forked from huggingface/diffusers
-
Notifications
You must be signed in to change notification settings - Fork 0
Commit
This commit does not belong to any branch on this repository, and may belong to a fork outside of the repository.
Merge pull request #122 from huggingface/main
Merge changes
- Loading branch information
Showing
144 changed files
with
9,187 additions
and
967 deletions.
There are no files selected for viewing
This file contains bidirectional Unicode text that may be interpreted or compiled differently than what appears below. To review, open the file in an editor that reveals hidden Unicode characters.
Learn more about bidirectional Unicode characters
This file contains bidirectional Unicode text that may be interpreted or compiled differently than what appears below. To review, open the file in an editor that reveals hidden Unicode characters.
Learn more about bidirectional Unicode characters
Original file line number | Diff line number | Diff line change |
---|---|---|
@@ -0,0 +1,13 @@ | ||
# UNetMotionModel | ||
|
||
The [UNet](https://huggingface.co/papers/1505.04597) model was originally introduced by Ronneberger et al for biomedical image segmentation, but it is also commonly used in 🤗 Diffusers because it outputs images that are the same size as the input. It is one of the most important components of a diffusion system because it facilitates the actual diffusion process. There are several variants of the UNet model in 🤗 Diffusers, depending on it's number of dimensions and whether it is a conditional model or not. This is a 2D UNet model. | ||
|
||
The abstract from the paper is: | ||
|
||
*There is large consent that successful training of deep networks requires many thousand annotated training samples. In this paper, we present a network and training strategy that relies on the strong use of data augmentation to use the available annotated samples more efficiently. The architecture consists of a contracting path to capture context and a symmetric expanding path that enables precise localization. We show that such a network can be trained end-to-end from very few images and outperforms the prior best method (a sliding-window convolutional network) on the ISBI challenge for segmentation of neuronal structures in electron microscopic stacks. Using the same network trained on transmitted light microscopy images (phase contrast and DIC) we won the ISBI cell tracking challenge 2015 in these categories by a large margin. Moreover, the network is fast. Segmentation of a 512x512 image takes less than a second on a recent GPU. The full implementation (based on Caffe) and the trained networks are available at http://lmb.informatik.uni-freiburg.de/people/ronneber/u-net.* | ||
|
||
## UNetMotionModel | ||
[[autodoc]] UNetMotionModel | ||
|
||
## UNet3DConditionOutput | ||
[[autodoc]] models.unet_3d_condition.UNet3DConditionOutput |
This file contains bidirectional Unicode text that may be interpreted or compiled differently than what appears below. To review, open the file in an editor that reveals hidden Unicode characters.
Learn more about bidirectional Unicode characters
Original file line number | Diff line number | Diff line change |
---|---|---|
@@ -0,0 +1,230 @@ | ||
<!--Copyright 2023 The HuggingFace Team. All rights reserved. | ||
Licensed under the Apache License, Version 2.0 (the "License"); you may not use this file except in compliance with | ||
the License. You may obtain a copy of the License at | ||
http://www.apache.org/licenses/LICENSE-2.0 | ||
Unless required by applicable law or agreed to in writing, software distributed under the License is distributed on | ||
an "AS IS" BASIS, WITHOUT WARRANTIES OR CONDITIONS OF ANY KIND, either express or implied. See the License for the | ||
specific language governing permissions and limitations under the License. | ||
--> | ||
|
||
# Text-to-Video Generation with AnimateDiff | ||
|
||
## Overview | ||
|
||
[AnimateDiff: Animate Your Personalized Text-to-Image Diffusion Models without Specific Tuning](https://arxiv.org/abs/2307.04725) by Yuwei Guo, Ceyuan Yang*, Anyi Rao, Yaohui Wang, Yu Qiao, Dahua Lin, Bo Dai | ||
|
||
The abstract of the paper is the following: | ||
|
||
With the advance of text-to-image models (e.g., Stable Diffusion) and corresponding personalization techniques such as DreamBooth and LoRA, everyone can manifest their imagination into high-quality images at an affordable cost. Subsequently, there is a great demand for image animation techniques to further combine generated static images with motion dynamics. In this report, we propose a practical framework to animate most of the existing personalized text-to-image models once and for all, saving efforts in model-specific tuning. At the core of the proposed framework is to insert a newly initialized motion modeling module into the frozen text-to-image model and train it on video clips to distill reasonable motion priors. Once trained, by simply injecting this motion modeling module, all personalized versions derived from the same base T2I readily become text-driven models that produce diverse and personalized animated images. We conduct our evaluation on several public representative personalized text-to-image models across anime pictures and realistic photographs, and demonstrate that our proposed framework helps these models generate temporally smooth animation clips while preserving the domain and diversity of their outputs. Code and pre-trained weights will be publicly available at this https URL . | ||
|
||
## Available Pipelines | ||
|
||
| Pipeline | Tasks | Demo | ||
|---|---|:---:| | ||
| [AnimateDiffPipeline](https://github.com/huggingface/diffusers/blob/main/src/diffusers/pipelines/animatediff/pipeline_animatediff.py) | *Text-to-Video Generation with AnimateDiff* | | ||
|
||
## Available checkpoints | ||
|
||
Motion Adapter checkpoints can be found under [guoyww](https://huggingface.co/guoyww/). These checkpoints are meant to work with any model based on Stable Diffusion 1.4/1.5 | ||
|
||
## Usage example | ||
|
||
AnimateDiff works with a MotionAdapter checkpoint and a Stable Diffusion model checkpoint. The MotionAdapter is a collection of Motion Modules that are responsible for adding coherent motion across image frames. These modules are applied after the Resnet and Attention blocks in Stable Diffusion UNet. | ||
|
||
The following example demonstrates how to use a *MotionAdapter* checkpoint with Diffusers for inference based on StableDiffusion-1.4/1.5. | ||
|
||
```python | ||
import torch | ||
from diffusers import MotionAdapter, AnimateDiffPipeline, DDIMScheduler | ||
from diffusers.utils import export_to_gif | ||
|
||
# Load the motion adapter | ||
adapter = MotionAdapter.from_pretrained("guoyww/animatediff-motion-adapter-v1-5-2") | ||
# load SD 1.5 based finetuned model | ||
model_id = "SG161222/Realistic_Vision_V5.1_noVAE" | ||
pipe = AnimateDiffPipeline.from_pretrained(model_id, motion_adapter=adapter) | ||
scheduler = DDIMScheduler.from_pretrained( | ||
model_id, subfolder="scheduler", clip_sample=False, timestep_spacing="linspace", steps_offset=1 | ||
) | ||
pipe.scheduler = scheduler | ||
|
||
# enable memory savings | ||
pipe.enable_vae_slicing() | ||
pipe.enable_model_cpu_offload() | ||
|
||
output = pipe( | ||
prompt=( | ||
"masterpiece, bestquality, highlydetailed, ultradetailed, sunset, " | ||
"orange sky, warm lighting, fishing boats, ocean waves seagulls, " | ||
"rippling water, wharf, silhouette, serene atmosphere, dusk, evening glow, " | ||
"golden hour, coastal landscape, seaside scenery" | ||
), | ||
negative_prompt="bad quality, worse quality", | ||
num_frames=16, | ||
guidance_scale=7.5, | ||
num_inference_steps=25, | ||
generator=torch.Generator("cpu").manual_seed(42), | ||
) | ||
frames = output.frames[0] | ||
export_to_gif(frames, "animation.gif") | ||
``` | ||
|
||
Here are some sample outputs: | ||
|
||
<table> | ||
<tr> | ||
<td><center> | ||
masterpiece, bestquality, sunset. | ||
<br> | ||
<img src="https://huggingface.co/datasets/huggingface/documentation-images/resolve/main/diffusers/animatediff-realistic-doc.gif" | ||
alt="masterpiece, bestquality, sunset" | ||
style="width: 300px;" /> | ||
</center></td> | ||
</tr> | ||
</table> | ||
|
||
<Tip> | ||
|
||
AnimateDiff tends to work better with finetuned Stable Diffusion models. If you plan on using a scheduler that can clip samples, make sure to disable it by setting `clip_sample=False` in the scheduler as this can also have an adverse effect on generated samples. | ||
|
||
</Tip> | ||
|
||
## Using Motion LoRAs | ||
|
||
Motion LoRAs are a collection of LoRAs that work with the `guoyww/animatediff-motion-adapter-v1-5-2` checkpoint. These LoRAs are responsible for adding specific types of motion to the animations. | ||
|
||
```python | ||
import torch | ||
from diffusers import MotionAdapter, AnimateDiffPipeline, DDIMScheduler | ||
from diffusers.utils import export_to_gif | ||
|
||
# Load the motion adapter | ||
adapter = MotionAdapter.from_pretrained("guoyww/animatediff-motion-adapter-v1-5-2") | ||
# load SD 1.5 based finetuned model | ||
model_id = "SG161222/Realistic_Vision_V5.1_noVAE" | ||
pipe = AnimateDiffPipeline.from_pretrained(model_id, motion_adapter=adapter) | ||
pipe.load_lora_weights("guoyww/animatediff-motion-lora-zoom-out", adapter_name="zoom-out") | ||
|
||
scheduler = DDIMScheduler.from_pretrained( | ||
model_id, subfolder="scheduler", clip_sample=False, timestep_spacing="linspace", steps_offset=1 | ||
) | ||
pipe.scheduler = scheduler | ||
|
||
# enable memory savings | ||
pipe.enable_vae_slicing() | ||
pipe.enable_model_cpu_offload() | ||
|
||
output = pipe( | ||
prompt=( | ||
"masterpiece, bestquality, highlydetailed, ultradetailed, sunset, " | ||
"orange sky, warm lighting, fishing boats, ocean waves seagulls, " | ||
"rippling water, wharf, silhouette, serene atmosphere, dusk, evening glow, " | ||
"golden hour, coastal landscape, seaside scenery" | ||
), | ||
negative_prompt="bad quality, worse quality", | ||
num_frames=16, | ||
guidance_scale=7.5, | ||
num_inference_steps=25, | ||
generator=torch.Generator("cpu").manual_seed(42), | ||
) | ||
frames = output.frames[0] | ||
export_to_gif(frames, "animation.gif") | ||
``` | ||
|
||
<table> | ||
<tr> | ||
<td><center> | ||
masterpiece, bestquality, sunset. | ||
<br> | ||
<img src="https://huggingface.co/datasets/huggingface/documentation-images/resolve/main/diffusers/animatediff-zoom-out-lora.gif" | ||
alt="masterpiece, bestquality, sunset" | ||
style="width: 300px;" /> | ||
</center></td> | ||
</tr> | ||
</table> | ||
|
||
## Using Motion LoRAs with PEFT | ||
|
||
You can also leverage the [PEFT](https://github.com/huggingface/peft) backend to combine Motion LoRA's and create more complex animations. | ||
|
||
First install PEFT with | ||
|
||
```shell | ||
pip install peft | ||
``` | ||
|
||
Then you can use the following code to combine Motion LoRAs. | ||
|
||
```python | ||
import torch | ||
from diffusers import MotionAdapter, AnimateDiffPipeline, DDIMScheduler | ||
from diffusers.utils import export_to_gif | ||
|
||
# Load the motion adapter | ||
adapter = MotionAdapter.from_pretrained("guoyww/animatediff-motion-adapter-v1-5-2") | ||
# load SD 1.5 based finetuned model | ||
model_id = "SG161222/Realistic_Vision_V5.1_noVAE" | ||
pipe = AnimateDiffPipeline.from_pretrained(model_id, motion_adapter=adapter) | ||
|
||
pipe.load_lora_weights("diffusers/animatediff-motion-lora-zoom-out", adapter_name="zoom-out") | ||
pipe.load_lora_weights("diffusers/animatediff-motion-lora-pan-left", adapter_name="pan-left") | ||
pipe.set_adapters(["zoom-out", "pan-left"], adapter_weights=[1.0, 1.0]) | ||
|
||
scheduler = DDIMScheduler.from_pretrained( | ||
model_id, subfolder="scheduler", clip_sample=False, timestep_spacing="linspace", steps_offset=1 | ||
) | ||
pipe.scheduler = scheduler | ||
|
||
# enable memory savings | ||
pipe.enable_vae_slicing() | ||
pipe.enable_model_cpu_offload() | ||
|
||
output = pipe( | ||
prompt=( | ||
"masterpiece, bestquality, highlydetailed, ultradetailed, sunset, " | ||
"orange sky, warm lighting, fishing boats, ocean waves seagulls, " | ||
"rippling water, wharf, silhouette, serene atmosphere, dusk, evening glow, " | ||
"golden hour, coastal landscape, seaside scenery" | ||
), | ||
negative_prompt="bad quality, worse quality", | ||
num_frames=16, | ||
guidance_scale=7.5, | ||
num_inference_steps=25, | ||
generator=torch.Generator("cpu").manual_seed(42), | ||
) | ||
frames = output.frames[0] | ||
export_to_gif(frames, "animation.gif") | ||
``` | ||
|
||
<table> | ||
<tr> | ||
<td><center> | ||
masterpiece, bestquality, sunset. | ||
<br> | ||
<img src="https://huggingface.co/datasets/huggingface/documentation-images/resolve/main/diffusers/animatediff-zoom-out-pan-left-lora.gif" | ||
alt="masterpiece, bestquality, sunset" | ||
style="width: 300px;" /> | ||
</center></td> | ||
</tr> | ||
</table> | ||
|
||
|
||
## AnimateDiffPipeline | ||
|
||
[[autodoc]] AnimateDiffPipeline | ||
- all | ||
- __call__ | ||
- enable_freeu | ||
- disable_freeu | ||
- enable_vae_slicing | ||
- disable_vae_slicing | ||
- enable_vae_tiling | ||
- disable_vae_tiling | ||
|
||
## AnimateDiffPipelineOutput | ||
|
||
[[autodoc]] pipelines.animatediff.AnimateDiffPipelineOutput | ||
|
This file contains bidirectional Unicode text that may be interpreted or compiled differently than what appears below. To review, open the file in an editor that reveals hidden Unicode characters.
Learn more about bidirectional Unicode characters
This file contains bidirectional Unicode text that may be interpreted or compiled differently than what appears below. To review, open the file in an editor that reveals hidden Unicode characters.
Learn more about bidirectional Unicode characters
Original file line number | Diff line number | Diff line change |
---|---|---|
@@ -0,0 +1,36 @@ | ||
<!--Copyright 2023 The HuggingFace Team. All rights reserved. | ||
Licensed under the Apache License, Version 2.0 (the "License"); you may not use this file except in compliance with | ||
the License. You may obtain a copy of the License at | ||
http://www.apache.org/licenses/LICENSE-2.0 | ||
Unless required by applicable law or agreed to in writing, software distributed under the License is distributed on | ||
an "AS IS" BASIS, WITHOUT WARRANTIES OR CONDITIONS OF ANY KIND, either express or implied. See the License for the | ||
specific language governing permissions and limitations under the License. | ||
--> | ||
|
||
# PixArt | ||
|
||
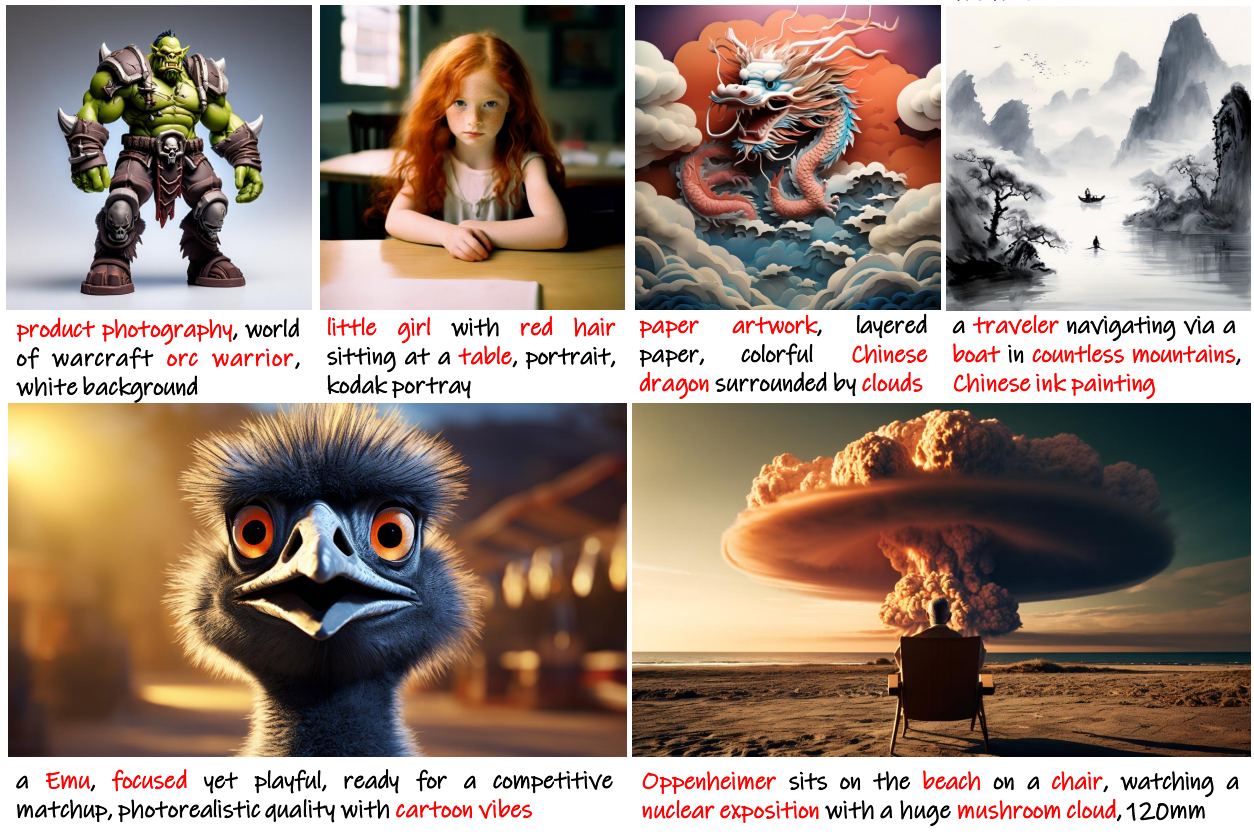 | ||
|
||
[PixArt-α: Fast Training of Diffusion Transformer for Photorealistic Text-to-Image Synthesis](https://huggingface.co/papers/2310.00426) is Junsong Chen, Jincheng Yu, Chongjian Ge, Lewei Yao, Enze Xie, Yue Wu, Zhongdao Wang, James Kwok, Ping Luo, Huchuan Lu, and Zhenguo Li. | ||
|
||
The abstract from the paper is: | ||
|
||
*The most advanced text-to-image (T2I) models require significant training costs (e.g., millions of GPU hours), seriously hindering the fundamental innovation for the AIGC community while increasing CO2 emissions. This paper introduces PIXART-α, a Transformer-based T2I diffusion model whose image generation quality is competitive with state-of-the-art image generators (e.g., Imagen, SDXL, and even Midjourney), reaching near-commercial application standards. Additionally, it supports high-resolution image synthesis up to 1024px resolution with low training cost, as shown in Figure 1 and 2. To achieve this goal, three core designs are proposed: (1) Training strategy decomposition: We devise three distinct training steps that separately optimize pixel dependency, text-image alignment, and image aesthetic quality; (2) Efficient T2I Transformer: We incorporate cross-attention modules into Diffusion Transformer (DiT) to inject text conditions and streamline the computation-intensive class-condition branch; (3) High-informative data: We emphasize the significance of concept density in text-image pairs and leverage a large Vision-Language model to auto-label dense pseudo-captions to assist text-image alignment learning. As a result, PIXART-α's training speed markedly surpasses existing large-scale T2I models, e.g., PIXART-α only takes 10.8% of Stable Diffusion v1.5's training time (675 vs. 6,250 A100 GPU days), saving nearly $300,000 ($26,000 vs. $320,000) and reducing 90% CO2 emissions. Moreover, compared with a larger SOTA model, RAPHAEL, our training cost is merely 1%. Extensive experiments demonstrate that PIXART-α excels in image quality, artistry, and semantic control. We hope PIXART-α will provide new insights to the AIGC community and startups to accelerate building their own high-quality yet low-cost generative models from scratch.* | ||
|
||
You can find the original codebase at [PixArt-alpha/PixArt-alpha](https://github.com/PixArt-alpha/PixArt-alpha) and all the available checkpoints at [PixArt-alpha](https://huggingface.co/PixArt-alpha). | ||
|
||
Some notes about this pipeline: | ||
|
||
* It uses a Transformer backbone (instead of a UNet) for denoising. As such it has a similar architecture as [DiT](./dit.md). | ||
* It was trained using text conditions computed from T5. This aspect makes the pipeline better at following complex text prompts with intricate details. | ||
* It is good at producing high-resolution images at different aspect ratios. To get the best results, the authors recommend some size brackets which can be found [here](https://github.com/PixArt-alpha/PixArt-alpha/blob/08fbbd281ec96866109bdd2cdb75f2f58fb17610/diffusion/data/datasets/utils.py). | ||
* It rivals the quality of state-of-the-art text-to-image generation systems (as of this writing) such as Stable Diffusion XL, Imagen, and DALL-E 2, while being more efficient than them. | ||
|
||
## PixArtAlphaPipeline | ||
|
||
[[autodoc]] PixArtAlphaPipeline | ||
- all | ||
- __call__ |
Oops, something went wrong.