You signed in with another tab or window. Reload to refresh your session.You signed out in another tab or window. Reload to refresh your session.You switched accounts on another tab or window. Reload to refresh your session.Dismiss alert
From my understanding the sparsity pattern for the block is fully random. This is concerning since it leads to non-full rank matrices when increasing sparsity. See the figure below which BlockSparseLinear generated for a 256x256 matrix with 25% density and 32x32 blocks:
If my computation is correct, at this size, block size and sparsity, only around 20% of matrices will be full rank or, for another example, only 10% of 1024x1024 matrices at 10% density will be full rank), and, if I am not completely mistaken (I might yet be), 0% of the matrices created in the README self.fc = BlockSparseLinear(1024, 256, density=0.1)
I don't have any good option to propose though sorry, I see only 2 complementary ways:
preselecting some of the tiles to make sure all input data is used and all output data is filled (eg: diagonal pattern for a square matrix),
adding an API for users to provide the sparsity pattern they want to use if they need more flexibility (eg: BlockSparseLinear.from_pattern(pattern: torch.Tensor, block_shape: Tuple[int, int]), but then it's no more a "drop in replacement" to a linear layer)
The text was updated successfully, but these errors were encountered:
Hi,
First, thanks for this code! ;)
From my understanding the sparsity pattern for the block is fully random. This is concerning since it leads to non-full rank matrices when increasing sparsity. See the figure below which
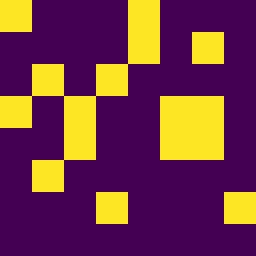
BlockSparseLinear
generated for a 256x256 matrix with 25% density and 32x32 blocks:If my computation is correct, at this size, block size and sparsity, only around 20% of matrices will be full rank or, for another example, only 10% of 1024x1024 matrices at 10% density will be full rank), and, if I am not completely mistaken (I might yet be), 0% of the matrices created in the README
self.fc = BlockSparseLinear(1024, 256, density=0.1)
I don't have any good option to propose though sorry, I see only 2 complementary ways:
BlockSparseLinear.from_pattern(pattern: torch.Tensor, block_shape: Tuple[int, int])
, but then it's no more a "drop in replacement" to a linear layer)The text was updated successfully, but these errors were encountered: