-
Notifications
You must be signed in to change notification settings - Fork 71
Commit
This commit does not belong to any branch on this repository, and may belong to a fork outside of the repository.
feat(diffusers/training): add training of controlnet
- Loading branch information
1 parent
aaa7102
commit 808048d
Showing
5 changed files
with
2,490 additions
and
9 deletions.
There are no files selected for viewing
This file contains bidirectional Unicode text that may be interpreted or compiled differently than what appears below. To review, open the file in an editor that reveals hidden Unicode characters.
Learn more about bidirectional Unicode characters
Original file line number | Diff line number | Diff line change |
---|---|---|
@@ -0,0 +1,136 @@ | ||
# ControlNet training example | ||
|
||
[Adding Conditional Control to Text-to-Image Diffusion Models](https://arxiv.org/abs/2302.05543) by Lvmin Zhang and Maneesh Agrawala. | ||
|
||
This example is based on the [training example in the original ControlNet repository](https://github.com/lllyasviel/ControlNet/blob/main/docs/train.md). It trains a ControlNet to fill circles using a [small synthetic dataset](https://huggingface.co/datasets/fusing/fill50k). | ||
|
||
## Installing the dependencies | ||
|
||
Before running the scripts, make sure to install the library's training dependencies: | ||
|
||
**Important** | ||
|
||
To make sure you can successfully run the latest versions of the example scripts, we highly recommend **installing from source** and keeping the install up to date as we update the example scripts frequently and install some example-specific requirements. To do this, execute the following steps in a new virtual environment: | ||
```bash | ||
git clone https://github.com/mindspore-lab/mindone | ||
cd mindone | ||
pip install -e . | ||
``` | ||
|
||
Then cd in the example folder and run | ||
```bash | ||
pip install -r requirements.txt | ||
``` | ||
|
||
## Circle filling dataset | ||
|
||
The original dataset is hosted in the [ControlNet repo](https://huggingface.co/lllyasviel/ControlNet/blob/main/training/fill50k.zip). We re-uploaded it to be compatible with `datasets` [here](https://huggingface.co/datasets/fusing/fill50k). Note that `datasets` handles dataloading within the training script. | ||
|
||
Our training examples use [Stable Diffusion 1.5](https://huggingface.co/runwayml/stable-diffusion-v1-5) as the original set of ControlNet models were trained from it. However, ControlNet can be trained to augment any Stable Diffusion compatible model (such as [CompVis/stable-diffusion-v1-4](https://huggingface.co/CompVis/stable-diffusion-v1-4)) or [stabilityai/stable-diffusion-2-1](https://huggingface.co/stabilityai/stable-diffusion-2-1). | ||
|
||
## Training | ||
|
||
Our training examples use two test conditioning images. They can be downloaded by running | ||
|
||
```sh | ||
wget https://huggingface.co/datasets/huggingface/documentation-images/resolve/main/diffusers/controlnet_training/conditioning_image_1.png | ||
|
||
wget https://huggingface.co/datasets/huggingface/documentation-images/resolve/main/diffusers/controlnet_training/conditioning_image_2.png | ||
``` | ||
|
||
```bash | ||
export MODEL_DIR="runwayml/stable-diffusion-v1-5" | ||
export OUTPUT_DIR="path to save model" | ||
|
||
python train_controlnet.py \ | ||
--pretrained_model_name_or_path=$MODEL_DIR \ | ||
--output_dir=$OUTPUT_DIR \ | ||
--dataset_name=fusing/fill50k \ | ||
--resolution=512 \ | ||
--learning_rate=1e-5 \ | ||
--validation_image "./conditioning_image_1.png" "./conditioning_image_2.png" \ | ||
--validation_prompt "red circle with blue background" "cyan circle with brown floral background" \ | ||
--train_batch_size=4 | ||
``` | ||
|
||
This default configuration requires ~38GB VRAM. | ||
|
||
By default, the training script logs outputs to tensorboard. | ||
|
||
Gradient accumulation with a smaller batch size can be used to reduce training requirements to ~20 GB VRAM. | ||
|
||
```bash | ||
export MODEL_DIR="runwayml/stable-diffusion-v1-5" | ||
export OUTPUT_DIR="path to save model" | ||
|
||
python train_controlnet.py \ | ||
--pretrained_model_name_or_path=$MODEL_DIR \ | ||
--output_dir=$OUTPUT_DIR \ | ||
--dataset_name=fusing/fill50k \ | ||
--resolution=512 \ | ||
--learning_rate=1e-5 \ | ||
--validation_image "./conditioning_image_1.png" "./conditioning_image_2.png" \ | ||
--validation_prompt "red circle with blue background" "cyan circle with brown floral background" \ | ||
--train_batch_size=1 \ | ||
--gradient_accumulation_steps=4 | ||
``` | ||
|
||
## Example results | ||
|
||
#### After 300 steps with batch size 8 | ||
|
||
| | | | ||
|-------------------|:-------------------------:| | ||
| | red circle with blue background | | ||
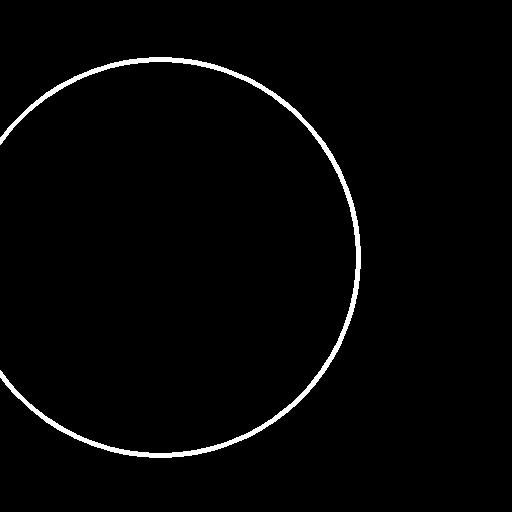 | 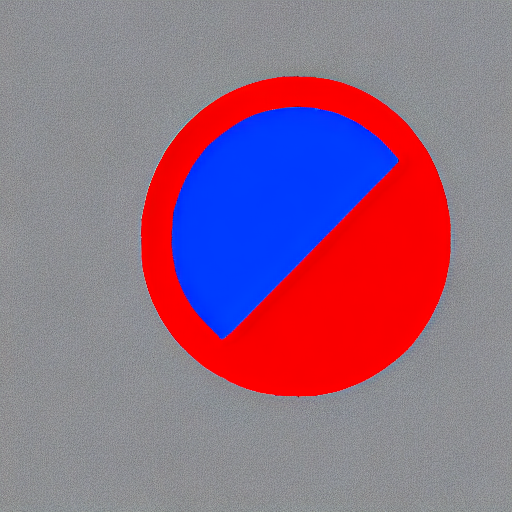 | | ||
| | cyan circle with brown floral background | | ||
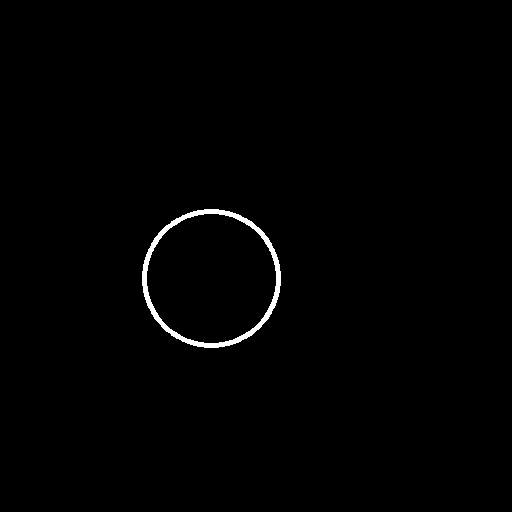 | 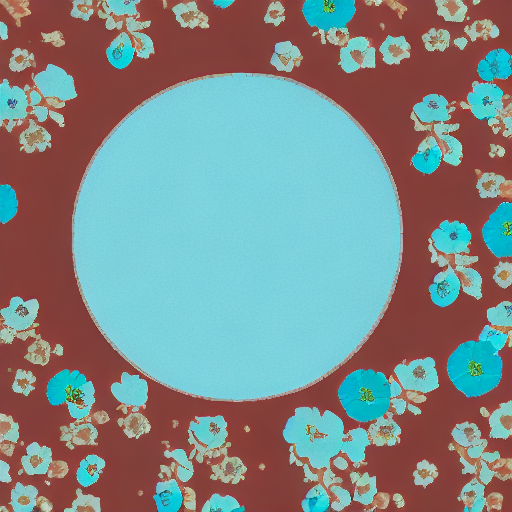 | | ||
|
||
|
||
#### After 6000 steps with batch size 8: | ||
|
||
| | | | ||
|-------------------|:-------------------------:| | ||
| | red circle with blue background | | ||
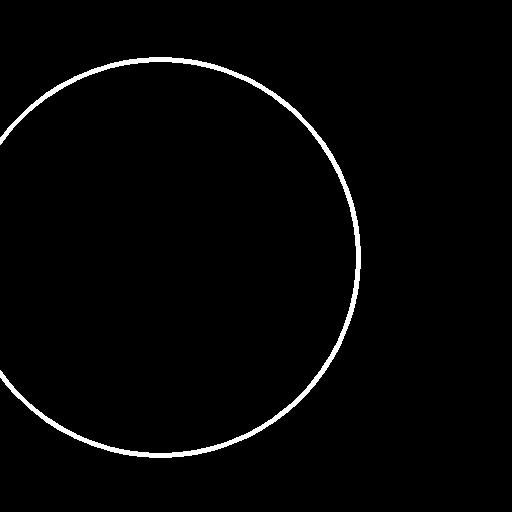 | 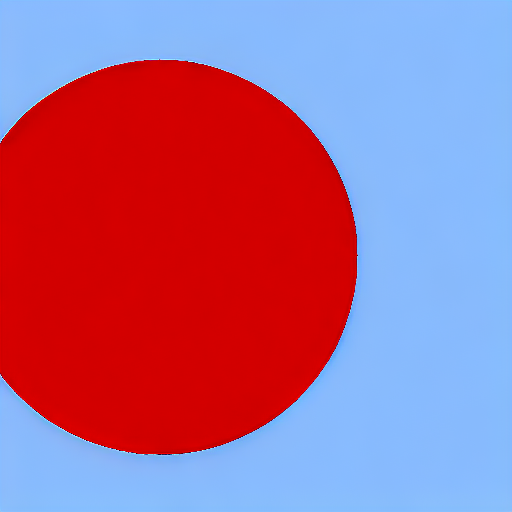 | | ||
| | cyan circle with brown floral background | | ||
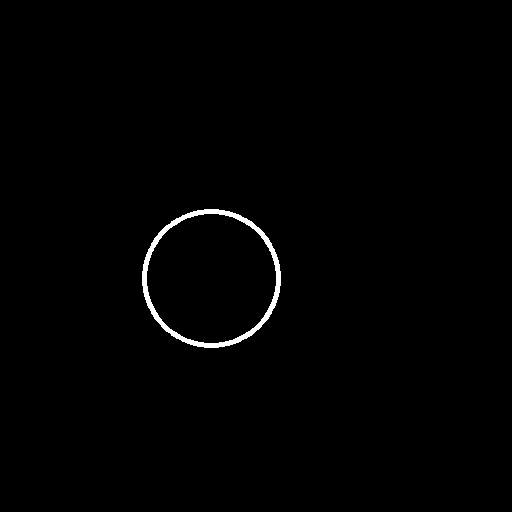 |  | | ||
|
||
|
||
## Performing inference with the trained ControlNet | ||
|
||
The trained model can be run the same as the original ControlNet pipeline with the newly trained ControlNet. | ||
Set `base_model_path` and `controlnet_path` to the values `--pretrained_model_name_or_path` and | ||
`--output_dir` were respectively set to in the training script. | ||
|
||
```py | ||
from mindone.diffusers import StableDiffusionControlNetPipeline, ControlNetModel, UniPCMultistepScheduler | ||
from mindone.diffusers.utils import load_image | ||
import mindspore as ms | ||
import numpy as np | ||
|
||
base_model_path = "path to model" | ||
controlnet_path = "path to controlnet" | ||
|
||
controlnet = ControlNetModel.from_pretrained(controlnet_path, mindspore_dtype=ms.float16) | ||
pipe = StableDiffusionControlNetPipeline.from_pretrained( | ||
base_model_path, controlnet=controlnet, mindspore_dtype=ms.float16 | ||
) | ||
|
||
# speed up diffusion process with faster scheduler | ||
pipe.scheduler = UniPCMultistepScheduler.from_config(pipe.scheduler.config) | ||
|
||
control_image = load_image("./conditioning_image_1.png") | ||
prompt = "pale golden rod circle with old lace background" | ||
|
||
# generate image | ||
generator = np.random.Generator(np.random.PCG64(0)) | ||
image = pipe( | ||
prompt, num_inference_steps=20, generator=generator, image=control_image | ||
)[0][0] | ||
image.save("./output.png") | ||
``` | ||
|
||
## Support for Stable Diffusion XL | ||
|
||
We provide a training script for training a ControlNet with [Stable Diffusion XL](https://huggingface.co/papers/2307.01952). Please refer to [README_sdxl.md](./README_sdxl.md) for more details. |
This file contains bidirectional Unicode text that may be interpreted or compiled differently than what appears below. To review, open the file in an editor that reveals hidden Unicode characters.
Learn more about bidirectional Unicode characters
Original file line number | Diff line number | Diff line change |
---|---|---|
@@ -0,0 +1,111 @@ | ||
# ControlNet training example for Stable Diffusion XL (SDXL) | ||
|
||
The `train_controlnet_sdxl.py` script shows how to implement the ControlNet training procedure and adapt it for [Stable Diffusion XL](https://huggingface.co/papers/2307.01952). | ||
|
||
## Running locally with MindSpore | ||
|
||
### Installing the dependencies | ||
|
||
Before running the scripts, make sure to install the library's training dependencies: | ||
|
||
**Important** | ||
|
||
To make sure you can successfully run the latest versions of the example scripts, we highly recommend **installing from source** and keeping the install up to date as we update the example scripts frequently and install some example-specific requirements. To do this, execute the following steps in a new virtual environment: | ||
|
||
```bash | ||
git clone https://github.com/mindspore-lab/mindone | ||
cd mindone | ||
pip install -e . | ||
``` | ||
|
||
Then cd in the `examples/controlnet` folder and run | ||
```bash | ||
pip install -r requirements_sdxl.txt | ||
``` | ||
|
||
## Circle filling dataset | ||
|
||
The original dataset is hosted in the [ControlNet repo](https://huggingface.co/lllyasviel/ControlNet/blob/main/training/fill50k.zip). We re-uploaded it to be compatible with `datasets` [here](https://huggingface.co/datasets/fusing/fill50k). Note that `datasets` handles dataloading within the training script. | ||
|
||
## Training | ||
|
||
Our training examples use two test conditioning images. They can be downloaded by running | ||
|
||
```sh | ||
wget https://huggingface.co/datasets/huggingface/documentation-images/resolve/main/diffusers/controlnet_training/conditioning_image_1.png | ||
|
||
wget https://huggingface.co/datasets/huggingface/documentation-images/resolve/main/diffusers/controlnet_training/conditioning_image_2.png | ||
``` | ||
|
||
```bash | ||
export MODEL_DIR="stabilityai/stable-diffusion-xl-base-1.0" | ||
export OUTPUT_DIR="path to save model" | ||
|
||
accelerate launch train_controlnet_sdxl.py \ | ||
--pretrained_model_name_or_path=$MODEL_DIR \ | ||
--output_dir=$OUTPUT_DIR \ | ||
--dataset_name=fusing/fill50k \ | ||
--mixed_precision="fp16" \ | ||
--resolution=1024 \ | ||
--learning_rate=1e-5 \ | ||
--max_train_steps=60000 \ | ||
--validation_image "./conditioning_image_1.png" "./conditioning_image_2.png" \ | ||
--validation_prompt "red circle with blue background" "cyan circle with brown floral background" \ | ||
--validation_steps=100 \ | ||
--train_batch_size=1 \ | ||
--seed=42 | ||
``` | ||
|
||
To better track our training experiments, we're using the following flags in the command above: | ||
|
||
* `validation_image`, `validation_prompt`, and `validation_steps` to allow the script to do a few validation inference runs. This allows us to qualitatively check if the training is progressing as expected. | ||
|
||
Our experiments were conducted on a single 40GB A100 GPU. | ||
|
||
### Inference | ||
|
||
Once training is done, we can perform inference like so: | ||
|
||
```python | ||
from mindone.diffusers import StableDiffusionXLControlNetPipeline, ControlNetModel, UniPCMultistepScheduler | ||
from mindone.diffusers.utils import load_image | ||
import mindspore as ms | ||
import numpy as np | ||
|
||
base_model_path = "stabilityai/stable-diffusion-xl-base-1.0" | ||
controlnet_path = "path to controlnet" | ||
|
||
controlnet = ControlNetModel.from_pretrained(controlnet_path, mindspore_dtype=ms.float16) | ||
pipe = StableDiffusionXLControlNetPipeline.from_pretrained( | ||
base_model_path, controlnet=controlnet, mindspore_dtype=ms.float16 | ||
) | ||
|
||
# speed up diffusion process with faster scheduler | ||
pipe.scheduler = UniPCMultistepScheduler.from_config(pipe.scheduler.config) | ||
|
||
control_image = load_image("./conditioning_image_1.png").resize((1024, 1024)) | ||
prompt = "pale golden rod circle with old lace background" | ||
|
||
# generate image | ||
generator = np.random.Generator(np.random.PCG64(0)) | ||
image = pipe( | ||
prompt, num_inference_steps=20, generator=generator, image=control_image | ||
)[0][0] | ||
image.save("./output.png") | ||
``` | ||
|
||
## Notes | ||
|
||
### Specifying a better VAE | ||
|
||
SDXL's VAE is known to suffer from numerical instability issues. This is why we also expose a CLI argument namely `--pretrained_vae_model_name_or_path` that lets you specify the location of an alternative VAE (such as [`madebyollin/sdxl-vae-fp16-fix`](https://huggingface.co/madebyollin/sdxl-vae-fp16-fix)). | ||
|
||
If you're using this VAE during training, you need to ensure you're using it during inference too. You do so by: | ||
|
||
```diff | ||
+ vae = AutoencoderKL.from_pretrained(vae_path_or_repo_id, torch_dtype=torch.float16) | ||
controlnet = ControlNetModel.from_pretrained(controlnet_path, torch_dtype=torch.float16) | ||
pipe = StableDiffusionXLControlNetPipeline.from_pretrained( | ||
base_model_path, controlnet=controlnet, torch_dtype=torch.float16, | ||
+ vae=vae, | ||
) |
Oops, something went wrong.