diff --git a/docs/Cloud_Chain/SenseCraft/SenseCraft_AI/Pretrained_Models/SenseCraft_Pretrained_Watcher.md b/docs/Cloud_Chain/SenseCraft/SenseCraft_AI/Pretrained_Models/SenseCraft_Pretrained_Watcher.md
new file mode 100644
index 000000000000..d38bc67abb2c
--- /dev/null
+++ b/docs/Cloud_Chain/SenseCraft/SenseCraft_AI/Pretrained_Models/SenseCraft_Pretrained_Watcher.md
@@ -0,0 +1,111 @@
+---
+description: How to use a model for SenseCAP Watcher
+title: for SenseCAP Watcher
+image: https://files.seeedstudio.com/wiki/SenseCraft_AI/img2/14.png
+slug: /sensecraft_ai_pretrained_models_for_watcher
+sidebar_position: 3
+last_update:
+ date: 12/03/2024
+ author: Citric
+---
+
+SenseCAP Watcher is a powerful monitoring device that can be configured to recognize specific objects and trigger alarms based on user-defined tasks. To enhance Watcher's object recognition capabilities, users can leverage custom models from the SenseCraft AI model repository. This wiki article will guide you through the process of using these custom models in your Watcher monitoring tasks.
+
+## Prerequisites
+
+Before you begin using custom models from the SenseCraft AI model repository, ensure that you have the following:
+
+- **SenseCAP Watcher**: You should have a SenseCAP Watcher device set up and ready to use. If you haven't already, follow the instructions in the [SenseCAP Watcher Quick Start Guide](https://wiki.seeedstudio.com/getting_started_with_watcher/) to set up your device.
+
+- **SenseCraft APP**: Install the SenseCraft APP on your mobile device. The app is available for both iOS and Android platforms and can be downloaded from the respective app stores.
+
+
+
+- **SenseCraft Account**: To access the SenseCraft AI model repository and use custom models, you need to have a SenseCraft AI account. If you don't have an account, sign up for one through the SenseCraft APP or the official SenseCraft AI website.
+
+- **Network Connectivity**: Ensure that your SenseCAP Watcher device and mobile device running the SenseCraft APP are connected to the internet. A stable network connection is required to access the SenseCraft AI model repository and download custom models.
+
+
+
+## Step 1. Issuing a Monitoring Task to Watcher via the SenseCraft APP
+
+To begin, open the SenseCraft APP and navigate to the Watcher device you want to configure. The app provides an intuitive interface for creating and managing monitoring tasks. For this example, let's create a task that says, *If a keyboard is recognized, play the sound 'Keyboard is awesome'*.
+
+
+
+When creating a task, it's essential to be clear and specific about the object you want Watcher to recognize and the action you want it to take when the object is detected. This helps ensure that Watcher understands and executes the task accurately.
+
+If you don't know enough about how Watcher places an appropriate task, read the [Task Assignment Guideline](https://wiki.seeedstudio.com/getting_started_with_watcher_task/).
+
+## Step 2. Enabling the Use of a Custom TinyML Model
+
+After issuing the task through the APP, click on the task card to enter the **Detail Configs** page. This page allows you to fine-tune various aspects of your monitoring task, including the selection of a custom TinyML model.
+
+In the **Scenario** section at the top of the page, you'll find the **Use TinyML Model** option. By checking this option, you enable Watcher to use a custom model from the SenseCraft AI model repository for object recognition. Click on the model name field to search or directly select the desired model, such as a **keyboard detection** model.
+
+
+
+The SenseCraft AI model repository hosts a wide range of pre-trained models that can be used for various object recognition tasks. These models have been optimized for use with Watcher, ensuring high accuracy and performance.
+
+:::note
+1. After selecting a model, the Watcher's alarm words may be cleared and need to be re-entered before the Run Task button can be clicked.
+
+2. After selecting the model, please reasonably configure the Confidence Threshold below the model. the default value is 0. If you directly send it to the task with 0 as the threshold, it may lead to anything being recognized as a wrong object, please adjust this value according to the actual situation to achieve the best detection effect.
+:::
+
+In addition to the pre-trained models available in the SenseCraft AI model repository, you can also use your own custom-trained models. If you have a specific object or scenario that isn't covered by the existing models, you can train your own model and share it with the SenseCraft AI community.
+
+:::tip
+Watcher can search and use private models under the same SenseCraft account. If you choose not to make your models public, you can also use your private models as long as Watcher is bound to your account.
+:::
+
+## Step 3. Confirming the Task and Starting Monitoring
+
+After selecting the custom model and confirming other task configuration details, click the "Run Task" button to start the monitoring task. This action deploys the task to your Watcher device and begins the monitoring process.
+
+Upon receiving the task, Watcher will automatically download the selected model from the SenseCraft AI model repository and use it as the basis for triggering alarm actions. This seamless integration ensures that Watcher has the most up-to-date and relevant model for accurate object recognition.
+
+With the custom model in place, Watcher will continuously monitor its environment for the presence of the specified object. In this example, when Watcher recognizes a keyboard using the selected model, it will trigger the specified alarm action—playing the sound "Keyboard is awesome".
+
+
+
+The combination of custom models and user-defined tasks allows Watcher to adapt to a wide range of monitoring scenarios. By leveraging the power of the SenseCraft AI model repository and the flexibility of the SenseCraft APP, users can tailor Watcher's capabilities to their specific needs, ensuring reliable and accurate object recognition and alarm triggering.
+
+## Conclusion
+
+Using custom models from the SenseCraft AI model repository empowers SenseCAP Watcher users to enhance the device's object recognition capabilities and expand its monitoring and alarm application scenarios. The SenseCraft APP provides an intuitive interface for searching, selecting, and applying these custom models to Watcher monitoring tasks. By following the steps outlined in this wiki article, users can easily configure Watcher to recognize specific objects and trigger alarms based on their unique requirements. Whether using pre-trained models or custom-trained models shared with the SenseCraft AI community, Watcher offers a powerful and adaptable solution for various monitoring needs.
+
+
+## Tech Support & Product Discussion
+
+Thank you for choosing our products! We are here to provide you with different support to ensure that your experience with our products is as smooth as possible. We offer several communication channels to cater to different preferences and needs.
+
+
+
diff --git a/docs/Cloud_Chain/SenseCraft/SenseCraft_AI/Pretrained_Models/SenseCraft_Pretrained_XIAO_ESP32S3.md b/docs/Cloud_Chain/SenseCraft/SenseCraft_AI/Pretrained_Models/SenseCraft_Pretrained_XIAO_ESP32S3.md
index f194eb238ab9..356583982670 100644
--- a/docs/Cloud_Chain/SenseCraft/SenseCraft_AI/Pretrained_Models/SenseCraft_Pretrained_XIAO_ESP32S3.md
+++ b/docs/Cloud_Chain/SenseCraft/SenseCraft_AI/Pretrained_Models/SenseCraft_Pretrained_XIAO_ESP32S3.md
@@ -139,19 +139,19 @@ Feel free to explore other models, experiment with different settings, and adapt
Happy experimenting and creating with SenseCraft AI models on your XIAO ESP32S3 Sense!
+
+
## Tech Support & Product Discussion
Thank you for choosing our products! We are here to provide you with different support to ensure that your experience with our products is as smooth as possible. We offer several communication channels to cater to different preferences and needs.
-
-
-
-
+
+
+
diff --git a/docs/Cloud_Chain/SenseCraft/SenseCraft_AI/Pretrained_Models/_category_.yml b/docs/Cloud_Chain/SenseCraft/SenseCraft_AI/Pretrained_Models/_category_.yml
index f7aa5ca52496..c42786c48ed8 100644
--- a/docs/Cloud_Chain/SenseCraft/SenseCraft_AI/Pretrained_Models/_category_.yml
+++ b/docs/Cloud_Chain/SenseCraft/SenseCraft_AI/Pretrained_Models/_category_.yml
@@ -2,3 +2,9 @@ position: 3 # float position is supported
label: 'Pretrained Models'
collapsible: true # make the category collapsible
collapsed: true # keep the category open by default
+className: sensecraft_ai_pretrained_models
+link:
+ type: generated-index
+ slug: sensecraft_ai_pretrained_models_main_page
+ title: SenseCraft AI Pretrained Models Part
+ description: This series will cover how to quickly deploy a model inside a model repository on Seeed Studio.
diff --git a/docs/Cloud_Chain/SenseCraft/SenseCraft_AI/SenseCraft_AI_main_page.md b/docs/Cloud_Chain/SenseCraft/SenseCraft_AI/SenseCraft_AI_main_page.md
index 8ec1920b6181..a7f39ff2e0df 100644
--- a/docs/Cloud_Chain/SenseCraft/SenseCraft_AI/SenseCraft_AI_main_page.md
+++ b/docs/Cloud_Chain/SenseCraft/SenseCraft_AI/SenseCraft_AI_main_page.md
@@ -9,4 +9,29 @@ last_update:
author: qiuyu
---
-# SenseCraft AI Wiki Center
\ No newline at end of file
+# SenseCraft AI Wiki Center
+
+
+
+
+
+
+
+
+
+
+
+## Tech Support & Product Discussion
+
+Thank you for choosing our products! We are here to provide you with different support to ensure that your experience with our products is as smooth as possible. We offer several communication channels to cater to different preferences and needs.
+
+
+
+
+
From 81f37b5f0ecb487fbc411a87a02a0293da58efc1 Mon Sep 17 00:00:00 2001
From: limengdu <747632169@qq.com>
Date: Wed, 4 Dec 2024 11:20:12 +0800
Subject: [PATCH 2/2] Update: SenseCraft AI Training wiki
---
.../SenseCraft_Pretrained_Watcher.md | 2 +-
.../SenseCraft_AI/Training/Classification.md | 132 +++++++++++++-----
.../Training/Object_Detection.md | 72 +++++++---
.../SenseCraft_AI/Training/_category_.yml | 6 +
4 files changed, 160 insertions(+), 52 deletions(-)
diff --git a/docs/Cloud_Chain/SenseCraft/SenseCraft_AI/Pretrained_Models/SenseCraft_Pretrained_Watcher.md b/docs/Cloud_Chain/SenseCraft/SenseCraft_AI/Pretrained_Models/SenseCraft_Pretrained_Watcher.md
index d38bc67abb2c..cd1ca02d19b1 100644
--- a/docs/Cloud_Chain/SenseCraft/SenseCraft_AI/Pretrained_Models/SenseCraft_Pretrained_Watcher.md
+++ b/docs/Cloud_Chain/SenseCraft/SenseCraft_AI/Pretrained_Models/SenseCraft_Pretrained_Watcher.md
@@ -1,7 +1,7 @@
---
description: How to use a model for SenseCAP Watcher
title: for SenseCAP Watcher
-image: https://files.seeedstudio.com/wiki/SenseCraft_AI/img2/14.png
+image: https://files.seeedstudio.com/wiki/SenseCraft_AI/img2/32.png
slug: /sensecraft_ai_pretrained_models_for_watcher
sidebar_position: 3
last_update:
diff --git a/docs/Cloud_Chain/SenseCraft/SenseCraft_AI/Training/Classification.md b/docs/Cloud_Chain/SenseCraft/SenseCraft_AI/Training/Classification.md
index ce9d6b901660..3d2470c0d12a 100644
--- a/docs/Cloud_Chain/SenseCraft/SenseCraft_AI/Training/Classification.md
+++ b/docs/Cloud_Chain/SenseCraft/SenseCraft_AI/Training/Classification.md
@@ -1,64 +1,132 @@
---
description: How to use Training(Classification)
title: Classification
-image: https://files.seeedstudio.com/wiki/SenseCraft_AI/img3/main_page.webp
+image: https://files.seeedstudio.com/wiki/SenseCraft_AI/img2/34.png
slug: /sensecraft_ai_Training_Classification
sidebar_position: 1
last_update:
- date: 11/27/2024
- author: qiuyu wei
+ date: 12/03/2024
+ author: Citric
---
# Type of training - Classification
-**Classification** training is a machine learning method that learns the relationship between data and categories by giving the model sample data labelled with categories, ultimately enabling the model to classify new data into predefined categories.
-
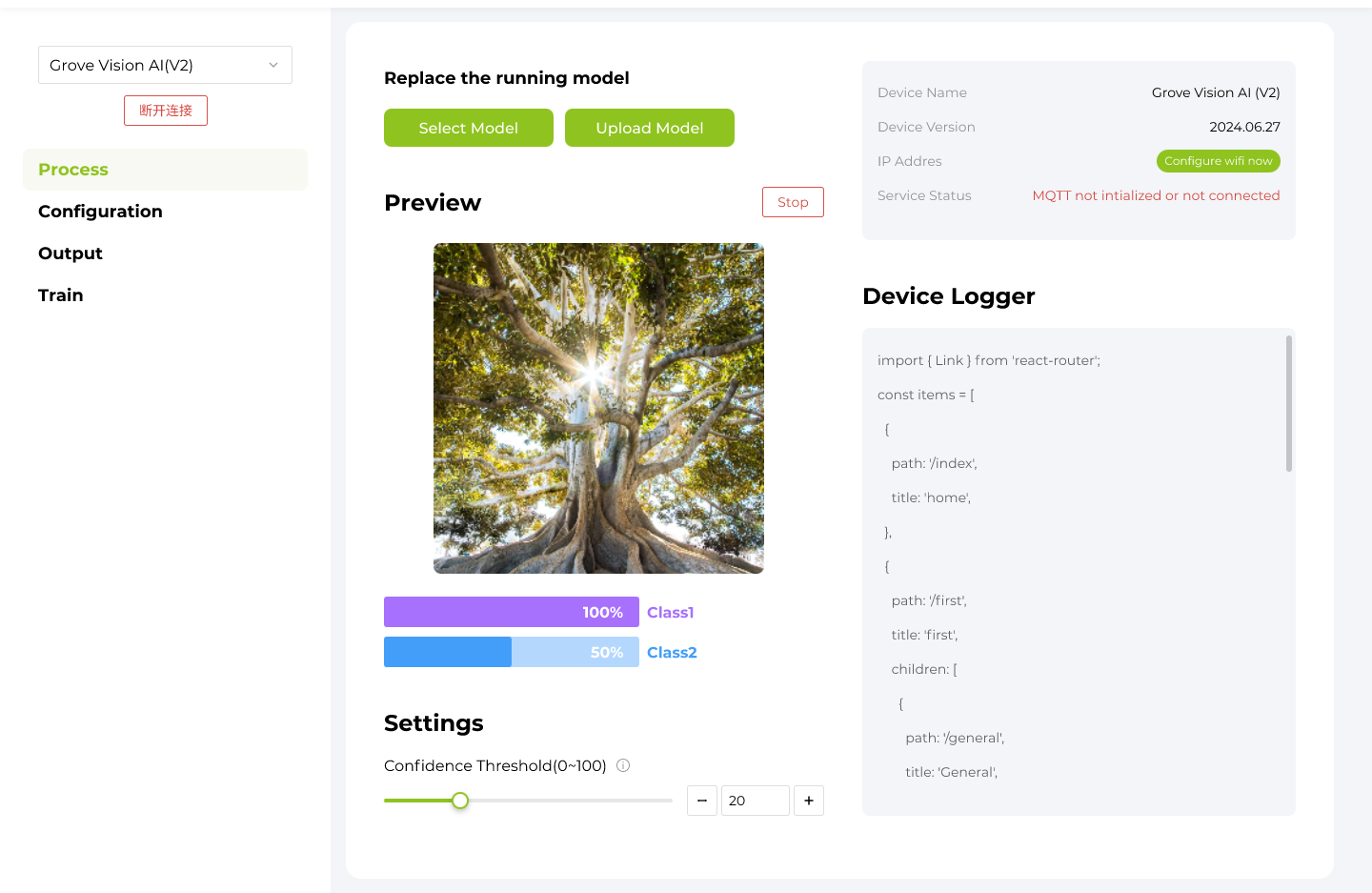
+
+Classification is a powerful tool in machine learning that allows you to train a model to recognize and categorize different types of data. In the SenseCraft AI platform, classification enables you to create models that can identify and distinguish between various objects, gestures, or scenes based on the images you provide during training.
+
+By training a classification model with SenseCraft AI, you can unlock a wide range of applications, such as:
+
+- Gesture recognition for interactive experiences
+
+- Object detection for inventory management or quality control
+
+- Scene classification for autonomous navigation or environmental monitoring
+
+The SenseCraft AI platform simplifies the classification process, allowing you to create custom models tailored to your specific needs without requiring extensive machine learning expertise.
+
+
+
+
## Getting Started
-Next, we will show you how to train a classification model of your own. The model will recognise whether people are wearing masks or not.
-
-## Training to recognise mask models
-
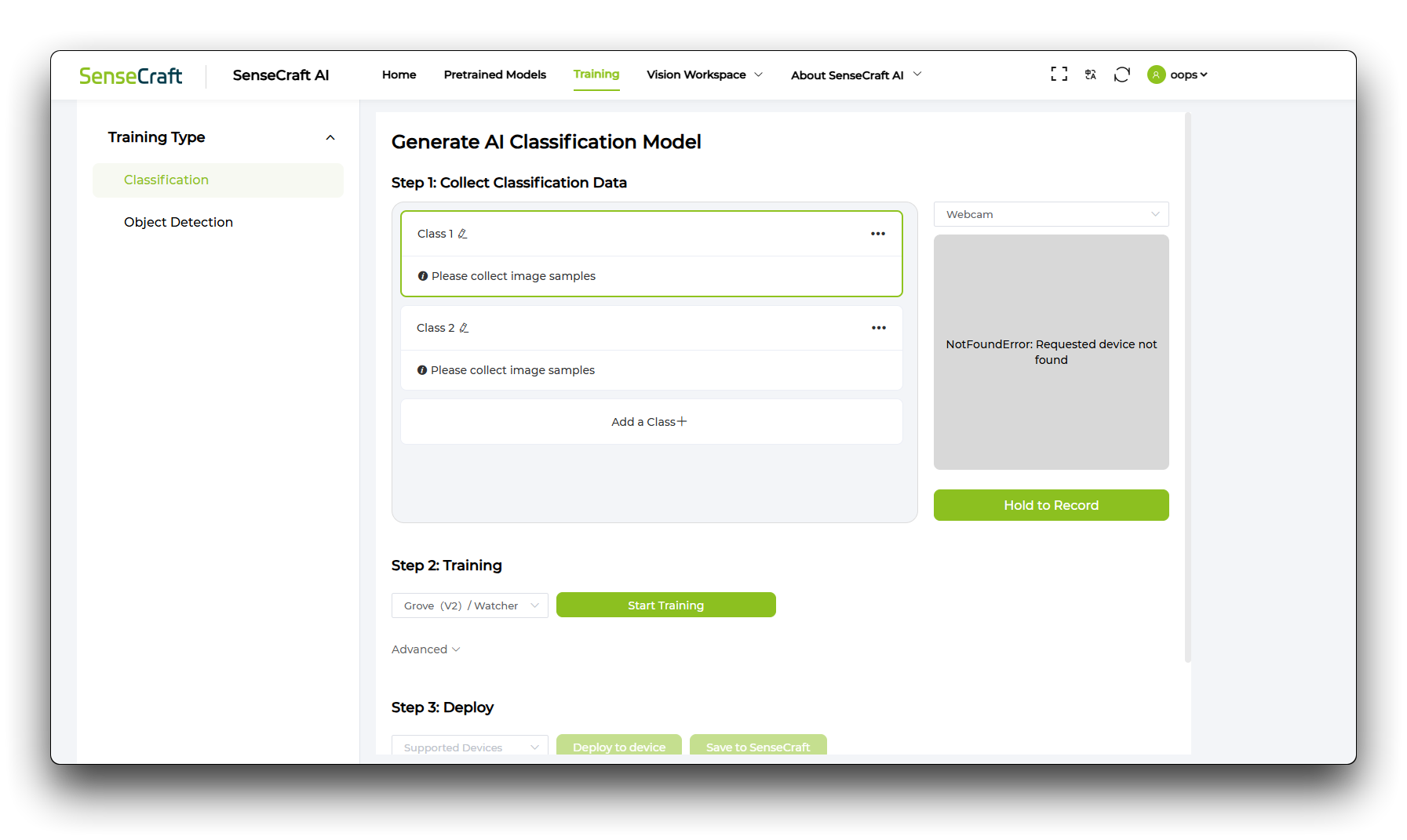
+In this comprehensive guide, we will walk you through the process of training a classification model using the SenseCraft AI platform. While our primary focus will be on training a model for the XIAO ESP32S3 Sense, it's important to note that this platform is also compatible with other Seeed Studio devices, such as the Grove Vision AI and Watcher.
+
+Don't have a Seeed Studio device? No problem! You can still follow along and experience the training process using your laptop's built-in camera. However, for optimal performance and the best results, we recommend using the target device to train and deploy your model.
+
+## Training a model to recognize body gestures
+
+For this tutorial, we will create a model that recognizes four distinct body gestures: crossed arms, open arms, standing at attention, and making a heart shape with hands.
-**step 1.** Connect the device to the computer via USB, the device selected in this demo is XIAO ESP32S3 Sense. Select the corresponding device and click **Connect**, then select the **correct serial port** for connection.
:::tip
-If the device is successfully connected, a live preview of the camera will appear in the right-hand box.
+The SenseCraft AI platform supports up to 200 categories for classification, giving you ample flexibility to create models tailored to your specific needs.
+:::
+
+### Step 1. Connect your device
+
+If you're using a Seeed Studio device like the XIAO ESP32S3 Sense, connect it to your computer via USB-C cable. Select the corresponding device from the dropdown menu and click **Connect**.
+
+
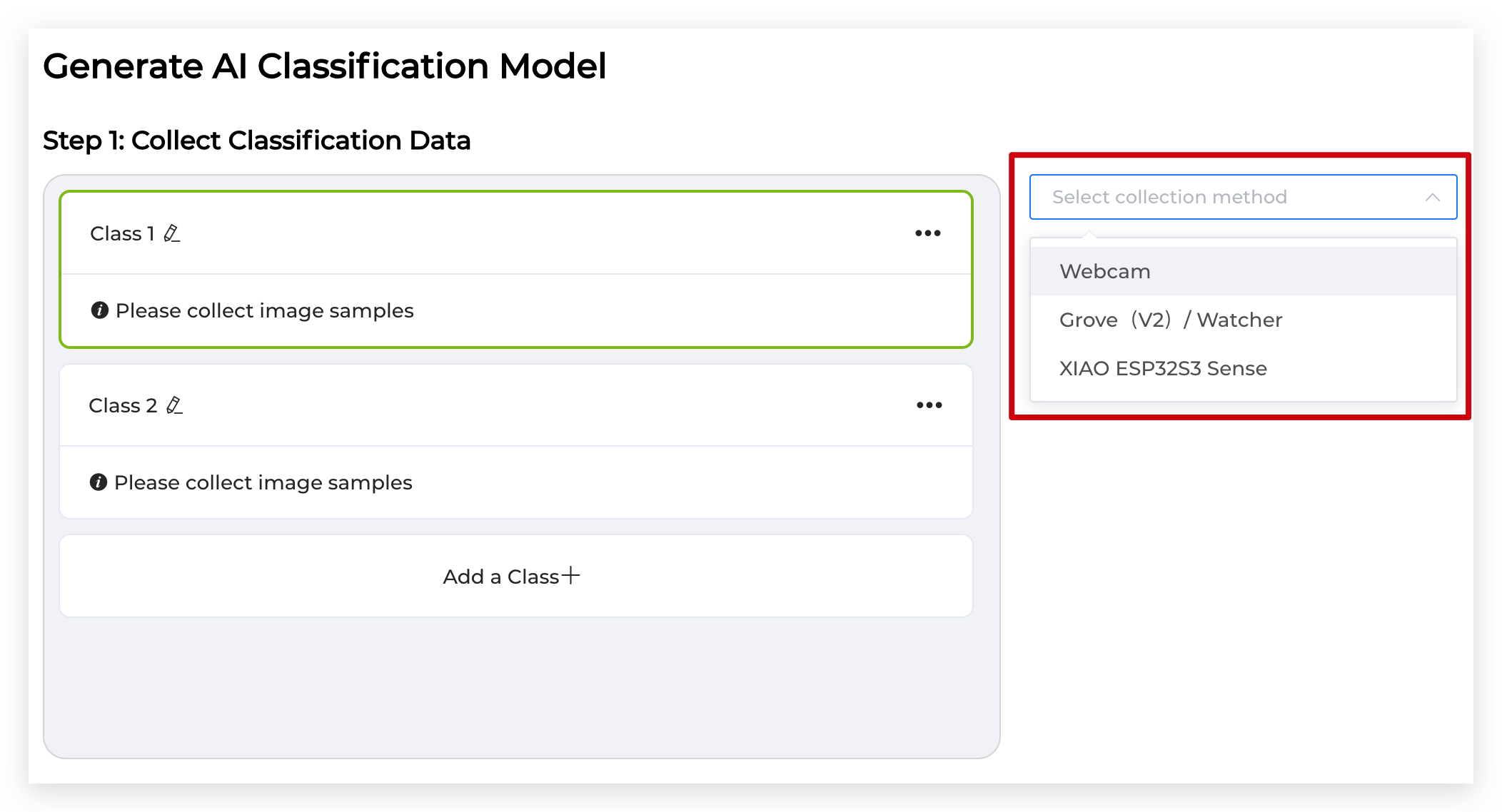
+
+Choose the **correct serial port** for the connection.
+
+
+
+If you're using your laptop's camera, you can skip this step. Because when you come to this page, it automatically shows the live feed of the camera. If it doesn't, please check your browser permissions.
+
+
+
+:::note
+Please use **Microsoft Edge** or **Google Chrome**.
:::
-
-**step 2.** This demo is to identify whether people are wearing masks or not, so we can see that we need to create two categories, after creating the categories you need to rename the different categories.
-**Category 1:** Wear masks;
-**Category 2:** No mask worn.
-
+### Step 2. Create and label categories
+
+Click the pencil button to the right of an existing class name to rename an already existing class. Click the **Add a Class** button below to create four categories for the body gestures you want to recognize.
+
+
+
+Label the categories as follows: "Crossed Arms," "Open Arms," "Standing at Attention," and "Heart Shape." Double-check that each category is named correctly.
+
+
+
+### Step 3. Capture training data
+
+Select the first category (e.g., "Crossed Arms") from the list. Position yourself in front of the camera, performing the corresponding body gesture. Press and hold the **Hold to Record** button to capture images of the gesture. Release the button to stop recording. Aim to capture **at least 40 images** per category to ensure a robust and accurate model.
+
+
+
+Repeat this process for each of the remaining categories, capturing a diverse range of images for each gesture.
-**step 3.** Select the appropriate category and capture the corresponding content with the camera.
:::tip
-Press and hold **‘Hold to Record’** to take a picture. The higher the number of relevant photos, the higher the recognition accuracy of the model.
+The more high-quality, relevant images you collect for each category, the better the model's performance will be. Aim for variety in lighting, angles, and backgrounds to improve the model's generalization capabilities.
:::
-
-
-**step 4.** Once you have collected a sufficient number of images by category, you can click **‘Start Training’** to train the model.
-
+### Step 4. Train the model
+
+Once you have collected a sufficient number of images for each category, click the **'Start Training'** button to initiate the model training process. The training process typically takes between 1-3 minutes, depending on the complexity of the model and the amount of training data.
+
+
:::tip
-Model training time is about 1-3 minutes, please be patient!
+Please **do not** immediately web page while training the model, otherwise the content of the page may be lost.
:::
-**step 5.** After the model training is completed, we can deploy the operation, in this demo we use the **XIAO ESP32S3 Sense**, so we need to select the appropriate device, and then click **‘Deploy to device’**.
-
+### Step 5. Deploy the trained model
-Then click **‘Confirm’**, and finally select the correct **serial port** for device connection, to complete the above operation model will officially start deployment. The process will also last 1-3 minutes, please be patient!
-
-
+:::caution
+Please note that if you want to save this model permanently, please make sure to click **Save to SenseCraft** first to save the model under your account to avoid losing it.
+:::
+
+After the model training is complete, it's time to deploy it to your target device. If you're using the XIAO ESP32S3 Sense or another Seeed Studio device, select the appropriate device from the dropdown menu and click **'Deploy to device'**. If you trained the model using your laptop's camera, you can skip this step and proceed to the results demonstration.
+
+
+
+Click **'Confirm'** and select the correct **serial port** for the device connection. The deployment process may take 1-3 minutes. Please be patient and wait for it to complete.
+
+
## Demonstration of results
-After completing the above steps, the mask recognition model has been successfully trained and deployed, next you can point the camera at yourself to test the actual effect.
-
-
+Congratulations! You have successfully trained and deployed your body gesture recognition model. It's time to put it to the test:
+
+- Point the camera at yourself or a test subject.
+- Perform each of the trained body gestures one at a time.
+- Observe the model's real-time predictions and classification results.
+- Verify that the model accurately recognizes and classifies each gesture.
+
+Feel free to experiment with training models for different objects, gestures, or scenarios using the SenseCraft AI platform. The process remains largely the same, regardless of whether you're using a Seeed Studio device or your laptop's camera.
+
+
+
+
+
+Remember, while the platform allows you to train models using any camera, for the best results and optimal performance, we recommend using the target device (currently limited to Seeed Studio devices) to train and deploy your model.
-You can try to train the model you want according to the above method, you can also replace Grove Vision AI (V2) for testing, the method and steps are the same.
+With this comprehensive guide, you should now have a solid understanding of how to train a classification model using the SenseCraft AI platform. Happy training, and enjoy creating powerful, custom AI models for your projects!
## Tech Support & Product Discussion
diff --git a/docs/Cloud_Chain/SenseCraft/SenseCraft_AI/Training/Object_Detection.md b/docs/Cloud_Chain/SenseCraft/SenseCraft_AI/Training/Object_Detection.md
index 9782324ec704..0d4d57de2a7e 100644
--- a/docs/Cloud_Chain/SenseCraft/SenseCraft_AI/Training/Object_Detection.md
+++ b/docs/Cloud_Chain/SenseCraft/SenseCraft_AI/Training/Object_Detection.md
@@ -10,75 +10,109 @@ last_update:
---
# Type of training - Object Detection
+
## Features of object detection
+
The Seeed SenseCraft AI Platform is an efficient AI training tool tailored for object detection tasks. Built on the advanced **YOLO - World object detection model**, it offers two convenient training methods:
+
- **Quick Training**
+
Features: No image data is required. Simply input the target name to quickly generate a single-class object detection model.
Advantages: Ideal for straightforward scenarios, enabling fast model creation and deployment.
+
- **Image Collection Training**
+
Features: Combines the target name with uploaded image data for training.
+
Advantages: Leverages diverse image data to significantly improve the detection accuracy of the generated model, making it suitable for applications requiring high precision.
+
With these two methods, the SenseCraft platform caters to diverse object detection model training needs, simplifying the complexities of AI development while ensuring both usability and precision.
+
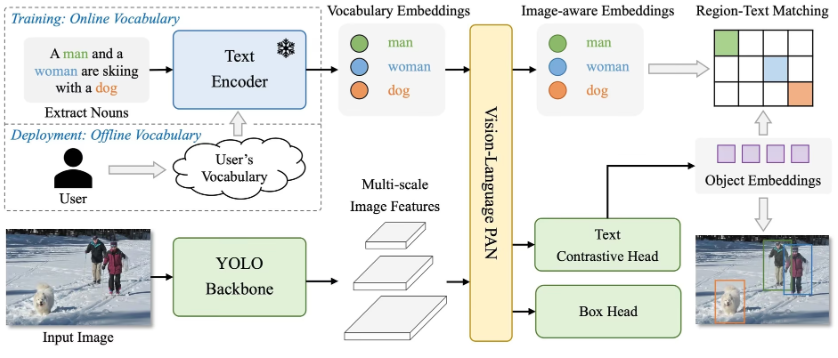
-## Quick Training
+## Quick Training
+
We will create a simple demo for **recognising human**. The quick training feature leverages the following core characteristics of the YOLO – World object detection model:
+
The quick training feature uses YOLO’s strengths to efficiently create single-class detection models. By combining pretrained weights, text semantics, and efficient feature extraction, it generates a tailored model, such as for "human", without requiring image data.
-### Step
-**Step 1:** Enter the target name in the text box. Then click on **'Start Training'**.
+
+### Step 1. Determine the object name
+
+Enter the target name in the text box. Then click on **'Start Training'**.
+
:::tip
The training session will last 1-3 minutes, so please be patient!
:::
-
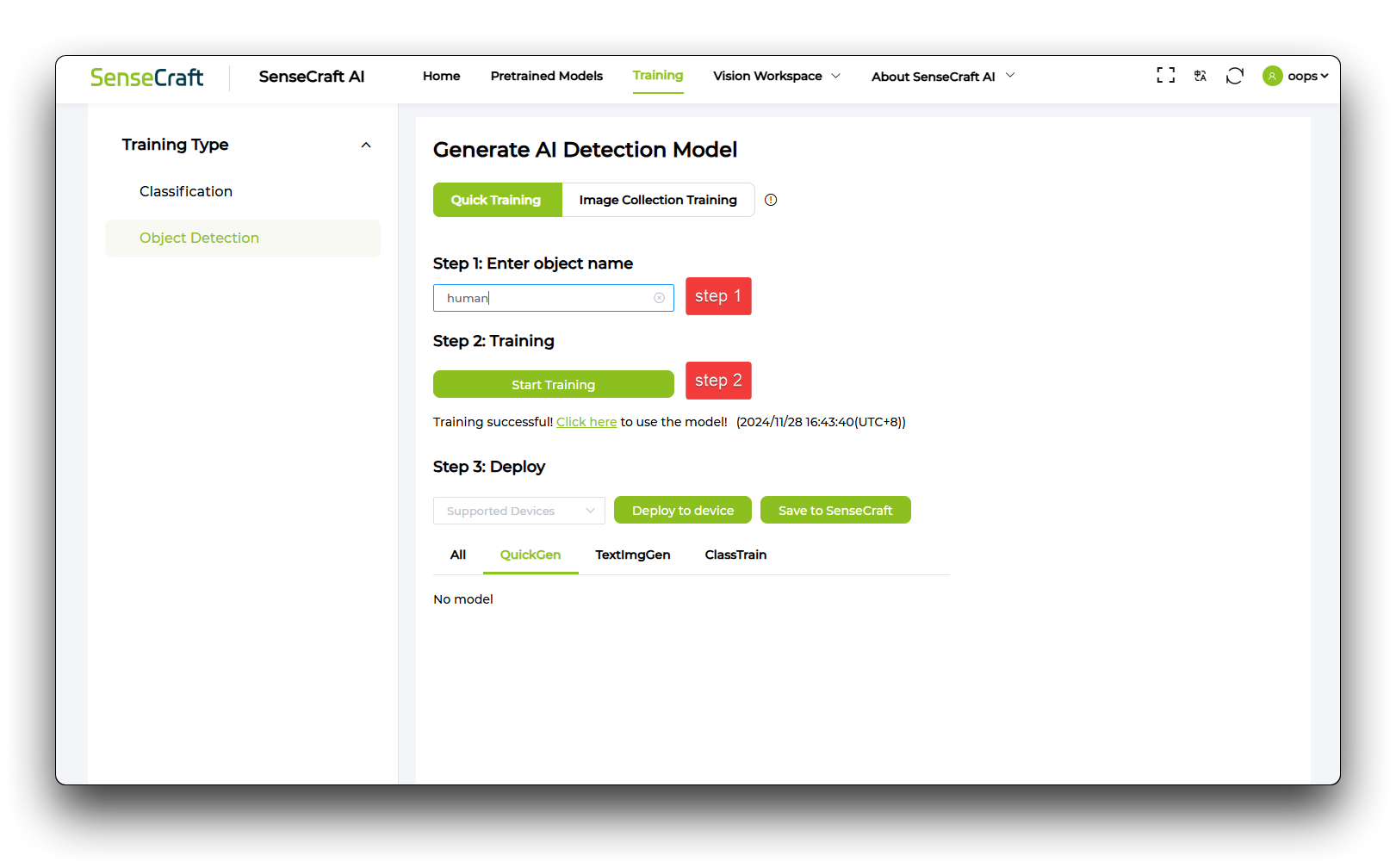
-**Step 2.** After completing the model training, the model will be deployed and Grove Vision AI (V2) will be selected for the deployment. Then choose the correct serial port to connect to, and finally wait patiently for 1-3 minutes to know that the model training is complete!
+
+
+### Step 2. Train and upload models
+
+After completing the model training, the model will be deployed and Grove Vision AI (V2) will be selected for the deployment. Then choose the correct serial port to connect to, and finally wait patiently for 1-3 minutes to know that the model training is complete!
:::caution
-Device selection in Object Detection can only support **Grove Vision AI (V2)**.
+Currently device selection in Object Detection can only support **Grove Vision AI (V2)**.
:::
-
-
+
+
+
### Demonstration of results
+
After completing the above steps, the model will be successfully deployed and run, but care needs to be taken with the **Confidence Threshold** and **IoU Threshold value** settings, which will affect the model's ability to recognise.
:::tip
**Confidence Threshold:** The minimum confidence score a model must have to consider a detection valid, filtering out low-confidence predictions.
+
**IoU Threshold:** The minimum Intersection over Union (IoU) value required to classify a predicted bounding box as a true positive, ensuring accuracy in overlap measurement between predicted and ground truth boxes.
:::
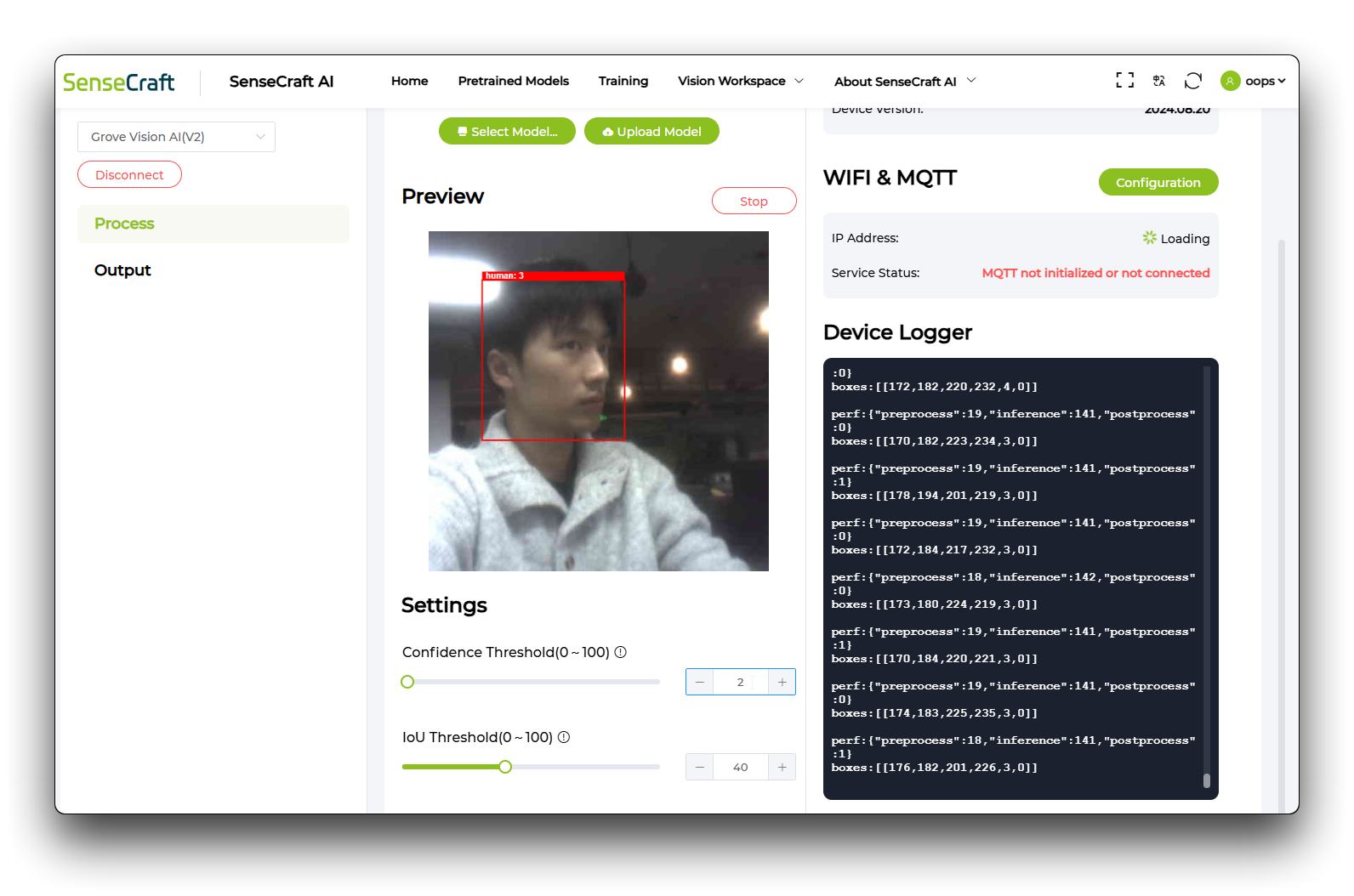
## Image Collection Training
+
We'll make a demo that **recognises earphones**. Based on YOLO – World object detection model, you can customize the training for text and image, which can improve the detection accuracy of the generated model.
-### Step
-**Step 3.** First enter the target name in the text box and then select **Grove Vision AI (V2)** to connect.
-
+### Step 1. Determine the object name
+
+First enter the target name in the text box and then select **Grove Vision AI (V2)** to connect.
+
+
:::tip
If the connection is successful, a live preview of the camera will appear in the box on the right.
:::
-
+
+
+### Step 2. Capture Image
+
+Then point the camera at the target object and click **'Capture'**, then box the target object with a red box and finally click **'Confirm'**.
-**Step 4.** Then point the camera at the target object and click **'Capture'**, then box the target object with a red box and finally click **'Confirm'**.
-
+
:::tip
The more image material, the better the recognition of model.
:::
-**Step 5.** Click on **'Training'** and then wait patiently for the model to finish training.
-
+### Step 3. Train and upload models
-**Step 6.** And finally it's time for model deployment.
-
+
+Click on **'Training'** and then wait patiently for the model to finish training.
+
+
+
+And finally it's time for model deployment.
+
+
### Demonstration of results
+
Once the above steps are completed, the model will be successfully trained and deployed.
-
+
+
+
## Tech Support & Product Discussion
diff --git a/docs/Cloud_Chain/SenseCraft/SenseCraft_AI/Training/_category_.yml b/docs/Cloud_Chain/SenseCraft/SenseCraft_AI/Training/_category_.yml
index e2734acb5ecb..f0b65e3df6b3 100644
--- a/docs/Cloud_Chain/SenseCraft/SenseCraft_AI/Training/_category_.yml
+++ b/docs/Cloud_Chain/SenseCraft/SenseCraft_AI/Training/_category_.yml
@@ -2,3 +2,9 @@ position: 4 # float position is supported
label: 'Training'
collapsible: true # make the category collapsible
collapsed: true # keep the category open by default
+className: sensecraft_ai_training
+link:
+ type: generated-index
+ slug: sensecraft_ai_training_main_page
+ title: SenseCraft AI Training Part
+ description: Inside this tutorial series, we will guide you on how to train classification models and object detection models using SenseCraft AI.