diff --git a/.gitignore b/.gitignore
new file mode 100644
index 0000000..5c4c9a5
--- /dev/null
+++ b/.gitignore
@@ -0,0 +1,135 @@
+# Byte-compiled / optimized / DLL files
+__pycache__/
+*.py[cod]
+*$py.class
+*.ipynb
+
+# C extensions
+*.so
+
+# Distribution / packaging
+.Python
+build/
+develop-eggs/
+dist/
+downloads/
+eggs/
+.eggs/
+lib/
+lib64/
+parts/
+sdist/
+var/
+wheels/
+*.egg-info/
+.installed.cfg
+*.egg
+MANIFEST
+
+# PyInstaller
+# Usually these files are written by a python script from a template
+# before PyInstaller builds the exe, so as to inject date/other infos into it.
+*.manifest
+*.spec
+
+# Installer logs
+pip-log.txt
+pip-delete-this-directory.txt
+
+# Unit test / coverage reports
+htmlcov/
+.tox/
+.coverage
+.coverage.*
+.cache
+nosetests.xml
+coverage.xml
+*.cover
+.hypothesis/
+.pytest_cache/
+
+# Translations
+*.mo
+*.pot
+
+# Django stuff:
+*.log
+local_settings.py
+db.sqlite3
+
+# Flask stuff:
+instance/
+.webassets-cache
+
+# Scrapy stuff:
+.scrapy
+
+# Sphinx documentation
+docs/en/_build/
+docs/zh_cn/_build/
+
+# PyBuilder
+target/
+
+# Jupyter Notebook
+.ipynb_checkpoints
+
+# pyenv
+.python-version
+
+# celery beat schedule file
+celerybeat-schedule
+
+# SageMath parsed files
+*.sage.py
+
+# Environments
+.env
+.venv
+env/
+venv/
+ENV/
+env.bak/
+venv.bak/
+
+# Spyder project settings
+.spyderproject
+.spyproject
+
+# Rope project settings
+.ropeproject
+
+# mkdocs documentation
+/site
+
+# mypy
+.mypy_cache/
+
+# cython generated cpp
+data
+.vscode
+.idea
+
+# custom
+*.pkl
+*.pkl.json
+*.log.json
+work_dirs/
+exps/
+*~
+mmdet3d/.mim
+
+# Pytorch
+*.pth
+
+# demo
+*.jpg
+*.png
+data/s3dis/Stanford3dDataset_v1.2_Aligned_Version/
+data/scannet/scans/
+data/sunrgbd/OFFICIAL_SUNRGBD/
+*.obj
+*.ply
+
+# Waymo evaluation
+mmdet3d/core/evaluation/waymo_utils/compute_detection_metrics_main
diff --git a/.pre-commit-config.yaml b/.pre-commit-config.yaml
new file mode 100644
index 0000000..790bfb1
--- /dev/null
+++ b/.pre-commit-config.yaml
@@ -0,0 +1,50 @@
+repos:
+ - repo: https://github.com/PyCQA/flake8
+ rev: 3.8.3
+ hooks:
+ - id: flake8
+ - repo: https://github.com/PyCQA/isort
+ rev: 5.10.1
+ hooks:
+ - id: isort
+ - repo: https://github.com/pre-commit/mirrors-yapf
+ rev: v0.30.0
+ hooks:
+ - id: yapf
+ - repo: https://github.com/pre-commit/pre-commit-hooks
+ rev: v3.1.0
+ hooks:
+ - id: trailing-whitespace
+ - id: check-yaml
+ - id: end-of-file-fixer
+ - id: requirements-txt-fixer
+ - id: double-quote-string-fixer
+ - id: check-merge-conflict
+ - id: fix-encoding-pragma
+ args: ["--remove"]
+ - id: mixed-line-ending
+ args: ["--fix=lf"]
+ - repo: https://github.com/codespell-project/codespell
+ rev: v2.1.0
+ hooks:
+ - id: codespell
+ - repo: https://github.com/executablebooks/mdformat
+ rev: 0.7.14
+ hooks:
+ - id: mdformat
+ args: [ "--number" ]
+ additional_dependencies:
+ - mdformat-gfm
+ - mdformat_frontmatter
+ - linkify-it-py
+ - repo: https://github.com/myint/docformatter
+ rev: v1.3.1
+ hooks:
+ - id: docformatter
+ args: ["--in-place", "--wrap-descriptions", "79"]
+ - repo: https://github.com/open-mmlab/pre-commit-hooks
+ rev: v0.2.0 # Use the ref you want to point at
+ hooks:
+ - id: check-algo-readme
+ - id: check-copyright
+ args: ["mmdet3d"] # replace the dir_to_check with your expected directory to check
diff --git a/.readthedocs.yml b/.readthedocs.yml
new file mode 100644
index 0000000..49178bb
--- /dev/null
+++ b/.readthedocs.yml
@@ -0,0 +1,10 @@
+version: 2
+
+formats: all
+
+python:
+ version: 3.7
+ install:
+ - requirements: requirements/docs.txt
+ - requirements: requirements/runtime.txt
+ - requirements: requirements/readthedocs.txt
diff --git a/LICENSE b/LICENSE
new file mode 100644
index 0000000..e4cf43e
--- /dev/null
+++ b/LICENSE
@@ -0,0 +1,159 @@
+# Attribution-NonCommercial 4.0 International
+
+> *Creative Commons Corporation (“Creative Commons”) is not a law firm and does not provide legal services or legal advice. Distribution of Creative Commons public licenses does not create a lawyer-client or other relationship. Creative Commons makes its licenses and related information available on an “as-is” basis. Creative Commons gives no warranties regarding its licenses, any material licensed under their terms and conditions, or any related information. Creative Commons disclaims all liability for damages resulting from their use to the fullest extent possible.*
+>
+> ### Using Creative Commons Public Licenses
+>
+> Creative Commons public licenses provide a standard set of terms and conditions that creators and other rights holders may use to share original works of authorship and other material subject to copyright and certain other rights specified in the public license below. The following considerations are for informational purposes only, are not exhaustive, and do not form part of our licenses.
+>
+> * __Considerations for licensors:__ Our public licenses are intended for use by those authorized to give the public permission to use material in ways otherwise restricted by copyright and certain other rights. Our licenses are irrevocable. Licensors should read and understand the terms and conditions of the license they choose before applying it. Licensors should also secure all rights necessary before applying our licenses so that the public can reuse the material as expected. Licensors should clearly mark any material not subject to the license. This includes other CC-licensed material, or material used under an exception or limitation to copyright. [More considerations for licensors](http://wiki.creativecommons.org/Considerations_for_licensors_and_licensees#Considerations_for_licensors).
+>
+> * __Considerations for the public:__ By using one of our public licenses, a licensor grants the public permission to use the licensed material under specified terms and conditions. If the licensor’s permission is not necessary for any reason–for example, because of any applicable exception or limitation to copyright–then that use is not regulated by the license. Our licenses grant only permissions under copyright and certain other rights that a licensor has authority to grant. Use of the licensed material may still be restricted for other reasons, including because others have copyright or other rights in the material. A licensor may make special requests, such as asking that all changes be marked or described. Although not required by our licenses, you are encouraged to respect those requests where reasonable. [More considerations for the public](http://wiki.creativecommons.org/Considerations_for_licensors_and_licensees#Considerations_for_licensees).
+
+## Creative Commons Attribution-NonCommercial 4.0 International Public License
+
+By exercising the Licensed Rights (defined below), You accept and agree to be bound by the terms and conditions of this Creative Commons Attribution-NonCommercial 4.0 International Public License ("Public License"). To the extent this Public License may be interpreted as a contract, You are granted the Licensed Rights in consideration of Your acceptance of these terms and conditions, and the Licensor grants You such rights in consideration of benefits the Licensor receives from making the Licensed Material available under these terms and conditions.
+
+### Section 1 – Definitions.
+
+a. __Adapted Material__ means material subject to Copyright and Similar Rights that is derived from or based upon the Licensed Material and in which the Licensed Material is translated, altered, arranged, transformed, or otherwise modified in a manner requiring permission under the Copyright and Similar Rights held by the Licensor. For purposes of this Public License, where the Licensed Material is a musical work, performance, or sound recording, Adapted Material is always produced where the Licensed Material is synched in timed relation with a moving image.
+
+b. __Adapter's License__ means the license You apply to Your Copyright and Similar Rights in Your contributions to Adapted Material in accordance with the terms and conditions of this Public License.
+
+c. __Copyright and Similar Rights__ means copyright and/or similar rights closely related to copyright including, without limitation, performance, broadcast, sound recording, and Sui Generis Database Rights, without regard to how the rights are labeled or categorized. For purposes of this Public License, the rights specified in Section 2(b)(1)-(2) are not Copyright and Similar Rights.
+
+d. __Effective Technological Measures__ means those measures that, in the absence of proper authority, may not be circumvented under laws fulfilling obligations under Article 11 of the WIPO Copyright Treaty adopted on December 20, 1996, and/or similar international agreements.
+
+e. __Exceptions and Limitations__ means fair use, fair dealing, and/or any other exception or limitation to Copyright and Similar Rights that applies to Your use of the Licensed Material.
+
+f. __Licensed Material__ means the artistic or literary work, database, or other material to which the Licensor applied this Public License.
+
+g. __Licensed Rights__ means the rights granted to You subject to the terms and conditions of this Public License, which are limited to all Copyright and Similar Rights that apply to Your use of the Licensed Material and that the Licensor has authority to license.
+
+h. __Licensor__ means the individual(s) or entity(ies) granting rights under this Public License.
+
+i. __NonCommercial__ means not primarily intended for or directed towards commercial advantage or monetary compensation. For purposes of this Public License, the exchange of the Licensed Material for other material subject to Copyright and Similar Rights by digital file-sharing or similar means is NonCommercial provided there is no payment of monetary compensation in connection with the exchange.
+
+j. __Share__ means to provide material to the public by any means or process that requires permission under the Licensed Rights, such as reproduction, public display, public performance, distribution, dissemination, communication, or importation, and to make material available to the public including in ways that members of the public may access the material from a place and at a time individually chosen by them.
+
+k. __Sui Generis Database Rights__ means rights other than copyright resulting from Directive 96/9/EC of the European Parliament and of the Council of 11 March 1996 on the legal protection of databases, as amended and/or succeeded, as well as other essentially equivalent rights anywhere in the world.
+
+l. __You__ means the individual or entity exercising the Licensed Rights under this Public License. Your has a corresponding meaning.
+
+### Section 2 – Scope.
+
+a. ___License grant.___
+
+ 1. Subject to the terms and conditions of this Public License, the Licensor hereby grants You a worldwide, royalty-free, non-sublicensable, non-exclusive, irrevocable license to exercise the Licensed Rights in the Licensed Material to:
+
+ A. reproduce and Share the Licensed Material, in whole or in part, for NonCommercial purposes only; and
+
+ B. produce, reproduce, and Share Adapted Material for NonCommercial purposes only.
+
+ 2. __Exceptions and Limitations.__ For the avoidance of doubt, where Exceptions and Limitations apply to Your use, this Public License does not apply, and You do not need to comply with its terms and conditions.
+
+ 3. __Term.__ The term of this Public License is specified in Section 6(a).
+
+ 4. __Media and formats; technical modifications allowed.__ The Licensor authorizes You to exercise the Licensed Rights in all media and formats whether now known or hereafter created, and to make technical modifications necessary to do so. The Licensor waives and/or agrees not to assert any right or authority to forbid You from making technical modifications necessary to exercise the Licensed Rights, including technical modifications necessary to circumvent Effective Technological Measures. For purposes of this Public License, simply making modifications authorized by this Section 2(a)(4) never produces Adapted Material.
+
+ 5. __Downstream recipients.__
+
+ A. __Offer from the Licensor – Licensed Material.__ Every recipient of the Licensed Material automatically receives an offer from the Licensor to exercise the Licensed Rights under the terms and conditions of this Public License.
+
+ B. __No downstream restrictions.__ You may not offer or impose any additional or different terms or conditions on, or apply any Effective Technological Measures to, the Licensed Material if doing so restricts exercise of the Licensed Rights by any recipient of the Licensed Material.
+
+ 6. __No endorsement.__ Nothing in this Public License constitutes or may be construed as permission to assert or imply that You are, or that Your use of the Licensed Material is, connected with, or sponsored, endorsed, or granted official status by, the Licensor or others designated to receive attribution as provided in Section 3(a)(1)(A)(i).
+
+b. ___Other rights.___
+
+ 1. Moral rights, such as the right of integrity, are not licensed under this Public License, nor are publicity, privacy, and/or other similar personality rights; however, to the extent possible, the Licensor waives and/or agrees not to assert any such rights held by the Licensor to the limited extent necessary to allow You to exercise the Licensed Rights, but not otherwise.
+
+ 2. Patent and trademark rights are not licensed under this Public License.
+
+ 3. To the extent possible, the Licensor waives any right to collect royalties from You for the exercise of the Licensed Rights, whether directly or through a collecting society under any voluntary or waivable statutory or compulsory licensing scheme. In all other cases the Licensor expressly reserves any right to collect such royalties, including when the Licensed Material is used other than for NonCommercial purposes.
+
+### Section 3 – License Conditions.
+
+Your exercise of the Licensed Rights is expressly made subject to the following conditions.
+
+a. ___Attribution.___
+
+ 1. If You Share the Licensed Material (including in modified form), You must:
+
+ A. retain the following if it is supplied by the Licensor with the Licensed Material:
+
+ i. identification of the creator(s) of the Licensed Material and any others designated to receive attribution, in any reasonable manner requested by the Licensor (including by pseudonym if designated);
+
+ ii. a copyright notice;
+
+ iii. a notice that refers to this Public License;
+
+ iv. a notice that refers to the disclaimer of warranties;
+
+ v. a URI or hyperlink to the Licensed Material to the extent reasonably practicable;
+
+ B. indicate if You modified the Licensed Material and retain an indication of any previous modifications; and
+
+ C. indicate the Licensed Material is licensed under this Public License, and include the text of, or the URI or hyperlink to, this Public License.
+
+ 2. You may satisfy the conditions in Section 3(a)(1) in any reasonable manner based on the medium, means, and context in which You Share the Licensed Material. For example, it may be reasonable to satisfy the conditions by providing a URI or hyperlink to a resource that includes the required information.
+
+ 3. If requested by the Licensor, You must remove any of the information required by Section 3(a)(1)(A) to the extent reasonably practicable.
+
+ 4. If You Share Adapted Material You produce, the Adapter's License You apply must not prevent recipients of the Adapted Material from complying with this Public License.
+
+### Section 4 – Sui Generis Database Rights.
+
+Where the Licensed Rights include Sui Generis Database Rights that apply to Your use of the Licensed Material:
+
+a. for the avoidance of doubt, Section 2(a)(1) grants You the right to extract, reuse, reproduce, and Share all or a substantial portion of the contents of the database for NonCommercial purposes only;
+
+b. if You include all or a substantial portion of the database contents in a database in which You have Sui Generis Database Rights, then the database in which You have Sui Generis Database Rights (but not its individual contents) is Adapted Material; and
+
+c. You must comply with the conditions in Section 3(a) if You Share all or a substantial portion of the contents of the database.
+
+For the avoidance of doubt, this Section 4 supplements and does not replace Your obligations under this Public License where the Licensed Rights include other Copyright and Similar Rights.
+
+### Section 5 – Disclaimer of Warranties and Limitation of Liability.
+
+a. __Unless otherwise separately undertaken by the Licensor, to the extent possible, the Licensor offers the Licensed Material as-is and as-available, and makes no representations or warranties of any kind concerning the Licensed Material, whether express, implied, statutory, or other. This includes, without limitation, warranties of title, merchantability, fitness for a particular purpose, non-infringement, absence of latent or other defects, accuracy, or the presence or absence of errors, whether or not known or discoverable. Where disclaimers of warranties are not allowed in full or in part, this disclaimer may not apply to You.__
+
+b. __To the extent possible, in no event will the Licensor be liable to You on any legal theory (including, without limitation, negligence) or otherwise for any direct, special, indirect, incidental, consequential, punitive, exemplary, or other losses, costs, expenses, or damages arising out of this Public License or use of the Licensed Material, even if the Licensor has been advised of the possibility of such losses, costs, expenses, or damages. Where a limitation of liability is not allowed in full or in part, this limitation may not apply to You.__
+
+c. The disclaimer of warranties and limitation of liability provided above shall be interpreted in a manner that, to the extent possible, most closely approximates an absolute disclaimer and waiver of all liability.
+
+### Section 6 – Term and Termination.
+
+a. This Public License applies for the term of the Copyright and Similar Rights licensed here. However, if You fail to comply with this Public License, then Your rights under this Public License terminate automatically.
+
+b. Where Your right to use the Licensed Material has terminated under Section 6(a), it reinstates:
+
+ 1. automatically as of the date the violation is cured, provided it is cured within 30 days of Your discovery of the violation; or
+
+ 2. upon express reinstatement by the Licensor.
+
+ For the avoidance of doubt, this Section 6(b) does not affect any right the Licensor may have to seek remedies for Your violations of this Public License.
+
+c. For the avoidance of doubt, the Licensor may also offer the Licensed Material under separate terms or conditions or stop distributing the Licensed Material at any time; however, doing so will not terminate this Public License.
+
+d. Sections 1, 5, 6, 7, and 8 survive termination of this Public License.
+
+### Section 7 – Other Terms and Conditions.
+
+a. The Licensor shall not be bound by any additional or different terms or conditions communicated by You unless expressly agreed.
+
+b. Any arrangements, understandings, or agreements regarding the Licensed Material not stated herein are separate from and independent of the terms and conditions of this Public License.
+
+### Section 8 – Interpretation.
+
+a. For the avoidance of doubt, this Public License does not, and shall not be interpreted to, reduce, limit, restrict, or impose conditions on any use of the Licensed Material that could lawfully be made without permission under this Public License.
+
+b. To the extent possible, if any provision of this Public License is deemed unenforceable, it shall be automatically reformed to the minimum extent necessary to make it enforceable. If the provision cannot be reformed, it shall be severed from this Public License without affecting the enforceability of the remaining terms and conditions.
+
+c. No term or condition of this Public License will be waived and no failure to comply consented to unless expressly agreed to by the Licensor.
+
+d. Nothing in this Public License constitutes or may be interpreted as a limitation upon, or waiver of, any privileges and immunities that apply to the Licensor or You, including from the legal processes of any jurisdiction or authority.
+
+> Creative Commons is not a party to its public licenses. Notwithstanding, Creative Commons may elect to apply one of its public licenses to material it publishes and in those instances will be considered the “Licensor.” Except for the limited purpose of indicating that material is shared under a Creative Commons public license or as otherwise permitted by the Creative Commons policies published at [creativecommons.org/policies](http://creativecommons.org/policies), Creative Commons does not authorize the use of the trademark “Creative Commons” or any other trademark or logo of Creative Commons without its prior written consent including, without limitation, in connection with any unauthorized modifications to any of its public licenses or any other arrangements, understandings, or agreements concerning use of licensed material. For the avoidance of doubt, this paragraph does not form part of the public licenses.
+>
+> Creative Commons may be contacted at creativecommons.org
\ No newline at end of file
diff --git a/MANIFEST.in b/MANIFEST.in
new file mode 100644
index 0000000..7b9cae6
--- /dev/null
+++ b/MANIFEST.in
@@ -0,0 +1,5 @@
+include mmdet3d/.mim/model-index.yml
+include requirements/*.txt
+recursive-include mmdet3d/.mim/ops *.cpp *.cu *.h *.cc
+recursive-include mmdet3d/.mim/configs *.py *.yml
+recursive-include mmdet3d/.mim/tools *.sh *.py
diff --git a/README.md b/README.md
new file mode 100644
index 0000000..f0e531a
--- /dev/null
+++ b/README.md
@@ -0,0 +1,78 @@
+## Top-Down Beats Bottom-Up in 3D Instance Segmentation
+
+**News**:
+ * :fire: February, 2023. Source code has been published.
+
+This repository contains an implementation of TD3D, a 3D instance segmentation method introduced in our paper:
+
+> **Top-Down Beats Bottom-Up in 3D Instance Segmentation**
+> [Maksim Kolodiazhnyi](https://github.com/col14m),
+> [Danila Rukhovich](https://github.com/filaPro),
+> [Anna Vorontsova](https://github.com/highrut),
+> [Anton Konushin](https://scholar.google.com/citations?user=ZT_k-wMAAAAJ)
+>
+> Samsung AI Center Moscow
+>
+
+### Installation
+For convenience, we provide a [Dockerfile](docker/Dockerfile).
+
+Alternatively, you can install all required packages manually. This implementation is based on [mmdetection3d](https://github.com/open-mmlab/mmdetection3d) framework.
+
+Please refer to the original installation guide [getting_started.md](docs/getting_started.md), including MinkowskiEngine installation, replacing open-mmlab/mmdetection3d with samsunglabs/td3d.
+
+Most of the `TD3D`-related code locates in the following files:
+[detectors/td3d_instance_segmentor.py](mmdet3d/models/detectors/td3d_instance_segmentor.py),
+[necks/ngfc_neck.py](mmdet3d/models/necks/ngfc_neck.py),
+[decode_heads/td3d_instance_head.py](mmdet3d/models/decode_heads/td3d_instance_head.py).
+
+### Getting Started
+
+Please see [getting_started.md](docs/getting_started.md) for basic usage examples.
+We follow the `mmdetection3d` data preparation protocol described in [scannet](data/scannet), [s3dis](data/s3dis).
+
+
+**Training**
+
+To start training, run [train](tools/train.py) with `TD3D` [configs](configs/td3d_is):
+```shell
+python tools/train.py configs/td3d_is/td3d_is_scannet-3d-18class.py
+```
+
+**Testing**
+
+Test pre-trained model using [test](tools/test.py) with `TD3D` [configs](configs/td3d_is). For best quality on Scannet and S3DIS, set `score_thr` to `0.1` and `nms_pre` to `1200` in configs. For best quality on Scannet200, set `score_thr` to `0.07` and `nms_pre` to `300`:
+```shell
+python tools/test.py configs/td3d_is/td3d_is_scannet-3d-18class.py \
+ work_dirs/td3d_is_scannet-3d-18class/latest.pth --eval mAP
+```
+
+**Visualization**
+
+Visualizations can be created with [test](tools/test.py) script.
+For better visualizations, you may set `score_thr` to `0.20` and `nms_pre` to `200` in configs:
+```shell
+python tools/test.py configs/td3d_is/td3d_is_scannet-3d-18class.py \
+ work_dirs/td3d_is_scannet-3d-18class/latest.pth --eval mAP --show \
+ --show-dir work_dirs/td3d_is_scannet-3d-18class
+```
+
+### Models (quality on validation subset)
+
+| Dataset | mAP@0.25 | mAP@0.5 | mAP | Download |
+|:-------:|:--------:|:-------:|:--------:|:--------:|
+| ScanNet | 81.3 | 71.1 | 46.2 | [model]() | [config]() |
+| S3DIS (5 area) | 82.8 | 66.5 | 47.4 | [model]() | [config]() |
+| S3DIS (5 area)
(ScanNet pretrain) | 85.6 | 75.5 | 61.1 | [model]() | [config]() |
+| Scannet200 | 39.7 | 33.3 | 22.2 | [model]() | [config]() |
+
+
+
+
diff --git a/configs/3dssd/3dssd_4x4_kitti-3d-car.py b/configs/3dssd/3dssd_4x4_kitti-3d-car.py
new file mode 100644
index 0000000..bcc8c82
--- /dev/null
+++ b/configs/3dssd/3dssd_4x4_kitti-3d-car.py
@@ -0,0 +1,121 @@
+_base_ = [
+ '../_base_/models/3dssd.py', '../_base_/datasets/kitti-3d-car.py',
+ '../_base_/default_runtime.py'
+]
+
+# dataset settings
+dataset_type = 'KittiDataset'
+data_root = 'data/kitti/'
+class_names = ['Car']
+point_cloud_range = [0, -40, -5, 70, 40, 3]
+input_modality = dict(use_lidar=True, use_camera=False)
+db_sampler = dict(
+ data_root=data_root,
+ info_path=data_root + 'kitti_dbinfos_train.pkl',
+ rate=1.0,
+ prepare=dict(filter_by_difficulty=[-1], filter_by_min_points=dict(Car=5)),
+ classes=class_names,
+ sample_groups=dict(Car=15))
+
+file_client_args = dict(backend='disk')
+# Uncomment the following if use ceph or other file clients.
+# See https://mmcv.readthedocs.io/en/latest/api.html#mmcv.fileio.FileClient
+# for more details.
+# file_client_args = dict(
+# backend='petrel', path_mapping=dict(data='s3://kitti_data/'))
+
+train_pipeline = [
+ dict(
+ type='LoadPointsFromFile',
+ coord_type='LIDAR',
+ load_dim=4,
+ use_dim=4,
+ file_client_args=file_client_args),
+ dict(
+ type='LoadAnnotations3D',
+ with_bbox_3d=True,
+ with_label_3d=True,
+ file_client_args=file_client_args),
+ dict(type='PointsRangeFilter', point_cloud_range=point_cloud_range),
+ dict(type='ObjectRangeFilter', point_cloud_range=point_cloud_range),
+ dict(type='ObjectSample', db_sampler=db_sampler),
+ dict(type='RandomFlip3D', flip_ratio_bev_horizontal=0.5),
+ dict(
+ type='ObjectNoise',
+ num_try=100,
+ translation_std=[1.0, 1.0, 0],
+ global_rot_range=[0.0, 0.0],
+ rot_range=[-1.0471975511965976, 1.0471975511965976]),
+ dict(
+ type='GlobalRotScaleTrans',
+ rot_range=[-0.78539816, 0.78539816],
+ scale_ratio_range=[0.9, 1.1]),
+ # 3DSSD can get a higher performance without this transform
+ # dict(type='BackgroundPointsFilter', bbox_enlarge_range=(0.5, 2.0, 0.5)),
+ dict(type='PointSample', num_points=16384),
+ dict(type='DefaultFormatBundle3D', class_names=class_names),
+ dict(type='Collect3D', keys=['points', 'gt_bboxes_3d', 'gt_labels_3d'])
+]
+
+test_pipeline = [
+ dict(
+ type='LoadPointsFromFile',
+ coord_type='LIDAR',
+ load_dim=4,
+ use_dim=4,
+ file_client_args=file_client_args),
+ dict(
+ type='MultiScaleFlipAug3D',
+ img_scale=(1333, 800),
+ pts_scale_ratio=1,
+ flip=False,
+ transforms=[
+ dict(
+ type='GlobalRotScaleTrans',
+ rot_range=[0, 0],
+ scale_ratio_range=[1., 1.],
+ translation_std=[0, 0, 0]),
+ dict(type='RandomFlip3D'),
+ dict(
+ type='PointsRangeFilter', point_cloud_range=point_cloud_range),
+ dict(type='PointSample', num_points=16384),
+ dict(
+ type='DefaultFormatBundle3D',
+ class_names=class_names,
+ with_label=False),
+ dict(type='Collect3D', keys=['points'])
+ ])
+]
+
+data = dict(
+ samples_per_gpu=4,
+ workers_per_gpu=4,
+ train=dict(dataset=dict(pipeline=train_pipeline)),
+ val=dict(pipeline=test_pipeline),
+ test=dict(pipeline=test_pipeline))
+
+evaluation = dict(interval=2)
+
+# model settings
+model = dict(
+ bbox_head=dict(
+ num_classes=1,
+ bbox_coder=dict(
+ type='AnchorFreeBBoxCoder', num_dir_bins=12, with_rot=True)))
+
+# optimizer
+lr = 0.002 # max learning rate
+optimizer = dict(type='AdamW', lr=lr, weight_decay=0)
+optimizer_config = dict(grad_clip=dict(max_norm=35, norm_type=2))
+lr_config = dict(policy='step', warmup=None, step=[45, 60])
+# runtime settings
+runner = dict(type='EpochBasedRunner', max_epochs=80)
+
+# yapf:disable
+log_config = dict(
+ interval=30,
+ hooks=[
+ dict(type='TextLoggerHook'),
+ dict(type='TensorboardLoggerHook')
+ ])
+# yapf:enable
diff --git a/configs/3dssd/README.md b/configs/3dssd/README.md
new file mode 100644
index 0000000..4feb6d7
--- /dev/null
+++ b/configs/3dssd/README.md
@@ -0,0 +1,45 @@
+# 3DSSD: Point-based 3D Single Stage Object Detector
+
+> [3DSSD: Point-based 3D Single Stage Object Detector](https://arxiv.org/abs/2002.10187)
+
+
+
+## Abstract
+
+Currently, there have been many kinds of voxel-based 3D single stage detectors, while point-based single stage methods are still underexplored. In this paper, we first present a lightweight and effective point-based 3D single stage object detector, named 3DSSD, achieving a good balance between accuracy and efficiency. In this paradigm, all upsampling layers and refinement stage, which are indispensable in all existing point-based methods, are abandoned to reduce the large computation cost. We novelly propose a fusion sampling strategy in downsampling process to make detection on less representative points feasible. A delicate box prediction network including a candidate generation layer, an anchor-free regression head with a 3D center-ness assignment strategy is designed to meet with our demand of accuracy and speed. Our paradigm is an elegant single stage anchor-free framework, showing great superiority to other existing methods. We evaluate 3DSSD on widely used KITTI dataset and more challenging nuScenes dataset. Our method outperforms all state-of-the-art voxel-based single stage methods by a large margin, and has comparable performance to two stage point-based methods as well, with inference speed more than 25 FPS, 2x faster than former state-of-the-art point-based methods.
+
+
+
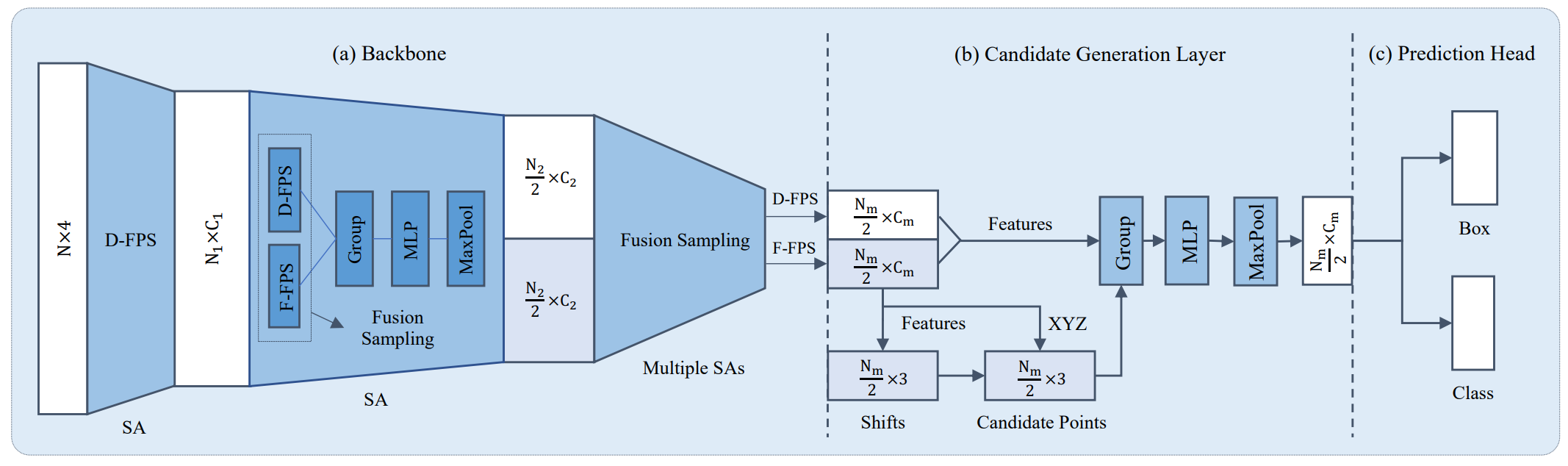
+
+
+## Introduction
+
+We implement 3DSSD and provide the results and checkpoints on KITTI datasets.
+
+Some settings in our implementation are different from the [official implementation](https://github.com/Jia-Research-Lab/3DSSD), which bring marginal differences to the performance on KITTI datasets in our experiments. To simplify and unify the models of our implementation, we skip them in our models. These differences are listed as below:
+
+1. We keep the scenes without any object while the official code skips these scenes in training. In the official implementation, only 3229 and 3394 samples are used as training and validation sets, respectively. In our implementation, we keep using 3712 and 3769 samples as training and validation sets, respectively, as those used for all the other models in our implementation on KITTI datasets.
+2. We do not modify the decay of `batch normalization` during training.
+3. While using [`DataBaseSampler`](https://github.com/open-mmlab/mmdetection3d/blob/master/mmdet3d/datasets/pipelines/dbsampler.py#L80) for data augmentation, the official code uses road planes as reference to place the sampled objects while we do not.
+4. We perform detection using LIDAR coordinates while the official code uses camera coordinates.
+
+## Results and models
+
+### KITTI
+
+| Backbone | Class | Lr schd | Mem (GB) | Inf time (fps) | mAP | Download |
+| :-------------------------------------------: | :---: | :-----: | :------: | :------------: | :----------------------: | :----------------------------------------------------------------------------------------------------------------------------------------------------------------------------------------------------------------------------------------------------------------------------------------------------: |
+| [PointNet2SAMSG](./3dssd_4x4_kitti-3d-car.py) | Car | 72e | 4.7 | | 78.58(81.27)1 | [model](https://download.openmmlab.com/mmdetection3d/v1.0.0_models/3dssd/3dssd_4x4_kitti-3d-car/3dssd_4x4_kitti-3d-car_20210818_203828-b89c8fc4.pth) \| [log](https://download.openmmlab.com/mmdetection3d/v1.0.0_models/3dssd/3dssd_4x4_kitti-3d-car/3dssd_4x4_kitti-3d-car_20210818_203828.log.json) |
+
+\[1\]: We report two different 3D object detection performance here. 78.58mAP is evaluated by our evaluation code and 81.27mAP is evaluated by the official development kit (so as that used in the paper and official code of 3DSSD ). We found that the commonly used Python implementation of [`rotate_iou`](https://github.com/traveller59/second.pytorch/blob/e42e4a0e17262ab7d180ee96a0a36427f2c20a44/second/core/non_max_suppression/nms_gpu.py#L605) which is used in our KITTI dataset evaluation, is different from the official implementation in [KITTI benchmark](http://www.cvlibs.net/datasets/kitti/eval_object.php?obj_benchmark=3d).
+
+## Citation
+
+```latex
+@inproceedings{yang20203dssd,
+ author = {Zetong Yang and Yanan Sun and Shu Liu and Jiaya Jia},
+ title = {3DSSD: Point-based 3D Single Stage Object Detector},
+ booktitle = {Proceedings of the IEEE/CVF Conference on Computer Vision and Pattern Recognition},
+ year = {2020}
+}
+```
diff --git a/configs/3dssd/metafile.yml b/configs/3dssd/metafile.yml
new file mode 100644
index 0000000..f6dbb3c
--- /dev/null
+++ b/configs/3dssd/metafile.yml
@@ -0,0 +1,29 @@
+Collections:
+ - Name: 3DSSD
+ Metadata:
+ Training Data: KITTI
+ Training Techniques:
+ - AdamW
+ Training Resources: 4x TITAN X
+ Architecture:
+ - PointNet++
+ Paper:
+ URL: https://arxiv.org/abs/2002.10187
+ Title: '3DSSD: Point-based 3D Single Stage Object Detector'
+ README: configs/3dssd/README.md
+ Code:
+ URL: https://github.com/open-mmlab/mmdetection3d/blob/master/mmdet3d/models/detectors/ssd3dnet.py#L7
+ Version: v0.6.0
+
+Models:
+ - Name: 3dssd_4x4_kitti-3d-car
+ In Collection: 3DSSD
+ Config: configs/3dssd/3dssd_4x4_kitti-3d-car.py
+ Metadata:
+ Training Memory (GB): 4.7
+ Results:
+ - Task: 3D Object Detection
+ Dataset: KITTI
+ Metrics:
+ mAP: 78.58
+ Weights: https://download.openmmlab.com/mmdetection3d/v1.0.0_models/3dssd/3dssd_4x4_kitti-3d-car/3dssd_4x4_kitti-3d-car_20210818_203828-b89c8fc4.pth
diff --git a/configs/_base_/datasets/coco_instance.py b/configs/_base_/datasets/coco_instance.py
new file mode 100644
index 0000000..f6ea4f4
--- /dev/null
+++ b/configs/_base_/datasets/coco_instance.py
@@ -0,0 +1,48 @@
+dataset_type = 'CocoDataset'
+data_root = 'data/coco/'
+img_norm_cfg = dict(
+ mean=[123.675, 116.28, 103.53], std=[58.395, 57.12, 57.375], to_rgb=True)
+train_pipeline = [
+ dict(type='LoadImageFromFile'),
+ dict(type='LoadAnnotations', with_bbox=True, with_mask=True),
+ dict(type='Resize', img_scale=(1333, 800), keep_ratio=True),
+ dict(type='RandomFlip', flip_ratio=0.5),
+ dict(type='Normalize', **img_norm_cfg),
+ dict(type='Pad', size_divisor=32),
+ dict(type='DefaultFormatBundle'),
+ dict(type='Collect', keys=['img', 'gt_bboxes', 'gt_labels', 'gt_masks']),
+]
+test_pipeline = [
+ dict(type='LoadImageFromFile'),
+ dict(
+ type='MultiScaleFlipAug',
+ img_scale=(1333, 800),
+ flip=False,
+ transforms=[
+ dict(type='Resize', keep_ratio=True),
+ dict(type='RandomFlip'),
+ dict(type='Normalize', **img_norm_cfg),
+ dict(type='Pad', size_divisor=32),
+ dict(type='ImageToTensor', keys=['img']),
+ dict(type='Collect', keys=['img']),
+ ])
+]
+data = dict(
+ samples_per_gpu=2,
+ workers_per_gpu=2,
+ train=dict(
+ type=dataset_type,
+ ann_file=data_root + 'annotations/instances_train2017.json',
+ img_prefix=data_root + 'train2017/',
+ pipeline=train_pipeline),
+ val=dict(
+ type=dataset_type,
+ ann_file=data_root + 'annotations/instances_val2017.json',
+ img_prefix=data_root + 'val2017/',
+ pipeline=test_pipeline),
+ test=dict(
+ type=dataset_type,
+ ann_file=data_root + 'annotations/instances_val2017.json',
+ img_prefix=data_root + 'val2017/',
+ pipeline=test_pipeline))
+evaluation = dict(metric=['bbox', 'segm'])
diff --git a/configs/_base_/datasets/kitti-3d-3class.py b/configs/_base_/datasets/kitti-3d-3class.py
new file mode 100644
index 0000000..1822af4
--- /dev/null
+++ b/configs/_base_/datasets/kitti-3d-3class.py
@@ -0,0 +1,140 @@
+# dataset settings
+dataset_type = 'KittiDataset'
+data_root = 'data/kitti/'
+class_names = ['Pedestrian', 'Cyclist', 'Car']
+point_cloud_range = [0, -40, -3, 70.4, 40, 1]
+input_modality = dict(use_lidar=True, use_camera=False)
+db_sampler = dict(
+ data_root=data_root,
+ info_path=data_root + 'kitti_dbinfos_train.pkl',
+ rate=1.0,
+ prepare=dict(
+ filter_by_difficulty=[-1],
+ filter_by_min_points=dict(Car=5, Pedestrian=10, Cyclist=10)),
+ classes=class_names,
+ sample_groups=dict(Car=12, Pedestrian=6, Cyclist=6))
+
+file_client_args = dict(backend='disk')
+# Uncomment the following if use ceph or other file clients.
+# See https://mmcv.readthedocs.io/en/latest/api.html#mmcv.fileio.FileClient
+# for more details.
+# file_client_args = dict(
+# backend='petrel', path_mapping=dict(data='s3://kitti_data/'))
+
+train_pipeline = [
+ dict(
+ type='LoadPointsFromFile',
+ coord_type='LIDAR',
+ load_dim=4,
+ use_dim=4,
+ file_client_args=file_client_args),
+ dict(
+ type='LoadAnnotations3D',
+ with_bbox_3d=True,
+ with_label_3d=True,
+ file_client_args=file_client_args),
+ dict(type='ObjectSample', db_sampler=db_sampler),
+ dict(
+ type='ObjectNoise',
+ num_try=100,
+ translation_std=[1.0, 1.0, 0.5],
+ global_rot_range=[0.0, 0.0],
+ rot_range=[-0.78539816, 0.78539816]),
+ dict(type='RandomFlip3D', flip_ratio_bev_horizontal=0.5),
+ dict(
+ type='GlobalRotScaleTrans',
+ rot_range=[-0.78539816, 0.78539816],
+ scale_ratio_range=[0.95, 1.05]),
+ dict(type='PointsRangeFilter', point_cloud_range=point_cloud_range),
+ dict(type='ObjectRangeFilter', point_cloud_range=point_cloud_range),
+ dict(type='PointShuffle'),
+ dict(type='DefaultFormatBundle3D', class_names=class_names),
+ dict(type='Collect3D', keys=['points', 'gt_bboxes_3d', 'gt_labels_3d'])
+]
+test_pipeline = [
+ dict(
+ type='LoadPointsFromFile',
+ coord_type='LIDAR',
+ load_dim=4,
+ use_dim=4,
+ file_client_args=file_client_args),
+ dict(
+ type='MultiScaleFlipAug3D',
+ img_scale=(1333, 800),
+ pts_scale_ratio=1,
+ flip=False,
+ transforms=[
+ dict(
+ type='GlobalRotScaleTrans',
+ rot_range=[0, 0],
+ scale_ratio_range=[1., 1.],
+ translation_std=[0, 0, 0]),
+ dict(type='RandomFlip3D'),
+ dict(
+ type='PointsRangeFilter', point_cloud_range=point_cloud_range),
+ dict(
+ type='DefaultFormatBundle3D',
+ class_names=class_names,
+ with_label=False),
+ dict(type='Collect3D', keys=['points'])
+ ])
+]
+# construct a pipeline for data and gt loading in show function
+# please keep its loading function consistent with test_pipeline (e.g. client)
+eval_pipeline = [
+ dict(
+ type='LoadPointsFromFile',
+ coord_type='LIDAR',
+ load_dim=4,
+ use_dim=4,
+ file_client_args=file_client_args),
+ dict(
+ type='DefaultFormatBundle3D',
+ class_names=class_names,
+ with_label=False),
+ dict(type='Collect3D', keys=['points'])
+]
+
+data = dict(
+ samples_per_gpu=6,
+ workers_per_gpu=4,
+ train=dict(
+ type='RepeatDataset',
+ times=2,
+ dataset=dict(
+ type=dataset_type,
+ data_root=data_root,
+ ann_file=data_root + 'kitti_infos_train.pkl',
+ split='training',
+ pts_prefix='velodyne_reduced',
+ pipeline=train_pipeline,
+ modality=input_modality,
+ classes=class_names,
+ test_mode=False,
+ # we use box_type_3d='LiDAR' in kitti and nuscenes dataset
+ # and box_type_3d='Depth' in sunrgbd and scannet dataset.
+ box_type_3d='LiDAR')),
+ val=dict(
+ type=dataset_type,
+ data_root=data_root,
+ ann_file=data_root + 'kitti_infos_val.pkl',
+ split='training',
+ pts_prefix='velodyne_reduced',
+ pipeline=test_pipeline,
+ modality=input_modality,
+ classes=class_names,
+ test_mode=True,
+ box_type_3d='LiDAR'),
+ test=dict(
+ type=dataset_type,
+ data_root=data_root,
+ ann_file=data_root + 'kitti_infos_val.pkl',
+ split='training',
+ pts_prefix='velodyne_reduced',
+ pipeline=test_pipeline,
+ modality=input_modality,
+ classes=class_names,
+ test_mode=True,
+ box_type_3d='LiDAR'))
+
+evaluation = dict(interval=1, pipeline=eval_pipeline)
diff --git a/configs/_base_/datasets/kitti-3d-car.py b/configs/_base_/datasets/kitti-3d-car.py
new file mode 100644
index 0000000..1e81226
--- /dev/null
+++ b/configs/_base_/datasets/kitti-3d-car.py
@@ -0,0 +1,138 @@
+# dataset settings
+dataset_type = 'KittiDataset'
+data_root = 'data/kitti/'
+class_names = ['Car']
+point_cloud_range = [0, -40, -3, 70.4, 40, 1]
+input_modality = dict(use_lidar=True, use_camera=False)
+db_sampler = dict(
+ data_root=data_root,
+ info_path=data_root + 'kitti_dbinfos_train.pkl',
+ rate=1.0,
+ prepare=dict(filter_by_difficulty=[-1], filter_by_min_points=dict(Car=5)),
+ classes=class_names,
+ sample_groups=dict(Car=15))
+
+file_client_args = dict(backend='disk')
+# Uncomment the following if use ceph or other file clients.
+# See https://mmcv.readthedocs.io/en/latest/api.html#mmcv.fileio.FileClient
+# for more details.
+# file_client_args = dict(
+# backend='petrel', path_mapping=dict(data='s3://kitti_data/'))
+
+train_pipeline = [
+ dict(
+ type='LoadPointsFromFile',
+ coord_type='LIDAR',
+ load_dim=4,
+ use_dim=4,
+ file_client_args=file_client_args),
+ dict(
+ type='LoadAnnotations3D',
+ with_bbox_3d=True,
+ with_label_3d=True,
+ file_client_args=file_client_args),
+ dict(type='ObjectSample', db_sampler=db_sampler),
+ dict(
+ type='ObjectNoise',
+ num_try=100,
+ translation_std=[1.0, 1.0, 0.5],
+ global_rot_range=[0.0, 0.0],
+ rot_range=[-0.78539816, 0.78539816]),
+ dict(type='RandomFlip3D', flip_ratio_bev_horizontal=0.5),
+ dict(
+ type='GlobalRotScaleTrans',
+ rot_range=[-0.78539816, 0.78539816],
+ scale_ratio_range=[0.95, 1.05]),
+ dict(type='PointsRangeFilter', point_cloud_range=point_cloud_range),
+ dict(type='ObjectRangeFilter', point_cloud_range=point_cloud_range),
+ dict(type='PointShuffle'),
+ dict(type='DefaultFormatBundle3D', class_names=class_names),
+ dict(type='Collect3D', keys=['points', 'gt_bboxes_3d', 'gt_labels_3d'])
+]
+test_pipeline = [
+ dict(
+ type='LoadPointsFromFile',
+ coord_type='LIDAR',
+ load_dim=4,
+ use_dim=4,
+ file_client_args=file_client_args),
+ dict(
+ type='MultiScaleFlipAug3D',
+ img_scale=(1333, 800),
+ pts_scale_ratio=1,
+ flip=False,
+ transforms=[
+ dict(
+ type='GlobalRotScaleTrans',
+ rot_range=[0, 0],
+ scale_ratio_range=[1., 1.],
+ translation_std=[0, 0, 0]),
+ dict(type='RandomFlip3D'),
+ dict(
+ type='PointsRangeFilter', point_cloud_range=point_cloud_range),
+ dict(
+ type='DefaultFormatBundle3D',
+ class_names=class_names,
+ with_label=False),
+ dict(type='Collect3D', keys=['points'])
+ ])
+]
+# construct a pipeline for data and gt loading in show function
+# please keep its loading function consistent with test_pipeline (e.g. client)
+eval_pipeline = [
+ dict(
+ type='LoadPointsFromFile',
+ coord_type='LIDAR',
+ load_dim=4,
+ use_dim=4,
+ file_client_args=file_client_args),
+ dict(
+ type='DefaultFormatBundle3D',
+ class_names=class_names,
+ with_label=False),
+ dict(type='Collect3D', keys=['points'])
+]
+
+data = dict(
+ samples_per_gpu=6,
+ workers_per_gpu=4,
+ train=dict(
+ type='RepeatDataset',
+ times=2,
+ dataset=dict(
+ type=dataset_type,
+ data_root=data_root,
+ ann_file=data_root + 'kitti_infos_train.pkl',
+ split='training',
+ pts_prefix='velodyne_reduced',
+ pipeline=train_pipeline,
+ modality=input_modality,
+ classes=class_names,
+ test_mode=False,
+ # we use box_type_3d='LiDAR' in kitti and nuscenes dataset
+ # and box_type_3d='Depth' in sunrgbd and scannet dataset.
+ box_type_3d='LiDAR')),
+ val=dict(
+ type=dataset_type,
+ data_root=data_root,
+ ann_file=data_root + 'kitti_infos_val.pkl',
+ split='training',
+ pts_prefix='velodyne_reduced',
+ pipeline=test_pipeline,
+ modality=input_modality,
+ classes=class_names,
+ test_mode=True,
+ box_type_3d='LiDAR'),
+ test=dict(
+ type=dataset_type,
+ data_root=data_root,
+ ann_file=data_root + 'kitti_infos_val.pkl',
+ split='training',
+ pts_prefix='velodyne_reduced',
+ pipeline=test_pipeline,
+ modality=input_modality,
+ classes=class_names,
+ test_mode=True,
+ box_type_3d='LiDAR'))
+
+evaluation = dict(interval=1, pipeline=eval_pipeline)
diff --git a/configs/_base_/datasets/kitti-mono3d.py b/configs/_base_/datasets/kitti-mono3d.py
new file mode 100644
index 0000000..5817dc7
--- /dev/null
+++ b/configs/_base_/datasets/kitti-mono3d.py
@@ -0,0 +1,92 @@
+dataset_type = 'KittiMonoDataset'
+data_root = 'data/kitti/'
+class_names = ['Pedestrian', 'Cyclist', 'Car']
+input_modality = dict(use_lidar=False, use_camera=True)
+img_norm_cfg = dict(
+ mean=[123.675, 116.28, 103.53], std=[58.395, 57.12, 57.375], to_rgb=True)
+train_pipeline = [
+ dict(type='LoadImageFromFileMono3D'),
+ dict(
+ type='LoadAnnotations3D',
+ with_bbox=True,
+ with_label=True,
+ with_attr_label=False,
+ with_bbox_3d=True,
+ with_label_3d=True,
+ with_bbox_depth=True),
+ dict(type='Resize', img_scale=(1242, 375), keep_ratio=True),
+ dict(type='RandomFlip3D', flip_ratio_bev_horizontal=0.5),
+ dict(type='Normalize', **img_norm_cfg),
+ dict(type='Pad', size_divisor=32),
+ dict(type='DefaultFormatBundle3D', class_names=class_names),
+ dict(
+ type='Collect3D',
+ keys=[
+ 'img', 'gt_bboxes', 'gt_labels', 'gt_bboxes_3d', 'gt_labels_3d',
+ 'centers2d', 'depths'
+ ]),
+]
+test_pipeline = [
+ dict(type='LoadImageFromFileMono3D'),
+ dict(
+ type='MultiScaleFlipAug',
+ img_scale=(1242, 375),
+ flip=False,
+ transforms=[
+ dict(type='RandomFlip3D'),
+ dict(type='Normalize', **img_norm_cfg),
+ dict(type='Pad', size_divisor=32),
+ dict(
+ type='DefaultFormatBundle3D',
+ class_names=class_names,
+ with_label=False),
+ dict(type='Collect3D', keys=['img']),
+ ])
+]
+# construct a pipeline for data and gt loading in show function
+# please keep its loading function consistent with test_pipeline (e.g. client)
+eval_pipeline = [
+ dict(type='LoadImageFromFileMono3D'),
+ dict(
+ type='DefaultFormatBundle3D',
+ class_names=class_names,
+ with_label=False),
+ dict(type='Collect3D', keys=['img'])
+]
+data = dict(
+ samples_per_gpu=2,
+ workers_per_gpu=2,
+ train=dict(
+ type=dataset_type,
+ data_root=data_root,
+ ann_file=data_root + 'kitti_infos_train_mono3d.coco.json',
+ info_file=data_root + 'kitti_infos_train.pkl',
+ img_prefix=data_root,
+ classes=class_names,
+ pipeline=train_pipeline,
+ modality=input_modality,
+ test_mode=False,
+ box_type_3d='Camera'),
+ val=dict(
+ type=dataset_type,
+ data_root=data_root,
+ ann_file=data_root + 'kitti_infos_val_mono3d.coco.json',
+ info_file=data_root + 'kitti_infos_val.pkl',
+ img_prefix=data_root,
+ classes=class_names,
+ pipeline=test_pipeline,
+ modality=input_modality,
+ test_mode=True,
+ box_type_3d='Camera'),
+ test=dict(
+ type=dataset_type,
+ data_root=data_root,
+ ann_file=data_root + 'kitti_infos_val_mono3d.coco.json',
+ info_file=data_root + 'kitti_infos_val.pkl',
+ img_prefix=data_root,
+ classes=class_names,
+ pipeline=test_pipeline,
+ modality=input_modality,
+ test_mode=True,
+ box_type_3d='Camera'))
+evaluation = dict(interval=2)
diff --git a/configs/_base_/datasets/lyft-3d.py b/configs/_base_/datasets/lyft-3d.py
new file mode 100644
index 0000000..71baff0
--- /dev/null
+++ b/configs/_base_/datasets/lyft-3d.py
@@ -0,0 +1,136 @@
+# If point cloud range is changed, the models should also change their point
+# cloud range accordingly
+point_cloud_range = [-80, -80, -5, 80, 80, 3]
+# For Lyft we usually do 9-class detection
+class_names = [
+ 'car', 'truck', 'bus', 'emergency_vehicle', 'other_vehicle', 'motorcycle',
+ 'bicycle', 'pedestrian', 'animal'
+]
+dataset_type = 'LyftDataset'
+data_root = 'data/lyft/'
+# Input modality for Lyft dataset, this is consistent with the submission
+# format which requires the information in input_modality.
+input_modality = dict(
+ use_lidar=True,
+ use_camera=False,
+ use_radar=False,
+ use_map=False,
+ use_external=False)
+file_client_args = dict(backend='disk')
+# Uncomment the following if use ceph or other file clients.
+# See https://mmcv.readthedocs.io/en/latest/api.html#mmcv.fileio.FileClient
+# for more details.
+# file_client_args = dict(
+# backend='petrel',
+# path_mapping=dict({
+# './data/lyft/': 's3://lyft/lyft/',
+# 'data/lyft/': 's3://lyft/lyft/'
+# }))
+train_pipeline = [
+ dict(
+ type='LoadPointsFromFile',
+ coord_type='LIDAR',
+ load_dim=5,
+ use_dim=5,
+ file_client_args=file_client_args),
+ dict(
+ type='LoadPointsFromMultiSweeps',
+ sweeps_num=10,
+ file_client_args=file_client_args),
+ dict(type='LoadAnnotations3D', with_bbox_3d=True, with_label_3d=True),
+ dict(
+ type='GlobalRotScaleTrans',
+ rot_range=[-0.3925, 0.3925],
+ scale_ratio_range=[0.95, 1.05],
+ translation_std=[0, 0, 0]),
+ dict(type='RandomFlip3D', flip_ratio_bev_horizontal=0.5),
+ dict(type='PointsRangeFilter', point_cloud_range=point_cloud_range),
+ dict(type='ObjectRangeFilter', point_cloud_range=point_cloud_range),
+ dict(type='PointShuffle'),
+ dict(type='DefaultFormatBundle3D', class_names=class_names),
+ dict(type='Collect3D', keys=['points', 'gt_bboxes_3d', 'gt_labels_3d'])
+]
+test_pipeline = [
+ dict(
+ type='LoadPointsFromFile',
+ coord_type='LIDAR',
+ load_dim=5,
+ use_dim=5,
+ file_client_args=file_client_args),
+ dict(
+ type='LoadPointsFromMultiSweeps',
+ sweeps_num=10,
+ file_client_args=file_client_args),
+ dict(
+ type='MultiScaleFlipAug3D',
+ img_scale=(1333, 800),
+ pts_scale_ratio=1,
+ flip=False,
+ transforms=[
+ dict(
+ type='GlobalRotScaleTrans',
+ rot_range=[0, 0],
+ scale_ratio_range=[1., 1.],
+ translation_std=[0, 0, 0]),
+ dict(type='RandomFlip3D'),
+ dict(
+ type='PointsRangeFilter', point_cloud_range=point_cloud_range),
+ dict(
+ type='DefaultFormatBundle3D',
+ class_names=class_names,
+ with_label=False),
+ dict(type='Collect3D', keys=['points'])
+ ])
+]
+# construct a pipeline for data and gt loading in show function
+# please keep its loading function consistent with test_pipeline (e.g. client)
+eval_pipeline = [
+ dict(
+ type='LoadPointsFromFile',
+ coord_type='LIDAR',
+ load_dim=5,
+ use_dim=5,
+ file_client_args=file_client_args),
+ dict(
+ type='LoadPointsFromMultiSweeps',
+ sweeps_num=10,
+ file_client_args=file_client_args),
+ dict(
+ type='DefaultFormatBundle3D',
+ class_names=class_names,
+ with_label=False),
+ dict(type='Collect3D', keys=['points'])
+]
+
+data = dict(
+ samples_per_gpu=2,
+ workers_per_gpu=2,
+ train=dict(
+ type=dataset_type,
+ data_root=data_root,
+ ann_file=data_root + 'lyft_infos_train.pkl',
+ pipeline=train_pipeline,
+ classes=class_names,
+ modality=input_modality,
+ test_mode=False),
+ val=dict(
+ type=dataset_type,
+ data_root=data_root,
+ ann_file=data_root + 'lyft_infos_val.pkl',
+ pipeline=test_pipeline,
+ classes=class_names,
+ modality=input_modality,
+ test_mode=True),
+ test=dict(
+ type=dataset_type,
+ data_root=data_root,
+ ann_file=data_root + 'lyft_infos_test.pkl',
+ pipeline=test_pipeline,
+ classes=class_names,
+ modality=input_modality,
+ test_mode=True))
+# For Lyft dataset, we usually evaluate the model at the end of training.
+# Since the models are trained by 24 epochs by default, we set evaluation
+# interval to be 24. Please change the interval accordingly if you do not
+# use a default schedule.
+evaluation = dict(interval=24, pipeline=eval_pipeline)
diff --git a/configs/_base_/datasets/nuim_instance.py b/configs/_base_/datasets/nuim_instance.py
new file mode 100644
index 0000000..82fce56
--- /dev/null
+++ b/configs/_base_/datasets/nuim_instance.py
@@ -0,0 +1,59 @@
+dataset_type = 'CocoDataset'
+data_root = 'data/nuimages/'
+class_names = [
+ 'car', 'truck', 'trailer', 'bus', 'construction_vehicle', 'bicycle',
+ 'motorcycle', 'pedestrian', 'traffic_cone', 'barrier'
+]
+img_norm_cfg = dict(
+ mean=[123.675, 116.28, 103.53], std=[58.395, 57.12, 57.375], to_rgb=True)
+train_pipeline = [
+ dict(type='LoadImageFromFile'),
+ dict(type='LoadAnnotations', with_bbox=True, with_mask=True),
+ dict(
+ type='Resize',
+ img_scale=[(1280, 720), (1920, 1080)],
+ multiscale_mode='range',
+ keep_ratio=True),
+ dict(type='RandomFlip', flip_ratio=0.5),
+ dict(type='Normalize', **img_norm_cfg),
+ dict(type='Pad', size_divisor=32),
+ dict(type='DefaultFormatBundle'),
+ dict(type='Collect', keys=['img', 'gt_bboxes', 'gt_labels', 'gt_masks']),
+]
+test_pipeline = [
+ dict(type='LoadImageFromFile'),
+ dict(
+ type='MultiScaleFlipAug',
+ img_scale=(1600, 900),
+ flip=False,
+ transforms=[
+ dict(type='Resize', keep_ratio=True),
+ dict(type='RandomFlip'),
+ dict(type='Normalize', **img_norm_cfg),
+ dict(type='Pad', size_divisor=32),
+ dict(type='ImageToTensor', keys=['img']),
+ dict(type='Collect', keys=['img']),
+ ])
+]
+data = dict(
+ samples_per_gpu=2,
+ workers_per_gpu=2,
+ train=dict(
+ type=dataset_type,
+ ann_file=data_root + 'annotations/nuimages_v1.0-train.json',
+ img_prefix=data_root,
+ classes=class_names,
+ pipeline=train_pipeline),
+ val=dict(
+ type=dataset_type,
+ ann_file=data_root + 'annotations/nuimages_v1.0-val.json',
+ img_prefix=data_root,
+ classes=class_names,
+ pipeline=test_pipeline),
+ test=dict(
+ type=dataset_type,
+ ann_file=data_root + 'annotations/nuimages_v1.0-val.json',
+ img_prefix=data_root,
+ classes=class_names,
+ pipeline=test_pipeline))
+evaluation = dict(metric=['bbox', 'segm'])
diff --git a/configs/_base_/datasets/nus-3d.py b/configs/_base_/datasets/nus-3d.py
new file mode 100644
index 0000000..1548171
--- /dev/null
+++ b/configs/_base_/datasets/nus-3d.py
@@ -0,0 +1,142 @@
+# If point cloud range is changed, the models should also change their point
+# cloud range accordingly
+point_cloud_range = [-50, -50, -5, 50, 50, 3]
+# For nuScenes we usually do 10-class detection
+class_names = [
+ 'car', 'truck', 'trailer', 'bus', 'construction_vehicle', 'bicycle',
+ 'motorcycle', 'pedestrian', 'traffic_cone', 'barrier'
+]
+dataset_type = 'NuScenesDataset'
+data_root = 'data/nuscenes/'
+# Input modality for nuScenes dataset, this is consistent with the submission
+# format which requires the information in input_modality.
+input_modality = dict(
+ use_lidar=True,
+ use_camera=False,
+ use_radar=False,
+ use_map=False,
+ use_external=False)
+file_client_args = dict(backend='disk')
+# Uncomment the following if use ceph or other file clients.
+# See https://mmcv.readthedocs.io/en/latest/api.html#mmcv.fileio.FileClient
+# for more details.
+# file_client_args = dict(
+# backend='petrel',
+# path_mapping=dict({
+# './data/nuscenes/': 's3://nuscenes/nuscenes/',
+# 'data/nuscenes/': 's3://nuscenes/nuscenes/'
+# }))
+train_pipeline = [
+ dict(
+ type='LoadPointsFromFile',
+ coord_type='LIDAR',
+ load_dim=5,
+ use_dim=5,
+ file_client_args=file_client_args),
+ dict(
+ type='LoadPointsFromMultiSweeps',
+ sweeps_num=10,
+ file_client_args=file_client_args),
+ dict(type='LoadAnnotations3D', with_bbox_3d=True, with_label_3d=True),
+ dict(
+ type='GlobalRotScaleTrans',
+ rot_range=[-0.3925, 0.3925],
+ scale_ratio_range=[0.95, 1.05],
+ translation_std=[0, 0, 0]),
+ dict(type='RandomFlip3D', flip_ratio_bev_horizontal=0.5),
+ dict(type='PointsRangeFilter', point_cloud_range=point_cloud_range),
+ dict(type='ObjectRangeFilter', point_cloud_range=point_cloud_range),
+ dict(type='ObjectNameFilter', classes=class_names),
+ dict(type='PointShuffle'),
+ dict(type='DefaultFormatBundle3D', class_names=class_names),
+ dict(type='Collect3D', keys=['points', 'gt_bboxes_3d', 'gt_labels_3d'])
+]
+test_pipeline = [
+ dict(
+ type='LoadPointsFromFile',
+ coord_type='LIDAR',
+ load_dim=5,
+ use_dim=5,
+ file_client_args=file_client_args),
+ dict(
+ type='LoadPointsFromMultiSweeps',
+ sweeps_num=10,
+ file_client_args=file_client_args),
+ dict(
+ type='MultiScaleFlipAug3D',
+ img_scale=(1333, 800),
+ pts_scale_ratio=1,
+ flip=False,
+ transforms=[
+ dict(
+ type='GlobalRotScaleTrans',
+ rot_range=[0, 0],
+ scale_ratio_range=[1., 1.],
+ translation_std=[0, 0, 0]),
+ dict(type='RandomFlip3D'),
+ dict(
+ type='PointsRangeFilter', point_cloud_range=point_cloud_range),
+ dict(
+ type='DefaultFormatBundle3D',
+ class_names=class_names,
+ with_label=False),
+ dict(type='Collect3D', keys=['points'])
+ ])
+]
+# construct a pipeline for data and gt loading in show function
+# please keep its loading function consistent with test_pipeline (e.g. client)
+eval_pipeline = [
+ dict(
+ type='LoadPointsFromFile',
+ coord_type='LIDAR',
+ load_dim=5,
+ use_dim=5,
+ file_client_args=file_client_args),
+ dict(
+ type='LoadPointsFromMultiSweeps',
+ sweeps_num=10,
+ file_client_args=file_client_args),
+ dict(
+ type='DefaultFormatBundle3D',
+ class_names=class_names,
+ with_label=False),
+ dict(type='Collect3D', keys=['points'])
+]
+
+data = dict(
+ samples_per_gpu=4,
+ workers_per_gpu=4,
+ train=dict(
+ type=dataset_type,
+ data_root=data_root,
+ ann_file=data_root + 'nuscenes_infos_train.pkl',
+ pipeline=train_pipeline,
+ classes=class_names,
+ modality=input_modality,
+ test_mode=False,
+ # we use box_type_3d='LiDAR' in kitti and nuscenes dataset
+ # and box_type_3d='Depth' in sunrgbd and scannet dataset.
+ box_type_3d='LiDAR'),
+ val=dict(
+ type=dataset_type,
+ data_root=data_root,
+ ann_file=data_root + 'nuscenes_infos_val.pkl',
+ pipeline=test_pipeline,
+ classes=class_names,
+ modality=input_modality,
+ test_mode=True,
+ box_type_3d='LiDAR'),
+ test=dict(
+ type=dataset_type,
+ data_root=data_root,
+ ann_file=data_root + 'nuscenes_infos_val.pkl',
+ pipeline=test_pipeline,
+ classes=class_names,
+ modality=input_modality,
+ test_mode=True,
+ box_type_3d='LiDAR'))
+# For nuScenes dataset, we usually evaluate the model at the end of training.
+# Since the models are trained by 24 epochs by default, we set evaluation
+# interval to be 24. Please change the interval accordingly if you do not
+# use a default schedule.
+evaluation = dict(interval=24, pipeline=eval_pipeline)
diff --git a/configs/_base_/datasets/nus-mono3d.py b/configs/_base_/datasets/nus-mono3d.py
new file mode 100644
index 0000000..5decdac
--- /dev/null
+++ b/configs/_base_/datasets/nus-mono3d.py
@@ -0,0 +1,100 @@
+dataset_type = 'NuScenesMonoDataset'
+data_root = 'data/nuscenes/'
+class_names = [
+ 'car', 'truck', 'trailer', 'bus', 'construction_vehicle', 'bicycle',
+ 'motorcycle', 'pedestrian', 'traffic_cone', 'barrier'
+]
+# Input modality for nuScenes dataset, this is consistent with the submission
+# format which requires the information in input_modality.
+input_modality = dict(
+ use_lidar=False,
+ use_camera=True,
+ use_radar=False,
+ use_map=False,
+ use_external=False)
+img_norm_cfg = dict(
+ mean=[123.675, 116.28, 103.53], std=[58.395, 57.12, 57.375], to_rgb=True)
+train_pipeline = [
+ dict(type='LoadImageFromFileMono3D'),
+ dict(
+ type='LoadAnnotations3D',
+ with_bbox=True,
+ with_label=True,
+ with_attr_label=True,
+ with_bbox_3d=True,
+ with_label_3d=True,
+ with_bbox_depth=True),
+ dict(type='Resize', img_scale=(1600, 900), keep_ratio=True),
+ dict(type='RandomFlip3D', flip_ratio_bev_horizontal=0.5),
+ dict(type='Normalize', **img_norm_cfg),
+ dict(type='Pad', size_divisor=32),
+ dict(type='DefaultFormatBundle3D', class_names=class_names),
+ dict(
+ type='Collect3D',
+ keys=[
+ 'img', 'gt_bboxes', 'gt_labels', 'attr_labels', 'gt_bboxes_3d',
+ 'gt_labels_3d', 'centers2d', 'depths'
+ ]),
+]
+test_pipeline = [
+ dict(type='LoadImageFromFileMono3D'),
+ dict(
+ type='MultiScaleFlipAug',
+ scale_factor=1.0,
+ flip=False,
+ transforms=[
+ dict(type='RandomFlip3D'),
+ dict(type='Normalize', **img_norm_cfg),
+ dict(type='Pad', size_divisor=32),
+ dict(
+ type='DefaultFormatBundle3D',
+ class_names=class_names,
+ with_label=False),
+ dict(type='Collect3D', keys=['img']),
+ ])
+]
+# construct a pipeline for data and gt loading in show function
+# please keep its loading function consistent with test_pipeline (e.g. client)
+eval_pipeline = [
+ dict(type='LoadImageFromFileMono3D'),
+ dict(
+ type='DefaultFormatBundle3D',
+ class_names=class_names,
+ with_label=False),
+ dict(type='Collect3D', keys=['img'])
+]
+
+data = dict(
+ samples_per_gpu=2,
+ workers_per_gpu=2,
+ train=dict(
+ type=dataset_type,
+ data_root=data_root,
+ ann_file=data_root + 'nuscenes_infos_train_mono3d.coco.json',
+ img_prefix=data_root,
+ classes=class_names,
+ pipeline=train_pipeline,
+ modality=input_modality,
+ test_mode=False,
+ box_type_3d='Camera'),
+ val=dict(
+ type=dataset_type,
+ data_root=data_root,
+ ann_file=data_root + 'nuscenes_infos_val_mono3d.coco.json',
+ img_prefix=data_root,
+ classes=class_names,
+ pipeline=test_pipeline,
+ modality=input_modality,
+ test_mode=True,
+ box_type_3d='Camera'),
+ test=dict(
+ type=dataset_type,
+ data_root=data_root,
+ ann_file=data_root + 'nuscenes_infos_val_mono3d.coco.json',
+ img_prefix=data_root,
+ classes=class_names,
+ pipeline=test_pipeline,
+ modality=input_modality,
+ test_mode=True,
+ box_type_3d='Camera'))
+evaluation = dict(interval=2)
diff --git a/configs/_base_/datasets/range100_lyft-3d.py b/configs/_base_/datasets/range100_lyft-3d.py
new file mode 100644
index 0000000..efa63ea
--- /dev/null
+++ b/configs/_base_/datasets/range100_lyft-3d.py
@@ -0,0 +1,136 @@
+# If point cloud range is changed, the models should also change their point
+# cloud range accordingly
+point_cloud_range = [-100, -100, -5, 100, 100, 3]
+# For Lyft we usually do 9-class detection
+class_names = [
+ 'car', 'truck', 'bus', 'emergency_vehicle', 'other_vehicle', 'motorcycle',
+ 'bicycle', 'pedestrian', 'animal'
+]
+dataset_type = 'LyftDataset'
+data_root = 'data/lyft/'
+# Input modality for Lyft dataset, this is consistent with the submission
+# format which requires the information in input_modality.
+input_modality = dict(
+ use_lidar=True,
+ use_camera=False,
+ use_radar=False,
+ use_map=False,
+ use_external=False)
+file_client_args = dict(backend='disk')
+# Uncomment the following if use ceph or other file clients.
+# See https://mmcv.readthedocs.io/en/latest/api.html#mmcv.fileio.FileClient
+# for more details.
+# file_client_args = dict(
+# backend='petrel',
+# path_mapping=dict({
+# './data/lyft/': 's3://lyft/lyft/',
+# 'data/lyft/': 's3://lyft/lyft/'
+# }))
+train_pipeline = [
+ dict(
+ type='LoadPointsFromFile',
+ coord_type='LIDAR',
+ load_dim=5,
+ use_dim=5,
+ file_client_args=file_client_args),
+ dict(
+ type='LoadPointsFromMultiSweeps',
+ sweeps_num=10,
+ file_client_args=file_client_args),
+ dict(type='LoadAnnotations3D', with_bbox_3d=True, with_label_3d=True),
+ dict(
+ type='GlobalRotScaleTrans',
+ rot_range=[-0.3925, 0.3925],
+ scale_ratio_range=[0.95, 1.05],
+ translation_std=[0, 0, 0]),
+ dict(type='RandomFlip3D', flip_ratio_bev_horizontal=0.5),
+ dict(type='PointsRangeFilter', point_cloud_range=point_cloud_range),
+ dict(type='ObjectRangeFilter', point_cloud_range=point_cloud_range),
+ dict(type='PointShuffle'),
+ dict(type='DefaultFormatBundle3D', class_names=class_names),
+ dict(type='Collect3D', keys=['points', 'gt_bboxes_3d', 'gt_labels_3d'])
+]
+test_pipeline = [
+ dict(
+ type='LoadPointsFromFile',
+ coord_type='LIDAR',
+ load_dim=5,
+ use_dim=5,
+ file_client_args=file_client_args),
+ dict(
+ type='LoadPointsFromMultiSweeps',
+ sweeps_num=10,
+ file_client_args=file_client_args),
+ dict(
+ type='MultiScaleFlipAug3D',
+ img_scale=(1333, 800),
+ pts_scale_ratio=1,
+ flip=False,
+ transforms=[
+ dict(
+ type='GlobalRotScaleTrans',
+ rot_range=[0, 0],
+ scale_ratio_range=[1., 1.],
+ translation_std=[0, 0, 0]),
+ dict(type='RandomFlip3D'),
+ dict(
+ type='PointsRangeFilter', point_cloud_range=point_cloud_range),
+ dict(
+ type='DefaultFormatBundle3D',
+ class_names=class_names,
+ with_label=False),
+ dict(type='Collect3D', keys=['points'])
+ ])
+]
+# construct a pipeline for data and gt loading in show function
+# please keep its loading function consistent with test_pipeline (e.g. client)
+eval_pipeline = [
+ dict(
+ type='LoadPointsFromFile',
+ coord_type='LIDAR',
+ load_dim=5,
+ use_dim=5,
+ file_client_args=file_client_args),
+ dict(
+ type='LoadPointsFromMultiSweeps',
+ sweeps_num=10,
+ file_client_args=file_client_args),
+ dict(
+ type='DefaultFormatBundle3D',
+ class_names=class_names,
+ with_label=False),
+ dict(type='Collect3D', keys=['points'])
+]
+
+data = dict(
+ samples_per_gpu=2,
+ workers_per_gpu=2,
+ train=dict(
+ type=dataset_type,
+ data_root=data_root,
+ ann_file=data_root + 'lyft_infos_train.pkl',
+ pipeline=train_pipeline,
+ classes=class_names,
+ modality=input_modality,
+ test_mode=False),
+ val=dict(
+ type=dataset_type,
+ data_root=data_root,
+ ann_file=data_root + 'lyft_infos_val.pkl',
+ pipeline=test_pipeline,
+ classes=class_names,
+ modality=input_modality,
+ test_mode=True),
+ test=dict(
+ type=dataset_type,
+ data_root=data_root,
+ ann_file=data_root + 'lyft_infos_test.pkl',
+ pipeline=test_pipeline,
+ classes=class_names,
+ modality=input_modality,
+ test_mode=True))
+# For Lyft dataset, we usually evaluate the model at the end of training.
+# Since the models are trained by 24 epochs by default, we set evaluation
+# interval to be 24. Please change the interval accordingly if you do not
+# use a default schedule.
+evaluation = dict(interval=24, pipeline=eval_pipeline)
diff --git a/configs/_base_/datasets/s3dis-3d-5class.py b/configs/_base_/datasets/s3dis-3d-5class.py
new file mode 100644
index 0000000..2422766
--- /dev/null
+++ b/configs/_base_/datasets/s3dis-3d-5class.py
@@ -0,0 +1,114 @@
+# dataset settings
+dataset_type = 'S3DISDataset'
+data_root = './data/s3dis/'
+class_names = ('table', 'chair', 'sofa', 'bookcase', 'board')
+train_area = [1, 2, 3, 4, 6]
+test_area = 5
+
+train_pipeline = [
+ dict(
+ type='LoadPointsFromFile',
+ coord_type='DEPTH',
+ shift_height=True,
+ load_dim=6,
+ use_dim=[0, 1, 2, 3, 4, 5]),
+ dict(type='LoadAnnotations3D', with_bbox_3d=True, with_label_3d=True),
+ dict(type='PointSample', num_points=40000),
+ dict(
+ type='RandomFlip3D',
+ sync_2d=False,
+ flip_ratio_bev_horizontal=0.5,
+ flip_ratio_bev_vertical=0.5),
+ dict(
+ type='GlobalRotScaleTrans',
+ # following ScanNet dataset the rotation range is 5 degrees
+ rot_range=[-0.087266, 0.087266],
+ scale_ratio_range=[1.0, 1.0],
+ shift_height=True),
+ dict(type='DefaultFormatBundle3D', class_names=class_names),
+ dict(type='Collect3D', keys=['points', 'gt_bboxes_3d', 'gt_labels_3d'])
+]
+test_pipeline = [
+ dict(
+ type='LoadPointsFromFile',
+ coord_type='DEPTH',
+ shift_height=True,
+ load_dim=6,
+ use_dim=[0, 1, 2, 3, 4, 5]),
+ dict(
+ type='MultiScaleFlipAug3D',
+ img_scale=(1333, 800),
+ pts_scale_ratio=1,
+ flip=False,
+ transforms=[
+ dict(
+ type='GlobalRotScaleTrans',
+ rot_range=[0, 0],
+ scale_ratio_range=[1., 1.],
+ translation_std=[0, 0, 0]),
+ dict(
+ type='RandomFlip3D',
+ sync_2d=False,
+ flip_ratio_bev_horizontal=0.5,
+ flip_ratio_bev_vertical=0.5),
+ dict(type='PointSample', num_points=40000),
+ dict(
+ type='DefaultFormatBundle3D',
+ class_names=class_names,
+ with_label=False),
+ dict(type='Collect3D', keys=['points'])
+ ])
+]
+# construct a pipeline for data and gt loading in show function
+# please keep its loading function consistent with test_pipeline (e.g. client)
+eval_pipeline = [
+ dict(
+ type='LoadPointsFromFile',
+ coord_type='DEPTH',
+ shift_height=False,
+ load_dim=6,
+ use_dim=[0, 1, 2, 3, 4, 5]),
+ dict(
+ type='DefaultFormatBundle3D',
+ class_names=class_names,
+ with_label=False),
+ dict(type='Collect3D', keys=['points'])
+]
+
+data = dict(
+ samples_per_gpu=8,
+ workers_per_gpu=4,
+ train=dict(
+ type='RepeatDataset',
+ times=5,
+ dataset=dict(
+ type='ConcatDataset',
+ datasets=[
+ dict(
+ type=dataset_type,
+ data_root=data_root,
+ ann_file=data_root + f's3dis_infos_Area_{i}.pkl',
+ pipeline=train_pipeline,
+ filter_empty_gt=False,
+ classes=class_names,
+ box_type_3d='Depth') for i in train_area
+ ],
+ separate_eval=False)),
+ val=dict(
+ type=dataset_type,
+ data_root=data_root,
+ ann_file=data_root + f's3dis_infos_Area_{test_area}.pkl',
+ pipeline=test_pipeline,
+ classes=class_names,
+ test_mode=True,
+ box_type_3d='Depth'),
+ test=dict(
+ type=dataset_type,
+ data_root=data_root,
+ ann_file=data_root + f's3dis_infos_Area_{test_area}.pkl',
+ pipeline=test_pipeline,
+ classes=class_names,
+ test_mode=True,
+ box_type_3d='Depth'))
+
+evaluation = dict(pipeline=eval_pipeline)
diff --git a/configs/_base_/datasets/s3dis_seg-3d-13class.py b/configs/_base_/datasets/s3dis_seg-3d-13class.py
new file mode 100644
index 0000000..4833154
--- /dev/null
+++ b/configs/_base_/datasets/s3dis_seg-3d-13class.py
@@ -0,0 +1,159 @@
+# dataset settings
+dataset_type = 'S3DISSegDataset'
+data_root = './data/s3dis/'
+class_names = ('ceiling', 'floor', 'wall', 'beam', 'column', 'window', 'door',
+ 'table', 'chair', 'sofa', 'bookcase', 'board', 'clutter')
+file_client_args = dict(backend='disk')
+# Uncomment the following if use ceph or other file clients.
+# See https://mmcv.readthedocs.io/en/latest/api.html#mmcv.fileio.FileClient
+# for more details.
+# file_client_args = dict(
+# backend='petrel',
+# path_mapping=dict({
+# './data/s3dis/':
+# 's3://openmmlab/datasets/detection3d/s3dis_processed/',
+# 'data/s3dis/':
+# 's3://openmmlab/datasets/detection3d/s3dis_processed/'
+# }))
+num_points = 4096
+train_area = [1, 2, 3, 4, 6]
+test_area = 5
+train_pipeline = [
+ dict(
+ type='LoadPointsFromFile',
+ file_client_args=file_client_args,
+ coord_type='DEPTH',
+ shift_height=False,
+ use_color=True,
+ load_dim=6,
+ use_dim=[0, 1, 2, 3, 4, 5]),
+ dict(
+ type='LoadAnnotations3D',
+ file_client_args=file_client_args,
+ with_bbox_3d=False,
+ with_label_3d=False,
+ with_mask_3d=False,
+ with_seg_3d=True),
+ dict(
+ type='PointSegClassMapping',
+ valid_cat_ids=tuple(range(len(class_names))),
+ max_cat_id=13),
+ dict(
+ type='IndoorPatchPointSample',
+ num_points=num_points,
+ block_size=1.0,
+ ignore_index=len(class_names),
+ use_normalized_coord=True,
+ enlarge_size=0.2,
+ min_unique_num=None),
+ dict(type='NormalizePointsColor', color_mean=None),
+ dict(type='DefaultFormatBundle3D', class_names=class_names),
+ dict(type='Collect3D', keys=['points', 'pts_semantic_mask'])
+]
+test_pipeline = [
+ dict(
+ type='LoadPointsFromFile',
+ file_client_args=file_client_args,
+ coord_type='DEPTH',
+ shift_height=False,
+ use_color=True,
+ load_dim=6,
+ use_dim=[0, 1, 2, 3, 4, 5]),
+ dict(type='NormalizePointsColor', color_mean=None),
+ dict(
+ # a wrapper in order to successfully call test function
+ # actually we don't perform test-time-aug
+ type='MultiScaleFlipAug3D',
+ img_scale=(1333, 800),
+ pts_scale_ratio=1,
+ flip=False,
+ transforms=[
+ dict(
+ type='GlobalRotScaleTrans',
+ rot_range=[0, 0],
+ scale_ratio_range=[1., 1.],
+ translation_std=[0, 0, 0]),
+ dict(
+ type='RandomFlip3D',
+ sync_2d=False,
+ flip_ratio_bev_horizontal=0.0,
+ flip_ratio_bev_vertical=0.0),
+ dict(
+ type='DefaultFormatBundle3D',
+ class_names=class_names,
+ with_label=False),
+ dict(type='Collect3D', keys=['points'])
+ ])
+]
+# construct a pipeline for data and gt loading in show function
+# please keep its loading function consistent with test_pipeline (e.g. client)
+# we need to load gt seg_mask!
+eval_pipeline = [
+ dict(
+ type='LoadPointsFromFile',
+ file_client_args=file_client_args,
+ coord_type='DEPTH',
+ shift_height=False,
+ use_color=True,
+ load_dim=6,
+ use_dim=[0, 1, 2, 3, 4, 5]),
+ dict(
+ type='LoadAnnotations3D',
+ file_client_args=file_client_args,
+ with_bbox_3d=False,
+ with_label_3d=False,
+ with_mask_3d=False,
+ with_seg_3d=True),
+ dict(
+ type='PointSegClassMapping',
+ valid_cat_ids=tuple(range(len(class_names))),
+ max_cat_id=13),
+ dict(
+ type='DefaultFormatBundle3D',
+ with_label=False,
+ class_names=class_names),
+ dict(type='Collect3D', keys=['points', 'pts_semantic_mask'])
+]
+
+data = dict(
+ samples_per_gpu=8,
+ workers_per_gpu=4,
+ # train on area 1, 2, 3, 4, 6
+ # test on area 5
+ train=dict(
+ type=dataset_type,
+ data_root=data_root,
+ ann_files=[
+ data_root + f's3dis_infos_Area_{i}.pkl' for i in train_area
+ ],
+ pipeline=train_pipeline,
+ classes=class_names,
+ test_mode=False,
+ ignore_index=len(class_names),
+ scene_idxs=[
+ data_root + f'seg_info/Area_{i}_resampled_scene_idxs.npy'
+ for i in train_area
+ ],
+ file_client_args=file_client_args),
+ val=dict(
+ type=dataset_type,
+ data_root=data_root,
+ ann_files=data_root + f's3dis_infos_Area_{test_area}.pkl',
+ pipeline=test_pipeline,
+ classes=class_names,
+ test_mode=True,
+ ignore_index=len(class_names),
+ scene_idxs=data_root +
+ f'seg_info/Area_{test_area}_resampled_scene_idxs.npy',
+ file_client_args=file_client_args),
+ test=dict(
+ type=dataset_type,
+ data_root=data_root,
+ ann_files=data_root + f's3dis_infos_Area_{test_area}.pkl',
+ pipeline=test_pipeline,
+ classes=class_names,
+ test_mode=True,
+ ignore_index=len(class_names),
+ file_client_args=file_client_args))
+
+evaluation = dict(pipeline=eval_pipeline)
diff --git a/configs/_base_/datasets/scannet-3d-18class.py b/configs/_base_/datasets/scannet-3d-18class.py
new file mode 100644
index 0000000..93da1e5
--- /dev/null
+++ b/configs/_base_/datasets/scannet-3d-18class.py
@@ -0,0 +1,128 @@
+# dataset settings
+dataset_type = 'ScanNetDataset'
+data_root = './data/scannet/'
+class_names = ('cabinet', 'bed', 'chair', 'sofa', 'table', 'door', 'window',
+ 'bookshelf', 'picture', 'counter', 'desk', 'curtain',
+ 'refrigerator', 'showercurtrain', 'toilet', 'sink', 'bathtub',
+ 'garbagebin')
+train_pipeline = [
+ dict(
+ type='LoadPointsFromFile',
+ coord_type='DEPTH',
+ shift_height=True,
+ load_dim=6,
+ use_dim=[0, 1, 2]),
+ dict(
+ type='LoadAnnotations3D',
+ with_bbox_3d=True,
+ with_label_3d=True,
+ with_mask_3d=True,
+ with_seg_3d=True),
+ dict(type='GlobalAlignment', rotation_axis=2),
+ dict(
+ type='PointSegClassMapping',
+ valid_cat_ids=(3, 4, 5, 6, 7, 8, 9, 10, 11, 12, 14, 16, 24, 28, 33, 34,
+ 36, 39),
+ max_cat_id=40),
+ dict(type='PointSample', num_points=40000),
+ dict(
+ type='RandomFlip3D',
+ sync_2d=False,
+ flip_ratio_bev_horizontal=0.5,
+ flip_ratio_bev_vertical=0.5),
+ dict(
+ type='GlobalRotScaleTrans',
+ rot_range=[-0.087266, 0.087266],
+ scale_ratio_range=[1.0, 1.0],
+ shift_height=True),
+ dict(type='DefaultFormatBundle3D', class_names=class_names),
+ dict(
+ type='Collect3D',
+ keys=[
+ 'points', 'gt_bboxes_3d', 'gt_labels_3d', 'pts_semantic_mask',
+ 'pts_instance_mask'
+ ])
+]
+test_pipeline = [
+ dict(
+ type='LoadPointsFromFile',
+ coord_type='DEPTH',
+ shift_height=True,
+ load_dim=6,
+ use_dim=[0, 1, 2]),
+ dict(type='GlobalAlignment', rotation_axis=2),
+ dict(
+ type='MultiScaleFlipAug3D',
+ img_scale=(1333, 800),
+ pts_scale_ratio=1,
+ flip=False,
+ transforms=[
+ dict(
+ type='GlobalRotScaleTrans',
+ rot_range=[0, 0],
+ scale_ratio_range=[1., 1.],
+ translation_std=[0, 0, 0]),
+ dict(
+ type='RandomFlip3D',
+ sync_2d=False,
+ flip_ratio_bev_horizontal=0.5,
+ flip_ratio_bev_vertical=0.5),
+ dict(type='PointSample', num_points=40000),
+ dict(
+ type='DefaultFormatBundle3D',
+ class_names=class_names,
+ with_label=False),
+ dict(type='Collect3D', keys=['points'])
+ ])
+]
+# construct a pipeline for data and gt loading in show function
+# please keep its loading function consistent with test_pipeline (e.g. client)
+eval_pipeline = [
+ dict(
+ type='LoadPointsFromFile',
+ coord_type='DEPTH',
+ shift_height=False,
+ load_dim=6,
+ use_dim=[0, 1, 2]),
+ dict(type='GlobalAlignment', rotation_axis=2),
+ dict(
+ type='DefaultFormatBundle3D',
+ class_names=class_names,
+ with_label=False),
+ dict(type='Collect3D', keys=['points'])
+]
+
+data = dict(
+ samples_per_gpu=8,
+ workers_per_gpu=4,
+ train=dict(
+ type='RepeatDataset',
+ times=5,
+ dataset=dict(
+ type=dataset_type,
+ data_root=data_root,
+ ann_file=data_root + 'scannet_infos_train.pkl',
+ pipeline=train_pipeline,
+ filter_empty_gt=False,
+ classes=class_names,
+ # we use box_type_3d='LiDAR' in kitti and nuscenes dataset
+ # and box_type_3d='Depth' in sunrgbd and scannet dataset.
+ box_type_3d='Depth')),
+ val=dict(
+ type=dataset_type,
+ data_root=data_root,
+ ann_file=data_root + 'scannet_infos_val.pkl',
+ pipeline=test_pipeline,
+ classes=class_names,
+ test_mode=True,
+ box_type_3d='Depth'),
+ test=dict(
+ type=dataset_type,
+ data_root=data_root,
+ ann_file=data_root + 'scannet_infos_val.pkl',
+ pipeline=test_pipeline,
+ classes=class_names,
+ test_mode=True,
+ box_type_3d='Depth'))
+
+evaluation = dict(pipeline=eval_pipeline)
diff --git a/configs/_base_/datasets/scannet_seg-3d-20class.py b/configs/_base_/datasets/scannet_seg-3d-20class.py
new file mode 100644
index 0000000..cf73b09
--- /dev/null
+++ b/configs/_base_/datasets/scannet_seg-3d-20class.py
@@ -0,0 +1,132 @@
+# dataset settings
+dataset_type = 'ScanNetSegDataset'
+data_root = './data/scannet/'
+class_names = ('wall', 'floor', 'cabinet', 'bed', 'chair', 'sofa', 'table',
+ 'door', 'window', 'bookshelf', 'picture', 'counter', 'desk',
+ 'curtain', 'refrigerator', 'showercurtrain', 'toilet', 'sink',
+ 'bathtub', 'otherfurniture')
+num_points = 8192
+train_pipeline = [
+ dict(
+ type='LoadPointsFromFile',
+ coord_type='DEPTH',
+ shift_height=False,
+ use_color=True,
+ load_dim=6,
+ use_dim=[0, 1, 2, 3, 4, 5]),
+ dict(
+ type='LoadAnnotations3D',
+ with_bbox_3d=False,
+ with_label_3d=False,
+ with_mask_3d=False,
+ with_seg_3d=True),
+ dict(
+ type='PointSegClassMapping',
+ valid_cat_ids=(1, 2, 3, 4, 5, 6, 7, 8, 9, 10, 11, 12, 14, 16, 24, 28,
+ 33, 34, 36, 39),
+ max_cat_id=40),
+ dict(
+ type='IndoorPatchPointSample',
+ num_points=num_points,
+ block_size=1.5,
+ ignore_index=len(class_names),
+ use_normalized_coord=False,
+ enlarge_size=0.2,
+ min_unique_num=None),
+ dict(type='NormalizePointsColor', color_mean=None),
+ dict(type='DefaultFormatBundle3D', class_names=class_names),
+ dict(type='Collect3D', keys=['points', 'pts_semantic_mask'])
+]
+test_pipeline = [
+ dict(
+ type='LoadPointsFromFile',
+ coord_type='DEPTH',
+ shift_height=False,
+ use_color=True,
+ load_dim=6,
+ use_dim=[0, 1, 2, 3, 4, 5]),
+ dict(type='NormalizePointsColor', color_mean=None),
+ dict(
+ # a wrapper in order to successfully call test function
+ # actually we don't perform test-time-aug
+ type='MultiScaleFlipAug3D',
+ img_scale=(1333, 800),
+ pts_scale_ratio=1,
+ flip=False,
+ transforms=[
+ dict(
+ type='GlobalRotScaleTrans',
+ rot_range=[0, 0],
+ scale_ratio_range=[1., 1.],
+ translation_std=[0, 0, 0]),
+ dict(
+ type='RandomFlip3D',
+ sync_2d=False,
+ flip_ratio_bev_horizontal=0.0,
+ flip_ratio_bev_vertical=0.0),
+ dict(
+ type='DefaultFormatBundle3D',
+ class_names=class_names,
+ with_label=False),
+ dict(type='Collect3D', keys=['points'])
+ ])
+]
+# construct a pipeline for data and gt loading in show function
+# please keep its loading function consistent with test_pipeline (e.g. client)
+# we need to load gt seg_mask!
+eval_pipeline = [
+ dict(
+ type='LoadPointsFromFile',
+ coord_type='DEPTH',
+ shift_height=False,
+ use_color=True,
+ load_dim=6,
+ use_dim=[0, 1, 2, 3, 4, 5]),
+ dict(
+ type='LoadAnnotations3D',
+ with_bbox_3d=False,
+ with_label_3d=False,
+ with_mask_3d=False,
+ with_seg_3d=True),
+ dict(
+ type='PointSegClassMapping',
+ valid_cat_ids=(1, 2, 3, 4, 5, 6, 7, 8, 9, 10, 11, 12, 14, 16, 24, 28,
+ 33, 34, 36, 39),
+ max_cat_id=40),
+ dict(
+ type='DefaultFormatBundle3D',
+ with_label=False,
+ class_names=class_names),
+ dict(type='Collect3D', keys=['points', 'pts_semantic_mask'])
+]
+
+data = dict(
+ samples_per_gpu=8,
+ workers_per_gpu=4,
+ train=dict(
+ type=dataset_type,
+ data_root=data_root,
+ ann_file=data_root + 'scannet_infos_train.pkl',
+ pipeline=train_pipeline,
+ classes=class_names,
+ test_mode=False,
+ ignore_index=len(class_names),
+ scene_idxs=data_root + 'seg_info/train_resampled_scene_idxs.npy'),
+ val=dict(
+ type=dataset_type,
+ data_root=data_root,
+ ann_file=data_root + 'scannet_infos_val.pkl',
+ pipeline=test_pipeline,
+ classes=class_names,
+ test_mode=True,
+ ignore_index=len(class_names)),
+ test=dict(
+ type=dataset_type,
+ data_root=data_root,
+ ann_file=data_root + 'scannet_infos_val.pkl',
+ pipeline=test_pipeline,
+ classes=class_names,
+ test_mode=True,
+ ignore_index=len(class_names)))
+
+evaluation = dict(pipeline=eval_pipeline)
diff --git a/configs/_base_/datasets/sunrgbd-3d-10class.py b/configs/_base_/datasets/sunrgbd-3d-10class.py
new file mode 100644
index 0000000..7121b75
--- /dev/null
+++ b/configs/_base_/datasets/sunrgbd-3d-10class.py
@@ -0,0 +1,107 @@
+dataset_type = 'SUNRGBDDataset'
+data_root = 'data/sunrgbd/'
+class_names = ('bed', 'table', 'sofa', 'chair', 'toilet', 'desk', 'dresser',
+ 'night_stand', 'bookshelf', 'bathtub')
+train_pipeline = [
+ dict(
+ type='LoadPointsFromFile',
+ coord_type='DEPTH',
+ shift_height=True,
+ load_dim=6,
+ use_dim=[0, 1, 2]),
+ dict(type='LoadAnnotations3D'),
+ dict(
+ type='RandomFlip3D',
+ sync_2d=False,
+ flip_ratio_bev_horizontal=0.5,
+ ),
+ dict(
+ type='GlobalRotScaleTrans',
+ rot_range=[-0.523599, 0.523599],
+ scale_ratio_range=[0.85, 1.15],
+ shift_height=True),
+ dict(type='PointSample', num_points=20000),
+ dict(type='DefaultFormatBundle3D', class_names=class_names),
+ dict(type='Collect3D', keys=['points', 'gt_bboxes_3d', 'gt_labels_3d'])
+]
+test_pipeline = [
+ dict(
+ type='LoadPointsFromFile',
+ coord_type='DEPTH',
+ shift_height=True,
+ load_dim=6,
+ use_dim=[0, 1, 2]),
+ dict(
+ type='MultiScaleFlipAug3D',
+ img_scale=(1333, 800),
+ pts_scale_ratio=1,
+ flip=False,
+ transforms=[
+ dict(
+ type='GlobalRotScaleTrans',
+ rot_range=[0, 0],
+ scale_ratio_range=[1., 1.],
+ translation_std=[0, 0, 0]),
+ dict(
+ type='RandomFlip3D',
+ sync_2d=False,
+ flip_ratio_bev_horizontal=0.5,
+ ),
+ dict(type='PointSample', num_points=20000),
+ dict(
+ type='DefaultFormatBundle3D',
+ class_names=class_names,
+ with_label=False),
+ dict(type='Collect3D', keys=['points'])
+ ])
+]
+# construct a pipeline for data and gt loading in show function
+# please keep its loading function consistent with test_pipeline (e.g. client)
+eval_pipeline = [
+ dict(
+ type='LoadPointsFromFile',
+ coord_type='DEPTH',
+ shift_height=False,
+ load_dim=6,
+ use_dim=[0, 1, 2]),
+ dict(
+ type='DefaultFormatBundle3D',
+ class_names=class_names,
+ with_label=False),
+ dict(type='Collect3D', keys=['points'])
+]
+
+data = dict(
+ samples_per_gpu=16,
+ workers_per_gpu=4,
+ train=dict(
+ type='RepeatDataset',
+ times=5,
+ dataset=dict(
+ type=dataset_type,
+ data_root=data_root,
+ ann_file=data_root + 'sunrgbd_infos_train.pkl',
+ pipeline=train_pipeline,
+ classes=class_names,
+ filter_empty_gt=False,
+ # we use box_type_3d='LiDAR' in kitti and nuscenes dataset
+ # and box_type_3d='Depth' in sunrgbd and scannet dataset.
+ box_type_3d='Depth')),
+ val=dict(
+ type=dataset_type,
+ data_root=data_root,
+ ann_file=data_root + 'sunrgbd_infos_val.pkl',
+ pipeline=test_pipeline,
+ classes=class_names,
+ test_mode=True,
+ box_type_3d='Depth'),
+ test=dict(
+ type=dataset_type,
+ data_root=data_root,
+ ann_file=data_root + 'sunrgbd_infos_val.pkl',
+ pipeline=test_pipeline,
+ classes=class_names,
+ test_mode=True,
+ box_type_3d='Depth'))
+
+evaluation = dict(pipeline=eval_pipeline)
diff --git a/configs/_base_/datasets/waymoD5-3d-3class.py b/configs/_base_/datasets/waymoD5-3d-3class.py
new file mode 100644
index 0000000..e3937fb
--- /dev/null
+++ b/configs/_base_/datasets/waymoD5-3d-3class.py
@@ -0,0 +1,145 @@
+# dataset settings
+# D5 in the config name means the whole dataset is divided into 5 folds
+# We only use one fold for efficient experiments
+dataset_type = 'WaymoDataset'
+data_root = 'data/waymo/kitti_format/'
+file_client_args = dict(backend='disk')
+# Uncomment the following if use ceph or other file clients.
+# See https://mmcv.readthedocs.io/en/latest/api.html#mmcv.fileio.FileClient
+# for more details.
+# file_client_args = dict(
+# backend='petrel', path_mapping=dict(data='s3://waymo_data/'))
+
+class_names = ['Car', 'Pedestrian', 'Cyclist']
+point_cloud_range = [-74.88, -74.88, -2, 74.88, 74.88, 4]
+input_modality = dict(use_lidar=True, use_camera=False)
+db_sampler = dict(
+ data_root=data_root,
+ info_path=data_root + 'waymo_dbinfos_train.pkl',
+ rate=1.0,
+ prepare=dict(
+ filter_by_difficulty=[-1],
+ filter_by_min_points=dict(Car=5, Pedestrian=10, Cyclist=10)),
+ classes=class_names,
+ sample_groups=dict(Car=15, Pedestrian=10, Cyclist=10),
+ points_loader=dict(
+ type='LoadPointsFromFile',
+ coord_type='LIDAR',
+ load_dim=6,
+ use_dim=[0, 1, 2, 3, 4],
+ file_client_args=file_client_args))
+
+train_pipeline = [
+ dict(
+ type='LoadPointsFromFile',
+ coord_type='LIDAR',
+ load_dim=6,
+ use_dim=5,
+ file_client_args=file_client_args),
+ dict(
+ type='LoadAnnotations3D',
+ with_bbox_3d=True,
+ with_label_3d=True,
+ file_client_args=file_client_args),
+ dict(type='ObjectSample', db_sampler=db_sampler),
+ dict(
+ type='RandomFlip3D',
+ sync_2d=False,
+ flip_ratio_bev_horizontal=0.5,
+ flip_ratio_bev_vertical=0.5),
+ dict(
+ type='GlobalRotScaleTrans',
+ rot_range=[-0.78539816, 0.78539816],
+ scale_ratio_range=[0.95, 1.05]),
+ dict(type='PointsRangeFilter', point_cloud_range=point_cloud_range),
+ dict(type='ObjectRangeFilter', point_cloud_range=point_cloud_range),
+ dict(type='PointShuffle'),
+ dict(type='DefaultFormatBundle3D', class_names=class_names),
+ dict(type='Collect3D', keys=['points', 'gt_bboxes_3d', 'gt_labels_3d'])
+]
+test_pipeline = [
+ dict(
+ type='LoadPointsFromFile',
+ coord_type='LIDAR',
+ load_dim=6,
+ use_dim=5,
+ file_client_args=file_client_args),
+ dict(
+ type='MultiScaleFlipAug3D',
+ img_scale=(1333, 800),
+ pts_scale_ratio=1,
+ flip=False,
+ transforms=[
+ dict(
+ type='GlobalRotScaleTrans',
+ rot_range=[0, 0],
+ scale_ratio_range=[1., 1.],
+ translation_std=[0, 0, 0]),
+ dict(type='RandomFlip3D'),
+ dict(
+ type='PointsRangeFilter', point_cloud_range=point_cloud_range),
+ dict(
+ type='DefaultFormatBundle3D',
+ class_names=class_names,
+ with_label=False),
+ dict(type='Collect3D', keys=['points'])
+ ])
+]
+# construct a pipeline for data and gt loading in show function
+# please keep its loading function consistent with test_pipeline (e.g. client)
+eval_pipeline = [
+ dict(
+ type='LoadPointsFromFile',
+ coord_type='LIDAR',
+ load_dim=6,
+ use_dim=5,
+ file_client_args=file_client_args),
+ dict(
+ type='DefaultFormatBundle3D',
+ class_names=class_names,
+ with_label=False),
+ dict(type='Collect3D', keys=['points'])
+]
+
+data = dict(
+ samples_per_gpu=2,
+ workers_per_gpu=4,
+ train=dict(
+ type='RepeatDataset',
+ times=2,
+ dataset=dict(
+ type=dataset_type,
+ data_root=data_root,
+ ann_file=data_root + 'waymo_infos_train.pkl',
+ split='training',
+ pipeline=train_pipeline,
+ modality=input_modality,
+ classes=class_names,
+ test_mode=False,
+ # we use box_type_3d='LiDAR' in kitti and nuscenes dataset
+ # and box_type_3d='Depth' in sunrgbd and scannet dataset.
+ box_type_3d='LiDAR',
+ # load one frame every five frames
+ load_interval=5)),
+ val=dict(
+ type=dataset_type,
+ data_root=data_root,
+ ann_file=data_root + 'waymo_infos_val.pkl',
+ split='training',
+ pipeline=test_pipeline,
+ modality=input_modality,
+ classes=class_names,
+ test_mode=True,
+ box_type_3d='LiDAR'),
+ test=dict(
+ type=dataset_type,
+ data_root=data_root,
+ ann_file=data_root + 'waymo_infos_val.pkl',
+ split='training',
+ pipeline=test_pipeline,
+ modality=input_modality,
+ classes=class_names,
+ test_mode=True,
+ box_type_3d='LiDAR'))
+
+evaluation = dict(interval=24, pipeline=eval_pipeline)
diff --git a/configs/_base_/datasets/waymoD5-3d-car.py b/configs/_base_/datasets/waymoD5-3d-car.py
new file mode 100644
index 0000000..e119e5a
--- /dev/null
+++ b/configs/_base_/datasets/waymoD5-3d-car.py
@@ -0,0 +1,143 @@
+# dataset settings
+# D5 in the config name means the whole dataset is divided into 5 folds
+# We only use one fold for efficient experiments
+dataset_type = 'WaymoDataset'
+data_root = 'data/waymo/kitti_format/'
+file_client_args = dict(backend='disk')
+# Uncomment the following if use ceph or other file clients.
+# See https://mmcv.readthedocs.io/en/latest/api.html#mmcv.fileio.FileClient
+# for more details.
+# file_client_args = dict(
+# backend='petrel', path_mapping=dict(data='s3://waymo_data/'))
+
+class_names = ['Car']
+point_cloud_range = [-74.88, -74.88, -2, 74.88, 74.88, 4]
+input_modality = dict(use_lidar=True, use_camera=False)
+db_sampler = dict(
+ data_root=data_root,
+ info_path=data_root + 'waymo_dbinfos_train.pkl',
+ rate=1.0,
+ prepare=dict(filter_by_difficulty=[-1], filter_by_min_points=dict(Car=5)),
+ classes=class_names,
+ sample_groups=dict(Car=15),
+ points_loader=dict(
+ type='LoadPointsFromFile',
+ coord_type='LIDAR',
+ load_dim=6,
+ use_dim=[0, 1, 2, 3, 4],
+ file_client_args=file_client_args))
+
+train_pipeline = [
+ dict(
+ type='LoadPointsFromFile',
+ coord_type='LIDAR',
+ load_dim=6,
+ use_dim=5,
+ file_client_args=file_client_args),
+ dict(
+ type='LoadAnnotations3D',
+ with_bbox_3d=True,
+ with_label_3d=True,
+ file_client_args=file_client_args),
+ dict(type='ObjectSample', db_sampler=db_sampler),
+ dict(
+ type='RandomFlip3D',
+ sync_2d=False,
+ flip_ratio_bev_horizontal=0.5,
+ flip_ratio_bev_vertical=0.5),
+ dict(
+ type='GlobalRotScaleTrans',
+ rot_range=[-0.78539816, 0.78539816],
+ scale_ratio_range=[0.95, 1.05]),
+ dict(type='PointsRangeFilter', point_cloud_range=point_cloud_range),
+ dict(type='ObjectRangeFilter', point_cloud_range=point_cloud_range),
+ dict(type='PointShuffle'),
+ dict(type='DefaultFormatBundle3D', class_names=class_names),
+ dict(type='Collect3D', keys=['points', 'gt_bboxes_3d', 'gt_labels_3d'])
+]
+test_pipeline = [
+ dict(
+ type='LoadPointsFromFile',
+ coord_type='LIDAR',
+ load_dim=6,
+ use_dim=5,
+ file_client_args=file_client_args),
+ dict(
+ type='MultiScaleFlipAug3D',
+ img_scale=(1333, 800),
+ pts_scale_ratio=1,
+ flip=False,
+ transforms=[
+ dict(
+ type='GlobalRotScaleTrans',
+ rot_range=[0, 0],
+ scale_ratio_range=[1., 1.],
+ translation_std=[0, 0, 0]),
+ dict(type='RandomFlip3D'),
+ dict(
+ type='PointsRangeFilter', point_cloud_range=point_cloud_range),
+ dict(
+ type='DefaultFormatBundle3D',
+ class_names=class_names,
+ with_label=False),
+ dict(type='Collect3D', keys=['points'])
+ ])
+]
+# construct a pipeline for data and gt loading in show function
+# please keep its loading function consistent with test_pipeline (e.g. client)
+eval_pipeline = [
+ dict(
+ type='LoadPointsFromFile',
+ coord_type='LIDAR',
+ load_dim=6,
+ use_dim=5,
+ file_client_args=file_client_args),
+ dict(
+ type='DefaultFormatBundle3D',
+ class_names=class_names,
+ with_label=False),
+ dict(type='Collect3D', keys=['points'])
+]
+
+data = dict(
+ samples_per_gpu=2,
+ workers_per_gpu=4,
+ train=dict(
+ type='RepeatDataset',
+ times=2,
+ dataset=dict(
+ type=dataset_type,
+ data_root=data_root,
+ ann_file=data_root + 'waymo_infos_train.pkl',
+ split='training',
+ pipeline=train_pipeline,
+ modality=input_modality,
+ classes=class_names,
+ test_mode=False,
+ # we use box_type_3d='LiDAR' in kitti and nuscenes dataset
+ # and box_type_3d='Depth' in sunrgbd and scannet dataset.
+ box_type_3d='LiDAR',
+ # load one frame every five frames
+ load_interval=5)),
+ val=dict(
+ type=dataset_type,
+ data_root=data_root,
+ ann_file=data_root + 'waymo_infos_val.pkl',
+ split='training',
+ pipeline=test_pipeline,
+ modality=input_modality,
+ classes=class_names,
+ test_mode=True,
+ box_type_3d='LiDAR'),
+ test=dict(
+ type=dataset_type,
+ data_root=data_root,
+ ann_file=data_root + 'waymo_infos_val.pkl',
+ split='training',
+ pipeline=test_pipeline,
+ modality=input_modality,
+ classes=class_names,
+ test_mode=True,
+ box_type_3d='LiDAR'))
+
+evaluation = dict(interval=24, pipeline=eval_pipeline)
diff --git a/configs/_base_/default_runtime.py b/configs/_base_/default_runtime.py
new file mode 100644
index 0000000..5fc198b
--- /dev/null
+++ b/configs/_base_/default_runtime.py
@@ -0,0 +1,23 @@
+checkpoint_config = dict(interval=1)
+# yapf:disable push
+# By default we use textlogger hook and tensorboard
+# For more loggers see
+# https://mmcv.readthedocs.io/en/latest/api.html#mmcv.runner.LoggerHook
+log_config = dict(
+ interval=50,
+ hooks=[
+ dict(type='TextLoggerHook'),
+ dict(type='TensorboardLoggerHook')
+ ])
+# yapf:enable
+dist_params = dict(backend='nccl')
+log_level = 'INFO'
+work_dir = None
+load_from = None
+resume_from = None
+workflow = [('train', 1)]
+
+# disable opencv multithreading to avoid system being overloaded
+opencv_num_threads = 0
+# set multi-process start method as `fork` to speed up the training
+mp_start_method = 'fork'
diff --git a/configs/_base_/models/3dssd.py b/configs/_base_/models/3dssd.py
new file mode 100644
index 0000000..55344c7
--- /dev/null
+++ b/configs/_base_/models/3dssd.py
@@ -0,0 +1,77 @@
+model = dict(
+ type='SSD3DNet',
+ backbone=dict(
+ type='PointNet2SAMSG',
+ in_channels=4,
+ num_points=(4096, 512, (256, 256)),
+ radii=((0.2, 0.4, 0.8), (0.4, 0.8, 1.6), (1.6, 3.2, 4.8)),
+ num_samples=((32, 32, 64), (32, 32, 64), (32, 32, 32)),
+ sa_channels=(((16, 16, 32), (16, 16, 32), (32, 32, 64)),
+ ((64, 64, 128), (64, 64, 128), (64, 96, 128)),
+ ((128, 128, 256), (128, 192, 256), (128, 256, 256))),
+ aggregation_channels=(64, 128, 256),
+ fps_mods=(('D-FPS'), ('FS'), ('F-FPS', 'D-FPS')),
+ fps_sample_range_lists=((-1), (-1), (512, -1)),
+ norm_cfg=dict(type='BN2d', eps=1e-3, momentum=0.1),
+ sa_cfg=dict(
+ type='PointSAModuleMSG',
+ pool_mod='max',
+ use_xyz=True,
+ normalize_xyz=False)),
+ bbox_head=dict(
+ type='SSD3DHead',
+ in_channels=256,
+ vote_module_cfg=dict(
+ in_channels=256,
+ num_points=256,
+ gt_per_seed=1,
+ conv_channels=(128, ),
+ conv_cfg=dict(type='Conv1d'),
+ norm_cfg=dict(type='BN1d', eps=1e-3, momentum=0.1),
+ with_res_feat=False,
+ vote_xyz_range=(3.0, 3.0, 2.0)),
+ vote_aggregation_cfg=dict(
+ type='PointSAModuleMSG',
+ num_point=256,
+ radii=(4.8, 6.4),
+ sample_nums=(16, 32),
+ mlp_channels=((256, 256, 256, 512), (256, 256, 512, 1024)),
+ norm_cfg=dict(type='BN2d', eps=1e-3, momentum=0.1),
+ use_xyz=True,
+ normalize_xyz=False,
+ bias=True),
+ pred_layer_cfg=dict(
+ in_channels=1536,
+ shared_conv_channels=(512, 128),
+ cls_conv_channels=(128, ),
+ reg_conv_channels=(128, ),
+ conv_cfg=dict(type='Conv1d'),
+ norm_cfg=dict(type='BN1d', eps=1e-3, momentum=0.1),
+ bias=True),
+ conv_cfg=dict(type='Conv1d'),
+ norm_cfg=dict(type='BN1d', eps=1e-3, momentum=0.1),
+ objectness_loss=dict(
+ type='CrossEntropyLoss',
+ use_sigmoid=True,
+ reduction='sum',
+ loss_weight=1.0),
+ center_loss=dict(
+ type='SmoothL1Loss', reduction='sum', loss_weight=1.0),
+ dir_class_loss=dict(
+ type='CrossEntropyLoss', reduction='sum', loss_weight=1.0),
+ dir_res_loss=dict(
+ type='SmoothL1Loss', reduction='sum', loss_weight=1.0),
+ size_res_loss=dict(
+ type='SmoothL1Loss', reduction='sum', loss_weight=1.0),
+ corner_loss=dict(
+ type='SmoothL1Loss', reduction='sum', loss_weight=1.0),
+ vote_loss=dict(type='SmoothL1Loss', reduction='sum', loss_weight=1.0)),
+ # model training and testing settings
+ train_cfg=dict(
+ sample_mod='spec', pos_distance_thr=10.0, expand_dims_length=0.05),
+ test_cfg=dict(
+ nms_cfg=dict(type='nms', iou_thr=0.1),
+ sample_mod='spec',
+ score_thr=0.0,
+ per_class_proposal=True,
+ max_output_num=100))
diff --git a/configs/_base_/models/cascade_mask_rcnn_r50_fpn.py b/configs/_base_/models/cascade_mask_rcnn_r50_fpn.py
new file mode 100644
index 0000000..cafb530
--- /dev/null
+++ b/configs/_base_/models/cascade_mask_rcnn_r50_fpn.py
@@ -0,0 +1,198 @@
+# model settings
+model = dict(
+ type='CascadeRCNN',
+ pretrained='torchvision://resnet50',
+ backbone=dict(
+ type='ResNet',
+ depth=50,
+ num_stages=4,
+ out_indices=(0, 1, 2, 3),
+ frozen_stages=1,
+ norm_cfg=dict(type='BN', requires_grad=True),
+ norm_eval=True,
+ style='pytorch'),
+ neck=dict(
+ type='FPN',
+ in_channels=[256, 512, 1024, 2048],
+ out_channels=256,
+ num_outs=5),
+ rpn_head=dict(
+ type='RPNHead',
+ in_channels=256,
+ feat_channels=256,
+ anchor_generator=dict(
+ type='AnchorGenerator',
+ scales=[8],
+ ratios=[0.5, 1.0, 2.0],
+ strides=[4, 8, 16, 32, 64]),
+ bbox_coder=dict(
+ type='DeltaXYWHBBoxCoder',
+ target_means=[.0, .0, .0, .0],
+ target_stds=[1.0, 1.0, 1.0, 1.0]),
+ loss_cls=dict(
+ type='CrossEntropyLoss', use_sigmoid=True, loss_weight=1.0),
+ loss_bbox=dict(type='SmoothL1Loss', beta=1.0 / 9.0, loss_weight=1.0)),
+ roi_head=dict(
+ type='CascadeRoIHead',
+ num_stages=3,
+ stage_loss_weights=[1, 0.5, 0.25],
+ bbox_roi_extractor=dict(
+ type='SingleRoIExtractor',
+ roi_layer=dict(type='RoIAlign', output_size=7, sampling_ratio=0),
+ out_channels=256,
+ featmap_strides=[4, 8, 16, 32]),
+ bbox_head=[
+ dict(
+ type='Shared2FCBBoxHead',
+ in_channels=256,
+ fc_out_channels=1024,
+ roi_feat_size=7,
+ num_classes=80,
+ bbox_coder=dict(
+ type='DeltaXYWHBBoxCoder',
+ target_means=[0., 0., 0., 0.],
+ target_stds=[0.1, 0.1, 0.2, 0.2]),
+ reg_class_agnostic=True,
+ loss_cls=dict(
+ type='CrossEntropyLoss',
+ use_sigmoid=False,
+ loss_weight=1.0),
+ loss_bbox=dict(type='SmoothL1Loss', beta=1.0,
+ loss_weight=1.0)),
+ dict(
+ type='Shared2FCBBoxHead',
+ in_channels=256,
+ fc_out_channels=1024,
+ roi_feat_size=7,
+ num_classes=80,
+ bbox_coder=dict(
+ type='DeltaXYWHBBoxCoder',
+ target_means=[0., 0., 0., 0.],
+ target_stds=[0.05, 0.05, 0.1, 0.1]),
+ reg_class_agnostic=True,
+ loss_cls=dict(
+ type='CrossEntropyLoss',
+ use_sigmoid=False,
+ loss_weight=1.0),
+ loss_bbox=dict(type='SmoothL1Loss', beta=1.0,
+ loss_weight=1.0)),
+ dict(
+ type='Shared2FCBBoxHead',
+ in_channels=256,
+ fc_out_channels=1024,
+ roi_feat_size=7,
+ num_classes=80,
+ bbox_coder=dict(
+ type='DeltaXYWHBBoxCoder',
+ target_means=[0., 0., 0., 0.],
+ target_stds=[0.033, 0.033, 0.067, 0.067]),
+ reg_class_agnostic=True,
+ loss_cls=dict(
+ type='CrossEntropyLoss',
+ use_sigmoid=False,
+ loss_weight=1.0),
+ loss_bbox=dict(type='SmoothL1Loss', beta=1.0, loss_weight=1.0))
+ ],
+ mask_roi_extractor=dict(
+ type='SingleRoIExtractor',
+ roi_layer=dict(type='RoIAlign', output_size=14, sampling_ratio=0),
+ out_channels=256,
+ featmap_strides=[4, 8, 16, 32]),
+ mask_head=dict(
+ type='FCNMaskHead',
+ num_convs=4,
+ in_channels=256,
+ conv_out_channels=256,
+ num_classes=80,
+ loss_mask=dict(
+ type='CrossEntropyLoss', use_mask=True, loss_weight=1.0))),
+ # model training and testing settings
+ train_cfg=dict(
+ rpn=dict(
+ assigner=dict(
+ type='MaxIoUAssigner',
+ pos_iou_thr=0.7,
+ neg_iou_thr=0.3,
+ min_pos_iou=0.3,
+ match_low_quality=True,
+ ignore_iof_thr=-1),
+ sampler=dict(
+ type='RandomSampler',
+ num=256,
+ pos_fraction=0.5,
+ neg_pos_ub=-1,
+ add_gt_as_proposals=False),
+ allowed_border=0,
+ pos_weight=-1,
+ debug=False),
+ rpn_proposal=dict(
+ nms_pre=2000,
+ nms_post=2000,
+ max_per_img=2000,
+ nms=dict(type='nms', iou_threshold=0.7),
+ min_bbox_size=0),
+ rcnn=[
+ dict(
+ assigner=dict(
+ type='MaxIoUAssigner',
+ pos_iou_thr=0.5,
+ neg_iou_thr=0.5,
+ min_pos_iou=0.5,
+ match_low_quality=False,
+ ignore_iof_thr=-1),
+ sampler=dict(
+ type='RandomSampler',
+ num=512,
+ pos_fraction=0.25,
+ neg_pos_ub=-1,
+ add_gt_as_proposals=True),
+ mask_size=28,
+ pos_weight=-1,
+ debug=False),
+ dict(
+ assigner=dict(
+ type='MaxIoUAssigner',
+ pos_iou_thr=0.6,
+ neg_iou_thr=0.6,
+ min_pos_iou=0.6,
+ match_low_quality=False,
+ ignore_iof_thr=-1),
+ sampler=dict(
+ type='RandomSampler',
+ num=512,
+ pos_fraction=0.25,
+ neg_pos_ub=-1,
+ add_gt_as_proposals=True),
+ mask_size=28,
+ pos_weight=-1,
+ debug=False),
+ dict(
+ assigner=dict(
+ type='MaxIoUAssigner',
+ pos_iou_thr=0.7,
+ neg_iou_thr=0.7,
+ min_pos_iou=0.7,
+ match_low_quality=False,
+ ignore_iof_thr=-1),
+ sampler=dict(
+ type='RandomSampler',
+ num=512,
+ pos_fraction=0.25,
+ neg_pos_ub=-1,
+ add_gt_as_proposals=True),
+ mask_size=28,
+ pos_weight=-1,
+ debug=False)
+ ]),
+ test_cfg=dict(
+ rpn=dict(
+ nms_pre=1000,
+ nms_post=1000,
+ max_per_img=1000,
+ nms=dict(type='nms', iou_threshold=0.7),
+ min_bbox_size=0),
+ rcnn=dict(
+ score_thr=0.05,
+ nms=dict(type='nms', iou_threshold=0.5),
+ max_per_img=100,
+ mask_thr_binary=0.5)))
diff --git a/configs/_base_/models/centerpoint_01voxel_second_secfpn_nus.py b/configs/_base_/models/centerpoint_01voxel_second_secfpn_nus.py
new file mode 100644
index 0000000..efdce59
--- /dev/null
+++ b/configs/_base_/models/centerpoint_01voxel_second_secfpn_nus.py
@@ -0,0 +1,83 @@
+voxel_size = [0.1, 0.1, 0.2]
+model = dict(
+ type='CenterPoint',
+ pts_voxel_layer=dict(
+ max_num_points=10, voxel_size=voxel_size, max_voxels=(90000, 120000)),
+ pts_voxel_encoder=dict(type='HardSimpleVFE', num_features=5),
+ pts_middle_encoder=dict(
+ type='SparseEncoder',
+ in_channels=5,
+ sparse_shape=[41, 1024, 1024],
+ output_channels=128,
+ order=('conv', 'norm', 'act'),
+ encoder_channels=((16, 16, 32), (32, 32, 64), (64, 64, 128), (128,
+ 128)),
+ encoder_paddings=((0, 0, 1), (0, 0, 1), (0, 0, [0, 1, 1]), (0, 0)),
+ block_type='basicblock'),
+ pts_backbone=dict(
+ type='SECOND',
+ in_channels=256,
+ out_channels=[128, 256],
+ layer_nums=[5, 5],
+ layer_strides=[1, 2],
+ norm_cfg=dict(type='BN', eps=1e-3, momentum=0.01),
+ conv_cfg=dict(type='Conv2d', bias=False)),
+ pts_neck=dict(
+ type='SECONDFPN',
+ in_channels=[128, 256],
+ out_channels=[256, 256],
+ upsample_strides=[1, 2],
+ norm_cfg=dict(type='BN', eps=1e-3, momentum=0.01),
+ upsample_cfg=dict(type='deconv', bias=False),
+ use_conv_for_no_stride=True),
+ pts_bbox_head=dict(
+ type='CenterHead',
+ in_channels=sum([256, 256]),
+ tasks=[
+ dict(num_class=1, class_names=['car']),
+ dict(num_class=2, class_names=['truck', 'construction_vehicle']),
+ dict(num_class=2, class_names=['bus', 'trailer']),
+ dict(num_class=1, class_names=['barrier']),
+ dict(num_class=2, class_names=['motorcycle', 'bicycle']),
+ dict(num_class=2, class_names=['pedestrian', 'traffic_cone']),
+ ],
+ common_heads=dict(
+ reg=(2, 2), height=(1, 2), dim=(3, 2), rot=(2, 2), vel=(2, 2)),
+ share_conv_channel=64,
+ bbox_coder=dict(
+ type='CenterPointBBoxCoder',
+ post_center_range=[-61.2, -61.2, -10.0, 61.2, 61.2, 10.0],
+ max_num=500,
+ score_threshold=0.1,
+ out_size_factor=8,
+ voxel_size=voxel_size[:2],
+ code_size=9),
+ separate_head=dict(
+ type='SeparateHead', init_bias=-2.19, final_kernel=3),
+ loss_cls=dict(type='GaussianFocalLoss', reduction='mean'),
+ loss_bbox=dict(type='L1Loss', reduction='mean', loss_weight=0.25),
+ norm_bbox=True),
+ # model training and testing settings
+ train_cfg=dict(
+ pts=dict(
+ grid_size=[1024, 1024, 40],
+ voxel_size=voxel_size,
+ out_size_factor=8,
+ dense_reg=1,
+ gaussian_overlap=0.1,
+ max_objs=500,
+ min_radius=2,
+ code_weights=[1.0, 1.0, 1.0, 1.0, 1.0, 1.0, 1.0, 1.0, 0.2, 0.2])),
+ test_cfg=dict(
+ pts=dict(
+ post_center_limit_range=[-61.2, -61.2, -10.0, 61.2, 61.2, 10.0],
+ max_per_img=500,
+ max_pool_nms=False,
+ min_radius=[4, 12, 10, 1, 0.85, 0.175],
+ score_threshold=0.1,
+ out_size_factor=8,
+ voxel_size=voxel_size[:2],
+ nms_type='rotate',
+ pre_max_size=1000,
+ post_max_size=83,
+ nms_thr=0.2)))
diff --git a/configs/_base_/models/centerpoint_02pillar_second_secfpn_nus.py b/configs/_base_/models/centerpoint_02pillar_second_secfpn_nus.py
new file mode 100644
index 0000000..311d763
--- /dev/null
+++ b/configs/_base_/models/centerpoint_02pillar_second_secfpn_nus.py
@@ -0,0 +1,83 @@
+voxel_size = [0.2, 0.2, 8]
+model = dict(
+ type='CenterPoint',
+ pts_voxel_layer=dict(
+ max_num_points=20, voxel_size=voxel_size, max_voxels=(30000, 40000)),
+ pts_voxel_encoder=dict(
+ type='PillarFeatureNet',
+ in_channels=5,
+ feat_channels=[64],
+ with_distance=False,
+ voxel_size=(0.2, 0.2, 8),
+ norm_cfg=dict(type='BN1d', eps=1e-3, momentum=0.01),
+ legacy=False),
+ pts_middle_encoder=dict(
+ type='PointPillarsScatter', in_channels=64, output_shape=(512, 512)),
+ pts_backbone=dict(
+ type='SECOND',
+ in_channels=64,
+ out_channels=[64, 128, 256],
+ layer_nums=[3, 5, 5],
+ layer_strides=[2, 2, 2],
+ norm_cfg=dict(type='BN', eps=1e-3, momentum=0.01),
+ conv_cfg=dict(type='Conv2d', bias=False)),
+ pts_neck=dict(
+ type='SECONDFPN',
+ in_channels=[64, 128, 256],
+ out_channels=[128, 128, 128],
+ upsample_strides=[0.5, 1, 2],
+ norm_cfg=dict(type='BN', eps=1e-3, momentum=0.01),
+ upsample_cfg=dict(type='deconv', bias=False),
+ use_conv_for_no_stride=True),
+ pts_bbox_head=dict(
+ type='CenterHead',
+ in_channels=sum([128, 128, 128]),
+ tasks=[
+ dict(num_class=1, class_names=['car']),
+ dict(num_class=2, class_names=['truck', 'construction_vehicle']),
+ dict(num_class=2, class_names=['bus', 'trailer']),
+ dict(num_class=1, class_names=['barrier']),
+ dict(num_class=2, class_names=['motorcycle', 'bicycle']),
+ dict(num_class=2, class_names=['pedestrian', 'traffic_cone']),
+ ],
+ common_heads=dict(
+ reg=(2, 2), height=(1, 2), dim=(3, 2), rot=(2, 2), vel=(2, 2)),
+ share_conv_channel=64,
+ bbox_coder=dict(
+ type='CenterPointBBoxCoder',
+ post_center_range=[-61.2, -61.2, -10.0, 61.2, 61.2, 10.0],
+ max_num=500,
+ score_threshold=0.1,
+ out_size_factor=4,
+ voxel_size=voxel_size[:2],
+ code_size=9),
+ separate_head=dict(
+ type='SeparateHead', init_bias=-2.19, final_kernel=3),
+ loss_cls=dict(type='GaussianFocalLoss', reduction='mean'),
+ loss_bbox=dict(type='L1Loss', reduction='mean', loss_weight=0.25),
+ norm_bbox=True),
+ # model training and testing settings
+ train_cfg=dict(
+ pts=dict(
+ grid_size=[512, 512, 1],
+ voxel_size=voxel_size,
+ out_size_factor=4,
+ dense_reg=1,
+ gaussian_overlap=0.1,
+ max_objs=500,
+ min_radius=2,
+ code_weights=[1.0, 1.0, 1.0, 1.0, 1.0, 1.0, 1.0, 1.0, 0.2, 0.2])),
+ test_cfg=dict(
+ pts=dict(
+ post_center_limit_range=[-61.2, -61.2, -10.0, 61.2, 61.2, 10.0],
+ max_per_img=500,
+ max_pool_nms=False,
+ min_radius=[4, 12, 10, 1, 0.85, 0.175],
+ score_threshold=0.1,
+ pc_range=[-51.2, -51.2],
+ out_size_factor=4,
+ voxel_size=voxel_size[:2],
+ nms_type='rotate',
+ pre_max_size=1000,
+ post_max_size=83,
+ nms_thr=0.2)))
diff --git a/configs/_base_/models/dgcnn.py b/configs/_base_/models/dgcnn.py
new file mode 100644
index 0000000..61e7272
--- /dev/null
+++ b/configs/_base_/models/dgcnn.py
@@ -0,0 +1,28 @@
+# model settings
+model = dict(
+ type='EncoderDecoder3D',
+ backbone=dict(
+ type='DGCNNBackbone',
+ in_channels=9, # [xyz, rgb, normal_xyz], modified with dataset
+ num_samples=(20, 20, 20),
+ knn_modes=('D-KNN', 'F-KNN', 'F-KNN'),
+ radius=(None, None, None),
+ gf_channels=((64, 64), (64, 64), (64, )),
+ fa_channels=(1024, ),
+ act_cfg=dict(type='LeakyReLU', negative_slope=0.2)),
+ decode_head=dict(
+ type='DGCNNHead',
+ fp_channels=(1216, 512),
+ channels=256,
+ dropout_ratio=0.5,
+ conv_cfg=dict(type='Conv1d'),
+ norm_cfg=dict(type='BN1d'),
+ act_cfg=dict(type='LeakyReLU', negative_slope=0.2),
+ loss_decode=dict(
+ type='CrossEntropyLoss',
+ use_sigmoid=False,
+ class_weight=None, # modified with dataset
+ loss_weight=1.0)),
+ # model training and testing settings
+ train_cfg=dict(),
+ test_cfg=dict(mode='slide'))
diff --git a/configs/_base_/models/fcaf3d.py b/configs/_base_/models/fcaf3d.py
new file mode 100644
index 0000000..b92bfad
--- /dev/null
+++ b/configs/_base_/models/fcaf3d.py
@@ -0,0 +1,16 @@
+model = dict(
+ type='MinkSingleStage3DDetector',
+ voxel_size=.01,
+ backbone=dict(type='MinkResNet', in_channels=3, depth=34),
+ head=dict(
+ type='FCAF3DHead',
+ in_channels=(64, 128, 256, 512),
+ out_channels=128,
+ voxel_size=.01,
+ pts_prune_threshold=100000,
+ pts_assign_threshold=27,
+ pts_center_threshold=18,
+ n_classes=18,
+ n_reg_outs=6),
+ train_cfg=dict(),
+ test_cfg=dict(nms_pre=1000, iou_thr=.5, score_thr=.01))
diff --git a/configs/_base_/models/fcos3d.py b/configs/_base_/models/fcos3d.py
new file mode 100644
index 0000000..be83001
--- /dev/null
+++ b/configs/_base_/models/fcos3d.py
@@ -0,0 +1,78 @@
+model = dict(
+ type='FCOSMono3D',
+ backbone=dict(
+ type='ResNet',
+ depth=101,
+ num_stages=4,
+ out_indices=(0, 1, 2, 3),
+ frozen_stages=1,
+ norm_cfg=dict(type='BN', requires_grad=False),
+ norm_eval=True,
+ style='caffe',
+ init_cfg=dict(
+ type='Pretrained',
+ checkpoint='open-mmlab://detectron2/resnet101_caffe')),
+ neck=dict(
+ type='FPN',
+ in_channels=[256, 512, 1024, 2048],
+ out_channels=256,
+ start_level=1,
+ add_extra_convs='on_output',
+ num_outs=5,
+ relu_before_extra_convs=True),
+ bbox_head=dict(
+ type='FCOSMono3DHead',
+ num_classes=10,
+ in_channels=256,
+ stacked_convs=2,
+ feat_channels=256,
+ use_direction_classifier=True,
+ diff_rad_by_sin=True,
+ pred_attrs=True,
+ pred_velo=True,
+ dir_offset=0.7854, # pi/4
+ dir_limit_offset=0,
+ strides=[8, 16, 32, 64, 128],
+ group_reg_dims=(2, 1, 3, 1, 2), # offset, depth, size, rot, velo
+ cls_branch=(256, ),
+ reg_branch=(
+ (256, ), # offset
+ (256, ), # depth
+ (256, ), # size
+ (256, ), # rot
+ () # velo
+ ),
+ dir_branch=(256, ),
+ attr_branch=(256, ),
+ loss_cls=dict(
+ type='FocalLoss',
+ use_sigmoid=True,
+ gamma=2.0,
+ alpha=0.25,
+ loss_weight=1.0),
+ loss_bbox=dict(type='SmoothL1Loss', beta=1.0 / 9.0, loss_weight=1.0),
+ loss_dir=dict(
+ type='CrossEntropyLoss', use_sigmoid=False, loss_weight=1.0),
+ loss_attr=dict(
+ type='CrossEntropyLoss', use_sigmoid=False, loss_weight=1.0),
+ loss_centerness=dict(
+ type='CrossEntropyLoss', use_sigmoid=True, loss_weight=1.0),
+ bbox_coder=dict(type='FCOS3DBBoxCoder', code_size=9),
+ norm_on_bbox=True,
+ centerness_on_reg=True,
+ center_sampling=True,
+ conv_bias=True,
+ dcn_on_last_conv=True),
+ train_cfg=dict(
+ allowed_border=0,
+ code_weight=[1.0, 1.0, 0.2, 1.0, 1.0, 1.0, 1.0, 0.05, 0.05],
+ pos_weight=-1,
+ debug=False),
+ test_cfg=dict(
+ use_rotate_nms=True,
+ nms_across_levels=False,
+ nms_pre=1000,
+ nms_thr=0.8,
+ score_thr=0.05,
+ min_bbox_size=0,
+ max_per_img=200))
diff --git a/configs/_base_/models/groupfree3d.py b/configs/_base_/models/groupfree3d.py
new file mode 100644
index 0000000..077d049
--- /dev/null
+++ b/configs/_base_/models/groupfree3d.py
@@ -0,0 +1,71 @@
+model = dict(
+ type='GroupFree3DNet',
+ backbone=dict(
+ type='PointNet2SASSG',
+ in_channels=3,
+ num_points=(2048, 1024, 512, 256),
+ radius=(0.2, 0.4, 0.8, 1.2),
+ num_samples=(64, 32, 16, 16),
+ sa_channels=((64, 64, 128), (128, 128, 256), (128, 128, 256),
+ (128, 128, 256)),
+ fp_channels=((256, 256), (256, 288)),
+ norm_cfg=dict(type='BN2d'),
+ sa_cfg=dict(
+ type='PointSAModule',
+ pool_mod='max',
+ use_xyz=True,
+ normalize_xyz=True)),
+ bbox_head=dict(
+ type='GroupFree3DHead',
+ in_channels=288,
+ num_decoder_layers=6,
+ num_proposal=256,
+ transformerlayers=dict(
+ type='BaseTransformerLayer',
+ attn_cfgs=dict(
+ type='GroupFree3DMHA',
+ embed_dims=288,
+ num_heads=8,
+ attn_drop=0.1,
+ dropout_layer=dict(type='Dropout', drop_prob=0.1)),
+ ffn_cfgs=dict(
+ embed_dims=288,
+ feedforward_channels=2048,
+ ffn_drop=0.1,
+ act_cfg=dict(type='ReLU', inplace=True)),
+ operation_order=('self_attn', 'norm', 'cross_attn', 'norm', 'ffn',
+ 'norm')),
+ pred_layer_cfg=dict(
+ in_channels=288, shared_conv_channels=(288, 288), bias=True),
+ sampling_objectness_loss=dict(
+ type='FocalLoss',
+ use_sigmoid=True,
+ gamma=2.0,
+ alpha=0.25,
+ loss_weight=8.0),
+ objectness_loss=dict(
+ type='FocalLoss',
+ use_sigmoid=True,
+ gamma=2.0,
+ alpha=0.25,
+ loss_weight=1.0),
+ center_loss=dict(
+ type='SmoothL1Loss', reduction='sum', loss_weight=10.0),
+ dir_class_loss=dict(
+ type='CrossEntropyLoss', reduction='sum', loss_weight=1.0),
+ dir_res_loss=dict(
+ type='SmoothL1Loss', reduction='sum', loss_weight=10.0),
+ size_class_loss=dict(
+ type='CrossEntropyLoss', reduction='sum', loss_weight=1.0),
+ size_res_loss=dict(
+ type='SmoothL1Loss', beta=1.0, reduction='sum', loss_weight=10.0),
+ semantic_loss=dict(
+ type='CrossEntropyLoss', reduction='sum', loss_weight=1.0)),
+ # model training and testing settings
+ train_cfg=dict(sample_mod='kps'),
+ test_cfg=dict(
+ sample_mod='kps',
+ nms_thr=0.25,
+ score_thr=0.0,
+ per_class_proposal=True,
+ prediction_stages='last'))
diff --git a/configs/_base_/models/h3dnet.py b/configs/_base_/models/h3dnet.py
new file mode 100644
index 0000000..7605667
--- /dev/null
+++ b/configs/_base_/models/h3dnet.py
@@ -0,0 +1,341 @@
+primitive_z_cfg = dict(
+ type='PrimitiveHead',
+ num_dims=2,
+ num_classes=18,
+ primitive_mode='z',
+ upper_thresh=100.0,
+ surface_thresh=0.5,
+ vote_module_cfg=dict(
+ in_channels=256,
+ vote_per_seed=1,
+ gt_per_seed=1,
+ conv_channels=(256, 256),
+ conv_cfg=dict(type='Conv1d'),
+ norm_cfg=dict(type='BN1d'),
+ norm_feats=True,
+ vote_loss=dict(
+ type='ChamferDistance',
+ mode='l1',
+ reduction='none',
+ loss_dst_weight=10.0)),
+ vote_aggregation_cfg=dict(
+ type='PointSAModule',
+ num_point=1024,
+ radius=0.3,
+ num_sample=16,
+ mlp_channels=[256, 128, 128, 128],
+ use_xyz=True,
+ normalize_xyz=True),
+ feat_channels=(128, 128),
+ conv_cfg=dict(type='Conv1d'),
+ norm_cfg=dict(type='BN1d'),
+ objectness_loss=dict(
+ type='CrossEntropyLoss',
+ class_weight=[0.4, 0.6],
+ reduction='mean',
+ loss_weight=30.0),
+ center_loss=dict(
+ type='ChamferDistance',
+ mode='l1',
+ reduction='sum',
+ loss_src_weight=0.5,
+ loss_dst_weight=0.5),
+ semantic_reg_loss=dict(
+ type='ChamferDistance',
+ mode='l1',
+ reduction='sum',
+ loss_src_weight=0.5,
+ loss_dst_weight=0.5),
+ semantic_cls_loss=dict(
+ type='CrossEntropyLoss', reduction='sum', loss_weight=1.0),
+ train_cfg=dict(
+ dist_thresh=0.2,
+ var_thresh=1e-2,
+ lower_thresh=1e-6,
+ num_point=100,
+ num_point_line=10,
+ line_thresh=0.2))
+
+primitive_xy_cfg = dict(
+ type='PrimitiveHead',
+ num_dims=1,
+ num_classes=18,
+ primitive_mode='xy',
+ upper_thresh=100.0,
+ surface_thresh=0.5,
+ vote_module_cfg=dict(
+ in_channels=256,
+ vote_per_seed=1,
+ gt_per_seed=1,
+ conv_channels=(256, 256),
+ conv_cfg=dict(type='Conv1d'),
+ norm_cfg=dict(type='BN1d'),
+ norm_feats=True,
+ vote_loss=dict(
+ type='ChamferDistance',
+ mode='l1',
+ reduction='none',
+ loss_dst_weight=10.0)),
+ vote_aggregation_cfg=dict(
+ type='PointSAModule',
+ num_point=1024,
+ radius=0.3,
+ num_sample=16,
+ mlp_channels=[256, 128, 128, 128],
+ use_xyz=True,
+ normalize_xyz=True),
+ feat_channels=(128, 128),
+ conv_cfg=dict(type='Conv1d'),
+ norm_cfg=dict(type='BN1d'),
+ objectness_loss=dict(
+ type='CrossEntropyLoss',
+ class_weight=[0.4, 0.6],
+ reduction='mean',
+ loss_weight=30.0),
+ center_loss=dict(
+ type='ChamferDistance',
+ mode='l1',
+ reduction='sum',
+ loss_src_weight=0.5,
+ loss_dst_weight=0.5),
+ semantic_reg_loss=dict(
+ type='ChamferDistance',
+ mode='l1',
+ reduction='sum',
+ loss_src_weight=0.5,
+ loss_dst_weight=0.5),
+ semantic_cls_loss=dict(
+ type='CrossEntropyLoss', reduction='sum', loss_weight=1.0),
+ train_cfg=dict(
+ dist_thresh=0.2,
+ var_thresh=1e-2,
+ lower_thresh=1e-6,
+ num_point=100,
+ num_point_line=10,
+ line_thresh=0.2))
+
+primitive_line_cfg = dict(
+ type='PrimitiveHead',
+ num_dims=0,
+ num_classes=18,
+ primitive_mode='line',
+ upper_thresh=100.0,
+ surface_thresh=0.5,
+ vote_module_cfg=dict(
+ in_channels=256,
+ vote_per_seed=1,
+ gt_per_seed=1,
+ conv_channels=(256, 256),
+ conv_cfg=dict(type='Conv1d'),
+ norm_cfg=dict(type='BN1d'),
+ norm_feats=True,
+ vote_loss=dict(
+ type='ChamferDistance',
+ mode='l1',
+ reduction='none',
+ loss_dst_weight=10.0)),
+ vote_aggregation_cfg=dict(
+ type='PointSAModule',
+ num_point=1024,
+ radius=0.3,
+ num_sample=16,
+ mlp_channels=[256, 128, 128, 128],
+ use_xyz=True,
+ normalize_xyz=True),
+ feat_channels=(128, 128),
+ conv_cfg=dict(type='Conv1d'),
+ norm_cfg=dict(type='BN1d'),
+ objectness_loss=dict(
+ type='CrossEntropyLoss',
+ class_weight=[0.4, 0.6],
+ reduction='mean',
+ loss_weight=30.0),
+ center_loss=dict(
+ type='ChamferDistance',
+ mode='l1',
+ reduction='sum',
+ loss_src_weight=1.0,
+ loss_dst_weight=1.0),
+ semantic_reg_loss=dict(
+ type='ChamferDistance',
+ mode='l1',
+ reduction='sum',
+ loss_src_weight=1.0,
+ loss_dst_weight=1.0),
+ semantic_cls_loss=dict(
+ type='CrossEntropyLoss', reduction='sum', loss_weight=2.0),
+ train_cfg=dict(
+ dist_thresh=0.2,
+ var_thresh=1e-2,
+ lower_thresh=1e-6,
+ num_point=100,
+ num_point_line=10,
+ line_thresh=0.2))
+
+model = dict(
+ type='H3DNet',
+ backbone=dict(
+ type='MultiBackbone',
+ num_streams=4,
+ suffixes=['net0', 'net1', 'net2', 'net3'],
+ conv_cfg=dict(type='Conv1d'),
+ norm_cfg=dict(type='BN1d', eps=1e-5, momentum=0.01),
+ act_cfg=dict(type='ReLU'),
+ backbones=dict(
+ type='PointNet2SASSG',
+ in_channels=4,
+ num_points=(2048, 1024, 512, 256),
+ radius=(0.2, 0.4, 0.8, 1.2),
+ num_samples=(64, 32, 16, 16),
+ sa_channels=((64, 64, 128), (128, 128, 256), (128, 128, 256),
+ (128, 128, 256)),
+ fp_channels=((256, 256), (256, 256)),
+ norm_cfg=dict(type='BN2d'),
+ sa_cfg=dict(
+ type='PointSAModule',
+ pool_mod='max',
+ use_xyz=True,
+ normalize_xyz=True))),
+ rpn_head=dict(
+ type='VoteHead',
+ vote_module_cfg=dict(
+ in_channels=256,
+ vote_per_seed=1,
+ gt_per_seed=3,
+ conv_channels=(256, 256),
+ conv_cfg=dict(type='Conv1d'),
+ norm_cfg=dict(type='BN1d'),
+ norm_feats=True,
+ vote_loss=dict(
+ type='ChamferDistance',
+ mode='l1',
+ reduction='none',
+ loss_dst_weight=10.0)),
+ vote_aggregation_cfg=dict(
+ type='PointSAModule',
+ num_point=256,
+ radius=0.3,
+ num_sample=16,
+ mlp_channels=[256, 128, 128, 128],
+ use_xyz=True,
+ normalize_xyz=True),
+ pred_layer_cfg=dict(
+ in_channels=128, shared_conv_channels=(128, 128), bias=True),
+ conv_cfg=dict(type='Conv1d'),
+ norm_cfg=dict(type='BN1d'),
+ objectness_loss=dict(
+ type='CrossEntropyLoss',
+ class_weight=[0.2, 0.8],
+ reduction='sum',
+ loss_weight=5.0),
+ center_loss=dict(
+ type='ChamferDistance',
+ mode='l2',
+ reduction='sum',
+ loss_src_weight=10.0,
+ loss_dst_weight=10.0),
+ dir_class_loss=dict(
+ type='CrossEntropyLoss', reduction='sum', loss_weight=1.0),
+ dir_res_loss=dict(
+ type='SmoothL1Loss', reduction='sum', loss_weight=10.0),
+ size_class_loss=dict(
+ type='CrossEntropyLoss', reduction='sum', loss_weight=1.0),
+ size_res_loss=dict(
+ type='SmoothL1Loss', reduction='sum', loss_weight=10.0),
+ semantic_loss=dict(
+ type='CrossEntropyLoss', reduction='sum', loss_weight=1.0)),
+ roi_head=dict(
+ type='H3DRoIHead',
+ primitive_list=[primitive_z_cfg, primitive_xy_cfg, primitive_line_cfg],
+ bbox_head=dict(
+ type='H3DBboxHead',
+ gt_per_seed=3,
+ num_proposal=256,
+ suface_matching_cfg=dict(
+ type='PointSAModule',
+ num_point=256 * 6,
+ radius=0.5,
+ num_sample=32,
+ mlp_channels=[128 + 6, 128, 64, 32],
+ use_xyz=True,
+ normalize_xyz=True),
+ line_matching_cfg=dict(
+ type='PointSAModule',
+ num_point=256 * 12,
+ radius=0.5,
+ num_sample=32,
+ mlp_channels=[128 + 12, 128, 64, 32],
+ use_xyz=True,
+ normalize_xyz=True),
+ feat_channels=(128, 128),
+ primitive_refine_channels=[128, 128, 128],
+ upper_thresh=100.0,
+ surface_thresh=0.5,
+ line_thresh=0.5,
+ conv_cfg=dict(type='Conv1d'),
+ norm_cfg=dict(type='BN1d'),
+ objectness_loss=dict(
+ type='CrossEntropyLoss',
+ class_weight=[0.2, 0.8],
+ reduction='sum',
+ loss_weight=5.0),
+ center_loss=dict(
+ type='ChamferDistance',
+ mode='l2',
+ reduction='sum',
+ loss_src_weight=10.0,
+ loss_dst_weight=10.0),
+ dir_class_loss=dict(
+ type='CrossEntropyLoss', reduction='sum', loss_weight=0.1),
+ dir_res_loss=dict(
+ type='SmoothL1Loss', reduction='sum', loss_weight=10.0),
+ size_class_loss=dict(
+ type='CrossEntropyLoss', reduction='sum', loss_weight=0.1),
+ size_res_loss=dict(
+ type='SmoothL1Loss', reduction='sum', loss_weight=10.0),
+ semantic_loss=dict(
+ type='CrossEntropyLoss', reduction='sum', loss_weight=0.1),
+ cues_objectness_loss=dict(
+ type='CrossEntropyLoss',
+ class_weight=[0.3, 0.7],
+ reduction='mean',
+ loss_weight=5.0),
+ cues_semantic_loss=dict(
+ type='CrossEntropyLoss',
+ class_weight=[0.3, 0.7],
+ reduction='mean',
+ loss_weight=5.0),
+ proposal_objectness_loss=dict(
+ type='CrossEntropyLoss',
+ class_weight=[0.2, 0.8],
+ reduction='none',
+ loss_weight=5.0),
+ primitive_center_loss=dict(
+ type='MSELoss', reduction='none', loss_weight=1.0))),
+ # model training and testing settings
+ train_cfg=dict(
+ rpn=dict(
+ pos_distance_thr=0.3, neg_distance_thr=0.6, sample_mod='vote'),
+ rpn_proposal=dict(use_nms=False),
+ rcnn=dict(
+ pos_distance_thr=0.3,
+ neg_distance_thr=0.6,
+ sample_mod='vote',
+ far_threshold=0.6,
+ near_threshold=0.3,
+ mask_surface_threshold=0.3,
+ label_surface_threshold=0.3,
+ mask_line_threshold=0.3,
+ label_line_threshold=0.3)),
+ test_cfg=dict(
+ rpn=dict(
+ sample_mod='seed',
+ nms_thr=0.25,
+ score_thr=0.05,
+ per_class_proposal=True,
+ use_nms=False),
+ rcnn=dict(
+ sample_mod='seed',
+ nms_thr=0.25,
+ score_thr=0.05,
+ per_class_proposal=True)))
diff --git a/configs/_base_/models/hv_pointpillars_fpn_lyft.py b/configs/_base_/models/hv_pointpillars_fpn_lyft.py
new file mode 100644
index 0000000..87c7fe0
--- /dev/null
+++ b/configs/_base_/models/hv_pointpillars_fpn_lyft.py
@@ -0,0 +1,22 @@
+_base_ = './hv_pointpillars_fpn_nus.py'
+
+# model settings (based on nuScenes model settings)
+# Voxel size for voxel encoder
+# Usually voxel size is changed consistently with the point cloud range
+# If point cloud range is modified, do remember to change all related
+# keys in the config.
+model = dict(
+ pts_voxel_layer=dict(
+ max_num_points=20,
+ point_cloud_range=[-80, -80, -5, 80, 80, 3],
+ max_voxels=(60000, 60000)),
+ pts_voxel_encoder=dict(
+ feat_channels=[64], point_cloud_range=[-80, -80, -5, 80, 80, 3]),
+ pts_middle_encoder=dict(output_shape=[640, 640]),
+ pts_bbox_head=dict(
+ num_classes=9,
+ anchor_generator=dict(
+ ranges=[[-80, -80, -1.8, 80, 80, -1.8]], custom_values=[]),
+ bbox_coder=dict(type='DeltaXYZWLHRBBoxCoder', code_size=7)),
+ # model training settings (based on nuScenes model settings)
+ train_cfg=dict(pts=dict(code_weight=[1.0, 1.0, 1.0, 1.0, 1.0, 1.0, 1.0])))
diff --git a/configs/_base_/models/hv_pointpillars_fpn_nus.py b/configs/_base_/models/hv_pointpillars_fpn_nus.py
new file mode 100644
index 0000000..be29269
--- /dev/null
+++ b/configs/_base_/models/hv_pointpillars_fpn_nus.py
@@ -0,0 +1,95 @@
+# model settings
+# Voxel size for voxel encoder
+# Usually voxel size is changed consistently with the point cloud range
+# If point cloud range is modified, do remember to change all related
+# keys in the config.
+voxel_size = [0.25, 0.25, 8]
+model = dict(
+ type='MVXFasterRCNN',
+ pts_voxel_layer=dict(
+ max_num_points=64,
+ point_cloud_range=[-50, -50, -5, 50, 50, 3],
+ voxel_size=voxel_size,
+ max_voxels=(30000, 40000)),
+ pts_voxel_encoder=dict(
+ type='HardVFE',
+ in_channels=4,
+ feat_channels=[64, 64],
+ with_distance=False,
+ voxel_size=voxel_size,
+ with_cluster_center=True,
+ with_voxel_center=True,
+ point_cloud_range=[-50, -50, -5, 50, 50, 3],
+ norm_cfg=dict(type='naiveSyncBN1d', eps=1e-3, momentum=0.01)),
+ pts_middle_encoder=dict(
+ type='PointPillarsScatter', in_channels=64, output_shape=[400, 400]),
+ pts_backbone=dict(
+ type='SECOND',
+ in_channels=64,
+ norm_cfg=dict(type='naiveSyncBN2d', eps=1e-3, momentum=0.01),
+ layer_nums=[3, 5, 5],
+ layer_strides=[2, 2, 2],
+ out_channels=[64, 128, 256]),
+ pts_neck=dict(
+ type='FPN',
+ norm_cfg=dict(type='naiveSyncBN2d', eps=1e-3, momentum=0.01),
+ act_cfg=dict(type='ReLU'),
+ in_channels=[64, 128, 256],
+ out_channels=256,
+ start_level=0,
+ num_outs=3),
+ pts_bbox_head=dict(
+ type='Anchor3DHead',
+ num_classes=10,
+ in_channels=256,
+ feat_channels=256,
+ use_direction_classifier=True,
+ anchor_generator=dict(
+ type='AlignedAnchor3DRangeGenerator',
+ ranges=[[-50, -50, -1.8, 50, 50, -1.8]],
+ scales=[1, 2, 4],
+ sizes=[
+ [2.5981, 0.8660, 1.], # 1.5 / sqrt(3)
+ [1.7321, 0.5774, 1.], # 1 / sqrt(3)
+ [1., 1., 1.],
+ [0.4, 0.4, 1],
+ ],
+ custom_values=[0, 0],
+ rotations=[0, 1.57],
+ reshape_out=True),
+ assigner_per_size=False,
+ diff_rad_by_sin=True,
+ dir_offset=-0.7854, # -pi / 4
+ bbox_coder=dict(type='DeltaXYZWLHRBBoxCoder', code_size=9),
+ loss_cls=dict(
+ type='FocalLoss',
+ use_sigmoid=True,
+ gamma=2.0,
+ alpha=0.25,
+ loss_weight=1.0),
+ loss_bbox=dict(type='SmoothL1Loss', beta=1.0 / 9.0, loss_weight=1.0),
+ loss_dir=dict(
+ type='CrossEntropyLoss', use_sigmoid=False, loss_weight=0.2)),
+ # model training and testing settings
+ train_cfg=dict(
+ pts=dict(
+ assigner=dict(
+ type='MaxIoUAssigner',
+ iou_calculator=dict(type='BboxOverlapsNearest3D'),
+ pos_iou_thr=0.6,
+ neg_iou_thr=0.3,
+ min_pos_iou=0.3,
+ ignore_iof_thr=-1),
+ allowed_border=0,
+ code_weight=[1.0, 1.0, 1.0, 1.0, 1.0, 1.0, 1.0, 0.2, 0.2],
+ pos_weight=-1,
+ debug=False)),
+ test_cfg=dict(
+ pts=dict(
+ use_rotate_nms=True,
+ nms_across_levels=False,
+ nms_pre=1000,
+ nms_thr=0.2,
+ score_thr=0.05,
+ min_bbox_size=0,
+ max_num=500)))
diff --git a/configs/_base_/models/hv_pointpillars_fpn_range100_lyft.py b/configs/_base_/models/hv_pointpillars_fpn_range100_lyft.py
new file mode 100644
index 0000000..9cd200f
--- /dev/null
+++ b/configs/_base_/models/hv_pointpillars_fpn_range100_lyft.py
@@ -0,0 +1,22 @@
+_base_ = './hv_pointpillars_fpn_nus.py'
+
+# model settings (based on nuScenes model settings)
+# Voxel size for voxel encoder
+# Usually voxel size is changed consistently with the point cloud range
+# If point cloud range is modified, do remember to change all related
+# keys in the config.
+model = dict(
+ pts_voxel_layer=dict(
+ max_num_points=20,
+ point_cloud_range=[-100, -100, -5, 100, 100, 3],
+ max_voxels=(60000, 60000)),
+ pts_voxel_encoder=dict(
+ feat_channels=[64], point_cloud_range=[-100, -100, -5, 100, 100, 3]),
+ pts_middle_encoder=dict(output_shape=[800, 800]),
+ pts_bbox_head=dict(
+ num_classes=9,
+ anchor_generator=dict(
+ ranges=[[-100, -100, -1.8, 100, 100, -1.8]], custom_values=[]),
+ bbox_coder=dict(type='DeltaXYZWLHRBBoxCoder', code_size=7)),
+ # model training settings (based on nuScenes model settings)
+ train_cfg=dict(pts=dict(code_weight=[1.0, 1.0, 1.0, 1.0, 1.0, 1.0, 1.0])))
diff --git a/configs/_base_/models/hv_pointpillars_secfpn_kitti.py b/configs/_base_/models/hv_pointpillars_secfpn_kitti.py
new file mode 100644
index 0000000..ac46475
--- /dev/null
+++ b/configs/_base_/models/hv_pointpillars_secfpn_kitti.py
@@ -0,0 +1,94 @@
+voxel_size = [0.16, 0.16, 4]
+
+model = dict(
+ type='VoxelNet',
+ voxel_layer=dict(
+ max_num_points=32, # max_points_per_voxel
+ point_cloud_range=[0, -39.68, -3, 69.12, 39.68, 1],
+ voxel_size=voxel_size,
+ max_voxels=(16000, 40000) # (training, testing) max_voxels
+ ),
+ voxel_encoder=dict(
+ type='PillarFeatureNet',
+ in_channels=4,
+ feat_channels=[64],
+ with_distance=False,
+ voxel_size=voxel_size,
+ point_cloud_range=[0, -39.68, -3, 69.12, 39.68, 1]),
+ middle_encoder=dict(
+ type='PointPillarsScatter', in_channels=64, output_shape=[496, 432]),
+ backbone=dict(
+ type='SECOND',
+ in_channels=64,
+ layer_nums=[3, 5, 5],
+ layer_strides=[2, 2, 2],
+ out_channels=[64, 128, 256]),
+ neck=dict(
+ type='SECONDFPN',
+ in_channels=[64, 128, 256],
+ upsample_strides=[1, 2, 4],
+ out_channels=[128, 128, 128]),
+ bbox_head=dict(
+ type='Anchor3DHead',
+ num_classes=3,
+ in_channels=384,
+ feat_channels=384,
+ use_direction_classifier=True,
+ assign_per_class=True,
+ anchor_generator=dict(
+ type='AlignedAnchor3DRangeGenerator',
+ ranges=[
+ [0, -39.68, -0.6, 69.12, 39.68, -0.6],
+ [0, -39.68, -0.6, 69.12, 39.68, -0.6],
+ [0, -39.68, -1.78, 69.12, 39.68, -1.78],
+ ],
+ sizes=[[0.8, 0.6, 1.73], [1.76, 0.6, 1.73], [3.9, 1.6, 1.56]],
+ rotations=[0, 1.57],
+ reshape_out=False),
+ diff_rad_by_sin=True,
+ bbox_coder=dict(type='DeltaXYZWLHRBBoxCoder'),
+ loss_cls=dict(
+ type='FocalLoss',
+ use_sigmoid=True,
+ gamma=2.0,
+ alpha=0.25,
+ loss_weight=1.0),
+ loss_bbox=dict(type='SmoothL1Loss', beta=1.0 / 9.0, loss_weight=2.0),
+ loss_dir=dict(
+ type='CrossEntropyLoss', use_sigmoid=False, loss_weight=0.2)),
+ # model training and testing settings
+ train_cfg=dict(
+ assigner=[
+ dict( # for Pedestrian
+ type='MaxIoUAssigner',
+ iou_calculator=dict(type='BboxOverlapsNearest3D'),
+ pos_iou_thr=0.5,
+ neg_iou_thr=0.35,
+ min_pos_iou=0.35,
+ ignore_iof_thr=-1),
+ dict( # for Cyclist
+ type='MaxIoUAssigner',
+ iou_calculator=dict(type='BboxOverlapsNearest3D'),
+ pos_iou_thr=0.5,
+ neg_iou_thr=0.35,
+ min_pos_iou=0.35,
+ ignore_iof_thr=-1),
+ dict( # for Car
+ type='MaxIoUAssigner',
+ iou_calculator=dict(type='BboxOverlapsNearest3D'),
+ pos_iou_thr=0.6,
+ neg_iou_thr=0.45,
+ min_pos_iou=0.45,
+ ignore_iof_thr=-1),
+ ],
+ allowed_border=0,
+ pos_weight=-1,
+ debug=False),
+ test_cfg=dict(
+ use_rotate_nms=True,
+ nms_across_levels=False,
+ nms_thr=0.01,
+ score_thr=0.1,
+ min_bbox_size=0,
+ nms_pre=100,
+ max_num=50))
diff --git a/configs/_base_/models/hv_pointpillars_secfpn_waymo.py b/configs/_base_/models/hv_pointpillars_secfpn_waymo.py
new file mode 100644
index 0000000..30e23e9
--- /dev/null
+++ b/configs/_base_/models/hv_pointpillars_secfpn_waymo.py
@@ -0,0 +1,107 @@
+# model settings
+# Voxel size for voxel encoder
+# Usually voxel size is changed consistently with the point cloud range
+# If point cloud range is modified, do remember to change all related
+# keys in the config.
+voxel_size = [0.32, 0.32, 6]
+model = dict(
+ type='MVXFasterRCNN',
+ pts_voxel_layer=dict(
+ max_num_points=20,
+ point_cloud_range=[-74.88, -74.88, -2, 74.88, 74.88, 4],
+ voxel_size=voxel_size,
+ max_voxels=(32000, 32000)),
+ pts_voxel_encoder=dict(
+ type='HardVFE',
+ in_channels=5,
+ feat_channels=[64],
+ with_distance=False,
+ voxel_size=voxel_size,
+ with_cluster_center=True,
+ with_voxel_center=True,
+ point_cloud_range=[-74.88, -74.88, -2, 74.88, 74.88, 4],
+ norm_cfg=dict(type='naiveSyncBN1d', eps=1e-3, momentum=0.01)),
+ pts_middle_encoder=dict(
+ type='PointPillarsScatter', in_channels=64, output_shape=[468, 468]),
+ pts_backbone=dict(
+ type='SECOND',
+ in_channels=64,
+ norm_cfg=dict(type='naiveSyncBN2d', eps=1e-3, momentum=0.01),
+ layer_nums=[3, 5, 5],
+ layer_strides=[1, 2, 2],
+ out_channels=[64, 128, 256]),
+ pts_neck=dict(
+ type='SECONDFPN',
+ norm_cfg=dict(type='naiveSyncBN2d', eps=1e-3, momentum=0.01),
+ in_channels=[64, 128, 256],
+ upsample_strides=[1, 2, 4],
+ out_channels=[128, 128, 128]),
+ pts_bbox_head=dict(
+ type='Anchor3DHead',
+ num_classes=3,
+ in_channels=384,
+ feat_channels=384,
+ use_direction_classifier=True,
+ anchor_generator=dict(
+ type='AlignedAnchor3DRangeGenerator',
+ ranges=[[-74.88, -74.88, -0.0345, 74.88, 74.88, -0.0345],
+ [-74.88, -74.88, -0.1188, 74.88, 74.88, -0.1188],
+ [-74.88, -74.88, 0, 74.88, 74.88, 0]],
+ sizes=[
+ [4.73, 2.08, 1.77], # car
+ [1.81, 0.84, 1.77], # cyclist
+ [0.91, 0.84, 1.74] # pedestrian
+ ],
+ rotations=[0, 1.57],
+ reshape_out=False),
+ diff_rad_by_sin=True,
+ dir_offset=-0.7854, # -pi / 4
+ bbox_coder=dict(type='DeltaXYZWLHRBBoxCoder', code_size=7),
+ loss_cls=dict(
+ type='FocalLoss',
+ use_sigmoid=True,
+ gamma=2.0,
+ alpha=0.25,
+ loss_weight=1.0),
+ loss_bbox=dict(type='SmoothL1Loss', beta=1.0 / 9.0, loss_weight=1.0),
+ loss_dir=dict(
+ type='CrossEntropyLoss', use_sigmoid=False, loss_weight=0.2)),
+ # model training and testing settings
+ train_cfg=dict(
+ pts=dict(
+ assigner=[
+ dict( # car
+ type='MaxIoUAssigner',
+ iou_calculator=dict(type='BboxOverlapsNearest3D'),
+ pos_iou_thr=0.55,
+ neg_iou_thr=0.4,
+ min_pos_iou=0.4,
+ ignore_iof_thr=-1),
+ dict( # cyclist
+ type='MaxIoUAssigner',
+ iou_calculator=dict(type='BboxOverlapsNearest3D'),
+ pos_iou_thr=0.5,
+ neg_iou_thr=0.3,
+ min_pos_iou=0.3,
+ ignore_iof_thr=-1),
+ dict( # pedestrian
+ type='MaxIoUAssigner',
+ iou_calculator=dict(type='BboxOverlapsNearest3D'),
+ pos_iou_thr=0.5,
+ neg_iou_thr=0.3,
+ min_pos_iou=0.3,
+ ignore_iof_thr=-1),
+ ],
+ allowed_border=0,
+ code_weight=[1.0, 1.0, 1.0, 1.0, 1.0, 1.0, 1.0],
+ pos_weight=-1,
+ debug=False)),
+ test_cfg=dict(
+ pts=dict(
+ use_rotate_nms=True,
+ nms_across_levels=False,
+ nms_pre=4096,
+ nms_thr=0.25,
+ score_thr=0.1,
+ min_bbox_size=0,
+ max_num=500)))
diff --git a/configs/_base_/models/hv_second_secfpn_kitti.py b/configs/_base_/models/hv_second_secfpn_kitti.py
new file mode 100644
index 0000000..e7d569a
--- /dev/null
+++ b/configs/_base_/models/hv_second_secfpn_kitti.py
@@ -0,0 +1,89 @@
+voxel_size = [0.05, 0.05, 0.1]
+
+model = dict(
+ type='VoxelNet',
+ voxel_layer=dict(
+ max_num_points=5,
+ point_cloud_range=[0, -40, -3, 70.4, 40, 1],
+ voxel_size=voxel_size,
+ max_voxels=(16000, 40000)),
+ voxel_encoder=dict(type='HardSimpleVFE'),
+ middle_encoder=dict(
+ type='SparseEncoder',
+ in_channels=4,
+ sparse_shape=[41, 1600, 1408],
+ order=('conv', 'norm', 'act')),
+ backbone=dict(
+ type='SECOND',
+ in_channels=256,
+ layer_nums=[5, 5],
+ layer_strides=[1, 2],
+ out_channels=[128, 256]),
+ neck=dict(
+ type='SECONDFPN',
+ in_channels=[128, 256],
+ upsample_strides=[1, 2],
+ out_channels=[256, 256]),
+ bbox_head=dict(
+ type='Anchor3DHead',
+ num_classes=3,
+ in_channels=512,
+ feat_channels=512,
+ use_direction_classifier=True,
+ anchor_generator=dict(
+ type='Anchor3DRangeGenerator',
+ ranges=[
+ [0, -40.0, -0.6, 70.4, 40.0, -0.6],
+ [0, -40.0, -0.6, 70.4, 40.0, -0.6],
+ [0, -40.0, -1.78, 70.4, 40.0, -1.78],
+ ],
+ sizes=[[0.8, 0.6, 1.73], [1.76, 0.6, 1.73], [3.9, 1.6, 1.56]],
+ rotations=[0, 1.57],
+ reshape_out=False),
+ diff_rad_by_sin=True,
+ bbox_coder=dict(type='DeltaXYZWLHRBBoxCoder'),
+ loss_cls=dict(
+ type='FocalLoss',
+ use_sigmoid=True,
+ gamma=2.0,
+ alpha=0.25,
+ loss_weight=1.0),
+ loss_bbox=dict(type='SmoothL1Loss', beta=1.0 / 9.0, loss_weight=2.0),
+ loss_dir=dict(
+ type='CrossEntropyLoss', use_sigmoid=False, loss_weight=0.2)),
+ # model training and testing settings
+ train_cfg=dict(
+ assigner=[
+ dict( # for Pedestrian
+ type='MaxIoUAssigner',
+ iou_calculator=dict(type='BboxOverlapsNearest3D'),
+ pos_iou_thr=0.35,
+ neg_iou_thr=0.2,
+ min_pos_iou=0.2,
+ ignore_iof_thr=-1),
+ dict( # for Cyclist
+ type='MaxIoUAssigner',
+ iou_calculator=dict(type='BboxOverlapsNearest3D'),
+ pos_iou_thr=0.35,
+ neg_iou_thr=0.2,
+ min_pos_iou=0.2,
+ ignore_iof_thr=-1),
+ dict( # for Car
+ type='MaxIoUAssigner',
+ iou_calculator=dict(type='BboxOverlapsNearest3D'),
+ pos_iou_thr=0.6,
+ neg_iou_thr=0.45,
+ min_pos_iou=0.45,
+ ignore_iof_thr=-1),
+ ],
+ allowed_border=0,
+ pos_weight=-1,
+ debug=False),
+ test_cfg=dict(
+ use_rotate_nms=True,
+ nms_across_levels=False,
+ nms_thr=0.01,
+ score_thr=0.1,
+ min_bbox_size=0,
+ nms_pre=100,
+ max_num=50))
diff --git a/configs/_base_/models/hv_second_secfpn_waymo.py b/configs/_base_/models/hv_second_secfpn_waymo.py
new file mode 100644
index 0000000..0fa39e1
--- /dev/null
+++ b/configs/_base_/models/hv_second_secfpn_waymo.py
@@ -0,0 +1,99 @@
+# model settings
+# Voxel size for voxel encoder
+# Usually voxel size is changed consistently with the point cloud range
+# If point cloud range is modified, do remember to change all related
+# keys in the config.
+voxel_size = [0.08, 0.08, 0.1]
+model = dict(
+ type='VoxelNet',
+ voxel_layer=dict(
+ max_num_points=10,
+ point_cloud_range=[-76.8, -51.2, -2, 76.8, 51.2, 4],
+ voxel_size=voxel_size,
+ max_voxels=(80000, 90000)),
+ voxel_encoder=dict(type='HardSimpleVFE', num_features=5),
+ middle_encoder=dict(
+ type='SparseEncoder',
+ in_channels=5,
+ sparse_shape=[61, 1280, 1920],
+ order=('conv', 'norm', 'act')),
+ backbone=dict(
+ type='SECOND',
+ in_channels=384,
+ norm_cfg=dict(type='naiveSyncBN2d', eps=1e-3, momentum=0.01),
+ layer_nums=[5, 5],
+ layer_strides=[1, 2],
+ out_channels=[128, 256]),
+ neck=dict(
+ type='SECONDFPN',
+ norm_cfg=dict(type='naiveSyncBN2d', eps=1e-3, momentum=0.01),
+ in_channels=[128, 256],
+ upsample_strides=[1, 2],
+ out_channels=[256, 256]),
+ bbox_head=dict(
+ type='Anchor3DHead',
+ num_classes=3,
+ in_channels=512,
+ feat_channels=512,
+ use_direction_classifier=True,
+ anchor_generator=dict(
+ type='AlignedAnchor3DRangeGenerator',
+ ranges=[[-76.8, -51.2, -0.0345, 76.8, 51.2, -0.0345],
+ [-76.8, -51.2, 0, 76.8, 51.2, 0],
+ [-76.8, -51.2, -0.1188, 76.8, 51.2, -0.1188]],
+ sizes=[
+ [4.73, 2.08, 1.77], # car
+ [0.91, 0.84, 1.74], # pedestrian
+ [1.81, 0.84, 1.77] # cyclist
+ ],
+ rotations=[0, 1.57],
+ reshape_out=False),
+ diff_rad_by_sin=True,
+ dir_offset=-0.7854, # -pi / 4
+ bbox_coder=dict(type='DeltaXYZWLHRBBoxCoder', code_size=7),
+ loss_cls=dict(
+ type='FocalLoss',
+ use_sigmoid=True,
+ gamma=2.0,
+ alpha=0.25,
+ loss_weight=1.0),
+ loss_bbox=dict(type='SmoothL1Loss', beta=1.0 / 9.0, loss_weight=1.0),
+ loss_dir=dict(
+ type='CrossEntropyLoss', use_sigmoid=False, loss_weight=0.2)),
+ # model training and testing settings
+ train_cfg=dict(
+ assigner=[
+ dict( # car
+ type='MaxIoUAssigner',
+ iou_calculator=dict(type='BboxOverlapsNearest3D'),
+ pos_iou_thr=0.55,
+ neg_iou_thr=0.4,
+ min_pos_iou=0.4,
+ ignore_iof_thr=-1),
+ dict( # pedestrian
+ type='MaxIoUAssigner',
+ iou_calculator=dict(type='BboxOverlapsNearest3D'),
+ pos_iou_thr=0.5,
+ neg_iou_thr=0.3,
+ min_pos_iou=0.3,
+ ignore_iof_thr=-1),
+ dict( # cyclist
+ type='MaxIoUAssigner',
+ iou_calculator=dict(type='BboxOverlapsNearest3D'),
+ pos_iou_thr=0.5,
+ neg_iou_thr=0.3,
+ min_pos_iou=0.3,
+ ignore_iof_thr=-1)
+ ],
+ allowed_border=0,
+ code_weight=[1.0, 1.0, 1.0, 1.0, 1.0, 1.0, 1.0],
+ pos_weight=-1,
+ debug=False),
+ test_cfg=dict(
+ use_rotate_nms=True,
+ nms_across_levels=False,
+ nms_pre=4096,
+ nms_thr=0.25,
+ score_thr=0.1,
+ min_bbox_size=0,
+ max_num=500))
diff --git a/configs/_base_/models/imvotenet_image.py b/configs/_base_/models/imvotenet_image.py
new file mode 100644
index 0000000..981f8bc
--- /dev/null
+++ b/configs/_base_/models/imvotenet_image.py
@@ -0,0 +1,108 @@
+model = dict(
+ type='ImVoteNet',
+ img_backbone=dict(
+ type='ResNet',
+ depth=50,
+ num_stages=4,
+ out_indices=(0, 1, 2, 3),
+ frozen_stages=1,
+ norm_cfg=dict(type='BN', requires_grad=False),
+ norm_eval=True,
+ style='caffe'),
+ img_neck=dict(
+ type='FPN',
+ in_channels=[256, 512, 1024, 2048],
+ out_channels=256,
+ num_outs=5),
+ img_rpn_head=dict(
+ type='RPNHead',
+ in_channels=256,
+ feat_channels=256,
+ anchor_generator=dict(
+ type='AnchorGenerator',
+ scales=[8],
+ ratios=[0.5, 1.0, 2.0],
+ strides=[4, 8, 16, 32, 64]),
+ bbox_coder=dict(
+ type='DeltaXYWHBBoxCoder',
+ target_means=[.0, .0, .0, .0],
+ target_stds=[1.0, 1.0, 1.0, 1.0]),
+ loss_cls=dict(
+ type='CrossEntropyLoss', use_sigmoid=True, loss_weight=1.0),
+ loss_bbox=dict(type='L1Loss', loss_weight=1.0)),
+ img_roi_head=dict(
+ type='StandardRoIHead',
+ bbox_roi_extractor=dict(
+ type='SingleRoIExtractor',
+ roi_layer=dict(type='RoIAlign', output_size=7, sampling_ratio=0),
+ out_channels=256,
+ featmap_strides=[4, 8, 16, 32]),
+ bbox_head=dict(
+ type='Shared2FCBBoxHead',
+ in_channels=256,
+ fc_out_channels=1024,
+ roi_feat_size=7,
+ num_classes=10,
+ bbox_coder=dict(
+ type='DeltaXYWHBBoxCoder',
+ target_means=[0., 0., 0., 0.],
+ target_stds=[0.1, 0.1, 0.2, 0.2]),
+ reg_class_agnostic=False,
+ loss_cls=dict(
+ type='CrossEntropyLoss', use_sigmoid=False, loss_weight=1.0),
+ loss_bbox=dict(type='L1Loss', loss_weight=1.0))),
+
+ # model training and testing settings
+ train_cfg=dict(
+ img_rpn=dict(
+ assigner=dict(
+ type='MaxIoUAssigner',
+ pos_iou_thr=0.7,
+ neg_iou_thr=0.3,
+ min_pos_iou=0.3,
+ match_low_quality=True,
+ ignore_iof_thr=-1),
+ sampler=dict(
+ type='RandomSampler',
+ num=256,
+ pos_fraction=0.5,
+ neg_pos_ub=-1,
+ add_gt_as_proposals=False),
+ allowed_border=-1,
+ pos_weight=-1,
+ debug=False),
+ img_rpn_proposal=dict(
+ nms_across_levels=False,
+ nms_pre=2000,
+ nms_post=1000,
+ max_per_img=1000,
+ nms=dict(type='nms', iou_threshold=0.7),
+ min_bbox_size=0),
+ img_rcnn=dict(
+ assigner=dict(
+ type='MaxIoUAssigner',
+ pos_iou_thr=0.5,
+ neg_iou_thr=0.5,
+ min_pos_iou=0.5,
+ match_low_quality=False,
+ ignore_iof_thr=-1),
+ sampler=dict(
+ type='RandomSampler',
+ num=512,
+ pos_fraction=0.25,
+ neg_pos_ub=-1,
+ add_gt_as_proposals=True),
+ pos_weight=-1,
+ debug=False)),
+ test_cfg=dict(
+ img_rpn=dict(
+ nms_across_levels=False,
+ nms_pre=1000,
+ nms_post=1000,
+ max_per_img=1000,
+ nms=dict(type='nms', iou_threshold=0.7),
+ min_bbox_size=0),
+ img_rcnn=dict(
+ score_thr=0.05,
+ nms=dict(type='nms', iou_threshold=0.5),
+ max_per_img=100)))
diff --git a/configs/_base_/models/mask_rcnn_r50_fpn.py b/configs/_base_/models/mask_rcnn_r50_fpn.py
new file mode 100644
index 0000000..4e670e9
--- /dev/null
+++ b/configs/_base_/models/mask_rcnn_r50_fpn.py
@@ -0,0 +1,124 @@
+# model settings
+model = dict(
+ type='MaskRCNN',
+ pretrained='torchvision://resnet50',
+ backbone=dict(
+ type='ResNet',
+ depth=50,
+ num_stages=4,
+ out_indices=(0, 1, 2, 3),
+ frozen_stages=1,
+ norm_cfg=dict(type='BN', requires_grad=True),
+ norm_eval=True,
+ style='pytorch'),
+ neck=dict(
+ type='FPN',
+ in_channels=[256, 512, 1024, 2048],
+ out_channels=256,
+ num_outs=5),
+ rpn_head=dict(
+ type='RPNHead',
+ in_channels=256,
+ feat_channels=256,
+ anchor_generator=dict(
+ type='AnchorGenerator',
+ scales=[8],
+ ratios=[0.5, 1.0, 2.0],
+ strides=[4, 8, 16, 32, 64]),
+ bbox_coder=dict(
+ type='DeltaXYWHBBoxCoder',
+ target_means=[.0, .0, .0, .0],
+ target_stds=[1.0, 1.0, 1.0, 1.0]),
+ loss_cls=dict(
+ type='CrossEntropyLoss', use_sigmoid=True, loss_weight=1.0),
+ loss_bbox=dict(type='L1Loss', loss_weight=1.0)),
+ roi_head=dict(
+ type='StandardRoIHead',
+ bbox_roi_extractor=dict(
+ type='SingleRoIExtractor',
+ roi_layer=dict(type='RoIAlign', output_size=7, sampling_ratio=0),
+ out_channels=256,
+ featmap_strides=[4, 8, 16, 32]),
+ bbox_head=dict(
+ type='Shared2FCBBoxHead',
+ in_channels=256,
+ fc_out_channels=1024,
+ roi_feat_size=7,
+ num_classes=80,
+ bbox_coder=dict(
+ type='DeltaXYWHBBoxCoder',
+ target_means=[0., 0., 0., 0.],
+ target_stds=[0.1, 0.1, 0.2, 0.2]),
+ reg_class_agnostic=False,
+ loss_cls=dict(
+ type='CrossEntropyLoss', use_sigmoid=False, loss_weight=1.0),
+ loss_bbox=dict(type='L1Loss', loss_weight=1.0)),
+ mask_roi_extractor=dict(
+ type='SingleRoIExtractor',
+ roi_layer=dict(type='RoIAlign', output_size=14, sampling_ratio=0),
+ out_channels=256,
+ featmap_strides=[4, 8, 16, 32]),
+ mask_head=dict(
+ type='FCNMaskHead',
+ num_convs=4,
+ in_channels=256,
+ conv_out_channels=256,
+ num_classes=80,
+ loss_mask=dict(
+ type='CrossEntropyLoss', use_mask=True, loss_weight=1.0))),
+ # model training and testing settings
+ train_cfg=dict(
+ rpn=dict(
+ assigner=dict(
+ type='MaxIoUAssigner',
+ pos_iou_thr=0.7,
+ neg_iou_thr=0.3,
+ min_pos_iou=0.3,
+ match_low_quality=True,
+ ignore_iof_thr=-1),
+ sampler=dict(
+ type='RandomSampler',
+ num=256,
+ pos_fraction=0.5,
+ neg_pos_ub=-1,
+ add_gt_as_proposals=False),
+ allowed_border=-1,
+ pos_weight=-1,
+ debug=False),
+ rpn_proposal=dict(
+ nms_across_levels=False,
+ nms_pre=2000,
+ nms_post=1000,
+ max_per_img=1000,
+ nms=dict(type='nms', iou_threshold=0.7),
+ min_bbox_size=0),
+ rcnn=dict(
+ assigner=dict(
+ type='MaxIoUAssigner',
+ pos_iou_thr=0.5,
+ neg_iou_thr=0.5,
+ min_pos_iou=0.5,
+ match_low_quality=True,
+ ignore_iof_thr=-1),
+ sampler=dict(
+ type='RandomSampler',
+ num=512,
+ pos_fraction=0.25,
+ neg_pos_ub=-1,
+ add_gt_as_proposals=True),
+ mask_size=28,
+ pos_weight=-1,
+ debug=False)),
+ test_cfg=dict(
+ rpn=dict(
+ nms_across_levels=False,
+ nms_pre=1000,
+ nms_post=1000,
+ max_per_img=1000,
+ nms=dict(type='nms', iou_threshold=0.7),
+ min_bbox_size=0),
+ rcnn=dict(
+ score_thr=0.05,
+ nms=dict(type='nms', iou_threshold=0.5),
+ max_per_img=100,
+ mask_thr_binary=0.5)))
diff --git a/configs/_base_/models/paconv_cuda_ssg.py b/configs/_base_/models/paconv_cuda_ssg.py
new file mode 100644
index 0000000..f513bd4
--- /dev/null
+++ b/configs/_base_/models/paconv_cuda_ssg.py
@@ -0,0 +1,7 @@
+_base_ = './paconv_ssg.py'
+
+model = dict(
+ backbone=dict(
+ sa_cfg=dict(
+ type='PAConvCUDASAModule',
+ scorenet_cfg=dict(mlp_channels=[8, 16, 16]))))
diff --git a/configs/_base_/models/paconv_ssg.py b/configs/_base_/models/paconv_ssg.py
new file mode 100644
index 0000000..1d4f1ed
--- /dev/null
+++ b/configs/_base_/models/paconv_ssg.py
@@ -0,0 +1,49 @@
+# model settings
+model = dict(
+ type='EncoderDecoder3D',
+ backbone=dict(
+ type='PointNet2SASSG',
+ in_channels=9, # [xyz, rgb, normalized_xyz]
+ num_points=(1024, 256, 64, 16),
+ radius=(None, None, None, None), # use kNN instead of ball query
+ num_samples=(32, 32, 32, 32),
+ sa_channels=((32, 32, 64), (64, 64, 128), (128, 128, 256), (256, 256,
+ 512)),
+ fp_channels=(),
+ norm_cfg=dict(type='BN2d', momentum=0.1),
+ sa_cfg=dict(
+ type='PAConvSAModule',
+ pool_mod='max',
+ use_xyz=True,
+ normalize_xyz=False,
+ paconv_num_kernels=[16, 16, 16],
+ paconv_kernel_input='w_neighbor',
+ scorenet_input='w_neighbor_dist',
+ scorenet_cfg=dict(
+ mlp_channels=[16, 16, 16],
+ score_norm='softmax',
+ temp_factor=1.0,
+ last_bn=False))),
+ decode_head=dict(
+ type='PAConvHead',
+ # PAConv model's decoder takes skip connections from beckbone
+ # different from PointNet++, it also concats input features in the last
+ # level of decoder, leading to `128 + 6` as the channel number
+ fp_channels=((768, 256, 256), (384, 256, 256), (320, 256, 128),
+ (128 + 6, 128, 128, 128)),
+ channels=128,
+ dropout_ratio=0.5,
+ conv_cfg=dict(type='Conv1d'),
+ norm_cfg=dict(type='BN1d'),
+ act_cfg=dict(type='ReLU'),
+ loss_decode=dict(
+ type='CrossEntropyLoss',
+ use_sigmoid=False,
+ class_weight=None, # should be modified with dataset
+ loss_weight=1.0)),
+ # correlation loss to regularize PAConv's kernel weights
+ loss_regularization=dict(
+ type='PAConvRegularizationLoss', reduction='sum', loss_weight=10.0),
+ # model training and testing settings
+ train_cfg=dict(),
+ test_cfg=dict(mode='slide'))
diff --git a/configs/_base_/models/parta2.py b/configs/_base_/models/parta2.py
new file mode 100644
index 0000000..aa15567
--- /dev/null
+++ b/configs/_base_/models/parta2.py
@@ -0,0 +1,201 @@
+# model settings
+voxel_size = [0.05, 0.05, 0.1]
+point_cloud_range = [0, -40, -3, 70.4, 40, 1]
+
+model = dict(
+ type='PartA2',
+ voxel_layer=dict(
+ max_num_points=5, # max_points_per_voxel
+ point_cloud_range=point_cloud_range,
+ voxel_size=voxel_size,
+ max_voxels=(16000, 40000) # (training, testing) max_voxels
+ ),
+ voxel_encoder=dict(type='HardSimpleVFE'),
+ middle_encoder=dict(
+ type='SparseUNet',
+ in_channels=4,
+ sparse_shape=[41, 1600, 1408],
+ order=('conv', 'norm', 'act')),
+ backbone=dict(
+ type='SECOND',
+ in_channels=256,
+ layer_nums=[5, 5],
+ layer_strides=[1, 2],
+ out_channels=[128, 256]),
+ neck=dict(
+ type='SECONDFPN',
+ in_channels=[128, 256],
+ upsample_strides=[1, 2],
+ out_channels=[256, 256]),
+ rpn_head=dict(
+ type='PartA2RPNHead',
+ num_classes=3,
+ in_channels=512,
+ feat_channels=512,
+ use_direction_classifier=True,
+ anchor_generator=dict(
+ type='Anchor3DRangeGenerator',
+ ranges=[[0, -40.0, -0.6, 70.4, 40.0, -0.6],
+ [0, -40.0, -0.6, 70.4, 40.0, -0.6],
+ [0, -40.0, -1.78, 70.4, 40.0, -1.78]],
+ sizes=[[0.8, 0.6, 1.73], [1.76, 0.6, 1.73], [3.9, 1.6, 1.56]],
+ rotations=[0, 1.57],
+ reshape_out=False),
+ diff_rad_by_sin=True,
+ assigner_per_size=True,
+ assign_per_class=True,
+ bbox_coder=dict(type='DeltaXYZWLHRBBoxCoder'),
+ loss_cls=dict(
+ type='FocalLoss',
+ use_sigmoid=True,
+ gamma=2.0,
+ alpha=0.25,
+ loss_weight=1.0),
+ loss_bbox=dict(type='SmoothL1Loss', beta=1.0 / 9.0, loss_weight=2.0),
+ loss_dir=dict(
+ type='CrossEntropyLoss', use_sigmoid=False, loss_weight=0.2)),
+ roi_head=dict(
+ type='PartAggregationROIHead',
+ num_classes=3,
+ semantic_head=dict(
+ type='PointwiseSemanticHead',
+ in_channels=16,
+ extra_width=0.2,
+ seg_score_thr=0.3,
+ num_classes=3,
+ loss_seg=dict(
+ type='FocalLoss',
+ use_sigmoid=True,
+ reduction='sum',
+ gamma=2.0,
+ alpha=0.25,
+ loss_weight=1.0),
+ loss_part=dict(
+ type='CrossEntropyLoss', use_sigmoid=True, loss_weight=1.0)),
+ seg_roi_extractor=dict(
+ type='Single3DRoIAwareExtractor',
+ roi_layer=dict(
+ type='RoIAwarePool3d',
+ out_size=14,
+ max_pts_per_voxel=128,
+ mode='max')),
+ part_roi_extractor=dict(
+ type='Single3DRoIAwareExtractor',
+ roi_layer=dict(
+ type='RoIAwarePool3d',
+ out_size=14,
+ max_pts_per_voxel=128,
+ mode='avg')),
+ bbox_head=dict(
+ type='PartA2BboxHead',
+ num_classes=3,
+ seg_in_channels=16,
+ part_in_channels=4,
+ seg_conv_channels=[64, 64],
+ part_conv_channels=[64, 64],
+ merge_conv_channels=[128, 128],
+ down_conv_channels=[128, 256],
+ bbox_coder=dict(type='DeltaXYZWLHRBBoxCoder'),
+ shared_fc_channels=[256, 512, 512, 512],
+ cls_channels=[256, 256],
+ reg_channels=[256, 256],
+ dropout_ratio=0.1,
+ roi_feat_size=14,
+ with_corner_loss=True,
+ loss_bbox=dict(
+ type='SmoothL1Loss',
+ beta=1.0 / 9.0,
+ reduction='sum',
+ loss_weight=1.0),
+ loss_cls=dict(
+ type='CrossEntropyLoss',
+ use_sigmoid=True,
+ reduction='sum',
+ loss_weight=1.0))),
+ # model training and testing settings
+ train_cfg=dict(
+ rpn=dict(
+ assigner=[
+ dict( # for Pedestrian
+ type='MaxIoUAssigner',
+ iou_calculator=dict(type='BboxOverlapsNearest3D'),
+ pos_iou_thr=0.5,
+ neg_iou_thr=0.35,
+ min_pos_iou=0.35,
+ ignore_iof_thr=-1),
+ dict( # for Cyclist
+ type='MaxIoUAssigner',
+ iou_calculator=dict(type='BboxOverlapsNearest3D'),
+ pos_iou_thr=0.5,
+ neg_iou_thr=0.35,
+ min_pos_iou=0.35,
+ ignore_iof_thr=-1),
+ dict( # for Car
+ type='MaxIoUAssigner',
+ iou_calculator=dict(type='BboxOverlapsNearest3D'),
+ pos_iou_thr=0.6,
+ neg_iou_thr=0.45,
+ min_pos_iou=0.45,
+ ignore_iof_thr=-1)
+ ],
+ allowed_border=0,
+ pos_weight=-1,
+ debug=False),
+ rpn_proposal=dict(
+ nms_pre=9000,
+ nms_post=512,
+ max_num=512,
+ nms_thr=0.8,
+ score_thr=0,
+ use_rotate_nms=False),
+ rcnn=dict(
+ assigner=[
+ dict( # for Pedestrian
+ type='MaxIoUAssigner',
+ iou_calculator=dict(
+ type='BboxOverlaps3D', coordinate='lidar'),
+ pos_iou_thr=0.55,
+ neg_iou_thr=0.55,
+ min_pos_iou=0.55,
+ ignore_iof_thr=-1),
+ dict( # for Cyclist
+ type='MaxIoUAssigner',
+ iou_calculator=dict(
+ type='BboxOverlaps3D', coordinate='lidar'),
+ pos_iou_thr=0.55,
+ neg_iou_thr=0.55,
+ min_pos_iou=0.55,
+ ignore_iof_thr=-1),
+ dict( # for Car
+ type='MaxIoUAssigner',
+ iou_calculator=dict(
+ type='BboxOverlaps3D', coordinate='lidar'),
+ pos_iou_thr=0.55,
+ neg_iou_thr=0.55,
+ min_pos_iou=0.55,
+ ignore_iof_thr=-1)
+ ],
+ sampler=dict(
+ type='IoUNegPiecewiseSampler',
+ num=128,
+ pos_fraction=0.55,
+ neg_piece_fractions=[0.8, 0.2],
+ neg_iou_piece_thrs=[0.55, 0.1],
+ neg_pos_ub=-1,
+ add_gt_as_proposals=False,
+ return_iou=True),
+ cls_pos_thr=0.75,
+ cls_neg_thr=0.25)),
+ test_cfg=dict(
+ rpn=dict(
+ nms_pre=1024,
+ nms_post=100,
+ max_num=100,
+ nms_thr=0.7,
+ score_thr=0,
+ use_rotate_nms=True),
+ rcnn=dict(
+ use_rotate_nms=True,
+ use_raw_score=True,
+ nms_thr=0.01,
+ score_thr=0.1)))
diff --git a/configs/_base_/models/pgd.py b/configs/_base_/models/pgd.py
new file mode 100644
index 0000000..e63fc1f
--- /dev/null
+++ b/configs/_base_/models/pgd.py
@@ -0,0 +1,55 @@
+_base_ = './fcos3d.py'
+# model settings
+model = dict(
+ bbox_head=dict(
+ _delete_=True,
+ type='PGDHead',
+ num_classes=10,
+ in_channels=256,
+ stacked_convs=2,
+ feat_channels=256,
+ use_direction_classifier=True,
+ diff_rad_by_sin=True,
+ pred_attrs=True,
+ pred_velo=True,
+ pred_bbox2d=True,
+ pred_keypoints=False,
+ dir_offset=0.7854, # pi/4
+ strides=[8, 16, 32, 64, 128],
+ group_reg_dims=(2, 1, 3, 1, 2), # offset, depth, size, rot, velo
+ cls_branch=(256, ),
+ reg_branch=(
+ (256, ), # offset
+ (256, ), # depth
+ (256, ), # size
+ (256, ), # rot
+ () # velo
+ ),
+ dir_branch=(256, ),
+ attr_branch=(256, ),
+ loss_cls=dict(
+ type='FocalLoss',
+ use_sigmoid=True,
+ gamma=2.0,
+ alpha=0.25,
+ loss_weight=1.0),
+ loss_bbox=dict(type='SmoothL1Loss', beta=1.0 / 9.0, loss_weight=1.0),
+ loss_dir=dict(
+ type='CrossEntropyLoss', use_sigmoid=False, loss_weight=1.0),
+ loss_attr=dict(
+ type='CrossEntropyLoss', use_sigmoid=False, loss_weight=1.0),
+ loss_centerness=dict(
+ type='CrossEntropyLoss', use_sigmoid=True, loss_weight=1.0),
+ norm_on_bbox=True,
+ centerness_on_reg=True,
+ center_sampling=True,
+ conv_bias=True,
+ dcn_on_last_conv=True,
+ use_depth_classifier=True,
+ depth_branch=(256, ),
+ depth_range=(0, 50),
+ depth_unit=10,
+ division='uniform',
+ depth_bins=6,
+ bbox_coder=dict(type='PGDBBoxCoder', code_size=9)),
+ test_cfg=dict(nms_pre=1000, nms_thr=0.8, score_thr=0.01, max_per_img=200))
diff --git a/configs/_base_/models/point_rcnn.py b/configs/_base_/models/point_rcnn.py
new file mode 100644
index 0000000..02a1414
--- /dev/null
+++ b/configs/_base_/models/point_rcnn.py
@@ -0,0 +1,131 @@
+model = dict(
+ type='PointRCNN',
+ backbone=dict(
+ type='PointNet2SAMSG',
+ in_channels=4,
+ num_points=(4096, 1024, 256, 64),
+ radii=((0.1, 0.5), (0.5, 1.0), (1.0, 2.0), (2.0, 4.0)),
+ num_samples=((16, 32), (16, 32), (16, 32), (16, 32)),
+ sa_channels=(((16, 16, 32), (32, 32, 64)), ((64, 64, 128), (64, 96,
+ 128)),
+ ((128, 196, 256), (128, 196, 256)), ((256, 256, 512),
+ (256, 384, 512))),
+ fps_mods=(('D-FPS'), ('D-FPS'), ('D-FPS'), ('D-FPS')),
+ fps_sample_range_lists=((-1), (-1), (-1), (-1)),
+ aggregation_channels=(None, None, None, None),
+ dilated_group=(False, False, False, False),
+ out_indices=(0, 1, 2, 3),
+ norm_cfg=dict(type='BN2d', eps=1e-3, momentum=0.1),
+ sa_cfg=dict(
+ type='PointSAModuleMSG',
+ pool_mod='max',
+ use_xyz=True,
+ normalize_xyz=False)),
+ neck=dict(
+ type='PointNetFPNeck',
+ fp_channels=((1536, 512, 512), (768, 512, 512), (608, 256, 256),
+ (257, 128, 128))),
+ rpn_head=dict(
+ type='PointRPNHead',
+ num_classes=3,
+ enlarge_width=0.1,
+ pred_layer_cfg=dict(
+ in_channels=128,
+ cls_linear_channels=(256, 256),
+ reg_linear_channels=(256, 256)),
+ cls_loss=dict(
+ type='FocalLoss',
+ use_sigmoid=True,
+ reduction='sum',
+ gamma=2.0,
+ alpha=0.25,
+ loss_weight=1.0),
+ bbox_loss=dict(
+ type='SmoothL1Loss',
+ beta=1.0 / 9.0,
+ reduction='sum',
+ loss_weight=1.0),
+ bbox_coder=dict(
+ type='PointXYZWHLRBBoxCoder',
+ code_size=8,
+ # code_size: (center residual (3), size regression (3),
+ # torch.cos(yaw) (1), torch.sin(yaw) (1)
+ use_mean_size=True,
+ mean_size=[[3.9, 1.6, 1.56], [0.8, 0.6, 1.73], [1.76, 0.6,
+ 1.73]])),
+ roi_head=dict(
+ type='PointRCNNRoIHead',
+ point_roi_extractor=dict(
+ type='Single3DRoIPointExtractor',
+ roi_layer=dict(type='RoIPointPool3d', num_sampled_points=512)),
+ bbox_head=dict(
+ type='PointRCNNBboxHead',
+ num_classes=1,
+ pred_layer_cfg=dict(
+ in_channels=512,
+ cls_conv_channels=(256, 256),
+ reg_conv_channels=(256, 256),
+ bias=True),
+ in_channels=5,
+ # 5 = 3 (xyz) + scores + depth
+ mlp_channels=[128, 128],
+ num_points=(128, 32, -1),
+ radius=(0.2, 0.4, 100),
+ num_samples=(16, 16, 16),
+ sa_channels=((128, 128, 128), (128, 128, 256), (256, 256, 512)),
+ with_corner_loss=True),
+ depth_normalizer=70.0),
+ # model training and testing settings
+ train_cfg=dict(
+ pos_distance_thr=10.0,
+ rpn=dict(
+ nms_cfg=dict(
+ use_rotate_nms=True, iou_thr=0.8, nms_pre=9000, nms_post=512),
+ score_thr=None),
+ rcnn=dict(
+ assigner=[
+ dict( # for Car
+ type='MaxIoUAssigner',
+ iou_calculator=dict(
+ type='BboxOverlaps3D', coordinate='lidar'),
+ pos_iou_thr=0.55,
+ neg_iou_thr=0.55,
+ min_pos_iou=0.55,
+ ignore_iof_thr=-1,
+ match_low_quality=False),
+ dict( # for Pedestrian
+ type='MaxIoUAssigner',
+ iou_calculator=dict(
+ type='BboxOverlaps3D', coordinate='lidar'),
+ pos_iou_thr=0.55,
+ neg_iou_thr=0.55,
+ min_pos_iou=0.55,
+ ignore_iof_thr=-1,
+ match_low_quality=False),
+ dict( # for Cyclist
+ type='MaxIoUAssigner',
+ iou_calculator=dict(
+ type='BboxOverlaps3D', coordinate='lidar'),
+ pos_iou_thr=0.55,
+ neg_iou_thr=0.55,
+ min_pos_iou=0.55,
+ ignore_iof_thr=-1,
+ match_low_quality=False)
+ ],
+ sampler=dict(
+ type='IoUNegPiecewiseSampler',
+ num=128,
+ pos_fraction=0.5,
+ neg_piece_fractions=[0.8, 0.2],
+ neg_iou_piece_thrs=[0.55, 0.1],
+ neg_pos_ub=-1,
+ add_gt_as_proposals=False,
+ return_iou=True),
+ cls_pos_thr=0.7,
+ cls_neg_thr=0.25)),
+ test_cfg=dict(
+ rpn=dict(
+ nms_cfg=dict(
+ use_rotate_nms=True, iou_thr=0.85, nms_pre=9000, nms_post=512),
+ score_thr=None),
+ rcnn=dict(use_rotate_nms=True, nms_thr=0.1, score_thr=0.1)))
diff --git a/configs/_base_/models/pointnet2_msg.py b/configs/_base_/models/pointnet2_msg.py
new file mode 100644
index 0000000..222ab88
--- /dev/null
+++ b/configs/_base_/models/pointnet2_msg.py
@@ -0,0 +1,28 @@
+_base_ = './pointnet2_ssg.py'
+
+# model settings
+model = dict(
+ backbone=dict(
+ _delete_=True,
+ type='PointNet2SAMSG',
+ in_channels=6, # [xyz, rgb], should be modified with dataset
+ num_points=(1024, 256, 64, 16),
+ radii=((0.05, 0.1), (0.1, 0.2), (0.2, 0.4), (0.4, 0.8)),
+ num_samples=((16, 32), (16, 32), (16, 32), (16, 32)),
+ sa_channels=(((16, 16, 32), (32, 32, 64)), ((64, 64, 128), (64, 96,
+ 128)),
+ ((128, 196, 256), (128, 196, 256)), ((256, 256, 512),
+ (256, 384, 512))),
+ aggregation_channels=(None, None, None, None),
+ fps_mods=(('D-FPS'), ('D-FPS'), ('D-FPS'), ('D-FPS')),
+ fps_sample_range_lists=((-1), (-1), (-1), (-1)),
+ dilated_group=(False, False, False, False),
+ out_indices=(0, 1, 2, 3),
+ sa_cfg=dict(
+ type='PointSAModuleMSG',
+ pool_mod='max',
+ use_xyz=True,
+ normalize_xyz=False)),
+ decode_head=dict(
+ fp_channels=((1536, 256, 256), (512, 256, 256), (352, 256, 128),
+ (128, 128, 128, 128))))
diff --git a/configs/_base_/models/pointnet2_ssg.py b/configs/_base_/models/pointnet2_ssg.py
new file mode 100644
index 0000000..58b4c24
--- /dev/null
+++ b/configs/_base_/models/pointnet2_ssg.py
@@ -0,0 +1,35 @@
+# model settings
+model = dict(
+ type='EncoderDecoder3D',
+ backbone=dict(
+ type='PointNet2SASSG',
+ in_channels=6, # [xyz, rgb], should be modified with dataset
+ num_points=(1024, 256, 64, 16),
+ radius=(0.1, 0.2, 0.4, 0.8),
+ num_samples=(32, 32, 32, 32),
+ sa_channels=((32, 32, 64), (64, 64, 128), (128, 128, 256), (256, 256,
+ 512)),
+ fp_channels=(),
+ norm_cfg=dict(type='BN2d'),
+ sa_cfg=dict(
+ type='PointSAModule',
+ pool_mod='max',
+ use_xyz=True,
+ normalize_xyz=False)),
+ decode_head=dict(
+ type='PointNet2Head',
+ fp_channels=((768, 256, 256), (384, 256, 256), (320, 256, 128),
+ (128, 128, 128, 128)),
+ channels=128,
+ dropout_ratio=0.5,
+ conv_cfg=dict(type='Conv1d'),
+ norm_cfg=dict(type='BN1d'),
+ act_cfg=dict(type='ReLU'),
+ loss_decode=dict(
+ type='CrossEntropyLoss',
+ use_sigmoid=False,
+ class_weight=None, # should be modified with dataset
+ loss_weight=1.0)),
+ # model training and testing settings
+ train_cfg=dict(),
+ test_cfg=dict(mode='slide'))
diff --git a/configs/_base_/models/smoke.py b/configs/_base_/models/smoke.py
new file mode 100644
index 0000000..0a7452b
--- /dev/null
+++ b/configs/_base_/models/smoke.py
@@ -0,0 +1,53 @@
+model = dict(
+ type='SMOKEMono3D',
+ backbone=dict(
+ type='DLANet',
+ depth=34,
+ in_channels=3,
+ norm_cfg=dict(type='GN', num_groups=32),
+ init_cfg=dict(
+ type='Pretrained',
+ checkpoint='http://dl.yf.io/dla/models/imagenet/dla34-ba72cf86.pth'
+ )),
+ neck=dict(
+ type='DLANeck',
+ in_channels=[16, 32, 64, 128, 256, 512],
+ start_level=2,
+ end_level=5,
+ norm_cfg=dict(type='GN', num_groups=32)),
+ bbox_head=dict(
+ type='SMOKEMono3DHead',
+ num_classes=3,
+ in_channels=64,
+ dim_channel=[3, 4, 5],
+ ori_channel=[6, 7],
+ stacked_convs=0,
+ feat_channels=64,
+ use_direction_classifier=False,
+ diff_rad_by_sin=False,
+ pred_attrs=False,
+ pred_velo=False,
+ dir_offset=0,
+ strides=None,
+ group_reg_dims=(8, ),
+ cls_branch=(256, ),
+ reg_branch=((256, ), ),
+ num_attrs=0,
+ bbox_code_size=7,
+ dir_branch=(),
+ attr_branch=(),
+ bbox_coder=dict(
+ type='SMOKECoder',
+ base_depth=(28.01, 16.32),
+ base_dims=((0.88, 1.73, 0.67), (1.78, 1.70, 0.58), (3.88, 1.63,
+ 1.53)),
+ code_size=7),
+ loss_cls=dict(type='GaussianFocalLoss', loss_weight=1.0),
+ loss_bbox=dict(type='L1Loss', reduction='sum', loss_weight=1 / 300),
+ loss_dir=dict(
+ type='CrossEntropyLoss', use_sigmoid=False, loss_weight=1.0),
+ loss_attr=None,
+ conv_bias=True,
+ dcn_on_last_conv=False),
+ train_cfg=None,
+ test_cfg=dict(topK=100, local_maximum_kernel=3, max_per_img=100))
diff --git a/configs/_base_/models/votenet.py b/configs/_base_/models/votenet.py
new file mode 100644
index 0000000..129339d
--- /dev/null
+++ b/configs/_base_/models/votenet.py
@@ -0,0 +1,73 @@
+model = dict(
+ type='VoteNet',
+ backbone=dict(
+ type='PointNet2SASSG',
+ in_channels=4,
+ num_points=(2048, 1024, 512, 256),
+ radius=(0.2, 0.4, 0.8, 1.2),
+ num_samples=(64, 32, 16, 16),
+ sa_channels=((64, 64, 128), (128, 128, 256), (128, 128, 256),
+ (128, 128, 256)),
+ fp_channels=((256, 256), (256, 256)),
+ norm_cfg=dict(type='BN2d'),
+ sa_cfg=dict(
+ type='PointSAModule',
+ pool_mod='max',
+ use_xyz=True,
+ normalize_xyz=True)),
+ bbox_head=dict(
+ type='VoteHead',
+ vote_module_cfg=dict(
+ in_channels=256,
+ vote_per_seed=1,
+ gt_per_seed=3,
+ conv_channels=(256, 256),
+ conv_cfg=dict(type='Conv1d'),
+ norm_cfg=dict(type='BN1d'),
+ norm_feats=True,
+ vote_loss=dict(
+ type='ChamferDistance',
+ mode='l1',
+ reduction='none',
+ loss_dst_weight=10.0)),
+ vote_aggregation_cfg=dict(
+ type='PointSAModule',
+ num_point=256,
+ radius=0.3,
+ num_sample=16,
+ mlp_channels=[256, 128, 128, 128],
+ use_xyz=True,
+ normalize_xyz=True),
+ pred_layer_cfg=dict(
+ in_channels=128, shared_conv_channels=(128, 128), bias=True),
+ conv_cfg=dict(type='Conv1d'),
+ norm_cfg=dict(type='BN1d'),
+ objectness_loss=dict(
+ type='CrossEntropyLoss',
+ class_weight=[0.2, 0.8],
+ reduction='sum',
+ loss_weight=5.0),
+ center_loss=dict(
+ type='ChamferDistance',
+ mode='l2',
+ reduction='sum',
+ loss_src_weight=10.0,
+ loss_dst_weight=10.0),
+ dir_class_loss=dict(
+ type='CrossEntropyLoss', reduction='sum', loss_weight=1.0),
+ dir_res_loss=dict(
+ type='SmoothL1Loss', reduction='sum', loss_weight=10.0),
+ size_class_loss=dict(
+ type='CrossEntropyLoss', reduction='sum', loss_weight=1.0),
+ size_res_loss=dict(
+ type='SmoothL1Loss', reduction='sum', loss_weight=10.0 / 3.0),
+ semantic_loss=dict(
+ type='CrossEntropyLoss', reduction='sum', loss_weight=1.0)),
+ # model training and testing settings
+ train_cfg=dict(
+ pos_distance_thr=0.3, neg_distance_thr=0.6, sample_mod='vote'),
+ test_cfg=dict(
+ sample_mod='seed',
+ nms_thr=0.25,
+ score_thr=0.05,
+ per_class_proposal=True))
diff --git a/configs/_base_/schedules/cosine.py b/configs/_base_/schedules/cosine.py
new file mode 100644
index 0000000..69cb7df
--- /dev/null
+++ b/configs/_base_/schedules/cosine.py
@@ -0,0 +1,20 @@
+# This schedule is mainly used by models with dynamic voxelization
+# optimizer
+lr = 0.003 # max learning rate
+optimizer = dict(
+ type='AdamW',
+ lr=lr,
+ betas=(0.95, 0.99), # the momentum is change during training
+ weight_decay=0.001)
+optimizer_config = dict(grad_clip=dict(max_norm=10, norm_type=2))
+
+lr_config = dict(
+ policy='CosineAnnealing',
+ warmup='linear',
+ warmup_iters=1000,
+ warmup_ratio=1.0 / 10,
+ min_lr_ratio=1e-5)
+
+momentum_config = None
+
+runner = dict(type='EpochBasedRunner', max_epochs=40)
diff --git a/configs/_base_/schedules/cyclic_20e.py b/configs/_base_/schedules/cyclic_20e.py
new file mode 100644
index 0000000..704740e
--- /dev/null
+++ b/configs/_base_/schedules/cyclic_20e.py
@@ -0,0 +1,24 @@
+# For nuScenes dataset, we usually evaluate the model at the end of training.
+# Since the models are trained by 24 epochs by default, we set evaluation
+# interval to be 20. Please change the interval accordingly if you do not
+# use a default schedule.
+# optimizer
+# This schedule is mainly used by models on nuScenes dataset
+optimizer = dict(type='AdamW', lr=1e-4, weight_decay=0.01)
+# max_norm=10 is better for SECOND
+optimizer_config = dict(grad_clip=dict(max_norm=35, norm_type=2))
+lr_config = dict(
+ policy='cyclic',
+ target_ratio=(10, 1e-4),
+ cyclic_times=1,
+ step_ratio_up=0.4,
+)
+momentum_config = dict(
+ policy='cyclic',
+ target_ratio=(0.85 / 0.95, 1),
+ cyclic_times=1,
+ step_ratio_up=0.4,
+)
+
+# runtime settings
+runner = dict(type='EpochBasedRunner', max_epochs=20)
diff --git a/configs/_base_/schedules/cyclic_40e.py b/configs/_base_/schedules/cyclic_40e.py
new file mode 100644
index 0000000..6649863
--- /dev/null
+++ b/configs/_base_/schedules/cyclic_40e.py
@@ -0,0 +1,31 @@
+# The schedule is usually used by models trained on KITTI dataset
+
+# The learning rate set in the cyclic schedule is the initial learning rate
+# rather than the max learning rate. Since the target_ratio is (10, 1e-4),
+# the learning rate will change from 0.0018 to 0.018, than go to 0.0018*1e-4
+lr = 0.0018
+# The optimizer follows the setting in SECOND.Pytorch, but here we use
+# the official AdamW optimizer implemented by PyTorch.
+optimizer = dict(type='AdamW', lr=lr, betas=(0.95, 0.99), weight_decay=0.01)
+optimizer_config = dict(grad_clip=dict(max_norm=10, norm_type=2))
+# We use cyclic learning rate and momentum schedule following SECOND.Pytorch
+# https://github.com/traveller59/second.pytorch/blob/3aba19c9688274f75ebb5e576f65cfe54773c021/torchplus/train/learning_schedules_fastai.py#L69 # noqa
+# We implement them in mmcv, for more details, please refer to
+# https://github.com/open-mmlab/mmcv/blob/f48241a65aebfe07db122e9db320c31b685dc674/mmcv/runner/hooks/lr_updater.py#L327 # noqa
+# https://github.com/open-mmlab/mmcv/blob/f48241a65aebfe07db122e9db320c31b685dc674/mmcv/runner/hooks/momentum_updater.py#L130 # noqa
+lr_config = dict(
+ policy='cyclic',
+ target_ratio=(10, 1e-4),
+ cyclic_times=1,
+ step_ratio_up=0.4,
+)
+momentum_config = dict(
+ policy='cyclic',
+ target_ratio=(0.85 / 0.95, 1),
+ cyclic_times=1,
+ step_ratio_up=0.4,
+)
+# Although the max_epochs is 40, this schedule is usually used we
+# RepeatDataset with repeat ratio N, thus the actual max epoch
+# number could be Nx40
+runner = dict(type='EpochBasedRunner', max_epochs=40)
diff --git a/configs/_base_/schedules/mmdet_schedule_1x.py b/configs/_base_/schedules/mmdet_schedule_1x.py
new file mode 100644
index 0000000..13b3783
--- /dev/null
+++ b/configs/_base_/schedules/mmdet_schedule_1x.py
@@ -0,0 +1,11 @@
+# optimizer
+optimizer = dict(type='SGD', lr=0.02, momentum=0.9, weight_decay=0.0001)
+optimizer_config = dict(grad_clip=None)
+# learning policy
+lr_config = dict(
+ policy='step',
+ warmup='linear',
+ warmup_iters=500,
+ warmup_ratio=0.001,
+ step=[8, 11])
+runner = dict(type='EpochBasedRunner', max_epochs=12)
diff --git a/configs/_base_/schedules/schedule_2x.py b/configs/_base_/schedules/schedule_2x.py
new file mode 100644
index 0000000..afde799
--- /dev/null
+++ b/configs/_base_/schedules/schedule_2x.py
@@ -0,0 +1,14 @@
+# optimizer
+# This schedule is mainly used by models on nuScenes dataset
+optimizer = dict(type='AdamW', lr=0.001, weight_decay=0.01)
+# max_norm=10 is better for SECOND
+optimizer_config = dict(grad_clip=dict(max_norm=35, norm_type=2))
+lr_config = dict(
+ policy='step',
+ warmup='linear',
+ warmup_iters=1000,
+ warmup_ratio=1.0 / 1000,
+ step=[20, 23])
+momentum_config = None
+# runtime settings
+runner = dict(type='EpochBasedRunner', max_epochs=24)
diff --git a/configs/_base_/schedules/schedule_3x.py b/configs/_base_/schedules/schedule_3x.py
new file mode 100644
index 0000000..115cd26
--- /dev/null
+++ b/configs/_base_/schedules/schedule_3x.py
@@ -0,0 +1,9 @@
+# optimizer
+# This schedule is mainly used by models on indoor dataset,
+# e.g., VoteNet on SUNRGBD and ScanNet
+lr = 0.008 # max learning rate
+optimizer = dict(type='AdamW', lr=lr, weight_decay=0.01)
+optimizer_config = dict(grad_clip=dict(max_norm=10, norm_type=2))
+lr_config = dict(policy='step', warmup=None, step=[24, 32])
+# runtime settings
+runner = dict(type='EpochBasedRunner', max_epochs=36)
diff --git a/configs/_base_/schedules/seg_cosine_100e.py b/configs/_base_/schedules/seg_cosine_100e.py
new file mode 100644
index 0000000..3b75932
--- /dev/null
+++ b/configs/_base_/schedules/seg_cosine_100e.py
@@ -0,0 +1,8 @@
+# optimizer
+# This schedule is mainly used on S3DIS dataset in segmentation task
+optimizer = dict(type='SGD', lr=0.1, momentum=0.9, weight_decay=0.0001)
+optimizer_config = dict(grad_clip=None)
+lr_config = dict(policy='CosineAnnealing', warmup=None, min_lr=1e-5)
+
+# runtime settings
+runner = dict(type='EpochBasedRunner', max_epochs=100)
diff --git a/configs/_base_/schedules/seg_cosine_150e.py b/configs/_base_/schedules/seg_cosine_150e.py
new file mode 100644
index 0000000..04b44e5
--- /dev/null
+++ b/configs/_base_/schedules/seg_cosine_150e.py
@@ -0,0 +1,9 @@
+# optimizer
+# This schedule is mainly used on S3DIS dataset in segmentation task
+optimizer = dict(type='SGD', lr=0.2, weight_decay=0.0001, momentum=0.9)
+optimizer_config = dict(grad_clip=None)
+lr_config = dict(policy='CosineAnnealing', warmup=None, min_lr=0.002)
+momentum_config = None
+
+# runtime settings
+runner = dict(type='EpochBasedRunner', max_epochs=150)
diff --git a/configs/_base_/schedules/seg_cosine_200e.py b/configs/_base_/schedules/seg_cosine_200e.py
new file mode 100644
index 0000000..6a49484
--- /dev/null
+++ b/configs/_base_/schedules/seg_cosine_200e.py
@@ -0,0 +1,9 @@
+# optimizer
+# This schedule is mainly used on ScanNet dataset in segmentation task
+optimizer = dict(type='Adam', lr=0.001, weight_decay=0.01)
+optimizer_config = dict(grad_clip=None)
+lr_config = dict(policy='CosineAnnealing', warmup=None, min_lr=1e-5)
+momentum_config = None
+
+# runtime settings
+runner = dict(type='EpochBasedRunner', max_epochs=200)
diff --git a/configs/_base_/schedules/seg_cosine_50e.py b/configs/_base_/schedules/seg_cosine_50e.py
new file mode 100644
index 0000000..975a8f9
--- /dev/null
+++ b/configs/_base_/schedules/seg_cosine_50e.py
@@ -0,0 +1,9 @@
+# optimizer
+# This schedule is mainly used on S3DIS dataset in segmentation task
+optimizer = dict(type='Adam', lr=0.001, weight_decay=0.001)
+optimizer_config = dict(grad_clip=None)
+lr_config = dict(policy='CosineAnnealing', warmup=None, min_lr=1e-5)
+momentum_config = None
+
+# runtime settings
+runner = dict(type='EpochBasedRunner', max_epochs=50)
diff --git a/configs/benchmark/hv_PartA2_secfpn_4x8_cyclic_80e_pcdet_kitti-3d-3class.py b/configs/benchmark/hv_PartA2_secfpn_4x8_cyclic_80e_pcdet_kitti-3d-3class.py
new file mode 100644
index 0000000..398a19c
--- /dev/null
+++ b/configs/benchmark/hv_PartA2_secfpn_4x8_cyclic_80e_pcdet_kitti-3d-3class.py
@@ -0,0 +1,332 @@
+# model settings
+voxel_size = [0.05, 0.05, 0.1]
+point_cloud_range = [0, -40, -3, 70.4, 40, 1] # velodyne coordinates, x, y, z
+
+model = dict(
+ type='PartA2',
+ voxel_layer=dict(
+ max_num_points=5, # max_points_per_voxel
+ point_cloud_range=point_cloud_range,
+ voxel_size=voxel_size,
+ max_voxels=(16000, 40000) # (training, testing) max_coxels
+ ),
+ voxel_encoder=dict(type='HardSimpleVFE'),
+ middle_encoder=dict(
+ type='SparseUNet',
+ in_channels=4,
+ sparse_shape=[41, 1600, 1408],
+ order=('conv', 'norm', 'act')),
+ backbone=dict(
+ type='SECOND',
+ in_channels=256,
+ layer_nums=[5, 5],
+ layer_strides=[1, 2],
+ out_channels=[128, 256]),
+ neck=dict(
+ type='SECONDFPN',
+ in_channels=[128, 256],
+ upsample_strides=[1, 2],
+ out_channels=[256, 256]),
+ rpn_head=dict(
+ type='PartA2RPNHead',
+ num_classes=3,
+ in_channels=512,
+ feat_channels=512,
+ use_direction_classifier=True,
+ anchor_generator=dict(
+ type='Anchor3DRangeGenerator',
+ ranges=[[0, -40.0, -0.6, 70.4, 40.0, -0.6],
+ [0, -40.0, -0.6, 70.4, 40.0, -0.6],
+ [0, -40.0, -1.78, 70.4, 40.0, -1.78]],
+ sizes=[[0.8, 0.6, 1.73], [1.76, 0.6, 1.73], [3.9, 1.6, 1.56]],
+ rotations=[0, 1.57],
+ reshape_out=False),
+ diff_rad_by_sin=True,
+ assigner_per_size=True,
+ assign_per_class=True,
+ bbox_coder=dict(type='DeltaXYZWLHRBBoxCoder'),
+ loss_cls=dict(
+ type='FocalLoss',
+ use_sigmoid=True,
+ gamma=2.0,
+ alpha=0.25,
+ loss_weight=1.0),
+ loss_bbox=dict(type='SmoothL1Loss', beta=1.0 / 9.0, loss_weight=2.0),
+ loss_dir=dict(
+ type='CrossEntropyLoss', use_sigmoid=False, loss_weight=0.2)),
+ roi_head=dict(
+ type='PartAggregationROIHead',
+ num_classes=3,
+ semantic_head=dict(
+ type='PointwiseSemanticHead',
+ in_channels=16,
+ extra_width=0.2,
+ seg_score_thr=0.3,
+ num_classes=3,
+ loss_seg=dict(
+ type='FocalLoss',
+ use_sigmoid=True,
+ reduction='sum',
+ gamma=2.0,
+ alpha=0.25,
+ loss_weight=1.0),
+ loss_part=dict(
+ type='CrossEntropyLoss', use_sigmoid=True, loss_weight=1.0)),
+ seg_roi_extractor=dict(
+ type='Single3DRoIAwareExtractor',
+ roi_layer=dict(
+ type='RoIAwarePool3d',
+ out_size=14,
+ max_pts_per_voxel=128,
+ mode='max')),
+ part_roi_extractor=dict(
+ type='Single3DRoIAwareExtractor',
+ roi_layer=dict(
+ type='RoIAwarePool3d',
+ out_size=14,
+ max_pts_per_voxel=128,
+ mode='avg')),
+ bbox_head=dict(
+ type='PartA2BboxHead',
+ num_classes=3,
+ seg_in_channels=16,
+ part_in_channels=4,
+ seg_conv_channels=[64, 64],
+ part_conv_channels=[64, 64],
+ merge_conv_channels=[128, 128],
+ down_conv_channels=[128, 256],
+ bbox_coder=dict(type='DeltaXYZWLHRBBoxCoder'),
+ shared_fc_channels=[256, 512, 512, 512],
+ cls_channels=[256, 256],
+ reg_channels=[256, 256],
+ dropout_ratio=0.1,
+ roi_feat_size=14,
+ with_corner_loss=True,
+ loss_bbox=dict(
+ type='SmoothL1Loss',
+ beta=1.0 / 9.0,
+ reduction='sum',
+ loss_weight=1.0),
+ loss_cls=dict(
+ type='CrossEntropyLoss',
+ use_sigmoid=True,
+ reduction='sum',
+ loss_weight=1.0))),
+ # model training and testing settings
+ train_cfg=dict(
+ rpn=dict(
+ assigner=[
+ dict( # for Pedestrian
+ type='MaxIoUAssigner',
+ iou_calculator=dict(type='BboxOverlapsNearest3D'),
+ pos_iou_thr=0.5,
+ neg_iou_thr=0.35,
+ min_pos_iou=0.35,
+ ignore_iof_thr=-1),
+ dict( # for Cyclist
+ type='MaxIoUAssigner',
+ iou_calculator=dict(type='BboxOverlapsNearest3D'),
+ pos_iou_thr=0.5,
+ neg_iou_thr=0.35,
+ min_pos_iou=0.35,
+ ignore_iof_thr=-1),
+ dict( # for Car
+ type='MaxIoUAssigner',
+ iou_calculator=dict(type='BboxOverlapsNearest3D'),
+ pos_iou_thr=0.6,
+ neg_iou_thr=0.45,
+ min_pos_iou=0.45,
+ ignore_iof_thr=-1)
+ ],
+ allowed_border=0,
+ pos_weight=-1,
+ debug=False),
+ rpn_proposal=dict(
+ nms_pre=9000,
+ nms_post=512,
+ max_num=512,
+ nms_thr=0.8,
+ score_thr=0,
+ use_rotate_nms=False),
+ rcnn=dict(
+ assigner=[
+ dict( # for Pedestrian
+ type='MaxIoUAssigner',
+ iou_calculator=dict(
+ type='BboxOverlaps3D', coordinate='lidar'),
+ pos_iou_thr=0.55,
+ neg_iou_thr=0.55,
+ min_pos_iou=0.55,
+ ignore_iof_thr=-1),
+ dict( # for Cyclist
+ type='MaxIoUAssigner',
+ iou_calculator=dict(
+ type='BboxOverlaps3D', coordinate='lidar'),
+ pos_iou_thr=0.55,
+ neg_iou_thr=0.55,
+ min_pos_iou=0.55,
+ ignore_iof_thr=-1),
+ dict( # for Car
+ type='MaxIoUAssigner',
+ iou_calculator=dict(
+ type='BboxOverlaps3D', coordinate='lidar'),
+ pos_iou_thr=0.55,
+ neg_iou_thr=0.55,
+ min_pos_iou=0.55,
+ ignore_iof_thr=-1)
+ ],
+ sampler=dict(
+ type='IoUNegPiecewiseSampler',
+ num=128,
+ pos_fraction=0.55,
+ neg_piece_fractions=[0.8, 0.2],
+ neg_iou_piece_thrs=[0.55, 0.1],
+ neg_pos_ub=-1,
+ add_gt_as_proposals=False,
+ return_iou=True),
+ cls_pos_thr=0.75,
+ cls_neg_thr=0.25)),
+ test_cfg=dict(
+ rpn=dict(
+ nms_pre=1024,
+ nms_post=100,
+ max_num=100,
+ nms_thr=0.7,
+ score_thr=0,
+ use_rotate_nms=True),
+ rcnn=dict(
+ use_rotate_nms=True,
+ use_raw_score=True,
+ nms_thr=0.01,
+ score_thr=0.3)))
+
+# dataset settings
+dataset_type = 'KittiDataset'
+data_root = 'data/kitti/'
+class_names = ['Pedestrian', 'Cyclist', 'Car']
+input_modality = dict(use_lidar=True, use_camera=False)
+db_sampler = dict(
+ data_root=data_root,
+ info_path=data_root + 'kitti_dbinfos_train.pkl',
+ rate=1.0,
+ prepare=dict(
+ filter_by_difficulty=[-1],
+ filter_by_min_points=dict(Car=5, Pedestrian=5, Cyclist=5)),
+ classes=class_names,
+ sample_groups=dict(Car=20, Pedestrian=15, Cyclist=15))
+train_pipeline = [
+ dict(type='LoadPointsFromFile', coord_type='LIDAR', load_dim=4, use_dim=4),
+ dict(type='LoadAnnotations3D', with_bbox_3d=True, with_label_3d=True),
+ dict(type='ObjectSample', db_sampler=db_sampler),
+ dict(type='RandomFlip3D', flip_ratio_bev_horizontal=0.5),
+ dict(
+ type='GlobalRotScaleTrans',
+ rot_range=[-0.78539816, 0.78539816],
+ scale_ratio_range=[0.95, 1.05]),
+ dict(type='PointsRangeFilter', point_cloud_range=point_cloud_range),
+ dict(type='ObjectRangeFilter', point_cloud_range=point_cloud_range),
+ dict(type='ObjectNameFilter', classes=class_names),
+ dict(type='PointShuffle'),
+ dict(type='DefaultFormatBundle3D', class_names=class_names),
+ dict(type='Collect3D', keys=['points', 'gt_bboxes_3d', 'gt_labels_3d'])
+]
+test_pipeline = [
+ dict(type='LoadPointsFromFile', coord_type='LIDAR', load_dim=4, use_dim=4),
+ dict(
+ type='MultiScaleFlipAug3D',
+ img_scale=(1333, 800),
+ pts_scale_ratio=1,
+ flip=False,
+ transforms=[
+ dict(
+ type='GlobalRotScaleTrans',
+ rot_range=[0, 0],
+ scale_ratio_range=[1., 1.],
+ translation_std=[0, 0, 0]),
+ dict(type='RandomFlip3D'),
+ dict(
+ type='PointsRangeFilter', point_cloud_range=point_cloud_range),
+ dict(
+ type='DefaultFormatBundle3D',
+ class_names=class_names,
+ with_label=False),
+ dict(type='Collect3D', keys=['points'])
+ ])
+]
+# construct a pipeline for data and gt loading in show function
+# please keep its loading function consistent with test_pipeline (e.g. client)
+eval_pipeline = [
+ dict(type='LoadPointsFromFile', coord_type='LIDAR', load_dim=4, use_dim=4),
+ dict(
+ type='DefaultFormatBundle3D',
+ class_names=class_names,
+ with_label=False),
+ dict(type='Collect3D', keys=['points'])
+]
+
+data = dict(
+ samples_per_gpu=4,
+ workers_per_gpu=4,
+ train=dict(
+ type=dataset_type,
+ data_root=data_root,
+ ann_file=data_root + 'kitti_infos_train.pkl',
+ split='training',
+ pts_prefix='velodyne_reduced',
+ pipeline=train_pipeline,
+ modality=input_modality,
+ classes=class_names,
+ test_mode=False),
+ val=dict(
+ type=dataset_type,
+ data_root=data_root,
+ ann_file=data_root + 'kitti_infos_val.pkl',
+ split='training',
+ pts_prefix='velodyne_reduced',
+ pipeline=test_pipeline,
+ modality=input_modality,
+ classes=class_names,
+ test_mode=True),
+ test=dict(
+ type=dataset_type,
+ data_root=data_root,
+ ann_file=data_root + 'kitti_infos_val.pkl',
+ split='training',
+ pts_prefix='velodyne_reduced',
+ pipeline=test_pipeline,
+ modality=input_modality,
+ classes=class_names,
+ test_mode=True))
+# optimizer
+lr = 0.001 # max learning rate
+optimizer = dict(type='AdamW', lr=lr, betas=(0.95, 0.99), weight_decay=0.01)
+optimizer_config = dict(grad_clip=dict(max_norm=10, norm_type=2))
+lr_config = dict(
+ policy='cyclic',
+ target_ratio=(10, 1e-4),
+ cyclic_times=1,
+ step_ratio_up=0.4)
+momentum_config = dict(
+ policy='cyclic',
+ target_ratio=(0.85 / 0.95, 1),
+ cyclic_times=1,
+ step_ratio_up=0.4)
+checkpoint_config = dict(interval=1)
+evaluation = dict(interval=1, pipeline=eval_pipeline)
+# yapf:disable
+log_config = dict(
+ interval=50,
+ hooks=[
+ dict(type='TextLoggerHook'),
+ dict(type='TensorboardLoggerHook')
+ ])
+# yapf:enable
+# runtime settings
+runner = dict(type='EpochBasedRunner', max_epochs=80)
+dist_params = dict(backend='nccl', port=29506)
+log_level = 'INFO'
+find_unused_parameters = True
+work_dir = './work_dirs/parta2_secfpn_80e'
+load_from = None
+resume_from = None
+workflow = [('train', 1)]
diff --git a/configs/benchmark/hv_pointpillars_secfpn_3x8_100e_det3d_kitti-3d-car.py b/configs/benchmark/hv_pointpillars_secfpn_3x8_100e_det3d_kitti-3d-car.py
new file mode 100644
index 0000000..72c7372
--- /dev/null
+++ b/configs/benchmark/hv_pointpillars_secfpn_3x8_100e_det3d_kitti-3d-car.py
@@ -0,0 +1,201 @@
+# model settings
+voxel_size = [0.16, 0.16, 4]
+point_cloud_range = [0, -39.68, -3, 69.12, 39.68, 1]
+model = dict(
+ type='VoxelNet',
+ voxel_layer=dict(
+ max_num_points=64,
+ point_cloud_range=point_cloud_range,
+ voxel_size=voxel_size,
+ max_voxels=(12000, 20000)),
+ voxel_encoder=dict(
+ type='PillarFeatureNet',
+ in_channels=4,
+ feat_channels=[64],
+ with_distance=False,
+ voxel_size=voxel_size,
+ point_cloud_range=point_cloud_range),
+ middle_encoder=dict(
+ type='PointPillarsScatter', in_channels=64, output_shape=[496, 432]),
+ backbone=dict(
+ type='SECOND',
+ in_channels=64,
+ layer_nums=[3, 5, 5],
+ layer_strides=[2, 2, 2],
+ out_channels=[64, 128, 256]),
+ neck=dict(
+ type='SECONDFPN',
+ in_channels=[64, 128, 256],
+ upsample_strides=[1, 2, 4],
+ out_channels=[128, 128, 128]),
+ bbox_head=dict(
+ type='Anchor3DHead',
+ num_classes=1,
+ in_channels=384,
+ feat_channels=384,
+ use_direction_classifier=True,
+ anchor_generator=dict(
+ type='Anchor3DRangeGenerator',
+ ranges=[[0, -39.68, -1.78, 69.12, 39.68, -1.78]],
+ sizes=[[3.9, 1.6, 1.56]],
+ rotations=[0, 1.57],
+ reshape_out=True),
+ diff_rad_by_sin=True,
+ bbox_coder=dict(type='DeltaXYZWLHRBBoxCoder'),
+ loss_cls=dict(
+ type='FocalLoss',
+ use_sigmoid=True,
+ gamma=2.0,
+ alpha=0.25,
+ loss_weight=1.0),
+ loss_bbox=dict(type='SmoothL1Loss', beta=1.0 / 9.0, loss_weight=2.0),
+ loss_dir=dict(
+ type='CrossEntropyLoss', use_sigmoid=False, loss_weight=0.2)),
+ # model training and testing settings
+ train_cfg=dict(
+ assigner=dict(
+ type='MaxIoUAssigner',
+ iou_calculator=dict(type='BboxOverlapsNearest3D'),
+ pos_iou_thr=0.6,
+ neg_iou_thr=0.45,
+ min_pos_iou=0.45,
+ ignore_iof_thr=-1),
+ allowed_border=0,
+ pos_weight=-1,
+ debug=False),
+ test_cfg=dict(
+ use_rotate_nms=True,
+ nms_across_levels=False,
+ nms_thr=0.01,
+ score_thr=0.1,
+ min_bbox_size=0,
+ nms_pre=100,
+ max_num=50))
+
+# dataset settings
+dataset_type = 'KittiDataset'
+data_root = 'data/kitti/'
+class_names = ['Car']
+input_modality = dict(use_lidar=True, use_camera=False)
+db_sampler = dict(
+ data_root=data_root,
+ info_path=data_root + 'kitti_dbinfos_train.pkl',
+ rate=1.0,
+ prepare=dict(filter_by_difficulty=[-1], filter_by_min_points=dict(Car=5)),
+ sample_groups=dict(Car=15),
+ classes=class_names)
+
+train_pipeline = [
+ dict(type='LoadPointsFromFile', coord_type='LIDAR', load_dim=4, use_dim=4),
+ dict(type='LoadAnnotations3D', with_bbox_3d=True, with_label_3d=True),
+ dict(type='ObjectSample', db_sampler=db_sampler),
+ dict(
+ type='ObjectNoise',
+ num_try=100,
+ translation_std=[0.25, 0.25, 0.25],
+ global_rot_range=[0.0, 0.0],
+ rot_range=[-0.15707963267, 0.15707963267]),
+ dict(type='RandomFlip3D', flip_ratio_bev_horizontal=0.5),
+ dict(
+ type='GlobalRotScaleTrans',
+ rot_range=[-0.78539816, 0.78539816],
+ scale_ratio_range=[0.95, 1.05]),
+ dict(type='PointsRangeFilter', point_cloud_range=point_cloud_range),
+ dict(type='ObjectRangeFilter', point_cloud_range=point_cloud_range),
+ dict(type='PointShuffle'),
+ dict(type='DefaultFormatBundle3D', class_names=class_names),
+ dict(type='Collect3D', keys=['points', 'gt_bboxes_3d', 'gt_labels_3d'])
+]
+test_pipeline = [
+ dict(type='LoadPointsFromFile', coord_type='LIDAR', load_dim=4, use_dim=4),
+ dict(type='PointsRangeFilter', point_cloud_range=point_cloud_range),
+ dict(
+ type='DefaultFormatBundle3D',
+ class_names=class_names,
+ with_label=False),
+ dict(type='Collect3D', keys=['points'])
+]
+# construct a pipeline for data and gt loading in show function
+# please keep its loading function consistent with test_pipeline (e.g. client)
+eval_pipeline = [
+ dict(type='LoadPointsFromFile', coord_type='LIDAR', load_dim=4, use_dim=4),
+ dict(
+ type='DefaultFormatBundle3D',
+ class_names=class_names,
+ with_label=False),
+ dict(type='Collect3D', keys=['points'])
+]
+
+data = dict(
+ samples_per_gpu=3,
+ workers_per_gpu=3,
+ train=dict(
+ type='RepeatDataset',
+ times=2,
+ dataset=dict(
+ type=dataset_type,
+ data_root=data_root,
+ ann_file=data_root + 'kitti_infos_train.pkl',
+ split='training',
+ pts_prefix='velodyne_reduced',
+ pipeline=train_pipeline,
+ modality=input_modality,
+ classes=class_names,
+ test_mode=False)),
+ val=dict(
+ type=dataset_type,
+ data_root=data_root,
+ ann_file=data_root + 'kitti_infos_val.pkl',
+ split='training',
+ pts_prefix='velodyne_reduced',
+ pipeline=test_pipeline,
+ modality=input_modality,
+ classes=class_names,
+ test_mode=True),
+ test=dict(
+ type=dataset_type,
+ data_root=data_root,
+ ann_file=data_root + 'kitti_infos_val.pkl',
+ split='training',
+ pts_prefix='velodyne_reduced',
+ pipeline=test_pipeline,
+ modality=input_modality,
+ classes=class_names,
+ test_mode=True))
+# optimizer
+lr = 0.001 # max learning rate
+optimizer = dict(
+ type='AdamW',
+ lr=lr,
+ betas=(0.95, 0.99), # the momentum is change during training
+ weight_decay=0.01)
+optimizer_config = dict(grad_clip=dict(max_norm=35, norm_type=2))
+# learning policy
+lr_config = dict(
+ policy='cyclic',
+ target_ratio=(10, 1e-4),
+ cyclic_times=1,
+ step_ratio_up=0.4)
+momentum_config = dict(
+ policy='cyclic',
+ target_ratio=(0.85 / 0.95, 1),
+ cyclic_times=1,
+ step_ratio_up=0.4)
+checkpoint_config = dict(interval=1)
+evaluation = dict(interval=1, pipeline=eval_pipeline)
+# yapf:disable
+log_config = dict(
+ interval=50,
+ hooks=[
+ dict(type='TextLoggerHook'),
+ dict(type='TensorboardLoggerHook')
+ ])
+# yapf:enable
+# runtime settings
+runner = dict(type='EpochBasedRunner', max_epochs=50)
+dist_params = dict(backend='nccl')
+log_level = 'INFO'
+work_dir = './work_dirs/pp_secfpn_100e'
+load_from = None
+resume_from = None
+workflow = [('train', 50)]
diff --git a/configs/benchmark/hv_pointpillars_secfpn_4x8_80e_pcdet_kitti-3d-3class.py b/configs/benchmark/hv_pointpillars_secfpn_4x8_80e_pcdet_kitti-3d-3class.py
new file mode 100644
index 0000000..02eed9f
--- /dev/null
+++ b/configs/benchmark/hv_pointpillars_secfpn_4x8_80e_pcdet_kitti-3d-3class.py
@@ -0,0 +1,244 @@
+# model settings
+point_cloud_range = [0, -39.68, -3, 69.12, 39.68, 1]
+voxel_size = [0.16, 0.16, 4]
+model = dict(
+ type='VoxelNet',
+ voxel_layer=dict(
+ max_num_points=32, # max_points_per_voxel
+ point_cloud_range=point_cloud_range,
+ voxel_size=voxel_size,
+ max_voxels=(16000, 40000) # (training, testing) max_coxels
+ ),
+ voxel_encoder=dict(
+ type='PillarFeatureNet',
+ in_channels=4,
+ feat_channels=[64],
+ with_distance=False,
+ voxel_size=voxel_size,
+ point_cloud_range=point_cloud_range,
+ ),
+ middle_encoder=dict(
+ type='PointPillarsScatter',
+ in_channels=64,
+ output_shape=[496, 432],
+ ),
+ backbone=dict(
+ type='SECOND',
+ in_channels=64,
+ layer_nums=[3, 5, 5],
+ layer_strides=[2, 2, 2],
+ out_channels=[64, 128, 256],
+ ),
+ neck=dict(
+ type='SECONDFPN',
+ in_channels=[64, 128, 256],
+ upsample_strides=[1, 2, 4],
+ out_channels=[128, 128, 128],
+ ),
+ bbox_head=dict(
+ type='Anchor3DHead',
+ num_classes=3,
+ in_channels=384,
+ feat_channels=384,
+ use_direction_classifier=True,
+ anchor_generator=dict(
+ type='Anchor3DRangeGenerator',
+ ranges=[
+ [0, -40.0, -0.6, 70.4, 40.0, -0.6],
+ [0, -40.0, -0.6, 70.4, 40.0, -0.6],
+ [0, -40.0, -1.78, 70.4, 40.0, -1.78],
+ ],
+ sizes=[[0.8, 0.6, 1.73], [1.76, 0.6, 1.73], [3.9, 1.6, 1.56]],
+ rotations=[0, 1.57],
+ reshape_out=False),
+ diff_rad_by_sin=True,
+ bbox_coder=dict(type='DeltaXYZWLHRBBoxCoder'),
+ loss_cls=dict(
+ type='FocalLoss',
+ use_sigmoid=True,
+ gamma=2.0,
+ alpha=0.25,
+ loss_weight=1.0),
+ loss_bbox=dict(type='SmoothL1Loss', beta=1.0 / 9.0, loss_weight=2.0),
+ loss_dir=dict(
+ type='CrossEntropyLoss', use_sigmoid=False, loss_weight=0.2),
+ ),
+ # model training and testing settings
+ train_cfg=dict(
+ assigner=[
+ dict( # for Pedestrian
+ type='MaxIoUAssigner',
+ iou_calculator=dict(type='BboxOverlapsNearest3D'),
+ pos_iou_thr=0.5,
+ neg_iou_thr=0.35,
+ min_pos_iou=0.35,
+ ignore_iof_thr=-1),
+ dict( # for Cyclist
+ type='MaxIoUAssigner',
+ iou_calculator=dict(type='BboxOverlapsNearest3D'),
+ pos_iou_thr=0.5,
+ neg_iou_thr=0.35,
+ min_pos_iou=0.35,
+ ignore_iof_thr=-1),
+ dict( # for Car
+ type='MaxIoUAssigner',
+ iou_calculator=dict(type='BboxOverlapsNearest3D'),
+ pos_iou_thr=0.6,
+ neg_iou_thr=0.45,
+ min_pos_iou=0.45,
+ ignore_iof_thr=-1),
+ ],
+ allowed_border=0,
+ pos_weight=-1,
+ debug=False),
+ test_cfg=dict(
+ use_rotate_nms=True,
+ nms_across_levels=False,
+ nms_thr=0.01,
+ score_thr=0.1,
+ min_bbox_size=0,
+ nms_pre=100,
+ max_num=50))
+
+# dataset settings
+dataset_type = 'KittiDataset'
+data_root = 'data/kitti/'
+class_names = ['Pedestrian', 'Cyclist', 'Car']
+input_modality = dict(use_lidar=True, use_camera=False)
+db_sampler = dict(
+ data_root=data_root,
+ info_path=data_root + 'kitti_dbinfos_train.pkl',
+ rate=1.0,
+ prepare=dict(
+ filter_by_difficulty=[-1],
+ filter_by_min_points=dict(
+ Car=5,
+ Pedestrian=5,
+ Cyclist=5,
+ )),
+ classes=class_names,
+ sample_groups=dict(
+ Car=15,
+ Pedestrian=15,
+ Cyclist=15,
+ ))
+
+train_pipeline = [
+ dict(type='LoadPointsFromFile', coord_type='LIDAR', load_dim=4, use_dim=4),
+ dict(type='LoadAnnotations3D', with_bbox_3d=True, with_label_3d=True),
+ dict(type='ObjectSample', db_sampler=db_sampler),
+ dict(type='RandomFlip3D', flip_ratio_bev_horizontal=0.5),
+ dict(
+ type='GlobalRotScaleTrans',
+ rot_range=[-0.78539816, 0.78539816],
+ scale_ratio_range=[0.95, 1.05]),
+ dict(type='PointsRangeFilter', point_cloud_range=point_cloud_range),
+ dict(type='ObjectRangeFilter', point_cloud_range=point_cloud_range),
+ dict(type='PointShuffle'),
+ dict(type='DefaultFormatBundle3D', class_names=class_names),
+ dict(type='Collect3D', keys=['points', 'gt_bboxes_3d', 'gt_labels_3d']),
+]
+test_pipeline = [
+ dict(type='LoadPointsFromFile', coord_type='LIDAR', load_dim=4, use_dim=4),
+ dict(
+ type='MultiScaleFlipAug3D',
+ img_scale=(1333, 800),
+ pts_scale_ratio=1,
+ flip=False,
+ transforms=[
+ dict(
+ type='GlobalRotScaleTrans',
+ rot_range=[0, 0],
+ scale_ratio_range=[1., 1.],
+ translation_std=[0, 0, 0]),
+ dict(type='RandomFlip3D'),
+ dict(
+ type='PointsRangeFilter', point_cloud_range=point_cloud_range),
+ dict(
+ type='DefaultFormatBundle3D',
+ class_names=class_names,
+ with_label=False),
+ dict(type='Collect3D', keys=['points'])
+ ])
+]
+# construct a pipeline for data and gt loading in show function
+# please keep its loading function consistent with test_pipeline (e.g. client)
+eval_pipeline = [
+ dict(type='LoadPointsFromFile', coord_type='LIDAR', load_dim=4, use_dim=4),
+ dict(
+ type='DefaultFormatBundle3D',
+ class_names=class_names,
+ with_label=False),
+ dict(type='Collect3D', keys=['points'])
+]
+
+data = dict(
+ samples_per_gpu=4,
+ workers_per_gpu=4,
+ train=dict(
+ type=dataset_type,
+ data_root=data_root,
+ ann_file=data_root + 'kitti_infos_train.pkl',
+ split='training',
+ pts_prefix='velodyne_reduced',
+ pipeline=train_pipeline,
+ modality=input_modality,
+ classes=class_names,
+ test_mode=False),
+ val=dict(
+ type=dataset_type,
+ data_root=data_root,
+ ann_file=data_root + 'kitti_infos_val.pkl',
+ split='training',
+ pts_prefix='velodyne_reduced',
+ pipeline=test_pipeline,
+ modality=input_modality,
+ classes=class_names,
+ test_mode=True),
+ test=dict(
+ type=dataset_type,
+ data_root=data_root,
+ ann_file=data_root + 'kitti_infos_val.pkl',
+ split='training',
+ pts_prefix='velodyne_reduced',
+ pipeline=test_pipeline,
+ modality=input_modality,
+ classes=class_names,
+ test_mode=True))
+# optimizer
+lr = 0.0003 # max learning rate
+optimizer = dict(
+ type='AdamW',
+ lr=lr,
+ betas=(0.95, 0.99), # the momentum is change during training
+ weight_decay=0.01)
+optimizer_config = dict(grad_clip=dict(max_norm=10, norm_type=2))
+# learning policy
+lr_config = dict(
+ policy='cyclic',
+ target_ratio=(10, 1e-4),
+ cyclic_times=1,
+ step_ratio_up=0.4)
+momentum_config = dict(
+ policy='cyclic',
+ target_ratio=(0.85 / 0.95, 1),
+ cyclic_times=1,
+ step_ratio_up=0.4)
+checkpoint_config = dict(interval=1)
+evaluation = dict(interval=2, pipeline=eval_pipeline)
+# yapf:disable
+log_config = dict(
+ interval=50,
+ hooks=[
+ dict(type='TextLoggerHook'),
+ dict(type='TensorboardLoggerHook')
+ ])
+# yapf:enable
+# runtime settings
+runner = dict(type='EpochBasedRunner', max_epochs=80)
+dist_params = dict(backend='nccl')
+log_level = 'INFO'
+work_dir = './work_dirs/pp_secfpn_80e'
+load_from = None
+resume_from = None
+workflow = [('train', 1)]
diff --git a/configs/benchmark/hv_second_secfpn_4x8_80e_pcdet_kitti-3d-3class.py b/configs/benchmark/hv_second_secfpn_4x8_80e_pcdet_kitti-3d-3class.py
new file mode 100644
index 0000000..d61a050
--- /dev/null
+++ b/configs/benchmark/hv_second_secfpn_4x8_80e_pcdet_kitti-3d-3class.py
@@ -0,0 +1,251 @@
+# model settings
+voxel_size = [0.05, 0.05, 0.1]
+point_cloud_range = [0, -40, -3, 70.4, 40, 1]
+
+model = dict(
+ type='VoxelNet',
+ voxel_layer=dict(
+ max_num_points=5,
+ point_cloud_range=point_cloud_range,
+ voxel_size=voxel_size,
+ max_voxels=(16000, 40000)),
+ voxel_encoder=dict(type='HardSimpleVFE'),
+ middle_encoder=dict(
+ type='SparseEncoder',
+ in_channels=4,
+ sparse_shape=[41, 1600, 1408],
+ order=('conv', 'norm', 'act')),
+ backbone=dict(
+ type='SECOND',
+ in_channels=256,
+ layer_nums=[5, 5],
+ layer_strides=[1, 2],
+ out_channels=[128, 256]),
+ neck=dict(
+ type='SECONDFPN',
+ in_channels=[128, 256],
+ upsample_strides=[1, 2],
+ out_channels=[256, 256]),
+ bbox_head=dict(
+ type='Anchor3DHead',
+ num_classes=3,
+ in_channels=512,
+ feat_channels=512,
+ use_direction_classifier=True,
+ anchor_generator=dict(
+ type='Anchor3DRangeGenerator',
+ ranges=[
+ [0, -40.0, -0.6, 70.4, 40.0, -0.6],
+ [0, -40.0, -0.6, 70.4, 40.0, -0.6],
+ [0, -40.0, -1.78, 70.4, 40.0, -1.78],
+ ],
+ sizes=[[0.8, 0.6, 1.73], [1.76, 0.6, 1.73], [3.9, 1.6, 1.56]],
+ rotations=[0, 1.57],
+ reshape_out=False),
+ diff_rad_by_sin=True,
+ bbox_coder=dict(type='DeltaXYZWLHRBBoxCoder'),
+ loss_cls=dict(
+ type='FocalLoss',
+ use_sigmoid=True,
+ gamma=2.0,
+ alpha=0.25,
+ loss_weight=1.0),
+ loss_bbox=dict(type='SmoothL1Loss', beta=1.0 / 9.0, loss_weight=2.0),
+ loss_dir=dict(
+ type='CrossEntropyLoss', use_sigmoid=False, loss_weight=0.2)),
+ # model training and testing settings
+ train_cfg=dict(
+ assigner=[
+ dict( # for Pedestrian
+ type='MaxIoUAssigner',
+ iou_calculator=dict(type='BboxOverlapsNearest3D'),
+ pos_iou_thr=0.5,
+ neg_iou_thr=0.35,
+ min_pos_iou=0.35,
+ ignore_iof_thr=-1),
+ dict( # for Cyclist
+ type='MaxIoUAssigner',
+ iou_calculator=dict(type='BboxOverlapsNearest3D'),
+ pos_iou_thr=0.5,
+ neg_iou_thr=0.35,
+ min_pos_iou=0.35,
+ ignore_iof_thr=-1),
+ dict( # for Car
+ type='MaxIoUAssigner',
+ iou_calculator=dict(type='BboxOverlapsNearest3D'),
+ pos_iou_thr=0.6,
+ neg_iou_thr=0.45,
+ min_pos_iou=0.45,
+ ignore_iof_thr=-1),
+ ],
+ allowed_border=0,
+ pos_weight=-1,
+ debug=False),
+ test_cfg=dict(
+ use_rotate_nms=True,
+ nms_across_levels=False,
+ nms_thr=0.01,
+ score_thr=0.1,
+ min_bbox_size=0,
+ nms_pre=100,
+ max_num=50))
+
+# dataset settings
+dataset_type = 'KittiDataset'
+data_root = 'data/kitti/'
+class_names = ['Pedestrian', 'Cyclist', 'Car']
+input_modality = dict(use_lidar=False, use_camera=False)
+db_sampler = dict(
+ data_root=data_root,
+ info_path=data_root + 'kitti_dbinfos_train.pkl',
+ rate=1.0,
+ prepare=dict(
+ filter_by_difficulty=[-1],
+ filter_by_min_points=dict(
+ Car=5,
+ Pedestrian=5,
+ Cyclist=5,
+ )),
+ classes=class_names,
+ sample_groups=dict(
+ Car=20,
+ Pedestrian=15,
+ Cyclist=15,
+ ))
+file_client_args = dict(backend='disk')
+# file_client_args = dict(
+# backend='petrel', path_mapping=dict(data='s3://kitti_data/'))
+
+train_pipeline = [
+ dict(
+ type='LoadPointsFromFile',
+ coord_type='LIDAR',
+ load_dim=4,
+ use_dim=4,
+ file_client_args=file_client_args),
+ dict(
+ type='LoadAnnotations3D',
+ with_bbox_3d=True,
+ with_label_3d=True,
+ file_client_args=file_client_args),
+ dict(type='ObjectSample', db_sampler=db_sampler),
+ dict(type='RandomFlip3D', flip_ratio_bev_horizontal=0.5),
+ dict(
+ type='GlobalRotScaleTrans',
+ rot_range=[-0.78539816, 0.78539816],
+ scale_ratio_range=[0.95, 1.05]),
+ dict(type='PointsRangeFilter', point_cloud_range=point_cloud_range),
+ dict(type='ObjectRangeFilter', point_cloud_range=point_cloud_range),
+ dict(type='PointShuffle'),
+ dict(type='DefaultFormatBundle3D', class_names=class_names),
+ dict(type='Collect3D', keys=['points', 'gt_bboxes_3d', 'gt_labels_3d'])
+]
+test_pipeline = [
+ dict(
+ type='LoadPointsFromFile',
+ coord_type='LIDAR',
+ load_dim=4,
+ use_dim=4,
+ file_client_args=file_client_args),
+ dict(
+ type='MultiScaleFlipAug3D',
+ img_scale=(1333, 800),
+ pts_scale_ratio=1,
+ flip=False,
+ transforms=[
+ dict(
+ type='GlobalRotScaleTrans',
+ rot_range=[0, 0],
+ scale_ratio_range=[1., 1.],
+ translation_std=[0, 0, 0]),
+ dict(type='RandomFlip3D'),
+ dict(
+ type='PointsRangeFilter', point_cloud_range=point_cloud_range),
+ dict(
+ type='DefaultFormatBundle3D',
+ class_names=class_names,
+ with_label=False),
+ dict(type='Collect3D', keys=['points'])
+ ])
+]
+# construct a pipeline for data and gt loading in show function
+# please keep its loading function consistent with test_pipeline (e.g. client)
+eval_pipeline = [
+ dict(
+ type='LoadPointsFromFile',
+ coord_type='LIDAR',
+ load_dim=4,
+ use_dim=4,
+ file_client_args=file_client_args),
+ dict(
+ type='DefaultFormatBundle3D',
+ class_names=class_names,
+ with_label=False),
+ dict(type='Collect3D', keys=['points'])
+]
+
+data = dict(
+ samples_per_gpu=4,
+ workers_per_gpu=4,
+ train=dict(
+ type=dataset_type,
+ data_root=data_root,
+ ann_file=data_root + 'kitti_infos_train.pkl',
+ split='training',
+ pts_prefix='velodyne_reduced',
+ pipeline=train_pipeline,
+ modality=input_modality,
+ classes=class_names,
+ test_mode=False),
+ val=dict(
+ type=dataset_type,
+ data_root=data_root,
+ ann_file=data_root + 'kitti_infos_val.pkl',
+ split='training',
+ pts_prefix='velodyne_reduced',
+ pipeline=test_pipeline,
+ modality=input_modality,
+ classes=class_names,
+ test_mode=True),
+ test=dict(
+ type=dataset_type,
+ data_root=data_root,
+ ann_file=data_root + 'kitti_infos_val.pkl',
+ split='training',
+ pts_prefix='velodyne_reduced',
+ pipeline=test_pipeline,
+ modality=input_modality,
+ classes=class_names,
+ test_mode=True))
+# optimizer
+lr = 0.0003 # max learning rate
+optimizer = dict(type='AdamW', lr=lr, betas=(0.95, 0.99), weight_decay=0.01)
+optimizer_config = dict(grad_clip=dict(max_norm=10, norm_type=2))
+lr_config = dict(
+ policy='cyclic',
+ target_ratio=(10, 1e-4),
+ cyclic_times=1,
+ step_ratio_up=0.4)
+momentum_config = dict(
+ policy='cyclic',
+ target_ratio=(0.85 / 0.95, 1),
+ cyclic_times=1,
+ step_ratio_up=0.4)
+checkpoint_config = dict(interval=1)
+evaluation = dict(interval=2, pipeline=eval_pipeline)
+# yapf:disable
+log_config = dict(
+ interval=50,
+ hooks=[
+ dict(type='TextLoggerHook'),
+ dict(type='TensorboardLoggerHook')
+ ])
+# yapf:enable
+# runtime settings
+runner = dict(type='EpochBasedRunner', max_epochs=80)
+dist_params = dict(backend='nccl')
+log_level = 'INFO'
+work_dir = './work_dirs/sec_secfpn_80e'
+load_from = None
+resume_from = None
+workflow = [('train', 1)]
diff --git a/configs/centerpoint/README.md b/configs/centerpoint/README.md
new file mode 100644
index 0000000..d9173c9
--- /dev/null
+++ b/configs/centerpoint/README.md
@@ -0,0 +1,138 @@
+# Center-based 3D Object Detection and Tracking
+
+> [Center-based 3D Object Detection and Tracking](https://arxiv.org/abs/2006.11275)
+
+
+
+## Abstract
+
+Three-dimensional objects are commonly represented as 3D boxes in a point-cloud. This representation mimics the well-studied image-based 2D bounding-box detection but comes with additional challenges. Objects in a 3D world do not follow any particular orientation, and box-based detectors have difficulties enumerating all orientations or fitting an axis-aligned bounding box to rotated objects. In this paper, we instead propose to represent, detect, and track 3D objects as points. Our framework, CenterPoint, first detects centers of objects using a keypoint detector and regresses to other attributes, including 3D size, 3D orientation, and velocity. In a second stage, it refines these estimates using additional point features on the object. In CenterPoint, 3D object tracking simplifies to greedy closest-point matching. The resulting detection and tracking algorithm is simple, efficient, and effective. CenterPoint achieved state-of-the-art performance on the nuScenes benchmark for both 3D detection and tracking, with 65.5 NDS and 63.8 AMOTA for a single model. On the Waymo Open Dataset, CenterPoint outperforms all previous single model method by a large margin and ranks first among all Lidar-only submissions.
+
+
+
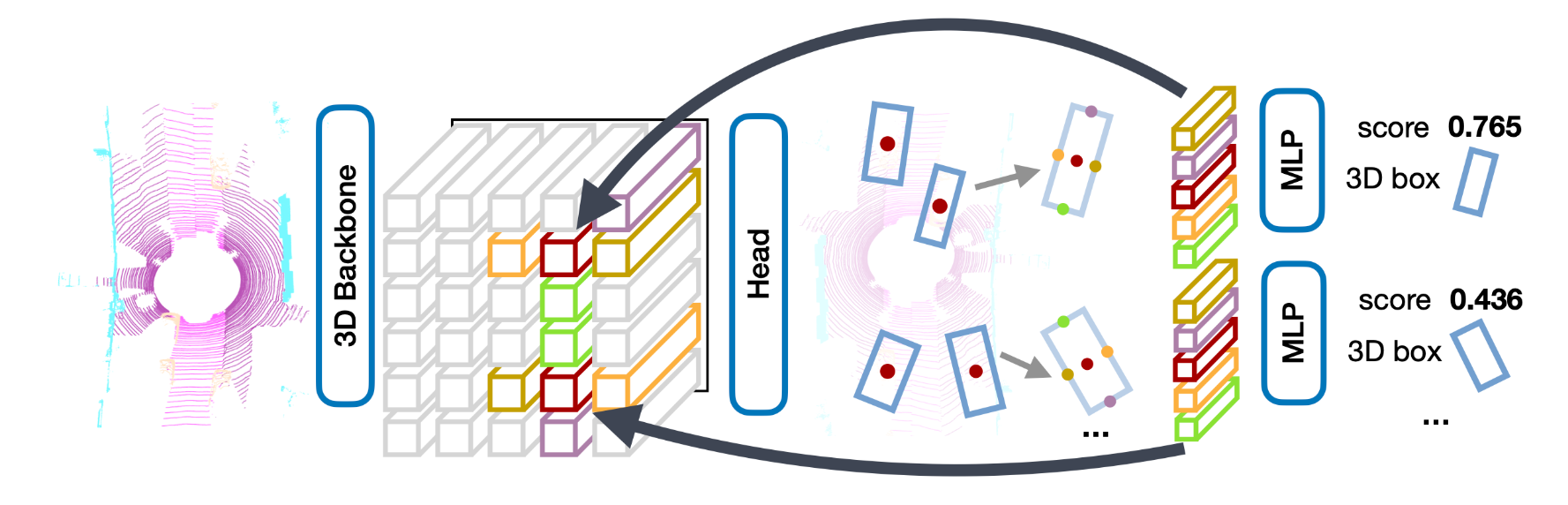
+
+
+## Introduction
+
+We implement CenterPoint and provide the result and checkpoints on nuScenes dataset.
+
+We follow the below style to name config files. Contributors are advised to follow the same style.
+`{xxx}` is required field and `[yyy]` is optional.
+
+`{model}`: model type like `centerpoint`.
+
+`{model setting}`: voxel size and voxel type like `01voxel`, `02pillar`.
+
+`{backbone}`: backbone type like `second`.
+
+`{neck}`: neck type like `secfpn`.
+
+`[dcn]`: Whether to use deformable convolution.
+
+`[circle]`: Whether to use circular nms.
+
+`[batch_per_gpu x gpu]`: GPUs and samples per GPU, 4x8 is used by default.
+
+`{schedule}`: training schedule, options are 1x, 2x, 20e, etc. 1x and 2x means 12 epochs and 24 epochs respectively. 20e is adopted in cascade models, which denotes 20 epochs. For 1x/2x, initial learning rate decays by a factor of 10 at the 8/16th and 11/22th epochs. For 20e, initial learning rate decays by a factor of 10 at the 16th and 19th epochs.
+
+`{dataset}`: dataset like nus-3d, kitti-3d, lyft-3d, scannet-3d, sunrgbd-3d. We also indicate the number of classes we are using if there exist multiple settings, e.g., kitti-3d-3class and kitti-3d-car means training on KITTI dataset with 3 classes and single class, respectively.
+
+## Usage
+
+### Test time augmentation
+
+We have supported double-flip and scale augmentation during test time. To use test time augmentation, users need to modify the
+`test_pipeline` and `test_cfg` in the config.
+For example, we change `centerpoint_0075voxel_second_secfpn_circlenms_4x8_cyclic_20e_nus.py` to the following.
+
+```python
+_base_ = './centerpoint_0075voxel_second_secfpn_circlenms' \
+ '_4x8_cyclic_20e_nus.py'
+
+model = dict(
+ test_cfg=dict(
+ pts=dict(
+ use_rotate_nms=True,
+ max_num=83)))
+
+point_cloud_range = [-54, -54, -5.0, 54, 54, 3.0]
+file_client_args = dict(backend='disk')
+class_names = [
+ 'car', 'truck', 'construction_vehicle', 'bus', 'trailer', 'barrier',
+ 'motorcycle', 'bicycle', 'pedestrian', 'traffic_cone'
+]
+
+test_pipeline = [
+ dict(
+ type='LoadPointsFromFile',
+ load_dim=5,
+ use_dim=5,
+ file_client_args=file_client_args),
+ dict(
+ type='LoadPointsFromMultiSweeps',
+ sweeps_num=9,
+ use_dim=[0, 1, 2, 3, 4],
+ file_client_args=file_client_args,
+ pad_empty_sweeps=True,
+ remove_close=True),
+ dict(
+ type='MultiScaleFlipAug3D',
+ img_scale=(1333, 800),
+ pts_scale_ratio=[0.95, 1.0, 1.05],
+ flip=True,
+ pcd_horizontal_flip=True,
+ pcd_vertical_flip=True,
+ transforms=[
+ dict(
+ type='GlobalRotScaleTrans',
+ rot_range=[0, 0],
+ scale_ratio_range=[1., 1.],
+ translation_std=[0, 0, 0]),
+ dict(type='RandomFlip3D', sync_2d=False),
+ dict(
+ type='PointsRangeFilter', point_cloud_range=point_cloud_range),
+ dict(
+ type='DefaultFormatBundle3D',
+ class_names=class_names,
+ with_label=False),
+ dict(type='Collect3D', keys=['points'])
+ ])
+]
+
+data = dict(
+ val=dict(pipeline=test_pipeline), test=dict(pipeline=test_pipeline))
+
+```
+
+## Results and models
+
+### CenterPoint
+
+| Backbone | Voxel type (voxel size) | Dcn | Circular nms | Mem (GB) | Inf time (fps) | mAP | NDS | Download |
+| :---------------------------------------------------------------------------------: | :---------------------: | :-: | :----------: | :------: | :------------: | :---: | :---: | :--------------------------------------------------------------------------------------------------------------------------------------------------------------------------------------------------------------------------------------------------------------------------------------------------------------------------------------------------------------------------------------------------------------------------------------------------------------------------------------------------------: |
+| [SECFPN](./centerpoint_01voxel_second_secfpn_circlenms_4x8_cyclic_20e_nus.py) | voxel (0.1) | ✗ | ✓ | 4.9 | | 56.19 | 64.43 | [model](https://download.openmmlab.com/mmdetection3d/v1.0.0_models/centerpoint/centerpoint_01voxel_second_secfpn_circlenms_4x8_cyclic_20e_nus/centerpoint_01voxel_second_secfpn_circlenms_4x8_cyclic_20e_nus_20210815_085857-9ba7f3a5.pth) \| [log](https://download.openmmlab.com/mmdetection3d/v1.0.0_models/centerpoint/centerpoint_01voxel_second_secfpn_circlenms_4x8_cyclic_20e_nus/centerpoint_01voxel_second_secfpn_circlenms_4x8_cyclic_20e_nus_20210815_085857.log.json) |
+| above w/o circle nms | voxel (0.1) | ✗ | ✗ | | | 56.56 | 64.46 | |
+| [SECFPN](./centerpoint_01voxel_second_secfpn_dcn_circlenms_4x8_cyclic_20e_nus.py) | voxel (0.1) | ✓ | ✓ | 5.2 | | 56.34 | 64.81 | [model](https://download.openmmlab.com/mmdetection3d/v1.0.0_models/centerpoint/centerpoint_01voxel_second_secfpn_dcn_circlenms_4x8_cyclic_20e_nus/centerpoint_01voxel_second_secfpn_dcn_circlenms_4x8_cyclic_20e_nus_20210814_060754-c9d535d2.pth) \| [log](https://download.openmmlab.com/mmdetection3d/v1.0.0_models/centerpoint/centerpoint_01voxel_second_secfpn_dcn_circlenms_4x8_cyclic_20e_nus/centerpoint_01voxel_second_secfpn_dcn_circlenms_4x8_cyclic_20e_nus_20210814_060754.log.json) |
+| above w/o circle nms | voxel (0.1) | ✓ | ✗ | | | 56.60 | 64.90 | |
+| [SECFPN](./centerpoint_0075voxel_second_secfpn_circlenms_4x8_cyclic_20e_nus.py) | voxel (0.075) | ✗ | ✓ | 7.8 | | 57.34 | 65.23 | [model](https://download.openmmlab.com/mmdetection3d/v1.0.0_models/centerpoint/centerpoint_0075voxel_second_secfpn_circlenms_4x8_cyclic_20e_nus/centerpoint_0075voxel_second_secfpn_circlenms_4x8_cyclic_20e_nus_20210814_113418-76ae0cf0.pth) \| [log](https://download.openmmlab.com/mmdetection3d/v1.0.0_models/centerpoint/centerpoint_0075voxel_second_secfpn_circlenms_4x8_cyclic_20e_nus/centerpoint_0075voxel_second_secfpn_circlenms_4x8_cyclic_20e_nus_20210814_113418.log.json) |
+| above w/o circle nms | voxel (0.075) | ✗ | ✗ | | | 57.63 | 65.39 | |
+| [SECFPN](./centerpoint_0075voxel_second_secfpn_dcn_circlenms_4x8_cyclic_20e_nus.py) | voxel (0.075) | ✓ | ✓ | 8.5 | | 57.27 | 65.58 | [model](https://download.openmmlab.com/mmdetection3d/v1.0.0_models/centerpoint/centerpoint_0075voxel_second_secfpn_dcn_circlenms_4x8_cyclic_20e_nus/centerpoint_0075voxel_second_secfpn_dcn_circlenms_4x8_cyclic_20e_nus_20210827_161135-1782af3e.pth) \| [log](https://download.openmmlab.com/mmdetection3d/v1.0.0_models/centerpoint/centerpoint_0075voxel_second_secfpn_dcn_circlenms_4x8_cyclic_20e_nus/centerpoint_0075voxel_second_secfpn_dcn_circlenms_4x8_cyclic_20e_nus_20210827_161135.log.json) |
+| above w/o circle nms | voxel (0.075) | ✓ | ✗ | | | 57.43 | 65.63 | |
+| above w/ double flip | voxel (0.075) | ✓ | ✗ | | | 59.73 | 67.39 | |
+| above w/ scale tta | voxel (0.075) | ✓ | ✗ | | | 60.43 | 67.65 | |
+| above w/ circle nms w/o scale tta | voxel (0.075) | ✓ | ✗ | | | 59.52 | 67.24 | |
+| [SECFPN](./centerpoint_02pillar_second_secfpn_circlenms_4x8_cyclic_20e_nus.py) | pillar (0.2) | ✗ | ✓ | 4.4 | | 49.07 | 59.66 | [model](https://download.openmmlab.com/mmdetection3d/v1.0.0_models/centerpoint/centerpoint_02pillar_second_secfpn_circlenms_4x8_cyclic_20e_nus/centerpoint_02pillar_second_secfpn_circlenms_4x8_cyclic_20e_nus_20210816_064624-0f3299c0.pth) \| [log](https://download.openmmlab.com/mmdetection3d/v1.0.0_models/centerpoint/centerpoint_02pillar_second_secfpn_circlenms_4x8_cyclic_20e_nus/centerpoint_02pillar_second_secfpn_circlenms_4x8_cyclic_20e_nus_20210816_064624.log.json) |
+| above w/o circle nms | pillar (0.2) | ✗ | ✗ | | | 49.12 | 59.66 | |
+| [SECFPN](./centerpoint_02pillar_second_secfpn_dcn_4x8_cyclic_20e_nus.py) | pillar (0.2) | ✓ | ✗ | 4.6 | | 48.8 | 59.67 | [model](https://download.openmmlab.com/mmdetection3d/v1.0.0_models/centerpoint/centerpoint_02pillar_second_secfpn_dcn_4x8_cyclic_20e_nus/centerpoint_02pillar_second_secfpn_dcn_4x8_cyclic_20e_nus_20210815_202702-f03ab9e4.pth) \| [log](https://download.openmmlab.com/mmdetection3d/v1.0.0_models/centerpoint/centerpoint_02pillar_second_secfpn_dcn_4x8_cyclic_20e_nus/centerpoint_02pillar_second_secfpn_dcn_4x8_cyclic_20e_nus_20210815_202702.log.json) |
+| above w/ circle nms | pillar (0.2) | ✓ | ✓ | | | 48.79 | 59.65 | |
+
+## Citation
+
+```latex
+@article{yin2021center,
+ title={Center-based 3D Object Detection and Tracking},
+ author={Yin, Tianwei and Zhou, Xingyi and Kr{\"a}henb{\"u}hl, Philipp},
+ journal={CVPR},
+ year={2021},
+}
+```
diff --git a/configs/centerpoint/centerpoint_0075voxel_second_secfpn_4x8_cyclic_20e_nus.py b/configs/centerpoint/centerpoint_0075voxel_second_secfpn_4x8_cyclic_20e_nus.py
new file mode 100644
index 0000000..f17d98e
--- /dev/null
+++ b/configs/centerpoint/centerpoint_0075voxel_second_secfpn_4x8_cyclic_20e_nus.py
@@ -0,0 +1,140 @@
+_base_ = ['./centerpoint_01voxel_second_secfpn_4x8_cyclic_20e_nus.py']
+
+# If point cloud range is changed, the models should also change their point
+# cloud range accordingly
+voxel_size = [0.075, 0.075, 0.2]
+point_cloud_range = [-54, -54, -5.0, 54, 54, 3.0]
+# For nuScenes we usually do 10-class detection
+class_names = [
+ 'car', 'truck', 'construction_vehicle', 'bus', 'trailer', 'barrier',
+ 'motorcycle', 'bicycle', 'pedestrian', 'traffic_cone'
+]
+
+model = dict(
+ pts_voxel_layer=dict(
+ voxel_size=voxel_size, point_cloud_range=point_cloud_range),
+ pts_middle_encoder=dict(sparse_shape=[41, 1440, 1440]),
+ pts_bbox_head=dict(
+ bbox_coder=dict(
+ voxel_size=voxel_size[:2], pc_range=point_cloud_range[:2])),
+ train_cfg=dict(
+ pts=dict(
+ grid_size=[1440, 1440, 40],
+ voxel_size=voxel_size,
+ point_cloud_range=point_cloud_range)),
+ test_cfg=dict(
+ pts=dict(voxel_size=voxel_size[:2], pc_range=point_cloud_range[:2])))
+
+dataset_type = 'NuScenesDataset'
+data_root = 'data/nuscenes/'
+file_client_args = dict(backend='disk')
+
+db_sampler = dict(
+ data_root=data_root,
+ info_path=data_root + 'nuscenes_dbinfos_train.pkl',
+ rate=1.0,
+ prepare=dict(
+ filter_by_difficulty=[-1],
+ filter_by_min_points=dict(
+ car=5,
+ truck=5,
+ bus=5,
+ trailer=5,
+ construction_vehicle=5,
+ traffic_cone=5,
+ barrier=5,
+ motorcycle=5,
+ bicycle=5,
+ pedestrian=5)),
+ classes=class_names,
+ sample_groups=dict(
+ car=2,
+ truck=3,
+ construction_vehicle=7,
+ bus=4,
+ trailer=6,
+ barrier=2,
+ motorcycle=6,
+ bicycle=6,
+ pedestrian=2,
+ traffic_cone=2),
+ points_loader=dict(
+ type='LoadPointsFromFile',
+ coord_type='LIDAR',
+ load_dim=5,
+ use_dim=[0, 1, 2, 3, 4],
+ file_client_args=file_client_args))
+
+train_pipeline = [
+ dict(
+ type='LoadPointsFromFile',
+ coord_type='LIDAR',
+ load_dim=5,
+ use_dim=5,
+ file_client_args=file_client_args),
+ dict(
+ type='LoadPointsFromMultiSweeps',
+ sweeps_num=9,
+ use_dim=[0, 1, 2, 3, 4],
+ file_client_args=file_client_args,
+ pad_empty_sweeps=True,
+ remove_close=True),
+ dict(type='LoadAnnotations3D', with_bbox_3d=True, with_label_3d=True),
+ dict(type='ObjectSample', db_sampler=db_sampler),
+ dict(
+ type='GlobalRotScaleTrans',
+ rot_range=[-0.3925, 0.3925],
+ scale_ratio_range=[0.95, 1.05],
+ translation_std=[0, 0, 0]),
+ dict(
+ type='RandomFlip3D',
+ sync_2d=False,
+ flip_ratio_bev_horizontal=0.5,
+ flip_ratio_bev_vertical=0.5),
+ dict(type='PointsRangeFilter', point_cloud_range=point_cloud_range),
+ dict(type='ObjectRangeFilter', point_cloud_range=point_cloud_range),
+ dict(type='ObjectNameFilter', classes=class_names),
+ dict(type='PointShuffle'),
+ dict(type='DefaultFormatBundle3D', class_names=class_names),
+ dict(type='Collect3D', keys=['points', 'gt_bboxes_3d', 'gt_labels_3d'])
+]
+test_pipeline = [
+ dict(
+ type='LoadPointsFromFile',
+ coord_type='LIDAR',
+ load_dim=5,
+ use_dim=5,
+ file_client_args=file_client_args),
+ dict(
+ type='LoadPointsFromMultiSweeps',
+ sweeps_num=9,
+ use_dim=[0, 1, 2, 3, 4],
+ file_client_args=file_client_args,
+ pad_empty_sweeps=True,
+ remove_close=True),
+ dict(
+ type='MultiScaleFlipAug3D',
+ img_scale=(1333, 800),
+ pts_scale_ratio=1,
+ flip=False,
+ transforms=[
+ dict(
+ type='GlobalRotScaleTrans',
+ rot_range=[0, 0],
+ scale_ratio_range=[1., 1.],
+ translation_std=[0, 0, 0]),
+ dict(type='RandomFlip3D'),
+ dict(
+ type='PointsRangeFilter', point_cloud_range=point_cloud_range),
+ dict(
+ type='DefaultFormatBundle3D',
+ class_names=class_names,
+ with_label=False),
+ dict(type='Collect3D', keys=['points'])
+ ])
+]
+
+data = dict(
+ train=dict(dataset=dict(pipeline=train_pipeline)),
+ val=dict(pipeline=test_pipeline),
+ test=dict(pipeline=test_pipeline))
diff --git a/configs/centerpoint/centerpoint_0075voxel_second_secfpn_circlenms_4x8_cyclic_20e_nus.py b/configs/centerpoint/centerpoint_0075voxel_second_secfpn_circlenms_4x8_cyclic_20e_nus.py
new file mode 100644
index 0000000..1541a10
--- /dev/null
+++ b/configs/centerpoint/centerpoint_0075voxel_second_secfpn_circlenms_4x8_cyclic_20e_nus.py
@@ -0,0 +1,3 @@
+_base_ = ['./centerpoint_0075voxel_second_secfpn_4x8_cyclic_20e_nus.py']
+
+model = dict(test_cfg=dict(pts=dict(nms_type='circle')))
diff --git a/configs/centerpoint/centerpoint_0075voxel_second_secfpn_dcn_4x8_cyclic_20e_nus.py b/configs/centerpoint/centerpoint_0075voxel_second_secfpn_dcn_4x8_cyclic_20e_nus.py
new file mode 100644
index 0000000..e479650
--- /dev/null
+++ b/configs/centerpoint/centerpoint_0075voxel_second_secfpn_dcn_4x8_cyclic_20e_nus.py
@@ -0,0 +1,15 @@
+_base_ = ['./centerpoint_0075voxel_second_secfpn_4x8_cyclic_20e_nus.py']
+
+model = dict(
+ pts_bbox_head=dict(
+ separate_head=dict(
+ type='DCNSeparateHead',
+ dcn_config=dict(
+ type='DCN',
+ in_channels=64,
+ out_channels=64,
+ kernel_size=3,
+ padding=1,
+ groups=4),
+ init_bias=-2.19,
+ final_kernel=3)))
diff --git a/configs/centerpoint/centerpoint_0075voxel_second_secfpn_dcn_4x8_cyclic_flip-tta_20e_nus.py b/configs/centerpoint/centerpoint_0075voxel_second_secfpn_dcn_4x8_cyclic_flip-tta_20e_nus.py
new file mode 100644
index 0000000..0090b3c
--- /dev/null
+++ b/configs/centerpoint/centerpoint_0075voxel_second_secfpn_dcn_4x8_cyclic_flip-tta_20e_nus.py
@@ -0,0 +1,50 @@
+_base_ = './centerpoint_0075voxel_second_secfpn_dcn_4x8_cyclic_20e_nus.py'
+
+point_cloud_range = [-54, -54, -5.0, 54, 54, 3.0]
+file_client_args = dict(backend='disk')
+class_names = [
+ 'car', 'truck', 'construction_vehicle', 'bus', 'trailer', 'barrier',
+ 'motorcycle', 'bicycle', 'pedestrian', 'traffic_cone'
+]
+
+test_pipeline = [
+ dict(
+ type='LoadPointsFromFile',
+ coord_type='LIDAR',
+ load_dim=5,
+ use_dim=5,
+ file_client_args=file_client_args),
+ dict(
+ type='LoadPointsFromMultiSweeps',
+ sweeps_num=9,
+ use_dim=[0, 1, 2, 3, 4],
+ file_client_args=file_client_args,
+ pad_empty_sweeps=True,
+ remove_close=True),
+ dict(
+ type='MultiScaleFlipAug3D',
+ img_scale=(1333, 800),
+ pts_scale_ratio=1,
+ # Add double-flip augmentation
+ flip=True,
+ pcd_horizontal_flip=True,
+ pcd_vertical_flip=True,
+ transforms=[
+ dict(
+ type='GlobalRotScaleTrans',
+ rot_range=[0, 0],
+ scale_ratio_range=[1., 1.],
+ translation_std=[0, 0, 0]),
+ dict(type='RandomFlip3D', sync_2d=False),
+ dict(
+ type='PointsRangeFilter', point_cloud_range=point_cloud_range),
+ dict(
+ type='DefaultFormatBundle3D',
+ class_names=class_names,
+ with_label=False),
+ dict(type='Collect3D', keys=['points'])
+ ])
+]
+
+data = dict(
+ val=dict(pipeline=test_pipeline), test=dict(pipeline=test_pipeline))
diff --git a/configs/centerpoint/centerpoint_0075voxel_second_secfpn_dcn_4x8_cyclic_tta_20e_nus.py b/configs/centerpoint/centerpoint_0075voxel_second_secfpn_dcn_4x8_cyclic_tta_20e_nus.py
new file mode 100644
index 0000000..cdbdf06
--- /dev/null
+++ b/configs/centerpoint/centerpoint_0075voxel_second_secfpn_dcn_4x8_cyclic_tta_20e_nus.py
@@ -0,0 +1,52 @@
+_base_ = './centerpoint_0075voxel_second_secfpn_dcn_4x8_cyclic_20e_nus.py'
+
+model = dict(test_cfg=dict(pts=dict(use_rotate_nms=True, max_num=500)))
+
+point_cloud_range = [-54, -54, -5.0, 54, 54, 3.0]
+file_client_args = dict(backend='disk')
+class_names = [
+ 'car', 'truck', 'construction_vehicle', 'bus', 'trailer', 'barrier',
+ 'motorcycle', 'bicycle', 'pedestrian', 'traffic_cone'
+]
+
+test_pipeline = [
+ dict(
+ type='LoadPointsFromFile',
+ coord_type='LIDAR',
+ load_dim=5,
+ use_dim=5,
+ file_client_args=file_client_args),
+ dict(
+ type='LoadPointsFromMultiSweeps',
+ sweeps_num=9,
+ use_dim=[0, 1, 2, 3, 4],
+ file_client_args=file_client_args,
+ pad_empty_sweeps=True,
+ remove_close=True),
+ dict(
+ type='MultiScaleFlipAug3D',
+ img_scale=(1333, 800),
+ pts_scale_ratio=[0.95, 1.0, 1.05],
+ # Add double-flip augmentation
+ flip=True,
+ pcd_horizontal_flip=True,
+ pcd_vertical_flip=True,
+ transforms=[
+ dict(
+ type='GlobalRotScaleTrans',
+ rot_range=[0, 0],
+ scale_ratio_range=[1., 1.],
+ translation_std=[0, 0, 0]),
+ dict(type='RandomFlip3D', sync_2d=False),
+ dict(
+ type='PointsRangeFilter', point_cloud_range=point_cloud_range),
+ dict(
+ type='DefaultFormatBundle3D',
+ class_names=class_names,
+ with_label=False),
+ dict(type='Collect3D', keys=['points'])
+ ])
+]
+
+data = dict(
+ val=dict(pipeline=test_pipeline), test=dict(pipeline=test_pipeline))
diff --git a/configs/centerpoint/centerpoint_0075voxel_second_secfpn_dcn_circlenms_4x8_cyclic_20e_nus.py b/configs/centerpoint/centerpoint_0075voxel_second_secfpn_dcn_circlenms_4x8_cyclic_20e_nus.py
new file mode 100644
index 0000000..1e7d14e
--- /dev/null
+++ b/configs/centerpoint/centerpoint_0075voxel_second_secfpn_dcn_circlenms_4x8_cyclic_20e_nus.py
@@ -0,0 +1,16 @@
+_base_ = ['./centerpoint_0075voxel_second_secfpn_4x8_cyclic_20e_nus.py']
+
+model = dict(
+ pts_bbox_head=dict(
+ separate_head=dict(
+ type='DCNSeparateHead',
+ dcn_config=dict(
+ type='DCN',
+ in_channels=64,
+ out_channels=64,
+ kernel_size=3,
+ padding=1,
+ groups=4),
+ init_bias=-2.19,
+ final_kernel=3)),
+ test_cfg=dict(pts=dict(nms_type='circle')))
diff --git a/configs/centerpoint/centerpoint_0075voxel_second_secfpn_dcn_circlenms_4x8_cyclic_flip-tta_20e_nus.py b/configs/centerpoint/centerpoint_0075voxel_second_secfpn_dcn_circlenms_4x8_cyclic_flip-tta_20e_nus.py
new file mode 100644
index 0000000..d3956fc
--- /dev/null
+++ b/configs/centerpoint/centerpoint_0075voxel_second_secfpn_dcn_circlenms_4x8_cyclic_flip-tta_20e_nus.py
@@ -0,0 +1,51 @@
+_base_ = './centerpoint_0075voxel_second_secfpn_dcn_' \
+ 'circlenms_4x8_cyclic_20e_nus.py'
+
+point_cloud_range = [-54, -54, -5.0, 54, 54, 3.0]
+file_client_args = dict(backend='disk')
+class_names = [
+ 'car', 'truck', 'construction_vehicle', 'bus', 'trailer', 'barrier',
+ 'motorcycle', 'bicycle', 'pedestrian', 'traffic_cone'
+]
+
+test_pipeline = [
+ dict(
+ type='LoadPointsFromFile',
+ coord_type='LIDAR',
+ load_dim=5,
+ use_dim=5,
+ file_client_args=file_client_args),
+ dict(
+ type='LoadPointsFromMultiSweeps',
+ sweeps_num=9,
+ use_dim=[0, 1, 2, 3, 4],
+ file_client_args=file_client_args,
+ pad_empty_sweeps=True,
+ remove_close=True),
+ dict(
+ type='MultiScaleFlipAug3D',
+ img_scale=(1333, 800),
+ pts_scale_ratio=1,
+ # Add double-flip augmentation
+ flip=True,
+ pcd_horizontal_flip=True,
+ pcd_vertical_flip=True,
+ transforms=[
+ dict(
+ type='GlobalRotScaleTrans',
+ rot_range=[0, 0],
+ scale_ratio_range=[1., 1.],
+ translation_std=[0, 0, 0]),
+ dict(type='RandomFlip3D', sync_2d=False),
+ dict(
+ type='PointsRangeFilter', point_cloud_range=point_cloud_range),
+ dict(
+ type='DefaultFormatBundle3D',
+ class_names=class_names,
+ with_label=False),
+ dict(type='Collect3D', keys=['points'])
+ ])
+]
+
+data = dict(
+ val=dict(pipeline=test_pipeline), test=dict(pipeline=test_pipeline))
diff --git a/configs/centerpoint/centerpoint_01voxel_second_secfpn_4x8_cyclic_20e_nus.py b/configs/centerpoint/centerpoint_01voxel_second_secfpn_4x8_cyclic_20e_nus.py
new file mode 100644
index 0000000..eae9284
--- /dev/null
+++ b/configs/centerpoint/centerpoint_01voxel_second_secfpn_4x8_cyclic_20e_nus.py
@@ -0,0 +1,171 @@
+_base_ = [
+ '../_base_/datasets/nus-3d.py',
+ '../_base_/models/centerpoint_01voxel_second_secfpn_nus.py',
+ '../_base_/schedules/cyclic_20e.py', '../_base_/default_runtime.py'
+]
+
+# If point cloud range is changed, the models should also change their point
+# cloud range accordingly
+point_cloud_range = [-51.2, -51.2, -5.0, 51.2, 51.2, 3.0]
+# For nuScenes we usually do 10-class detection
+class_names = [
+ 'car', 'truck', 'construction_vehicle', 'bus', 'trailer', 'barrier',
+ 'motorcycle', 'bicycle', 'pedestrian', 'traffic_cone'
+]
+
+model = dict(
+ pts_voxel_layer=dict(point_cloud_range=point_cloud_range),
+ pts_bbox_head=dict(bbox_coder=dict(pc_range=point_cloud_range[:2])),
+ # model training and testing settings
+ train_cfg=dict(pts=dict(point_cloud_range=point_cloud_range)),
+ test_cfg=dict(pts=dict(pc_range=point_cloud_range[:2])))
+
+dataset_type = 'NuScenesDataset'
+data_root = 'data/nuscenes/'
+file_client_args = dict(backend='disk')
+
+db_sampler = dict(
+ data_root=data_root,
+ info_path=data_root + 'nuscenes_dbinfos_train.pkl',
+ rate=1.0,
+ prepare=dict(
+ filter_by_difficulty=[-1],
+ filter_by_min_points=dict(
+ car=5,
+ truck=5,
+ bus=5,
+ trailer=5,
+ construction_vehicle=5,
+ traffic_cone=5,
+ barrier=5,
+ motorcycle=5,
+ bicycle=5,
+ pedestrian=5)),
+ classes=class_names,
+ sample_groups=dict(
+ car=2,
+ truck=3,
+ construction_vehicle=7,
+ bus=4,
+ trailer=6,
+ barrier=2,
+ motorcycle=6,
+ bicycle=6,
+ pedestrian=2,
+ traffic_cone=2),
+ points_loader=dict(
+ type='LoadPointsFromFile',
+ coord_type='LIDAR',
+ load_dim=5,
+ use_dim=[0, 1, 2, 3, 4],
+ file_client_args=file_client_args))
+
+train_pipeline = [
+ dict(
+ type='LoadPointsFromFile',
+ coord_type='LIDAR',
+ load_dim=5,
+ use_dim=5,
+ file_client_args=file_client_args),
+ dict(
+ type='LoadPointsFromMultiSweeps',
+ sweeps_num=9,
+ use_dim=[0, 1, 2, 3, 4],
+ file_client_args=file_client_args,
+ pad_empty_sweeps=True,
+ remove_close=True),
+ dict(type='LoadAnnotations3D', with_bbox_3d=True, with_label_3d=True),
+ dict(type='ObjectSample', db_sampler=db_sampler),
+ dict(
+ type='GlobalRotScaleTrans',
+ rot_range=[-0.3925, 0.3925],
+ scale_ratio_range=[0.95, 1.05],
+ translation_std=[0, 0, 0]),
+ dict(
+ type='RandomFlip3D',
+ sync_2d=False,
+ flip_ratio_bev_horizontal=0.5,
+ flip_ratio_bev_vertical=0.5),
+ dict(type='PointsRangeFilter', point_cloud_range=point_cloud_range),
+ dict(type='ObjectRangeFilter', point_cloud_range=point_cloud_range),
+ dict(type='ObjectNameFilter', classes=class_names),
+ dict(type='PointShuffle'),
+ dict(type='DefaultFormatBundle3D', class_names=class_names),
+ dict(type='Collect3D', keys=['points', 'gt_bboxes_3d', 'gt_labels_3d'])
+]
+test_pipeline = [
+ dict(
+ type='LoadPointsFromFile',
+ coord_type='LIDAR',
+ load_dim=5,
+ use_dim=5,
+ file_client_args=file_client_args),
+ dict(
+ type='LoadPointsFromMultiSweeps',
+ sweeps_num=9,
+ use_dim=[0, 1, 2, 3, 4],
+ file_client_args=file_client_args,
+ pad_empty_sweeps=True,
+ remove_close=True),
+ dict(
+ type='MultiScaleFlipAug3D',
+ img_scale=(1333, 800),
+ pts_scale_ratio=1,
+ flip=False,
+ transforms=[
+ dict(
+ type='GlobalRotScaleTrans',
+ rot_range=[0, 0],
+ scale_ratio_range=[1., 1.],
+ translation_std=[0, 0, 0]),
+ dict(type='RandomFlip3D'),
+ dict(
+ type='PointsRangeFilter', point_cloud_range=point_cloud_range),
+ dict(
+ type='DefaultFormatBundle3D',
+ class_names=class_names,
+ with_label=False),
+ dict(type='Collect3D', keys=['points'])
+ ])
+]
+# construct a pipeline for data and gt loading in show function
+# please keep its loading function consistent with test_pipeline (e.g. client)
+eval_pipeline = [
+ dict(
+ type='LoadPointsFromFile',
+ coord_type='LIDAR',
+ load_dim=5,
+ use_dim=5,
+ file_client_args=file_client_args),
+ dict(
+ type='LoadPointsFromMultiSweeps',
+ sweeps_num=9,
+ use_dim=[0, 1, 2, 3, 4],
+ file_client_args=file_client_args,
+ pad_empty_sweeps=True,
+ remove_close=True),
+ dict(
+ type='DefaultFormatBundle3D',
+ class_names=class_names,
+ with_label=False),
+ dict(type='Collect3D', keys=['points'])
+]
+
+data = dict(
+ train=dict(
+ type='CBGSDataset',
+ dataset=dict(
+ type=dataset_type,
+ data_root=data_root,
+ ann_file=data_root + 'nuscenes_infos_train.pkl',
+ pipeline=train_pipeline,
+ classes=class_names,
+ test_mode=False,
+ use_valid_flag=True,
+ # we use box_type_3d='LiDAR' in kitti and nuscenes dataset
+ # and box_type_3d='Depth' in sunrgbd and scannet dataset.
+ box_type_3d='LiDAR')),
+ val=dict(pipeline=test_pipeline, classes=class_names),
+ test=dict(pipeline=test_pipeline, classes=class_names))
+
+evaluation = dict(interval=20, pipeline=eval_pipeline)
diff --git a/configs/centerpoint/centerpoint_01voxel_second_secfpn_circlenms_4x8_cyclic_20e_nus.py b/configs/centerpoint/centerpoint_01voxel_second_secfpn_circlenms_4x8_cyclic_20e_nus.py
new file mode 100644
index 0000000..ae56032
--- /dev/null
+++ b/configs/centerpoint/centerpoint_01voxel_second_secfpn_circlenms_4x8_cyclic_20e_nus.py
@@ -0,0 +1,3 @@
+_base_ = ['./centerpoint_01voxel_second_secfpn_4x8_cyclic_20e_nus.py']
+
+model = dict(test_cfg=dict(pts=dict(nms_type='circle')))
diff --git a/configs/centerpoint/centerpoint_01voxel_second_secfpn_dcn_4x8_cyclic_20e_nus.py b/configs/centerpoint/centerpoint_01voxel_second_secfpn_dcn_4x8_cyclic_20e_nus.py
new file mode 100644
index 0000000..5f31c44
--- /dev/null
+++ b/configs/centerpoint/centerpoint_01voxel_second_secfpn_dcn_4x8_cyclic_20e_nus.py
@@ -0,0 +1,15 @@
+_base_ = ['./centerpoint_01voxel_second_secfpn_4x8_cyclic_20e_nus.py']
+
+model = dict(
+ pts_bbox_head=dict(
+ separate_head=dict(
+ type='DCNSeparateHead',
+ dcn_config=dict(
+ type='DCN',
+ in_channels=64,
+ out_channels=64,
+ kernel_size=3,
+ padding=1,
+ groups=4),
+ init_bias=-2.19,
+ final_kernel=3)))
diff --git a/configs/centerpoint/centerpoint_01voxel_second_secfpn_dcn_circlenms_4x8_cyclic_20e_nus.py b/configs/centerpoint/centerpoint_01voxel_second_secfpn_dcn_circlenms_4x8_cyclic_20e_nus.py
new file mode 100644
index 0000000..cc5488e
--- /dev/null
+++ b/configs/centerpoint/centerpoint_01voxel_second_secfpn_dcn_circlenms_4x8_cyclic_20e_nus.py
@@ -0,0 +1,16 @@
+_base_ = ['./centerpoint_01voxel_second_secfpn_4x8_cyclic_20e_nus.py']
+
+model = dict(
+ pts_bbox_head=dict(
+ separate_head=dict(
+ type='DCNSeparateHead',
+ dcn_config=dict(
+ type='DCN',
+ in_channels=64,
+ out_channels=64,
+ kernel_size=3,
+ padding=1,
+ groups=4),
+ init_bias=-2.19,
+ final_kernel=3)),
+ test_cfg=dict(pts=dict(nms_type='circle')))
diff --git a/configs/centerpoint/centerpoint_02pillar_second_secfpn_4x8_cyclic_20e_nus.py b/configs/centerpoint/centerpoint_02pillar_second_secfpn_4x8_cyclic_20e_nus.py
new file mode 100644
index 0000000..cd90349
--- /dev/null
+++ b/configs/centerpoint/centerpoint_02pillar_second_secfpn_4x8_cyclic_20e_nus.py
@@ -0,0 +1,170 @@
+_base_ = [
+ '../_base_/datasets/nus-3d.py',
+ '../_base_/models/centerpoint_02pillar_second_secfpn_nus.py',
+ '../_base_/schedules/cyclic_20e.py', '../_base_/default_runtime.py'
+]
+
+# If point cloud range is changed, the models should also change their point
+# cloud range accordingly
+point_cloud_range = [-51.2, -51.2, -5.0, 51.2, 51.2, 3.0]
+# For nuScenes we usually do 10-class detection
+class_names = [
+ 'car', 'truck', 'construction_vehicle', 'bus', 'trailer', 'barrier',
+ 'motorcycle', 'bicycle', 'pedestrian', 'traffic_cone'
+]
+
+model = dict(
+ pts_voxel_layer=dict(point_cloud_range=point_cloud_range),
+ pts_voxel_encoder=dict(point_cloud_range=point_cloud_range),
+ pts_bbox_head=dict(bbox_coder=dict(pc_range=point_cloud_range[:2])),
+ # model training and testing settings
+ train_cfg=dict(pts=dict(point_cloud_range=point_cloud_range)),
+ test_cfg=dict(pts=dict(pc_range=point_cloud_range[:2])))
+
+dataset_type = 'NuScenesDataset'
+data_root = 'data/nuscenes/'
+file_client_args = dict(backend='disk')
+
+db_sampler = dict(
+ data_root=data_root,
+ info_path=data_root + 'nuscenes_dbinfos_train.pkl',
+ rate=1.0,
+ prepare=dict(
+ filter_by_difficulty=[-1],
+ filter_by_min_points=dict(
+ car=5,
+ truck=5,
+ bus=5,
+ trailer=5,
+ construction_vehicle=5,
+ traffic_cone=5,
+ barrier=5,
+ motorcycle=5,
+ bicycle=5,
+ pedestrian=5)),
+ classes=class_names,
+ sample_groups=dict(
+ car=2,
+ truck=3,
+ construction_vehicle=7,
+ bus=4,
+ trailer=6,
+ barrier=2,
+ motorcycle=6,
+ bicycle=6,
+ pedestrian=2,
+ traffic_cone=2),
+ points_loader=dict(
+ type='LoadPointsFromFile',
+ coord_type='LIDAR',
+ load_dim=5,
+ use_dim=[0, 1, 2, 3, 4],
+ file_client_args=file_client_args))
+
+train_pipeline = [
+ dict(
+ type='LoadPointsFromFile',
+ coord_type='LIDAR',
+ load_dim=5,
+ use_dim=5,
+ file_client_args=file_client_args),
+ dict(
+ type='LoadPointsFromMultiSweeps',
+ sweeps_num=9,
+ use_dim=[0, 1, 2, 3, 4],
+ file_client_args=file_client_args,
+ pad_empty_sweeps=True,
+ remove_close=True),
+ dict(type='LoadAnnotations3D', with_bbox_3d=True, with_label_3d=True),
+ dict(type='ObjectSample', db_sampler=db_sampler),
+ dict(
+ type='GlobalRotScaleTrans',
+ rot_range=[-0.3925, 0.3925],
+ scale_ratio_range=[0.95, 1.05],
+ translation_std=[0, 0, 0]),
+ dict(
+ type='RandomFlip3D',
+ sync_2d=False,
+ flip_ratio_bev_horizontal=0.5,
+ flip_ratio_bev_vertical=0.5),
+ dict(type='PointsRangeFilter', point_cloud_range=point_cloud_range),
+ dict(type='ObjectRangeFilter', point_cloud_range=point_cloud_range),
+ dict(type='ObjectNameFilter', classes=class_names),
+ dict(type='PointShuffle'),
+ dict(type='DefaultFormatBundle3D', class_names=class_names),
+ dict(type='Collect3D', keys=['points', 'gt_bboxes_3d', 'gt_labels_3d'])
+]
+test_pipeline = [
+ dict(
+ type='LoadPointsFromFile',
+ coord_type='LIDAR',
+ load_dim=5,
+ use_dim=5,
+ file_client_args=file_client_args),
+ dict(
+ type='LoadPointsFromMultiSweeps',
+ sweeps_num=9,
+ use_dim=[0, 1, 2, 3, 4],
+ file_client_args=file_client_args,
+ pad_empty_sweeps=True,
+ remove_close=True),
+ dict(
+ type='MultiScaleFlipAug3D',
+ img_scale=(1333, 800),
+ pts_scale_ratio=1,
+ flip=False,
+ transforms=[
+ dict(
+ type='GlobalRotScaleTrans',
+ rot_range=[0, 0],
+ scale_ratio_range=[1., 1.],
+ translation_std=[0, 0, 0]),
+ dict(type='RandomFlip3D'),
+ dict(
+ type='DefaultFormatBundle3D',
+ class_names=class_names,
+ with_label=False),
+ dict(type='Collect3D', keys=['points'])
+ ])
+]
+# construct a pipeline for data and gt loading in show function
+# please keep its loading function consistent with test_pipeline (e.g. client)
+eval_pipeline = [
+ dict(
+ type='LoadPointsFromFile',
+ coord_type='LIDAR',
+ load_dim=5,
+ use_dim=5,
+ file_client_args=file_client_args),
+ dict(
+ type='LoadPointsFromMultiSweeps',
+ sweeps_num=9,
+ use_dim=[0, 1, 2, 3, 4],
+ file_client_args=file_client_args,
+ pad_empty_sweeps=True,
+ remove_close=True),
+ dict(
+ type='DefaultFormatBundle3D',
+ class_names=class_names,
+ with_label=False),
+ dict(type='Collect3D', keys=['points'])
+]
+
+data = dict(
+ train=dict(
+ type='CBGSDataset',
+ dataset=dict(
+ type=dataset_type,
+ data_root=data_root,
+ ann_file=data_root + 'nuscenes_infos_train.pkl',
+ pipeline=train_pipeline,
+ classes=class_names,
+ test_mode=False,
+ use_valid_flag=True,
+ # we use box_type_3d='LiDAR' in kitti and nuscenes dataset
+ # and box_type_3d='Depth' in sunrgbd and scannet dataset.
+ box_type_3d='LiDAR')),
+ val=dict(pipeline=test_pipeline, classes=class_names),
+ test=dict(pipeline=test_pipeline, classes=class_names))
+
+evaluation = dict(interval=20, pipeline=eval_pipeline)
diff --git a/configs/centerpoint/centerpoint_02pillar_second_secfpn_circlenms_4x8_cyclic_20e_nus.py b/configs/centerpoint/centerpoint_02pillar_second_secfpn_circlenms_4x8_cyclic_20e_nus.py
new file mode 100644
index 0000000..67a1cf6
--- /dev/null
+++ b/configs/centerpoint/centerpoint_02pillar_second_secfpn_circlenms_4x8_cyclic_20e_nus.py
@@ -0,0 +1,3 @@
+_base_ = ['./centerpoint_02pillar_second_secfpn_4x8_cyclic_20e_nus.py']
+
+model = dict(test_cfg=dict(pts=dict(nms_type='circle')))
diff --git a/configs/centerpoint/centerpoint_02pillar_second_secfpn_dcn_4x8_cyclic_20e_nus.py b/configs/centerpoint/centerpoint_02pillar_second_secfpn_dcn_4x8_cyclic_20e_nus.py
new file mode 100644
index 0000000..e694892
--- /dev/null
+++ b/configs/centerpoint/centerpoint_02pillar_second_secfpn_dcn_4x8_cyclic_20e_nus.py
@@ -0,0 +1,15 @@
+_base_ = ['./centerpoint_02pillar_second_secfpn_4x8_cyclic_20e_nus.py']
+
+model = dict(
+ pts_bbox_head=dict(
+ separate_head=dict(
+ type='DCNSeparateHead',
+ dcn_config=dict(
+ type='DCN',
+ in_channels=64,
+ out_channels=64,
+ kernel_size=3,
+ padding=1,
+ groups=4),
+ init_bias=-2.19,
+ final_kernel=3)))
diff --git a/configs/centerpoint/centerpoint_02pillar_second_secfpn_dcn_circlenms_4x8_cyclic_20e_nus.py b/configs/centerpoint/centerpoint_02pillar_second_secfpn_dcn_circlenms_4x8_cyclic_20e_nus.py
new file mode 100644
index 0000000..c62488d
--- /dev/null
+++ b/configs/centerpoint/centerpoint_02pillar_second_secfpn_dcn_circlenms_4x8_cyclic_20e_nus.py
@@ -0,0 +1,16 @@
+_base_ = ['./centerpoint_02pillar_second_secfpn_4x8_cyclic_20e_nus.py']
+
+model = dict(
+ pts_bbox_head=dict(
+ separate_head=dict(
+ type='DCNSeparateHead',
+ dcn_config=dict(
+ type='DCN',
+ in_channels=64,
+ out_channels=64,
+ kernel_size=3,
+ padding=1,
+ groups=4),
+ init_bias=-2.19,
+ final_kernel=3)),
+ test_cfg=dict(pts=dict(nms_type='circle')))
diff --git a/configs/centerpoint/metafile.yml b/configs/centerpoint/metafile.yml
new file mode 100644
index 0000000..1651689
--- /dev/null
+++ b/configs/centerpoint/metafile.yml
@@ -0,0 +1,95 @@
+Collections:
+ - Name: CenterPoint
+ Metadata:
+ Training Data: nuScenes
+ Training Techniques:
+ - AdamW
+ Training Resources: 8x V100 GPUs
+ Architecture:
+ - Hard Voxelization
+ Paper:
+ URL: https://arxiv.org/abs/2006.11275
+ Title: 'Center-based 3D Object Detection and Tracking'
+ README: configs/centerpoint/README.md
+ Code:
+ URL: https://github.com/open-mmlab/mmdetection3d/blob/master/mmdet3d/models/detectors/centerpoint.py#L10
+ Version: v0.6.0
+
+Models:
+ - Name: centerpoint_01voxel_second_secfpn_circlenms_4x8_cyclic_20e_nus
+ In Collection: CenterPoint
+ Config: configs/centerpoint/centerpoint_01voxel_second_secfpn_circlenms_4x8_cyclic_20e_nus.py
+ Metadata:
+ Training Memory (GB): 4.9
+ Results:
+ - Task: 3D Object Detection
+ Dataset: nuScenes
+ Metrics:
+ mAP: 56.19
+ NDS: 64.43
+ Weights: https://download.openmmlab.com/mmdetection3d/v0.1.0_models/centerpoint/centerpoint_01voxel_second_secfpn_circlenms_4x8_cyclic_20e_nus/centerpoint_01voxel_second_secfpn_circlenms_4x8_cyclic_20e_nus_20201001_135205-5db91e00.pth
+
+ - Name: centerpoint_01voxel_second_secfpn_dcn_circlenms_4x8_cyclic_20e_nus
+ In Collection: CenterPoint
+ Config: configs/centerpoint/centerpoint_01voxel_second_secfpn_dcn_circlenms_4x8_cyclic_20e_nus.py
+ Metadata:
+ Training Memory (GB): 5.2
+ Results:
+ - Task: 3D Object Detection
+ Dataset: nuScenes
+ Metrics:
+ mAP: 56.34
+ NDS: 64.81
+ Weights: https://download.openmmlab.com/mmdetection3d/v0.1.0_models/centerpoint/centerpoint_01voxel_second_secfpn_dcn_circlenms_4x8_cyclic_20e_nus/centerpoint_01voxel_second_secfpn_dcn_circlenms_4x8_cyclic_20e_nus_20201004_075317-26d8176c.pth
+
+ - Name: centerpoint_0075voxel_second_secfpn_circlenms_4x8_cyclic_20e_nus
+ In Collection: CenterPoint
+ Config: configs/centerpoint/centerpoint_0075voxel_second_secfpn_circlenms_4x8_cyclic_20e_nus.py
+ Metadata:
+ Training Memory (GB): 7.8
+ Results:
+ - Task: 3D Object Detection
+ Dataset: nuScenes
+ Metrics:
+ mAP: 57.34
+ NDS: 65.23
+ Weights: https://download.openmmlab.com/mmdetection3d/v0.1.0_models/centerpoint/centerpoint_0075voxel_second_secfpn_circlenms_4x8_cyclic_20e_nus/centerpoint_0075voxel_second_secfpn_circlenms_4x8_cyclic_20e_nus_20200925_230905-358fbe3b.pth
+
+ - Name: centerpoint_0075voxel_second_secfpn_dcn_circlenms_4x8_cyclic_20e_nus
+ In Collection: CenterPoint
+ Config: configs/centerpoint/centerpoint_0075voxel_second_secfpn_dcn_circlenms_4x8_cyclic_20e_nus.py
+ Metadata:
+ Training Memory (GB): 8.5
+ Results:
+ - Task: 3D Object Detection
+ Dataset: nuScenes
+ Metrics:
+ mAP: 57.27
+ NDS: 65.58
+ Weights: https://download.openmmlab.com/mmdetection3d/v0.1.0_models/centerpoint/centerpoint_0075voxel_second_secfpn_dcn_circlenms_4x8_cyclic_20e_nus/centerpoint_0075voxel_second_secfpn_dcn_circlenms_4x8_cyclic_20e_nus_20200930_201619-67c8496f.pth
+
+ - Name: centerpoint_02pillar_second_secfpn_circlenms_4x8_cyclic_20e_nus
+ In Collection: CenterPoint
+ Config: configs/centerpoint/centerpoint_02pillar_second_secfpn_circlenms_4x8_cyclic_20e_nus.py
+ Metadata:
+ Training Memory (GB): 4.4
+ Results:
+ - Task: 3D Object Detection
+ Dataset: nuScenes
+ Metrics:
+ mAP: 49.07
+ NDS: 59.66
+ Weights: https://download.openmmlab.com/mmdetection3d/v0.1.0_models/centerpoint/centerpoint_01voxel_second_secfpn_circlenms_4x8_cyclic_20e_nus/centerpoint_02pillar_second_secfpn_circlenms_4x8_cyclic_20e_nus_20201004_170716-a134a233.pth
+
+ - Name: centerpoint_02pillar_second_secfpn_dcn_4x8_cyclic_20e_nus
+ In Collection: CenterPoint
+ Config: configs/centerpoint/centerpoint_02pillar_second_secfpn_dcn_4x8_cyclic_20e_nus.py
+ Metadata:
+ Training Memory (GB): 4.6
+ Results:
+ - Task: 3D Object Detection
+ Dataset: nuScenes
+ Metrics:
+ mAP: 48.8
+ NDS: 59.67
+ Weights: https://download.openmmlab.com/mmdetection3d/v0.1.0_models/centerpoint/centerpoint_02pillar_second_secfpn_dcn_4x8_cyclic_20e_nus/centerpoint_02pillar_second_secfpn_dcn_4x8_cyclic_20e_nus_20200930_103722-3bb135f2.pth
diff --git a/configs/dgcnn/README.md b/configs/dgcnn/README.md
new file mode 100644
index 0000000..5255435
--- /dev/null
+++ b/configs/dgcnn/README.md
@@ -0,0 +1,55 @@
+# Dynamic Graph CNN for Learning on Point Clouds
+
+> [Dynamic Graph CNN for Learning on Point Clouds](https://arxiv.org/abs/1801.07829)
+
+
+
+## Abstract
+
+Point clouds provide a flexible geometric representation suitable for countless applications in computer graphics; they also comprise the raw output of most 3D data acquisition devices. While hand-designed features on point clouds have long been proposed in graphics and vision, however, the recent overwhelming success of convolutional neural networks (CNNs) for image analysis suggests the value of adapting insight from CNN to the point cloud world. Point clouds inherently lack topological information so designing a model to recover topology can enrich the representation power of point clouds. To this end, we propose a new neural network module dubbed EdgeConv suitable for CNN-based high-level tasks on point clouds including classification and segmentation. EdgeConv acts on graphs dynamically computed in each layer of the network. It is differentiable and can be plugged into existing architectures. Compared to existing modules operating in extrinsic space or treating each point independently, EdgeConv has several appealing properties: It incorporates local neighborhood information; it can be stacked applied to learn global shape properties; and in multi-layer systems affinity in feature space captures semantic characteristics over potentially long distances in the original embedding. We show the performance of our model on standard benchmarks including ModelNet40, ShapeNetPart, and S3DIS.
+
+
+
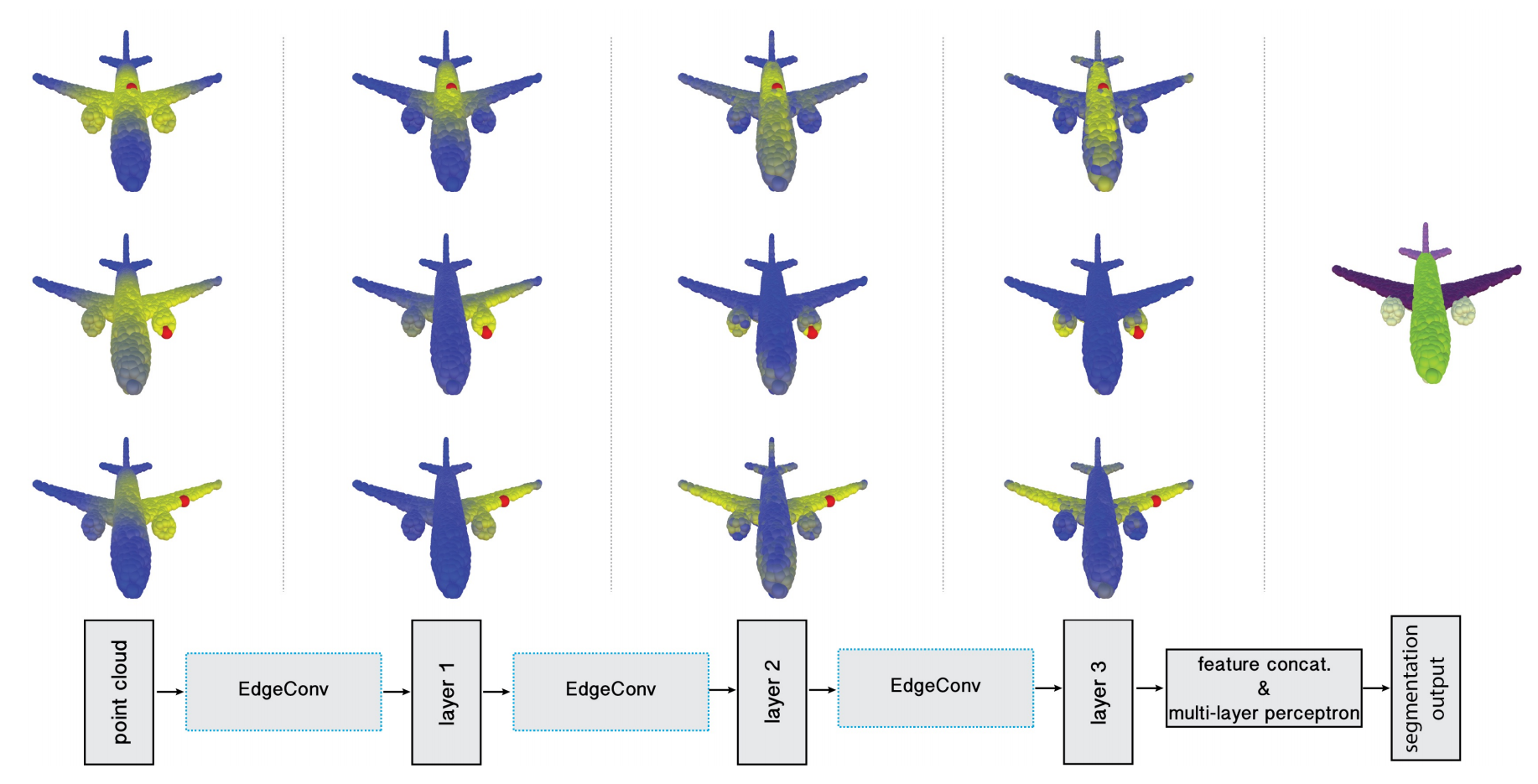
+
+
+## Introduction
+
+We implement DGCNN and provide the results and checkpoints on S3DIS dataset.
+
+**Notice**: We follow the implementations in the original DGCNN paper and a PyTorch implementation of DGCNN [code](https://github.com/AnTao97/dgcnn.pytorch).
+
+## Results and models
+
+### S3DIS
+
+| Method | Split | Lr schd | Mem (GB) | Inf time (fps) | mIoU (Val set) | Download |
+| :-------------------------------------------------------: | :----: | :---------: | :------: | :------------: | :------------: | :------------------------------------------------------------------------------------------------------------------------------------------------------------------------------------------------------------------------------------------------------------------------------------------------------------------------------------------------------------------------------------------------------: |
+| [DGCNN](./dgcnn_32x4_cosine_100e_s3dis_seg-3d-13class.py) | Area_1 | cosine 100e | 13.1 | | 68.33 | [model](https://download.openmmlab.com/mmdetection3d/v0.17.0_models/dgcnn/dgcnn_32x4_cosine_100e_s3dis_seg-3d-13class/area1/dgcnn_32x4_cosine_100e_s3dis_seg-3d-13class_20210731_000734-39658f14.pth) \| [log](https://download.openmmlab.com/mmdetection3d/v0.17.0_models/dgcnn/dgcnn_32x4_cosine_100e_s3dis_seg-3d-13class/area1/dgcnn_32x4_cosine_100e_s3dis_seg-3d-13class_20210731_000734.log.json) |
+| [DGCNN](./dgcnn_32x4_cosine_100e_s3dis_seg-3d-13class.py) | Area_2 | cosine 100e | 13.1 | | 40.68 | [model](https://download.openmmlab.com/mmdetection3d/v0.17.0_models/dgcnn/dgcnn_32x4_cosine_100e_s3dis_seg-3d-13class/area2/dgcnn_32x4_cosine_100e_s3dis_seg-3d-13class_20210731_144648-aea9ecb6.pth) \| [log](https://download.openmmlab.com/mmdetection3d/v0.17.0_models/dgcnn/dgcnn_32x4_cosine_100e_s3dis_seg-3d-13class/area2/dgcnn_32x4_cosine_100e_s3dis_seg-3d-13class_20210731_144648.log.json) |
+| [DGCNN](./dgcnn_32x4_cosine_100e_s3dis_seg-3d-13class.py) | Area_3 | cosine 100e | 13.1 | | 69.38 | [model](https://download.openmmlab.com/mmdetection3d/v0.17.0_models/dgcnn/dgcnn_32x4_cosine_100e_s3dis_seg-3d-13class/area3/dgcnn_32x4_cosine_100e_s3dis_seg-3d-13class_20210801_154629-2ff50ee0.pth) \| [log](https://download.openmmlab.com/mmdetection3d/v0.17.0_models/dgcnn/dgcnn_32x4_cosine_100e_s3dis_seg-3d-13class/area3/dgcnn_32x4_cosine_100e_s3dis_seg-3d-13class_20210801_154629.log.json) |
+| [DGCNN](./dgcnn_32x4_cosine_100e_s3dis_seg-3d-13class.py) | Area_4 | cosine 100e | 13.1 | | 50.07 | [model](https://download.openmmlab.com/mmdetection3d/v0.17.0_models/dgcnn/dgcnn_32x4_cosine_100e_s3dis_seg-3d-13class/area4/dgcnn_32x4_cosine_100e_s3dis_seg-3d-13class_20210802_073551-dffab9cd.pth) \| [log](https://download.openmmlab.com/mmdetection3d/v0.17.0_models/dgcnn/dgcnn_32x4_cosine_100e_s3dis_seg-3d-13class/area4/dgcnn_32x4_cosine_100e_s3dis_seg-3d-13class_20210802_073551.log.json) |
+| [DGCNN](./dgcnn_32x4_cosine_100e_s3dis_seg-3d-13class.py) | Area_5 | cosine 100e | 13.1 | | 50.59 | [model](https://download.openmmlab.com/mmdetection3d/v0.17.0_models/dgcnn/dgcnn_32x4_cosine_100e_s3dis_seg-3d-13class/area5/dgcnn_32x4_cosine_100e_s3dis_seg-3d-13class_20210730_235824-f277e0c5.pth) \| [log](https://download.openmmlab.com/mmdetection3d/v0.17.0_models/dgcnn/dgcnn_32x4_cosine_100e_s3dis_seg-3d-13class/area5/dgcnn_32x4_cosine_100e_s3dis_seg-3d-13class_20210730_235824.log.json) |
+| [DGCNN](./dgcnn_32x4_cosine_100e_s3dis_seg-3d-13class.py) | Area_6 | cosine 100e | 13.1 | | 77.94 | [model](https://download.openmmlab.com/mmdetection3d/v0.17.0_models/dgcnn/dgcnn_32x4_cosine_100e_s3dis_seg-3d-13class/area6/dgcnn_32x4_cosine_100e_s3dis_seg-3d-13class_20210802_154317-e3511b32.pth) \| [log](https://download.openmmlab.com/mmdetection3d/v0.17.0_models/dgcnn/dgcnn_32x4_cosine_100e_s3dis_seg-3d-13class/area6/dgcnn_32x4_cosine_100e_s3dis_seg-3d-13class_20210802_154317.log.json) |
+| [DGCNN](./dgcnn_32x4_cosine_100e_s3dis_seg-3d-13class.py) | 6-fold | | | | 59.43 | |
+
+**Notes:**
+
+- We use XYZ+Color+Normalized_XYZ as input in all the experiments on S3DIS datasets.
+- `Area_5` Split means training the model on Area_1, 2, 3, 4, 6 and testing on Area_5.
+- `6-fold` Split means the overall result of 6 different splits (Area_1, Area_2, Area_3, Area_4, Area_5 and Area_6 Splits).
+- Users need to modify `train_area` and `test_area` in the S3DIS dataset's [config](./configs/_base_/datasets/s3dis_seg-3d-13class.py) to set the training and testing areas, respectively.
+
+## Indeterminism
+
+Since DGCNN testing adopts sliding patch inference which involves random point sampling, and the test script uses fixed random seeds while the random seeds of validation in training are not fixed, the test results may be slightly different from the results reported above.
+
+## Citation
+
+```latex
+@article{dgcnn,
+ title={Dynamic Graph CNN for Learning on Point Clouds},
+ author={Wang, Yue and Sun, Yongbin and Liu, Ziwei and Sarma, Sanjay E. and Bronstein, Michael M. and Solomon, Justin M.},
+ journal={ACM Transactions on Graphics (TOG)},
+ year={2019}
+}
+```
diff --git a/configs/dgcnn/dgcnn_32x4_cosine_100e_s3dis_seg-3d-13class.py b/configs/dgcnn/dgcnn_32x4_cosine_100e_s3dis_seg-3d-13class.py
new file mode 100644
index 0000000..6f1b582
--- /dev/null
+++ b/configs/dgcnn/dgcnn_32x4_cosine_100e_s3dis_seg-3d-13class.py
@@ -0,0 +1,24 @@
+_base_ = [
+ '../_base_/datasets/s3dis_seg-3d-13class.py', '../_base_/models/dgcnn.py',
+ '../_base_/schedules/seg_cosine_100e.py', '../_base_/default_runtime.py'
+]
+
+# data settings
+data = dict(samples_per_gpu=32)
+evaluation = dict(interval=2)
+
+# model settings
+model = dict(
+ backbone=dict(in_channels=9), # [xyz, rgb, normalized_xyz]
+ decode_head=dict(
+ num_classes=13, ignore_index=13,
+ loss_decode=dict(class_weight=None)), # S3DIS doesn't use class_weight
+ test_cfg=dict(
+ num_points=4096,
+ block_size=1.0,
+ sample_rate=0.5,
+ use_normalized_coord=True,
+ batch_size=24))
+
+# runtime settings
+checkpoint_config = dict(interval=2)
diff --git a/configs/dgcnn/metafile.yml b/configs/dgcnn/metafile.yml
new file mode 100644
index 0000000..87ff915
--- /dev/null
+++ b/configs/dgcnn/metafile.yml
@@ -0,0 +1,24 @@
+Collections:
+ - Name: DGCNN
+ Metadata:
+ Training Techniques:
+ - SGD
+ Training Resources: 4x Titan XP GPUs
+ Architecture:
+ - DGCNN
+ Paper: https://arxiv.org/abs/1801.07829
+ README: configs/dgcnn/README.md
+
+Models:
+ - Name: dgcnn_32x4_cosine_100e_s3dis_seg-3d-13class.py
+ In Collection: DGCNN
+ Config: configs/dgcnn/dgcnn_32x4_cosine_100e_s3dis_seg-3d-13class.py
+ Metadata:
+ Training Data: S3DIS
+ Training Memory (GB): 13.3
+ Results:
+ - Task: 3D Semantic Segmentation
+ Dataset: S3DIS
+ Metrics:
+ mIoU: 50.59
+ Weights: https://download.openmmlab.com/mmdetection3d/v0.17.0_models/dgcnn/dgcnn_32x4_cosine_100e_s3dis_seg-3d-13class/area5/dgcnn_32x4_cosine_100e_s3dis_seg-3d-13class_20210730_235824-f277e0c5.pth
diff --git a/configs/dynamic_voxelization/README.md b/configs/dynamic_voxelization/README.md
new file mode 100644
index 0000000..ab2bbc6
--- /dev/null
+++ b/configs/dynamic_voxelization/README.md
@@ -0,0 +1,40 @@
+# Dynamic Voxelization
+
+> [End-to-End Multi-View Fusion for 3D Object Detection in LiDAR Point Clouds](https://arxiv.org/abs/1910.06528)
+
+
+
+## Abstract
+
+Recent work on 3D object detection advocates point cloud voxelization in birds-eye view, where objects preserve their physical dimensions and are naturally separable. When represented in this view, however, point clouds are sparse and have highly variable point density, which may cause detectors difficulties in detecting distant or small objects (pedestrians, traffic signs, etc.). On the other hand, perspective view provides dense observations, which could allow more favorable feature encoding for such cases. In this paper, we aim to synergize the birds-eye view and the perspective view and propose a novel end-to-end multi-view fusion (MVF) algorithm, which can effectively learn to utilize the complementary information from both. Specifically, we introduce dynamic voxelization, which has four merits compared to existing voxelization methods, i) removing the need of pre-allocating a tensor with fixed size; ii) overcoming the information loss due to stochastic point/voxel dropout; iii) yielding deterministic voxel embeddings and more stable detection outcomes; iv) establishing the bi-directional relationship between points and voxels, which potentially lays a natural foundation for cross-view feature fusion. By employing dynamic voxelization, the proposed feature fusion architecture enables each point to learn to fuse context information from different views. MVF operates on points and can be naturally extended to other approaches using LiDAR point clouds. We evaluate our MVF model extensively on the newly released Waymo Open Dataset and on the KITTI dataset and demonstrate that it significantly improves detection accuracy over the comparable single-view PointPillars baseline.
+
+
+
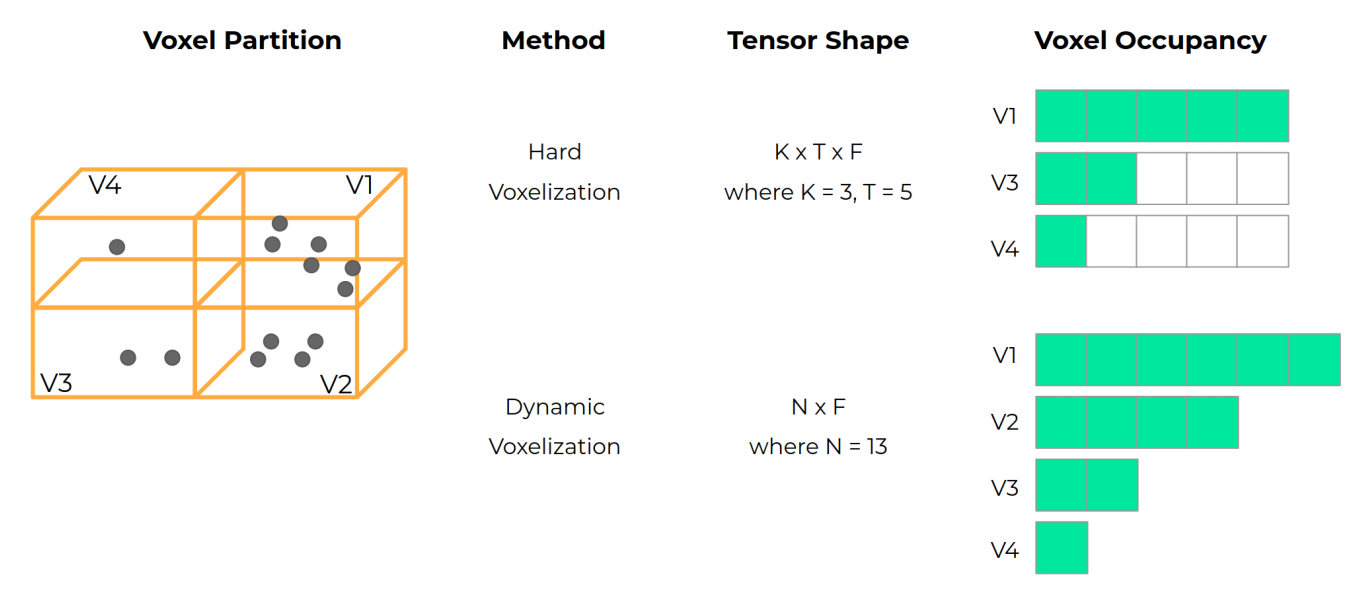
+
+
+## Introduction
+
+We implement Dynamic Voxelization proposed in and provide its results and models on KITTI dataset.
+
+## Results and models
+
+### KITTI
+
+| Model | Class | Lr schd | Mem (GB) | Inf time (fps) | mAP | Download |
+| :---------------------------------------------------------------: | :-----: | :--------: | :------: | :------------: | :---: | :--------------------------------------------------------------------------------------------------------------------------------------------------------------------------------------------------------------------------------------------------------------------------------------------------------------------------------------------------------------------------------------------------------------------------------------: |
+| [SECOND](./dv_second_secfpn_6x8_80e_kitti-3d-car.py) | Car | cyclic 80e | 5.5 | | 78.83 | [model](https://download.openmmlab.com/mmdetection3d/v0.1.0_models/dynamic_voxelization/dv_second_secfpn_6x8_80e_kitti-3d-car/dv_second_secfpn_6x8_80e_kitti-3d-car_20200620_235228-ac2c1c0c.pth) \| [log](https://download.openmmlab.com/mmdetection3d/v0.1.0_models/dynamic_voxelization/dv_second_secfpn_6x8_80e_kitti-3d-car/dv_second_secfpn_6x8_80e_kitti-3d-car_20200620_235228.log.json) |
+| [SECOND](./dv_second_secfpn_2x8_cosine_80e_kitti-3d-3class.py) | 3 Class | cosine 80e | 5.5 | | 65.27 | [model](https://download.openmmlab.com/mmdetection3d/v1.0.0_models/dynamic_voxelization/dv_second_secfpn_2x8_cosine_80e_kitti-3d-3class/dv_second_secfpn_2x8_cosine_80e_kitti-3d-3class_20210831_054106-e742d163.pth) \| [log](https://download.openmmlab.com/mmdetection3d/v1.0.0_models/dynamic_voxelization/dv_second_secfpn_2x8_cosine_80e_kitti-3d-3class/dv_second_secfpn_2x8_cosine_80e_kitti-3d-3class_20210831_054106.log.json) |
+| [PointPillars](./dv_pointpillars_secfpn_6x8_160e_kitti-3d-car.py) | Car | cyclic 80e | 4.7 | | 77.76 | [model](https://download.openmmlab.com/mmdetection3d/v0.1.0_models/dynamic_voxelization/dv_pointpillars_secfpn_6x8_160e_kitti-3d-car/dv_pointpillars_secfpn_6x8_160e_kitti-3d-car_20200620_230844-ee7b75c9.pth) \| [log](https://download.openmmlab.com/mmdetection3d/v0.1.0_models/dynamic_voxelization/dv_pointpillars_secfpn_6x8_160e_kitti-3d-car/dv_pointpillars_secfpn_6x8_160e_kitti-3d-car_20200620_230844.log.json) |
+
+## Citation
+
+```latex
+@article{zhou2019endtoend,
+ title={End-to-End Multi-View Fusion for 3D Object Detection in LiDAR Point Clouds},
+ author={Yin Zhou and Pei Sun and Yu Zhang and Dragomir Anguelov and Jiyang Gao and Tom Ouyang and James Guo and Jiquan Ngiam and Vijay Vasudevan},
+ year={2019},
+ eprint={1910.06528},
+ archivePrefix={arXiv},
+ primaryClass={cs.CV}
+}
+```
diff --git a/configs/dynamic_voxelization/dv_pointpillars_secfpn_6x8_160e_kitti-3d-car.py b/configs/dynamic_voxelization/dv_pointpillars_secfpn_6x8_160e_kitti-3d-car.py
new file mode 100644
index 0000000..68baae9
--- /dev/null
+++ b/configs/dynamic_voxelization/dv_pointpillars_secfpn_6x8_160e_kitti-3d-car.py
@@ -0,0 +1,19 @@
+_base_ = '../pointpillars/hv_pointpillars_secfpn_6x8_160e_kitti-3d-car.py'
+
+voxel_size = [0.16, 0.16, 4]
+point_cloud_range = [0, -39.68, -3, 69.12, 39.68, 1]
+
+model = dict(
+ type='DynamicVoxelNet',
+ voxel_layer=dict(
+ max_num_points=-1,
+ point_cloud_range=point_cloud_range,
+ voxel_size=voxel_size,
+ max_voxels=(-1, -1)),
+ voxel_encoder=dict(
+ type='DynamicPillarFeatureNet',
+ in_channels=4,
+ feat_channels=[64],
+ with_distance=False,
+ voxel_size=voxel_size,
+ point_cloud_range=point_cloud_range))
diff --git a/configs/dynamic_voxelization/dv_second_secfpn_2x8_cosine_80e_kitti-3d-3class.py b/configs/dynamic_voxelization/dv_second_secfpn_2x8_cosine_80e_kitti-3d-3class.py
new file mode 100644
index 0000000..87fefad
--- /dev/null
+++ b/configs/dynamic_voxelization/dv_second_secfpn_2x8_cosine_80e_kitti-3d-3class.py
@@ -0,0 +1,22 @@
+_base_ = [
+ '../_base_/models/hv_second_secfpn_kitti.py',
+ '../_base_/datasets/kitti-3d-3class.py', '../_base_/schedules/cosine.py',
+ '../_base_/default_runtime.py'
+]
+
+point_cloud_range = [0, -40, -3, 70.4, 40, 1]
+voxel_size = [0.05, 0.05, 0.1]
+
+model = dict(
+ type='DynamicVoxelNet',
+ voxel_layer=dict(
+ _delete_=True,
+ max_num_points=-1,
+ point_cloud_range=point_cloud_range,
+ voxel_size=voxel_size,
+ max_voxels=(-1, -1)),
+ voxel_encoder=dict(
+ _delete_=True,
+ type='DynamicSimpleVFE',
+ voxel_size=voxel_size,
+ point_cloud_range=point_cloud_range))
diff --git a/configs/dynamic_voxelization/dv_second_secfpn_6x8_80e_kitti-3d-car.py b/configs/dynamic_voxelization/dv_second_secfpn_6x8_80e_kitti-3d-car.py
new file mode 100644
index 0000000..9da4ffe
--- /dev/null
+++ b/configs/dynamic_voxelization/dv_second_secfpn_6x8_80e_kitti-3d-car.py
@@ -0,0 +1,18 @@
+_base_ = '../second/hv_second_secfpn_6x8_80e_kitti-3d-car.py'
+
+point_cloud_range = [0, -40, -3, 70.4, 40, 1]
+voxel_size = [0.05, 0.05, 0.1]
+
+model = dict(
+ type='DynamicVoxelNet',
+ voxel_layer=dict(
+ _delete_=True,
+ max_num_points=-1,
+ point_cloud_range=point_cloud_range,
+ voxel_size=voxel_size,
+ max_voxels=(-1, -1)),
+ voxel_encoder=dict(
+ _delete_=True,
+ type='DynamicSimpleVFE',
+ voxel_size=voxel_size,
+ point_cloud_range=point_cloud_range))
diff --git a/configs/dynamic_voxelization/metafile.yml b/configs/dynamic_voxelization/metafile.yml
new file mode 100644
index 0000000..190c51d
--- /dev/null
+++ b/configs/dynamic_voxelization/metafile.yml
@@ -0,0 +1,53 @@
+Collections:
+ - Name: Dynamic Voxelization
+ Metadata:
+ Training Data: KITTI
+ Training Techniques:
+ - AdamW
+ Training Resources: 8x V100 GPUs
+ Architecture:
+ - Dynamic Voxelization
+ Paper:
+ URL: https://arxiv.org/abs/1910.06528
+ Title: 'End-to-End Multi-View Fusion for 3D Object Detection in LiDAR Point Clouds'
+ README: configs/dynamic_voxelization/README.md
+ Code:
+ URL: https://github.com/open-mmlab/mmdetection3d/blob/master/mmdet3d/models/detectors/dynamic_voxelnet.py#L11
+ Version: v0.5.0
+
+Models:
+ - Name: dv_second_secfpn_6x8_80e_kitti-3d-car
+ In Collection: Dynamic Voxelization
+ Config: configs/dynamic_voxelization/dv_second_secfpn_6x8_80e_kitti-3d-car.py
+ Metadata:
+ Training Memory (GB): 5.5
+ Results:
+ - Task: 3D Object Detection
+ Dataset: KITTI
+ Metrics:
+ mAP: 78.83
+ Weights: https://download.openmmlab.com/mmdetection3d/v0.1.0_models/dynamic_voxelization/dv_second_secfpn_6x8_80e_kitti-3d-car/dv_second_secfpn_6x8_80e_kitti-3d-car_20200620_235228-ac2c1c0c.pth
+
+ - Name: dv_second_secfpn_2x8_cosine_80e_kitti-3d-3class
+ In Collection: Dynamic Voxelization
+ Config: configs/dynamic_voxelization/dv_second_secfpn_2x8_cosine_80e_kitti-3d-3class.py
+ Metadata:
+ Training Memory (GB): 5.5
+ Results:
+ - Task: 3D Object Detection
+ Dataset: KITTI
+ Metrics:
+ mAP: 65.27
+ Weights: https://download.openmmlab.com/mmdetection3d/v1.0.0_models/dynamic_voxelization/dv_second_secfpn_2x8_cosine_80e_kitti-3d-3class/dv_second_secfpn_2x8_cosine_80e_kitti-3d-3class_20210831_054106-e742d163.pth
+
+ - Name: dv_pointpillars_secfpn_6x8_160e_kitti-3d-car
+ In Collection: Dynamic Voxelization
+ Config: configs/dynamic_voxelization/dv_pointpillars_secfpn_6x8_160e_kitti-3d-car.py
+ Metadata:
+ Training Memory (GB): 4.7
+ Results:
+ - Task: 3D Object Detection
+ Dataset: KITTI
+ Metrics:
+ mAP: 77.76
+ Weights: https://download.openmmlab.com/mmdetection3d/v0.1.0_models/dynamic_voxelization/dv_pointpillars_secfpn_6x8_160e_kitti-3d-car/dv_pointpillars_secfpn_6x8_160e_kitti-3d-car_20200620_230844-ee7b75c9.pth
diff --git a/configs/fcaf3d/fcaf3d.py b/configs/fcaf3d/fcaf3d.py
new file mode 100644
index 0000000..9a531e5
--- /dev/null
+++ b/configs/fcaf3d/fcaf3d.py
@@ -0,0 +1,7 @@
+_base_ = ['../_base_/models/fcaf3d.py', '../_base_/default_runtime.py']
+
+optimizer = dict(type='AdamW', lr=0.001, weight_decay=0.0001)
+optimizer_config = dict(grad_clip=dict(max_norm=10, norm_type=2))
+lr_config = dict(policy='step', warmup=None, step=[8, 11])
+runner = dict(type='EpochBasedRunner', max_epochs=12)
+custom_hooks = [dict(type='EmptyCacheHook', after_iter=True)]
diff --git a/configs/fcaf3d/fcaf3d_s3dis-3d-5class.py b/configs/fcaf3d/fcaf3d_s3dis-3d-5class.py
new file mode 100644
index 0000000..1aed8e9
--- /dev/null
+++ b/configs/fcaf3d/fcaf3d_s3dis-3d-5class.py
@@ -0,0 +1,104 @@
+_base_ = ['fcaf3d.py']
+n_points = 100000
+
+model = dict(head=dict(n_classes=5))
+
+dataset_type = 'S3DISDataset'
+data_root = './data/s3dis/'
+class_names = ('table', 'chair', 'sofa', 'bookcase', 'board')
+train_area = [1, 2, 3, 4, 6]
+test_area = 5
+
+train_pipeline = [
+ dict(
+ type='LoadPointsFromFile',
+ coord_type='DEPTH',
+ shift_height=False,
+ use_color=True,
+ load_dim=6,
+ use_dim=[0, 1, 2, 3, 4, 5]),
+ dict(type='LoadAnnotations3D'),
+ dict(type='PointSample', num_points=n_points),
+ dict(
+ type='RandomFlip3D',
+ sync_2d=False,
+ flip_ratio_bev_horizontal=0.5,
+ flip_ratio_bev_vertical=0.5),
+ dict(
+ type='GlobalRotScaleTrans',
+ rot_range=[-0.087266, 0.087266],
+ scale_ratio_range=[.9, 1.1],
+ translation_std=[.1, .1, .1],
+ shift_height=False),
+ dict(type='NormalizePointsColor', color_mean=None),
+ dict(type='DefaultFormatBundle3D', class_names=class_names),
+ dict(type='Collect3D', keys=['points', 'gt_bboxes_3d', 'gt_labels_3d'])
+]
+test_pipeline = [
+ dict(
+ type='LoadPointsFromFile',
+ coord_type='DEPTH',
+ shift_height=False,
+ use_color=True,
+ load_dim=6,
+ use_dim=[0, 1, 2, 3, 4, 5]),
+ dict(
+ type='MultiScaleFlipAug3D',
+ img_scale=(1333, 800),
+ pts_scale_ratio=1,
+ flip=False,
+ transforms=[
+ dict(
+ type='GlobalRotScaleTrans',
+ rot_range=[0, 0],
+ scale_ratio_range=[1., 1.],
+ translation_std=[0, 0, 0]),
+ dict(
+ type='RandomFlip3D',
+ sync_2d=False,
+ flip_ratio_bev_horizontal=0.5,
+ flip_ratio_bev_vertical=0.5),
+ dict(type='PointSample', num_points=n_points),
+ dict(type='NormalizePointsColor', color_mean=None),
+ dict(
+ type='DefaultFormatBundle3D',
+ class_names=class_names,
+ with_label=False),
+ dict(type='Collect3D', keys=['points'])
+ ])
+]
+data = dict(
+ samples_per_gpu=8,
+ workers_per_gpu=4,
+ train=dict(
+ type='RepeatDataset',
+ times=13,
+ dataset=dict(
+ type='ConcatDataset',
+ datasets=[
+ dict(
+ type=dataset_type,
+ data_root=data_root,
+ ann_file=data_root + f's3dis_infos_Area_{i}.pkl',
+ pipeline=train_pipeline,
+ filter_empty_gt=True,
+ classes=class_names,
+ box_type_3d='Depth') for i in train_area
+ ],
+ separate_eval=False)),
+ val=dict(
+ type=dataset_type,
+ data_root=data_root,
+ ann_file=data_root + f's3dis_infos_Area_{test_area}.pkl',
+ pipeline=test_pipeline,
+ classes=class_names,
+ test_mode=True,
+ box_type_3d='Depth'),
+ test=dict(
+ type=dataset_type,
+ data_root=data_root,
+ ann_file=data_root + f's3dis_infos_Area_{test_area}.pkl',
+ pipeline=test_pipeline,
+ classes=class_names,
+ test_mode=True,
+ box_type_3d='Depth'))
diff --git a/configs/fcaf3d/fcaf3d_scannet-3d-18class.py b/configs/fcaf3d/fcaf3d_scannet-3d-18class.py
new file mode 100644
index 0000000..c81a901
--- /dev/null
+++ b/configs/fcaf3d/fcaf3d_scannet-3d-18class.py
@@ -0,0 +1,99 @@
+_base_ = ['fcaf3d.py']
+n_points = 100000
+
+dataset_type = 'ScanNetDataset'
+data_root = './data/scannet/'
+class_names = ('cabinet', 'bed', 'chair', 'sofa', 'table', 'door', 'window',
+ 'bookshelf', 'picture', 'counter', 'desk', 'curtain',
+ 'refrigerator', 'showercurtrain', 'toilet', 'sink', 'bathtub',
+ 'garbagebin')
+train_pipeline = [
+ dict(
+ type='LoadPointsFromFile',
+ coord_type='DEPTH',
+ shift_height=False,
+ use_color=True,
+ load_dim=6,
+ use_dim=[0, 1, 2, 3, 4, 5]),
+ dict(type='LoadAnnotations3D'),
+ dict(type='GlobalAlignment', rotation_axis=2),
+ dict(type='PointSample', num_points=n_points),
+ dict(
+ type='RandomFlip3D',
+ sync_2d=False,
+ flip_ratio_bev_horizontal=0.5,
+ flip_ratio_bev_vertical=0.5),
+ dict(
+ type='GlobalRotScaleTrans',
+ rot_range=[-0.087266, 0.087266],
+ scale_ratio_range=[.9, 1.1],
+ translation_std=[.1, .1, .1],
+ shift_height=False),
+ dict(type='NormalizePointsColor', color_mean=None),
+ dict(type='DefaultFormatBundle3D', class_names=class_names),
+ dict(type='Collect3D', keys=['points', 'gt_bboxes_3d', 'gt_labels_3d'])
+]
+test_pipeline = [
+ dict(
+ type='LoadPointsFromFile',
+ coord_type='DEPTH',
+ shift_height=False,
+ use_color=True,
+ load_dim=6,
+ use_dim=[0, 1, 2, 3, 4, 5]),
+ dict(type='GlobalAlignment', rotation_axis=2),
+ dict(
+ type='MultiScaleFlipAug3D',
+ img_scale=(1333, 800),
+ pts_scale_ratio=1,
+ flip=False,
+ transforms=[
+ dict(
+ type='GlobalRotScaleTrans',
+ rot_range=[0, 0],
+ scale_ratio_range=[1., 1.],
+ translation_std=[0, 0, 0]),
+ dict(
+ type='RandomFlip3D',
+ sync_2d=False,
+ flip_ratio_bev_horizontal=0.5,
+ flip_ratio_bev_vertical=0.5),
+ dict(type='PointSample', num_points=n_points),
+ dict(type='NormalizePointsColor', color_mean=None),
+ dict(
+ type='DefaultFormatBundle3D',
+ class_names=class_names,
+ with_label=False),
+ dict(type='Collect3D', keys=['points'])
+ ])
+]
+data = dict(
+ samples_per_gpu=8,
+ workers_per_gpu=4,
+ train=dict(
+ type='RepeatDataset',
+ times=10,
+ dataset=dict(
+ type=dataset_type,
+ data_root=data_root,
+ ann_file=data_root + 'scannet_infos_train.pkl',
+ pipeline=train_pipeline,
+ filter_empty_gt=True,
+ classes=class_names,
+ box_type_3d='Depth')),
+ val=dict(
+ type=dataset_type,
+ data_root=data_root,
+ ann_file=data_root + 'scannet_infos_val.pkl',
+ pipeline=test_pipeline,
+ classes=class_names,
+ test_mode=True,
+ box_type_3d='Depth'),
+ test=dict(
+ type=dataset_type,
+ data_root=data_root,
+ ann_file=data_root + 'scannet_infos_val.pkl',
+ pipeline=test_pipeline,
+ classes=class_names,
+ test_mode=True,
+ box_type_3d='Depth'))
diff --git a/configs/fcaf3d/fcaf3d_sunrgbd-3d-10class.py b/configs/fcaf3d/fcaf3d_sunrgbd-3d-10class.py
new file mode 100644
index 0000000..6c7c3b4
--- /dev/null
+++ b/configs/fcaf3d/fcaf3d_sunrgbd-3d-10class.py
@@ -0,0 +1,94 @@
+_base_ = ['fcaf3d.py']
+n_points = 100000
+
+model = dict(
+ head=dict(
+ n_classes=10, n_reg_outs=8, bbox_loss=dict(type='RotatedIoU3DLoss')))
+
+dataset_type = 'SUNRGBDDataset'
+data_root = 'data/sunrgbd/'
+class_names = ('bed', 'table', 'sofa', 'chair', 'toilet', 'desk', 'dresser',
+ 'night_stand', 'bookshelf', 'bathtub')
+train_pipeline = [
+ dict(
+ type='LoadPointsFromFile',
+ coord_type='DEPTH',
+ shift_height=False,
+ load_dim=6,
+ use_dim=[0, 1, 2, 3, 4, 5]),
+ dict(type='LoadAnnotations3D'),
+ dict(type='PointSample', num_points=n_points),
+ dict(type='RandomFlip3D', sync_2d=False, flip_ratio_bev_horizontal=0.5),
+ dict(
+ type='GlobalRotScaleTrans',
+ rot_range=[-0.523599, 0.523599],
+ scale_ratio_range=[0.85, 1.15],
+ translation_std=[.1, .1, .1],
+ shift_height=False),
+ dict(type='DefaultFormatBundle3D', class_names=class_names),
+ dict(type='Collect3D', keys=['points', 'gt_bboxes_3d', 'gt_labels_3d'])
+]
+test_pipeline = [
+ dict(
+ type='LoadPointsFromFile',
+ coord_type='DEPTH',
+ shift_height=False,
+ load_dim=6,
+ use_dim=[0, 1, 2, 3, 4, 5]),
+ dict(
+ type='MultiScaleFlipAug3D',
+ img_scale=(1333, 800),
+ pts_scale_ratio=1,
+ flip=False,
+ transforms=[
+ dict(
+ type='GlobalRotScaleTrans',
+ rot_range=[0, 0],
+ scale_ratio_range=[1., 1.],
+ translation_std=[0, 0, 0]),
+ dict(
+ type='RandomFlip3D',
+ sync_2d=False,
+ flip_ratio_bev_horizontal=0.5,
+ flip_ratio_bev_vertical=0.5),
+ dict(type='PointSample', num_points=n_points),
+ dict(
+ type='DefaultFormatBundle3D',
+ class_names=class_names,
+ with_label=False),
+ dict(type='Collect3D', keys=['points'])
+ ])
+]
+data = dict(
+ samples_per_gpu=8,
+ workers_per_gpu=4,
+ train=dict(
+ type='RepeatDataset',
+ times=3,
+ dataset=dict(
+ type=dataset_type,
+ modality=dict(use_camera=False, use_lidar=True),
+ data_root=data_root,
+ ann_file=data_root + 'sunrgbd_infos_train.pkl',
+ pipeline=train_pipeline,
+ filter_empty_gt=True,
+ classes=class_names,
+ box_type_3d='Depth')),
+ val=dict(
+ type=dataset_type,
+ modality=dict(use_camera=False, use_lidar=True),
+ data_root=data_root,
+ ann_file=data_root + 'sunrgbd_infos_val.pkl',
+ pipeline=test_pipeline,
+ classes=class_names,
+ test_mode=True,
+ box_type_3d='Depth'),
+ test=dict(
+ type=dataset_type,
+ modality=dict(use_camera=False, use_lidar=True),
+ data_root=data_root,
+ ann_file=data_root + 'sunrgbd_infos_val.pkl',
+ pipeline=test_pipeline,
+ classes=class_names,
+ test_mode=True,
+ box_type_3d='Depth'))
diff --git a/configs/fcos3d/README.md b/configs/fcos3d/README.md
new file mode 100644
index 0000000..e47a489
--- /dev/null
+++ b/configs/fcos3d/README.md
@@ -0,0 +1,75 @@
+# FCOS3D: Fully Convolutional One-Stage Monocular 3D Object Detection
+
+> [FCOS3D: Fully Convolutional One-Stage Monocular 3D Object Detection](https://arxiv.org/abs/2104.10956)
+
+
+
+## Abstract
+
+Monocular 3D object detection is an important task for autonomous driving considering its advantage of low cost. It is much more challenging than conventional 2D cases due to its inherent ill-posed property, which is mainly reflected in the lack of depth information. Recent progress on 2D detection offers opportunities to better solving this problem. However, it is non-trivial to make a general adapted 2D detector work in this 3D task. In this paper, we study this problem with a practice built on a fully convolutional single-stage detector and propose a general framework FCOS3D. Specifically, we first transform the commonly defined 7-DoF 3D targets to the image domain and decouple them as 2D and 3D attributes. Then the objects are distributed to different feature levels with consideration of their 2D scales and assigned only according to the projected 3D-center for the training procedure. Furthermore, the center-ness is redefined with a 2D Gaussian distribution based on the 3D-center to fit the 3D target formulation. All of these make this framework simple yet effective, getting rid of any 2D detection or 2D-3D correspondence priors. Our solution achieves 1st place out of all the vision-only methods in the nuScenes 3D detection challenge of NeurIPS 2020.
+
+
+
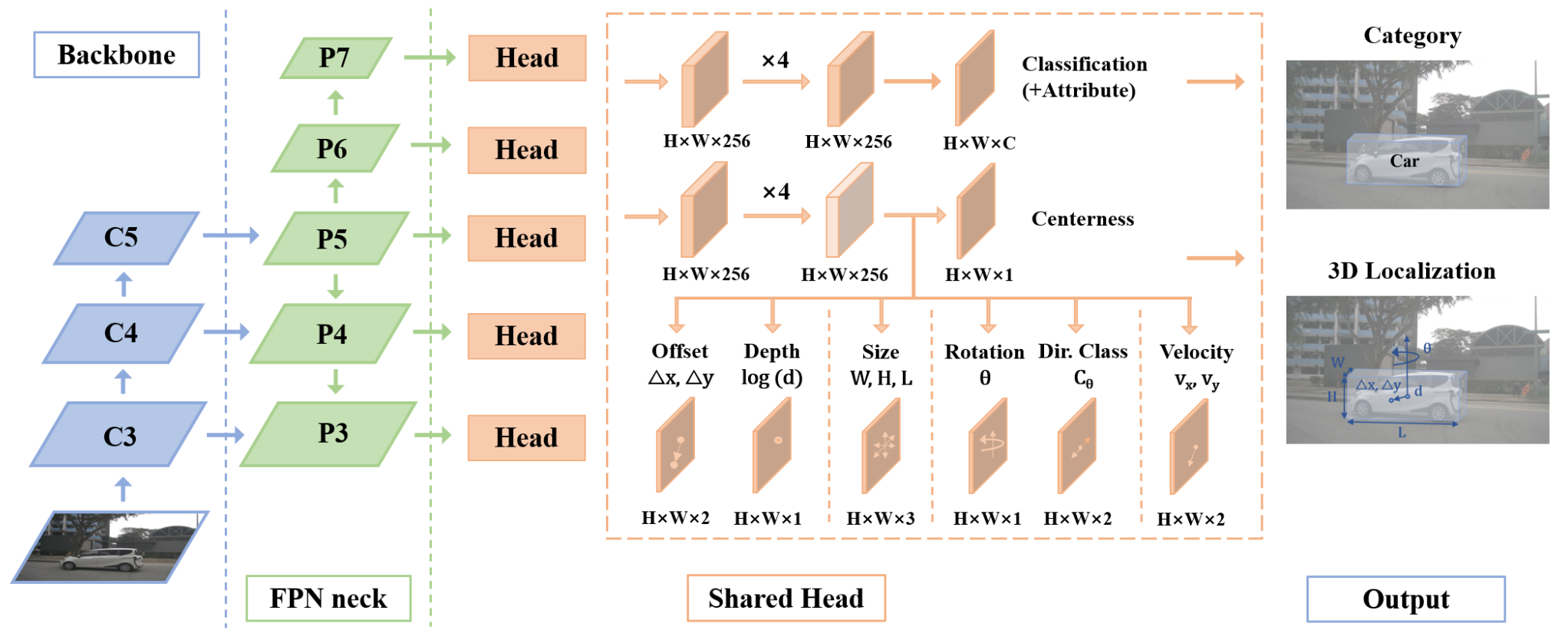
+
+
+## Introduction
+
+FCOS3D is a general anchor-free, one-stage monocular 3D object detector adapted from the original 2D version FCOS.
+It serves as a baseline built on top of mmdetection and mmdetection3d for 3D detection based on monocular vision.
+
+Currently we first support the benchmark on the large-scale nuScenes dataset, which achieved 1st place out of all the vision-only methods in the [nuScenes 3D detecton challenge](https://www.nuscenes.org/object-detection?externalData=all&mapData=all&modalities=Camera) of NeurIPS 2020.
+
+
+
+## Usage
+
+### Data Preparation
+
+After supporting FCOS3D and monocular 3D object detection in v0.13.0, the coco-style 2D json info files will include related annotations by default
+(see [here](https://github.com/open-mmlab/mmdetection3d/blob/master/tools/data_converter/nuscenes_converter.py#L333) if you would like to change the parameter).
+So you can just follow the data preparation steps given in the documentation, then all the needed infos are ready together.
+
+### Training and Inference
+
+The way to training and inference a monocular 3D object detector is the same as others in mmdetection and mmdetection3d. You can basically follow the [documentation](https://mmdetection3d.readthedocs.io/en/latest/1_exist_data_model.html#train-predefined-models-on-standard-datasets) and change the `config`, `work_dirs`, etc. accordingly.
+
+### Test time augmentation
+
+We implement test time augmentation for the dense outputs of detection heads, which is more effective than merging predicted boxes at last.
+You can turn on it by setting `flip=True` in the `test_pipeline`.
+
+### Training with finetune
+
+Due to the scale and measurements of depth is different from those of other regression targets, we first train the model with depth weight equal to 0.2 for a more stable training procedure. For a stronger detector with better performance, please finetune the model with depth weight changed to 1.0 as shown in the [config](./fcos3d_r101_caffe_fpn_gn-head_dcn_2x8_1x_nus-mono3d_finetune.py). Note that the path of `load_from` needs to be changed to yours accordingly.
+
+### Visualizing prediction results
+
+We also provide visualization functions to show the monocular 3D detection results. Simply follow the [documentation](https://mmdetection3d.readthedocs.io/en/latest/1_exist_data_model.html#test-existing-models-on-standard-datasets) and use the `single-gpu testing` command. You only need to add the `--show` flag and specify `--show-dir` to store the visualization results.
+
+## Results and models
+
+### NuScenes
+
+| Backbone | Lr schd | Mem (GB) | Inf time (fps) | mAP | NDS | Download |
+| :------------------------------------------------------------------------------------: | :-----: | :------: | :------------: | :--: | :--: | :--------------------------------------------------------------------------------------------------------------------------------------------------------------------------------------------------------------------------------------------------------------------------------------------------------------------------------------------------------------------------------------------------------------------------------------------------------------: |
+| [ResNet101 w/ DCN](./fcos3d_r101_caffe_fpn_gn-head_dcn_2x8_1x_nus-mono3d.py) | 1x | 8.69 | | 29.8 | 37.7 | [model](https://download.openmmlab.com/mmdetection3d/v0.1.0_models/fcos3d/fcos3d_r101_caffe_fpn_gn-head_dcn_2x8_1x_nus-mono3d/fcos3d_r101_caffe_fpn_gn-head_dcn_2x8_1x_nus-mono3d_20210715_235813-4bed5239.pth) \| [log](https://download.openmmlab.com/mmdetection3d/v0.1.0_models/fcos3d/fcos3d_r101_caffe_fpn_gn-head_dcn_2x8_1x_nus-mono3d/fcos3d_r101_caffe_fpn_gn-head_dcn_2x8_1x_nus-mono3d_20210715_235813.log.json) |
+| [above w/ finetune](./fcos3d_r101_caffe_fpn_gn-head_dcn_2x8_1x_nus-mono3d_finetune.py) | 1x | 8.69 | | 32.1 | 39.5 | [model](https://download.openmmlab.com/mmdetection3d/v0.1.0_models/fcos3d/fcos3d_r101_caffe_fpn_gn-head_dcn_2x8_1x_nus-mono3d_finetune/fcos3d_r101_caffe_fpn_gn-head_dcn_2x8_1x_nus-mono3d_finetune_20210717_095645-8d806dc2.pth) \| [log](https://download.openmmlab.com/mmdetection3d/v0.1.0_models/fcos3d/fcos3d_r101_caffe_fpn_gn-head_dcn_2x8_1x_nus-mono3d_finetune/fcos3d_r101_caffe_fpn_gn-head_dcn_2x8_1x_nus-mono3d_finetune_20210717_095645.log.json) |
+| above w/ tta | 1x | 8.69 | | 33.1 | 40.3 | |
+
+## Citation
+
+```latex
+@inproceedings{wang2021fcos3d,
+ title={{FCOS3D: Fully} Convolutional One-Stage Monocular 3D Object Detection},
+ author={Wang, Tai and Zhu, Xinge and Pang, Jiangmiao and Lin, Dahua},
+ booktitle={Proceedings of the IEEE/CVF International Conference on Computer Vision (ICCV) Workshops},
+ year={2021}
+}
+# For the original 2D version
+@inproceedings{tian2019fcos,
+ title = {{FCOS: Fully} Convolutional One-Stage Object Detection},
+ author = {Tian, Zhi and Shen, Chunhua and Chen, Hao and He, Tong},
+ booktitle = {Proceedings of the IEEE/CVF International Conference on Computer Vision (ICCV)},
+ year = {2019}
+}
+```
diff --git a/configs/fcos3d/fcos3d_r101_caffe_fpn_gn-head_dcn_2x8_1x_nus-mono3d.py b/configs/fcos3d/fcos3d_r101_caffe_fpn_gn-head_dcn_2x8_1x_nus-mono3d.py
new file mode 100644
index 0000000..3b7eb99
--- /dev/null
+++ b/configs/fcos3d/fcos3d_r101_caffe_fpn_gn-head_dcn_2x8_1x_nus-mono3d.py
@@ -0,0 +1,75 @@
+_base_ = [
+ '../_base_/datasets/nus-mono3d.py', '../_base_/models/fcos3d.py',
+ '../_base_/schedules/mmdet_schedule_1x.py', '../_base_/default_runtime.py'
+]
+# model settings
+model = dict(
+ backbone=dict(
+ dcn=dict(type='DCNv2', deform_groups=1, fallback_on_stride=False),
+ stage_with_dcn=(False, False, True, True)))
+
+class_names = [
+ 'car', 'truck', 'trailer', 'bus', 'construction_vehicle', 'bicycle',
+ 'motorcycle', 'pedestrian', 'traffic_cone', 'barrier'
+]
+img_norm_cfg = dict(
+ mean=[103.530, 116.280, 123.675], std=[1.0, 1.0, 1.0], to_rgb=False)
+train_pipeline = [
+ dict(type='LoadImageFromFileMono3D'),
+ dict(
+ type='LoadAnnotations3D',
+ with_bbox=True,
+ with_label=True,
+ with_attr_label=True,
+ with_bbox_3d=True,
+ with_label_3d=True,
+ with_bbox_depth=True),
+ dict(type='Resize', img_scale=(1600, 900), keep_ratio=True),
+ dict(type='RandomFlip3D', flip_ratio_bev_horizontal=0.5),
+ dict(type='Normalize', **img_norm_cfg),
+ dict(type='Pad', size_divisor=32),
+ dict(type='DefaultFormatBundle3D', class_names=class_names),
+ dict(
+ type='Collect3D',
+ keys=[
+ 'img', 'gt_bboxes', 'gt_labels', 'attr_labels', 'gt_bboxes_3d',
+ 'gt_labels_3d', 'centers2d', 'depths'
+ ]),
+]
+test_pipeline = [
+ dict(type='LoadImageFromFileMono3D'),
+ dict(
+ type='MultiScaleFlipAug',
+ scale_factor=1.0,
+ flip=False,
+ transforms=[
+ dict(type='RandomFlip3D'),
+ dict(type='Normalize', **img_norm_cfg),
+ dict(type='Pad', size_divisor=32),
+ dict(
+ type='DefaultFormatBundle3D',
+ class_names=class_names,
+ with_label=False),
+ dict(type='Collect3D', keys=['img']),
+ ])
+]
+data = dict(
+ samples_per_gpu=2,
+ workers_per_gpu=2,
+ train=dict(pipeline=train_pipeline),
+ val=dict(pipeline=test_pipeline),
+ test=dict(pipeline=test_pipeline))
+# optimizer
+optimizer = dict(
+ lr=0.002, paramwise_cfg=dict(bias_lr_mult=2., bias_decay_mult=0.))
+optimizer_config = dict(
+ _delete_=True, grad_clip=dict(max_norm=35, norm_type=2))
+# learning policy
+lr_config = dict(
+ policy='step',
+ warmup='linear',
+ warmup_iters=500,
+ warmup_ratio=1.0 / 3,
+ step=[8, 11])
+total_epochs = 12
+evaluation = dict(interval=2)
diff --git a/configs/fcos3d/fcos3d_r101_caffe_fpn_gn-head_dcn_2x8_1x_nus-mono3d_finetune.py b/configs/fcos3d/fcos3d_r101_caffe_fpn_gn-head_dcn_2x8_1x_nus-mono3d_finetune.py
new file mode 100644
index 0000000..ade5b4e
--- /dev/null
+++ b/configs/fcos3d/fcos3d_r101_caffe_fpn_gn-head_dcn_2x8_1x_nus-mono3d_finetune.py
@@ -0,0 +1,8 @@
+_base_ = './fcos3d_r101_caffe_fpn_gn-head_dcn_2x8_1x_nus-mono3d.py'
+# model settings
+model = dict(
+ train_cfg=dict(
+ code_weight=[1.0, 1.0, 1.0, 1.0, 1.0, 1.0, 1.0, 0.05, 0.05]))
+# optimizer
+optimizer = dict(lr=0.001)
+load_from = 'work_dirs/fcos3d_nus/latest.pth'
diff --git a/configs/fcos3d/metafile.yml b/configs/fcos3d/metafile.yml
new file mode 100644
index 0000000..11de491
--- /dev/null
+++ b/configs/fcos3d/metafile.yml
@@ -0,0 +1,43 @@
+Collections:
+ - Name: FCOS3D
+ Metadata:
+ Training Data: NuScenes
+ Training Techniques:
+ - SGD
+ Training Resources: 8x GeForce RTX 2080 Ti
+ Architecture:
+ - FCOSMono3DHead
+ Paper:
+ URL: https://arxiv.org/abs/2104.10956
+ Title: 'FCOS3D: Fully Convolutional One-Stage Monocular 3D Object Detection'
+ README: configs/fcos3d/README.md
+ Code:
+ URL: https://github.com/open-mmlab/mmdetection3d/blob/master/mmdet3d/models/detectors/fcos_mono3d.py#L7
+ Version: v0.13.0
+
+Models:
+ - Name: fcos3d_r101_caffe_fpn_gn-head_dcn_2x8_1x_nus-mono3d
+ In Collection: FCOS3D
+ Config: configs/fcos3d/fcos3d_r101_caffe_fpn_gn-head_dcn_2x8_1x_nus-mono3d.py
+ Metadata:
+ Training Memory (GB): 8.7
+ Results:
+ - Task: 3D Object Detection
+ Dataset: NuScenes
+ Metrics:
+ mAP: 29.9
+ NDS: 37.3
+ Weights: https://download.openmmlab.com/mmdetection3d/v0.1.0_models/fcos3d/fcos3d_r101_caffe_fpn_gn-head_dcn_2x8_1x_nus-mono3d/fcos3d_r101_caffe_fpn_gn-head_dcn_2x8_1x_nus-mono3d_20210425_181341-8d5a21fe.pth
+
+ - Name: fcos3d_r101_caffe_fpn_gn-head_dcn_2x8_1x_nus-mono3d_finetune
+ In Collection: FCOS3D
+ Config: configs/fcos3d/fcos3d_r101_caffe_fpn_gn-head_dcn_2x8_1x_nus-mono3d_finetune.py
+ Metadata:
+ Training Memory (GB): 8.7
+ Results:
+ - Task: 3D Object Detection
+ Dataset: NuScenes
+ Metrics:
+ mAP: 32.1
+ NDS: 39.3
+ Weights: https://download.openmmlab.com/mmdetection3d/v0.1.0_models/fcos3d/fcos3d_r101_caffe_fpn_gn-head_dcn_2x8_1x_nus-mono3d_finetune/fcos3d_r101_caffe_fpn_gn-head_dcn_2x8_1x_nus-mono3d_finetune_20210427_091419-35aaaad0.pth
diff --git a/configs/free_anchor/README.md b/configs/free_anchor/README.md
new file mode 100644
index 0000000..727a700
--- /dev/null
+++ b/configs/free_anchor/README.md
@@ -0,0 +1,105 @@
+# FreeAnchor for 3D Object Detection
+
+> [FreeAnchor: Learning to Match Anchors for Visual Object Detection](https://arxiv.org/abs/1909.02466)
+
+
+
+## Abstract
+
+Modern CNN-based object detectors assign anchors for ground-truth objects under the restriction of object-anchor Intersection-over-Unit (IoU). In this study, we propose a learning-to-match approach to break IoU restriction, allowing objects to match anchors in a flexible manner. Our approach, referred to as FreeAnchor, updates hand-crafted anchor assignment to “free" anchor matching by formulating detector training as a maximum likelihood estimation (MLE) procedure. FreeAnchor targets at learning features which best explain a class of objects in terms of both classification and localization. FreeAnchor is implemented by optimizing detection customized likelihood and can be fused with CNN-based detectors in a plug-and-play manner. Experiments on COCO demonstrate that FreeAnchor consistently outperforms the counterparts with significant margins.
+
+
+
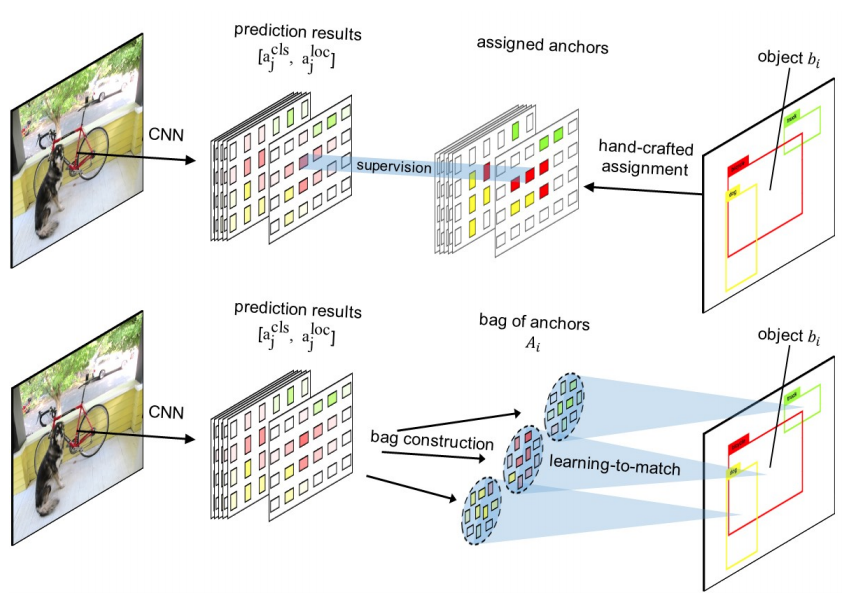
+
+
+## Introduction
+
+We implement FreeAnchor in 3D detection systems and provide their first results with PointPillars on nuScenes dataset.
+With the implemented `FreeAnchor3DHead`, a PointPillar detector with a big backbone (e.g., RegNet-3.2GF) achieves top performance
+on the nuScenes benchmark.
+
+## Usage
+
+### Modify config
+
+As in the [baseline config](hv_pointpillars_fpn_sbn-all_free-anchor_4x8_2x_nus-3d.py), we only need to replace the head of an existing one-stage detector to use FreeAnchor head.
+Since the config is inherit from a common detector head, `_delete_=True` is necessary to avoid conflicts.
+The hyperparameters are specifically tuned according to the original paper.
+
+```python
+_base_ = [
+ '../_base_/models/hv_pointpillars_fpn_lyft.py',
+ '../_base_/datasets/nus-3d.py', '../_base_/schedules/schedule_2x.py',
+ '../_base_/default_runtime.py'
+]
+
+model = dict(
+ pts_bbox_head=dict(
+ _delete_=True,
+ type='FreeAnchor3DHead',
+ num_classes=10,
+ in_channels=256,
+ feat_channels=256,
+ use_direction_classifier=True,
+ pre_anchor_topk=25,
+ bbox_thr=0.5,
+ gamma=2.0,
+ alpha=0.5,
+ anchor_generator=dict(
+ type='AlignedAnchor3DRangeGenerator',
+ ranges=[[-50, -50, -1.8, 50, 50, -1.8]],
+ scales=[1, 2, 4],
+ sizes=[
+ [2.5981, 0.8660, 1.], # 1.5 / sqrt(3)
+ [1.7321, 0.5774, 1.], # 1 / sqrt(3)
+ [1., 1., 1.],
+ [0.4, 0.4, 1],
+ ],
+ custom_values=[0, 0],
+ rotations=[0, 1.57],
+ reshape_out=True),
+ assigner_per_size=False,
+ diff_rad_by_sin=True,
+ dir_offset=-0.7854, # -pi / 4
+ bbox_coder=dict(type='DeltaXYZWLHRBBoxCoder', code_size=9),
+ loss_cls=dict(
+ type='FocalLoss',
+ use_sigmoid=True,
+ gamma=2.0,
+ alpha=0.25,
+ loss_weight=1.0),
+ loss_bbox=dict(type='SmoothL1Loss', beta=1.0 / 9.0, loss_weight=0.8),
+ loss_dir=dict(
+ type='CrossEntropyLoss', use_sigmoid=False, loss_weight=0.2)),
+ # model training and testing settings
+ train_cfg = dict(
+ pts=dict(code_weight=[1.0, 1.0, 1.0, 1.0, 1.0, 1.0, 1.0, 0.25, 0.25])))
+```
+
+## Results and models
+
+### PointPillars
+
+| Backbone | FreeAnchor | Lr schd | Mem (GB) | Inf time (fps) | mAP | NDS | Download |
+| :-------------------------------------------------------------------------------------------------------: | :--------: | :-----: | :------: | :------------: | :---: | :---: | :--------------------------------------------------------------------------------------------------------------------------------------------------------------------------------------------------------------------------------------------------------------------------------------------------------------------------------------------------------------------------------------------------------------------------------------------------------------------------------------------------------------------------------------------: |
+| [FPN](../pointpillars/hv_pointpillars_fpn_sbn-all_4x8_2x_nus-3d.py) | ✗ | 2x | 17.1 | | 40.0 | 53.3 | [model](https://download.openmmlab.com/mmdetection3d/v0.1.0_models/pointpillars/hv_pointpillars_fpn_sbn-all_4x8_2x_nus-3d/hv_pointpillars_fpn_sbn-all_4x8_2x_nus-3d_20200620_230405-2fa62f3d.pth) \| [log](https://download.openmmlab.com/mmdetection3d/v0.1.0_models/pointpillars/hv_pointpillars_fpn_sbn-all_4x8_2x_nus-3d/hv_pointpillars_fpn_sbn-all_4x8_2x_nus-3d_20200620_230405.log.json) |
+| [FPN](./hv_pointpillars_fpn_sbn-all_free-anchor_4x8_2x_nus-3d.py) | ✓ | 2x | 16.3 | | 43.82 | 54.86 | [model](https://download.openmmlab.com/mmdetection3d/v1.0.0_models/free_anchor/hv_pointpillars_fpn_sbn-all_free-anchor_4x8_2x_nus-3d/hv_pointpillars_fpn_sbn-all_free-anchor_4x8_2x_nus-3d_20210816_163441-ae0897e7.pth) \| [log](https://download.openmmlab.com/mmdetection3d/v1.0.0_models/free_anchor/hv_pointpillars_fpn_sbn-all_free-anchor_4x8_2x_nus-3d/hv_pointpillars_fpn_sbn-all_free-anchor_4x8_2x_nus-3d_20210816_163441.log.json) |
+| [RegNetX-400MF-FPN](../regnet/hv_pointpillars_regnet-400mf_fpn_sbn-all_4x8_2x_nus-3d.py) | ✗ | 2x | 17.3 | | 44.8 | 56.4 | [model](https://download.openmmlab.com/mmdetection3d/v0.1.0_models/regnet/hv_pointpillars_regnet-400mf_fpn_sbn-all_4x8_2x_nus-3d/hv_pointpillars_regnet-400mf_fpn_sbn-all_4x8_2x_nus-3d_20200620_230239-c694dce7.pth) \| [log](https://download.openmmlab.com/mmdetection3d/v0.1.0_models/regnet/hv_pointpillars_regnet-400mf_fpn_sbn-all_4x8_2x_nus-3d/hv_pointpillars_regnet-400mf_fpn_sbn-all_4x8_2x_nus-3d_20200620_230239.log.json) |
+| [RegNetX-400MF-FPN](./hv_pointpillars_regnet-400mf_fpn_sbn-all_free-anchor_4x8_2x_nus-3d.py) | ✓ | 2x | 17.6 | | 48.3 | 58.65 | [model](https://download.openmmlab.com/mmdetection3d/v1.0.0_models/free_anchor/hv_pointpillars_regnet-400mf_fpn_sbn-all_free-anchor_4x8_2x_nus-3d/hv_pointpillars_regnet-400mf_fpn_sbn-all_free-anchor_4x8_2x_nus-3d_20210827_213939-a2dd3fff.pth) \| [log](https://download.openmmlab.com/mmdetection3d/v1.0.0_models/free_anchor/hv_pointpillars_regnet-400mf_fpn_sbn-all_free-anchor_4x8_2x_nus-3d/hv_pointpillars_regnet-400mf_fpn_sbn-all_free-anchor_4x8_2x_nus-3d_20210827_213939.log.json) |
+| [RegNetX-1.6GF-FPN](./hv_pointpillars_regnet-1.6gf_fpn_sbn-all_free-anchor_4x8_2x_nus-3d.py) | ✓ | 2x | 24.3 | | 52.04 | 61.49 | [model](https://download.openmmlab.com/mmdetection3d/v1.0.0_models/free_anchor/hv_pointpillars_regnet-1.6gf_fpn_sbn-all_free-anchor_4x8_2x_nus-3d/hv_pointpillars_regnet-1.6gf_fpn_sbn-all_free-anchor_4x8_2x_nus-3d_20210828_025608-bfbd506e.pth) \| [log](https://download.openmmlab.com/mmdetection3d/v1.0.0_models/free_anchor/hv_pointpillars_regnet-1.6gf_fpn_sbn-all_free-anchor_4x8_2x_nus-3d/hv_pointpillars_regnet-1.6gf_fpn_sbn-all_free-anchor_4x8_2x_nus-3d_20210828_025608.log.json) |
+| [RegNetX-1.6GF-FPN](./hv_pointpillars_regnet-1.6gf_fpn_sbn-all_free-anchor_strong-aug_4x8_3x_nus-3d.py)\* | ✓ | 3x | 24.4 | | 52.69 | 62.45 | [model](https://download.openmmlab.com/mmdetection3d/v1.0.0_models/free_anchor/hv_pointpillars_regnet-1.6gf_fpn_sbn-all_free-anchor_strong-aug_4x8_3x_nus-3d/hv_pointpillars_regnet-1.6gf_fpn_sbn-all_free-anchor_strong-aug_4x8_3x_nus-3d_20210827_184909-14d2dbd1.pth) \| [log](https://download.openmmlab.com/mmdetection3d/v1.0.0_models/free_anchor/hv_pointpillars_regnet-1.6gf_fpn_sbn-all_free-anchor_strong-aug_4x8_3x_nus-3d/hv_pointpillars_regnet-1.6gf_fpn_sbn-all_free-anchor_strong-aug_4x8_3x_nus-3d_20210827_184909.log.json) |
+| [RegNetX-3.2GF-FPN](./hv_pointpillars_regnet-3.2gf_fpn_sbn-all_free-anchor_4x8_2x_nus-3d.py) | ✓ | 2x | 29.4 | | 52.4 | 61.94 | [model](https://download.openmmlab.com/mmdetection3d/v1.0.0_models/free_anchor/hv_pointpillars_regnet-3.2gf_fpn_sbn-all_free-anchor_4x8_2x_nus-3d/hv_pointpillars_regnet-3.2gf_fpn_sbn-all_free-anchor_4x8_2x_nus-3d_20210827_181237-e385c35a.pth) \| [log](https://download.openmmlab.com/mmdetection3d/v1.0.0_models/free_anchor/hv_pointpillars_regnet-3.2gf_fpn_sbn-all_free-anchor_4x8_2x_nus-3d/hv_pointpillars_regnet-3.2gf_fpn_sbn-all_free-anchor_4x8_2x_nus-3d_20210827_181237.log.json) |
+| [RegNetX-3.2GF-FPN](./hv_pointpillars_regnet-3.2gf_fpn_sbn-all_free-anchor_strong-aug_4x8_3x_nus-3d.py)\* | ✓ | 3x | 29.2 | | 54.23 | 63.41 | [model](https://download.openmmlab.com/mmdetection3d/v1.0.0_models/free_anchor/hv_pointpillars_regnet-3.2gf_fpn_sbn-all_free-anchor_strong-aug_4x8_3x_nus-3d/hv_pointpillars_regnet-3.2gf_fpn_sbn-all_free-anchor_strong-aug_4x8_3x_nus-3d_20210828_030816-06708918.pth) \| [log](https://download.openmmlab.com/mmdetection3d/v1.0.0_models/free_anchor/hv_pointpillars_regnet-3.2gf_fpn_sbn-all_free-anchor_strong-aug_4x8_3x_nus-3d/hv_pointpillars_regnet-3.2gf_fpn_sbn-all_free-anchor_strong-aug_4x8_3x_nus-3d_20210828_030816.log.json) |
+
+**Note**: Models noted by `*` means it is trained using stronger augmentation with vertical flip under bird-eye-view, global translation, and larger range of global rotation.
+
+## Citation
+
+```latex
+@inproceedings{zhang2019freeanchor,
+ title = {{FreeAnchor}: Learning to Match Anchors for Visual Object Detection},
+ author = {Zhang, Xiaosong and Wan, Fang and Liu, Chang and Ji, Rongrong and Ye, Qixiang},
+ booktitle = {Neural Information Processing Systems},
+ year = {2019}
+}
+```
diff --git a/configs/free_anchor/hv_pointpillars_fpn_sbn-all_free-anchor_4x8_2x_nus-3d.py b/configs/free_anchor/hv_pointpillars_fpn_sbn-all_free-anchor_4x8_2x_nus-3d.py
new file mode 100644
index 0000000..7412b93
--- /dev/null
+++ b/configs/free_anchor/hv_pointpillars_fpn_sbn-all_free-anchor_4x8_2x_nus-3d.py
@@ -0,0 +1,47 @@
+_base_ = [
+ '../_base_/models/hv_pointpillars_fpn_nus.py',
+ '../_base_/datasets/nus-3d.py', '../_base_/schedules/schedule_2x.py',
+ '../_base_/default_runtime.py'
+]
+
+model = dict(
+ pts_bbox_head=dict(
+ _delete_=True,
+ type='FreeAnchor3DHead',
+ num_classes=10,
+ in_channels=256,
+ feat_channels=256,
+ use_direction_classifier=True,
+ pre_anchor_topk=25,
+ bbox_thr=0.5,
+ gamma=2.0,
+ alpha=0.5,
+ anchor_generator=dict(
+ type='AlignedAnchor3DRangeGenerator',
+ ranges=[[-50, -50, -1.8, 50, 50, -1.8]],
+ scales=[1, 2, 4],
+ sizes=[
+ [2.5981, 0.8660, 1.], # 1.5 / sqrt(3)
+ [1.7321, 0.5774, 1.], # 1 / sqrt(3)
+ [1., 1., 1.],
+ [0.4, 0.4, 1],
+ ],
+ custom_values=[0, 0],
+ rotations=[0, 1.57],
+ reshape_out=True),
+ assigner_per_size=False,
+ diff_rad_by_sin=True,
+ dir_offset=-0.7854, # -pi / 4
+ bbox_coder=dict(type='DeltaXYZWLHRBBoxCoder', code_size=9),
+ loss_cls=dict(
+ type='FocalLoss',
+ use_sigmoid=True,
+ gamma=2.0,
+ alpha=0.25,
+ loss_weight=1.0),
+ loss_bbox=dict(type='SmoothL1Loss', beta=1.0 / 9.0, loss_weight=0.8),
+ loss_dir=dict(
+ type='CrossEntropyLoss', use_sigmoid=False, loss_weight=0.2)),
+ # model training and testing settings
+ train_cfg=dict(
+ pts=dict(code_weight=[1.0, 1.0, 1.0, 1.0, 1.0, 1.0, 1.0, 0.25, 0.25])))
diff --git a/configs/free_anchor/hv_pointpillars_regnet-1.6gf_fpn_sbn-all_free-anchor_4x8_2x_nus-3d.py b/configs/free_anchor/hv_pointpillars_regnet-1.6gf_fpn_sbn-all_free-anchor_4x8_2x_nus-3d.py
new file mode 100644
index 0000000..ef740a8
--- /dev/null
+++ b/configs/free_anchor/hv_pointpillars_regnet-1.6gf_fpn_sbn-all_free-anchor_4x8_2x_nus-3d.py
@@ -0,0 +1,18 @@
+_base_ = './hv_pointpillars_fpn_sbn-all_free-anchor_4x8_2x_nus-3d.py'
+
+model = dict(
+ pts_backbone=dict(
+ _delete_=True,
+ type='NoStemRegNet',
+ arch='regnetx_1.6gf',
+ init_cfg=dict(
+ type='Pretrained', checkpoint='open-mmlab://regnetx_1.6gf'),
+ out_indices=(1, 2, 3),
+ frozen_stages=-1,
+ strides=(1, 2, 2, 2),
+ base_channels=64,
+ stem_channels=64,
+ norm_cfg=dict(type='naiveSyncBN2d', eps=1e-3, momentum=0.01),
+ norm_eval=False,
+ style='pytorch'),
+ pts_neck=dict(in_channels=[168, 408, 912]))
diff --git a/configs/free_anchor/hv_pointpillars_regnet-1.6gf_fpn_sbn-all_free-anchor_strong-aug_4x8_3x_nus-3d.py b/configs/free_anchor/hv_pointpillars_regnet-1.6gf_fpn_sbn-all_free-anchor_strong-aug_4x8_3x_nus-3d.py
new file mode 100644
index 0000000..d4e48d3
--- /dev/null
+++ b/configs/free_anchor/hv_pointpillars_regnet-1.6gf_fpn_sbn-all_free-anchor_strong-aug_4x8_3x_nus-3d.py
@@ -0,0 +1,70 @@
+_base_ = './hv_pointpillars_fpn_sbn-all_free-anchor_4x8_2x_nus-3d.py'
+
+model = dict(
+ pts_backbone=dict(
+ _delete_=True,
+ type='NoStemRegNet',
+ arch='regnetx_1.6gf',
+ init_cfg=dict(
+ type='Pretrained', checkpoint='open-mmlab://regnetx_1.6gf'),
+ out_indices=(1, 2, 3),
+ frozen_stages=-1,
+ strides=(1, 2, 2, 2),
+ base_channels=64,
+ stem_channels=64,
+ norm_cfg=dict(type='naiveSyncBN2d', eps=1e-3, momentum=0.01),
+ norm_eval=False,
+ style='pytorch'),
+ pts_neck=dict(in_channels=[168, 408, 912]))
+
+# If point cloud range is changed, the models should also change their point
+# cloud range accordingly
+point_cloud_range = [-50, -50, -5, 50, 50, 3]
+# For nuScenes we usually do 10-class detection
+class_names = [
+ 'car', 'truck', 'trailer', 'bus', 'construction_vehicle', 'bicycle',
+ 'motorcycle', 'pedestrian', 'traffic_cone', 'barrier'
+]
+file_client_args = dict(backend='disk')
+# Uncomment the following if use ceph or other file clients.
+# See https://mmcv.readthedocs.io/en/latest/api.html#mmcv.fileio.FileClient
+# for more details.
+# file_client_args = dict(
+# backend='petrel',
+# path_mapping=dict({
+# './data/nuscenes/': 's3://nuscenes/nuscenes/',
+# 'data/nuscenes/': 's3://nuscenes/nuscenes/'
+# }))
+train_pipeline = [
+ dict(
+ type='LoadPointsFromFile',
+ coord_type='LIDAR',
+ load_dim=5,
+ use_dim=5,
+ file_client_args=file_client_args),
+ dict(
+ type='LoadPointsFromMultiSweeps',
+ sweeps_num=10,
+ file_client_args=file_client_args),
+ dict(type='LoadAnnotations3D', with_bbox_3d=True, with_label_3d=True),
+ dict(
+ type='GlobalRotScaleTrans',
+ rot_range=[-0.7854, 0.7854],
+ scale_ratio_range=[0.95, 1.05],
+ translation_std=[0.2, 0.2, 0.2]),
+ dict(
+ type='RandomFlip3D',
+ flip_ratio_bev_horizontal=0.5,
+ flip_ratio_bev_vertical=0.5),
+ dict(type='PointsRangeFilter', point_cloud_range=point_cloud_range),
+ dict(type='ObjectRangeFilter', point_cloud_range=point_cloud_range),
+ dict(type='ObjectNameFilter', classes=class_names),
+ dict(type='PointShuffle'),
+ dict(type='DefaultFormatBundle3D', class_names=class_names),
+ dict(type='Collect3D', keys=['points', 'gt_bboxes_3d', 'gt_labels_3d'])
+]
+data = dict(train=dict(pipeline=train_pipeline))
+
+lr_config = dict(step=[28, 34])
+runner = dict(max_epochs=36)
+evaluation = dict(interval=36)
diff --git a/configs/free_anchor/hv_pointpillars_regnet-3.2gf_fpn_sbn-all_free-anchor_4x8_2x_nus-3d.py b/configs/free_anchor/hv_pointpillars_regnet-3.2gf_fpn_sbn-all_free-anchor_4x8_2x_nus-3d.py
new file mode 100644
index 0000000..13bc0d6
--- /dev/null
+++ b/configs/free_anchor/hv_pointpillars_regnet-3.2gf_fpn_sbn-all_free-anchor_4x8_2x_nus-3d.py
@@ -0,0 +1,18 @@
+_base_ = './hv_pointpillars_fpn_sbn-all_free-anchor_4x8_2x_nus-3d.py'
+
+model = dict(
+ pts_backbone=dict(
+ _delete_=True,
+ type='NoStemRegNet',
+ arch='regnetx_3.2gf',
+ init_cfg=dict(
+ type='Pretrained', checkpoint='open-mmlab://regnetx_3.2gf'),
+ out_indices=(1, 2, 3),
+ frozen_stages=-1,
+ strides=(1, 2, 2, 2),
+ base_channels=64,
+ stem_channels=64,
+ norm_cfg=dict(type='naiveSyncBN2d', eps=1e-3, momentum=0.01),
+ norm_eval=False,
+ style='pytorch'),
+ pts_neck=dict(in_channels=[192, 432, 1008]))
diff --git a/configs/free_anchor/hv_pointpillars_regnet-3.2gf_fpn_sbn-all_free-anchor_strong-aug_4x8_3x_nus-3d.py b/configs/free_anchor/hv_pointpillars_regnet-3.2gf_fpn_sbn-all_free-anchor_strong-aug_4x8_3x_nus-3d.py
new file mode 100644
index 0000000..6fbce89
--- /dev/null
+++ b/configs/free_anchor/hv_pointpillars_regnet-3.2gf_fpn_sbn-all_free-anchor_strong-aug_4x8_3x_nus-3d.py
@@ -0,0 +1,70 @@
+_base_ = './hv_pointpillars_fpn_sbn-all_free-anchor_4x8_2x_nus-3d.py'
+
+model = dict(
+ pts_backbone=dict(
+ _delete_=True,
+ type='NoStemRegNet',
+ arch='regnetx_3.2gf',
+ init_cfg=dict(
+ type='Pretrained', checkpoint='open-mmlab://regnetx_3.2gf'),
+ out_indices=(1, 2, 3),
+ frozen_stages=-1,
+ strides=(1, 2, 2, 2),
+ base_channels=64,
+ stem_channels=64,
+ norm_cfg=dict(type='naiveSyncBN2d', eps=1e-3, momentum=0.01),
+ norm_eval=False,
+ style='pytorch'),
+ pts_neck=dict(in_channels=[192, 432, 1008]))
+
+# If point cloud range is changed, the models should also change their point
+# cloud range accordingly
+point_cloud_range = [-50, -50, -5, 50, 50, 3]
+# For nuScenes we usually do 10-class detection
+class_names = [
+ 'car', 'truck', 'trailer', 'bus', 'construction_vehicle', 'bicycle',
+ 'motorcycle', 'pedestrian', 'traffic_cone', 'barrier'
+]
+file_client_args = dict(backend='disk')
+# Uncomment the following if use ceph or other file clients.
+# See https://mmcv.readthedocs.io/en/latest/api.html#mmcv.fileio.FileClient
+# for more details.
+# file_client_args = dict(
+# backend='petrel',
+# path_mapping=dict({
+# './data/nuscenes/': 's3://nuscenes/nuscenes/',
+# 'data/nuscenes/': 's3://nuscenes/nuscenes/'
+# }))
+train_pipeline = [
+ dict(
+ type='LoadPointsFromFile',
+ coord_type='LIDAR',
+ load_dim=5,
+ use_dim=5,
+ file_client_args=file_client_args),
+ dict(
+ type='LoadPointsFromMultiSweeps',
+ sweeps_num=10,
+ file_client_args=file_client_args),
+ dict(type='LoadAnnotations3D', with_bbox_3d=True, with_label_3d=True),
+ dict(
+ type='GlobalRotScaleTrans',
+ rot_range=[-0.7854, 0.7854],
+ scale_ratio_range=[0.9, 1.1],
+ translation_std=[0.2, 0.2, 0.2]),
+ dict(
+ type='RandomFlip3D',
+ flip_ratio_bev_horizontal=0.5,
+ flip_ratio_bev_vertical=0.5),
+ dict(type='PointsRangeFilter', point_cloud_range=point_cloud_range),
+ dict(type='ObjectRangeFilter', point_cloud_range=point_cloud_range),
+ dict(type='ObjectNameFilter', classes=class_names),
+ dict(type='PointShuffle'),
+ dict(type='DefaultFormatBundle3D', class_names=class_names),
+ dict(type='Collect3D', keys=['points', 'gt_bboxes_3d', 'gt_labels_3d'])
+]
+
+data = dict(train=dict(pipeline=train_pipeline))
+lr_config = dict(step=[28, 34])
+runner = dict(max_epochs=36)
+evaluation = dict(interval=36)
diff --git a/configs/free_anchor/hv_pointpillars_regnet-400mf_fpn_sbn-all_free-anchor_4x8_2x_nus-3d.py b/configs/free_anchor/hv_pointpillars_regnet-400mf_fpn_sbn-all_free-anchor_4x8_2x_nus-3d.py
new file mode 100644
index 0000000..2b5f254
--- /dev/null
+++ b/configs/free_anchor/hv_pointpillars_regnet-400mf_fpn_sbn-all_free-anchor_4x8_2x_nus-3d.py
@@ -0,0 +1,18 @@
+_base_ = './hv_pointpillars_fpn_sbn-all_free-anchor_4x8_2x_nus-3d.py'
+
+model = dict(
+ pts_backbone=dict(
+ _delete_=True,
+ type='NoStemRegNet',
+ arch='regnetx_400mf',
+ init_cfg=dict(
+ type='Pretrained', checkpoint='open-mmlab://regnetx_400mf'),
+ out_indices=(1, 2, 3),
+ frozen_stages=-1,
+ strides=(1, 2, 2, 2),
+ base_channels=64,
+ stem_channels=64,
+ norm_cfg=dict(type='naiveSyncBN2d', eps=1e-3, momentum=0.01),
+ norm_eval=False,
+ style='pytorch'),
+ pts_neck=dict(in_channels=[64, 160, 384]))
diff --git a/configs/free_anchor/metafile.yml b/configs/free_anchor/metafile.yml
new file mode 100644
index 0000000..73b55f5
--- /dev/null
+++ b/configs/free_anchor/metafile.yml
@@ -0,0 +1,96 @@
+Collections:
+ - Name: FreeAnchor
+ Metadata:
+ Training Data: nuScenes
+ Training Techniques:
+ - AdamW
+ Training Resources: 8x V100 GPUs
+ Architecture:
+ - Hard Voxelization
+ - Free Anchor
+ Paper:
+ URL: https://arxiv.org/abs/1909.02466
+ Title: 'FreeAnchor: Learning to Match Anchors for Visual Object Detection'
+ README: configs/free_anchor/README.md
+ Code:
+ URL: https://github.com/open-mmlab/mmdetection3d/blob/master/mmdet3d/models/dense_heads/free_anchor3d_head.py#L13
+ Version: v0.5.0
+
+Models:
+ - Name: hv_pointpillars_fpn_sbn-all_free-anchor_4x8_2x_nus-3d
+ In Collection: FreeAnchor
+ Config: free_anchor/hv_pointpillars_fpn_sbn-all_free-anchor_4x8_2x_nus-3d.py
+ Metadata:
+ Training Memory (GB): 16.3
+ Results:
+ - Task: 3D Object Detection
+ Dataset: nuScenes
+ Metrics:
+ mAP: 43.82
+ NDS: 54.86
+ Weights: https://download.openmmlab.com/mmdetection3d/v1.0.0_models/free_anchor/hv_pointpillars_fpn_sbn-all_free-anchor_4x8_2x_nus-3d/hv_pointpillars_fpn_sbn-all_free-anchor_4x8_2x_nus-3d_20210816_163441-ae0897e7.pth
+
+ - Name: hv_pointpillars_regnet-400mf_fpn_sbn-all_free-anchor_4x8_2x_nus-3d
+ In Collection: FreeAnchor
+ Config: configs/free_anchor/hv_pointpillars_regnet-400mf_fpn_sbn-all_free-anchor_4x8_2x_nus-3d.py
+ Metadata:
+ Training Memory (GB): 17.6
+ Results:
+ - Task: 3D Object Detection
+ Dataset: nuScenes
+ Metrics:
+ mAP: 48.3
+ NDS: 58.65
+ Weights: https://download.openmmlab.com/mmdetection3d/v1.0.0_models/free_anchor/hv_pointpillars_regnet-400mf_fpn_sbn-all_free-anchor_4x8_2x_nus-3d/hv_pointpillars_regnet-400mf_fpn_sbn-all_free-anchor_4x8_2x_nus-3d_20210827_213939-a2dd3fff.pth
+
+ - Name: hv_pointpillars_regnet-1.6gf_fpn_sbn-all_free-anchor_4x8_2x_nus-3d
+ In Collection: FreeAnchor
+ Config: configs/free_anchor/hv_pointpillars_regnet-1.6gf_fpn_sbn-all_free-anchor_4x8_2x_nus-3d.py
+ Metadata:
+ Training Memory (GB): 24.3
+ Results:
+ - Task: 3D Object Detection
+ Dataset: nuScenes
+ Metrics:
+ mAP: 52.04
+ NDS: 61.49
+ Weights: https://download.openmmlab.com/mmdetection3d/v1.0.0_models/free_anchor/hv_pointpillars_regnet-1.6gf_fpn_sbn-all_free-anchor_4x8_2x_nus-3d/hv_pointpillars_regnet-1.6gf_fpn_sbn-all_free-anchor_4x8_2x_nus-3d_20210828_025608-bfbd506e.pth
+
+ - Name: hv_pointpillars_regnet-1.6gf_fpn_sbn-all_free-anchor_strong-aug_4x8_3x_nus-3d
+ In Collection: FreeAnchor
+ Config: configs/free_anchor/hv_pointpillars_regnet-1.6gf_fpn_sbn-all_free-anchor_strong-aug_4x8_3x_nus-3d.py
+ Metadata:
+ Training Memory (GB): 24.4
+ Results:
+ - Task: 3D Object Detection
+ Dataset: nuScenes
+ Metrics:
+ mAP: 52.69
+ NDS: 62.45
+ Weights: https://download.openmmlab.com/mmdetection3d/v1.0.0_models/free_anchor/hv_pointpillars_regnet-1.6gf_fpn_sbn-all_free-anchor_strong-aug_4x8_3x_nus-3d/hv_pointpillars_regnet-1.6gf_fpn_sbn-all_free-anchor_strong-aug_4x8_3x_nus-3d_20210827_184909-14d2dbd1.pth
+
+ - Name: hv_pointpillars_regnet-3.2gf_fpn_sbn-all_free-anchor_4x8_2x_nus-3d
+ In Collection: FreeAnchor
+ Config: configs/free_anchor/hv_pointpillars_regnet-3.2gf_fpn_sbn-all_free-anchor_4x8_2x_nus-3d.py
+ Metadata:
+ Training Memory (GB): 29.4
+ Results:
+ - Task: 3D Object Detection
+ Dataset: nuScenes
+ Metrics:
+ mAP: 52.4
+ NDS: 61.94
+ Weights: https://download.openmmlab.com/mmdetection3d/v1.0.0_models/free_anchor/hv_pointpillars_regnet-3.2gf_fpn_sbn-all_free-anchor_4x8_2x_nus-3d/hv_pointpillars_regnet-3.2gf_fpn_sbn-all_free-anchor_4x8_2x_nus-3d_20210827_181237-e385c35a.pth
+
+ - Name: hv_pointpillars_regnet-3.2gf_fpn_sbn-all_free-anchor_strong-aug_4x8_3x_nus-3d
+ In Collection: FreeAnchor
+ Config: configs/free_anchor/hv_pointpillars_regnet-3.2gf_fpn_sbn-all_free-anchor_strong-aug_4x8_3x_nus-3d.py
+ Metadata:
+ Training Memory (GB): 29.2
+ Results:
+ - Task: 3D Object Detection
+ Dataset: nuScenes
+ Metrics:
+ mAP: 54.23
+ NDS: 63.41
+ Weights: https://download.openmmlab.com/mmdetection3d/v1.0.0_models/free_anchor/hv_pointpillars_regnet-3.2gf_fpn_sbn-all_free-anchor_strong-aug_4x8_3x_nus-3d/hv_pointpillars_regnet-3.2gf_fpn_sbn-all_free-anchor_strong-aug_4x8_3x_nus-3d_20210828_030816-06708918.pth
diff --git a/configs/groupfree3d/README.md b/configs/groupfree3d/README.md
new file mode 100644
index 0000000..5b055e7
--- /dev/null
+++ b/configs/groupfree3d/README.md
@@ -0,0 +1,44 @@
+# Group-Free 3D Object Detection via Transformers
+
+> [Group-Free 3D Object Detection via Transformers](https://arxiv.org/abs/2104.00678)
+
+
+
+## Abstract
+
+Recently, directly detecting 3D objects from 3D point clouds has received increasing attention. To extract object representation from an irregular point cloud, existing methods usually take a point grouping step to assign the points to an object candidate so that a PointNet-like network could be used to derive object features from the grouped points. However, the inaccurate point assignments caused by the hand-crafted grouping scheme decrease the performance of 3D object detection. In this paper, we present a simple yet effective method for directly detecting 3D objects from the 3D point cloud. Instead of grouping local points to each object candidate, our method computes the feature of an object from all the points in the point cloud with the help of an attention mechanism in the Transformers, where the contribution of each point is automatically learned in the network training. With an improved attention stacking scheme, our method fuses object features in different stages and generates more accurate object detection results. With few bells and whistles, the proposed method achieves state-of-the-art 3D object detection performance on two widely used benchmarks, ScanNet V2 and SUN RGB-D.
+
+
+
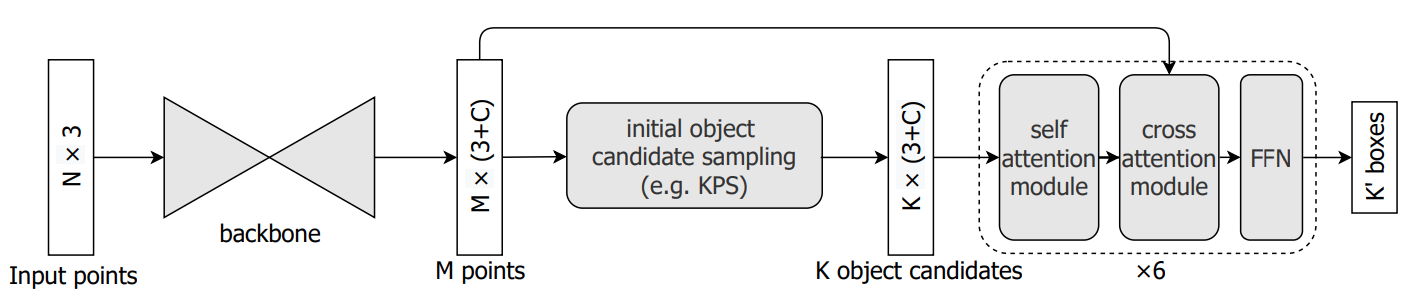
+
+
+## Introduction
+
+We implement Group-Free-3D and provide the result and checkpoints on ScanNet datasets.
+
+## Results and models
+
+### ScanNet
+
+| Method | Backbone | Lr schd | Mem (GB) | Inf time (fps) | AP@0.25 | AP@0.5 | Download |
+| :---------------------------------------------------------------: | :-----------: | :-----: | :------: | :------------: | :-------------: | :-------------: | :--------------------------------------------------------------------------------------------------------------------------------------------------------------------------------------------------------------------------------------------------------------------------------------------------------------------------------------------------------------------------------------------------------------------: |
+| [L6, O256](./groupfree3d_8x4_scannet-3d-18class-L6-O256.py) | PointNet++ | 3x | 6.7 | | 66.32 (65.67\*) | 47.82 (47.74\*) | [model](https://download.openmmlab.com/mmdetection3d/v0.1.0_models/groupfree3d/groupfree3d_8x4_scannet-3d-18class-L6-O256/groupfree3d_8x4_scannet-3d-18class-L6-O256_20210702_145347-3499eb55.pth) \| [log](https://download.openmmlab.com/mmdetection3d/v0.1.0_models/groupfree3d/groupfree3d_8x4_scannet-3d-18class-L6-O256/groupfree3d_8x4_scannet-3d-18class-L6-O256_20210702_145347.log.json) |
+| [L12, O256](./groupfree3d_8x4_scannet-3d-18class-L12-O256.py) | PointNet++ | 3x | 9.4 | | 66.57 (66.22\*) | 48.21 (48.95\*) | [model](https://download.openmmlab.com/mmdetection3d/v0.1.0_models/groupfree3d/groupfree3d_8x4_scannet-3d-18class-L12-O256/groupfree3d_8x4_scannet-3d-18class-L12-O256_20210702_150907-1c5551ad.pth) \| [log](https://download.openmmlab.com/mmdetection3d/v0.1.0_models/groupfree3d/groupfree3d_8x4_scannet-3d-18class-L12-O256/groupfree3d_8x4_scannet-3d-18class-L12-O256_20210702_150907.log.json) |
+| [L12, O256](./groupfree3d_8x4_scannet-3d-18class-w2x-L12-O256.py) | PointNet++w2x | 3x | 13.3 | | 68.20 (67.30\*) | 51.02 (50.44\*) | [model](https://download.openmmlab.com/mmdetection3d/v0.1.0_models/groupfree3d/groupfree3d_8x4_scannet-3d-18class-w2x-L12-O256/groupfree3d_8x4_scannet-3d-18class-w2x-L12-O256_20210702_200301-944f0ac0.pth) \| [log](https://download.openmmlab.com/mmdetection3d/v0.1.0_models/groupfree3d/groupfree3d_8x4_scannet-3d-18class-w2x-L12-O256/groupfree3d_8x4_scannet-3d-18class-w2x-L12-O256_20210702_200301.log.json) |
+| [L12, O512](./groupfree3d_8x4_scannet-3d-18class-w2x-L12-O512.py) | PointNet++w2x | 3x | 18.8 | | 68.22 (68.20\*) | 52.61 (51.31\*) | [model](https://download.openmmlab.com/mmdetection3d/v0.1.0_models/groupfree3d/groupfree3d_8x4_scannet-3d-18class-w2x-L12-O512/groupfree3d_8x4_scannet-3d-18class-w2x-L12-O512_20210702_220204-187b71c7.pth) \| [log](https://download.openmmlab.com/mmdetection3d/v0.1.0_models/groupfree3d/groupfree3d_8x4_scannet-3d-18class-w2x-L12-O512/groupfree3d_8x4_scannet-3d-18class-w2x-L12-O512_20210702_220204.log.json) |
+
+**Notes:**
+
+- We report the best results (AP@0.50) on validation set during each training. * means the evaluation method in the paper: we train each setting 5 times and test each training trial 5 times, then the average performance of these 25 trials is reported to account for algorithm randomness.
+- We use 4 GPUs for training by default as the original code.
+
+## Citation
+
+```latex
+@article{liu2021,
+ title={Group-Free 3D Object Detection via Transformers},
+ author={Liu, Ze and Zhang, Zheng and Cao, Yue and Hu, Han and Tong, Xin},
+ journal={Proceedings of the IEEE/CVF International Conference on Computer Vision (ICCV)},
+ year={2021}
+}
+```
diff --git a/configs/groupfree3d/groupfree3d_8x4_scannet-3d-18class-L12-O256.py b/configs/groupfree3d/groupfree3d_8x4_scannet-3d-18class-L12-O256.py
new file mode 100644
index 0000000..987bcec
--- /dev/null
+++ b/configs/groupfree3d/groupfree3d_8x4_scannet-3d-18class-L12-O256.py
@@ -0,0 +1,199 @@
+_base_ = [
+ '../_base_/datasets/scannet-3d-18class.py',
+ '../_base_/models/groupfree3d.py', '../_base_/schedules/schedule_3x.py',
+ '../_base_/default_runtime.py'
+]
+
+# model settings
+model = dict(
+ bbox_head=dict(
+ num_classes=18,
+ num_decoder_layers=12,
+ size_cls_agnostic=False,
+ bbox_coder=dict(
+ type='GroupFree3DBBoxCoder',
+ num_sizes=18,
+ num_dir_bins=1,
+ with_rot=False,
+ size_cls_agnostic=False,
+ mean_sizes=[[0.76966727, 0.8116021, 0.92573744],
+ [1.876858, 1.8425595, 1.1931566],
+ [0.61328, 0.6148609, 0.7182701],
+ [1.3955007, 1.5121545, 0.83443564],
+ [0.97949594, 1.0675149, 0.6329687],
+ [0.531663, 0.5955577, 1.7500148],
+ [0.9624706, 0.72462326, 1.1481868],
+ [0.83221924, 1.0490936, 1.6875663],
+ [0.21132214, 0.4206159, 0.5372846],
+ [1.4440073, 1.8970833, 0.26985747],
+ [1.0294262, 1.4040797, 0.87554324],
+ [1.3766412, 0.65521795, 1.6813129],
+ [0.6650819, 0.71111923, 1.298853],
+ [0.41999173, 0.37906948, 1.7513971],
+ [0.59359556, 0.5912492, 0.73919016],
+ [0.50867593, 0.50656086, 0.30136237],
+ [1.1511526, 1.0546296, 0.49706793],
+ [0.47535285, 0.49249494, 0.5802117]]),
+ sampling_objectness_loss=dict(
+ type='FocalLoss',
+ use_sigmoid=True,
+ gamma=2.0,
+ alpha=0.25,
+ loss_weight=8.0),
+ objectness_loss=dict(
+ type='FocalLoss',
+ use_sigmoid=True,
+ gamma=2.0,
+ alpha=0.25,
+ loss_weight=1.0),
+ center_loss=dict(
+ type='SmoothL1Loss', beta=0.04, reduction='sum', loss_weight=10.0),
+ dir_class_loss=dict(
+ type='CrossEntropyLoss', reduction='sum', loss_weight=1.0),
+ dir_res_loss=dict(
+ type='SmoothL1Loss', reduction='sum', loss_weight=10.0),
+ size_class_loss=dict(
+ type='CrossEntropyLoss', reduction='sum', loss_weight=1.0),
+ size_res_loss=dict(
+ type='SmoothL1Loss',
+ beta=1.0 / 9.0,
+ reduction='sum',
+ loss_weight=10.0 / 9.0),
+ semantic_loss=dict(
+ type='CrossEntropyLoss', reduction='sum', loss_weight=1.0)),
+ test_cfg=dict(
+ sample_mod='kps',
+ nms_thr=0.25,
+ score_thr=0.0,
+ per_class_proposal=True,
+ prediction_stages='last_three'))
+
+# dataset settings
+dataset_type = 'ScanNetDataset'
+data_root = './data/scannet/'
+class_names = ('cabinet', 'bed', 'chair', 'sofa', 'table', 'door', 'window',
+ 'bookshelf', 'picture', 'counter', 'desk', 'curtain',
+ 'refrigerator', 'showercurtrain', 'toilet', 'sink', 'bathtub',
+ 'garbagebin')
+train_pipeline = [
+ dict(
+ type='LoadPointsFromFile',
+ coord_type='DEPTH',
+ load_dim=6,
+ use_dim=[0, 1, 2]),
+ dict(
+ type='LoadAnnotations3D',
+ with_bbox_3d=True,
+ with_label_3d=True,
+ with_mask_3d=True,
+ with_seg_3d=True),
+ dict(type='GlobalAlignment', rotation_axis=2),
+ dict(
+ type='PointSegClassMapping',
+ valid_cat_ids=(3, 4, 5, 6, 7, 8, 9, 10, 11, 12, 14, 16, 24, 28, 33, 34,
+ 36, 39)),
+ dict(type='PointSample', num_points=50000),
+ dict(
+ type='RandomFlip3D',
+ sync_2d=False,
+ flip_ratio_bev_horizontal=0.5,
+ flip_ratio_bev_vertical=0.5),
+ dict(
+ type='GlobalRotScaleTrans',
+ rot_range=[-0.087266, 0.087266],
+ scale_ratio_range=[1.0, 1.0]),
+ dict(type='DefaultFormatBundle3D', class_names=class_names),
+ dict(
+ type='Collect3D',
+ keys=[
+ 'points', 'gt_bboxes_3d', 'gt_labels_3d', 'pts_semantic_mask',
+ 'pts_instance_mask'
+ ])
+]
+test_pipeline = [
+ dict(
+ type='LoadPointsFromFile',
+ coord_type='DEPTH',
+ load_dim=6,
+ use_dim=[0, 1, 2]),
+ dict(type='GlobalAlignment', rotation_axis=2),
+ dict(
+ type='MultiScaleFlipAug3D',
+ img_scale=(1333, 800),
+ pts_scale_ratio=1,
+ flip=False,
+ transforms=[
+ dict(
+ type='GlobalRotScaleTrans',
+ rot_range=[0, 0],
+ scale_ratio_range=[1., 1.],
+ translation_std=[0, 0, 0]),
+ dict(
+ type='RandomFlip3D',
+ sync_2d=False,
+ flip_ratio_bev_horizontal=0.5,
+ flip_ratio_bev_vertical=0.5),
+ dict(type='PointSample', num_points=50000),
+ dict(
+ type='DefaultFormatBundle3D',
+ class_names=class_names,
+ with_label=False),
+ dict(type='Collect3D', keys=['points'])
+ ])
+]
+
+data = dict(
+ samples_per_gpu=8,
+ workers_per_gpu=4,
+ train=dict(
+ type='RepeatDataset',
+ times=5,
+ dataset=dict(
+ type=dataset_type,
+ data_root=data_root,
+ ann_file=data_root + 'scannet_infos_train.pkl',
+ pipeline=train_pipeline,
+ filter_empty_gt=False,
+ classes=class_names,
+ # we use box_type_3d='LiDAR' in kitti and nuscenes dataset
+ # and box_type_3d='Depth' in sunrgbd and scannet dataset.
+ box_type_3d='Depth')),
+ val=dict(
+ type=dataset_type,
+ data_root=data_root,
+ ann_file=data_root + 'scannet_infos_val.pkl',
+ pipeline=test_pipeline,
+ classes=class_names,
+ test_mode=True,
+ box_type_3d='Depth'),
+ test=dict(
+ type=dataset_type,
+ data_root=data_root,
+ ann_file=data_root + 'scannet_infos_val.pkl',
+ pipeline=test_pipeline,
+ classes=class_names,
+ test_mode=True,
+ box_type_3d='Depth'))
+
+# optimizer
+lr = 0.006
+optimizer = dict(
+ lr=lr,
+ weight_decay=0.0005,
+ paramwise_cfg=dict(
+ custom_keys={
+ 'bbox_head.decoder_layers': dict(lr_mult=0.1, decay_mult=1.0),
+ 'bbox_head.decoder_self_posembeds': dict(
+ lr_mult=0.1, decay_mult=1.0),
+ 'bbox_head.decoder_cross_posembeds': dict(
+ lr_mult=0.1, decay_mult=1.0),
+ 'bbox_head.decoder_query_proj': dict(lr_mult=0.1, decay_mult=1.0),
+ 'bbox_head.decoder_key_proj': dict(lr_mult=0.1, decay_mult=1.0)
+ }))
+
+optimizer_config = dict(grad_clip=dict(max_norm=0.1, norm_type=2))
+lr_config = dict(policy='step', warmup=None, step=[56, 68])
+
+# runtime settings
+runner = dict(type='EpochBasedRunner', max_epochs=80)
+checkpoint_config = dict(interval=1, max_keep_ckpts=10)
diff --git a/configs/groupfree3d/groupfree3d_8x4_scannet-3d-18class-L6-O256.py b/configs/groupfree3d/groupfree3d_8x4_scannet-3d-18class-L6-O256.py
new file mode 100644
index 0000000..6282129
--- /dev/null
+++ b/configs/groupfree3d/groupfree3d_8x4_scannet-3d-18class-L6-O256.py
@@ -0,0 +1,198 @@
+_base_ = [
+ '../_base_/datasets/scannet-3d-18class.py',
+ '../_base_/models/groupfree3d.py', '../_base_/schedules/schedule_3x.py',
+ '../_base_/default_runtime.py'
+]
+
+# model settings
+model = dict(
+ bbox_head=dict(
+ num_classes=18,
+ size_cls_agnostic=False,
+ bbox_coder=dict(
+ type='GroupFree3DBBoxCoder',
+ num_sizes=18,
+ num_dir_bins=1,
+ with_rot=False,
+ size_cls_agnostic=False,
+ mean_sizes=[[0.76966727, 0.8116021, 0.92573744],
+ [1.876858, 1.8425595, 1.1931566],
+ [0.61328, 0.6148609, 0.7182701],
+ [1.3955007, 1.5121545, 0.83443564],
+ [0.97949594, 1.0675149, 0.6329687],
+ [0.531663, 0.5955577, 1.7500148],
+ [0.9624706, 0.72462326, 1.1481868],
+ [0.83221924, 1.0490936, 1.6875663],
+ [0.21132214, 0.4206159, 0.5372846],
+ [1.4440073, 1.8970833, 0.26985747],
+ [1.0294262, 1.4040797, 0.87554324],
+ [1.3766412, 0.65521795, 1.6813129],
+ [0.6650819, 0.71111923, 1.298853],
+ [0.41999173, 0.37906948, 1.7513971],
+ [0.59359556, 0.5912492, 0.73919016],
+ [0.50867593, 0.50656086, 0.30136237],
+ [1.1511526, 1.0546296, 0.49706793],
+ [0.47535285, 0.49249494, 0.5802117]]),
+ sampling_objectness_loss=dict(
+ type='FocalLoss',
+ use_sigmoid=True,
+ gamma=2.0,
+ alpha=0.25,
+ loss_weight=8.0),
+ objectness_loss=dict(
+ type='FocalLoss',
+ use_sigmoid=True,
+ gamma=2.0,
+ alpha=0.25,
+ loss_weight=1.0),
+ center_loss=dict(
+ type='SmoothL1Loss', beta=0.04, reduction='sum', loss_weight=10.0),
+ dir_class_loss=dict(
+ type='CrossEntropyLoss', reduction='sum', loss_weight=1.0),
+ dir_res_loss=dict(
+ type='SmoothL1Loss', reduction='sum', loss_weight=10.0),
+ size_class_loss=dict(
+ type='CrossEntropyLoss', reduction='sum', loss_weight=1.0),
+ size_res_loss=dict(
+ type='SmoothL1Loss',
+ beta=1.0 / 9.0,
+ reduction='sum',
+ loss_weight=10.0 / 9.0),
+ semantic_loss=dict(
+ type='CrossEntropyLoss', reduction='sum', loss_weight=1.0)),
+ test_cfg=dict(
+ sample_mod='kps',
+ nms_thr=0.25,
+ score_thr=0.0,
+ per_class_proposal=True,
+ prediction_stages='last_three'))
+
+# dataset settings
+dataset_type = 'ScanNetDataset'
+data_root = './data/scannet/'
+class_names = ('cabinet', 'bed', 'chair', 'sofa', 'table', 'door', 'window',
+ 'bookshelf', 'picture', 'counter', 'desk', 'curtain',
+ 'refrigerator', 'showercurtrain', 'toilet', 'sink', 'bathtub',
+ 'garbagebin')
+train_pipeline = [
+ dict(
+ type='LoadPointsFromFile',
+ coord_type='DEPTH',
+ load_dim=6,
+ use_dim=[0, 1, 2]),
+ dict(
+ type='LoadAnnotations3D',
+ with_bbox_3d=True,
+ with_label_3d=True,
+ with_mask_3d=True,
+ with_seg_3d=True),
+ dict(type='GlobalAlignment', rotation_axis=2),
+ dict(
+ type='PointSegClassMapping',
+ valid_cat_ids=(3, 4, 5, 6, 7, 8, 9, 10, 11, 12, 14, 16, 24, 28, 33, 34,
+ 36, 39)),
+ dict(type='PointSample', num_points=50000),
+ dict(
+ type='RandomFlip3D',
+ sync_2d=False,
+ flip_ratio_bev_horizontal=0.5,
+ flip_ratio_bev_vertical=0.5),
+ dict(
+ type='GlobalRotScaleTrans',
+ rot_range=[-0.087266, 0.087266],
+ scale_ratio_range=[1.0, 1.0]),
+ dict(type='DefaultFormatBundle3D', class_names=class_names),
+ dict(
+ type='Collect3D',
+ keys=[
+ 'points', 'gt_bboxes_3d', 'gt_labels_3d', 'pts_semantic_mask',
+ 'pts_instance_mask'
+ ])
+]
+test_pipeline = [
+ dict(
+ type='LoadPointsFromFile',
+ coord_type='DEPTH',
+ load_dim=6,
+ use_dim=[0, 1, 2]),
+ dict(type='GlobalAlignment', rotation_axis=2),
+ dict(
+ type='MultiScaleFlipAug3D',
+ img_scale=(1333, 800),
+ pts_scale_ratio=1,
+ flip=False,
+ transforms=[
+ dict(
+ type='GlobalRotScaleTrans',
+ rot_range=[0, 0],
+ scale_ratio_range=[1., 1.],
+ translation_std=[0, 0, 0]),
+ dict(
+ type='RandomFlip3D',
+ sync_2d=False,
+ flip_ratio_bev_horizontal=0.5,
+ flip_ratio_bev_vertical=0.5),
+ dict(type='PointSample', num_points=50000),
+ dict(
+ type='DefaultFormatBundle3D',
+ class_names=class_names,
+ with_label=False),
+ dict(type='Collect3D', keys=['points'])
+ ])
+]
+
+data = dict(
+ samples_per_gpu=8,
+ workers_per_gpu=4,
+ train=dict(
+ type='RepeatDataset',
+ times=5,
+ dataset=dict(
+ type=dataset_type,
+ data_root=data_root,
+ ann_file=data_root + 'scannet_infos_train.pkl',
+ pipeline=train_pipeline,
+ filter_empty_gt=False,
+ classes=class_names,
+ # we use box_type_3d='LiDAR' in kitti and nuscenes dataset
+ # and box_type_3d='Depth' in sunrgbd and scannet dataset.
+ box_type_3d='Depth')),
+ val=dict(
+ type=dataset_type,
+ data_root=data_root,
+ ann_file=data_root + 'scannet_infos_val.pkl',
+ pipeline=test_pipeline,
+ classes=class_names,
+ test_mode=True,
+ box_type_3d='Depth'),
+ test=dict(
+ type=dataset_type,
+ data_root=data_root,
+ ann_file=data_root + 'scannet_infos_val.pkl',
+ pipeline=test_pipeline,
+ classes=class_names,
+ test_mode=True,
+ box_type_3d='Depth'))
+
+# optimizer
+lr = 0.006
+optimizer = dict(
+ lr=lr,
+ weight_decay=0.0005,
+ paramwise_cfg=dict(
+ custom_keys={
+ 'bbox_head.decoder_layers': dict(lr_mult=0.1, decay_mult=1.0),
+ 'bbox_head.decoder_self_posembeds': dict(
+ lr_mult=0.1, decay_mult=1.0),
+ 'bbox_head.decoder_cross_posembeds': dict(
+ lr_mult=0.1, decay_mult=1.0),
+ 'bbox_head.decoder_query_proj': dict(lr_mult=0.1, decay_mult=1.0),
+ 'bbox_head.decoder_key_proj': dict(lr_mult=0.1, decay_mult=1.0)
+ }))
+
+optimizer_config = dict(grad_clip=dict(max_norm=0.1, norm_type=2))
+lr_config = dict(policy='step', warmup=None, step=[56, 68])
+
+# runtime settings
+runner = dict(type='EpochBasedRunner', max_epochs=80)
+checkpoint_config = dict(interval=1, max_keep_ckpts=10)
diff --git a/configs/groupfree3d/groupfree3d_8x4_scannet-3d-18class-w2x-L12-O256.py b/configs/groupfree3d/groupfree3d_8x4_scannet-3d-18class-w2x-L12-O256.py
new file mode 100644
index 0000000..8551b74
--- /dev/null
+++ b/configs/groupfree3d/groupfree3d_8x4_scannet-3d-18class-w2x-L12-O256.py
@@ -0,0 +1,214 @@
+_base_ = [
+ '../_base_/datasets/scannet-3d-18class.py',
+ '../_base_/models/groupfree3d.py', '../_base_/schedules/schedule_3x.py',
+ '../_base_/default_runtime.py'
+]
+
+# model settings
+model = dict(
+ backbone=dict(
+ type='PointNet2SASSG',
+ in_channels=3,
+ num_points=(2048, 1024, 512, 256),
+ radius=(0.2, 0.4, 0.8, 1.2),
+ num_samples=(64, 32, 16, 16),
+ sa_channels=((128, 128, 256), (256, 256, 512), (256, 256, 512),
+ (256, 256, 512)),
+ fp_channels=((512, 512), (512, 288)),
+ norm_cfg=dict(type='BN2d'),
+ sa_cfg=dict(
+ type='PointSAModule',
+ pool_mod='max',
+ use_xyz=True,
+ normalize_xyz=True)),
+ bbox_head=dict(
+ num_classes=18,
+ num_decoder_layers=12,
+ size_cls_agnostic=False,
+ bbox_coder=dict(
+ type='GroupFree3DBBoxCoder',
+ num_sizes=18,
+ num_dir_bins=1,
+ with_rot=False,
+ size_cls_agnostic=False,
+ mean_sizes=[[0.76966727, 0.8116021, 0.92573744],
+ [1.876858, 1.8425595, 1.1931566],
+ [0.61328, 0.6148609, 0.7182701],
+ [1.3955007, 1.5121545, 0.83443564],
+ [0.97949594, 1.0675149, 0.6329687],
+ [0.531663, 0.5955577, 1.7500148],
+ [0.9624706, 0.72462326, 1.1481868],
+ [0.83221924, 1.0490936, 1.6875663],
+ [0.21132214, 0.4206159, 0.5372846],
+ [1.4440073, 1.8970833, 0.26985747],
+ [1.0294262, 1.4040797, 0.87554324],
+ [1.3766412, 0.65521795, 1.6813129],
+ [0.6650819, 0.71111923, 1.298853],
+ [0.41999173, 0.37906948, 1.7513971],
+ [0.59359556, 0.5912492, 0.73919016],
+ [0.50867593, 0.50656086, 0.30136237],
+ [1.1511526, 1.0546296, 0.49706793],
+ [0.47535285, 0.49249494, 0.5802117]]),
+ sampling_objectness_loss=dict(
+ type='FocalLoss',
+ use_sigmoid=True,
+ gamma=2.0,
+ alpha=0.25,
+ loss_weight=8.0),
+ objectness_loss=dict(
+ type='FocalLoss',
+ use_sigmoid=True,
+ gamma=2.0,
+ alpha=0.25,
+ loss_weight=1.0),
+ center_loss=dict(
+ type='SmoothL1Loss', beta=0.04, reduction='sum', loss_weight=10.0),
+ dir_class_loss=dict(
+ type='CrossEntropyLoss', reduction='sum', loss_weight=1.0),
+ dir_res_loss=dict(
+ type='SmoothL1Loss', reduction='sum', loss_weight=10.0),
+ size_class_loss=dict(
+ type='CrossEntropyLoss', reduction='sum', loss_weight=1.0),
+ size_res_loss=dict(
+ type='SmoothL1Loss',
+ beta=1.0 / 9.0,
+ reduction='sum',
+ loss_weight=10.0 / 9.0),
+ semantic_loss=dict(
+ type='CrossEntropyLoss', reduction='sum', loss_weight=1.0)),
+ test_cfg=dict(
+ sample_mod='kps',
+ nms_thr=0.25,
+ score_thr=0.0,
+ per_class_proposal=True,
+ prediction_stages='last_three'))
+
+# dataset settings
+dataset_type = 'ScanNetDataset'
+data_root = './data/scannet/'
+class_names = ('cabinet', 'bed', 'chair', 'sofa', 'table', 'door', 'window',
+ 'bookshelf', 'picture', 'counter', 'desk', 'curtain',
+ 'refrigerator', 'showercurtrain', 'toilet', 'sink', 'bathtub',
+ 'garbagebin')
+train_pipeline = [
+ dict(
+ type='LoadPointsFromFile',
+ coord_type='DEPTH',
+ load_dim=6,
+ use_dim=[0, 1, 2]),
+ dict(
+ type='LoadAnnotations3D',
+ with_bbox_3d=True,
+ with_label_3d=True,
+ with_mask_3d=True,
+ with_seg_3d=True),
+ dict(type='GlobalAlignment', rotation_axis=2),
+ dict(
+ type='PointSegClassMapping',
+ valid_cat_ids=(3, 4, 5, 6, 7, 8, 9, 10, 11, 12, 14, 16, 24, 28, 33, 34,
+ 36, 39)),
+ dict(type='PointSample', num_points=50000),
+ dict(
+ type='RandomFlip3D',
+ sync_2d=False,
+ flip_ratio_bev_horizontal=0.5,
+ flip_ratio_bev_vertical=0.5),
+ dict(
+ type='GlobalRotScaleTrans',
+ rot_range=[-0.087266, 0.087266],
+ scale_ratio_range=[1.0, 1.0]),
+ dict(type='DefaultFormatBundle3D', class_names=class_names),
+ dict(
+ type='Collect3D',
+ keys=[
+ 'points', 'gt_bboxes_3d', 'gt_labels_3d', 'pts_semantic_mask',
+ 'pts_instance_mask'
+ ])
+]
+test_pipeline = [
+ dict(
+ type='LoadPointsFromFile',
+ coord_type='DEPTH',
+ load_dim=6,
+ use_dim=[0, 1, 2]),
+ dict(type='GlobalAlignment', rotation_axis=2),
+ dict(
+ type='MultiScaleFlipAug3D',
+ img_scale=(1333, 800),
+ pts_scale_ratio=1,
+ flip=False,
+ transforms=[
+ dict(
+ type='GlobalRotScaleTrans',
+ rot_range=[0, 0],
+ scale_ratio_range=[1., 1.],
+ translation_std=[0, 0, 0]),
+ dict(
+ type='RandomFlip3D',
+ sync_2d=False,
+ flip_ratio_bev_horizontal=0.5,
+ flip_ratio_bev_vertical=0.5),
+ dict(type='PointSample', num_points=50000),
+ dict(
+ type='DefaultFormatBundle3D',
+ class_names=class_names,
+ with_label=False),
+ dict(type='Collect3D', keys=['points'])
+ ])
+]
+
+data = dict(
+ samples_per_gpu=8,
+ workers_per_gpu=4,
+ train=dict(
+ type='RepeatDataset',
+ times=5,
+ dataset=dict(
+ type=dataset_type,
+ data_root=data_root,
+ ann_file=data_root + 'scannet_infos_train.pkl',
+ pipeline=train_pipeline,
+ filter_empty_gt=False,
+ classes=class_names,
+ # we use box_type_3d='LiDAR' in kitti and nuscenes dataset
+ # and box_type_3d='Depth' in sunrgbd and scannet dataset.
+ box_type_3d='Depth')),
+ val=dict(
+ type=dataset_type,
+ data_root=data_root,
+ ann_file=data_root + 'scannet_infos_val.pkl',
+ pipeline=test_pipeline,
+ classes=class_names,
+ test_mode=True,
+ box_type_3d='Depth'),
+ test=dict(
+ type=dataset_type,
+ data_root=data_root,
+ ann_file=data_root + 'scannet_infos_val.pkl',
+ pipeline=test_pipeline,
+ classes=class_names,
+ test_mode=True,
+ box_type_3d='Depth'))
+
+# optimizer
+lr = 0.006
+optimizer = dict(
+ lr=lr,
+ weight_decay=0.0005,
+ paramwise_cfg=dict(
+ custom_keys={
+ 'bbox_head.decoder_layers': dict(lr_mult=0.1, decay_mult=1.0),
+ 'bbox_head.decoder_self_posembeds': dict(
+ lr_mult=0.1, decay_mult=1.0),
+ 'bbox_head.decoder_cross_posembeds': dict(
+ lr_mult=0.1, decay_mult=1.0),
+ 'bbox_head.decoder_query_proj': dict(lr_mult=0.1, decay_mult=1.0),
+ 'bbox_head.decoder_key_proj': dict(lr_mult=0.1, decay_mult=1.0)
+ }))
+
+optimizer_config = dict(grad_clip=dict(max_norm=0.1, norm_type=2))
+lr_config = dict(policy='step', warmup=None, step=[56, 68])
+
+# runtime settings
+runner = dict(type='EpochBasedRunner', max_epochs=80)
+checkpoint_config = dict(interval=1, max_keep_ckpts=10)
diff --git a/configs/groupfree3d/groupfree3d_8x4_scannet-3d-18class-w2x-L12-O512.py b/configs/groupfree3d/groupfree3d_8x4_scannet-3d-18class-w2x-L12-O512.py
new file mode 100644
index 0000000..199e08b
--- /dev/null
+++ b/configs/groupfree3d/groupfree3d_8x4_scannet-3d-18class-w2x-L12-O512.py
@@ -0,0 +1,215 @@
+_base_ = [
+ '../_base_/datasets/scannet-3d-18class.py',
+ '../_base_/models/groupfree3d.py', '../_base_/schedules/schedule_3x.py',
+ '../_base_/default_runtime.py'
+]
+
+# model settings
+model = dict(
+ backbone=dict(
+ type='PointNet2SASSG',
+ in_channels=3,
+ num_points=(2048, 1024, 512, 256),
+ radius=(0.2, 0.4, 0.8, 1.2),
+ num_samples=(64, 32, 16, 16),
+ sa_channels=((128, 128, 256), (256, 256, 512), (256, 256, 512),
+ (256, 256, 512)),
+ fp_channels=((512, 512), (512, 288)),
+ norm_cfg=dict(type='BN2d'),
+ sa_cfg=dict(
+ type='PointSAModule',
+ pool_mod='max',
+ use_xyz=True,
+ normalize_xyz=True)),
+ bbox_head=dict(
+ num_classes=18,
+ num_decoder_layers=12,
+ num_proposal=512,
+ size_cls_agnostic=False,
+ bbox_coder=dict(
+ type='GroupFree3DBBoxCoder',
+ num_sizes=18,
+ num_dir_bins=1,
+ with_rot=False,
+ size_cls_agnostic=False,
+ mean_sizes=[[0.76966727, 0.8116021, 0.92573744],
+ [1.876858, 1.8425595, 1.1931566],
+ [0.61328, 0.6148609, 0.7182701],
+ [1.3955007, 1.5121545, 0.83443564],
+ [0.97949594, 1.0675149, 0.6329687],
+ [0.531663, 0.5955577, 1.7500148],
+ [0.9624706, 0.72462326, 1.1481868],
+ [0.83221924, 1.0490936, 1.6875663],
+ [0.21132214, 0.4206159, 0.5372846],
+ [1.4440073, 1.8970833, 0.26985747],
+ [1.0294262, 1.4040797, 0.87554324],
+ [1.3766412, 0.65521795, 1.6813129],
+ [0.6650819, 0.71111923, 1.298853],
+ [0.41999173, 0.37906948, 1.7513971],
+ [0.59359556, 0.5912492, 0.73919016],
+ [0.50867593, 0.50656086, 0.30136237],
+ [1.1511526, 1.0546296, 0.49706793],
+ [0.47535285, 0.49249494, 0.5802117]]),
+ sampling_objectness_loss=dict(
+ type='FocalLoss',
+ use_sigmoid=True,
+ gamma=2.0,
+ alpha=0.25,
+ loss_weight=8.0),
+ objectness_loss=dict(
+ type='FocalLoss',
+ use_sigmoid=True,
+ gamma=2.0,
+ alpha=0.25,
+ loss_weight=1.0),
+ center_loss=dict(
+ type='SmoothL1Loss', beta=0.04, reduction='sum', loss_weight=10.0),
+ dir_class_loss=dict(
+ type='CrossEntropyLoss', reduction='sum', loss_weight=1.0),
+ dir_res_loss=dict(
+ type='SmoothL1Loss', reduction='sum', loss_weight=10.0),
+ size_class_loss=dict(
+ type='CrossEntropyLoss', reduction='sum', loss_weight=1.0),
+ size_res_loss=dict(
+ type='SmoothL1Loss',
+ beta=1.0 / 9.0,
+ reduction='sum',
+ loss_weight=10.0 / 9.0),
+ semantic_loss=dict(
+ type='CrossEntropyLoss', reduction='sum', loss_weight=1.0)),
+ test_cfg=dict(
+ sample_mod='kps',
+ nms_thr=0.25,
+ score_thr=0.0,
+ per_class_proposal=True,
+ prediction_stages='last_three'))
+
+# dataset settings
+dataset_type = 'ScanNetDataset'
+data_root = './data/scannet/'
+class_names = ('cabinet', 'bed', 'chair', 'sofa', 'table', 'door', 'window',
+ 'bookshelf', 'picture', 'counter', 'desk', 'curtain',
+ 'refrigerator', 'showercurtrain', 'toilet', 'sink', 'bathtub',
+ 'garbagebin')
+train_pipeline = [
+ dict(
+ type='LoadPointsFromFile',
+ coord_type='DEPTH',
+ load_dim=6,
+ use_dim=[0, 1, 2]),
+ dict(
+ type='LoadAnnotations3D',
+ with_bbox_3d=True,
+ with_label_3d=True,
+ with_mask_3d=True,
+ with_seg_3d=True),
+ dict(type='GlobalAlignment', rotation_axis=2),
+ dict(
+ type='PointSegClassMapping',
+ valid_cat_ids=(3, 4, 5, 6, 7, 8, 9, 10, 11, 12, 14, 16, 24, 28, 33, 34,
+ 36, 39)),
+ dict(type='PointSample', num_points=50000),
+ dict(
+ type='RandomFlip3D',
+ sync_2d=False,
+ flip_ratio_bev_horizontal=0.5,
+ flip_ratio_bev_vertical=0.5),
+ dict(
+ type='GlobalRotScaleTrans',
+ rot_range=[-0.087266, 0.087266],
+ scale_ratio_range=[1.0, 1.0]),
+ dict(type='DefaultFormatBundle3D', class_names=class_names),
+ dict(
+ type='Collect3D',
+ keys=[
+ 'points', 'gt_bboxes_3d', 'gt_labels_3d', 'pts_semantic_mask',
+ 'pts_instance_mask'
+ ])
+]
+test_pipeline = [
+ dict(
+ type='LoadPointsFromFile',
+ coord_type='DEPTH',
+ load_dim=6,
+ use_dim=[0, 1, 2]),
+ dict(type='GlobalAlignment', rotation_axis=2),
+ dict(
+ type='MultiScaleFlipAug3D',
+ img_scale=(1333, 800),
+ pts_scale_ratio=1,
+ flip=False,
+ transforms=[
+ dict(
+ type='GlobalRotScaleTrans',
+ rot_range=[0, 0],
+ scale_ratio_range=[1., 1.],
+ translation_std=[0, 0, 0]),
+ dict(
+ type='RandomFlip3D',
+ sync_2d=False,
+ flip_ratio_bev_horizontal=0.5,
+ flip_ratio_bev_vertical=0.5),
+ dict(type='PointSample', num_points=50000),
+ dict(
+ type='DefaultFormatBundle3D',
+ class_names=class_names,
+ with_label=False),
+ dict(type='Collect3D', keys=['points'])
+ ])
+]
+
+data = dict(
+ samples_per_gpu=8,
+ workers_per_gpu=4,
+ train=dict(
+ type='RepeatDataset',
+ times=5,
+ dataset=dict(
+ type=dataset_type,
+ data_root=data_root,
+ ann_file=data_root + 'scannet_infos_train.pkl',
+ pipeline=train_pipeline,
+ filter_empty_gt=False,
+ classes=class_names,
+ # we use box_type_3d='LiDAR' in kitti and nuscenes dataset
+ # and box_type_3d='Depth' in sunrgbd and scannet dataset.
+ box_type_3d='Depth')),
+ val=dict(
+ type=dataset_type,
+ data_root=data_root,
+ ann_file=data_root + 'scannet_infos_val.pkl',
+ pipeline=test_pipeline,
+ classes=class_names,
+ test_mode=True,
+ box_type_3d='Depth'),
+ test=dict(
+ type=dataset_type,
+ data_root=data_root,
+ ann_file=data_root + 'scannet_infos_val.pkl',
+ pipeline=test_pipeline,
+ classes=class_names,
+ test_mode=True,
+ box_type_3d='Depth'))
+
+# optimizer
+lr = 0.006
+optimizer = dict(
+ lr=lr,
+ weight_decay=0.0005,
+ paramwise_cfg=dict(
+ custom_keys={
+ 'bbox_head.decoder_layers': dict(lr_mult=0.1, decay_mult=1.0),
+ 'bbox_head.decoder_self_posembeds': dict(
+ lr_mult=0.1, decay_mult=1.0),
+ 'bbox_head.decoder_cross_posembeds': dict(
+ lr_mult=0.1, decay_mult=1.0),
+ 'bbox_head.decoder_query_proj': dict(lr_mult=0.1, decay_mult=1.0),
+ 'bbox_head.decoder_key_proj': dict(lr_mult=0.1, decay_mult=1.0)
+ }))
+
+optimizer_config = dict(grad_clip=dict(max_norm=0.1, norm_type=2))
+lr_config = dict(policy='step', warmup=None, step=[56, 68])
+
+# runtime settings
+runner = dict(type='EpochBasedRunner', max_epochs=80)
+checkpoint_config = dict(interval=1, max_keep_ckpts=10)
diff --git a/configs/groupfree3d/metafile.yml b/configs/groupfree3d/metafile.yml
new file mode 100644
index 0000000..ff0b63c
--- /dev/null
+++ b/configs/groupfree3d/metafile.yml
@@ -0,0 +1,72 @@
+Collections:
+ - Name: Group-Free-3D
+ Metadata:
+ Training Techniques:
+ - AdamW
+ Training Resources: 4x V100 GPUs
+ Architecture:
+ - PointNet++
+ Paper:
+ URL: https://arxiv.org/abs/2104.00678
+ Title: 'Group-Free 3D Object Detection via Transformers'
+ README: configs/groupfree3d/README.md
+ Code:
+ URL: https://github.com/open-mmlab/mmdetection3d/blob/master/mmdet3d/models/detectors/groupfree3dnet.py#L10
+ Version: v0.15.0
+
+Models:
+ - Name: groupfree3d_8x4_scannet-3d-18class-L6-O256.py
+ In Collection: Group-Free-3D
+ Config: configs/groupfree3d/groupfree3d_8x4_scannet-3d-18class-L6-O256.py
+ Metadata:
+ Training Data: ScanNet
+ Training Memory (GB): 6.7
+ Results:
+ - Task: 3D Object Detection
+ Dataset: ScanNet
+ Metrics:
+ AP@0.25: 66.32
+ AP@0.5: 47.82
+ Weights: https://download.openmmlab.com/mmdetection3d/v0.1.0_models/groupfree3d/groupfree3d_8x4_scannet-3d-18class-L6-O256/groupfree3d_8x4_scannet-3d-18class-L6-O256_20210702_145347-3499eb55.pth
+
+ - Name: groupfree3d_8x4_scannet-3d-18class-L12-O256.py
+ In Collection: Group-Free-3D
+ Config: configs/groupfree3d/groupfree3d_8x4_scannet-3d-18class-L12-O256.py
+ Metadata:
+ Training Data: ScanNet
+ Training Memory (GB): 9.4
+ Results:
+ - Task: 3D Object Detection
+ Dataset: ScanNet
+ Metrics:
+ AP@0.25: 66.57
+ AP@0.5: 48.21
+ Weights: https://download.openmmlab.com/mmdetection3d/v0.1.0_models/groupfree3d/groupfree3d_8x4_scannet-3d-18class-L12-O256/groupfree3d_8x4_scannet-3d-18class-L12-O256_20210702_150907-1c5551ad.pth
+
+ - Name: groupfree3d_8x4_scannet-3d-18class-w2x-L12-O256.py
+ In Collection: Group-Free-3D
+ Config: configs/groupfree3d/groupfree3d_8x4_scannet-3d-18class-w2x-L12-O256.py
+ Metadata:
+ Training Data: ScanNet
+ Training Memory (GB): 13.3
+ Results:
+ - Task: 3D Object Detection
+ Dataset: ScanNet
+ Metrics:
+ AP@0.25: 68.20
+ AP@0.5: 51.02
+ Weights: https://download.openmmlab.com/mmdetection3d/v0.1.0_models/groupfree3d/groupfree3d_8x4_scannet-3d-18class-w2x-L12-O256/groupfree3d_8x4_scannet-3d-18class-w2x-L12-O256_20210702_200301-944f0ac0.pth
+
+ - Name: groupfree3d_8x4_scannet-3d-18class-w2x-L12-O512.py
+ In Collection: Group-Free-3D
+ Config: configs/groupfree3d/groupfree3d_8x4_scannet-3d-18class-w2x-L12-O512.py
+ Metadata:
+ Training Data: ScanNet
+ Training Memory (GB): 18.8
+ Results:
+ - Task: 3D Object Detection
+ Dataset: ScanNet
+ Metrics:
+ AP@0.25: 68.22
+ AP@0.5: 52.61
+ Weights: https://download.openmmlab.com/mmdetection3d/v0.1.0_models/groupfree3d/groupfree3d_8x4_scannet-3d-18class-w2x-L12-O512/groupfree3d_8x4_scannet-3d-18class-w2x-L12-O512_20210702_220204-187b71c7.pth
diff --git a/configs/h3dnet/README.md b/configs/h3dnet/README.md
new file mode 100644
index 0000000..60cc30f
--- /dev/null
+++ b/configs/h3dnet/README.md
@@ -0,0 +1,44 @@
+# H3DNet: 3D Object Detection Using Hybrid Geometric Primitives
+
+> [H3DNet: 3D Object Detection Using Hybrid Geometric Primitives](https://arxiv.org/abs/2006.05682)
+
+
+
+## Abstract
+
+We introduce H3DNet, which takes a colorless 3D point cloud as input and outputs a collection of oriented object bounding boxes (or BB) and their semantic labels. The critical idea of H3DNet is to predict a hybrid set of geometric primitives, i.e., BB centers, BB face centers, and BB edge centers. We show how to convert the predicted geometric primitives into object proposals by defining a distance function between an object and the geometric primitives. This distance function enables continuous optimization of object proposals, and its local minimums provide high-fidelity object proposals. H3DNet then utilizes a matching and refinement module to classify object proposals into detected objects and fine-tune the geometric parameters of the detected objects. The hybrid set of geometric primitives not only provides more accurate signals for object detection than using a single type of geometric primitives, but it also provides an overcomplete set of constraints on the resulting 3D layout. Therefore, H3DNet can tolerate outliers in predicted geometric primitives. Our model achieves state-of-the-art 3D detection results on two large datasets with real 3D scans, ScanNet and SUN RGB-D.
+
+
+
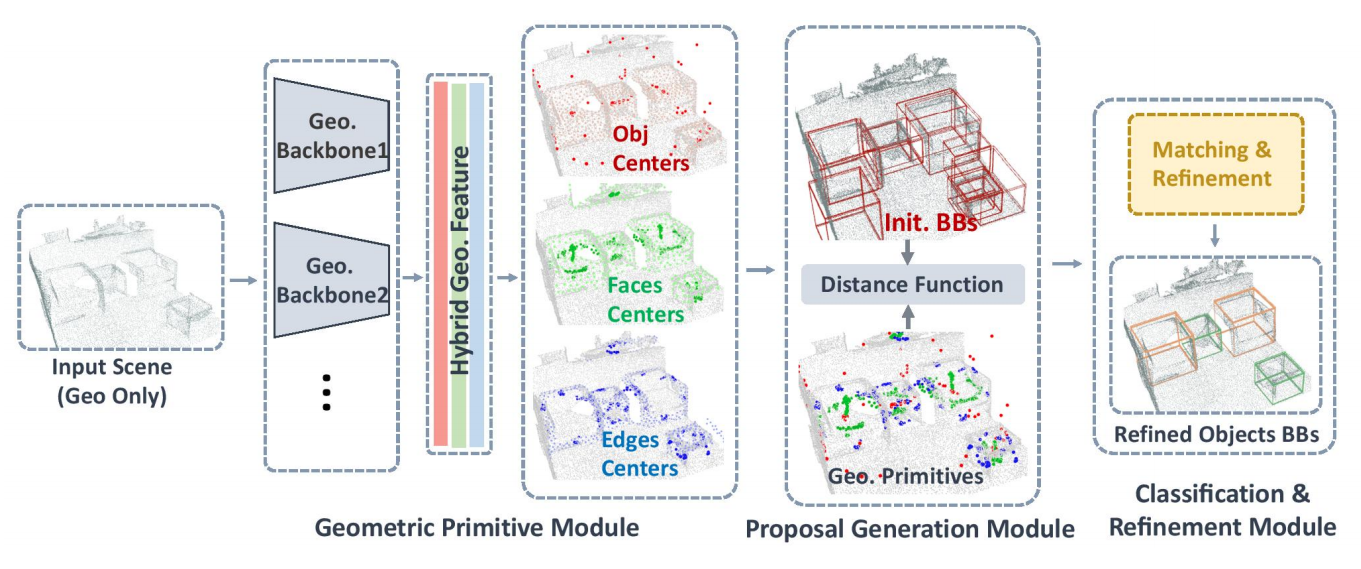
+
+
+## Introduction
+
+We implement H3DNet and provide the result and checkpoints on ScanNet datasets.
+
+## Results and models
+
+### ScanNet
+
+| Backbone | Lr schd | Mem (GB) | Inf time (fps) | AP@0.25 | AP@0.5 | Download |
+| :-------------------------------------------------: | :-----: | :------: | :------------: | :-----: | :----: | :--------------------------------------------------------------------------------------------------------------------------------------------------------------------------------------------------------------------------------------------------------------------------------------------------------------------------: |
+| [MultiBackbone](./h3dnet_3x8_scannet-3d-18class.py) | 3x | 7.9 | | 66.07 | 47.68 | [model](https://download.openmmlab.com/mmdetection3d/v1.0.0_models/h3dnet/h3dnet_scannet-3d-18class/h3dnet_3x8_scannet-3d-18class_20210824_003149-414bd304.pth) \| [log](https://download.openmmlab.com/mmdetection3d/v1.0.0_models/h3dnet/h3dnet_scannet-3d-18class/h3dnet_3x8_scannet-3d-18class_20210824_003149.log.json) |
+
+**Notice**: If your current mmdetection3d version >= 0.6.0, and you are using the checkpoints downloaded from the above links or using checkpoints trained with mmdetection3d version \< 0.6.0, the checkpoints have to be first converted via [tools/model_converters/convert_h3dnet_checkpoints.py](../../tools/model_converters/convert_h3dnet_checkpoints.py):
+
+```
+python ./tools/model_converters/convert_h3dnet_checkpoints.py ${ORIGINAL_CHECKPOINT_PATH} --out=${NEW_CHECKPOINT_PATH}
+```
+
+Then you can use the converted checkpoints following [getting_started.md](../../docs/en/getting_started.md).
+
+## Citation
+
+```latex
+@inproceedings{zhang2020h3dnet,
+ author = {Zhang, Zaiwei and Sun, Bo and Yang, Haitao and Huang, Qixing},
+ title = {H3DNet: 3D Object Detection Using Hybrid Geometric Primitives},
+ booktitle = {Proceedings of the European Conference on Computer Vision},
+ year = {2020}
+}
+```
diff --git a/configs/h3dnet/h3dnet_3x8_scannet-3d-18class.py b/configs/h3dnet/h3dnet_3x8_scannet-3d-18class.py
new file mode 100644
index 0000000..e6534a4
--- /dev/null
+++ b/configs/h3dnet/h3dnet_3x8_scannet-3d-18class.py
@@ -0,0 +1,64 @@
+_base_ = [
+ '../_base_/datasets/scannet-3d-18class.py', '../_base_/models/h3dnet.py',
+ '../_base_/schedules/schedule_3x.py', '../_base_/default_runtime.py'
+]
+
+# model settings
+model = dict(
+ rpn_head=dict(
+ num_classes=18,
+ bbox_coder=dict(
+ type='PartialBinBasedBBoxCoder',
+ num_sizes=18,
+ num_dir_bins=24,
+ with_rot=False,
+ mean_sizes=[[0.76966727, 0.8116021, 0.92573744],
+ [1.876858, 1.8425595, 1.1931566],
+ [0.61328, 0.6148609, 0.7182701],
+ [1.3955007, 1.5121545, 0.83443564],
+ [0.97949594, 1.0675149, 0.6329687],
+ [0.531663, 0.5955577, 1.7500148],
+ [0.9624706, 0.72462326, 1.1481868],
+ [0.83221924, 1.0490936, 1.6875663],
+ [0.21132214, 0.4206159, 0.5372846],
+ [1.4440073, 1.8970833, 0.26985747],
+ [1.0294262, 1.4040797, 0.87554324],
+ [1.3766412, 0.65521795, 1.6813129],
+ [0.6650819, 0.71111923, 1.298853],
+ [0.41999173, 0.37906948, 1.7513971],
+ [0.59359556, 0.5912492, 0.73919016],
+ [0.50867593, 0.50656086, 0.30136237],
+ [1.1511526, 1.0546296, 0.49706793],
+ [0.47535285, 0.49249494, 0.5802117]])),
+ roi_head=dict(
+ bbox_head=dict(
+ num_classes=18,
+ bbox_coder=dict(
+ type='PartialBinBasedBBoxCoder',
+ num_sizes=18,
+ num_dir_bins=24,
+ with_rot=False,
+ mean_sizes=[[0.76966727, 0.8116021, 0.92573744],
+ [1.876858, 1.8425595, 1.1931566],
+ [0.61328, 0.6148609, 0.7182701],
+ [1.3955007, 1.5121545, 0.83443564],
+ [0.97949594, 1.0675149, 0.6329687],
+ [0.531663, 0.5955577, 1.7500148],
+ [0.9624706, 0.72462326, 1.1481868],
+ [0.83221924, 1.0490936, 1.6875663],
+ [0.21132214, 0.4206159, 0.5372846],
+ [1.4440073, 1.8970833, 0.26985747],
+ [1.0294262, 1.4040797, 0.87554324],
+ [1.3766412, 0.65521795, 1.6813129],
+ [0.6650819, 0.71111923, 1.298853],
+ [0.41999173, 0.37906948, 1.7513971],
+ [0.59359556, 0.5912492, 0.73919016],
+ [0.50867593, 0.50656086, 0.30136237],
+ [1.1511526, 1.0546296, 0.49706793],
+ [0.47535285, 0.49249494, 0.5802117]]))))
+
+data = dict(samples_per_gpu=3, workers_per_gpu=2)
+
+# yapf:disable
+log_config = dict(interval=30)
+# yapf:enable
diff --git a/configs/h3dnet/metafile.yml b/configs/h3dnet/metafile.yml
new file mode 100644
index 0000000..6d731d6
--- /dev/null
+++ b/configs/h3dnet/metafile.yml
@@ -0,0 +1,29 @@
+Collections:
+ - Name: H3DNet
+ Metadata:
+ Training Data: ScanNet
+ Training Techniques:
+ - AdamW
+ Training Resources: 8x GeForce GTX 1080 Ti
+ Architecture:
+ Paper:
+ URL: https://arxiv.org/abs/2006.05682
+ Title: 'H3DNet: 3D Object Detection Using Hybrid Geometric Primitives'
+ README: configs/h3dnet/README.md
+ Code:
+ URL: https://github.com/open-mmlab/mmdetection3d/blob/master/mmdet3d/models/detectors/h3dnet.py#L10
+ Version: v0.6.0
+
+Models:
+ - Name: h3dnet_3x8_scannet-3d-18class
+ In Collection: H3DNet
+ Config: configs/h3dnet/h3dnet_3x8_scannet-3d-18class.py
+ Metadata:
+ Training Memory (GB): 7.9
+ Results:
+ - Task: 3D Object Detection
+ Dataset: ScanNet
+ Metrics:
+ AP@0.25: 66.07
+ AP@0.5: 47.68
+ Weights: https://download.openmmlab.com/mmdetection3d/v1.0.0_models/h3dnet/h3dnet_scannet-3d-18class/h3dnet_3x8_scannet-3d-18class_20210824_003149-414bd304.pth
diff --git a/configs/imvotenet/README.md b/configs/imvotenet/README.md
new file mode 100644
index 0000000..a491b9d
--- /dev/null
+++ b/configs/imvotenet/README.md
@@ -0,0 +1,43 @@
+# ImVoteNet: Boosting 3D Object Detection in Point Clouds with Image Votes
+
+> [ImVoteNet: Boosting 3D Object Detection in Point Clouds with Image Votes](https://arxiv.org/abs/2001.10692)
+
+
+
+## Abstract
+
+3D object detection has seen quick progress thanks to advances in deep learning on point clouds. A few recent works have even shown state-of-the-art performance with just point clouds input (e.g. VOTENET). However, point cloud data have inherent limitations. They are sparse, lack color information and often suffer from sensor noise. Images, on the other hand, have high resolution and rich texture. Thus they can complement the 3D geometry provided by point clouds. Yet how to effectively use image information to assist point cloud based detection is still an open question. In this work, we build on top of VOTENET and propose a 3D detection architecture called IMVOTENET specialized for RGB-D scenes. IMVOTENET is based on fusing 2D votes in images and 3D votes in point clouds. Compared to prior work on multi-modal detection, we explicitly extract both geometric and semantic features from the 2D images. We leverage camera parameters to lift these features to 3D. To improve the synergy of 2D-3D feature fusion, we also propose a multi-tower training scheme. We validate our model on the challenging SUN RGB-D dataset, advancing state-of-the-art results by 5.7 mAP. We also provide rich ablation studies to analyze the contribution of each design choice.
+
+
+
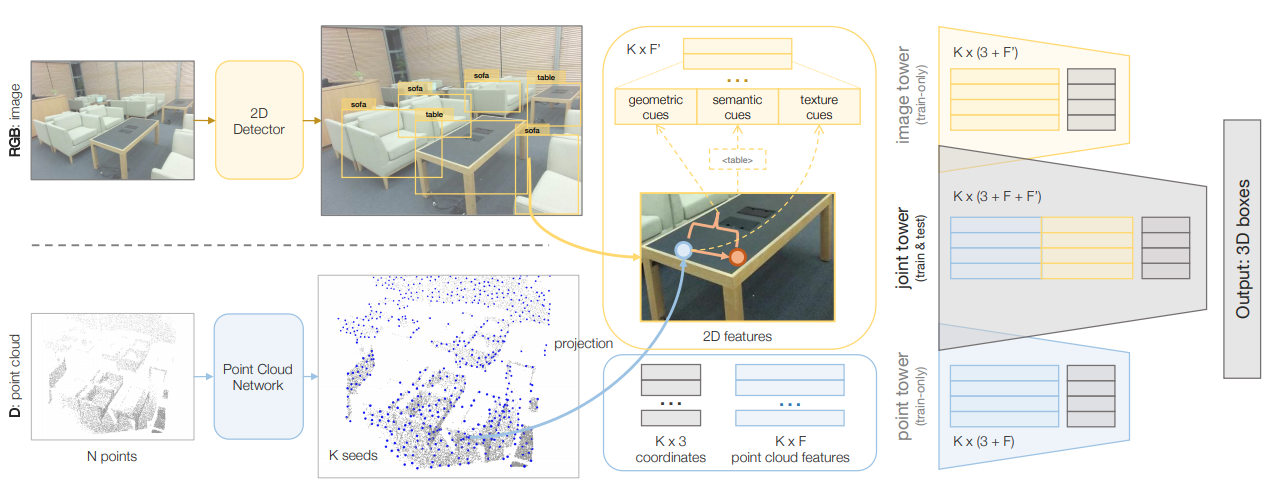
+
+
+## Introduction
+
+We implement ImVoteNet and provide the result and checkpoints on SUNRGBD.
+
+## Results and models
+
+### SUNRGBD-2D (Stage 1, image branch pre-train)
+
+| Backbone | Lr schd | Mem (GB) | Inf time (fps) | AP@0.25 | AP@0.5 | Download |
+| :---------------------------------------------------------------------: | :-----: | :------: | :------------: | :-----: | :----: | :--------------------------------------------------------------------------------------------------------------------------------------------------------------------------------------------------------------------------------------------------------------------------------------------------------------------------------------------------------------------------------------------------------------------------------: |
+| [PointNet++](./imvotenet_faster_rcnn_r50_fpn_2x4_sunrgbd-3d-10class.py) | | 2.1 | | | 62.70 | [model](https://download.openmmlab.com/mmdetection3d/v1.0.0_models/imvotenet/imvotenet_faster_rcnn_r50_fpn_2x4_sunrgbd-3d-10class/imvotenet_faster_rcnn_r50_fpn_2x4_sunrgbd-3d-10class_20210819_225618-62eba6ce.pth) \| [log](https://download.openmmlab.com/mmdetection3d/v1.0.0_models/imvotenet/imvotenet_faster_rcnn_r50_fpn_2x4_sunrgbd-3d-10class/imvotenet_faster_rcnn_r50_fpn_2x4_sunrgbd-3d-10class_20210819_225618.json) |
+
+### SUNRGBD-3D (Stage 2)
+
+| Backbone | Lr schd | Mem (GB) | Inf time (fps) | AP@0.25 | AP@0.5 | Download |
+| :---------------------------------------------------------: | :-----: | :------: | :------------: | :-----: | :----: | :------------------------------------------------------------------------------------------------------------------------------------------------------------------------------------------------------------------------------------------------------------------------------------------------------------------------------------------------------------------------------------: |
+| [PointNet++](./imvotenet_stage2_16x8_sunrgbd-3d-10class.py) | 3x | 9.4 | | 64.55 | | [model](https://download.openmmlab.com/mmdetection3d/v1.0.0_models/imvotenet/imvotenet_stage2_16x8_sunrgbd-3d-10class/imvotenet_stage2_16x8_sunrgbd-3d-10class_20210819_192851-1bcd1b97.pth) \| [log](https://download.openmmlab.com/mmdetection3d/v1.0.0_models/imvotenet/imvotenet_stage2_16x8_sunrgbd-3d-10class/imvotenet_stage2_16x8_sunrgbd-3d-10class_20210819_192851.log.json) |
+
+## Citation
+
+```latex
+@inproceedings{qi2020imvotenet,
+ title={Imvotenet: Boosting 3D object detection in point clouds with image votes},
+ author={Qi, Charles R and Chen, Xinlei and Litany, Or and Guibas, Leonidas J},
+ booktitle={Proceedings of the IEEE/CVF conference on computer vision and pattern recognition},
+ pages={4404--4413},
+ year={2020}
+}
+```
diff --git a/configs/imvotenet/imvotenet_faster_rcnn_r50_fpn_2x4_sunrgbd-3d-10class.py b/configs/imvotenet/imvotenet_faster_rcnn_r50_fpn_2x4_sunrgbd-3d-10class.py
new file mode 100644
index 0000000..e999c65
--- /dev/null
+++ b/configs/imvotenet/imvotenet_faster_rcnn_r50_fpn_2x4_sunrgbd-3d-10class.py
@@ -0,0 +1,58 @@
+_base_ = [
+ '../_base_/datasets/sunrgbd-3d-10class.py', '../_base_/default_runtime.py',
+ '../_base_/models/imvotenet_image.py'
+]
+
+# use caffe img_norm
+img_norm_cfg = dict(
+ mean=[103.530, 116.280, 123.675], std=[1.0, 1.0, 1.0], to_rgb=False)
+
+train_pipeline = [
+ dict(type='LoadImageFromFile'),
+ dict(type='LoadAnnotations', with_bbox=True),
+ dict(
+ type='Resize',
+ img_scale=[(1333, 480), (1333, 504), (1333, 528), (1333, 552),
+ (1333, 576), (1333, 600)],
+ multiscale_mode='value',
+ keep_ratio=True),
+ dict(type='RandomFlip', flip_ratio=0.5),
+ dict(type='Normalize', **img_norm_cfg),
+ dict(type='Pad', size_divisor=32),
+ dict(type='DefaultFormatBundle'),
+ dict(type='Collect', keys=['img', 'gt_bboxes', 'gt_labels']),
+]
+test_pipeline = [
+ dict(type='LoadImageFromFile'),
+ dict(
+ type='MultiScaleFlipAug',
+ img_scale=(1333, 600),
+ flip=False,
+ transforms=[
+ dict(type='Resize', keep_ratio=True),
+ dict(type='RandomFlip'),
+ dict(type='Normalize', **img_norm_cfg),
+ dict(type='Pad', size_divisor=32),
+ dict(type='ImageToTensor', keys=['img']),
+ dict(type='Collect', keys=['img']),
+ ])
+]
+
+data = dict(
+ samples_per_gpu=2,
+ workers_per_gpu=2,
+ train=dict(times=1, dataset=dict(pipeline=train_pipeline)),
+ val=dict(pipeline=test_pipeline),
+ test=dict(pipeline=test_pipeline))
+
+optimizer = dict(type='SGD', lr=0.01, momentum=0.9, weight_decay=0.0001)
+optimizer_config = dict(grad_clip=None)
+lr_config = dict(
+ policy='step',
+ warmup='linear',
+ warmup_iters=500,
+ warmup_ratio=0.001,
+ step=[6])
+runner = dict(type='EpochBasedRunner', max_epochs=8)
+
+load_from = 'http://download.openmmlab.com/mmdetection/v2.0/mask_rcnn/mask_rcnn_r50_caffe_fpn_mstrain-poly_3x_coco/mask_rcnn_r50_caffe_fpn_mstrain-poly_3x_coco_bbox_mAP-0.408__segm_mAP-0.37_20200504_163245-42aa3d00.pth' # noqa
diff --git a/configs/imvotenet/imvotenet_stage2_16x8_sunrgbd-3d-10class.py b/configs/imvotenet/imvotenet_stage2_16x8_sunrgbd-3d-10class.py
new file mode 100644
index 0000000..ef1e553
--- /dev/null
+++ b/configs/imvotenet/imvotenet_stage2_16x8_sunrgbd-3d-10class.py
@@ -0,0 +1,260 @@
+_base_ = [
+ '../_base_/datasets/sunrgbd-3d-10class.py',
+ '../_base_/schedules/schedule_3x.py', '../_base_/default_runtime.py',
+ '../_base_/models/imvotenet_image.py'
+]
+
+class_names = ('bed', 'table', 'sofa', 'chair', 'toilet', 'desk', 'dresser',
+ 'night_stand', 'bookshelf', 'bathtub')
+
+# use caffe img_norm
+img_norm_cfg = dict(
+ mean=[103.530, 116.280, 123.675], std=[1.0, 1.0, 1.0], to_rgb=False)
+
+model = dict(
+ pts_backbone=dict(
+ type='PointNet2SASSG',
+ in_channels=4,
+ num_points=(2048, 1024, 512, 256),
+ radius=(0.2, 0.4, 0.8, 1.2),
+ num_samples=(64, 32, 16, 16),
+ sa_channels=((64, 64, 128), (128, 128, 256), (128, 128, 256),
+ (128, 128, 256)),
+ fp_channels=((256, 256), (256, 256)),
+ norm_cfg=dict(type='BN2d'),
+ sa_cfg=dict(
+ type='PointSAModule',
+ pool_mod='max',
+ use_xyz=True,
+ normalize_xyz=True)),
+ pts_bbox_heads=dict(
+ common=dict(
+ type='VoteHead',
+ num_classes=10,
+ bbox_coder=dict(
+ type='PartialBinBasedBBoxCoder',
+ num_sizes=10,
+ num_dir_bins=12,
+ with_rot=True,
+ mean_sizes=[[2.114256, 1.620300, 0.927272],
+ [0.791118, 1.279516, 0.718182],
+ [0.923508, 1.867419, 0.845495],
+ [0.591958, 0.552978, 0.827272],
+ [0.699104, 0.454178, 0.75625],
+ [0.69519, 1.346299, 0.736364],
+ [0.528526, 1.002642, 1.172878],
+ [0.500618, 0.632163, 0.683424],
+ [0.404671, 1.071108, 1.688889],
+ [0.76584, 1.398258, 0.472728]]),
+ pred_layer_cfg=dict(
+ in_channels=128, shared_conv_channels=(128, 128), bias=True),
+ conv_cfg=dict(type='Conv1d'),
+ norm_cfg=dict(type='BN1d'),
+ objectness_loss=dict(
+ type='CrossEntropyLoss',
+ class_weight=[0.2, 0.8],
+ reduction='sum',
+ loss_weight=5.0),
+ center_loss=dict(
+ type='ChamferDistance',
+ mode='l2',
+ reduction='sum',
+ loss_src_weight=10.0,
+ loss_dst_weight=10.0),
+ dir_class_loss=dict(
+ type='CrossEntropyLoss', reduction='sum', loss_weight=1.0),
+ dir_res_loss=dict(
+ type='SmoothL1Loss', reduction='sum', loss_weight=10.0),
+ size_class_loss=dict(
+ type='CrossEntropyLoss', reduction='sum', loss_weight=1.0),
+ size_res_loss=dict(
+ type='SmoothL1Loss', reduction='sum', loss_weight=10.0 / 3.0),
+ semantic_loss=dict(
+ type='CrossEntropyLoss', reduction='sum', loss_weight=1.0)),
+ joint=dict(
+ vote_module_cfg=dict(
+ in_channels=512,
+ vote_per_seed=1,
+ gt_per_seed=3,
+ conv_channels=(512, 256),
+ conv_cfg=dict(type='Conv1d'),
+ norm_cfg=dict(type='BN1d'),
+ norm_feats=True,
+ vote_loss=dict(
+ type='ChamferDistance',
+ mode='l1',
+ reduction='none',
+ loss_dst_weight=10.0)),
+ vote_aggregation_cfg=dict(
+ type='PointSAModule',
+ num_point=256,
+ radius=0.3,
+ num_sample=16,
+ mlp_channels=[512, 128, 128, 128],
+ use_xyz=True,
+ normalize_xyz=True)),
+ pts=dict(
+ vote_module_cfg=dict(
+ in_channels=256,
+ vote_per_seed=1,
+ gt_per_seed=3,
+ conv_channels=(256, 256),
+ conv_cfg=dict(type='Conv1d'),
+ norm_cfg=dict(type='BN1d'),
+ norm_feats=True,
+ vote_loss=dict(
+ type='ChamferDistance',
+ mode='l1',
+ reduction='none',
+ loss_dst_weight=10.0)),
+ vote_aggregation_cfg=dict(
+ type='PointSAModule',
+ num_point=256,
+ radius=0.3,
+ num_sample=16,
+ mlp_channels=[256, 128, 128, 128],
+ use_xyz=True,
+ normalize_xyz=True)),
+ img=dict(
+ vote_module_cfg=dict(
+ in_channels=256,
+ vote_per_seed=1,
+ gt_per_seed=3,
+ conv_channels=(256, 256),
+ conv_cfg=dict(type='Conv1d'),
+ norm_cfg=dict(type='BN1d'),
+ norm_feats=True,
+ vote_loss=dict(
+ type='ChamferDistance',
+ mode='l1',
+ reduction='none',
+ loss_dst_weight=10.0)),
+ vote_aggregation_cfg=dict(
+ type='PointSAModule',
+ num_point=256,
+ radius=0.3,
+ num_sample=16,
+ mlp_channels=[256, 128, 128, 128],
+ use_xyz=True,
+ normalize_xyz=True)),
+ loss_weights=[0.4, 0.3, 0.3]),
+ img_mlp=dict(
+ in_channel=18,
+ conv_channels=(256, 256),
+ conv_cfg=dict(type='Conv1d'),
+ norm_cfg=dict(type='BN1d'),
+ act_cfg=dict(type='ReLU')),
+ fusion_layer=dict(
+ type='VoteFusion',
+ num_classes=len(class_names),
+ max_imvote_per_pixel=3),
+ num_sampled_seed=1024,
+ freeze_img_branch=True,
+
+ # model training and testing settings
+ train_cfg=dict(
+ pts=dict(
+ pos_distance_thr=0.3, neg_distance_thr=0.6, sample_mod='vote')),
+ test_cfg=dict(
+ img_rcnn=dict(score_thr=0.1),
+ pts=dict(
+ sample_mod='seed',
+ nms_thr=0.25,
+ score_thr=0.05,
+ per_class_proposal=True)))
+
+train_pipeline = [
+ dict(
+ type='LoadPointsFromFile',
+ coord_type='DEPTH',
+ shift_height=True,
+ load_dim=6,
+ use_dim=[0, 1, 2]),
+ dict(type='LoadImageFromFile'),
+ dict(type='LoadAnnotations3D'),
+ dict(type='LoadAnnotations', with_bbox=True),
+ dict(type='Resize', img_scale=(1333, 600), keep_ratio=True),
+ dict(type='RandomFlip', flip_ratio=0.0),
+ dict(type='Normalize', **img_norm_cfg),
+ dict(type='Pad', size_divisor=32),
+ dict(
+ type='RandomFlip3D',
+ sync_2d=False,
+ flip_ratio_bev_horizontal=0.5,
+ ),
+ dict(
+ type='GlobalRotScaleTrans',
+ rot_range=[-0.523599, 0.523599],
+ scale_ratio_range=[0.85, 1.15],
+ shift_height=True),
+ dict(type='PointSample', num_points=20000),
+ dict(type='DefaultFormatBundle3D', class_names=class_names),
+ dict(
+ type='Collect3D',
+ keys=[
+ 'img', 'gt_bboxes', 'gt_labels', 'points', 'gt_bboxes_3d',
+ 'gt_labels_3d'
+ ])
+]
+
+test_pipeline = [
+ dict(type='LoadImageFromFile'),
+ dict(
+ type='LoadPointsFromFile',
+ coord_type='DEPTH',
+ shift_height=True,
+ load_dim=6,
+ use_dim=[0, 1, 2]),
+ dict(
+ type='MultiScaleFlipAug3D',
+ img_scale=(1333, 600),
+ pts_scale_ratio=1,
+ flip=False,
+ transforms=[
+ dict(type='Resize', keep_ratio=True),
+ dict(type='RandomFlip', flip_ratio=0.0),
+ dict(type='Normalize', **img_norm_cfg),
+ dict(type='Pad', size_divisor=32),
+ dict(
+ type='GlobalRotScaleTrans',
+ rot_range=[0, 0],
+ scale_ratio_range=[1., 1.],
+ translation_std=[0, 0, 0]),
+ dict(
+ type='RandomFlip3D',
+ sync_2d=False,
+ flip_ratio_bev_horizontal=0.5,
+ ),
+ dict(type='PointSample', num_points=20000),
+ dict(
+ type='DefaultFormatBundle3D',
+ class_names=class_names,
+ with_label=False),
+ dict(type='Collect3D', keys=['img', 'points'])
+ ]),
+]
+# construct a pipeline for data and gt loading in show function
+# please keep its loading function consistent with test_pipeline (e.g. client)
+eval_pipeline = [
+ dict(type='LoadImageFromFile'),
+ dict(
+ type='LoadPointsFromFile',
+ coord_type='DEPTH',
+ shift_height=False,
+ load_dim=6,
+ use_dim=[0, 1, 2]),
+ dict(
+ type='DefaultFormatBundle3D',
+ class_names=class_names,
+ with_label=False),
+ dict(type='Collect3D', keys=['img', 'points'])
+]
+
+data = dict(
+ train=dict(dataset=dict(pipeline=train_pipeline)),
+ val=dict(pipeline=test_pipeline),
+ test=dict(pipeline=test_pipeline))
+evaluation = dict(pipeline=eval_pipeline)
+
+# may also use your own pre-trained image branch
+load_from = 'https://download.openmmlab.com/mmdetection3d/v0.1.0_models/imvotenet/imvotenet_faster_rcnn_r50_fpn_2x4_sunrgbd-3d-10class/imvotenet_faster_rcnn_r50_fpn_2x4_sunrgbd-3d-10class_20210323_173222-cad62aeb.pth' # noqa
diff --git a/configs/imvotenet/metafile.yml b/configs/imvotenet/metafile.yml
new file mode 100644
index 0000000..28051c4
--- /dev/null
+++ b/configs/imvotenet/metafile.yml
@@ -0,0 +1,43 @@
+Collections:
+ - Name: ImVoteNet
+ Metadata:
+ Training Data: SUNRGBD
+ Training Techniques:
+ - AdamW
+ Training Resources: 8x TITAN Xp
+ Architecture:
+ - Faster R-CNN
+ - VoteNet
+ - Feature Pyramid Network
+ Paper:
+ URL: https://arxiv.org/abs/2001.10692
+ Title: 'ImVoteNet: Boosting 3D Object Detection in Point Clouds with Image Votes'
+ README: configs/imvotenet/README.md
+ Code:
+ URL: https://github.com/open-mmlab/mmdetection3d/blob/master/mmdet3d/models/detectors/imvotenet.py#L56
+ Version: v0.12.0
+
+Models:
+ - Name: imvotenet_faster_rcnn_r50_fpn_2x4_sunrgbd-3d-10class
+ In Collection: ImVoteNet
+ Config: configs/imvotenet/imvotenet_faster_rcnn_r50_fpn_2x4_sunrgbd-3d-10class.py
+ Metadata:
+ Training Memory (GB): 2.1
+ Results:
+ - Task: Object Detection
+ Dataset: SUNRGBD-2D
+ Metrics:
+ AP@0.5: 62.70
+ Weights: https://download.openmmlab.com/mmdetection3d/v1.0.0_models/imvotenet/imvotenet_faster_rcnn_r50_fpn_2x4_sunrgbd-3d-10class/imvotenet_faster_rcnn_r50_fpn_2x4_sunrgbd-3d-10class_20210819_225618-62eba6ce.pth
+
+ - Name: imvotenet_stage2_16x8_sunrgbd-3d-10class
+ In Collection: ImVoteNet
+ Config: configs/imvotenet/imvotenet_stage2_16x8_sunrgbd-3d-10class.py
+ Metadata:
+ Training Memory (GB): 9.4
+ Results:
+ - Task: 3D Object Detection
+ Dataset: SUNRGBD-3D
+ Metrics:
+ AP@0.25: 64.55
+ Weights: https://download.openmmlab.com/mmdetection3d/v1.0.0_models/imvotenet/imvotenet_stage2_16x8_sunrgbd-3d-10class/imvotenet_stage2_16x8_sunrgbd-3d-10class_20210819_192851-1bcd1b97.pth
diff --git a/configs/imvoxelnet/README.md b/configs/imvoxelnet/README.md
new file mode 100644
index 0000000..faaddf2
--- /dev/null
+++ b/configs/imvoxelnet/README.md
@@ -0,0 +1,38 @@
+# ImVoxelNet: Image to Voxels Projection for Monocular and Multi-View General-Purpose 3D Object Detection
+
+> [ImVoxelNet: Image to Voxels Projection for Monocular and Multi-View General-Purpose 3D Object Detection](https://arxiv.org/abs/2106.01178)
+
+
+
+## Abstract
+
+In this paper, we introduce the task of multi-view RGB-based 3D object detection as an end-to-end optimization problem. To address this problem, we propose ImVoxelNet, a novel fully convolutional method of 3D object detection based on posed monocular or multi-view RGB images. The number of monocular images in each multiview input can variate during training and inference; actually, this number might be unique for each multi-view input. ImVoxelNet successfully handles both indoor and outdoor scenes, which makes it general-purpose. Specifically, it achieves state-of-the-art results in car detection on KITTI (monocular) and nuScenes (multi-view) benchmarks among all methods that accept RGB images. Moreover, it surpasses existing RGB-based 3D object detection methods on the SUN RGB-D dataset. On ScanNet, ImVoxelNet sets a new benchmark for multi-view 3D object detection.
+
+
+
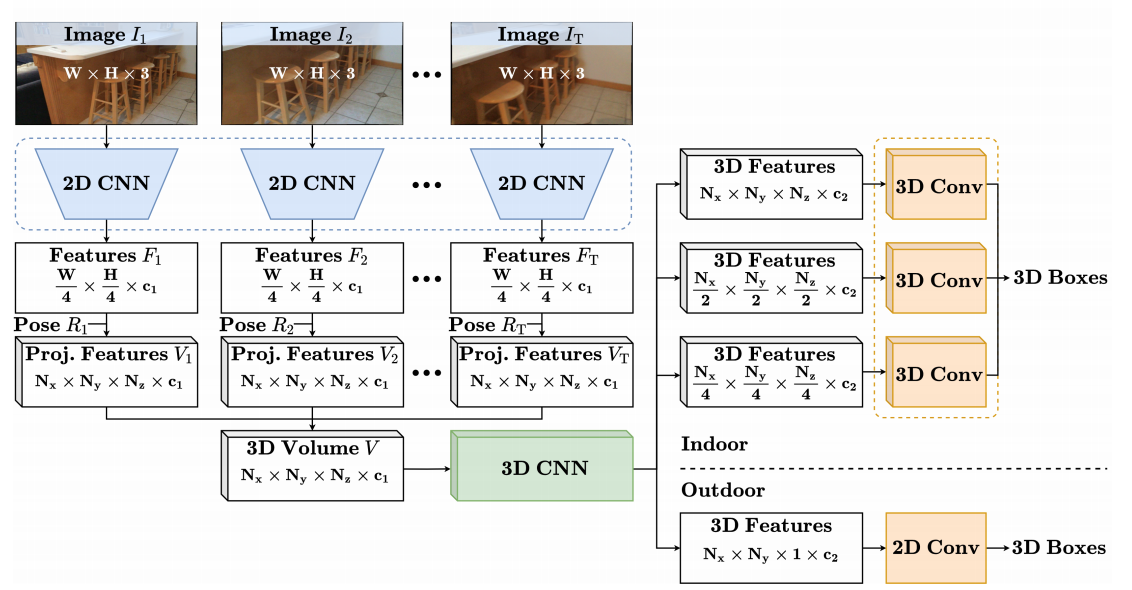
+
+
+## Introduction
+
+We implement a monocular 3D detector ImVoxelNet and provide its results and checkpoints on KITTI dataset.
+Results for SUN RGB-D, ScanNet and nuScenes are currently available in ImVoxelNet authors
+[repo](https://github.com/saic-vul/imvoxelnet) (based on mmdetection3d).
+
+## Results and models
+
+### KITTI
+
+| Backbone | Class | Lr schd | Mem (GB) | Inf time (fps) | mAP | Download |
+| :---------------------------------------: | :---: | :-----: | :------: | :------------: | :---: | :----------------------------------------------------------------------------------------------------------------------------------------------------------------------------------------------------------------------------------------------------------------------------------------------------------------------------------: |
+| [ResNet-50](./imvoxelnet_kitti-3d-car.py) | Car | 3x | | | 17.26 | [model](https://download.openmmlab.com/mmdetection3d/v1.0.0_models/imvoxelnet/imvoxelnet_4x8_kitti-3d-car/imvoxelnet_4x8_kitti-3d-car_20210830_003014-3d0ffdf4.pth) \| [log](https://download.openmmlab.com/mmdetection3d/v1.0.0_models/imvoxelnet/imvoxelnet_4x8_kitti-3d-car/imvoxelnet_4x8_kitti-3d-car_20210830_003014.log.json) |
+
+## Citation
+
+```latex
+@article{rukhovich2021imvoxelnet,
+ title={ImVoxelNet: Image to Voxels Projection for Monocular and Multi-View General-Purpose 3D Object Detection},
+ author={Danila Rukhovich, Anna Vorontsova, Anton Konushin},
+ journal={arXiv preprint arXiv:2106.01178},
+ year={2021}
+}
+```
diff --git a/configs/imvoxelnet/imvoxelnet_4x8_kitti-3d-car.py b/configs/imvoxelnet/imvoxelnet_4x8_kitti-3d-car.py
new file mode 100644
index 0000000..06ebe62
--- /dev/null
+++ b/configs/imvoxelnet/imvoxelnet_4x8_kitti-3d-car.py
@@ -0,0 +1,160 @@
+model = dict(
+ type='ImVoxelNet',
+ backbone=dict(
+ type='ResNet',
+ depth=50,
+ num_stages=4,
+ out_indices=(0, 1, 2, 3),
+ frozen_stages=1,
+ norm_cfg=dict(type='BN', requires_grad=False),
+ norm_eval=True,
+ init_cfg=dict(type='Pretrained', checkpoint='torchvision://resnet50'),
+ style='pytorch'),
+ neck=dict(
+ type='FPN',
+ in_channels=[256, 512, 1024, 2048],
+ out_channels=64,
+ num_outs=4),
+ neck_3d=dict(type='OutdoorImVoxelNeck', in_channels=64, out_channels=256),
+ bbox_head=dict(
+ type='Anchor3DHead',
+ num_classes=1,
+ in_channels=256,
+ feat_channels=256,
+ use_direction_classifier=True,
+ anchor_generator=dict(
+ type='AlignedAnchor3DRangeGenerator',
+ ranges=[[-0.16, -39.68, -1.78, 68.96, 39.68, -1.78]],
+ sizes=[[3.9, 1.6, 1.56]],
+ rotations=[0, 1.57],
+ reshape_out=True),
+ diff_rad_by_sin=True,
+ bbox_coder=dict(type='DeltaXYZWLHRBBoxCoder'),
+ loss_cls=dict(
+ type='FocalLoss',
+ use_sigmoid=True,
+ gamma=2.0,
+ alpha=0.25,
+ loss_weight=1.0),
+ loss_bbox=dict(type='SmoothL1Loss', beta=1.0 / 9.0, loss_weight=2.0),
+ loss_dir=dict(
+ type='CrossEntropyLoss', use_sigmoid=False, loss_weight=0.2)),
+ n_voxels=[216, 248, 12],
+ anchor_generator=dict(
+ type='AlignedAnchor3DRangeGenerator',
+ ranges=[[-0.16, -39.68, -3.08, 68.96, 39.68, 0.76]],
+ rotations=[.0]),
+ train_cfg=dict(
+ assigner=dict(
+ type='MaxIoUAssigner',
+ iou_calculator=dict(type='BboxOverlapsNearest3D'),
+ pos_iou_thr=0.6,
+ neg_iou_thr=0.45,
+ min_pos_iou=0.45,
+ ignore_iof_thr=-1),
+ allowed_border=0,
+ pos_weight=-1,
+ debug=False),
+ test_cfg=dict(
+ use_rotate_nms=True,
+ nms_across_levels=False,
+ nms_thr=0.01,
+ score_thr=0.1,
+ min_bbox_size=0,
+ nms_pre=100,
+ max_num=50))
+
+dataset_type = 'KittiDataset'
+data_root = 'data/kitti/'
+class_names = ['Car']
+input_modality = dict(use_lidar=False, use_camera=True)
+point_cloud_range = [0, -39.68, -3, 69.12, 39.68, 1]
+img_norm_cfg = dict(
+ mean=[123.675, 116.28, 103.53], std=[58.395, 57.12, 57.375], to_rgb=True)
+
+train_pipeline = [
+ dict(type='LoadAnnotations3D'),
+ dict(type='LoadImageFromFile'),
+ dict(type='RandomFlip3D', flip_ratio_bev_horizontal=0.5),
+ dict(
+ type='Resize',
+ img_scale=[(1173, 352), (1387, 416)],
+ keep_ratio=True,
+ multiscale_mode='range'),
+ dict(type='Normalize', **img_norm_cfg),
+ dict(type='Pad', size_divisor=32),
+ dict(type='ObjectRangeFilter', point_cloud_range=point_cloud_range),
+ dict(type='DefaultFormatBundle3D', class_names=class_names),
+ dict(type='Collect3D', keys=['img', 'gt_bboxes_3d', 'gt_labels_3d'])
+]
+test_pipeline = [
+ dict(type='LoadImageFromFile'),
+ dict(type='Resize', img_scale=(1280, 384), keep_ratio=True),
+ dict(type='Normalize', **img_norm_cfg),
+ dict(type='Pad', size_divisor=32),
+ dict(
+ type='DefaultFormatBundle3D',
+ class_names=class_names,
+ with_label=False),
+ dict(type='Collect3D', keys=['img'])
+]
+
+data = dict(
+ samples_per_gpu=4,
+ workers_per_gpu=4,
+ train=dict(
+ type='RepeatDataset',
+ times=3,
+ dataset=dict(
+ type=dataset_type,
+ data_root=data_root,
+ ann_file=data_root + 'kitti_infos_train.pkl',
+ split='training',
+ pts_prefix='velodyne_reduced',
+ pipeline=train_pipeline,
+ modality=input_modality,
+ classes=class_names,
+ test_mode=False)),
+ val=dict(
+ type=dataset_type,
+ data_root=data_root,
+ ann_file=data_root + 'kitti_infos_val.pkl',
+ split='training',
+ pts_prefix='velodyne_reduced',
+ pipeline=test_pipeline,
+ modality=input_modality,
+ classes=class_names,
+ test_mode=True),
+ test=dict(
+ type=dataset_type,
+ data_root=data_root,
+ ann_file=data_root + 'kitti_infos_val.pkl',
+ split='training',
+ pts_prefix='velodyne_reduced',
+ pipeline=test_pipeline,
+ modality=input_modality,
+ classes=class_names,
+ test_mode=True))
+
+optimizer = dict(
+ type='AdamW',
+ lr=0.0001,
+ weight_decay=0.0001,
+ paramwise_cfg=dict(
+ custom_keys={'backbone': dict(lr_mult=0.1, decay_mult=1.0)}))
+optimizer_config = dict(grad_clip=dict(max_norm=35., norm_type=2))
+lr_config = dict(policy='step', step=[8, 11])
+total_epochs = 12
+
+checkpoint_config = dict(interval=1, max_keep_ckpts=1)
+log_config = dict(
+ interval=50,
+ hooks=[dict(type='TextLoggerHook'),
+ dict(type='TensorboardLoggerHook')])
+evaluation = dict(interval=1)
+dist_params = dict(backend='nccl')
+find_unused_parameters = True # only 1 of 4 FPN outputs is used
+log_level = 'INFO'
+load_from = None
+resume_from = None
+workflow = [('train', 1)]
diff --git a/configs/imvoxelnet/metafile.yml b/configs/imvoxelnet/metafile.yml
new file mode 100644
index 0000000..0dea486
--- /dev/null
+++ b/configs/imvoxelnet/metafile.yml
@@ -0,0 +1,29 @@
+Collections:
+ - Name: ImVoxelNet
+ Metadata:
+ Training Data: KITTI
+ Training Techniques:
+ - AdamW
+ Training Resources: 8x Tesla P40
+ Architecture:
+ - Anchor3DHead
+ Paper:
+ URL: https://arxiv.org/abs/2106.01178
+ Title: 'ImVoxelNet: Image to Voxels Projection for Monocular and Multi-View General-Purpose 3D Object Detection'
+ README: configs/imvoxelnet/README.md
+ Code:
+ URL: https://github.com/open-mmlab/mmdetection3d/blob/master/mmdet3d/models/detectors/imvoxelnet.py#L11
+ Version: v0.15.0
+
+Models:
+ - Name: imvoxelnet_kitti-3d-car
+ In Collection: ImVoxelNet
+ Config: configs/imvoxelnet/imvoxelnet_kitti-3d-car.py
+ Metadata:
+ Training Memory (GB): 15.0
+ Results:
+ - Task: 3D Object Detection
+ Dataset: KITTI
+ Metrics:
+ mAP: 17.26
+ Weights: https://download.openmmlab.com/mmdetection3d/v1.0.0_models/imvoxelnet/imvoxelnet_4x8_kitti-3d-car/imvoxelnet_4x8_kitti-3d-car_20210830_003014-3d0ffdf4.pth
diff --git a/configs/monoflex/README.md b/configs/monoflex/README.md
new file mode 100644
index 0000000..0f402be
--- /dev/null
+++ b/configs/monoflex/README.md
@@ -0,0 +1,48 @@
+# Objects are Different: Flexible Monocular 3D Object Detection
+
+> [Objects are Different: Flexible Monocular 3D Object Detection](https://arxiv.org/abs/2104.02323)
+
+
+
+## Abstract
+
+The precise localization of 3D objects from a single image without depth information is a highly challenging problem. Most existing methods adopt the same approach for all objects regardless of their diverse distributions, leading to limited performance for truncated objects. In this paper, we propose a flexible framework for monocular 3D object detection which explicitly decouples the truncated objects and adaptively combines multiple approaches for object depth estimation. Specifically, we decouple the edge of the feature map for predicting long-tail truncated objects so that the optimization of normal objects is not influenced. Furthermore, we formulate the object depth estimation as an uncertainty-guided ensemble of directly regressed object depth and solved depths from different groups of keypoints. Experiments demonstrate that our method outperforms the state-of-the-art method by relatively 27% for the moderate level and 30% for the hard level in the test set of KITTI benchmark while maintaining real-time efficiency.
+
+
+
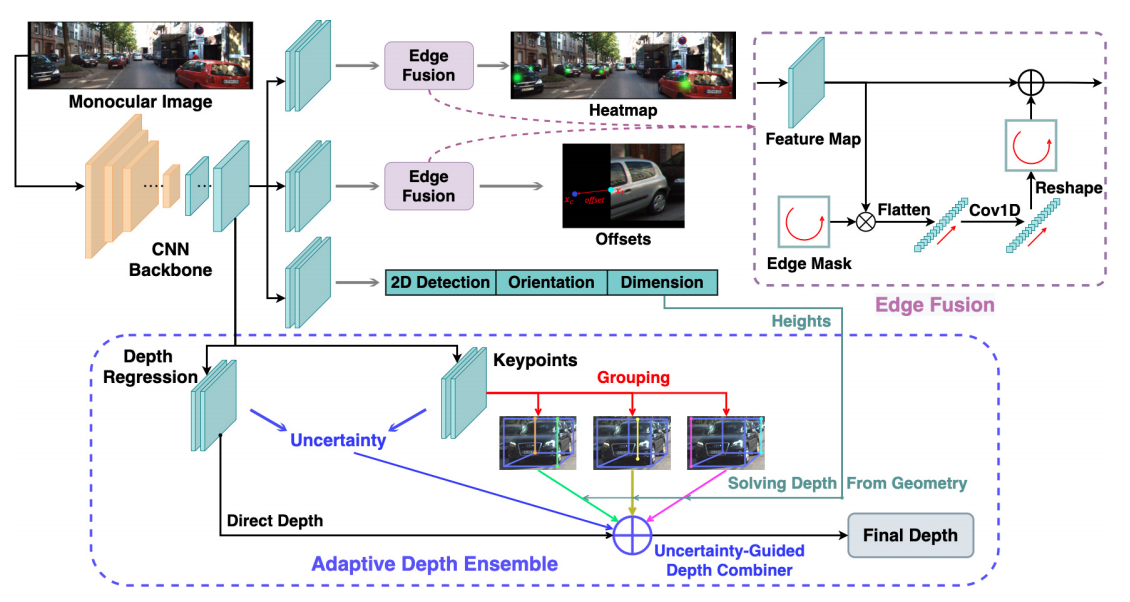
+
+
+## Introduction
+
+We implement MonoFlex and provide the results and checkpoints on KITTI dataset.
+
+## Results and models
+
+### KITTI
+
+| Backbone | Lr schd | Mem (GB) | Inf time (fps) | mAP | Download |
+| :---------------------------------------------------------------------: | :-----: | :------: | :------------: | :---: | :----------------------------------------------------------------------------------------------------------------------------------------------------------------------------------------------------------------------------------------------------------------------------------------------------------------------------------: |
+| [DLA34](./monoflex_dla34_pytorch_dlaneck_gn-all_2x4_6x_kitti-mono3d.py) | 6x | 9.64 | | 21.86 | [model](https://download.openmmlab.com/mmdetection3d/v0.1.0_models/monoflex/monoflex_dla34_pytorch_dlaneck_gn-all_2x4_6x_kitti-mono3d_20211228_027553-d46d9bb0.pth) \| [log](https://download.openmmlab.com/mmdetection3d/v0.1.0_models/monoflex/monoflex_dla34_pytorch_dlaneck_gn-all_2x4_6x_kitti-mono3d_20211228_027553.log.json) |
+
+Note: mAP represents Car moderate 3D strict AP11 results.
+Detailed performance on KITTI 3D detection (3D/BEV) is as follows, evaluated by AP11 and AP40 metric:
+
+| | Easy | Moderate | Hard |
+| ---------- | :-----------: | :-----------: | :-----------: |
+| Car (AP11) | 28.02 / 36.11 | 21.86 / 29.46 | 19.01 / 24.83 |
+| Car (AP40) | 23.22 / 32.74 | 17.18 / 24.02 | 15.13 / 20.67 |
+
+Note: mAP represents Car moderate 3D strict AP11 / AP40 results. Because of the limited data for pedestrians and cyclists, the detection performance for these two classes is usually unstable. Therefore, we only list car detection results here. In addition, the AP11 result may fluctuate in a larger range (~1 AP), so AP40 is a more recommended metric for reference due to its much better stability.
+
+## Citation
+
+```latex
+@InProceedings{MonoFlex,
+ author = {Zhang, Yunpeng and Lu, Jiwen and Zhou, Jie},
+ title = {Objects Are Different: Flexible Monocular 3D Object Detection},
+ booktitle = {Proceedings of the IEEE/CVF Conference on Computer Vision and Pattern Recognition (CVPR)},
+ month = {June},
+ year = {2021},
+ pages = {3289-3298}
+}
+```
diff --git a/configs/monoflex/metafile.yml b/configs/monoflex/metafile.yml
new file mode 100644
index 0000000..c64dd6f
--- /dev/null
+++ b/configs/monoflex/metafile.yml
@@ -0,0 +1,30 @@
+Collections:
+ - Name: MonoFlex
+ Metadata:
+ Training Data: KITTI
+ Training Techniques:
+ - Adam
+ Training Resources: 2x V100 GPUS
+ Architecture:
+ - MonoFlexHead
+ - DLA
+ Paper:
+ URL: https://arxiv.org/abs/2104.02323
+ Title: 'Objects are Different: Flexible Monocular 3D Object Detection'
+ README: configs/monoflex/README.md
+ Code:
+ URL: https://github.com/open-mmlab/mmdetection3d/blob/v1.0.0.dev0/mmdet3d/models/detectors/monoflex.py#L7
+ Version: v1.0.0
+
+Models:
+ - Name: monoflex_dla34_pytorch_dlaneck_gn-all_2x4_6x_kitti-mono3d
+ In Collection: MonoFlex
+ Config: configs/monoflex/monoflex_dla34_pytorch_dlaneck_gn-all_2x4_6x_kitti-mono3d.py
+ Metadata:
+ Training Memory (GB): 9.64
+ Results:
+ - Task: 3D Object Detection
+ Dataset: KITTI
+ Metrics:
+ mAP: 21.98
+ Weights: https://download.openmmlab.com/mmdetection3d/v0.1.0_models/monoflex/monoflex_dla34_pytorch_dlaneck_gn-all_2x4_6x_kitti-mono3d_20211228_027553-d46d9bb0.pth
diff --git a/configs/mvxnet/README.md b/configs/mvxnet/README.md
new file mode 100644
index 0000000..d786efa
--- /dev/null
+++ b/configs/mvxnet/README.md
@@ -0,0 +1,38 @@
+# MVX-Net: Multimodal VoxelNet for 3D Object Detection
+
+> [MVX-Net: Multimodal VoxelNet for 3D Object Detection](https://arxiv.org/abs/1904.01649)
+
+
+
+## Abstract
+
+Many recent works on 3D object detection have focused on designing neural network architectures that can consume point cloud data. While these approaches demonstrate encouraging performance, they are typically based on a single modality and are unable to leverage information from other modalities, such as a camera. Although a few approaches fuse data from different modalities, these methods either use a complicated pipeline to process the modalities sequentially, or perform late-fusion and are unable to learn interaction between different modalities at early stages. In this work, we present PointFusion and VoxelFusion: two simple yet effective early-fusion approaches to combine the RGB and point cloud modalities, by leveraging the recently introduced VoxelNet architecture. Evaluation on the KITTI dataset demonstrates significant improvements in performance over approaches which only use point cloud data. Furthermore, the proposed method provides results competitive with the state-of-the-art multimodal algorithms, achieving top-2 ranking in five of the six bird's eye view and 3D detection categories on the KITTI benchmark, by using a simple single stage network.
+
+
+
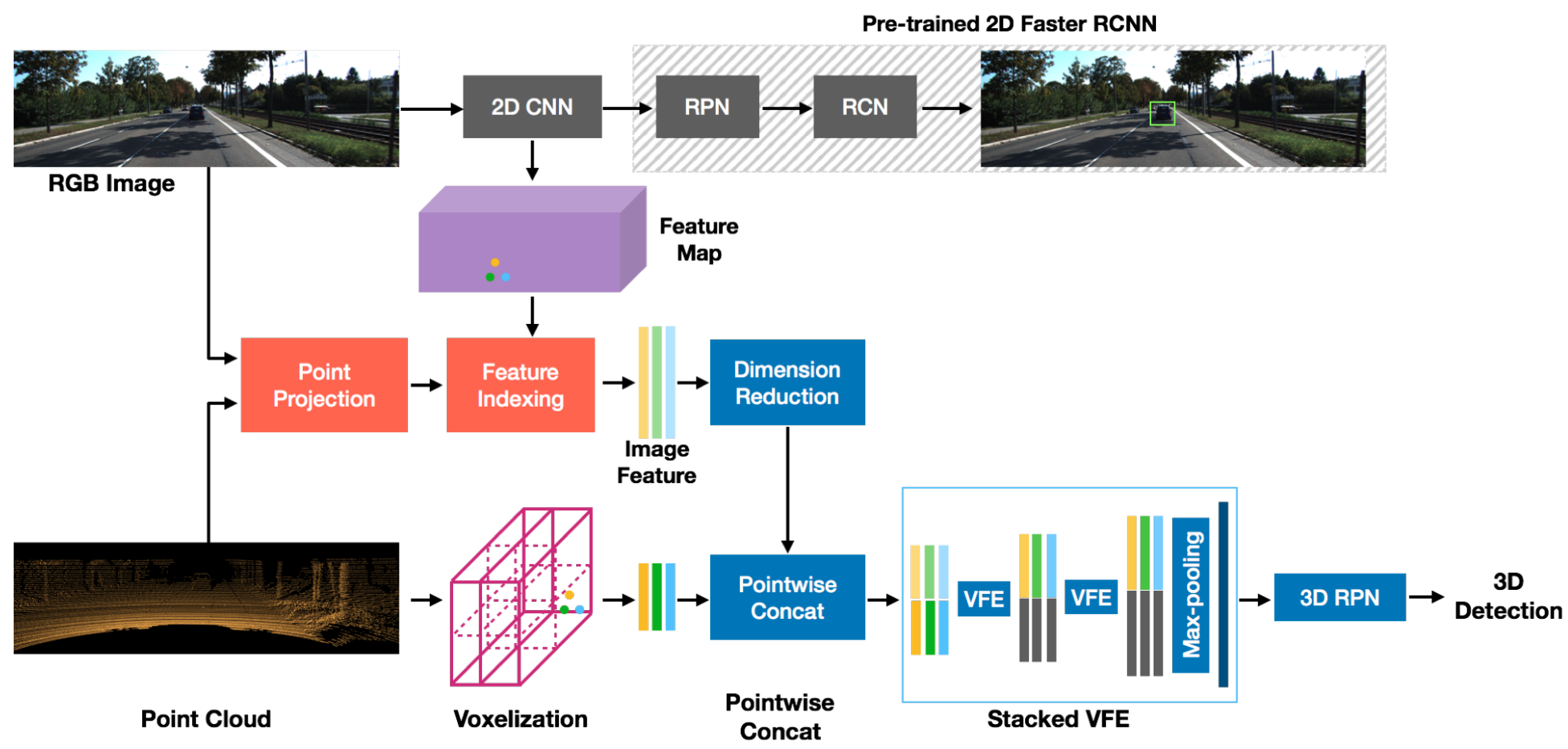
+
+
+## Introduction
+
+We implement MVX-Net and provide its results and models on KITTI dataset.
+
+## Results and models
+
+### KITTI
+
+| Backbone | Class | Lr schd | Mem (GB) | Inf time (fps) | mAP | Download |
+| :-------------------------------------------------------------------: | :-----: | :--------: | :------: | :------------: | :---: | :--------------------------------------------------------------------------------------------------------------------------------------------------------------------------------------------------------------------------------------------------------------------------------------------------------------------------------------------------------------------------------------------------------------------------------------: |
+| [SECFPN](./dv_mvx-fpn_second_secfpn_adamw_2x8_80e_kitti-3d-3class.py) | 3 Class | cosine 80e | 6.7 | | 63.22 | [model](https://download.openmmlab.com/mmdetection3d/v1.0.0_models/mvxnet/dv_mvx-fpn_second_secfpn_adamw_2x8_80e_kitti-3d-3class/dv_mvx-fpn_second_secfpn_adamw_2x8_80e_kitti-3d-3class_20210831_060805-83442923.pth) \| [log](https://download.openmmlab.com/mmdetection3d/v1.0.0_models/mvxnet/dv_mvx-fpn_second_secfpn_adamw_2x8_80e_kitti-3d-3class/dv_mvx-fpn_second_secfpn_adamw_2x8_80e_kitti-3d-3class_20210831_060805.log.json) |
+
+## Citation
+
+```latex
+@inproceedings{sindagi2019mvx,
+ title={MVX-Net: Multimodal voxelnet for 3D object detection},
+ author={Sindagi, Vishwanath A and Zhou, Yin and Tuzel, Oncel},
+ booktitle={2019 International Conference on Robotics and Automation (ICRA)},
+ pages={7276--7282},
+ year={2019},
+ organization={IEEE}
+}
+```
diff --git a/configs/mvxnet/dv_mvx-fpn_second_secfpn_adamw_2x8_80e_kitti-3d-3class.py b/configs/mvxnet/dv_mvx-fpn_second_secfpn_adamw_2x8_80e_kitti-3d-3class.py
new file mode 100644
index 0000000..e9f592f
--- /dev/null
+++ b/configs/mvxnet/dv_mvx-fpn_second_secfpn_adamw_2x8_80e_kitti-3d-3class.py
@@ -0,0 +1,251 @@
+_base_ = ['../_base_/schedules/cosine.py', '../_base_/default_runtime.py']
+
+# model settings
+voxel_size = [0.05, 0.05, 0.1]
+point_cloud_range = [0, -40, -3, 70.4, 40, 1]
+
+model = dict(
+ type='DynamicMVXFasterRCNN',
+ img_backbone=dict(
+ type='ResNet',
+ depth=50,
+ num_stages=4,
+ out_indices=(0, 1, 2, 3),
+ frozen_stages=1,
+ norm_cfg=dict(type='BN', requires_grad=False),
+ norm_eval=True,
+ style='caffe'),
+ img_neck=dict(
+ type='FPN',
+ in_channels=[256, 512, 1024, 2048],
+ out_channels=256,
+ num_outs=5),
+ pts_voxel_layer=dict(
+ max_num_points=-1,
+ point_cloud_range=point_cloud_range,
+ voxel_size=voxel_size,
+ max_voxels=(-1, -1),
+ ),
+ pts_voxel_encoder=dict(
+ type='DynamicVFE',
+ in_channels=4,
+ feat_channels=[64, 64],
+ with_distance=False,
+ voxel_size=voxel_size,
+ with_cluster_center=True,
+ with_voxel_center=True,
+ point_cloud_range=point_cloud_range,
+ fusion_layer=dict(
+ type='PointFusion',
+ img_channels=256,
+ pts_channels=64,
+ mid_channels=128,
+ out_channels=128,
+ img_levels=[0, 1, 2, 3, 4],
+ align_corners=False,
+ activate_out=True,
+ fuse_out=False)),
+ pts_middle_encoder=dict(
+ type='SparseEncoder',
+ in_channels=128,
+ sparse_shape=[41, 1600, 1408],
+ order=('conv', 'norm', 'act')),
+ pts_backbone=dict(
+ type='SECOND',
+ in_channels=256,
+ layer_nums=[5, 5],
+ layer_strides=[1, 2],
+ out_channels=[128, 256]),
+ pts_neck=dict(
+ type='SECONDFPN',
+ in_channels=[128, 256],
+ upsample_strides=[1, 2],
+ out_channels=[256, 256]),
+ pts_bbox_head=dict(
+ type='Anchor3DHead',
+ num_classes=3,
+ in_channels=512,
+ feat_channels=512,
+ use_direction_classifier=True,
+ anchor_generator=dict(
+ type='Anchor3DRangeGenerator',
+ ranges=[
+ [0, -40.0, -0.6, 70.4, 40.0, -0.6],
+ [0, -40.0, -0.6, 70.4, 40.0, -0.6],
+ [0, -40.0, -1.78, 70.4, 40.0, -1.78],
+ ],
+ sizes=[[0.8, 0.6, 1.73], [1.76, 0.6, 1.73], [3.9, 1.6, 1.56]],
+ rotations=[0, 1.57],
+ reshape_out=False),
+ assigner_per_size=True,
+ diff_rad_by_sin=True,
+ assign_per_class=True,
+ bbox_coder=dict(type='DeltaXYZWLHRBBoxCoder'),
+ loss_cls=dict(
+ type='FocalLoss',
+ use_sigmoid=True,
+ gamma=2.0,
+ alpha=0.25,
+ loss_weight=1.0),
+ loss_bbox=dict(type='SmoothL1Loss', beta=1.0 / 9.0, loss_weight=2.0),
+ loss_dir=dict(
+ type='CrossEntropyLoss', use_sigmoid=False, loss_weight=0.2)),
+ # model training and testing settings
+ train_cfg=dict(
+ pts=dict(
+ assigner=[
+ dict( # for Pedestrian
+ type='MaxIoUAssigner',
+ iou_calculator=dict(type='BboxOverlapsNearest3D'),
+ pos_iou_thr=0.35,
+ neg_iou_thr=0.2,
+ min_pos_iou=0.2,
+ ignore_iof_thr=-1),
+ dict( # for Cyclist
+ type='MaxIoUAssigner',
+ iou_calculator=dict(type='BboxOverlapsNearest3D'),
+ pos_iou_thr=0.35,
+ neg_iou_thr=0.2,
+ min_pos_iou=0.2,
+ ignore_iof_thr=-1),
+ dict( # for Car
+ type='MaxIoUAssigner',
+ iou_calculator=dict(type='BboxOverlapsNearest3D'),
+ pos_iou_thr=0.6,
+ neg_iou_thr=0.45,
+ min_pos_iou=0.45,
+ ignore_iof_thr=-1),
+ ],
+ allowed_border=0,
+ pos_weight=-1,
+ debug=False)),
+ test_cfg=dict(
+ pts=dict(
+ use_rotate_nms=True,
+ nms_across_levels=False,
+ nms_thr=0.01,
+ score_thr=0.1,
+ min_bbox_size=0,
+ nms_pre=100,
+ max_num=50)))
+
+# dataset settings
+dataset_type = 'KittiDataset'
+data_root = 'data/kitti/'
+class_names = ['Pedestrian', 'Cyclist', 'Car']
+img_norm_cfg = dict(
+ mean=[103.530, 116.280, 123.675], std=[1.0, 1.0, 1.0], to_rgb=False)
+input_modality = dict(use_lidar=True, use_camera=True)
+train_pipeline = [
+ dict(type='LoadPointsFromFile', coord_type='LIDAR', load_dim=4, use_dim=4),
+ dict(type='LoadImageFromFile'),
+ dict(type='LoadAnnotations3D', with_bbox_3d=True, with_label_3d=True),
+ dict(
+ type='Resize',
+ img_scale=[(640, 192), (2560, 768)],
+ multiscale_mode='range',
+ keep_ratio=True),
+ dict(
+ type='GlobalRotScaleTrans',
+ rot_range=[-0.78539816, 0.78539816],
+ scale_ratio_range=[0.95, 1.05],
+ translation_std=[0.2, 0.2, 0.2]),
+ dict(type='RandomFlip3D', flip_ratio_bev_horizontal=0.5),
+ dict(type='PointsRangeFilter', point_cloud_range=point_cloud_range),
+ dict(type='ObjectRangeFilter', point_cloud_range=point_cloud_range),
+ dict(type='PointShuffle'),
+ dict(type='Normalize', **img_norm_cfg),
+ dict(type='Pad', size_divisor=32),
+ dict(type='DefaultFormatBundle3D', class_names=class_names),
+ dict(
+ type='Collect3D',
+ keys=['points', 'img', 'gt_bboxes_3d', 'gt_labels_3d']),
+]
+test_pipeline = [
+ dict(type='LoadPointsFromFile', coord_type='LIDAR', load_dim=4, use_dim=4),
+ dict(type='LoadImageFromFile'),
+ dict(
+ type='MultiScaleFlipAug3D',
+ img_scale=(1280, 384),
+ pts_scale_ratio=1,
+ flip=False,
+ transforms=[
+ dict(type='Resize', multiscale_mode='value', keep_ratio=True),
+ dict(
+ type='GlobalRotScaleTrans',
+ rot_range=[0, 0],
+ scale_ratio_range=[1., 1.],
+ translation_std=[0, 0, 0]),
+ dict(type='RandomFlip3D'),
+ dict(type='Normalize', **img_norm_cfg),
+ dict(type='Pad', size_divisor=32),
+ dict(
+ type='PointsRangeFilter', point_cloud_range=point_cloud_range),
+ dict(
+ type='DefaultFormatBundle3D',
+ class_names=class_names,
+ with_label=False),
+ dict(type='Collect3D', keys=['points', 'img'])
+ ])
+]
+# construct a pipeline for data and gt loading in show function
+# please keep its loading function consistent with test_pipeline (e.g. client)
+eval_pipeline = [
+ dict(type='LoadPointsFromFile', coord_type='LIDAR', load_dim=4, use_dim=4),
+ dict(type='LoadImageFromFile'),
+ dict(
+ type='DefaultFormatBundle3D',
+ class_names=class_names,
+ with_label=False),
+ dict(type='Collect3D', keys=['points', 'img'])
+]
+
+data = dict(
+ samples_per_gpu=2,
+ workers_per_gpu=2,
+ train=dict(
+ type='RepeatDataset',
+ times=2,
+ dataset=dict(
+ type=dataset_type,
+ data_root=data_root,
+ ann_file=data_root + 'kitti_infos_train.pkl',
+ split='training',
+ pts_prefix='velodyne_reduced',
+ pipeline=train_pipeline,
+ modality=input_modality,
+ classes=class_names,
+ test_mode=False,
+ box_type_3d='LiDAR')),
+ val=dict(
+ type=dataset_type,
+ data_root=data_root,
+ ann_file=data_root + 'kitti_infos_val.pkl',
+ split='training',
+ pts_prefix='velodyne_reduced',
+ pipeline=test_pipeline,
+ modality=input_modality,
+ classes=class_names,
+ test_mode=True,
+ box_type_3d='LiDAR'),
+ test=dict(
+ type=dataset_type,
+ data_root=data_root,
+ ann_file=data_root + 'kitti_infos_val.pkl',
+ split='training',
+ pts_prefix='velodyne_reduced',
+ pipeline=test_pipeline,
+ modality=input_modality,
+ classes=class_names,
+ test_mode=True,
+ box_type_3d='LiDAR'))
+
+# Training settings
+optimizer = dict(weight_decay=0.01)
+# max_norm=10 is better for SECOND
+optimizer_config = dict(grad_clip=dict(max_norm=35, norm_type=2))
+
+evaluation = dict(interval=1, pipeline=eval_pipeline)
+
+# You may need to download the model first is the network is unstable
+load_from = 'https://download.openmmlab.com/mmdetection3d/pretrain_models/mvx_faster_rcnn_detectron2-caffe_20e_coco-pretrain_gt-sample_kitti-3-class_moderate-79.3_20200207-a4a6a3c7.pth' # noqa
diff --git a/configs/mvxnet/metafile.yml b/configs/mvxnet/metafile.yml
new file mode 100644
index 0000000..4ce10b7
--- /dev/null
+++ b/configs/mvxnet/metafile.yml
@@ -0,0 +1,30 @@
+Collections:
+ - Name: MVX-Net
+ Metadata:
+ Training Data: KITTI
+ Training Techniques:
+ - AdamW
+ Training Resources: 8x V100 GPUs
+ Architecture:
+ - Feature Pyramid Network
+ - Dynamic Voxelization
+ Paper:
+ URL: https://arxiv.org/abs/1904.01649
+ Title: 'MVX-Net: Multimodal VoxelNet for 3D Object Detection'
+ README: configs/mvxnet/README.md
+ Code:
+ URL: https://github.com/open-mmlab/mmdetection3d/blob/master/mmdet3d/models/detectors/mvx_two_stage.py#L20
+ Version: v0.5.0
+
+Models:
+ - Name: dv_mvx-fpn_second_secfpn_adamw_2x8_80e_kitti-3d-3class
+ In Collection: MVX-Net
+ Config: configs/mvxnet/dv_mvx-fpn_second_secfpn_adamw_2x8_80e_kitti-3d-3class.py
+ Metadata:
+ Training Memory (GB): 6.7
+ Results:
+ - Task: 3D Object Detection
+ Dataset: KITTI
+ Metrics:
+ mAP: 63.22
+ Weights: https://download.openmmlab.com/mmdetection3d/v1.0.0_models/mvxnet/dv_mvx-fpn_second_secfpn_adamw_2x8_80e_kitti-3d-3class/dv_mvx-fpn_second_secfpn_adamw_2x8_80e_kitti-3d-3class_20210831_060805-83442923.pth
diff --git a/configs/nuimages/README.md b/configs/nuimages/README.md
new file mode 100644
index 0000000..9106229
--- /dev/null
+++ b/configs/nuimages/README.md
@@ -0,0 +1,59 @@
+# NuImages Results
+
+
+
+## Introduction
+
+We support and provide some baseline results on [nuImages dataset](https://www.nuscenes.org/nuimages).
+We follow the class mapping in nuScenes dataset, which maps the original categories into 10 foreground categories.
+The convert script can be found [here](https://github.com/open-mmlab/mmdetection3d/blob/master/tools/data_converter/nuimage_converter.py).
+The baseline results include instance segmentation models, e.g., Mask R-CNN, Cascade Mask R-CNN, and HTC.
+We will support panoptic segmentation models in the future.
+
+
+
+The dataset converted by the script of v0.6.0 only supports instance segmentation. Since v0.7.0, we also support to produce semantic segmentation mask of each image; thus, we can train HTC or semantic segmentation models using the dataset. To convert the nuImages dataset into COCO format, please use the command below:
+
+```shell
+python -u tools/data_converter/nuimage_converter.py --data-root ${DATA_ROOT} --version ${VERSIONS} \
+ --out-dir ${OUT_DIR} --nproc ${NUM_WORKERS} --extra-tag ${TAG}
+```
+
+- `--data-root`: the root of the dataset, defaults to `./data/nuimages`.
+- `--version`: the version of the dataset, defaults to `v1.0-mini`. To get the full dataset, please use `--version v1.0-train v1.0-val v1.0-mini`
+- `--out-dir`: the output directory of annotations and semantic masks, defaults to `./data/nuimages/annotations/`.
+- `--nproc`: number of workers for data preparation, defaults to `4`. Larger number could reduce the preparation time as images are processed in parallel.
+- `--extra-tag`: extra tag of the annotations, defaults to `nuimages`. This can be used to separate different annotations processed in different time for study.
+
+## Results and models
+
+### Instance Segmentation
+
+We report Mask R-CNN and Cascade Mask R-CNN results on nuimages.
+
+| Method | Backbone | Pretraining | Lr schd | Mem (GB) | Box AP | Mask AP | Download |
+| :----------------: | :-----------------------------------------------------------------------------------: | :---------: | :-----: | :------: | :------------------------------------------------------------------------------------------------------------------------------------------------------------------------: | :-----: | :----------------------------------------------------------------------------------------------------------------------------------------------------------------------------------------------------------------------------------------------------------------------------------------------------------------------------------------------------------------------------------------------------------------------------------------------------: |
+| Mask R-CNN | [R-50](./mask_rcnn_r50_fpn_1x_nuim.py) | IN | 1x | 7.4 | 47.8 | 38.4 | [model](https://download.openmmlab.com/mmdetection3d/v0.1.0_models/nuimages_semseg/mask_rcnn_r50_fpn_1x_nuim/mask_rcnn_r50_fpn_1x_nuim_20201008_195238-e99f5182.pth) \| [log](https://download.openmmlab.com/mmdetection3d/v0.1.0_models/nuimages_semseg/mask_rcnn_r50_fpn_1x_nuim/mask_rcnn_r50_fpn_1x_nuim_20201008_195238.log.json) |
+| Mask R-CNN | [R-50](./mask_rcnn_r50_fpn_coco-2x_1x_nuim.py) | IN+COCO-2x | 1x | 7.4 | 49.7 | 40.5 | [model](https://download.openmmlab.com/mmdetection3d/v0.1.0_models/nuimages_semseg/mask_rcnn_r50_fpn_coco-2x_1x_nuim/mask_rcnn_r50_fpn_coco-2x_1x_nuim_20201008_195238-b1742a60.pth) \| [log](https://download.openmmlab.com/mmdetection3d/v0.1.0_models/nuimages_semseg/mask_rcnn_r50_fpn_coco-2x_1x_nuim/mask_rcnn_r50_fpn_coco-2x_1x_nuim_20201008_195238.log.json) |
+| Mask R-CNN | [R-50-CAFFE](./mask_rcnn_r50_caffe_fpn_1x_nuim.py) | IN | 1x | 7.0 | 47.7 | 38.2 | [model](https://download.openmmlab.com/mmdetection3d/v0.1.0_models/nuimages_semseg/mask_rcnn_r50_caffe_fpn_1x_nuim/) \| [log](https://download.openmmlab.com/mmdetection3d/v0.1.0_models/nuimages_semseg/mask_rcnn_r50_caffe_fpn_1x_nuim/) |
+| Mask R-CNN | [R-50-CAFFE](./mask_rcnn_r50_caffe_fpn_coco-3x_1x_nuim.py) | IN+COCO-3x | 1x | 7.0 | 49.9 | 40.8 | [model](https://download.openmmlab.com/mmdetection3d/v0.1.0_models/nuimages_semseg/mask_rcnn_r50_caffe_fpn_coco-3x_1x_nuim/mask_rcnn_r50_caffe_fpn_coco-3x_1x_nuim_20201008_195305-661a992e.pth) \| [log](https://download.openmmlab.com/mmdetection3d/v0.1.0_models/nuimages_semseg/mask_rcnn_r50_caffe_fpn_coco-3x_1x_nuim/mask_rcnn_r50_caffe_fpn_coco-3x_1x_nuim_20201008_195305.log.json) |
+| Mask R-CNN | [R-50-CAFFE](./mask_rcnn_r50_caffe_fpn_coco-3x_20e_nuim.py) | IN+COCO-3x | 20e | 7.0 | 50.6 | 41.3 | [model](https://download.openmmlab.com/mmdetection3d/v0.1.0_models/nuimages_semseg/mask_rcnn_r50_caffe_fpn_coco-3x_20e_nuim/mask_rcnn_r50_caffe_fpn_coco-3x_20e_nuim_20201009_125002-5529442c.pth) \| [log](https://download.openmmlab.com/mmdetection3d/v0.1.0_models/nuimages_semseg/mask_rcnn_r50_caffe_fpn_coco-3x_20e_nuim/mask_rcnn_r50_caffe_fpn_coco-3x_20e_nuim_20201009_125002.log.json) |
+| Mask R-CNN | [R-101](./mask_rcnn_r101_fpn_1x_nuim.py) | IN | 1x | 10.9 | 48.9 | 39.1 | [model](https://download.openmmlab.com/mmdetection3d/v0.1.0_models/nuimages_semseg/mask_rcnn_r101_fpn_1x_nuim/mask_rcnn_r101_fpn_1x_nuim_20201024_134803-65c7623a.pth) \| [log](https://download.openmmlab.com/mmdetection3d/v0.1.0_models/nuimages_semseg/mask_rcnn_r101_fpn_1x_nuim/mask_rcnn_r101_fpn_1x_nuim_20201024_134803.log.json) |
+| Mask R-CNN | [X-101_32x4d](./mask_rcnn_x101_32x4d_fpn_1x_nuim.py) | IN | 1x | 13.3 | 50.4 | 40.5 | [model](https://download.openmmlab.com/mmdetection3d/v0.1.0_models/nuimages_semseg/mask_rcnn_x101_32x4d_fpn_1x_nuim/mask_rcnn_x101_32x4d_fpn_1x_nuim_20201024_135741-b699ab37.pth) \| [log](https://download.openmmlab.com/mmdetection3d/v0.1.0_models/nuimages_semseg/mask_rcnn_x101_32x4d_fpn_1x_nuim/mask_rcnn_x101_32x4d_fpn_1x_nuim_20201024_135741.log.json) |
+| Cascade Mask R-CNN | [R-50](./cascade_mask_rcnn_r50_fpn_1x_nuim.py) | IN | 1x | 8.9 | 50.8 | 40.4 | [model](https://download.openmmlab.com/mmdetection3d/v0.1.0_models/nuimages_semseg/cascade_mask_rcnn_r50_fpn_1x_nuim/cascade_mask_rcnn_r50_fpn_1x_nuim_20201008_195342-1147c036.pth) \| [log](https://download.openmmlab.com/mmdetection3d/v0.1.0_models/nuimages_semseg/cascade_mask_rcnn_r50_fpn_1x_nuim/cascade_mask_rcnn_r50_fpn_1x_nuim_20201008_195342.log.json) |
+| Cascade Mask R-CNN | [R-50](./cascade_mask_rcnn_r50_fpn_coco-20e_1x_nuim.py) | IN+COCO-20e | 1x | 8.9 | 52.8 | 42.2 | [model](https://download.openmmlab.com/mmdetection3d/v0.1.0_models/nuimages_semseg/cascade_mask_rcnn_r50_fpn_coco-20e_1x_nuim/cascade_mask_rcnn_r50_fpn_coco-20e_1x_nuim_20201009_124158-ad0540e3.pth) \| [log](https://download.openmmlab.com/mmdetection3d/v0.1.0_models/nuimages_semseg/cascade_mask_rcnn_r50_fpn_coco-20e_1x_nuim/cascade_mask_rcnn_r50_fpn_coco-20e_1x_nuim_20201009_124158.log.json) |
+| Cascade Mask R-CNN | [R-50](./cascade_mask_rcnn_r50_fpn_coco-20e_20e_nuim.py) | IN+COCO-20e | 20e | 8.9 | 52.8 | 42.2 | [model](https://download.openmmlab.com/mmdetection3d/v0.1.0_models/nuimages_semseg/cascade_mask_rcnn_r50_fpn_coco-20e_20e_nuim/cascade_mask_rcnn_r50_fpn_coco-20e_20e_nuim_20201009_124951-40963960.pth) \| [log](https://download.openmmlab.com/mmdetection3d/v0.1.0_models/nuimages_semseg/cascade_mask_rcnn_r50_fpn_coco-20e_20e_nuim/cascade_mask_rcnn_r50_fpn_coco-20e_20e_nuim_20201009_124951.log.json) |
+| Cascade Mask R-CNN | [R-101](./cascade_mask_rcnn_r101_fpn_1x_nuim.py) | IN | 1x | 12.5 | 51.5 | 40.7 | [model](https://download.openmmlab.com/mmdetection3d/v0.1.0_models/nuimages_semseg/cascade_mask_rcnn_r101_fpn_1x_nuim/cascade_mask_rcnn_r101_fpn_1x_nuim_20201024_134804-45215b1e.pth) \| [log](https://download.openmmlab.com/mmdetection3d/v0.1.0_models/nuimages_semseg/cascade_mask_rcnn_r101_fpn_1x_nuim/cascade_mask_rcnn_r101_fpn_1x_nuim_20201024_134804.log.json) |
+| Cascade Mask R-CNN | [X-101_32x4d](./cascade_mask_rcnn_x101_32x4d_fpn_1x_nuim.py) | IN | 1x | 14.9 | 52.8 | 41.6 | [model](https://download.openmmlab.com/mmdetection3d/v0.1.0_models/nuimages_semseg/cascade_mask_rcnn_x101_32x4d_fpn_1x_nuim/cascade_mask_rcnn_x101_32x4d_fpn_1x_nuim_20201024_135753-e0e49778.pth) \| [log](https://download.openmmlab.com/mmdetection3d/v0.1.0_models/nuimages_semseg/cascade_mask_rcnn_x101_32x4d_fpn_1x_nuim/cascade_mask_rcnn_x101_32x4d_fpn_1x_nuim_20201024_135753.log.json) |
+| HTC w/o semantic | [R-50](./htc_without_semantic_r50_fpn_1x_nuim.py) | IN | 1x | | [model](<>) \| [log](<>) | | |
+| HTC | [R-50](./htc_r50_fpn_1x_nuim.py) | IN | 1x | | [model](https://download.openmmlab.com/mmdetection3d/v0.1.0_models/nuimages_semseg/) \| [log](https://download.openmmlab.com/mmdetection3d/v0.1.0_models/nuimages_semseg/) | | |
+| HTC | [R-50](./htc_r50_fpn_coco-20e_1x_nuim.py) | IN+COCO-20e | 1x | 11.6 | 53.8 | 43.8 | [model](https://download.openmmlab.com/mmdetection3d/v0.1.0_models/nuimages_semseg/htc_r50_fpn_coco-20e_1x_nuim/htc_r50_fpn_coco-20e_1x_nuim_20201010_070203-0b53a65e.pth) \| [log](https://download.openmmlab.com/mmdetection3d/v0.1.0_models/nuimages_semseg/htc_r50_fpn_coco-20e_1x_nuim/htc_r50_fpn_coco-20e_1x_nuim_20201010_070203.log.json) |
+| HTC | [R-50](./htc_r50_fpn_coco-20e_20e_nuim.py) | IN+COCO-20e | 20e | 11.6 | 54.8 | 44.4 | [model](https://download.openmmlab.com/mmdetection3d/v0.1.0_models/nuimages_semseg/htc_r50_fpn_coco-20e_20e_nuim/htc_r50_fpn_coco-20e_20e_nuim_20201008_211415-d6c60a2c.pth) \| [log](https://download.openmmlab.com/mmdetection3d/v0.1.0_models/nuimages_semseg/htc_r50_fpn_coco-20e_20e_nuim/htc_r50_fpn_coco-20e_20e_nuim_20201008_211415.log.json) |
+| HTC | [X-101_64x4d + DCN_c3-c5](./htc_x101_64x4d_fpn_dconv_c3-c5_coco-20e_16x1_20e_nuim.py) | IN+COCO-20e | 20e | 13.3 | 57.3 | 46.4 | [model](https://download.openmmlab.com/mmdetection3d/v0.1.0_models/nuimages_semseg/htc_x101_64x4d_fpn_dconv_c3-c5_coco-20e_16x1_20e_nuim/htc_x101_64x4d_fpn_dconv_c3-c5_coco-20e_16x1_20e_nuim_20201008_211222-0b16ac4b.pth) \| [log](https://download.openmmlab.com/mmdetection3d/v0.1.0_models/nuimages_semseg/htc_x101_64x4d_fpn_dconv_c3-c5_coco-20e_16x1_20e_nuim/htc_x101_64x4d_fpn_dconv_c3-c5_coco-20e_16x1_20e_nuim_20201008_211222.log.json) |
+
+**Note**:
+
+1. `IN` means only using ImageNet pre-trained backbone. `IN+COCO-Nx` and `IN+COCO-Ne` means the backbone is first pre-trained on ImageNet, and then the detector is pre-trained on COCO train2017 dataset by `Nx` and `N` epochs schedules, respectively.
+2. All the training hyper-parameters follow the standard schedules on COCO dataset except that the images are resized from
+ 1280 x 720 to 1920 x 1080 (relative ratio 0.8 to 1.2) since the images are in size 1600 x 900.
+3. The class order in the detectors released in v0.6.0 is different from the order in the configs because the bug in the conversion script. This bug has been fixed since v0.7.0 and the models trained by the correct class order are also released. If you used nuImages since v0.6.0, please re-convert the data through the conversion script using the above-mentioned command.
diff --git a/configs/nuimages/cascade_mask_rcnn_r101_fpn_1x_nuim.py b/configs/nuimages/cascade_mask_rcnn_r101_fpn_1x_nuim.py
new file mode 100644
index 0000000..28a54f7
--- /dev/null
+++ b/configs/nuimages/cascade_mask_rcnn_r101_fpn_1x_nuim.py
@@ -0,0 +1,2 @@
+_base_ = './cascade_mask_rcnn_r50_fpn_1x_nuim.py'
+model = dict(pretrained='torchvision://resnet101', backbone=dict(depth=101))
diff --git a/configs/nuimages/cascade_mask_rcnn_r50_fpn_1x_nuim.py b/configs/nuimages/cascade_mask_rcnn_r50_fpn_1x_nuim.py
new file mode 100644
index 0000000..c6ce25e
--- /dev/null
+++ b/configs/nuimages/cascade_mask_rcnn_r50_fpn_1x_nuim.py
@@ -0,0 +1,60 @@
+_base_ = [
+ '../_base_/models/cascade_mask_rcnn_r50_fpn.py',
+ '../_base_/datasets/nuim_instance.py',
+ '../_base_/schedules/mmdet_schedule_1x.py', '../_base_/default_runtime.py'
+]
+model = dict(
+ roi_head=dict(
+ bbox_head=[
+ dict(
+ type='Shared2FCBBoxHead',
+ in_channels=256,
+ fc_out_channels=1024,
+ roi_feat_size=7,
+ num_classes=10,
+ bbox_coder=dict(
+ type='DeltaXYWHBBoxCoder',
+ target_means=[0., 0., 0., 0.],
+ target_stds=[0.1, 0.1, 0.2, 0.2]),
+ reg_class_agnostic=True,
+ loss_cls=dict(
+ type='CrossEntropyLoss',
+ use_sigmoid=False,
+ loss_weight=1.0),
+ loss_bbox=dict(type='SmoothL1Loss', beta=1.0,
+ loss_weight=1.0)),
+ dict(
+ type='Shared2FCBBoxHead',
+ in_channels=256,
+ fc_out_channels=1024,
+ roi_feat_size=7,
+ num_classes=10,
+ bbox_coder=dict(
+ type='DeltaXYWHBBoxCoder',
+ target_means=[0., 0., 0., 0.],
+ target_stds=[0.05, 0.05, 0.1, 0.1]),
+ reg_class_agnostic=True,
+ loss_cls=dict(
+ type='CrossEntropyLoss',
+ use_sigmoid=False,
+ loss_weight=1.0),
+ loss_bbox=dict(type='SmoothL1Loss', beta=1.0,
+ loss_weight=1.0)),
+ dict(
+ type='Shared2FCBBoxHead',
+ in_channels=256,
+ fc_out_channels=1024,
+ roi_feat_size=7,
+ num_classes=10,
+ bbox_coder=dict(
+ type='DeltaXYWHBBoxCoder',
+ target_means=[0., 0., 0., 0.],
+ target_stds=[0.033, 0.033, 0.067, 0.067]),
+ reg_class_agnostic=True,
+ loss_cls=dict(
+ type='CrossEntropyLoss',
+ use_sigmoid=False,
+ loss_weight=1.0),
+ loss_bbox=dict(type='SmoothL1Loss', beta=1.0, loss_weight=1.0))
+ ],
+ mask_head=dict(num_classes=10)))
diff --git a/configs/nuimages/cascade_mask_rcnn_r50_fpn_coco-20e_1x_nuim.py b/configs/nuimages/cascade_mask_rcnn_r50_fpn_coco-20e_1x_nuim.py
new file mode 100644
index 0000000..bf3ffed
--- /dev/null
+++ b/configs/nuimages/cascade_mask_rcnn_r50_fpn_coco-20e_1x_nuim.py
@@ -0,0 +1,3 @@
+_base_ = './cascade_mask_rcnn_r50_fpn_1x_nuim.py'
+
+load_from = 'http://download.openmmlab.com/mmdetection/v2.0/cascade_rcnn/cascade_mask_rcnn_r50_fpn_20e_coco/cascade_mask_rcnn_r50_fpn_20e_coco_bbox_mAP-0.419__segm_mAP-0.365_20200504_174711-4af8e66e.pth' # noqa
diff --git a/configs/nuimages/cascade_mask_rcnn_r50_fpn_coco-20e_20e_nuim.py b/configs/nuimages/cascade_mask_rcnn_r50_fpn_coco-20e_20e_nuim.py
new file mode 100644
index 0000000..5d69466
--- /dev/null
+++ b/configs/nuimages/cascade_mask_rcnn_r50_fpn_coco-20e_20e_nuim.py
@@ -0,0 +1,7 @@
+_base_ = './cascade_mask_rcnn_r50_fpn_1x_nuim.py'
+
+# learning policy
+lr_config = dict(step=[16, 19])
+runner = dict(max_epochs=20)
+
+load_from = 'http://download.openmmlab.com/mmdetection/v2.0/cascade_rcnn/cascade_mask_rcnn_r50_fpn_20e_coco/cascade_mask_rcnn_r50_fpn_20e_coco_bbox_mAP-0.419__segm_mAP-0.365_20200504_174711-4af8e66e.pth' # noqa
diff --git a/configs/nuimages/cascade_mask_rcnn_x101_32x4d_fpn_1x_nuim.py b/configs/nuimages/cascade_mask_rcnn_x101_32x4d_fpn_1x_nuim.py
new file mode 100644
index 0000000..19f35ae
--- /dev/null
+++ b/configs/nuimages/cascade_mask_rcnn_x101_32x4d_fpn_1x_nuim.py
@@ -0,0 +1,13 @@
+_base_ = './cascade_mask_rcnn_r50_fpn_1x_nuim.py'
+model = dict(
+ pretrained='open-mmlab://resnext101_32x4d',
+ backbone=dict(
+ type='ResNeXt',
+ depth=101,
+ groups=32,
+ base_width=4,
+ num_stages=4,
+ out_indices=(0, 1, 2, 3),
+ frozen_stages=1,
+ norm_cfg=dict(type='BN', requires_grad=True),
+ style='pytorch'))
diff --git a/configs/nuimages/htc_r50_fpn_1x_nuim.py b/configs/nuimages/htc_r50_fpn_1x_nuim.py
new file mode 100644
index 0000000..4680683
--- /dev/null
+++ b/configs/nuimages/htc_r50_fpn_1x_nuim.py
@@ -0,0 +1,44 @@
+_base_ = './htc_without_semantic_r50_fpn_1x_nuim.py'
+model = dict(
+ roi_head=dict(
+ semantic_roi_extractor=dict(
+ type='SingleRoIExtractor',
+ roi_layer=dict(type='RoIAlign', output_size=14, sampling_ratio=0),
+ out_channels=256,
+ featmap_strides=[8]),
+ semantic_head=dict(
+ type='FusedSemanticHead',
+ num_ins=5,
+ fusion_level=1,
+ num_convs=4,
+ in_channels=256,
+ conv_out_channels=256,
+ num_classes=32,
+ ignore_label=0,
+ loss_weight=0.2)))
+
+data_root = 'data/nuimages/'
+img_norm_cfg = dict(
+ mean=[123.675, 116.28, 103.53], std=[58.395, 57.12, 57.375], to_rgb=True)
+train_pipeline = [
+ dict(type='LoadImageFromFile'),
+ dict(
+ type='LoadAnnotations', with_bbox=True, with_mask=True, with_seg=True),
+ dict(
+ type='Resize',
+ img_scale=[(1280, 720), (1920, 1080)],
+ multiscale_mode='range',
+ keep_ratio=True),
+ dict(type='RandomFlip', flip_ratio=0.5),
+ dict(type='Normalize', **img_norm_cfg),
+ dict(type='Pad', size_divisor=32),
+ dict(type='SegRescale', scale_factor=1 / 8),
+ dict(type='DefaultFormatBundle'),
+ dict(
+ type='Collect',
+ keys=['img', 'gt_bboxes', 'gt_labels', 'gt_masks', 'gt_semantic_seg'])
+]
+data = dict(
+ train=dict(
+ seg_prefix=data_root + 'annotations/semantic_masks/',
+ pipeline=train_pipeline))
diff --git a/configs/nuimages/htc_r50_fpn_coco-20e_1x_nuim.py b/configs/nuimages/htc_r50_fpn_coco-20e_1x_nuim.py
new file mode 100644
index 0000000..e5f6052
--- /dev/null
+++ b/configs/nuimages/htc_r50_fpn_coco-20e_1x_nuim.py
@@ -0,0 +1,3 @@
+_base_ = './htc_r50_fpn_1x_nuim.py'
+
+load_from = 'http://download.openmmlab.com/mmdetection/v2.0/htc/htc_r50_fpn_20e_coco/htc_r50_fpn_20e_coco_20200319-fe28c577.pth' # noqa
diff --git a/configs/nuimages/htc_r50_fpn_coco-20e_20e_nuim.py b/configs/nuimages/htc_r50_fpn_coco-20e_20e_nuim.py
new file mode 100644
index 0000000..2274900
--- /dev/null
+++ b/configs/nuimages/htc_r50_fpn_coco-20e_20e_nuim.py
@@ -0,0 +1,4 @@
+_base_ = './htc_r50_fpn_coco-20e_1x_nuim.py'
+# learning policy
+lr_config = dict(step=[16, 19])
+runner = dict(max_epochs=20)
diff --git a/configs/nuimages/htc_without_semantic_r50_fpn_1x_nuim.py b/configs/nuimages/htc_without_semantic_r50_fpn_1x_nuim.py
new file mode 100644
index 0000000..09fde67
--- /dev/null
+++ b/configs/nuimages/htc_without_semantic_r50_fpn_1x_nuim.py
@@ -0,0 +1,221 @@
+_base_ = [
+ '../_base_/datasets/nuim_instance.py',
+ '../_base_/schedules/mmdet_schedule_1x.py', '../_base_/default_runtime.py'
+]
+# model settings
+model = dict(
+ type='HybridTaskCascade',
+ pretrained='torchvision://resnet50',
+ backbone=dict(
+ type='ResNet',
+ depth=50,
+ num_stages=4,
+ out_indices=(0, 1, 2, 3),
+ frozen_stages=1,
+ norm_cfg=dict(type='BN', requires_grad=True),
+ norm_eval=True,
+ style='pytorch'),
+ neck=dict(
+ type='FPN',
+ in_channels=[256, 512, 1024, 2048],
+ out_channels=256,
+ num_outs=5),
+ rpn_head=dict(
+ type='RPNHead',
+ in_channels=256,
+ feat_channels=256,
+ anchor_generator=dict(
+ type='AnchorGenerator',
+ scales=[8],
+ ratios=[0.5, 1.0, 2.0],
+ strides=[4, 8, 16, 32, 64]),
+ bbox_coder=dict(
+ type='DeltaXYWHBBoxCoder',
+ target_means=[.0, .0, .0, .0],
+ target_stds=[1.0, 1.0, 1.0, 1.0]),
+ loss_cls=dict(
+ type='CrossEntropyLoss', use_sigmoid=True, loss_weight=1.0),
+ loss_bbox=dict(type='SmoothL1Loss', beta=1.0 / 9.0, loss_weight=1.0)),
+ roi_head=dict(
+ type='HybridTaskCascadeRoIHead',
+ interleaved=True,
+ mask_info_flow=True,
+ num_stages=3,
+ stage_loss_weights=[1, 0.5, 0.25],
+ bbox_roi_extractor=dict(
+ type='SingleRoIExtractor',
+ roi_layer=dict(type='RoIAlign', output_size=7, sampling_ratio=0),
+ out_channels=256,
+ featmap_strides=[4, 8, 16, 32]),
+ bbox_head=[
+ dict(
+ type='Shared2FCBBoxHead',
+ in_channels=256,
+ fc_out_channels=1024,
+ roi_feat_size=7,
+ num_classes=10,
+ bbox_coder=dict(
+ type='DeltaXYWHBBoxCoder',
+ target_means=[0., 0., 0., 0.],
+ target_stds=[0.1, 0.1, 0.2, 0.2]),
+ reg_class_agnostic=True,
+ loss_cls=dict(
+ type='CrossEntropyLoss',
+ use_sigmoid=False,
+ loss_weight=1.0),
+ loss_bbox=dict(type='SmoothL1Loss', beta=1.0,
+ loss_weight=1.0)),
+ dict(
+ type='Shared2FCBBoxHead',
+ in_channels=256,
+ fc_out_channels=1024,
+ roi_feat_size=7,
+ num_classes=10,
+ bbox_coder=dict(
+ type='DeltaXYWHBBoxCoder',
+ target_means=[0., 0., 0., 0.],
+ target_stds=[0.05, 0.05, 0.1, 0.1]),
+ reg_class_agnostic=True,
+ loss_cls=dict(
+ type='CrossEntropyLoss',
+ use_sigmoid=False,
+ loss_weight=1.0),
+ loss_bbox=dict(type='SmoothL1Loss', beta=1.0,
+ loss_weight=1.0)),
+ dict(
+ type='Shared2FCBBoxHead',
+ in_channels=256,
+ fc_out_channels=1024,
+ roi_feat_size=7,
+ num_classes=10,
+ bbox_coder=dict(
+ type='DeltaXYWHBBoxCoder',
+ target_means=[0., 0., 0., 0.],
+ target_stds=[0.033, 0.033, 0.067, 0.067]),
+ reg_class_agnostic=True,
+ loss_cls=dict(
+ type='CrossEntropyLoss',
+ use_sigmoid=False,
+ loss_weight=1.0),
+ loss_bbox=dict(type='SmoothL1Loss', beta=1.0, loss_weight=1.0))
+ ],
+ mask_roi_extractor=dict(
+ type='SingleRoIExtractor',
+ roi_layer=dict(type='RoIAlign', output_size=14, sampling_ratio=0),
+ out_channels=256,
+ featmap_strides=[4, 8, 16, 32]),
+ mask_head=[
+ dict(
+ type='HTCMaskHead',
+ with_conv_res=False,
+ num_convs=4,
+ in_channels=256,
+ conv_out_channels=256,
+ num_classes=10,
+ loss_mask=dict(
+ type='CrossEntropyLoss', use_mask=True, loss_weight=1.0)),
+ dict(
+ type='HTCMaskHead',
+ num_convs=4,
+ in_channels=256,
+ conv_out_channels=256,
+ num_classes=10,
+ loss_mask=dict(
+ type='CrossEntropyLoss', use_mask=True, loss_weight=1.0)),
+ dict(
+ type='HTCMaskHead',
+ num_convs=4,
+ in_channels=256,
+ conv_out_channels=256,
+ num_classes=10,
+ loss_mask=dict(
+ type='CrossEntropyLoss', use_mask=True, loss_weight=1.0))
+ ]),
+ # model training and testing settings
+ train_cfg=dict(
+ rpn=dict(
+ assigner=dict(
+ type='MaxIoUAssigner',
+ pos_iou_thr=0.7,
+ neg_iou_thr=0.3,
+ min_pos_iou=0.3,
+ ignore_iof_thr=-1),
+ sampler=dict(
+ type='RandomSampler',
+ num=256,
+ pos_fraction=0.5,
+ neg_pos_ub=-1,
+ add_gt_as_proposals=False),
+ allowed_border=0,
+ pos_weight=-1,
+ debug=False),
+ rpn_proposal=dict(
+ nms_across_levels=False,
+ nms_pre=2000,
+ nms_post=2000,
+ max_per_img=2000,
+ nms=dict(type='nms', iou_threshold=0.7),
+ min_bbox_size=0),
+ rcnn=[
+ dict(
+ assigner=dict(
+ type='MaxIoUAssigner',
+ pos_iou_thr=0.5,
+ neg_iou_thr=0.5,
+ min_pos_iou=0.5,
+ ignore_iof_thr=-1),
+ sampler=dict(
+ type='RandomSampler',
+ num=512,
+ pos_fraction=0.25,
+ neg_pos_ub=-1,
+ add_gt_as_proposals=True),
+ mask_size=28,
+ pos_weight=-1,
+ debug=False),
+ dict(
+ assigner=dict(
+ type='MaxIoUAssigner',
+ pos_iou_thr=0.6,
+ neg_iou_thr=0.6,
+ min_pos_iou=0.6,
+ ignore_iof_thr=-1),
+ sampler=dict(
+ type='RandomSampler',
+ num=512,
+ pos_fraction=0.25,
+ neg_pos_ub=-1,
+ add_gt_as_proposals=True),
+ mask_size=28,
+ pos_weight=-1,
+ debug=False),
+ dict(
+ assigner=dict(
+ type='MaxIoUAssigner',
+ pos_iou_thr=0.7,
+ neg_iou_thr=0.7,
+ min_pos_iou=0.7,
+ ignore_iof_thr=-1),
+ sampler=dict(
+ type='RandomSampler',
+ num=512,
+ pos_fraction=0.25,
+ neg_pos_ub=-1,
+ add_gt_as_proposals=True),
+ mask_size=28,
+ pos_weight=-1,
+ debug=False)
+ ]),
+ test_cfg=dict(
+ rpn=dict(
+ nms_across_levels=False,
+ nms_pre=1000,
+ nms_post=1000,
+ max_per_img=1000,
+ nms=dict(type='nms', iou_threshold=0.7),
+ min_bbox_size=0),
+ rcnn=dict(
+ score_thr=0.001,
+ nms=dict(type='nms', iou_threshold=0.5),
+ max_per_img=100,
+ mask_thr_binary=0.5)))
diff --git a/configs/nuimages/htc_x101_64x4d_fpn_dconv_c3-c5_coco-20e_16x1_20e_nuim.py b/configs/nuimages/htc_x101_64x4d_fpn_dconv_c3-c5_coco-20e_16x1_20e_nuim.py
new file mode 100644
index 0000000..4ab095a
--- /dev/null
+++ b/configs/nuimages/htc_x101_64x4d_fpn_dconv_c3-c5_coco-20e_16x1_20e_nuim.py
@@ -0,0 +1,23 @@
+_base_ = './htc_r50_fpn_1x_nuim.py'
+model = dict(
+ pretrained='open-mmlab://resnext101_64x4d',
+ backbone=dict(
+ type='ResNeXt',
+ depth=101,
+ groups=64,
+ base_width=4,
+ num_stages=4,
+ out_indices=(0, 1, 2, 3),
+ frozen_stages=1,
+ norm_cfg=dict(type='BN', requires_grad=True),
+ norm_eval=True,
+ style='pytorch',
+ dcn=dict(type='DCN', deform_groups=1, fallback_on_stride=False),
+ stage_with_dcn=(False, True, True, True)))
+
+data = dict(samples_per_gpu=1, workers_per_gpu=1)
+# learning policy
+lr_config = dict(step=[16, 19])
+runner = dict(max_epochs=20)
+
+load_from = 'http://download.openmmlab.com/mmdetection/v2.0/htc/htc_x101_64x4d_fpn_dconv_c3-c5_mstrain_400_1400_16x1_20e_coco/htc_x101_64x4d_fpn_dconv_c3-c5_mstrain_400_1400_16x1_20e_coco_20200312-946fd751.pth' # noqa
diff --git a/configs/nuimages/mask_rcnn_r101_fpn_1x_nuim.py b/configs/nuimages/mask_rcnn_r101_fpn_1x_nuim.py
new file mode 100644
index 0000000..6245194
--- /dev/null
+++ b/configs/nuimages/mask_rcnn_r101_fpn_1x_nuim.py
@@ -0,0 +1,2 @@
+_base_ = './mask_rcnn_r50_fpn_1x_nuim.py'
+model = dict(pretrained='torchvision://resnet101', backbone=dict(depth=101))
diff --git a/configs/nuimages/mask_rcnn_r50_caffe_fpn_1x_nuim.py b/configs/nuimages/mask_rcnn_r50_caffe_fpn_1x_nuim.py
new file mode 100644
index 0000000..4af79e5
--- /dev/null
+++ b/configs/nuimages/mask_rcnn_r50_caffe_fpn_1x_nuim.py
@@ -0,0 +1,46 @@
+_base_ = [
+ '../_base_/models/mask_rcnn_r50_fpn.py',
+ '../_base_/datasets/nuim_instance.py',
+ '../_base_/schedules/mmdet_schedule_1x.py', '../_base_/default_runtime.py'
+]
+model = dict(
+ pretrained='open-mmlab://detectron2/resnet50_caffe',
+ backbone=dict(norm_cfg=dict(requires_grad=False), style='caffe'),
+ roi_head=dict(
+ bbox_head=dict(num_classes=10), mask_head=dict(num_classes=10)))
+# use caffe img_norm
+img_norm_cfg = dict(
+ mean=[103.530, 116.280, 123.675], std=[1.0, 1.0, 1.0], to_rgb=False)
+train_pipeline = [
+ dict(type='LoadImageFromFile'),
+ dict(type='LoadAnnotations', with_bbox=True, with_mask=True),
+ dict(
+ type='Resize',
+ img_scale=[(1280, 720), (1920, 1080)],
+ multiscale_mode='range',
+ keep_ratio=True),
+ dict(type='RandomFlip', flip_ratio=0.5),
+ dict(type='Normalize', **img_norm_cfg),
+ dict(type='Pad', size_divisor=32),
+ dict(type='DefaultFormatBundle'),
+ dict(type='Collect', keys=['img', 'gt_bboxes', 'gt_labels', 'gt_masks']),
+]
+test_pipeline = [
+ dict(type='LoadImageFromFile'),
+ dict(
+ type='MultiScaleFlipAug',
+ img_scale=(1600, 900),
+ flip=False,
+ transforms=[
+ dict(type='Resize', keep_ratio=True),
+ dict(type='RandomFlip'),
+ dict(type='Normalize', **img_norm_cfg),
+ dict(type='Pad', size_divisor=32),
+ dict(type='ImageToTensor', keys=['img']),
+ dict(type='Collect', keys=['img']),
+ ])
+]
+data = dict(
+ train=dict(pipeline=train_pipeline),
+ val=dict(pipeline=test_pipeline),
+ test=dict(pipeline=test_pipeline))
diff --git a/configs/nuimages/mask_rcnn_r50_caffe_fpn_coco-3x_1x_nuim.py b/configs/nuimages/mask_rcnn_r50_caffe_fpn_coco-3x_1x_nuim.py
new file mode 100644
index 0000000..32c3f44
--- /dev/null
+++ b/configs/nuimages/mask_rcnn_r50_caffe_fpn_coco-3x_1x_nuim.py
@@ -0,0 +1,48 @@
+_base_ = [
+ '../_base_/models/mask_rcnn_r50_fpn.py',
+ '../_base_/datasets/nuim_instance.py',
+ '../_base_/schedules/mmdet_schedule_1x.py', '../_base_/default_runtime.py'
+]
+model = dict(
+ pretrained='open-mmlab://detectron2/resnet50_caffe',
+ backbone=dict(norm_cfg=dict(requires_grad=False), style='caffe'),
+ roi_head=dict(
+ bbox_head=dict(num_classes=10), mask_head=dict(num_classes=10)))
+# use caffe img_norm
+img_norm_cfg = dict(
+ mean=[103.530, 116.280, 123.675], std=[1.0, 1.0, 1.0], to_rgb=False)
+train_pipeline = [
+ dict(type='LoadImageFromFile'),
+ dict(type='LoadAnnotations', with_bbox=True, with_mask=True),
+ dict(
+ type='Resize',
+ img_scale=[(1280, 720), (1920, 1080)],
+ multiscale_mode='range',
+ keep_ratio=True),
+ dict(type='RandomFlip', flip_ratio=0.5),
+ dict(type='Normalize', **img_norm_cfg),
+ dict(type='Pad', size_divisor=32),
+ dict(type='DefaultFormatBundle'),
+ dict(type='Collect', keys=['img', 'gt_bboxes', 'gt_labels', 'gt_masks']),
+]
+test_pipeline = [
+ dict(type='LoadImageFromFile'),
+ dict(
+ type='MultiScaleFlipAug',
+ img_scale=(1600, 900),
+ flip=False,
+ transforms=[
+ dict(type='Resize', keep_ratio=True),
+ dict(type='RandomFlip'),
+ dict(type='Normalize', **img_norm_cfg),
+ dict(type='Pad', size_divisor=32),
+ dict(type='ImageToTensor', keys=['img']),
+ dict(type='Collect', keys=['img']),
+ ])
+]
+data = dict(
+ train=dict(pipeline=train_pipeline),
+ val=dict(pipeline=test_pipeline),
+ test=dict(pipeline=test_pipeline))
+
+load_from = 'https://download.openmmlab.com/mmdetection/v2.0/mask_rcnn/mask_rcnn_r50_caffe_fpn_mstrain-poly_3x_coco/mask_rcnn_r50_caffe_fpn_mstrain-poly_3x_coco_bbox_mAP-0.408__segm_mAP-0.37_20200504_163245-42aa3d00.pth' # noqa
diff --git a/configs/nuimages/mask_rcnn_r50_caffe_fpn_coco-3x_20e_nuim.py b/configs/nuimages/mask_rcnn_r50_caffe_fpn_coco-3x_20e_nuim.py
new file mode 100644
index 0000000..6097353
--- /dev/null
+++ b/configs/nuimages/mask_rcnn_r50_caffe_fpn_coco-3x_20e_nuim.py
@@ -0,0 +1,52 @@
+_base_ = [
+ '../_base_/models/mask_rcnn_r50_fpn.py',
+ '../_base_/datasets/nuim_instance.py',
+ '../_base_/schedules/mmdet_schedule_1x.py', '../_base_/default_runtime.py'
+]
+model = dict(
+ pretrained='open-mmlab://detectron2/resnet50_caffe',
+ backbone=dict(norm_cfg=dict(requires_grad=False), style='caffe'),
+ roi_head=dict(
+ bbox_head=dict(num_classes=10), mask_head=dict(num_classes=10)))
+# use caffe img_norm
+img_norm_cfg = dict(
+ mean=[103.530, 116.280, 123.675], std=[1.0, 1.0, 1.0], to_rgb=False)
+train_pipeline = [
+ dict(type='LoadImageFromFile'),
+ dict(type='LoadAnnotations', with_bbox=True, with_mask=True),
+ dict(
+ type='Resize',
+ img_scale=[(1280, 720), (1920, 1080)],
+ multiscale_mode='range',
+ keep_ratio=True),
+ dict(type='RandomFlip', flip_ratio=0.5),
+ dict(type='Normalize', **img_norm_cfg),
+ dict(type='Pad', size_divisor=32),
+ dict(type='DefaultFormatBundle'),
+ dict(type='Collect', keys=['img', 'gt_bboxes', 'gt_labels', 'gt_masks']),
+]
+test_pipeline = [
+ dict(type='LoadImageFromFile'),
+ dict(
+ type='MultiScaleFlipAug',
+ img_scale=(1600, 900),
+ flip=False,
+ transforms=[
+ dict(type='Resize', keep_ratio=True),
+ dict(type='RandomFlip'),
+ dict(type='Normalize', **img_norm_cfg),
+ dict(type='Pad', size_divisor=32),
+ dict(type='ImageToTensor', keys=['img']),
+ dict(type='Collect', keys=['img']),
+ ])
+]
+data = dict(
+ train=dict(pipeline=train_pipeline),
+ val=dict(pipeline=test_pipeline),
+ test=dict(pipeline=test_pipeline))
+
+# learning policy
+lr_config = dict(step=[16, 19])
+runner = dict(max_epochs=20)
+
+load_from = 'http://download.openmmlab.com/mmdetection/v2.0/mask_rcnn/mask_rcnn_r50_caffe_fpn_mstrain-poly_3x_coco/mask_rcnn_r50_caffe_fpn_mstrain-poly_3x_coco_bbox_mAP-0.408__segm_mAP-0.37_20200504_163245-42aa3d00.pth' # noqa
diff --git a/configs/nuimages/mask_rcnn_r50_fpn_1x_nuim.py b/configs/nuimages/mask_rcnn_r50_fpn_1x_nuim.py
new file mode 100644
index 0000000..ec999ec
--- /dev/null
+++ b/configs/nuimages/mask_rcnn_r50_fpn_1x_nuim.py
@@ -0,0 +1,8 @@
+_base_ = [
+ '../_base_/models/mask_rcnn_r50_fpn.py',
+ '../_base_/datasets/nuim_instance.py',
+ '../_base_/schedules/mmdet_schedule_1x.py', '../_base_/default_runtime.py'
+]
+model = dict(
+ roi_head=dict(
+ bbox_head=dict(num_classes=10), mask_head=dict(num_classes=10)))
diff --git a/configs/nuimages/mask_rcnn_r50_fpn_coco-2x_1x_nuim.py b/configs/nuimages/mask_rcnn_r50_fpn_coco-2x_1x_nuim.py
new file mode 100644
index 0000000..fd60353
--- /dev/null
+++ b/configs/nuimages/mask_rcnn_r50_fpn_coco-2x_1x_nuim.py
@@ -0,0 +1,9 @@
+_base_ = [
+ '../_base_/models/mask_rcnn_r50_fpn.py',
+ '../_base_/datasets/nuim_instance.py',
+ '../_base_/schedules/mmdet_schedule_1x.py', '../_base_/default_runtime.py'
+]
+model = dict(
+ roi_head=dict(
+ bbox_head=dict(num_classes=10), mask_head=dict(num_classes=10)))
+load_from = 'https://download.openmmlab.com/mmdetection/v2.0/mask_rcnn/mask_rcnn_r50_fpn_2x_coco/mask_rcnn_r50_fpn_2x_coco_bbox_mAP-0.392__segm_mAP-0.354_20200505_003907-3e542a40.pth' # noqa
diff --git a/configs/nuimages/mask_rcnn_r50_fpn_coco-2x_1x_nus-2d.py b/configs/nuimages/mask_rcnn_r50_fpn_coco-2x_1x_nus-2d.py
new file mode 100644
index 0000000..06d2745
--- /dev/null
+++ b/configs/nuimages/mask_rcnn_r50_fpn_coco-2x_1x_nus-2d.py
@@ -0,0 +1,39 @@
+_base_ = [
+ '../_base_/models/mask_rcnn_r50_fpn.py',
+ '../_base_/datasets/nuim_instance.py',
+ '../_base_/schedules/mmdet_schedule_1x.py', '../_base_/default_runtime.py'
+]
+model = dict(
+ roi_head=dict(
+ bbox_head=dict(num_classes=10), mask_head=dict(num_classes=10)))
+
+file_client_args = dict(
+ backend='petrel',
+ path_mapping=dict({
+ './data/nuscenes/': 's3://nuscenes/nuscenes/',
+ 'data/nuscenes/': 's3://nuscenes/nuscenes/'
+ }))
+img_norm_cfg = dict(
+ mean=[123.675, 116.28, 103.53], std=[58.395, 57.12, 57.375], to_rgb=True)
+
+test_pipeline = [
+ dict(type='LoadImageFromFile', file_client_args=file_client_args),
+ dict(
+ type='MultiScaleFlipAug',
+ img_scale=(1600, 900),
+ flip=False,
+ transforms=[
+ dict(type='Resize', keep_ratio=True),
+ dict(type='RandomFlip'),
+ dict(type='Normalize', **img_norm_cfg),
+ dict(type='Pad', size_divisor=32),
+ dict(type='ImageToTensor', keys=['img']),
+ dict(type='Collect', keys=['img']),
+ ])
+]
+data_root = 'data/nuimages/'
+# data = dict(
+# val=dict(
+# ann_file=data_root + 'annotations/nuimages_v1.0-mini.json'),
+# test=dict(
+# ann_file=data_root + 'annotations/nuimages_v1.0-mini.json'))
diff --git a/configs/nuimages/mask_rcnn_x101_32x4d_fpn_1x_nuim.py b/configs/nuimages/mask_rcnn_x101_32x4d_fpn_1x_nuim.py
new file mode 100644
index 0000000..eb3e81b
--- /dev/null
+++ b/configs/nuimages/mask_rcnn_x101_32x4d_fpn_1x_nuim.py
@@ -0,0 +1,13 @@
+_base_ = './mask_rcnn_r50_fpn_1x_nuim.py'
+model = dict(
+ pretrained='open-mmlab://resnext101_32x4d',
+ backbone=dict(
+ type='ResNeXt',
+ depth=101,
+ groups=32,
+ base_width=4,
+ num_stages=4,
+ out_indices=(0, 1, 2, 3),
+ frozen_stages=1,
+ norm_cfg=dict(type='BN', requires_grad=True),
+ style='pytorch'))
diff --git a/configs/nuimages/metafile.yml b/configs/nuimages/metafile.yml
new file mode 100644
index 0000000..7b94ce7
--- /dev/null
+++ b/configs/nuimages/metafile.yml
@@ -0,0 +1,255 @@
+Models:
+ - Name: mask_rcnn_r50_fpn_1x_nuim
+ In Collection: Mask R-CNN
+ Config: configs/nuimages/mask_rcnn_r50_fpn_1x_nuim.py
+ Metadata:
+ Training Memory (GB): 7.4
+ Training Resources: 8x TITAN Xp
+ Results:
+ - Task: Object Detection
+ Dataset: nuImages
+ Metrics:
+ Box AP: 47.8
+ - Task: Instance Segmentation
+ Dataset: nuImages
+ Metrics:
+ Mask AP: 38.4
+ Weights: https://download.openmmlab.com/mmdetection3d/v0.1.0_models/nuimages_semseg/mask_rcnn_r50_fpn_1x_nuim/mask_rcnn_r50_fpn_1x_nuim_20201008_195238-e99f5182.pth
+
+ - Name: mask_rcnn_r50_fpn_coco-2x_1x_nuim
+ In Collection: Mask R-CNN
+ Config: configs/nuimages/mask_rcnn_r50_fpn_coco-2x_1x_nuim.py
+ Metadata:
+ Training Memory (GB): 7.4
+ Training Resources: 8x TITAN Xp
+ Results:
+ - Task: Object Detection
+ Dataset: nuImages
+ Metrics:
+ Box AP: 49.7
+ - Task: Instance Segmentation
+ Dataset: nuImages
+ Metrics:
+ Mask AP: 40.5
+ Weights: https://download.openmmlab.com/mmdetection3d/v0.1.0_models/nuimages_semseg/mask_rcnn_r50_fpn_coco-2x_1x_nuim/mask_rcnn_r50_fpn_coco-2x_1x_nuim_20201008_195238-b1742a60.pth
+
+ - Name: mask_rcnn_r50_caffe_fpn_1x_nuim
+ In Collection: Mask R-CNN
+ Config: configs/nuimages/mask_rcnn_r50_caffe_fpn_1x_nuim.py
+ Metadata:
+ Training Memory (GB): 7.0
+ Training Resources: 8x TITAN Xp
+ Results:
+ - Task: Object Detection
+ Dataset: nuImages
+ Metrics:
+ Box AP: 47.7
+ - Task: Instance Segmentation
+ Dataset: nuImages
+ Metrics:
+ Mask AP: 38.2
+ Weights: https://download.openmmlab.com/mmdetection3d/v0.1.0_models/nuimages_semseg/mask_rcnn_r50_caffe_fpn_1x_nuim/
+
+ - Name: mask_rcnn_r50_caffe_fpn_coco-3x_1x_nuim
+ In Collection: Mask R-CNN
+ Config: configs/nuimages/mask_rcnn_r50_caffe_fpn_coco-3x_1x_nuim.py
+ Metadata:
+ Training Memory (GB): 7.0
+ Training Resources: 8x TITAN Xp
+ Results:
+ - Task: Object Detection
+ Dataset: nuImages
+ Metrics:
+ Box AP: 49.9
+ - Task: Instance Segmentation
+ Dataset: nuImages
+ Metrics:
+ Mask AP: 40.8
+ Weights: https://download.openmmlab.com/mmdetection3d/v0.1.0_models/nuimages_semseg/mask_rcnn_r50_caffe_fpn_coco-3x_1x_nuim/mask_rcnn_r50_caffe_fpn_coco-3x_1x_nuim_20201008_195305-661a992e.pth
+
+ - Name: mask_rcnn_r50_caffe_fpn_coco-3x_20e_nuim
+ In Collection: Mask R-CNN
+ Config: configs/nuimages/mask_rcnn_r50_caffe_fpn_coco-3x_20e_nuim.py
+ Metadata:
+ Training Memory (GB): 7.0
+ Training Resources: 8x TITAN Xp
+ Results:
+ - Task: Object Detection
+ Dataset: nuImages
+ Metrics:
+ Box AP: 50.6
+ - Task: Instance Segmentation
+ Dataset: nuImages
+ Metrics:
+ Mask AP: 41.3
+ Weights: https://download.openmmlab.com/mmdetection3d/v0.1.0_models/nuimages_semseg/mask_rcnn_r50_caffe_fpn_coco-3x_20e_nuim/mask_rcnn_r50_caffe_fpn_coco-3x_20e_nuim_20201009_125002-5529442c.pth
+
+ - Name: mask_rcnn_r101_fpn_1x_nuim
+ In Collection: Mask R-CNN
+ Config: configs/nuimages/mask_rcnn_r101_fpn_1x_nuim.py
+ Metadata:
+ Training Memory (GB): 10.9
+ Training Resources: 8x TITAN Xp
+ Results:
+ - Task: Object Detection
+ Dataset: nuImages
+ Metrics:
+ Box AP: 48.9
+ - Task: Instance Segmentation
+ Dataset: nuImages
+ Metrics:
+ Mask AP: 39.1
+ Weights: https://download.openmmlab.com/mmdetection3d/v0.1.0_models/nuimages_semseg/mask_rcnn_r101_fpn_1x_nuim/mask_rcnn_r101_fpn_1x_nuim_20201024_134803-65c7623a.pth
+
+ - Name: mask_rcnn_x101_32x4d_fpn_1x_nuim
+ In Collection: Mask R-CNN
+ Config: configs/nuimages/mask_rcnn_x101_32x4d_fpn_1x_nuim.py
+ Metadata:
+ Training Memory (GB): 13.3
+ Training Resources: 8x TITAN Xp
+ Results:
+ - Task: Object Detection
+ Dataset: nuImages
+ Metrics:
+ Box AP: 50.4
+ - Task: Instance Segmentation
+ Dataset: nuImages
+ Metrics:
+ Mask AP: 40.5
+ Weights: https://download.openmmlab.com/mmdetection3d/v0.1.0_models/nuimages_semseg/mask_rcnn_x101_32x4d_fpn_1x_nuim/mask_rcnn_x101_32x4d_fpn_1x_nuim_20201024_135741-b699ab37.pth
+
+ - Name: cascade_mask_rcnn_r50_fpn_1x_nuim
+ In Collection: Mask R-CNN
+ Config: configs/nuimages/cascade_mask_rcnn_r50_fpn_1x_nuim.py
+ Metadata:
+ Training Memory (GB): 8.9
+ Training Resources: 8x TITAN Xp
+ Results:
+ - Task: Object Detection
+ Dataset: nuImages
+ Metrics:
+ Box AP: 50.8
+ - Task: Instance Segmentation
+ Dataset: nuImages
+ Metrics:
+ Mask AP: 40.4
+ Weights: https://download.openmmlab.com/mmdetection3d/v0.1.0_models/nuimages_semseg/cascade_mask_rcnn_r50_fpn_1x_nuim/cascade_mask_rcnn_r50_fpn_1x_nuim_20201008_195342-1147c036.pth
+
+ - Name: cascade_mask_rcnn_r50_fpn_coco-20e_1x_nuim
+ In Collection: Mask R-CNN
+ Config: configs/nuimages/cascade_mask_rcnn_r50_fpn_coco-20e_1x_nuim.py
+ Metadata:
+ Training Memory (GB): 8.9
+ Training Resources: 8x TITAN Xp
+ Results:
+ - Task: Object Detection
+ Dataset: nuImages
+ Metrics:
+ Box AP: 52.8
+ - Task: Instance Segmentation
+ Dataset: nuImages
+ Metrics:
+ Mask AP: 42.2
+ Weights: https://download.openmmlab.com/mmdetection3d/v0.1.0_models/nuimages_semseg/cascade_mask_rcnn_r50_fpn_coco-20e_1x_nuim/cascade_mask_rcnn_r50_fpn_coco-20e_1x_nuim_20201009_124158-ad0540e3.pth
+
+ - Name: cascade_mask_rcnn_r50_fpn_coco-20e_20e_nuim
+ In Collection: Mask R-CNN
+ Config: configs/nuimages/cascade_mask_rcnn_r50_fpn_coco-20e_20e_nuim.py
+ Metadata:
+ Training Memory (GB): 8.9
+ Training Resources: 8x TITAN Xp
+ Results:
+ - Task: Object Detection
+ Dataset: nuImages
+ Metrics:
+ Box AP: 52.8
+ - Task: Instance Segmentation
+ Dataset: nuImages
+ Metrics:
+ Mask AP: 42.2
+ Weights: https://download.openmmlab.com/mmdetection3d/v0.1.0_models/nuimages_semseg/cascade_mask_rcnn_r50_fpn_coco-20e_20e_nuim/cascade_mask_rcnn_r50_fpn_coco-20e_20e_nuim_20201009_124951-40963960.pth
+
+ - Name: cascade_mask_rcnn_r101_fpn_1x_nuim
+ In Collection: Mask R-CNN
+ Config: configs/nuimages/cascade_mask_rcnn_r101_fpn_1x_nuim.py
+ Metadata:
+ Training Memory (GB): 12.5
+ Training Resources: 8x TITAN Xp
+ Results:
+ - Task: Object Detection
+ Dataset: nuImages
+ Metrics:
+ Box AP: 51.5
+ - Task: Instance Segmentation
+ Dataset: nuImages
+ Metrics:
+ Mask AP: 40.7
+ Weights: https://download.openmmlab.com/mmdetection3d/v0.1.0_models/nuimages_semseg/cascade_mask_rcnn_r101_fpn_1x_nuim/cascade_mask_rcnn_r101_fpn_1x_nuim_20201024_134804-45215b1e.pth
+
+ - Name: cascade_mask_rcnn_x101_32x4d_fpn_1x_nuim
+ In Collection: Mask R-CNN
+ Config: configs/nuimages/cascade_mask_rcnn_x101_32x4d_fpn_1x_nuim.py
+ Metadata:
+ Training Memory (GB): 14.9
+ Training Resources: 8x TITAN Xp
+ Results:
+ - Task: Object Detection
+ Dataset: nuImages
+ Metrics:
+ Box AP: 52.8
+ - Task: Instance Segmentation
+ Dataset: nuImages
+ Metrics:
+ Mask AP: 41.6
+ Weights: https://download.openmmlab.com/mmdetection3d/v0.1.0_models/nuimages_semseg/cascade_mask_rcnn_x101_32x4d_fpn_1x_nuim/cascade_mask_rcnn_x101_32x4d_fpn_1x_nuim_20201024_135753-e0e49778.pth
+
+ - Name: htc_r50_fpn_coco-20e_1x_nuim
+ In Collection: Mask R-CNN
+ Config: configs/nuimages/htc_r50_fpn_coco-20e_1x_nuim.py
+ Metadata:
+ Training Memory (GB): 11.6
+ Training Resources: 8x V100 GPUs
+ Results:
+ - Task: Object Detection
+ Dataset: nuImages
+ Metrics:
+ Box AP: 53.8
+ - Task: Instance Segmentation
+ Dataset: nuImages
+ Metrics:
+ Mask AP: 43.8
+ Weights: https://download.openmmlab.com/mmdetection3d/v0.1.0_models/nuimages_semseg/htc_r50_fpn_coco-20e_1x_nuim/htc_r50_fpn_coco-20e_1x_nuim_20201010_070203-0b53a65e.pth
+
+ - Name: htc_r50_fpn_coco-20e_20e_nuim
+ In Collection: Mask R-CNN
+ Config: configs/nuimages/htc_r50_fpn_coco-20e_20e_nuim.py
+ Metadata:
+ Training Memory (GB): 11.6
+ Training Resources: 8x V100 GPUs
+ Results:
+ - Task: Object Detection
+ Dataset: nuImages
+ Metrics:
+ Box AP: 54.8
+ - Task: Instance Segmentation
+ Dataset: nuImages
+ Metrics:
+ Mask AP: 44.4
+ Weights: https://download.openmmlab.com/mmdetection3d/v0.1.0_models/nuimages_semseg/htc_r50_fpn_coco-20e_20e_nuim/htc_r50_fpn_coco-20e_20e_nuim_20201008_211415-d6c60a2c.pth
+
+ - Name: htc_x101_64x4d_fpn_dconv_c3-c5_coco-20e_16x1_20e_nuim
+ In Collection: Mask R-CNN
+ Config: configs/nuimages/htc_x101_64x4d_fpn_dconv_c3-c5_coco-20e_16x1_20e_nuim.py
+ Metadata:
+ Training Memory (GB): 13.3
+ Training Resources: 8x V100 GPUs
+ Results:
+ - Task: Object Detection
+ Dataset: nuImages
+ Metrics:
+ Box AP: 57.3
+ - Task: Instance Segmentation
+ Dataset: nuImages
+ Metrics:
+ Mask AP: 46.4
+ Weights: https://download.openmmlab.com/mmdetection3d/v0.1.0_models/nuimages_semseg/htc_x101_64x4d_fpn_dconv_c3-c5_coco-20e_16x1_20e_nuim/htc_x101_64x4d_fpn_dconv_c3-c5_coco-20e_16x1_20e_nuim_20201008_211222-0b16ac4b.pth
diff --git a/configs/paconv/README.md b/configs/paconv/README.md
new file mode 100644
index 0000000..83ab5b0
--- /dev/null
+++ b/configs/paconv/README.md
@@ -0,0 +1,51 @@
+# PAConv: Position Adaptive Convolution with Dynamic Kernel Assembling on Point Clouds
+
+> [PAConv: Position Adaptive Convolution with Dynamic Kernel Assembling on Point Clouds](https://arxiv.org/abs/2103.14635)
+
+
+
+## Abstract
+
+We introduce Position Adaptive Convolution (PAConv), a generic convolution operation for 3D point cloud processing. The key of PAConv is to construct the convolution kernel by dynamically assembling basic weight matrices stored in Weight Bank, where the coefficients of these weight matrices are self-adaptively learned from point positions through ScoreNet. In this way, the kernel is built in a data-driven manner, endowing PAConv with more flexibility than 2D convolutions to better handle the irregular and unordered point cloud data. Besides, the complexity of the learning process is reduced by combining weight matrices instead of brutally predicting kernels from point positions.
+Furthermore, different from the existing point convolution operators whose network architectures are often heavily engineered, we integrate our PAConv into classical MLP-based point cloud pipelines without changing network configurations. Even built on simple networks, our method still approaches or even surpasses the state-of-the-art models, and significantly improves baseline performance on both classification and segmentation tasks, yet with decent efficiency. Thorough ablation studies and visualizations are provided to understand PAConv.
+
+
+
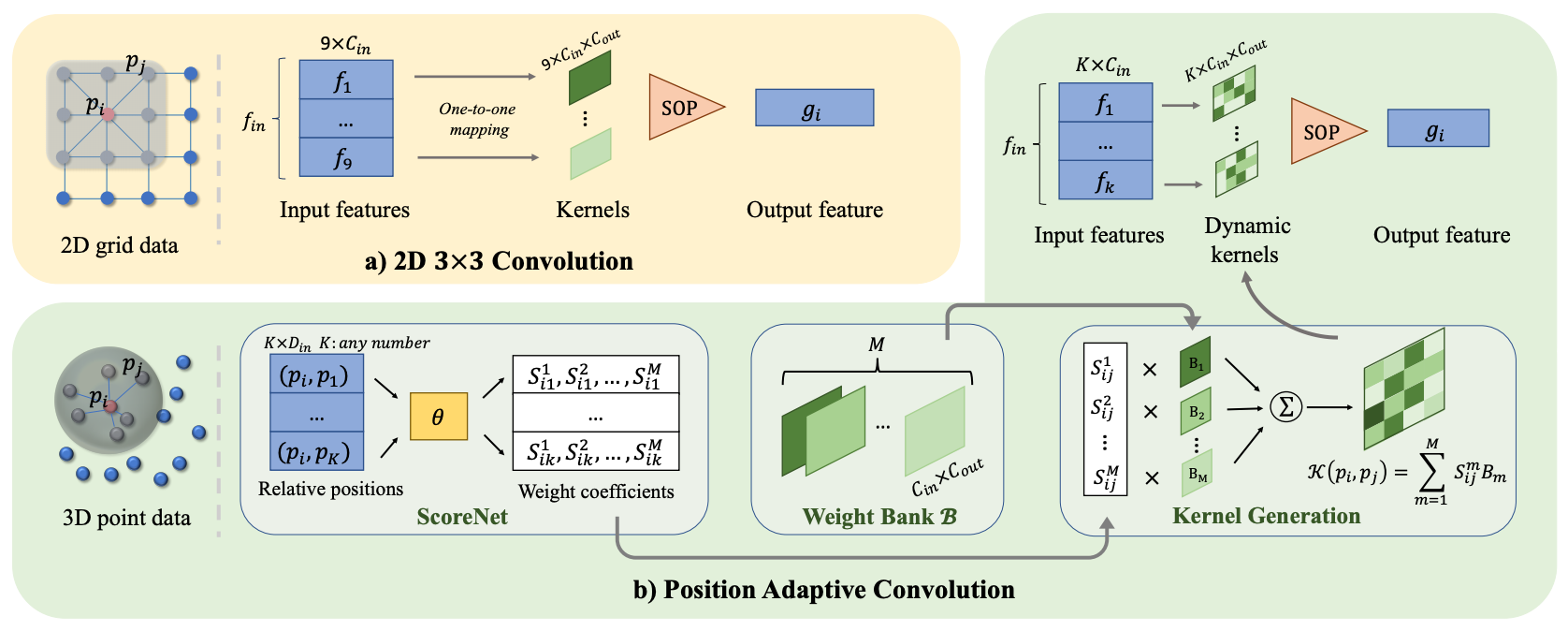
+
+
+## Introduction
+
+We implement PAConv and provide the result and checkpoints on S3DIS dataset.
+
+**Notice**: The original PAConv paper used step learning rate schedule. We discovered that cosine schedule achieves slightly better results and adopt it in our implementations.
+
+## Results and models
+
+### S3DIS
+
+| Method | Split | Lr schd | Mem (GB) | Inf time (fps) | mIoU (Val set) | Download |
+| :-------------------------------------------------------------------------: | :----: | :---------: | :------: | :------------: | :------------: | :------------------------------------------------------------------------------------------------------------------------------------------------------------------------------------------------------------------------------------------------------------------------------------------------------------------------------------------------------------------------------------------------------------------------------: |
+| [PAConv (SSG)](./paconv_ssg_8x8_cosine_150e_s3dis_seg-3d-13class.py) | Area_5 | cosine 150e | 5.8 | | 66.65 | [model](https://download.openmmlab.com/mmdetection3d/v0.1.0_models/paconv/paconv_ssg_8x8_cosine_150e_s3dis_seg-3d-13class/paconv_ssg_8x8_cosine_150e_s3dis_seg-3d-13class_20210729_200615-2147b2d1.pth) \| [log](https://download.openmmlab.com/mmdetection3d/v0.1.0_models/paconv/paconv_ssg_8x8_cosine_150e_s3dis_seg-3d-13class/paconv_ssg_8x8_cosine_150e_s3dis_seg-3d-13class_20210729_200615.log.json) |
+| [PAConv\* (SSG)](./paconv_cuda_ssg_8x8_cosine_200e_s3dis_seg-3d-13class.py) | Area_5 | cosine 200e | 3.8 | | 65.33 | [model](https://download.openmmlab.com/mmdetection3d/v0.1.0_models/paconv/paconv_cuda_ssg_8x8_cosine_200e_s3dis_seg-3d-13class/paconv_cuda_ssg_8x8_cosine_200e_s3dis_seg-3d-13class_20210802_171802-e5ea9bb9.pth) \| [log](https://download.openmmlab.com/mmdetection3d/v0.1.0_models/paconv/paconv_cuda_ssg_8x8_cosine_200e_s3dis_seg-3d-13class/paconv_cuda_ssg_8x8_cosine_200e_s3dis_seg-3d-13class_20210802_171802.log.json) |
+
+**Notes:**
+
+- We use XYZ+Color+Normalized_XYZ as input in all the experiments on S3DIS datasets.
+- `Area_5` Split means training the model on Area_1, 2, 3, 4, 6 and testing on Area_5.
+- PAConv\* stands for the CUDA implementation of PAConv operations. See the [paper](https://arxiv.org/pdf/2103.14635.pdf) appendix section D for more details. In our experiments, the training of PAConv\* is found to be very unstable. We achieved slightly lower mIoU than the result in the paper, but is consistent with the result obtained by running their [official code](https://github.com/CVMI-Lab/PAConv/tree/main/scene_seg). Besides, although the GPU memory consumption of PAConv\* is significantly lower than PAConv, its training and inference speed are actually slower (by ~10%).
+
+## Indeterminism
+
+Since PAConv testing adopts sliding patch inference which involves random point sampling, and the test script uses fixed random seeds while the random seeds of validation in training are not fixed, the test results may be slightly different from the results reported above.
+
+## Citation
+
+```latex
+@inproceedings{xu2021paconv,
+ title={PAConv: Position Adaptive Convolution with Dynamic Kernel Assembling on Point Clouds},
+ author={Xu, Mutian and Ding, Runyu and Zhao, Hengshuang and Qi, Xiaojuan},
+ booktitle={Proceedings of the IEEE/CVF Conference on Computer Vision and Pattern Recognition},
+ pages={3173--3182},
+ year={2021}
+}
+```
diff --git a/configs/paconv/metafile.yml b/configs/paconv/metafile.yml
new file mode 100644
index 0000000..589f807
--- /dev/null
+++ b/configs/paconv/metafile.yml
@@ -0,0 +1,29 @@
+Collections:
+ - Name: PAConv
+ Metadata:
+ Training Techniques:
+ - SGD
+ Training Resources: 8x Titan XP GPUs
+ Architecture:
+ - PAConv
+ Paper:
+ URL: https://arxiv.org/abs/2103.14635
+ Title: 'PAConv: Position Adaptive Convolution with Dynamic Kernel Assembling on Point Clouds'
+ README: configs/paconv/README.md
+ Code:
+ URL: https://github.com/open-mmlab/mmdetection3d/blob/master/mmdet3d/ops/paconv/paconv.py#L106
+ Version: v0.16.0
+
+Models:
+ - Name: paconv_ssg_8x8_cosine_150e_s3dis_seg-3d-13class.py
+ In Collection: PAConv
+ Config: configs/paconv/paconv_ssg_8x8_cosine_150e_s3dis_seg-3d-13class.py
+ Metadata:
+ Training Data: S3DIS
+ Training Memory (GB): 5.8
+ Results:
+ - Task: 3D Semantic Segmentation
+ Dataset: S3DIS
+ Metrics:
+ mIoU: 66.65
+ Weights: https://download.openmmlab.com/mmdetection3d/v0.1.0_models/paconv/paconv_ssg_8x8_cosine_150e_s3dis_seg-3d-13class/paconv_ssg_8x8_cosine_150e_s3dis_seg-3d-13class_20210729_200615-2147b2d1.pth
diff --git a/configs/paconv/paconv_cuda_ssg_8x8_cosine_200e_s3dis_seg-3d-13class.py b/configs/paconv/paconv_cuda_ssg_8x8_cosine_200e_s3dis_seg-3d-13class.py
new file mode 100644
index 0000000..b2a1440
--- /dev/null
+++ b/configs/paconv/paconv_cuda_ssg_8x8_cosine_200e_s3dis_seg-3d-13class.py
@@ -0,0 +1,69 @@
+_base_ = [
+ '../_base_/datasets/s3dis_seg-3d-13class.py',
+ '../_base_/models/paconv_cuda_ssg.py',
+ '../_base_/schedules/seg_cosine_150e.py', '../_base_/default_runtime.py'
+]
+
+# data settings
+class_names = ('ceiling', 'floor', 'wall', 'beam', 'column', 'window', 'door',
+ 'table', 'chair', 'sofa', 'bookcase', 'board', 'clutter')
+num_points = 4096
+train_pipeline = [
+ dict(
+ type='LoadPointsFromFile',
+ coord_type='DEPTH',
+ shift_height=False,
+ use_color=True,
+ load_dim=6,
+ use_dim=[0, 1, 2, 3, 4, 5]),
+ dict(
+ type='LoadAnnotations3D',
+ with_bbox_3d=False,
+ with_label_3d=False,
+ with_mask_3d=False,
+ with_seg_3d=True),
+ dict(
+ type='PointSegClassMapping',
+ valid_cat_ids=tuple(range(len(class_names))),
+ max_cat_id=13),
+ dict(
+ type='IndoorPatchPointSample',
+ num_points=num_points,
+ block_size=1.0,
+ use_normalized_coord=True,
+ num_try=10000,
+ enlarge_size=None,
+ min_unique_num=num_points // 4,
+ eps=0.0),
+ dict(type='NormalizePointsColor', color_mean=None),
+ dict(
+ type='GlobalRotScaleTrans',
+ rot_range=[0.0, 6.283185307179586], # [0, 2 * pi]
+ scale_ratio_range=[0.8, 1.2],
+ translation_std=[0, 0, 0]),
+ dict(
+ type='RandomJitterPoints',
+ jitter_std=[0.01, 0.01, 0.01],
+ clip_range=[-0.05, 0.05]),
+ dict(type='RandomDropPointsColor', drop_ratio=0.2),
+ dict(type='DefaultFormatBundle3D', class_names=class_names),
+ dict(type='Collect3D', keys=['points', 'pts_semantic_mask'])
+]
+
+data = dict(samples_per_gpu=8, train=dict(pipeline=train_pipeline))
+evaluation = dict(interval=1)
+
+# model settings
+model = dict(
+ decode_head=dict(
+ num_classes=13, ignore_index=13,
+ loss_decode=dict(class_weight=None)), # S3DIS doesn't use class_weight
+ test_cfg=dict(
+ num_points=4096,
+ block_size=1.0,
+ sample_rate=0.5,
+ use_normalized_coord=True,
+ batch_size=12))
+
+# runtime settings
+runner = dict(max_epochs=200)
diff --git a/configs/paconv/paconv_ssg_8x8_cosine_150e_s3dis_seg-3d-13class.py b/configs/paconv/paconv_ssg_8x8_cosine_150e_s3dis_seg-3d-13class.py
new file mode 100644
index 0000000..6b22a67
--- /dev/null
+++ b/configs/paconv/paconv_ssg_8x8_cosine_150e_s3dis_seg-3d-13class.py
@@ -0,0 +1,66 @@
+_base_ = [
+ '../_base_/datasets/s3dis_seg-3d-13class.py',
+ '../_base_/models/paconv_ssg.py', '../_base_/schedules/seg_cosine_150e.py',
+ '../_base_/default_runtime.py'
+]
+
+# data settings
+class_names = ('ceiling', 'floor', 'wall', 'beam', 'column', 'window', 'door',
+ 'table', 'chair', 'sofa', 'bookcase', 'board', 'clutter')
+num_points = 4096
+train_pipeline = [
+ dict(
+ type='LoadPointsFromFile',
+ coord_type='DEPTH',
+ shift_height=False,
+ use_color=True,
+ load_dim=6,
+ use_dim=[0, 1, 2, 3, 4, 5]),
+ dict(
+ type='LoadAnnotations3D',
+ with_bbox_3d=False,
+ with_label_3d=False,
+ with_mask_3d=False,
+ with_seg_3d=True),
+ dict(
+ type='PointSegClassMapping',
+ valid_cat_ids=tuple(range(len(class_names))),
+ max_cat_id=13),
+ dict(
+ type='IndoorPatchPointSample',
+ num_points=num_points,
+ block_size=1.0,
+ use_normalized_coord=True,
+ num_try=10000,
+ enlarge_size=None,
+ min_unique_num=num_points // 4,
+ eps=0.0),
+ dict(type='NormalizePointsColor', color_mean=None),
+ dict(
+ type='GlobalRotScaleTrans',
+ rot_range=[0.0, 6.283185307179586], # [0, 2 * pi]
+ scale_ratio_range=[0.8, 1.2],
+ translation_std=[0, 0, 0]),
+ dict(
+ type='RandomJitterPoints',
+ jitter_std=[0.01, 0.01, 0.01],
+ clip_range=[-0.05, 0.05]),
+ dict(type='RandomDropPointsColor', drop_ratio=0.2),
+ dict(type='DefaultFormatBundle3D', class_names=class_names),
+ dict(type='Collect3D', keys=['points', 'pts_semantic_mask'])
+]
+
+data = dict(samples_per_gpu=8, train=dict(pipeline=train_pipeline))
+evaluation = dict(interval=1)
+
+# model settings
+model = dict(
+ decode_head=dict(
+ num_classes=13, ignore_index=13,
+ loss_decode=dict(class_weight=None)), # S3DIS doesn't use class_weight
+ test_cfg=dict(
+ num_points=4096,
+ block_size=1.0,
+ sample_rate=0.5,
+ use_normalized_coord=True,
+ batch_size=12))
diff --git a/configs/parta2/README.md b/configs/parta2/README.md
new file mode 100644
index 0000000..b94b849
--- /dev/null
+++ b/configs/parta2/README.md
@@ -0,0 +1,38 @@
+# From Points to Parts: 3D Object Detection from Point Cloud with Part-aware and Part-aggregation Network
+
+> [From Points to Parts: 3D Object Detection from Point Cloud with Part-aware and Part-aggregation Network](https://arxiv.org/abs/1907.03670)
+
+
+
+## Abstract
+
+3D object detection from LiDAR point cloud is a challenging problem in 3D scene understanding and has many practical applications. In this paper, we extend our preliminary work PointRCNN to a novel and strong point-cloud-based 3D object detection framework, the part-aware and aggregation neural network (Part-A2 net). The whole framework consists of the part-aware stage and the part-aggregation stage. Firstly, the part-aware stage for the first time fully utilizes free-of-charge part supervisions derived from 3D ground-truth boxes to simultaneously predict high quality 3D proposals and accurate intra-object part locations. The predicted intra-object part locations within the same proposal are grouped by our new-designed RoI-aware point cloud pooling module, which results in an effective representation to encode the geometry-specific features of each 3D proposal. Then the part-aggregation stage learns to re-score the box and refine the box location by exploring the spatial relationship of the pooled intra-object part locations. Extensive experiments are conducted to demonstrate the performance improvements from each component of our proposed framework. Our Part-A2 net outperforms all existing 3D detection methods and achieves new state-of-the-art on KITTI 3D object detection dataset by utilizing only the LiDAR point cloud data.
+
+
+
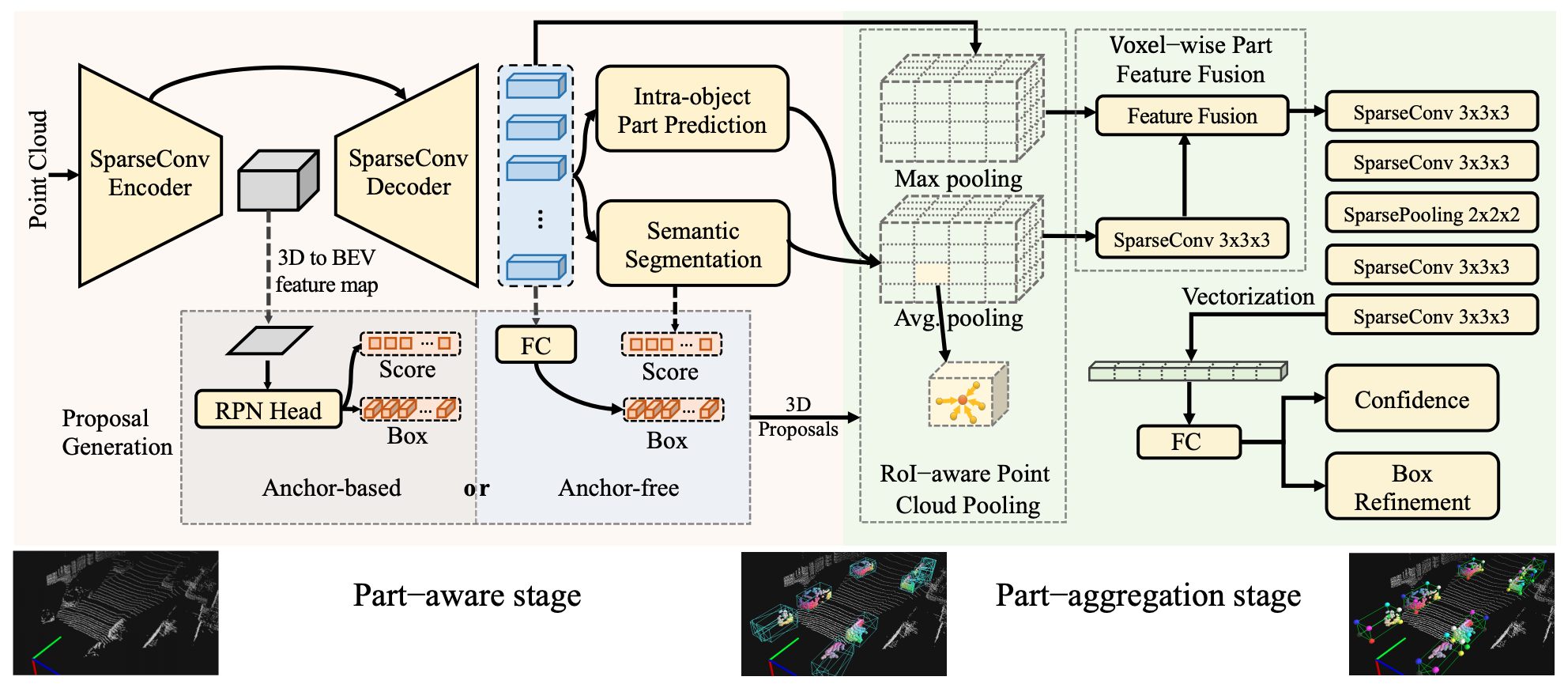
+
+
+## Introduction
+
+We implement Part-A^2 and provide its results and checkpoints on KITTI dataset.
+
+## Results and models
+
+### KITTI
+
+| Backbone | Class | Lr schd | Mem (GB) | Inf time (fps) | mAP | Download |
+| :------------------------------------------------------------: | :-----: | :--------: | :------: | :------------: | :---: | :----------------------------------------------------------------------------------------------------------------------------------------------------------------------------------------------------------------------------------------------------------------------------------------------------------------------------------------------------------------------------------------------------------: |
+| [SECFPN](./hv_PartA2_secfpn_2x8_cyclic_80e_kitti-3d-3class.py) | 3 Class | cyclic 80e | 4.1 | | 68.33 | [model](https://download.openmmlab.com/mmdetection3d/v1.0.0_models/parta2/hv_PartA2_secfpn_2x8_cyclic_80e_kitti-3d-3class/hv_PartA2_secfpn_2x8_cyclic_80e_kitti-3d-3class_20210831_022017-454a5344.pth) \| [log](https://download.openmmlab.com/mmdetection3d/v1.0.0_models/parta2/hv_PartA2_secfpn_2x8_cyclic_80e_kitti-3d-3class/hv_PartA2_secfpn_2x8_cyclic_80e_kitti-3d-3class_20210831_022017.log.json) |
+| [SECFPN](./hv_PartA2_secfpn_2x8_cyclic_80e_kitti-3d-car.py) | Car | cyclic 80e | 4.0 | | 79.08 | [model](https://download.openmmlab.com/mmdetection3d/v1.0.0_models/parta2/hv_PartA2_secfpn_2x8_cyclic_80e_kitti-3d-car/hv_PartA2_secfpn_2x8_cyclic_80e_kitti-3d-car_20210831_022017-cb7ff621.pth) \| [log](https://download.openmmlab.com/mmdetection3d/v1.0.0_models/parta2/hv_PartA2_secfpn_2x8_cyclic_80e_kitti-3d-car/hv_PartA2_secfpn_2x8_cyclic_80e_kitti-3d-car_20210831_022017.log.json) |
+
+## Citation
+
+```latex
+@article{shi2020points,
+ title={From points to parts: 3d object detection from point cloud with part-aware and part-aggregation network},
+ author={Shi, Shaoshuai and Wang, Zhe and Shi, Jianping and Wang, Xiaogang and Li, Hongsheng},
+ journal={IEEE Transactions on Pattern Analysis and Machine Intelligence},
+ year={2020},
+ publisher={IEEE}
+}
+```
diff --git a/configs/parta2/hv_PartA2_secfpn_2x8_cyclic_80e_kitti-3d-3class.py b/configs/parta2/hv_PartA2_secfpn_2x8_cyclic_80e_kitti-3d-3class.py
new file mode 100644
index 0000000..1166231
--- /dev/null
+++ b/configs/parta2/hv_PartA2_secfpn_2x8_cyclic_80e_kitti-3d-3class.py
@@ -0,0 +1,122 @@
+_base_ = [
+ '../_base_/schedules/cyclic_40e.py', '../_base_/default_runtime.py',
+ '../_base_/models/parta2.py'
+]
+
+point_cloud_range = [0, -40, -3, 70.4, 40, 1]
+
+# dataset settings
+dataset_type = 'KittiDataset'
+data_root = 'data/kitti/'
+class_names = ['Pedestrian', 'Cyclist', 'Car']
+input_modality = dict(use_lidar=True, use_camera=False)
+db_sampler = dict(
+ data_root=data_root,
+ info_path=data_root + 'kitti_dbinfos_train.pkl',
+ rate=1.0,
+ prepare=dict(
+ filter_by_difficulty=[-1],
+ filter_by_min_points=dict(Car=5, Pedestrian=10, Cyclist=10)),
+ classes=class_names,
+ sample_groups=dict(Car=12, Pedestrian=6, Cyclist=6))
+train_pipeline = [
+ dict(type='LoadPointsFromFile', coord_type='LIDAR', load_dim=4, use_dim=4),
+ dict(type='LoadAnnotations3D', with_bbox_3d=True, with_label_3d=True),
+ dict(type='ObjectSample', db_sampler=db_sampler),
+ dict(
+ type='ObjectNoise',
+ num_try=100,
+ translation_std=[1.0, 1.0, 0.5],
+ global_rot_range=[0.0, 0.0],
+ rot_range=[-0.78539816, 0.78539816]),
+ dict(type='RandomFlip3D', flip_ratio_bev_horizontal=0.5),
+ dict(
+ type='GlobalRotScaleTrans',
+ rot_range=[-0.78539816, 0.78539816],
+ scale_ratio_range=[0.95, 1.05]),
+ dict(type='PointsRangeFilter', point_cloud_range=point_cloud_range),
+ dict(type='ObjectRangeFilter', point_cloud_range=point_cloud_range),
+ dict(type='ObjectNameFilter', classes=class_names),
+ dict(type='PointShuffle'),
+ dict(type='DefaultFormatBundle3D', class_names=class_names),
+ dict(type='Collect3D', keys=['points', 'gt_bboxes_3d', 'gt_labels_3d'])
+]
+test_pipeline = [
+ dict(type='LoadPointsFromFile', coord_type='LIDAR', load_dim=4, use_dim=4),
+ dict(
+ type='MultiScaleFlipAug3D',
+ img_scale=(1333, 800),
+ pts_scale_ratio=1,
+ flip=False,
+ transforms=[
+ dict(
+ type='GlobalRotScaleTrans',
+ rot_range=[0, 0],
+ scale_ratio_range=[1., 1.],
+ translation_std=[0, 0, 0]),
+ dict(type='RandomFlip3D'),
+ dict(
+ type='PointsRangeFilter', point_cloud_range=point_cloud_range),
+ dict(
+ type='DefaultFormatBundle3D',
+ class_names=class_names,
+ with_label=False),
+ dict(type='Collect3D', keys=['points'])
+ ])
+]
+# construct a pipeline for data and gt loading in show function
+# please keep its loading function consistent with test_pipeline (e.g. client)
+eval_pipeline = [
+ dict(type='LoadPointsFromFile', coord_type='LIDAR', load_dim=4, use_dim=4),
+ dict(
+ type='DefaultFormatBundle3D',
+ class_names=class_names,
+ with_label=False),
+ dict(type='Collect3D', keys=['points'])
+]
+
+data = dict(
+ samples_per_gpu=2,
+ workers_per_gpu=2,
+ train=dict(
+ type='RepeatDataset',
+ times=2,
+ dataset=dict(
+ type=dataset_type,
+ data_root=data_root,
+ ann_file=data_root + 'kitti_infos_train.pkl',
+ split='training',
+ pts_prefix='velodyne_reduced',
+ pipeline=train_pipeline,
+ modality=input_modality,
+ classes=class_names,
+ box_type_3d='LiDAR',
+ test_mode=False)),
+ val=dict(
+ type=dataset_type,
+ data_root=data_root,
+ ann_file=data_root + 'kitti_infos_val.pkl',
+ split='training',
+ pts_prefix='velodyne_reduced',
+ pipeline=test_pipeline,
+ modality=input_modality,
+ classes=class_names,
+ box_type_3d='LiDAR',
+ test_mode=True),
+ test=dict(
+ type=dataset_type,
+ data_root=data_root,
+ ann_file=data_root + 'kitti_infos_val.pkl',
+ split='training',
+ pts_prefix='velodyne_reduced',
+ pipeline=test_pipeline,
+ modality=input_modality,
+ classes=class_names,
+ box_type_3d='LiDAR',
+ test_mode=True))
+
+# Part-A2 uses a different learning rate from what SECOND uses.
+lr = 0.001
+optimizer = dict(lr=lr)
+evaluation = dict(pipeline=eval_pipeline)
+find_unused_parameters = True
diff --git a/configs/parta2/hv_PartA2_secfpn_2x8_cyclic_80e_kitti-3d-car.py b/configs/parta2/hv_PartA2_secfpn_2x8_cyclic_80e_kitti-3d-car.py
new file mode 100644
index 0000000..89be085
--- /dev/null
+++ b/configs/parta2/hv_PartA2_secfpn_2x8_cyclic_80e_kitti-3d-car.py
@@ -0,0 +1,137 @@
+_base_ = './hv_PartA2_secfpn_2x8_cyclic_80e_kitti-3d-3class.py'
+
+point_cloud_range = [0, -40, -3, 70.4, 40, 1] # velodyne coordinates, x, y, z
+
+model = dict(
+ rpn_head=dict(
+ type='PartA2RPNHead',
+ num_classes=1,
+ anchor_generator=dict(
+ _delete_=True,
+ type='Anchor3DRangeGenerator',
+ ranges=[[0, -40.0, -1.78, 70.4, 40.0, -1.78]],
+ sizes=[[3.9, 1.6, 1.56]],
+ rotations=[0, 1.57],
+ reshape_out=False)),
+ roi_head=dict(
+ num_classes=1,
+ semantic_head=dict(num_classes=1),
+ bbox_head=dict(num_classes=1)),
+ # model training and testing settings
+ train_cfg=dict(
+ _delete_=True,
+ rpn=dict(
+ assigner=dict(
+ type='MaxIoUAssigner',
+ iou_calculator=dict(type='BboxOverlapsNearest3D'),
+ pos_iou_thr=0.6,
+ neg_iou_thr=0.45,
+ min_pos_iou=0.45,
+ ignore_iof_thr=-1),
+ allowed_border=0,
+ pos_weight=-1,
+ debug=False),
+ rpn_proposal=dict(
+ nms_pre=9000,
+ nms_post=512,
+ max_num=512,
+ nms_thr=0.8,
+ score_thr=0,
+ use_rotate_nms=False),
+ rcnn=dict(
+ assigner=dict( # for Car
+ type='MaxIoUAssigner',
+ iou_calculator=dict(type='BboxOverlaps3D', coordinate='lidar'),
+ pos_iou_thr=0.55,
+ neg_iou_thr=0.55,
+ min_pos_iou=0.55,
+ ignore_iof_thr=-1),
+ sampler=dict(
+ type='IoUNegPiecewiseSampler',
+ num=128,
+ pos_fraction=0.55,
+ neg_piece_fractions=[0.8, 0.2],
+ neg_iou_piece_thrs=[0.55, 0.1],
+ neg_pos_ub=-1,
+ add_gt_as_proposals=False,
+ return_iou=True),
+ cls_pos_thr=0.75,
+ cls_neg_thr=0.25)),
+ test_cfg=dict(
+ rpn=dict(
+ nms_pre=1024,
+ nms_post=100,
+ max_num=100,
+ nms_thr=0.7,
+ score_thr=0,
+ use_rotate_nms=True),
+ rcnn=dict(
+ use_rotate_nms=True,
+ use_raw_score=True,
+ nms_thr=0.01,
+ score_thr=0.1)))
+
+# dataset settings
+dataset_type = 'KittiDataset'
+data_root = 'data/kitti/'
+class_names = ['Car']
+input_modality = dict(use_lidar=True, use_camera=False)
+db_sampler = dict(
+ data_root=data_root,
+ info_path=data_root + 'kitti_dbinfos_train.pkl',
+ rate=1.0,
+ prepare=dict(filter_by_difficulty=[-1], filter_by_min_points=dict(Car=5)),
+ classes=class_names,
+ sample_groups=dict(Car=15))
+train_pipeline = [
+ dict(type='LoadPointsFromFile', coord_type='LIDAR', load_dim=4, use_dim=4),
+ dict(type='LoadAnnotations3D', with_bbox_3d=True, with_label_3d=True),
+ dict(type='ObjectSample', db_sampler=db_sampler),
+ dict(
+ type='ObjectNoise',
+ num_try=100,
+ translation_std=[1.0, 1.0, 0.5],
+ global_rot_range=[0.0, 0.0],
+ rot_range=[-0.78539816, 0.78539816]),
+ dict(type='RandomFlip3D', flip_ratio_bev_horizontal=0.5),
+ dict(
+ type='GlobalRotScaleTrans',
+ rot_range=[-0.78539816, 0.78539816],
+ scale_ratio_range=[0.95, 1.05]),
+ dict(type='PointsRangeFilter', point_cloud_range=point_cloud_range),
+ dict(type='ObjectRangeFilter', point_cloud_range=point_cloud_range),
+ dict(type='ObjectNameFilter', classes=class_names),
+ dict(type='PointShuffle'),
+ dict(type='DefaultFormatBundle3D', class_names=class_names),
+ dict(type='Collect3D', keys=['points', 'gt_bboxes_3d', 'gt_labels_3d'])
+]
+test_pipeline = [
+ dict(type='LoadPointsFromFile', coord_type='LIDAR', load_dim=4, use_dim=4),
+ dict(
+ type='MultiScaleFlipAug3D',
+ img_scale=(1333, 800),
+ pts_scale_ratio=1,
+ flip=False,
+ transforms=[
+ dict(
+ type='GlobalRotScaleTrans',
+ rot_range=[0, 0],
+ scale_ratio_range=[1., 1.],
+ translation_std=[0, 0, 0]),
+ dict(type='RandomFlip3D'),
+ dict(
+ type='PointsRangeFilter', point_cloud_range=point_cloud_range),
+ dict(
+ type='DefaultFormatBundle3D',
+ class_names=class_names,
+ with_label=False),
+ dict(type='Collect3D', keys=['points'])
+ ])
+]
+
+data = dict(
+ train=dict(dataset=dict(pipeline=train_pipeline, classes=class_names)),
+ val=dict(pipeline=test_pipeline, classes=class_names),
+ test=dict(pipeline=test_pipeline, classes=class_names))
+
+find_unused_parameters = True
diff --git a/configs/parta2/metafile.yml b/configs/parta2/metafile.yml
new file mode 100644
index 0000000..d626fcb
--- /dev/null
+++ b/configs/parta2/metafile.yml
@@ -0,0 +1,41 @@
+Collections:
+ - Name: Part-A^2
+ Metadata:
+ Training Data: KITTI
+ Training Techniques:
+ - AdamW
+ Training Resources: 8x V100 GPUs
+ Architecture:
+ - Sparse U-Net
+ Paper:
+ URL: https://arxiv.org/abs/1907.03670
+ Title: 'From Points to Parts: 3D Object Detection from Point Cloud with Part-aware and Part-aggregation Network'
+ README: configs/parta2/README.md
+ Code:
+ URL: https://github.com/open-mmlab/mmdetection3d/blob/master/mmdet3d/models/detectors/parta2.py#L12
+ Version: v0.5.0
+
+Models:
+ - Name: hv_PartA2_secfpn_2x8_cyclic_80e_kitti-3d-3class
+ In Collection: Part-A^2
+ Config: configs/parta2/hv_PartA2_secfpn_2x8_cyclic_80e_kitti-3d-3class.py
+ Metadata:
+ Training Memory (GB): 4.1
+ Results:
+ - Task: 3D Object Detection
+ Dataset: KITTI
+ Metrics:
+ mAP: 68.33
+ Weights: https://download.openmmlab.com/mmdetection3d/v1.0.0_models/parta2/hv_PartA2_secfpn_2x8_cyclic_80e_kitti-3d-3class/hv_PartA2_secfpn_2x8_cyclic_80e_kitti-3d-3class_20210831_022017-454a5344.pth
+
+ - Name: hv_PartA2_secfpn_2x8_cyclic_80e_kitti-3d-car
+ In Collection: Part-A^2
+ Config: configs/parta2/hv_PartA2_secfpn_2x8_cyclic_80e_kitti-3d-car.py
+ Metadata:
+ Training Memory (GB): 4.0
+ Results:
+ - Task: 3D Object Detection
+ Dataset: KITTI
+ Metrics:
+ mAP: 79.08
+ Weights: https://download.openmmlab.com/mmdetection3d/v1.0.0_models/parta2/hv_PartA2_secfpn_2x8_cyclic_80e_kitti-3d-car/hv_PartA2_secfpn_2x8_cyclic_80e_kitti-3d-car_20210831_022017-cb7ff621.pth
diff --git a/configs/pgd/README.md b/configs/pgd/README.md
new file mode 100644
index 0000000..f805f53
--- /dev/null
+++ b/configs/pgd/README.md
@@ -0,0 +1,69 @@
+# Probabilistic and Geometric Depth: Detecting Objects in Perspective
+
+> [Probabilistic and Geometric Depth: Detecting Objects in Perspective](https://arxiv.org/abs/2107.14160)
+
+
+
+## Abstract
+
+3D object detection is an important capability needed in various practical applications such as driver assistance systems. Monocular 3D detection, as a representative general setting among image-based approaches, provides a more economical solution than conventional settings relying on LiDARs but still yields unsatisfactory results. This paper first presents a systematic study on this problem. We observe that the current monocular 3D detection can be simplified as an instance depth estimation problem: The inaccurate instance depth blocks all the other 3D attribute predictions from improving the overall detection performance. Moreover, recent methods directly estimate the depth based on isolated instances or pixels while ignoring the geometric relations across different objects. To this end, we construct geometric relation graphs across predicted objects and use the graph to facilitate depth estimation. As the preliminary depth estimation of each instance is usually inaccurate in this ill-posed setting, we incorporate a probabilistic representation to capture the uncertainty. It provides an important indicator to identify confident predictions and further guide the depth propagation. Despite the simplicity of the basic idea, our method, PGD, obtains significant improvements on KITTI and nuScenes benchmarks, achieving 1st place out of all monocular vision-only methods while still maintaining real-time efficiency. Code and models will be released at [this https URL](https://github.com/open-mmlab/mmdetection3d).
+
+
+
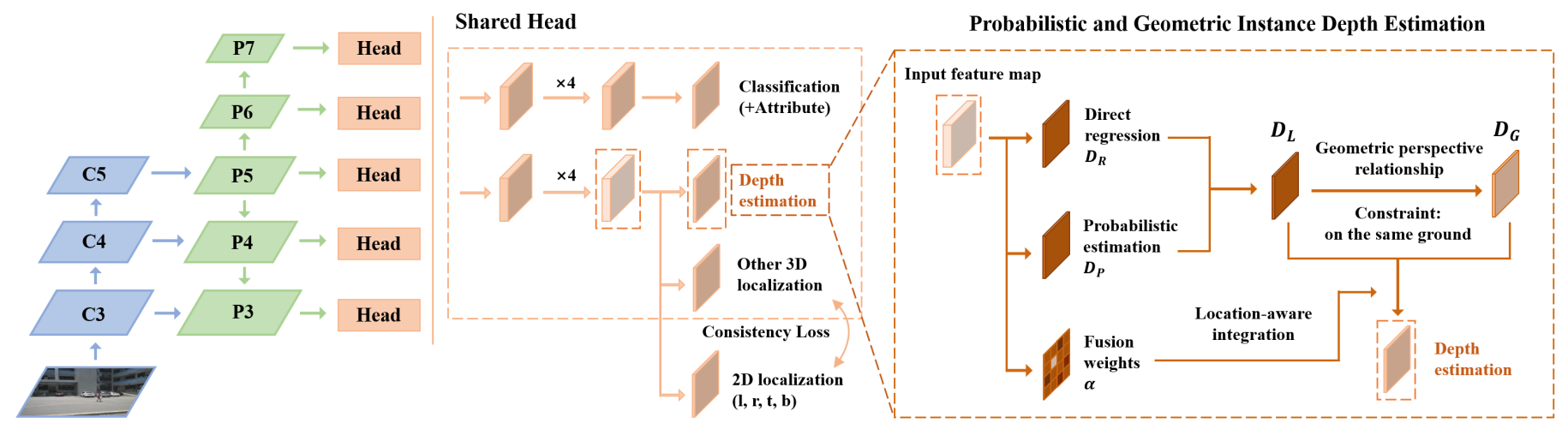
+
+
+## Introduction
+
+PGD, also can be regarded as FCOS3D++, is a simple yet effective monocular 3D detector. It enhances the FCOS3D baseline by involving local geometric constraints and improving instance depth estimation.
+
+We release the code and model for both KITTI and nuScenes benchmark, which is a good supplement for the original FCOS3D baseline (only supported on nuScenes).
+
+For clean implementation, our preliminary release supports base models with proposed local geometric constraints and the probabilistic depth representation. We will involve the geometric graph part in the future.
+
+A more extensive study based on FCOS3D and PGD is on-going. Please stay tuned.
+
+## Results and models
+
+### KITTI
+
+| Backbone | Lr schd | Mem (GB) | Inf time (fps) | mAP_11 / mAP_40 | Download |
+| :--------------------------------------------------------------: | :-----: | :------: | :------------: | :-------------: | :------------------------------------------------------------------------------------------------------------------------------------------------------------------------------------------------------------------------------------------------------------------------------------------------------------------------------------------------------------------------------------------------: |
+| [ResNet101](./pgd_r101_caffe_fpn_gn-head_3x4_4x_kitti-mono3d.py) | 4x | 9.07 | | 18.33 / 13.23 | [model](https://download.openmmlab.com/mmdetection3d/v1.0.0_models/pgd/pgd_r101_caffe_fpn_gn-head_3x4_4x_kitti-mono3d/pgd_r101_caffe_fpn_gn-head_3x4_4x_kitti-mono3d_20211022_102608-8a97533b.pth) \| [log](https://download.openmmlab.com/mmdetection3d/v1.0.0_models/pgd/pgd_r101_caffe_fpn_gn-head_3x4_4x_kitti-mono3d/pgd_r101_caffe_fpn_gn-head_3x4_4x_kitti-mono3d_20211022_102608.log.json) |
+
+Detailed performance on KITTI 3D detection (3D/BEV) is as follows, evaluated by AP11 and AP40 metric:
+
+| | Easy | Moderate | Hard |
+| ---------- | :-----------: | :-----------: | :-----------: |
+| Car (AP11) | 24.09 / 30.11 | 18.33 / 23.46 | 16.90 / 19.33 |
+| Car (AP40) | 19.27 / 26.60 | 13.23 / 18.23 | 10.65 / 15.00 |
+
+Note: mAP represents Car moderate 3D strict AP11 / AP40 results. Because of the limited data for pedestrians and cyclists, the detection performance for these two classes is usually unstable. Therefore, we only list car detection results here. In addition, AP40 is a more recommended metric for reference due to its much better stability.
+
+### NuScenes
+
+| Backbone | Lr schd | Mem (GB) | mAP | NDS | Download |
+| :------------------------------------------------------------------------------: | :-----: | :------: | :--: | :--: | :--------------------------------------------------------------------------------------------------------------------------------------------------------------------------------------------------------------------------------------------------------------------------------------------------------------------------------------------------------------------------------------------------------------------------------: |
+| [ResNet101 w/ DCN](./pgd_r101_caffe_fpn_gn-head_2x16_1x_nus-mono3d.py) | 1x | 9.20 | 31.7 | 39.3 | [model](https://download.openmmlab.com/mmdetection3d/v1.0.0_models/pgd/pgd_r101_caffe_fpn_gn-head_2x16_1x_nus-mono3d/pgd_r101_caffe_fpn_gn-head_2x16_1x_nus-mono3d_20211116_195350-f4b5eec2.pth) \| [log](https://download.openmmlab.com/mmdetection3d/v1.0.0_models/pgd/pgd_r101_caffe_fpn_gn-head_2x16_1x_nus-mono3d/pgd_r101_caffe_fpn_gn-head_2x16_1x_nus-mono3d_20211116_195350.log.json) |
+| [above w/ finetune](./pgd_r101_caffe_fpn_gn-head_2x16_1x_nus-mono3d_finetune.py) | 1x | 9.20 | 34.6 | 41.1 | [model](https://download.openmmlab.com/mmdetection3d/v1.0.0_models/pgd/pgd_r101_caffe_fpn_gn-head_2x16_1x_nus-mono3d_finetune/pgd_r101_caffe_fpn_gn-head_2x16_1x_nus-mono3d_finetune_20211118_093245-fd419681.pth) \| [log](https://download.openmmlab.com/mmdetection3d/v1.0.0_models/pgd/pgd_r101_caffe_fpn_gn-head_2x16_1x_nus-mono3d_finetune/pgd_r101_caffe_fpn_gn-head_2x16_1x_nus-mono3d_finetune_20211118_093245.log.json) |
+| above w/ tta | 1x | 9.20 | 35.5 | 41.8 | |
+| [ResNet101 w/ DCN](./pgd_r101_caffe_fpn_gn-head_2x16_2x_nus-mono3d.py) | 2x | 9.20 | 33.6 | 40.9 | [model](https://download.openmmlab.com/mmdetection3d/v1.0.0_models/pgd/pgd_r101_caffe_fpn_gn-head_2x16_2x_nus-mono3d/pgd_r101_caffe_fpn_gn-head_2x16_2x_nus-mono3d_20211112_125314-cb677266.pth) \| [log](https://download.openmmlab.com/mmdetection3d/v1.0.0_models/pgd/pgd_r101_caffe_fpn_gn-head_2x16_2x_nus-mono3d/pgd_r101_caffe_fpn_gn-head_2x16_2x_nus-mono3d_20211112_125314.log.json) |
+| [above w/ finetune](./pgd_r101_caffe_fpn_gn-head_2x16_2x_nus-mono3d_finetune.py) | 2x | 9.20 | 35.8 | 42.5 | [model](https://download.openmmlab.com/mmdetection3d/v1.0.0_models/pgd/pgd_r101_caffe_fpn_gn-head_2x16_2x_nus-mono3d_finetune/pgd_r101_caffe_fpn_gn-head_2x16_2x_nus-mono3d_finetune_20211114_162135-5ec7c1cd.pth) \| [log](https://download.openmmlab.com/mmdetection3d/v1.0.0_models/pgd/pgd_r101_caffe_fpn_gn-head_2x16_2x_nus-mono3d_finetune/pgd_r101_caffe_fpn_gn-head_2x16_2x_nus-mono3d_finetune_20211114_162135.log.json) |
+| above w/ tta | 2x | 9.20 | 36.8 | 43.1 | |
+
+## Citation
+
+```latex
+@inproceedings{wang2021pgd,
+ title={{Probabilistic and Geometric Depth: Detecting} Objects in Perspective},
+ author={Wang, Tai and Zhu, Xinge and Pang, Jiangmiao and Lin, Dahua},
+ booktitle={Conference on Robot Learning (CoRL) 2021},
+ year={2021}
+}
+# For the baseline version
+@inproceedings{wang2021fcos3d,
+ title={{FCOS3D: Fully} Convolutional One-Stage Monocular 3D Object Detection},
+ author={Wang, Tai and Zhu, Xinge and Pang, Jiangmiao and Lin, Dahua},
+ booktitle={Proceedings of the IEEE/CVF International Conference on Computer Vision (ICCV) Workshops},
+ year={2021}
+}
+```
diff --git a/configs/pgd/metafile.yml b/configs/pgd/metafile.yml
new file mode 100644
index 0000000..d7d6626
--- /dev/null
+++ b/configs/pgd/metafile.yml
@@ -0,0 +1,81 @@
+Collections:
+ - Name: PGD
+ Metadata:
+ Training Data: KITTI
+ Training Techniques:
+ - SGD
+ Training Resources: 4x TITAN XP
+ Architecture:
+ - PGDHead
+ Paper:
+ URL: https://arxiv.org/abs/2107.14160
+ Title: 'Probabilistic and Geometric Depth: Detecting Objects in Perspective'
+ README: configs/pgd/README.md
+ Code:
+ URL: https://github.com/open-mmlab/mmdetection3d/blob/v1.0.0.dev0/mmdet3d/models/dense_heads/pgd_head.py#17
+ Version: v1.0.0
+
+Models:
+ - Name: pgd_r101_caffe_fpn_gn-head_3x4_4x_kitti-mono3d
+ In Collection: PGD
+ Config: configs/pgd/pgd_r101_caffe_fpn_gn-head_3x4_4x_kitti-mono3d.py
+ Metadata:
+ Training Memory (GB): 9.1
+ Results:
+ - Task: 3D Object Detection
+ Dataset: KITTI
+ Metrics:
+ mAP: 18.33
+ Weights: https://download.openmmlab.com/mmdetection3d/v1.0.0_models/pgd/pgd_r101_caffe_fpn_gn-head_3x4_4x_kitti-mono3d/pgd_r101_caffe_fpn_gn-head_3x4_4x_kitti-mono3d_20211022_102608-8a97533b.pth
+
+ - Name: pgd_r101_caffe_fpn_gn-head_2x16_1x_nus-mono3d
+ In Collection: PGD
+ Config: configs/pgd/pgd_r101_caffe_fpn_gn-head_2x16_1x_nus-mono3d.py
+ Metadata:
+ Training Memory (GB): 9.2
+ Results:
+ - Task: 3D Object Detection
+ Dataset: nuScenes
+ Metrics:
+ mAP: 31.7
+ NDS: 39.3
+ Weights: https://download.openmmlab.com/mmdetection3d/v1.0.0_models/pgd/pgd_r101_caffe_fpn_gn-head_2x16_1x_nus-mono3d/pgd_r101_caffe_fpn_gn-head_2x16_1x_nus-mono3d_20211116_195350-f4b5eec2.pth
+
+ - Name: pgd_r101_caffe_fpn_gn-head_2x16_1x_nus-mono3d_finetune
+ In Collection: PGD
+ Config: configs/pgd/pgd_r101_caffe_fpn_gn-head_2x16_1x_nus-mono3d_finetune.py
+ Metadata:
+ Training Memory (GB): 9.2
+ Results:
+ - Task: 3D Object Detection
+ Dataset: nuScenes
+ Metrics:
+ mAP: 34.6
+ NDS: 41.1
+ Weights: https://download.openmmlab.com/mmdetection3d/v1.0.0_models/pgd/pgd_r101_caffe_fpn_gn-head_2x16_1x_nus-mono3d_finetune/pgd_r101_caffe_fpn_gn-head_2x16_1x_nus-mono3d_finetune_20211118_093245-fd419681.pth
+
+ - Name: pgd_r101_caffe_fpn_gn-head_2x16_2x_nus-mono3d
+ In Collection: PGD
+ Config: configs/pgd/pgd_r101_caffe_fpn_gn-head_2x16_2x_nus-mono3d.py
+ Metadata:
+ Training Memory (GB): 9.2
+ Results:
+ - Task: 3D Object Detection
+ Dataset: nuScenes
+ Metrics:
+ mAP: 33.6
+ NDS: 40.9
+ Weights: https://download.openmmlab.com/mmdetection3d/v1.0.0_models/pgd/pgd_r101_caffe_fpn_gn-head_2x16_2x_nus-mono3d/pgd_r101_caffe_fpn_gn-head_2x16_2x_nus-mono3d_20211112_125314-cb677266.pth
+
+ - Name: pgd_r101_caffe_fpn_gn-head_2x16_2x_nus-mono3d_finetune
+ In Collection: PGD
+ Config: configs/pgd/pgd_r101_caffe_fpn_gn-head_2x16_2x_nus-mono3d_finetune.py
+ Metadata:
+ Training Memory (GB): 9.2
+ Results:
+ - Task: 3D Object Detection
+ Dataset: nuScenes
+ Metrics:
+ mAP: 35.8
+ NDS: 42.5
+ Weights: https://download.openmmlab.com/mmdetection3d/v1.0.0_models/pgd/pgd_r101_caffe_fpn_gn-head_2x16_2x_nus-mono3d_finetune/pgd_r101_caffe_fpn_gn-head_2x16_2x_nus-mono3d_finetune_20211114_162135-5ec7c1cd.pth
diff --git a/configs/pgd/pgd_r101_caffe_fpn_gn-head_2x16_1x_nus-mono3d.py b/configs/pgd/pgd_r101_caffe_fpn_gn-head_2x16_1x_nus-mono3d.py
new file mode 100644
index 0000000..37b5049
--- /dev/null
+++ b/configs/pgd/pgd_r101_caffe_fpn_gn-head_2x16_1x_nus-mono3d.py
@@ -0,0 +1,107 @@
+_base_ = [
+ '../_base_/datasets/nus-mono3d.py', '../_base_/models/pgd.py',
+ '../_base_/schedules/mmdet_schedule_1x.py', '../_base_/default_runtime.py'
+]
+# model settings
+model = dict(
+ backbone=dict(
+ dcn=dict(type='DCNv2', deform_groups=1, fallback_on_stride=False),
+ stage_with_dcn=(False, False, True, True)),
+ bbox_head=dict(
+ pred_bbox2d=True,
+ group_reg_dims=(2, 1, 3, 1, 2,
+ 4), # offset, depth, size, rot, velo, bbox2d
+ reg_branch=(
+ (256, ), # offset
+ (256, ), # depth
+ (256, ), # size
+ (256, ), # rot
+ (), # velo
+ (256, ) # bbox2d
+ ),
+ loss_depth=dict(type='SmoothL1Loss', beta=1.0 / 9.0, loss_weight=1.0),
+ bbox_coder=dict(
+ type='PGDBBoxCoder',
+ base_depths=((31.99, 21.12), (37.15, 24.63), (39.69, 23.97),
+ (40.91, 26.34), (34.16, 20.11), (22.35, 13.70),
+ (24.28, 16.05), (27.26, 15.50), (20.61, 13.68),
+ (22.74, 15.01)),
+ base_dims=((4.62, 1.73, 1.96), (6.93, 2.83, 2.51),
+ (12.56, 3.89, 2.94), (11.22, 3.50, 2.95),
+ (6.68, 3.21, 2.85), (6.68, 3.21, 2.85),
+ (2.11, 1.46, 0.78), (0.73, 1.77, 0.67),
+ (0.41, 1.08, 0.41), (0.50, 0.99, 2.52)),
+ code_size=9)),
+ # set weight 1.0 for base 7 dims (offset, depth, size, rot)
+ # 0.05 for 2-dim velocity and 0.2 for 4-dim 2D distance targets
+ train_cfg=dict(code_weight=[
+ 1.0, 1.0, 0.2, 1.0, 1.0, 1.0, 1.0, 0.05, 0.05, 0.2, 0.2, 0.2, 0.2
+ ]),
+ test_cfg=dict(nms_pre=1000, nms_thr=0.8, score_thr=0.01, max_per_img=200))
+
+class_names = [
+ 'car', 'truck', 'trailer', 'bus', 'construction_vehicle', 'bicycle',
+ 'motorcycle', 'pedestrian', 'traffic_cone', 'barrier'
+]
+img_norm_cfg = dict(
+ mean=[103.530, 116.280, 123.675], std=[1.0, 1.0, 1.0], to_rgb=False)
+train_pipeline = [
+ dict(type='LoadImageFromFileMono3D'),
+ dict(
+ type='LoadAnnotations3D',
+ with_bbox=True,
+ with_label=True,
+ with_attr_label=True,
+ with_bbox_3d=True,
+ with_label_3d=True,
+ with_bbox_depth=True),
+ dict(type='Resize', img_scale=(1600, 900), keep_ratio=True),
+ dict(type='RandomFlip3D', flip_ratio_bev_horizontal=0.5),
+ dict(type='Normalize', **img_norm_cfg),
+ dict(type='Pad', size_divisor=32),
+ dict(type='DefaultFormatBundle3D', class_names=class_names),
+ dict(
+ type='Collect3D',
+ keys=[
+ 'img', 'gt_bboxes', 'gt_labels', 'attr_labels', 'gt_bboxes_3d',
+ 'gt_labels_3d', 'centers2d', 'depths'
+ ]),
+]
+test_pipeline = [
+ dict(type='LoadImageFromFileMono3D'),
+ dict(
+ type='MultiScaleFlipAug',
+ scale_factor=1.0,
+ flip=False,
+ transforms=[
+ dict(type='RandomFlip3D'),
+ dict(type='Normalize', **img_norm_cfg),
+ dict(type='Pad', size_divisor=32),
+ dict(
+ type='DefaultFormatBundle3D',
+ class_names=class_names,
+ with_label=False),
+ dict(type='Collect3D', keys=['img']),
+ ])
+]
+data = dict(
+ samples_per_gpu=2,
+ workers_per_gpu=2,
+ train=dict(pipeline=train_pipeline),
+ val=dict(pipeline=test_pipeline),
+ test=dict(pipeline=test_pipeline))
+# optimizer
+optimizer = dict(
+ lr=0.004, paramwise_cfg=dict(bias_lr_mult=2., bias_decay_mult=0.))
+optimizer_config = dict(
+ _delete_=True, grad_clip=dict(max_norm=35, norm_type=2))
+# learning policy
+lr_config = dict(
+ policy='step',
+ warmup='linear',
+ warmup_iters=500,
+ warmup_ratio=1.0 / 3,
+ step=[8, 11])
+total_epochs = 12
+evaluation = dict(interval=4)
+runner = dict(max_epochs=total_epochs)
diff --git a/configs/pgd/pgd_r101_caffe_fpn_gn-head_2x16_1x_nus-mono3d_finetune.py b/configs/pgd/pgd_r101_caffe_fpn_gn-head_2x16_1x_nus-mono3d_finetune.py
new file mode 100644
index 0000000..f5d6423
--- /dev/null
+++ b/configs/pgd/pgd_r101_caffe_fpn_gn-head_2x16_1x_nus-mono3d_finetune.py
@@ -0,0 +1,9 @@
+_base_ = './pgd_r101_caffe_fpn_gn-head_2x16_1x_nus-mono3d.py'
+# model settings
+model = dict(
+ train_cfg=dict(code_weight=[
+ 1.0, 1.0, 1.0, 1.0, 1.0, 1.0, 1.0, 0.05, 0.05, 0.2, 0.2, 0.2, 0.2
+ ]))
+# optimizer
+optimizer = dict(lr=0.002)
+load_from = 'work_dirs/pgd_nus_benchmark_1x/latest.pth'
diff --git a/configs/pgd/pgd_r101_caffe_fpn_gn-head_2x16_2x_nus-mono3d.py b/configs/pgd/pgd_r101_caffe_fpn_gn-head_2x16_2x_nus-mono3d.py
new file mode 100644
index 0000000..2dd5957
--- /dev/null
+++ b/configs/pgd/pgd_r101_caffe_fpn_gn-head_2x16_2x_nus-mono3d.py
@@ -0,0 +1,5 @@
+_base_ = './pgd_r101_caffe_fpn_gn-head_2x16_1x_nus-mono3d.py'
+# learning policy
+lr_config = dict(step=[16, 22])
+total_epochs = 24
+runner = dict(max_epochs=total_epochs)
diff --git a/configs/pgd/pgd_r101_caffe_fpn_gn-head_2x16_2x_nus-mono3d_finetune.py b/configs/pgd/pgd_r101_caffe_fpn_gn-head_2x16_2x_nus-mono3d_finetune.py
new file mode 100644
index 0000000..19a3d63
--- /dev/null
+++ b/configs/pgd/pgd_r101_caffe_fpn_gn-head_2x16_2x_nus-mono3d_finetune.py
@@ -0,0 +1,9 @@
+_base_ = './pgd_r101_caffe_fpn_gn-head_2x16_2x_nus-mono3d.py'
+# model settings
+model = dict(
+ train_cfg=dict(code_weight=[
+ 1.0, 1.0, 1.0, 1.0, 1.0, 1.0, 1.0, 0.05, 0.05, 0.2, 0.2, 0.2, 0.2
+ ]))
+# optimizer
+optimizer = dict(lr=0.002)
+load_from = 'work_dirs/pgd_nus_benchmark_2x/latest.pth'
diff --git a/configs/pgd/pgd_r101_caffe_fpn_gn-head_3x4_4x_kitti-mono3d.py b/configs/pgd/pgd_r101_caffe_fpn_gn-head_3x4_4x_kitti-mono3d.py
new file mode 100644
index 0000000..832b34e
--- /dev/null
+++ b/configs/pgd/pgd_r101_caffe_fpn_gn-head_3x4_4x_kitti-mono3d.py
@@ -0,0 +1,127 @@
+_base_ = [
+ '../_base_/datasets/kitti-mono3d.py', '../_base_/models/pgd.py',
+ '../_base_/schedules/mmdet_schedule_1x.py', '../_base_/default_runtime.py'
+]
+# model settings
+model = dict(
+ backbone=dict(frozen_stages=0),
+ neck=dict(start_level=0, num_outs=4),
+ bbox_head=dict(
+ num_classes=3,
+ bbox_code_size=7,
+ pred_attrs=False,
+ pred_velo=False,
+ pred_bbox2d=True,
+ use_onlyreg_proj=True,
+ strides=(4, 8, 16, 32),
+ regress_ranges=((-1, 64), (64, 128), (128, 256), (256, 1e8)),
+ group_reg_dims=(2, 1, 3, 1, 16,
+ 4), # offset, depth, size, rot, kpts, bbox2d
+ reg_branch=(
+ (256, ), # offset
+ (256, ), # depth
+ (256, ), # size
+ (256, ), # rot
+ (256, ), # kpts
+ (256, ) # bbox2d
+ ),
+ centerness_branch=(256, ),
+ loss_cls=dict(
+ type='FocalLoss',
+ use_sigmoid=True,
+ gamma=2.0,
+ alpha=0.25,
+ loss_weight=1.0),
+ loss_bbox=dict(type='SmoothL1Loss', beta=1.0 / 9.0, loss_weight=1.0),
+ loss_dir=dict(
+ type='CrossEntropyLoss', use_sigmoid=False, loss_weight=1.0),
+ loss_centerness=dict(
+ type='CrossEntropyLoss', use_sigmoid=True, loss_weight=1.0),
+ use_depth_classifier=True,
+ depth_branch=(256, ),
+ depth_range=(0, 70),
+ depth_unit=10,
+ division='uniform',
+ depth_bins=8,
+ pred_keypoints=True,
+ weight_dim=1,
+ loss_depth=dict(
+ type='UncertainSmoothL1Loss', alpha=1.0, beta=3.0,
+ loss_weight=1.0),
+ bbox_coder=dict(
+ type='PGDBBoxCoder',
+ base_depths=((28.01, 16.32), ),
+ base_dims=((0.8, 1.73, 0.6), (1.76, 1.73, 0.6), (3.9, 1.56, 1.6)),
+ code_size=7)),
+ # set weight 1.0 for base 7 dims (offset, depth, size, rot)
+ # 0.2 for 16-dim keypoint offsets and 1.0 for 4-dim 2D distance targets
+ train_cfg=dict(code_weight=[
+ 1.0, 1.0, 1.0, 1.0, 1.0, 1.0, 1.0, 0.2, 0.2, 0.2, 0.2, 0.2, 0.2, 0.2,
+ 0.2, 0.2, 0.2, 0.2, 0.2, 0.2, 0.2, 0.2, 0.2, 1.0, 1.0, 1.0, 1.0
+ ]),
+ test_cfg=dict(nms_pre=100, nms_thr=0.05, score_thr=0.001, max_per_img=20))
+
+class_names = ['Pedestrian', 'Cyclist', 'Car']
+img_norm_cfg = dict(
+ mean=[103.530, 116.280, 123.675], std=[1.0, 1.0, 1.0], to_rgb=False)
+train_pipeline = [
+ dict(type='LoadImageFromFileMono3D'),
+ dict(
+ type='LoadAnnotations3D',
+ with_bbox=True,
+ with_label=True,
+ with_attr_label=False,
+ with_bbox_3d=True,
+ with_label_3d=True,
+ with_bbox_depth=True),
+ dict(type='Resize', img_scale=(1242, 375), keep_ratio=True),
+ dict(type='RandomFlip3D', flip_ratio_bev_horizontal=0.5),
+ dict(type='Normalize', **img_norm_cfg),
+ dict(type='Pad', size_divisor=32),
+ dict(type='DefaultFormatBundle3D', class_names=class_names),
+ dict(
+ type='Collect3D',
+ keys=[
+ 'img', 'gt_bboxes', 'gt_labels', 'gt_bboxes_3d', 'gt_labels_3d',
+ 'centers2d', 'depths'
+ ]),
+]
+test_pipeline = [
+ dict(type='LoadImageFromFileMono3D'),
+ dict(
+ type='MultiScaleFlipAug',
+ scale_factor=1.0,
+ flip=False,
+ transforms=[
+ dict(type='RandomFlip3D'),
+ dict(type='Normalize', **img_norm_cfg),
+ dict(type='Pad', size_divisor=32),
+ dict(
+ type='DefaultFormatBundle3D',
+ class_names=class_names,
+ with_label=False),
+ dict(type='Collect3D', keys=['img']),
+ ])
+]
+data = dict(
+ samples_per_gpu=3,
+ workers_per_gpu=3,
+ train=dict(pipeline=train_pipeline),
+ val=dict(pipeline=test_pipeline),
+ test=dict(pipeline=test_pipeline))
+# optimizer
+optimizer = dict(
+ lr=0.001, paramwise_cfg=dict(bias_lr_mult=2., bias_decay_mult=0.))
+optimizer_config = dict(
+ _delete_=True, grad_clip=dict(max_norm=35, norm_type=2))
+# learning policy
+lr_config = dict(
+ policy='step',
+ warmup='linear',
+ warmup_iters=500,
+ warmup_ratio=1.0 / 3,
+ step=[32, 44])
+total_epochs = 48
+runner = dict(type='EpochBasedRunner', max_epochs=48)
+evaluation = dict(interval=2)
+checkpoint_config = dict(interval=8)
diff --git a/configs/point_rcnn/README.md b/configs/point_rcnn/README.md
new file mode 100644
index 0000000..eddbdc7
--- /dev/null
+++ b/configs/point_rcnn/README.md
@@ -0,0 +1,47 @@
+# PointRCNN: 3D Object Proposal Generation and Detection from Point Cloud
+
+> [PointRCNN: 3D Object Proposal Generation and Detection from Point Cloud](https://arxiv.org/abs/1812.04244)
+
+
+
+## Abstract
+
+In this paper, we propose PointRCNN for 3D object detection from raw point cloud. The whole framework is composed of two stages: stage-1 for the bottom-up 3D proposal generation and stage-2 for refining proposals in the canonical coordinates to obtain the final detection results. Instead of generating proposals from RGB image or projecting point cloud to bird's view or voxels as previous methods do, our stage-1 sub-network directly generates a small number of high-quality 3D proposals from point cloud in a bottom-up manner via segmenting the point cloud of the whole scene into foreground points and background. The stage-2 sub-network transforms the pooled points of each proposal to canonical coordinates to learn better local spatial features, which is combined with global semantic features of each point learned in stage-1 for accurate box refinement and confidence prediction. Extensive experiments on the 3D detection benchmark of KITTI dataset show that our proposed architecture outperforms state-of-the-art methods with remarkable margins by using only point cloud as input.
+
+
+
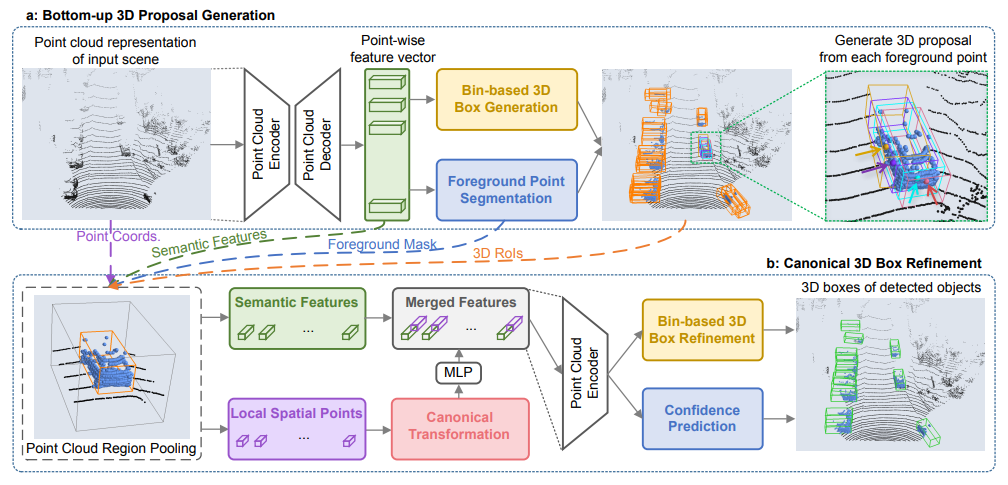
+
+
+## Introduction
+
+We implement PointRCNN and provide the result with checkpoints on KITTI dataset.
+
+## Results and models
+
+### KITTI
+
+| Backbone | Class | Lr schd | Mem (GB) | Inf time (fps) | mAP | Download |
+| :-------------------------------------------------: | :-----: | :--------: | :------: | :------------: | :---: | :---------------------------------------------------------------------------------------------------------------------------------------------------------------------------------------------------------------------------------------------------------------------------: |
+| [PointNet++](./point_rcnn_2x8_kitti-3d-3classes.py) | 3 Class | cyclic 40e | 4.6 | | 70.83 | [model](https://download.openmmlab.com/mmdetection3d/v1.0.0_models/point_rcnn/point_rcnn_2x8_kitti-3d-3classes_20211208_151344.pth) \| [log](https://download.openmmlab.com/mmdetection3d/v1.0.0_models/point_rcnn/point_rcnn_2x8_kitti-3d-3classes_20211208_151344.log.json) |
+
+Note: mAP represents AP11 results on 3 Class under the moderate setting.
+
+Detailed performance on KITTI 3D detection (3D) is as follows, evaluated by AP11 metric:
+
+| | Easy | Moderate | Hard |
+| ---------- | :---: | :------: | :---: |
+| Car | 89.13 | 78.72 | 78.24 |
+| Pedestrian | 65.81 | 59.57 | 52.75 |
+| Cyclist | 93.51 | 74.19 | 70.73 |
+
+## Citation
+
+```latex
+@inproceedings{Shi_2019_CVPR,
+ title = {PointRCNN: 3D Object Proposal Generation and Detection from Point Cloud},
+ author = {Shi, Shaoshuai and Wang, Xiaogang and Li, Hongsheng},
+ booktitle = {The IEEE Conference on Computer Vision and Pattern Recognition (CVPR)},
+ month = {June},
+ year = {2019}
+}
+```
diff --git a/configs/point_rcnn/metafile.yml b/configs/point_rcnn/metafile.yml
new file mode 100644
index 0000000..a7627ce
--- /dev/null
+++ b/configs/point_rcnn/metafile.yml
@@ -0,0 +1,29 @@
+Collections:
+ - Name: PointRCNN
+ Metadata:
+ Training Data: KITTI
+ Training Techniques:
+ - AdamW
+ Training Resources: 8x Titan XP GPUs
+ Architecture:
+ - PointNet++
+ Paper:
+ URL: https://arxiv.org/abs/1812.04244
+ Title: 'PointRCNN: 3D Object Proposal Generation and Detection from Point Cloud'
+ README: configs/point_rcnn/README.md
+ Code:
+ URL: https://github.com/open-mmlab/mmdetection3d/blob/v1.0.0.dev0/mmdet3d/models/detectors/point_rcnn.py#L8
+ Version: v1.0.0
+
+Models:
+ - Name: point_rcnn_2x8_kitti-3d-3classes.py
+ In Collection: PointRCNN
+ Config: configs/point_rcnn/point_rcnn_2x8_kitti-3d-3classes.py
+ Metadata:
+ Training Memory (GB): 4.6
+ Results:
+ - Task: 3D Object Detection
+ Dataset: KITTI
+ Metrics:
+ mAP: 70.83
+ Weights: https://download.openmmlab.com/mmdetection3d/v1.0.0_models/point_rcnn/point_rcnn_2x8_kitti-3d-3classes_20211208_151344.pth
diff --git a/configs/point_rcnn/point_rcnn_2x8_kitti-3d-3classes.py b/configs/point_rcnn/point_rcnn_2x8_kitti-3d-3classes.py
new file mode 100644
index 0000000..1344aca
--- /dev/null
+++ b/configs/point_rcnn/point_rcnn_2x8_kitti-3d-3classes.py
@@ -0,0 +1,94 @@
+_base_ = [
+ '../_base_/datasets/kitti-3d-car.py', '../_base_/models/point_rcnn.py',
+ '../_base_/default_runtime.py', '../_base_/schedules/cyclic_40e.py'
+]
+
+# dataset settings
+dataset_type = 'KittiDataset'
+data_root = 'data/kitti/'
+class_names = ['Car', 'Pedestrian', 'Cyclist']
+point_cloud_range = [0, -40, -3, 70.4, 40, 1]
+input_modality = dict(use_lidar=True, use_camera=False)
+
+db_sampler = dict(
+ data_root=data_root,
+ info_path=data_root + 'kitti_dbinfos_train.pkl',
+ rate=1.0,
+ prepare=dict(
+ filter_by_difficulty=[-1],
+ filter_by_min_points=dict(Car=5, Pedestrian=5, Cyclist=5)),
+ sample_groups=dict(Car=20, Pedestrian=15, Cyclist=15),
+ classes=class_names)
+
+train_pipeline = [
+ dict(type='LoadPointsFromFile', coord_type='LIDAR', load_dim=4, use_dim=4),
+ dict(type='LoadAnnotations3D', with_bbox_3d=True, with_label_3d=True),
+ dict(type='PointsRangeFilter', point_cloud_range=point_cloud_range),
+ dict(type='ObjectRangeFilter', point_cloud_range=point_cloud_range),
+ dict(type='ObjectSample', db_sampler=db_sampler),
+ dict(type='RandomFlip3D', flip_ratio_bev_horizontal=0.5),
+ dict(
+ type='ObjectNoise',
+ num_try=100,
+ translation_std=[1.0, 1.0, 0.5],
+ global_rot_range=[0.0, 0.0],
+ rot_range=[-0.78539816, 0.78539816]),
+ dict(
+ type='GlobalRotScaleTrans',
+ rot_range=[-0.78539816, 0.78539816],
+ scale_ratio_range=[0.95, 1.05]),
+ dict(type='PointsRangeFilter', point_cloud_range=point_cloud_range),
+ dict(type='PointSample', num_points=16384, sample_range=40.0),
+ dict(type='PointShuffle'),
+ dict(type='DefaultFormatBundle3D', class_names=class_names),
+ dict(type='Collect3D', keys=['points', 'gt_bboxes_3d', 'gt_labels_3d'])
+]
+test_pipeline = [
+ dict(type='LoadPointsFromFile', coord_type='LIDAR', load_dim=4, use_dim=4),
+ dict(
+ type='MultiScaleFlipAug3D',
+ img_scale=(1333, 800),
+ pts_scale_ratio=1,
+ flip=False,
+ transforms=[
+ dict(
+ type='GlobalRotScaleTrans',
+ rot_range=[0, 0],
+ scale_ratio_range=[1., 1.],
+ translation_std=[0, 0, 0]),
+ dict(type='RandomFlip3D'),
+ dict(
+ type='PointsRangeFilter', point_cloud_range=point_cloud_range),
+ dict(type='PointSample', num_points=16384, sample_range=40.0),
+ dict(
+ type='DefaultFormatBundle3D',
+ class_names=class_names,
+ with_label=False),
+ dict(type='Collect3D', keys=['points'])
+ ])
+]
+
+data = dict(
+ samples_per_gpu=2,
+ workers_per_gpu=2,
+ train=dict(
+ type='RepeatDataset',
+ times=2,
+ dataset=dict(pipeline=train_pipeline, classes=class_names)),
+ val=dict(pipeline=test_pipeline, classes=class_names),
+ test=dict(pipeline=test_pipeline, classes=class_names))
+
+# optimizer
+lr = 0.001 # max learning rate
+optimizer = dict(lr=lr, betas=(0.95, 0.85))
+# runtime settings
+runner = dict(type='EpochBasedRunner', max_epochs=80)
+evaluation = dict(interval=2)
+# yapf:disable
+log_config = dict(
+ interval=30,
+ hooks=[
+ dict(type='TextLoggerHook'),
+ dict(type='TensorboardLoggerHook')
+ ])
+# yapf:enable
diff --git a/configs/pointnet2/README.md b/configs/pointnet2/README.md
new file mode 100644
index 0000000..c9204eb
--- /dev/null
+++ b/configs/pointnet2/README.md
@@ -0,0 +1,72 @@
+# PointNet++: Deep Hierarchical Feature Learning on Point Sets in a Metric Space
+
+> [PointNet++: Deep Hierarchical Feature Learning on Point Sets in a Metric Space](https://arxiv.org/abs/1706.02413)
+
+
+
+## Abstract
+
+Few prior works study deep learning on point sets. PointNet by Qi et al. is a pioneer in this direction. However, by design PointNet does not capture local structures induced by the metric space points live in, limiting its ability to recognize fine-grained patterns and generalizability to complex scenes. In this work, we introduce a hierarchical neural network that applies PointNet recursively on a nested partitioning of the input point set. By exploiting metric space distances, our network is able to learn local features with increasing contextual scales. With further observation that point sets are usually sampled with varying densities, which results in greatly decreased performance for networks trained on uniform densities, we propose novel set learning layers to adaptively combine features from multiple scales. Experiments show that our network called PointNet++ is able to learn deep point set features efficiently and robustly. In particular, results significantly better than state-of-the-art have been obtained on challenging benchmarks of 3D point clouds.
+
+
+
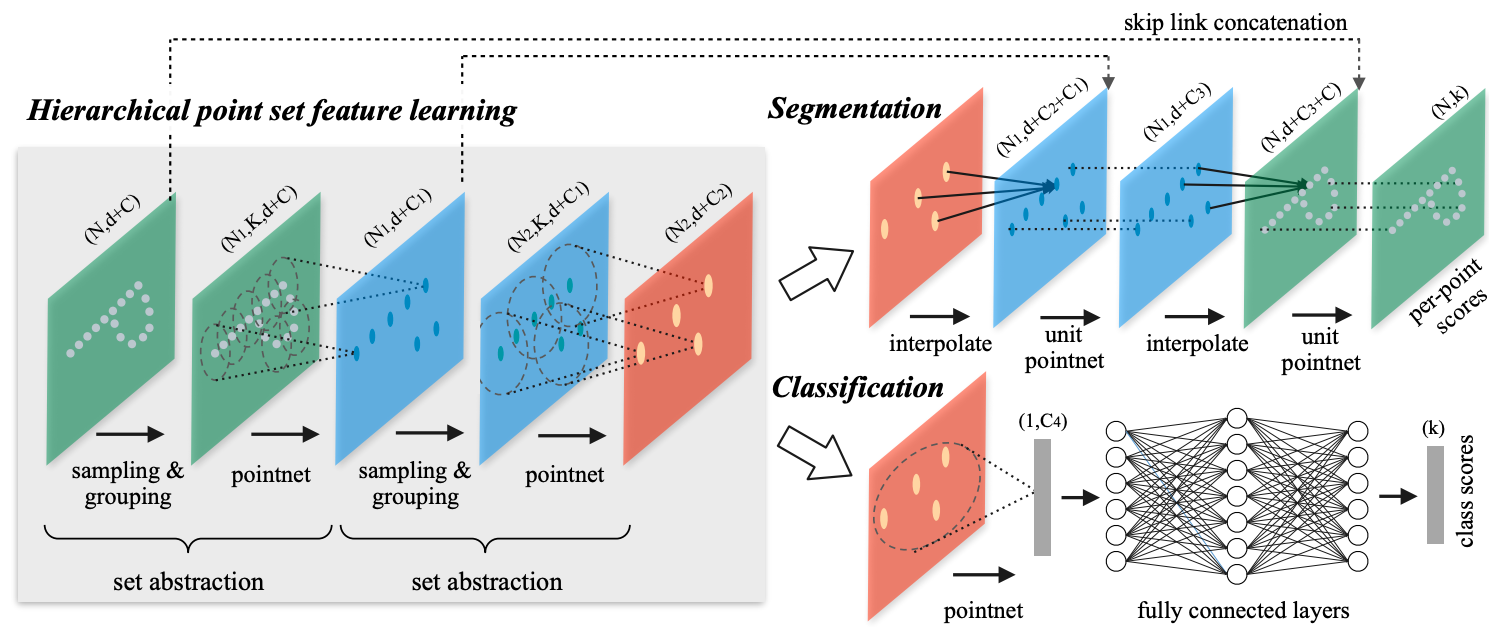
+
+
+## Introduction
+
+We implement PointNet++ and provide the result and checkpoints on ScanNet and S3DIS datasets.
+
+**Notice**: The original PointNet++ paper used step learning rate schedule. We discovered that cosine schedule achieves much better results and adopt it in our implementations. We also use a larger `weight_decay` factor because we find it consistently improves the performance.
+
+## Results and models
+
+### ScanNet
+
+| Method | Input | Lr schd | Mem (GB) | Inf time (fps) | mIoU (Val set) | mIoU (Test set) | Download |
+| :-------------------------------------------------------------------------------------: | :-------: | :---------: | :------: | :------------: | :------------: | :-------------: | ------------------------------------------------------------------------------------------------------------------------------------------------------------------------------------------------------------------------------------------------------------------------------------------------------------------------------------------------------------------------------------------------------------------------------------------------------------------------------ |
+| [PointNet++ (SSG)](./pointnet2_ssg_xyz-only_16x2_cosine_200e_scannet_seg-3d-20class.py) | XYZ | cosine 200e | 1.9 | | 53.91 | | [model](https://download.openmmlab.com/mmdetection3d/v0.1.0_models/pointnet2/pointnet2_ssg_xyz-only_16x2_cosine_200e_scannet_seg-3d-20class/pointnet2_ssg_xyz-only_16x2_cosine_200e_scannet_seg-3d-20class_20210514_143628-4e341a48.pth) \| [log](https://download.openmmlab.com/mmdetection3d/v0.1.0_models/pointnet2/pointnet2_ssg_xyz-only_16x2_cosine_200e_scannet_seg-3d-20class/pointnet2_ssg_xyz-only_16x2_cosine_200e_scannet_seg-3d-20class_20210514_143628.log.json) |
+| [PointNet++ (SSG)](./pointnet2_ssg_16x2_cosine_200e_scannet_seg-3d-20class.py) | XYZ+Color | cosine 200e | 1.9 | | 54.44 | | [model](https://download.openmmlab.com/mmdetection3d/v0.1.0_models/pointnet2/pointnet2_ssg_16x2_cosine_200e_scannet_seg-3d-20class/pointnet2_ssg_16x2_cosine_200e_scannet_seg-3d-20class_20210514_143644-ee73704a.pth) \| [log](https://download.openmmlab.com/mmdetection3d/v0.1.0_models/pointnet2/pointnet2_ssg_16x2_cosine_200e_scannet_seg-3d-20class/pointnet2_ssg_16x2_cosine_200e_scannet_seg-3d-20class_20210514_143644.log.json) |
+| [PointNet++ (MSG)](./pointnet2_msg_xyz-only_16x2_cosine_250e_scannet_seg-3d-20class.py) | XYZ | cosine 250e | 2.4 | | 54.26 | | [model](https://download.openmmlab.com/mmdetection3d/v0.1.0_models/pointnet2/pointnet2_msg_xyz-only_16x2_cosine_250e_scannet_seg-3d-20class/pointnet2_msg_xyz-only_16x2_cosine_250e_scannet_seg-3d-20class_20210514_143838-b4a3cf89.pth) \| [log](https://download.openmmlab.com/mmdetection3d/v0.1.0_models/pointnet2/pointnet2_msg_xyz-only_16x2_cosine_250e_scannet_seg-3d-20class/pointnet2_msg_xyz-only_16x2_cosine_250e_scannet_seg-3d-20class_20210514_143838.log.json) |
+| [PointNet++ (MSG)](./pointnet2_msg_16x2_cosine_250e_scannet_seg-3d-20class.py) | XYZ+Color | cosine 250e | 2.4 | | 55.05 | | [model](https://download.openmmlab.com/mmdetection3d/v0.1.0_models/pointnet2/pointnet2_msg_16x2_cosine_250e_scannet_seg-3d-20class/pointnet2_msg_16x2_cosine_250e_scannet_seg-3d-20class_20210514_144009-24477ab1.pth) \| [log](https://download.openmmlab.com/mmdetection3d/v0.1.0_models/pointnet2/pointnet2_msg_16x2_cosine_250e_scannet_seg-3d-20class/pointnet2_msg_16x2_cosine_250e_scannet_seg-3d-20class_20210514_144009.log.json) |
+
+**Notes:**
+
+- The original PointNet++ paper conducted experiments on the ScanNet V1 dataset, while later point cloud segmentor papers often used ScanNet V2. Following common practice, we report results on the ScanNet V2 dataset.
+
+- Since ScanNet dataset doesn't provide ground-truth labels for the test set, users can only evaluate test set performance by submitting to its online benchmark [website](http://kaldir.vc.in.tum.de/scannet_benchmark/). However, users are only allowed to submit once every two weeks. Therefore, we currently report val set mIoU. Test set performance may be added in the future.
+
+- To generate submission file for ScanNet online benchmark, you need to modify the ScanNet dataset's [config](https://github.com/open-mmlab/mmdetection3d/blob/master/configs/_base_/datasets/scannet_seg-3d-20class.py#L126). Change `ann_file=data_root + 'scannet_infos_val.pkl'` to `ann_file=data_root + 'scannet_infos_test.pkl'`, and then simply run:
+
+ ```shell
+ python tools/test.py ${CONFIG_FILE} ${CHECKPOINT_FILE} --format-only --options 'txt_prefix=exps/pointnet2_scannet_results'
+ ```
+
+ This will save the prediction results as `txt` files in `exps/pointnet2_scannet_results/`. Then, go to this folder and zip all files into `pn2_scannet.zip`. Now you can submit it to the online benchmark and wait for the test set result. More instructions can be found at their official [website](http://kaldir.vc.in.tum.de/scannet_benchmark/documentation#submission-policy).
+
+### S3DIS
+
+| Method | Split | Lr schd | Mem (GB) | Inf time (fps) | mIoU (Val set) | Download |
+| :-------------------------------------------------------------------------: | :----: | :--------: | :------: | :------------: | :------------: | :----------------------------------------------------------------------------------------------------------------------------------------------------------------------------------------------------------------------------------------------------------------------------------------------------------------------------------------------------------------------------------------------------------------------------: |
+| [PointNet++ (SSG)](./pointnet2_ssg_16x2_cosine_50e_s3dis_seg-3d-13class.py) | Area_5 | cosine 50e | 3.6 | | 56.93 | [model](https://download.openmmlab.com/mmdetection3d/v0.1.0_models/pointnet2/pointnet2_ssg_16x2_cosine_50e_s3dis_seg-3d-13class/pointnet2_ssg_16x2_cosine_50e_s3dis_seg-3d-13class_20210514_144205-995d0119.pth) \| [log](https://download.openmmlab.com/mmdetection3d/v0.1.0_models/pointnet2/pointnet2_ssg_16x2_cosine_50e_s3dis_seg-3d-13class/pointnet2_ssg_16x2_cosine_50e_s3dis_seg-3d-13class_20210514_144205.log.json) |
+| [PointNet++ (MSG)](./pointnet2_msg_16x2_cosine_80e_s3dis_seg-3d-13class.py) | Area_5 | cosine 80e | 3.6 | | 58.04 | [model](https://download.openmmlab.com/mmdetection3d/v0.1.0_models/pointnet2/pointnet2_msg_16x2_cosine_80e_s3dis_seg-3d-13class/pointnet2_msg_16x2_cosine_80e_s3dis_seg-3d-13class_20210514_144307-b2059817.pth) \| [log](https://download.openmmlab.com/mmdetection3d/v0.1.0_models/pointnet2/pointnet2_msg_16x2_cosine_80e_s3dis_seg-3d-13class/pointnet2_msg_16x2_cosine_80e_s3dis_seg-3d-13class_20210514_144307.log.json) |
+
+**Notes:**
+
+- We use XYZ+Color+Normalized_XYZ as input in all the experiments on S3DIS datasets.
+- `Area_5` Split means training the model on Area_1, 2, 3, 4, 6 and testing on Area_5.
+
+## Indeterminism
+
+Since PointNet++ testing adopts sliding patch inference which involves random point sampling, and the test script uses fixed random seeds while the random seeds of validation in training are not fixed, the test results may be slightly different from the results reported above.
+
+## Citation
+
+```latex
+@inproceedings{qi2017pointnet++,
+ title={PointNet++ deep hierarchical feature learning on point sets in a metric space},
+ author={Qi, Charles R and Yi, Li and Su, Hao and Guibas, Leonidas J},
+ booktitle={Proceedings of the 31st International Conference on Neural Information Processing Systems},
+ pages={5105--5114},
+ year={2017}
+}
+```
diff --git a/configs/pointnet2/metafile.yml b/configs/pointnet2/metafile.yml
new file mode 100644
index 0000000..e7e5175
--- /dev/null
+++ b/configs/pointnet2/metafile.yml
@@ -0,0 +1,94 @@
+Collections:
+ - Name: PointNet++
+ Metadata:
+ Training Techniques:
+ - Adam
+ Training Resources: 2x Titan XP GPUs
+ Architecture:
+ - PointNet++
+ Paper:
+ URL: https://arxiv.org/abs/1706.02413
+ Title: 'PointNet++: Deep Hierarchical Feature Learning on Point Sets in a Metric Space'
+ README: configs/pointnet2/README.md
+ Code:
+ URL: https://github.com/open-mmlab/mmdetection3d/blob/master/mmdet3d/models/backbones/pointnet2_sa_ssg.py#L12
+ Version: v0.14.0
+
+Models:
+ - Name: pointnet2_ssg_xyz-only_16x2_cosine_200e_scannet_seg-3d-20class.py
+ In Collection: PointNet++
+ Config: configs/pointnet/pointnet2_ssg_xyz-only_16x2_cosine_200e_scannet_seg-3d-20class.py
+ Metadata:
+ Training Data: ScanNet
+ Training Memory (GB): 1.9
+ Results:
+ - Task: 3D Semantic Segmentation
+ Dataset: ScanNet
+ Metrics:
+ mIoU: 53.91
+ Weights: https://download.openmmlab.com/mmdetection3d/v0.1.0_models/pointnet2/pointnet2_ssg_xyz-only_16x2_cosine_200e_scannet_seg-3d-20class/pointnet2_ssg_xyz-only_16x2_cosine_200e_scannet_seg-3d-20class_20210514_143628-4e341a48.pth
+
+ - Name: pointnet2_ssg_16x2_cosine_200e_scannet_seg-3d-20class.py
+ In Collection: PointNet++
+ Config: configs/pointnet/pointnet2_ssg_16x2_cosine_200e_scannet_seg-3d-20class.py
+ Metadata:
+ Training Data: ScanNet
+ Training Memory (GB): 1.9
+ Results:
+ - Task: 3D Semantic Segmentation
+ Dataset: ScanNet
+ Metrics:
+ mIoU: 54.44
+ Weights: https://download.openmmlab.com/mmdetection3d/v0.1.0_models/pointnet2/pointnet2_ssg_16x2_cosine_200e_scannet_seg-3d-20class/pointnet2_ssg_16x2_cosine_200e_scannet_seg-3d-20class_20210514_143644-ee73704a.pth
+
+ - Name: pointnet2_msg_xyz-only_16x2_cosine_250e_scannet_seg-3d-20class.py
+ In Collection: PointNet++
+ Config: configs/pointnet/pointnet2_msg_xyz-only_16x2_cosine_250e_scannet_seg-3d-20class.py
+ Metadata:
+ Training Data: ScanNet
+ Training Memory (GB): 2.4
+ Results:
+ - Task: 3D Semantic Segmentation
+ Dataset: ScanNet
+ Metrics:
+ mIoU: 54.26
+ Weights: https://download.openmmlab.com/mmdetection3d/v0.1.0_models/pointnet2/pointnet2_msg_xyz-only_16x2_cosine_250e_scannet_seg-3d-20class/pointnet2_msg_xyz-only_16x2_cosine_250e_scannet_seg-3d-20class_20210514_143838-b4a3cf89.pth
+
+ - Name: pointnet2_msg_16x2_cosine_250e_scannet_seg-3d-20class.py
+ In Collection: PointNet++
+ Config: configs/pointnet/pointnet2_msg_16x2_cosine_250e_scannet_seg-3d-20class.py
+ Metadata:
+ Training Data: ScanNet
+ Training Memory (GB): 2.4
+ Results:
+ - Task: 3D Semantic Segmentation
+ Dataset: ScanNet
+ Metrics:
+ mIoU: 55.05
+ Weights: https://download.openmmlab.com/mmdetection3d/v0.1.0_models/pointnet2/pointnet2_msg_16x2_cosine_250e_scannet_seg-3d-20class/pointnet2_msg_16x2_cosine_250e_scannet_seg-3d-20class_20210514_144009-24477ab1.pth
+
+ - Name: pointnet2_ssg_16x2_cosine_50e_s3dis_seg-3d-13class.py
+ In Collection: PointNet++
+ Config: configs/pointnet/pointnet2_ssg_16x2_cosine_50e_s3dis_seg-3d-13class.py
+ Metadata:
+ Training Data: S3DIS
+ Training Memory (GB): 3.6
+ Results:
+ - Task: 3D Semantic Segmentation
+ Dataset: S3DIS
+ Metrics:
+ mIoU: 56.93
+ Weights: https://download.openmmlab.com/mmdetection3d/v0.1.0_models/pointnet2/pointnet2_ssg_16x2_cosine_50e_s3dis_seg-3d-13class/pointnet2_ssg_16x2_cosine_50e_s3dis_seg-3d-13class_20210514_144205-995d0119.pth
+
+ - Name: pointnet2_msg_16x2_cosine_80e_s3dis_seg-3d-13class.py
+ In Collection: PointNet++
+ Config: configs/pointnet/pointnet2_msg_16x2_cosine_80e_s3dis_seg-3d-13class.py
+ Metadata:
+ Training Data: S3DIS
+ Training Memory (GB): 3.6
+ Results:
+ - Task: 3D Semantic Segmentation
+ Dataset: S3DIS
+ Metrics:
+ mIoU: 58.04
+ Weights: https://download.openmmlab.com/mmdetection3d/v0.1.0_models/pointnet2/pointnet2_msg_16x2_cosine_80e_s3dis_seg-3d-13class/pointnet2_msg_16x2_cosine_80e_s3dis_seg-3d-13class_20210514_144307-b2059817.pth
diff --git a/configs/pointnet2/pointnet2_msg_16x2_cosine_250e_scannet_seg-3d-20class.py b/configs/pointnet2/pointnet2_msg_16x2_cosine_250e_scannet_seg-3d-20class.py
new file mode 100644
index 0000000..fbad158
--- /dev/null
+++ b/configs/pointnet2/pointnet2_msg_16x2_cosine_250e_scannet_seg-3d-20class.py
@@ -0,0 +1,36 @@
+_base_ = [
+ '../_base_/datasets/scannet_seg-3d-20class.py',
+ '../_base_/models/pointnet2_msg.py',
+ '../_base_/schedules/seg_cosine_200e.py', '../_base_/default_runtime.py'
+]
+
+# data settings
+data = dict(samples_per_gpu=16)
+evaluation = dict(interval=5)
+
+# model settings
+model = dict(
+ decode_head=dict(
+ num_classes=20,
+ ignore_index=20,
+ # `class_weight` is generated in data pre-processing, saved in
+ # `data/scannet/seg_info/train_label_weight.npy`
+ # you can copy paste the values here, or input the file path as
+ # `class_weight=data/scannet/seg_info/train_label_weight.npy`
+ loss_decode=dict(class_weight=[
+ 2.389689, 2.7215734, 4.5944676, 4.8543367, 4.096086, 4.907941,
+ 4.690836, 4.512031, 4.623311, 4.9242644, 5.358117, 5.360071,
+ 5.019636, 4.967126, 5.3502126, 5.4023647, 5.4027233, 5.4169416,
+ 5.3954206, 4.6971426
+ ])),
+ test_cfg=dict(
+ num_points=8192,
+ block_size=1.5,
+ sample_rate=0.5,
+ use_normalized_coord=False,
+ batch_size=24))
+
+# runtime settings
+checkpoint_config = dict(interval=5)
+# PointNet2-MSG needs longer training time than PointNet2-SSG
+runner = dict(type='EpochBasedRunner', max_epochs=250)
diff --git a/configs/pointnet2/pointnet2_msg_16x2_cosine_80e_s3dis_seg-3d-13class.py b/configs/pointnet2/pointnet2_msg_16x2_cosine_80e_s3dis_seg-3d-13class.py
new file mode 100644
index 0000000..ed1e3c4
--- /dev/null
+++ b/configs/pointnet2/pointnet2_msg_16x2_cosine_80e_s3dis_seg-3d-13class.py
@@ -0,0 +1,27 @@
+_base_ = [
+ '../_base_/datasets/s3dis_seg-3d-13class.py',
+ '../_base_/models/pointnet2_msg.py',
+ '../_base_/schedules/seg_cosine_50e.py', '../_base_/default_runtime.py'
+]
+
+# data settings
+data = dict(samples_per_gpu=16)
+evaluation = dict(interval=2)
+
+# model settings
+model = dict(
+ backbone=dict(in_channels=9), # [xyz, rgb, normalized_xyz]
+ decode_head=dict(
+ num_classes=13, ignore_index=13,
+ loss_decode=dict(class_weight=None)), # S3DIS doesn't use class_weight
+ test_cfg=dict(
+ num_points=4096,
+ block_size=1.0,
+ sample_rate=0.5,
+ use_normalized_coord=True,
+ batch_size=24))
+
+# runtime settings
+checkpoint_config = dict(interval=2)
+# PointNet2-MSG needs longer training time than PointNet2-SSG
+runner = dict(type='EpochBasedRunner', max_epochs=80)
diff --git a/configs/pointnet2/pointnet2_msg_xyz-only_16x2_cosine_250e_scannet_seg-3d-20class.py b/configs/pointnet2/pointnet2_msg_xyz-only_16x2_cosine_250e_scannet_seg-3d-20class.py
new file mode 100644
index 0000000..2cb7ee1
--- /dev/null
+++ b/configs/pointnet2/pointnet2_msg_xyz-only_16x2_cosine_250e_scannet_seg-3d-20class.py
@@ -0,0 +1,166 @@
+_base_ = [
+ '../_base_/datasets/scannet_seg-3d-20class.py',
+ '../_base_/models/pointnet2_msg.py',
+ '../_base_/schedules/seg_cosine_200e.py', '../_base_/default_runtime.py'
+]
+
+# dataset settings
+# in this setting, we only use xyz as network input
+# so we need to re-write all the data pipeline
+dataset_type = 'ScanNetSegDataset'
+data_root = './data/scannet/'
+class_names = ('wall', 'floor', 'cabinet', 'bed', 'chair', 'sofa', 'table',
+ 'door', 'window', 'bookshelf', 'picture', 'counter', 'desk',
+ 'curtain', 'refrigerator', 'showercurtrain', 'toilet', 'sink',
+ 'bathtub', 'otherfurniture')
+num_points = 8192
+train_pipeline = [
+ dict(
+ type='LoadPointsFromFile',
+ coord_type='DEPTH',
+ shift_height=False,
+ use_color=False,
+ load_dim=6,
+ use_dim=[0, 1, 2]), # only load xyz coordinates
+ dict(
+ type='LoadAnnotations3D',
+ with_bbox_3d=False,
+ with_label_3d=False,
+ with_mask_3d=False,
+ with_seg_3d=True),
+ dict(
+ type='PointSegClassMapping',
+ valid_cat_ids=(1, 2, 3, 4, 5, 6, 7, 8, 9, 10, 11, 12, 14, 16, 24, 28,
+ 33, 34, 36, 39),
+ max_cat_id=40),
+ dict(
+ type='IndoorPatchPointSample',
+ num_points=num_points,
+ block_size=1.5,
+ ignore_index=len(class_names),
+ use_normalized_coord=False,
+ enlarge_size=0.2,
+ min_unique_num=None),
+ dict(type='DefaultFormatBundle3D', class_names=class_names),
+ dict(type='Collect3D', keys=['points', 'pts_semantic_mask'])
+]
+test_pipeline = [
+ dict(
+ type='LoadPointsFromFile',
+ coord_type='DEPTH',
+ shift_height=False,
+ use_color=False,
+ load_dim=6,
+ use_dim=[0, 1, 2]),
+ dict(
+ # a wrapper in order to successfully call test function
+ # actually we don't perform test-time-aug
+ type='MultiScaleFlipAug3D',
+ img_scale=(1333, 800),
+ pts_scale_ratio=1,
+ flip=False,
+ transforms=[
+ dict(
+ type='GlobalRotScaleTrans',
+ rot_range=[0, 0],
+ scale_ratio_range=[1., 1.],
+ translation_std=[0, 0, 0]),
+ dict(
+ type='RandomFlip3D',
+ sync_2d=False,
+ flip_ratio_bev_horizontal=0.0,
+ flip_ratio_bev_vertical=0.0),
+ dict(
+ type='DefaultFormatBundle3D',
+ class_names=class_names,
+ with_label=False),
+ dict(type='Collect3D', keys=['points'])
+ ])
+]
+# construct a pipeline for data and gt loading in show function
+# please keep its loading function consistent with test_pipeline (e.g. client)
+# we need to load gt seg_mask!
+eval_pipeline = [
+ dict(
+ type='LoadPointsFromFile',
+ coord_type='DEPTH',
+ shift_height=False,
+ use_color=False,
+ load_dim=6,
+ use_dim=[0, 1, 2]),
+ dict(
+ type='LoadAnnotations3D',
+ with_bbox_3d=False,
+ with_label_3d=False,
+ with_mask_3d=False,
+ with_seg_3d=True),
+ dict(
+ type='PointSegClassMapping',
+ valid_cat_ids=(1, 2, 3, 4, 5, 6, 7, 8, 9, 10, 11, 12, 14, 16, 24, 28,
+ 33, 34, 36, 39),
+ max_cat_id=40),
+ dict(
+ type='DefaultFormatBundle3D',
+ with_label=False,
+ class_names=class_names),
+ dict(type='Collect3D', keys=['points', 'pts_semantic_mask'])
+]
+
+data = dict(
+ samples_per_gpu=16,
+ workers_per_gpu=4,
+ train=dict(
+ type=dataset_type,
+ data_root=data_root,
+ ann_file=data_root + 'scannet_infos_train.pkl',
+ pipeline=train_pipeline,
+ classes=class_names,
+ test_mode=False,
+ ignore_index=len(class_names),
+ scene_idxs=data_root + 'seg_info/train_resampled_scene_idxs.npy'),
+ val=dict(
+ type=dataset_type,
+ data_root=data_root,
+ ann_file=data_root + 'scannet_infos_val.pkl',
+ pipeline=test_pipeline,
+ classes=class_names,
+ test_mode=True,
+ ignore_index=len(class_names)),
+ test=dict(
+ type=dataset_type,
+ data_root=data_root,
+ ann_file=data_root + 'scannet_infos_val.pkl',
+ pipeline=test_pipeline,
+ classes=class_names,
+ test_mode=True,
+ ignore_index=len(class_names)))
+
+evaluation = dict(pipeline=eval_pipeline, interval=5)
+
+# model settings
+model = dict(
+ backbone=dict(in_channels=3), # only [xyz]
+ decode_head=dict(
+ num_classes=20,
+ ignore_index=20,
+ # `class_weight` is generated in data pre-processing, saved in
+ # `data/scannet/seg_info/train_label_weight.npy`
+ # you can copy paste the values here, or input the file path as
+ # `class_weight=data/scannet/seg_info/train_label_weight.npy`
+ loss_decode=dict(class_weight=[
+ 2.389689, 2.7215734, 4.5944676, 4.8543367, 4.096086, 4.907941,
+ 4.690836, 4.512031, 4.623311, 4.9242644, 5.358117, 5.360071,
+ 5.019636, 4.967126, 5.3502126, 5.4023647, 5.4027233, 5.4169416,
+ 5.3954206, 4.6971426
+ ])),
+ test_cfg=dict(
+ num_points=8192,
+ block_size=1.5,
+ sample_rate=0.5,
+ use_normalized_coord=False,
+ batch_size=24))
+
+# runtime settings
+checkpoint_config = dict(interval=5)
+# PointNet2-MSG needs longer training time than PointNet2-SSG
+runner = dict(type='EpochBasedRunner', max_epochs=250)
diff --git a/configs/pointnet2/pointnet2_ssg_16x2_cosine_200e_scannet_seg-3d-20class.py b/configs/pointnet2/pointnet2_ssg_16x2_cosine_200e_scannet_seg-3d-20class.py
new file mode 100644
index 0000000..b526107
--- /dev/null
+++ b/configs/pointnet2/pointnet2_ssg_16x2_cosine_200e_scannet_seg-3d-20class.py
@@ -0,0 +1,34 @@
+_base_ = [
+ '../_base_/datasets/scannet_seg-3d-20class.py',
+ '../_base_/models/pointnet2_ssg.py',
+ '../_base_/schedules/seg_cosine_200e.py', '../_base_/default_runtime.py'
+]
+
+# data settings
+data = dict(samples_per_gpu=16)
+evaluation = dict(interval=5)
+
+# model settings
+model = dict(
+ decode_head=dict(
+ num_classes=20,
+ ignore_index=20,
+ # `class_weight` is generated in data pre-processing, saved in
+ # `data/scannet/seg_info/train_label_weight.npy`
+ # you can copy paste the values here, or input the file path as
+ # `class_weight=data/scannet/seg_info/train_label_weight.npy`
+ loss_decode=dict(class_weight=[
+ 2.389689, 2.7215734, 4.5944676, 4.8543367, 4.096086, 4.907941,
+ 4.690836, 4.512031, 4.623311, 4.9242644, 5.358117, 5.360071,
+ 5.019636, 4.967126, 5.3502126, 5.4023647, 5.4027233, 5.4169416,
+ 5.3954206, 4.6971426
+ ])),
+ test_cfg=dict(
+ num_points=8192,
+ block_size=1.5,
+ sample_rate=0.5,
+ use_normalized_coord=False,
+ batch_size=24))
+
+# runtime settings
+checkpoint_config = dict(interval=5)
diff --git a/configs/pointnet2/pointnet2_ssg_16x2_cosine_50e_s3dis_seg-3d-13class.py b/configs/pointnet2/pointnet2_ssg_16x2_cosine_50e_s3dis_seg-3d-13class.py
new file mode 100644
index 0000000..b14100d
--- /dev/null
+++ b/configs/pointnet2/pointnet2_ssg_16x2_cosine_50e_s3dis_seg-3d-13class.py
@@ -0,0 +1,25 @@
+_base_ = [
+ '../_base_/datasets/s3dis_seg-3d-13class.py',
+ '../_base_/models/pointnet2_ssg.py',
+ '../_base_/schedules/seg_cosine_50e.py', '../_base_/default_runtime.py'
+]
+
+# data settings
+data = dict(samples_per_gpu=16)
+evaluation = dict(interval=2)
+
+# model settings
+model = dict(
+ backbone=dict(in_channels=9), # [xyz, rgb, normalized_xyz]
+ decode_head=dict(
+ num_classes=13, ignore_index=13,
+ loss_decode=dict(class_weight=None)), # S3DIS doesn't use class_weight
+ test_cfg=dict(
+ num_points=4096,
+ block_size=1.0,
+ sample_rate=0.5,
+ use_normalized_coord=True,
+ batch_size=24))
+
+# runtime settings
+checkpoint_config = dict(interval=2)
diff --git a/configs/pointnet2/pointnet2_ssg_xyz-only_16x2_cosine_200e_scannet_seg-3d-20class.py b/configs/pointnet2/pointnet2_ssg_xyz-only_16x2_cosine_200e_scannet_seg-3d-20class.py
new file mode 100644
index 0000000..9dff449
--- /dev/null
+++ b/configs/pointnet2/pointnet2_ssg_xyz-only_16x2_cosine_200e_scannet_seg-3d-20class.py
@@ -0,0 +1,164 @@
+_base_ = [
+ '../_base_/datasets/scannet_seg-3d-20class.py',
+ '../_base_/models/pointnet2_ssg.py',
+ '../_base_/schedules/seg_cosine_200e.py', '../_base_/default_runtime.py'
+]
+
+# dataset settings
+# in this setting, we only use xyz as network input
+# so we need to re-write all the data pipeline
+dataset_type = 'ScanNetSegDataset'
+data_root = './data/scannet/'
+class_names = ('wall', 'floor', 'cabinet', 'bed', 'chair', 'sofa', 'table',
+ 'door', 'window', 'bookshelf', 'picture', 'counter', 'desk',
+ 'curtain', 'refrigerator', 'showercurtrain', 'toilet', 'sink',
+ 'bathtub', 'otherfurniture')
+num_points = 8192
+train_pipeline = [
+ dict(
+ type='LoadPointsFromFile',
+ coord_type='DEPTH',
+ shift_height=False,
+ use_color=False,
+ load_dim=6,
+ use_dim=[0, 1, 2]), # only load xyz coordinates
+ dict(
+ type='LoadAnnotations3D',
+ with_bbox_3d=False,
+ with_label_3d=False,
+ with_mask_3d=False,
+ with_seg_3d=True),
+ dict(
+ type='PointSegClassMapping',
+ valid_cat_ids=(1, 2, 3, 4, 5, 6, 7, 8, 9, 10, 11, 12, 14, 16, 24, 28,
+ 33, 34, 36, 39),
+ max_cat_id=40),
+ dict(
+ type='IndoorPatchPointSample',
+ num_points=num_points,
+ block_size=1.5,
+ ignore_index=len(class_names),
+ use_normalized_coord=False,
+ enlarge_size=0.2,
+ min_unique_num=None),
+ dict(type='DefaultFormatBundle3D', class_names=class_names),
+ dict(type='Collect3D', keys=['points', 'pts_semantic_mask'])
+]
+test_pipeline = [
+ dict(
+ type='LoadPointsFromFile',
+ coord_type='DEPTH',
+ shift_height=False,
+ use_color=False,
+ load_dim=6,
+ use_dim=[0, 1, 2]),
+ dict(
+ # a wrapper in order to successfully call test function
+ # actually we don't perform test-time-aug
+ type='MultiScaleFlipAug3D',
+ img_scale=(1333, 800),
+ pts_scale_ratio=1,
+ flip=False,
+ transforms=[
+ dict(
+ type='GlobalRotScaleTrans',
+ rot_range=[0, 0],
+ scale_ratio_range=[1., 1.],
+ translation_std=[0, 0, 0]),
+ dict(
+ type='RandomFlip3D',
+ sync_2d=False,
+ flip_ratio_bev_horizontal=0.0,
+ flip_ratio_bev_vertical=0.0),
+ dict(
+ type='DefaultFormatBundle3D',
+ class_names=class_names,
+ with_label=False),
+ dict(type='Collect3D', keys=['points'])
+ ])
+]
+# construct a pipeline for data and gt loading in show function
+# please keep its loading function consistent with test_pipeline (e.g. client)
+# we need to load gt seg_mask!
+eval_pipeline = [
+ dict(
+ type='LoadPointsFromFile',
+ coord_type='DEPTH',
+ shift_height=False,
+ use_color=False,
+ load_dim=6,
+ use_dim=[0, 1, 2]),
+ dict(
+ type='LoadAnnotations3D',
+ with_bbox_3d=False,
+ with_label_3d=False,
+ with_mask_3d=False,
+ with_seg_3d=True),
+ dict(
+ type='PointSegClassMapping',
+ valid_cat_ids=(1, 2, 3, 4, 5, 6, 7, 8, 9, 10, 11, 12, 14, 16, 24, 28,
+ 33, 34, 36, 39),
+ max_cat_id=40),
+ dict(
+ type='DefaultFormatBundle3D',
+ with_label=False,
+ class_names=class_names),
+ dict(type='Collect3D', keys=['points', 'pts_semantic_mask'])
+]
+
+data = dict(
+ samples_per_gpu=16,
+ workers_per_gpu=4,
+ train=dict(
+ type=dataset_type,
+ data_root=data_root,
+ ann_file=data_root + 'scannet_infos_train.pkl',
+ pipeline=train_pipeline,
+ classes=class_names,
+ test_mode=False,
+ ignore_index=len(class_names),
+ scene_idxs=data_root + 'seg_info/train_resampled_scene_idxs.npy'),
+ val=dict(
+ type=dataset_type,
+ data_root=data_root,
+ ann_file=data_root + 'scannet_infos_val.pkl',
+ pipeline=test_pipeline,
+ classes=class_names,
+ test_mode=True,
+ ignore_index=len(class_names)),
+ test=dict(
+ type=dataset_type,
+ data_root=data_root,
+ ann_file=data_root + 'scannet_infos_val.pkl',
+ pipeline=test_pipeline,
+ classes=class_names,
+ test_mode=True,
+ ignore_index=len(class_names)))
+
+evaluation = dict(pipeline=eval_pipeline, interval=5)
+
+# model settings
+model = dict(
+ backbone=dict(in_channels=3), # only [xyz]
+ decode_head=dict(
+ num_classes=20,
+ ignore_index=20,
+ # `class_weight` is generated in data pre-processing, saved in
+ # `data/scannet/seg_info/train_label_weight.npy`
+ # you can copy paste the values here, or input the file path as
+ # `class_weight=data/scannet/seg_info/train_label_weight.npy`
+ loss_decode=dict(class_weight=[
+ 2.389689, 2.7215734, 4.5944676, 4.8543367, 4.096086, 4.907941,
+ 4.690836, 4.512031, 4.623311, 4.9242644, 5.358117, 5.360071,
+ 5.019636, 4.967126, 5.3502126, 5.4023647, 5.4027233, 5.4169416,
+ 5.3954206, 4.6971426
+ ])),
+ test_cfg=dict(
+ num_points=8192,
+ block_size=1.5,
+ sample_rate=0.5,
+ use_normalized_coord=False,
+ batch_size=24))
+
+# runtime settings
+checkpoint_config = dict(interval=5)
diff --git a/configs/pointpillars/README.md b/configs/pointpillars/README.md
new file mode 100644
index 0000000..6209097
--- /dev/null
+++ b/configs/pointpillars/README.md
@@ -0,0 +1,78 @@
+# PointPillars: Fast Encoders for Object Detection from Point Clouds
+
+> [PointPillars: Fast Encoders for Object Detection from Point Clouds](https://arxiv.org/abs/1812.05784)
+
+
+
+## Abstract
+
+Object detection in point clouds is an important aspect of many robotics applications such as autonomous driving. In this paper we consider the problem of encoding a point cloud into a format appropriate for a downstream detection pipeline. Recent literature suggests two types of encoders; fixed encoders tend to be fast but sacrifice accuracy, while encoders that are learned from data are more accurate, but slower. In this work we propose PointPillars, a novel encoder which utilizes PointNets to learn a representation of point clouds organized in vertical columns (pillars). While the encoded features can be used with any standard 2D convolutional detection architecture, we further propose a lean downstream network. Extensive experimentation shows that PointPillars outperforms previous encoders with respect to both speed and accuracy by a large margin. Despite only using lidar, our full detection pipeline significantly outperforms the state of the art, even among fusion methods, with respect to both the 3D and bird's eye view KITTI benchmarks. This detection performance is achieved while running at 62 Hz: a 2 - 4 fold runtime improvement. A faster version of our method matches the state of the art at 105 Hz. These benchmarks suggest that PointPillars is an appropriate encoding for object detection in point clouds.
+
+
+
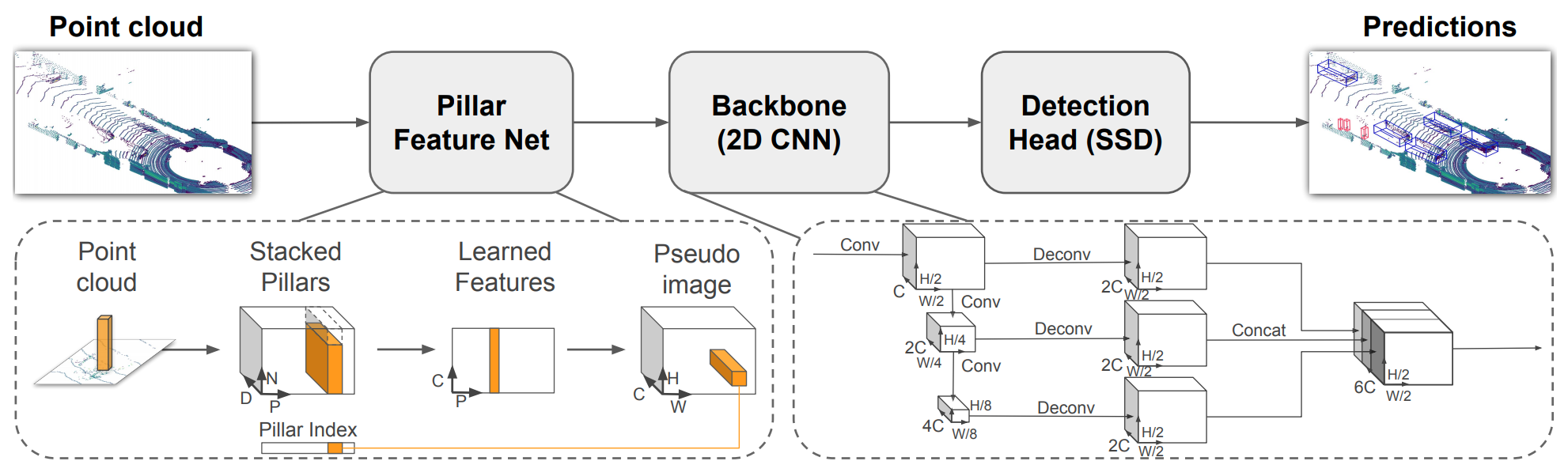
+
+
+## Introduction
+
+We implement PointPillars and provide the results and checkpoints on KITTI, nuScenes, Lyft and Waymo datasets.
+
+## Results and models
+
+### KITTI
+
+| Backbone | Class | Lr schd | Mem (GB) | Inf time (fps) | AP | Download |
+| :------------------------------------------------------------: | :-----: | :---------: | :------: | :------------: | :---: | :----------------------------------------------------------------------------------------------------------------------------------------------------------------------------------------------------------------------------------------------------------------------------------------------------------------------------------------------------------------------------------------------------------------------: |
+| [SECFPN](./hv_pointpillars_secfpn_6x8_160e_kitti-3d-car.py) | Car | cyclic 160e | 5.4 | | 77.6 | [model](https://download.openmmlab.com/mmdetection3d/v1.0.0_models/pointpillars/hv_pointpillars_secfpn_6x8_160e_kitti-3d-car/hv_pointpillars_secfpn_6x8_160e_kitti-3d-car_20220331_134606-d42d15ed.pth) \| [log](https://download.openmmlab.com/mmdetection3d/v1.0.0_models/pointpillars/hv_pointpillars_secfpn_6x8_160e_kitti-3d-car/hv_pointpillars_secfpn_6x8_160e_kitti-3d-car_20220331_134606.log.json) |
+| [SECFPN](./hv_pointpillars_secfpn_6x8_160e_kitti-3d-3class.py) | 3 Class | cyclic 160e | 5.5 | | 64.07 | [model](https://download.openmmlab.com/mmdetection3d/v1.0.0_models/pointpillars/hv_pointpillars_secfpn_6x8_160e_kitti-3d-3class/hv_pointpillars_secfpn_6x8_160e_kitti-3d-3class_20220301_150306-37dc2420.pth) \| [log](https://download.openmmlab.com/mmdetection3d/v1.0.0_models/pointpillars/hv_pointpillars_secfpn_6x8_160e_kitti-3d-3class/hv_pointpillars_secfpn_6x8_160e_kitti-3d-3class_20220301_150306.log.json) |
+
+### nuScenes
+
+| Backbone | Lr schd | Mem (GB) | Inf time (fps) | mAP | NDS | Download |
+| :---------------------------------------------------------------------: | :-----: | :------: | :------------: | :---: | :---: | :--------------------------------------------------------------------------------------------------------------------------------------------------------------------------------------------------------------------------------------------------------------------------------------------------------------------------------------------------------------------------------------------------------------: |
+| [SECFPN](./hv_pointpillars_secfpn_sbn-all_4x8_2x_nus-3d.py) | 2x | 16.4 | | 34.33 | 49.1 | [model](https://download.openmmlab.com/mmdetection3d/v1.0.0_models/pointpillars/hv_pointpillars_secfpn_sbn-all_4x8_2x_nus-3d/hv_pointpillars_secfpn_sbn-all_4x8_2x_nus-3d_20210826_225857-f19d00a3.pth) \| [log](https://download.openmmlab.com/mmdetection3d/v1.0.0_models/pointpillars/hv_pointpillars_secfpn_sbn-all_4x8_2x_nus-3d/hv_pointpillars_secfpn_sbn-all_4x8_2x_nus-3d_20210826_225857.log.json) |
+| [SECFPN (FP16)](./hv_pointpillars_secfpn_sbn-all_fp16_2x8_2x_nus-3d.py) | 2x | 8.37 | | 35.19 | 50.27 | [model](https://download.openmmlab.com/mmdetection3d/v0.1.0_models/fp16/hv_pointpillars_secfpn_sbn-all_fp16_2x8_2x_nus-3d/hv_pointpillars_secfpn_sbn-all_fp16_2x8_2x_nus-3d_20201020_222626-c3f0483e.pth) \| [log](https://download.openmmlab.com/mmdetection3d/v0.1.0_models/fp16/hv_pointpillars_secfpn_sbn-all_fp16_2x8_2x_nus-3d/hv_pointpillars_secfpn_sbn-all_fp16_2x8_2x_nus-3d_20201020_222626.log.json) |
+| [FPN](./hv_pointpillars_fpn_sbn-all_4x8_2x_nus-3d.py) | 2x | 16.3 | | 39.7 | 53.2 | [model](https://download.openmmlab.com/mmdetection3d/v1.0.0_models/pointpillars/hv_pointpillars_fpn_sbn-all_4x8_2x_nus-3d/hv_pointpillars_fpn_sbn-all_4x8_2x_nus-3d_20210826_104936-fca299c1.pth) \| [log](https://download.openmmlab.com/mmdetection3d/v1.0.0_models/pointpillars/hv_pointpillars_fpn_sbn-all_4x8_2x_nus-3d/hv_pointpillars_fpn_sbn-all_4x8_2x_nus-3d_20210826_104936.log.json) |
+| [FPN (FP16)](./hv_pointpillars_fpn_sbn-all_fp16_2x8_2x_nus-3d.py) | 2x | 8.40 | | 39.26 | 53.26 | [model](https://download.openmmlab.com/mmdetection3d/v0.1.0_models/fp16/hv_pointpillars_fpn_sbn-all_fp16_2x8_2x_nus-3d/hv_pointpillars_fpn_sbn-all_fp16_2x8_2x_nus-3d_20201021_120719-269f9dd6.pth) \| [log](https://download.openmmlab.com/mmdetection3d/v0.1.0_models/fp16/hv_pointpillars_fpn_sbn-all_fp16_2x8_2x_nus-3d/hv_pointpillars_fpn_sbn-all_fp16_2x8_2x_nus-3d_20201021_120719.log.json) |
+
+### Lyft
+
+| Backbone | Lr schd | Mem (GB) | Inf time (fps) | Private Score | Public Score | Download |
+| :----------------------------------------------------------: | :-----: | :------: | :------------: | :-----------: | :----------: | :--------------------------------------------------------------------------------------------------------------------------------------------------------------------------------------------------------------------------------------------------------------------------------------------------------------------------------------------------------------------------------------------------------------: |
+| [SECFPN](./hv_pointpillars_secfpn_sbn-all_2x8_2x_lyft-3d.py) | 2x | 12.2 | | 13.8 | 14.1 | [model](https://download.openmmlab.com/mmdetection3d/v1.0.0_models/pointpillars/hv_pointpillars_secfpn_sbn-all_2x8_2x_lyft-3d/hv_pointpillars_secfpn_sbn-all_2x8_2x_lyft-3d_20210829_100455-82b81c39.pth) \| [log](https://download.openmmlab.com/mmdetection3d/v1.0.0_models/pointpillars/hv_pointpillars_secfpn_sbn-all_2x8_2x_lyft-3d/hv_pointpillars_secfpn_sbn-all_2x8_2x_lyft-3d_20210829_100455.log.json) |
+| [FPN](./hv_pointpillars_fpn_sbn-all_2x8_2x_lyft-3d.py) | 2x | 9.2 | | 14.8 | 15.0 | [model](https://download.openmmlab.com/mmdetection3d/v1.0.0_models/pointpillars/hv_pointpillars_fpn_sbn-all_2x8_2x_lyft-3d/hv_pointpillars_fpn_sbn-all_2x8_2x_lyft-3d_20210822_095429-0b3d6196.pth) \| [log](https://download.openmmlab.com/mmdetection3d/v1.0.0_models/pointpillars/hv_pointpillars_fpn_sbn-all_2x8_2x_lyft-3d/hv_pointpillars_fpn_sbn-all_2x8_2x_lyft-3d_20210822_095429.log.json) |
+
+### Waymo
+
+| Backbone | Load Interval | Class | Lr schd | Mem (GB) | Inf time (fps) | mAP@L1 | mAPH@L1 | mAP@L2 | **mAPH@L2** | Download |
+| :-----------------------------------------------------------------: | :-----------: | :-----: | :-----: | :------: | :------------: | :----: | :-----: | :----: | :---------: | :------------------------------------------------------------------------------------------------------------------------------------------------------------------------------------------------------------------------------------------------------------------------------------------------------------------------------------------------------------------------------------------------------------------------------------------: |
+| [SECFPN](./hv_pointpillars_secfpn_sbn_2x16_2x_waymoD5-3d-car.py) | 5 | Car | 2x | 7.76 | | 70.2 | 69.6 | 62.6 | 62.1 | [model](https://download.openmmlab.com/mmdetection3d/v0.1.0_models/pointpillars/hv_pointpillars_secfpn_sbn_2x16_2x_waymoD5-3d-car/hv_pointpillars_secfpn_sbn_2x16_2x_waymoD5-3d-car_20200901_204315-302fc3e7.pth) \| [log](https://download.openmmlab.com/mmdetection3d/v0.1.0_models/pointpillars/hv_pointpillars_secfpn_sbn_2x16_2x_waymoD5-3d-car/hv_pointpillars_secfpn_sbn_2x16_2x_waymoD5-3d-car_20200901_204315.log.json) |
+| [SECFPN](./hv_pointpillars_secfpn_sbn_2x16_2x_waymoD5-3d-3class.py) | 5 | 3 Class | 2x | 8.12 | | 64.7 | 57.6 | 58.4 | 52.1 | [model](https://download.openmmlab.com/mmdetection3d/v0.1.0_models/pointpillars/hv_pointpillars_secfpn_sbn_2x16_2x_waymoD5-3d-3class/hv_pointpillars_secfpn_sbn_2x16_2x_waymoD5-3d-3class_20200831_204144-d1a706b1.pth) \| [log](https://download.openmmlab.com/mmdetection3d/v0.1.0_models/pointpillars/hv_pointpillars_secfpn_sbn_2x16_2x_waymoD5-3d-3class/hv_pointpillars_secfpn_sbn_2x16_2x_waymoD5-3d-3class_20200831_204144.log.json) |
+| above @ Car | | | 2x | 8.12 | | 68.5 | 67.9 | 60.1 | 59.6 | |
+| above @ Pedestrian | | | 2x | 8.12 | | 67.8 | 50.6 | 59.6 | 44.3 | |
+| above @ Cyclist | | | 2x | 8.12 | | 57.7 | 54.4 | 55.5 | 52.4 | |
+| [SECFPN](./hv_pointpillars_secfpn_sbn_2x16_2x_waymo-3d-car.py) | 1 | Car | 2x | 7.76 | | 72.1 | 71.5 | 63.6 | 63.1 | [log](https://download.openmmlab.com/mmdetection3d/v0.1.0_models/pointpillars/hv_pointpillars_secfpn_sbn_2x16_2x_waymo-3d-car/hv_pointpillars_secfpn_sbn_2x16_2x_waymo-3d-car.log.json) |
+| [SECFPN](./hv_pointpillars_secfpn_sbn_2x16_2x_waymo-3d-3class.py) | 1 | 3 Class | 2x | 8.12 | | 68.8 | 63.3 | 62.6 | 57.6 | [log](https://download.openmmlab.com/mmdetection3d/v0.1.0_models/pointpillars/hv_pointpillars_secfpn_sbn_2x16_2x_waymo-3d-3class/hv_pointpillars_secfpn_sbn_2x16_2x_waymo-3d-3class.log.json) |
+| above @ Car | | | 2x | 8.12 | | 71.6 | 71.0 | 63.1 | 62.5 | |
+| above @ Pedestrian | | | 2x | 8.12 | | 70.6 | 56.7 | 62.9 | 50.2 | |
+| above @ Cyclist | | | 2x | 8.12 | | 64.4 | 62.3 | 61.9 | 59.9 | |
+
+#### Note:
+
+- **Metric**: For model trained with 3 classes, the average APH@L2 (mAPH@L2) of all the categories is reported and used to rank the model. For model trained with only 1 class, the APH@L2 is reported and used to rank the model.
+- **Data Split**: Here we provide several baselines for waymo dataset, among which D5 means that we divide the dataset into 5 folds and only use one fold for efficient experiments. Using the complete dataset can boost the performance a lot, especially for the detection of cyclist and pedestrian, where more than 5 mAP or mAPH improvement can be expected.
+- **Implementation Details**: We basically follow the implementation in the [paper](https://arxiv.org/pdf/1912.04838.pdf) in terms of the network architecture (having a
+ stride of 1 for the first convolutional block). Different settings of voxelization, data augmentation and hyper parameters make these baselines outperform those in the paper by about 7 mAP for car and 4 mAP for pedestrian with only a subset of the whole dataset. All of these results are achieved without bells-and-whistles, e.g. ensemble, multi-scale training and test augmentation.
+- **License Aggrement**: To comply the [license agreement of Waymo dataset](https://waymo.com/open/terms/), the pre-trained models on Waymo dataset are not released. We still release the training log as a reference to ease the future research.
+- `FP16` means Mixed Precision (FP16) is adopted in training. With mixed precision training, we can train PointPillars with nuScenes dataset on 8 Titan XP GPUS with batch size of 2. This will cause OOM error without mixed precision training. The loss scale for PointPillars on nuScenes dataset is specifically tuned to avoid the loss to be Nan. We find 32 is more stable than 512, though loss scale 32 still cause Nan sometimes.
+
+## Citation
+
+```latex
+@inproceedings{lang2019pointpillars,
+ title={Pointpillars: Fast encoders for object detection from point clouds},
+ author={Lang, Alex H and Vora, Sourabh and Caesar, Holger and Zhou, Lubing and Yang, Jiong and Beijbom, Oscar},
+ booktitle={Proceedings of the IEEE Conference on Computer Vision and Pattern Recognition},
+ pages={12697--12705},
+ year={2019}
+}
+```
diff --git a/configs/pointpillars/hv_pointpillars_fpn_sbn-all_2x8_2x_lyft-3d.py b/configs/pointpillars/hv_pointpillars_fpn_sbn-all_2x8_2x_lyft-3d.py
new file mode 100644
index 0000000..6cc3e2d
--- /dev/null
+++ b/configs/pointpillars/hv_pointpillars_fpn_sbn-all_2x8_2x_lyft-3d.py
@@ -0,0 +1,5 @@
+_base_ = [
+ '../_base_/models/hv_pointpillars_fpn_lyft.py',
+ '../_base_/datasets/lyft-3d.py', '../_base_/schedules/schedule_2x.py',
+ '../_base_/default_runtime.py'
+]
diff --git a/configs/pointpillars/hv_pointpillars_fpn_sbn-all_4x8_2x_nus-3d.py b/configs/pointpillars/hv_pointpillars_fpn_sbn-all_4x8_2x_nus-3d.py
new file mode 100644
index 0000000..2c6ba49
--- /dev/null
+++ b/configs/pointpillars/hv_pointpillars_fpn_sbn-all_4x8_2x_nus-3d.py
@@ -0,0 +1,5 @@
+_base_ = [
+ '../_base_/models/hv_pointpillars_fpn_nus.py',
+ '../_base_/datasets/nus-3d.py', '../_base_/schedules/schedule_2x.py',
+ '../_base_/default_runtime.py'
+]
diff --git a/configs/pointpillars/hv_pointpillars_fpn_sbn-all_fp16_2x8_2x_nus-3d.py b/configs/pointpillars/hv_pointpillars_fpn_sbn-all_fp16_2x8_2x_nus-3d.py
new file mode 100644
index 0000000..9764aa3
--- /dev/null
+++ b/configs/pointpillars/hv_pointpillars_fpn_sbn-all_fp16_2x8_2x_nus-3d.py
@@ -0,0 +1,4 @@
+_base_ = './hv_pointpillars_fpn_sbn-all_4x8_2x_nus-3d.py'
+data = dict(samples_per_gpu=2, workers_per_gpu=2)
+# fp16 settings, the loss scale is specifically tuned to avoid Nan
+fp16 = dict(loss_scale=32.)
diff --git a/configs/pointpillars/hv_pointpillars_fpn_sbn-all_range100_2x8_2x_lyft-3d.py b/configs/pointpillars/hv_pointpillars_fpn_sbn-all_range100_2x8_2x_lyft-3d.py
new file mode 100644
index 0000000..57c90db
--- /dev/null
+++ b/configs/pointpillars/hv_pointpillars_fpn_sbn-all_range100_2x8_2x_lyft-3d.py
@@ -0,0 +1,5 @@
+_base_ = [
+ '../_base_/models/hv_pointpillars_fpn_range100_lyft.py',
+ '../_base_/datasets/range100_lyft-3d.py',
+ '../_base_/schedules/schedule_2x.py', '../_base_/default_runtime.py'
+]
diff --git a/configs/pointpillars/hv_pointpillars_secfpn_6x8_160e_kitti-3d-3class.py b/configs/pointpillars/hv_pointpillars_secfpn_6x8_160e_kitti-3d-3class.py
new file mode 100644
index 0000000..d8aad2f
--- /dev/null
+++ b/configs/pointpillars/hv_pointpillars_secfpn_6x8_160e_kitti-3d-3class.py
@@ -0,0 +1,81 @@
+_base_ = [
+ '../_base_/models/hv_pointpillars_secfpn_kitti.py',
+ '../_base_/datasets/kitti-3d-3class.py',
+ '../_base_/schedules/cyclic_40e.py', '../_base_/default_runtime.py'
+]
+
+point_cloud_range = [0, -39.68, -3, 69.12, 39.68, 1]
+# dataset settings
+data_root = 'data/kitti/'
+class_names = ['Pedestrian', 'Cyclist', 'Car']
+# PointPillars adopted a different sampling strategies among classes
+db_sampler = dict(
+ data_root=data_root,
+ info_path=data_root + 'kitti_dbinfos_train.pkl',
+ rate=1.0,
+ prepare=dict(
+ filter_by_difficulty=[-1],
+ filter_by_min_points=dict(Car=5, Pedestrian=5, Cyclist=5)),
+ classes=class_names,
+ sample_groups=dict(Car=15, Pedestrian=15, Cyclist=15))
+
+# PointPillars uses different augmentation hyper parameters
+train_pipeline = [
+ dict(type='LoadPointsFromFile', coord_type='LIDAR', load_dim=4, use_dim=4),
+ dict(type='LoadAnnotations3D', with_bbox_3d=True, with_label_3d=True),
+ dict(type='ObjectSample', db_sampler=db_sampler, use_ground_plane=True),
+ dict(type='RandomFlip3D', flip_ratio_bev_horizontal=0.5),
+ dict(
+ type='GlobalRotScaleTrans',
+ rot_range=[-0.78539816, 0.78539816],
+ scale_ratio_range=[0.95, 1.05]),
+ dict(type='PointsRangeFilter', point_cloud_range=point_cloud_range),
+ dict(type='ObjectRangeFilter', point_cloud_range=point_cloud_range),
+ dict(type='PointShuffle'),
+ dict(type='DefaultFormatBundle3D', class_names=class_names),
+ dict(type='Collect3D', keys=['points', 'gt_bboxes_3d', 'gt_labels_3d'])
+]
+test_pipeline = [
+ dict(type='LoadPointsFromFile', coord_type='LIDAR', load_dim=4, use_dim=4),
+ dict(
+ type='MultiScaleFlipAug3D',
+ img_scale=(1333, 800),
+ pts_scale_ratio=1,
+ flip=False,
+ transforms=[
+ dict(
+ type='GlobalRotScaleTrans',
+ rot_range=[0, 0],
+ scale_ratio_range=[1., 1.],
+ translation_std=[0, 0, 0]),
+ dict(type='RandomFlip3D'),
+ dict(
+ type='PointsRangeFilter', point_cloud_range=point_cloud_range),
+ dict(
+ type='DefaultFormatBundle3D',
+ class_names=class_names,
+ with_label=False),
+ dict(type='Collect3D', keys=['points'])
+ ])
+]
+
+data = dict(
+ train=dict(dataset=dict(pipeline=train_pipeline, classes=class_names)),
+ val=dict(pipeline=test_pipeline, classes=class_names),
+ test=dict(pipeline=test_pipeline, classes=class_names))
+
+# In practice PointPillars also uses a different schedule
+# optimizer
+lr = 0.001
+optimizer = dict(lr=lr)
+# max_norm=35 is slightly better than 10 for PointPillars in the earlier
+# development of the codebase thus we keep the setting. But we does not
+# specifically tune this parameter.
+optimizer_config = dict(grad_clip=dict(max_norm=35, norm_type=2))
+# PointPillars usually need longer schedule than second, we simply double
+# the training schedule. Do remind that since we use RepeatDataset and
+# repeat factor is 2, so we actually train 160 epochs.
+runner = dict(max_epochs=80)
+
+# Use evaluation interval=2 reduce the number of evaluation timese
+evaluation = dict(interval=2)
diff --git a/configs/pointpillars/hv_pointpillars_secfpn_6x8_160e_kitti-3d-car.py b/configs/pointpillars/hv_pointpillars_secfpn_6x8_160e_kitti-3d-car.py
new file mode 100644
index 0000000..3537ce3
--- /dev/null
+++ b/configs/pointpillars/hv_pointpillars_secfpn_6x8_160e_kitti-3d-car.py
@@ -0,0 +1,87 @@
+# model settings
+_base_ = './hv_pointpillars_secfpn_6x8_160e_kitti-3d-3class.py'
+
+point_cloud_range = [0, -39.68, -3, 69.12, 39.68, 1]
+model = dict(
+ bbox_head=dict(
+ type='Anchor3DHead',
+ num_classes=1,
+ anchor_generator=dict(
+ _delete_=True,
+ type='AlignedAnchor3DRangeGenerator',
+ ranges=[[0, -39.68, -1.78, 69.12, 39.68, -1.78]],
+ sizes=[[3.9, 1.6, 1.56]],
+ rotations=[0, 1.57],
+ reshape_out=True)),
+ # model training and testing settings
+ train_cfg=dict(
+ _delete_=True,
+ assigner=dict(
+ type='MaxIoUAssigner',
+ iou_calculator=dict(type='BboxOverlapsNearest3D'),
+ pos_iou_thr=0.6,
+ neg_iou_thr=0.45,
+ min_pos_iou=0.45,
+ ignore_iof_thr=-1),
+ allowed_border=0,
+ pos_weight=-1,
+ debug=False))
+
+# dataset settings
+dataset_type = 'KittiDataset'
+data_root = 'data/kitti/'
+class_names = ['Car']
+db_sampler = dict(
+ data_root=data_root,
+ info_path=data_root + 'kitti_dbinfos_train.pkl',
+ rate=1.0,
+ prepare=dict(filter_by_difficulty=[-1], filter_by_min_points=dict(Car=5)),
+ sample_groups=dict(Car=15),
+ classes=class_names)
+
+train_pipeline = [
+ dict(type='LoadPointsFromFile', coord_type='LIDAR', load_dim=4, use_dim=4),
+ dict(type='LoadAnnotations3D', with_bbox_3d=True, with_label_3d=True),
+ dict(type='ObjectSample', db_sampler=db_sampler, use_ground_plane=True),
+ dict(type='RandomFlip3D', flip_ratio_bev_horizontal=0.5),
+ dict(
+ type='GlobalRotScaleTrans',
+ rot_range=[-0.78539816, 0.78539816],
+ scale_ratio_range=[0.95, 1.05]),
+ dict(type='PointsRangeFilter', point_cloud_range=point_cloud_range),
+ dict(type='ObjectRangeFilter', point_cloud_range=point_cloud_range),
+ dict(type='PointShuffle'),
+ dict(type='DefaultFormatBundle3D', class_names=class_names),
+ dict(type='Collect3D', keys=['points', 'gt_bboxes_3d', 'gt_labels_3d'])
+]
+test_pipeline = [
+ dict(type='LoadPointsFromFile', coord_type='LIDAR', load_dim=4, use_dim=4),
+ dict(
+ type='MultiScaleFlipAug3D',
+ img_scale=(1333, 800),
+ pts_scale_ratio=1,
+ flip=False,
+ transforms=[
+ dict(
+ type='GlobalRotScaleTrans',
+ rot_range=[0, 0],
+ scale_ratio_range=[1., 1.],
+ translation_std=[0, 0, 0]),
+ dict(type='RandomFlip3D'),
+ dict(
+ type='PointsRangeFilter', point_cloud_range=point_cloud_range),
+ dict(
+ type='DefaultFormatBundle3D',
+ class_names=class_names,
+ with_label=False),
+ dict(type='Collect3D', keys=['points'])
+ ])
+]
+
+data = dict(
+ train=dict(
+ type='RepeatDataset',
+ times=2,
+ dataset=dict(pipeline=train_pipeline, classes=class_names)),
+ val=dict(pipeline=test_pipeline, classes=class_names),
+ test=dict(pipeline=test_pipeline, classes=class_names))
diff --git a/configs/pointpillars/hv_pointpillars_secfpn_sbn-all_2x8_2x_lyft-3d.py b/configs/pointpillars/hv_pointpillars_secfpn_sbn-all_2x8_2x_lyft-3d.py
new file mode 100644
index 0000000..1a0400e
--- /dev/null
+++ b/configs/pointpillars/hv_pointpillars_secfpn_sbn-all_2x8_2x_lyft-3d.py
@@ -0,0 +1,43 @@
+_base_ = [
+ '../_base_/models/hv_pointpillars_fpn_lyft.py',
+ '../_base_/datasets/lyft-3d.py',
+ '../_base_/schedules/schedule_2x.py',
+ '../_base_/default_runtime.py',
+]
+# model settings
+model = dict(
+ pts_neck=dict(
+ _delete_=True,
+ type='SECONDFPN',
+ norm_cfg=dict(type='naiveSyncBN2d', eps=1e-3, momentum=0.01),
+ in_channels=[64, 128, 256],
+ upsample_strides=[1, 2, 4],
+ out_channels=[128, 128, 128]),
+ pts_bbox_head=dict(
+ in_channels=384,
+ feat_channels=384,
+ anchor_generator=dict(
+ _delete_=True,
+ type='AlignedAnchor3DRangeGenerator',
+ ranges=[[-80, -80, -1.0715024, 80, 80, -1.0715024],
+ [-80, -80, -0.3033737, 80, 80, -0.3033737],
+ [-80, -80, -0.3519405, 80, 80, -0.3519405],
+ [-80, -80, -0.8871424, 80, 80, -0.8871424],
+ [-80, -80, -0.6276341, 80, 80, -0.6276341],
+ [-80, -80, -1.3220503, 80, 80, -1.3220503],
+ [-80, -80, -1.0709302, 80, 80, -1.0709302],
+ [-80, -80, -0.9122268, 80, 80, -0.9122268],
+ [-80, -80, -1.8012227, 80, 80, -1.8012227]],
+ sizes=[
+ [4.75, 1.92, 1.71], # car
+ [10.24, 2.84, 3.44], # truck
+ [12.70, 2.92, 3.42], # bus
+ [6.52, 2.42, 2.34], # emergency vehicle
+ [8.17, 2.75, 3.20], # other vehicle
+ [2.35, 0.96, 1.59], # motorcycle
+ [1.76, 0.63, 1.44], # bicycle
+ [0.80, 0.76, 1.76], # pedestrian
+ [0.73, 0.35, 0.50] # animal
+ ],
+ rotations=[0, 1.57],
+ reshape_out=True)))
diff --git a/configs/pointpillars/hv_pointpillars_secfpn_sbn-all_4x8_2x_nus-3d.py b/configs/pointpillars/hv_pointpillars_secfpn_sbn-all_4x8_2x_nus-3d.py
new file mode 100644
index 0000000..afff99c
--- /dev/null
+++ b/configs/pointpillars/hv_pointpillars_secfpn_sbn-all_4x8_2x_nus-3d.py
@@ -0,0 +1,42 @@
+_base_ = [
+ '../_base_/models/hv_pointpillars_fpn_nus.py',
+ '../_base_/datasets/nus-3d.py',
+ '../_base_/schedules/schedule_2x.py',
+ '../_base_/default_runtime.py',
+]
+# model settings
+model = dict(
+ pts_neck=dict(
+ _delete_=True,
+ type='SECONDFPN',
+ norm_cfg=dict(type='naiveSyncBN2d', eps=1e-3, momentum=0.01),
+ in_channels=[64, 128, 256],
+ upsample_strides=[1, 2, 4],
+ out_channels=[128, 128, 128]),
+ pts_bbox_head=dict(
+ in_channels=384,
+ feat_channels=384,
+ anchor_generator=dict(
+ _delete_=True,
+ type='AlignedAnchor3DRangeGenerator',
+ ranges=[
+ [-49.6, -49.6, -1.80032795, 49.6, 49.6, -1.80032795],
+ [-49.6, -49.6, -1.74440365, 49.6, 49.6, -1.74440365],
+ [-49.6, -49.6, -1.68526504, 49.6, 49.6, -1.68526504],
+ [-49.6, -49.6, -1.67339111, 49.6, 49.6, -1.67339111],
+ [-49.6, -49.6, -1.61785072, 49.6, 49.6, -1.61785072],
+ [-49.6, -49.6, -1.80984986, 49.6, 49.6, -1.80984986],
+ [-49.6, -49.6, -1.763965, 49.6, 49.6, -1.763965],
+ ],
+ sizes=[
+ [4.60718145, 1.95017717, 1.72270761], # car
+ [6.73778078, 2.4560939, 2.73004906], # truck
+ [12.01320693, 2.87427237, 3.81509561], # trailer
+ [1.68452161, 0.60058911, 1.27192197], # bicycle
+ [0.7256437, 0.66344886, 1.75748069], # pedestrian
+ [0.40359262, 0.39694519, 1.06232151], # traffic_cone
+ [0.48578221, 2.49008838, 0.98297065], # barrier
+ ],
+ custom_values=[0, 0],
+ rotations=[0, 1.57],
+ reshape_out=True)))
diff --git a/configs/pointpillars/hv_pointpillars_secfpn_sbn-all_fp16_2x8_2x_nus-3d.py b/configs/pointpillars/hv_pointpillars_secfpn_sbn-all_fp16_2x8_2x_nus-3d.py
new file mode 100644
index 0000000..ff0f67a
--- /dev/null
+++ b/configs/pointpillars/hv_pointpillars_secfpn_sbn-all_fp16_2x8_2x_nus-3d.py
@@ -0,0 +1,4 @@
+_base_ = './hv_pointpillars_secfpn_sbn-all_4x8_2x_nus-3d.py'
+data = dict(samples_per_gpu=2, workers_per_gpu=2)
+# fp16 settings, the loss scale is specifically tuned to avoid Nan
+fp16 = dict(loss_scale=32.)
diff --git a/configs/pointpillars/hv_pointpillars_secfpn_sbn-all_range100_2x8_2x_lyft-3d.py b/configs/pointpillars/hv_pointpillars_secfpn_sbn-all_range100_2x8_2x_lyft-3d.py
new file mode 100644
index 0000000..7964b79
--- /dev/null
+++ b/configs/pointpillars/hv_pointpillars_secfpn_sbn-all_range100_2x8_2x_lyft-3d.py
@@ -0,0 +1,42 @@
+_base_ = [
+ '../_base_/models/hv_pointpillars_fpn_range100_lyft.py',
+ '../_base_/datasets/range100_lyft-3d.py',
+ '../_base_/schedules/schedule_2x.py', '../_base_/default_runtime.py'
+]
+# model settings
+model = dict(
+ pts_neck=dict(
+ _delete_=True,
+ type='SECONDFPN',
+ norm_cfg=dict(type='naiveSyncBN2d', eps=1e-3, momentum=0.01),
+ in_channels=[64, 128, 256],
+ upsample_strides=[1, 2, 4],
+ out_channels=[128, 128, 128]),
+ pts_bbox_head=dict(
+ in_channels=384,
+ feat_channels=384,
+ anchor_generator=dict(
+ _delete_=True,
+ type='AlignedAnchor3DRangeGenerator',
+ ranges=[[-100, -100, -1.0715024, 100, 100, -1.0715024],
+ [-100, -100, -0.3033737, 100, 100, -0.3033737],
+ [-100, -100, -0.3519405, 100, 100, -0.3519405],
+ [-100, -100, -0.8871424, 100, 100, -0.8871424],
+ [-100, -100, -0.6276341, 100, 100, -0.6276341],
+ [-100, -100, -1.3220503, 100, 100, -1.3220503],
+ [-100, -100, -1.0709302, 100, 100, -1.0709302],
+ [-100, -100, -0.9122268, 100, 100, -0.9122268],
+ [-100, -100, -1.8012227, 100, 100, -1.8012227]],
+ sizes=[
+ [4.75, 1.92, 1.71], # car
+ [10.24, 2.84, 3.44], # truck
+ [12.70, 2.92, 3.42], # bus
+ [6.52, 2.42, 2.34], # emergency vehicle
+ [8.17, 2.75, 3.20], # other vehicle
+ [2.35, 0.96, 1.59], # motorcycle
+ [1.76, 0.63, 1.44], # bicycle
+ [0.80, 0.76, 1.76], # pedestrian
+ [0.73, 0.35, 0.50] # animal
+ ],
+ rotations=[0, 1.57],
+ reshape_out=True)))
diff --git a/configs/pointpillars/hv_pointpillars_secfpn_sbn_2x16_2x_waymo-3d-3class.py b/configs/pointpillars/hv_pointpillars_secfpn_sbn_2x16_2x_waymo-3d-3class.py
new file mode 100644
index 0000000..8655691
--- /dev/null
+++ b/configs/pointpillars/hv_pointpillars_secfpn_sbn_2x16_2x_waymo-3d-3class.py
@@ -0,0 +1,9 @@
+_base_ = [
+ '../_base_/models/hv_pointpillars_secfpn_waymo.py',
+ '../_base_/datasets/waymoD5-3d-3class.py',
+ '../_base_/schedules/schedule_2x.py',
+ '../_base_/default_runtime.py',
+]
+
+# data settings
+data = dict(train=dict(dataset=dict(load_interval=1)))
diff --git a/configs/pointpillars/hv_pointpillars_secfpn_sbn_2x16_2x_waymo-3d-car.py b/configs/pointpillars/hv_pointpillars_secfpn_sbn_2x16_2x_waymo-3d-car.py
new file mode 100644
index 0000000..90f2a42
--- /dev/null
+++ b/configs/pointpillars/hv_pointpillars_secfpn_sbn_2x16_2x_waymo-3d-car.py
@@ -0,0 +1,37 @@
+_base_ = [
+ '../_base_/models/hv_pointpillars_secfpn_waymo.py',
+ '../_base_/datasets/waymoD5-3d-car.py',
+ '../_base_/schedules/schedule_2x.py',
+ '../_base_/default_runtime.py',
+]
+
+# data settings
+data = dict(train=dict(dataset=dict(load_interval=1)))
+
+# model settings
+model = dict(
+ type='MVXFasterRCNN',
+ pts_bbox_head=dict(
+ type='Anchor3DHead',
+ num_classes=1,
+ anchor_generator=dict(
+ type='AlignedAnchor3DRangeGenerator',
+ ranges=[[-74.88, -74.88, -0.0345, 74.88, 74.88, -0.0345]],
+ sizes=[[4.73, 2.08, 1.77]],
+ rotations=[0, 1.57],
+ reshape_out=True)),
+ # model training and testing settings
+ train_cfg=dict(
+ _delete_=True,
+ pts=dict(
+ assigner=dict(
+ type='MaxIoUAssigner',
+ iou_calculator=dict(type='BboxOverlapsNearest3D'),
+ pos_iou_thr=0.55,
+ neg_iou_thr=0.4,
+ min_pos_iou=0.4,
+ ignore_iof_thr=-1),
+ allowed_border=0,
+ code_weight=[1.0, 1.0, 1.0, 1.0, 1.0, 1.0, 1.0],
+ pos_weight=-1,
+ debug=False)))
diff --git a/configs/pointpillars/hv_pointpillars_secfpn_sbn_2x16_2x_waymoD5-3d-3class.py b/configs/pointpillars/hv_pointpillars_secfpn_sbn_2x16_2x_waymoD5-3d-3class.py
new file mode 100644
index 0000000..e4f1ce5
--- /dev/null
+++ b/configs/pointpillars/hv_pointpillars_secfpn_sbn_2x16_2x_waymoD5-3d-3class.py
@@ -0,0 +1,6 @@
+_base_ = [
+ '../_base_/models/hv_pointpillars_secfpn_waymo.py',
+ '../_base_/datasets/waymoD5-3d-3class.py',
+ '../_base_/schedules/schedule_2x.py',
+ '../_base_/default_runtime.py',
+]
diff --git a/configs/pointpillars/hv_pointpillars_secfpn_sbn_2x16_2x_waymoD5-3d-car.py b/configs/pointpillars/hv_pointpillars_secfpn_sbn_2x16_2x_waymoD5-3d-car.py
new file mode 100644
index 0000000..3a3e326
--- /dev/null
+++ b/configs/pointpillars/hv_pointpillars_secfpn_sbn_2x16_2x_waymoD5-3d-car.py
@@ -0,0 +1,34 @@
+_base_ = [
+ '../_base_/models/hv_pointpillars_secfpn_waymo.py',
+ '../_base_/datasets/waymoD5-3d-car.py',
+ '../_base_/schedules/schedule_2x.py',
+ '../_base_/default_runtime.py',
+]
+
+# model settings
+model = dict(
+ type='MVXFasterRCNN',
+ pts_bbox_head=dict(
+ type='Anchor3DHead',
+ num_classes=1,
+ anchor_generator=dict(
+ type='AlignedAnchor3DRangeGenerator',
+ ranges=[[-74.88, -74.88, -0.0345, 74.88, 74.88, -0.0345]],
+ sizes=[[4.73, 2.08, 1.77]],
+ rotations=[0, 1.57],
+ reshape_out=True)),
+ # model training and testing settings
+ train_cfg=dict(
+ _delete_=True,
+ pts=dict(
+ assigner=dict(
+ type='MaxIoUAssigner',
+ iou_calculator=dict(type='BboxOverlapsNearest3D'),
+ pos_iou_thr=0.55,
+ neg_iou_thr=0.4,
+ min_pos_iou=0.4,
+ ignore_iof_thr=-1),
+ allowed_border=0,
+ code_weight=[1.0, 1.0, 1.0, 1.0, 1.0, 1.0, 1.0],
+ pos_weight=-1,
+ debug=False)))
diff --git a/configs/pointpillars/metafile.yml b/configs/pointpillars/metafile.yml
new file mode 100644
index 0000000..9a898c4
--- /dev/null
+++ b/configs/pointpillars/metafile.yml
@@ -0,0 +1,213 @@
+Collections:
+ - Name: PointPillars
+ Metadata:
+ Training Techniques:
+ - AdamW
+ Architecture:
+ - Feature Pyramid Network
+ Paper:
+ URL: https://arxiv.org/abs/1812.05784
+ Title: 'PointPillars: Fast Encoders for Object Detection from Point Clouds'
+ README: configs/pointpillars/README.md
+ Code:
+ URL: https://github.com/open-mmlab/mmdetection3d/blob/master/mmdet3d/models/voxel_encoders/pillar_encoder.py#L13
+ Version: v0.6.0
+
+Models:
+ - Name: hv_pointpillars_secfpn_6x8_160e_kitti-3d-car
+ In Collection: PointPillars
+ Config: configs/pointpillars/hv_pointpillars_secfpn_6x8_160e_kitti-3d-car.py
+ Metadata:
+ Training Data: KITTI
+ Training Memory (GB): 5.4
+ Training Resources: 8x V100 GPUs
+ Results:
+ - Task: 3D Object Detection
+ Dataset: KITTI
+ Metrics:
+ AP: 77.6
+ Weights: https://download.openmmlab.com/mmdetection3d/v1.0.0_models/pointpillars/hv_pointpillars_secfpn_6x8_160e_kitti-3d-car/hv_pointpillars_secfpn_6x8_160e_kitti-3d-car_20220331_134606-d42d15ed.pth
+
+ - Name: hv_pointpillars_secfpn_6x8_160e_kitti-3d-3class
+ In Collection: PointPillars
+ Config: configs/pointpillars/hv_pointpillars_secfpn_6x8_160e_kitti-3d-3class.py
+ Metadata:
+ Training Data: KITTI
+ Training Memory (GB): 5.5
+ Training Resources: 8x V100 GPUs
+ Results:
+ - Task: 3D Object Detection
+ Dataset: KITTI
+ Metrics:
+ AP: 64.07
+ Weights: https://download.openmmlab.com/mmdetection3d/v1.0.0_models/pointpillars/hv_pointpillars_secfpn_6x8_160e_kitti-3d-3class/hv_pointpillars_secfpn_6x8_160e_kitti-3d-3class_20220301_150306-37dc2420.pth
+
+ - Name: hv_pointpillars_secfpn_sbn-all_4x8_2x_nus-3d
+ In Collection: PointPillars
+ Config: configs/pointpillars/hv_pointpillars_secfpn_sbn-all_4x8_2x_nus-3d.py
+ Metadata:
+ Training Data: nuScenes
+ Training Memory (GB): 16.4
+ Training Resources: 8x V100 GPUs
+ Results:
+ - Task: 3D Object Detection
+ Dataset: nuScenes
+ Metrics:
+ mAP: 34.33
+ NDS: 49.1
+ Weights: https://download.openmmlab.com/mmdetection3d/v1.0.0_models/pointpillars/hv_pointpillars_secfpn_sbn-all_4x8_2x_nus-3d/hv_pointpillars_secfpn_sbn-all_4x8_2x_nus-3d_20210826_225857-f19d00a3.pth
+
+ - Name: hv_pointpillars_fpn_sbn-all_4x8_2x_nus-3d
+ In Collection: PointPillars
+ Config: configs/pointpillars/hv_pointpillars_fpn_sbn-all_4x8_2x_nus-3d.py
+ Metadata:
+ Training Data: nuScenes
+ Training Memory (GB): 16.3
+ Training Resources: 8x V100 GPUs
+ Results:
+ - Task: 3D Object Detection
+ Dataset: nuScenes
+ Metrics:
+ mAP: 39.71
+ NDS: 53.15
+ Weights: https://download.openmmlab.com/mmdetection3d/v1.0.0_models/pointpillars/hv_pointpillars_fpn_sbn-all_4x8_2x_nus-3d/hv_pointpillars_fpn_sbn-all_4x8_2x_nus-3d_20210826_104936-fca299c1.pth
+
+ - Name: hv_pointpillars_secfpn_sbn-all_2x8_2x_lyft-3d
+ In Collection: PointPillars
+ Config: configs/pointpillars/hv_pointpillars_secfpn_sbn-all_2x8_2x_lyft-3d.py
+ Metadata:
+ Training Data: Lyft
+ Training Memory (GB): 12.2
+ Training Resources: 8x V100 GPUs
+ Results:
+ - Task: 3D Object Detection
+ Dataset: Lyft
+ Metrics:
+ Private Score: 13.8
+ Public Score: 14.1
+ Weights: https://download.openmmlab.com/mmdetection3d/v1.0.0_models/pointpillars/hv_pointpillars_secfpn_sbn-all_2x8_2x_lyft-3d/hv_pointpillars_secfpn_sbn-all_2x8_2x_lyft-3d_20210829_100455-82b81c39.pth
+
+ - Name: hv_pointpillars_fpn_sbn-all_2x8_2x_lyft-3d
+ In Collection: PointPillars
+ Config: configs/pointpillars/hv_pointpillars_fpn_sbn-all_2x8_2x_lyft-3d.py
+ Metadata:
+ Training Data: Lyft
+ Training Memory (GB): 9.2
+ Training Resources: 8x V100 GPUs
+ Results:
+ - Task: 3D Object Detection
+ Dataset: Lyft
+ Metrics:
+ Private Score: 14.0
+ Public Score: 15.0
+ Weights: https://download.openmmlab.com/mmdetection3d/v1.0.0_models/pointpillars/hv_pointpillars_fpn_sbn-all_2x8_2x_lyft-3d/hv_pointpillars_fpn_sbn-all_2x8_2x_lyft-3d_20210822_095429-0b3d6196.pth
+
+ - Name: hv_pointpillars_secfpn_sbn_2x16_2x_waymoD5-3d-car
+ In Collection: PointPillars
+ Config: configs/pointpillars/hv_pointpillars_secfpn_sbn_2x16_2x_waymoD5-3d-car.py
+ Metadata:
+ Training Data: Waymo
+ Training Memory (GB): 7.76
+ Training Resources: 8x GeForce GTX 1080 Ti
+ Results:
+ - Task: 3D Object Detection
+ Dataset: Waymo
+ Metrics:
+ mAP@L1: 70.2
+ mAPH@L1: 69.6
+ mAP@L2: 62.6
+ mAPH@L2: 62.1
+ Weights: https://download.openmmlab.com/mmdetection3d/v0.1.0_models/pointpillars/hv_pointpillars_secfpn_sbn_2x16_2x_waymoD5-3d-car/hv_pointpillars_secfpn_sbn_2x16_2x_waymoD5-3d-car_20200901_204315-302fc3e7.pth
+
+ - Name: hv_pointpillars_secfpn_sbn_2x16_2x_waymoD5-3d-3class
+ In Collection: PointPillars
+ Config: configs/pointpillars/hv_pointpillars_secfpn_sbn_2x16_2x_waymoD5-3d-3class.py
+ Metadata:
+ Training Data: Waymo
+ Training Memory (GB): 8.12
+ Training Resources: 8x GeForce GTX 1080 Ti
+ Results:
+ - Task: 3D Object Detection
+ Dataset: Waymo
+ Metrics:
+ mAP@L1: 64.7
+ mAPH@L1: 57.6
+ mAP@L2: 58.4
+ mAPH@L2: 52.1
+ Weights: https://download.openmmlab.com/mmdetection3d/v0.1.0_models/pointpillars/hv_pointpillars_secfpn_sbn_2x16_2x_waymoD5-3d-3class/hv_pointpillars_secfpn_sbn_2x16_2x_waymoD5-3d-3class_20200831_204144-d1a706b1.pth
+
+ - Name: hv_pointpillars_secfpn_sbn_2x16_2x_waymo-3d-car
+ In Collection: PointPillars
+ Config: configs/pointpillars/hv_pointpillars_secfpn_sbn_2x16_2x_waymo-3d-car.py
+ Metadata:
+ Training Data: Waymo
+ Training Memory (GB): 7.76
+ Training Resources: 8x GeForce GTX 1080 Ti
+ Results:
+ - Task: 3D Object Detection
+ Dataset: Waymo
+ Metrics:
+ mAP@L1: 72.1
+ mAPH@L1: 71.5
+ mAP@L2: 63.6
+ mAPH@L2: 63.1
+
+ - Name: hv_pointpillars_secfpn_sbn_2x16_2x_waymo-3d-3class
+ In Collection: PointPillars
+ Config: configs/pointpillars/hv_pointpillars_secfpn_sbn_2x16_2x_waymo-3d-3class.py
+ Metadata:
+ Training Data: Waymo
+ Training Memory (GB): 8.12
+ Training Resources: 8x GeForce GTX 1080 Ti
+ Results:
+ - Task: 3D Object Detection
+ Dataset: Waymo
+ Metrics:
+ mAP@L1: 68.8
+ mAPH@L1: 63.3
+ mAP@L2: 62.6
+ mAPH@L2: 57.6
+
+ - Name: hv_pointpillars_secfpn_sbn-all_fp16_2x8_2x_nus-3d
+ In Collection: PointPillars
+ Config: configs/pointpillars/hv_pointpillars_secfpn_sbn-all_fp16_2x8_2x_nus-3d.py
+ Metadata:
+ Training Techniques:
+ - AdamW
+ - Mixed Precision Training
+ Training Resources: 8x TITAN Xp
+ Architecture:
+ - Hard Voxelization
+ Training Data: nuScenes
+ Training Memory (GB): 8.37
+ Results:
+ - Task: 3D Object Detection
+ Dataset: nuScenes
+ Metrics:
+ mAP: 35.19
+ NDS: 50.27
+ Weights: https://download.openmmlab.com/mmdetection3d/v0.1.0_models/fp16/hv_pointpillars_secfpn_sbn-all_fp16_2x8_2x_nus-3d/hv_pointpillars_secfpn_sbn-all_fp16_2x8_2x_nus-3d_20201020_222626-c3f0483e.pth
+ Code:
+ Version: v0.7.0
+
+ - Name: hv_pointpillars_fpn_sbn-all_fp16_2x8_2x_nus-3d
+ In Collection: PointPillars
+ Config: configs/pointpillars/hv_pointpillars_fpn_sbn-all_fp16_2x8_2x_nus-3d.py
+ Metadata:
+ Training Techniques:
+ - AdamW
+ - Mixed Precision Training
+ Training Resources: 8x TITAN Xp
+ Architecture:
+ - Hard Voxelization
+ Training Data: nuScenes
+ Training Memory (GB): 8.40
+ Results:
+ - Task: 3D Object Detection
+ Dataset: nuScenes
+ Metrics:
+ mAP: 39.26
+ NDS: 53.26
+ Weights: https://download.openmmlab.com/mmdetection3d/v0.1.0_models/fp16/hv_pointpillars_fpn_sbn-all_fp16_2x8_2x_nus-3d/hv_pointpillars_fpn_sbn-all_fp16_2x8_2x_nus-3d_20201021_120719-269f9dd6.pth
+ Code:
+ Version: v0.7.0
diff --git a/configs/regnet/README.md b/configs/regnet/README.md
new file mode 100644
index 0000000..f15b94f
--- /dev/null
+++ b/configs/regnet/README.md
@@ -0,0 +1,82 @@
+# Designing Network Design Spaces
+
+> [Designing Network Design Spaces](https://arxiv.org/abs/2003.13678)
+
+
+
+## Abstract
+
+In this work, we present a new network design paradigm. Our goal is to help advance the understanding of network design and discover design principles that generalize across settings. Instead of focusing on designing individual network instances, we design network design spaces that parametrize populations of networks. The overall process is analogous to classic manual design of networks, but elevated to the design space level. Using our methodology we explore the structure aspect of network design and arrive at a low-dimensional design space consisting of simple, regular networks that we call RegNet. The core insight of the RegNet parametrization is surprisingly simple: widths and depths of good networks can be explained by a quantized linear function. We analyze the RegNet design space and arrive at interesting findings that do not match the current practice of network design. The RegNet design space provides simple and fast networks that work well across a wide range of flop regimes. Under comparable training settings and flops, the RegNet models outperform the popular EfficientNet models while being up to 5x faster on GPUs.
+
+
+

+
+
+## Introduction
+
+We implement RegNetX models in 3D detection systems and provide their first results with PointPillars on nuScenes and Lyft dataset.
+
+The pre-trained modles are converted from [model zoo of pycls](https://github.com/facebookresearch/pycls/blob/master/MODEL_ZOO.md) and maintained in [mmcv](https://github.com/open-mmlab/mmcv).
+
+## Usage
+
+To use a regnet model, there are two steps to do:
+
+1. Convert the model to ResNet-style supported by MMDetection
+2. Modify backbone and neck in config accordingly
+
+### Convert model
+
+We already prepare models of FLOPs from 800M to 12G in our model zoo.
+
+For more general usage, we also provide script `regnet2mmdet.py` in the tools directory to convert the key of models pretrained by [pycls](https://github.com/facebookresearch/pycls/) to
+ResNet-style checkpoints used in MMDetection.
+
+```bash
+python -u tools/model_converters/regnet2mmdet.py ${PRETRAIN_PATH} ${STORE_PATH}
+```
+
+This script convert model from `PRETRAIN_PATH` and store the converted model in `STORE_PATH`.
+
+### Modify config
+
+The users can modify the config's `depth` of backbone and corresponding keys in `arch` according to the configs in the [pycls model zoo](https://github.com/facebookresearch/pycls/blob/master/MODEL_ZOO.md).
+The parameter `in_channels` in FPN can be found in the Figure 15 & 16 of the paper (`wi` in the legend).
+This directory already provides some configs with their performance, using RegNetX from 800MF to 12GF level.
+For other pre-trained models or self-implemented regnet models, the users are responsible to check these parameters by themselves.
+
+**Note**: Although Fig. 15 & 16 also provide `w0`, `wa`, `wm`, `group_w`, and `bot_mul` for `arch`, they are quantized thus inaccurate, using them sometimes produces different backbone that does not match the key in the pre-trained model.
+
+## Results and models
+
+### nuScenes
+
+| Backbone | Lr schd | Mem (GB) | Inf time (fps) | mAP | NDS | Download |
+| :------------------------------------------------------------------------------------: | :-----: | :------: | :------------: | :---: | :--: | :--------------------------------------------------------------------------------------------------------------------------------------------------------------------------------------------------------------------------------------------------------------------------------------------------------------------------------------------------------------------------------------------------------------------------------------------------: |
+| [SECFPN](../pointpillars/hv_pointpillars_secfpn_sbn-all_4x8_2x_nus-3d.py) | 2x | 16.4 | | 35.17 | 49.7 | [model](https://download.openmmlab.com/mmdetection3d/v0.1.0_models/pointpillars/hv_pointpillars_secfpn_sbn-all_4x8_2x_nus-3d/hv_pointpillars_secfpn_sbn-all_4x8_2x_nus-3d_20200620_230725-0817d270.pth) \| [log](https://download.openmmlab.com/mmdetection3d/v0.1.0_models/pointpillars/hv_pointpillars_secfpn_sbn-all_4x8_2x_nus-3d/hv_pointpillars_secfpn_sbn-all_4x8_2x_nus-3d_20200620_230725.log.json) |
+| [RegNetX-400MF-SECFPN](./hv_pointpillars_regnet-400mf_secfpn_sbn-all_4x8_2x_nus-3d.py) | 2x | 16.4 | | 41.2 | 55.2 | [model](https://download.openmmlab.com/mmdetection3d/v0.1.0_models/regnet/hv_pointpillars_regnet-400mf_secfpn_sbn-all_4x8_2x_nus-3d/hv_pointpillars_regnet-400mf_secfpn_sbn-all_4x8_2x_nus-3d_20200620_230334-53044f32.pth) \| [log](https://download.openmmlab.com/mmdetection3d/v0.1.0_models/regnet/hv_pointpillars_regnet-400mf_secfpn_sbn-all_4x8_2x_nus-3d/hv_pointpillars_regnet-400mf_secfpn_sbn-all_4x8_2x_nus-3d_20200620_230334.log.json) |
+| [FPN](../pointpillars/hv_pointpillars_fpn_sbn-all_4x8_2x_nus-3d.py) | 2x | 17.1 | | 40.0 | 53.3 | [model](https://download.openmmlab.com/mmdetection3d/v0.1.0_models/pointpillars/hv_pointpillars_fpn_sbn-all_4x8_2x_nus-3d/hv_pointpillars_fpn_sbn-all_4x8_2x_nus-3d_20200620_230405-2fa62f3d.pth) \| [log](https://download.openmmlab.com/mmdetection3d/v0.1.0_models/pointpillars/hv_pointpillars_fpn_sbn-all_4x8_2x_nus-3d/hv_pointpillars_fpn_sbn-all_4x8_2x_nus-3d_20200620_230405.log.json) |
+| [RegNetX-400MF-FPN](./hv_pointpillars_regnet-400mf_fpn_sbn-all_4x8_2x_nus-3d.py) | 2x | 17.3 | | 44.8 | 56.4 | [model](https://download.openmmlab.com/mmdetection3d/v0.1.0_models/regnet/hv_pointpillars_regnet-400mf_fpn_sbn-all_4x8_2x_nus-3d/hv_pointpillars_regnet-400mf_fpn_sbn-all_4x8_2x_nus-3d_20200620_230239-c694dce7.pth) \| [log](https://download.openmmlab.com/mmdetection3d/v0.1.0_models/regnet/hv_pointpillars_regnet-400mf_fpn_sbn-all_4x8_2x_nus-3d/hv_pointpillars_regnet-400mf_fpn_sbn-all_4x8_2x_nus-3d_20200620_230239.log.json) |
+| [RegNetX-1.6gF-FPN](./hv_pointpillars_regnet-1.6gf_fpn_sbn-all_4x8_2x_nus-3d.py) | 2x | 24.0 | | 48.2 | 59.3 | [model](https://download.openmmlab.com/mmdetection3d/v0.1.0_models/regnet/hv_pointpillars_regnet-1.6gf_fpn_sbn-all_4x8_2x_nus-3d/hv_pointpillars_regnet-1.6gf_fpn_sbn-all_4x8_2x_nus-3d_20200629_050311-dcd4e090.pth) \| [log](https://download.openmmlab.com/mmdetection3d/v0.1.0_models/regnet/hv_pointpillars_regnet-1.6gf_fpn_sbn-all_4x8_2x_nus-3d/hv_pointpillars_regnet-1.6gf_fpn_sbn-all_4x8_2x_nus-3d_20200629_050311.log.json) |
+
+### Lyft
+
+| Backbone | Lr schd | Mem (GB) | Inf time (fps) | Private Score | Public Score | Download |
+| :-------------------------------------------------------------------------------------: | :-----: | :------: | :------------: | :-----------: | :----------: | :------------------------------------------------------------------------------------------------------------------------------------------------------------------------------------------------------------------------------------------------------------------------------------------------------------------------------------------------------------------------------------------------------------------------------------------------------: |
+| [SECFPN](../pointpillars/hv_pointpillars_secfpn_sbn-all_2x8_2x_lyft-3d.py) | 2x | 12.2 | | 13.9 | 14.1 | [model](https://download.openmmlab.com/mmdetection3d/v0.1.0_models/pointpillars/hv_pointpillars_secfpn_sbn-all_2x8_2x_lyft-3d/hv_pointpillars_secfpn_sbn-all_2x8_2x_lyft-3d_20210517_204807-2518e3de.pth) \| [log](https://download.openmmlab.com/mmdetection3d/v0.1.0_models/pointpillars/hv_pointpillars_secfpn_sbn-all_2x8_2x_lyft-3d/hv_pointpillars_secfpn_sbn-all_2x8_2x_lyft-3d_20210517_204807.log.json) |
+| [RegNetX-400MF-SECFPN](./hv_pointpillars_regnet-400mf_secfpn_sbn-all_4x8_2x_lyft-3d.py) | 2x | 15.9 | | 14.9 | 15.1 | [model](https://download.openmmlab.com/mmdetection3d/v0.1.0_models/regnet/hv_pointpillars_regnet-400mf_secfpn_sbn-all_2x8_2x_lyft-3d/hv_pointpillars_regnet-400mf_secfpn_sbn-all_2x8_2x_lyft-3d_20210524_092151-42513826.pth) \| [log](https://download.openmmlab.com/mmdetection3d/v0.1.0_models/regnet/hv_pointpillars_regnet-400mf_secfpn_sbn-all_2x8_2x_lyft-3d/hv_pointpillars_regnet-400mf_secfpn_sbn-all_2x8_2x_lyft-3d_20210524_092151.log.json) |
+| [FPN](../pointpillars/hv_pointpillars_fpn_sbn-all_2x8_2x_lyft-3d.py) | 2x | 9.2 | | 14.9 | 15.1 | [model](https://download.openmmlab.com/mmdetection3d/v0.1.0_models/pointpillars/hv_pointpillars_fpn_sbn-all_2x8_2x_lyft-3d/hv_pointpillars_fpn_sbn-all_2x8_2x_lyft-3d_20210517_202818-fc6904c3.pth) \| [log](https://download.openmmlab.com/mmdetection3d/v0.1.0_models/pointpillars/hv_pointpillars_fpn_sbn-all_2x8_2x_lyft-3d/hv_pointpillars_fpn_sbn-all_2x8_2x_lyft-3d_20210517_202818.log.json) |
+| [RegNetX-400MF-FPN](./hv_pointpillars_regnet-400mf_fpn_sbn-all_4x8_2x_lyft-3d.py) | 2x | 13.0 | | 16.0 | 16.1 | [model](https://download.openmmlab.com/mmdetection3d/v0.1.0_models/regnet/hv_pointpillars_regnet-400mf_fpn_sbn-all_2x8_2x_lyft-3d/hv_pointpillars_regnet-400mf_fpn_sbn-all_2x8_2x_lyft-3d_20210521_115618-823dcf18.pth) \| [log](https://download.openmmlab.com/mmdetection3d/v0.1.0_models/regnet/hv_pointpillars_regnet-400mf_fpn_sbn-all_2x8_2x_lyft-3d/hv_pointpillars_regnet-400mf_fpn_sbn-all_2x8_2x_lyft-3d_20210521_115618.log.json) |
+
+## Citation
+
+```latex
+@article{radosavovic2020designing,
+ title={Designing Network Design Spaces},
+ author={Ilija Radosavovic and Raj Prateek Kosaraju and Ross Girshick and Kaiming He and Piotr Dollár},
+ year={2020},
+ eprint={2003.13678},
+ archivePrefix={arXiv},
+ primaryClass={cs.CV}
+}
+```
diff --git a/configs/regnet/hv_pointpillars_regnet-1.6gf_fpn_sbn-all_4x8_2x_nus-3d.py b/configs/regnet/hv_pointpillars_regnet-1.6gf_fpn_sbn-all_4x8_2x_nus-3d.py
new file mode 100644
index 0000000..0574be5
--- /dev/null
+++ b/configs/regnet/hv_pointpillars_regnet-1.6gf_fpn_sbn-all_4x8_2x_nus-3d.py
@@ -0,0 +1,24 @@
+_base_ = [
+ '../_base_/models/hv_pointpillars_fpn_nus.py',
+ '../_base_/datasets/nus-3d.py',
+ '../_base_/schedules/schedule_2x.py',
+ '../_base_/default_runtime.py',
+]
+# model settings
+model = dict(
+ type='MVXFasterRCNN',
+ pts_backbone=dict(
+ _delete_=True,
+ type='NoStemRegNet',
+ arch='regnetx_1.6gf',
+ init_cfg=dict(
+ type='Pretrained', checkpoint='open-mmlab://regnetx_1.6gf'),
+ out_indices=(1, 2, 3),
+ frozen_stages=-1,
+ strides=(1, 2, 2, 2),
+ base_channels=64,
+ stem_channels=64,
+ norm_cfg=dict(type='naiveSyncBN2d', eps=1e-3, momentum=0.01),
+ norm_eval=False,
+ style='pytorch'),
+ pts_neck=dict(in_channels=[168, 408, 912]))
diff --git a/configs/regnet/hv_pointpillars_regnet-400mf_fpn_sbn-all_2x8_2x_lyft-3d.py b/configs/regnet/hv_pointpillars_regnet-400mf_fpn_sbn-all_2x8_2x_lyft-3d.py
new file mode 100644
index 0000000..1f391a3
--- /dev/null
+++ b/configs/regnet/hv_pointpillars_regnet-400mf_fpn_sbn-all_2x8_2x_lyft-3d.py
@@ -0,0 +1,24 @@
+_base_ = [
+ '../_base_/models/hv_pointpillars_fpn_lyft.py',
+ '../_base_/datasets/lyft-3d.py',
+ '../_base_/schedules/schedule_2x.py',
+ '../_base_/default_runtime.py',
+]
+# model settings
+model = dict(
+ type='MVXFasterRCNN',
+ pts_backbone=dict(
+ _delete_=True,
+ type='NoStemRegNet',
+ arch=dict(w0=24, wa=24.48, wm=2.54, group_w=16, depth=22, bot_mul=1.0),
+ init_cfg=dict(
+ type='Pretrained', checkpoint='open-mmlab://regnetx_400mf'),
+ out_indices=(1, 2, 3),
+ frozen_stages=-1,
+ strides=(1, 2, 2, 2),
+ base_channels=64,
+ stem_channels=64,
+ norm_cfg=dict(type='naiveSyncBN2d', eps=1e-3, momentum=0.01),
+ norm_eval=False,
+ style='pytorch'),
+ pts_neck=dict(in_channels=[64, 160, 384]))
diff --git a/configs/regnet/hv_pointpillars_regnet-400mf_fpn_sbn-all_4x8_2x_nus-3d.py b/configs/regnet/hv_pointpillars_regnet-400mf_fpn_sbn-all_4x8_2x_nus-3d.py
new file mode 100644
index 0000000..884729c
--- /dev/null
+++ b/configs/regnet/hv_pointpillars_regnet-400mf_fpn_sbn-all_4x8_2x_nus-3d.py
@@ -0,0 +1,24 @@
+_base_ = [
+ '../_base_/models/hv_pointpillars_fpn_nus.py',
+ '../_base_/datasets/nus-3d.py',
+ '../_base_/schedules/schedule_2x.py',
+ '../_base_/default_runtime.py',
+]
+# model settings
+model = dict(
+ type='MVXFasterRCNN',
+ pts_backbone=dict(
+ _delete_=True,
+ type='NoStemRegNet',
+ arch=dict(w0=24, wa=24.48, wm=2.54, group_w=16, depth=22, bot_mul=1.0),
+ init_cfg=dict(
+ type='Pretrained', checkpoint='open-mmlab://regnetx_400mf'),
+ out_indices=(1, 2, 3),
+ frozen_stages=-1,
+ strides=(1, 2, 2, 2),
+ base_channels=64,
+ stem_channels=64,
+ norm_cfg=dict(type='naiveSyncBN2d', eps=1e-3, momentum=0.01),
+ norm_eval=False,
+ style='pytorch'),
+ pts_neck=dict(in_channels=[64, 160, 384]))
diff --git a/configs/regnet/hv_pointpillars_regnet-400mf_fpn_sbn-all_fp16_2x8_2x_nus-3d.py b/configs/regnet/hv_pointpillars_regnet-400mf_fpn_sbn-all_fp16_2x8_2x_nus-3d.py
new file mode 100644
index 0000000..e586365
--- /dev/null
+++ b/configs/regnet/hv_pointpillars_regnet-400mf_fpn_sbn-all_fp16_2x8_2x_nus-3d.py
@@ -0,0 +1,4 @@
+_base_ = './hv_pointpillars_regnet-400mf_fpn_sbn-all_4x8_2x_nus-3d.py'
+data = dict(samples_per_gpu=2, workers_per_gpu=2)
+# fp16 settings, the loss scale is specifically tuned to avoid Nan
+fp16 = dict(loss_scale=32.)
diff --git a/configs/regnet/hv_pointpillars_regnet-400mf_fpn_sbn-all_range100_2x8_2x_lyft-3d.py b/configs/regnet/hv_pointpillars_regnet-400mf_fpn_sbn-all_range100_2x8_2x_lyft-3d.py
new file mode 100644
index 0000000..fef308d
--- /dev/null
+++ b/configs/regnet/hv_pointpillars_regnet-400mf_fpn_sbn-all_range100_2x8_2x_lyft-3d.py
@@ -0,0 +1,24 @@
+_base_ = [
+ '../_base_/models/hv_pointpillars_fpn_range100_lyft.py',
+ '../_base_/datasets/range100_lyft-3d.py',
+ '../_base_/schedules/schedule_2x.py',
+ '../_base_/default_runtime.py',
+]
+# model settings
+model = dict(
+ type='MVXFasterRCNN',
+ pts_backbone=dict(
+ _delete_=True,
+ type='NoStemRegNet',
+ arch=dict(w0=24, wa=24.48, wm=2.54, group_w=16, depth=22, bot_mul=1.0),
+ init_cfg=dict(
+ type='Pretrained', checkpoint='open-mmlab://regnetx_400mf'),
+ out_indices=(1, 2, 3),
+ frozen_stages=-1,
+ strides=(1, 2, 2, 2),
+ base_channels=64,
+ stem_channels=64,
+ norm_cfg=dict(type='naiveSyncBN2d', eps=1e-3, momentum=0.01),
+ norm_eval=False,
+ style='pytorch'),
+ pts_neck=dict(in_channels=[64, 160, 384]))
diff --git a/configs/regnet/hv_pointpillars_regnet-400mf_secfpn_sbn-all_2x8_2x_lyft-3d.py b/configs/regnet/hv_pointpillars_regnet-400mf_secfpn_sbn-all_2x8_2x_lyft-3d.py
new file mode 100644
index 0000000..fb330d7
--- /dev/null
+++ b/configs/regnet/hv_pointpillars_regnet-400mf_secfpn_sbn-all_2x8_2x_lyft-3d.py
@@ -0,0 +1,39 @@
+_base_ = './hv_pointpillars_regnet-400mf_fpn_sbn-all_2x8_2x_lyft-3d.py'
+# model settings
+model = dict(
+ pts_neck=dict(
+ type='SECONDFPN',
+ _delete_=True,
+ norm_cfg=dict(type='naiveSyncBN2d', eps=1e-3, momentum=0.01),
+ in_channels=[64, 160, 384],
+ upsample_strides=[1, 2, 4],
+ out_channels=[128, 128, 128]),
+ pts_bbox_head=dict(
+ type='Anchor3DHead',
+ in_channels=384,
+ feat_channels=384,
+ anchor_generator=dict(
+ _delete_=True,
+ type='AlignedAnchor3DRangeGenerator',
+ ranges=[[-80, -80, -1.0715024, 80, 80, -1.0715024],
+ [-80, -80, -0.3033737, 80, 80, -0.3033737],
+ [-80, -80, -0.3519405, 80, 80, -0.3519405],
+ [-80, -80, -0.8871424, 80, 80, -0.8871424],
+ [-80, -80, -0.6276341, 80, 80, -0.6276341],
+ [-80, -80, -1.3220503, 80, 80, -1.3220503],
+ [-80, -80, -1.0709302, 80, 80, -1.0709302],
+ [-80, -80, -0.9122268, 80, 80, -0.9122268],
+ [-80, -80, -1.8012227, 80, 80, -1.8012227]],
+ sizes=[
+ [4.75, 1.92, 1.71], # car
+ [10.24, 2.84, 3.44], # truck
+ [12.70, 2.92, 3.42], # bus
+ [6.52, 2.42, 2.34], # emergency vehicle
+ [8.17, 2.75, 3.20], # other vehicle
+ [2.35, 0.96, 1.59], # motorcycle
+ [1.76, 0.63, 1.44], # bicycle
+ [0.80, 0.76, 1.76], # pedestrian
+ [0.73, 0.35, 0.50] # animal
+ ],
+ rotations=[0, 1.57],
+ reshape_out=True)))
diff --git a/configs/regnet/hv_pointpillars_regnet-400mf_secfpn_sbn-all_4x8_2x_nus-3d.py b/configs/regnet/hv_pointpillars_regnet-400mf_secfpn_sbn-all_4x8_2x_nus-3d.py
new file mode 100644
index 0000000..ef8996a
--- /dev/null
+++ b/configs/regnet/hv_pointpillars_regnet-400mf_secfpn_sbn-all_4x8_2x_nus-3d.py
@@ -0,0 +1,38 @@
+_base_ = './hv_pointpillars_regnet-400mf_fpn_sbn-all_4x8_2x_nus-3d.py'
+# model settings
+model = dict(
+ pts_neck=dict(
+ type='SECONDFPN',
+ _delete_=True,
+ norm_cfg=dict(type='naiveSyncBN2d', eps=1e-3, momentum=0.01),
+ in_channels=[64, 160, 384],
+ upsample_strides=[1, 2, 4],
+ out_channels=[128, 128, 128]),
+ pts_bbox_head=dict(
+ type='Anchor3DHead',
+ in_channels=384,
+ feat_channels=384,
+ anchor_generator=dict(
+ _delete_=True,
+ type='AlignedAnchor3DRangeGenerator',
+ ranges=[
+ [-49.6, -49.6, -1.80032795, 49.6, 49.6, -1.80032795],
+ [-49.6, -49.6, -1.74440365, 49.6, 49.6, -1.74440365],
+ [-49.6, -49.6, -1.68526504, 49.6, 49.6, -1.68526504],
+ [-49.6, -49.6, -1.67339111, 49.6, 49.6, -1.67339111],
+ [-49.6, -49.6, -1.61785072, 49.6, 49.6, -1.61785072],
+ [-49.6, -49.6, -1.80984986, 49.6, 49.6, -1.80984986],
+ [-49.6, -49.6, -1.763965, 49.6, 49.6, -1.763965],
+ ],
+ sizes=[
+ [4.60718145, 1.95017717, 1.72270761], # car
+ [6.73778078, 2.4560939, 2.73004906], # truck
+ [12.01320693, 2.87427237, 3.81509561], # trailer
+ [1.68452161, 0.60058911, 1.27192197], # bicycle
+ [0.7256437, 0.66344886, 1.75748069], # pedestrian
+ [0.40359262, 0.39694519, 1.06232151], # traffic_cone
+ [0.48578221, 2.49008838, 0.98297065], # barrier
+ ],
+ custom_values=[0, 0],
+ rotations=[0, 1.57],
+ reshape_out=True)))
diff --git a/configs/regnet/hv_pointpillars_regnet-400mf_secfpn_sbn-all_range100_2x8_2x_lyft-3d.py b/configs/regnet/hv_pointpillars_regnet-400mf_secfpn_sbn-all_range100_2x8_2x_lyft-3d.py
new file mode 100644
index 0000000..2af3719
--- /dev/null
+++ b/configs/regnet/hv_pointpillars_regnet-400mf_secfpn_sbn-all_range100_2x8_2x_lyft-3d.py
@@ -0,0 +1,40 @@
+_base_ = \
+ './hv_pointpillars_regnet-400mf_fpn_sbn-all_range100_2x8_2x_lyft-3d.py'
+# model settings
+model = dict(
+ pts_neck=dict(
+ type='SECONDFPN',
+ _delete_=True,
+ norm_cfg=dict(type='naiveSyncBN2d', eps=1e-3, momentum=0.01),
+ in_channels=[64, 160, 384],
+ upsample_strides=[1, 2, 4],
+ out_channels=[128, 128, 128]),
+ pts_bbox_head=dict(
+ type='Anchor3DHead',
+ in_channels=384,
+ feat_channels=384,
+ anchor_generator=dict(
+ _delete_=True,
+ type='AlignedAnchor3DRangeGenerator',
+ ranges=[[-100, -100, -1.0715024, 100, 100, -1.0715024],
+ [-100, -100, -0.3033737, 100, 100, -0.3033737],
+ [-100, -100, -0.3519405, 100, 100, -0.3519405],
+ [-100, -100, -0.8871424, 100, 100, -0.8871424],
+ [-100, -100, -0.6276341, 100, 100, -0.6276341],
+ [-100, -100, -1.3220503, 100, 100, -1.3220503],
+ [-100, -100, -1.0709302, 100, 100, -1.0709302],
+ [-100, -100, -0.9122268, 100, 100, -0.9122268],
+ [-100, -100, -1.8012227, 100, 100, -1.8012227]],
+ sizes=[
+ [4.75, 1.92, 1.71], # car
+ [10.24, 2.84, 3.44], # truck
+ [12.70, 2.92, 3.42], # bus
+ [6.52, 2.42, 2.34], # emergency vehicle
+ [8.17, 2.75, 3.20], # other vehicle
+ [2.35, 0.96, 1.59], # motorcycle
+ [1.76, 0.63, 1.44], # bicycle
+ [0.80, 0.76, 1.76], # pedestrian
+ [0.73, 0.35, 0.50] # animal
+ ],
+ rotations=[0, 1.57],
+ reshape_out=True)))
diff --git a/configs/regnet/metafile.yml b/configs/regnet/metafile.yml
new file mode 100644
index 0000000..18f13b1
--- /dev/null
+++ b/configs/regnet/metafile.yml
@@ -0,0 +1,85 @@
+Models:
+ - Name: hv_pointpillars_regnet-400mf_secfpn_sbn-all_4x8_2x_nus-3d
+ In Collection: PointPillars
+ Config: configs/regnet/hv_pointpillars_regnet-400mf_secfpn_sbn-all_4x8_2x_nus-3d.py
+ Metadata:
+ Training Data: nuScenes
+ Training Memory (GB): 16.4
+ Architecture:
+ - RegNetX
+ - Hard Voxelization
+ Results:
+ - Task: 3D Object Detection
+ Dataset: nuScenes
+ Metrics:
+ mAP: 41.2
+ NDS: 55.2
+ Weights: https://download.openmmlab.com/mmdetection3d/v0.1.0_models/regnet/hv_pointpillars_regnet-400mf_secfpn_sbn-all_4x8_2x_nus-3d/hv_pointpillars_regnet-400mf_secfpn_sbn-all_4x8_2x_nus-3d_20200620_230334-53044f32.pth
+
+ - Name: hv_pointpillars_regnet-400mf_fpn_sbn-all_4x8_2x_nus-3d
+ In Collection: PointPillars
+ Config: configs/regnet/hv_pointpillars_regnet-400mf_fpn_sbn-all_4x8_2x_nus-3d.py
+ Metadata:
+ Training Data: nuScenes
+ Training Memory (GB): 17.3
+ Architecture:
+ - RegNetX
+ - Hard Voxelization
+ Results:
+ - Task: 3D Object Detection
+ Dataset: nuScenes
+ Metrics:
+ mAP: 44.8
+ NDS: 56.4
+ Weights: https://download.openmmlab.com/mmdetection3d/v0.1.0_models/regnet/hv_pointpillars_regnet-400mf_fpn_sbn-all_4x8_2x_nus-3d/hv_pointpillars_regnet-400mf_fpn_sbn-all_4x8_2x_nus-3d_20200620_230239-c694dce7.pth
+
+ - Name: hv_pointpillars_regnet-1.6gf_fpn_sbn-all_4x8_2x_nus-3d
+ In Collection: PointPillars
+ Config: configs/regnet/hv_pointpillars_regnet-1.6gf_fpn_sbn-all_4x8_2x_nus-3d.py
+ Metadata:
+ Training Data: nuScenes
+ Training Memory (GB): 24.0
+ Architecture:
+ - RegNetX
+ - Hard Voxelization
+ Results:
+ - Task: 3D Object Detection
+ Dataset: nuScenes
+ Metrics:
+ mAP: 48.2
+ NDS: 59.3
+ Weights: https://download.openmmlab.com/mmdetection3d/v0.1.0_models/regnet/hv_pointpillars_regnet-1.6gf_fpn_sbn-all_4x8_2x_nus-3d/hv_pointpillars_regnet-1.6gf_fpn_sbn-all_4x8_2x_nus-3d_20200629_050311-dcd4e090.pth
+
+ - Name: hv_pointpillars_regnet-400mf_secfpn_sbn-all_2x8_2x_lyft-3d
+ In Collection: PointPillars
+ Config: configs/regnet/hv_pointpillars_regnet-400mf_secfpn_sbn-all_2x8_2x_lyft-3d.py
+ Metadata:
+ Training Data: Lyft
+ Training Memory (GB): 15.9
+ Architecture:
+ - RegNetX
+ - Hard Voxelization
+ Results:
+ - Task: 3D Object Detection
+ Dataset: Lyft
+ Metrics:
+ Private Score: 14.9
+ Public Score: 15.1
+ Weights: https://download.openmmlab.com/mmdetection3d/v0.1.0_models/regnet/hv_pointpillars_regnet-400mf_secfpn_sbn-all_2x8_2x_lyft-3d/hv_pointpillars_regnet-400mf_secfpn_sbn-all_2x8_2x_lyft-3d_20210524_092151-42513826.pth
+
+ - Name: hv_pointpillars_regnet-400mf_fpn_sbn-all_2x8_2x_lyft-3d
+ In Collection: PointPillars
+ Config: configs/regnet/hv_pointpillars_regnet-400mf_fpn_sbn-all_2x8_2x_lyft-3d.py
+ Metadata:
+ Training Data: Lyft
+ Training Memory (GB): 13.0
+ Architecture:
+ - RegNetX
+ - Hard Voxelization
+ Results:
+ - Task: 3D Object Detection
+ Dataset: Lyft
+ Metrics:
+ Private Score: 16.0
+ Public Score: 16.1
+ Weights: https://download.openmmlab.com/mmdetection3d/v0.1.0_models/regnet/hv_pointpillars_regnet-400mf_fpn_sbn-all_2x8_2x_lyft-3d/hv_pointpillars_regnet-400mf_fpn_sbn-all_2x8_2x_lyft-3d_20210521_115618-823dcf18.pth
diff --git a/configs/sassd/README.md b/configs/sassd/README.md
new file mode 100644
index 0000000..3a4444a
--- /dev/null
+++ b/configs/sassd/README.md
@@ -0,0 +1,28 @@
+# Structure Aware Single-stage 3D Object Detection from Point Cloud
+
+> [Structure Aware Single-stage 3D Object Detection from Point Cloud]([https://arxiv.org/abs/2104.02323](https://openaccess.thecvf.com/content_CVPR_2020/papers/He_Structure_Aware_Single-Stage_3D_Object_Detection_From_Point_Cloud_CVPR_2020_paper.pdf))
+
+
+
+## Abstract
+
+3D object detection from point cloud data plays an essential role in autonomous driving. Current single-stage detectors are efficient by progressively downscaling the 3D point clouds in a fully convolutional manner. However, the downscaled features inevitably lose spatial information and cannot make full use of the structure information of 3D point cloud, degrading their localization precision. In this work, we propose to improve the localization precision of single-stage detectors by explicitly leveraging the structure information of 3D point cloud. Specifically, we design an auxiliary network which converts the convolutional features in the backbone network back to point-level representations. The auxiliary network is jointly optimized, by two point-level supervisions, to guide the convolutional features in the backbone network to be aware of the object structure. The auxiliary network can be detached after training and therefore introduces no extra computation in the inference stage. Besides, considering that single-stage detectors suffer from the discordance between the predicted bounding boxes and corresponding classification confidences, we develop an efficient part-sensitive warping operation to align the confidences to the predicted bounding boxes. Our proposed detector ranks at the top of KITTI 3D/BEV detection leaderboards and runs at 25 FPS for inference.
+
+
+
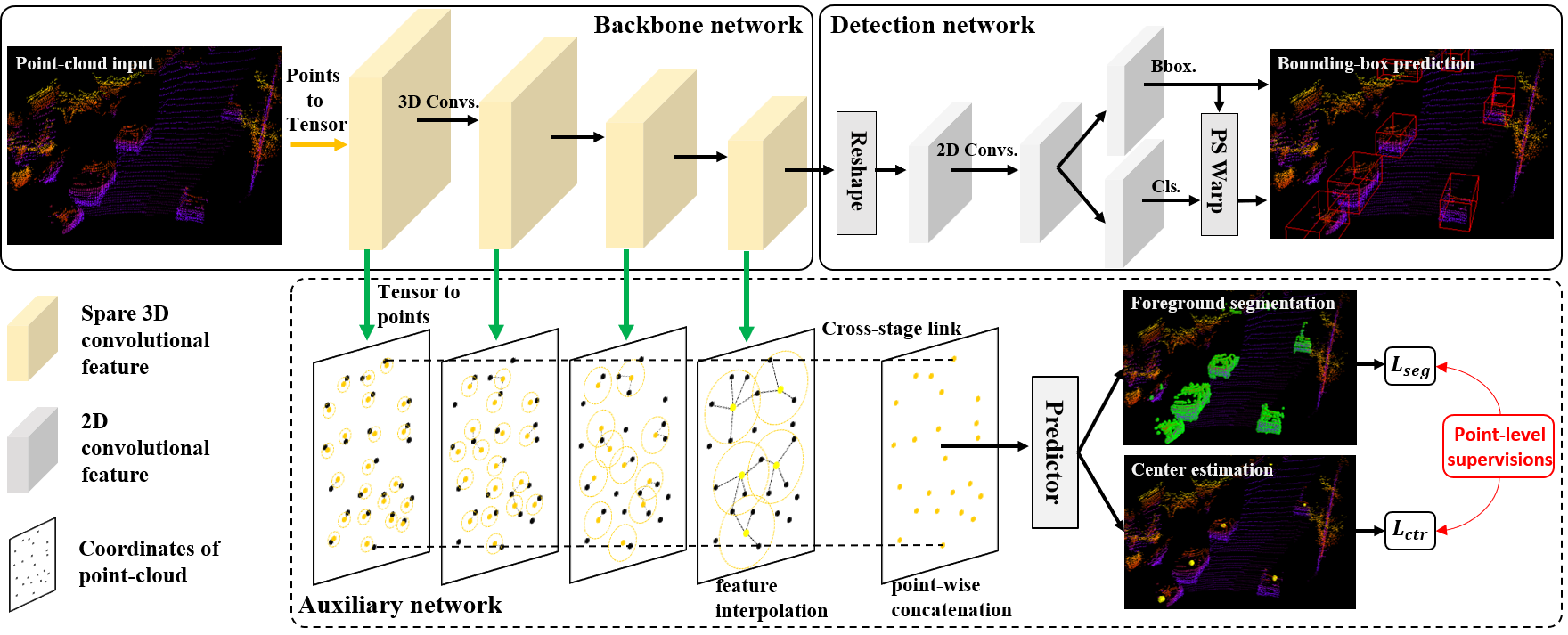
+
+
+## Introduction
+
+We implement SA-SSD and provide the results and checkpoints on KITTI dataset.
+
+## Citation
+
+```latex
+@InProceedings{he2020sassd,
+ title={Structure Aware Single-stage 3D Object Detection from Point Cloud},
+ author={He, Chenhang and Zeng, Hui and Huang, Jianqiang and Hua, Xian-Sheng and Zhang, Lei},
+ booktitle={Proceedings of the IEEE Conference on Computer Vision and Pattern Recognition},
+ year={2020}
+}
+```
diff --git a/configs/sassd/sassd_6x8_80e_kitti-3d-3class.py b/configs/sassd/sassd_6x8_80e_kitti-3d-3class.py
new file mode 100644
index 0000000..efc67c7
--- /dev/null
+++ b/configs/sassd/sassd_6x8_80e_kitti-3d-3class.py
@@ -0,0 +1,94 @@
+_base_ = [
+ '../_base_/datasets/kitti-3d-3class.py',
+ '../_base_/schedules/cyclic_40e.py', '../_base_/default_runtime.py'
+]
+
+voxel_size = [0.05, 0.05, 0.1]
+
+model = dict(
+ type='SASSD',
+ voxel_layer=dict(
+ max_num_points=5,
+ point_cloud_range=[0, -40, -3, 70.4, 40, 1],
+ voxel_size=voxel_size,
+ max_voxels=(16000, 40000)),
+ voxel_encoder=dict(type='HardSimpleVFE'),
+ middle_encoder=dict(
+ type='SparseEncoderSASSD',
+ in_channels=4,
+ sparse_shape=[41, 1600, 1408],
+ order=('conv', 'norm', 'act')),
+ backbone=dict(
+ type='SECOND',
+ in_channels=256,
+ layer_nums=[5, 5],
+ layer_strides=[1, 2],
+ out_channels=[128, 256]),
+ neck=dict(
+ type='SECONDFPN',
+ in_channels=[128, 256],
+ upsample_strides=[1, 2],
+ out_channels=[256, 256]),
+ bbox_head=dict(
+ type='Anchor3DHead',
+ num_classes=3,
+ in_channels=512,
+ feat_channels=512,
+ use_direction_classifier=True,
+ anchor_generator=dict(
+ type='Anchor3DRangeGenerator',
+ ranges=[
+ [0, -40.0, -0.6, 70.4, 40.0, -0.6],
+ [0, -40.0, -0.6, 70.4, 40.0, -0.6],
+ [0, -40.0, -1.78, 70.4, 40.0, -1.78],
+ ],
+ sizes=[[0.6, 0.8, 1.73], [0.6, 1.76, 1.73], [1.6, 3.9, 1.56]],
+ rotations=[0, 1.57],
+ reshape_out=False),
+ diff_rad_by_sin=True,
+ bbox_coder=dict(type='DeltaXYZWLHRBBoxCoder'),
+ loss_cls=dict(
+ type='FocalLoss',
+ use_sigmoid=True,
+ gamma=2.0,
+ alpha=0.25,
+ loss_weight=1.0),
+ loss_bbox=dict(type='SmoothL1Loss', beta=1.0 / 9.0, loss_weight=2.0),
+ loss_dir=dict(
+ type='CrossEntropyLoss', use_sigmoid=False, loss_weight=0.2)),
+ # model training and testing settings
+ train_cfg=dict(
+ assigner=[
+ dict( # for Pedestrian
+ type='MaxIoUAssigner',
+ iou_calculator=dict(type='BboxOverlapsNearest3D'),
+ pos_iou_thr=0.35,
+ neg_iou_thr=0.2,
+ min_pos_iou=0.2,
+ ignore_iof_thr=-1),
+ dict( # for Cyclist
+ type='MaxIoUAssigner',
+ iou_calculator=dict(type='BboxOverlapsNearest3D'),
+ pos_iou_thr=0.35,
+ neg_iou_thr=0.2,
+ min_pos_iou=0.2,
+ ignore_iof_thr=-1),
+ dict( # for Car
+ type='MaxIoUAssigner',
+ iou_calculator=dict(type='BboxOverlapsNearest3D'),
+ pos_iou_thr=0.6,
+ neg_iou_thr=0.45,
+ min_pos_iou=0.45,
+ ignore_iof_thr=-1),
+ ],
+ allowed_border=0,
+ pos_weight=-1,
+ debug=False),
+ test_cfg=dict(
+ use_rotate_nms=True,
+ nms_across_levels=False,
+ nms_thr=0.01,
+ score_thr=0.1,
+ min_bbox_size=0,
+ nms_pre=100,
+ max_num=50))
diff --git a/configs/second/README.md b/configs/second/README.md
new file mode 100644
index 0000000..1aa9650
--- /dev/null
+++ b/configs/second/README.md
@@ -0,0 +1,54 @@
+# Second: Sparsely embedded convolutional detection
+
+> [SECOND: Sparsely Embedded Convolutional Detection](https://www.mdpi.com/1424-8220/18/10/3337)
+
+
+
+## Abstract
+
+LiDAR-based or RGB-D-based object detection is used in numerous applications, ranging from autonomous driving to robot vision. Voxel-based 3D convolutional networks have been used for some time to enhance the retention of information when processing point cloud LiDAR data. However, problems remain, including a slow inference speed and low orientation estimation performance. We therefore investigate an improved sparse convolution method for such networks, which significantly increases the speed of both training and inference. We also introduce a new form of angle loss regression to improve the orientation estimation performance and a new data augmentation approach that can enhance the convergence speed and performance. The proposed network produces state-of-the-art results on the KITTI 3D object detection benchmarks while maintaining a fast inference speed.
+
+
+
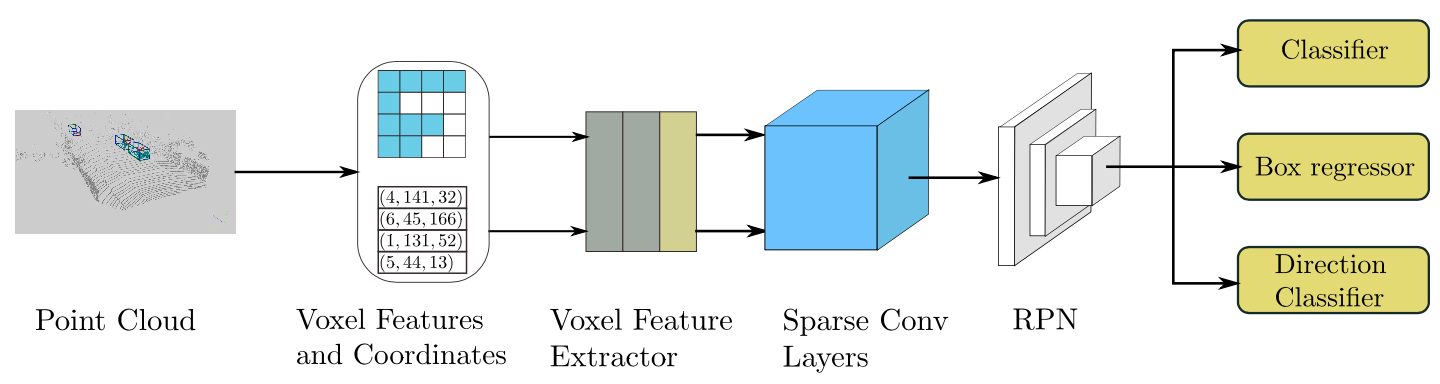
+
+
+## Introduction
+
+We implement SECOND and provide the results and checkpoints on KITTI dataset.
+
+## Results and models
+
+### KITTI
+
+| Backbone | Class | Lr schd | Mem (GB) | Inf time (fps) | mAP | Download |
+| :-----------------------------------------------------------------: | :-----: | :--------: | :------: | :------------: | :---: | :----------------------------------------------------------------------------------------------------------------------------------------------------------------------------------------------------------------------------------------------------------------------------------------------------------------------------------------------------------------------------------------------: |
+| [SECFPN](./hv_second_secfpn_6x8_80e_kitti-3d-car.py) | Car | cyclic 80e | 5.4 | | 79.07 | [model](https://download.openmmlab.com/mmdetection3d/v0.1.0_models/second/hv_second_secfpn_6x8_80e_kitti-3d-car/hv_second_secfpn_6x8_80e_kitti-3d-car_20200620_230238-393f000c.pth) \| [log](https://download.openmmlab.com/mmdetection3d/v0.1.0_models/second/hv_second_secfpn_6x8_80e_kitti-3d-car/hv_second_secfpn_6x8_80e_kitti-3d-car_20200620_230238.log.json) |
+| [SECFPN (FP16)](./hv_second_secfpn_fp16_6x8_80e_kitti-3d-car.py) | Car | cyclic 80e | 2.9 | | 78.72 | [model](https://download.openmmlab.com/mmdetection3d/v0.1.0_models/fp16/hv_second_secfpn_fp16_6x8_80e_kitti-3d-car/hv_second_secfpn_fp16_6x8_80e_kitti-3d-car_20200924_211301-1f5ad833.pth)\| [log](https://download.openmmlab.com/mmdetection3d/v0.1.0_models/fp16/hv_second_secfpn_fp16_6x8_80e_kitti-3d-car/hv_second_secfpn_fp16_6x8_80e_kitti-3d-car_20200924_211301.log.json) |
+| [SECFPN](./hv_second_secfpn_6x8_80e_kitti-3d-3class.py) | 3 Class | cyclic 80e | 5.4 | | 65.74 | [model](https://download.openmmlab.com/mmdetection3d/v1.0.0_models/second/hv_second_secfpn_6x8_80e_kitti-3d-3class/hv_second_secfpn_6x8_80e_kitti-3d-3class_20210831_022017-ae782e87.pth) \| [log](https://download.openmmlab.com/mmdetection3d/v1.0.0_models/second/hv_second_secfpn_6x8_80e_kitti-3d-3class/hv_second_secfpn_6x8_80e_kitti-3d-3class_20210831_022017log.json) |
+| [SECFPN (FP16)](./hv_second_secfpn_fp16_6x8_80e_kitti-3d-3class.py) | 3 Class | cyclic 80e | 2.9 | | 67.4 | [model](https://download.openmmlab.com/mmdetection3d/v0.1.0_models/fp16/hv_second_secfpn_fp16_6x8_80e_kitti-3d-3class/hv_second_secfpn_fp16_6x8_80e_kitti-3d-3class_20200925_110059-05f67bdf.pth) \| [log](https://download.openmmlab.com/mmdetection3d/v0.1.0_models/fp16/hv_second_secfpn_fp16_6x8_80e_kitti-3d-3class/hv_second_secfpn_fp16_6x8_80e_kitti-3d-3class_20200925_110059.log.json) |
+
+### Waymo
+
+| Backbone | Load Interval | Class | Lr schd | Mem (GB) | Inf time (fps) | mAP@L1 | mAPH@L1 | mAP@L2 | **mAPH@L2** | Download |
+| :-----------------------------------------------------------: | :-----------: | :-----: | :-----: | :------: | :------------: | :----: | :-----: | :----: | :---------: | :-------------------------------------------------------------------------------------------------------------------------------------------------------------------------------------------: |
+| [SECFPN](./hv_second_secfpn_sbn_2x16_2x_waymoD5-3d-3class.py) | 5 | 3 Class | 2x | 8.12 | | 65.3 | 61.7 | 58.9 | 55.7 | [log](https://download.openmmlab.com/mmdetection3d/v0.1.0_models/second/hv_second_secfpn_sbn_4x8_2x_waymoD5-3d-3class/hv_second_secfpn_sbn_4x8_2x_waymoD5-3d-3class_20201115_112448.log.json) |
+| above @ Car | | | 2x | 8.12 | | 67.1 | 66.6 | 58.7 | 58.2 | |
+| above @ Pedestrian | | | 2x | 8.12 | | 68.1 | 59.1 | 59.5 | 51.5 | |
+| above @ Cyclist | | | 2x | 8.12 | | 60.7 | 59.5 | 58.4 | 57.3 | |
+
+Note:
+
+- See more details about metrics and data split on Waymo [HERE](https://github.com/open-mmlab/mmdetection3d/tree/master/configs/pointpillars). For implementation details, we basically follow the original settings. All of these results are achieved without bells-and-whistles, e.g. ensemble, multi-scale training and test augmentation.
+- `FP16` means Mixed Precision (FP16) is adopted in training.
+
+## Citation
+
+```latex
+@article{yan2018second,
+ title={Second: Sparsely embedded convolutional detection},
+ author={Yan, Yan and Mao, Yuxing and Li, Bo},
+ journal={Sensors},
+ year={2018},
+ publisher={Multidisciplinary Digital Publishing Institute}
+}
+```
diff --git a/configs/second/hv_second_secfpn_6x8_80e_kitti-3d-3class.py b/configs/second/hv_second_secfpn_6x8_80e_kitti-3d-3class.py
new file mode 100644
index 0000000..0f28921
--- /dev/null
+++ b/configs/second/hv_second_secfpn_6x8_80e_kitti-3d-3class.py
@@ -0,0 +1,5 @@
+_base_ = [
+ '../_base_/models/hv_second_secfpn_kitti.py',
+ '../_base_/datasets/kitti-3d-3class.py',
+ '../_base_/schedules/cyclic_40e.py', '../_base_/default_runtime.py'
+]
diff --git a/configs/second/hv_second_secfpn_6x8_80e_kitti-3d-car.py b/configs/second/hv_second_secfpn_6x8_80e_kitti-3d-car.py
new file mode 100644
index 0000000..9ab7350
--- /dev/null
+++ b/configs/second/hv_second_secfpn_6x8_80e_kitti-3d-car.py
@@ -0,0 +1,30 @@
+_base_ = [
+ '../_base_/models/hv_second_secfpn_kitti.py',
+ '../_base_/datasets/kitti-3d-car.py', '../_base_/schedules/cyclic_40e.py',
+ '../_base_/default_runtime.py'
+]
+point_cloud_range = [0, -40, -3, 70.4, 40, 1]
+model = dict(
+ bbox_head=dict(
+ type='Anchor3DHead',
+ num_classes=1,
+ anchor_generator=dict(
+ _delete_=True,
+ type='Anchor3DRangeGenerator',
+ ranges=[[0, -40.0, -1.78, 70.4, 40.0, -1.78]],
+ sizes=[[3.9, 1.6, 1.56]],
+ rotations=[0, 1.57],
+ reshape_out=True)),
+ # model training and testing settings
+ train_cfg=dict(
+ _delete_=True,
+ assigner=dict(
+ type='MaxIoUAssigner',
+ iou_calculator=dict(type='BboxOverlapsNearest3D'),
+ pos_iou_thr=0.6,
+ neg_iou_thr=0.45,
+ min_pos_iou=0.45,
+ ignore_iof_thr=-1),
+ allowed_border=0,
+ pos_weight=-1,
+ debug=False))
diff --git a/configs/second/hv_second_secfpn_fp16_6x8_80e_kitti-3d-3class.py b/configs/second/hv_second_secfpn_fp16_6x8_80e_kitti-3d-3class.py
new file mode 100644
index 0000000..bf0336a
--- /dev/null
+++ b/configs/second/hv_second_secfpn_fp16_6x8_80e_kitti-3d-3class.py
@@ -0,0 +1,3 @@
+_base_ = './hv_second_secfpn_6x8_80e_kitti-3d-3class.py'
+# fp16 settings
+fp16 = dict(loss_scale=512.)
diff --git a/configs/second/hv_second_secfpn_fp16_6x8_80e_kitti-3d-car.py b/configs/second/hv_second_secfpn_fp16_6x8_80e_kitti-3d-car.py
new file mode 100644
index 0000000..efba553
--- /dev/null
+++ b/configs/second/hv_second_secfpn_fp16_6x8_80e_kitti-3d-car.py
@@ -0,0 +1,3 @@
+_base_ = './hv_second_secfpn_6x8_80e_kitti-3d-car.py'
+# fp16 settings
+fp16 = dict(loss_scale=512.)
diff --git a/configs/second/hv_second_secfpn_sbn_2x16_2x_waymoD5-3d-3class.py b/configs/second/hv_second_secfpn_sbn_2x16_2x_waymoD5-3d-3class.py
new file mode 100644
index 0000000..758827f
--- /dev/null
+++ b/configs/second/hv_second_secfpn_sbn_2x16_2x_waymoD5-3d-3class.py
@@ -0,0 +1,112 @@
+_base_ = [
+ '../_base_/models/hv_second_secfpn_waymo.py',
+ '../_base_/datasets/waymoD5-3d-3class.py',
+ '../_base_/schedules/schedule_2x.py',
+ '../_base_/default_runtime.py',
+]
+
+dataset_type = 'WaymoDataset'
+data_root = 'data/waymo/kitti_format/'
+class_names = ['Car', 'Pedestrian', 'Cyclist']
+point_cloud_range = [-76.8, -51.2, -2, 76.8, 51.2, 4]
+input_modality = dict(use_lidar=True, use_camera=False)
+
+db_sampler = dict(
+ data_root=data_root,
+ info_path=data_root + 'waymo_dbinfos_train.pkl',
+ rate=1.0,
+ prepare=dict(
+ filter_by_difficulty=[-1],
+ filter_by_min_points=dict(Car=5, Pedestrian=5, Cyclist=5)),
+ classes=class_names,
+ sample_groups=dict(Car=15, Pedestrian=10, Cyclist=10),
+ points_loader=dict(
+ type='LoadPointsFromFile',
+ coord_type='LIDAR',
+ load_dim=6,
+ use_dim=[0, 1, 2, 3, 4]))
+
+train_pipeline = [
+ dict(type='LoadPointsFromFile', coord_type='LIDAR', load_dim=6, use_dim=5),
+ dict(type='LoadAnnotations3D', with_bbox_3d=True, with_label_3d=True),
+ dict(type='ObjectSample', db_sampler=db_sampler),
+ dict(
+ type='RandomFlip3D',
+ sync_2d=False,
+ flip_ratio_bev_horizontal=0.5,
+ flip_ratio_bev_vertical=0.5),
+ dict(
+ type='GlobalRotScaleTrans',
+ rot_range=[-0.78539816, 0.78539816],
+ scale_ratio_range=[0.95, 1.05]),
+ dict(type='PointsRangeFilter', point_cloud_range=point_cloud_range),
+ dict(type='ObjectRangeFilter', point_cloud_range=point_cloud_range),
+ dict(type='PointShuffle'),
+ dict(type='DefaultFormatBundle3D', class_names=class_names),
+ dict(type='Collect3D', keys=['points', 'gt_bboxes_3d', 'gt_labels_3d'])
+]
+
+test_pipeline = [
+ dict(type='LoadPointsFromFile', coord_type='LIDAR', load_dim=6, use_dim=5),
+ dict(
+ type='MultiScaleFlipAug3D',
+ img_scale=(1333, 800),
+ pts_scale_ratio=1,
+ flip=False,
+ transforms=[
+ dict(
+ type='GlobalRotScaleTrans',
+ rot_range=[0, 0],
+ scale_ratio_range=[1., 1.],
+ translation_std=[0, 0, 0]),
+ dict(type='RandomFlip3D'),
+ dict(
+ type='PointsRangeFilter', point_cloud_range=point_cloud_range),
+ dict(
+ type='DefaultFormatBundle3D',
+ class_names=class_names,
+ with_label=False),
+ dict(type='Collect3D', keys=['points'])
+ ])
+]
+
+data = dict(
+ samples_per_gpu=4,
+ workers_per_gpu=4,
+ train=dict(
+ type='RepeatDataset',
+ times=2,
+ dataset=dict(
+ type=dataset_type,
+ data_root=data_root,
+ ann_file=data_root + 'waymo_infos_train.pkl',
+ split='training',
+ pipeline=train_pipeline,
+ modality=input_modality,
+ classes=class_names,
+ test_mode=False,
+ # we use box_type_3d='LiDAR' in kitti and nuscenes dataset
+ # and box_type_3d='Depth' in sunrgbd and scannet dataset.
+ box_type_3d='LiDAR',
+ # load one frame every five frames
+ load_interval=5)),
+ val=dict(
+ type=dataset_type,
+ data_root=data_root,
+ ann_file=data_root + 'waymo_infos_val.pkl',
+ split='training',
+ pipeline=test_pipeline,
+ modality=input_modality,
+ classes=class_names,
+ test_mode=True,
+ box_type_3d='LiDAR'),
+ test=dict(
+ type=dataset_type,
+ data_root=data_root,
+ ann_file=data_root + 'waymo_infos_val.pkl',
+ split='training',
+ pipeline=test_pipeline,
+ modality=input_modality,
+ classes=class_names,
+ test_mode=True,
+ box_type_3d='LiDAR'))
diff --git a/configs/second/metafile.yml b/configs/second/metafile.yml
new file mode 100644
index 0000000..5b68fe9
--- /dev/null
+++ b/configs/second/metafile.yml
@@ -0,0 +1,97 @@
+Collections:
+ - Name: SECOND
+ Metadata:
+ Training Techniques:
+ - AdamW
+ Architecture:
+ - Hard Voxelization
+ Paper:
+ URL: https://www.mdpi.com/1424-8220/18/10/3337
+ Title: 'SECOND: Sparsely Embedded Convolutional Detection'
+ README: configs/second/README.md
+ Code:
+ URL: https://github.com/open-mmlab/mmdetection3d/blob/master/mmdet3d/models/backbones/second.py#L11
+ Version: v0.5.0
+
+Models:
+ - Name: hv_second_secfpn_6x8_80e_kitti-3d-car
+ In Collection: SECOND
+ Config: configs/second/hv_second_secfpn_6x8_80e_kitti-3d-car.py
+ Metadata:
+ Training Data: KITTI
+ Training Memory (GB): 5.4
+ Training Resources: 8x V100 GPUs
+ Results:
+ - Task: 3D Object Detection
+ Dataset: KITTI
+ Metrics:
+ mAP: 79.07
+ Weights: https://download.openmmlab.com/mmdetection3d/v0.1.0_models/second/hv_second_secfpn_6x8_80e_kitti-3d-car/hv_second_secfpn_6x8_80e_kitti-3d-car_20200620_230238-393f000c.pth
+
+ - Name: hv_second_secfpn_6x8_80e_kitti-3d-3class
+ In Collection: SECOND
+ Config: configs/second/hv_second_secfpn_6x8_80e_kitti-3d-3class.py
+ Metadata:
+ Training Data: KITTI
+ Training Memory (GB): 5.4
+ Training Resources: 8x V100 GPUs
+ Results:
+ - Task: 3D Object Detection
+ Dataset: KITTI
+ Metrics:
+ mAP: 65.74
+ Weights: https://download.openmmlab.com/mmdetection3d/v1.0.0_models/second/hv_second_secfpn_6x8_80e_kitti-3d-3class/hv_second_secfpn_6x8_80e_kitti-3d-3class_20210831_022017-ae782e87.pth
+
+ - Name: hv_second_secfpn_sbn_2x16_2x_waymoD5-3d-3class
+ In Collection: SECOND
+ Config: configs/second/hv_second_secfpn_sbn_2x16_2x_waymoD5-3d-3class.py
+ Metadata:
+ Training Data: Waymo
+ Training Memory (GB): 8.12
+ Training Resources: 8x GeForce GTX 1080 Ti
+ Results:
+ - Task: 3D Object Detection
+ Dataset: Waymo
+ Metrics:
+ mAP@L1: 65.3
+ mAPH@L1: 61.7
+ mAP@L2: 58.9
+ mAPH@L2: 55.7
+
+ - Name: hv_second_secfpn_fp16_6x8_80e_kitti-3d-car
+ In Collection: SECOND
+ Config: configs/second/hv_second_secfpn_fp16_6x8_80e_kitti-3d-car.py
+ Metadata:
+ Training Techniques:
+ - AdamW
+ - Mixed Precision Training
+ Training Resources: 8x TITAN Xp
+ Training Data: KITTI
+ Training Memory (GB): 2.9
+ Results:
+ - Task: 3D Object Detection
+ Dataset: KITTI
+ Metrics:
+ mAP: 78.72
+ Weights: https://download.openmmlab.com/mmdetection3d/v0.1.0_models/fp16/hv_second_secfpn_fp16_6x8_80e_kitti-3d-car/hv_second_secfpn_fp16_6x8_80e_kitti-3d-car_20200924_211301-1f5ad833.pth
+ Code:
+ Version: v0.7.0
+
+ - Name: hv_second_secfpn_fp16_6x8_80e_kitti-3d-3class
+ In Collection: SECOND
+ Config: configs/second/hv_second_secfpn_fp16_6x8_80e_kitti-3d-3class.py
+ Metadata:
+ Training Techniques:
+ - AdamW
+ - Mixed Precision Training
+ Training Resources: 8x TITAN Xp
+ Training Data: KITTI
+ Training Memory (GB): 2.9
+ Results:
+ - Task: 3D Object Detection
+ Dataset: KITTI
+ Metrics:
+ mAP: 67.4
+ Weights: https://download.openmmlab.com/mmdetection3d/v0.1.0_models/fp16/hv_second_secfpn_fp16_6x8_80e_kitti-3d-3class/hv_second_secfpn_fp16_6x8_80e_kitti-3d-3class_20200925_110059-05f67bdf.pth
+ Code:
+ Version: v0.7.0
diff --git a/configs/smoke/README.md b/configs/smoke/README.md
new file mode 100644
index 0000000..8d91314
--- /dev/null
+++ b/configs/smoke/README.md
@@ -0,0 +1,47 @@
+# SMOKE: Single-Stage Monocular 3D Object Detection via Keypoint Estimation
+
+> [SMOKE: Single-Stage Monocular 3D Object Detection via Keypoint Estimation](https://arxiv.org/abs/2002.10111)
+
+
+
+## Abstract
+
+Estimating 3D orientation and translation of objects is essential for infrastructure-less autonomous navigation and driving. In case of monocular vision, successful methods have been mainly based on two ingredients: (i) a network generating 2D region proposals, (ii) a R-CNN structure predicting 3D object pose by utilizing the acquired regions of interest. We argue that the 2D detection network is redundant and introduces non-negligible noise for 3D detection. Hence, we propose a novel 3D object detection method, named SMOKE, in this paper that predicts a 3D bounding box for each detected object by combining a single keypoint estimate with regressed 3D variables. As a second contribution, we propose a multi-step disentangling approach for constructing the 3D bounding box, which significantly improves both training convergence and detection accuracy. In contrast to previous 3D detection techniques, our method does not require complicated pre/post-processing, extra data, and a refinement stage. Despite of its structural simplicity, our proposed SMOKE network outperforms all existing monocular 3D detection methods on the KITTI dataset, giving the best state-of-the-art result on both 3D object detection and Bird's eye view evaluation.
+
+
+
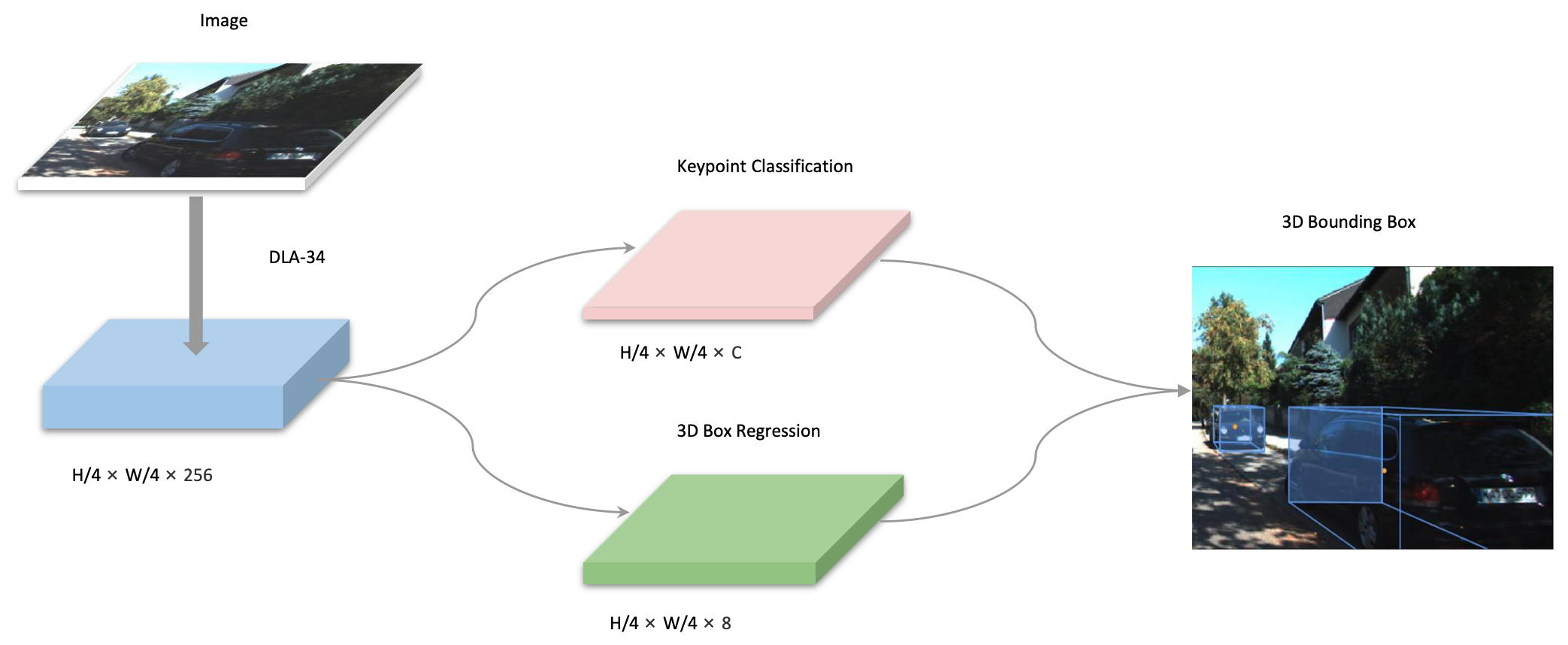
+
+
+## Introduction
+
+We implement SMOKE and provide the results and checkpoints on KITTI dataset.
+
+## Results and models
+
+### KITTI
+
+| Backbone | Lr schd | Mem (GB) | Inf time (fps) | mAP | Download |
+| :------------------------------------------------------------------: | :-----: | :------: | :------------: | :---: | :----------------------------------------------------------------------------------------------------------------------------------------------------------------------------------------------------------------------------------------------------------------------------------------------------------------------: |
+| [DLA34](./smoke_dla34_pytorch_dlaneck_gn-all_8x4_6x_kitti-mono3d.py) | 6x | 9.64 | | 13.85 | [model](https://download.openmmlab.com/mmdetection3d/v0.1.0_models/smoke/smoke_dla34_pytorch_dlaneck_gn-all_8x4_6x_kitti-mono3d_20210929_015553-d46d9bb0.pth) \| [log](https://download.openmmlab.com/mmdetection3d/v0.1.0_models/smoke/smoke_dla34_pytorch_dlaneck_gn-all_8x4_6x_kitti-mono3d_20210929_015553.log.json) |
+
+Note: mAP represents Car moderate 3D strict AP11 results.
+
+Detailed performance on KITTI 3D detection (3D/BEV) is as follows, evaluated by AP11 metric:
+
+| | Easy | Moderate | Hard |
+| ---------- | :-----------: | :-----------: | :-----------: |
+| Car | 16.92 / 22.97 | 13.85 / 18.32 | 11.90 / 15.88 |
+| Pedestrian | 11.13 / 12.61 | 11.10 / 11.32 | 10.67 / 11.14 |
+| Cyclist | 0.99 / 1.47 | 0.54 / 0.65 | 0.55 / 0.67 |
+
+## Citation
+
+```latex
+@inproceedings{liu2020smoke,
+ title={Smoke: Single-stage monocular 3d object detection via keypoint estimation},
+ author={Liu, Zechen and Wu, Zizhang and T{\'o}th, Roland},
+ booktitle={Proceedings of the IEEE/CVF Conference on Computer Vision and Pattern Recognition Workshops},
+ pages={996--997},
+ year={2020}
+}
+```
diff --git a/configs/smoke/metafile.yml b/configs/smoke/metafile.yml
new file mode 100644
index 0000000..df956e4
--- /dev/null
+++ b/configs/smoke/metafile.yml
@@ -0,0 +1,30 @@
+Collections:
+ - Name: SMOKE
+ Metadata:
+ Training Data: KITTI
+ Training Techniques:
+ - Adam
+ Training Resources: 4x V100 GPUS
+ Architecture:
+ - SMOKEMono3DHead
+ - DLA
+ Paper:
+ URL: https://arxiv.org/abs/2002.10111
+ Title: 'SMOKE: Single-Stage Monocular 3D Object Detection via Keypoint Estimation'
+ README: configs/smoke/README.md
+ Code:
+ URL: https://github.com/open-mmlab/mmdetection3d/blob/v1.0.0.dev0/mmdet3d/models/detectors/smoke_mono3d.py#L7
+ Version: v1.0.0
+
+Models:
+ - Name: smoke_dla34_pytorch_dlaneck_gn-all_8x4_6x_kitti-mono3d
+ In Collection: SMOKE
+ Config: configs/smoke/smoke_dla34_pytorch_dlaneck_gn-all_8x4_6x_kitti-mono3d.py
+ Metadata:
+ Training Memory (GB): 9.6
+ Results:
+ - Task: 3D Object Detection
+ Dataset: KITTI
+ Metrics:
+ mAP: 13.8
+ Weights: https://download.openmmlab.com/mmdetection3d/v0.1.0_models/smoke/smoke_dla34_pytorch_dlaneck_gn-all_8x4_6x_kitti-mono3d_20210929_015553-d46d9bb0.pth
diff --git a/configs/smoke/smoke_dla34_pytorch_dlaneck_gn-all_8x4_6x_kitti-mono3d.py b/configs/smoke/smoke_dla34_pytorch_dlaneck_gn-all_8x4_6x_kitti-mono3d.py
new file mode 100644
index 0000000..c802ce3
--- /dev/null
+++ b/configs/smoke/smoke_dla34_pytorch_dlaneck_gn-all_8x4_6x_kitti-mono3d.py
@@ -0,0 +1,64 @@
+_base_ = [
+ '../_base_/datasets/kitti-mono3d.py', '../_base_/models/smoke.py',
+ '../_base_/default_runtime.py'
+]
+
+# optimizer
+optimizer = dict(type='Adam', lr=2.5e-4)
+optimizer_config = dict(grad_clip=None)
+lr_config = dict(policy='step', warmup=None, step=[50])
+
+# runtime settings
+runner = dict(type='EpochBasedRunner', max_epochs=72)
+log_config = dict(interval=10)
+
+find_unused_parameters = True
+class_names = ['Pedestrian', 'Cyclist', 'Car']
+img_norm_cfg = dict(
+ mean=[123.675, 116.28, 103.53], std=[58.395, 57.12, 57.375], to_rgb=True)
+train_pipeline = [
+ dict(type='LoadImageFromFileMono3D'),
+ dict(
+ type='LoadAnnotations3D',
+ with_bbox=True,
+ with_label=True,
+ with_attr_label=False,
+ with_bbox_3d=True,
+ with_label_3d=True,
+ with_bbox_depth=True),
+ dict(type='RandomFlip3D', flip_ratio_bev_horizontal=0.5),
+ dict(type='RandomShiftScale', shift_scale=(0.2, 0.4), aug_prob=0.3),
+ dict(type='AffineResize', img_scale=(1280, 384), down_ratio=4),
+ dict(type='Normalize', **img_norm_cfg),
+ dict(type='Pad', size_divisor=32),
+ dict(type='DefaultFormatBundle3D', class_names=class_names),
+ dict(
+ type='Collect3D',
+ keys=[
+ 'img', 'gt_bboxes', 'gt_labels', 'gt_bboxes_3d', 'gt_labels_3d',
+ 'centers2d', 'depths'
+ ]),
+]
+test_pipeline = [
+ dict(type='LoadImageFromFileMono3D'),
+ dict(
+ type='MultiScaleFlipAug',
+ img_scale=(1280, 384),
+ flip=False,
+ transforms=[
+ dict(type='AffineResize', img_scale=(1280, 384), down_ratio=4),
+ dict(type='Normalize', **img_norm_cfg),
+ dict(type='Pad', size_divisor=32),
+ dict(
+ type='DefaultFormatBundle3D',
+ class_names=class_names,
+ with_label=False),
+ dict(type='Collect3D', keys=['img']),
+ ])
+]
+data = dict(
+ samples_per_gpu=8,
+ workers_per_gpu=4,
+ train=dict(pipeline=train_pipeline),
+ val=dict(pipeline=test_pipeline),
+ test=dict(pipeline=test_pipeline))
diff --git a/configs/ssn/README.md b/configs/ssn/README.md
new file mode 100644
index 0000000..dad03f8
--- /dev/null
+++ b/configs/ssn/README.md
@@ -0,0 +1,53 @@
+# SSN: Shape Signature Networks for Multi-class Object Detection from Point Clouds
+
+> [SSN: Shape Signature Networks for Multi-class Object Detection from Point Clouds](https://arxiv.org/abs/2004.02774)
+
+
+
+## Abstract
+
+Multi-class 3D object detection aims to localize and classify objects of multiple categories from point clouds. Due to the nature of point clouds, i.e. unstructured, sparse and noisy, some features benefit-ting multi-class discrimination are underexploited, such as shape information. In this paper, we propose a novel 3D shape signature to explore the shape information from point clouds. By incorporating operations of symmetry, convex hull and chebyshev fitting, the proposed shape sig-nature is not only compact and effective but also robust to the noise, which serves as a soft constraint to improve the feature capability of multi-class discrimination. Based on the proposed shape signature, we develop the shape signature networks (SSN) for 3D object detection, which consist of pyramid feature encoding part, shape-aware grouping heads and explicit shape encoding objective. Experiments show that the proposed method performs remarkably better than existing methods on two large-scale datasets. Furthermore, our shape signature can act as a plug-and-play component and ablation study shows its effectiveness and good scalability.
+
+
+
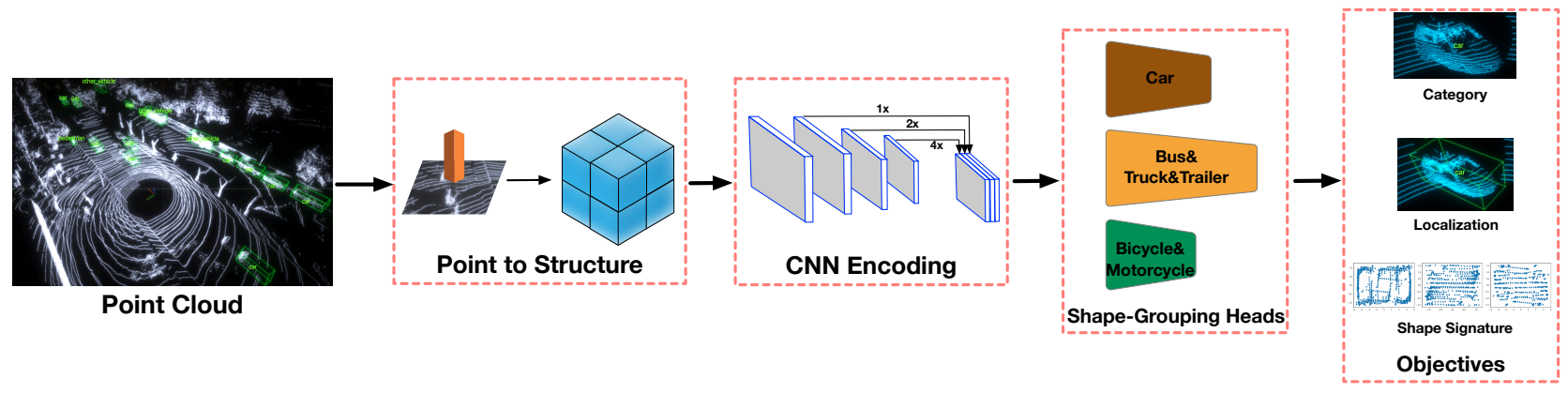
+
+
+## Introduction
+
+We implement PointPillars with Shape-aware grouping heads used in the SSN and provide the results and checkpoints on the nuScenes and Lyft dataset.
+
+## Results and models
+
+### NuScenes
+
+| Backbone | Lr schd | Mem (GB) | Inf time (fps) | mAP | NDS | Download |
+| :--------------------------------------------------------------------------------------------: | :-----: | :------: | :------------: | :---: | :---: | :--------------------------------------------------------------------------------------------------------------------------------------------------------------------------------------------------------------------------------------------------------------------------------------------------------------------------------------------------------------------------------------------------------------------------------------------------: |
+| [SECFPN](../pointpillars/hv_pointpillars_secfpn_sbn-all_4x8_2x_nus-3d.py) | 2x | 16.4 | | 35.17 | 49.76 | [model](https://download.openmmlab.com/mmdetection3d/v0.1.0_models/pointpillars/hv_pointpillars_secfpn_sbn-all_4x8_2x_nus-3d/hv_pointpillars_secfpn_sbn-all_4x8_2x_nus-3d_20200620_230725-0817d270.pth) \| [log](https://download.openmmlab.com/mmdetection3d/v0.1.0_models/pointpillars/hv_pointpillars_secfpn_sbn-all_4x8_2x_nus-3d/hv_pointpillars_secfpn_sbn-all_4x8_2x_nus-3d_20200620_230725.log.json) |
+| [SSN](./hv_ssn_secfpn_sbn-all_2x16_2x_nus-3d.py) | 2x | 3.6 | | 40.91 | 54.44 | [model](https://download.openmmlab.com/mmdetection3d/v1.0.0_models/ssn/hv_ssn_secfpn_sbn-all_2x16_2x_nus-3d/hv_ssn_secfpn_sbn-all_2x16_2x_nus-3d_20210830_101351-51915986.pth) \| [log](https://download.openmmlab.com/mmdetection3d/v1.0.0_models/ssn/hv_ssn_secfpn_sbn-all_2x16_2x_nus-3d/hv_ssn_secfpn_sbn-all_2x16_2x_nus-3d_20210830_101351.log.json) |
+| [RegNetX-400MF-SECFPN](../regnet/hv_pointpillars_regnet-400mf_secfpn_sbn-all_4x8_2x_nus-3d.py) | 2x | 16.4 | | 41.15 | 55.20 | [model](https://download.openmmlab.com/mmdetection3d/v0.1.0_models/regnet/hv_pointpillars_regnet-400mf_secfpn_sbn-all_4x8_2x_nus-3d/hv_pointpillars_regnet-400mf_secfpn_sbn-all_4x8_2x_nus-3d_20200620_230334-53044f32.pth) \| [log](https://download.openmmlab.com/mmdetection3d/v0.1.0_models/regnet/hv_pointpillars_regnet-400mf_secfpn_sbn-all_4x8_2x_nus-3d/hv_pointpillars_regnet-400mf_secfpn_sbn-all_4x8_2x_nus-3d_20200620_230334.log.json) |
+| [RegNetX-400MF-SSN](./hv_ssn_regnet-400mf_secfpn_sbn-all_2x16_2x_nus-3d.py) | 2x | 5.1 | | 46.65 | 58.24 | [model](https://download.openmmlab.com/mmdetection3d/v1.0.0_models/ssn/hv_ssn_regnet-400mf_secfpn_sbn-all_2x16_2x_nus-3d/hv_ssn_regnet-400mf_secfpn_sbn-all_2x16_2x_nus-3d_20210829_210615-361e5e04.pth) \| [log](https://download.openmmlab.com/mmdetection3d/v1.0.0_models/ssn/hv_ssn_regnet-400mf_secfpn_sbn-all_2x16_2x_nus-3d/hv_ssn_regnet-400mf_secfpn_sbn-all_2x16_2x_nus-3d_20210829_210615.log.json) |
+
+### Lyft
+
+| Backbone | Lr schd | Mem (GB) | Inf time (fps) | Private Score | Public Score | Download |
+| :--------------------------------------------------------------------------: | :-----: | :------: | :------------: | :-----------: | :----------: | :----------------------------------------------------------------------------------------------------------------------------------------------------------------------------------------------------------------------------------------------------------------------------------------------------------------------------------------------------------------------------------------------------------------: |
+| [SECFPN](../pointpillars/hv_pointpillars_secfpn_sbn-all_2x8_2x_lyft-3d.py) | 2x | 12.2 | | 13.9 | 14.1 | [model](https://download.openmmlab.com/mmdetection3d/v0.1.0_models/pointpillars/hv_pointpillars_secfpn_sbn-all_2x8_2x_lyft-3d/hv_pointpillars_secfpn_sbn-all_2x8_2x_lyft-3d_20210517_204807-2518e3de.pth) \| [log](https://download.openmmlab.com/mmdetection3d/v0.1.0_models/pointpillars/hv_pointpillars_secfpn_sbn-all_2x8_2x_lyft-3d/hv_pointpillars_secfpn_sbn-all_2x8_2x_lyft-3d_20210517_204807.log.json) |
+| [SSN](./hv_ssn_secfpn_sbn-all_2x16_2x_lyft-3d.py) | 2x | 8.5 | | 17.5 | 17.5 | [model](https://download.openmmlab.com/mmdetection3d/v1.0.0_models/ssn/hv_ssn_secfpn_sbn-all_2x16_2x_lyft-3d/hv_ssn_secfpn_sbn-all_2x16_2x_lyft-3d_20210822_134731-46841b41.pth) \| [log](https://download.openmmlab.com/mmdetection3d/v1.0.0_models/ssn/hv_ssn_secfpn_sbn-all_2x16_2x_lyft-3d/hv_ssn_secfpn_sbn-all_2x16_2x_lyft-3d_20210822_134731.log.json) |
+| [RegNetX-400MF-SSN](./hv_ssn_regnet-400mf_secfpn_sbn-all_1x16_2x_lyft-3d.py) | 2x | 7.4 | | 17.9 | 18 | [model](https://download.openmmlab.com/mmdetection3d/v1.0.0_models/ssn/hv_ssn_regnet-400mf_secfpn_sbn-all_1x16_2x_lyft-3d/hv_ssn_regnet-400mf_secfpn_sbn-all_1x16_2x_lyft-3d_20210829_122825-d93475a1.pth) \| [log](https://download.openmmlab.com/mmdetection3d/v1.0.0_models/ssn/hv_ssn_regnet-400mf_secfpn_sbn-all_1x16_2x_lyft-3d/hv_ssn_regnet-400mf_secfpn_sbn-all_1x16_2x_lyft-3d_20210829_122825.log.json) |
+
+Note:
+
+The main difference of the shape-aware grouping heads with the original SECOND FPN heads is that the former groups objects with similar sizes and shapes together, and design shape-specific heads for each group. Heavier heads (with more convolutions and large strides) are designed for large objects while smaller heads for small objects. Note that there may appear different feature map sizes in the outputs, so an anchor generator tailored to these feature maps is also needed in the implementation.
+
+Users could try other settings in terms of the head design. Here we basically refer to the implementation [HERE](https://github.com/xinge008/SSN).
+
+## Citation
+
+```latex
+@inproceedings{zhu2020ssn,
+ title={SSN: Shape Signature Networks for Multi-class Object Detection from Point Clouds},
+ author={Zhu, Xinge and Ma, Yuexin and Wang, Tai and Xu, Yan and Shi, Jianping and Lin, Dahua},
+ booktitle={Proceedings of the European Conference on Computer Vision},
+ year={2020}
+}
+```
diff --git a/configs/ssn/hv_ssn_regnet-400mf_secfpn_sbn-all_1x16_2x_lyft-3d.py b/configs/ssn/hv_ssn_regnet-400mf_secfpn_sbn-all_1x16_2x_lyft-3d.py
new file mode 100644
index 0000000..1103bcf
--- /dev/null
+++ b/configs/ssn/hv_ssn_regnet-400mf_secfpn_sbn-all_1x16_2x_lyft-3d.py
@@ -0,0 +1,21 @@
+_base_ = './hv_ssn_secfpn_sbn-all_2x16_2x_lyft-3d.py'
+# model settings
+model = dict(
+ type='MVXFasterRCNN',
+ pts_backbone=dict(
+ _delete_=True,
+ type='NoStemRegNet',
+ arch=dict(w0=24, wa=24.48, wm=2.54, group_w=16, depth=22, bot_mul=1.0),
+ init_cfg=dict(
+ type='Pretrained', checkpoint='open-mmlab://regnetx_400mf'),
+ out_indices=(1, 2, 3),
+ frozen_stages=-1,
+ strides=(1, 2, 2, 2),
+ base_channels=64,
+ stem_channels=64,
+ norm_cfg=dict(type='naiveSyncBN2d', eps=1e-3, momentum=0.01),
+ norm_eval=False,
+ style='pytorch'),
+ pts_neck=dict(in_channels=[64, 160, 384]))
+# dataset settings
+data = dict(samples_per_gpu=1, workers_per_gpu=2)
diff --git a/configs/ssn/hv_ssn_regnet-400mf_secfpn_sbn-all_2x16_2x_nus-3d.py b/configs/ssn/hv_ssn_regnet-400mf_secfpn_sbn-all_2x16_2x_nus-3d.py
new file mode 100644
index 0000000..fb9ef31
--- /dev/null
+++ b/configs/ssn/hv_ssn_regnet-400mf_secfpn_sbn-all_2x16_2x_nus-3d.py
@@ -0,0 +1,19 @@
+_base_ = './hv_ssn_secfpn_sbn-all_2x16_2x_nus-3d.py'
+# model settings
+model = dict(
+ type='MVXFasterRCNN',
+ pts_backbone=dict(
+ _delete_=True,
+ type='NoStemRegNet',
+ arch=dict(w0=24, wa=24.48, wm=2.54, group_w=16, depth=22, bot_mul=1.0),
+ init_cfg=dict(
+ type='Pretrained', checkpoint='open-mmlab://regnetx_400mf'),
+ out_indices=(1, 2, 3),
+ frozen_stages=-1,
+ strides=(1, 2, 2, 2),
+ base_channels=64,
+ stem_channels=64,
+ norm_cfg=dict(type='naiveSyncBN2d', eps=1e-3, momentum=0.01),
+ norm_eval=False,
+ style='pytorch'),
+ pts_neck=dict(in_channels=[64, 160, 384]))
diff --git a/configs/ssn/hv_ssn_secfpn_sbn-all_2x16_2x_lyft-3d.py b/configs/ssn/hv_ssn_secfpn_sbn-all_2x16_2x_lyft-3d.py
new file mode 100644
index 0000000..50b33c8
--- /dev/null
+++ b/configs/ssn/hv_ssn_secfpn_sbn-all_2x16_2x_lyft-3d.py
@@ -0,0 +1,224 @@
+_base_ = [
+ '../_base_/models/hv_pointpillars_fpn_lyft.py',
+ '../_base_/datasets/lyft-3d.py',
+ '../_base_/schedules/schedule_2x.py',
+ '../_base_/default_runtime.py',
+]
+point_cloud_range = [-100, -100, -5, 100, 100, 3]
+# Note that the order of class names should be consistent with
+# the following anchors' order
+class_names = [
+ 'bicycle', 'motorcycle', 'pedestrian', 'animal', 'car',
+ 'emergency_vehicle', 'bus', 'other_vehicle', 'truck'
+]
+
+train_pipeline = [
+ dict(type='LoadPointsFromFile', coord_type='LIDAR', load_dim=5, use_dim=5),
+ dict(type='LoadPointsFromMultiSweeps', sweeps_num=10),
+ dict(type='LoadAnnotations3D', with_bbox_3d=True, with_label_3d=True),
+ dict(
+ type='GlobalRotScaleTrans',
+ rot_range=[-0.3925, 0.3925],
+ scale_ratio_range=[0.95, 1.05],
+ translation_std=[0, 0, 0]),
+ dict(
+ type='RandomFlip3D',
+ sync_2d=False,
+ flip_ratio_bev_horizontal=0.5,
+ flip_ratio_bev_vertical=0.5),
+ dict(type='PointsRangeFilter', point_cloud_range=point_cloud_range),
+ dict(type='ObjectRangeFilter', point_cloud_range=point_cloud_range),
+ dict(type='PointShuffle'),
+ dict(type='DefaultFormatBundle3D', class_names=class_names),
+ dict(type='Collect3D', keys=['points', 'gt_bboxes_3d', 'gt_labels_3d'])
+]
+test_pipeline = [
+ dict(type='LoadPointsFromFile', coord_type='LIDAR', load_dim=5, use_dim=5),
+ dict(type='LoadPointsFromMultiSweeps', sweeps_num=10),
+ dict(
+ type='MultiScaleFlipAug3D',
+ img_scale=(1333, 800),
+ pts_scale_ratio=1,
+ flip=False,
+ transforms=[
+ dict(
+ type='GlobalRotScaleTrans',
+ rot_range=[0, 0],
+ scale_ratio_range=[1., 1.],
+ translation_std=[0, 0, 0]),
+ dict(type='RandomFlip3D'),
+ dict(
+ type='PointsRangeFilter', point_cloud_range=point_cloud_range),
+ dict(
+ type='DefaultFormatBundle3D',
+ class_names=class_names,
+ with_label=False),
+ dict(type='Collect3D', keys=['points'])
+ ])
+]
+data = dict(
+ samples_per_gpu=2,
+ workers_per_gpu=4,
+ train=dict(pipeline=train_pipeline, classes=class_names),
+ val=dict(pipeline=test_pipeline, classes=class_names),
+ test=dict(pipeline=test_pipeline, classes=class_names))
+
+# model settings
+model = dict(
+ pts_voxel_layer=dict(point_cloud_range=[-100, -100, -5, 100, 100, 3]),
+ pts_voxel_encoder=dict(
+ feat_channels=[32, 64],
+ point_cloud_range=[-100, -100, -5, 100, 100, 3]),
+ pts_middle_encoder=dict(output_shape=[800, 800]),
+ pts_neck=dict(
+ _delete_=True,
+ type='SECONDFPN',
+ norm_cfg=dict(type='naiveSyncBN2d', eps=1e-3, momentum=0.01),
+ in_channels=[64, 128, 256],
+ upsample_strides=[1, 2, 4],
+ out_channels=[128, 128, 128]),
+ pts_bbox_head=dict(
+ _delete_=True,
+ type='ShapeAwareHead',
+ num_classes=9,
+ in_channels=384,
+ feat_channels=384,
+ use_direction_classifier=True,
+ anchor_generator=dict(
+ type='AlignedAnchor3DRangeGeneratorPerCls',
+ ranges=[[-100, -100, -1.0709302, 100, 100, -1.0709302],
+ [-100, -100, -1.3220503, 100, 100, -1.3220503],
+ [-100, -100, -0.9122268, 100, 100, -0.9122268],
+ [-100, -100, -1.8012227, 100, 100, -1.8012227],
+ [-100, -100, -1.0715024, 100, 100, -1.0715024],
+ [-100, -100, -0.8871424, 100, 100, -0.8871424],
+ [-100, -100, -0.3519405, 100, 100, -0.3519405],
+ [-100, -100, -0.6276341, 100, 100, -0.6276341],
+ [-100, -100, -0.3033737, 100, 100, -0.3033737]],
+ sizes=[
+ [1.76, 0.63, 1.44], # bicycle
+ [2.35, 0.96, 1.59], # motorcycle
+ [0.80, 0.76, 1.76], # pedestrian
+ [0.73, 0.35, 0.50], # animal
+ [4.75, 1.92, 1.71], # car
+ [6.52, 2.42, 2.34], # emergency vehicle
+ [12.70, 2.92, 3.42], # bus
+ [8.17, 2.75, 3.20], # other vehicle
+ [10.24, 2.84, 3.44] # truck
+ ],
+ custom_values=[],
+ rotations=[0, 1.57],
+ reshape_out=False),
+ tasks=[
+ dict(
+ num_class=2,
+ class_names=['bicycle', 'motorcycle'],
+ shared_conv_channels=(64, 64),
+ shared_conv_strides=(1, 1),
+ norm_cfg=dict(type='naiveSyncBN2d', eps=1e-3, momentum=0.01)),
+ dict(
+ num_class=2,
+ class_names=['pedestrian', 'animal'],
+ shared_conv_channels=(64, 64),
+ shared_conv_strides=(1, 1),
+ norm_cfg=dict(type='naiveSyncBN2d', eps=1e-3, momentum=0.01)),
+ dict(
+ num_class=2,
+ class_names=['car', 'emergency_vehicle'],
+ shared_conv_channels=(64, 64, 64),
+ shared_conv_strides=(2, 1, 1),
+ norm_cfg=dict(type='naiveSyncBN2d', eps=1e-3, momentum=0.01)),
+ dict(
+ num_class=3,
+ class_names=['bus', 'other_vehicle', 'truck'],
+ shared_conv_channels=(64, 64, 64),
+ shared_conv_strides=(2, 1, 1),
+ norm_cfg=dict(type='naiveSyncBN2d', eps=1e-3, momentum=0.01))
+ ],
+ assign_per_class=True,
+ diff_rad_by_sin=True,
+ dir_offset=-0.7854, # -pi/4
+ dir_limit_offset=0,
+ bbox_coder=dict(type='DeltaXYZWLHRBBoxCoder', code_size=7),
+ loss_cls=dict(
+ type='FocalLoss',
+ use_sigmoid=True,
+ gamma=2.0,
+ alpha=0.25,
+ loss_weight=1.0),
+ loss_bbox=dict(type='SmoothL1Loss', beta=1.0 / 9.0, loss_weight=1.0),
+ loss_dir=dict(
+ type='CrossEntropyLoss', use_sigmoid=False, loss_weight=0.2)),
+ # model training and testing settings
+ train_cfg=dict(
+ _delete_=True,
+ pts=dict(
+ assigner=[
+ dict( # bicycle
+ type='MaxIoUAssigner',
+ iou_calculator=dict(type='BboxOverlapsNearest3D'),
+ pos_iou_thr=0.55,
+ neg_iou_thr=0.4,
+ min_pos_iou=0.4,
+ ignore_iof_thr=-1),
+ dict( # motorcycle
+ type='MaxIoUAssigner',
+ iou_calculator=dict(type='BboxOverlapsNearest3D'),
+ pos_iou_thr=0.55,
+ neg_iou_thr=0.4,
+ min_pos_iou=0.4,
+ ignore_iof_thr=-1),
+ dict( # pedestrian
+ type='MaxIoUAssigner',
+ iou_calculator=dict(type='BboxOverlapsNearest3D'),
+ pos_iou_thr=0.55,
+ neg_iou_thr=0.4,
+ min_pos_iou=0.4,
+ ignore_iof_thr=-1),
+ dict( # animal
+ type='MaxIoUAssigner',
+ iou_calculator=dict(type='BboxOverlapsNearest3D'),
+ pos_iou_thr=0.55,
+ neg_iou_thr=0.4,
+ min_pos_iou=0.4,
+ ignore_iof_thr=-1),
+ dict( # car
+ type='MaxIoUAssigner',
+ iou_calculator=dict(type='BboxOverlapsNearest3D'),
+ pos_iou_thr=0.6,
+ neg_iou_thr=0.45,
+ min_pos_iou=0.45,
+ ignore_iof_thr=-1),
+ dict( # emergency vehicle
+ type='MaxIoUAssigner',
+ iou_calculator=dict(type='BboxOverlapsNearest3D'),
+ pos_iou_thr=0.55,
+ neg_iou_thr=0.4,
+ min_pos_iou=0.4,
+ ignore_iof_thr=-1),
+ dict( # bus
+ type='MaxIoUAssigner',
+ iou_calculator=dict(type='BboxOverlapsNearest3D'),
+ pos_iou_thr=0.6,
+ neg_iou_thr=0.45,
+ min_pos_iou=0.45,
+ ignore_iof_thr=-1),
+ dict( # other vehicle
+ type='MaxIoUAssigner',
+ iou_calculator=dict(type='BboxOverlapsNearest3D'),
+ pos_iou_thr=0.55,
+ neg_iou_thr=0.4,
+ min_pos_iou=0.4,
+ ignore_iof_thr=-1),
+ dict( # truck
+ type='MaxIoUAssigner',
+ iou_calculator=dict(type='BboxOverlapsNearest3D'),
+ pos_iou_thr=0.6,
+ neg_iou_thr=0.45,
+ min_pos_iou=0.45,
+ ignore_iof_thr=-1)
+ ],
+ allowed_border=0,
+ code_weight=[1.0, 1.0, 1.0, 1.0, 1.0, 1.0, 1.0],
+ pos_weight=-1,
+ debug=False)))
diff --git a/configs/ssn/hv_ssn_secfpn_sbn-all_2x16_2x_nus-3d.py b/configs/ssn/hv_ssn_secfpn_sbn-all_2x16_2x_nus-3d.py
new file mode 100644
index 0000000..8550201
--- /dev/null
+++ b/configs/ssn/hv_ssn_secfpn_sbn-all_2x16_2x_nus-3d.py
@@ -0,0 +1,238 @@
+_base_ = [
+ '../_base_/models/hv_pointpillars_fpn_nus.py',
+ '../_base_/datasets/nus-3d.py',
+ '../_base_/schedules/schedule_2x.py',
+ '../_base_/default_runtime.py',
+]
+# Note that the order of class names should be consistent with
+# the following anchors' order
+point_cloud_range = [-50, -50, -5, 50, 50, 3]
+class_names = [
+ 'bicycle', 'motorcycle', 'pedestrian', 'traffic_cone', 'barrier', 'car',
+ 'truck', 'trailer', 'bus', 'construction_vehicle'
+]
+
+train_pipeline = [
+ dict(type='LoadPointsFromFile', coord_type='LIDAR', load_dim=5, use_dim=5),
+ dict(type='LoadPointsFromMultiSweeps', sweeps_num=10),
+ dict(type='LoadAnnotations3D', with_bbox_3d=True, with_label_3d=True),
+ dict(
+ type='GlobalRotScaleTrans',
+ rot_range=[-0.3925, 0.3925],
+ scale_ratio_range=[0.95, 1.05],
+ translation_std=[0, 0, 0]),
+ dict(
+ type='RandomFlip3D',
+ sync_2d=False,
+ flip_ratio_bev_horizontal=0.5,
+ flip_ratio_bev_vertical=0.5),
+ dict(type='PointsRangeFilter', point_cloud_range=point_cloud_range),
+ dict(type='ObjectRangeFilter', point_cloud_range=point_cloud_range),
+ dict(type='PointShuffle'),
+ dict(type='DefaultFormatBundle3D', class_names=class_names),
+ dict(type='Collect3D', keys=['points', 'gt_bboxes_3d', 'gt_labels_3d'])
+]
+test_pipeline = [
+ dict(type='LoadPointsFromFile', coord_type='LIDAR', load_dim=5, use_dim=5),
+ dict(type='LoadPointsFromMultiSweeps', sweeps_num=10),
+ dict(
+ type='MultiScaleFlipAug3D',
+ img_scale=(1333, 800),
+ pts_scale_ratio=1,
+ flip=False,
+ transforms=[
+ dict(
+ type='GlobalRotScaleTrans',
+ rot_range=[0, 0],
+ scale_ratio_range=[1., 1.],
+ translation_std=[0, 0, 0]),
+ dict(type='RandomFlip3D'),
+ dict(
+ type='PointsRangeFilter', point_cloud_range=point_cloud_range),
+ dict(
+ type='DefaultFormatBundle3D',
+ class_names=class_names,
+ with_label=False),
+ dict(type='Collect3D', keys=['points'])
+ ])
+]
+data = dict(
+ samples_per_gpu=2,
+ workers_per_gpu=4,
+ train=dict(pipeline=train_pipeline, classes=class_names),
+ val=dict(pipeline=test_pipeline, classes=class_names),
+ test=dict(pipeline=test_pipeline, classes=class_names))
+
+# model settings
+model = dict(
+ pts_voxel_layer=dict(max_num_points=20),
+ pts_voxel_encoder=dict(feat_channels=[64, 64]),
+ pts_neck=dict(
+ _delete_=True,
+ type='SECONDFPN',
+ norm_cfg=dict(type='naiveSyncBN2d', eps=1e-3, momentum=0.01),
+ in_channels=[64, 128, 256],
+ upsample_strides=[1, 2, 4],
+ out_channels=[128, 128, 128]),
+ pts_bbox_head=dict(
+ _delete_=True,
+ type='ShapeAwareHead',
+ num_classes=10,
+ in_channels=384,
+ feat_channels=384,
+ use_direction_classifier=True,
+ anchor_generator=dict(
+ type='AlignedAnchor3DRangeGeneratorPerCls',
+ ranges=[[-50, -50, -1.67339111, 50, 50, -1.67339111],
+ [-50, -50, -1.71396371, 50, 50, -1.71396371],
+ [-50, -50, -1.61785072, 50, 50, -1.61785072],
+ [-50, -50, -1.80984986, 50, 50, -1.80984986],
+ [-50, -50, -1.76396500, 50, 50, -1.76396500],
+ [-50, -50, -1.80032795, 50, 50, -1.80032795],
+ [-50, -50, -1.74440365, 50, 50, -1.74440365],
+ [-50, -50, -1.68526504, 50, 50, -1.68526504],
+ [-50, -50, -1.80673031, 50, 50, -1.80673031],
+ [-50, -50, -1.64824291, 50, 50, -1.64824291]],
+ sizes=[
+ [1.68452161, 0.60058911, 1.27192197], # bicycle
+ [2.09973778, 0.76279481, 1.44403034], # motorcycle
+ [0.72564370, 0.66344886, 1.75748069], # pedestrian
+ [0.40359262, 0.39694519, 1.06232151], # traffic cone
+ [0.48578221, 2.49008838, 0.98297065], # barrier
+ [4.60718145, 1.95017717, 1.72270761], # car
+ [6.73778078, 2.45609390, 2.73004906], # truck
+ [12.01320693, 2.87427237, 3.81509561], # trailer
+ [11.1885991, 2.94046906, 3.47030982], # bus
+ [6.38352896, 2.73050468, 3.13312415] # construction vehicle
+ ],
+ custom_values=[0, 0],
+ rotations=[0, 1.57],
+ reshape_out=False),
+ tasks=[
+ dict(
+ num_class=2,
+ class_names=['bicycle', 'motorcycle'],
+ shared_conv_channels=(64, 64),
+ shared_conv_strides=(1, 1),
+ norm_cfg=dict(type='naiveSyncBN2d', eps=1e-3, momentum=0.01)),
+ dict(
+ num_class=1,
+ class_names=['pedestrian'],
+ shared_conv_channels=(64, 64),
+ shared_conv_strides=(1, 1),
+ norm_cfg=dict(type='naiveSyncBN2d', eps=1e-3, momentum=0.01)),
+ dict(
+ num_class=2,
+ class_names=['traffic_cone', 'barrier'],
+ shared_conv_channels=(64, 64),
+ shared_conv_strides=(1, 1),
+ norm_cfg=dict(type='naiveSyncBN2d', eps=1e-3, momentum=0.01)),
+ dict(
+ num_class=1,
+ class_names=['car'],
+ shared_conv_channels=(64, 64, 64),
+ shared_conv_strides=(2, 1, 1),
+ norm_cfg=dict(type='naiveSyncBN2d', eps=1e-3, momentum=0.01)),
+ dict(
+ num_class=4,
+ class_names=[
+ 'truck', 'trailer', 'bus', 'construction_vehicle'
+ ],
+ shared_conv_channels=(64, 64, 64),
+ shared_conv_strides=(2, 1, 1),
+ norm_cfg=dict(type='naiveSyncBN2d', eps=1e-3, momentum=0.01))
+ ],
+ assign_per_class=True,
+ diff_rad_by_sin=True,
+ dir_offset=-0.7854, # -pi/4
+ dir_limit_offset=0,
+ bbox_coder=dict(type='DeltaXYZWLHRBBoxCoder', code_size=9),
+ loss_cls=dict(
+ type='FocalLoss',
+ use_sigmoid=True,
+ gamma=2.0,
+ alpha=0.25,
+ loss_weight=1.0),
+ loss_bbox=dict(type='SmoothL1Loss', beta=1.0 / 9.0, loss_weight=1.0),
+ loss_dir=dict(
+ type='CrossEntropyLoss', use_sigmoid=False, loss_weight=0.2)),
+ # model training and testing settings
+ train_cfg=dict(
+ _delete_=True,
+ pts=dict(
+ assigner=[
+ dict( # bicycle
+ type='MaxIoUAssigner',
+ iou_calculator=dict(type='BboxOverlapsNearest3D'),
+ pos_iou_thr=0.5,
+ neg_iou_thr=0.35,
+ min_pos_iou=0.35,
+ ignore_iof_thr=-1),
+ dict( # motorcycle
+ type='MaxIoUAssigner',
+ iou_calculator=dict(type='BboxOverlapsNearest3D'),
+ pos_iou_thr=0.5,
+ neg_iou_thr=0.3,
+ min_pos_iou=0.3,
+ ignore_iof_thr=-1),
+ dict( # pedestrian
+ type='MaxIoUAssigner',
+ iou_calculator=dict(type='BboxOverlapsNearest3D'),
+ pos_iou_thr=0.6,
+ neg_iou_thr=0.4,
+ min_pos_iou=0.4,
+ ignore_iof_thr=-1),
+ dict( # traffic cone
+ type='MaxIoUAssigner',
+ iou_calculator=dict(type='BboxOverlapsNearest3D'),
+ pos_iou_thr=0.6,
+ neg_iou_thr=0.4,
+ min_pos_iou=0.4,
+ ignore_iof_thr=-1),
+ dict( # barrier
+ type='MaxIoUAssigner',
+ iou_calculator=dict(type='BboxOverlapsNearest3D'),
+ pos_iou_thr=0.55,
+ neg_iou_thr=0.4,
+ min_pos_iou=0.4,
+ ignore_iof_thr=-1),
+ dict( # car
+ type='MaxIoUAssigner',
+ iou_calculator=dict(type='BboxOverlapsNearest3D'),
+ pos_iou_thr=0.6,
+ neg_iou_thr=0.45,
+ min_pos_iou=0.45,
+ ignore_iof_thr=-1),
+ dict( # truck
+ type='MaxIoUAssigner',
+ iou_calculator=dict(type='BboxOverlapsNearest3D'),
+ pos_iou_thr=0.55,
+ neg_iou_thr=0.4,
+ min_pos_iou=0.4,
+ ignore_iof_thr=-1),
+ dict( # trailer
+ type='MaxIoUAssigner',
+ iou_calculator=dict(type='BboxOverlapsNearest3D'),
+ pos_iou_thr=0.5,
+ neg_iou_thr=0.35,
+ min_pos_iou=0.35,
+ ignore_iof_thr=-1),
+ dict( # bus
+ type='MaxIoUAssigner',
+ iou_calculator=dict(type='BboxOverlapsNearest3D'),
+ pos_iou_thr=0.55,
+ neg_iou_thr=0.4,
+ min_pos_iou=0.4,
+ ignore_iof_thr=-1),
+ dict( # construction vehicle
+ type='MaxIoUAssigner',
+ iou_calculator=dict(type='BboxOverlapsNearest3D'),
+ pos_iou_thr=0.5,
+ neg_iou_thr=0.35,
+ min_pos_iou=0.35,
+ ignore_iof_thr=-1)
+ ],
+ allowed_border=0,
+ code_weight=[1.0, 1.0, 1.0, 1.0, 1.0, 1.0, 1.0, 0.2, 0.2],
+ pos_weight=-1,
+ debug=False)))
diff --git a/configs/ssn/metafile.yml b/configs/ssn/metafile.yml
new file mode 100644
index 0000000..df6dd9e
--- /dev/null
+++ b/configs/ssn/metafile.yml
@@ -0,0 +1,72 @@
+Collections:
+ - Name: SSN
+ Metadata:
+ Training Techniques:
+ - AdamW
+ Training Resources: 8x GeForce GTX 1080 Ti
+ Architecture:
+ - Hard Voxelization
+ Paper:
+ URL: https://arxiv.org/abs/2004.02774
+ Title: 'SSN: Shape Signature Networks for Multi-class Object Detection from Point Clouds'
+ README: configs/ssn/README.md
+ Code:
+ URL: https://github.com/open-mmlab/mmdetection3d/blob/master/mmdet3d/models/dense_heads/shape_aware_head.py#L166
+ Version: v0.7.0
+
+Models:
+ - Name: hv_ssn_secfpn_sbn-all_2x16_2x_nus-3d
+ In Collection: SSN
+ Config: configs/ssn/hv_ssn_secfpn_sbn-all_2x16_2x_nus-3d.py
+ Metadata:
+ Training Data: nuScenes
+ Training Memory (GB): 3.6
+ Results:
+ - Task: 3D Object Detection
+ Dataset: nuScenes
+ Metrics:
+ mAP: 40.91
+ NDS: 54.44
+ Weights: https://download.openmmlab.com/mmdetection3d/v1.0.0_models/ssn/hv_ssn_secfpn_sbn-all_2x16_2x_nus-3d/hv_ssn_secfpn_sbn-all_2x16_2x_nus-3d_20210830_101351-51915986.pth
+
+ - Name: hv_ssn_regnet-400mf_secfpn_sbn-all_2x16_2x_nus-3d
+ In Collection: SSN
+ Config: configs/ssn/hv_ssn_regnet-400mf_secfpn_sbn-all_2x16_2x_nus-3d.py
+ Metadata:
+ Training Data: nuScenes
+ Training Memory (GB): 5.1
+ Results:
+ - Task: 3D Object Detection
+ Dataset: nuScenes
+ Metrics:
+ mAP: 46.65
+ NDS: 58.24
+ Weights: https://download.openmmlab.com/mmdetection3d/v1.0.0_models/ssn/hv_ssn_regnet-400mf_secfpn_sbn-all_2x16_2x_nus-3d/hv_ssn_regnet-400mf_secfpn_sbn-all_2x16_2x_nus-3d_20210829_210615-361e5e04.pth
+
+ - Name: hv_ssn_secfpn_sbn-all_2x16_2x_lyft-3d
+ In Collection: SSN
+ Config: configs/ssn/hv_ssn_secfpn_sbn-all_2x16_2x_lyft-3d.py
+ Metadata:
+ Training Data: Lyft
+ Training Memory (GB): 8.5
+ Results:
+ - Task: 3D Object Detection
+ Dataset: Lyft
+ Metrics:
+ Private Score: 17.5
+ Public Score: 17.5
+ Weights: https://download.openmmlab.com/mmdetection3d/v1.0.0_models/ssn/hv_ssn_secfpn_sbn-all_2x16_2x_lyft-3d/hv_ssn_secfpn_sbn-all_2x16_2x_lyft-3d_20210822_134731-46841b41.pth
+
+ - Name: hv_ssn_regnet-400mf_secfpn_sbn-all_1x16_2x_lyft-3d
+ In Collection: SSN
+ Config: configs/ssn/hv_ssn_regnet-400mf_secfpn_sbn-all_1x16_2x_lyft-3d.py
+ Metadata:
+ Training Data: Lyft
+ Training Memory (GB): 7.4
+ Results:
+ - Task: 3D Object Detection
+ Dataset: Lyft
+ Metrics:
+ Private Score: 17.9
+ Public Score: 18.0
+ Weights: https://download.openmmlab.com/mmdetection3d/v1.0.0_models/ssn/hv_ssn_regnet-400mf_secfpn_sbn-all_1x16_2x_lyft-3d/hv_ssn_regnet-400mf_secfpn_sbn-all_1x16_2x_lyft-3d_20210829_122825-d93475a1.pth
diff --git a/configs/td3d_is/td3d_is_s3dis-3d-5class.py b/configs/td3d_is/td3d_is_s3dis-3d-5class.py
new file mode 100644
index 0000000..f304129
--- /dev/null
+++ b/configs/td3d_is/td3d_is_s3dis-3d-5class.py
@@ -0,0 +1,164 @@
+voxel_size = .02
+padding = .08
+n_points = 100000
+
+model = dict(
+ type='TD3DInstanceSegmentor',
+ voxel_size=voxel_size,
+ backbone=dict(type='MinkResNet', in_channels=3, depth=34, norm='batch', return_stem=True, stride=1),
+ neck=dict(
+ type='NgfcTinySegmentationNeck',
+ in_channels=(64, 128, 256, 512),
+ out_channels=128),
+ head=dict(
+ type='TD3DInstanceHead',
+ in_channels=128,
+ n_reg_outs=6,
+ n_classes=5,
+ n_levels=4,
+ padding=padding,
+ voxel_size=voxel_size,
+ unet=dict(
+ type='MinkUNet14B',
+ in_channels=32,
+ out_channels=5 + 1,
+ D=3),
+ first_assigner=dict(
+ type='NgfcV2Assigner',
+ min_pts_threshold=18,
+ top_pts_threshold=8,
+ padding=padding),
+ second_assigner=dict(
+ type='MaxIoU3DAssigner',
+ threshold=.25),
+ roi_extractor=dict(
+ type='Mink3DRoIExtractor',
+ voxel_size=voxel_size,
+ padding=padding,
+ min_pts_threshold=10)),
+ train_cfg=dict(num_rois=2),
+ test_cfg=dict(
+ nms_pre=100,
+ iou_thr=.4,
+ score_thr=.15,
+ binary_score_thr=0.2))
+
+optimizer = dict(type='AdamW', lr=0.001, weight_decay=0.0001)
+optimizer_config = dict(grad_clip=dict(max_norm=10, norm_type=2))
+lr_config = dict(policy='step', warmup=None, step=[28, 32])
+runner = dict(type='EpochBasedRunner', max_epochs=33)
+custom_hooks = [dict(type='EmptyCacheHook', after_iter=True)]
+
+checkpoint_config = dict(interval=1, max_keep_ckpts=50)
+log_config = dict(
+ interval=50,
+ hooks=[
+ dict(type='TextLoggerHook'),
+ # dict(type='TensorboardLoggerHook')
+])
+dist_params = dict(backend='nccl')
+log_level = 'INFO'
+work_dir = None
+load_from = None
+resume_from = None
+workflow = [('train', 1)]
+
+dataset_type = 'S3DISInstanceSegDataset'
+data_root = './data/s3dis/'
+
+class_names = ('table', 'chair', 'sofa', 'bookcase', 'board')
+train_area = [1, 2, 3, 4, 6]
+test_area = 5
+
+train_pipeline = [
+ dict(
+ type='LoadPointsFromFile',
+ coord_type='DEPTH',
+ shift_height=False,
+ use_color=True,
+ load_dim=6,
+ use_dim=[0, 1, 2, 3, 4, 5]),
+ dict(
+ type='LoadAnnotations3D',
+ with_mask_3d=True,
+ with_seg_3d=True),
+ dict(type='PointSample', num_points=n_points),
+ dict(type='PointSegClassMappingV2',
+ valid_cat_ids=(7, 8, 9, 10, 11),
+ max_cat_id=13),
+ dict(
+ type='RandomFlip3D',
+ sync_2d=False,
+ flip_ratio_bev_horizontal=0.5,
+ flip_ratio_bev_vertical=0.5),
+ dict(
+ type='GlobalRotScaleTrans',
+ rot_range_z=[-3.14, 3.14],
+ rot_range_x_y=[-0.1308, 0.1308],
+ scale_ratio_range=[.8, 1.2],
+ translation_std=[.1, .1, .1],
+ shift_height=False),
+ dict(type='NormalizePointsColor', color_mean=None),
+ dict(type='DefaultFormatBundle3D', class_names=class_names),
+ dict(type='Collect3D', keys=['points', 'gt_bboxes_3d', 'gt_labels_3d',
+ 'pts_semantic_mask', 'pts_instance_mask'])
+]
+test_pipeline = [
+ dict(
+ type='LoadPointsFromFile',
+ coord_type='DEPTH',
+ shift_height=False,
+ use_color=True,
+ load_dim=6,
+ use_dim=[0, 1, 2, 3, 4, 5]),
+ dict(
+ type='MultiScaleFlipAug3D',
+ img_scale=(1333, 800),
+ pts_scale_ratio=1,
+ flip=False,
+ transforms=[
+ dict(type='NormalizePointsColor', color_mean=None),
+ dict(
+ type='DefaultFormatBundle3D',
+ class_names=class_names,
+ with_label=False),
+ dict(type='Collect3D', keys=['points'])
+ ])
+]
+data = dict(
+ samples_per_gpu=6,
+ workers_per_gpu=10,
+ train=dict(
+ type='RepeatDataset',
+ times=13,
+ dataset=dict(
+ type='ConcatDataset',
+ datasets=[
+ dict(
+ type=dataset_type,
+ data_root=data_root,
+ ann_file=data_root + f's3dis_infos_Area_{i}.pkl',
+ pipeline=train_pipeline,
+ filter_empty_gt=True,
+ classes=class_names,
+ box_type_3d='Depth') for i in train_area
+ ],
+ separate_eval=False)),
+ val=dict(
+ type=dataset_type,
+ data_root=data_root,
+ ann_file=data_root + f's3dis_infos_Area_{test_area}.pkl',
+ pipeline=test_pipeline,
+ filter_empty_gt=False,
+ classes=class_names,
+ test_mode=True,
+ box_type_3d='Depth'),
+ test=dict(
+ type=dataset_type,
+ data_root=data_root,
+ ann_file=data_root + f's3dis_infos_Area_{test_area}.pkl',
+ pipeline=test_pipeline,
+ filter_empty_gt=False,
+ classes=class_names,
+ test_mode=True,
+ box_type_3d='Depth'))
\ No newline at end of file
diff --git a/configs/td3d_is/td3d_is_s3dis-3d-5class_public.py b/configs/td3d_is/td3d_is_s3dis-3d-5class_public.py
new file mode 100644
index 0000000..39d56ea
--- /dev/null
+++ b/configs/td3d_is/td3d_is_s3dis-3d-5class_public.py
@@ -0,0 +1,164 @@
+voxel_size = .02
+padding = .08
+n_points = 100000
+
+model = dict(
+ type='TD3DInstanceSegmentor',
+ voxel_size=voxel_size,
+ backbone=dict(type='MinkResNet', in_channels=3, depth=34, norm='batch', return_stem=True, stride=1),
+ neck=dict(
+ type='NgfcTinySegmentationNeck',
+ in_channels=(64, 128, 256, 512),
+ out_channels=128),
+ head=dict(
+ type='TD3DInstanceHead',
+ in_channels=128,
+ n_reg_outs=6,
+ n_classes=5,
+ n_levels=4,
+ padding=padding,
+ voxel_size=voxel_size,
+ unet=dict(
+ type='MinkUNet14B',
+ in_channels=32,
+ out_channels=5 + 1,
+ D=3),
+ first_assigner=dict(
+ type='NgfcV2Assigner',
+ min_pts_threshold=18,
+ top_pts_threshold=8,
+ padding=padding),
+ second_assigner=dict(
+ type='MaxIoU3DAssigner',
+ threshold=.25),
+ roi_extractor=dict(
+ type='Mink3DRoIExtractor',
+ voxel_size=voxel_size,
+ padding=padding,
+ min_pts_threshold=10)),
+ train_cfg=dict(num_rois=2),
+ test_cfg=dict(
+ nms_pre=800,
+ iou_thr=.4,
+ score_thr=.06,
+ binary_score_thr=0.2))
+
+optimizer = dict(type='AdamW', lr=0.001, weight_decay=0.0001)
+optimizer_config = dict(grad_clip=dict(max_norm=10, norm_type=2))
+lr_config = dict(policy='step', warmup=None, step=[28, 32])
+runner = dict(type='EpochBasedRunner', max_epochs=33)
+custom_hooks = [dict(type='EmptyCacheHook', after_iter=True)]
+
+checkpoint_config = dict(interval=1, max_keep_ckpts=50)
+log_config = dict(
+ interval=50,
+ hooks=[
+ dict(type='TextLoggerHook'),
+ # dict(type='TensorboardLoggerHook')
+])
+dist_params = dict(backend='nccl')
+log_level = 'INFO'
+work_dir = None
+load_from = None
+resume_from = None
+workflow = [('train', 1)]
+
+dataset_type = 'S3DISInstanceSegDataset'
+data_root = './data/s3dis/'
+
+class_names = ('table', 'chair', 'sofa', 'bookcase', 'board')
+train_area = [1, 2, 3, 4, 6]
+test_area = 5
+
+train_pipeline = [
+ dict(
+ type='LoadPointsFromFile',
+ coord_type='DEPTH',
+ shift_height=False,
+ use_color=True,
+ load_dim=6,
+ use_dim=[0, 1, 2, 3, 4, 5]),
+ dict(
+ type='LoadAnnotations3D',
+ with_mask_3d=True,
+ with_seg_3d=True),
+ dict(type='PointSample', num_points=n_points),
+ dict(type='PointSegClassMappingV2',
+ valid_cat_ids=(7, 8, 9, 10, 11),
+ max_cat_id=13),
+ dict(
+ type='RandomFlip3D',
+ sync_2d=False,
+ flip_ratio_bev_horizontal=0.5,
+ flip_ratio_bev_vertical=0.5),
+ dict(
+ type='GlobalRotScaleTrans',
+ rot_range_z=[-3.14, 3.14],
+ rot_range_x_y=[-0.1308, 0.1308],
+ scale_ratio_range=[.8, 1.2],
+ translation_std=[.1, .1, .1],
+ shift_height=False),
+ dict(type='NormalizePointsColor', color_mean=None),
+ dict(type='DefaultFormatBundle3D', class_names=class_names),
+ dict(type='Collect3D', keys=['points', 'gt_bboxes_3d', 'gt_labels_3d',
+ 'pts_semantic_mask', 'pts_instance_mask'])
+]
+test_pipeline = [
+ dict(
+ type='LoadPointsFromFile',
+ coord_type='DEPTH',
+ shift_height=False,
+ use_color=True,
+ load_dim=6,
+ use_dim=[0, 1, 2, 3, 4, 5]),
+ dict(
+ type='MultiScaleFlipAug3D',
+ img_scale=(1333, 800),
+ pts_scale_ratio=1,
+ flip=False,
+ transforms=[
+ dict(type='NormalizePointsColor', color_mean=None),
+ dict(
+ type='DefaultFormatBundle3D',
+ class_names=class_names,
+ with_label=False),
+ dict(type='Collect3D', keys=['points'])
+ ])
+]
+data = dict(
+ samples_per_gpu=6,
+ workers_per_gpu=10,
+ train=dict(
+ type='RepeatDataset',
+ times=13,
+ dataset=dict(
+ type='ConcatDataset',
+ datasets=[
+ dict(
+ type=dataset_type,
+ data_root=data_root,
+ ann_file=data_root + f's3dis_infos_Area_{i}.pkl',
+ pipeline=train_pipeline,
+ filter_empty_gt=True,
+ classes=class_names,
+ box_type_3d='Depth') for i in train_area
+ ],
+ separate_eval=False)),
+ val=dict(
+ type=dataset_type,
+ data_root=data_root,
+ ann_file=data_root + f's3dis_infos_Area_{test_area}.pkl',
+ pipeline=test_pipeline,
+ filter_empty_gt=False,
+ classes=class_names,
+ test_mode=True,
+ box_type_3d='Depth'),
+ test=dict(
+ type=dataset_type,
+ data_root=data_root,
+ ann_file=data_root + f's3dis_infos_Area_{test_area}.pkl',
+ pipeline=test_pipeline,
+ filter_empty_gt=False,
+ classes=class_names,
+ test_mode=True,
+ box_type_3d='Depth'))
\ No newline at end of file
diff --git a/configs/td3d_is/td3d_is_scannet-3d-18class.py b/configs/td3d_is/td3d_is_scannet-3d-18class.py
new file mode 100644
index 0000000..b60f296
--- /dev/null
+++ b/configs/td3d_is/td3d_is_scannet-3d-18class.py
@@ -0,0 +1,164 @@
+voxel_size = .02
+padding = .08
+n_points = 100000
+
+model = dict(
+ type='TD3DInstanceSegmentor',
+ voxel_size=voxel_size,
+ backbone=dict(type='MinkResNet', in_channels=3, depth=34, norm='batch', return_stem=True, stride=1),
+ neck=dict(
+ type='NgfcTinySegmentationNeck',
+ in_channels=(64, 128, 256, 512),
+ out_channels=128),
+ head=dict(
+ type='TD3DInstanceHead',
+ in_channels=128,
+ n_reg_outs=6,
+ n_classes=18,
+ n_levels=4,
+ padding=padding,
+ voxel_size=voxel_size,
+ unet=dict(
+ type='MinkUNet14B',
+ in_channels=32,
+ out_channels=18 + 1,
+ D=3),
+ first_assigner=dict(
+ type='NgfcV2Assigner',
+ min_pts_threshold=18,
+ top_pts_threshold=8,
+ padding=padding),
+ second_assigner=dict(
+ type='MaxIoU3DAssigner',
+ threshold=.25),
+ roi_extractor=dict(
+ type='Mink3DRoIExtractor',
+ voxel_size=voxel_size,
+ padding=padding,
+ min_pts_threshold=10)),
+ train_cfg=dict(num_rois=2),
+ test_cfg=dict(
+ nms_pre=100,
+ iou_thr=.4,
+ score_thr=.15,
+ binary_score_thr=0.2))
+
+optimizer = dict(type='AdamW', lr=0.001, weight_decay=0.0001)
+optimizer_config = dict(grad_clip=dict(max_norm=10, norm_type=2))
+lr_config = dict(policy='step', warmup=None, step=[28, 32])
+runner = dict(type='EpochBasedRunner', max_epochs=33)
+custom_hooks = [dict(type='EmptyCacheHook', after_iter=True)]
+
+checkpoint_config = dict(interval=1, max_keep_ckpts=40)
+log_config = dict(
+ interval=50,
+ hooks=[
+ dict(type='TextLoggerHook'),
+ # dict(type='TensorboardLoggerHook')
+])
+dist_params = dict(backend='nccl')
+log_level = 'INFO'
+work_dir = None
+load_from = None
+resume_from = None
+workflow = [('train', 1)]
+
+dataset_type = 'ScanNetInstanceSegV2Dataset'
+data_root = './data/scannet/'
+class_names = ('cabinet', 'bed', 'chair', 'sofa', 'table', 'door', 'window',
+ 'bookshelf', 'picture', 'counter', 'desk', 'curtain',
+ 'refrigerator', 'showercurtrain', 'toilet', 'sink', 'bathtub',
+ 'garbagebin')
+
+train_pipeline = [
+ dict(
+ type='LoadPointsFromFile',
+ coord_type='DEPTH',
+ shift_height=False,
+ use_color=True,
+ load_dim=6,
+ use_dim=[0, 1, 2, 3, 4, 5]),
+ dict(
+ type='LoadAnnotations3D',
+ with_mask_3d=True,
+ with_seg_3d=True),
+ dict(type='GlobalAlignment', rotation_axis=2),
+ dict(type='PointSample', num_points=n_points),
+ dict(
+ type='PointSegClassMappingV2',
+ valid_cat_ids=(3, 4, 5, 6, 7, 8, 9, 10, 11, 12, 14, 16, 24, 28,
+ 33, 34, 36, 39),
+ max_cat_id=40),
+ dict(
+ type='RandomFlip3D',
+ sync_2d=False,
+ flip_ratio_bev_horizontal=0.5,
+ flip_ratio_bev_vertical=0.5),
+ dict(
+ type='GlobalRotScaleTrans',
+ rot_range_z=[-3.14, 3.14],
+ rot_range_x_y=[-0.1308, 0.1308],
+ scale_ratio_range=[.8, 1.2],
+ translation_std=[.1, .1, .1],
+ shift_height=False),
+ dict(type='NormalizePointsColor', color_mean=None),
+ dict(type='DefaultFormatBundle3D', class_names=class_names),
+ dict(type='Collect3D', keys=['points', 'gt_bboxes_3d', 'gt_labels_3d',
+ 'pts_semantic_mask', 'pts_instance_mask'])
+]
+test_pipeline = [
+ dict(
+ type='LoadPointsFromFile',
+ coord_type='DEPTH',
+ shift_height=False,
+ use_color=True,
+ load_dim=6,
+ use_dim=[0, 1, 2, 3, 4, 5]),
+ dict(
+ type='MultiScaleFlipAug3D',
+ img_scale=(1333, 800),
+ pts_scale_ratio=1,
+ flip=False,
+ transforms=[
+ dict(type='NormalizePointsColor', color_mean=None),
+ dict(
+ type='DefaultFormatBundle3D',
+ class_names=class_names,
+ with_label=False),
+ dict(type='Collect3D', keys=['points'])
+ ])
+]
+
+data = dict(
+ samples_per_gpu=6,
+ workers_per_gpu=10,
+ train=dict(
+ type='RepeatDataset',
+ times=10,
+ dataset=dict(
+ type=dataset_type,
+ data_root=data_root,
+ ann_file=data_root + 'scannet_infos_train.pkl',
+ pipeline=train_pipeline,
+ filter_empty_gt=True,
+ classes=class_names,
+ box_type_3d='Depth')),
+ val=dict(
+ type=dataset_type,
+ data_root=data_root,
+ ann_file=data_root + 'scannet_infos_val.pkl',
+ pipeline=test_pipeline,
+ filter_empty_gt=False,
+ classes=class_names,
+ test_mode=True,
+ box_type_3d='Depth'),
+ test=dict(
+ type=dataset_type,
+ data_root=data_root,
+ ann_file=data_root + 'scannet_infos_val.pkl',
+ pipeline=test_pipeline,
+ filter_empty_gt=False,
+ classes=class_names,
+ test_mode=True,
+ box_type_3d='Depth')
+)
diff --git a/configs/td3d_is/td3d_is_scannet-3d-18class_public.py b/configs/td3d_is/td3d_is_scannet-3d-18class_public.py
new file mode 100644
index 0000000..dfedd0f
--- /dev/null
+++ b/configs/td3d_is/td3d_is_scannet-3d-18class_public.py
@@ -0,0 +1,164 @@
+voxel_size = .02
+padding = .08
+n_points = 100000
+
+model = dict(
+ type='TD3DInstanceSegmentor',
+ voxel_size=voxel_size,
+ backbone=dict(type='MinkResNet', in_channels=3, depth=34, norm='batch', return_stem=True, stride=1),
+ neck=dict(
+ type='NgfcTinySegmentationNeck',
+ in_channels=(64, 128, 256, 512),
+ out_channels=128),
+ head=dict(
+ type='TD3DInstanceHead',
+ in_channels=128,
+ n_reg_outs=6,
+ n_classes=18,
+ n_levels=4,
+ padding=padding,
+ voxel_size=voxel_size,
+ unet=dict(
+ type='MinkUNet14B',
+ in_channels=32,
+ out_channels=18 + 1,
+ D=3),
+ first_assigner=dict(
+ type='NgfcV2Assigner',
+ min_pts_threshold=18,
+ top_pts_threshold=8,
+ padding=padding),
+ second_assigner=dict(
+ type='MaxIoU3DAssigner',
+ threshold=.25),
+ roi_extractor=dict(
+ type='Mink3DRoIExtractor',
+ voxel_size=voxel_size,
+ padding=padding,
+ min_pts_threshold=10)),
+ train_cfg=dict(num_rois=2),
+ test_cfg=dict(
+ nms_pre=1200,
+ iou_thr=.4,
+ score_thr=.1,
+ binary_score_thr=0.2))
+
+optimizer = dict(type='AdamW', lr=0.001, weight_decay=0.0001)
+optimizer_config = dict(grad_clip=dict(max_norm=10, norm_type=2))
+lr_config = dict(policy='step', warmup=None, step=[28, 32])
+runner = dict(type='EpochBasedRunner', max_epochs=33)
+custom_hooks = [dict(type='EmptyCacheHook', after_iter=True)]
+
+checkpoint_config = dict(interval=1, max_keep_ckpts=40)
+log_config = dict(
+ interval=50,
+ hooks=[
+ dict(type='TextLoggerHook'),
+ # dict(type='TensorboardLoggerHook')
+])
+dist_params = dict(backend='nccl')
+log_level = 'INFO'
+work_dir = None
+load_from = None
+resume_from = None
+workflow = [('train', 1)]
+
+dataset_type = 'ScanNetInstanceSegV2Dataset'
+data_root = './data/scannet/'
+class_names = ('cabinet', 'bed', 'chair', 'sofa', 'table', 'door', 'window',
+ 'bookshelf', 'picture', 'counter', 'desk', 'curtain',
+ 'refrigerator', 'showercurtrain', 'toilet', 'sink', 'bathtub',
+ 'garbagebin')
+
+train_pipeline = [
+ dict(
+ type='LoadPointsFromFile',
+ coord_type='DEPTH',
+ shift_height=False,
+ use_color=True,
+ load_dim=6,
+ use_dim=[0, 1, 2, 3, 4, 5]),
+ dict(
+ type='LoadAnnotations3D',
+ with_mask_3d=True,
+ with_seg_3d=True),
+ dict(type='GlobalAlignment', rotation_axis=2),
+ dict(type='PointSample', num_points=n_points),
+ dict(
+ type='PointSegClassMappingV2',
+ valid_cat_ids=(3, 4, 5, 6, 7, 8, 9, 10, 11, 12, 14, 16, 24, 28,
+ 33, 34, 36, 39),
+ max_cat_id=40),
+ dict(
+ type='RandomFlip3D',
+ sync_2d=False,
+ flip_ratio_bev_horizontal=0.5,
+ flip_ratio_bev_vertical=0.5),
+ dict(
+ type='GlobalRotScaleTrans',
+ rot_range_z=[-3.14, 3.14],
+ rot_range_x_y=[-0.1308, 0.1308],
+ scale_ratio_range=[.8, 1.2],
+ translation_std=[.1, .1, .1],
+ shift_height=False),
+ dict(type='NormalizePointsColor', color_mean=None),
+ dict(type='DefaultFormatBundle3D', class_names=class_names),
+ dict(type='Collect3D', keys=['points', 'gt_bboxes_3d', 'gt_labels_3d',
+ 'pts_semantic_mask', 'pts_instance_mask'])
+]
+test_pipeline = [
+ dict(
+ type='LoadPointsFromFile',
+ coord_type='DEPTH',
+ shift_height=False,
+ use_color=True,
+ load_dim=6,
+ use_dim=[0, 1, 2, 3, 4, 5]),
+ dict(
+ type='MultiScaleFlipAug3D',
+ img_scale=(1333, 800),
+ pts_scale_ratio=1,
+ flip=False,
+ transforms=[
+ dict(type='NormalizePointsColor', color_mean=None),
+ dict(
+ type='DefaultFormatBundle3D',
+ class_names=class_names,
+ with_label=False),
+ dict(type='Collect3D', keys=['points'])
+ ])
+]
+
+data = dict(
+ samples_per_gpu=6,
+ workers_per_gpu=10,
+ train=dict(
+ type='RepeatDataset',
+ times=10,
+ dataset=dict(
+ type=dataset_type,
+ data_root=data_root,
+ ann_file=data_root + 'scannet_infos_train.pkl',
+ pipeline=train_pipeline,
+ filter_empty_gt=True,
+ classes=class_names,
+ box_type_3d='Depth')),
+ val=dict(
+ type=dataset_type,
+ data_root=data_root,
+ ann_file=data_root + 'scannet_infos_val.pkl',
+ pipeline=test_pipeline,
+ filter_empty_gt=False,
+ classes=class_names,
+ test_mode=True,
+ box_type_3d='Depth'),
+ test=dict(
+ type=dataset_type,
+ data_root=data_root,
+ ann_file=data_root + 'scannet_infos_val.pkl',
+ pipeline=test_pipeline,
+ filter_empty_gt=False,
+ classes=class_names,
+ test_mode=True,
+ box_type_3d='Depth')
+)
diff --git a/configs/td3d_is/td3d_is_scannet200-3d-198class.py b/configs/td3d_is/td3d_is_scannet200-3d-198class.py
new file mode 100644
index 0000000..33dafda
--- /dev/null
+++ b/configs/td3d_is/td3d_is_scannet200-3d-198class.py
@@ -0,0 +1,170 @@
+voxel_size = .02
+padding = .08
+n_points = 100000
+
+model = dict(
+ type='TD3DInstanceSegmentor',
+ voxel_size=voxel_size,
+ backbone=dict(type='MinkResNet', in_channels=3, depth=34, norm='batch', return_stem=True, stride=1),
+ neck=dict(
+ type='NgfcTinySegmentationNeck',
+ in_channels=(64, 128, 256, 512),
+ out_channels=128),
+ head=dict(
+ type='TD3DInstanceHead',
+ in_channels=128,
+ n_reg_outs=6,
+ n_classes=198,
+ n_levels=4,
+ padding=padding,
+ voxel_size=voxel_size,
+ unet=dict(
+ type='MinkUNet14B',
+ in_channels=32,
+ out_channels=198 + 1,
+ D=3),
+ first_assigner=dict(
+ type='NgfcV2Assigner',
+ min_pts_threshold=18,
+ top_pts_threshold=8,
+ padding=padding),
+ second_assigner=dict(
+ type='MaxIoU3DAssigner',
+ threshold=.25),
+ roi_extractor=dict(
+ type='Mink3DRoIExtractor',
+ voxel_size=voxel_size,
+ padding=padding,
+ min_pts_threshold=10)),
+ train_cfg=dict(num_rois=2),
+ test_cfg=dict(
+ nms_pre=100,
+ iou_thr=.4,
+ score_thr=.15,
+ binary_score_thr=0.2))
+
+optimizer = dict(type='AdamW', lr=0.001, weight_decay=0.0001)
+optimizer_config = dict(grad_clip=dict(max_norm=10, norm_type=2))
+lr_config = dict(policy='step', warmup=None, step=[28, 32])
+runner = dict(type='EpochBasedRunner', max_epochs=33)
+custom_hooks = [dict(type='EmptyCacheHook', after_iter=True)]
+
+checkpoint_config = dict(interval=1, max_keep_ckpts=40)
+log_config = dict(
+ interval=50,
+ hooks=[
+ dict(type='TextLoggerHook'),
+ # dict(type='TensorboardLoggerHook')
+])
+dist_params = dict(backend='nccl')
+log_level = 'INFO'
+work_dir = None
+load_from = None
+resume_from = None
+workflow = [('train', 1)]
+
+dataset_type = 'ScanNet200InstanceSegDataset'
+data_root = './data/scannet200/'
+class_names = ('chair', 'table', 'door', 'couch', 'cabinet', 'shelf', 'desk', 'office chair', 'bed', 'pillow', 'sink', 'picture', 'window', 'toilet', 'bookshelf', 'monitor', 'curtain', 'book', 'armchair', 'coffee table', 'box',
+'refrigerator', 'lamp', 'kitchen cabinet', 'towel', 'clothes', 'tv', 'nightstand', 'counter', 'dresser', 'stool', 'cushion', 'plant', 'ceiling', 'bathtub', 'end table', 'dining table', 'keyboard', 'bag', 'backpack', 'toilet paper',
+'printer', 'tv stand', 'whiteboard', 'blanket', 'shower curtain', 'trash can', 'closet', 'stairs', 'microwave', 'stove', 'shoe', 'computer tower', 'bottle', 'bin', 'ottoman', 'bench', 'board', 'washing machine', 'mirror', 'copier',
+'basket', 'sofa chair', 'file cabinet', 'fan', 'laptop', 'shower', 'paper', 'person', 'paper towel dispenser', 'oven', 'blinds', 'rack', 'plate', 'blackboard', 'piano', 'suitcase', 'rail', 'radiator', 'recycling bin', 'container',
+'wardrobe', 'soap dispenser', 'telephone', 'bucket', 'clock', 'stand', 'light', 'laundry basket', 'pipe', 'clothes dryer', 'guitar', 'toilet paper holder', 'seat', 'speaker', 'column', 'bicycle', 'ladder', 'bathroom stall', 'shower wall',
+'cup', 'jacket', 'storage bin', 'coffee maker', 'dishwasher', 'paper towel roll', 'machine', 'mat', 'windowsill', 'bar', 'toaster', 'bulletin board', 'ironing board', 'fireplace', 'soap dish', 'kitchen counter', 'doorframe',
+'toilet paper dispenser', 'mini fridge', 'fire extinguisher', 'ball', 'hat', 'shower curtain rod', 'water cooler', 'paper cutter', 'tray', 'shower door', 'pillar', 'ledge', 'toaster oven', 'mouse', 'toilet seat cover dispenser',
+'furniture', 'cart', 'storage container', 'scale', 'tissue box', 'light switch', 'crate', 'power outlet', 'decoration', 'sign', 'projector', 'closet door', 'vacuum cleaner', 'candle', 'plunger', 'stuffed animal', 'headphones', 'dish rack',
+'broom', 'guitar case', 'range hood', 'dustpan', 'hair dryer', 'water bottle', 'handicap bar', 'purse', 'vent', 'shower floor', 'water pitcher', 'mailbox', 'bowl', 'paper bag', 'alarm clock', 'music stand', 'projector screen', 'divider',
+'laundry detergent', 'bathroom counter', 'object', 'bathroom vanity', 'closet wall', 'laundry hamper', 'bathroom stall door', 'ceiling light', 'trash bin', 'dumbbell', 'stair rail', 'tube', 'bathroom cabinet', 'cd case', 'closet rod',
+'coffee kettle', 'structure', 'shower head', 'keyboard piano', 'case of water bottles', 'coat rack', 'storage organizer', 'folded chair', 'fire alarm', 'power strip', 'calendar', 'poster', 'potted plant', 'luggage', 'mattress')
+
+train_pipeline = [
+ dict(
+ type='LoadPointsFromFile',
+ coord_type='DEPTH',
+ shift_height=False,
+ use_color=True,
+ load_dim=6,
+ use_dim=[0, 1, 2, 3, 4, 5]),
+ dict(
+ type='LoadAnnotations3D',
+ with_mask_3d=True,
+ with_seg_3d=True),
+ dict(type='GlobalAlignment', rotation_axis=2),
+ dict(type='PointSample', num_points=n_points),
+ dict(type='PointSegClassMappingV2',
+ valid_cat_ids=(2, 4, 5, 6, 7, 8, 9, 10, 11, 13, 14, 15, 16, 17, 18, 19, 21, 22, 23, 24, 26, 27, 28, 29, 31, 32, 33, 34, 35, 36, 38, 39, 40, 41, 42, 44, 45, 46, 47, 48, 49, 50, 51, 52, 54, 55, 56, 57, 58, 59, 62, 63, 64, 65, 66, 67, 68, 69, 70, 71,
+ 72, 73, 74, 75, 76, 77, 78, 79, 80, 82, 84, 86, 87, 88, 89, 90, 93, 95, 96, 97, 98, 99, 100, 101, 102, 103, 104, 105, 106, 107, 110, 112, 115, 116, 118, 120, 121, 122, 125, 128, 130, 131, 132, 134, 136, 138, 139, 140, 141, 145, 148, 154,
+ 155, 156, 157, 159, 161, 163, 165, 166, 168, 169, 170, 177, 180, 185, 188, 191, 193, 195, 202, 208, 213, 214, 221, 229, 230, 232, 233, 242, 250, 261, 264, 276, 283, 286, 300, 304, 312, 323, 325, 331, 342, 356, 370, 392, 395, 399, 408, 417,
+ 488, 540, 562, 570, 572, 581, 609, 748, 776, 1156, 1163, 1164, 1165, 1166, 1167, 1168, 1169, 1170, 1171, 1172, 1173, 1174, 1175, 1176, 1178, 1179, 1180, 1181, 1182, 1183, 1184, 1185, 1186, 1187, 1188, 1189, 1190, 1191),
+ max_cat_id=1357),
+ dict(
+ type='RandomFlip3D',
+ sync_2d=False,
+ flip_ratio_bev_horizontal=0.5,
+ flip_ratio_bev_vertical=0.5),
+ dict(
+ type='GlobalRotScaleTrans',
+ rot_range_z=[-3.14, 3.14],
+ rot_range_x_y=[-0.1308, 0.1308],
+ scale_ratio_range=[.8, 1.2],
+ translation_std=[.1, .1, .1],
+ shift_height=False),
+ dict(type='NormalizePointsColor', color_mean=None),
+ dict(type='DefaultFormatBundle3D', class_names=class_names),
+ dict(type='Collect3D', keys=['points', 'gt_bboxes_3d', 'gt_labels_3d',
+ 'pts_semantic_mask', 'pts_instance_mask'])
+]
+test_pipeline = [
+ dict(
+ type='LoadPointsFromFile',
+ coord_type='DEPTH',
+ shift_height=False,
+ use_color=True,
+ load_dim=6,
+ use_dim=[0, 1, 2, 3, 4, 5]),
+ dict(
+ type='MultiScaleFlipAug3D',
+ img_scale=(1333, 800),
+ pts_scale_ratio=1,
+ flip=False,
+ transforms=[
+ dict(type='NormalizePointsColor', color_mean=None),
+ dict(
+ type='DefaultFormatBundle3D',
+ class_names=class_names,
+ with_label=False),
+ dict(type='Collect3D', keys=['points'])
+ ])
+]
+data = dict(
+ samples_per_gpu=5,
+ workers_per_gpu=9,
+ train=dict(
+ type='RepeatDataset',
+ times=10,
+ dataset=dict(
+ type=dataset_type,
+ data_root=data_root,
+ ann_file=data_root + 'scannet200_infos_train.pkl',
+ pipeline=train_pipeline,
+ filter_empty_gt=True,
+ classes=class_names,
+ box_type_3d='Depth')),
+ val=dict(
+ type=dataset_type,
+ data_root=data_root,
+ ann_file=data_root + 'scannet200_infos_val.pkl',
+ pipeline=test_pipeline,
+ filter_empty_gt=False,
+ classes=class_names,
+ test_mode=True,
+ box_type_3d='Depth'),
+ test=dict(
+ type=dataset_type,
+ data_root=data_root,
+ ann_file=data_root + 'scannet200_infos_val.pkl',
+ pipeline=test_pipeline,
+ filter_empty_gt=False,
+ classes=class_names,
+ test_mode=True,
+ box_type_3d='Depth'))
diff --git a/configs/td3d_is/td3d_is_scannet200-3d-198class_public.py b/configs/td3d_is/td3d_is_scannet200-3d-198class_public.py
new file mode 100644
index 0000000..703c896
--- /dev/null
+++ b/configs/td3d_is/td3d_is_scannet200-3d-198class_public.py
@@ -0,0 +1,170 @@
+voxel_size = .02
+padding = .08
+n_points = 100000
+
+model = dict(
+ type='TD3DInstanceSegmentor',
+ voxel_size=voxel_size,
+ backbone=dict(type='MinkResNet', in_channels=3, depth=34, norm='batch', return_stem=True, stride=1),
+ neck=dict(
+ type='NgfcTinySegmentationNeck',
+ in_channels=(64, 128, 256, 512),
+ out_channels=128),
+ head=dict(
+ type='TD3DInstanceHead',
+ in_channels=128,
+ n_reg_outs=6,
+ n_classes=198,
+ n_levels=4,
+ padding=padding,
+ voxel_size=voxel_size,
+ unet=dict(
+ type='MinkUNet14B',
+ in_channels=32,
+ out_channels=3,
+ D=3),
+ first_assigner=dict(
+ type='NgfcV2Assigner',
+ min_pts_threshold=18,
+ top_pts_threshold=8,
+ padding=padding),
+ second_assigner=dict(
+ type='MaxIoU3DAssigner',
+ threshold=.25),
+ roi_extractor=dict(
+ type='Mink3DRoIExtractor',
+ voxel_size=voxel_size,
+ padding=padding,
+ min_pts_threshold=10)),
+ train_cfg=dict(num_rois=2),
+ test_cfg=dict(
+ nms_pre=300,
+ iou_thr=.4,
+ score_thr=.07,
+ binary_score_thr=0.2))
+
+optimizer = dict(type='AdamW', lr=0.001, weight_decay=0.0001)
+optimizer_config = dict(grad_clip=dict(max_norm=10, norm_type=2))
+lr_config = dict(policy='step', warmup=None, step=[28, 32])
+runner = dict(type='EpochBasedRunner', max_epochs=33)
+custom_hooks = [dict(type='EmptyCacheHook', after_iter=True)]
+
+checkpoint_config = dict(interval=1, max_keep_ckpts=40)
+log_config = dict(
+ interval=50,
+ hooks=[
+ dict(type='TextLoggerHook'),
+ # dict(type='TensorboardLoggerHook')
+])
+dist_params = dict(backend='nccl')
+log_level = 'INFO'
+work_dir = None
+load_from = None
+resume_from = None
+workflow = [('train', 1)]
+
+dataset_type = 'ScanNet200InstanceSegDataset'
+data_root = './data/scannet200/'
+class_names = ('chair', 'table', 'door', 'couch', 'cabinet', 'shelf', 'desk', 'office chair', 'bed', 'pillow', 'sink', 'picture', 'window', 'toilet', 'bookshelf', 'monitor', 'curtain', 'book', 'armchair', 'coffee table', 'box',
+'refrigerator', 'lamp', 'kitchen cabinet', 'towel', 'clothes', 'tv', 'nightstand', 'counter', 'dresser', 'stool', 'cushion', 'plant', 'ceiling', 'bathtub', 'end table', 'dining table', 'keyboard', 'bag', 'backpack', 'toilet paper',
+'printer', 'tv stand', 'whiteboard', 'blanket', 'shower curtain', 'trash can', 'closet', 'stairs', 'microwave', 'stove', 'shoe', 'computer tower', 'bottle', 'bin', 'ottoman', 'bench', 'board', 'washing machine', 'mirror', 'copier',
+'basket', 'sofa chair', 'file cabinet', 'fan', 'laptop', 'shower', 'paper', 'person', 'paper towel dispenser', 'oven', 'blinds', 'rack', 'plate', 'blackboard', 'piano', 'suitcase', 'rail', 'radiator', 'recycling bin', 'container',
+'wardrobe', 'soap dispenser', 'telephone', 'bucket', 'clock', 'stand', 'light', 'laundry basket', 'pipe', 'clothes dryer', 'guitar', 'toilet paper holder', 'seat', 'speaker', 'column', 'bicycle', 'ladder', 'bathroom stall', 'shower wall',
+'cup', 'jacket', 'storage bin', 'coffee maker', 'dishwasher', 'paper towel roll', 'machine', 'mat', 'windowsill', 'bar', 'toaster', 'bulletin board', 'ironing board', 'fireplace', 'soap dish', 'kitchen counter', 'doorframe',
+'toilet paper dispenser', 'mini fridge', 'fire extinguisher', 'ball', 'hat', 'shower curtain rod', 'water cooler', 'paper cutter', 'tray', 'shower door', 'pillar', 'ledge', 'toaster oven', 'mouse', 'toilet seat cover dispenser',
+'furniture', 'cart', 'storage container', 'scale', 'tissue box', 'light switch', 'crate', 'power outlet', 'decoration', 'sign', 'projector', 'closet door', 'vacuum cleaner', 'candle', 'plunger', 'stuffed animal', 'headphones', 'dish rack',
+'broom', 'guitar case', 'range hood', 'dustpan', 'hair dryer', 'water bottle', 'handicap bar', 'purse', 'vent', 'shower floor', 'water pitcher', 'mailbox', 'bowl', 'paper bag', 'alarm clock', 'music stand', 'projector screen', 'divider',
+'laundry detergent', 'bathroom counter', 'object', 'bathroom vanity', 'closet wall', 'laundry hamper', 'bathroom stall door', 'ceiling light', 'trash bin', 'dumbbell', 'stair rail', 'tube', 'bathroom cabinet', 'cd case', 'closet rod',
+'coffee kettle', 'structure', 'shower head', 'keyboard piano', 'case of water bottles', 'coat rack', 'storage organizer', 'folded chair', 'fire alarm', 'power strip', 'calendar', 'poster', 'potted plant', 'luggage', 'mattress')
+
+train_pipeline = [
+ dict(
+ type='LoadPointsFromFile',
+ coord_type='DEPTH',
+ shift_height=False,
+ use_color=True,
+ load_dim=6,
+ use_dim=[0, 1, 2, 3, 4, 5]),
+ dict(
+ type='LoadAnnotations3D',
+ with_mask_3d=True,
+ with_seg_3d=True),
+ dict(type='GlobalAlignment', rotation_axis=2),
+ dict(type='PointSample', num_points=n_points),
+ dict(type='PointSegClassMappingV2',
+ valid_cat_ids=(2, 4, 5, 6, 7, 8, 9, 10, 11, 13, 14, 15, 16, 17, 18, 19, 21, 22, 23, 24, 26, 27, 28, 29, 31, 32, 33, 34, 35, 36, 38, 39, 40, 41, 42, 44, 45, 46, 47, 48, 49, 50, 51, 52, 54, 55, 56, 57, 58, 59, 62, 63, 64, 65, 66, 67, 68, 69, 70, 71,
+ 72, 73, 74, 75, 76, 77, 78, 79, 80, 82, 84, 86, 87, 88, 89, 90, 93, 95, 96, 97, 98, 99, 100, 101, 102, 103, 104, 105, 106, 107, 110, 112, 115, 116, 118, 120, 121, 122, 125, 128, 130, 131, 132, 134, 136, 138, 139, 140, 141, 145, 148, 154,
+ 155, 156, 157, 159, 161, 163, 165, 166, 168, 169, 170, 177, 180, 185, 188, 191, 193, 195, 202, 208, 213, 214, 221, 229, 230, 232, 233, 242, 250, 261, 264, 276, 283, 286, 300, 304, 312, 323, 325, 331, 342, 356, 370, 392, 395, 399, 408, 417,
+ 488, 540, 562, 570, 572, 581, 609, 748, 776, 1156, 1163, 1164, 1165, 1166, 1167, 1168, 1169, 1170, 1171, 1172, 1173, 1174, 1175, 1176, 1178, 1179, 1180, 1181, 1182, 1183, 1184, 1185, 1186, 1187, 1188, 1189, 1190, 1191),
+ max_cat_id=1357),
+ dict(
+ type='RandomFlip3D',
+ sync_2d=False,
+ flip_ratio_bev_horizontal=0.5,
+ flip_ratio_bev_vertical=0.5),
+ dict(
+ type='GlobalRotScaleTrans',
+ rot_range_z=[-3.14, 3.14],
+ rot_range_x_y=[-0.1308, 0.1308],
+ scale_ratio_range=[.8, 1.2],
+ translation_std=[.1, .1, .1],
+ shift_height=False),
+ dict(type='NormalizePointsColor', color_mean=None),
+ dict(type='DefaultFormatBundle3D', class_names=class_names),
+ dict(type='Collect3D', keys=['points', 'gt_bboxes_3d', 'gt_labels_3d',
+ 'pts_semantic_mask', 'pts_instance_mask'])
+]
+test_pipeline = [
+ dict(
+ type='LoadPointsFromFile',
+ coord_type='DEPTH',
+ shift_height=False,
+ use_color=True,
+ load_dim=6,
+ use_dim=[0, 1, 2, 3, 4, 5]),
+ dict(
+ type='MultiScaleFlipAug3D',
+ img_scale=(1333, 800),
+ pts_scale_ratio=1,
+ flip=False,
+ transforms=[
+ dict(type='NormalizePointsColor', color_mean=None),
+ dict(
+ type='DefaultFormatBundle3D',
+ class_names=class_names,
+ with_label=False),
+ dict(type='Collect3D', keys=['points'])
+ ])
+]
+data = dict(
+ samples_per_gpu=5,
+ workers_per_gpu=9,
+ train=dict(
+ type='RepeatDataset',
+ times=10,
+ dataset=dict(
+ type=dataset_type,
+ data_root=data_root,
+ ann_file=data_root + 'scannet200_infos_train.pkl',
+ pipeline=train_pipeline,
+ filter_empty_gt=True,
+ classes=class_names,
+ box_type_3d='Depth')),
+ val=dict(
+ type=dataset_type,
+ data_root=data_root,
+ ann_file=data_root + 'scannet200_infos_val.pkl',
+ pipeline=test_pipeline,
+ filter_empty_gt=False,
+ classes=class_names,
+ test_mode=True,
+ box_type_3d='Depth'),
+ test=dict(
+ type=dataset_type,
+ data_root=data_root,
+ ann_file=data_root + 'scannet200_infos_val.pkl',
+ pipeline=test_pipeline,
+ filter_empty_gt=False,
+ classes=class_names,
+ test_mode=True,
+ box_type_3d='Depth'))
diff --git a/configs/votenet/README.md b/configs/votenet/README.md
new file mode 100644
index 0000000..d74486f
--- /dev/null
+++ b/configs/votenet/README.md
@@ -0,0 +1,68 @@
+# Deep Hough Voting for 3D Object Detection in Point Clouds
+
+> [Deep Hough Voting for 3D Object Detection in Point Clouds](https://arxiv.org/abs/1904.09664)
+
+
+
+## Abstract
+
+Current 3D object detection methods are heavily influenced by 2D detectors. In order to leverage architectures in 2D detectors, they often convert 3D point clouds to regular grids (i.e., to voxel grids or to bird's eye view images), or rely on detection in 2D images to propose 3D boxes. Few works have attempted to directly detect objects in point clouds. In this work, we return to first principles to construct a 3D detection pipeline for point cloud data and as generic as possible. However, due to the sparse nature of the data -- samples from 2D manifolds in 3D space -- we face a major challenge when directly predicting bounding box parameters from scene points: a 3D object centroid can be far from any surface point thus hard to regress accurately in one step. To address the challenge, we propose VoteNet, an end-to-end 3D object detection network based on a synergy of deep point set networks and Hough voting. Our model achieves state-of-the-art 3D detection on two large datasets of real 3D scans, ScanNet and SUN RGB-D with a simple design, compact model size and high efficiency. Remarkably, VoteNet outperforms previous methods by using purely geometric information without relying on color images.
+
+
+
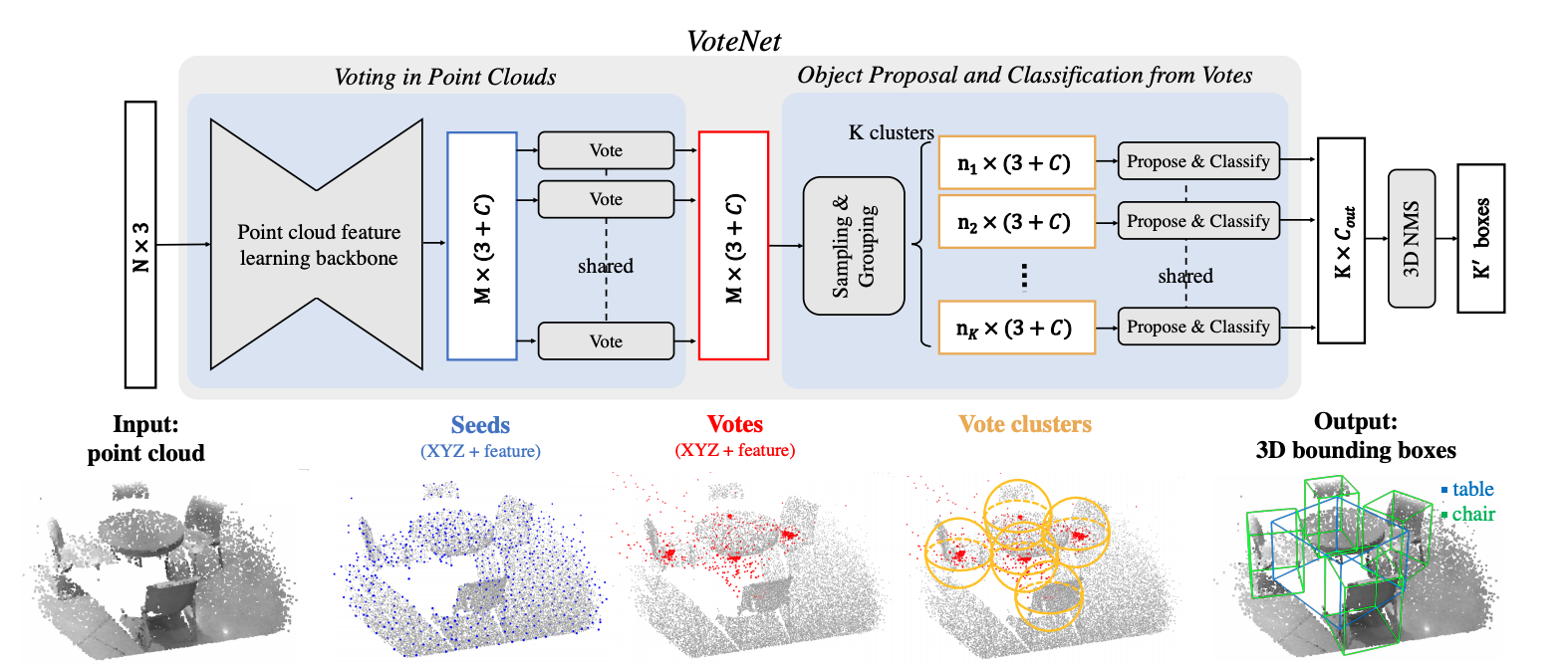
+
+
+## Introduction
+
+We implement VoteNet and provide the result and checkpoints on ScanNet and SUNRGBD datasets.
+
+## Results and models
+
+### ScanNet
+
+| Backbone | Lr schd | Mem (GB) | Inf time (fps) | AP@0.25 | AP@0.5 | Download |
+| :-----------------------------------------------: | :-----: | :------: | :------------: | :-----: | :----: | :----------------------------------------------------------------------------------------------------------------------------------------------------------------------------------------------------------------------------------------------------------------------------------------------------------------------------------------: |
+| [PointNet++](./votenet_8x8_scannet-3d-18class.py) | 3x | 4.1 | | 62.34 | 40.82 | [model](https://download.openmmlab.com/mmdetection3d/v1.0.0_models/votenet/votenet_8x8_scannet-3d-18class/votenet_8x8_scannet-3d-18class_20210823_234503-cf8134fa.pth) \| [log](https://download.openmmlab.com/mmdetection3d/v1.0.0_models/votenet/votenet_8x8_scannet-3d-18class/votenet_8x8_scannet-3d-18class_20210823_234503.log.json) |
+
+### SUNRGBD
+
+| Backbone | Lr schd | Mem (GB) | Inf time (fps) | AP@0.25 | AP@0.5 | Download |
+| :------------------------------------------------: | :-----: | :------: | :------------: | :-----: | :----: | :--------------------------------------------------------------------------------------------------------------------------------------------------------------------------------------------------------------------------------------------------------------------------------------------------------------------------------------------: |
+| [PointNet++](./votenet_16x8_sunrgbd-3d-10class.py) | 3x | 8.1 | | 59.78 | 35.77 | [model](https://download.openmmlab.com/mmdetection3d/v1.0.0_models/votenet/votenet_16x8_sunrgbd-3d-10class/votenet_16x8_sunrgbd-3d-10class_20210820_162823-bf11f014.pth) \| [log](https://download.openmmlab.com/mmdetection3d/v1.0.0_models/votenet/votenet_16x8_sunrgbd-3d-10class/votenet_16x8_sunrgbd-3d-10class_20210820_162823.log.json) |
+
+**Notice**: If your current mmdetection3d version >= 0.6.0, and you are using the checkpoints downloaded from the above links or using checkpoints trained with mmdetection3d version \< 0.6.0, the checkpoints have to be first converted via [tools/model_converters/convert_votenet_checkpoints.py](../../tools/model_converters/convert_votenet_checkpoints.py):
+
+```
+python ./tools/model_converters/convert_votenet_checkpoints.py ${ORIGINAL_CHECKPOINT_PATH} --out=${NEW_CHECKPOINT_PATH}
+```
+
+Then you can use the converted checkpoints following [getting_started.md](../../docs/en/getting_started.md).
+
+## Indeterminism
+
+Since test data preparation randomly downsamples the points, and the test script uses fixed random seeds while the random seeds of validation in training are not fixed, the test results may be slightly different from the results reported above.
+
+## IoU loss
+
+Adding IoU loss (simply = 1-IoU) boosts VoteNet's performance. To use IoU loss, add this loss term to the config file:
+
+```python
+iou_loss=dict(type='AxisAlignedIoULoss', reduction='sum', loss_weight=10.0 / 3.0)
+```
+
+| Backbone | Lr schd | Mem (GB) | Inf time (fps) | AP@0.25 | AP@0.5 | Download |
+| :-------------------------------------------------------: | :-----: | :------: | :------------: | :-----: | :----: | :------: |
+| [PointNet++](./votenet_iouloss_8x8_scannet-3d-18class.py) | 3x | 4.1 | | 63.81 | 44.21 | / |
+
+For now, we only support calculating IoU loss for axis-aligned bounding boxes since the CUDA op of general 3D IoU calculation does not implement the backward method. Therefore, IoU loss can only be used for ScanNet dataset for now.
+
+## Citation
+
+```latex
+@inproceedings{qi2019deep,
+ author = {Qi, Charles R and Litany, Or and He, Kaiming and Guibas, Leonidas J},
+ title = {Deep Hough Voting for 3D Object Detection in Point Clouds},
+ booktitle = {Proceedings of the IEEE International Conference on Computer Vision},
+ year = {2019}
+}
+```
diff --git a/configs/votenet/metafile.yml b/configs/votenet/metafile.yml
new file mode 100644
index 0000000..cd18680
--- /dev/null
+++ b/configs/votenet/metafile.yml
@@ -0,0 +1,59 @@
+Collections:
+ - Name: VoteNet
+ Metadata:
+ Training Techniques:
+ - AdamW
+ Training Resources: 8x V100 GPUs
+ Architecture:
+ - PointNet++
+ Paper:
+ URL: https://arxiv.org/abs/1904.09664
+ Title: 'Deep Hough Voting for 3D Object Detection in Point Clouds'
+ README: configs/votenet/README.md
+ Code:
+ URL: https://github.com/open-mmlab/mmdetection3d/blob/master/mmdet3d/models/detectors/votenet.py#L10
+ Version: v0.5.0
+
+Models:
+ - Name: votenet_16x8_sunrgbd-3d-10class.py
+ In Collection: VoteNet
+ Config: configs/votenet/votenet_16x8_sunrgbd-3d-10class.py
+ Metadata:
+ Training Data: SUNRGBD
+ Training Memory (GB): 8.1
+ Results:
+ - Task: 3D Object Detection
+ Dataset: SUNRGBD
+ Metrics:
+ AP@0.25: 59.78
+ AP@0.5: 35.77
+ Weights: https://download.openmmlab.com/mmdetection3d/v1.0.0_models/votenet/votenet_16x8_sunrgbd-3d-10class/votenet_16x8_sunrgbd-3d-10class_20210820_162823-bf11f014.pth
+
+ - Name: votenet_8x8_scannet-3d-18class.py
+ In Collection: VoteNet
+ Config: configs/votenet/votenet_8x8_scannet-3d-18class.py
+ Metadata:
+ Training Data: ScanNet
+ Training Memory (GB): 4.1
+ Results:
+ - Task: 3D Object Detection
+ Dataset: ScanNet
+ Metrics:
+ AP@0.25: 62.34
+ AP@0.5: 40.82
+ Weights: https://download.openmmlab.com/mmdetection3d/v1.0.0_models/votenet/votenet_8x8_scannet-3d-18class/votenet_8x8_scannet-3d-18class_20210823_234503-cf8134fa.pth
+
+ - Name: votenet_iouloss_8x8_scannet-3d-18class
+ In Collection: VoteNet
+ Config: configs/votenet/votenet_iouloss_8x8_scannet-3d-18class.py
+ Metadata:
+ Training Data: ScanNet
+ Training Memory (GB): 4.1
+ Architecture:
+ - IoU Loss
+ Results:
+ - Task: 3D Object Detection
+ Dataset: ScanNet
+ Metrics:
+ AP@0.25: 63.81
+ AP@0.5: 44.21
diff --git a/configs/votenet/votenet_16x8_sunrgbd-3d-10class.py b/configs/votenet/votenet_16x8_sunrgbd-3d-10class.py
new file mode 100644
index 0000000..5ddfa7a
--- /dev/null
+++ b/configs/votenet/votenet_16x8_sunrgbd-3d-10class.py
@@ -0,0 +1,21 @@
+_base_ = [
+ '../_base_/datasets/sunrgbd-3d-10class.py', '../_base_/models/votenet.py',
+ '../_base_/schedules/schedule_3x.py', '../_base_/default_runtime.py'
+]
+# model settings
+model = dict(
+ bbox_head=dict(
+ num_classes=10,
+ bbox_coder=dict(
+ type='PartialBinBasedBBoxCoder',
+ num_sizes=10,
+ num_dir_bins=12,
+ with_rot=True,
+ mean_sizes=[
+ [2.114256, 1.620300, 0.927272], [0.791118, 1.279516, 0.718182],
+ [0.923508, 1.867419, 0.845495], [0.591958, 0.552978, 0.827272],
+ [0.699104, 0.454178, 0.75625], [0.69519, 1.346299, 0.736364],
+ [0.528526, 1.002642, 1.172878], [0.500618, 0.632163, 0.683424],
+ [0.404671, 1.071108, 1.688889], [0.76584, 1.398258, 0.472728]
+ ]),
+ ))
diff --git a/configs/votenet/votenet_8x8_scannet-3d-18class.py b/configs/votenet/votenet_8x8_scannet-3d-18class.py
new file mode 100644
index 0000000..62e5630
--- /dev/null
+++ b/configs/votenet/votenet_8x8_scannet-3d-18class.py
@@ -0,0 +1,36 @@
+_base_ = [
+ '../_base_/datasets/scannet-3d-18class.py', '../_base_/models/votenet.py',
+ '../_base_/schedules/schedule_3x.py', '../_base_/default_runtime.py'
+]
+
+# model settings
+model = dict(
+ bbox_head=dict(
+ num_classes=18,
+ bbox_coder=dict(
+ type='PartialBinBasedBBoxCoder',
+ num_sizes=18,
+ num_dir_bins=1,
+ with_rot=False,
+ mean_sizes=[[0.76966727, 0.8116021, 0.92573744],
+ [1.876858, 1.8425595, 1.1931566],
+ [0.61328, 0.6148609, 0.7182701],
+ [1.3955007, 1.5121545, 0.83443564],
+ [0.97949594, 1.0675149, 0.6329687],
+ [0.531663, 0.5955577, 1.7500148],
+ [0.9624706, 0.72462326, 1.1481868],
+ [0.83221924, 1.0490936, 1.6875663],
+ [0.21132214, 0.4206159, 0.5372846],
+ [1.4440073, 1.8970833, 0.26985747],
+ [1.0294262, 1.4040797, 0.87554324],
+ [1.3766412, 0.65521795, 1.6813129],
+ [0.6650819, 0.71111923, 1.298853],
+ [0.41999173, 0.37906948, 1.7513971],
+ [0.59359556, 0.5912492, 0.73919016],
+ [0.50867593, 0.50656086, 0.30136237],
+ [1.1511526, 1.0546296, 0.49706793],
+ [0.47535285, 0.49249494, 0.5802117]])))
+
+# yapf:disable
+log_config = dict(interval=30)
+# yapf:enable
diff --git a/configs/votenet/votenet_iouloss_8x8_scannet-3d-18class.py b/configs/votenet/votenet_iouloss_8x8_scannet-3d-18class.py
new file mode 100644
index 0000000..ac2a6c0
--- /dev/null
+++ b/configs/votenet/votenet_iouloss_8x8_scannet-3d-18class.py
@@ -0,0 +1,8 @@
+_base_ = ['./votenet_8x8_scannet-3d-18class.py']
+
+# model settings, add iou loss
+model = dict(
+ bbox_head=dict(
+ iou_loss=dict(
+ type='AxisAlignedIoULoss', reduction='sum', loss_weight=10.0 /
+ 3.0)))
diff --git a/demo/mono_det_demo.py b/demo/mono_det_demo.py
new file mode 100644
index 0000000..c2bd502
--- /dev/null
+++ b/demo/mono_det_demo.py
@@ -0,0 +1,46 @@
+# Copyright (c) OpenMMLab. All rights reserved.
+from argparse import ArgumentParser
+
+from mmdet3d.apis import (inference_mono_3d_detector, init_model,
+ show_result_meshlab)
+
+
+def main():
+ parser = ArgumentParser()
+ parser.add_argument('image', help='image file')
+ parser.add_argument('ann', help='ann file')
+ parser.add_argument('config', help='Config file')
+ parser.add_argument('checkpoint', help='Checkpoint file')
+ parser.add_argument(
+ '--device', default='cuda:0', help='Device used for inference')
+ parser.add_argument(
+ '--score-thr', type=float, default=0.15, help='bbox score threshold')
+ parser.add_argument(
+ '--out-dir', type=str, default='demo', help='dir to save results')
+ parser.add_argument(
+ '--show',
+ action='store_true',
+ help='show online visualization results')
+ parser.add_argument(
+ '--snapshot',
+ action='store_true',
+ help='whether to save online visualization results')
+ args = parser.parse_args()
+
+ # build the model from a config file and a checkpoint file
+ model = init_model(args.config, args.checkpoint, device=args.device)
+ # test a single image
+ result, data = inference_mono_3d_detector(model, args.image, args.ann)
+ # show the results
+ show_result_meshlab(
+ data,
+ result,
+ args.out_dir,
+ args.score_thr,
+ show=args.show,
+ snapshot=args.snapshot,
+ task='mono-det')
+
+
+if __name__ == '__main__':
+ main()
diff --git a/demo/multi_modality_demo.py b/demo/multi_modality_demo.py
new file mode 100644
index 0000000..9ef1969
--- /dev/null
+++ b/demo/multi_modality_demo.py
@@ -0,0 +1,48 @@
+# Copyright (c) OpenMMLab. All rights reserved.
+from argparse import ArgumentParser
+
+from mmdet3d.apis import (inference_multi_modality_detector, init_model,
+ show_result_meshlab)
+
+
+def main():
+ parser = ArgumentParser()
+ parser.add_argument('pcd', help='Point cloud file')
+ parser.add_argument('image', help='image file')
+ parser.add_argument('ann', help='ann file')
+ parser.add_argument('config', help='Config file')
+ parser.add_argument('checkpoint', help='Checkpoint file')
+ parser.add_argument(
+ '--device', default='cuda:0', help='Device used for inference')
+ parser.add_argument(
+ '--score-thr', type=float, default=0.0, help='bbox score threshold')
+ parser.add_argument(
+ '--out-dir', type=str, default='demo', help='dir to save results')
+ parser.add_argument(
+ '--show',
+ action='store_true',
+ help='show online visualization results')
+ parser.add_argument(
+ '--snapshot',
+ action='store_true',
+ help='whether to save online visualization results')
+ args = parser.parse_args()
+
+ # build the model from a config file and a checkpoint file
+ model = init_model(args.config, args.checkpoint, device=args.device)
+ # test a single image
+ result, data = inference_multi_modality_detector(model, args.pcd,
+ args.image, args.ann)
+ # show the results
+ show_result_meshlab(
+ data,
+ result,
+ args.out_dir,
+ args.score_thr,
+ show=args.show,
+ snapshot=args.snapshot,
+ task='multi_modality-det')
+
+
+if __name__ == '__main__':
+ main()
diff --git a/demo/pc_seg_demo.py b/demo/pc_seg_demo.py
new file mode 100644
index 0000000..c80c3f4
--- /dev/null
+++ b/demo/pc_seg_demo.py
@@ -0,0 +1,42 @@
+# Copyright (c) OpenMMLab. All rights reserved.
+from argparse import ArgumentParser
+
+from mmdet3d.apis import inference_segmentor, init_model, show_result_meshlab
+
+
+def main():
+ parser = ArgumentParser()
+ parser.add_argument('pcd', help='Point cloud file')
+ parser.add_argument('config', help='Config file')
+ parser.add_argument('checkpoint', help='Checkpoint file')
+ parser.add_argument(
+ '--device', default='cuda:0', help='Device used for inference')
+ parser.add_argument(
+ '--out-dir', type=str, default='demo', help='dir to save results')
+ parser.add_argument(
+ '--show',
+ action='store_true',
+ help='show online visualization results')
+ parser.add_argument(
+ '--snapshot',
+ action='store_true',
+ help='whether to save online visualization results')
+ args = parser.parse_args()
+
+ # build the model from a config file and a checkpoint file
+ model = init_model(args.config, args.checkpoint, device=args.device)
+ # test a single image
+ result, data = inference_segmentor(model, args.pcd)
+ # show the results
+ show_result_meshlab(
+ data,
+ result,
+ args.out_dir,
+ show=args.show,
+ snapshot=args.snapshot,
+ task='seg',
+ palette=model.PALETTE)
+
+
+if __name__ == '__main__':
+ main()
diff --git a/demo/pcd_demo.py b/demo/pcd_demo.py
new file mode 100644
index 0000000..5822714
--- /dev/null
+++ b/demo/pcd_demo.py
@@ -0,0 +1,44 @@
+# Copyright (c) OpenMMLab. All rights reserved.
+from argparse import ArgumentParser
+
+from mmdet3d.apis import inference_detector, init_model, show_result_meshlab
+
+
+def main():
+ parser = ArgumentParser()
+ parser.add_argument('pcd', help='Point cloud file')
+ parser.add_argument('config', help='Config file')
+ parser.add_argument('checkpoint', help='Checkpoint file')
+ parser.add_argument(
+ '--device', default='cuda:0', help='Device used for inference')
+ parser.add_argument(
+ '--score-thr', type=float, default=0.0, help='bbox score threshold')
+ parser.add_argument(
+ '--out-dir', type=str, default='demo', help='dir to save results')
+ parser.add_argument(
+ '--show',
+ action='store_true',
+ help='show online visualization results')
+ parser.add_argument(
+ '--snapshot',
+ action='store_true',
+ help='whether to save online visualization results')
+ args = parser.parse_args()
+
+ # build the model from a config file and a checkpoint file
+ model = init_model(args.config, args.checkpoint, device=args.device)
+ # test a single image
+ result, data = inference_detector(model, args.pcd)
+ # show the results
+ show_result_meshlab(
+ data,
+ result,
+ args.out_dir,
+ args.score_thr,
+ show=args.show,
+ snapshot=args.snapshot,
+ task='det')
+
+
+if __name__ == '__main__':
+ main()
diff --git a/docker/Dockerfile b/docker/Dockerfile
new file mode 100644
index 0000000..953bd51
--- /dev/null
+++ b/docker/Dockerfile
@@ -0,0 +1,32 @@
+ARG PYTORCH="1.9.1"
+ARG CUDA="11.1"
+ARG CUDNN="8"
+
+FROM pytorch/pytorch:${PYTORCH}-cuda${CUDA}-cudnn${CUDNN}-devel
+
+RUN apt-key adv --fetch-keys https://developer.download.nvidia.com/compute/cuda/repos/ubuntu1804/x86_64/3bf863cc.pub \
+ && apt-key adv --fetch-keys https://developer.download.nvidia.com/compute/machine-learning/repos/ubuntu1804/x86_64/7fa2af80.pub \
+ && apt-get update \
+ && apt-get install -y ffmpeg libsm6 libxext6 git ninja-build libglib2.0-0 libsm6 libxrender-dev libxext6 \
+ && apt-get clean \
+ && rm -rf /var/lib/apt/lists/*
+
+# Install MMCV, MMDetection and MMSegmentation
+RUN pip install mmcv-full==1.6.0 -f https://download.openmmlab.com/mmcv/dist/cu111/torch1.10.0/index.html
+RUN pip install mmdet==2.24.1
+RUN pip install mmsegmentation==0.24.1
+
+# Install albumentation
+RUN pip install albumentations==1.3.0
+
+# Install MMDetection3D
+RUN git clone https://github.com/samsunglabs/td3d.git /mmdetection3d
+WORKDIR /mmdetection3d
+RUN pip install --no-cache-dir -e .
+
+# Install MinkowskiEngine
+RUN conda install openblas-devel -c anaconda
+RUN pip install -U git+https://github.com/NVIDIA/MinkowskiEngine@405b39cb7e68c2ec1b4ac8c4b4ca9fa6fcfcb2fc -v --no-deps \
+ --install-option="--blas_include_dirs=/opt/conda/include" \
+ --install-option="--blas=openblas" \
+ --install-option="--force_cuda"
diff --git a/docker/serve/Dockerfile b/docker/serve/Dockerfile
new file mode 100644
index 0000000..d4c1a39
--- /dev/null
+++ b/docker/serve/Dockerfile
@@ -0,0 +1,54 @@
+ARG PYTORCH="1.6.0"
+ARG CUDA="10.1"
+ARG CUDNN="7"
+FROM pytorch/pytorch:${PYTORCH}-cuda${CUDA}-cudnn${CUDNN}-devel
+
+ARG MMCV="1.3.8"
+ARG MMSEGMENTATION="0.14.1"
+ARG MMDET="2.14.0"
+ARG MMDET3D="0.17.1"
+
+ENV PYTHONUNBUFFERED TRUE
+
+RUN apt-get update && \
+ DEBIAN_FRONTEND=noninteractive apt-get install --no-install-recommends -y \
+ ca-certificates \
+ g++ \
+ openjdk-11-jre-headless \
+ # MMDet3D Requirements
+ ffmpeg libsm6 libxext6 git ninja-build libglib2.0-0 libsm6 libxrender-dev libxext6 \
+ && rm -rf /var/lib/apt/lists/*
+
+ENV PATH="/opt/conda/bin:$PATH"
+RUN export FORCE_CUDA=1
+
+# TORCHSEVER
+RUN pip install torchserve torch-model-archiver
+
+# MMLAB
+ARG PYTORCH
+ARG CUDA
+RUN ["/bin/bash", "-c", "pip install mmcv-full==${MMCV} -f https://download.openmmlab.com/mmcv/dist/cu${CUDA//./}/torch${PYTORCH}/index.html"]
+RUN pip install mmdet==${MMDET}
+RUN pip install mmsegmentation==${MMSEGMENTATION}
+RUN pip install mmdet3d==${MMDET3D}
+
+
+RUN useradd -m model-server \
+ && mkdir -p /home/model-server/tmp
+
+COPY entrypoint.sh /usr/local/bin/entrypoint.sh
+
+RUN chmod +x /usr/local/bin/entrypoint.sh \
+ && chown -R model-server /home/model-server
+
+COPY config.properties /home/model-server/config.properties
+RUN mkdir /home/model-server/model-store && chown -R model-server /home/model-server/model-store
+
+EXPOSE 8080 8081 8082
+
+USER model-server
+WORKDIR /home/model-server
+ENV TEMP=/home/model-server/tmp
+ENTRYPOINT ["/usr/local/bin/entrypoint.sh"]
+CMD ["serve"]
diff --git a/docker/serve/config.properties b/docker/serve/config.properties
new file mode 100644
index 0000000..efb9c47
--- /dev/null
+++ b/docker/serve/config.properties
@@ -0,0 +1,5 @@
+inference_address=http://0.0.0.0:8080
+management_address=http://0.0.0.0:8081
+metrics_address=http://0.0.0.0:8082
+model_store=/home/model-server/model-store
+load_models=all
diff --git a/docker/serve/entrypoint.sh b/docker/serve/entrypoint.sh
new file mode 100644
index 0000000..41ba00b
--- /dev/null
+++ b/docker/serve/entrypoint.sh
@@ -0,0 +1,12 @@
+#!/bin/bash
+set -e
+
+if [[ "$1" = "serve" ]]; then
+ shift 1
+ torchserve --start --ts-config /home/model-server/config.properties
+else
+ eval "$@"
+fi
+
+# prevent docker exit
+tail -f /dev/null
diff --git a/docs/en/1_exist_data_model.md b/docs/en/1_exist_data_model.md
new file mode 100644
index 0000000..96039c9
--- /dev/null
+++ b/docs/en/1_exist_data_model.md
@@ -0,0 +1,262 @@
+# 1: Inference and train with existing models and standard datasets
+
+## Inference with existing models
+
+Here we provide testing scripts to evaluate a whole dataset (SUNRGBD, ScanNet, KITTI, etc.).
+
+For high-level apis easier to integrated into other projects and basic demos, please refer to Verification/Demo under [Get Started](https://mmdetection3d.readthedocs.io/en/latest/getting_started.html).
+
+### Test existing models on standard datasets
+
+- single GPU
+- CPU
+- single node multiple GPU
+- multiple node
+
+You can use the following commands to test a dataset.
+
+```shell
+# single-gpu testing
+python tools/test.py ${CONFIG_FILE} ${CHECKPOINT_FILE} [--out ${RESULT_FILE}] [--eval ${EVAL_METRICS}] [--show] [--show-dir ${SHOW_DIR}]
+
+# CPU: disable GPUs and run single-gpu testing script (experimental)
+export CUDA_VISIBLE_DEVICES=-1
+python tools/test.py ${CONFIG_FILE} ${CHECKPOINT_FILE} [--out ${RESULT_FILE}] [--eval ${EVAL_METRICS}] [--show] [--show-dir ${SHOW_DIR}]
+
+# multi-gpu testing
+./tools/dist_test.sh ${CONFIG_FILE} ${CHECKPOINT_FILE} ${GPU_NUM} [--out ${RESULT_FILE}] [--eval ${EVAL_METRICS}]
+```
+
+**Note**:
+
+For now, CPU testing is only supported for SMOKE.
+
+Optional arguments:
+
+- `RESULT_FILE`: Filename of the output results in pickle format. If not specified, the results will not be saved to a file.
+- `EVAL_METRICS`: Items to be evaluated on the results. Allowed values depend on the dataset. Typically we default to use official metrics for evaluation on different datasets, so it can be simply set to `mAP` as a placeholder for detection tasks, which applies to nuScenes, Lyft, ScanNet and SUNRGBD. For KITTI, if we only want to evaluate the 2D detection performance, we can simply set the metric to `img_bbox` (unstable, stay tuned). For Waymo, we provide both KITTI-style evaluation (unstable) and Waymo-style official protocol, corresponding to metric `kitti` and `waymo` respectively. We recommend to use the default official metric for stable performance and fair comparison with other methods. Similarly, the metric can be set to `mIoU` for segmentation tasks, which applies to S3DIS and ScanNet.
+- `--show`: If specified, detection results will be plotted in the silient mode. It is only applicable to single GPU testing and used for debugging and visualization. This should be used with `--show-dir`.
+- `--show-dir`: If specified, detection results will be plotted on the `***_points.obj` and `***_pred.obj` files in the specified directory. It is only applicable to single GPU testing and used for debugging and visualization. You do NOT need a GUI available in your environment for using this option.
+
+Examples:
+
+Assume that you have already downloaded the checkpoints to the directory `checkpoints/`.
+
+1. Test VoteNet on ScanNet and save the points and prediction visualization results.
+
+ ```shell
+ python tools/test.py configs/votenet/votenet_8x8_scannet-3d-18class.py \
+ checkpoints/votenet_8x8_scannet-3d-18class_20200620_230238-2cea9c3a.pth \
+ --show --show-dir ./data/scannet/show_results
+ ```
+
+2. Test VoteNet on ScanNet, save the points, prediction, groundtruth visualization results, and evaluate the mAP.
+
+ ```shell
+ python tools/test.py configs/votenet/votenet_8x8_scannet-3d-18class.py \
+ checkpoints/votenet_8x8_scannet-3d-18class_20200620_230238-2cea9c3a.pth \
+ --eval mAP
+ --eval-options 'show=True' 'out_dir=./data/scannet/show_results'
+ ```
+
+3. Test VoteNet on ScanNet (without saving the test results) and evaluate the mAP.
+
+ ```shell
+ python tools/test.py configs/votenet/votenet_8x8_scannet-3d-18class.py \
+ checkpoints/votenet_8x8_scannet-3d-18class_20200620_230238-2cea9c3a.pth \
+ --eval mAP
+ ```
+
+4. Test SECOND on KITTI with 8 GPUs, and evaluate the mAP.
+
+ ```shell
+ ./tools/slurm_test.sh ${PARTITION} ${JOB_NAME} configs/second/hv_second_secfpn_6x8_80e_kitti-3d-3class.py \
+ checkpoints/hv_second_secfpn_6x8_80e_kitti-3d-3class_20200620_230238-9208083a.pth \
+ --out results.pkl --eval mAP
+ ```
+
+5. Test PointPillars on nuScenes with 8 GPUs, and generate the json file to be submit to the official evaluation server.
+
+ ```shell
+ ./tools/slurm_test.sh ${PARTITION} ${JOB_NAME} configs/pointpillars/hv_pointpillars_fpn_sbn-all_4x8_2x_nus-3d.py \
+ checkpoints/hv_pointpillars_fpn_sbn-all_4x8_2x_nus-3d_20200620_230405-2fa62f3d.pth \
+ --format-only --eval-options 'jsonfile_prefix=./pointpillars_nuscenes_results'
+ ```
+
+ The generated results be under `./pointpillars_nuscenes_results` directory.
+
+6. Test SECOND on KITTI with 8 GPUs, and generate the pkl files and submission data to be submit to the official evaluation server.
+
+ ```shell
+ ./tools/slurm_test.sh ${PARTITION} ${JOB_NAME} configs/second/hv_second_secfpn_6x8_80e_kitti-3d-3class.py \
+ checkpoints/hv_second_secfpn_6x8_80e_kitti-3d-3class_20200620_230238-9208083a.pth \
+ --format-only --eval-options 'pklfile_prefix=./second_kitti_results' 'submission_prefix=./second_kitti_results'
+ ```
+
+ The generated results be under `./second_kitti_results` directory.
+
+7. Test PointPillars on Lyft with 8 GPUs, generate the pkl files and make a submission to the leaderboard.
+
+ ```shell
+ ./tools/slurm_test.sh ${PARTITION} ${JOB_NAME} configs/pointpillars/hv_pointpillars_fpn_sbn-2x8_2x_lyft-3d.py \
+ checkpoints/hv_pointpillars_fpn_sbn-2x8_2x_lyft-3d_latest.pth --out results/pp_lyft/results_challenge.pkl \
+ --format-only --eval-options 'jsonfile_prefix=results/pp_lyft/results_challenge' \
+ 'csv_savepath=results/pp_lyft/results_challenge.csv'
+ ```
+
+ **Notice**: To generate submissions on Lyft, `csv_savepath` must be given in the `--eval-options`. After generating the csv file, you can make a submission with kaggle commands given on the [website](https://www.kaggle.com/c/3d-object-detection-for-autonomous-vehicles/submit).
+
+ Note that in the [config of Lyft dataset](../../configs/_base_/datasets/lyft-3d.py), the value of `ann_file` keyword in `test` is `data_root + 'lyft_infos_test.pkl'`, which is the official test set of Lyft without annotation. To test on the validation set, please change this to `data_root + 'lyft_infos_val.pkl'`.
+
+8. Test PointPillars on waymo with 8 GPUs, and evaluate the mAP with waymo metrics.
+
+ ```shell
+ ./tools/slurm_test.sh ${PARTITION} ${JOB_NAME} configs/pointpillars/hv_pointpillars_secfpn_sbn-2x16_2x_waymo-3d-car.py \
+ checkpoints/hv_pointpillars_secfpn_sbn-2x16_2x_waymo-3d-car_latest.pth --out results/waymo-car/results_eval.pkl \
+ --eval waymo --eval-options 'pklfile_prefix=results/waymo-car/kitti_results' \
+ 'submission_prefix=results/waymo-car/kitti_results'
+ ```
+
+ **Notice**: For evaluation on waymo, please follow the [instruction](https://github.com/waymo-research/waymo-open-dataset/blob/master/docs/quick_start.md/) to build the binary file `compute_detection_metrics_main` for metrics computation and put it into `mmdet3d/core/evaluation/waymo_utils/`.(Sometimes when using bazel to build `compute_detection_metrics_main`, an error `'round' is not a member of 'std'` may appear. We just need to remove the `std::` before `round` in that file.) `pklfile_prefix` should be given in the `--eval-options` for the bin file generation. For metrics, `waymo` is the recommended official evaluation prototype. Currently, evaluating with choice `kitti` is adapted from KITTI and the results for each difficulty are not exactly the same as the definition of KITTI. Instead, most of objects are marked with difficulty 0 currently, which will be fixed in the future. The reasons of its instability include the large computation for evaluation, the lack of occlusion and truncation in the converted data, different definition of difficulty and different methods of computing average precision.
+
+9. Test PointPillars on waymo with 8 GPUs, generate the bin files and make a submission to the leaderboard.
+
+ ```shell
+ ./tools/slurm_test.sh ${PARTITION} ${JOB_NAME} configs/pointpillars/hv_pointpillars_secfpn_sbn-2x16_2x_waymo-3d-car.py \
+ checkpoints/hv_pointpillars_secfpn_sbn-2x16_2x_waymo-3d-car_latest.pth --out results/waymo-car/results_eval.pkl \
+ --format-only --eval-options 'pklfile_prefix=results/waymo-car/kitti_results' \
+ 'submission_prefix=results/waymo-car/kitti_results'
+ ```
+
+ **Notice**: After generating the bin file, you can simply build the binary file `create_submission` and use them to create a submission file by following the [instruction](https://github.com/waymo-research/waymo-open-dataset/blob/master/docs/quick_start.md/). For evaluation on the validation set with the eval server, you can also use the same way to generate a submission.
+
+## Train predefined models on standard datasets
+
+MMDetection3D implements distributed training and non-distributed training,
+which uses `MMDistributedDataParallel` and `MMDataParallel` respectively.
+
+All outputs (log files and checkpoints) will be saved to the working directory,
+which is specified by `work_dir` in the config file.
+
+By default we evaluate the model on the validation set after each epoch, you can change the evaluation interval by adding the interval argument in the training config.
+
+```python
+evaluation = dict(interval=12) # This evaluate the model per 12 epoch.
+```
+
+**Important**: The default learning rate in config files is for 8 GPUs and the exact batch size is marked by the config's file name, e.g. '2x8' means 2 samples per GPU using 8 GPUs.
+According to the [Linear Scaling Rule](https://arxiv.org/abs/1706.02677), you need to set the learning rate proportional to the batch size if you use different GPUs or images per GPU, e.g., lr=0.01 for 4 GPUs * 2 img/gpu and lr=0.08 for 16 GPUs * 4 img/gpu. However, since most of the models in this repo use ADAM rather than SGD for optimization, the rule may not hold and users need to tune the learning rate by themselves.
+
+### Train with a single GPU
+
+```shell
+python tools/train.py ${CONFIG_FILE} [optional arguments]
+```
+
+If you want to specify the working directory in the command, you can add an argument `--work-dir ${YOUR_WORK_DIR}`.
+
+### Training with CPU (experimental)
+
+The process of training on the CPU is consistent with single GPU training. We just need to disable GPUs before the training process.
+
+```shell
+export CUDA_VISIBLE_DEVICES=-1
+```
+
+And then run the script of train with a single GPU.
+
+**Note**:
+
+For now, most of the point cloud related algorithms rely on 3D CUDA op, which can not be trained on CPU. Some monocular 3D object detection algorithms, like FCOS3D and SMOKE can be trained on CPU. We do not recommend users to use CPU for training because it is too slow. We support this feature to allow users to debug certain models on machines without GPU for convenience.
+
+### Train with multiple GPUs
+
+```shell
+./tools/dist_train.sh ${CONFIG_FILE} ${GPU_NUM} [optional arguments]
+```
+
+Optional arguments are:
+
+- `--no-validate` (**not suggested**): By default, the codebase will perform evaluation at every k (default value is 1, which can be modified like [this](https://github.com/open-mmlab/mmdetection3d/blob/master/configs/fcos3d/fcos3d_r101_caffe_fpn_gn-head_dcn_2x8_1x_nus-mono3d.py#L75)) epochs during the training. To disable this behavior, use `--no-validate`.
+- `--work-dir ${WORK_DIR}`: Override the working directory specified in the config file.
+- `--resume-from ${CHECKPOINT_FILE}`: Resume from a previous checkpoint file.
+- `--options 'Key=value'`: Override some settings in the used config.
+
+Difference between `resume-from` and `load-from`:
+
+- `resume-from` loads both the model weights and optimizer status, and the epoch is also inherited from the specified checkpoint. It is usually used for resuming the training process that is interrupted accidentally.
+- `load-from` only loads the model weights and the training epoch starts from 0. It is usually used for finetuning.
+
+### Train with multiple machines
+
+If you run MMDetection3D on a cluster managed with [slurm](https://slurm.schedmd.com/), you can use the script `slurm_train.sh`. (This script also supports single machine training.)
+
+```shell
+[GPUS=${GPUS}] ./tools/slurm_train.sh ${PARTITION} ${JOB_NAME} ${CONFIG_FILE} ${WORK_DIR}
+```
+
+Here is an example of using 16 GPUs to train Mask R-CNN on the dev partition.
+
+```shell
+GPUS=16 ./tools/slurm_train.sh dev pp_kitti_3class hv_pointpillars_secfpn_6x8_160e_kitti-3d-3class.py /nfs/xxxx/pp_kitti_3class
+```
+
+You can check [slurm_train.sh](https://github.com/open-mmlab/mmdetection/blob/master/tools/slurm_train.sh) for full arguments and environment variables.
+
+If you launch with multiple machines simply connected with ethernet, you can simply run following commands:
+
+On the first machine:
+
+```shell
+NNODES=2 NODE_RANK=0 PORT=$MASTER_PORT MASTER_ADDR=$MASTER_ADDR ./tools/dist_train.sh $CONFIG $GPUS
+```
+
+On the second machine:
+
+```shell
+NNODES=2 NODE_RANK=1 PORT=$MASTER_PORT MASTER_ADDR=$MASTER_ADDR ./tools/dist_train.sh $CONFIG $GPUS
+```
+
+Usually it is slow if you do not have high speed networking like InfiniBand.
+
+### Launch multiple jobs on a single machine
+
+If you launch multiple jobs on a single machine, e.g., 2 jobs of 4-GPU training on a machine with 8 GPUs,
+you need to specify different ports (29500 by default) for each job to avoid communication conflict.
+
+If you use `dist_train.sh` to launch training jobs, you can set the port in commands.
+
+```shell
+CUDA_VISIBLE_DEVICES=0,1,2,3 PORT=29500 ./tools/dist_train.sh ${CONFIG_FILE} 4
+CUDA_VISIBLE_DEVICES=4,5,6,7 PORT=29501 ./tools/dist_train.sh ${CONFIG_FILE} 4
+```
+
+If you use launch training jobs with Slurm, there are two ways to specify the ports.
+
+1. Set the port through `--options`. This is more recommended since it does not change the original configs.
+
+ ```shell
+ CUDA_VISIBLE_DEVICES=0,1,2,3 GPUS=4 ./tools/slurm_train.sh ${PARTITION} ${JOB_NAME} config1.py ${WORK_DIR} --options 'dist_params.port=29500'
+ CUDA_VISIBLE_DEVICES=4,5,6,7 GPUS=4 ./tools/slurm_train.sh ${PARTITION} ${JOB_NAME} config2.py ${WORK_DIR} --options 'dist_params.port=29501'
+ ```
+
+2. Modify the config files (usually the 6th line from the bottom in config files) to set different communication ports.
+
+ In `config1.py`,
+
+ ```python
+ dist_params = dict(backend='nccl', port=29500)
+ ```
+
+ In `config2.py`,
+
+ ```python
+ dist_params = dict(backend='nccl', port=29501)
+ ```
+
+ Then you can launch two jobs with `config1.py` and `config2.py`.
+
+ ```shell
+ CUDA_VISIBLE_DEVICES=0,1,2,3 GPUS=4 ./tools/slurm_train.sh ${PARTITION} ${JOB_NAME} config1.py ${WORK_DIR}
+ CUDA_VISIBLE_DEVICES=4,5,6,7 GPUS=4 ./tools/slurm_train.sh ${PARTITION} ${JOB_NAME} config2.py ${WORK_DIR}
+ ```
diff --git a/docs/en/2_new_data_model.md b/docs/en/2_new_data_model.md
new file mode 100644
index 0000000..ef4da47
--- /dev/null
+++ b/docs/en/2_new_data_model.md
@@ -0,0 +1,104 @@
+# 2: Train with customized datasets
+
+In this note, you will know how to train and test predefined models with customized datasets. We use the Waymo dataset as an example to describe the whole process.
+
+The basic steps are as below:
+
+1. Prepare the customized dataset
+2. Prepare a config
+3. Train, test, inference models on the customized dataset.
+
+## Prepare the customized dataset
+
+There are three ways to support a new dataset in MMDetection3D:
+
+1. reorganize the dataset into existing format.
+2. reorganize the dataset into a middle format.
+3. implement a new dataset.
+
+Usually we recommend to use the first two methods which are usually easier than the third.
+
+In this note, we give an example for converting the data into KITTI format.
+
+**Note**: We take Waymo as the example here considering its format is totally different from other existing formats. For other datasets using similar methods to organize data, like Lyft compared to nuScenes, it would be easier to directly implement the new data converter (for the second approach above) instead of converting it to another format (for the first approach above).
+
+### KITTI dataset format
+
+Firstly, the raw data for 3D object detection from KITTI are typically organized as follows, where `ImageSets` contains split files indicating which files belong to training/validation/testing set, `calib` contains calibration information files, `image_2` and `velodyne` include image data and point cloud data, and `label_2` includes label files for 3D detection.
+
+```
+mmdetection3d
+├── mmdet3d
+├── tools
+├── configs
+├── data
+│ ├── kitti
+│ │ ├── ImageSets
+│ │ ├── testing
+│ │ │ ├── calib
+│ │ │ ├── image_2
+│ │ │ ├── velodyne
+│ │ ├── training
+│ │ │ ├── calib
+│ │ │ ├── image_2
+│ │ │ ├── label_2
+│ │ │ ├── velodyne
+```
+
+Specific annotation format is described in the official object development [kit](https://s3.eu-central-1.amazonaws.com/avg-kitti/devkit_object.zip). For example, it consists of the following labels:
+
+```
+#Values Name Description
+----------------------------------------------------------------------------
+ 1 type Describes the type of object: 'Car', 'Van', 'Truck',
+ 'Pedestrian', 'Person_sitting', 'Cyclist', 'Tram',
+ 'Misc' or 'DontCare'
+ 1 truncated Float from 0 (non-truncated) to 1 (truncated), where
+ truncated refers to the object leaving image boundaries
+ 1 occluded Integer (0,1,2,3) indicating occlusion state:
+ 0 = fully visible, 1 = partly occluded
+ 2 = largely occluded, 3 = unknown
+ 1 alpha Observation angle of object, ranging [-pi..pi]
+ 4 bbox 2D bounding box of object in the image (0-based index):
+ contains left, top, right, bottom pixel coordinates
+ 3 dimensions 3D object dimensions: height, width, length (in meters)
+ 3 location 3D object location x,y,z in camera coordinates (in meters)
+ 1 rotation_y Rotation ry around Y-axis in camera coordinates [-pi..pi]
+ 1 score Only for results: Float, indicating confidence in
+ detection, needed for p/r curves, higher is better.
+```
+
+Assume we use the Waymo dataset.
+After downloading the data, we need to implement a function to convert both the input data and annotation format into the KITTI style. Then we can implement WaymoDataset inherited from KittiDataset to load the data and perform training and evaluation.
+
+Specifically, we implement a waymo [converter](https://github.com/open-mmlab/mmdetection3d/blob/master/tools/data_converter/waymo_converter.py) to convert Waymo data into KITTI format and a waymo dataset [class](https://github.com/open-mmlab/mmdetection3d/blob/master/mmdet3d/datasets/waymo_dataset.py) to process it. Because we preprocess the raw data and reorganize it like KITTI, the dataset class could be implemented more easily by inheriting from KittiDataset. The last thing needed to be noted is the evaluation protocol you would like to use. Because Waymo has its own evaluation approach, we further incorporate it into our dataset class. Afterwards, users can successfully convert the data format and use `WaymoDataset` to train and evaluate the model.
+
+For more details about the intermediate results of preprocessing of Waymo dataset, please refer to its [tutorial](https://mmdetection3d.readthedocs.io/en/latest/datasets/waymo_det.html).
+
+## Prepare a config
+
+The second step is to prepare configs such that the dataset could be successfully loaded. In addition, adjusting hyperparameters is usually necessary to obtain decent performance in 3D detection.
+
+Suppose we would like to train PointPillars on Waymo to achieve 3D detection for 3 classes, vehicle, cyclist and pedestrian, we need to prepare dataset config like [this](https://github.com/open-mmlab/mmdetection3d/blob/master/configs/_base_/datasets/waymoD5-3d-3class.py), model config like [this](https://github.com/open-mmlab/mmdetection3d/blob/master/configs/_base_/models/hv_pointpillars_secfpn_waymo.py) and combine them like [this](https://github.com/open-mmlab/mmdetection3d/blob/master/configs/pointpillars/hv_pointpillars_secfpn_sbn_2x16_2x_waymoD5-3d-3class.py), compared to KITTI [dataset config](https://github.com/open-mmlab/mmdetection3d/blob/master/configs/_base_/datasets/kitti-3d-3class.py), [model config](https://github.com/open-mmlab/mmdetection3d/blob/master/configs/_base_/models/hv_pointpillars_secfpn_kitti.py) and [overall](https://github.com/open-mmlab/mmdetection3d/blob/master/configs/pointpillars/hv_pointpillars_secfpn_6x8_160e_kitti-3d-3class.py).
+
+## Train a new model
+
+To train a model with the new config, you can simply run
+
+```shell
+python tools/train.py configs/pointpillars/hv_pointpillars_secfpn_sbn_2x16_2x_waymoD5-3d-3class.py
+```
+
+For more detailed usages, please refer to the [Case 1](https://mmdetection3d.readthedocs.io/en/latest/1_exist_data_model.html).
+
+## Test and inference
+
+To test the trained model, you can simply run
+
+```shell
+python tools/test.py configs/pointpillars/hv_pointpillars_secfpn_sbn_2x16_2x_waymoD5-3d-3class.py work_dirs/hv_pointpillars_secfpn_sbn_2x16_2x_waymoD5-3d-3class/latest.pth --eval waymo
+```
+
+**Note**: To use Waymo evaluation protocol, you need to follow the [tutorial](https://mmdetection3d.readthedocs.io/en/latest/datasets/waymo_det.html) and prepare files related to metrics computation as official instructions.
+
+For more detailed usages for test and inference, please refer to the [Case 1](https://mmdetection3d.readthedocs.io/en/latest/1_exist_data_model.html).
diff --git a/docs/en/Makefile b/docs/en/Makefile
new file mode 100644
index 0000000..d4bb2cb
--- /dev/null
+++ b/docs/en/Makefile
@@ -0,0 +1,20 @@
+# Minimal makefile for Sphinx documentation
+#
+
+# You can set these variables from the command line, and also
+# from the environment for the first two.
+SPHINXOPTS ?=
+SPHINXBUILD ?= sphinx-build
+SOURCEDIR = .
+BUILDDIR = _build
+
+# Put it first so that "make" without argument is like "make help".
+help:
+ @$(SPHINXBUILD) -M help "$(SOURCEDIR)" "$(BUILDDIR)" $(SPHINXOPTS) $(O)
+
+.PHONY: help Makefile
+
+# Catch-all target: route all unknown targets to Sphinx using the new
+# "make mode" option. $(O) is meant as a shortcut for $(SPHINXOPTS).
+%: Makefile
+ @$(SPHINXBUILD) -M $@ "$(SOURCEDIR)" "$(BUILDDIR)" $(SPHINXOPTS) $(O)
diff --git a/docs/en/_static/css/readthedocs.css b/docs/en/_static/css/readthedocs.css
new file mode 100644
index 0000000..cc61ab8
--- /dev/null
+++ b/docs/en/_static/css/readthedocs.css
@@ -0,0 +1,6 @@
+.header-logo {
+ background-image: url("../image/mmdet3d-logo.png");
+ background-size: 182.5px 40px;
+ height: 40px;
+ width: 182.5px;
+}
diff --git a/docs/en/api.rst b/docs/en/api.rst
new file mode 100644
index 0000000..00153b9
--- /dev/null
+++ b/docs/en/api.rst
@@ -0,0 +1,86 @@
+mmdet3d.core
+--------------
+
+anchor
+^^^^^^^^^^
+.. automodule:: mmdet3d.core.anchor
+ :members:
+
+bbox
+^^^^^^^^^^
+.. automodule:: mmdet3d.core.bbox
+ :members:
+
+evaluation
+^^^^^^^^^^
+.. automodule:: mmdet3d.core.evaluation
+ :members:
+
+visualizer
+^^^^^^^^^^^^^^^
+.. automodule:: mmdet3d.core.visualizer
+ :members:
+
+voxel
+^^^^^^^^^^^^^^^
+.. automodule:: mmdet3d.core.voxel
+ :members:
+
+post_processing
+^^^^^^^^^^^^^^^
+.. automodule:: mmdet3d.core.post_processing
+ :members:
+
+mmdet3d.datasets
+----------------
+
+.. automodule:: mmdet3d.datasets
+ :members:
+
+mmdet3d.models
+--------------
+
+detectors
+^^^^^^^^^^
+.. automodule:: mmdet3d.models.detectors
+ :members:
+
+backbones
+^^^^^^^^^^
+.. automodule:: mmdet3d.models.backbones
+ :members:
+
+necks
+^^^^^^^^^^
+.. automodule:: mmdet3d.models.necks
+ :members:
+
+dense_heads
+^^^^^^^^^^^^
+.. automodule:: mmdet3d.models.dense_heads
+ :members:
+
+roi_heads
+^^^^^^^^^^
+.. automodule:: mmdet3d.models.roi_heads
+ :members:
+
+fusion_layers
+^^^^^^^^^^^^^
+.. automodule:: mmdet3d.models.fusion_layers
+ :members:
+
+losses
+^^^^^^^^^^
+.. automodule:: mmdet3d.models.losses
+ :members:
+
+middle_encoders
+^^^^^^^^^^^^^^^
+.. automodule:: mmdet3d.models.middle_encoders
+ :members:
+
+model_utils
+^^^^^^^^^^^^^
+.. automodule:: mmdet3d.models.model_utils
+ :members:
diff --git a/docs/en/benchmarks.md b/docs/en/benchmarks.md
new file mode 100644
index 0000000..8c71b40
--- /dev/null
+++ b/docs/en/benchmarks.md
@@ -0,0 +1,286 @@
+# Benchmarks
+
+Here we benchmark the training and testing speed of models in MMDetection3D,
+with some other open source 3D detection codebases.
+
+## Settings
+
+- Hardwares: 8 NVIDIA Tesla V100 (32G) GPUs, Intel(R) Xeon(R) Gold 6148 CPU @ 2.40GHz
+- Software: Python 3.7, CUDA 10.1, cuDNN 7.6.5, PyTorch 1.3, numba 0.48.0.
+- Model: Since all the other codebases implements different models, we compare the corresponding models including SECOND, PointPillars, Part-A2, and VoteNet with them separately.
+- Metrics: We use the average throughput in iterations of the entire training run and skip the first 50 iterations of each epoch to skip GPU warmup time.
+
+## Main Results
+
+We compare the training speed (samples/s) with other codebases if they implement the similar models. The results are as below, the greater the numbers in the table, the faster of the training process. The models that are not supported by other codebases are marked by `×`.
+
+| Methods | MMDetection3D | OpenPCDet | votenet | Det3D |
+| :-----------------: | :-----------: | :-------: | :-----: | :---: |
+| VoteNet | 358 | × | 77 | × |
+| PointPillars-car | 141 | × | × | 140 |
+| PointPillars-3class | 107 | 44 | × | × |
+| SECOND | 40 | 30 | × | × |
+| Part-A2 | 17 | 14 | × | × |
+
+## Details of Comparison
+
+### Modification for Calculating Speed
+
+- __MMDetection3D__: We try to use as similar settings as those of other codebases as possible using [benchmark configs](https://github.com/open-mmlab/MMDetection3D/blob/master/configs/benchmark).
+
+- __Det3D__: For comparison with Det3D, we use the commit [519251e](https://github.com/poodarchu/Det3D/tree/519251e72a5c1fdd58972eabeac67808676b9bb7).
+
+- __OpenPCDet__: For comparison with OpenPCDet, we use the commit [b32fbddb](https://github.com/open-mmlab/OpenPCDet/tree/b32fbddbe06183507bad433ed99b407cbc2175c2).
+
+ For training speed, we add code to record the running time in the file `./tools/train_utils/train_utils.py`. We calculate the speed of each epoch, and report the average speed of all the epochs.
+
+
+
+ (diff to make it use the same method for benchmarking speed - click to expand)
+
+
+ ```diff
+ diff --git a/tools/train_utils/train_utils.py b/tools/train_utils/train_utils.py
+ index 91f21dd..021359d 100644
+ --- a/tools/train_utils/train_utils.py
+ +++ b/tools/train_utils/train_utils.py
+ @@ -2,6 +2,7 @@ import torch
+ import os
+ import glob
+ import tqdm
+ +import datetime
+ from torch.nn.utils import clip_grad_norm_
+
+
+ @@ -13,7 +14,10 @@ def train_one_epoch(model, optimizer, train_loader, model_func, lr_scheduler, ac
+ if rank == 0:
+ pbar = tqdm.tqdm(total=total_it_each_epoch, leave=leave_pbar, desc='train', dynamic_ncols=True)
+
+ + start_time = None
+ for cur_it in range(total_it_each_epoch):
+ + if cur_it > 49 and start_time is None:
+ + start_time = datetime.datetime.now()
+ try:
+ batch = next(dataloader_iter)
+ except StopIteration:
+ @@ -55,9 +59,11 @@ def train_one_epoch(model, optimizer, train_loader, model_func, lr_scheduler, ac
+ tb_log.add_scalar('learning_rate', cur_lr, accumulated_iter)
+ for key, val in tb_dict.items():
+ tb_log.add_scalar('train_' + key, val, accumulated_iter)
+ + endtime = datetime.datetime.now()
+ + speed = (endtime - start_time).seconds / (total_it_each_epoch - 50)
+ if rank == 0:
+ pbar.close()
+ - return accumulated_iter
+ + return accumulated_iter, speed
+
+
+ def train_model(model, optimizer, train_loader, model_func, lr_scheduler, optim_cfg,
+ @@ -65,6 +71,7 @@ def train_model(model, optimizer, train_loader, model_func, lr_scheduler, optim_
+ lr_warmup_scheduler=None, ckpt_save_interval=1, max_ckpt_save_num=50,
+ merge_all_iters_to_one_epoch=False):
+ accumulated_iter = start_iter
+ + speeds = []
+ with tqdm.trange(start_epoch, total_epochs, desc='epochs', dynamic_ncols=True, leave=(rank == 0)) as tbar:
+ total_it_each_epoch = len(train_loader)
+ if merge_all_iters_to_one_epoch:
+ @@ -82,7 +89,7 @@ def train_model(model, optimizer, train_loader, model_func, lr_scheduler, optim_
+ cur_scheduler = lr_warmup_scheduler
+ else:
+ cur_scheduler = lr_scheduler
+ - accumulated_iter = train_one_epoch(
+ + accumulated_iter, speed = train_one_epoch(
+ model, optimizer, train_loader, model_func,
+ lr_scheduler=cur_scheduler,
+ accumulated_iter=accumulated_iter, optim_cfg=optim_cfg,
+ @@ -91,7 +98,7 @@ def train_model(model, optimizer, train_loader, model_func, lr_scheduler, optim_
+ total_it_each_epoch=total_it_each_epoch,
+ dataloader_iter=dataloader_iter
+ )
+ -
+ + speeds.append(speed)
+ # save trained model
+ trained_epoch = cur_epoch + 1
+ if trained_epoch % ckpt_save_interval == 0 and rank == 0:
+ @@ -107,6 +114,8 @@ def train_model(model, optimizer, train_loader, model_func, lr_scheduler, optim_
+ save_checkpoint(
+ checkpoint_state(model, optimizer, trained_epoch, accumulated_iter), filename=ckpt_name,
+ )
+ + print(speed)
+ + print(f'*******{sum(speeds) / len(speeds)}******')
+
+
+ def model_state_to_cpu(model_state):
+ ```
+
+
+
+### VoteNet
+
+- __MMDetection3D__: With release v0.1.0, run
+
+ ```bash
+ ./tools/dist_train.sh configs/votenet/votenet_16x8_sunrgbd-3d-10class.py 8 --no-validate
+ ```
+
+- __votenet__: At commit [2f6d6d3](https://github.com/facebookresearch/votenet/tree/2f6d6d36ff98d96901182e935afe48ccee82d566), run
+
+ ```bash
+ python train.py --dataset sunrgbd --batch_size 16
+ ```
+
+ Then benchmark the test speed by running
+
+ ```bash
+ python eval.py --dataset sunrgbd --checkpoint_path log_sunrgbd/checkpoint.tar --batch_size 1 --dump_dir eval_sunrgbd --cluster_sampling seed_fps --use_3d_nms --use_cls_nms --per_class_proposal
+ ```
+
+ Note that eval.py is modified to compute inference time.
+
+
+
+ (diff to benchmark the similar models - click to expand)
+
+
+ ```diff
+ diff --git a/eval.py b/eval.py
+ index c0b2886..04921e9 100644
+ --- a/eval.py
+ +++ b/eval.py
+ @@ -10,6 +10,7 @@ import os
+ import sys
+ import numpy as np
+ from datetime import datetime
+ +import time
+ import argparse
+ import importlib
+ import torch
+ @@ -28,7 +29,7 @@ parser.add_argument('--checkpoint_path', default=None, help='Model checkpoint pa
+ parser.add_argument('--dump_dir', default=None, help='Dump dir to save sample outputs [default: None]')
+ parser.add_argument('--num_point', type=int, default=20000, help='Point Number [default: 20000]')
+ parser.add_argument('--num_target', type=int, default=256, help='Point Number [default: 256]')
+ -parser.add_argument('--batch_size', type=int, default=8, help='Batch Size during training [default: 8]')
+ +parser.add_argument('--batch_size', type=int, default=1, help='Batch Size during training [default: 8]')
+ parser.add_argument('--vote_factor', type=int, default=1, help='Number of votes generated from each seed [default: 1]')
+ parser.add_argument('--cluster_sampling', default='vote_fps', help='Sampling strategy for vote clusters: vote_fps, seed_fps, random [default: vote_fps]')
+ parser.add_argument('--ap_iou_thresholds', default='0.25,0.5', help='A list of AP IoU thresholds [default: 0.25,0.5]')
+ @@ -132,6 +133,7 @@ CONFIG_DICT = {'remove_empty_box': (not FLAGS.faster_eval), 'use_3d_nms': FLAGS.
+ # ------------------------------------------------------------------------- GLOBAL CONFIG END
+
+ def evaluate_one_epoch():
+ + time_list = list()
+ stat_dict = {}
+ ap_calculator_list = [APCalculator(iou_thresh, DATASET_CONFIG.class2type) \
+ for iou_thresh in AP_IOU_THRESHOLDS]
+ @@ -144,6 +146,8 @@ def evaluate_one_epoch():
+
+ # Forward pass
+ inputs = {'point_clouds': batch_data_label['point_clouds']}
+ + torch.cuda.synchronize()
+ + start_time = time.perf_counter()
+ with torch.no_grad():
+ end_points = net(inputs)
+
+ @@ -161,6 +165,12 @@ def evaluate_one_epoch():
+
+ batch_pred_map_cls = parse_predictions(end_points, CONFIG_DICT)
+ batch_gt_map_cls = parse_groundtruths(end_points, CONFIG_DICT)
+ + torch.cuda.synchronize()
+ + elapsed = time.perf_counter() - start_time
+ + time_list.append(elapsed)
+ +
+ + if len(time_list==200):
+ + print("average inference time: %4f"%(sum(time_list[5:])/len(time_list[5:])))
+ for ap_calculator in ap_calculator_list:
+ ap_calculator.step(batch_pred_map_cls, batch_gt_map_cls)
+
+ ```
+
+### PointPillars-car
+
+- __MMDetection3D__: With release v0.1.0, run
+
+ ```bash
+ ./tools/dist_train.sh configs/benchmark/hv_pointpillars_secfpn_3x8_100e_det3d_kitti-3d-car.py 8 --no-validate
+ ```
+
+- __Det3D__: At commit [519251e](https://github.com/poodarchu/Det3D/tree/519251e72a5c1fdd58972eabeac67808676b9bb7), use `kitti_point_pillars_mghead_syncbn.py` and run
+
+ ```bash
+ ./tools/scripts/train.sh --launcher=slurm --gpus=8
+ ```
+
+ Note that the config in train.sh is modified to train point pillars.
+
+
+
+ (diff to benchmark the similar models - click to expand)
+
+
+ ```diff
+ diff --git a/tools/scripts/train.sh b/tools/scripts/train.sh
+ index 3a93f95..461e0ea 100755
+ --- a/tools/scripts/train.sh
+ +++ b/tools/scripts/train.sh
+ @@ -16,9 +16,9 @@ then
+ fi
+
+ # Voxelnet
+ -python -m torch.distributed.launch --nproc_per_node=8 ./tools/train.py examples/second/configs/ kitti_car_vfev3_spmiddlefhd_rpn1_mghead_syncbn.py --work_dir=$SECOND_WORK_DIR
+ +# python -m torch.distributed.launch --nproc_per_node=8 ./tools/train.py examples/second/configs/ kitti_car_vfev3_spmiddlefhd_rpn1_mghead_syncbn.py --work_dir=$SECOND_WORK_DIR
+ # python -m torch.distributed.launch --nproc_per_node=8 ./tools/train.py examples/cbgs/configs/ nusc_all_vfev3_spmiddleresnetfhd_rpn2_mghead_syncbn.py --work_dir=$NUSC_CBGS_WORK_DIR
+ # python -m torch.distributed.launch --nproc_per_node=8 ./tools/train.py examples/second/configs/ lyft_all_vfev3_spmiddleresnetfhd_rpn2_mghead_syncbn.py --work_dir=$LYFT_CBGS_WORK_DIR
+
+ # PointPillars
+ -# python -m torch.distributed.launch --nproc_per_node=8 ./tools/train.py ./examples/point_pillars/configs/ original_pp_mghead_syncbn_kitti.py --work_dir=$PP_WORK_DIR
+ +python -m torch.distributed.launch --nproc_per_node=8 ./tools/train.py ./examples/point_pillars/configs/ kitti_point_pillars_mghead_syncbn.py
+ ```
+
+
+
+### PointPillars-3class
+
+- __MMDetection3D__: With release v0.1.0, run
+
+ ```bash
+ ./tools/dist_train.sh configs/benchmark/hv_pointpillars_secfpn_4x8_80e_pcdet_kitti-3d-3class.py 8 --no-validate
+ ```
+
+- __OpenPCDet__: At commit [b32fbddb](https://github.com/open-mmlab/OpenPCDet/tree/b32fbddbe06183507bad433ed99b407cbc2175c2), run
+
+ ```bash
+ cd tools
+ sh scripts/slurm_train.sh ${PARTITION} ${JOB_NAME} 8 --cfg_file ./cfgs/kitti_models/pointpillar.yaml --batch_size 32 --workers 32 --epochs 80
+ ```
+
+### SECOND
+
+For SECOND, we mean the [SECONDv1.5](https://github.com/traveller59/second.pytorch/blob/master/second/configs/all.fhd.config) that was first implemented in [second.Pytorch](https://github.com/traveller59/second.pytorch). Det3D's implementation of SECOND uses its self-implemented Multi-Group Head, so its speed is not compatible with other codebases.
+
+- __MMDetection3D__: With release v0.1.0, run
+
+ ```bash
+ ./tools/dist_train.sh configs/benchmark/hv_second_secfpn_4x8_80e_pcdet_kitti-3d-3class.py 8 --no-validate
+ ```
+
+- __OpenPCDet__: At commit [b32fbddb](https://github.com/open-mmlab/OpenPCDet/tree/b32fbddbe06183507bad433ed99b407cbc2175c2), run
+
+ ```bash
+ cd tools
+ sh ./scripts/slurm_train.sh ${PARTITION} ${JOB_NAME} 8 --cfg_file ./cfgs/kitti_models/second.yaml --batch_size 32 --workers 32 --epochs 80
+ ```
+
+### Part-A2
+
+- __MMDetection3D__: With release v0.1.0, run
+
+ ```bash
+ ./tools/dist_train.sh configs/benchmark/hv_PartA2_secfpn_4x8_cyclic_80e_pcdet_kitti-3d-3class.py 8 --no-validate
+ ```
+
+- __OpenPCDet__: At commit [b32fbddb](https://github.com/open-mmlab/OpenPCDet/tree/b32fbddbe06183507bad433ed99b407cbc2175c2), train the model by running
+
+ ```bash
+ cd tools
+ sh ./scripts/slurm_train.sh ${PARTITION} ${JOB_NAME} 8 --cfg_file ./cfgs/kitti_models/PartA2.yaml --batch_size 32 --workers 32 --epochs 80
+ ```
diff --git a/docs/en/changelog.md b/docs/en/changelog.md
new file mode 100644
index 0000000..748aa94
--- /dev/null
+++ b/docs/en/changelog.md
@@ -0,0 +1,822 @@
+## Changelog
+
+### v1.0.0rc3 (8/6/2022)
+
+#### Highlights
+
+- Support [SA-SSD](https://openaccess.thecvf.com/content_CVPR_2020/papers/He_Structure_Aware_Single-Stage_3D_Object_Detection_From_Point_Cloud_CVPR_2020_paper.pdf)
+
+#### New Features
+
+- Support [SA-SSD](https://openaccess.thecvf.com/content_CVPR_2020/papers/He_Structure_Aware_Single-Stage_3D_Object_Detection_From_Point_Cloud_CVPR_2020_paper.pdf) (#1337)
+
+#### Improvements
+
+- Add Chinese documentation for vision-only 3D detection (#1438)
+- Update CenterPoint pretrained models that are compatible with refactored coordinate systems (#1450)
+- Configure myst-parser to parse anchor tag in the documentation (#1488)
+- Replace markdownlint with mdformat for avoiding installing ruby (#1489)
+- Add missing `gt_names` when getting annotation info in Custom3DDataset (#1519)
+- Support S3DIS full ceph training (#1542)
+- Rewrite the installation and FAQ documentation (#1545)
+
+#### Bug Fixes
+
+- Fix the incorrect registry name when building RoI extractors (#1460)
+- Fix the potential problems caused by the registry scope update when composing pipelines (#1466) and using CocoDataset (#1536)
+- Fix the missing selection with `order` in the [box3d_nms](https://github.com/open-mmlab/mmdetection3d/blob/master/mmdet3d/core/post_processing/box3d_nms.py) introduced by [#1403](https://github.com/open-mmlab/mmdetection3d/pull/1403) (#1479)
+- Update the [PointPillars config](https://github.com/open-mmlab/mmdetection3d/blob/master/configs/pointpillars/hv_pointpillars_secfpn_6x8_160e_kitti-3d-car.py) to make it consistent with the log (#1486)
+- Fix heading anchor in documentation (#1490)
+- Fix the compatibility of mmcv in the dockerfile (#1508)
+- Make overwrite_spconv packaged when building whl (#1516)
+- Fix the requirement of mmcv and mmdet (#1537)
+- Update configs of PartA2 and support its compatibility with spconv 2.0 (#1538)
+
+#### Contributors
+
+A total of 13 developers contributed to this release.
+
+@Xiangxu-0103, @ZCMax, @jshilong, @filaPro, @atinfinity, @Tai-Wang, @wenbo-yu, @yi-chen-isuzu, @ZwwWayne, @wchen61, @VVsssssk, @AlexPasqua, @lianqing11
+
+### v1.0.0rc2 (1/5/2022)
+
+#### Highlights
+
+- Support spconv 2.0
+- Support MinkowskiEngine with MinkResNet
+- Support training models on custom datasets with only point clouds
+- Update Registry to distinguish the scope of built functions
+- Replace mmcv.iou3d with a set of bird-eye-view (BEV) operators to unify the operations of rotated boxes
+
+#### New Features
+
+- Add loader arguments in the configuration files (#1388)
+- Support [spconv 2.0](https://github.com/traveller59/spconv) when the package is installed. Users can still use spconv 1.x in MMCV with CUDA 9.0 (only cost more memory) without losing the compatibility of model weights between two versions (#1421)
+- Support MinkowskiEngine with MinkResNet (#1422)
+
+#### Improvements
+
+- Add the documentation for model deployment (#1373, #1436)
+- Add Chinese documentation of
+ - Speed benchmark (#1379)
+ - LiDAR-based 3D detection (#1368)
+ - LiDAR 3D segmentation (#1420)
+ - Coordinate system refactoring (#1384)
+- Support training models on custom datasets with only point clouds (#1393)
+- Replace mmcv.iou3d with a set of bird-eye-view (BEV) operators to unify the operations of rotated boxes (#1403, #1418)
+- Update Registry to distinguish the scope of building functions (#1412, #1443)
+- Replace recommonmark with myst_parser for documentation rendering (#1414)
+
+#### Bug Fixes
+
+- Fix the show pipeline in the [browse_dataset.py](https://github.com/open-mmlab/mmdetection3d/blob/master/tools/misc/browse_dataset.py) (#1376)
+- Fix missing __init__ files after coordinate system refactoring (#1383)
+- Fix the incorrect yaw in the visualization caused by coordinate system refactoring (#1407)
+- Fix `NaiveSyncBatchNorm1d` and `NaiveSyncBatchNorm2d` to support non-distributed cases and more general inputs (#1435)
+
+#### Contributors
+
+A total of 11 developers contributed to this release.
+
+@ZCMax, @ZwwWayne, @Tai-Wang, @VVsssssk, @HanaRo, @JoeyforJoy, @ansonlcy, @filaPro, @jshilong, @Xiangxu-0103, @deleomike
+
+### v1.0.0rc1 (1/4/2022)
+
+#### Compatibility
+
+- We migrate all the mmdet3d ops to mmcv and do not need to compile them when installing mmdet3d.
+- To fix the imprecise timestamp and optimize its saving method, we reformat the point cloud data during Waymo data conversion. The data conversion time is also optimized significantly by supporting parallel processing. Please re-generate KITTI format Waymo data if necessary. See more details in the [compatibility documentation](https://github.com/open-mmlab/mmdetection3d/blob/master/docs/en/compatibility.md).
+- We update some of the model checkpoints after the refactor of coordinate systems. Please stay tuned for the release of the remaining model checkpoints.
+
+| | Fully Updated | Partially Updated | In Progress | No Influcence |
+| ------------- | :-----------: | :---------------: | :---------: | :-----------: |
+| SECOND | | ✓ | | |
+| PointPillars | | ✓ | | |
+| FreeAnchor | ✓ | | | |
+| VoteNet | ✓ | | | |
+| H3DNet | ✓ | | | |
+| 3DSSD | | ✓ | | |
+| Part-A2 | ✓ | | | |
+| MVXNet | ✓ | | | |
+| CenterPoint | | | ✓ | |
+| SSN | ✓ | | | |
+| ImVoteNet | ✓ | | | |
+| FCOS3D | | | | ✓ |
+| PointNet++ | | | | ✓ |
+| Group-Free-3D | | | | ✓ |
+| ImVoxelNet | ✓ | | | |
+| PAConv | | | | ✓ |
+| DGCNN | | | | ✓ |
+| SMOKE | | | | ✓ |
+| PGD | | | | ✓ |
+| MonoFlex | | | | ✓ |
+
+#### Highlights
+
+- Migrate all the mmdet3d ops to mmcv
+- Support parallel waymo data converter
+- Add ScanNet instance segmentation dataset with metrics
+- Better compatibility for windows with CI support, op migration and bug fixes
+- Support loading annotations from Ceph
+
+#### New Features
+
+- Add ScanNet instance segmentation dataset with metrics (#1230)
+- Support different random seeds for different ranks (#1321)
+- Support loading annotations from Ceph (#1325)
+- Support resuming from the latest checkpoint automatically (#1329)
+- Add windows CI (#1345)
+
+#### Improvements
+
+- Update the table format and OpenMMLab project orders in [README.md](https://github.com/open-mmlab/mmdetection3d/blob/master/README.md) (#1272, #1283)
+- Migrate all the mmdet3d ops to mmcv (#1240, #1286, #1290, #1333)
+- Add `with_plane` flag in the KITTI data conversion (#1278)
+- Update instructions and links in the documentation (#1300, 1309, #1319)
+- Support parallel Waymo dataset converter and ground truth database generator (#1327)
+- Add quick installation commands to [getting_started.md](https://github.com/open-mmlab/mmdetection3d/blob/master/docs/en/getting_started.md) (#1366)
+
+#### Bug Fixes
+
+- Update nuimages configs to use new nms config style (#1258)
+- Fix the usage of np.long for windows compatibility (#1270)
+- Fix the incorrect indexing in `BasePoints` (#1274)
+- Fix the incorrect indexing in the [pillar_scatter.forward_single](https://github.com/open-mmlab/mmdetection3d/blob/dev/mmdet3d/models/middle_encoders/pillar_scatter.py#L38) (#1280)
+- Fix unit tests that use GPUs (#1301)
+- Fix incorrect feature dimensions in `DynamicPillarFeatureNet` caused by previous upgrading of `PillarFeatureNet` (#1302)
+- Remove the `CameraPoints` constraint in `PointSample` (#1314)
+- Fix imprecise timestamps saving of Waymo dataset (#1327)
+
+#### Contributors
+
+A total of 9 developers contributed to this release.
+
+@ZCMax, @ZwwWayne, @wHao-Wu, @Tai-Wang, @wangruohui, @zjwzcx, @Xiangxu-0103, @EdAyers, @hongye-dev, @zhanggefan
+
+### v1.0.0rc0 (18/2/2022)
+
+#### Compatibility
+
+- We refactor our three coordinate systems to make their rotation directions and origins more consistent, and further remove unnecessary hacks in different datasets and models. Therefore, please re-generate data infos or convert the old version to the new one with our provided scripts. We will also provide updated checkpoints in the next version. Please refer to the [compatibility documentation](https://github.com/open-mmlab/mmdetection3d/blob/v1.0.0.dev0/docs/en/compatibility.md) for more details.
+- Unify the camera keys for consistent transformation between coordinate systems on different datasets. The modification changes the key names to `lidar2img`, `depth2img`, `cam2img`, etc., for easier understanding. Customized codes using legacy keys may be influenced.
+- The next release will begin to move files of CUDA ops to [MMCV](https://github.com/open-mmlab/mmcv). It will influence the way to import related functions. We will not break the compatibility but will raise a warning first and please prepare to migrate it.
+
+#### Highlights
+
+- Support new monocular 3D detectors: [PGD](https://github.com/open-mmlab/mmdetection3d/tree/v1.0.0.dev0/configs/pgd), [SMOKE](https://github.com/open-mmlab/mmdetection3d/tree/v1.0.0.dev0/configs/smoke), [MonoFlex](https://github.com/open-mmlab/mmdetection3d/tree/v1.0.0.dev0/configs/monoflex)
+- Support a new LiDAR-based detector: [PointRCNN](https://github.com/open-mmlab/mmdetection3d/tree/v1.0.0.dev0/configs/point_rcnn)
+- Support a new backbone: [DGCNN](https://github.com/open-mmlab/mmdetection3d/tree/v1.0.0.dev0/configs/dgcnn)
+- Support 3D object detection on the S3DIS dataset
+- Support compilation on Windows
+- Full benchmark for PAConv on S3DIS
+- Further enhancement for documentation, especially on the Chinese documentation
+
+#### New Features
+
+- Support 3D object detection on the S3DIS dataset (#835)
+- Support PointRCNN (#842, #843, #856, #974, #1022, #1109, #1125)
+- Support DGCNN (#896)
+- Support PGD (#938, #940, #948, #950, #964, #1014, #1065, #1070, #1157)
+- Support SMOKE (#939, #955, #959, #975, #988, #999, #1029)
+- Support MonoFlex (#1026, #1044, #1114, #1115, #1183)
+- Support CPU Training (#1196)
+
+#### Improvements
+
+- Support point sampling based on distance metric (#667, #840)
+- Refactor coordinate systems (#677, #774, #803, #899, #906, #912, #968, #1001)
+- Unify camera keys in PointFusion and transformations between different systems (#791, #805)
+- Refine documentation (#792, #827, #829, #836, #849, #854, #859, #1111, #1113, #1116, #1121, #1132, #1135, #1185, #1193, #1226)
+- Add a script to support benchmark regression (#808)
+- Benchmark PAConvCUDA on S3DIS (#847)
+- Support to download pdf and epub documentation (#850)
+- Change the `repeat` setting in Group-Free-3D configs to reduce training epochs (#855)
+- Support KITTI AP40 evaluation metric (#927)
+- Add the mmdet3d2torchserve tool for SECOND (#977)
+- Add code-spell pre-commit hook and fix typos (#995)
+- Support the latest numba version (#1043)
+- Set a default seed to use when the random seed is not specified (#1072)
+- Distribute mix-precision models to each algorithm folder (#1074)
+- Add abstract and a representative figure for each algorithm (#1086)
+- Upgrade pre-commit hook (#1088, #1217)
+- Support augmented data and ground truth visualization (#1092)
+- Add local yaw property for `CameraInstance3DBoxes` (#1130)
+- Lock the required numba version to 0.53.0 (#1159)
+- Support the usage of plane information for KITTI dataset (#1162)
+- Deprecate the support for "python setup.py test" (#1164)
+- Reduce the number of multi-process threads to accelerate training (#1168)
+- Support 3D flip augmentation for semantic segmentation (#1181)
+- Update README format for each model (#1195)
+
+#### Bug Fixes
+
+- Fix compiling errors on Windows (#766)
+- Fix the deprecated nms setting in the ImVoteNet config (#828)
+- Use the latest `wrap_fp16_model` import from mmcv (#861)
+- Remove 2D annotations generation on Lyft (#867)
+- Update index files for the Chinese documentation to be consistent with the English version (#873)
+- Fix the nested list transpose in the CenterPoint head (#879)
+- Fix deprecated pretrained model loading for RegNet (#889)
+- Fix the incorrect dimension indices of rotations and testing config in the CenterPoint test time augmentation (#892)
+- Fix and improve visualization tools (#956, #1066, #1073)
+- Fix PointPillars FLOPs calculation error (#1075)
+- Fix missing dimension information in the SUN RGB-D data generation (#1120)
+- Fix incorrect anchor range settings in the PointPillars [config](https://github.com/open-mmlab/mmdetection3d/blob/master/configs/_base_/models/hv_pointpillars_secfpn_kitti.py) for KITTI (#1163)
+- Fix incorrect model information in the RegNet metafile (#1184)
+- Fix bugs in non-distributed multi-gpu training and testing (#1197)
+- Fix a potential assertion error when generating corners from an empty box (#1212)
+- Upgrade bazel version according to the requirement of Waymo Devkit (#1223)
+
+#### Contributors
+
+A total of 12 developers contributed to this release.
+
+@THU17cyz, @wHao-Wu, @wangruohui, @Wuziyi616, @filaPro, @ZwwWayne, @Tai-Wang, @DCNSW, @xieenze, @robin-karlsson0, @ZCMax, @Otteri
+
+### v0.18.1 (1/2/2022)
+
+#### Improvements
+
+- Support Flip3D augmentation in semantic segmentation task (#1182)
+- Update regnet metafile (#1184)
+- Add point cloud annotation tools introduction in FAQ (#1185)
+- Add missing explanations of `cam_intrinsic` in the nuScenes dataset doc (#1193)
+
+#### Bug Fixes
+
+- Deprecate the support for "python setup.py test" (#1164)
+- Fix the rotation matrix while rotation axis=0 (#1182)
+- Fix the bug in non-distributed multi-gpu training/testing (#1197)
+- Fix a potential bug when generating corners for empty bounding boxes (#1212)
+
+#### Contributors
+
+A total of 4 developers contributed to this release.
+
+@ZwwWayne, @ZCMax, @Tai-Wang, @wHao-Wu
+
+### v0.18.0 (1/1/2022)
+
+#### Highlights
+
+- Update the required minimum version of mmdet and mmseg
+
+#### Improvements
+
+- Use the official markdownlint hook and add codespell hook for pre-committing (#1088)
+- Improve CI operation (#1095, #1102, #1103)
+- Use shared menu content from OpenMMLab's theme and remove duplicated contents from config (#1111)
+- Refactor the structure of documentation (#1113, #1121)
+- Update the required minimum version of mmdet and mmseg (#1147)
+
+#### Bug Fixes
+
+- Fix symlink failure on Windows (#1096)
+- Fix the upper bound of mmcv version in the mminstall requirements (#1104)
+- Fix API documentation compilation and mmcv build errors (#1116)
+- Fix figure links and pdf documentation compilation (#1132, #1135)
+
+#### Contributors
+
+A total of 4 developers contributed to this release.
+
+@ZwwWayne, @ZCMax, @Tai-Wang, @wHao-Wu
+
+### v0.17.3 (1/12/2021)
+
+#### Improvements
+
+- Change the default show value to `False` in show_result function to avoid unnecessary errors (#1034)
+- Improve the visualization of detection results with colorized points in [single_gpu_test](https://github.com/open-mmlab/mmdetection3d/blob/master/mmdet3d/apis/test.py#L11) (#1050)
+- Clean unnecessary custom_imports in entrypoints (#1068)
+
+#### Bug Fixes
+
+- Update mmcv version in the Dockerfile (#1036)
+- Fix the memory-leak problem when loading checkpoints in [init_model](https://github.com/open-mmlab/mmdetection3d/blob/master/mmdet3d/apis/inference.py#L36) (#1045)
+- Fix incorrect velocity indexing when formatting boxes on nuScenes (#1049)
+- Explicitly set cuda device ID in [init_model](https://github.com/open-mmlab/mmdetection3d/blob/master/mmdet3d/apis/inference.py#L36) to avoid memory allocation on unexpected devices (#1056)
+- Fix PointPillars FLOPs calculation error (#1076)
+
+#### Contributors
+
+A total of 5 developers contributed to this release.
+
+@wHao-Wu, @Tai-Wang, @ZCMax, @MilkClouds, @aldakata
+
+### v0.17.2 (1/11/2021)
+
+#### Improvements
+
+- Update Group-Free-3D and FCOS3D bibtex (#985)
+- Update the solutions for incompatibility of pycocotools in the FAQ (#993)
+- Add Chinese documentation for the KITTI (#1003) and Lyft (#1010) dataset tutorial
+- Add the H3DNet checkpoint converter for incompatible keys (#1007)
+
+#### Bug Fixes
+
+- Update mmdetection and mmsegmentation version in the Dockerfile (#992)
+- Fix links in the Chinese documentation (#1015)
+
+#### Contributors
+
+A total of 4 developers contributed to this release.
+
+@Tai-Wang, @wHao-Wu, @ZwwWayne, @ZCMax
+
+### v0.17.1 (1/10/2021)
+
+#### Highlights
+
+- Support a faster but non-deterministic version of hard voxelization
+- Completion of dataset tutorials and the Chinese documentation
+- Improved the aesthetics of the documentation format
+
+#### Improvements
+
+- Add Chinese documentation for training on customized datasets and designing customized models (#729, #820)
+- Support a faster but non-deterministic version of hard voxelization (#904)
+- Update paper titles and code details for metafiles (#917)
+- Add a tutorial for KITTI dataset (#953)
+- Use Pytorch sphinx theme to improve the format of documentation (#958)
+- Use the docker to accelerate CI (#971)
+
+#### Bug Fixes
+
+- Fix the sphinx version used in the documentation (#902)
+- Fix a dynamic scatter bug that discards the first voxel by mistake when all input points are valid (#915)
+- Fix the inconsistent variable names used in the [unit test](https://github.com/open-mmlab/mmdetection3d/blob/master/tests/test_models/test_voxel_encoder/test_voxel_generator.py) for voxel generator (#919)
+- Upgrade to use `build_prior_generator` to replace the legacy `build_anchor_generator` (#941)
+- Fix a minor bug caused by a too small difference set in the FreeAnchor Head (#944)
+
+#### Contributors
+
+A total of 8 developers contributed to this release.
+
+@DCNSW, @zhanggefan, @mickeyouyou, @ZCMax, @wHao-Wu, @tojimahammatov, @xiliu8006, @Tai-Wang
+
+### v0.17.0 (1/9/2021)
+
+#### Compatibility
+
+- Unify the camera keys for consistent transformation between coordinate systems on different datasets. The modification change the key names to `lidar2img`, `depth2img`, `cam2img`, etc. for easier understanding. Customized codes using legacy keys may be influenced.
+- The next release will begin to move files of CUDA ops to [MMCV](https://github.com/open-mmlab/mmcv). It will influence the way to import related functions. We will not break the compatibility but will raise a warning first and please prepare to migrate it.
+
+#### Highlights
+
+- Support 3D object detection on the S3DIS dataset
+- Support compilation on Windows
+- Full benchmark for PAConv on S3DIS
+- Further enhancement for documentation, especially on the Chinese documentation
+
+#### New Features
+
+- Support 3D object detection on the S3DIS dataset (#835)
+
+#### Improvements
+
+- Support point sampling based on distance metric (#667, #840)
+- Update PointFusion to support unified camera keys (#791)
+- Add Chinese documentation for customized dataset (#792), data pipeline (#827), customized runtime (#829), 3D Detection on ScanNet (#836), nuScenes (#854) and Waymo (#859)
+- Unify camera keys used in transformation between different systems (#805)
+- Add a script to support benchmark regression (#808)
+- Benchmark PAConvCUDA on S3DIS (#847)
+- Add a tutorial for 3D detection on the Lyft dataset (#849)
+- Support to download pdf and epub documentation (#850)
+- Change the `repeat` setting in Group-Free-3D configs to reduce training epochs (#855)
+
+#### Bug Fixes
+
+- Fix compiling errors on Windows (#766)
+- Fix the deprecated nms setting in the ImVoteNet config (#828)
+- Use the latest `wrap_fp16_model` import from mmcv (#861)
+- Remove 2D annotations generation on Lyft (#867)
+- Update index files for the Chinese documentation to be consistent with the English version (#873)
+- Fix the nested list transpose in the CenterPoint head (#879)
+- Fix deprecated pretrained model loading for RegNet (#889)
+
+#### Contributors
+
+A total of 11 developers contributed to this release.
+
+@THU17cyz, @wHao-Wu, @wangruohui, @Wuziyi616, @filaPro, @ZwwWayne, @Tai-Wang, @DCNSW, @xieenze, @robin-karlsson0, @ZCMax
+
+### v0.16.0 (1/8/2021)
+
+#### Compatibility
+
+- Remove the rotation and dimension hack in the monocular 3D detection on nuScenes by applying corresponding transformation in the pre-processing and post-processing. The modification only influences nuScenes coco-style json files. Please re-run the data preparation scripts if necessary. See more details in the PR #744.
+- Add a new pre-processing module for the ScanNet dataset in order to support multi-view detectors. Please run the updated scripts to extract the RGB data and its annotations. See more details in the PR #696.
+
+#### Highlights
+
+- Support to use [MIM](https://github.com/open-mmlab/mim) with pip installation
+- Support PAConv [models and benchmarks](https://github.com/open-mmlab/mmdetection3d/tree/master/configs/paconv) on S3DIS
+- Enhance the documentation especially on dataset tutorials
+
+#### New Features
+
+- Support RGB images on ScanNet for multi-view detectors (#696)
+- Support FLOPs and number of parameters calculation (#736)
+- Support to use [MIM](https://github.com/open-mmlab/mim) with pip installation (#782)
+- Support PAConv models and benchmarks on the S3DIS dataset (#783, #809)
+
+#### Improvements
+
+- Refactor Group-Free-3D to make it inherit BaseModule from MMCV (#704)
+- Modify the initialization methods of FCOS3D to be consistent with the refactored approach (#705)
+- Benchmark the Group-Free-3D [models](https://github.com/open-mmlab/mmdetection3d/tree/master/configs/groupfree3d) on ScanNet (#710)
+- Add Chinese documentation for Getting Started (#725), FAQ (#730), Model Zoo (#735), Demo (#745), Quick Run (#746), Data Preparation (#787) and Configs (#788)
+- Add documentation for semantic segmentation on ScanNet and S3DIS (#743, #747, #806, #807)
+- Add a parameter `max_keep_ckpts` to limit the maximum number of saved Group-Free-3D checkpoints (#765)
+- Add documentation for 3D detection on SUN RGB-D and nuScenes (#770, #793)
+- Remove mmpycocotools in the Dockerfile (#785)
+
+#### Bug Fixes
+
+- Fix versions of OpenMMLab dependencies (#708)
+- Convert `rt_mat` to `torch.Tensor` in coordinate transformation for compatibility (#709)
+- Fix the `bev_range` initialization in `ObjectRangeFilter` according to the `gt_bboxes_3d` type (#717)
+- Fix Chinese documentation and incorrect doc format due to the incompatible Sphinx version (#718)
+- Fix a potential bug when setting `interval == 1` in [analyze_logs.py](https://github.com/open-mmlab/mmdetection3d/blob/master/tools/analysis_tools/analyze_logs.py) (#720)
+- Update the structure of Chinese documentation (#722)
+- Fix FCOS3D FPN BC-Breaking caused by the code refactoring in MMDetection (#739)
+- Fix wrong `in_channels` when `with_distance=True` in the [Dynamic VFE Layers](https://github.com/open-mmlab/mmdetection3d/blob/master/mmdet3d/models/voxel_encoders/voxel_encoder.py#L87) (#749)
+- Fix the dimension and yaw hack of FCOS3D on nuScenes (#744, #794, #795, #818)
+- Fix the missing default `bbox_mode` in the `show_multi_modality_result` (#825)
+
+#### Contributors
+
+A total of 12 developers contributed to this release.
+
+@yinchimaoliang, @gopi231091, @filaPro, @ZwwWayne, @ZCMax, @hjin2902, @wHao-Wu, @Wuziyi616, @xiliu8006, @THU17cyz, @DCNSW, @Tai-Wang
+
+### v0.15.0 (1/7/2021)
+
+#### Compatibility
+
+In order to fix the problem that the priority of EvalHook is too low, all hook priorities have been re-adjusted in 1.3.8, so MMDetection 2.14.0 needs to rely on the latest MMCV 1.3.8 version. For related information, please refer to [#1120](https://github.com/open-mmlab/mmcv/pull/1120), for related issues, please refer to [#5343](https://github.com/open-mmlab/mmdetection/issues/5343).
+
+#### Highlights
+
+- Support [PAConv](https://arxiv.org/abs/2103.14635)
+- Support monocular/multi-view 3D detector [ImVoxelNet](https://arxiv.org/abs/2106.01178) on KITTI
+- Support Transformer-based 3D detection method [Group-Free-3D](https://arxiv.org/abs/2104.00678) on ScanNet
+- Add documentation for tasks including LiDAR-based 3D detection, vision-only 3D detection and point-based 3D semantic segmentation
+- Add dataset documents like ScanNet
+
+#### New Features
+
+- Support Group-Free-3D on ScanNet (#539)
+- Support PAConv modules (#598, #599)
+- Support ImVoxelNet on KITTI (#627, #654)
+
+#### Improvements
+
+- Add unit tests for pipeline functions `LoadImageFromFileMono3D`, `ObjectNameFilter` and `ObjectRangeFilter` (#615)
+- Enhance [IndoorPatchPointSample](https://github.com/open-mmlab/mmdetection3d/blob/master/mmdet3d/datasets/pipelines/transforms_3d.py) (#617)
+- Refactor model initialization methods based MMCV (#622)
+- Add Chinese docs (#629)
+- Add documentation for LiDAR-based 3D detection (#642)
+- Unify intrinsic and extrinsic matrices for all datasets (#653)
+- Add documentation for point-based 3D semantic segmentation (#663)
+- Add documentation of ScanNet for 3D detection (#664)
+- Refine docs for tutorials (#666)
+- Add documentation for vision-only 3D detection (#669)
+- Refine docs for Quick Run and Useful Tools (#686)
+
+#### Bug Fixes
+
+- Fix the bug of [BackgroundPointsFilter](https://github.com/open-mmlab/mmdetection3d/blob/master/mmdet3d/datasets/pipelines/transforms_3d.py) using the bottom center of ground truth (#609)
+- Fix [LoadMultiViewImageFromFiles](https://github.com/open-mmlab/mmdetection3d/blob/master/mmdet3d/datasets/pipelines/loading.py) to unravel stacked multi-view images to list to be consistent with DefaultFormatBundle (#611)
+- Fix the potential bug in [analyze_logs](https://github.com/open-mmlab/mmdetection3d/blob/master/tools/analysis_tools/analyze_logs.py) when the training resumes from a checkpoint or is stopped before evaluation (#634)
+- Fix test commands in docs and make some refinements (#635)
+- Fix wrong config paths in unit tests (#641)
+
+### v0.14.0 (1/6/2021)
+
+#### Highlights
+
+- Support the point cloud segmentation method [PointNet++](https://arxiv.org/abs/1706.02413)
+
+#### New Features
+
+- Support PointNet++ (#479, #528, #532, #541)
+- Support RandomJitterPoints transform for point cloud segmentation (#584)
+- Support RandomDropPointsColor transform for point cloud segmentation (#585)
+
+#### Improvements
+
+- Move the point alignment of ScanNet from data pre-processing to pipeline (#439, #470)
+- Add compatibility document to provide detailed descriptions of BC-breaking changes (#504)
+- Add MMSegmentation installation requirement (#535)
+- Support points rotation even without bounding box in GlobalRotScaleTrans for point cloud segmentaiton (#540)
+- Support visualization of detection results and dataset browse for nuScenes Mono-3D dataset (#542, #582)
+- Support faster implementation of KNN (#586)
+- Support RegNetX models on Lyft dataset (#589)
+- Remove a useless parameter `label_weight` from segmentation datasets including `Custom3DSegDataset`, `ScanNetSegDataset` and `S3DISSegDataset` (#607)
+
+#### Bug Fixes
+
+- Fix a corrupted lidar data file in Lyft dataset in [data_preparation](https://github.com/open-mmlab/mmdetection3d/tree/master/docs/data_preparation.md) (#546)
+- Fix evaluation bugs in nuScenes and Lyft dataset (#549)
+- Fix converting points between coordinates with specific transformation matrix in the [coord_3d_mode.py](https://github.com/open-mmlab/mmdetection3d/blob/master/mmdet3d/core/bbox/structures/coord_3d_mode.py) (#556)
+- Support PointPillars models on Lyft dataset (#578)
+- Fix the bug of demo with pre-trained VoteNet model on ScanNet (#600)
+
+### v0.13.0 (1/5/2021)
+
+#### Highlights
+
+- Support a monocular 3D detection method [FCOS3D](https://arxiv.org/abs/2104.10956)
+- Support ScanNet and S3DIS semantic segmentation dataset
+- Enhancement of visualization tools for dataset browsing and demos, including support of visualization for multi-modality data and point cloud segmentation.
+
+#### New Features
+
+- Support ScanNet semantic segmentation dataset (#390)
+- Support monocular 3D detection on nuScenes (#392)
+- Support multi-modality visualization (#405)
+- Support nuimages visualization (#408)
+- Support monocular 3D detection on KITTI (#415)
+- Support online visualization of semantic segmentation results (#416)
+- Support ScanNet test results submission to online benchmark (#418)
+- Support S3DIS data pre-processing and dataset class (#433)
+- Support FCOS3D (#436, #442, #482, #484)
+- Support dataset browse for multiple types of datasets (#467)
+- Adding paper-with-code (PWC) metafile for each model in the model zoo (#485)
+
+#### Improvements
+
+- Support dataset browsing for SUNRGBD, ScanNet or KITTI points and detection results (#367)
+- Add the pipeline to load data using file client (#430)
+- Support to customize the type of runner (#437)
+- Make pipeline functions process points and masks simultaneously when sampling points (#444)
+- Add waymo unit tests (#455)
+- Split the visualization of projecting points onto image from that for only points (#480)
+- Efficient implementation of PointSegClassMapping (#489)
+- Use the new model registry from mmcv (#495)
+
+#### Bug Fixes
+
+- Fix Pytorch 1.8 Compilation issue in the [scatter_points_cuda.cu](https://github.com/open-mmlab/mmdetection3d/blob/master/mmdet3d/ops/voxel/src/scatter_points_cuda.cu) (#404)
+- Fix [dynamic_scatter](https://github.com/open-mmlab/mmdetection3d/blob/master/mmdet3d/ops/voxel/src/scatter_points_cuda.cu) errors triggered by empty point input (#417)
+- Fix the bug of missing points caused by using break incorrectly in the voxelization (#423)
+- Fix the missing `coord_type` in the waymo dataset [config](https://github.com/open-mmlab/mmdetection3d/blob/master/configs/_base_/datasets/waymoD5-3d-3class.py) (#441)
+- Fix errors in four unittest functions of [configs](https://github.com/open-mmlab/mmdetection3d/blob/master/configs/ssn/hv_ssn_secfpn_sbn-all_2x16_2x_lyft-3d.py), [test_detectors.py](https://github.com/open-mmlab/mmdetection3d/blob/master/tests/test_models/test_detectors.py), [test_heads.py](https://github.com/open-mmlab/mmdetection3d/blob/master/tests/test_models/test_heads/test_heads.py) (#453)
+- Fix 3DSSD training errors and simplify configs (#462)
+- Clamp 3D votes projections to image boundaries in ImVoteNet (#463)
+- Update out-of-date names of pipelines in the [config](https://github.com/open-mmlab/mmdetection3d/blob/master/configs/benchmark/hv_pointpillars_secfpn_3x8_100e_det3d_kitti-3d-car.py) of pointpillars benchmark (#474)
+- Fix the lack of a placeholder when unpacking RPN targets in the [h3d_bbox_head.py](https://github.com/open-mmlab/mmdetection3d/blob/master/mmdet3d/models/roi_heads/bbox_heads/h3d_bbox_head.py) (#508)
+- Fix the incorrect value of `K` when creating pickle files for SUN RGB-D (#511)
+
+### v0.12.0 (1/4/2021)
+
+#### Highlights
+
+- Support a new multi-modality method [ImVoteNet](https://arxiv.org/abs/2001.10692).
+- Support PyTorch 1.7 and 1.8
+- Refactor the structure of tools and [train.py](https://github.com/open-mmlab/mmdetection3d/blob/master/tools/train.py)/[test.py](https://github.com/open-mmlab/mmdetection3d/blob/master/tools/test.py)
+
+#### New Features
+
+- Support LiDAR-based semantic segmentation metrics (#332)
+- Support [ImVoteNet](https://arxiv.org/abs/2001.10692) (#352, #384)
+- Support the KNN GPU operation (#360, #371)
+
+#### Improvements
+
+- Add FAQ for common problems in the documentation (#333)
+- Refactor the structure of tools (#339)
+- Refactor [train.py](https://github.com/open-mmlab/mmdetection3d/blob/master/tools/train.py) and [test.py](https://github.com/open-mmlab/mmdetection3d/blob/master/tools/test.py) (#343)
+- Support demo on nuScenes (#353)
+- Add 3DSSD checkpoints (#359)
+- Update the Bibtex of CenterPoint (#368)
+- Add citation format and reference to other OpenMMLab projects in the README (#374)
+- Upgrade the mmcv version requirements (#376)
+- Add numba and numpy version requirements in FAQ (#379)
+- Avoid unnecessary for-loop execution of vfe layer creation (#389)
+- Update SUNRGBD dataset documentation to stress the requirements for training ImVoteNet (#391)
+- Modify vote head to support 3DSSD (#396)
+
+#### Bug Fixes
+
+- Fix missing keys `coord_type` in database sampler config (#345)
+- Rename H3DNet configs (#349)
+- Fix CI by using ubuntu 18.04 in github workflow (#350)
+- Add assertions to avoid 4-dim points being input to [points_in_boxes](https://github.com/open-mmlab/mmdetection3d/blob/master/mmdet3d/ops/roiaware_pool3d/points_in_boxes.py) (#357)
+- Fix the SECOND results on Waymo in the corresponding [README](https://github.com/open-mmlab/mmdetection3d/tree/master/configs/second) (#363)
+- Fix the incorrect adopted pipeline when adding val to workflow (#370)
+- Fix a potential bug when indices used in the backwarding in ThreeNN (#377)
+- Fix a compilation error triggered by [scatter_points_cuda.cu](https://github.com/open-mmlab/mmdetection3d/blob/master/mmdet3d/ops/voxel/src/scatter_points_cuda.cu) in PyTorch 1.7 (#393)
+
+### v0.11.0 (1/3/2021)
+
+#### Highlights
+
+- Support more friendly visualization interfaces based on open3d
+- Support a faster and more memory-efficient implementation of DynamicScatter
+- Refactor unit tests and details of configs
+
+#### New Features
+
+- Support new visualization methods based on open3d (#284, #323)
+
+#### Improvements
+
+- Refactor unit tests (#303)
+- Move the key `train_cfg` and `test_cfg` into the model configs (#307)
+- Update [README](https://github.com/open-mmlab/mmdetection3d/blob/master/README.md/) with [Chinese version](https://github.com/open-mmlab/mmdetection3d/blob/master/README_zh-CN.md/) and [instructions for getting started](https://github.com/open-mmlab/mmdetection3d/blob/master/docs/getting_started.md/). (#310, #316)
+- Support a faster and more memory-efficient implementation of DynamicScatter (#318, #326)
+
+#### Bug Fixes
+
+- Fix an unsupported bias setting in the unit test for centerpoint head (#304)
+- Fix errors due to typos in the centerpoint head (#308)
+- Fix a minor bug in [points_in_boxes.py](https://github.com/open-mmlab/mmdetection3d/blob/master/mmdet3d/ops/roiaware_pool3d/points_in_boxes.py) when tensors are not in the same device. (#317)
+- Fix warning of deprecated usages of nonzero during training with PyTorch 1.6 (#330)
+
+### v0.10.0 (1/2/2021)
+
+#### Highlights
+
+- Preliminary release of API for SemanticKITTI dataset.
+- Documentation and demo enhancement for better user experience.
+- Fix a number of underlying minor bugs and add some corresponding important unit tests.
+
+#### New Features
+
+- Support SemanticKITTI dataset preliminarily (#287)
+
+#### Improvements
+
+- Add tag to README in configurations for specifying different uses (#262)
+- Update instructions for evaluation metrics in the documentation (#265)
+- Add nuImages entry in [README.md](https://github.com/open-mmlab/mmdetection3d/blob/master/README.md/) and gif demo (#266, #268)
+- Add unit test for voxelization (#275)
+
+#### Bug Fixes
+
+- Fixed the issue of unpacking size in [furthest_point_sample.py](https://github.com/open-mmlab/mmdetection3d/blob/master/mmdet3d/ops/furthest_point_sample/furthest_point_sample.py) (#248)
+- Fix bugs for 3DSSD triggered by empty ground truths (#258)
+- Remove models without checkpoints in model zoo statistics of documentation (#259)
+- Fix some unclear installation instructions in [getting_started.md](https://github.com/open-mmlab/mmdetection3d/blob/master/docs/getting_started.md/) (#269)
+- Fix relative paths/links in the documentation (#271)
+- Fix a minor bug in [scatter_points_cuda.cu](https://github.com/open-mmlab/mmdetection3d/blob/master/mmdet3d/ops/voxel/src/scatter_points_cuda.cu) when num_features != 4 (#275)
+- Fix the bug about missing text files when testing on KITTI (#278)
+- Fix issues caused by inplace modification of tensors in `BaseInstance3DBoxes` (#283)
+- Fix log analysis for evaluation and adjust the documentation accordingly (#285)
+
+### v0.9.0 (31/12/2020)
+
+#### Highlights
+
+- Documentation refactoring with better structure, especially about how to implement new models and customized datasets.
+- More compatible with refactored point structure by bug fixes in ground truth sampling.
+
+#### Improvements
+
+- Documentation refactoring (#242)
+
+#### Bug Fixes
+
+- Fix point structure related bugs in ground truth sampling (#211)
+- Fix loading points in ground truth sampling augmentation on nuScenes (#221)
+- Fix channel setting in the SeparateHead of CenterPoint (#228)
+- Fix evaluation for indoors 3D detection in case of less classes in prediction (#231)
+- Remove unreachable lines in nuScenes data converter (#235)
+- Minor adjustments of numpy implementation for perspective projection and prediction filtering criterion in KITTI evaluation (#241)
+
+### v0.8.0 (30/11/2020)
+
+#### Highlights
+
+- Refactor points structure with more constructive and clearer implementation.
+- Support axis-aligned IoU loss for VoteNet with better performance.
+- Update and enhance [SECOND](https://github.com/open-mmlab/mmdetection3d/tree/master/configs/second) benchmark on Waymo.
+
+#### New Features
+
+- Support axis-aligned IoU loss for VoteNet. (#194)
+- Support points structure for consistent processing of all the point related representation. (#196, #204)
+
+#### Improvements
+
+- Enhance [SECOND](https://github.com/open-mmlab/mmdetection3d/tree/master/configs/second) benchmark on Waymo with stronger baselines. (#205)
+- Add model zoo statistics and polish the documentation. (#201)
+
+### v0.7.0 (1/11/2020)
+
+#### Highlights
+
+- Support a new method [SSN](https://www.ecva.net/papers/eccv_2020/papers_ECCV/papers/123700579.pdf) with benchmarks on nuScenes and Lyft datasets.
+- Update benchmarks for SECOND on Waymo, CenterPoint with TTA on nuScenes and models with mixed precision training on KITTI and nuScenes.
+- Support semantic segmentation on nuImages and provide [HTC](https://arxiv.org/abs/1901.07518) models with configurations and performance for reference.
+
+#### New Features
+
+- Modified primitive head which can support the setting on SUN-RGBD dataset (#136)
+- Support semantic segmentation and [HTC](https://github.com/open-mmlab/mmdetection3d/tree/master/configs/nuimages) with models for reference on nuImages dataset (#155)
+- Support [SSN](https://github.com/open-mmlab/mmdetection3d/tree/master/configs/ssn) on nuScenes and Lyft datasets (#147, #174, #166, #182)
+- Support double flip for test time augmentation of CenterPoint with updated benchmark (#143)
+
+#### Improvements
+
+- Update [SECOND](https://github.com/open-mmlab/mmdetection3d/tree/master/configs/second) benchmark with configurations for reference on Waymo (#166)
+- Delete checkpoints on Waymo to comply its specific license agreement (#180)
+- Update models and instructions with [mixed precision training](https://github.com/open-mmlab/mmdetection3d/tree/master/configs/fp16) on KITTI and nuScenes (#178)
+
+#### Bug Fixes
+
+- Fix incorrect code weights in anchor3d_head when introducing mixed precision training (#173)
+- Fix the incorrect label mapping on nuImages dataset (#155)
+
+### v0.6.1 (11/10/2020)
+
+#### Highlights
+
+- Support mixed precision training of voxel-based methods
+- Support docker with PyTorch 1.6.0
+- Update baseline configs and results ([CenterPoint](https://github.com/open-mmlab/mmdetection3d/tree/master/configs/centerpoint) on nuScenes and [PointPillars](https://github.com/open-mmlab/mmdetection3d/tree/master/configs/pointpillars) on Waymo with full dataset)
+- Switch model zoo to download.openmmlab.com
+
+#### New Features
+
+- Support dataset pipeline `VoxelBasedPointSampler` to sample multi-sweep points based on voxelization. (#125)
+- Support mixed precision training of voxel-based methods (#132)
+- Support docker with PyTorch 1.6.0 (#160)
+
+#### Improvements
+
+- Reduce requirements for the case exclusive of Waymo (#121)
+- Switch model zoo to download.openmmlab.com (#126)
+- Update docs related to Waymo (#128)
+- Add version assertion in the [init file](https://github.com/open-mmlab/mmdetection3d/blob/master/mmdet3d/__init__.py) (#129)
+- Add evaluation interval setting for CenterPoint (#131)
+- Add unit test for CenterPoint (#133)
+- Update [PointPillars](https://github.com/open-mmlab/mmdetection3d/tree/master/configs/pointpillars) baselines on Waymo with full dataset (#142)
+- Update [CenterPoint](https://github.com/open-mmlab/mmdetection3d/tree/master/configs/centerpoint) results with models and logs (#154)
+
+#### Bug Fixes
+
+- Fix a bug of visualization in multi-batch case (#120)
+- Fix bugs in dcn unit test (#130)
+- Fix dcn bias bug in centerpoint (#137)
+- Fix dataset mapping in the evaluation of nuScenes mini dataset (#140)
+- Fix origin initialization in `CameraInstance3DBoxes` (#148, #150)
+- Correct documentation link in the getting_started.md (#159)
+- Fix model save path bug in gather_models.py (#153)
+- Fix image padding shape bug in `PointFusion` (#162)
+
+### v0.6.0 (20/9/2020)
+
+#### Highlights
+
+- Support new methods [H3DNet](https://arxiv.org/abs/2006.05682), [3DSSD](https://arxiv.org/abs/2002.10187), [CenterPoint](https://arxiv.org/abs/2006.11275).
+- Support new dataset [Waymo](https://waymo.com/open/) (with PointPillars baselines) and [nuImages](https://www.nuscenes.org/nuimages) (with Mask R-CNN and Cascade Mask R-CNN baselines).
+- Support Batch Inference
+- Support Pytorch 1.6
+- Start to publish `mmdet3d` package to PyPI since v0.5.0. You can use mmdet3d through `pip install mmdet3d`.
+
+#### Backwards Incompatible Changes
+
+- Support Batch Inference (#95, #103, #116): MMDetection3D v0.6.0 migrates to support batch inference based on MMDetection >= v2.4.0. This change influences all the test APIs in MMDetection3D and downstream codebases.
+- Start to use collect environment function from MMCV (#113): MMDetection3D v0.6.0 migrates to use `collect_env` function in MMCV.
+ `get_compiler_version` and `get_compiling_cuda_version` compiled in `mmdet3d.ops.utils` are removed. Please import these two functions from `mmcv.ops`.
+
+#### New Features
+
+- Support [nuImages](https://www.nuscenes.org/nuimages) dataset by converting them into coco format and release Mask R-CNN and Cascade Mask R-CNN baseline models (#91, #94)
+- Support to publish to PyPI in github-action (#17, #19, #25, #39, #40)
+- Support CBGSDataset and make it generally applicable to all the supported datasets (#75, #94)
+- Support [H3DNet](https://arxiv.org/abs/2006.05682) and release models on ScanNet dataset (#53, #58, #105)
+- Support Fusion Point Sampling used in [3DSSD](https://arxiv.org/abs/2002.10187) (#66)
+- Add `BackgroundPointsFilter` to filter background points in data pipeline (#84)
+- Support pointnet2 with multi-scale grouping in backbone and refactor pointnets (#82)
+- Support dilated ball query used in [3DSSD](https://arxiv.org/abs/2002.10187) (#96)
+- Support [3DSSD](https://arxiv.org/abs/2002.10187) and release models on KITTI dataset (#83, #100, #104)
+- Support [CenterPoint](https://arxiv.org/abs/2006.11275) and release models on nuScenes dataset (#49, #92)
+- Support [Waymo](https://waymo.com/open/) dataset and release PointPillars baseline models (#118)
+- Allow `LoadPointsFromMultiSweeps` to pad empty sweeps and select multiple sweeps randomly (#67)
+
+#### Improvements
+
+- Fix all warnings and bugs in PyTorch 1.6.0 (#70, #72)
+- Update issue templates (#43)
+- Update unit tests (#20, #24, #30)
+- Update documentation for using `ply` format point cloud data (#41)
+- Use points loader to load point cloud data in ground truth (GT) samplers (#87)
+- Unify version file of OpenMMLab projects by using `version.py` (#112)
+- Remove unnecessary data preprocessing commands of SUN RGB-D dataset (#110)
+
+#### Bug Fixes
+
+- Rename CosineAnealing to CosineAnnealing (#57)
+- Fix device inconsistent bug in 3D IoU computation (#69)
+- Fix a minor bug in json2csv of lyft dataset (#78)
+- Add missed test data for pointnet modules (#85)
+- Fix `use_valid_flag` bug in `CustomDataset` (#106)
+
+### v0.5.0 (9/7/2020)
+
+MMDetection3D is released.
diff --git a/docs/en/compatibility.md b/docs/en/compatibility.md
new file mode 100644
index 0000000..6b11fc8
--- /dev/null
+++ b/docs/en/compatibility.md
@@ -0,0 +1,170 @@
+## v1.0.0rc1
+
+### Operators Migration
+
+We have adopted CUDA operators compiled from [mmcv](https://github.com/open-mmlab/mmcv/blob/master/mmcv/ops/__init__.py) and removed all the CUDA operators in mmdet3d. We now do not need to compile the CUDA operators in mmdet3d anymore.
+
+### Waymo dataset converter refactoring
+
+In this version we did a major code refactoring that boosted the performance of waymo dataset conversion by multiprocessing.
+Meanwhile, we also fixed the imprecise timestamps saving issue in waymo dataset conversion. This change introduces following backward compatibility breaks:
+
+- The point cloud .bin files of waymo dataset need to be regenerated.
+ In the .bin files each point occupies 6 `float32` and the meaning of the last `float32` now changed from **imprecise timestamps** to **range frame offset**.
+ The **range frame offset** for each point is calculated as`ri * h * w + row * w + col` if the point is from the **TOP** lidar or `-1` otherwise.
+ The `h`, `w` denote the height and width of the TOP lidar's range frame.
+ The `ri`, `row`, `col` denote the return index, the row and the column of the range frame where each point locates.
+ Following tables show the difference across the change:
+
+Before
+
+| Element offset (float32) | 0 | 1 | 2 | 3 | 4 | 5 |
+| ------------------------ | :-: | :-: | :-: | :-------: | :--------: | :---------------------: |
+| Bytes offset | 0 | 4 | 8 | 12 | 16 | 20 |
+| Meaning | x | y | z | intensity | elongation | **imprecise timestamp** |
+
+After
+
+| Element offset (float32) | 0 | 1 | 2 | 3 | 4 | 5 |
+| ------------------------ | :-: | :-: | :-: | :-------: | :--------: | :--------------------: |
+| Bytes offset | 0 | 4 | 8 | 12 | 16 | 20 |
+| Meaning | x | y | z | intensity | elongation | **range frame offset** |
+
+- The objects' point cloud .bin files in the GT-database of waymo dataset need to be regenerated because we also dumped the range frame offset for each point into it.
+ Following tables show the difference across the change:
+
+Before
+
+| Element offset (float32) | 0 | 1 | 2 | 3 | 4 |
+| ------------------------ | :-: | :-: | :-: | :-------: | :--------: |
+| Bytes offset | 0 | 4 | 8 | 12 | 16 |
+| Meaning | x | y | z | intensity | elongation |
+
+After
+
+| Element offset (float32) | 0 | 1 | 2 | 3 | 4 | 5 |
+| ------------------------ | :-: | :-: | :-: | :-------: | :--------: | :--------------------: |
+| Bytes offset | 0 | 4 | 8 | 12 | 16 | 20 |
+| Meaning | x | y | z | intensity | elongation | **range frame offset** |
+
+- Any configuration that uses waymo dataset with GT Augmentation should change the `db_sampler.points_loader.load_dim` from `5` to `6`.
+
+## v1.0.0rc0
+
+### Coordinate system refactoring
+
+In this version, we did a major code refactoring which improved the consistency among the three coordinate systems (and corresponding box representation), LiDAR, Camera, and Depth. A brief summary for this refactoring is as follows:
+
+- The three coordinate systems are all right-handed now (which means the yaw angle increases in the counterclockwise direction).
+- The LiDAR system `(x_size, y_size, z_size)` corresponds to `(l, w, h)` instead of `(w, l, h)`. This is more natural since `l` is parallel with the direction where the yaw angle is zero, and we prefer using the positive direction of the `x` axis as that direction, which is exactly how we define yaw angle in Depth and Camera coordinate systems.
+- The APIs for box-related operations are improved and now are more user-friendly.
+
+#### ***NOTICE!!***
+
+Since definitions of box representation have changed, the annotation data of most datasets require updating:
+
+- SUN RGB-D: Yaw angles in the annotation should be reversed.
+- KITTI: For LiDAR boxes in GT databases, (x_size, y_size, z_size, yaw) out of (x, y, z, x_size, y_size, z_size) should be converted from the old LiDAR coordinate system to the new one. The training/validation data annotations should be left unchanged since they are under the Camera coordinate system, which is unmodified after the refactoring.
+- Waymo: Same as KITTI.
+- nuScenes: For LiDAR boxes in training/validation data and GT databases, (x_size, y_size, z_size, yaw) out of (x, y, z, x_size, y_size, z_size) should be converted.
+- Lyft: Same as nuScenes.
+
+Please regenerate the data annotation/GT database files or use [`update_data_coords.py`](https://github.com/open-mmlab/mmdetection3d/blob/v1.0.0rc0/tools/update_data_coords.py) to update the data.
+
+To use boxes under Depth and LiDAR coordinate systems, or to convert boxes between different coordinate systems, users should be aware of the difference between the old and new definitions. For example, the rotation, flipping, and bev functions of [`DepthInstance3DBoxes`](https://github.com/open-mmlab/mmdetection3d/blob/v1.0.0rc0/mmdet3d/core/bbox/structures/depth_box3d.py) and [`LiDARInstance3DBoxes`](https://github.com/open-mmlab/mmdetection3d/blob/v1.0.0rc0/mdet3d/core/bbox/structures/lidar_box3d.py) and box conversion [functions](https://github.com/open-mmlab/mmdetection3d/blob/v1.0.0rc0/mmdet3d/core/bbox/structures/box_3d_mode.py) have all been reimplemented in the refactoring.
+
+Consequently, functions like [`output_to_lyft_box`](https://github.com/open-mmlab/mmdetection3d/blob/v1.0.0rc0/mmdet3d/datasets/lyft_dataset.py) undergo small modification to adapt to the new LiDAR/Depth box.
+
+Since the LiDAR system `(x_size, y_size, z_size)` now corresponds to `(l, w, h)` instead of `(w, l, h)`, the anchor sizes for LiDAR boxes are also changed, e.g., from `[1.6, 3.9, 1.56]` to `[3.9, 1.6, 1.56]`.
+
+Functions only involving points are generally unaffected except if they rely on some refactored utility functions such as `rotation_3d_in_axis`.
+
+#### Other BC-breaking or new features:
+
+- `array_converter`: Please refer to [array_converter.py](https://github.com/open-mmlab/mmdetection3d/blob/v1.0.0rc0/mmdet3d/core/utils/array_converter.py). Functions wrapped with `array_converter` can convert array-like input types of `torch.Tensor`, `np.ndarray`, and `list/tuple/float` to `torch.Tensor` to process in an unified PyTorch pipeline. The result may finally be converted back to the input type. Most functions in [utils.py](https://github.com/open-mmlab/mmdetection3d/blob/v1.0.0rc0/mmdet3d/core/bbox/structures/utils.py) are wrapped with `array_converter`.
+- [`points_in_boxes`](https://github.com/open-mmlab/mmdetection3d/blob/v1.0.0rc0/mmdet3d/core/bbox/structures/base_box3d.py) and [`points_in_boxes_batch`](https://github.com/open-mmlab/mmdetection3d/blob/v1.0.0rc0/mmdet3d/core/bbox/structures/base_box3d.py) will be deprecated soon. They are renamed to `points_in_boxes_part` and `points_in_boxes_all` respectively, with more detailed docstrings. The major difference of the two functions is that if a point is enclosed by multiple boxes, `points_in_boxes_part` will only return the index of the first enclosing box while `points_in_boxes_all` will return all the indices of enclosing boxes.
+- `rotation_3d_in_axis`: Please refer to [utils.py](https://github.com/open-mmlab/mmdetection3d/blob/v1.0.0rc0/mmdet3d/core/bbox/structures/utils.py). Now this function supports multiple input types and more options. The function with the same name in [box_np_ops.py](https://github.com/open-mmlab/mmdetection3d/blob/v1.0.0rc0/mmdet3d/core/bbox/box_np_ops.py) is deleted since we do not need another function to tackle with NumPy data. `rotation_2d`, `points_cam2img`, and `limit_period` in box_np_ops.py are also deleted for the same reason.
+- `bev` method of [`CameraInstance3DBoxes`](https://github.com/open-mmlab/mmdetection3d/blob/v1.0.0rc0/mmdet3d/core/bbox/structures/cam_box3d.py): Changed it to be consistent with the definition of bev in Depth and LiDAR coordinate systems.
+- Data augmentation utils in [data_augment_utils.py](https://github.com/open-mmlab/mmdetection3d/blob/v1.0.0rc0/mmdet3d/datasets/pipelines/data_augment_utils.py) now follow the rules of a right-handed system.
+- We do not need the yaw hacking in KITTI anymore after refining [`get_direction_target`](https://github.com/open-mmlab/mmdetection3d/blob/v1.0.0rc0/mmdet3d/models/dense_heads/train_mixins.py). Interested users may refer to PR [#677](https://github.com/open-mmlab/mmdetection3d/pull/677) .
+
+## 0.16.0
+
+### Returned values of `QueryAndGroup` operation
+
+We modified the returned `grouped_xyz` value of operation `QueryAndGroup` to support PAConv segmentor. Originally, the `grouped_xyz` is centered by subtracting the grouping centers, which represents the relative positions of grouped points. Now, we didn't perform such subtraction and the returned `grouped_xyz` stands for the absolute coordinates of these points.
+
+Note that, the other returned variables of `QueryAndGroup` such as `new_features`, `unique_cnt` and `grouped_idx` are not affected.
+
+### NuScenes coco-style data pre-processing
+
+We remove the rotation and dimension hack in the monocular 3D detection on nuScenes. Specifically, we transform the rotation and dimension of boxes defined by nuScenes devkit to the coordinate system of our `CameraInstance3DBoxes` in the pre-processing and transform them back in the post-processing. In this way, we can remove the corresponding [hack](https://github.com/open-mmlab/mmdetection3d/pull/744/files#diff-5bee5062bd84e6fa25a2fdd71353f6f283dfdc4a66a0316c3b1ca26078c978b6L165) used in the visualization tools. The modification also guarantees the correctness of all the operations based on our `CameraInstance3DBoxes` (such as NMS and flip augmentation) when training monocular 3D detectors.
+
+The modification only influences nuScenes coco-style json files. Please re-run the nuScenes data preparation script if necessary. See more details in the PR [#744](https://github.com/open-mmlab/mmdetection3d/pull/744).
+
+### ScanNet dataset for ImVoxelNet
+
+We adopt a new pre-processing procedure for the ScanNet dataset in order to support ImVoxelNet, which is a multi-view method requiring image data. In previous versions of MMDetection3D, ScanNet dataset was only used for point cloud based 3D detection and segmentation methods. We plan adding ImVoxelNet to our model zoo, thus updating ScanNet correspondingly by adding image-related pre-processing steps. Specifically, we made these changes:
+
+- Add [script](https://github.com/open-mmlab/mmdetection3d/blob/master/data/scannet/extract_posed_images.py) for extracting RGB data.
+- Update [script](https://github.com/open-mmlab/mmdetection3d/blob/master/tools/data_converter/scannet_data_utils.py) for annotation creating.
+- Add instructions in the documents on preparing image data.
+
+Please refer to the ScanNet [README.md](https://github.com/open-mmlab/mmdetection3d/blob/master/data/scannet/README.md/) for more details.
+
+## 0.15.0
+
+### MMCV Version
+
+In order to fix the problem that the priority of EvalHook is too low, all hook priorities have been re-adjusted in 1.3.8, so MMDetection 2.14.0 needs to rely on the latest MMCV 1.3.8 version. For related information, please refer to [#1120](https://github.com/open-mmlab/mmcv/pull/1120), for related issues, please refer to [#5343](https://github.com/open-mmlab/mmdetection/issues/5343).
+
+### Unified parameter initialization
+
+To unify the parameter initialization in OpenMMLab projects, MMCV supports `BaseModule` that accepts `init_cfg` to allow the modules' parameters initialized in a flexible and unified manner. Now the users need to explicitly call `model.init_weights()` in the training script to initialize the model (as in [here](https://github.com/open-mmlab/mmdetection3d/blob/master/tools/train.py#L183), previously this was handled by the detector. Please refer to PR [#622](https://github.com/open-mmlab/mmdetection3d/pull/622) for details.
+
+### BackgroundPointsFilter
+
+We modified the dataset augmentation function `BackgroundPointsFilter`([here](https://github.com/open-mmlab/mmdetection3d/blob/v0.15.0/mmdet3d/datasets/pipelines/transforms_3d.py#L1132)). In previous version of MMdetection3D, `BackgroundPointsFilter` changes the gt_bboxes_3d's bottom center to the gravity center. In MMDetection3D 0.15.0,
+`BackgroundPointsFilter` will not change it. Please refer to PR [#609](https://github.com/open-mmlab/mmdetection3d/pull/609) for details.
+
+### Enhance `IndoorPatchPointSample` transform
+
+We enhance the pipeline function `IndoorPatchPointSample` used in point cloud segmentation task by adding more choices for patch selection. Also, we plan to remove the unused parameter `sample_rate` in the future. Please modify the code as well as the config files accordingly if you use this transform.
+
+## 0.14.0
+
+### Dataset class for 3D segmentation task
+
+We remove a useless parameter `label_weight` from segmentation datasets including `Custom3DSegDataset`, `ScanNetSegDataset` and `S3DISSegDataset` since this weight is utilized in the loss function of model class. Please modify the code as well as the config files accordingly if you use or inherit from these codes.
+
+### ScanNet data pre-processing
+
+We adopt new pre-processing and conversion steps of ScanNet dataset. In previous versions of MMDetection3D, ScanNet dataset was only used for 3D detection task, where we trained on the training set and tested on the validation set. In MMDetection3D 0.14.0, we further support 3D segmentation task on ScanNet, which includes online benchmarking on test set. Since the alignment matrix is not provided for test set data, we abandon the alignment of points in data generation steps to support both tasks. Besides, as 3D segmentation requires per-point prediction, we also remove the down-sampling step in data generation.
+
+- In the new ScanNet processing scripts, we save the unaligned points for all the training, validation and test set. For train and val set with annotations, we also store the `axis_align_matrix` in data infos. For ground-truth bounding boxes, we store boxes in both aligned and unaligned coordinates with key `gt_boxes_upright_depth` and key `unaligned_gt_boxes_upright_depth` respectively in data infos.
+
+- In `ScanNetDataset`, we now load the `axis_align_matrix` as a part of data annotations. If it is not contained in old data infos, we will use identity matrix for compatibility. We also add a transform function `GlobalAlignment` in ScanNet detection data pipeline to align the points.
+
+- Since the aligned boxes share the same key as in old data infos, we do not need to modify the code related to it. But do remember that they are not in the same coordinate system as the saved points.
+
+- There is an `PointSample` pipeline in the data pipelines for ScanNet detection task which down-samples points. So removing down-sampling in data generation will not affect the code.
+
+We have trained a [VoteNet](https://github.com/open-mmlab/mmdetection3d/blob/master/configs/votenet/votenet_8x8_scannet-3d-18class.py) model on the newly processed ScanNet dataset and get similar benchmark results. In order to prepare ScanNet data for both detection and segmentation tasks, please re-run the new pre-processing scripts following the ScanNet [README.md](https://github.com/open-mmlab/mmdetection3d/blob/master/data/scannet/README.md/).
+
+## 0.12.0
+
+### SUNRGBD dataset for ImVoteNet
+
+We adopt a new pre-processing procedure for the SUNRGBD dataset in order to support ImVoteNet, which is a multi-modality method requiring both image and point cloud data. In previous versions of MMDetection3D, SUNRGBD dataset was only used for point cloud based 3D detection methods. In MMDetection3D 0.12.0, we add ImVoteNet to our model zoo, thus updating SUNRGBD correspondingly by adding image-related pre-processing steps. Specifically, we made these changes:
+
+- Fix a bug in the image file path in meta data.
+- Convert calibration matrices from double to float to avoid type mismatch in further operations.
+- Add instructions in the documents on preparing image data.
+
+Please refer to the SUNRGBD [README.md](https://github.com/open-mmlab/mmdetection3d/blob/master/data/sunrgbd/README.md/) for more details.
+
+## 0.6.0
+
+### VoteNet and H3DNet model structure update
+
+In MMDetection 0.6.0, we updated the model structures of VoteNet and H3DNet, therefore model checkpoints generated by MMDetection \< 0.6.0 should be first converted to a format compatible with the latest structures via [convert_votenet_checkpoints.py](https://github.com/open-mmlab/mmdetection3d/blob/master/tools/model_converters/convert_votenet_checkpoints.py) and [convert_h3dnet_checkpoints.py](https://github.com/open-mmlab/mmdetection3d/blob/master/tools/model_converters/convert_h3dnet_checkpoints.py) . For more details, please refer to the VoteNet [README.md](https://github.com/open-mmlab/mmdetection3d/tree/master/configs/votenet/README.md/) and H3DNet [README.md](https://github.com/open-mmlab/mmdetection3d/tree/master/configs/h3dnet/README.md/).
diff --git a/docs/en/conf.py b/docs/en/conf.py
new file mode 100644
index 0000000..f13c638
--- /dev/null
+++ b/docs/en/conf.py
@@ -0,0 +1,161 @@
+# Configuration file for the Sphinx documentation builder.
+#
+# This file only contains a selection of the most common options. For a full
+# list see the documentation:
+# https://www.sphinx-doc.org/en/master/usage/configuration.html
+
+# -- Path setup --------------------------------------------------------------
+
+# If extensions (or modules to document with autodoc) are in another directory,
+# add these directories to sys.path here. If the directory is relative to the
+# documentation root, use os.path.abspath to make it absolute, like shown here.
+#
+import os
+import subprocess
+import sys
+
+import pytorch_sphinx_theme
+from m2r import MdInclude
+from recommonmark.transform import AutoStructify
+from sphinx.builders.html import StandaloneHTMLBuilder
+
+sys.path.insert(0, os.path.abspath('../../'))
+
+# -- Project information -----------------------------------------------------
+
+project = 'MMDetection3D'
+copyright = '2020-2023, OpenMMLab'
+author = 'MMDetection3D Authors'
+
+version_file = '../../mmdet3d/version.py'
+
+
+def get_version():
+ with open(version_file, 'r') as f:
+ exec(compile(f.read(), version_file, 'exec'))
+ return locals()['__version__']
+
+
+# The full version, including alpha/beta/rc tags
+release = get_version()
+
+# -- General configuration ---------------------------------------------------
+
+# Add any Sphinx extension module names here, as strings. They can be
+# extensions coming with Sphinx (named 'sphinx.ext.*') or your custom
+# ones.
+extensions = [
+ 'sphinx.ext.autodoc',
+ 'sphinx.ext.napoleon',
+ 'sphinx.ext.viewcode',
+ 'myst_parser',
+ 'sphinx_markdown_tables',
+ 'sphinx.ext.autosectionlabel',
+ 'sphinx_copybutton',
+]
+
+autodoc_mock_imports = [
+ 'matplotlib', 'nuscenes', 'PIL', 'pycocotools', 'pyquaternion',
+ 'terminaltables', 'mmdet3d.version', 'mmdet3d.ops', 'mmcv.ops'
+]
+autosectionlabel_prefix_document = True
+
+# Add any paths that contain templates here, relative to this directory.
+templates_path = ['_templates']
+
+# The suffix(es) of source filenames.
+# You can specify multiple suffix as a list of string:
+#
+source_suffix = {
+ '.rst': 'restructuredtext',
+ '.md': 'markdown',
+}
+
+# The master toctree document.
+master_doc = 'index'
+
+# List of patterns, relative to source directory, that match files and
+# directories to ignore when looking for source files.
+# This pattern also affects html_static_path and html_extra_path.
+exclude_patterns = ['_build', 'Thumbs.db', '.DS_Store']
+
+# -- Options for HTML output -------------------------------------------------
+
+# The theme to use for HTML and HTML Help pages. See the documentation for
+# a list of builtin themes.
+#
+# html_theme = 'sphinx_rtd_theme'
+html_theme = 'pytorch_sphinx_theme'
+html_theme_path = [pytorch_sphinx_theme.get_html_theme_path()]
+
+html_theme_options = {
+ # 'logo_url': 'https://mmocr.readthedocs.io/en/latest/',
+ 'menu': [
+ {
+ 'name': 'GitHub',
+ 'url': 'https://github.com/open-mmlab/mmdetection3d'
+ },
+ {
+ 'name':
+ 'Upstream',
+ 'children': [
+ {
+ 'name': 'MMCV',
+ 'url': 'https://github.com/open-mmlab/mmcv',
+ 'description': 'Foundational library for computer vision'
+ },
+ {
+ 'name': 'MMDetection',
+ 'url': 'https://github.com/open-mmlab/mmdetection',
+ 'description': 'Object detection toolbox and benchmark'
+ },
+ ]
+ },
+ ],
+ # Specify the language of shared menu
+ 'menu_lang':
+ 'en'
+}
+
+language = 'en'
+
+master_doc = 'index'
+
+# Add any paths that contain custom static files (such as style sheets) here,
+# relative to this directory. They are copied after the builtin static files,
+# so a file named "default.css" will overwrite the builtin "default.css".
+html_static_path = ['_static']
+html_css_files = ['css/readthedocs.css']
+
+latex_documents = [
+ (master_doc, 'mmcv.tex', 'mmcv Documentation', 'MMCV Contributors',
+ 'manual'),
+]
+
+# set priority when building html
+StandaloneHTMLBuilder.supported_image_types = [
+ 'image/svg+xml', 'image/gif', 'image/png', 'image/jpeg'
+]
+# Enable ::: for my_st
+myst_enable_extensions = ['colon_fence']
+myst_heading_anchors = 3
+
+language = 'en'
+
+
+def builder_inited_handler(app):
+ subprocess.run(['./stat.py'])
+
+
+def setup(app):
+ app.connect('builder-inited', builder_inited_handler)
+ app.add_config_value('no_underscore_emphasis', False, 'env')
+ app.add_config_value('m2r_parse_relative_links', False, 'env')
+ app.add_config_value('m2r_anonymous_references', False, 'env')
+ app.add_config_value('m2r_disable_inline_math', False, 'env')
+ app.add_directive('mdinclude', MdInclude)
+ app.add_config_value('recommonmark_config', {
+ 'auto_toc_tree_section': 'Contents',
+ 'enable_eval_rst': True,
+ }, True)
+ app.add_transform(AutoStructify)
diff --git a/docs/en/data_preparation.md b/docs/en/data_preparation.md
new file mode 100644
index 0000000..159f248
--- /dev/null
+++ b/docs/en/data_preparation.md
@@ -0,0 +1,144 @@
+# Dataset Preparation
+
+## Before Preparation
+
+It is recommended to symlink the dataset root to `$MMDETECTION3D/data`.
+If your folder structure is different from the following, you may need to change the corresponding paths in config files.
+
+```
+mmdetection3d
+├── mmdet3d
+├── tools
+├── configs
+├── data
+│ ├── nuscenes
+│ │ ├── maps
+│ │ ├── samples
+│ │ ├── sweeps
+│ │ ├── v1.0-test
+| | ├── v1.0-trainval
+│ ├── kitti
+│ │ ├── ImageSets
+│ │ ├── testing
+│ │ │ ├── calib
+│ │ │ ├── image_2
+│ │ │ ├── velodyne
+│ │ ├── training
+│ │ │ ├── calib
+│ │ │ ├── image_2
+│ │ │ ├── label_2
+│ │ │ ├── velodyne
+│ ├── waymo
+│ │ ├── waymo_format
+│ │ │ ├── training
+│ │ │ ├── validation
+│ │ │ ├── testing
+│ │ │ ├── gt.bin
+│ │ ├── kitti_format
+│ │ │ ├── ImageSets
+│ ├── lyft
+│ │ ├── v1.01-train
+│ │ │ ├── v1.01-train (train_data)
+│ │ │ ├── lidar (train_lidar)
+│ │ │ ├── images (train_images)
+│ │ │ ├── maps (train_maps)
+│ │ ├── v1.01-test
+│ │ │ ├── v1.01-test (test_data)
+│ │ │ ├── lidar (test_lidar)
+│ │ │ ├── images (test_images)
+│ │ │ ├── maps (test_maps)
+│ │ ├── train.txt
+│ │ ├── val.txt
+│ │ ├── test.txt
+│ │ ├── sample_submission.csv
+│ ├── s3dis
+│ │ ├── meta_data
+│ │ ├── Stanford3dDataset_v1.2_Aligned_Version
+│ │ ├── collect_indoor3d_data.py
+│ │ ├── indoor3d_util.py
+│ │ ├── README.md
+│ ├── scannet
+│ │ ├── meta_data
+│ │ ├── scans
+│ │ ├── scans_test
+│ │ ├── batch_load_scannet_data.py
+│ │ ├── load_scannet_data.py
+│ │ ├── scannet_utils.py
+│ │ ├── README.md
+│ ├── sunrgbd
+│ │ ├── OFFICIAL_SUNRGBD
+│ │ ├── matlab
+│ │ ├── sunrgbd_data.py
+│ │ ├── sunrgbd_utils.py
+│ │ ├── README.md
+
+```
+
+## Download and Data Preparation
+
+### KITTI
+
+Download KITTI 3D detection data [HERE](http://www.cvlibs.net/datasets/kitti/eval_object.php?obj_benchmark=3d). Prepare KITTI data splits by running
+
+```bash
+mkdir ./data/kitti/ && mkdir ./data/kitti/ImageSets
+
+# Download data split
+wget -c https://raw.githubusercontent.com/traveller59/second.pytorch/master/second/data/ImageSets/test.txt --no-check-certificate --content-disposition -O ./data/kitti/ImageSets/test.txt
+wget -c https://raw.githubusercontent.com/traveller59/second.pytorch/master/second/data/ImageSets/train.txt --no-check-certificate --content-disposition -O ./data/kitti/ImageSets/train.txt
+wget -c https://raw.githubusercontent.com/traveller59/second.pytorch/master/second/data/ImageSets/val.txt --no-check-certificate --content-disposition -O ./data/kitti/ImageSets/val.txt
+wget -c https://raw.githubusercontent.com/traveller59/second.pytorch/master/second/data/ImageSets/trainval.txt --no-check-certificate --content-disposition -O ./data/kitti/ImageSets/trainval.txt
+```
+
+Then generate info files by running
+
+```
+python tools/create_data.py kitti --root-path ./data/kitti --out-dir ./data/kitti --extra-tag kitti
+```
+
+In an environment using slurm, users may run the following command instead
+
+```
+sh tools/create_data.sh kitti
+```
+
+### Waymo
+
+Download Waymo open dataset V1.2 [HERE](https://waymo.com/open/download/) and its data split [HERE](https://drive.google.com/drive/folders/18BVuF_RYJF0NjZpt8SnfzANiakoRMf0o?usp=sharing). Then put tfrecord files into corresponding folders in `data/waymo/waymo_format/` and put the data split txt files into `data/waymo/kitti_format/ImageSets`. Download ground truth bin file for validation set [HERE](https://console.cloud.google.com/storage/browser/waymo_open_dataset_v_1_2_0/validation/ground_truth_objects) and put it into `data/waymo/waymo_format/`. A tip is that you can use `gsutil` to download the large-scale dataset with commands. You can take this [tool](https://github.com/RalphMao/Waymo-Dataset-Tool) as an example for more details. Subsequently, prepare waymo data by running
+
+```bash
+python tools/create_data.py waymo --root-path ./data/waymo/ --out-dir ./data/waymo/ --workers 128 --extra-tag waymo
+```
+
+Note that if your local disk does not have enough space for saving converted data, you can change the `out-dir` to anywhere else. Just remember to create folders and prepare data there in advance and link them back to `data/waymo/kitti_format` after the data conversion.
+
+### NuScenes
+
+Download nuScenes V1.0 full dataset data [HERE](https://www.nuscenes.org/download). Prepare nuscenes data by running
+
+```bash
+python tools/create_data.py nuscenes --root-path ./data/nuscenes --out-dir ./data/nuscenes --extra-tag nuscenes
+```
+
+### Lyft
+
+Download Lyft 3D detection data [HERE](https://www.kaggle.com/c/3d-object-detection-for-autonomous-vehicles/data). Prepare Lyft data by running
+
+```bash
+python tools/create_data.py lyft --root-path ./data/lyft --out-dir ./data/lyft --extra-tag lyft --version v1.01
+python tools/data_converter/lyft_data_fixer.py --version v1.01 --root-folder ./data/lyft
+```
+
+Note that we follow the original folder names for clear organization. Please rename the raw folders as shown above. Also note that the second command serves the purpose of fixing a corrupted lidar data file. Please refer to the discussion [here](https://www.kaggle.com/c/3d-object-detection-for-autonomous-vehicles/discussion/110000) for more details.
+
+### S3DIS, ScanNet and SUN RGB-D
+
+To prepare S3DIS data, please see its [README](https://github.com/open-mmlab/mmdetection3d/blob/master/data/s3dis/README.md/).
+
+To prepare ScanNet data, please see its [README](https://github.com/open-mmlab/mmdetection3d/blob/master/data/scannet/README.md/).
+
+To prepare SUN RGB-D data, please see its [README](https://github.com/open-mmlab/mmdetection3d/blob/master/data/sunrgbd/README.md/).
+
+### Customized Datasets
+
+For using custom datasets, please refer to [Tutorials 2: Customize Datasets](https://mmdetection3d.readthedocs.io/en/latest/tutorials/customize_dataset.html).
diff --git a/docs/en/datasets/index.rst b/docs/en/datasets/index.rst
new file mode 100644
index 0000000..c25295d
--- /dev/null
+++ b/docs/en/datasets/index.rst
@@ -0,0 +1,11 @@
+.. toctree::
+ :maxdepth: 2
+
+ kitti_det.md
+ nuscenes_det.md
+ lyft_det.md
+ waymo_det.md
+ sunrgbd_det.md
+ scannet_det.md
+ scannet_sem_seg.md
+ s3dis_sem_seg.md
diff --git a/docs/en/datasets/kitti_det.md b/docs/en/datasets/kitti_det.md
new file mode 100644
index 0000000..c0eaac9
--- /dev/null
+++ b/docs/en/datasets/kitti_det.md
@@ -0,0 +1,194 @@
+# KITTI Dataset for 3D Object Detection
+
+This page provides specific tutorials about the usage of MMDetection3D for KITTI dataset.
+
+**Note**: Current tutorial is only for LiDAR-based and multi-modality 3D detection methods. Contents related to monocular methods will be supplemented afterwards.
+
+## Prepare dataset
+
+You can download KITTI 3D detection data [HERE](http://www.cvlibs.net/datasets/kitti/eval_object.php?obj_benchmark=3d) and unzip all zip files. Besides, the road planes could be downloaded from [HERE](https://download.openmmlab.com/mmdetection3d/data/train_planes.zip), which are optional for data augmentation during training for better performance. The road planes are generated by [AVOD](https://github.com/kujason/avod), you can see more details [HERE](https://github.com/kujason/avod/issues/19).
+
+Like the general way to prepare dataset, it is recommended to symlink the dataset root to `$MMDETECTION3D/data`.
+
+The folder structure should be organized as follows before our processing.
+
+```
+mmdetection3d
+├── mmdet3d
+├── tools
+├── configs
+├── data
+│ ├── kitti
+│ │ ├── ImageSets
+│ │ ├── testing
+│ │ │ ├── calib
+│ │ │ ├── image_2
+│ │ │ ├── velodyne
+│ │ ├── training
+│ │ │ ├── calib
+│ │ │ ├── image_2
+│ │ │ ├── label_2
+│ │ │ ├── velodyne
+│ │ │ ├── planes (optional)
+```
+
+### Create KITTI dataset
+
+To create KITTI point cloud data, we load the raw point cloud data and generate the relevant annotations including object labels and bounding boxes. We also generate all single training objects' point cloud in KITTI dataset and save them as `.bin` files in `data/kitti/kitti_gt_database`. Meanwhile, `.pkl` info files are also generated for training or validation. Subsequently, create KITTI data by running
+
+```bash
+mkdir ./data/kitti/ && mkdir ./data/kitti/ImageSets
+
+# Download data split
+wget -c https://raw.githubusercontent.com/traveller59/second.pytorch/master/second/data/ImageSets/test.txt --no-check-certificate --content-disposition -O ./data/kitti/ImageSets/test.txt
+wget -c https://raw.githubusercontent.com/traveller59/second.pytorch/master/second/data/ImageSets/train.txt --no-check-certificate --content-disposition -O ./data/kitti/ImageSets/train.txt
+wget -c https://raw.githubusercontent.com/traveller59/second.pytorch/master/second/data/ImageSets/val.txt --no-check-certificate --content-disposition -O ./data/kitti/ImageSets/val.txt
+wget -c https://raw.githubusercontent.com/traveller59/second.pytorch/master/second/data/ImageSets/trainval.txt --no-check-certificate --content-disposition -O ./data/kitti/ImageSets/trainval.txt
+
+
+python tools/create_data.py kitti --root-path ./data/kitti --out-dir ./data/kitti --extra-tag kitti --with-plane
+
+```
+
+Note that if your local disk does not have enough space for saving converted data, you can change the `out-dir` to anywhere else, and you need to remove the `--with-plane` flag if `planes` are not prepared.
+
+The folder structure after processing should be as below
+
+```
+kitti
+├── ImageSets
+│ ├── test.txt
+│ ├── train.txt
+│ ├── trainval.txt
+│ ├── val.txt
+├── testing
+│ ├── calib
+│ ├── image_2
+│ ├── velodyne
+│ ├── velodyne_reduced
+├── training
+│ ├── calib
+│ ├── image_2
+│ ├── label_2
+│ ├── velodyne
+│ ├── velodyne_reduced
+│ ├── planes (optional)
+├── kitti_gt_database
+│ ├── xxxxx.bin
+├── kitti_infos_train.pkl
+├── kitti_infos_val.pkl
+├── kitti_dbinfos_train.pkl
+├── kitti_infos_test.pkl
+├── kitti_infos_trainval.pkl
+├── kitti_infos_train_mono3d.coco.json
+├── kitti_infos_trainval_mono3d.coco.json
+├── kitti_infos_test_mono3d.coco.json
+├── kitti_infos_val_mono3d.coco.json
+```
+
+- `kitti_gt_database/xxxxx.bin`: point cloud data included in each 3D bounding box of the training dataset
+- `kitti_infos_train.pkl`: training dataset infos, each frame info contains following details:
+ - info\['point_cloud'\]: {'num_features': 4, 'velodyne_path': velodyne_path}.
+ - info\['annos'\]: {
+ - location: x,y,z are bottom center in referenced camera coordinate system (in meters), an Nx3 array
+ - dimensions: height, width, length (in meters), an Nx3 array
+ - rotation_y: rotation ry around Y-axis in camera coordinates \[-pi..pi\], an N array
+ - name: ground truth name array, an N array
+ - difficulty: kitti difficulty, Easy, Moderate, Hard
+ - group_ids: used for multi-part object
+ }
+ - (optional) info\['calib'\]: {
+ - P0: camera0 projection matrix after rectification, an 3x4 array
+ - P1: camera1 projection matrix after rectification, an 3x4 array
+ - P2: camera2 projection matrix after rectification, an 3x4 array
+ - P3: camera3 projection matrix after rectification, an 3x4 array
+ - R0_rect: rectifying rotation matrix, an 4x4 array
+ - Tr_velo_to_cam: transformation from Velodyne coordinate to camera coordinate, an 4x4 array
+ - Tr_imu_to_velo: transformation from IMU coordinate to Velodyne coordinate, an 4x4 array
+ }
+ - (optional) info\['image'\]:{'image_idx': idx, 'image_path': image_path, 'image_shape', image_shape}.
+
+**Note:** the info\['annos'\] is in the referenced camera coordinate system. More details please refer to [this](http://www.cvlibs.net/publications/Geiger2013IJRR.pdf)
+
+The core function to get kitti_infos_xxx.pkl and kitti_infos_xxx_mono3d.coco.json are [get_kitti_image_info](https://github.com/open-mmlab/mmdetection3d/blob/7873c8f62b99314f35079f369d1dab8d63f8a3ce/tools/data_converter/kitti_data_utils.py#L140) and [get_2d_boxes](https://github.com/open-mmlab/mmdetection3d/blob/7873c8f62b99314f35079f369d1dab8d63f8a3ce/tools/data_converter/kitti_converter.py#L378). Please refer to [kitti_converter.py](https://github.com/open-mmlab/mmdetection3d/blob/7873c8f62b99314f35079f369d1dab8d63f8a3ce/tools/data_converter/kitti_converter.py) for more details.
+
+## Train pipeline
+
+A typical train pipeline of 3D detection on KITTI is as below.
+
+```python
+train_pipeline = [
+ dict(
+ type='LoadPointsFromFile',
+ coord_type='LIDAR',
+ load_dim=4, # x, y, z, intensity
+ use_dim=4, # x, y, z, intensity
+ file_client_args=file_client_args),
+ dict(
+ type='LoadAnnotations3D',
+ with_bbox_3d=True,
+ with_label_3d=True,
+ file_client_args=file_client_args),
+ dict(type='ObjectSample', db_sampler=db_sampler),
+ dict(
+ type='ObjectNoise',
+ num_try=100,
+ translation_std=[1.0, 1.0, 0.5],
+ global_rot_range=[0.0, 0.0],
+ rot_range=[-0.78539816, 0.78539816]),
+ dict(type='RandomFlip3D', flip_ratio_bev_horizontal=0.5),
+ dict(
+ type='GlobalRotScaleTrans',
+ rot_range=[-0.78539816, 0.78539816],
+ scale_ratio_range=[0.95, 1.05]),
+ dict(type='PointsRangeFilter', point_cloud_range=point_cloud_range),
+ dict(type='ObjectRangeFilter', point_cloud_range=point_cloud_range),
+ dict(type='PointShuffle'),
+ dict(type='DefaultFormatBundle3D', class_names=class_names),
+ dict(type='Collect3D', keys=['points', 'gt_bboxes_3d', 'gt_labels_3d'])
+]
+```
+
+- Data augmentation:
+ - `ObjectNoise`: apply noise to each GT objects in the scene.
+ - `RandomFlip3D`: randomly flip input point cloud horizontally or vertically.
+ - `GlobalRotScaleTrans`: rotate input point cloud.
+
+## Evaluation
+
+An example to evaluate PointPillars with 8 GPUs with kitti metrics is as follows:
+
+```shell
+bash tools/dist_test.sh configs/pointpillars/hv_pointpillars_secfpn_6x8_160e_kitti-3d-3class.py work_dirs/hv_pointpillars_secfpn_6x8_160e_kitti-3d-3class/latest.pth 8 --eval bbox
+```
+
+## Metrics
+
+KITTI evaluates 3D object detection performance using mean Average Precision (mAP) and Average Orientation Similarity (AOS), Please refer to its [official website](http://www.cvlibs.net/datasets/kitti/eval_3dobject.php) and [original paper](http://www.cvlibs.net/publications/Geiger2012CVPR.pdf) for more details.
+
+We also adopt this approach for evaluation on KITTI. An example of printed evaluation results is as follows:
+
+```
+Car AP@0.70, 0.70, 0.70:
+bbox AP:97.9252, 89.6183, 88.1564
+bev AP:90.4196, 87.9491, 85.1700
+3d AP:88.3891, 77.1624, 74.4654
+aos AP:97.70, 89.11, 87.38
+Car AP@0.70, 0.50, 0.50:
+bbox AP:97.9252, 89.6183, 88.1564
+bev AP:98.3509, 90.2042, 89.6102
+3d AP:98.2800, 90.1480, 89.4736
+aos AP:97.70, 89.11, 87.38
+```
+
+## Testing and make a submission
+
+An example to test PointPillars on KITTI with 8 GPUs and generate a submission to the leaderboard is as follows:
+
+```shell
+mkdir -p results/kitti-3class
+
+./tools/dist_test.sh configs/pointpillars/hv_pointpillars_secfpn_6x8_160e_kitti-3d-3class.py work_dirs/hv_pointpillars_secfpn_6x8_160e_kitti-3d-3class/latest.pth 8 --out results/kitti-3class/results_eval.pkl --format-only --eval-options 'pklfile_prefix=results/kitti-3class/kitti_results' 'submission_prefix=results/kitti-3class/kitti_results'
+```
+
+After generating `results/kitti-3class/kitti_results/xxxxx.txt` files, you can submit these files to KITTI benchmark. Please refer to the [KITTI official website](http://www.cvlibs.net/datasets/kitti/index.php) for more details.
diff --git a/docs/en/datasets/lyft_det.md b/docs/en/datasets/lyft_det.md
new file mode 100644
index 0000000..3bc1927
--- /dev/null
+++ b/docs/en/datasets/lyft_det.md
@@ -0,0 +1,197 @@
+# Lyft Dataset for 3D Object Detection
+
+This page provides specific tutorials about the usage of MMDetection3D for Lyft dataset.
+
+## Before Preparation
+
+You can download Lyft 3D detection data [HERE](https://www.kaggle.com/c/3d-object-detection-for-autonomous-vehicles/data) and unzip all zip files.
+
+Like the general way to prepare a dataset, it is recommended to symlink the dataset root to `$MMDETECTION3D/data`.
+
+The folder structure should be organized as follows before our processing.
+
+```
+mmdetection3d
+├── mmdet3d
+├── tools
+├── configs
+├── data
+│ ├── lyft
+│ │ ├── v1.01-train
+│ │ │ ├── v1.01-train (train_data)
+│ │ │ ├── lidar (train_lidar)
+│ │ │ ├── images (train_images)
+│ │ │ ├── maps (train_maps)
+│ │ ├── v1.01-test
+│ │ │ ├── v1.01-test (test_data)
+│ │ │ ├── lidar (test_lidar)
+│ │ │ ├── images (test_images)
+│ │ │ ├── maps (test_maps)
+│ │ ├── train.txt
+│ │ ├── val.txt
+│ │ ├── test.txt
+│ │ ├── sample_submission.csv
+```
+
+Here `v1.01-train` and `v1.01-test` contain the metafiles which are similar to those of nuScenes. `.txt` files contain the data split information.
+Lyft does not have an official split for training and validation set, so we provide a split considering the number of objects from different categories in different scenes.
+`sample_submission.csv` is the base file for submission on the Kaggle evaluation server.
+Note that we follow the original folder names for clear organization. Please rename the raw folders as shown above.
+
+## Dataset Preparation
+
+The way to organize Lyft dataset is similar to nuScenes. We also generate the .pkl and .json files which share almost the same structure.
+Next, we will mainly focus on the difference between these two datasets. For a more detailed explanation of the info structure, please refer to [nuScenes tutorial](https://github.com/open-mmlab/mmdetection3d/blob/master/docs/en/datasets/nuscenes_det.md).
+
+To prepare info files for Lyft, run the following commands:
+
+```bash
+python tools/create_data.py lyft --root-path ./data/lyft --out-dir ./data/lyft --extra-tag lyft --version v1.01
+python tools/data_converter/lyft_data_fixer.py --version v1.01 --root-folder ./data/lyft
+```
+
+Note that the second command serves the purpose of fixing a corrupted lidar data file. Please refer to the discussion [here](https://www.kaggle.com/c/3d-object-detection-for-autonomous-vehicles/discussion/110000) for more details.
+
+The folder structure after processing should be as below.
+
+```
+mmdetection3d
+├── mmdet3d
+├── tools
+├── configs
+├── data
+│ ├── lyft
+│ │ ├── v1.01-train
+│ │ │ ├── v1.01-train (train_data)
+│ │ │ ├── lidar (train_lidar)
+│ │ │ ├── images (train_images)
+│ │ │ ├── maps (train_maps)
+│ │ ├── v1.01-test
+│ │ │ ├── v1.01-test (test_data)
+│ │ │ ├── lidar (test_lidar)
+│ │ │ ├── images (test_images)
+│ │ │ ├── maps (test_maps)
+│ │ ├── train.txt
+│ │ ├── val.txt
+│ │ ├── test.txt
+│ │ ├── sample_submission.csv
+│ │ ├── lyft_infos_train.pkl
+│ │ ├── lyft_infos_val.pkl
+│ │ ├── lyft_infos_test.pkl
+│ │ ├── lyft_infos_train_mono3d.coco.json
+│ │ ├── lyft_infos_val_mono3d.coco.json
+│ │ ├── lyft_infos_test_mono3d.coco.json
+```
+
+Here, .pkl files are generally used for methods involving point clouds, and coco-style .json files are more suitable for image-based methods, such as image-based 2D and 3D detection.
+Different from nuScenes, we only support using the json files for 2D detection experiments. Image-based 3D detection may be further supported in the future.
+
+Next, we will elaborate on the difference compared to nuScenes in terms of the details recorded in these info files.
+
+- without `lyft_database/xxxxx.bin`: This folder and `.bin` files are not extracted on the Lyft dataset due to the negligible effect of ground-truth sampling in the experiments.
+- `lyft_infos_train.pkl`: training dataset infos, each frame info has two keys: `metadata` and `infos`.
+ `metadata` contains the basic information for the dataset itself, such as `{'version': 'v1.01-train'}`, while `infos` contains the detailed information the same as nuScenes except for the following details:
+ - info\['sweeps'\]: Sweeps information.
+ - info\['sweeps'\]\[i\]\['type'\]: The sweep data type, e.g., `'lidar'`.
+ Lyft has different LiDAR settings for some samples, but we always take only the points collected by the top LiDAR for the consistency of data distribution.
+ - info\['gt_names'\]: There are 9 categories on the Lyft dataset, and the imbalance of annotations for different categories is even more significant than nuScenes.
+ - without info\['gt_velocity'\]: There is no velocity measurement on Lyft.
+ - info\['num_lidar_pts'\]: Set to -1 by default.
+ - info\['num_radar_pts'\]: Set to 0 by default.
+ - without info\['valid_flag'\]: This flag does recorded due to invalid `num_lidar_pts` and `num_radar_pts`.
+- `nuscenes_infos_train_mono3d.coco.json`: training dataset coco-style info. This file only contains 2D information, without the information required by 3D detection, such as camera intrinsics.
+ - info\['images'\]: A list containing all the image info.
+ - only containing `'file_name'`, `'id'`, `'width'`, `'height'`.
+ - info\['annotations'\]: A list containing all the annotation info.
+ - only containing `'file_name'`, `'image_id'`, `'area'`, `'category_name'`, `'category_id'`, `'bbox'`, `'is_crowd'`, `'segmentation'`, `'id'`, where `'is_crowd'`, `'segmentation'` are set to `0` and `[]` by default.
+ There is no attribute annotation on Lyft.
+
+Here we only explain the data recorded in the training info files. The same applies to the testing set.
+
+The core function to get `lyft_infos_xxx.pkl` is [\_fill_trainval_infos](https://github.com/open-mmlab/mmdetection3d/blob/master/tools/data_converter/lyft_converter.py#L93).
+Please refer to [lyft_converter.py](https://github.com/open-mmlab/mmdetection3d/blob/master/tools/data_converter/lyft_converter.py) for more details.
+
+## Training pipeline
+
+### LiDAR-Based Methods
+
+A typical training pipeline of LiDAR-based 3D detection (including multi-modality methods) on Lyft is almost the same as nuScenes as below.
+
+```python
+train_pipeline = [
+ dict(
+ type='LoadPointsFromFile',
+ coord_type='LIDAR',
+ load_dim=5,
+ use_dim=5,
+ file_client_args=file_client_args),
+ dict(
+ type='LoadPointsFromMultiSweeps',
+ sweeps_num=10,
+ file_client_args=file_client_args),
+ dict(type='LoadAnnotations3D', with_bbox_3d=True, with_label_3d=True),
+ dict(
+ type='GlobalRotScaleTrans',
+ rot_range=[-0.3925, 0.3925],
+ scale_ratio_range=[0.95, 1.05],
+ translation_std=[0, 0, 0]),
+ dict(type='RandomFlip3D', flip_ratio_bev_horizontal=0.5),
+ dict(type='PointsRangeFilter', point_cloud_range=point_cloud_range),
+ dict(type='ObjectRangeFilter', point_cloud_range=point_cloud_range),
+ dict(type='PointShuffle'),
+ dict(type='DefaultFormatBundle3D', class_names=class_names),
+ dict(type='Collect3D', keys=['points', 'gt_bboxes_3d', 'gt_labels_3d'])
+]
+```
+
+Similar to nuScenes, models on Lyft also need the `'LoadPointsFromMultiSweeps'` pipeline to load point clouds from consecutive frames.
+In addition, considering the intensity of LiDAR points collected by Lyft is invalid, we also set the `use_dim` in `'LoadPointsFromMultiSweeps'` to `[0, 1, 2, 4]` by default,
+where the first 3 dimensions refer to point coordinates, and the last refers to timestamp differences.
+
+## Evaluation
+
+An example to evaluate PointPillars with 8 GPUs with Lyft metrics is as follows.
+
+```shell
+bash ./tools/dist_test.sh configs/pointpillars/hv_pointpillars_fpn_sbn-all_2x8_2x_lyft-3d.py checkpoints/hv_pointpillars_fpn_sbn-all_2x8_2x_lyft-3d_20210517_202818-fc6904c3.pth 8 --eval bbox
+```
+
+## Metrics
+
+Lyft proposes a more strict metric for evaluating the predicted 3D bounding boxes.
+The basic criteria to judge whether a predicted box is positive or not is the same as KITTI, i.e. the 3D Intersection over Union (IoU).
+However, it adopts a way similar to COCO to compute the mean average precision (mAP) -- compute the average precision under different thresholds of 3D IoU from 0.5-0.95.
+Actually, overlap more than 0.7 3D IoU is a quite strict criterion for 3D detection methods, so the overall performance seems a little low.
+The imbalance of annotations for different categories is another important reason for the finally lower results compared to other datasets.
+Please refer to its [official website](https://www.kaggle.com/c/3d-object-detection-for-autonomous-vehicles/overview/evaluation) for more details about the definition of this metric.
+
+We employ this official method for evaluation on Lyft. An example of printed evaluation results is as follows:
+
+```
++mAPs@0.5:0.95------+--------------+
+| class | mAP@0.5:0.95 |
++-------------------+--------------+
+| animal | 0.0 |
+| bicycle | 0.099 |
+| bus | 0.177 |
+| car | 0.422 |
+| emergency_vehicle | 0.0 |
+| motorcycle | 0.049 |
+| other_vehicle | 0.359 |
+| pedestrian | 0.066 |
+| truck | 0.176 |
+| Overall | 0.15 |
++-------------------+--------------+
+```
+
+## Testing and make a submission
+
+An example to test PointPillars on Lyft with 8 GPUs and generate a submission to the leaderboard is as follows.
+
+```shell
+./tools/dist_test.sh configs/pointpillars/hv_pointpillars_fpn_sbn-all_2x8_2x_lyft-3d.py work_dirs/pp-lyft/latest.pth 8 --out work_dirs/pp-lyft/results_challenge.pkl --format-only --eval-options 'jsonfile_prefix=work_dirs/pp-lyft/results_challenge' 'csv_savepath=results/pp-lyft/results_challenge.csv'
+```
+
+After generating the `work_dirs/pp-lyft/results_challenge.csv`, you can submit it to the Kaggle evaluation server. Please refer to the [official website](https://www.kaggle.com/c/3d-object-detection-for-autonomous-vehicles) for more information.
+
+We can also visualize the prediction results with our developed visualization tools. Please refer to the [visualization doc](https://mmdetection3d.readthedocs.io/en/latest/useful_tools.html#visualization) for more details.
diff --git a/docs/en/datasets/nuscenes_det.md b/docs/en/datasets/nuscenes_det.md
new file mode 100644
index 0000000..60e1935
--- /dev/null
+++ b/docs/en/datasets/nuscenes_det.md
@@ -0,0 +1,263 @@
+# NuScenes Dataset for 3D Object Detection
+
+This page provides specific tutorials about the usage of MMDetection3D for nuScenes dataset.
+
+## Before Preparation
+
+You can download nuScenes 3D detection data [HERE](https://www.nuscenes.org/download) and unzip all zip files.
+
+Like the general way to prepare dataset, it is recommended to symlink the dataset root to `$MMDETECTION3D/data`.
+
+The folder structure should be organized as follows before our processing.
+
+```
+mmdetection3d
+├── mmdet3d
+├── tools
+├── configs
+├── data
+│ ├── nuscenes
+│ │ ├── maps
+│ │ ├── samples
+│ │ ├── sweeps
+│ │ ├── v1.0-test
+| | ├── v1.0-trainval
+```
+
+## Dataset Preparation
+
+We typically need to organize the useful data information with a .pkl or .json file in a specific style, e.g., coco-style for organizing images and their annotations.
+To prepare these files for nuScenes, run the following command:
+
+```bash
+python tools/create_data.py nuscenes --root-path ./data/nuscenes --out-dir ./data/nuscenes --extra-tag nuscenes
+```
+
+The folder structure after processing should be as below.
+
+```
+mmdetection3d
+├── mmdet3d
+├── tools
+├── configs
+├── data
+│ ├── nuscenes
+│ │ ├── maps
+│ │ ├── samples
+│ │ ├── sweeps
+│ │ ├── v1.0-test
+| | ├── v1.0-trainval
+│ │ ├── nuscenes_database
+│ │ ├── nuscenes_infos_train.pkl
+│ │ ├── nuscenes_infos_val.pkl
+│ │ ├── nuscenes_infos_test.pkl
+│ │ ├── nuscenes_dbinfos_train.pkl
+│ │ ├── nuscenes_infos_train_mono3d.coco.json
+│ │ ├── nuscenes_infos_val_mono3d.coco.json
+│ │ ├── nuscenes_infos_test_mono3d.coco.json
+```
+
+Here, .pkl files are generally used for methods involving point clouds and coco-style .json files are more suitable for image-based methods, such as image-based 2D and 3D detection.
+Next, we will elaborate on the details recorded in these info files.
+
+- `nuscenes_database/xxxxx.bin`: point cloud data included in each 3D bounding box of the training dataset
+- `nuscenes_infos_train.pkl`: training dataset info, each frame info has two keys: `metadata` and `infos`.
+ `metadata` contains the basic information for the dataset itself, such as `{'version': 'v1.0-trainval'}`, while `infos` contains the detailed information as follows:
+ - info\['lidar_path'\]: The file path of the lidar point cloud data.
+ - info\['token'\]: Sample data token.
+ - info\['sweeps'\]: Sweeps information (`sweeps` in the nuScenes refer to the intermediate frames without annotations, while `samples` refer to those key frames with annotations).
+ - info\['sweeps'\]\[i\]\['data_path'\]: The data path of i-th sweep.
+ - info\['sweeps'\]\[i\]\['type'\]: The sweep data type, e.g., `'lidar'`.
+ - info\['sweeps'\]\[i\]\['sample_data_token'\]: The sweep sample data token.
+ - info\['sweeps'\]\[i\]\['sensor2ego_translation'\]: The translation from the current sensor (for collecting the sweep data) to ego vehicle. (1x3 list)
+ - info\['sweeps'\]\[i\]\['sensor2ego_rotation'\]: The rotation from the current sensor (for collecting the sweep data) to ego vehicle. (1x4 list in the quaternion format)
+ - info\['sweeps'\]\[i\]\['ego2global_translation'\]: The translation from the ego vehicle to global coordinates. (1x3 list)
+ - info\['sweeps'\]\[i\]\['ego2global_rotation'\]: The rotation from the ego vehicle to global coordinates. (1x4 list in the quaternion format)
+ - info\['sweeps'\]\[i\]\['timestamp'\]: Timestamp of the sweep data.
+ - info\['sweeps'\]\[i\]\['sensor2lidar_translation'\]: The translation from the current sensor (for collecting the sweep data) to lidar. (1x3 list)
+ - info\['sweeps'\]\[i\]\['sensor2lidar_rotation'\]: The rotation from the current sensor (for collecting the sweep data) to lidar. (1x4 list in the quaternion format)
+ - info\['cams'\]: Cameras calibration information. It contains six keys corresponding to each camera: `'CAM_FRONT'`, `'CAM_FRONT_RIGHT'`, `'CAM_FRONT_LEFT'`, `'CAM_BACK'`, `'CAM_BACK_LEFT'`, `'CAM_BACK_RIGHT'`.
+ Each dictionary contains detailed information following the above way for each sweep data (has the same keys for each information as above). In addition, each camera has a key `'cam_intrinsic'` for recording the intrinsic parameters when projecting 3D points to each image plane.
+ - info\['lidar2ego_translation'\]: The translation from lidar to ego vehicle. (1x3 list)
+ - info\['lidar2ego_rotation'\]: The rotation from lidar to ego vehicle. (1x4 list in the quaternion format)
+ - info\['ego2global_translation'\]: The translation from the ego vehicle to global coordinates. (1x3 list)
+ - info\['ego2global_rotation'\]: The rotation from the ego vehicle to global coordinates. (1x4 list in the quaternion format)
+ - info\['timestamp'\]: Timestamp of the sample data.
+ - info\['gt_boxes'\]: 7-DoF annotations of 3D bounding boxes, an Nx7 array.
+ - info\['gt_names'\]: Categories of 3D bounding boxes, an 1xN array.
+ - info\['gt_velocity'\]: Velocities of 3D bounding boxes (no vertical measurements due to inaccuracy), an Nx2 array.
+ - info\['num_lidar_pts'\]: Number of lidar points included in each 3D bounding box.
+ - info\['num_radar_pts'\]: Number of radar points included in each 3D bounding box.
+ - info\['valid_flag'\]: Whether each bounding box is valid. In general, we only take the 3D boxes that include at least one lidar or radar point as valid boxes.
+- `nuscenes_infos_train_mono3d.coco.json`: training dataset coco-style info. This file organizes image-based data into three categories (keys): `'categories'`, `'images'`, `'annotations'`.
+ - info\['categories'\]: A list containing all the category names. Each element follows the dictionary format and consists of two keys: `'id'` and `'name'`.
+ - info\['images'\]: A list containing all the image info.
+ - info\['images'\]\[i\]\['file_name'\]: The file name of the i-th image.
+ - info\['images'\]\[i\]\['id'\]: Sample data token of the i-th image.
+ - info\['images'\]\[i\]\['token'\]: Sample token corresponding to this frame.
+ - info\['images'\]\[i\]\['cam2ego_rotation'\]: The rotation from the camera to ego vehicle. (1x4 list in the quaternion format)
+ - info\['images'\]\[i\]\['cam2ego_translation'\]: The translation from the camera to ego vehicle. (1x3 list)
+ - info\['images'\]\[i\]\['ego2global_rotation''\]: The rotation from the ego vehicle to global coordinates. (1x4 list in the quaternion format)
+ - info\['images'\]\[i\]\['ego2global_translation'\]: The translation from the ego vehicle to global coordinates. (1x3 list)
+ - info\['images'\]\[i\]\['cam_intrinsic'\]: Camera intrinsic matrix. (3x3 list)
+ - info\['images'\]\[i\]\['width'\]: Image width, 1600 by default in nuScenes.
+ - info\['images'\]\[i\]\['height'\]: Image height, 900 by default in nuScenes.
+ - info\['annotations'\]: A list containing all the annotation info.
+ - info\['annotations'\]\[i\]\['file_name'\]: The file name of the corresponding image.
+ - info\['annotations'\]\[i\]\['image_id'\]: The image id (token) of the corresponding image.
+ - info\['annotations'\]\[i\]\['area'\]: Area of the 2D bounding box.
+ - info\['annotations'\]\[i\]\['category_name'\]: Category name.
+ - info\['annotations'\]\[i\]\['category_id'\]: Category id.
+ - info\['annotations'\]\[i\]\['bbox'\]: 2D bounding box annotation (exterior rectangle of the projected 3D box), 1x4 list following \[x1, y1, x2-x1, y2-y1\].
+ x1/y1 are minimum coordinates along horizontal/vertical direction of the image.
+ - info\['annotations'\]\[i\]\['iscrowd'\]: Whether the region is crowded. Defaults to 0.
+ - info\['annotations'\]\[i\]\['bbox_cam3d'\]: 3D bounding box (gravity) center location (3), size (3), (global) yaw angle (1), 1x7 list.
+ - info\['annotations'\]\[i\]\['velo_cam3d'\]: Velocities of 3D bounding boxes (no vertical measurements due to inaccuracy), an Nx2 array.
+ - info\['annotations'\]\[i\]\['center2d'\]: Projected 3D-center containing 2.5D information: projected center location on the image (2) and depth (1), 1x3 list.
+ - info\['annotations'\]\[i\]\['attribute_name'\]: Attribute name.
+ - info\['annotations'\]\[i\]\['attribute_id'\]: Attribute id.
+ We maintain a default attribute collection and mapping for attribute classification.
+ Please refer to [here](https://github.com/open-mmlab/mmdetection3d/blob/master/mmdet3d/datasets/nuscenes_mono_dataset.py#L53) for more details.
+ - info\['annotations'\]\[i\]\['id'\]: Annotation id. Defaults to `i`.
+
+Here we only explain the data recorded in the training info files. The same applies to validation and testing set.
+
+The core function to get `nuscenes_infos_xxx.pkl` and `nuscenes_infos_xxx_mono3d.coco.json` are [\_fill_trainval_infos](https://github.com/open-mmlab/mmdetection3d/blob/master/tools/data_converter/nuscenes_converter.py#L143) and [get_2d_boxes](https://github.com/open-mmlab/mmdetection3d/blob/master/tools/data_converter/nuscenes_converter.py#L397), respectively.
+Please refer to [nuscenes_converter.py](https://github.com/open-mmlab/mmdetection3d/blob/master/tools/data_converter/nuscenes_converter.py) for more details.
+
+## Training pipeline
+
+### LiDAR-Based Methods
+
+A typical training pipeline of LiDAR-based 3D detection (including multi-modality methods) on nuScenes is as below.
+
+```python
+train_pipeline = [
+ dict(
+ type='LoadPointsFromFile',
+ coord_type='LIDAR',
+ load_dim=5,
+ use_dim=5,
+ file_client_args=file_client_args),
+ dict(
+ type='LoadPointsFromMultiSweeps',
+ sweeps_num=10,
+ file_client_args=file_client_args),
+ dict(type='LoadAnnotations3D', with_bbox_3d=True, with_label_3d=True),
+ dict(
+ type='GlobalRotScaleTrans',
+ rot_range=[-0.3925, 0.3925],
+ scale_ratio_range=[0.95, 1.05],
+ translation_std=[0, 0, 0]),
+ dict(type='RandomFlip3D', flip_ratio_bev_horizontal=0.5),
+ dict(type='PointsRangeFilter', point_cloud_range=point_cloud_range),
+ dict(type='ObjectRangeFilter', point_cloud_range=point_cloud_range),
+ dict(type='ObjectNameFilter', classes=class_names),
+ dict(type='PointShuffle'),
+ dict(type='DefaultFormatBundle3D', class_names=class_names),
+ dict(type='Collect3D', keys=['points', 'gt_bboxes_3d', 'gt_labels_3d'])
+]
+```
+
+Compared to general cases, nuScenes has a specific `'LoadPointsFromMultiSweeps'` pipeline to load point clouds from consecutive frames. This is a common practice used in this setting.
+Please refer to the nuScenes [original paper](https://arxiv.org/abs/1903.11027) for more details.
+The default `use_dim` in `'LoadPointsFromMultiSweeps'` is `[0, 1, 2, 4]`, where the first 3 dimensions refer to point coordinates and the last refers to timestamp differences.
+Intensity is not used by default due to its yielded noise when concatenating the points from different frames.
+
+### Vision-Based Methods
+
+A typical training pipeline of image-based 3D detection on nuScenes is as below.
+
+```python
+train_pipeline = [
+ dict(type='LoadImageFromFileMono3D'),
+ dict(
+ type='LoadAnnotations3D',
+ with_bbox=True,
+ with_label=True,
+ with_attr_label=True,
+ with_bbox_3d=True,
+ with_label_3d=True,
+ with_bbox_depth=True),
+ dict(type='Resize', img_scale=(1600, 900), keep_ratio=True),
+ dict(type='RandomFlip3D', flip_ratio_bev_horizontal=0.5),
+ dict(type='Normalize', **img_norm_cfg),
+ dict(type='Pad', size_divisor=32),
+ dict(type='DefaultFormatBundle3D', class_names=class_names),
+ dict(
+ type='Collect3D',
+ keys=[
+ 'img', 'gt_bboxes', 'gt_labels', 'attr_labels', 'gt_bboxes_3d',
+ 'gt_labels_3d', 'centers2d', 'depths'
+ ]),
+]
+```
+
+It follows the general pipeline of 2D detection while differs in some details:
+
+- It uses monocular pipelines to load images, which includes additional required information like camera intrinsics.
+- It needs to load 3D annotations.
+- Some data augmentation techniques need to be adjusted, such as `RandomFlip3D`.
+ Currently we do not support more augmentation methods, because how to transfer and apply other techniques is still under explored.
+
+## Evaluation
+
+An example to evaluate PointPillars with 8 GPUs with nuScenes metrics is as follows.
+
+```shell
+bash ./tools/dist_test.sh configs/pointpillars/hv_pointpillars_fpn_sbn-all_4x8_2x_nus-3d.py checkpoints/hv_pointpillars_fpn_sbn-all_4x8_2x_nus-3d_20200620_230405-2fa62f3d.pth 8 --eval bbox
+```
+
+## Metrics
+
+NuScenes proposes a comprehensive metric, namely nuScenes detection score (NDS), to evaluate different methods and set up the benchmark.
+It consists of mean Average Precision (mAP), Average Translation Error (ATE), Average Scale Error (ASE), Average Orientation Error (AOE), Average Velocity Error (AVE) and Average Attribute Error (AAE).
+Please refer to its [official website](https://www.nuscenes.org/object-detection?externalData=all&mapData=all&modalities=Any) for more details.
+
+We also adopt this approach for evaluation on nuScenes. An example of printed evaluation results is as follows:
+
+```
+mAP: 0.3197
+mATE: 0.7595
+mASE: 0.2700
+mAOE: 0.4918
+mAVE: 1.3307
+mAAE: 0.1724
+NDS: 0.3905
+Eval time: 170.8s
+
+Per-class results:
+Object Class AP ATE ASE AOE AVE AAE
+car 0.503 0.577 0.152 0.111 2.096 0.136
+truck 0.223 0.857 0.224 0.220 1.389 0.179
+bus 0.294 0.855 0.204 0.190 2.689 0.283
+trailer 0.081 1.094 0.243 0.553 0.742 0.167
+construction_vehicle 0.058 1.017 0.450 1.019 0.137 0.341
+pedestrian 0.392 0.687 0.284 0.694 0.876 0.158
+motorcycle 0.317 0.737 0.265 0.580 2.033 0.104
+bicycle 0.308 0.704 0.299 0.892 0.683 0.010
+traffic_cone 0.555 0.486 0.309 nan nan nan
+barrier 0.466 0.581 0.269 0.169 nan nan
+```
+
+## Testing and make a submission
+
+An example to test PointPillars on nuScenes with 8 GPUs and generate a submission to the leaderboard is as follows.
+
+```shell
+./tools/dist_test.sh configs/pointpillars/hv_pointpillars_fpn_sbn-all_4x8_2x_nus-3d.py work_dirs/pp-nus/latest.pth 8 --out work_dirs/pp-nus/results_eval.pkl --format-only --eval-options 'jsonfile_prefix=work_dirs/pp-nus/results_eval'
+```
+
+Note that the testing info should be changed to that for testing set instead of validation set [here](https://github.com/open-mmlab/mmdetection3d/blob/master/configs/_base_/datasets/nus-3d.py#L132).
+
+After generating the `work_dirs/pp-nus/results_eval.json`, you can compress it and submit it to nuScenes benchmark. Please refer to the [nuScenes official website](https://www.nuscenes.org/object-detection?externalData=all&mapData=all&modalities=Any) for more information.
+
+We can also visualize the prediction results with our developed visualization tools. Please refer to the [visualization doc](https://mmdetection3d.readthedocs.io/en/latest/useful_tools.html#visualization) for more details.
+
+## Notes
+
+### Transformation between `NuScenesBox` and our `CameraInstanceBoxes`.
+
+In general, the main difference of `NuScenesBox` and our `CameraInstanceBoxes` is mainly reflected in the yaw definition. `NuScenesBox` defines the rotation with a quaternion or three Euler angles while ours only defines one yaw angle due to the practical scenario. It requires us to add some additional rotations manually in the pre-processing and post-processing, such as [here](https://github.com/open-mmlab/mmdetection3d/blob/master/mmdet3d/datasets/nuscenes_mono_dataset.py#L673).
+
+In addition, please note that the definition of corners and locations are detached in the `NuScenesBox`. For example, in monocular 3D detection, the definition of the box location is in its camera coordinate (see its official [illustration](https://www.nuscenes.org/nuscenes#data-collection) for car setup), which is consistent with [ours](https://github.com/open-mmlab/mmdetection3d/blob/master/mmdet3d/core/bbox/structures/cam_box3d.py). In contrast, its corners are defined with the [convention](https://github.com/nutonomy/nuscenes-devkit/blob/02e9200218977193a1058dd7234f935834378319/python-sdk/nuscenes/utils/data_classes.py#L527) "x points forward, y to the left, z up". It results in different philosophy of dimension and rotation definitions from our `CameraInstanceBoxes`. An example to remove similar hacks is PR [#744](https://github.com/open-mmlab/mmdetection3d/pull/744). The same problem also exists in the LiDAR system. To deal with them, we typically add some transformation in the pre-processing and post-processing to guarantee the box will be in our coordinate system during the entire training and inference procedure.
diff --git a/docs/en/datasets/s3dis_sem_seg.md b/docs/en/datasets/s3dis_sem_seg.md
new file mode 100644
index 0000000..d11162f
--- /dev/null
+++ b/docs/en/datasets/s3dis_sem_seg.md
@@ -0,0 +1,254 @@
+# S3DIS for 3D Semantic Segmentation
+
+## Dataset preparation
+
+For the overall process, please refer to the [README](https://github.com/open-mmlab/mmdetection3d/blob/master/data/s3dis/README.md/) page for S3DIS.
+
+### Export S3DIS data
+
+By exporting S3DIS data, we load the raw point cloud data and generate the relevant annotations including semantic labels and instance labels.
+
+The directory structure before exporting should be as below:
+
+```
+mmdetection3d
+├── mmdet3d
+├── tools
+├── configs
+├── data
+│ ├── s3dis
+│ │ ├── meta_data
+│ │ ├── Stanford3dDataset_v1.2_Aligned_Version
+│ │ │ ├── Area_1
+│ │ │ │ ├── conferenceRoom_1
+│ │ │ │ ├── office_1
+│ │ │ │ ├── ...
+│ │ │ ├── Area_2
+│ │ │ ├── Area_3
+│ │ │ ├── Area_4
+│ │ │ ├── Area_5
+│ │ │ ├── Area_6
+│ │ ├── indoor3d_util.py
+│ │ ├── collect_indoor3d_data.py
+│ │ ├── README.md
+```
+
+Under folder `Stanford3dDataset_v1.2_Aligned_Version`, the rooms are spilted into 6 areas. We use 5 areas for training and 1 for evaluation (typically `Area_5`). Under the directory of each area, there are folders in which raw point cloud data and relevant annotations are saved. For instance, under folder `Area_1/office_1` the files are as below:
+
+- `office_1.txt`: A txt file storing coordinates and colors of each point in the raw point cloud data.
+
+- `Annotations/`: This folder contains txt files for different object instances. Each txt file represents one instance, e.g.
+
+ - `chair_1.txt`: A txt file storing raw point cloud data of one chair in this room.
+
+ If we concat all the txt files under `Annotations/`, we will get the same point cloud as denoted by `office_1.txt`.
+
+Export S3DIS data by running `python collect_indoor3d_data.py`. The main steps include:
+
+- Export original txt files to point cloud, instance label and semantic label.
+- Save point cloud data and relevant annotation files.
+
+And the core function `export` in `indoor3d_util.py` is as follows:
+
+```python
+def export(anno_path, out_filename):
+ """Convert original dataset files to points, instance mask and semantic
+ mask files. We aggregated all the points from each instance in the room.
+
+ Args:
+ anno_path (str): path to annotations. e.g. Area_1/office_2/Annotations/
+ out_filename (str): path to save collected points and labels.
+ file_format (str): txt or numpy, determines what file format to save.
+
+ Note:
+ the points are shifted before save, the most negative point is now
+ at origin.
+ """
+ points_list = []
+ ins_idx = 1 # instance ids should be indexed from 1, so 0 is unannotated
+
+ # an example of `anno_path`: Area_1/office_1/Annotations
+ # which contains all object instances in this room as txt files
+ for f in glob.glob(osp.join(anno_path, '*.txt')):
+ # get class name of this instance
+ one_class = osp.basename(f).split('_')[0]
+ if one_class not in class_names: # some rooms have 'staris' class
+ one_class = 'clutter'
+ points = np.loadtxt(f)
+ labels = np.ones((points.shape[0], 1)) * class2label[one_class]
+ ins_labels = np.ones((points.shape[0], 1)) * ins_idx
+ ins_idx += 1
+ points_list.append(np.concatenate([points, labels, ins_labels], 1))
+
+ data_label = np.concatenate(points_list, 0) # [N, 8], (pts, rgb, sem, ins)
+ # align point cloud to the origin
+ xyz_min = np.amin(data_label, axis=0)[0:3]
+ data_label[:, 0:3] -= xyz_min
+
+ np.save(f'{out_filename}_point.npy', data_label[:, :6].astype(np.float32))
+ np.save(f'{out_filename}_sem_label.npy', data_label[:, 6].astype(np.int))
+ np.save(f'{out_filename}_ins_label.npy', data_label[:, 7].astype(np.int))
+
+```
+
+where we load and concatenate all the point cloud instances under `Annotations/` to form raw point cloud and generate semantic/instance labels. After exporting each room, the point cloud data, semantic labels and instance labels should be saved in `.npy` files.
+
+### Create dataset
+
+```shell
+python tools/create_data.py s3dis --root-path ./data/s3dis \
+--out-dir ./data/s3dis --extra-tag s3dis
+```
+
+The above exported point cloud files, semantic label files and instance label files are further saved in `.bin` format. Meanwhile `.pkl` info files are also generated for each area.
+
+The directory structure after process should be as below:
+
+```
+s3dis
+├── meta_data
+├── indoor3d_util.py
+├── collect_indoor3d_data.py
+├── README.md
+├── Stanford3dDataset_v1.2_Aligned_Version
+├── s3dis_data
+├── points
+│ ├── xxxxx.bin
+├── instance_mask
+│ ├── xxxxx.bin
+├── semantic_mask
+│ ├── xxxxx.bin
+├── seg_info
+│ ├── Area_1_label_weight.npy
+│ ├── Area_1_resampled_scene_idxs.npy
+│ ├── Area_2_label_weight.npy
+│ ├── Area_2_resampled_scene_idxs.npy
+│ ├── Area_3_label_weight.npy
+│ ├── Area_3_resampled_scene_idxs.npy
+│ ├── Area_4_label_weight.npy
+│ ├── Area_4_resampled_scene_idxs.npy
+│ ├── Area_5_label_weight.npy
+│ ├── Area_5_resampled_scene_idxs.npy
+│ ├── Area_6_label_weight.npy
+│ ├── Area_6_resampled_scene_idxs.npy
+├── s3dis_infos_Area_1.pkl
+├── s3dis_infos_Area_2.pkl
+├── s3dis_infos_Area_3.pkl
+├── s3dis_infos_Area_4.pkl
+├── s3dis_infos_Area_5.pkl
+├── s3dis_infos_Area_6.pkl
+```
+
+- `points/xxxxx.bin`: The exported point cloud data.
+- `instance_mask/xxxxx.bin`: The instance label for each point, value range: \[0, ${NUM_INSTANCES}\], 0: unannotated.
+- `semantic_mask/xxxxx.bin`: The semantic label for each point, value range: \[0, 12\].
+- `s3dis_infos_Area_1.pkl`: Area 1 data infos, the detailed info of each room is as follows:
+ - info\['point_cloud'\]: {'num_features': 6, 'lidar_idx': sample_idx}.
+ - info\['pts_path'\]: The path of `points/xxxxx.bin`.
+ - info\['pts_instance_mask_path'\]: The path of `instance_mask/xxxxx.bin`.
+ - info\['pts_semantic_mask_path'\]: The path of `semantic_mask/xxxxx.bin`.
+- `seg_info`: The generated infos to support semantic segmentation model training.
+ - `Area_1_label_weight.npy`: Weighting factor for each semantic class. Since the number of points in different classes varies greatly, it's a common practice to use label re-weighting to get a better performance.
+ - `Area_1_resampled_scene_idxs.npy`: Re-sampling index for each scene. Different rooms will be sampled multiple times according to their number of points to balance training data.
+
+## Training pipeline
+
+A typical training pipeline of S3DIS for 3D semantic segmentation is as below.
+
+```python
+class_names = ('ceiling', 'floor', 'wall', 'beam', 'column', 'window', 'door',
+ 'table', 'chair', 'sofa', 'bookcase', 'board', 'clutter')
+num_points = 4096
+train_pipeline = [
+ dict(
+ type='LoadPointsFromFile',
+ coord_type='DEPTH',
+ shift_height=False,
+ use_color=True,
+ load_dim=6,
+ use_dim=[0, 1, 2, 3, 4, 5]),
+ dict(
+ type='LoadAnnotations3D',
+ with_bbox_3d=False,
+ with_label_3d=False,
+ with_mask_3d=False,
+ with_seg_3d=True),
+ dict(
+ type='PointSegClassMapping',
+ valid_cat_ids=tuple(range(len(class_names))),
+ max_cat_id=13),
+ dict(
+ type='IndoorPatchPointSample',
+ num_points=num_points,
+ block_size=1.0,
+ ignore_index=None,
+ use_normalized_coord=True,
+ enlarge_size=None,
+ min_unique_num=num_points // 4,
+ eps=0.0),
+ dict(type='NormalizePointsColor', color_mean=None),
+ dict(
+ type='GlobalRotScaleTrans',
+ rot_range=[-3.141592653589793, 3.141592653589793], # [-pi, pi]
+ scale_ratio_range=[0.8, 1.2],
+ translation_std=[0, 0, 0]),
+ dict(
+ type='RandomJitterPoints',
+ jitter_std=[0.01, 0.01, 0.01],
+ clip_range=[-0.05, 0.05]),
+ dict(type='RandomDropPointsColor', drop_ratio=0.2),
+ dict(type='DefaultFormatBundle3D', class_names=class_names),
+ dict(type='Collect3D', keys=['points', 'pts_semantic_mask'])
+]
+```
+
+- `PointSegClassMapping`: Only the valid category ids will be mapped to class label ids like \[0, 13) during training. Other class ids will be converted to `ignore_index` which equals to `13`.
+- `IndoorPatchPointSample`: Crop a patch containing a fixed number of points from input point cloud. `block_size` indicates the size of the cropped block, typically `1.0` for S3DIS.
+- `NormalizePointsColor`: Normalize the RGB color values of input point cloud by dividing `255`.
+- Data augmentation:
+ - `GlobalRotScaleTrans`: randomly rotate and scale input point cloud.
+ - `RandomJitterPoints`: randomly jitter point cloud by adding different noise vector to each point.
+ - `RandomDropPointsColor`: set the colors of point cloud to all zeros by a probability `drop_ratio`.
+
+## Metrics
+
+Typically mean intersection over union (mIoU) is used for evaluation on S3DIS. In detail, we first compute IoU for multiple classes and then average them to get mIoU, please refer to [seg_eval.py](https://github.com/open-mmlab/mmdetection3d/blob/master/mmdet3d/core/evaluation/seg_eval.py).
+
+As introduced in section `Export S3DIS data`, S3DIS trains on 5 areas and evaluates on the remaining 1 area. But there are also other area split schemes in different papers.
+To enable flexible combination of train-val splits, we use sub-dataset to represent one area, and concatenate them to form a larger training set. An example of training on area 1, 2, 3, 4, 6 and evaluating on area 5 is shown as below:
+
+```python
+dataset_type = 'S3DISSegDataset'
+data_root = './data/s3dis/'
+class_names = ('ceiling', 'floor', 'wall', 'beam', 'column', 'window', 'door',
+ 'table', 'chair', 'sofa', 'bookcase', 'board', 'clutter')
+train_area = [1, 2, 3, 4, 6]
+test_area = 5
+data = dict(
+ train=dict(
+ type=dataset_type,
+ data_root=data_root,
+ ann_files=[
+ data_root + f's3dis_infos_Area_{i}.pkl' for i in train_area
+ ],
+ pipeline=train_pipeline,
+ classes=class_names,
+ test_mode=False,
+ ignore_index=len(class_names),
+ scene_idxs=[
+ data_root + f'seg_info/Area_{i}_resampled_scene_idxs.npy'
+ for i in train_area
+ ]),
+ val=dict(
+ type=dataset_type,
+ data_root=data_root,
+ ann_files=data_root + f's3dis_infos_Area_{test_area}.pkl',
+ pipeline=test_pipeline,
+ classes=class_names,
+ test_mode=True,
+ ignore_index=len(class_names),
+ scene_idxs=data_root +
+ f'seg_info/Area_{test_area}_resampled_scene_idxs.npy'))
+```
+
+where we specify the areas used for training/validation by setting `ann_files` and `scene_idxs` with lists that include corresponding paths. The train-val split can be simply modified via changing the `train_area` and `test_area` variables.
diff --git a/docs/en/datasets/scannet_det.md b/docs/en/datasets/scannet_det.md
new file mode 100644
index 0000000..540c8ca
--- /dev/null
+++ b/docs/en/datasets/scannet_det.md
@@ -0,0 +1,303 @@
+# ScanNet for 3D Object Detection
+
+## Dataset preparation
+
+For the overall process, please refer to the [README](https://github.com/open-mmlab/mmdetection3d/blob/master/data/scannet/README.md/) page for ScanNet.
+
+### Export ScanNet point cloud data
+
+By exporting ScanNet data, we load the raw point cloud data and generate the relevant annotations including semantic labels, instance labels and ground truth bounding boxes.
+
+```shell
+python batch_load_scannet_data.py
+```
+
+The directory structure before data preparation should be as below
+
+```
+mmdetection3d
+├── mmdet3d
+├── tools
+├── configs
+├── data
+│ ├── scannet
+│ │ ├── meta_data
+│ │ ├── scans
+│ │ │ ├── scenexxxx_xx
+│ │ ├── batch_load_scannet_data.py
+│ │ ├── load_scannet_data.py
+│ │ ├── scannet_utils.py
+│ │ ├── README.md
+```
+
+Under folder `scans` there are overall 1201 train and 312 validation folders in which raw point cloud data and relevant annotations are saved. For instance, under folder `scene0001_01` the files are as below:
+
+- `scene0001_01_vh_clean_2.ply`: Mesh file storing coordinates and colors of each vertex. The mesh's vertices are taken as raw point cloud data.
+- `scene0001_01.aggregation.json`: Aggregation file including object ID, segments ID and label.
+- `scene0001_01_vh_clean_2.0.010000.segs.json`: Segmentation file including segments ID and vertex.
+- `scene0001_01.txt`: Meta file including axis-aligned matrix, etc.
+- `scene0001_01_vh_clean_2.labels.ply`: Annotation file containing the category of each vertex.
+
+Export ScanNet data by running `python batch_load_scannet_data.py`. The main steps include:
+
+- Export original files to point cloud, instance label, semantic label and bounding box file.
+- Downsample raw point cloud and filter invalid classes.
+- Save point cloud data and relevant annotation files.
+
+And the core function `export` in `load_scannet_data.py` is as follows:
+
+```python
+def export(mesh_file,
+ agg_file,
+ seg_file,
+ meta_file,
+ label_map_file,
+ output_file=None,
+ test_mode=False):
+
+ # label map file: ./data/scannet/meta_data/scannetv2-labels.combined.tsv
+ # the various label standards in the label map file, e.g. 'nyu40id'
+ label_map = scannet_utils.read_label_mapping(
+ label_map_file, label_from='raw_category', label_to='nyu40id')
+ # load raw point cloud data, 6-dims feature: XYZRGB
+ mesh_vertices = scannet_utils.read_mesh_vertices_rgb(mesh_file)
+
+ # Load scene axis alignment matrix: a 4x4 transformation matrix
+ # transform raw points in sensor coordinate system to a coordinate system
+ # which is axis-aligned with the length/width of the room
+ lines = open(meta_file).readlines()
+ # test set data doesn't have align_matrix
+ axis_align_matrix = np.eye(4)
+ for line in lines:
+ if 'axisAlignment' in line:
+ axis_align_matrix = [
+ float(x)
+ for x in line.rstrip().strip('axisAlignment = ').split(' ')
+ ]
+ break
+ axis_align_matrix = np.array(axis_align_matrix).reshape((4, 4))
+
+ # perform global alignment of mesh vertices
+ pts = np.ones((mesh_vertices.shape[0], 4))
+ # raw point cloud in homogeneous coordinates, each row: [x, y, z, 1]
+ pts[:, 0:3] = mesh_vertices[:, 0:3]
+ # transform raw mesh vertices to aligned mesh vertices
+ pts = np.dot(pts, axis_align_matrix.transpose()) # Nx4
+ aligned_mesh_vertices = np.concatenate([pts[:, 0:3], mesh_vertices[:, 3:]],
+ axis=1)
+
+ # Load semantic and instance labels
+ if not test_mode:
+ # each object has one semantic label and consists of several segments
+ object_id_to_segs, label_to_segs = read_aggregation(agg_file)
+ # many points may belong to the same segment
+ seg_to_verts, num_verts = read_segmentation(seg_file)
+ label_ids = np.zeros(shape=(num_verts), dtype=np.uint32)
+ object_id_to_label_id = {}
+ for label, segs in label_to_segs.items():
+ label_id = label_map[label]
+ for seg in segs:
+ verts = seg_to_verts[seg]
+ # each point has one semantic label
+ label_ids[verts] = label_id
+ instance_ids = np.zeros(
+ shape=(num_verts), dtype=np.uint32) # 0: unannotated
+ for object_id, segs in object_id_to_segs.items():
+ for seg in segs:
+ verts = seg_to_verts[seg]
+ # object_id is 1-indexed, i.e. 1,2,3,.,,,.NUM_INSTANCES
+ # each point belongs to one object
+ instance_ids[verts] = object_id
+ if object_id not in object_id_to_label_id:
+ object_id_to_label_id[object_id] = label_ids[verts][0]
+ # bbox format is [x, y, z, x_size, y_size, z_size, label_id]
+ # [x, y, z] is gravity center of bbox, [x_size, y_size, z_size] is axis-aligned
+ # [label_id] is semantic label id in 'nyu40id' standard
+ # Note: since 3D bbox is axis-aligned, the yaw is 0.
+ unaligned_bboxes = extract_bbox(mesh_vertices, object_id_to_segs,
+ object_id_to_label_id, instance_ids)
+ aligned_bboxes = extract_bbox(aligned_mesh_vertices, object_id_to_segs,
+ object_id_to_label_id, instance_ids)
+ ...
+
+ return mesh_vertices, label_ids, instance_ids, unaligned_bboxes, \
+ aligned_bboxes, object_id_to_label_id, axis_align_matrix
+
+```
+
+After exporting each scan, the raw point cloud could be downsampled, e.g. to 50000, if the number of points is too large (the raw point cloud won't be downsampled if it's also used in 3D semantic segmentation task). In addition, invalid semantic labels outside of `nyu40id` standard or optional `DONOT CARE` classes should be filtered. Finally, the point cloud data, semantic labels, instance labels and ground truth bounding boxes should be saved in `.npy` files.
+
+### Export ScanNet RGB data (optional)
+
+By exporting ScanNet RGB data, for each scene we load a set of RGB images with corresponding 4x4 pose matrices, and a single 4x4 camera intrinsic matrix. Note, that this step is optional and can be skipped if multi-view detection is not planned to use.
+
+```shell
+python extract_posed_images.py
+```
+
+Each of 1201 train, 312 validation and 100 test scenes contains a single `.sens` file. For instance, for scene `0001_01` we have `data/scannet/scans/scene0001_01/0001_01.sens`. For this scene all images and poses are extracted to `data/scannet/posed_images/scene0001_01`. Specifically, there will be 300 image files xxxxx.jpg, 300 camera pose files xxxxx.txt and a single `intrinsic.txt` file. Typically, single scene contains several thousand images. By default, we extract only 300 of them with resulting space occupation of \<100 Gb. To extract more images, use `--max-images-per-scene` parameter.
+
+### Create dataset
+
+```shell
+python tools/create_data.py scannet --root-path ./data/scannet \
+--out-dir ./data/scannet --extra-tag scannet
+```
+
+The above exported point cloud file, semantic label file and instance label file are further saved in `.bin` format. Meanwhile `.pkl` info files are also generated for train or validation. The core function `process_single_scene` of getting data infos is as follows.
+
+```python
+def process_single_scene(sample_idx):
+
+ # save point cloud, instance label and semantic label in .bin file respectively, get info['pts_path'], info['pts_instance_mask_path'] and info['pts_semantic_mask_path']
+ ...
+
+ # get annotations
+ if has_label:
+ annotations = {}
+ # box is of shape [k, 6 + class]
+ aligned_box_label = self.get_aligned_box_label(sample_idx)
+ unaligned_box_label = self.get_unaligned_box_label(sample_idx)
+ annotations['gt_num'] = aligned_box_label.shape[0]
+ if annotations['gt_num'] != 0:
+ aligned_box = aligned_box_label[:, :-1] # k, 6
+ unaligned_box = unaligned_box_label[:, :-1]
+ classes = aligned_box_label[:, -1] # k
+ annotations['name'] = np.array([
+ self.label2cat[self.cat_ids2class[classes[i]]]
+ for i in range(annotations['gt_num'])
+ ])
+ # default names are given to aligned bbox for compatibility
+ # we also save unaligned bbox info with marked names
+ annotations['location'] = aligned_box[:, :3]
+ annotations['dimensions'] = aligned_box[:, 3:6]
+ annotations['gt_boxes_upright_depth'] = aligned_box
+ annotations['unaligned_location'] = unaligned_box[:, :3]
+ annotations['unaligned_dimensions'] = unaligned_box[:, 3:6]
+ annotations[
+ 'unaligned_gt_boxes_upright_depth'] = unaligned_box
+ annotations['index'] = np.arange(
+ annotations['gt_num'], dtype=np.int32)
+ annotations['class'] = np.array([
+ self.cat_ids2class[classes[i]]
+ for i in range(annotations['gt_num'])
+ ])
+ axis_align_matrix = self.get_axis_align_matrix(sample_idx)
+ annotations['axis_align_matrix'] = axis_align_matrix # 4x4
+ info['annos'] = annotations
+ return info
+```
+
+The directory structure after process should be as below
+
+```
+scannet
+├── meta_data
+├── batch_load_scannet_data.py
+├── load_scannet_data.py
+├── scannet_utils.py
+├── README.md
+├── scans
+├── scans_test
+├── scannet_instance_data
+├── points
+│ ├── xxxxx.bin
+├── instance_mask
+│ ├── xxxxx.bin
+├── semantic_mask
+│ ├── xxxxx.bin
+├── seg_info
+│ ├── train_label_weight.npy
+│ ├── train_resampled_scene_idxs.npy
+│ ├── val_label_weight.npy
+│ ├── val_resampled_scene_idxs.npy
+├── posed_images
+│ ├── scenexxxx_xx
+│ │ ├── xxxxxx.txt
+│ │ ├── xxxxxx.jpg
+│ │ ├── intrinsic.txt
+├── scannet_infos_train.pkl
+├── scannet_infos_val.pkl
+├── scannet_infos_test.pkl
+```
+
+- `points/xxxxx.bin`: The `axis-unaligned` point cloud data after downsample. Since ScanNet 3D detection task takes axis-aligned point clouds as input, while ScanNet 3D semantic segmentation task takes unaligned points, we choose to store unaligned points and their axis-align transform matrix. Note: the points would be axis-aligned in pre-processing pipeline [`GlobalAlignment`](https://github.com/open-mmlab/mmdetection3d/blob/9f0b01caf6aefed861ef4c3eb197c09362d26b32/mmdet3d/datasets/pipelines/transforms_3d.py#L423) of 3D detection task.
+- `instance_mask/xxxxx.bin`: The instance label for each point, value range: \[0, NUM_INSTANCES\], 0: unannotated.
+- `semantic_mask/xxxxx.bin`: The semantic label for each point, value range: \[1, 40\], i.e. `nyu40id` standard. Note: the `nyu40id` ID will be mapped to train ID in train pipeline `PointSegClassMapping`.
+- `posed_images/scenexxxx_xx`: The set of `.jpg` images with `.txt` 4x4 poses and the single `.txt` file with camera intrinsic matrix.
+- `scannet_infos_train.pkl`: The train data infos, the detailed info of each scan is as follows:
+ - info\['point_cloud'\]: {'num_features': 6, 'lidar_idx': sample_idx}.
+ - info\['pts_path'\]: The path of `points/xxxxx.bin`.
+ - info\['pts_instance_mask_path'\]: The path of `instance_mask/xxxxx.bin`.
+ - info\['pts_semantic_mask_path'\]: The path of `semantic_mask/xxxxx.bin`.
+ - info\['annos'\]: The annotations of each scan.
+ - annotations\['gt_num'\]: The number of ground truths.
+ - annotations\['name'\]: The semantic name of all ground truths, e.g. `chair`.
+ - annotations\['location'\]: The gravity center of the axis-aligned 3D bounding boxes in depth coordinate system. Shape: \[K, 3\], K is the number of ground truths.
+ - annotations\['dimensions'\]: The dimensions of the axis-aligned 3D bounding boxes in depth coordinate system, i.e. (x_size, y_size, z_size), shape: \[K, 3\].
+ - annotations\['gt_boxes_upright_depth'\]: The axis-aligned 3D bounding boxes in depth coordinate system, each bounding box is (x, y, z, x_size, y_size, z_size), shape: \[K, 6\].
+ - annotations\['unaligned_location'\]: The gravity center of the axis-unaligned 3D bounding boxes in depth coordinate system.
+ - annotations\['unaligned_dimensions'\]: The dimensions of the axis-unaligned 3D bounding boxes in depth coordinate system.
+ - annotations\['unaligned_gt_boxes_upright_depth'\]: The axis-unaligned 3D bounding boxes in depth coordinate system.
+ - annotations\['index'\]: The index of all ground truths, i.e. \[0, K).
+ - annotations\['class'\]: The train class ID of the bounding boxes, value range: \[0, 18), shape: \[K, \].
+- `scannet_infos_val.pkl`: The val data infos, which shares the same format as `scannet_infos_train.pkl`.
+- `scannet_infos_test.pkl`: The test data infos, which almost shares the same format as `scannet_infos_train.pkl` except for the lack of annotation.
+
+## Training pipeline
+
+A typical training pipeline of ScanNet for 3D detection is as follows.
+
+```python
+train_pipeline = [
+ dict(
+ type='LoadPointsFromFile',
+ coord_type='DEPTH',
+ shift_height=True,
+ load_dim=6,
+ use_dim=[0, 1, 2]),
+ dict(
+ type='LoadAnnotations3D',
+ with_bbox_3d=True,
+ with_label_3d=True,
+ with_mask_3d=True,
+ with_seg_3d=True),
+ dict(type='GlobalAlignment', rotation_axis=2),
+ dict(
+ type='PointSegClassMapping',
+ valid_cat_ids=(3, 4, 5, 6, 7, 8, 9, 10, 11, 12, 14, 16, 24, 28, 33, 34,
+ 36, 39),
+ max_cat_id=40),
+ dict(type='PointSample', num_points=40000),
+ dict(
+ type='RandomFlip3D',
+ sync_2d=False,
+ flip_ratio_bev_horizontal=0.5,
+ flip_ratio_bev_vertical=0.5),
+ dict(
+ type='GlobalRotScaleTrans',
+ rot_range=[-0.087266, 0.087266],
+ scale_ratio_range=[1.0, 1.0],
+ shift_height=True),
+ dict(type='DefaultFormatBundle3D', class_names=class_names),
+ dict(
+ type='Collect3D',
+ keys=[
+ 'points', 'gt_bboxes_3d', 'gt_labels_3d', 'pts_semantic_mask',
+ 'pts_instance_mask'
+ ])
+]
+```
+
+- `GlobalAlignment`: The previous point cloud would be axis-aligned using the axis-aligned matrix.
+- `PointSegClassMapping`: Only the valid category IDs will be mapped to class label IDs like \[0, 18) during training.
+- Data augmentation:
+ - `PointSample`: downsample the input point cloud.
+ - `RandomFlip3D`: randomly flip the input point cloud horizontally or vertically.
+ - `GlobalRotScaleTrans`: rotate the input point cloud, usually in the range of \[-5, 5\] (degrees) for ScanNet; then scale the input point cloud, usually by 1.0 for ScanNet (which means no scaling); finally translate the input point cloud, usually by 0 for ScanNet (which means no translation).
+
+## Metrics
+
+Typically mean Average Precision (mAP) is used for evaluation on ScanNet, e.g. `mAP@0.25` and `mAP@0.5`. In detail, a generic function to compute precision and recall for 3D object detection for multiple classes is called, please refer to [indoor_eval](https://github.com/open-mmlab/mmdetection3d/blob/master/mmdet3D/core/evaluation/indoor_eval.py).
+
+As introduced in section `Export ScanNet data`, all ground truth 3D bounding box are axis-aligned, i.e. the yaw is zero. So the yaw target of network predicted 3D bounding box is also zero and axis-aligned 3D Non-Maximum Suppression (NMS), which is regardless of rotation, is adopted during post-processing .
diff --git a/docs/en/datasets/scannet_sem_seg.md b/docs/en/datasets/scannet_sem_seg.md
new file mode 100644
index 0000000..edb1394
--- /dev/null
+++ b/docs/en/datasets/scannet_sem_seg.md
@@ -0,0 +1,132 @@
+# ScanNet for 3D Semantic Segmentation
+
+## Dataset preparation
+
+The overall process is similar to ScanNet 3D detection task. Please refer to this [section](https://github.com/open-mmlab/mmdetection3d/blob/master/docs/en/datasets/scannet_det.md#dataset-preparation). Only a few differences and additional information about the 3D semantic segmentation data will be listed below.
+
+### Export ScanNet data
+
+Since ScanNet provides online benchmark for 3D semantic segmentation evaluation on the test set, we need to also download the test scans and put it under `scannet` folder.
+
+The directory structure before data preparation should be as below:
+
+```
+mmdetection3d
+├── mmdet3d
+├── tools
+├── configs
+├── data
+│ ├── scannet
+│ │ ├── meta_data
+│ │ ├── scans
+│ │ │ ├── scenexxxx_xx
+│ │ ├── scans_test
+│ │ │ ├── scenexxxx_xx
+│ │ ├── batch_load_scannet_data.py
+│ │ ├── load_scannet_data.py
+│ │ ├── scannet_utils.py
+│ │ ├── README.md
+```
+
+Under folder `scans_test` there are 100 test folders in which only raw point cloud data and its meta file are saved. For instance, under folder `scene0707_00` the files are as below:
+
+- `scene0707_00_vh_clean_2.ply`: Mesh file storing coordinates and colors of each vertex. The mesh's vertices are taken as raw point cloud data.
+- `scene0707_00.txt`: Meta file including sensor parameters, etc. Note: different from data under `scans`, axis-aligned matrix is not provided for test scans.
+
+Export ScanNet data by running `python batch_load_scannet_data.py`. Note: only point cloud data will be saved for test set scans because no annotations are provided.
+
+### Create dataset
+
+Similar to the 3D detection task, we create dataset by running `python tools/create_data.py scannet --root-path ./data/scannet --out-dir ./data/scannet --extra-tag scannet`.
+The directory structure after processing should be as below:
+
+```
+scannet
+├── scannet_utils.py
+├── batch_load_scannet_data.py
+├── load_scannet_data.py
+├── scannet_utils.py
+├── README.md
+├── scans
+├── scans_test
+├── scannet_instance_data
+├── points
+│ ├── xxxxx.bin
+├── instance_mask
+│ ├── xxxxx.bin
+├── semantic_mask
+│ ├── xxxxx.bin
+├── seg_info
+│ ├── train_label_weight.npy
+│ ├── train_resampled_scene_idxs.npy
+│ ├── val_label_weight.npy
+│ ├── val_resampled_scene_idxs.npy
+├── scannet_infos_train.pkl
+├── scannet_infos_val.pkl
+├── scannet_infos_test.pkl
+```
+
+- `seg_info`: The generated infos to support semantic segmentation model training.
+ - `train_label_weight.npy`: Weighting factor for each semantic class. Since the number of points in different classes varies greatly, it's a common practice to use label re-weighting to get a better performance.
+ - `train_resampled_scene_idxs.npy`: Re-sampling index for each scene. Different rooms will be sampled multiple times according to their number of points to balance training data.
+
+## Training pipeline
+
+A typical training pipeline of ScanNet for 3D semantic segmentation is as below:
+
+```python
+train_pipeline = [
+ dict(
+ type='LoadPointsFromFile',
+ coord_type='DEPTH',
+ shift_height=False,
+ use_color=True,
+ load_dim=6,
+ use_dim=[0, 1, 2, 3, 4, 5]),
+ dict(
+ type='LoadAnnotations3D',
+ with_bbox_3d=False,
+ with_label_3d=False,
+ with_mask_3d=False,
+ with_seg_3d=True),
+ dict(
+ type='PointSegClassMapping',
+ valid_cat_ids=(1, 2, 3, 4, 5, 6, 7, 8, 9, 10, 11, 12, 14, 16, 24, 28,
+ 33, 34, 36, 39),
+ max_cat_id=40),
+ dict(
+ type='IndoorPatchPointSample',
+ num_points=num_points,
+ block_size=1.5,
+ ignore_index=len(class_names),
+ use_normalized_coord=False,
+ enlarge_size=0.2,
+ min_unique_num=None),
+ dict(type='NormalizePointsColor', color_mean=None),
+ dict(type='DefaultFormatBundle3D', class_names=class_names),
+ dict(type='Collect3D', keys=['points', 'pts_semantic_mask'])
+]
+```
+
+- `PointSegClassMapping`: Only the valid category ids will be mapped to class label ids like \[0, 20) during training. Other class ids will be converted to `ignore_index` which equals to `20`.
+- `IndoorPatchPointSample`: Crop a patch containing a fixed number of points from input point cloud. `block_size` indicates the size of the cropped block, typically `1.5` for ScanNet.
+- `NormalizePointsColor`: Normalize the RGB color values of input point cloud by dividing `255`.
+
+## Metrics
+
+Typically mean Intersection over Union (mIoU) is used for evaluation on ScanNet. In detail, we first compute IoU for multiple classes and then average them to get mIoU, please refer to [seg_eval](https://github.com/open-mmlab/mmdetection3d/blob/master/mmdet3d/core/evaluation/seg_eval.py).
+
+## Testing and Making a Submission
+
+By default, our codebase evaluates semantic segmentation results on the validation set.
+If you would like to test the model performance on the online benchmark, add `--format-only` flag in the evaluation script and change `ann_file=data_root + 'scannet_infos_val.pkl'` to `ann_file=data_root + 'scannet_infos_test.pkl'` in the ScanNet dataset's [config](https://github.com/open-mmlab/mmdetection3d/blob/master/configs/_base_/datasets/scannet_seg-3d-20class.py#L126). Remember to specify the `txt_prefix` as the directory to save the testing results.
+
+Taking PointNet++ (SSG) on ScanNet for example, the following command can be used to do inference on test set:
+
+```
+./tools/dist_test.sh configs/pointnet2/pointnet2_ssg_16x2_cosine_200e_scannet_seg-3d-20class.py \
+ work_dirs/pointnet2_ssg/latest.pth --format-only \
+ --eval-options txt_prefix=work_dirs/pointnet2_ssg/test_submission
+```
+
+After generating the results, you can basically compress the folder and upload to the [ScanNet evaluation server](http://kaldir.vc.in.tum.de/scannet_benchmark/semantic_label_3d).
diff --git a/docs/en/datasets/sunrgbd_det.md b/docs/en/datasets/sunrgbd_det.md
new file mode 100644
index 0000000..16aa914
--- /dev/null
+++ b/docs/en/datasets/sunrgbd_det.md
@@ -0,0 +1,347 @@
+# SUN RGB-D for 3D Object Detection
+
+## Dataset preparation
+
+For the overall process, please refer to the [README](https://github.com/open-mmlab/mmdetection3d/blob/master/data/sunrgbd/README.md/) page for SUN RGB-D.
+
+### Download SUN RGB-D data and toolbox
+
+Download SUNRGBD data [HERE](http://rgbd.cs.princeton.edu/data/). Then, move `SUNRGBD.zip`, `SUNRGBDMeta2DBB_v2.mat`, `SUNRGBDMeta3DBB_v2.mat` and `SUNRGBDtoolbox.zip` to the `OFFICIAL_SUNRGBD` folder, unzip the zip files.
+
+The directory structure before data preparation should be as below:
+
+```
+sunrgbd
+├── README.md
+├── matlab
+│ ├── extract_rgbd_data_v1.m
+│ ├── extract_rgbd_data_v2.m
+│ ├── extract_split.m
+├── OFFICIAL_SUNRGBD
+│ ├── SUNRGBD
+│ ├── SUNRGBDMeta2DBB_v2.mat
+│ ├── SUNRGBDMeta3DBB_v2.mat
+│ ├── SUNRGBDtoolbox
+```
+
+### Extract data and annotations for 3D detection from raw data
+
+Extract SUN RGB-D annotation data from raw annotation data by running (this requires MATLAB installed on your machine):
+
+```bash
+matlab -nosplash -nodesktop -r 'extract_split;quit;'
+matlab -nosplash -nodesktop -r 'extract_rgbd_data_v2;quit;'
+matlab -nosplash -nodesktop -r 'extract_rgbd_data_v1;quit;'
+```
+
+The main steps include:
+
+- Extract train and val split.
+- Extract data for 3D detection from raw data.
+- Extract and format detection annotation from raw data.
+
+The main component of `extract_rgbd_data_v2.m` which extracts point cloud data from depth map is as follows:
+
+```matlab
+data = SUNRGBDMeta(imageId);
+data.depthpath(1:16) = '';
+data.depthpath = strcat('../OFFICIAL_SUNRGBD', data.depthpath);
+data.rgbpath(1:16) = '';
+data.rgbpath = strcat('../OFFICIAL_SUNRGBD', data.rgbpath);
+
+% extract point cloud from depth map
+[rgb,points3d,depthInpaint,imsize]=read3dPoints(data);
+rgb(isnan(points3d(:,1)),:) = [];
+points3d(isnan(points3d(:,1)),:) = [];
+points3d_rgb = [points3d, rgb];
+
+% MAT files are 3x smaller than TXT files. In Python we can use
+% scipy.io.loadmat('xxx.mat')['points3d_rgb'] to load the data.
+mat_filename = strcat(num2str(imageId,'%06d'), '.mat');
+txt_filename = strcat(num2str(imageId,'%06d'), '.txt');
+% save point cloud data
+parsave(strcat(depth_folder, mat_filename), points3d_rgb);
+```
+
+The main component of `extract_rgbd_data_v1.m` which extracts annotation is as follows:
+
+```matlab
+% Write 2D and 3D box label
+data2d = data;
+fid = fopen(strcat(det_label_folder, txt_filename), 'w');
+for j = 1:length(data.groundtruth3DBB)
+ centroid = data.groundtruth3DBB(j).centroid; % 3D bbox center
+ classname = data.groundtruth3DBB(j).classname; % class name
+ orientation = data.groundtruth3DBB(j).orientation; % 3D bbox orientation
+ coeffs = abs(data.groundtruth3DBB(j).coeffs); % 3D bbox size
+ box2d = data2d.groundtruth2DBB(j).gtBb2D; % 2D bbox
+ fprintf(fid, '%s %d %d %d %d %f %f %f %f %f %f %f %f\n', classname, box2d(1), box2d(2), box2d(3), box2d(4), centroid(1), centroid(2), centroid(3), coeffs(1), coeffs(2), coeffs(3), orientation(1), orientation(2));
+end
+fclose(fid);
+```
+
+The above two scripts call functions such as `read3dPoints` from the [toolbox](https://rgbd.cs.princeton.edu/data/SUNRGBDtoolbox.zip) provided by SUN RGB-D.
+
+The directory structure after extraction should be as follows.
+
+```
+sunrgbd
+├── README.md
+├── matlab
+│ ├── extract_rgbd_data_v1.m
+│ ├── extract_rgbd_data_v2.m
+│ ├── extract_split.m
+├── OFFICIAL_SUNRGBD
+│ ├── SUNRGBD
+│ ├── SUNRGBDMeta2DBB_v2.mat
+│ ├── SUNRGBDMeta3DBB_v2.mat
+│ ├── SUNRGBDtoolbox
+├── sunrgbd_trainval
+│ ├── calib
+│ ├── depth
+│ ├── image
+│ ├── label
+│ ├── label_v1
+│ ├── seg_label
+│ ├── train_data_idx.txt
+│ ├── val_data_idx.txt
+```
+
+Under each following folder there are overall 5285 train files and 5050 val files:
+
+- `calib`: Camera calibration information in `.txt`
+- `depth`: Point cloud saved in `.mat` (xyz+rgb)
+- `image`: Image data in `.jpg`
+- `label`: Detection annotation data in `.txt` (version 2)
+- `label_v1`: Detection annotation data in `.txt` (version 1)
+- `seg_label`: Segmentation annotation data in `.txt`
+
+Currently, we use v1 data for training and testing, so the version 2 labels are unused.
+
+### Create dataset
+
+Please run the command below to create the dataset.
+
+```shell
+python tools/create_data.py sunrgbd --root-path ./data/sunrgbd \
+--out-dir ./data/sunrgbd --extra-tag sunrgbd
+```
+
+or (if in a slurm environment)
+
+```
+bash tools/create_data.sh sunrgbd
+```
+
+The above point cloud data are further saved in `.bin` format. Meanwhile `.pkl` info files are also generated for saving annotation and metadata. The core function `process_single_scene` of getting data infos is as follows.
+
+```python
+def process_single_scene(sample_idx):
+ print(f'{self.split} sample_idx: {sample_idx}')
+ # convert depth to points
+ # and downsample the points
+ SAMPLE_NUM = 50000
+ pc_upright_depth = self.get_depth(sample_idx)
+ pc_upright_depth_subsampled = random_sampling(
+ pc_upright_depth, SAMPLE_NUM)
+
+ info = dict()
+ pc_info = {'num_features': 6, 'lidar_idx': sample_idx}
+ info['point_cloud'] = pc_info
+
+ # save point cloud data in `.bin` format
+ mmcv.mkdir_or_exist(osp.join(self.root_dir, 'points'))
+ pc_upright_depth_subsampled.tofile(
+ osp.join(self.root_dir, 'points', f'{sample_idx:06d}.bin'))
+
+ # save point cloud file path
+ info['pts_path'] = osp.join('points', f'{sample_idx:06d}.bin')
+
+ # save image file path and metainfo
+ img_path = osp.join('image', f'{sample_idx:06d}.jpg')
+ image_info = {
+ 'image_idx': sample_idx,
+ 'image_shape': self.get_image_shape(sample_idx),
+ 'image_path': img_path
+ }
+ info['image'] = image_info
+
+ # save calibration information
+ K, Rt = self.get_calibration(sample_idx)
+ calib_info = {'K': K, 'Rt': Rt}
+ info['calib'] = calib_info
+
+ # save all annotation
+ if has_label:
+ obj_list = self.get_label_objects(sample_idx)
+ annotations = {}
+ annotations['gt_num'] = len([
+ obj.classname for obj in obj_list
+ if obj.classname in self.cat2label.keys()
+ ])
+ if annotations['gt_num'] != 0:
+ # class name
+ annotations['name'] = np.array([
+ obj.classname for obj in obj_list
+ if obj.classname in self.cat2label.keys()
+ ])
+ # 2D image bounding boxes
+ annotations['bbox'] = np.concatenate([
+ obj.box2d.reshape(1, 4) for obj in obj_list
+ if obj.classname in self.cat2label.keys()
+ ], axis=0)
+ # 3D bounding box center location (in depth coordinate system)
+ annotations['location'] = np.concatenate([
+ obj.centroid.reshape(1, 3) for obj in obj_list
+ if obj.classname in self.cat2label.keys()
+ ], axis=0)
+ # 3D bounding box dimension/size (in depth coordinate system)
+ annotations['dimensions'] = 2 * np.array([
+ [obj.l, obj.h, obj.w] for obj in obj_list
+ if obj.classname in self.cat2label.keys()
+ ])
+ # 3D bounding box rotation angle/yaw angle (in depth coordinate system)
+ annotations['rotation_y'] = np.array([
+ obj.heading_angle for obj in obj_list
+ if obj.classname in self.cat2label.keys()
+ ])
+ annotations['index'] = np.arange(
+ len(obj_list), dtype=np.int32)
+ # class label (number)
+ annotations['class'] = np.array([
+ self.cat2label[obj.classname] for obj in obj_list
+ if obj.classname in self.cat2label.keys()
+ ])
+ # 3D bounding box (in depth coordinate system)
+ annotations['gt_boxes_upright_depth'] = np.stack(
+ [
+ obj.box3d for obj in obj_list
+ if obj.classname in self.cat2label.keys()
+ ], axis=0) # (K,8)
+ info['annos'] = annotations
+ return info
+```
+
+The directory structure after processing should be as follows.
+
+```
+sunrgbd
+├── README.md
+├── matlab
+│ ├── ...
+├── OFFICIAL_SUNRGBD
+│ ├── ...
+├── sunrgbd_trainval
+│ ├── ...
+├── points
+├── sunrgbd_infos_train.pkl
+├── sunrgbd_infos_val.pkl
+```
+
+- `points/0xxxxx.bin`: The point cloud data after downsample.
+- `sunrgbd_infos_train.pkl`: The train data infos, the detailed info of each scene is as follows:
+ - info\['point_cloud'\]: `·`{'num_features': 6, 'lidar_idx': sample_idx}`, where `sample_idx\` is the index of the scene.
+ - info\['pts_path'\]: The path of `points/0xxxxx.bin`.
+ - info\['image'\]: The image path and metainfo:
+ - image\['image_idx'\]: The index of the image.
+ - image\['image_shape'\]: The shape of the image tensor.
+ - image\['image_path'\]: The path of the image.
+ - info\['annos'\]: The annotations of each scene.
+ - annotations\['gt_num'\]: The number of ground truths.
+ - annotations\['name'\]: The semantic name of all ground truths, e.g. `chair`.
+ - annotations\['location'\]: The gravity center of the 3D bounding boxes in depth coordinate system. Shape: \[K, 3\], K is the number of ground truths.
+ - annotations\['dimensions'\]: The dimensions of the 3D bounding boxes in depth coordinate system, i.e. `(x_size, y_size, z_size)`, shape: \[K, 3\].
+ - annotations\['rotation_y'\]: The yaw angle of the 3D bounding boxes in depth coordinate system. Shape: \[K, \].
+ - annotations\['gt_boxes_upright_depth'\]: The 3D bounding boxes in depth coordinate system, each bounding box is `(x, y, z, x_size, y_size, z_size, yaw)`, shape: \[K, 7\].
+ - annotations\['bbox'\]: The 2D bounding boxes, each bounding box is `(x, y, x_size, y_size)`, shape: \[K, 4\].
+ - annotations\['index'\]: The index of all ground truths, range \[0, K).
+ - annotations\['class'\]: The train class id of the bounding boxes, value range: \[0, 10), shape: \[K, \].
+- `sunrgbd_infos_val.pkl`: The val data infos, which shares the same format as `sunrgbd_infos_train.pkl`.
+
+## Train pipeline
+
+A typical train pipeline of SUN RGB-D for point cloud only 3D detection is as follows.
+
+```python
+train_pipeline = [
+ dict(
+ type='LoadPointsFromFile',
+ coord_type='DEPTH',
+ shift_height=True,
+ load_dim=6,
+ use_dim=[0, 1, 2]),
+ dict(type='LoadAnnotations3D'),
+ dict(
+ type='RandomFlip3D',
+ sync_2d=False,
+ flip_ratio_bev_horizontal=0.5,
+ ),
+ dict(
+ type='GlobalRotScaleTrans',
+ rot_range=[-0.523599, 0.523599],
+ scale_ratio_range=[0.85, 1.15],
+ shift_height=True),
+ dict(type='PointSample', num_points=20000),
+ dict(type='DefaultFormatBundle3D', class_names=class_names),
+ dict(type='Collect3D', keys=['points', 'gt_bboxes_3d', 'gt_labels_3d'])
+]
+```
+
+Data augmentation for point clouds:
+
+- `RandomFlip3D`: randomly flip the input point cloud horizontally or vertically.
+- `GlobalRotScaleTrans`: rotate the input point cloud, usually in the range of \[-30, 30\] (degrees) for SUN RGB-D; then scale the input point cloud, usually in the range of \[0.85, 1.15\] for SUN RGB-D; finally translate the input point cloud, usually by 0 for SUN RGB-D (which means no translation).
+- `PointSample`: downsample the input point cloud.
+
+A typical train pipeline of SUN RGB-D for multi-modality (point cloud and image) 3D detection is as follows.
+
+```python
+train_pipeline = [
+ dict(
+ type='LoadPointsFromFile',
+ coord_type='DEPTH',
+ shift_height=True,
+ load_dim=6,
+ use_dim=[0, 1, 2]),
+ dict(type='LoadImageFromFile'),
+ dict(type='LoadAnnotations3D'),
+ dict(type='LoadAnnotations', with_bbox=True),
+ dict(type='Resize', img_scale=(1333, 600), keep_ratio=True),
+ dict(type='RandomFlip', flip_ratio=0.0),
+ dict(type='Normalize', **img_norm_cfg),
+ dict(type='Pad', size_divisor=32),
+ dict(
+ type='RandomFlip3D',
+ sync_2d=False,
+ flip_ratio_bev_horizontal=0.5,
+ ),
+ dict(
+ type='GlobalRotScaleTrans',
+ rot_range=[-0.523599, 0.523599],
+ scale_ratio_range=[0.85, 1.15],
+ shift_height=True),
+ dict(type='PointSample', num_points=20000),
+ dict(type='DefaultFormatBundle3D', class_names=class_names),
+ dict(
+ type='Collect3D',
+ keys=[
+ 'img', 'gt_bboxes', 'gt_labels', 'points', 'gt_bboxes_3d',
+ 'gt_labels_3d'
+ ])
+]
+```
+
+Data augmentation/normalization for images:
+
+- `Resize`: resize the input image, `keep_ratio=True` means the ratio of the image is kept unchanged.
+- `Normalize`: normalize the RGB channels of the input image.
+- `RandomFlip`: randomly flip the input image.
+- `Pad`: pad the input image with zeros by default.
+
+The image augmentation and normalization functions are implemented in [MMDetection](https://github.com/open-mmlab/mmdetection/tree/master/mmdet/datasets/pipelines).
+
+## Metrics
+
+Same as ScanNet, typically mean Average Precision (mAP) is used for evaluation on SUN RGB-D, e.g. `mAP@0.25` and `mAP@0.5`. In detail, a generic function to compute precision and recall for 3D object detection for multiple classes is called, please refer to [indoor_eval](https://github.com/open-mmlab/mmdetection3d/blob/master/mmdet3d/core/evaluation/indoor_eval.py).
+
+Since SUN RGB-D consists of image data, detection on image data is also feasible. For instance, in ImVoteNet, we first train an image detector, and we also use mAP for evaluation, e.g. `mAP@0.5`. We use the `eval_map` function from [MMDetection](https://github.com/open-mmlab/mmdetection) to calculate mAP.
diff --git a/docs/en/datasets/waymo_det.md b/docs/en/datasets/waymo_det.md
new file mode 100644
index 0000000..a1772c9
--- /dev/null
+++ b/docs/en/datasets/waymo_det.md
@@ -0,0 +1,175 @@
+# Waymo Dataset
+
+This page provides specific tutorials about the usage of MMDetection3D for Waymo dataset.
+
+## Prepare dataset
+
+Before preparing Waymo dataset, if you only installed requirements in `requirements/build.txt` and `requirements/runtime.txt` before, please install the official package for this dataset at first by running
+
+```
+# tf 2.1.0.
+pip install waymo-open-dataset-tf-2-1-0==1.2.0
+# tf 2.0.0
+# pip install waymo-open-dataset-tf-2-0-0==1.2.0
+# tf 1.15.0
+# pip install waymo-open-dataset-tf-1-15-0==1.2.0
+```
+
+or
+
+```
+pip install -r requirements/optional.txt
+```
+
+Like the general way to prepare dataset, it is recommended to symlink the dataset root to `$MMDETECTION3D/data`.
+Due to the original Waymo data format is based on `tfrecord`, we need to preprocess the raw data for convenient usage in the training and evaluation procedure. Our approach is to convert them into KITTI format.
+
+The folder structure should be organized as follows before our processing.
+
+```
+mmdetection3d
+├── mmdet3d
+├── tools
+├── configs
+├── data
+│ ├── waymo
+│ │ ├── waymo_format
+│ │ │ ├── training
+│ │ │ ├── validation
+│ │ │ ├── testing
+│ │ │ ├── gt.bin
+│ │ ├── kitti_format
+│ │ │ ├── ImageSets
+
+```
+
+You can download Waymo open dataset V1.2 [HERE](https://waymo.com/open/download/) and its data split [HERE](https://drive.google.com/drive/folders/18BVuF_RYJF0NjZpt8SnfzANiakoRMf0o?usp=sharing). Then put `tfrecord` files into corresponding folders in `data/waymo/waymo_format/` and put the data split txt files into `data/waymo/kitti_format/ImageSets`. Download ground truth bin files for validation set [HERE](https://console.cloud.google.com/storage/browser/waymo_open_dataset_v_1_2_0/validation/ground_truth_objects) and put it into `data/waymo/waymo_format/`. A tip is that you can use `gsutil` to download the large-scale dataset with commands. You can take this [tool](https://github.com/RalphMao/Waymo-Dataset-Tool) as an example for more details. Subsequently, prepare Waymo data by running
+
+```bash
+python tools/create_data.py waymo --root-path ./data/waymo/ --out-dir ./data/waymo/ --workers 128 --extra-tag waymo
+```
+
+Note that if your local disk does not have enough space for saving converted data, you can change the `--out-dir` to anywhere else. Just remember to create folders and prepare data there in advance and link them back to `data/waymo/kitti_format` after the data conversion.
+
+After the data conversion, the folder structure and info files should be organized as below.
+
+```
+mmdetection3d
+├── mmdet3d
+├── tools
+├── configs
+├── data
+│ ├── waymo
+│ │ ├── waymo_format
+│ │ │ ├── training
+│ │ │ ├── validation
+│ │ │ ├── testing
+│ │ │ ├── gt.bin
+│ │ ├── kitti_format
+│ │ │ ├── ImageSets
+│ │ │ ├── training
+│ │ │ │ ├── calib
+│ │ │ │ ├── image_0
+│ │ │ │ ├── image_1
+│ │ │ │ ├── image_2
+│ │ │ │ ├── image_3
+│ │ │ │ ├── image_4
+│ │ │ │ ├── label_0
+│ │ │ │ ├── label_1
+│ │ │ │ ├── label_2
+│ │ │ │ ├── label_3
+│ │ │ │ ├── label_4
+│ │ │ │ ├── label_all
+│ │ │ │ ├── pose
+│ │ │ │ ├── velodyne
+│ │ │ ├── testing
+│ │ │ │ ├── (the same as training)
+│ │ │ ├── waymo_gt_database
+│ │ │ ├── waymo_infos_trainval.pkl
+│ │ │ ├── waymo_infos_train.pkl
+│ │ │ ├── waymo_infos_val.pkl
+│ │ │ ├── waymo_infos_test.pkl
+│ │ │ ├── waymo_dbinfos_train.pkl
+
+```
+
+Here because there are several cameras, we store the corresponding image and labels that can be projected to that camera respectively and save pose for further usage of consecutive frames point clouds. We use a coding way `{a}{bbb}{ccc}` to name the data for each frame, where `a` is the prefix for different split (`0` for training, `1` for validation and `2` for testing), `bbb` for segment index and `ccc` for frame index. You can easily locate the required frame according to this naming rule. We gather the data for training and validation together as KITTI and store the indices for different set in the `ImageSet` files.
+
+## Training
+
+Considering there are many similar frames in the original dataset, we can basically use a subset to train our model primarily. In our preliminary baselines, we load one frame every five frames, and thanks to our hyper parameters settings and data augmentation, we obtain a better result compared with the performance given in the original dataset [paper](https://arxiv.org/pdf/1912.04838.pdf). For more details about the configuration and performance, please refer to README.md in the `configs/pointpillars/`. A more complete benchmark based on other settings and methods is coming soon.
+
+## Evaluation
+
+For evaluation on Waymo, please follow the [instruction](https://github.com/waymo-research/waymo-open-dataset/blob/master/docs/quick_start.md/) to build the binary file `compute_detection_metrics_main` for metrics computation and put it into `mmdet3d/core/evaluation/waymo_utils/`. Basically, you can follow the commands below to install `bazel` and build the file.
+
+```shell
+# download the code and enter the base directory
+git clone https://github.com/waymo-research/waymo-open-dataset.git waymo-od
+cd waymo-od
+git checkout remotes/origin/master
+
+# use the Bazel build system
+sudo apt-get install --assume-yes pkg-config zip g++ zlib1g-dev unzip python3 python3-pip
+BAZEL_VERSION=3.1.0
+wget https://github.com/bazelbuild/bazel/releases/download/${BAZEL_VERSION}/bazel-${BAZEL_VERSION}-installer-linux-x86_64.sh
+sudo bash bazel-${BAZEL_VERSION}-installer-linux-x86_64.sh
+sudo apt install build-essential
+
+# configure .bazelrc
+./configure.sh
+# delete previous bazel outputs and reset internal caches
+bazel clean
+
+bazel build waymo_open_dataset/metrics/tools/compute_detection_metrics_main
+cp bazel-bin/waymo_open_dataset/metrics/tools/compute_detection_metrics_main ../mmdetection3d/mmdet3d/core/evaluation/waymo_utils/
+```
+
+Then you can evaluate your models on Waymo. An example to evaluate PointPillars on Waymo with 8 GPUs with Waymo metrics is as follows.
+
+```shell
+./tools/slurm_test.sh ${PARTITION} ${JOB_NAME} configs/pointpillars/hv_pointpillars_secfpn_sbn-2x16_2x_waymo-3d-car.py \
+ checkpoints/hv_pointpillars_secfpn_sbn-2x16_2x_waymo-3d-car_latest.pth --out results/waymo-car/results_eval.pkl \
+ --eval waymo --eval-options 'pklfile_prefix=results/waymo-car/kitti_results' \
+ 'submission_prefix=results/waymo-car/kitti_results'
+```
+
+`pklfile_prefix` should be given in the `--eval-options` if the bin file is needed to be generated. For metrics, `waymo` is the recommended official evaluation prototype. Currently, evaluating with choice `kitti` is adapted from KITTI and the results for each difficulty are not exactly the same as the definition of KITTI. Instead, most of objects are marked with difficulty 0 currently, which will be fixed in the future. The reasons of its instability include the large computation for evaluation, the lack of occlusion and truncation in the converted data, different definitions of difficulty and different methods of computing Average Precision.
+
+**Notice**:
+
+1. Sometimes when using `bazel` to build `compute_detection_metrics_main`, an error `'round' is not a member of 'std'` may appear. We just need to remove the `std::` before `round` in that file.
+
+2. Considering it takes a little long time to evaluate once, we recommend to evaluate only once at the end of model training.
+
+3. To use TensorFlow with CUDA 9, it is recommended to compile it from source. Apart from official tutorials, you can refer to this [link](https://github.com/SmileTM/Tensorflow2.X-GPU-CUDA9.0) for possibly suitable precompiled packages and useful information for compiling it from source.
+
+## Testing and make a submission
+
+An example to test PointPillars on Waymo with 8 GPUs, generate the bin files and make a submission to the leaderboard.
+
+```shell
+./tools/slurm_test.sh ${PARTITION} ${JOB_NAME} configs/pointpillars/hv_pointpillars_secfpn_sbn-2x16_2x_waymo-3d-car.py \
+ checkpoints/hv_pointpillars_secfpn_sbn-2x16_2x_waymo-3d-car_latest.pth --out results/waymo-car/results_eval.pkl \
+ --format-only --eval-options 'pklfile_prefix=results/waymo-car/kitti_results' \
+ 'submission_prefix=results/waymo-car/kitti_results'
+```
+
+After generating the bin file, you can simply build the binary file `create_submission` and use them to create a submission file by following the [instruction](https://github.com/waymo-research/waymo-open-dataset/blob/master/docs/quick_start.md/). Basically, here are some example commands.
+
+```shell
+cd ../waymo-od/
+bazel build waymo_open_dataset/metrics/tools/create_submission
+cp bazel-bin/waymo_open_dataset/metrics/tools/create_submission ../mmdetection3d/mmdet3d/core/evaluation/waymo_utils/
+vim waymo_open_dataset/metrics/tools/submission.txtpb # set the metadata information
+cp waymo_open_dataset/metrics/tools/submission.txtpb ../mmdetection3d/mmdet3d/core/evaluation/waymo_utils/
+
+cd ../mmdetection3d
+# suppose the result bin is in `results/waymo-car/submission`
+mmdet3d/core/evaluation/waymo_utils/create_submission --input_filenames='results/waymo-car/kitti_results_test.bin' --output_filename='results/waymo-car/submission/model' --submission_filename='mmdet3d/core/evaluation/waymo_utils/submission.txtpb'
+
+tar cvf results/waymo-car/submission/my_model.tar results/waymo-car/submission/my_model/
+gzip results/waymo-car/submission/my_model.tar
+```
+
+For evaluation on the validation set with the eval server, you can also use the same way to generate a submission. Make sure you change the fields in `submission.txtpb` before running the command above.
diff --git a/docs/en/demo.md b/docs/en/demo.md
new file mode 100644
index 0000000..bf84870
--- /dev/null
+++ b/docs/en/demo.md
@@ -0,0 +1,89 @@
+# Demo
+
+## Introduction
+
+We provide scripts for multi-modality/single-modality (LiDAR-based/vision-based), indoor/outdoor 3D detection and 3D semantic segmentation demos. The pre-trained models can be downloaded from [model zoo](https://github.com/open-mmlab/mmdetection3d/blob/master/docs/en/model_zoo.md/). We provide pre-processed sample data from KITTI, SUN RGB-D, nuScenes and ScanNet dataset. You can use any other data following our pre-processing steps.
+
+## Testing
+
+### 3D Detection
+
+#### Single-modality demo
+
+To test a 3D detector on point cloud data, simply run:
+
+```shell
+python demo/pcd_demo.py ${PCD_FILE} ${CONFIG_FILE} ${CHECKPOINT_FILE} [--device ${GPU_ID}] [--score-thr ${SCORE_THR}] [--out-dir ${OUT_DIR}] [--show]
+```
+
+The visualization results including a point cloud and predicted 3D bounding boxes will be saved in `${OUT_DIR}/PCD_NAME`, which you can open using [MeshLab](http://www.meshlab.net/). Note that if you set the flag `--show`, the prediction result will be displayed online using [Open3D](http://www.open3d.org/).
+
+Example on KITTI data using [SECOND](https://github.com/open-mmlab/mmdetection3d/tree/master/configs/second) model:
+
+```shell
+python demo/pcd_demo.py demo/data/kitti/kitti_000008.bin configs/second/hv_second_secfpn_6x8_80e_kitti-3d-car.py checkpoints/hv_second_secfpn_6x8_80e_kitti-3d-car_20200620_230238-393f000c.pth
+```
+
+Example on SUN RGB-D data using [VoteNet](https://github.com/open-mmlab/mmdetection3d/tree/master/configs/votenet) model:
+
+```shell
+python demo/pcd_demo.py demo/data/sunrgbd/sunrgbd_000017.bin configs/votenet/votenet_16x8_sunrgbd-3d-10class.py checkpoints/votenet_16x8_sunrgbd-3d-10class_20200620_230238-4483c0c0.pth
+```
+
+Remember to convert the VoteNet checkpoint if you are using mmdetection3d version >= 0.6.0. See its [README](https://github.com/open-mmlab/mmdetection3d/blob/master/configs/votenet/README.md/) for detailed instructions on how to convert the checkpoint.
+
+#### Multi-modality demo
+
+To test a 3D detector on multi-modality data (typically point cloud and image), simply run:
+
+```shell
+python demo/multi_modality_demo.py ${PCD_FILE} ${IMAGE_FILE} ${ANNOTATION_FILE} ${CONFIG_FILE} ${CHECKPOINT_FILE} [--device ${GPU_ID}] [--score-thr ${SCORE_THR}] [--out-dir ${OUT_DIR}] [--show]
+```
+
+where the `ANNOTATION_FILE` should provide the 3D to 2D projection matrix. The visualization results including a point cloud, an image, predicted 3D bounding boxes and their projection on the image will be saved in `${OUT_DIR}/PCD_NAME`.
+
+Example on KITTI data using [MVX-Net](https://github.com/open-mmlab/mmdetection3d/tree/master/configs/mvxnet) model:
+
+```shell
+python demo/multi_modality_demo.py demo/data/kitti/kitti_000008.bin demo/data/kitti/kitti_000008.png demo/data/kitti/kitti_000008_infos.pkl configs/mvxnet/dv_mvx-fpn_second_secfpn_adamw_2x8_80e_kitti-3d-3class.py checkpoints/dv_mvx-fpn_second_secfpn_adamw_2x8_80e_kitti-3d-3class_20200621_003904-10140f2d.pth
+```
+
+Example on SUN RGB-D data using [ImVoteNet](https://github.com/open-mmlab/mmdetection3d/tree/master/configs/imvotenet) model:
+
+```shell
+python demo/multi_modality_demo.py demo/data/sunrgbd/sunrgbd_000017.bin demo/data/sunrgbd/sunrgbd_000017.jpg demo/data/sunrgbd/sunrgbd_000017_infos.pkl configs/imvotenet/imvotenet_stage2_16x8_sunrgbd-3d-10class.py checkpoints/imvotenet_stage2_16x8_sunrgbd-3d-10class_20210323_184021-d44dcb66.pth
+```
+
+### Monocular 3D Detection
+
+To test a monocular 3D detector on image data, simply run:
+
+```shell
+python demo/mono_det_demo.py ${IMAGE_FILE} ${ANNOTATION_FILE} ${CONFIG_FILE} ${CHECKPOINT_FILE} [--device ${GPU_ID}] [--out-dir ${OUT_DIR}] [--show]
+```
+
+where the `ANNOTATION_FILE` should provide the 3D to 2D projection matrix (camera intrinsic matrix). The visualization results including an image and its predicted 3D bounding boxes projected on the image will be saved in `${OUT_DIR}/PCD_NAME`.
+
+Example on nuScenes data using [FCOS3D](https://github.com/open-mmlab/mmdetection3d/tree/master/configs/fcos3d) model:
+
+```shell
+python demo/mono_det_demo.py demo/data/nuscenes/n015-2018-07-24-11-22-45+0800__CAM_BACK__1532402927637525.jpg demo/data/nuscenes/n015-2018-07-24-11-22-45+0800__CAM_BACK__1532402927637525_mono3d.coco.json configs/fcos3d/fcos3d_r101_caffe_fpn_gn-head_dcn_2x8_1x_nus-mono3d_finetune.py checkpoints/fcos3d_r101_caffe_fpn_gn-head_dcn_2x8_1x_nus-mono3d_finetune_20210717_095645-8d806dc2.pth
+```
+
+Note that when visualizing results of monocular 3D detection for flipped images, the camera intrinsic matrix should also be modified accordingly. See more details and examples in PR [#744](https://github.com/open-mmlab/mmdetection3d/pull/744).
+
+### 3D Segmentation
+
+To test a 3D segmentor on point cloud data, simply run:
+
+```shell
+python demo/pc_seg_demo.py ${PCD_FILE} ${CONFIG_FILE} ${CHECKPOINT_FILE} [--device ${GPU_ID}] [--out-dir ${OUT_DIR}] [--show]
+```
+
+The visualization results including a point cloud and its predicted 3D segmentation mask will be saved in `${OUT_DIR}/PCD_NAME`.
+
+Example on ScanNet data using [PointNet++ (SSG)](https://github.com/open-mmlab/mmdetection3d/tree/master/configs/pointnet2) model:
+
+```shell
+python demo/pc_seg_demo.py demo/data/scannet/scene0000_00.bin configs/pointnet2/pointnet2_ssg_16x2_cosine_200e_scannet_seg-3d-20class.py checkpoints/pointnet2_ssg_16x2_cosine_200e_scannet_seg-3d-20class_20210514_143644-ee73704a.pth
+```
diff --git a/docs/en/faq.md b/docs/en/faq.md
new file mode 100644
index 0000000..38b87af
--- /dev/null
+++ b/docs/en/faq.md
@@ -0,0 +1,71 @@
+# FAQ
+
+We list some potential troubles encountered by users and developers, along with their corresponding solutions. Feel free to enrich the list if you find any frequent issues and contribute your solutions to solve them. If you have any trouble with environment configuration, model training, etc, please create an issue using the [provided templates](https://github.com/open-mmlab/mmdetection3d/blob/master/.github/ISSUE_TEMPLATE/error-report.md/) and fill in all required information in the template.
+
+## MMCV/MMDet/MMDet3D Installation
+
+- Compatibility issue between MMCV, MMDetection, MMSegmentation and MMDection3D; "ConvWS is already registered in conv layer"; "AssertionError: MMCV==xxx is used but incompatible. Please install mmcv>=xxx, \<=xxx."
+
+ The required versions of MMCV, MMDetection and MMSegmentation for different versions of MMDetection3D are as below. Please install the correct version of MMCV, MMDetection and MMSegmentation to avoid installation issues.
+
+ | MMDetection3D version | MMDetection version | MMSegmentation version | MMCV version |
+ | :-------------------: | :---------------------: | :--------------------: | :------------------------: |
+ | master | mmdet>=2.24.0, <=3.0.0 | mmseg>=0.20.0, <=1.0.0 | mmcv-full>=1.4.8, <=1.6.0 |
+ | v1.0.0rc3 | mmdet>=2.24.0, <=3.0.0 | mmseg>=0.20.0, <=1.0.0 | mmcv-full>=1.4.8, <=1.6.0 |
+ | v1.0.0rc2 | mmdet>=2.24.0, <=3.0.0 | mmseg>=0.20.0, <=1.0.0 | mmcv-full>=1.4.8, <=1.6.0 |
+ | v1.0.0rc1 | mmdet>=2.19.0, <=3.0.0 | mmseg>=0.20.0, <=1.0.0 | mmcv-full>=1.4.8, <=1.5.0 |
+ | v1.0.0rc0 | mmdet>=2.19.0, <=3.0.0 | mmseg>=0.20.0, <=1.0.0 | mmcv-full>=1.3.17, <=1.5.0 |
+ | 0.18.1 | mmdet>=2.19.0, <=3.0.0 | mmseg>=0.20.0, <=1.0.0 | mmcv-full>=1.3.17, <=1.5.0 |
+ | 0.18.0 | mmdet>=2.19.0, <=3.0.0 | mmseg>=0.20.0, <=1.0.0 | mmcv-full>=1.3.17, <=1.5.0 |
+ | 0.17.3 | mmdet>=2.14.0, <=3.0.0 | mmseg>=0.14.1, <=1.0.0 | mmcv-full>=1.3.8, <=1.4.0 |
+ | 0.17.2 | mmdet>=2.14.0, <=3.0.0 | mmseg>=0.14.1, <=1.0.0 | mmcv-full>=1.3.8, <=1.4.0 |
+ | 0.17.1 | mmdet>=2.14.0, <=3.0.0 | mmseg>=0.14.1, <=1.0.0 | mmcv-full>=1.3.8, <=1.4.0 |
+ | 0.17.0 | mmdet>=2.14.0, <=3.0.0 | mmseg>=0.14.1, <=1.0.0 | mmcv-full>=1.3.8, <=1.4.0 |
+ | 0.16.0 | mmdet>=2.14.0, <=3.0.0 | mmseg>=0.14.1, <=1.0.0 | mmcv-full>=1.3.8, <=1.4.0 |
+ | 0.15.0 | mmdet>=2.14.0, <=3.0.0 | mmseg>=0.14.1, <=1.0.0 | mmcv-full>=1.3.8, <=1.4.0 |
+ | 0.14.0 | mmdet>=2.10.0, <=2.11.0 | mmseg==0.14.0 | mmcv-full>=1.3.1, <=1.4.0 |
+ | 0.13.0 | mmdet>=2.10.0, <=2.11.0 | Not required | mmcv-full>=1.2.4, <=1.4.0 |
+ | 0.12.0 | mmdet>=2.5.0, <=2.11.0 | Not required | mmcv-full>=1.2.4, <=1.4.0 |
+ | 0.11.0 | mmdet>=2.5.0, <=2.11.0 | Not required | mmcv-full>=1.2.4, <=1.3.0 |
+ | 0.10.0 | mmdet>=2.5.0, <=2.11.0 | Not required | mmcv-full>=1.2.4, <=1.3.0 |
+ | 0.9.0 | mmdet>=2.5.0, <=2.11.0 | Not required | mmcv-full>=1.2.4, <=1.3.0 |
+ | 0.8.0 | mmdet>=2.5.0, <=2.11.0 | Not required | mmcv-full>=1.1.5, <=1.3.0 |
+ | 0.7.0 | mmdet>=2.5.0, <=2.11.0 | Not required | mmcv-full>=1.1.5, <=1.3.0 |
+ | 0.6.0 | mmdet>=2.4.0, <=2.11.0 | Not required | mmcv-full>=1.1.3, <=1.2.0 |
+ | 0.5.0 | 2.3.0 | Not required | mmcv-full==1.0.5 |
+
+- If you faced the error shown below when importing open3d:
+
+ `OSError: /lib/x86_64-linux-gnu/libm.so.6: version 'GLIBC_2.27' not found`
+
+ please downgrade open3d to 0.9.0.0, because the latest open3d needs the support of file 'GLIBC_2.27', which only exists in Ubuntu 18.04, not in Ubuntu 16.04.
+
+- If you faced the error when importing pycocotools, this is because nuscenes-devkit installs pycocotools but mmdet relies on mmpycocotools. The current workaround is as below. We will migrate to use pycocotools in the future.
+
+ ```shell
+ pip uninstall pycocotools mmpycocotools
+ pip install mmpycocotools
+ ```
+
+ **NOTE**: We have migrated to use pycocotools in mmdet3d >= 0.13.0.
+
+- If you face the error shown below when importing pycocotools:
+
+ `ValueError: numpy.ndarray size changed, may indicate binary incompatibility. Expected 88 from C header, got 80 from PyObject`
+
+ please downgrade pycocotools to 2.0.1 because of the incompatibility between the newest pycocotools and numpy \< 1.20.0. Or you can compile and install the latest pycocotools from source as below:
+
+ `pip install -e "git+https://github.com/cocodataset/cocoapi#egg=pycocotools&subdirectory=PythonAPI"`
+
+ or
+
+ `pip install -e "git+https://github.com/ppwwyyxx/cocoapi#egg=pycocotools&subdirectory=PythonAPI"`
+
+## How to annotate point cloud?
+
+MMDetection3D does not support point cloud annotation. Some open-source annotation tool are offered for reference:
+
+- [SUSTechPOINTS](https://github.com/naurril/SUSTechPOINTS)
+- [LATTE](https://github.com/bernwang/latte)
+
+Besides, we improved [LATTE](https://github.com/bernwang/latte) for better use. More details can be found [here](https://arxiv.org/abs/2011.10174).
diff --git a/docs/en/getting_started.md b/docs/en/getting_started.md
new file mode 100644
index 0000000..74fdf12
--- /dev/null
+++ b/docs/en/getting_started.md
@@ -0,0 +1,278 @@
+# Prerequisites
+In this section we demonstrate how to prepare an environment with PyTorch.
+MMDection3D works on Linux, Windows (experimental support) and macOS and requires the following packages:
+
+- Python 3.6+
+- PyTorch 1.3+
+- CUDA 9.2+ (If you build PyTorch from source, CUDA 9.0 is also compatible)
+- GCC 5+
+- [MMCV](https://mmcv.readthedocs.io/en/latest/#installation)
+
+```{note}
+If you are experienced with PyTorch and have already installed it, just skip this part and jump to the [next section](#installation). Otherwise, you can follow these steps for the preparation.
+```
+
+**Step 0.** Download and install Miniconda from the [official website](https://docs.conda.io/en/latest/miniconda.html).
+
+**Step 1.** Create a conda environment and activate it.
+
+```shell
+conda create --name openmmlab python=3.8 -y
+conda activate openmmlab
+```
+
+**Step 2.** Install PyTorch following [official instructions](https://pytorch.org/get-started/locally/), e.g.
+
+On GPU platforms:
+
+```shell
+conda install pytorch torchvision -c pytorch
+```
+
+On CPU platforms:
+
+```shell
+conda install pytorch torchvision cpuonly -c pytorch
+```
+
+# Installation
+
+We recommend that users follow our best practices to install MMDetection3D. However, the whole process is highly customizable. See [Customize Installation](#customize-installation) section for more information.
+
+## Best Practices
+Assuming that you already have CUDA 11.0 installed, here is a full script for quick installation of MMDetection3D with conda.
+Otherwise, you should refer to the step-by-step installation instructions in the next section.
+
+```shell
+pip install openmim
+mim install mmcv-full
+mim install mmdet
+mim install mmsegmentation
+git clone https://github.com/open-mmlab/mmdetection3d.git
+cd mmdetection3d
+pip install -e .
+```
+
+**Step 0.** Install [MMCV](https://github.com/open-mmlab/mmcv) using [MIM](https://github.com/open-mmlab/mim).
+
+**Step 1.** Install [MMDetection](https://github.com/open-mmlab/mmdetection).
+
+
+```shell
+pip install mmdet
+```
+
+Optionally, you could also build MMDetection from source in case you want to modify the code:
+
+```shell
+git clone https://github.com/open-mmlab/mmdetection.git
+cd mmdetection
+git checkout v2.24.0 # switch to v2.24.0 branch
+pip install -r requirements/build.txt
+pip install -v -e . # or "python setup.py develop"
+```
+
+**Step 2.** Install [MMSegmentation](https://github.com/open-mmlab/mmsegmentation).
+
+```shell
+pip install mmsegmentation
+```
+
+Optionally, you could also build MMSegmentation from source in case you want to modify the code:
+
+```shell
+git clone https://github.com/open-mmlab/mmsegmentation.git
+cd mmsegmentation
+git checkout v0.20.0 # switch to v0.20.0 branch
+pip install -e . # or "python setup.py develop"
+```
+
+**Step 3.** Clone the MMDetection3D repository.
+
+```shell
+git clone https://github.com/open-mmlab/mmdetection3d.git
+cd mmdetection3d
+```
+
+**Step 4.** Install build requirements and then install MMDetection3D.
+
+```shell
+pip install -v -e . # or "python setup.py develop"
+```
+
+Note:
+
+1. The git commit id will be written to the version number with step d, e.g. 0.6.0+2e7045c. The version will also be saved in trained models.
+It is recommended that you run step d each time you pull some updates from github. If C++/CUDA codes are modified, then this step is compulsory.
+
+ > Important: Be sure to remove the `./build` folder if you reinstall mmdet with a different CUDA/PyTorch version.
+
+ ```shell
+ pip uninstall mmdet3d
+ rm -rf ./build
+ find . -name "*.so" | xargs rm
+ ```
+
+2. Following the above instructions, MMDetection3D is installed on `dev` mode, any local modifications made to the code will take effect without the need to reinstall it (unless you submit some commits and want to update the version number).
+
+3. If you would like to use `opencv-python-headless` instead of `opencv-python`,
+you can install it before installing MMCV.
+
+4. Some dependencies are optional. Simply running `pip install -v -e .` will only install the minimum runtime requirements. To use optional dependencies like `albumentations` and `imagecorruptions` either install them manually with `pip install -r requirements/optional.txt` or specify desired extras when calling `pip` (e.g. `pip install -v -e .[optional]`). Valid keys for the extras field are: `all`, `tests`, `build`, and `optional`.
+
+ We have supported spconv2.0. If the user has installed spconv2.0, the code will use spconv2.0 first, which will take up less GPU memory than using the default mmcv spconv. Users can use the following commands to install spconv2.0:
+
+ ```bash
+ pip install cumm-cuxxx
+ pip install spconv-cuxxx
+ ```
+
+ Where xxx is the CUDA version in the environment.
+
+ For example, using CUDA 10.2, the command will be `pip install cumm-cu102 && pip install spconv-cu102`.
+
+ Supported CUDA versions include 10.2, 11.1, 11.3, and 11.4. Users can also install it by building from the source. For more details please refer to [spconv v2.x](https://github.com/traveller59/spconv).
+
+ We also support Minkowski Engine as a sparse convolution backend. If necessary please follow original [installation guide](https://github.com/NVIDIA/MinkowskiEngine#installation) or use `pip`:
+
+ ```shell
+ conda install openblas-devel -c anaconda
+ pip install -U git+https://github.com/NVIDIA/MinkowskiEngine -v --no-deps --install-option="--blas_include_dirs=/opt/conda/include" --install-option="--blas=openblas"
+ ```
+
+5. The code can not be built for CPU only environment (where CUDA isn't available) for now.
+
+
+## Verification
+
+### Verify with point cloud demo
+
+We provide several demo scripts to test a single sample. Pre-trained models can be downloaded from [model zoo](model_zoo.md). To test a single-modality 3D detection on point cloud scenes:
+
+```shell
+python demo/pcd_demo.py ${PCD_FILE} ${CONFIG_FILE} ${CHECKPOINT_FILE} [--device ${GPU_ID}] [--score-thr ${SCORE_THR}] [--out-dir ${OUT_DIR}]
+```
+
+Examples:
+
+```shell
+python demo/pcd_demo.py demo/data/kitti/kitti_000008.bin configs/second/hv_second_secfpn_6x8_80e_kitti-3d-car.py checkpoints/hv_second_secfpn_6x8_80e_kitti-3d-car_20200620_230238-393f000c.pth
+```
+
+If you want to input a `ply` file, you can use the following function and convert it to `bin` format. Then you can use the converted `bin` file to generate demo.
+Note that you need to install `pandas` and `plyfile` before using this script. This function can also be used for data preprocessing for training ```ply data```.
+
+```python
+import numpy as np
+import pandas as pd
+from plyfile import PlyData
+
+def convert_ply(input_path, output_path):
+ plydata = PlyData.read(input_path) # read file
+ data = plydata.elements[0].data # read data
+ data_pd = pd.DataFrame(data) # convert to DataFrame
+ data_np = np.zeros(data_pd.shape, dtype=np.float) # initialize array to store data
+ property_names = data[0].dtype.names # read names of properties
+ for i, name in enumerate(
+ property_names): # read data by property
+ data_np[:, i] = data_pd[name]
+ data_np.astype(np.float32).tofile(output_path)
+```
+
+Examples:
+
+```python
+convert_ply('./test.ply', './test.bin')
+```
+
+If you have point clouds in other format (`off`, `obj`, etc.), you can use `trimesh` to convert them into `ply`.
+
+```python
+import trimesh
+
+def to_ply(input_path, output_path, original_type):
+ mesh = trimesh.load(input_path, file_type=original_type) # read file
+ mesh.export(output_path, file_type='ply') # convert to ply
+```
+
+Examples:
+
+```python
+to_ply('./test.obj', './test.ply', 'obj')
+```
+
+More demos about single/multi-modality and indoor/outdoor 3D detection can be found in [demo](demo.md).
+
+## Customize Installation
+
+### CUDA Versions
+When installing PyTorch, you need to specify the version of CUDA. If you are not clear on which to choose, follow our recommendations:
+
+- For Ampere-based NVIDIA GPUs, such as GeForce 30 series and NVIDIA A100, CUDA 11 is a must.
+- For older NVIDIA GPUs, CUDA 11 is backward compatible, but CUDA 10.2 offers better compatibility and is more lightweight.
+
+Please make sure the GPU driver satisfies the minimum version requirements. See [this table](https://docs.nvidia.com/cuda/cuda-toolkit-release-notes/index.html#cuda-major-component-versions__table-cuda-toolkit-driver-versions) for more information.
+
+```{note}
+Installing CUDA runtime libraries is enough if you follow our best practices, because no CUDA code will be compiled locally. However if you hope to compile MMCV from source or develop other CUDA operators, you need to install the complete CUDA toolkit from NVIDIA's [website](https://developer.nvidia.com/cuda-downloads), and its version should match the CUDA version of PyTorch. i.e., the specified version of cudatoolkit in `conda install` command.
+```
+
+### Install MMCV without MIM
+
+MMCV contains C++ and CUDA extensions, thus depending on PyTorch in a complex way. MIM solves such dependencies automatically and makes the installation easier. However, it is not a must.
+
+To install MMCV with pip instead of MIM, please follow [MMCV installation guides](https://mmcv.readthedocs.io/en/latest/get_started/installation.html). This requires manually specifying a find-url based on PyTorch version and its CUDA version.
+
+For example, the following command install mmcv-full built for PyTorch 1.10.x and CUDA 11.3.
+
+```shell
+pip install mmcv-full -f https://download.openmmlab.com/mmcv/dist/cu113/torch1.10/index.html
+```
+
+
+
+### Using MMDetection3D with Docker
+
+We provide a [Dockerfile](https://github.com/open-mmlab/mmdetection3d/blob/master/docker/Dockerfile) to build an image.
+
+```shell
+# build an image with PyTorch 1.6, CUDA 10.1
+docker build -t mmdetection3d -f docker/Dockerfile .
+```
+
+Run it with
+
+```shell
+docker run --gpus all --shm-size=8g -it -v {DATA_DIR}:/mmdetection3d/data mmdetection3d
+```
+
+### A from-scratch setup script
+
+Here is a full script for setting up MMdetection3D with conda.
+
+```shell
+conda create -n open-mmlab python=3.7 -y
+conda activate open-mmlab
+
+# install latest PyTorch prebuilt with the default prebuilt CUDA version (usually the latest)
+conda install -c pytorch pytorch torchvision -y
+
+# install mmcv
+pip install mmcv-full
+
+# install mmdetection
+pip install git+https://github.com/open-mmlab/mmdetection.git
+
+# install mmsegmentation
+pip install git+https://github.com/open-mmlab/mmsegmentation.git
+
+# install mmdetection3d
+git clone https://github.com/open-mmlab/mmdetection3d.git
+cd mmdetection3d
+pip install -v -e .
+```
+
+## Trouble shooting
+
+If you have some issues during the installation, please first view the [FAQ](faq.md) page.
+You may [open an issue](https://github.com/open-mmlab/mmdetection3d/issues/new/choose) on GitHub if no solution is found.
diff --git a/docs/en/index.rst b/docs/en/index.rst
new file mode 100644
index 0000000..0d21d0b
--- /dev/null
+++ b/docs/en/index.rst
@@ -0,0 +1,98 @@
+Welcome to MMDetection3D's documentation!
+==========================================
+
+.. toctree::
+ :maxdepth: 1
+ :caption: Get Started
+
+ getting_started.md
+
+.. toctree::
+ :maxdepth: 1
+ :caption: Demo
+
+ demo.md
+
+.. toctree::
+ :maxdepth: 1
+ :caption: Model Zoo
+
+ model_zoo.md
+
+.. toctree::
+ :maxdepth: 1
+ :caption: Data Preparation
+
+ data_preparation.md
+
+.. toctree::
+ :maxdepth: 1
+ :caption: Exist Data and Model
+
+ 1_exist_data_model.md
+
+.. toctree::
+ :maxdepth: 1
+ :caption: New Data and Model
+
+ 2_new_data_model.md
+
+.. toctree::
+ :maxdepth: 1
+ :caption: Supported Tasks
+
+ supported_tasks/index.rst
+
+.. toctree::
+ :maxdepth: 1
+ :caption: Datasets
+
+ datasets/index.rst
+
+.. toctree::
+ :maxdepth: 1
+ :caption: Tutorials
+
+ tutorials/index.rst
+
+.. toctree::
+ :maxdepth: 1
+ :caption: Useful Tools and Scripts
+
+ useful_tools.md
+
+.. toctree::
+ :maxdepth: 1
+ :caption: Notes
+
+ benchmarks.md
+
+.. toctree::
+ :maxdepth: 1
+ :caption: FAQ
+
+ faq.md
+
+.. toctree::
+ :maxdepth: 1
+ :caption: Compatibility
+
+ compatibility.md
+
+.. toctree::
+ :maxdepth: 1
+ :caption: API Reference
+
+ api.rst
+
+.. toctree::
+ :maxdepth: 1
+ :caption: Switch Language
+
+ switch_language.md
+
+Indices and tables
+==================
+
+* :ref:`genindex`
+* :ref:`search`
diff --git a/docs/en/make.bat b/docs/en/make.bat
new file mode 100644
index 0000000..922152e
--- /dev/null
+++ b/docs/en/make.bat
@@ -0,0 +1,35 @@
+@ECHO OFF
+
+pushd %~dp0
+
+REM Command file for Sphinx documentation
+
+if "%SPHINXBUILD%" == "" (
+ set SPHINXBUILD=sphinx-build
+)
+set SOURCEDIR=.
+set BUILDDIR=_build
+
+if "%1" == "" goto help
+
+%SPHINXBUILD% >NUL 2>NUL
+if errorlevel 9009 (
+ echo.
+ echo.The 'sphinx-build' command was not found. Make sure you have Sphinx
+ echo.installed, then set the SPHINXBUILD environment variable to point
+ echo.to the full path of the 'sphinx-build' executable. Alternatively you
+ echo.may add the Sphinx directory to PATH.
+ echo.
+ echo.If you don't have Sphinx installed, grab it from
+ echo.http://sphinx-doc.org/
+ exit /b 1
+)
+
+%SPHINXBUILD% -M %1 %SOURCEDIR% %BUILDDIR% %SPHINXOPTS% %O%
+goto end
+
+:help
+%SPHINXBUILD% -M help %SOURCEDIR% %BUILDDIR% %SPHINXOPTS% %O%
+
+:end
+popd
diff --git a/docs/en/model_zoo.md b/docs/en/model_zoo.md
new file mode 100644
index 0000000..1a647ce
--- /dev/null
+++ b/docs/en/model_zoo.md
@@ -0,0 +1,109 @@
+# Model Zoo
+
+## Common settings
+
+- We use distributed training.
+- For fair comparison with other codebases, we report the GPU memory as the maximum value of `torch.cuda.max_memory_allocated()` for all 8 GPUs. Note that this value is usually less than what `nvidia-smi` shows.
+- We report the inference time as the total time of network forwarding and post-processing, excluding the data loading time. Results are obtained with the script [benchmark.py](https://github.com/open-mmlab/mmdetection/blob/master/tools/analysis_tools/benchmark.py) which computes the average time on 2000 images.
+
+## Baselines
+
+### SECOND
+
+Please refer to [SECOND](https://github.com/open-mmlab/mmdetection3d/blob/master/configs/second) for details. We provide SECOND baselines on KITTI and Waymo datasets.
+
+### PointPillars
+
+Please refer to [PointPillars](https://github.com/open-mmlab/mmdetection3d/blob/master/configs/pointpillars) for details. We provide pointpillars baselines on KITTI, nuScenes, Lyft, and Waymo datasets.
+
+### Part-A2
+
+Please refer to [Part-A2](https://github.com/open-mmlab/mmdetection3d/blob/master/configs/parta2) for details.
+
+### VoteNet
+
+Please refer to [VoteNet](https://github.com/open-mmlab/mmdetection3d/blob/master/configs/votenet) for details. We provide VoteNet baselines on ScanNet and SUNRGBD datasets.
+
+### Dynamic Voxelization
+
+Please refer to [Dynamic Voxelization](https://github.com/open-mmlab/mmdetection3d/blob/master/configs/dynamic_voxelization) for details.
+
+### MVXNet
+
+Please refer to [MVXNet](https://github.com/open-mmlab/mmdetection3d/blob/master/configs/mvxnet) for details.
+
+### RegNetX
+
+Please refer to [RegNet](https://github.com/open-mmlab/mmdetection3d/blob/master/configs/regnet) for details. We provide pointpillars baselines with RegNetX backbones on nuScenes and Lyft datasets currently.
+
+### nuImages
+
+We also support baseline models on [nuImages dataset](https://www.nuscenes.org/nuimages). Please refer to [nuImages](https://github.com/open-mmlab/mmdetection3d/blob/master/configs/nuimages) for details. We report Mask R-CNN, Cascade Mask R-CNN and HTC results currently.
+
+### H3DNet
+
+Please refer to [H3DNet](https://github.com/open-mmlab/mmdetection3d/blob/master/configs/h3dnet) for details.
+
+### 3DSSD
+
+Please refer to [3DSSD](https://github.com/open-mmlab/mmdetection3d/blob/master/configs/3dssd) for details.
+
+### CenterPoint
+
+Please refer to [CenterPoint](https://github.com/open-mmlab/mmdetection3d/blob/master/configs/centerpoint) for details.
+
+### SSN
+
+Please refer to [SSN](https://github.com/open-mmlab/mmdetection3d/blob/master/configs/ssn) for details. We provide pointpillars with shape-aware grouping heads used in SSN on the nuScenes and Lyft datasets currently.
+
+### ImVoteNet
+
+Please refer to [ImVoteNet](https://github.com/open-mmlab/mmdetection3d/blob/master/configs/imvotenet) for details. We provide ImVoteNet baselines on SUNRGBD dataset.
+
+### FCOS3D
+
+Please refer to [FCOS3D](https://github.com/open-mmlab/mmdetection3d/blob/master/configs/fcos3d) for details. We provide FCOS3D baselines on the nuScenes dataset.
+
+### PointNet++
+
+Please refer to [PointNet++](https://github.com/open-mmlab/mmdetection3d/blob/master/configs/pointnet2) for details. We provide PointNet++ baselines on ScanNet and S3DIS datasets.
+
+### Group-Free-3D
+
+Please refer to [Group-Free-3D](https://github.com/open-mmlab/mmdetection3d/blob/master/configs/groupfree3d) for details. We provide Group-Free-3D baselines on ScanNet dataset.
+
+### ImVoxelNet
+
+Please refer to [ImVoxelNet](https://github.com/open-mmlab/mmdetection3d/blob/master/configs/imvoxelnet) for details. We provide ImVoxelNet baselines on KITTI dataset.
+
+### PAConv
+
+Please refer to [PAConv](https://github.com/open-mmlab/mmdetection3d/blob/master/configs/paconv) for details. We provide PAConv baselines on S3DIS dataset.
+
+### DGCNN
+
+Please refer to [DGCNN](https://github.com/open-mmlab/mmdetection3d/tree/v1.0.0.dev0/configs/dgcnn) for details. We provide DGCNN baselines on S3DIS dataset.
+
+### SMOKE
+
+Please refer to [SMOKE](https://github.com/open-mmlab/mmdetection3d/tree/v1.0.0.dev0/configs/smoke) for details. We provide SMOKE baselines on KITTI dataset.
+
+### PGD
+
+Please refer to [PGD](https://github.com/open-mmlab/mmdetection3d/tree/v1.0.0.dev0/configs/pgd) for details. We provide PGD baselines on KITTI and nuScenes dataset.
+
+### PointRCNN
+
+Please refer to [PointRCNN](https://github.com/open-mmlab/mmdetection3d/tree/v1.0.0.dev0/configs/point_rcnn) for details. We provide PointRCNN baselines on KITTI dataset.
+
+### MonoFlex
+
+Please refer to [MonoFlex](https://github.com/open-mmlab/mmdetection3d/tree/v1.0.0.dev0/configs/monoflex) for details. We provide MonoFlex baselines on KITTI dataset.
+
+### SA-SSD
+
+Please refer to [SA-SSD](https://github.com/open-mmlab/mmdetection3d/blob/master/configs/sassd) for details. We provide SA-SSD baselines on the KITTI dataset.
+
+### Mixed Precision (FP16) Training
+
+Please refer to [Mixed Precision (FP16) Training on PointPillars](https://github.com/open-mmlab/mmdetection3d/tree/v1.0.0.dev0/configs/pointpillars/hv_pointpillars_fpn_sbn-all_fp16_2x8_2x_nus-3d.py) for details.
diff --git a/docs/en/stat.py b/docs/en/stat.py
new file mode 100755
index 0000000..b5f10a8
--- /dev/null
+++ b/docs/en/stat.py
@@ -0,0 +1,62 @@
+#!/usr/bin/env python
+import functools as func
+import glob
+import re
+from os import path as osp
+
+import numpy as np
+
+url_prefix = 'https://github.com/open-mmlab/mmdetection3d/blob/master/'
+
+files = sorted(glob.glob('../configs/*/README.md'))
+
+stats = []
+titles = []
+num_ckpts = 0
+
+for f in files:
+ url = osp.dirname(f.replace('../', url_prefix))
+
+ with open(f, 'r') as content_file:
+ content = content_file.read()
+
+ title = content.split('\n')[0].replace('#', '').strip()
+ ckpts = set(x.lower().strip()
+ for x in re.findall(r'https?://download.*\.pth', content)
+ if 'mmdetection3d' in x)
+ if len(ckpts) == 0:
+ continue
+
+ _papertype = [x for x in re.findall(r'', content)]
+ assert len(_papertype) > 0
+ papertype = _papertype[0]
+
+ paper = set([(papertype, title)])
+
+ titles.append(title)
+ num_ckpts += len(ckpts)
+ statsmsg = f"""
+\t* [{papertype}] [{title}]({url}) ({len(ckpts)} ckpts)
+"""
+ stats.append((paper, ckpts, statsmsg))
+
+allpapers = func.reduce(lambda a, b: a.union(b), [p for p, _, _ in stats])
+msglist = '\n'.join(x for _, _, x in stats)
+
+papertypes, papercounts = np.unique([t for t, _ in allpapers],
+ return_counts=True)
+countstr = '\n'.join(
+ [f' - {t}: {c}' for t, c in zip(papertypes, papercounts)])
+
+modelzoo = f"""
+\n## Model Zoo Statistics
+
+* Number of papers: {len(set(titles))}
+{countstr}
+
+* Number of checkpoints: {num_ckpts}
+{msglist}
+"""
+
+with open('model_zoo.md', 'a') as f:
+ f.write(modelzoo)
diff --git a/docs/en/supported_tasks/index.rst b/docs/en/supported_tasks/index.rst
new file mode 100644
index 0000000..7b30c59
--- /dev/null
+++ b/docs/en/supported_tasks/index.rst
@@ -0,0 +1,6 @@
+.. toctree::
+ :maxdepth: 2
+
+ lidar_det3d.md
+ vision_det3d.md
+ lidar_sem_seg3d.md
diff --git a/docs/en/supported_tasks/lidar_det3d.md b/docs/en/supported_tasks/lidar_det3d.md
new file mode 100644
index 0000000..2d860b6
--- /dev/null
+++ b/docs/en/supported_tasks/lidar_det3d.md
@@ -0,0 +1,102 @@
+# LiDAR-Based 3D Detection
+
+LiDAR-based 3D detection is one of the most basic tasks supported in MMDetection3D.
+It expects the given model to take any number of points with features collected by LiDAR as input, and predict the 3D bounding boxes and category labels for each object of interest.
+Next, taking PointPillars on the KITTI dataset as an example, we will show how to prepare data, train and test a model on a standard 3D detection benchmark, and how to visualize and validate the results.
+
+## Data Preparation
+
+To begin with, we need to download the raw data and reorganize the data in a standard way presented in the [doc for data preparation](https://mmdetection3d.readthedocs.io/en/latest/data_preparation.html).
+Note that for KITTI, we need extra txt files for data splits.
+
+Due to different ways of organizing the raw data in different datasets, we typically need to collect the useful data information with a .pkl or .json file.
+So after getting all the raw data ready, we need to run the scripts provided in the `create_data.py` for different datasets to generate data infos.
+For example, for KITTI we need to run:
+
+```
+python tools/create_data.py kitti --root-path ./data/kitti --out-dir ./data/kitti --extra-tag kitti
+```
+
+Afterwards, the related folder structure should be as follows:
+
+```
+mmdetection3d
+├── mmdet3d
+├── tools
+├── configs
+├── data
+│ ├── kitti
+│ │ ├── ImageSets
+│ │ ├── testing
+│ │ │ ├── calib
+│ │ │ ├── image_2
+│ │ │ ├── velodyne
+│ │ ├── training
+│ │ │ ├── calib
+│ │ │ ├── image_2
+│ │ │ ├── label_2
+│ │ │ ├── velodyne
+│ │ ├── kitti_gt_database
+│ │ ├── kitti_infos_train.pkl
+│ │ ├── kitti_infos_trainval.pkl
+│ │ ├── kitti_infos_val.pkl
+│ │ ├── kitti_infos_test.pkl
+│ │ ├── kitti_dbinfos_train.pkl
+```
+
+## Training
+
+Then let us train a model with provided configs for PointPillars.
+You can basically follow this [tutorial](https://mmdetection3d.readthedocs.io/en/latest/1_exist_data_model.html#inference-with-existing-models) for sample scripts when training with different GPU settings.
+Suppose we use 8 GPUs on a single machine with distributed training:
+
+```
+./tools/dist_train.sh configs/pointpillars/hv_pointpillars_secfpn_6x8_160e_kitti-3d-3class.py 8
+```
+
+Note that `6x8` in the config name refers to the training is completed with 8 GPUs and 6 samples on each GPU.
+If your customized setting is different from this, sometimes you need to adjust the learning rate accordingly.
+A basic rule can be referred to [here](https://arxiv.org/abs/1706.02677).
+
+## Quantitative Evaluation
+
+During training, the model checkpoints will be evaluated regularly according to the setting of `evaluation = dict(interval=xxx)` in the config.
+We support official evaluation protocols for different datasets.
+For KITTI, the model will be evaluated with mean average precision (mAP) with Intersection over Union (IoU) thresholds 0.5/0.7 for 3 categories respectively.
+The evaluation results will be printed in the command like:
+
+```
+Car AP@0.70, 0.70, 0.70:
+bbox AP:98.1839, 89.7606, 88.7837
+bev AP:89.6905, 87.4570, 85.4865
+3d AP:87.4561, 76.7569, 74.1302
+aos AP:97.70, 88.73, 87.34
+Car AP@0.70, 0.50, 0.50:
+bbox AP:98.1839, 89.7606, 88.7837
+bev AP:98.4400, 90.1218, 89.6270
+3d AP:98.3329, 90.0209, 89.4035
+aos AP:97.70, 88.73, 87.34
+```
+
+In addition, you can also evaluate a specific model checkpoint after training is finished. Simply run scripts like the following:
+
+```
+./tools/dist_test.sh configs/pointpillars/hv_pointpillars_secfpn_6x8_160e_kitti-3d-3class.py \
+ work_dirs/pointpillars/latest.pth --eval mAP
+```
+
+## Testing and Making a Submission
+
+If you would like to only conduct inference or test the model performance on the online benchmark,
+you just need to replace the `--eval mAP` with `--format-only` in the previous evaluation script and specify the `pklfile_prefix` and `submission_prefix` if necessary,
+e.g., adding an option `--eval-options submission_prefix=work_dirs/pointpillars/test_submission`.
+Please guarantee the [info for testing](https://github.com/open-mmlab/mmdetection3d/blob/master/configs/_base_/datasets/kitti-3d-3class.py#L131) in the config corresponds to the test set instead of validation set.
+After generating the results, you can basically compress the folder and upload to the KITTI evaluation server.
+
+## Qualitative Validation
+
+MMDetection3D also provides versatile tools for visualization such that we can have an intuitive feeling of the detection results predicted by our trained models.
+You can either set the `--eval-options 'show=True' 'out_dir=${SHOW_DIR}'` option to visualize the detection results online during evaluation,
+or using `tools/misc/visualize_results.py` for offline visualization.
+Besides, we also provide scripts `tools/misc/browse_dataset.py` to visualize the dataset without inference.
+Please refer more details in the [doc for visualization](https://mmdetection3d.readthedocs.io/en/latest/useful_tools.html#visualization).
diff --git a/docs/en/supported_tasks/lidar_sem_seg3d.md b/docs/en/supported_tasks/lidar_sem_seg3d.md
new file mode 100644
index 0000000..07f48d4
--- /dev/null
+++ b/docs/en/supported_tasks/lidar_sem_seg3d.md
@@ -0,0 +1,94 @@
+# LiDAR-Based 3D Semantic Segmentation
+
+LiDAR-based 3D semantic segmentation is one of the most basic tasks supported in MMDetection3D.
+It expects the given model to take any number of points with features collected by LiDAR as input, and predict the semantic labels for each input point.
+Next, taking PointNet++ (SSG) on the ScanNet dataset as an example, we will show how to prepare data, train and test a model on a standard 3D semantic segmentation benchmark, and how to visualize and validate the results.
+
+## Data Preparation
+
+To begin with, we need to download the raw data from ScanNet's [official website](http://kaldir.vc.in.tum.de/scannet_benchmark/documentation).
+
+Due to different ways of organizing the raw data in different datasets, we typically need to collect the useful data information with a .pkl or .json file.
+
+So after getting all the raw data ready, we can follow the instructions presented in [ScanNet README doc](https://github.com/open-mmlab/mmdetection3d/blob/master/data/scannet/README.md/) to generate data infos.
+
+Afterwards, the related folder structure should be as follows:
+
+```
+mmdetection3d
+├── mmdet3d
+├── tools
+├── configs
+├── data
+│ ├── scannet
+│ │ ├── scannet_utils.py
+│ │ ├── batch_load_scannet_data.py
+│ │ ├── load_scannet_data.py
+│ │ ├── scannet_utils.py
+│ │ ├── README.md
+│ │ ├── scans
+│ │ ├── scans_test
+│ │ ├── scannet_instance_data
+│ │ ├── points
+│ │ ├── instance_mask
+│ │ ├── semantic_mask
+│ │ ├── seg_info
+│ │ │ ├── train_label_weight.npy
+│ │ │ ├── train_resampled_scene_idxs.npy
+│ │ │ ├── val_label_weight.npy
+│ │ │ ├── val_resampled_scene_idxs.npy
+│ │ ├── scannet_infos_train.pkl
+│ │ ├── scannet_infos_val.pkl
+│ │ ├── scannet_infos_test.pkl
+```
+
+## Training
+
+Then let us train a model with provided configs for PointNet++ (SSG).
+You can basically follow this [tutorial](https://mmdetection3d.readthedocs.io/en/latest/1_exist_data_model.html#inference-with-existing-models) for sample scripts when training with different GPU settings.
+Suppose we use 2 GPUs on a single machine with distributed training:
+
+```
+./tools/dist_train.sh configs/pointnet2/pointnet2_ssg_16x2_cosine_200e_scannet_seg-3d-20class.py 2
+```
+
+Note that `16x2` in the config name refers to the training is completed with 2 GPUs and 16 samples on each GPU.
+If your customized setting is different from this, sometimes you need to adjust the learning rate accordingly.
+A basic rule can be referred to [here](https://arxiv.org/abs/1706.02677).
+
+## Quantitative Evaluation
+
+During training, the model checkpoints will be evaluated regularly according to the setting of `evaluation = dict(interval=xxx)` in the config.
+We support official evaluation protocols for different datasets.
+For ScanNet, the model will be evaluated with mean Intersection over Union (mIoU) over all 20 categories.
+The evaluation results will be printed in the command like:
+
+```
++---------+--------+--------+---------+--------+--------+--------+--------+--------+--------+-----------+---------+---------+--------+---------+--------------+----------------+--------+--------+---------+----------------+--------+--------+---------+
+| classes | wall | floor | cabinet | bed | chair | sofa | table | door | window | bookshelf | picture | counter | desk | curtain | refrigerator | showercurtrain | toilet | sink | bathtub | otherfurniture | miou | acc | acc_cls |
++---------+--------+--------+---------+--------+--------+--------+--------+--------+--------+-----------+---------+---------+--------+---------+--------------+----------------+--------+--------+---------+----------------+--------+--------+---------+
+| results | 0.7257 | 0.9373 | 0.4625 | 0.6613 | 0.7707 | 0.5562 | 0.5864 | 0.4010 | 0.4558 | 0.7011 | 0.2500 | 0.4645 | 0.4540 | 0.5399 | 0.2802 | 0.3488 | 0.7359 | 0.4971 | 0.6922 | 0.3681 | 0.5444 | 0.8118 | 0.6695 |
++---------+--------+--------+---------+--------+--------+--------+--------+--------+--------+-----------+---------+---------+--------+---------+--------------+----------------+--------+--------+---------+----------------+--------+--------+---------+
+```
+
+In addition, you can also evaluate a specific model checkpoint after training is finished. Simply run scripts like the following:
+
+```
+./tools/dist_test.sh configs/pointnet2/pointnet2_ssg_16x2_cosine_200e_scannet_seg-3d-20class.py \
+ work_dirs/pointnet2_ssg/latest.pth --eval mIoU
+```
+
+## Testing and Making a Submission
+
+If you would like to only conduct inference or test the model performance on the online benchmark,
+you need to replace the `--eval mIoU` with `--format-only` in the previous evaluation script and change `ann_file=data_root + 'scannet_infos_val.pkl'` to `ann_file=data_root + 'scannet_infos_test.pkl'` in the ScanNet dataset's [config](https://github.com/open-mmlab/mmdetection3d/blob/master/configs/_base_/datasets/scannet_seg-3d-20class.py#L126). Remember to specify the `txt_prefix` as the directory to save the testing results,
+e.g., adding an option `--eval-options txt_prefix=work_dirs/pointnet2_ssg/test_submission`.
+After generating the results, you can basically compress the folder and upload to the [ScanNet evaluation server](http://kaldir.vc.in.tum.de/scannet_benchmark/semantic_label_3d).
+
+## Qualitative Validation
+
+MMDetection3D also provides versatile tools for visualization such that we can have an intuitive feeling of the segmentation results predicted by our trained models.
+You can either set the `--eval-options 'show=True' 'out_dir=${SHOW_DIR}'` option to visualize the segmentation results online during evaluation,
+or using `tools/misc/visualize_results.py` for offline visualization.
+Besides, we also provide scripts `tools/misc/browse_dataset.py` to visualize the dataset without inference.
+Please refer more details in the [doc for visualization](https://mmdetection3d.readthedocs.io/en/latest/useful_tools.html#visualization).
diff --git a/docs/en/supported_tasks/vision_det3d.md b/docs/en/supported_tasks/vision_det3d.md
new file mode 100644
index 0000000..2dc4f6f
--- /dev/null
+++ b/docs/en/supported_tasks/vision_det3d.md
@@ -0,0 +1,133 @@
+# Vision-Based 3D Detection
+
+Vision-based 3D detection refers to the 3D detection solutions based on vision-only input, such as monocular, binocular, and multi-view image based 3D detection.
+Currently, we only support monocular and multi-view 3D detection methods. Other approaches should be also compatible with our framework and will be supported in the future.
+
+It expects the given model to take any number of images as input, and predict the 3D bounding boxes and category labels for each object of interest.
+Taking FCOS3D on the nuScenes dataset as an example, we will show how to prepare data, train and test a model on a standard 3D detection benchmark, and how to visualize and validate the results.
+
+## Data Preparation
+
+To begin with, we need to download the raw data and reorganize the data in a standard way presented in the [doc for data preparation](https://mmdetection3d.readthedocs.io/en/latest/data_preparation.html).
+
+Due to different ways of organizing the raw data in different datasets, we typically need to collect the useful data information with a .pkl or .json file.
+So after getting all the raw data ready, we need to run the scripts provided in the `create_data.py` for different datasets to generate data infos.
+For example, for nuScenes we need to run:
+
+```
+python tools/create_data.py nuscenes --root-path ./data/nuscenes --out-dir ./data/nuscenes --extra-tag nuscenes
+```
+
+Afterwards, the related folder structure should be as follows:
+
+```
+mmdetection3d
+├── mmdet3d
+├── tools
+├── configs
+├── data
+│ ├── nuscenes
+│ │ ├── maps
+│ │ ├── samples
+│ │ ├── sweeps
+│ │ ├── v1.0-test
+| | ├── v1.0-trainval
+│ │ ├── nuscenes_database
+│ │ ├── nuscenes_infos_train.pkl
+│ │ ├── nuscenes_infos_trainval.pkl
+│ │ ├── nuscenes_infos_val.pkl
+│ │ ├── nuscenes_infos_test.pkl
+│ │ ├── nuscenes_dbinfos_train.pkl
+│ │ ├── nuscenes_infos_train_mono3d.coco.json
+│ │ ├── nuscenes_infos_trainval_mono3d.coco.json
+│ │ ├── nuscenes_infos_val_mono3d.coco.json
+│ │ ├── nuscenes_infos_test_mono3d.coco.json
+```
+
+Note that the .pkl files here are mainly used for methods using LiDAR data and .json files are used for 2D detection/vision-only 3D detection.
+The .json files only contain infos for 2D detection before supporting monocular 3D detection in v0.13.0, so if you need the latest infos, please checkout the branches after v0.13.0.
+
+## Training
+
+Then let us train a model with provided configs for FCOS3D. The basic script is the same as other models.
+You can basically follow the examples provided in this [tutorial](https://mmdetection3d.readthedocs.io/en/latest/1_exist_data_model.html#inference-with-existing-models) when training with different GPU settings.
+Suppose we use 8 GPUs on a single machine with distributed training:
+
+```
+./tools/dist_train.sh configs/fcos3d/fcos3d_r101_caffe_fpn_gn-head_dcn_2x8_1x_nus-mono3d.py 8
+```
+
+Note that `2x8` in the config name refers to the training is completed with 8 GPUs and 2 data samples on each GPU.
+If your customized setting is different from this, sometimes you need to adjust the learning rate accordingly.
+A basic rule can be referred to [here](https://arxiv.org/abs/1706.02677).
+
+We can also achieve better performance with finetuned FCOS3D by running:
+
+```
+./tools/dist_train.sh fcos3d_r101_caffe_fpn_gn-head_dcn_2x8_1x_nus-mono3d_finetune.py 8
+```
+
+After training a baseline model with the previous script,
+please remember to modify the path [here](https://github.com/open-mmlab/mmdetection3d/blob/master/configs/fcos3d/fcos3d_r101_caffe_fpn_gn-head_dcn_2x8_1x_nus-mono3d_finetune.py#L8) correspondingly.
+
+## Quantitative Evaluation
+
+During training, the model checkpoints will be evaluated regularly according to the setting of `evaluation = dict(interval=xxx)` in the config.
+
+We support official evaluation protocols for different datasets.
+Due to the output format is the same as 3D detection based on other modalities, the evaluation methods are also the same.
+
+For nuScenes, the model will be evaluated with distance-based mean AP (mAP) and NuScenes Detection Score (NDS) for 10 categories respectively.
+The evaluation results will be printed in the command like:
+
+```
+mAP: 0.3197
+mATE: 0.7595
+mASE: 0.2700
+mAOE: 0.4918
+mAVE: 1.3307
+mAAE: 0.1724
+NDS: 0.3905
+Eval time: 170.8s
+
+Per-class results:
+Object Class AP ATE ASE AOE AVE AAE
+car 0.503 0.577 0.152 0.111 2.096 0.136
+truck 0.223 0.857 0.224 0.220 1.389 0.179
+bus 0.294 0.855 0.204 0.190 2.689 0.283
+trailer 0.081 1.094 0.243 0.553 0.742 0.167
+construction_vehicle 0.058 1.017 0.450 1.019 0.137 0.341
+pedestrian 0.392 0.687 0.284 0.694 0.876 0.158
+motorcycle 0.317 0.737 0.265 0.580 2.033 0.104
+bicycle 0.308 0.704 0.299 0.892 0.683 0.010
+traffic_cone 0.555 0.486 0.309 nan nan nan
+barrier 0.466 0.581 0.269 0.169 nan nan
+```
+
+In addition, you can also evaluate a specific model checkpoint after training is finished. Simply run scripts like the following:
+
+```
+./tools/dist_test.sh configs/fcos3d/fcos3d_r101_caffe_fpn_gn-head_dcn_2x8_1x_nus-mono3d.py \
+ work_dirs/fcos3d/latest.pth --eval mAP
+```
+
+## Testing and Making a Submission
+
+If you would like to only conduct inference or test the model performance on the online benchmark,
+you just need to replace the `--eval mAP` with `--format-only` in the previous evaluation script and specify the `jsonfile_prefix` if necessary,
+e.g., adding an option `--eval-options jsonfile_prefix=work_dirs/fcos3d/test_submission`.
+Please guarantee the [info for testing](https://github.com/open-mmlab/mmdetection3d/blob/master/configs/_base_/datasets/nus-mono3d.py#L93) in the config corresponds to the test set instead of validation set.
+
+After generating the results, you can basically compress the folder and upload to the evalAI evaluation server for nuScenes 3D detection challenge.
+
+## Qualitative Validation
+
+MMDetection3D also provides versatile tools for visualization such that we can have an intuitive feeling of the detection results predicted by our trained models.
+You can either set the `--eval-options 'show=True' 'out_dir=${SHOW_DIR}'` option to visualize the detection results online during evaluation,
+or using `tools/misc/visualize_results.py` for offline visualization.
+
+Besides, we also provide scripts `tools/misc/browse_dataset.py` to visualize the dataset without inference.
+Please refer more details in the [doc for visualization](https://mmdetection3d.readthedocs.io/en/latest/useful_tools.html#visualization).
+
+Note that currently we only support the visualization on images for vision-only methods.
+The visualization in the perspective view and bird-eye-view (BEV) will be integrated in the future.
diff --git a/docs/en/switch_language.md b/docs/en/switch_language.md
new file mode 100644
index 0000000..d33d080
--- /dev/null
+++ b/docs/en/switch_language.md
@@ -0,0 +1,3 @@
+## English
+
+## 简体中文
diff --git a/docs/en/tutorials/backends_support.md b/docs/en/tutorials/backends_support.md
new file mode 100644
index 0000000..5304ccd
--- /dev/null
+++ b/docs/en/tutorials/backends_support.md
@@ -0,0 +1,154 @@
+# Tutorial 7: Backends Support
+
+We support different file client backends: Disk, Ceph and LMDB, etc. Here is an example of how to modify configs for Ceph-based data loading and saving.
+
+## Load data and annotations from Ceph
+
+We support loading data and generated annotation info files (pkl and json) from Ceph:
+
+```python
+# set file client backends as Ceph
+file_client_args = dict(
+ backend='petrel',
+ path_mapping=dict({
+ './data/nuscenes/':
+ 's3://openmmlab/datasets/detection3d/nuscenes/', # replace the path with your data path on Ceph
+ 'data/nuscenes/':
+ 's3://openmmlab/datasets/detection3d/nuscenes/' # replace the path with your data path on Ceph
+ }))
+
+db_sampler = dict(
+ data_root=data_root,
+ info_path=data_root + 'kitti_dbinfos_train.pkl',
+ rate=1.0,
+ prepare=dict(filter_by_difficulty=[-1], filter_by_min_points=dict(Car=5)),
+ sample_groups=dict(Car=15),
+ classes=class_names,
+ # set file client for points loader to load training data
+ points_loader=dict(
+ type='LoadPointsFromFile',
+ coord_type='LIDAR',
+ load_dim=4,
+ use_dim=4,
+ file_client_args=file_client_args),
+ # set file client for data base sampler to load db info file
+ file_client_args=file_client_args)
+
+train_pipeline = [
+ # set file client for loading training data
+ dict(type='LoadPointsFromFile', coord_type='LIDAR', load_dim=4, use_dim=4, file_client_args=file_client_args),
+ # set file client for loading training data annotations
+ dict(type='LoadAnnotations3D', with_bbox_3d=True, with_label_3d=True, file_client_args=file_client_args),
+ dict(type='ObjectSample', db_sampler=db_sampler),
+ dict(
+ type='ObjectNoise',
+ num_try=100,
+ translation_std=[0.25, 0.25, 0.25],
+ global_rot_range=[0.0, 0.0],
+ rot_range=[-0.15707963267, 0.15707963267]),
+ dict(type='RandomFlip3D', flip_ratio_bev_horizontal=0.5),
+ dict(
+ type='GlobalRotScaleTrans',
+ rot_range=[-0.78539816, 0.78539816],
+ scale_ratio_range=[0.95, 1.05]),
+ dict(type='PointsRangeFilter', point_cloud_range=point_cloud_range),
+ dict(type='ObjectRangeFilter', point_cloud_range=point_cloud_range),
+ dict(type='PointShuffle'),
+ dict(type='DefaultFormatBundle3D', class_names=class_names),
+ dict(type='Collect3D', keys=['points', 'gt_bboxes_3d', 'gt_labels_3d'])
+]
+test_pipeline = [
+ # set file client for loading validation/testing data
+ dict(type='LoadPointsFromFile', coord_type='LIDAR', load_dim=4, use_dim=4, file_client_args=file_client_args),
+ dict(
+ type='MultiScaleFlipAug3D',
+ img_scale=(1333, 800),
+ pts_scale_ratio=1,
+ flip=False,
+ transforms=[
+ dict(
+ type='GlobalRotScaleTrans',
+ rot_range=[0, 0],
+ scale_ratio_range=[1., 1.],
+ translation_std=[0, 0, 0]),
+ dict(type='RandomFlip3D'),
+ dict(
+ type='PointsRangeFilter', point_cloud_range=point_cloud_range),
+ dict(
+ type='DefaultFormatBundle3D',
+ class_names=class_names,
+ with_label=False),
+ dict(type='Collect3D', keys=['points'])
+ ])
+]
+
+data = dict(
+ # set file client for loading training info files (.pkl)
+ train=dict(
+ type='RepeatDataset',
+ times=2,
+ dataset=dict(pipeline=train_pipeline, classes=class_names, file_client_args=file_client_args)),
+ # set file client for loading validation info files (.pkl)
+ val=dict(pipeline=test_pipeline, classes=class_names,file_client_args=file_client_args),
+ # set file client for loading testing info files (.pkl)
+ test=dict(pipeline=test_pipeline, classes=class_names, file_client_args=file_client_args))
+```
+
+## Load pretrained model from Ceph
+
+```python
+model = dict(
+ pts_backbone=dict(
+ _delete_=True,
+ type='NoStemRegNet',
+ arch='regnetx_1.6gf',
+ init_cfg=dict(
+ type='Pretrained', checkpoint='s3://openmmlab/checkpoints/mmdetection3d/regnetx_1.6gf'), # replace the path with your pretrained model path on Ceph
+ ...
+```
+
+## Load checkpoint from Ceph
+
+```python
+# replace the path with your checkpoint path on Ceph
+load_from = 's3://openmmlab/checkpoints/mmdetection3d/v0.1.0_models/pointpillars/hv_pointpillars_secfpn_6x8_160e_kitti-3d-car/hv_pointpillars_secfpn_6x8_160e_kitti-3d-car_20200620_230614-77663cd6.pth.pth'
+resume_from = None
+workflow = [('train', 1)]
+```
+
+## Save checkpoint into Ceph
+
+```python
+# checkpoint saving
+# replace the path with your checkpoint saving path on Ceph
+checkpoint_config = dict(interval=1, max_keep_ckpts=2, out_dir='s3://openmmlab/mmdetection3d')
+```
+
+## EvalHook saves the best checkpoint into Ceph
+
+```python
+# replace the path with your checkpoint saving path on Ceph
+evaluation = dict(interval=1, save_best='bbox', out_dir='s3://openmmlab/mmdetection3d')
+```
+
+## Save the training log into Ceph
+
+The training log will be backed up to the specified Ceph path after training.
+
+```python
+log_config = dict(
+ interval=50,
+ hooks=[
+ dict(type='TextLoggerHook', out_dir='s3://openmmlab/mmdetection3d'),
+ ])
+```
+
+You can also delete the local training log after backing up to the specified Ceph path by setting `keep_local = False`.
+
+```python
+log_config = dict(
+ interval=50,
+ hooks=[
+ dict(type='TextLoggerHook', out_dir='s3://openmmlab/mmdetection3d'', keep_local=False),
+ ])
+```
diff --git a/docs/en/tutorials/config.md b/docs/en/tutorials/config.md
new file mode 100644
index 0000000..9b4261f
--- /dev/null
+++ b/docs/en/tutorials/config.md
@@ -0,0 +1,526 @@
+# Tutorial 1: Learn about Configs
+
+We incorporate modular and inheritance design into our config system, which is convenient to conduct various experiments.
+If you wish to inspect the config file, you may run `python tools/misc/print_config.py /PATH/TO/CONFIG` to see the complete config.
+You may also pass `--options xxx.yyy=zzz` to see updated config.
+
+## Config File Structure
+
+There are 4 basic component types under `config/_base_`, dataset, model, schedule, default_runtime.
+Many methods could be easily constructed with one of each like SECOND, PointPillars, PartA2, and VoteNet.
+The configs that are composed by components from `_base_` are called _primitive_.
+
+For all configs under the same folder, it is recommended to have only **one** _primitive_ config. All other configs should inherit from the _primitive_ config. In this way, the maximum of inheritance level is 3.
+
+For easy understanding, we recommend contributors to inherit from exiting methods.
+For example, if some modification is made based on PointPillars, user may first inherit the basic PointPillars structure by specifying `_base_ = ../pointpillars/hv_pointpillars_fpn_sbn-all_4x8_2x_nus-3d.py`, then modify the necessary fields in the config files.
+
+If you are building an entirely new method that does not share the structure with any of the existing methods, you may create a folder `xxx_rcnn` under `configs`,
+
+Please refer to [mmcv](https://mmcv.readthedocs.io/en/latest/understand_mmcv/config.html) for detailed documentation.
+
+## Config Name Style
+
+We follow the below style to name config files. Contributors are advised to follow the same style.
+
+```
+{model}_[model setting]_{backbone}_[neck]_[norm setting]_[misc]_[batch_per_gpu x gpu]_{schedule}_{dataset}
+```
+
+`{xxx}` is required field and `[yyy]` is optional.
+
+- `{model}`: model type like `hv_pointpillars` (Hard Voxelization PointPillars), `VoteNet`, etc.
+- `[model setting]`: specific setting for some model.
+- `{backbone}`: backbone type like `regnet-400mf`, `regnet-1.6gf`.
+- `[neck]`: neck type like `fpn`, `secfpn`.
+- `[norm_setting]`: `bn` (Batch Normalization) is used unless specified, other norm layer type could be `gn` (Group Normalization), `sbn` (Synchronized Batch Normalization).
+ `gn-head`/`gn-neck` indicates GN is applied in head/neck only, while `gn-all` means GN is applied in the entire model, e.g. backbone, neck, head.
+- `[misc]`: miscellaneous setting/plugins of model, e.g. `strong-aug` means using stronger augmentation strategies for training.
+- `[batch_per_gpu x gpu]`: samples per GPU and GPUs, `4x8` is used by default.
+- `{schedule}`: training schedule, options are `1x`, `2x`, `20e`, etc.
+ `1x` and `2x` means 12 epochs and 24 epochs respectively.
+ `20e` is adopted in cascade models, which denotes 20 epochs.
+ For `1x`/`2x`, initial learning rate decays by a factor of 10 at the 8/16th and 11/22th epochs.
+ For `20e`, initial learning rate decays by a factor of 10 at the 16th and 19th epochs.
+- `{dataset}`: dataset like `nus-3d`, `kitti-3d`, `lyft-3d`, `scannet-3d`, `sunrgbd-3d`. We also indicate the number of classes we are using if there exist multiple settings, e.g., `kitti-3d-3class` and `kitti-3d-car` means training on KITTI dataset with 3 classes and single class, respectively.
+
+## Deprecated train_cfg/test_cfg
+
+Following MMDetection, the `train_cfg` and `test_cfg` are deprecated in config file, please specify them in the model config. The original config structure is as below.
+
+```python
+# deprecated
+model = dict(
+ type=...,
+ ...
+)
+train_cfg=dict(...)
+test_cfg=dict(...)
+```
+
+The migration example is as below.
+
+```python
+# recommended
+model = dict(
+ type=...,
+ ...
+ train_cfg=dict(...),
+ test_cfg=dict(...)
+)
+```
+
+## An example of VoteNet
+
+```python
+model = dict(
+ type='VoteNet', # The type of detector, refer to mmdet3d.models.detectors for more details
+ backbone=dict(
+ type='PointNet2SASSG', # The type of the backbone, refer to mmdet3d.models.backbones for more details
+ in_channels=4, # Input channels of point cloud
+ num_points=(2048, 1024, 512, 256), # The number of points which each SA module samples
+ radius=(0.2, 0.4, 0.8, 1.2), # Radius for each set abstraction layer
+ num_samples=(64, 32, 16, 16), # Number of samples for each set abstraction layer
+ sa_channels=((64, 64, 128), (128, 128, 256), (128, 128, 256),
+ (128, 128, 256)), # Out channels of each mlp in SA module
+ fp_channels=((256, 256), (256, 256)), # Out channels of each mlp in FP module
+ norm_cfg=dict(type='BN2d'), # Config of normalization layer
+ sa_cfg=dict( # Config of point set abstraction (SA) module
+ type='PointSAModule', # type of SA module
+ pool_mod='max', # Pool method ('max' or 'avg') for SA modules
+ use_xyz=True, # Whether to use xyz as features during feature gathering
+ normalize_xyz=True)), # Whether to use normalized xyz as feature during feature gathering
+ bbox_head=dict(
+ type='VoteHead', # The type of bbox head, refer to mmdet3d.models.dense_heads for more details
+ num_classes=18, # Number of classes for classification
+ bbox_coder=dict(
+ type='PartialBinBasedBBoxCoder', # The type of bbox_coder, refer to mmdet3d.core.bbox.coders for more details
+ num_sizes=18, # Number of size clusters
+ num_dir_bins=1, # Number of bins to encode direction angle
+ with_rot=False, # Whether the bbox is with rotation
+ mean_sizes=[[0.76966727, 0.8116021, 0.92573744],
+ [1.876858, 1.8425595, 1.1931566],
+ [0.61328, 0.6148609, 0.7182701],
+ [1.3955007, 1.5121545, 0.83443564],
+ [0.97949594, 1.0675149, 0.6329687],
+ [0.531663, 0.5955577, 1.7500148],
+ [0.9624706, 0.72462326, 1.1481868],
+ [0.83221924, 1.0490936, 1.6875663],
+ [0.21132214, 0.4206159, 0.5372846],
+ [1.4440073, 1.8970833, 0.26985747],
+ [1.0294262, 1.4040797, 0.87554324],
+ [1.3766412, 0.65521795, 1.6813129],
+ [0.6650819, 0.71111923, 1.298853],
+ [0.41999173, 0.37906948, 1.7513971],
+ [0.59359556, 0.5912492, 0.73919016],
+ [0.50867593, 0.50656086, 0.30136237],
+ [1.1511526, 1.0546296, 0.49706793],
+ [0.47535285, 0.49249494, 0.5802117]]), # Mean sizes for each class, the order is consistent with class_names.
+ vote_moudule_cfg=dict( # Config of vote module branch, refer to mmdet3d.models.model_utils for more details
+ in_channels=256, # Input channels for vote_module
+ vote_per_seed=1, # Number of votes to generate for each seed
+ gt_per_seed=3, # Number of gts for each seed
+ conv_channels=(256, 256), # Channels for convolution
+ conv_cfg=dict(type='Conv1d'), # Config of convolution
+ norm_cfg=dict(type='BN1d'), # Config of normalization
+ norm_feats=True, # Whether to normalize features
+ vote_loss=dict( # Config of the loss function for voting branch
+ type='ChamferDistance', # Type of loss for voting branch
+ mode='l1', # Loss mode of voting branch
+ reduction='none', # Specifies the reduction to apply to the output
+ loss_dst_weight=10.0)), # Destination loss weight of the voting branch
+ vote_aggregation_cfg=dict( # Config of vote aggregation branch
+ type='PointSAModule', # type of vote aggregation module
+ num_point=256, # Number of points for the set abstraction layer in vote aggregation branch
+ radius=0.3, # Radius for the set abstraction layer in vote aggregation branch
+ num_sample=16, # Number of samples for the set abstraction layer in vote aggregation branch
+ mlp_channels=[256, 128, 128, 128], # Mlp channels for the set abstraction layer in vote aggregation branch
+ use_xyz=True, # Whether to use xyz
+ normalize_xyz=True), # Whether to normalize xyz
+ feat_channels=(128, 128), # Channels for feature convolution
+ conv_cfg=dict(type='Conv1d'), # Config of convolution
+ norm_cfg=dict(type='BN1d'), # Config of normalization
+ objectness_loss=dict( # Config of objectness loss
+ type='CrossEntropyLoss', # Type of loss
+ class_weight=[0.2, 0.8], # Class weight of the objectness loss
+ reduction='sum', # Specifies the reduction to apply to the output
+ loss_weight=5.0), # Loss weight of the objectness loss
+ center_loss=dict( # Config of center loss
+ type='ChamferDistance', # Type of loss
+ mode='l2', # Loss mode of center loss
+ reduction='sum', # Specifies the reduction to apply to the output
+ loss_src_weight=10.0, # Source loss weight of the voting branch.
+ loss_dst_weight=10.0), # Destination loss weight of the voting branch.
+ dir_class_loss=dict( # Config of direction classification loss
+ type='CrossEntropyLoss', # Type of loss
+ reduction='sum', # Specifies the reduction to apply to the output
+ loss_weight=1.0), # Loss weight of the direction classification loss
+ dir_res_loss=dict( # Config of direction residual loss
+ type='SmoothL1Loss', # Type of loss
+ reduction='sum', # Specifies the reduction to apply to the output
+ loss_weight=10.0), # Loss weight of the direction residual loss
+ size_class_loss=dict( # Config of size classification loss
+ type='CrossEntropyLoss', # Type of loss
+ reduction='sum', # Specifies the reduction to apply to the output
+ loss_weight=1.0), # Loss weight of the size classification loss
+ size_res_loss=dict( # Config of size residual loss
+ type='SmoothL1Loss', # Type of loss
+ reduction='sum', # Specifies the reduction to apply to the output
+ loss_weight=3.3333333333333335), # Loss weight of the size residual loss
+ semantic_loss=dict( # Config of semantic loss
+ type='CrossEntropyLoss', # Type of loss
+ reduction='sum', # Specifies the reduction to apply to the output
+ loss_weight=1.0)), # Loss weight of the semantic loss
+ train_cfg = dict( # Config of training hyperparameters for VoteNet
+ pos_distance_thr=0.3, # distance >= threshold 0.3 will be taken as positive samples
+ neg_distance_thr=0.6, # distance < threshold 0.6 will be taken as negative samples
+ sample_mod='vote'), # Mode of the sampling method
+ test_cfg = dict( # Config of testing hyperparameters for VoteNet
+ sample_mod='seed', # Mode of the sampling method
+ nms_thr=0.25, # The threshold to be used during NMS
+ score_thr=0.8, # Threshold to filter out boxes
+ per_class_proposal=False)) # Whether to use per_class_proposal
+dataset_type = 'ScanNetDataset' # Type of the dataset
+data_root = './data/scannet/' # Root path of the data
+class_names = ('cabinet', 'bed', 'chair', 'sofa', 'table', 'door', 'window',
+ 'bookshelf', 'picture', 'counter', 'desk', 'curtain',
+ 'refrigerator', 'showercurtrain', 'toilet', 'sink', 'bathtub',
+ 'garbagebin') # Names of classes
+train_pipeline = [ # Training pipeline, refer to mmdet3d.datasets.pipelines for more details
+ dict(
+ type='LoadPointsFromFile', # First pipeline to load points, refer to mmdet3d.datasets.pipelines.indoor_loading for more details
+ shift_height=True, # Whether to use shifted height
+ load_dim=6, # The dimension of the loaded points
+ use_dim=[0, 1, 2]), # Which dimensions of the points to be used
+ dict(
+ type='LoadAnnotations3D', # Second pipeline to load annotations, refer to mmdet3d.datasets.pipelines.indoor_loading for more details
+ with_bbox_3d=True, # Whether to load 3D boxes
+ with_label_3d=True, # Whether to load 3D labels corresponding to each 3D box
+ with_mask_3d=True, # Whether to load 3D instance masks
+ with_seg_3d=True), # Whether to load 3D semantic masks
+ dict(
+ type='PointSegClassMapping', # Declare valid categories, refer to mmdet3d.datasets.pipelines.point_seg_class_mapping for more details
+ valid_cat_ids=(3, 4, 5, 6, 7, 8, 9, 10, 11, 12, 14, 16, 24, 28, 33, 34,
+ 36, 39), # all valid categories ids
+ max_cat_id=40), # max possible category id in input segmentation mask
+ dict(type='PointSample', # Sample points, refer to mmdet3d.datasets.pipelines.transforms_3d for more details
+ num_points=40000), # Number of points to be sampled
+ dict(type='IndoorFlipData', # Augmentation pipeline that flip points and 3d boxes
+ flip_ratio_yz=0.5, # Probability of being flipped along yz plane
+ flip_ratio_xz=0.5), # Probability of being flipped along xz plane
+ dict(
+ type='IndoorGlobalRotScale', # Augmentation pipeline that rotate and scale points and 3d boxes, refer to mmdet3d.datasets.pipelines.indoor_augment for more details
+ shift_height=True, # Whether the loaded points use `shift_height` attribute
+ rot_range=[-0.027777777777777776, 0.027777777777777776], # Range of rotation
+ scale_range=None), # Range of scale
+ dict(
+ type='DefaultFormatBundle3D', # Default format bundle to gather data in the pipeline, refer to mmdet3d.datasets.pipelines.formatting for more details
+ class_names=('cabinet', 'bed', 'chair', 'sofa', 'table', 'door',
+ 'window', 'bookshelf', 'picture', 'counter', 'desk',
+ 'curtain', 'refrigerator', 'showercurtrain', 'toilet',
+ 'sink', 'bathtub', 'garbagebin')),
+ dict(
+ type='Collect3D', # Pipeline that decides which keys in the data should be passed to the detector, refer to mmdet3d.datasets.pipelines.formatting for more details
+ keys=[
+ 'points', 'gt_bboxes_3d', 'gt_labels_3d', 'pts_semantic_mask',
+ 'pts_instance_mask'
+ ])
+]
+test_pipeline = [ # Testing pipeline, refer to mmdet3d.datasets.pipelines for more details
+ dict(
+ type='LoadPointsFromFile', # First pipeline to load points, refer to mmdet3d.datasets.pipelines.indoor_loading for more details
+ shift_height=True, # Whether to use shifted height
+ load_dim=6, # The dimension of the loaded points
+ use_dim=[0, 1, 2]), # Which dimensions of the points to be used
+ dict(type='PointSample', # Sample points, refer to mmdet3d.datasets.pipelines.transforms_3d for more details
+ num_points=40000), # Number of points to be sampled
+ dict(
+ type='DefaultFormatBundle3D', # Default format bundle to gather data in the pipeline, refer to mmdet3d.datasets.pipelines.formatting for more details
+ class_names=('cabinet', 'bed', 'chair', 'sofa', 'table', 'door',
+ 'window', 'bookshelf', 'picture', 'counter', 'desk',
+ 'curtain', 'refrigerator', 'showercurtrain', 'toilet',
+ 'sink', 'bathtub', 'garbagebin')),
+ dict(type='Collect3D', # Pipeline that decides which keys in the data should be passed to the detector, refer to mmdet3d.datasets.pipelines.formatting for more details
+ keys=['points'])
+]
+eval_pipeline = [ # Pipeline used for evaluation or visualization, refer to mmdet3d.datasets.pipelines for more details
+ dict(
+ type='LoadPointsFromFile', # First pipeline to load points, refer to mmdet3d.datasets.pipelines.indoor_loading for more details
+ shift_height=True, # Whether to use shifted height
+ load_dim=6, # The dimension of the loaded points
+ use_dim=[0, 1, 2]), # Which dimensions of the points to be used
+ dict(
+ type='DefaultFormatBundle3D', # Default format bundle to gather data in the pipeline, refer to mmdet3d.datasets.pipelines.formatting for more details
+ class_names=('cabinet', 'bed', 'chair', 'sofa', 'table', 'door',
+ 'window', 'bookshelf', 'picture', 'counter', 'desk',
+ 'curtain', 'refrigerator', 'showercurtrain', 'toilet',
+ 'sink', 'bathtub', 'garbagebin')),
+ with_label=False),
+ dict(type='Collect3D', # Pipeline that decides which keys in the data should be passed to the detector, refer to mmdet3d.datasets.pipelines.formatting for more details
+ keys=['points'])
+]
+data = dict(
+ samples_per_gpu=8, # Batch size of a single GPU
+ workers_per_gpu=4, # Number of workers to pre-fetch data for each single GPU
+ train=dict( # Train dataset config
+ type='RepeatDataset', # Wrapper of dataset, refer to https://github.com/open-mmlab/mmdetection/blob/master/mmdet/datasets/dataset_wrappers.py for details.
+ times=5, # Repeat times
+ dataset=dict(
+ type='ScanNetDataset', # Type of dataset
+ data_root='./data/scannet/', # Root path of the data
+ ann_file='./data/scannet/scannet_infos_train.pkl', # Ann path of the data
+ pipeline=[ # pipeline, this is passed by the train_pipeline created before.
+ dict(
+ type='LoadPointsFromFile',
+ shift_height=True,
+ load_dim=6,
+ use_dim=[0, 1, 2]),
+ dict(
+ type='LoadAnnotations3D',
+ with_bbox_3d=True,
+ with_label_3d=True,
+ with_mask_3d=True,
+ with_seg_3d=True),
+ dict(
+ type='PointSegClassMapping',
+ valid_cat_ids=(3, 4, 5, 6, 7, 8, 9, 10, 11, 12, 14, 16, 24,
+ 28, 33, 34, 36, 39),
+ max_cat_id=40),
+ dict(type='PointSample', num_points=40000),
+ dict(
+ type='IndoorFlipData',
+ flip_ratio_yz=0.5,
+ flip_ratio_xz=0.5),
+ dict(
+ type='IndoorGlobalRotScale',
+ shift_height=True,
+ rot_range=[-0.027777777777777776, 0.027777777777777776],
+ scale_range=None),
+ dict(
+ type='DefaultFormatBundle3D',
+ class_names=('cabinet', 'bed', 'chair', 'sofa', 'table',
+ 'door', 'window', 'bookshelf', 'picture',
+ 'counter', 'desk', 'curtain', 'refrigerator',
+ 'showercurtrain', 'toilet', 'sink', 'bathtub',
+ 'garbagebin')),
+ dict(
+ type='Collect3D',
+ keys=[
+ 'points', 'gt_bboxes_3d', 'gt_labels_3d',
+ 'pts_semantic_mask', 'pts_instance_mask'
+ ])
+ ],
+ filter_empty_gt=False, # Whether to filter empty ground truth boxes
+ classes=('cabinet', 'bed', 'chair', 'sofa', 'table', 'door',
+ 'window', 'bookshelf', 'picture', 'counter', 'desk',
+ 'curtain', 'refrigerator', 'showercurtrain', 'toilet',
+ 'sink', 'bathtub', 'garbagebin'))), # Names of classes
+ val=dict( # Validation dataset config
+ type='ScanNetDataset', # Type of dataset
+ data_root='./data/scannet/', # Root path of the data
+ ann_file='./data/scannet/scannet_infos_val.pkl', # Ann path of the data
+ pipeline=[ # Pipeline is passed by test_pipeline created before
+ dict(
+ type='LoadPointsFromFile',
+ shift_height=True,
+ load_dim=6,
+ use_dim=[0, 1, 2]),
+ dict(type='PointSample', num_points=40000),
+ dict(
+ type='DefaultFormatBundle3D',
+ class_names=('cabinet', 'bed', 'chair', 'sofa', 'table',
+ 'door', 'window', 'bookshelf', 'picture',
+ 'counter', 'desk', 'curtain', 'refrigerator',
+ 'showercurtrain', 'toilet', 'sink', 'bathtub',
+ 'garbagebin')),
+ dict(type='Collect3D', keys=['points'])
+ ],
+ classes=('cabinet', 'bed', 'chair', 'sofa', 'table', 'door', 'window',
+ 'bookshelf', 'picture', 'counter', 'desk', 'curtain',
+ 'refrigerator', 'showercurtrain', 'toilet', 'sink', 'bathtub',
+ 'garbagebin'), # Names of classes
+ test_mode=True), # Whether to use test mode
+ test=dict( # Test dataset config
+ type='ScanNetDataset', # Type of dataset
+ data_root='./data/scannet/', # Root path of the data
+ ann_file='./data/scannet/scannet_infos_val.pkl', # Ann path of the data
+ pipeline=[ # Pipeline is passed by test_pipeline created before
+ dict(
+ type='LoadPointsFromFile',
+ shift_height=True,
+ load_dim=6,
+ use_dim=[0, 1, 2]),
+ dict(type='PointSample', num_points=40000),
+ dict(
+ type='DefaultFormatBundle3D',
+ class_names=('cabinet', 'bed', 'chair', 'sofa', 'table',
+ 'door', 'window', 'bookshelf', 'picture',
+ 'counter', 'desk', 'curtain', 'refrigerator',
+ 'showercurtrain', 'toilet', 'sink', 'bathtub',
+ 'garbagebin')),
+ dict(type='Collect3D', keys=['points'])
+ ],
+ classes=('cabinet', 'bed', 'chair', 'sofa', 'table', 'door', 'window',
+ 'bookshelf', 'picture', 'counter', 'desk', 'curtain',
+ 'refrigerator', 'showercurtrain', 'toilet', 'sink', 'bathtub',
+ 'garbagebin'), # Names of classes
+ test_mode=True)) # Whether to use test mode
+evaluation = dict(pipeline=[ # Pipeline is passed by eval_pipeline created before
+ dict(
+ type='LoadPointsFromFile',
+ coord_type='DEPTH',
+ shift_height=False,
+ load_dim=6,
+ use_dim=[0, 1, 2]),
+ dict(
+ type='DefaultFormatBundle3D',
+ class_names=('cabinet', 'bed', 'chair', 'sofa', 'table', 'door',
+ 'window', 'bookshelf', 'picture', 'counter', 'desk',
+ 'curtain', 'refrigerator', 'showercurtrain', 'toilet',
+ 'sink', 'bathtub', 'garbagebin'),
+ with_label=False),
+ dict(type='Collect3D', keys=['points'])
+])
+lr = 0.008 # Learning rate of optimizers
+optimizer = dict( # Config used to build optimizer, support all the optimizers in PyTorch whose arguments are also the same as those in PyTorch
+ type='Adam', # Type of optimizers, refer to https://github.com/open-mmlab/mmcv/blob/v1.3.7/mmcv/runner/optimizer/default_constructor.py#L12 for more details
+ lr=0.008) # Learning rate of optimizers, see detail usages of the parameters in the documentation of PyTorch
+optimizer_config = dict( # Config used to build the optimizer hook, refer to https://github.com/open-mmlab/mmcv/blob/v1.3.7/mmcv/runner/hooks/optimizer.py#L22 for implementation details.
+ grad_clip=dict( # Config used to grad_clip
+ max_norm=10, # max norm of the gradients
+ norm_type=2)) # Type of the used p-norm. Can be 'inf' for infinity norm.
+lr_config = dict( # Learning rate scheduler config used to register LrUpdater hook
+ policy='step', # The policy of scheduler, also support CosineAnnealing, Cyclic, etc. Refer to details of supported LrUpdater from https://github.com/open-mmlab/mmcv/blob/v1.3.7/mmcv/runner/hooks/lr_updater.py#L9.
+ warmup=None, # The warmup policy, also support `exp` and `constant`.
+ step=[24, 32]) # Steps to decay the learning rate
+checkpoint_config = dict( # Config of set the checkpoint hook, Refer to https://github.com/open-mmlab/mmcv/blob/master/mmcv/runner/hooks/checkpoint.py for implementation.
+ interval=1) # The save interval is 1
+log_config = dict( # config of register logger hook
+ interval=50, # Interval to print the log
+ hooks=[dict(type='TextLoggerHook'),
+ dict(type='TensorboardLoggerHook')]) # The logger used to record the training process.
+runner = dict(type='EpochBasedRunner', max_epochs=36) # Runner that runs the `workflow` in total `max_epochs`
+dist_params = dict(backend='nccl') # Parameters to setup distributed training, the port can also be set.
+log_level = 'INFO' # The level of logging.
+find_unused_parameters = True # Whether to find unused parameters
+work_dir = None # Directory to save the model checkpoints and logs for the current experiments.
+load_from = None # load models as a pre-trained model from a given path. This will not resume training.
+resume_from = None # Resume checkpoints from a given path, the training will be resumed from the epoch when the checkpoint's is saved. The training state such as the epoch number and optimizer state will be restored.
+workflow = [('train', 1)] # Workflow for runner. [('train', 1)] means there is only one workflow and the workflow named 'train' is executed once. The workflow trains the model by 36 epochs according to the max_epochs.
+gpu_ids = range(0, 1) # ids of gpus
+```
+
+## FAQ
+
+### Ignore some fields in the base configs
+
+Sometimes, you may set `_delete_=True` to ignore some of fields in base configs.
+You may refer to [mmcv](https://mmcv.readthedocs.io/en/latest/utils.html#inherit-from-base-config-with-ignored-fields) for simple illustration.
+
+In MMDetection3D, for example, to change the FPN neck of PointPillars with the following config.
+
+```python
+model = dict(
+ type='MVXFasterRCNN',
+ pts_voxel_layer=dict(...),
+ pts_voxel_encoder=dict(...),
+ pts_middle_encoder=dict(...),
+ pts_backbone=dict(...),
+ pts_neck=dict(
+ type='FPN',
+ norm_cfg=dict(type='naiveSyncBN2d', eps=1e-3, momentum=0.01),
+ act_cfg=dict(type='ReLU'),
+ in_channels=[64, 128, 256],
+ out_channels=256,
+ start_level=0,
+ num_outs=3),
+ pts_bbox_head=dict(...))
+```
+
+`FPN` and `SECONDFPN` use different keywords to construct.
+
+```python
+_base_ = '../_base_/models/hv_pointpillars_fpn_nus.py'
+model = dict(
+ pts_neck=dict(
+ _delete_=True,
+ type='SECONDFPN',
+ norm_cfg=dict(type='naiveSyncBN2d', eps=1e-3, momentum=0.01),
+ in_channels=[64, 128, 256],
+ upsample_strides=[1, 2, 4],
+ out_channels=[128, 128, 128]),
+ pts_bbox_head=dict(...))
+```
+
+The `_delete_=True` would replace all old keys in `pts_neck` field with new keys.
+
+### Use intermediate variables in configs
+
+Some intermediate variables are used in the configs files, like `train_pipeline`/`test_pipeline` in datasets.
+It's worth noting that when modifying intermediate variables in the children configs, user needs to pass the intermediate variables into corresponding fields again.
+For example, we would like to use multi scale strategy to train and test a PointPillars. `train_pipeline`/`test_pipeline` are intermediate variable we would like modify.
+
+```python
+_base_ = './nus-3d.py'
+train_pipeline = [
+ dict(
+ type='LoadPointsFromFile',
+ load_dim=5,
+ use_dim=5,
+ file_client_args=file_client_args),
+ dict(
+ type='LoadPointsFromMultiSweeps',
+ sweeps_num=10,
+ file_client_args=file_client_args),
+ dict(type='LoadAnnotations3D', with_bbox_3d=True, with_label_3d=True),
+ dict(
+ type='GlobalRotScaleTrans',
+ rot_range=[-0.3925, 0.3925],
+ scale_ratio_range=[0.95, 1.05],
+ translation_std=[0, 0, 0]),
+ dict(type='RandomFlip3D', flip_ratio_bev_horizontal=0.5),
+ dict(type='PointsRangeFilter', point_cloud_range=point_cloud_range),
+ dict(type='ObjectRangeFilter', point_cloud_range=point_cloud_range),
+ dict(type='ObjectNameFilter', classes=class_names),
+ dict(type='PointShuffle'),
+ dict(type='DefaultFormatBundle3D', class_names=class_names),
+ dict(type='Collect3D', keys=['points', 'gt_bboxes_3d', 'gt_labels_3d'])
+]
+test_pipeline = [
+ dict(
+ type='LoadPointsFromFile',
+ load_dim=5,
+ use_dim=5,
+ file_client_args=file_client_args),
+ dict(
+ type='LoadPointsFromMultiSweeps',
+ sweeps_num=10,
+ file_client_args=file_client_args),
+ dict(
+ type='MultiScaleFlipAug3D',
+ img_scale=(1333, 800),
+ pts_scale_ratio=[0.95, 1.0, 1.05],
+ flip=False,
+ transforms=[
+ dict(
+ type='GlobalRotScaleTrans',
+ rot_range=[0, 0],
+ scale_ratio_range=[1., 1.],
+ translation_std=[0, 0, 0]),
+ dict(type='RandomFlip3D'),
+ dict(
+ type='PointsRangeFilter', point_cloud_range=point_cloud_range),
+ dict(
+ type='DefaultFormatBundle3D',
+ class_names=class_names,
+ with_label=False),
+ dict(type='Collect3D', keys=['points'])
+ ])
+]
+data = dict(
+ train=dict(pipeline=train_pipeline),
+ val=dict(pipeline=test_pipeline),
+ test=dict(pipeline=test_pipeline))
+```
+
+We first define the new `train_pipeline`/`test_pipeline` and pass them into `data`.
diff --git a/docs/en/tutorials/coord_sys_tutorial.md b/docs/en/tutorials/coord_sys_tutorial.md
new file mode 100644
index 0000000..4ddb2b8
--- /dev/null
+++ b/docs/en/tutorials/coord_sys_tutorial.md
@@ -0,0 +1,240 @@
+# Tutorial 6: Coordinate System
+
+## Overview
+
+MMDetection3D uses three different coordinate systems. The existence of different coordinate systems in the society of 3D object detection is necessary, because for various 3D data collection devices, such as LiDAR, depth camera, etc., the coordinate systems are not consistent, and different 3D datasets also follow different data formats. Early works, such as SECOND, VoteNet, convert the raw data to another format, forming conventions that some later works also follow, making the conversion between coordinate systems even more complicated.
+
+Despite the variety of datasets and equipment, by summarizing the line of works on 3D object detection we can roughly categorize coordinate systems into three:
+
+- Camera coordinate system -- the coordinate system of most cameras, in which the positive direction of the y-axis points to the ground, the positive direction of the x-axis points to the right, and the positive direction of the z-axis points to the front.
+ ```
+ up z front
+ | ^
+ | /
+ | /
+ | /
+ |/
+ left ------ 0 ------> x right
+ |
+ |
+ |
+ |
+ v
+ y down
+ ```
+- LiDAR coordinate system -- the coordinate system of many LiDARs, in which the negative direction of the z-axis points to the ground, the positive direction of the x-axis points to the front, and the positive direction of the y-axis points to the left.
+ ```
+ z up x front
+ ^ ^
+ | /
+ | /
+ | /
+ |/
+ y left <------ 0 ------ right
+ ```
+- Depth coordinate system -- the coordinate system used by VoteNet, H3DNet, etc., in which the negative direction of the z-axis points to the ground, the positive direction of the x-axis points to the right, and the positive direction of the y-axis points to the front.
+ ```
+ z up y front
+ ^ ^
+ | /
+ | /
+ | /
+ |/
+ left ------ 0 ------> x right
+ ```
+
+The definition of coordinate systems in this tutorial is actually **more than just defining the three axes**. For a box in the form of `` $$`(x, y, z, dx, dy, dz, r)`$$ ``, our coordinate systems also define how to interpret the box dimensions `` $$`(dx, dy, dz)`$$ `` and the yaw angle `` $$`r`$$ ``.
+
+The illustration of the three coordinate systems is shown below:
+
+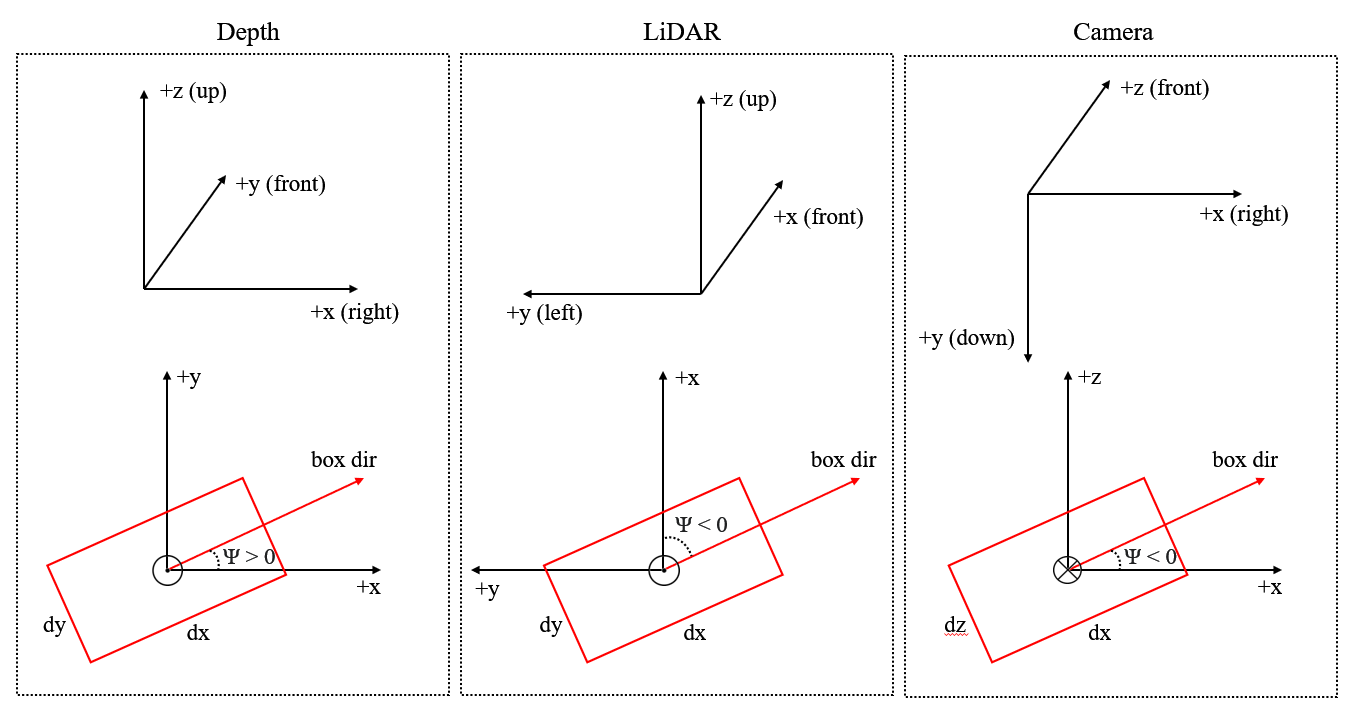
+
+The three figures above are the 3D coordinate systems while the three figures below are the bird's eye view.
+
+We will stick to the three coordinate systems defined in this tutorial in the future.
+
+## Definition of the yaw angle
+
+Please refer to [wikipedia](https://en.wikipedia.org/wiki/Euler_angles#Tait%E2%80%93Bryan_angles) for the standard definition of the yaw angle. In object detection, we choose an axis as the gravity axis, and a reference direction on the plane `` $$`\Pi`$$ `` perpendicular to the gravity axis, then the reference direction has a yaw angle of 0, and other directions on `` $$`\Pi`$$ `` have non-zero yaw angles depending on its angle with the reference direction.
+
+Currently, for all supported datasets, annotations do not include pitch angle and roll angle, which means we need only consider the yaw angle when predicting boxes and calculating overlap between boxes.
+
+In MMDetection3D, all three coordinate systems are right-handed coordinate systems, which means the ascending direction of the yaw angle is counter-clockwise if viewed from the negative direction of the gravity axis (the axis is pointing at one's eyes).
+
+The figure below shows that, in this right-handed coordinate system, if we set the positive direction of the x-axis as a reference direction, then the positive direction of the y-axis has a yaw angle of `` $$`\frac{\pi}{2}`$$ ``.
+
+```
+ z up y front (yaw=0.5*pi)
+ ^ ^
+ | /
+ | /
+ | /
+ |/
+left (yaw=pi) ------ 0 ------> x right (yaw=0)
+```
+
+For a box, the value of its yaw angle equals its direction minus a reference direction. In all three coordinate systems in MMDetection3D, the reference direction is always the positive direction of the x-axis, while the direction of a box is defined to be parallel with the x-axis if its yaw angle is 0. The definition of the yaw angle of a box is illustrated in the figure below.
+
+```
+y front
+ ^ box direction (yaw=0.5*pi)
+ /|\ ^
+ | /|\
+ | ____|____
+ | | | |
+ | | | |
+__|____|____|____|______\ x right
+ | | | | /
+ | | | |
+ | |____|____|
+ |
+```
+
+## Definition of the box dimensions
+
+The definition of the box dimensions cannot be disentangled with the definition of the yaw angle. In the previous section, we said that the direction of a box is defined to be parallel with the x-axis if its yaw angle is 0. Then naturally, the dimension of a box which corresponds to the x-axis should be `` $$`dx`$$ ``. However, this is not always the case in some datasets (we will address that later).
+
+The following figures show the meaning of the correspondence between the x-axis and `` $$`dx`$$ ``, and between the y-axis and `` $$`dy`$$ ``.
+
+```
+y front
+ ^ box direction (yaw=0.5*pi)
+ /|\ ^
+ | /|\
+ | ____|____
+ | | | |
+ | | | | dx
+__|____|____|____|______\ x right
+ | | | | /
+ | | | |
+ | |____|____|
+ | dy
+```
+
+Note that the box direction is always parallel with the edge `` $$`dx`$$ ``.
+
+```
+y front
+ ^ _________
+ /|\ | | |
+ | | | |
+ | | | | dy
+ | |____|____|____\ box direction (yaw=0)
+ | | | | /
+__|____|____|____|_________\ x right
+ | | | | /
+ | |____|____|
+ | dx
+ |
+```
+
+## Relation with raw coordinate systems of supported datasets
+
+### KITTI
+
+The raw annotation of KITTI is under camera coordinate system, see [get_label_anno](https://github.com/open-mmlab/mmdetection3d/blob/master/tools/data_converter/kitti_data_utils.py). In MMDetection3D, to train LiDAR-based models on KITTI, the data is first converted from camera coordinate system to LiDAR coordinate system, see [get_ann_info](https://github.com/open-mmlab/mmdetection3d/blob/master/mmdet3d/datasets/kitti_dataset.py). For training vision-based models, the data is kept in the camera coordinate system.
+
+In SECOND, the LiDAR coordinate system for a box is defined as follows (a bird's eye view):
+
+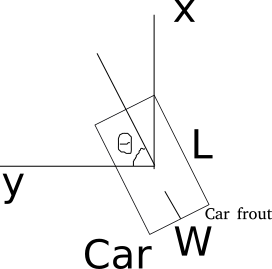
+
+For each box, the dimensions are `` $$`(w, l, h)`$$ ``, and the reference direction for the yaw angle is the positive direction of the y axis. For more details, refer to the [repo](https://github.com/traveller59/second.pytorch#concepts).
+
+Our LiDAR coordinate system has two changes:
+
+- The yaw angle is defined to be right-handed instead of left-handed for consistency;
+- The box dimensions are `` $$`(l, w, h)`$$ `` instead of `` $$`(w, l, h)`$$ ``, since `` $$`w`$$ `` corresponds to `` $$`dy`$$ `` and `` $$`l`$$ `` corresponds to `` $$`dx`$$ `` in KITTI.
+
+### Waymo
+
+We use the KITTI-format data of Waymo dataset. Therefore, KITTI and Waymo also share the same coordinate system in our implementation.
+
+### NuScenes
+
+NuScenes provides a toolkit for evaluation, in which each box is wrapped into a `Box` instance. The coordinate system of `Box` is different from our LiDAR coordinate system in that the first two elements of the box dimension correspond to `` $$`(dy, dx)`$$ ``, or `` $$`(w, l)`$$ ``, respectively, instead of the reverse. For more details, please refer to the NuScenes [tutorial](https://github.com/open-mmlab/mmdetection3d/blob/master/docs/en/datasets/nuscenes_det.md#notes).
+
+Readers may refer to the [NuScenes development kit](https://github.com/nutonomy/nuscenes-devkit/tree/master/python-sdk/nuscenes/eval/detection) for the definition of a [NuScenes box](https://github.com/nutonomy/nuscenes-devkit/blob/2c6a752319f23910d5f55cc995abc547a9e54142/python-sdk/nuscenes/utils/data_classes.py#L457) and implementation of [NuScenes evaluation](https://github.com/nutonomy/nuscenes-devkit/blob/master/python-sdk/nuscenes/eval/detection/evaluate.py).
+
+### Lyft
+
+Lyft shares the same data format with NuScenes as far as coordinate system is involved.
+
+Please refer to the [official website](https://www.kaggle.com/c/3d-object-detection-for-autonomous-vehicles/data) for more information.
+
+### ScanNet
+
+The raw data of ScanNet is not point cloud but mesh. The sampled point cloud data is under our depth coordinate system. For ScanNet detection task, the box annotations are axis-aligned, and the yaw angle is always zero. Therefore the direction of the yaw angle in our depth coordinate system makes no difference regarding ScanNet.
+
+### SUN RGB-D
+
+The raw data of SUN RGB-D is not point cloud but RGB-D image. By back projection, we obtain the corresponding point cloud for each image, which is under our Depth coordinate system. However, the annotation is not under our system and thus needs conversion.
+
+For the conversion from raw annotation to annotation under our Depth coordinate system, please refer to [sunrgbd_data_utils.py](https://github.com/open-mmlab/mmdetection3d/blob/master/tools/data_converter/sunrgbd_data_utils.py).
+
+### S3DIS
+
+S3DIS shares the same coordinate system as ScanNet in our implementation. However, S3DIS is a segmentation-task-only dataset, and thus no annotation is coordinate system sensitive.
+
+## Examples
+
+### Box conversion (between different coordinate systems)
+
+Take the conversion between our Camera coordinate system and LiDAR coordinate system as an example:
+
+First, for points and box centers, the coordinates before and after the conversion satisfy the following relationship:
+
+- `` $$`x_{LiDAR}=z_{camera}`$$ ``
+- `` $$`y_{LiDAR}=-x_{camera}`$$ ``
+- `` $$`z_{LiDAR}=-y_{camera}`$$ ``
+
+Then, the box dimensions before and after the conversion satisfy the following relationship:
+
+- `` $$`dx_{LiDAR}=dx_{camera}`$$ ``
+- `` $$`dy_{LiDAR}=dz_{camera}`$$ ``
+- `` $$`dz_{LiDAR}=dy_{camera}`$$ ``
+
+Finally, the yaw angle should also be converted:
+
+- `` $$`r_{LiDAR}=-\frac{\pi}{2}-r_{camera}`$$ ``
+
+See the code [here](https://github.com/open-mmlab/mmdetection3d/blob/master/mmdet3d/core/bbox/structures/box_3d_mode.py) for more details.
+
+### Bird's Eye View
+
+The BEV of a camera coordinate system box is `` $$`(x, z, dx, dz, -r)`$$ `` if the 3D box is `` $$`(x, y, z, dx, dy, dz, r)`$$ ``. The inversion of the sign of the yaw angle is because the positive direction of the gravity axis of the Camera coordinate system points to the ground.
+
+See the code [here](https://github.com/open-mmlab/mmdetection3d/blob/master/mmdet3d/core/bbox/structures/cam_box3d.py) for more details.
+
+### Rotation of boxes
+
+We set the rotation of all kinds of boxes to be counter-clockwise about the gravity axis. Therefore, to rotate a 3D box we first calculate the new box center, and then we add the rotation angle to the yaw angle.
+
+See the code [here](https://github.com/open-mmlab/mmdetection3d/blob/master/mmdet3d/core/bbox/structures/cam_box3d.py) for more details.
+
+## Common FAQ
+
+#### Q1: Are the box related ops universal to all coordinate system types?
+
+No. For example, [RoI-Aware Pooling ops](https://github.com/open-mmlab/mmcv/blob/master/mmcv/ops/roiaware_pool3d.py) is applicable to boxes under Depth or LiDAR coordinate system only. The evaluation functions for KITTI dataset [here](https://github.com/open-mmlab/mmdetection3d/blob/master/mmdet3d/core/evaluation/kitti_utils) are only applicable to boxes under Camera coordinate system since the rotation is clockwise if viewed from above.
+
+For each box related op, we have marked the type of boxes to which we can apply the op.
+
+#### Q2: In every coordinate system, do the three axes point exactly to the right, the front, and the ground, respectively?
+
+No. For example, in KITTI, we need a calibration matrix when converting from Camera coordinate system to LiDAR coordinate system.
+
+#### Q3: How does a phase difference of `` $$`2\pi`$$ `` in the yaw angle of a box affect evaluation?
+
+For IoU calculation, a phase difference of `` $$`2\pi`$$ `` in the yaw angle will result in the same box, thus not affecting evaluation.
+
+For angle prediction evaluation such as the NDS metric in NuScenes and the AOS metric in KITTI, the angle of predicted boxes will be first standardized, so the phase difference of `` $$`2\pi`$$ `` will not change the result.
+
+#### Q4: How does a phase difference of `` $$`\pi`$$ `` in the yaw angle of a box affect evaluation?
+
+For IoU calculation, a phase difference of `` $$`\pi`$$ `` in the yaw angle will result in the same box, thus not affecting evaluation.
+
+However, for angle prediction evaluation, this will result in the exact opposite direction.
+
+Just think about a car. The yaw angle is the angle between the direction of the car front and the positive direction of the x-axis. If we add `` $$`\pi`$$ `` to this angle, the car front will become the car rear.
+
+For categories such as barrier, the front and the rear have no difference, therefore a phase difference of `` $$`\pi`$$ `` will not affect the angle prediction score.
diff --git a/docs/en/tutorials/customize_dataset.md b/docs/en/tutorials/customize_dataset.md
new file mode 100644
index 0000000..772cd0a
--- /dev/null
+++ b/docs/en/tutorials/customize_dataset.md
@@ -0,0 +1,367 @@
+# Tutorial 2: Customize Datasets
+
+## Support new data format
+
+To support a new data format, you can either convert them to existing formats or directly convert them to the middle format. You could also choose to convert them offline (before training by a script) or online (implement a new dataset and do the conversion at training). In MMDetection3D, for the data that is inconvenient to read directly online, we recommend to convert it into KITTI format and do the conversion offline, thus you only need to modify the config's data annotation paths and classes after the conversion.
+For data sharing similar format with existing datasets, like Lyft compared to nuScenes, we recommend to directly implement data converter and dataset class. During the procedure, inheritation could be taken into consideration to reduce the implementation workload.
+
+### Reorganize new data formats to existing format
+
+For data that is inconvenient to read directly online, the simplest way is to convert your dataset to existing dataset formats.
+
+Typically we need a data converter to reorganize the raw data and convert the annotation format into KITTI style. Then a new dataset class inherited from existing ones is sometimes necessary for dealing with some specific differences between datasets. Finally, the users need to further modify the config files to use the dataset. An [example](https://mmdetection3d.readthedocs.io/en/latest/2_new_data_model.html) training predefined models on Waymo dataset by converting it into KITTI style can be taken for reference.
+
+### Reorganize new data format to middle format
+
+It is also fine if you do not want to convert the annotation format to existing formats.
+Actually, we convert all the supported datasets into pickle files, which summarize useful information for model training and inference.
+
+The annotation of a dataset is a list of dict, each dict corresponds to a frame.
+A basic example (used in KITTI) is as follows. A frame consists of several keys, like `image`, `point_cloud`, `calib` and `annos`.
+As long as we could directly read data according to these information, the organization of raw data could also be different from existing ones.
+With this design, we provide an alternative choice for customizing datasets.
+
+```python
+
+[
+ {'image': {'image_idx': 0, 'image_path': 'training/image_2/000000.png', 'image_shape': array([ 370, 1224], dtype=int32)},
+ 'point_cloud': {'num_features': 4, 'velodyne_path': 'training/velodyne/000000.bin'},
+ 'calib': {'P0': array([[707.0493, 0. , 604.0814, 0. ],
+ [ 0. , 707.0493, 180.5066, 0. ],
+ [ 0. , 0. , 1. , 0. ],
+ [ 0. , 0. , 0. , 1. ]]),
+ 'P1': array([[ 707.0493, 0. , 604.0814, -379.7842],
+ [ 0. , 707.0493, 180.5066, 0. ],
+ [ 0. , 0. , 1. , 0. ],
+ [ 0. , 0. , 0. , 1. ]]),
+ 'P2': array([[ 7.070493e+02, 0.000000e+00, 6.040814e+02, 4.575831e+01],
+ [ 0.000000e+00, 7.070493e+02, 1.805066e+02, -3.454157e-01],
+ [ 0.000000e+00, 0.000000e+00, 1.000000e+00, 4.981016e-03],
+ [ 0.000000e+00, 0.000000e+00, 0.000000e+00, 1.000000e+00]]),
+ 'P3': array([[ 7.070493e+02, 0.000000e+00, 6.040814e+02, -3.341081e+02],
+ [ 0.000000e+00, 7.070493e+02, 1.805066e+02, 2.330660e+00],
+ [ 0.000000e+00, 0.000000e+00, 1.000000e+00, 3.201153e-03],
+ [ 0.000000e+00, 0.000000e+00, 0.000000e+00, 1.000000e+00]]),
+ 'R0_rect': array([[ 0.9999128 , 0.01009263, -0.00851193, 0. ],
+ [-0.01012729, 0.9999406 , -0.00403767, 0. ],
+ [ 0.00847068, 0.00412352, 0.9999556 , 0. ],
+ [ 0. , 0. , 0. , 1. ]]),
+ 'Tr_velo_to_cam': array([[ 0.00692796, -0.9999722 , -0.00275783, -0.02457729],
+ [-0.00116298, 0.00274984, -0.9999955 , -0.06127237],
+ [ 0.9999753 , 0.00693114, -0.0011439 , -0.3321029 ],
+ [ 0. , 0. , 0. , 1. ]]),
+ 'Tr_imu_to_velo': array([[ 9.999976e-01, 7.553071e-04, -2.035826e-03, -8.086759e-01],
+ [-7.854027e-04, 9.998898e-01, -1.482298e-02, 3.195559e-01],
+ [ 2.024406e-03, 1.482454e-02, 9.998881e-01, -7.997231e-01],
+ [ 0.000000e+00, 0.000000e+00, 0.000000e+00, 1.000000e+00]])},
+ 'annos': {'name': array(['Pedestrian'], dtype=' 0
+ loss = torch.abs(pred - target)
+ return loss
+
+@LOSSES.register_module()
+class MyLoss(nn.Module):
+
+ def __init__(self, reduction='mean', loss_weight=1.0):
+ super(MyLoss, self).__init__()
+ self.reduction = reduction
+ self.loss_weight = loss_weight
+
+ def forward(self,
+ pred,
+ target,
+ weight=None,
+ avg_factor=None,
+ reduction_override=None):
+ assert reduction_override in (None, 'none', 'mean', 'sum')
+ reduction = (
+ reduction_override if reduction_override else self.reduction)
+ loss_bbox = self.loss_weight * my_loss(
+ pred, target, weight, reduction=reduction, avg_factor=avg_factor)
+ return loss_bbox
+```
+
+Then the users need to add it in the `mmdet3d/models/losses/__init__.py`.
+
+```python
+from .my_loss import MyLoss, my_loss
+
+```
+
+Alternatively, you can add
+
+```python
+custom_imports=dict(
+ imports=['mmdet3d.models.losses.my_loss'])
+```
+
+to the config file and achieve the same goal.
+
+To use it, modify the `loss_xxx` field.
+Since MyLoss is for regression, you need to modify the `loss_bbox` field in the head.
+
+```python
+loss_bbox=dict(type='MyLoss', loss_weight=1.0))
+```
diff --git a/docs/en/tutorials/customize_runtime.md b/docs/en/tutorials/customize_runtime.md
new file mode 100644
index 0000000..8b5596e
--- /dev/null
+++ b/docs/en/tutorials/customize_runtime.md
@@ -0,0 +1,333 @@
+# Tutorial 5: Customize Runtime Settings
+
+## Customize optimization settings
+
+### Customize optimizer supported by PyTorch
+
+We already support to use all the optimizers implemented by PyTorch, and the only modification is to change the `optimizer` field of config files.
+For example, if you want to use `ADAM` (note that the performance could drop a lot), the modification could be as the following.
+
+```python
+optimizer = dict(type='Adam', lr=0.0003, weight_decay=0.0001)
+```
+
+To modify the learning rate of the model, the users only need to modify the `lr` in the config of optimizer. The users can directly set arguments following the [API doc](https://pytorch.org/docs/stable/optim.html?highlight=optim#module-torch.optim) of PyTorch.
+
+### Customize self-implemented optimizer
+
+#### 1. Define a new optimizer
+
+A customized optimizer could be defined as following.
+
+Assume you want to add a optimizer named `MyOptimizer`, which has arguments `a`, `b`, and `c`.
+You need to create a new directory named `mmdet3d/core/optimizer`.
+And then implement the new optimizer in a file, e.g., in `mmdet3d/core/optimizer/my_optimizer.py`:
+
+```python
+from mmcv.runner.optimizer import OPTIMIZERS
+from torch.optim import Optimizer
+
+
+@OPTIMIZERS.register_module()
+class MyOptimizer(Optimizer):
+
+ def __init__(self, a, b, c)
+
+```
+
+#### 2. Add the optimizer to registry
+
+To find the above module defined above, this module should be imported into the main namespace at first. There are two options to achieve it.
+
+- Add `mmdet3d/core/optimizer/__init__.py` to import it.
+
+ The newly defined module should be imported in `mmdet3d/core/optimizer/__init__.py` so that the registry will
+ find the new module and add it:
+
+```python
+from .my_optimizer import MyOptimizer
+
+__all__ = ['MyOptimizer']
+
+```
+
+You also need to import `optimizer` in `mmdet3d/core/__init__.py` by adding:
+
+```python
+from .optimizer import *
+```
+
+Or use `custom_imports` in the config to manually import it
+
+```python
+custom_imports = dict(imports=['mmdet3d.core.optimizer.my_optimizer'], allow_failed_imports=False)
+```
+
+The module `mmdet3d.core.optimizer.my_optimizer` will be imported at the beginning of the program and the class `MyOptimizer` is then automatically registered.
+Note that only the package containing the class `MyOptimizer` should be imported.
+`mmdet3d.core.optimizer.my_optimizer.MyOptimizer` **cannot** be imported directly.
+
+Actually users can use a totally different file directory structure in this importing method, as long as the module root can be located in `PYTHONPATH`.
+
+#### 3. Specify the optimizer in the config file
+
+Then you can use `MyOptimizer` in `optimizer` field of config files.
+In the configs, the optimizers are defined by the field `optimizer` like the following:
+
+```python
+optimizer = dict(type='SGD', lr=0.02, momentum=0.9, weight_decay=0.0001)
+```
+
+To use your own optimizer, the field can be changed to
+
+```python
+optimizer = dict(type='MyOptimizer', a=a_value, b=b_value, c=c_value)
+```
+
+### Customize optimizer constructor
+
+Some models may have some parameter-specific settings for optimization, e.g. weight decay for BatchNorm layers.
+The users can tune those fine-grained parameters through customizing optimizer constructor.
+
+```python
+from mmcv.utils import build_from_cfg
+
+from mmcv.runner.optimizer import OPTIMIZER_BUILDERS, OPTIMIZERS
+from mmdet.utils import get_root_logger
+from .my_optimizer import MyOptimizer
+
+
+@OPTIMIZER_BUILDERS.register_module()
+class MyOptimizerConstructor(object):
+
+ def __init__(self, optimizer_cfg, paramwise_cfg=None):
+
+ def __call__(self, model):
+
+ return my_optimizer
+
+```
+
+The default optimizer constructor is implemented [here](https://github.com/open-mmlab/mmcv/blob/v1.3.7/mmcv/runner/optimizer/default_constructor.py#L11), which could also serve as a template for new optimizer constructor.
+
+### Additional settings
+
+Tricks not implemented by the optimizer should be implemented through optimizer constructor (e.g., set parameter-wise learning rates) or hooks. We list some common settings that could stabilize the training or accelerate the training. Feel free to create PR, issue for more settings.
+
+- __Use gradient clip to stabilize training__:
+
+ Some models need gradient clip to clip the gradients to stabilize the training process. An example is as below:
+
+ ```python
+ optimizer_config = dict(
+ _delete_=True, grad_clip=dict(max_norm=35, norm_type=2))
+ ```
+
+ If your config inherits the base config which already sets the `optimizer_config`, you might need `_delete_=True` to override the unnecessary settings in the base config. See the [config documentation](https://mmdetection.readthedocs.io/en/latest/tutorials/config.html) for more details.
+
+- __Use momentum schedule to accelerate model convergence__:
+
+ We support momentum scheduler to modify model's momentum according to learning rate, which could make the model converge in a faster way.
+ Momentum scheduler is usually used with LR scheduler, for example, the following config is used in 3D detection to accelerate convergence.
+ For more details, please refer to the implementation of [CyclicLrUpdater](https://github.com/open-mmlab/mmcv/blob/v1.3.7/mmcv/runner/hooks/lr_updater.py#L358) and [CyclicMomentumUpdater](https://github.com/open-mmlab/mmcv/blob/v1.3.7/mmcv/runner/hooks/momentum_updater.py#L225).
+
+ ```python
+ lr_config = dict(
+ policy='cyclic',
+ target_ratio=(10, 1e-4),
+ cyclic_times=1,
+ step_ratio_up=0.4,
+ )
+ momentum_config = dict(
+ policy='cyclic',
+ target_ratio=(0.85 / 0.95, 1),
+ cyclic_times=1,
+ step_ratio_up=0.4,
+ )
+ ```
+
+## Customize training schedules
+
+By default we use step learning rate with 1x schedule, this calls [`StepLRHook`](https://github.com/open-mmlab/mmcv/blob/v1.3.7/mmcv/runner/hooks/lr_updater.py#L167) in MMCV.
+We support many other learning rate schedule [here](https://github.com/open-mmlab/mmcv/blob/v1.3.7/mmcv/runner/hooks/lr_updater.py), such as `CosineAnnealing` and `Poly` schedule. Here are some examples
+
+- Poly schedule:
+
+ ```python
+ lr_config = dict(policy='poly', power=0.9, min_lr=1e-4, by_epoch=False)
+ ```
+
+- ConsineAnnealing schedule:
+
+ ```python
+ lr_config = dict(
+ policy='CosineAnnealing',
+ warmup='linear',
+ warmup_iters=1000,
+ warmup_ratio=1.0 / 10,
+ min_lr_ratio=1e-5)
+ ```
+
+## Customize workflow
+
+Workflow is a list of (phase, epochs) to specify the running order and epochs.
+By default it is set to be
+
+```python
+workflow = [('train', 1)]
+```
+
+which means running 1 epoch for training.
+Sometimes user may want to check some metrics (e.g. loss, accuracy) about the model on the validate set.
+In such case, we can set the workflow as
+
+```python
+[('train', 1), ('val', 1)]
+```
+
+so that 1 epoch for training and 1 epoch for validation will be run iteratively.
+
+**Note**:
+
+1. The parameters of model will not be updated during val epoch.
+2. Keyword `max_epochs` in `runner` in the config only controls the number of training epochs and will not affect the validation workflow.
+3. Workflows `[('train', 1), ('val', 1)]` and `[('train', 1)]` will not change the behavior of `EvalHook` because `EvalHook` is called by `after_train_epoch` and validation workflow only affect hooks that are called through `after_val_epoch`. Therefore, the only difference between `[('train', 1), ('val', 1)]` and `[('train', 1)]` is that the runner will calculate losses on validation set after each training epoch.
+
+## Customize hooks
+
+### Customize self-implemented hooks
+
+#### 1. Implement a new hook
+
+There are some occasions when the users might need to implement a new hook. MMDetection supports customized hooks in training (#3395) since v2.3.0. Thus the users could implement a hook directly in mmdet or their mmdet-based codebases and use the hook by only modifying the config in training.
+Before v2.3.0, the users need to modify the code to get the hook registered before training starts.
+Here we give an example of creating a new hook in mmdet3d and using it in training.
+
+```python
+from mmcv.runner import HOOKS, Hook
+
+
+@HOOKS.register_module()
+class MyHook(Hook):
+
+ def __init__(self, a, b):
+ pass
+
+ def before_run(self, runner):
+ pass
+
+ def after_run(self, runner):
+ pass
+
+ def before_epoch(self, runner):
+ pass
+
+ def after_epoch(self, runner):
+ pass
+
+ def before_iter(self, runner):
+ pass
+
+ def after_iter(self, runner):
+ pass
+```
+
+Depending on the functionality of the hook, the users need to specify what the hook will do at each stage of the training in `before_run`, `after_run`, `before_epoch`, `after_epoch`, `before_iter`, and `after_iter`.
+
+#### 2. Register the new hook
+
+Then we need to make `MyHook` imported. Assuming the hook is in `mmdet3d/core/utils/my_hook.py` there are two ways to do that:
+
+- Modify `mmdet3d/core/utils/__init__.py` to import it.
+
+ The newly defined module should be imported in `mmdet3d/core/utils/__init__.py` so that the registry will
+ find the new module and add it:
+
+```python
+from .my_hook import MyHook
+
+__all__ = [..., 'MyHook']
+
+```
+
+Or use `custom_imports` in the config to manually import it
+
+```python
+custom_imports = dict(imports=['mmdet3d.core.utils.my_hook'], allow_failed_imports=False)
+```
+
+#### 3. Modify the config
+
+```python
+custom_hooks = [
+ dict(type='MyHook', a=a_value, b=b_value)
+]
+```
+
+You can also set the priority of the hook by setting key `priority` to `'NORMAL'` or `'HIGHEST'` as below
+
+```python
+custom_hooks = [
+ dict(type='MyHook', a=a_value, b=b_value, priority='NORMAL')
+]
+```
+
+By default the hook's priority is set as `NORMAL` during registration.
+
+### Use hooks implemented in MMCV
+
+If the hook is already implemented in MMCV, you can directly modify the config to use the hook as below
+
+```python
+custom_hooks = [
+ dict(type='MyHook', a=a_value, b=b_value, priority='NORMAL')
+]
+```
+
+### Modify default runtime hooks
+
+There are some common hooks that are not registered through `custom_hooks`, they are
+
+- log_config
+- checkpoint_config
+- evaluation
+- lr_config
+- optimizer_config
+- momentum_config
+
+In those hooks, only the logger hook has the `VERY_LOW` priority, others' priority are `NORMAL`.
+The above-mentioned tutorials already covers how to modify `optimizer_config`, `momentum_config`, and `lr_config`.
+Here we reveal what we can do with `log_config`, `checkpoint_config`, and `evaluation`.
+
+#### Checkpoint config
+
+The MMCV runner will use `checkpoint_config` to initialize [`CheckpointHook`](https://github.com/open-mmlab/mmcv/blob/v1.3.7/mmcv/runner/hooks/checkpoint.py#L9).
+
+```python
+checkpoint_config = dict(interval=1)
+```
+
+The users could set `max_keep_ckpts` to save only small number of checkpoints or decide whether to store state dict of optimizer by `save_optimizer`. More details of the arguments are [here](https://mmcv.readthedocs.io/en/latest/api.html#mmcv.runner.CheckpointHook).
+
+#### Log config
+
+The `log_config` wraps multiple logger hooks and enables to set intervals. Now MMCV supports `WandbLoggerHook`, `MlflowLoggerHook`, and `TensorboardLoggerHook`.
+The detailed usages can be found in the [docs](https://mmcv.readthedocs.io/en/latest/api.html#mmcv.runner.LoggerHook).
+
+```python
+log_config = dict(
+ interval=50,
+ hooks=[
+ dict(type='TextLoggerHook'),
+ dict(type='TensorboardLoggerHook')
+ ])
+```
+
+#### Evaluation config
+
+The config of `evaluation` will be used to initialize the [`EvalHook`](https://github.com/open-mmlab/mmdetection/blob/v2.13.0/mmdet/core/evaluation/eval_hooks.py#L9).
+Except the key `interval`, other arguments such as `metric` will be passed to the `dataset.evaluate()`.
+
+```python
+evaluation = dict(interval=1, metric='bbox')
+```
diff --git a/docs/en/tutorials/data_pipeline.md b/docs/en/tutorials/data_pipeline.md
new file mode 100644
index 0000000..60dc187
--- /dev/null
+++ b/docs/en/tutorials/data_pipeline.md
@@ -0,0 +1,198 @@
+# Tutorial 3: Customize Data Pipelines
+
+## Design of Data pipelines
+
+Following typical conventions, we use `Dataset` and `DataLoader` for data loading
+with multiple workers. `Dataset` returns a dict of data items corresponding
+the arguments of models' forward method.
+Since the data in object detection may not be the same size (point number, gt bbox size, etc.),
+we introduce a new `DataContainer` type in MMCV to help collect and distribute
+data of different size.
+See [here](https://github.com/open-mmlab/mmcv/blob/master/mmcv/parallel/data_container.py) for more details.
+
+The data preparation pipeline and the dataset is decomposed. Usually a dataset
+defines how to process the annotations and a data pipeline defines all the steps to prepare a data dict.
+A pipeline consists of a sequence of operations. Each operation takes a dict as input and also output a dict for the next transform.
+
+We present a classical pipeline in the following figure. The blue blocks are pipeline operations. With the pipeline going on, each operator can add new keys (marked as green) to the result dict or update the existing keys (marked as orange).
+
+
+The operations are categorized into data loading, pre-processing, formatting and test-time augmentation.
+
+Here is an pipeline example for PointPillars.
+
+```python
+train_pipeline = [
+ dict(
+ type='LoadPointsFromFile',
+ load_dim=5,
+ use_dim=5,
+ file_client_args=file_client_args),
+ dict(
+ type='LoadPointsFromMultiSweeps',
+ sweeps_num=10,
+ file_client_args=file_client_args),
+ dict(type='LoadAnnotations3D', with_bbox_3d=True, with_label_3d=True),
+ dict(
+ type='GlobalRotScaleTrans',
+ rot_range=[-0.3925, 0.3925],
+ scale_ratio_range=[0.95, 1.05],
+ translation_std=[0, 0, 0]),
+ dict(type='RandomFlip3D', flip_ratio_bev_horizontal=0.5),
+ dict(type='PointsRangeFilter', point_cloud_range=point_cloud_range),
+ dict(type='ObjectRangeFilter', point_cloud_range=point_cloud_range),
+ dict(type='ObjectNameFilter', classes=class_names),
+ dict(type='PointShuffle'),
+ dict(type='DefaultFormatBundle3D', class_names=class_names),
+ dict(type='Collect3D', keys=['points', 'gt_bboxes_3d', 'gt_labels_3d'])
+]
+test_pipeline = [
+ dict(
+ type='LoadPointsFromFile',
+ load_dim=5,
+ use_dim=5,
+ file_client_args=file_client_args),
+ dict(
+ type='LoadPointsFromMultiSweeps',
+ sweeps_num=10,
+ file_client_args=file_client_args),
+ dict(
+ type='MultiScaleFlipAug',
+ img_scale=(1333, 800),
+ pts_scale_ratio=1.0,
+ flip=False,
+ pcd_horizontal_flip=False,
+ pcd_vertical_flip=False,
+ transforms=[
+ dict(
+ type='GlobalRotScaleTrans',
+ rot_range=[0, 0],
+ scale_ratio_range=[1., 1.],
+ translation_std=[0, 0, 0]),
+ dict(type='RandomFlip3D'),
+ dict(
+ type='PointsRangeFilter', point_cloud_range=point_cloud_range),
+ dict(
+ type='DefaultFormatBundle3D',
+ class_names=class_names,
+ with_label=False),
+ dict(type='Collect3D', keys=['points'])
+ ])
+]
+```
+
+For each operation, we list the related dict fields that are added/updated/removed.
+
+### Data loading
+
+`LoadPointsFromFile`
+
+- add: points
+
+`LoadPointsFromMultiSweeps`
+
+- update: points
+
+`LoadAnnotations3D`
+
+- add: gt_bboxes_3d, gt_labels_3d, gt_bboxes, gt_labels, pts_instance_mask, pts_semantic_mask, bbox3d_fields, pts_mask_fields, pts_seg_fields
+
+### Pre-processing
+
+`GlobalRotScaleTrans`
+
+- add: pcd_trans, pcd_rotation, pcd_scale_factor
+- update: points, \*bbox3d_fields
+
+`RandomFlip3D`
+
+- add: flip, pcd_horizontal_flip, pcd_vertical_flip
+- update: points, \*bbox3d_fields
+
+`PointsRangeFilter`
+
+- update: points
+
+`ObjectRangeFilter`
+
+- update: gt_bboxes_3d, gt_labels_3d
+
+`ObjectNameFilter`
+
+- update: gt_bboxes_3d, gt_labels_3d
+
+`PointShuffle`
+
+- update: points
+
+`PointsRangeFilter`
+
+- update: points
+
+### Formatting
+
+`DefaultFormatBundle3D`
+
+- update: points, gt_bboxes_3d, gt_labels_3d, gt_bboxes, gt_labels
+
+`Collect3D`
+
+- add: img_meta (the keys of img_meta is specified by `meta_keys`)
+- remove: all other keys except for those specified by `keys`
+
+### Test time augmentation
+
+`MultiScaleFlipAug`
+
+- update: scale, pcd_scale_factor, flip, flip_direction, pcd_horizontal_flip, pcd_vertical_flip with list of augmented data with these specific parameters
+
+## Extend and use custom pipelines
+
+1. Write a new pipeline in any file, e.g., `my_pipeline.py`. It takes a dict as input and return a dict.
+
+ ```python
+ from mmdet.datasets import PIPELINES
+
+ @PIPELINES.register_module()
+ class MyTransform:
+
+ def __call__(self, results):
+ results['dummy'] = True
+ return results
+ ```
+
+2. Import the new class.
+
+ ```python
+ from .my_pipeline import MyTransform
+ ```
+
+3. Use it in config files.
+
+ ```python
+ train_pipeline = [
+ dict(
+ type='LoadPointsFromFile',
+ load_dim=5,
+ use_dim=5,
+ file_client_args=file_client_args),
+ dict(
+ type='LoadPointsFromMultiSweeps',
+ sweeps_num=10,
+ file_client_args=file_client_args),
+ dict(type='LoadAnnotations3D', with_bbox_3d=True, with_label_3d=True),
+ dict(
+ type='GlobalRotScaleTrans',
+ rot_range=[-0.3925, 0.3925],
+ scale_ratio_range=[0.95, 1.05],
+ translation_std=[0, 0, 0]),
+ dict(type='RandomFlip3D', flip_ratio_bev_horizontal=0.5),
+ dict(type='PointsRangeFilter', point_cloud_range=point_cloud_range),
+ dict(type='ObjectRangeFilter', point_cloud_range=point_cloud_range),
+ dict(type='ObjectNameFilter', classes=class_names),
+ dict(type='MyTransform'),
+ dict(type='PointShuffle'),
+ dict(type='DefaultFormatBundle3D', class_names=class_names),
+ dict(type='Collect3D', keys=['points', 'gt_bboxes_3d', 'gt_labels_3d'])
+ ]
+ ```
diff --git a/docs/en/tutorials/index.rst b/docs/en/tutorials/index.rst
new file mode 100644
index 0000000..866df3f
--- /dev/null
+++ b/docs/en/tutorials/index.rst
@@ -0,0 +1,12 @@
+.. toctree::
+ :maxdepth: 2
+
+ config.md
+ customize_dataset.md
+ data_pipeline.md
+ customize_models.md
+ customize_runtime.md
+ coord_sys_tutorial.md
+ backends_support.md
+ model_deployment.md
+ pure_point_cloud_dataset.md
diff --git a/docs/en/tutorials/model_deployment.md b/docs/en/tutorials/model_deployment.md
new file mode 100644
index 0000000..ff8c06a
--- /dev/null
+++ b/docs/en/tutorials/model_deployment.md
@@ -0,0 +1,121 @@
+# Tutorial 8: MMDetection3D model deployment
+
+To meet the speed requirement of the model in practical use, usually, we deploy the trained model to inference backends. [MMDeploy](https://github.com/open-mmlab/mmdeploy) is OpenMMLab model deployment framework. Now MMDeploy has supported MMDetection3D model deployment, and you can deploy the trained model to inference backends by MMDeploy.
+
+## Prerequisite
+
+### Install MMDeploy
+
+```bash
+git clone -b master git@github.com:open-mmlab/mmdeploy.git
+cd mmdeploy
+git submodule update --init --recursive
+```
+
+### Install backend and build custom ops
+
+According to MMDeploy documentation, choose to install the inference backend and build custom ops. Now supported inference backends for MMDetection3D include [OnnxRuntime](https://mmdeploy.readthedocs.io/en/latest/backends/onnxruntime.html), [TensorRT](https://mmdeploy.readthedocs.io/en/latest/backends/tensorrt.html), [OpenVINO](https://mmdeploy.readthedocs.io/en/latest/backends/openvino.html).
+
+## Export model
+
+Export the Pytorch model of MMDetection3D to the ONNX model file and the model file required by the backend. You could refer to MMDeploy docs [how to convert model](https://mmdeploy.readthedocs.io/en/latest/tutorials/how_to_convert_model.html).
+
+```bash
+python ./tools/deploy.py \
+ ${DEPLOY_CFG_PATH} \
+ ${MODEL_CFG_PATH} \
+ ${MODEL_CHECKPOINT_PATH} \
+ ${INPUT_IMG} \
+ --test-img ${TEST_IMG} \
+ --work-dir ${WORK_DIR} \
+ --calib-dataset-cfg ${CALIB_DATA_CFG} \
+ --device ${DEVICE} \
+ --log-level INFO \
+ --show \
+ --dump-info
+```
+
+### Description of all arguments
+
+- `deploy_cfg` : The path of deploy config file in MMDeploy codebase.
+- `model_cfg` : The path of model config file in OpenMMLab codebase.
+- `checkpoint` : The path of model checkpoint file.
+- `img` : The path of point cloud file or image file that used to convert model.
+- `--test-img` : The path of image file that used to test model. If not specified, it will be set to `None`.
+- `--work-dir` : The path of work directory that used to save logs and models.
+- `--calib-dataset-cfg` : Only valid in int8 mode. Config used for calibration. If not specified, it will be set to `None` and use "val" dataset in model config for calibration.
+- `--device` : The device used for conversion. If not specified, it will be set to `cpu`.
+- `--log-level` : To set log level which in `'CRITICAL', 'FATAL', 'ERROR', 'WARN', 'WARNING', 'INFO', 'DEBUG', 'NOTSET'`. If not specified, it will be set to `INFO`.
+- `--show` : Whether to show detection outputs.
+- `--dump-info` : Whether to output information for SDK.
+
+### Example
+
+```bash
+cd mmdeploy
+python tools/deploy.py \
+ configs/mmdet3d/voxel-detection/voxel-detection_tensorrt_dynamic-kitti.py \
+ ${$MMDET3D_DIR}/configs/pointpillars/hv_pointpillars_secfpn_6x8_160e_kitti-3d-3class.py \
+ ${$MMDET3D_DIR}/checkpoints/hv_pointpillars_secfpn_6x8_160e_kitti-3d-3class_20200620_230421-aa0f3adb.pth \
+ ${$MMDET3D_DIR}/demo/data/kitti/kitti_000008.bin \
+ --work-dir work-dir \
+ --device cuda:0 \
+ --show
+```
+
+## Inference Model
+
+Now you can do model inference with the APIs provided by the backend. But what if you want to test the model instantly? We have some backend wrappers for you.
+
+```python
+from mmdeploy.apis import inference_model
+
+result = inference_model(model_cfg, deploy_cfg, backend_files, img=img, device=device)
+```
+
+The `inference_model` will create a wrapper module and do the inference for you. The result has the same format as the original OpenMMLab repo.
+
+## Evaluate model (Optional)
+
+You can test the accuracy and speed of the model in the inference backend. You could refer to MMDeploy docs [how to measure performance of models](https://mmdeploy.readthedocs.io/en/latest/tutorials/how_to_measure_performance_of_models.html).
+
+```bash
+python tools/test.py \
+ ${DEPLOY_CFG} \
+ ${MODEL_CFG} \
+ --model ${BACKEND_MODEL_FILES} \
+ [--out ${OUTPUT_PKL_FILE}] \
+ [--format-only] \
+ [--metrics ${METRICS}] \
+ [--show] \
+ [--show-dir ${OUTPUT_IMAGE_DIR}] \
+ [--show-score-thr ${SHOW_SCORE_THR}] \
+ --device ${DEVICE} \
+ [--cfg-options ${CFG_OPTIONS}] \
+ [--metric-options ${METRIC_OPTIONS}] \
+ [--log2file work_dirs/output.txt]
+```
+
+### Example
+
+```bash
+cd mmdeploy
+python tools/test.py \
+ configs/mmdet3d/voxel-detection/voxel-detection_onnxruntime_dynamic.py \
+ ${MMDET3D_DIR}/configs/centerpoint/centerpoint_02pillar_second_secfpn_circlenms_4x8_cyclic_20e_nus.py \
+ --model work-dir/end2end.onnx \
+ --metrics bbox \
+ --device cpu
+```
+
+## Supported models
+
+| Model | TorchScript | OnnxRuntime | TensorRT | NCNN | PPLNN | OpenVINO | Model config |
+| -------------------- | :---------: | :---------: | :------: | :--: | :---: | :------: | -------------------------------------------------------------------------------------- |
+| PointPillars | ? | Y | Y | N | N | Y | [config](https://github.com/open-mmlab/mmdetection3d/blob/master/configs/pointpillars) |
+| CenterPoint (pillar) | ? | Y | Y | N | N | Y | [config](https://github.com/open-mmlab/mmdetection3d/blob/master/configs/centerpoint) |
+
+## Note
+
+- MMDeploy version >= 0.4.0.
+- Currently, CenterPoint has only supported the pillar version.
diff --git a/docs/en/tutorials/pure_point_cloud_dataset.md b/docs/en/tutorials/pure_point_cloud_dataset.md
new file mode 100644
index 0000000..ae7ddaa
--- /dev/null
+++ b/docs/en/tutorials/pure_point_cloud_dataset.md
@@ -0,0 +1,461 @@
+# Tutorial 9: Use Pure Point Cloud Dataset
+
+## Data Pre-Processing
+
+### Convert Point cloud format
+
+Currently, we only support bin format point cloud training and inference, before training on your own datasets, you need to transform your point cloud format to bin file. The common point cloud data formats include pcd and las, we provide some open-source tools for reference.
+
+1. Convert pcd to bin: https://github.com/leofansq/Tools_RosBag2KITTI
+2. Convert las to bin: The common conversion path is las -> pcd -> bin, and the conversion from las -> pcd can be achieved through [this tool](https://github.com/Hitachi-Automotive-And-Industry-Lab/semantic-segmentation-editor).
+
+### Point cloud annotation
+
+MMDetection3D does not support point cloud annotation. Some open-source annotation tools are offered for reference:
+
+- [SUSTechPOINTS](https://github.com/naurril/SUSTechPOINTS)
+- [LATTE](https://github.com/bernwang/latte)
+
+Besides, we improved [LATTE](https://github.com/bernwang/latte) for better usage. More details can be found [here](https://arxiv.org/abs/2011.10174).
+
+## Support new data format
+
+To support a new data format, you can either convert them to existing formats or directly convert them to the middle format. You could also choose to convert them offline (before training by a script) or online (implement a new dataset and do the conversion at training).
+
+### Reorganize new data formats to existing format
+
+Once your datasets only contain point cloud file and 3D Bounding box annotations, without calib file. We recommend converting it into the basic formats, the annotations files in basic format has the following necessary keys:
+
+```python
+
+[
+ {'sample_idx':
+ 'lidar_points': {'lidar_path': velodyne_path,
+ ....
+ },
+ 'annos': {'box_type_3d': (str) 'LiDAR/Camera/Depth'
+ 'gt_bboxes_3d': (n, 7)
+ 'gt_names': [list]
+ ....
+ }
+ 'calib': { .....}
+ 'images': { .....}
+ }
+]
+
+```
+
+In MMDetection3D, for the data that is inconvenient to read directly online, we recommend converting it into into basic format as above and do the conversion offline, thus you only need to modify the config's data annotation paths and classes after the conversion.
+To use data that share a similar format as the existing datasets, e.g., Lyft has a similar format as the nuScenes dataset, we recommend directly implementing a new data converter and a dataset class to convert the data and load the data, respectively. In this procedure, the code can inherit from the existing dataset classes to reuse the code.
+
+### Reorganize new data format to middle format
+
+There is also a way if users do not want to convert the annotation format to existing formats.
+Actually, we convert all the supported datasets into pickle files, which summarize useful information for model training and inference.
+
+The annotation of a dataset is a list of dict, each dict corresponds to a frame.
+A basic example (used in KITTI) is as follows. A frame consists of several keys, like `image`, `point_cloud`, `calib` and `annos`.
+As long as we could directly read data according to these information, the organization of raw data could also be different from existing ones.
+With this design, we provide an alternative choice for customizing datasets.
+
+```python
+
+[
+ {'image': {'image_idx': 0, 'image_path': 'training/image_2/000000.png', 'image_shape': array([ 370, 1224], dtype=int32)},
+ 'point_cloud': {'num_features': 4, 'velodyne_path': 'training/velodyne/000000.bin'},
+ 'calib': {'P0': array([[707.0493, 0. , 604.0814, 0. ],
+ [ 0. , 707.0493, 180.5066, 0. ],
+ [ 0. , 0. , 1. , 0. ],
+ [ 0. , 0. , 0. , 1. ]]),
+ 'P1': array([[ 707.0493, 0. , 604.0814, -379.7842],
+ [ 0. , 707.0493, 180.5066, 0. ],
+ [ 0. , 0. , 1. , 0. ],
+ [ 0. , 0. , 0. , 1. ]]),
+ 'P2': array([[ 7.070493e+02, 0.000000e+00, 6.040814e+02, 4.575831e+01],
+ [ 0.000000e+00, 7.070493e+02, 1.805066e+02, -3.454157e-01],
+ [ 0.000000e+00, 0.000000e+00, 1.000000e+00, 4.981016e-03],
+ [ 0.000000e+00, 0.000000e+00, 0.000000e+00, 1.000000e+00]]),
+ 'P3': array([[ 7.070493e+02, 0.000000e+00, 6.040814e+02, -3.341081e+02],
+ [ 0.000000e+00, 7.070493e+02, 1.805066e+02, 2.330660e+00],
+ [ 0.000000e+00, 0.000000e+00, 1.000000e+00, 3.201153e-03],
+ [ 0.000000e+00, 0.000000e+00, 0.000000e+00, 1.000000e+00]]),
+ 'R0_rect': array([[ 0.9999128 , 0.01009263, -0.00851193, 0. ],
+ [-0.01012729, 0.9999406 , -0.00403767, 0. ],
+ [ 0.00847068, 0.00412352, 0.9999556 , 0. ],
+ [ 0. , 0. , 0. , 1. ]]),
+ 'Tr_velo_to_cam': array([[ 0.00692796, -0.9999722 , -0.00275783, -0.02457729],
+ [-0.00116298, 0.00274984, -0.9999955 , -0.06127237],
+ [ 0.9999753 , 0.00693114, -0.0011439 , -0.3321029 ],
+ [ 0. , 0. , 0. , 1. ]]),
+ 'Tr_imu_to_velo': array([[ 9.999976e-01, 7.553071e-04, -2.035826e-03, -8.086759e-01],
+ [-7.854027e-04, 9.998898e-01, -1.482298e-02, 3.195559e-01],
+ [ 2.024406e-03, 1.482454e-02, 9.998881e-01, -7.997231e-01],
+ [ 0.000000e+00, 0.000000e+00, 0.000000e+00, 1.000000e+00]])},
+ 'annos': {'name': array(['Pedestrian'], dtype='
+
+ (为了使用相同方法进行测试所做的具体修改 - 点击展开)
+
+
+ ```diff
+ diff --git a/tools/train_utils/train_utils.py b/tools/train_utils/train_utils.py
+ index 91f21dd..021359d 100644
+ --- a/tools/train_utils/train_utils.py
+ +++ b/tools/train_utils/train_utils.py
+ @@ -2,6 +2,7 @@ import torch
+ import os
+ import glob
+ import tqdm
+ +import datetime
+ from torch.nn.utils import clip_grad_norm_
+
+
+ @@ -13,7 +14,10 @@ def train_one_epoch(model, optimizer, train_loader, model_func, lr_scheduler, ac
+ if rank == 0:
+ pbar = tqdm.tqdm(total=total_it_each_epoch, leave=leave_pbar, desc='train', dynamic_ncols=True)
+
+ + start_time = None
+ for cur_it in range(total_it_each_epoch):
+ + if cur_it > 49 and start_time is None:
+ + start_time = datetime.datetime.now()
+ try:
+ batch = next(dataloader_iter)
+ except StopIteration:
+ @@ -55,9 +59,11 @@ def train_one_epoch(model, optimizer, train_loader, model_func, lr_scheduler, ac
+ tb_log.add_scalar('learning_rate', cur_lr, accumulated_iter)
+ for key, val in tb_dict.items():
+ tb_log.add_scalar('train_' + key, val, accumulated_iter)
+ + endtime = datetime.datetime.now()
+ + speed = (endtime - start_time).seconds / (total_it_each_epoch - 50)
+ if rank == 0:
+ pbar.close()
+ - return accumulated_iter
+ + return accumulated_iter, speed
+
+
+ def train_model(model, optimizer, train_loader, model_func, lr_scheduler, optim_cfg,
+ @@ -65,6 +71,7 @@ def train_model(model, optimizer, train_loader, model_func, lr_scheduler, optim_
+ lr_warmup_scheduler=None, ckpt_save_interval=1, max_ckpt_save_num=50,
+ merge_all_iters_to_one_epoch=False):
+ accumulated_iter = start_iter
+ + speeds = []
+ with tqdm.trange(start_epoch, total_epochs, desc='epochs', dynamic_ncols=True, leave=(rank == 0)) as tbar:
+ total_it_each_epoch = len(train_loader)
+ if merge_all_iters_to_one_epoch:
+ @@ -82,7 +89,7 @@ def train_model(model, optimizer, train_loader, model_func, lr_scheduler, optim_
+ cur_scheduler = lr_warmup_scheduler
+ else:
+ cur_scheduler = lr_scheduler
+ - accumulated_iter = train_one_epoch(
+ + accumulated_iter, speed = train_one_epoch(
+ model, optimizer, train_loader, model_func,
+ lr_scheduler=cur_scheduler,
+ accumulated_iter=accumulated_iter, optim_cfg=optim_cfg,
+ @@ -91,7 +98,7 @@ def train_model(model, optimizer, train_loader, model_func, lr_scheduler, optim_
+ total_it_each_epoch=total_it_each_epoch,
+ dataloader_iter=dataloader_iter
+ )
+ -
+ + speeds.append(speed)
+ # save trained model
+ trained_epoch = cur_epoch + 1
+ if trained_epoch % ckpt_save_interval == 0 and rank == 0:
+ @@ -107,6 +114,8 @@ def train_model(model, optimizer, train_loader, model_func, lr_scheduler, optim_
+ save_checkpoint(
+ checkpoint_state(model, optimizer, trained_epoch, accumulated_iter), filename=ckpt_name,
+ )
+ + print(speed)
+ + print(f'*******{sum(speeds) / len(speeds)}******')
+
+
+ def model_state_to_cpu(model_state):
+ ```
+
+
+
+### VoteNet
+
+- __MMDetection3D__:在 v0.1.0 版本下, 执行如下命令:
+
+ ```bash
+ ./tools/dist_train.sh configs/votenet/votenet_16x8_sunrgbd-3d-10class.py 8 --no-validate
+ ```
+
+- __votenet__:在 commit [2f6d6d3](https://github.com/facebookresearch/votenet/tree/2f6d6d36ff98d96901182e935afe48ccee82d566) 版本下,执行如下命令:
+
+ ```bash
+ python train.py --dataset sunrgbd --batch_size 16
+ ```
+
+ 然后执行如下命令,对测试速度进行评估:
+
+ ```bash
+ python eval.py --dataset sunrgbd --checkpoint_path log_sunrgbd/checkpoint.tar --batch_size 1 --dump_dir eval_sunrgbd --cluster_sampling seed_fps --use_3d_nms --use_cls_nms --per_class_proposal
+ ```
+
+ 注意,为了计算推理速度,我们对 `eval.py` 进行了修改。
+
+
+
+ (为了对相同模型进行测试所做的具体修改 - 点击展开)
+
+
+ ```diff
+ diff --git a/eval.py b/eval.py
+ index c0b2886..04921e9 100644
+ --- a/eval.py
+ +++ b/eval.py
+ @@ -10,6 +10,7 @@ import os
+ import sys
+ import numpy as np
+ from datetime import datetime
+ +import time
+ import argparse
+ import importlib
+ import torch
+ @@ -28,7 +29,7 @@ parser.add_argument('--checkpoint_path', default=None, help='Model checkpoint pa
+ parser.add_argument('--dump_dir', default=None, help='Dump dir to save sample outputs [default: None]')
+ parser.add_argument('--num_point', type=int, default=20000, help='Point Number [default: 20000]')
+ parser.add_argument('--num_target', type=int, default=256, help='Point Number [default: 256]')
+ -parser.add_argument('--batch_size', type=int, default=8, help='Batch Size during training [default: 8]')
+ +parser.add_argument('--batch_size', type=int, default=1, help='Batch Size during training [default: 8]')
+ parser.add_argument('--vote_factor', type=int, default=1, help='Number of votes generated from each seed [default: 1]')
+ parser.add_argument('--cluster_sampling', default='vote_fps', help='Sampling strategy for vote clusters: vote_fps, seed_fps, random [default: vote_fps]')
+ parser.add_argument('--ap_iou_thresholds', default='0.25,0.5', help='A list of AP IoU thresholds [default: 0.25,0.5]')
+ @@ -132,6 +133,7 @@ CONFIG_DICT = {'remove_empty_box': (not FLAGS.faster_eval), 'use_3d_nms': FLAGS.
+ # ------------------------------------------------------------------------- GLOBAL CONFIG END
+
+ def evaluate_one_epoch():
+ + time_list = list()
+ stat_dict = {}
+ ap_calculator_list = [APCalculator(iou_thresh, DATASET_CONFIG.class2type) \
+ for iou_thresh in AP_IOU_THRESHOLDS]
+ @@ -144,6 +146,8 @@ def evaluate_one_epoch():
+
+ # Forward pass
+ inputs = {'point_clouds': batch_data_label['point_clouds']}
+ + torch.cuda.synchronize()
+ + start_time = time.perf_counter()
+ with torch.no_grad():
+ end_points = net(inputs)
+
+ @@ -161,6 +165,12 @@ def evaluate_one_epoch():
+
+ batch_pred_map_cls = parse_predictions(end_points, CONFIG_DICT)
+ batch_gt_map_cls = parse_groundtruths(end_points, CONFIG_DICT)
+ + torch.cuda.synchronize()
+ + elapsed = time.perf_counter() - start_time
+ + time_list.append(elapsed)
+ +
+ + if len(time_list==200):
+ + print("average inference time: %4f"%(sum(time_list[5:])/len(time_list[5:])))
+ for ap_calculator in ap_calculator_list:
+ ap_calculator.step(batch_pred_map_cls, batch_gt_map_cls)
+
+ ```
+
+### PointPillars-car
+
+- __MMDetection3D__:在 v0.1.0 版本下, 执行如下命令:
+
+ ```bash
+ ./tools/dist_train.sh configs/benchmark/hv_pointpillars_secfpn_3x8_100e_det3d_kitti-3d-car.py 8 --no-validate
+ ```
+
+- __Det3D__:在 commit [519251e](https://github.com/poodarchu/Det3D/tree/519251e72a5c1fdd58972eabeac67808676b9bb7) 版本下,使用 `kitti_point_pillars_mghead_syncbn.py` 并执行如下命令:
+
+ ```bash
+ ./tools/scripts/train.sh --launcher=slurm --gpus=8
+ ```
+
+ 注意,为了训练 PointPillars,我们对 `train.sh` 进行了修改。
+
+
+
+ (为了对相同模型进行测试所做的具体修改 - 点击展开)
+
+
+ ```diff
+ diff --git a/tools/scripts/train.sh b/tools/scripts/train.sh
+ index 3a93f95..461e0ea 100755
+ --- a/tools/scripts/train.sh
+ +++ b/tools/scripts/train.sh
+ @@ -16,9 +16,9 @@ then
+ fi
+
+ # Voxelnet
+ -python -m torch.distributed.launch --nproc_per_node=8 ./tools/train.py examples/second/configs/ kitti_car_vfev3_spmiddlefhd_rpn1_mghead_syncbn.py --work_dir=$SECOND_WORK_DIR
+ +# python -m torch.distributed.launch --nproc_per_node=8 ./tools/train.py examples/second/configs/ kitti_car_vfev3_spmiddlefhd_rpn1_mghead_syncbn.py --work_dir=$SECOND_WORK_DIR
+ # python -m torch.distributed.launch --nproc_per_node=8 ./tools/train.py examples/cbgs/configs/ nusc_all_vfev3_spmiddleresnetfhd_rpn2_mghead_syncbn.py --work_dir=$NUSC_CBGS_WORK_DIR
+ # python -m torch.distributed.launch --nproc_per_node=8 ./tools/train.py examples/second/configs/ lyft_all_vfev3_spmiddleresnetfhd_rpn2_mghead_syncbn.py --work_dir=$LYFT_CBGS_WORK_DIR
+
+ # PointPillars
+ -# python -m torch.distributed.launch --nproc_per_node=8 ./tools/train.py ./examples/point_pillars/configs/ original_pp_mghead_syncbn_kitti.py --work_dir=$PP_WORK_DIR
+ +python -m torch.distributed.launch --nproc_per_node=8 ./tools/train.py ./examples/point_pillars/configs/ kitti_point_pillars_mghead_syncbn.py
+ ```
+
+
+
+### PointPillars-3class
+
+- __MMDetection3D__:在 v0.1.0 版本下, 执行如下命令:
+
+ ```bash
+ ./tools/dist_train.sh configs/benchmark/hv_pointpillars_secfpn_4x8_80e_pcdet_kitti-3d-3class.py 8 --no-validate
+ ```
+
+- __OpenPCDet__:在 commit [b32fbddb](https://github.com/open-mmlab/OpenPCDet/tree/b32fbddbe06183507bad433ed99b407cbc2175c2) 版本下,执行如下命令:
+
+ ```bash
+ cd tools
+ sh scripts/slurm_train.sh ${PARTITION} ${JOB_NAME} 8 --cfg_file ./cfgs/kitti_models/pointpillar.yaml --batch_size 32 --workers 32 --epochs 80
+ ```
+
+### SECOND
+
+基准测试中的 SECOND 指在 [second.Pytorch](https://github.com/traveller59/second.pytorch) 首次被实现的 [SECONDv1.5](https://github.com/traveller59/second.pytorch/blob/master/second/configs/all.fhd.config)。Det3D 实现的 SECOND 中,使用了自己实现的 Multi-Group Head,因此无法将它的速度与其他代码库进行对比。
+
+- __MMDetection3D__:在 v0.1.0 版本下, 执行如下命令:
+
+ ```bash
+ ./tools/dist_train.sh configs/benchmark/hv_second_secfpn_4x8_80e_pcdet_kitti-3d-3class.py 8 --no-validate
+ ```
+
+- __OpenPCDet__:在 commit [b32fbddb](https://github.com/open-mmlab/OpenPCDet/tree/b32fbddbe06183507bad433ed99b407cbc2175c2) 版本下,执行如下命令:
+
+ ```bash
+ cd tools
+ sh ./scripts/slurm_train.sh ${PARTITION} ${JOB_NAME} 8 --cfg_file ./cfgs/kitti_models/second.yaml --batch_size 32 --workers 32 --epochs 80
+ ```
+
+### Part-A2
+
+- __MMDetection3D__:在 v0.1.0 版本下, 执行如下命令:
+
+ ```bash
+ ./tools/dist_train.sh configs/benchmark/hv_PartA2_secfpn_4x8_cyclic_80e_pcdet_kitti-3d-3class.py 8 --no-validate
+ ```
+
+- __OpenPCDet__:在 commit [b32fbddb](https://github.com/open-mmlab/OpenPCDet/tree/b32fbddbe06183507bad433ed99b407cbc2175c2) 版本下,执行如下命令以进行模型训练:
+
+ ```bash
+ cd tools
+ sh ./scripts/slurm_train.sh ${PARTITION} ${JOB_NAME} 8 --cfg_file ./cfgs/kitti_models/PartA2.yaml --batch_size 32 --workers 32 --epochs 80
+ ```
diff --git a/docs/zh_cn/changelog.md b/docs/zh_cn/changelog.md
new file mode 100644
index 0000000..9017dfd
--- /dev/null
+++ b/docs/zh_cn/changelog.md
@@ -0,0 +1 @@
+# 变更日志
diff --git a/docs/zh_cn/compatibility.md b/docs/zh_cn/compatibility.md
new file mode 100644
index 0000000..3b34d36
--- /dev/null
+++ b/docs/zh_cn/compatibility.md
@@ -0,0 +1 @@
+## 0.16.0
diff --git a/docs/zh_cn/conf.py b/docs/zh_cn/conf.py
new file mode 100644
index 0000000..348059d
--- /dev/null
+++ b/docs/zh_cn/conf.py
@@ -0,0 +1,161 @@
+# Configuration file for the Sphinx documentation builder.
+#
+# This file only contains a selection of the most common options. For a full
+# list see the documentation:
+# https://www.sphinx-doc.org/en/master/usage/configuration.html
+
+# -- Path setup --------------------------------------------------------------
+
+# If extensions (or modules to document with autodoc) are in another directory,
+# add these directories to sys.path here. If the directory is relative to the
+# documentation root, use os.path.abspath to make it absolute, like shown here.
+#
+import os
+import subprocess
+import sys
+
+import pytorch_sphinx_theme
+from m2r import MdInclude
+from recommonmark.transform import AutoStructify
+from sphinx.builders.html import StandaloneHTMLBuilder
+
+sys.path.insert(0, os.path.abspath('../../'))
+
+# -- Project information -----------------------------------------------------
+
+project = 'MMDetection3D'
+copyright = '2020-2023, OpenMMLab'
+author = 'MMDetection3D Authors'
+
+version_file = '../../mmdet3d/version.py'
+
+
+def get_version():
+ with open(version_file, 'r') as f:
+ exec(compile(f.read(), version_file, 'exec'))
+ return locals()['__version__']
+
+
+# The full version, including alpha/beta/rc tags
+release = get_version()
+
+# -- General configuration ---------------------------------------------------
+
+# Add any Sphinx extension module names here, as strings. They can be
+# extensions coming with Sphinx (named 'sphinx.ext.*') or your custom
+# ones.
+extensions = [
+ 'sphinx.ext.autodoc',
+ 'sphinx.ext.napoleon',
+ 'sphinx.ext.viewcode',
+ 'myst_parser',
+ 'sphinx_markdown_tables',
+ 'sphinx.ext.autosectionlabel',
+ 'sphinx_copybutton',
+]
+
+autodoc_mock_imports = [
+ 'matplotlib', 'nuscenes', 'PIL', 'pycocotools', 'pyquaternion',
+ 'terminaltables', 'mmdet3d.version', 'mmdet3d.ops', 'mmcv.ops'
+]
+autosectionlabel_prefix_document = True
+
+# Add any paths that contain templates here, relative to this directory.
+templates_path = ['_templates']
+
+# The suffix(es) of source filenames.
+# You can specify multiple suffix as a list of string:
+#
+source_suffix = {
+ '.rst': 'restructuredtext',
+ '.md': 'markdown',
+}
+
+# The master toctree document.
+master_doc = 'index'
+
+# List of patterns, relative to source directory, that match files and
+# directories to ignore when looking for source files.
+# This pattern also affects html_static_path and html_extra_path.
+exclude_patterns = ['_build', 'Thumbs.db', '.DS_Store']
+
+# -- Options for HTML output -------------------------------------------------
+
+# The theme to use for HTML and HTML Help pages. See the documentation for
+# a list of builtin themes.
+#
+# html_theme = 'sphinx_rtd_theme'
+html_theme = 'pytorch_sphinx_theme'
+html_theme_path = [pytorch_sphinx_theme.get_html_theme_path()]
+
+html_theme_options = {
+ # 'logo_url': 'https://mmocr.readthedocs.io/en/latest/',
+ 'menu': [
+ {
+ 'name': 'GitHub',
+ 'url': 'https://github.com/open-mmlab/mmdetection3d'
+ },
+ {
+ 'name':
+ '上游库',
+ 'children': [
+ {
+ 'name': 'MMCV',
+ 'url': 'https://github.com/open-mmlab/mmcv',
+ 'description': '基础视觉库'
+ },
+ {
+ 'name': 'MMDetection',
+ 'url': 'https://github.com/open-mmlab/mmdetection',
+ 'description': '目标检测工具箱'
+ },
+ ]
+ },
+ ],
+ # Specify the language of shared menu
+ 'menu_lang':
+ 'cn',
+}
+
+language = 'zh_CN'
+
+master_doc = 'index'
+
+# Add any paths that contain custom static files (such as style sheets) here,
+# relative to this directory. They are copied after the builtin static files,
+# so a file named "default.css" will overwrite the builtin "default.css".
+html_static_path = ['_static']
+html_css_files = ['css/readthedocs.css']
+
+latex_documents = [
+ (master_doc, 'mmcv.tex', 'mmcv Documentation', 'MMCV Contributors',
+ 'manual'),
+]
+
+# set priority when building html
+StandaloneHTMLBuilder.supported_image_types = [
+ 'image/svg+xml', 'image/gif', 'image/png', 'image/jpeg'
+]
+# Enable ::: for my_st
+myst_enable_extensions = ['colon_fence']
+myst_heading_anchors = 3
+
+language = 'zh_CN'
+
+
+def builder_inited_handler(app):
+ subprocess.run(['./stat.py'])
+
+
+def setup(app):
+ app.connect('builder-inited', builder_inited_handler)
+ app.add_config_value('no_underscore_emphasis', False, 'env')
+ app.add_config_value('m2r_parse_relative_links', False, 'env')
+ app.add_config_value('m2r_anonymous_references', False, 'env')
+ app.add_config_value('m2r_disable_inline_math', False, 'env')
+ app.add_directive('mdinclude', MdInclude)
+ app.add_config_value('recommonmark_config', {
+ 'auto_toc_tree_section': 'Contents',
+ 'enable_eval_rst': True,
+ }, True)
+ app.add_transform(AutoStructify)
diff --git a/docs/zh_cn/data_preparation.md b/docs/zh_cn/data_preparation.md
new file mode 100644
index 0000000..62bebd8
--- /dev/null
+++ b/docs/zh_cn/data_preparation.md
@@ -0,0 +1,140 @@
+# 数据预处理
+
+## 在数据预处理前
+
+我们推荐用户将数据集的路径软链接到 `$MMDETECTION3D/data`。
+如果你的文件夹结构和以下所展示的结构相异,你可能需要改变配置文件中相应的数据路径。
+
+```
+mmdetection3d
+├── mmdet3d
+├── tools
+├── configs
+├── data
+│ ├── nuscenes
+│ │ ├── maps
+│ │ ├── samples
+│ │ ├── sweeps
+│ │ ├── v1.0-test
+| | ├── v1.0-trainval
+│ ├── kitti
+│ │ ├── ImageSets
+│ │ ├── testing
+│ │ │ ├── calib
+│ │ │ ├── image_2
+│ │ │ ├── velodyne
+│ │ ├── training
+│ │ │ ├── calib
+│ │ │ ├── image_2
+│ │ │ ├── label_2
+│ │ │ ├── velodyne
+│ ├── waymo
+│ │ ├── waymo_format
+│ │ │ ├── training
+│ │ │ ├── validation
+│ │ │ ├── testing
+│ │ │ ├── gt.bin
+│ │ ├── kitti_format
+│ │ │ ├── ImageSets
+│ ├── lyft
+│ │ ├── v1.01-train
+│ │ │ ├── v1.01-train (训练数据)
+│ │ │ ├── lidar (训练激光雷达)
+│ │ │ ├── images (训练图片)
+│ │ │ ├── maps (训练地图)
+│ │ ├── v1.01-test
+│ │ │ ├── v1.01-test (测试数据)
+│ │ │ ├── lidar (测试激光雷达)
+│ │ │ ├── images (测试图片)
+│ │ │ ├── maps (测试地图)
+│ │ ├── train.txt
+│ │ ├── val.txt
+│ │ ├── test.txt
+│ │ ├── sample_submission.csv
+│ ├── s3dis
+│ │ ├── meta_data
+│ │ ├── Stanford3dDataset_v1.2_Aligned_Version
+│ │ ├── collect_indoor3d_data.py
+│ │ ├── indoor3d_util.py
+│ │ ├── README.md
+│ ├── scannet
+│ │ ├── meta_data
+│ │ ├── scans
+│ │ ├── scans_test
+│ │ ├── batch_load_scannet_data.py
+│ │ ├── load_scannet_data.py
+│ │ ├── scannet_utils.py
+│ │ ├── README.md
+│ ├── sunrgbd
+│ │ ├── OFFICIAL_SUNRGBD
+│ │ ├── matlab
+│ │ ├── sunrgbd_data.py
+│ │ ├── sunrgbd_utils.py
+│ │ ├── README.md
+
+```
+
+## 数据下载和预处理
+
+### KITTI
+
+在[这里](http://www.cvlibs.net/datasets/kitti/eval_object.php?obj_benchmark=3d)下载 KITTI 的 3D 检测数据。通过运行以下指令对 KITTI 数据进行预处理:
+
+```bash
+mkdir ./data/kitti/ && mkdir ./data/kitti/ImageSets
+
+# 下载数据划分文件
+wget -c https://raw.githubusercontent.com/traveller59/second.pytorch/master/second/data/ImageSets/test.txt --no-check-certificate --content-disposition -O ./data/kitti/ImageSets/test.txt
+wget -c https://raw.githubusercontent.com/traveller59/second.pytorch/master/second/data/ImageSets/train.txt --no-check-certificate --content-disposition -O ./data/kitti/ImageSets/train.txt
+wget -c https://raw.githubusercontent.com/traveller59/second.pytorch/master/second/data/ImageSets/val.txt --no-check-certificate --content-disposition -O ./data/kitti/ImageSets/val.txt
+wget -c https://raw.githubusercontent.com/traveller59/second.pytorch/master/second/data/ImageSets/trainval.txt --no-check-certificate --content-disposition -O ./data/kitti/ImageSets/trainval.txt
+
+python tools/create_data.py kitti --root-path ./data/kitti --out-dir ./data/kitti --extra-tag kitti
+```
+
+### Waymo
+
+在[这里](https://waymo.com/open/download/)下载 Waymo 公开数据集1.2版本,在[这里](https://drive.google.com/drive/folders/18BVuF_RYJF0NjZpt8SnfzANiakoRMf0o?usp=sharing)下载其数据划分文件。
+然后,将 tfrecord 文件置于 `data/waymo/waymo_format/` 目录下的相应位置,并将数据划分的 txt 文件置于 `data/waymo/kitti_format/ImageSets` 目录下。
+在[这里](https://console.cloud.google.com/storage/browser/waymo_open_dataset_v_1_2_0/validation/ground_truth_objects)下载验证集的真实标签 (bin 文件) 并将其置于 `data/waymo/waymo_format/`。
+提示,你可以使用 `gsutil` 来用命令下载大规模的数据集。你可以参考这个[工具](https://github.com/RalphMao/Waymo-Dataset-Tool)来获取更多实现细节。
+完成以上各步后,可以通过运行以下指令对 Waymo 数据进行预处理:
+
+```bash
+python tools/create_data.py waymo --root-path ./data/waymo/ --out-dir ./data/waymo/ --workers 128 --extra-tag waymo
+```
+
+注意,如果你的硬盘空间大小不足以存储转换后的数据,你可以将 `out-dir` 参数设定为别的路径。
+你只需要记得在那个路径下创建文件夹并下载数据,然后在数据预处理完成后将其链接回 `data/waymo/kitti_format` 即可。
+
+### NuScenes
+
+在[这里](https://www.nuscenes.org/download)下载 nuScenes 数据集 1.0 版本的完整数据文件。通过运行以下指令对 nuScenes 数据进行预处理:
+
+```bash
+python tools/create_data.py nuscenes --root-path ./data/nuscenes --out-dir ./data/nuscenes --extra-tag nuscenes
+```
+
+### Lyft
+
+在[这里](https://www.kaggle.com/c/3d-object-detection-for-autonomous-vehicles/data)下载 Lyft 3D 检测数据。通过运行以下指令对 Lyft 数据进行预处理:
+
+```bash
+python tools/create_data.py lyft --root-path ./data/lyft --out-dir ./data/lyft --extra-tag lyft --version v1.01
+python tools/data_converter/lyft_data_fixer.py --version v1.01 --root-folder ./data/lyft
+```
+
+注意,为了文件结构的清晰性,我们遵从了 Lyft 数据原先的文件夹名称。请按照上面展示出的文件结构对原始文件夹进行重命名。
+同样值得注意的是,第二行命令的目的是为了修复一个损坏的激光雷达数据文件。请参考[这一](https://www.kaggle.com/c/3d-object-detection-for-autonomous-vehicles/discussion/110000)讨论来获取更多细节。
+
+### S3DIS、ScanNet 和 SUN RGB-D
+
+请参考 S3DIS [README](https://github.com/open-mmlab/mmdetection3d/blob/master/data/s3dis/README.md/) 文件以对其进行数据预处理。
+
+请参考 ScanNet [README](https://github.com/open-mmlab/mmdetection3d/blob/master/data/scannet/README.md/) 文件以对其进行数据预处理。
+
+请参考 SUN RGB-D [README](https://github.com/open-mmlab/mmdetection3d/blob/master/data/sunrgbd/README.md/) 文件以对其进行数据预处理。
+
+### 自定义数据集
+
+关于如何使用自定义数据集,请参考[教程 2: 自定义数据集](https://mmdetection3d.readthedocs.io/zh_CN/latest/tutorials/customize_dataset.html)。
diff --git a/docs/zh_cn/datasets/index.rst b/docs/zh_cn/datasets/index.rst
new file mode 100644
index 0000000..c25295d
--- /dev/null
+++ b/docs/zh_cn/datasets/index.rst
@@ -0,0 +1,11 @@
+.. toctree::
+ :maxdepth: 2
+
+ kitti_det.md
+ nuscenes_det.md
+ lyft_det.md
+ waymo_det.md
+ sunrgbd_det.md
+ scannet_det.md
+ scannet_sem_seg.md
+ s3dis_sem_seg.md
diff --git a/docs/zh_cn/datasets/kitti_det.md b/docs/zh_cn/datasets/kitti_det.md
new file mode 100644
index 0000000..01a2421
--- /dev/null
+++ b/docs/zh_cn/datasets/kitti_det.md
@@ -0,0 +1,194 @@
+# 3D 目标检测 KITTI 数据集
+
+本页提供了有关在 MMDetection3D 中使用 KITTI 数据集的具体教程。
+
+**注意**:此教程目前仅适用于基于雷达和多模态的 3D 目标检测的相关方法,与基于单目图像的 3D 目标检测相关的内容会在之后进行补充。
+
+## 数据准备
+
+您可以在[这里](http://www.cvlibs.net/datasets/kitti/eval_object.php?obj_benchmark=3d)下载 KITTI 3D 检测数据并解压缩所有 zip 文件。此外,您可以在[这里](https://download.openmmlab.com/mmdetection3d/data/train_planes.zip)下载道路平面信息,其在训练过程中作为一个可选项,用来提高模型的性能。道路平面信息由 [AVOD](https://github.com/kujason/avod) 生成,你可以在[这里](https://github.com/kujason/avod/issues/19)查看更多细节。
+
+像准备数据集的一般方法一样,建议将数据集根目录链接到 `$MMDETECTION3D/data`。
+
+在我们处理之前,文件夹结构应按如下方式组织:
+
+```
+mmdetection3d
+├── mmdet3d
+├── tools
+├── configs
+├── data
+│ ├── kitti
+│ │ ├── ImageSets
+│ │ ├── testing
+│ │ │ ├── calib
+│ │ │ ├── image_2
+│ │ │ ├── velodyne
+│ │ ├── training
+│ │ │ ├── calib
+│ │ │ ├── image_2
+│ │ │ ├── label_2
+│ │ │ ├── velodyne
+│ │ │ ├── planes (optional)
+```
+
+### 创建 KITTI 数据集
+
+为了创建 KITTI 点云数据,首先需要加载原始的点云数据并生成相关的包含目标标签和标注框的数据标注文件,同时还需要为 KITTI 数据集生成每个单独的训练目标的点云数据,并将其存储在 `data/kitti/kitti_gt_database` 的 `.bin` 格式的文件中,此外,需要为训练数据或者验证数据生成 `.pkl` 格式的包含数据信息的文件。随后,通过运行下面的命令来创建最终的 KITTI 数据:
+
+```bash
+mkdir ./data/kitti/ && mkdir ./data/kitti/ImageSets
+
+# Download data split
+wget -c https://raw.githubusercontent.com/traveller59/second.pytorch/master/second/data/ImageSets/test.txt --no-check-certificate --content-disposition -O ./data/kitti/ImageSets/test.txt
+wget -c https://raw.githubusercontent.com/traveller59/second.pytorch/master/second/data/ImageSets/train.txt --no-check-certificate --content-disposition -O ./data/kitti/ImageSets/train.txt
+wget -c https://raw.githubusercontent.com/traveller59/second.pytorch/master/second/data/ImageSets/val.txt --no-check-certificate --content-disposition -O ./data/kitti/ImageSets/val.txt
+wget -c https://raw.githubusercontent.com/traveller59/second.pytorch/master/second/data/ImageSets/trainval.txt --no-check-certificate --content-disposition -O ./data/kitti/ImageSets/trainval.txt
+
+python tools/create_data.py kitti --root-path ./data/kitti --out-dir ./data/kitti --extra-tag kitti --with-plane
+```
+
+需要注意的是,如果您的本地磁盘没有充足的存储空间来存储转换后的数据,您可以通过改变 `out-dir` 来指定其他任意的存储路径。如果您没有准备 `planes` 数据,您需要移除 `--with-plane` 标志。
+
+处理后的文件夹结构应该如下:
+
+```
+kitti
+├── ImageSets
+│ ├── test.txt
+│ ├── train.txt
+│ ├── trainval.txt
+│ ├── val.txt
+├── testing
+│ ├── calib
+│ ├── image_2
+│ ├── velodyne
+│ ├── velodyne_reduced
+├── training
+│ ├── calib
+│ ├── image_2
+│ ├── label_2
+│ ├── velodyne
+│ ├── velodyne_reduced
+│ ├── planes (optional)
+├── kitti_gt_database
+│ ├── xxxxx.bin
+├── kitti_infos_train.pkl
+├── kitti_infos_val.pkl
+├── kitti_dbinfos_train.pkl
+├── kitti_infos_test.pkl
+├── kitti_infos_trainval.pkl
+├── kitti_infos_train_mono3d.coco.json
+├── kitti_infos_trainval_mono3d.coco.json
+├── kitti_infos_test_mono3d.coco.json
+├── kitti_infos_val_mono3d.coco.json
+```
+
+其中的各项文件的含义如下所示:
+
+- `kitti_gt_database/xxxxx.bin`: 训练数据集中包含在 3D 标注框中的点云数据
+- `kitti_infos_train.pkl`:训练数据集的信息,其中每一帧的信息包含下面的内容:
+ - info\['point_cloud'\]: {'num_features': 4, 'velodyne_path': velodyne_path}.
+ - info\['annos'\]: {
+ - 位置:其中 x,y,z 为相机参考坐标系下的目标的底部中心(单位为米),是一个尺寸为 Nx3 的数组
+ - 维度: 目标的高、宽、长(单位为米),是一个尺寸为 Nx3 的数组
+ - 旋转角:相机坐标系下目标绕着 Y 轴的旋转角 ry,其取值范围为 \[-pi..pi\] ,是一个尺寸为 N 的数组
+ - 名称:标准框所包含的目标的名称,是一个尺寸为 N 的数组
+ - 困难度:kitti 官方所定义的困难度,包括 简单,适中,困难
+ - 组别标识符:用于多部件的目标
+ }
+ - (optional) info\['calib'\]: {
+ - P0:校对后的 camera0 投影矩阵,是一个 3x4 数组
+ - P1:校对后的 camera1 投影矩阵,是一个 3x4 数组
+ - P2:校对后的 camera2 投影矩阵,是一个 3x4 数组
+ - P3:校对后的 camera3 投影矩阵,是一个 3x4 数组
+ - R0_rect:校准旋转矩阵,是一个 4x4 数组
+ - Tr_velo_to_cam:从 Velodyne 坐标到相机坐标的变换矩阵,是一个 4x4 数组
+ - Tr_imu_to_velo:从 IMU 坐标到 Velodyne 坐标的变换矩阵,是一个 4x4 数组
+ }
+ - (optional) info\['image'\]:{'image_idx': idx, 'image_path': image_path, 'image_shape', image_shape}.
+
+**注意**:其中的 info\['annos'\] 中的数据均位于相机参考坐标系中,更多的细节请参考[此处](http://www.cvlibs.net/publications/Geiger2013IJRR.pdf)。
+
+获取 kitti_infos_xxx.pkl 和 kitti_infos_xxx_mono3d.coco.json 的核心函数分别为 [get_kitti_image_info](https://github.com/open-mmlab/mmdetection3d/blob/7873c8f62b99314f35079f369d1dab8d63f8a3ce/tools/data_converter/kitti_data_utils.py#L140) 和 [get_2d_boxes](https://github.com/open-mmlab/mmdetection3d/blob/7873c8f62b99314f35079f369d1dab8d63f8a3ce/tools/data_converter/kitti_converter.py#L378).
+
+## 训练流程
+
+下面展示了一个使用 KITTI 数据集进行 3D 目标检测的典型流程:
+
+```python
+train_pipeline = [
+ dict(
+ type='LoadPointsFromFile',
+ coord_type='LIDAR',
+ load_dim=4, # x, y, z, intensity
+ use_dim=4, # x, y, z, intensity
+ file_client_args=file_client_args),
+ dict(
+ type='LoadAnnotations3D',
+ with_bbox_3d=True,
+ with_label_3d=True,
+ file_client_args=file_client_args),
+ dict(type='ObjectSample', db_sampler=db_sampler),
+ dict(
+ type='ObjectNoise',
+ num_try=100,
+ translation_std=[1.0, 1.0, 0.5],
+ global_rot_range=[0.0, 0.0],
+ rot_range=[-0.78539816, 0.78539816]),
+ dict(type='RandomFlip3D', flip_ratio_bev_horizontal=0.5),
+ dict(
+ type='GlobalRotScaleTrans',
+ rot_range=[-0.78539816, 0.78539816],
+ scale_ratio_range=[0.95, 1.05]),
+ dict(type='PointsRangeFilter', point_cloud_range=point_cloud_range),
+ dict(type='ObjectRangeFilter', point_cloud_range=point_cloud_range),
+ dict(type='PointShuffle'),
+ dict(type='DefaultFormatBundle3D', class_names=class_names),
+ dict(type='Collect3D', keys=['points', 'gt_bboxes_3d', 'gt_labels_3d'])
+]
+```
+
+- 数据增强:
+ - `ObjectNoise`:对场景中的每个真实标注框目标添加噪音。
+ - `RandomFlip3D`:对输入点云数据进行随机地水平翻转或者垂直翻转。
+ - `GlobalRotScaleTrans`:对输入点云数据进行旋转。
+
+## 评估
+
+使用 8 个 GPU 以及 KITTI 指标评估的 PointPillars 的示例如下:
+
+```shell
+bash tools/dist_test.sh configs/pointpillars/hv_pointpillars_secfpn_6x8_160e_kitti-3d-3class.py work_dirs/hv_pointpillars_secfpn_6x8_160e_kitti-3d-3class/latest.pth 8 --eval bbox
+```
+
+## 度量指标
+
+KITTI 官方使用全类平均精度(mAP)和平均方向相似度(AOS)来评估 3D 目标检测的性能,请参考[官方网站](http://www.cvlibs.net/datasets/kitti/eval_3dobject.php)和[论文](http://www.cvlibs.net/publications/Geiger2012CVPR.pdf)获取更多细节。
+
+MMDetection3D 采用相同的方法在 KITTI 数据集上进行评估,下面展示了一个评估结果的例子:
+
+```
+Car AP@0.70, 0.70, 0.70:
+bbox AP:97.9252, 89.6183, 88.1564
+bev AP:90.4196, 87.9491, 85.1700
+3d AP:88.3891, 77.1624, 74.4654
+aos AP:97.70, 89.11, 87.38
+Car AP@0.70, 0.50, 0.50:
+bbox AP:97.9252, 89.6183, 88.1564
+bev AP:98.3509, 90.2042, 89.6102
+3d AP:98.2800, 90.1480, 89.4736
+aos AP:97.70, 89.11, 87.38
+```
+
+## 测试和提交
+
+使用 8 个 GPU 在 KITTI 上测试 PointPillars 并生成对排行榜的提交的示例如下:
+
+```shell
+mkdir -p results/kitti-3class
+
+./tools/dist_test.sh configs/pointpillars/hv_pointpillars_secfpn_6x8_160e_kitti-3d-3class.py work_dirs/hv_pointpillars_secfpn_6x8_160e_kitti-3d-3class/latest.pth 8 --out results/kitti-3class/results_eval.pkl --format-only --eval-options 'pklfile_prefix=results/kitti-3class/kitti_results' 'submission_prefix=results/kitti-3class/kitti_results'
+```
+
+在生成 `results/kitti-3class/kitti_results/xxxxx.txt` 后,您可以提交这些文件到 KITTI 官方网站进行基准测试,请参考 [KITTI 官方网站](<(http://www.cvlibs.net/datasets/kitti/index.php)>)获取更多细节。
diff --git a/docs/zh_cn/datasets/lyft_det.md b/docs/zh_cn/datasets/lyft_det.md
new file mode 100644
index 0000000..f02e792
--- /dev/null
+++ b/docs/zh_cn/datasets/lyft_det.md
@@ -0,0 +1,194 @@
+# 3D 目标检测 Lyft 数据集
+
+本页提供了有关在 MMDetection3D 中使用 Lyft 数据集的具体教程。
+
+## 准备之前
+
+您可以在[这里](https://www.kaggle.com/c/3d-object-detection-for-autonomous-vehicles/data)下载 Lyft 3D 检测数据并解压缩所有 zip 文件。
+
+像准备数据集的一般方法一样,建议将数据集根目录链接到 `$MMDETECTION3D/data`。
+
+在进行处理之前,文件夹结构应按如下方式组织:
+
+```
+mmdetection3d
+├── mmdet3d
+├── tools
+├── configs
+├── data
+│ ├── lyft
+│ │ ├── v1.01-train
+│ │ │ ├── v1.01-train (train_data)
+│ │ │ ├── lidar (train_lidar)
+│ │ │ ├── images (train_images)
+│ │ │ ├── maps (train_maps)
+│ │ ├── v1.01-test
+│ │ │ ├── v1.01-test (test_data)
+│ │ │ ├── lidar (test_lidar)
+│ │ │ ├── images (test_images)
+│ │ │ ├── maps (test_maps)
+│ │ ├── train.txt
+│ │ ├── val.txt
+│ │ ├── test.txt
+│ │ ├── sample_submission.csv
+```
+
+其中 `v1.01-train` 和 `v1.01-test` 包含与 nuScenes 数据集相同的元文件,`.txt` 文件包含数据划分的信息。
+Lyft 不提供训练集和验证集的官方划分方案,因此 MMDetection3D 对不同场景下的不同类别的目标数量进行分析,并提供了一个数据集划分方案。
+`sample_submission.csv` 是用于提交到 Kaggle 评估服务器的基本文件。
+需要注意的是,我们遵循了 Lyft 最初的文件夹命名以实现更清楚的文件组织。请将下载下来的原始文件夹重命名按照上述组织结构重新命名。
+
+## 数据准备
+
+组织 Lyft 数据集的方式和组织 nuScenes 的方式相同,首先会生成几乎具有相同结构的 .pkl 和 .json 文件,接着需要重点关注这两个数据集之间的不同点,请参考 [nuScenes 教程](https://github.com/open-mmlab/mmdetection3d/blob/master/docs_zh-CN/datasets/nuscenes_det.md)获取更加详细的数据集信息文件结构的说明。
+
+请通过运行下面的命令来生成 Lyft 的数据集信息文件:
+
+```bash
+python tools/create_data.py lyft --root-path ./data/lyft --out-dir ./data/lyft --extra-tag lyft --version v1.01
+python tools/data_converter/lyft_data_fixer.py --version v1.01 --root-folder ./data/lyft
+```
+
+请注意,上面的第二行命令用于修复损坏的 lidar 数据文件,请参考[此处](https://www.kaggle.com/c/3d-object-detection-for-autonomous-vehicles/discussion/110000)获取更多细节。
+
+处理后的文件夹结构应该如下:
+
+```
+mmdetection3d
+├── mmdet3d
+├── tools
+├── configs
+├── data
+│ ├── lyft
+│ │ ├── v1.01-train
+│ │ │ ├── v1.01-train (train_data)
+│ │ │ ├── lidar (train_lidar)
+│ │ │ ├── images (train_images)
+│ │ │ ├── maps (train_maps)
+│ │ ├── v1.01-test
+│ │ │ ├── v1.01-test (test_data)
+│ │ │ ├── lidar (test_lidar)
+│ │ │ ├── images (test_images)
+│ │ │ ├── maps (test_maps)
+│ │ ├── train.txt
+│ │ ├── val.txt
+│ │ ├── test.txt
+│ │ ├── sample_submission.csv
+│ │ ├── lyft_infos_train.pkl
+│ │ ├── lyft_infos_val.pkl
+│ │ ├── lyft_infos_test.pkl
+│ │ ├── lyft_infos_train_mono3d.coco.json
+│ │ ├── lyft_infos_val_mono3d.coco.json
+│ │ ├── lyft_infos_test_mono3d.coco.json
+```
+
+其中,.pkl 文件通常适用于涉及到点云的相关方法,coco 类型的 .json 文件更加适用于涉及到基于图像的相关方法,如基于图像的 2D 和 3D 目标检测。
+不同于 nuScenes 数据集,这里仅能使用 json 文件进行 2D 检测相关的实验,未来将会进一步支持基于图像的 3D 检测。
+
+接下来将详细介绍 Lyft 数据集和 nuScenes 数据集之间的数据集信息文件中的不同点:
+
+- `lyft_database/xxxxx.bin` 文件不存在:由于真实标注框的采样对实验的影响可以忽略不计,在 Lyft 数据集中不会提取该目录和相关的 `.bin` 文件。
+- `lyft_infos_train.pkl`:包含训练数据集信息,每一帧包含两个关键字:`metadata` 和 `infos`。
+ `metadata` 包含数据集自身的基础信息,如 `{'version': 'v1.01-train'}`,然而 `infos` 包含和 nuScenes 数据集相似的数据集详细信息,但是并不包含一下几点:
+ - info\['sweeps'\]:扫描信息.
+ - info\['sweeps'\]\[i\]\['type'\]:扫描信息的数据类型,如 `'lidar'`。
+ Lyft 数据集中的一些样例具有不同的 LiDAR 设置,然而为了数据分布的一致性,这里将一直采用顶部的 LiDAR 设备所采集的数据点信息。
+ - info\['gt_names'\]:在 Lyft 数据集中有 9 个类别,相比于 nuScenes 数据集,不同类别的标注不平衡问题更加突出。
+ - info\['gt_velocity'\] 不存在:Lyft 数据集中不存在速度评估信息。
+ - info\['num_lidar_pts'\]:默认值设置为 -1。
+ - info\['num_radar_pts'\]:默认值设置为 0。
+ - info\['valid_flag'\] 不存在:这个标志信息因无效的 `num_lidar_pts` 和 `num_radar_pts` 的存在而存在。
+- `nuscenes_infos_train_mono3d.coco.json`:包含 coco 类型的训练数据集相关的信息。这个文件仅包含 2D 相关的信息,不包含 3D 目标检测所需要的信息,如相机内参。
+ - info\['images'\]:包含所有图像信息的列表。
+ - 仅包含 `'file_name'`, `'id'`, `'width'`, `'height'`。
+ - info\['annotations'\]:包含所有标注信息的列表。
+ - 仅包含 `'file_name'`,`'image_id'`,`'area'`,`'category_name'`,`'category_id'`,`'bbox'`,`'is_crowd'`,`'segmentation'`,`'id'`,其中 `'is_crowd'` 和 `'segmentation'` 默认设置为 `0` 和 `[]`。
+ Lyft 数据集中不包含属性标注信息。
+
+这里仅介绍存储在训练数据文件的数据记录信息,在测试数据集也采用上述的数据记录方式。
+
+获取 `lyft_infos_xxx.pkl` 的核心函数是 [\_fill_trainval_infos](https://github.com/open-mmlab/mmdetection3d/blob/master/tools/data_converter/lyft_converter.py#L93)。
+请参考 [lyft_converter.py](https://github.com/open-mmlab/mmdetection3d/blob/master/tools/data_converter/lyft_converter.py) 获取更多细节。
+
+## 训练流程
+
+### 基于 LiDAR 的方法
+
+Lyft 上基于 LiDAR 的 3D 检测(包括多模态方法)的训练流程与 nuScenes 几乎相同,如下所示:
+
+```python
+train_pipeline = [
+ dict(
+ type='LoadPointsFromFile',
+ coord_type='LIDAR',
+ load_dim=5,
+ use_dim=5,
+ file_client_args=file_client_args),
+ dict(
+ type='LoadPointsFromMultiSweeps',
+ sweeps_num=10,
+ file_client_args=file_client_args),
+ dict(type='LoadAnnotations3D', with_bbox_3d=True, with_label_3d=True),
+ dict(
+ type='GlobalRotScaleTrans',
+ rot_range=[-0.3925, 0.3925],
+ scale_ratio_range=[0.95, 1.05],
+ translation_std=[0, 0, 0]),
+ dict(type='RandomFlip3D', flip_ratio_bev_horizontal=0.5),
+ dict(type='PointsRangeFilter', point_cloud_range=point_cloud_range),
+ dict(type='ObjectRangeFilter', point_cloud_range=point_cloud_range),
+ dict(type='PointShuffle'),
+ dict(type='DefaultFormatBundle3D', class_names=class_names),
+ dict(type='Collect3D', keys=['points', 'gt_bboxes_3d', 'gt_labels_3d'])
+]
+```
+
+与 nuScenes 相似,在 Lyft 上进行训练的模型也需要 `LoadPointsFromMultiSweeps` 步骤来从连续帧中加载点云数据。
+另外,考虑到 Lyft 中所收集的激光雷达点的强度是无效的,因此将 `LoadPointsFromMultiSweeps` 中的 `use_dim` 默认值设置为 `[0, 1, 2, 4]`,其中前三个维度表示点的坐标,最后一个维度表示时间戳的差异。
+
+## 评估
+
+使用 8 个 GPU 以及 Lyft 指标评估的 PointPillars 的示例如下:
+
+```shell
+bash ./tools/dist_test.sh configs/pointpillars/hv_pointpillars_fpn_sbn-all_2x8_2x_lyft-3d.py checkpoints/hv_pointpillars_fpn_sbn-all_2x8_2x_lyft-3d_20210517_202818-fc6904c3.pth 8 --eval bbox
+```
+
+## 度量指标
+
+Lyft 提出了一个更加严格的用以评估所预测的 3D 检测框的度量指标。
+判断一个预测框是否是正类的基本评判标准和 KITTI 一样,如基于 3D 交并比进行评估,然而,Lyft 采用与 COCO 相似的方式来计算平均精度 -- 计算 3D 交并比在 0.5-0.95 之间的不同阈值下的平均精度。
+实际上,重叠部分大于 0.7 的 3D 交并比是一项对于 3D 检测方法比较严格的标准,因此整体的性能似乎会偏低。
+相比于其他数据集,Lyft 上不同类别的标注不平衡是导致最终结果偏低的另一个重要原因。
+请参考[官方网址](https://www.kaggle.com/c/3d-object-detection-for-autonomous-vehicles/overview/evaluation)获取更多关于度量指标的定义的细节。
+
+这里将采用官方方法对 Lyft 进行评估,下面展示了一个评估结果的例子:
+
+```
++mAPs@0.5:0.95------+--------------+
+| class | mAP@0.5:0.95 |
++-------------------+--------------+
+| animal | 0.0 |
+| bicycle | 0.099 |
+| bus | 0.177 |
+| car | 0.422 |
+| emergency_vehicle | 0.0 |
+| motorcycle | 0.049 |
+| other_vehicle | 0.359 |
+| pedestrian | 0.066 |
+| truck | 0.176 |
+| Overall | 0.15 |
++-------------------+--------------+
+```
+
+## 测试和提交
+
+使用 8 个 GPU 在 Lyft 上测试 PointPillars 并生成对排行榜的提交的示例如下:
+
+```shell
+./tools/dist_test.sh configs/pointpillars/hv_pointpillars_fpn_sbn-all_2x8_2x_lyft-3d.py work_dirs/pp-lyft/latest.pth 8 --out work_dirs/pp-lyft/results_challenge.pkl --format-only --eval-options 'jsonfile_prefix=work_dirs/pp-lyft/results_challenge' 'csv_savepath=results/pp-lyft/results_challenge.csv'
+```
+
+在生成 `work_dirs/pp-lyft/results_challenge.csv`,您可以将生成的文件提交到 Kaggle 评估服务器,请参考[官方网址](https://www.kaggle.com/c/3d-object-detection-for-autonomous-vehicles)获取更多细节。
+
+同时还可以使用可视化工具将预测结果进行可视化,请参考[可视化文档](https://mmdetection3d.readthedocs.io/zh_CN/latest/useful_tools.html#visualization)获取更多细节。
diff --git a/docs/zh_cn/datasets/nuscenes_det.md b/docs/zh_cn/datasets/nuscenes_det.md
new file mode 100644
index 0000000..6bc054e
--- /dev/null
+++ b/docs/zh_cn/datasets/nuscenes_det.md
@@ -0,0 +1,260 @@
+# 3D 目标检测 NuScenes 数据集
+
+本页提供了有关在 MMDetection3D 中使用 nuScenes 数据集的具体教程。
+
+## 准备之前
+
+您可以在[这里](https://www.nuscenes.org/download)下载 nuScenes 3D 检测数据并解压缩所有 zip 文件。
+
+像准备数据集的一般方法一样,建议将数据集根目录链接到 `$MMDETECTION3D/data`。
+
+在我们处理之前,文件夹结构应按如下方式组织。
+
+```
+mmdetection3d
+├── mmdet3d
+├── tools
+├── configs
+├── data
+│ ├── nuscenes
+│ │ ├── maps
+│ │ ├── samples
+│ │ ├── sweeps
+│ │ ├── v1.0-test
+| | ├── v1.0-trainval
+```
+
+## 数据准备
+
+我们通常需要通过特定样式来使用 .pkl 或 .json 文件组织有用的数据信息,例如用于组织图像及其标注的 coco 样式。
+要为 nuScenes 准备这些文件,请运行以下命令:
+
+```bash
+python tools/create_data.py nuscenes --root-path ./data/nuscenes --out-dir ./data/nuscenes --extra-tag nuscenes
+```
+
+处理后的文件夹结构应该如下
+
+```
+mmdetection3d
+├── mmdet3d
+├── tools
+├── configs
+├── data
+│ ├── nuscenes
+│ │ ├── maps
+│ │ ├── samples
+│ │ ├── sweeps
+│ │ ├── v1.0-test
+| | ├── v1.0-trainval
+│ │ ├── nuscenes_database
+│ │ ├── nuscenes_infos_train.pkl
+│ │ ├── nuscenes_infos_trainval.pkl
+│ │ ├── nuscenes_infos_val.pkl
+│ │ ├── nuscenes_infos_test.pkl
+│ │ ├── nuscenes_dbinfos_train.pkl
+│ │ ├── nuscenes_infos_train_mono3d.coco.json
+│ │ ├── nuscenes_infos_trainval_mono3d.coco.json
+│ │ ├── nuscenes_infos_val_mono3d.coco.json
+│ │ ├── nuscenes_infos_test_mono3d.coco.json
+```
+
+这里,.pkl 文件一般用于涉及点云的方法,coco 风格的 .json 文件更适合基于图像的方法,例如基于图像的 2D 和 3D 检测。
+接下来,我们将详细说明这些信息文件中记录的细节。
+
+- `nuscenes_database/xxxxx.bin`:训练数据集的每个 3D 包围框中包含的点云数据。
+- `nuscenes_infos_train.pkl`:训练数据集信息,每帧信息有两个键值: `metadata` 和 `infos`。 `metadata` 包含数据集本身的基本信息,例如 `{'version': 'v1.0-trainval'}`,而 `infos` 包含详细信息如下:
+ - info\['lidar_path'\]:激光雷达点云数据的文件路径。
+ - info\['token'\]:样本数据标记。
+ - info\['sweeps'\]:扫描信息(nuScenes 中的 `sweeps` 是指没有标注的中间帧,而 `samples` 是指那些带有标注的关键帧)。
+ - info\['sweeps'\]\[i\]\['data_path'\]:第 i 次扫描的数据路径。
+ - info\['sweeps'\]\[i\]\['type'\]:扫描数据类型,例如“激光雷达”。
+ - info\['sweeps'\]\[i\]\['sample_data_token'\]:扫描样本数据标记。
+ - info\['sweeps'\]\[i\]\['sensor2ego_translation'\]:从当前传感器(用于收集扫描数据)到自车(包含感知周围环境传感器的车辆,车辆坐标系固连在自车上)的转换(1x3 列表)。
+ - info\['sweeps'\]\[i\]\['sensor2ego_rotation'\]:从当前传感器(用于收集扫描数据)到自车的旋转(四元数格式的 1x4 列表)。
+ - info\['sweeps'\]\[i\]\['ego2global_translation'\]:从自车到全局坐标的转换(1x3 列表)。
+ - info\['sweeps'\]\[i\]\['ego2global_rotation'\]:从自车到全局坐标的旋转(四元数格式的 1x4 列表)。
+ - info\['sweeps'\]\[i\]\['timestamp'\]:扫描数据的时间戳。
+ - info\['sweeps'\]\[i\]\['sensor2lidar_translation'\]:从当前传感器(用于收集扫描数据)到激光雷达的转换(1x3 列表)。
+ - info\['sweeps'\]\[i\]\['sensor2lidar_rotation'\]:从当前传感器(用于收集扫描数据)到激光雷达的旋转(四元数格式的 1x4 列表)。
+ - info\['cams'\]:相机校准信息。它包含与每个摄像头对应的六个键值: `'CAM_FRONT'`, `'CAM_FRONT_RIGHT'`, `'CAM_FRONT_LEFT'`, `'CAM_BACK'`, `'CAM_BACK_LEFT'`, `'CAM_BACK_RIGHT'`。
+ 每个字典包含每个扫描数据按照上述方式的详细信息(每个信息的关键字与上述相同)。除此之外,每个相机还包含了一个键值 `'cam_intrinsic'` 用来保存 3D 点投影到图像平面上需要的内参信息。
+ - info\['lidar2ego_translation'\]:从激光雷达到自车的转换(1x3 列表)。
+ - info\['lidar2ego_rotation'\]:从激光雷达到自车的旋转(四元数格式的 1x4 列表)。
+ - info\['ego2global_translation'\]:从自车到全局坐标的转换(1x3 列表)。
+ - info\['ego2global_rotation'\]:从自我车辆到全局坐标的旋转(四元数格式的 1x4 列表)。
+ - info\['timestamp'\]:样本数据的时间戳。
+ - info\['gt_boxes'\]:7 个自由度的 3D 包围框,一个 Nx7 数组。
+ - info\['gt_names'\]:3D 包围框的类别,一个 1xN 数组。
+ - info\['gt_velocity'\]:3D 包围框的速度(由于不准确,没有垂直测量),一个 Nx2 数组。
+ - info\['num_lidar_pts'\]:每个 3D 包围框中包含的激光雷达点数。
+ - info\['num_radar_pts'\]:每个 3D 包围框中包含的雷达点数。
+ - info\['valid_flag'\]:每个包围框是否有效。一般情况下,我们只将包含至少一个激光雷达或雷达点的 3D 框作为有效框。
+- `nuscenes_infos_train_mono3d.coco.json`:训练数据集 coco 风格的信息。该文件将基于图像的数据组织为三类(键值):`'categories'`, `'images'`, `'annotations'`。
+ - info\['categories'\]:包含所有类别名称的列表。每个元素都遵循字典格式并由两个键值组成:`'id'` 和 `'name'`。
+ - info\['images'\]:包含所有图像信息的列表。
+ - info\['images'\]\[i\]\['file_name'\]:第 i 张图像的文件名。
+ - info\['images'\]\[i\]\['id'\]:第 i 张图像的样本数据标记。
+ - info\['images'\]\[i\]\['token'\]:与该帧对应的样本标记。
+ - info\['images'\]\[i\]\['cam2ego_rotation'\]:从相机到自车的旋转(四元数格式的 1x4 列表)。
+ - info\['images'\]\[i\]\['cam2ego_translation'\]:从相机到自车的转换(1x3 列表)。
+ - info\['images'\]\[i\]\['ego2global_rotation''\]:从自车到全局坐标的旋转(四元数格式的 1x4 列表)。
+ - info\['images'\]\[i\]\['ego2global_translation'\]:从自车到全局坐标的转换(1x3 列表)。
+ - info\['images'\]\[i\]\['cam_intrinsic'\]: 相机内参矩阵(3x3 列表)。
+ - info\['images'\]\[i\]\['width'\]:图片宽度, nuScenes 中默认为 1600。
+ - info\['images'\]\[i\]\['height'\]:图像高度, nuScenes 中默认为 900。
+ - info\['annotations'\]: 包含所有标注信息的列表。
+ - info\['annotations'\]\[i\]\['file_name'\]:对应图像的文件名。
+ - info\['annotations'\]\[i\]\['image_id'\]:对应图像的图像 ID (标记)。
+ - info\['annotations'\]\[i\]\['area'\]:2D 包围框的面积。
+ - info\['annotations'\]\[i\]\['category_name'\]:类别名称。
+ - info\['annotations'\]\[i\]\['category_id'\]:类别 id。
+ - info\['annotations'\]\[i\]\['bbox'\]:2D 包围框标注(3D 投影框的外部矩形),1x4 列表跟随 \[x1, y1, x2-x1, y2-y1\]。x1/y1 是沿图像水平/垂直方向的最小坐标。
+ - info\['annotations'\]\[i\]\['iscrowd'\]:该区域是否拥挤。默认为 0。
+ - info\['annotations'\]\[i\]\['bbox_cam3d'\]:3D 包围框(重力)中心位置(3)、大小(3)、(全局)偏航角(1)、1x7 列表。
+ - info\['annotations'\]\[i\]\['velo_cam3d'\]:3D 包围框的速度(由于不准确,没有垂直测量),一个 Nx2 数组。
+ - info\['annotations'\]\[i\]\['center2d'\]:包含 2.5D 信息的投影 3D 中心:图像上的投影中心位置(2)和深度(1),1x3 列表。
+ - info\['annotations'\]\[i\]\['attribute_name'\]:属性名称。
+ - info\['annotations'\]\[i\]\['attribute_id'\]:属性 ID。
+ 我们为属性分类维护了一个属性集合和映射。更多的细节请参考[这里](https://github.com/open-mmlab/mmdetection3d/blob/master/mmdet3d/datasets/nuscenes_mono_dataset.py#L53)。
+ - info\['annotations'\]\[i\]\['id'\]:标注 ID。默认为 `i`。
+
+这里我们只解释训练信息文件中记录的数据。这同样适用于验证和测试集。
+获取 `nuscenes_infos_xxx.pkl` 和 `nuscenes_infos_xxx_mono3d.coco.json` 的核心函数分别为 [\_fill_trainval_infos](https://github.com/open-mmlab/mmdetection3d/blob/master/tools/data_converter/nuscenes_converter.py#L143) 和 [get_2d_boxes](https://github.com/open-mmlab/mmdetection3d/blob/master/tools/data_converter/nuscenes_converter.py#L397)。更多细节请参考 [nuscenes_converter.py](https://github.com/open-mmlab/mmdetection3d/blob/master/tools/data_converter/nuscenes_converter.py)。
+
+## 训练流程
+
+### 基于 LiDAR 的方法
+
+nuScenes 上基于 LiDAR 的 3D 检测(包括多模态方法)的典型训练流程如下。
+
+```python
+train_pipeline = [
+ dict(
+ type='LoadPointsFromFile',
+ coord_type='LIDAR',
+ load_dim=5,
+ use_dim=5,
+ file_client_args=file_client_args),
+ dict(
+ type='LoadPointsFromMultiSweeps',
+ sweeps_num=10,
+ file_client_args=file_client_args),
+ dict(type='LoadAnnotations3D', with_bbox_3d=True, with_label_3d=True),
+ dict(
+ type='GlobalRotScaleTrans',
+ rot_range=[-0.3925, 0.3925],
+ scale_ratio_range=[0.95, 1.05],
+ translation_std=[0, 0, 0]),
+ dict(type='RandomFlip3D', flip_ratio_bev_horizontal=0.5),
+ dict(type='PointsRangeFilter', point_cloud_range=point_cloud_range),
+ dict(type='ObjectRangeFilter', point_cloud_range=point_cloud_range),
+ dict(type='ObjectNameFilter', classes=class_names),
+ dict(type='PointShuffle'),
+ dict(type='DefaultFormatBundle3D', class_names=class_names),
+ dict(type='Collect3D', keys=['points', 'gt_bboxes_3d', 'gt_labels_3d'])
+]
+```
+
+与一般情况相比,nuScenes 有一个特定的 `'LoadPointsFromMultiSweeps'` 流水线来从连续帧加载点云。这是此设置中使用的常见做法。
+更多细节请参考 nuScenes [原始论文](https://arxiv.org/abs/1903.11027)。
+`'LoadPointsFromMultiSweeps'` 中的默认 `use_dim` 是 `[0, 1, 2, 4]`,其中前 3 个维度是指点坐标,最后一个是指时间戳差异。
+由于在拼接来自不同帧的点时使用点云的强度信息会产生噪声,因此默认情况下不使用点云的强度信息。
+
+### 基于视觉的方法
+
+nuScenes 上基于图像的 3D 检测的典型训练流水线如下。
+
+```python
+train_pipeline = [
+ dict(type='LoadImageFromFileMono3D'),
+ dict(
+ type='LoadAnnotations3D',
+ with_bbox=True,
+ with_label=True,
+ with_attr_label=True,
+ with_bbox_3d=True,
+ with_label_3d=True,
+ with_bbox_depth=True),
+ dict(type='Resize', img_scale=(1600, 900), keep_ratio=True),
+ dict(type='RandomFlip3D', flip_ratio_bev_horizontal=0.5),
+ dict(type='Normalize', **img_norm_cfg),
+ dict(type='Pad', size_divisor=32),
+ dict(type='DefaultFormatBundle3D', class_names=class_names),
+ dict(
+ type='Collect3D',
+ keys=[
+ 'img', 'gt_bboxes', 'gt_labels', 'attr_labels', 'gt_bboxes_3d',
+ 'gt_labels_3d', 'centers2d', 'depths'
+ ]),
+]
+```
+
+它遵循 2D 检测的一般流水线,但在一些细节上有所不同:
+
+- 它使用单目流水线加载图像,其中包括额外的必需信息,如相机内参矩阵。
+- 它需要加载 3D 标注。
+- 一些数据增强技术需要调整,例如`RandomFlip3D`。
+ 目前我们不支持更多的增强方法,因为如何迁移和应用其他技术仍在探索中。
+
+## 评估
+
+使用 8 个 GPU 以及 nuScenes 指标评估的 PointPillars 的示例如下
+
+```shell
+bash ./tools/dist_test.sh configs/pointpillars/hv_pointpillars_fpn_sbn-all_4x8_2x_nus-3d.py checkpoints/hv_pointpillars_fpn_sbn-all_4x8_2x_nus-3d_20200620_230405-2fa62f3d.pth 8 --eval bbox
+```
+
+## 指标
+
+NuScenes 提出了一个综合指标,即 nuScenes 检测分数(NDS),以评估不同的方法并设置基准测试。
+它由平均精度(mAP)、平均平移误差(ATE)、平均尺度误差(ASE)、平均方向误差(AOE)、平均速度误差(AVE)和平均属性误差(AAE)组成。
+更多细节请参考其[官方网站](https://www.nuscenes.org/object-detection?externalData=all&mapData=all&modalities=Any)。
+
+我们也采用这种方法对 nuScenes 进行评估。打印的评估结果示例如下:
+
+```
+mAP: 0.3197
+mATE: 0.7595
+mASE: 0.2700
+mAOE: 0.4918
+mAVE: 1.3307
+mAAE: 0.1724
+NDS: 0.3905
+Eval time: 170.8s
+
+Per-class results:
+Object Class AP ATE ASE AOE AVE AAE
+car 0.503 0.577 0.152 0.111 2.096 0.136
+truck 0.223 0.857 0.224 0.220 1.389 0.179
+bus 0.294 0.855 0.204 0.190 2.689 0.283
+trailer 0.081 1.094 0.243 0.553 0.742 0.167
+construction_vehicle 0.058 1.017 0.450 1.019 0.137 0.341
+pedestrian 0.392 0.687 0.284 0.694 0.876 0.158
+motorcycle 0.317 0.737 0.265 0.580 2.033 0.104
+bicycle 0.308 0.704 0.299 0.892 0.683 0.010
+traffic_cone 0.555 0.486 0.309 nan nan nan
+barrier 0.466 0.581 0.269 0.169 nan nan
+```
+
+## 测试和提交
+
+使用 8 个 GPU 在 nuScenes 上测试 PointPillars 并生成对排行榜的提交的示例如下
+
+```shell
+./tools/dist_test.sh configs/pointpillars/hv_pointpillars_fpn_sbn-all_4x8_2x_nus-3d.py work_dirs/hv_pointpillars_secfpn_6x8_160e_kitti-3d-3class/latest.pth 8 --out work_dirs/pp-nus/results_eval.pkl --format-only --eval-options 'jsonfile_prefix=work_dirs/pp-nus/results_eval'
+```
+
+请注意,在[这里](https://github.com/open-mmlab/mmdetection3d/blob/master/configs/_base_/datasets/nus-3d.py#L132)测试信息应更改为测试集而不是验证集。
+
+生成 `work_dirs/pp-nus/results_eval.json` 后,您可以压缩并提交给 nuScenes 基准测试。更多信息请参考 [nuScenes 官方网站](https://www.nuscenes.org/object-detection?externalData=all&mapData=all&modalities=Any)。
+
+我们还可以使用我们开发的可视化工具将预测结果可视化。更多细节请参考[可视化文档](https://mmdetection3d.readthedocs.io/zh_CN/latest/useful_tools.html#id2)。
+
+## 注意
+
+### `NuScenesBox` 和我们的 `CameraInstanceBoxes` 之间的转换。
+
+总的来说,`NuScenesBox` 和我们的 `CameraInstanceBoxes` 的主要区别主要体现在转向角(yaw)定义上。 `NuScenesBox` 定义了一个四元数或三个欧拉角的旋转,而我们的由于实际情况只定义了一个转向角(yaw),它需要我们在预处理和后处理中手动添加一些额外的旋转,例如[这里](https://github.com/open-mmlab/mmdetection3d/blob/master/mmdet3d/datasets/nuscenes_mono_dataset.py#L673)。
+
+另外,请注意,角点和位置的定义在 `NuScenesBox` 中是分离的。例如,在单目 3D 检测中,框位置的定义在其相机坐标中(有关汽车设置,请参阅其官方[插图](https://www.nuscenes.org/nuscenes#data-collection)),即与[我们的](https://github.com/open-mmlab/mmdetection3d/blob/master/mmdet3d/core/bbox/structures/cam_box3d.py)一致。相比之下,它的角点是通过[惯例](https://github.com/nutonomy/nuscenes-devkit/blob/02e9200218977193a1058dd7234f935834378319/python-sdk/nuscenes/utils/data_classes.py#L527) 定义的,“x 向前, y 向左, z 向上”。它导致了与我们的 `CameraInstanceBoxes` 不同的维度和旋转定义理念。一个移除相似冲突的例子是 PR [#744](https://github.com/open-mmlab/mmdetection3d/pull/744)。同样的问题也存在于 LiDAR 系统中。为了解决它们,我们通常会在预处理和后处理中添加一些转换,以保证在整个训练和推理过程中框都在我们的坐标系系统里。
diff --git a/docs/zh_cn/datasets/s3dis_sem_seg.md b/docs/zh_cn/datasets/s3dis_sem_seg.md
new file mode 100644
index 0000000..86adb02
--- /dev/null
+++ b/docs/zh_cn/datasets/s3dis_sem_seg.md
@@ -0,0 +1,263 @@
+# 3D 语义分割 S3DIS 数据集
+
+## 数据集的准备
+
+对于数据集准备的整体流程,请参考 S3DIS 的[指南](https://github.com/open-mmlab/mmdetection3d/blob/master/data/s3dis/README.md/)。
+
+### 提取 S3DIS 数据
+
+通过从原始数据中提取 S3DIS 数据,我们将点云数据读取并保存下相关的标注信息,例如语义分割标签和实例分割标签。
+
+数据提取前的目录结构应该如下所示:
+
+```
+mmdetection3d
+├── mmdet3d
+├── tools
+├── configs
+├── data
+│ ├── s3dis
+│ │ ├── meta_data
+│ │ ├── Stanford3dDataset_v1.2_Aligned_Version
+│ │ │ ├── Area_1
+│ │ │ │ ├── conferenceRoom_1
+│ │ │ │ ├── office_1
+│ │ │ │ ├── ...
+│ │ │ ├── Area_2
+│ │ │ ├── Area_3
+│ │ │ ├── Area_4
+│ │ │ ├── Area_5
+│ │ │ ├── Area_6
+│ │ ├── indoor3d_util.py
+│ │ ├── collect_indoor3d_data.py
+│ │ ├── README.md
+```
+
+在 `Stanford3dDataset_v1.2_Aligned_Version` 目录下,所有房间依据所属区域被分为 6 组。
+我们通常使用 5 个区域进行训练,然后在余下 1 个区域上进行测试 (被余下的 1 个区域通常为区域 5)。
+在每个区域的目录下包含有多个房间的文件夹,每个文件夹是一个房间的原始点云数据和相关的标注信息。
+例如,在 `Area_1/office_1` 目录下的文件如下所示:
+
+- `office_1.txt`:一个 txt 文件存储着原始点云数据每个点的坐标和颜色信息。
+
+- `Annotations/`:这个文件夹里包含有此房间中实例物体的信息 (以 txt 文件的形式存储)。每个 txt 文件表示一个实例,例如:
+
+ - `chair_1.txt`:存储有该房间中一把椅子的点云数据。
+
+ 如果我们将 `Annotations/` 下的所有 txt 文件合并起来,得到的点云就和 `office_1.txt` 中的点云是一致的。
+
+你可以通过 `python collect_indoor3d_data.py` 指令进行 S3DIS 数据的提取。
+主要步骤包括:
+
+- 从原始 txt 文件中读取点云数据、语义分割标签和实例分割标签。
+- 将点云数据和相关标注文件存储下来。
+
+这其中的核心函数 `indoor3d_util.py` 中的 `export` 函数实现如下:
+
+```python
+def export(anno_path, out_filename):
+ """将原始数据集的文件转化为点云、语义分割标签和实例分割掩码文件。
+ 我们将同一房间中所有实例的点进行聚合。
+
+ 参数列表:
+ anno_path (str): 标注信息的路径,例如 Area_1/office_2/Annotations/
+ out_filename (str): 保存点云和标签的路径
+ file_format (str): txt 或 numpy,指定保存的文件格式
+
+ 注意:
+ 点云在处理过程中被整体移动了,保存下的点最小位于原点 (即没有负数坐标值)
+ """
+ points_list = []
+ ins_idx = 1 # 实例标签从 1 开始,因此最终实例标签为 0 的点就是无标注的点
+
+ # `anno_path` 的一个例子:Area_1/office_1/Annotations
+ # 其中以 txt 文件存储有该房间中所有实例物体的点云
+ for f in glob.glob(osp.join(anno_path, '*.txt')):
+ # get class name of this instance
+ one_class = osp.basename(f).split('_')[0]
+ if one_class not in class_names: # 某些房间有 'staris' 类物体
+ one_class = 'clutter'
+ points = np.loadtxt(f)
+ labels = np.ones((points.shape[0], 1)) * class2label[one_class]
+ ins_labels = np.ones((points.shape[0], 1)) * ins_idx
+ ins_idx += 1
+ points_list.append(np.concatenate([points, labels, ins_labels], 1))
+
+ data_label = np.concatenate(points_list, 0) # [N, 8], (pts, rgb, sem, ins)
+ # 将点云对齐到原点
+ xyz_min = np.amin(data_label, axis=0)[0:3]
+ data_label[:, 0:3] -= xyz_min
+
+ np.save(f'{out_filename}_point.npy', data_label[:, :6].astype(np.float32))
+ np.save(f'{out_filename}_sem_label.npy', data_label[:, 6].astype(np.int))
+ np.save(f'{out_filename}_ins_label.npy', data_label[:, 7].astype(np.int))
+
+```
+
+上述代码中,我们读取 `Annotations/` 下的所有点云实例,将其合并得到整体房屋的点云,同时生成语义/实例分割的标签。
+在提取完每个房间的数据后,点云、语义分割和实例分割的标签文件应以 `.npy` 的格式被保存下来。
+
+### 创建数据集
+
+```shell
+python tools/create_data.py s3dis --root-path ./data/s3dis \
+--out-dir ./data/s3dis --extra-tag s3dis
+```
+
+上述指令首先读取以 `.npy` 格式存储的点云、语义分割和实例分割标签文件,然后进一步将它们以 `.bin` 格式保存。
+同时,每个区域 `.pkl` 格式的信息文件也会被保存下来。
+
+数据预处理后的目录结构如下所示:
+
+```
+s3dis
+├── meta_data
+├── indoor3d_util.py
+├── collect_indoor3d_data.py
+├── README.md
+├── Stanford3dDataset_v1.2_Aligned_Version
+├── s3dis_data
+├── points
+│ ├── xxxxx.bin
+├── instance_mask
+│ ├── xxxxx.bin
+├── semantic_mask
+│ ├── xxxxx.bin
+├── seg_info
+│ ├── Area_1_label_weight.npy
+│ ├── Area_1_resampled_scene_idxs.npy
+│ ├── Area_2_label_weight.npy
+│ ├── Area_2_resampled_scene_idxs.npy
+│ ├── Area_3_label_weight.npy
+│ ├── Area_3_resampled_scene_idxs.npy
+│ ├── Area_4_label_weight.npy
+│ ├── Area_4_resampled_scene_idxs.npy
+│ ├── Area_5_label_weight.npy
+│ ├── Area_5_resampled_scene_idxs.npy
+│ ├── Area_6_label_weight.npy
+│ ├── Area_6_resampled_scene_idxs.npy
+├── s3dis_infos_Area_1.pkl
+├── s3dis_infos_Area_2.pkl
+├── s3dis_infos_Area_3.pkl
+├── s3dis_infos_Area_4.pkl
+├── s3dis_infos_Area_5.pkl
+├── s3dis_infos_Area_6.pkl
+```
+
+- `points/xxxxx.bin`:提取的点云数据。
+- `instance_mask/xxxxx.bin`:每个点云的实例标签,取值范围为 \[0, ${实例个数}\],其中 0 代表未标注的点。
+- `semantic_mask/xxxxx.bin`:每个点云的语义标签,取值范围为 \[0, 12\]。
+- `s3dis_infos_Area_1.pkl`:区域 1 的数据信息,每个房间的详细信息如下:
+ - info\['point_cloud'\]: {'num_features': 6, 'lidar_idx': sample_idx}.
+ - info\['pts_path'\]: `points/xxxxx.bin` 点云的路径。
+ - info\['pts_instance_mask_path'\]: `instance_mask/xxxxx.bin` 实例标签的路径。
+ - info\['pts_semantic_mask_path'\]: `semantic_mask/xxxxx.bin` 语义标签的路径。
+- `seg_info`:为支持语义分割任务所生成的信息文件。
+ - `Area_1_label_weight.npy`:每一语义类别的权重系数。因为 S3DIS 中属于不同类的点的数量相差很大,一个常见的操作是在计算损失时对不同类别进行加权 (label re-weighting) 以得到更好的分割性能。
+ - `Area_1_resampled_scene_idxs.npy`:每一个场景 (房间) 的重采样标签。在训练过程中,我们依据每个场景的点的数量,会对其进行不同次数的重采样,以保证训练数据均衡。
+
+## 训练流程
+
+S3DIS 上 3D 语义分割的一种典型数据载入流程如下所示:
+
+```python
+class_names = ('ceiling', 'floor', 'wall', 'beam', 'column', 'window', 'door',
+ 'table', 'chair', 'sofa', 'bookcase', 'board', 'clutter')
+num_points = 4096
+train_pipeline = [
+ dict(
+ type='LoadPointsFromFile',
+ coord_type='DEPTH',
+ shift_height=False,
+ use_color=True,
+ load_dim=6,
+ use_dim=[0, 1, 2, 3, 4, 5]),
+ dict(
+ type='LoadAnnotations3D',
+ with_bbox_3d=False,
+ with_label_3d=False,
+ with_mask_3d=False,
+ with_seg_3d=True),
+ dict(
+ type='PointSegClassMapping',
+ valid_cat_ids=tuple(range(len(class_names))),
+ max_cat_id=13),
+ dict(
+ type='IndoorPatchPointSample',
+ num_points=num_points,
+ block_size=1.0,
+ ignore_index=None,
+ use_normalized_coord=True,
+ enlarge_size=None,
+ min_unique_num=num_points // 4,
+ eps=0.0),
+ dict(type='NormalizePointsColor', color_mean=None),
+ dict(
+ type='GlobalRotScaleTrans',
+ rot_range=[-3.141592653589793, 3.141592653589793], # [-pi, pi]
+ scale_ratio_range=[0.8, 1.2],
+ translation_std=[0, 0, 0]),
+ dict(
+ type='RandomJitterPoints',
+ jitter_std=[0.01, 0.01, 0.01],
+ clip_range=[-0.05, 0.05]),
+ dict(type='RandomDropPointsColor', drop_ratio=0.2),
+ dict(type='DefaultFormatBundle3D', class_names=class_names),
+ dict(type='Collect3D', keys=['points', 'pts_semantic_mask'])
+]
+```
+
+- `PointSegClassMapping`:在训练过程中,只有被使用的类别的序号会被映射到类似 \[0, 13) 范围内的类别标签。其余的类别序号会被转换为 `ignore_index` 所制定的忽略标签,在本例中是 `13`。
+- `IndoorPatchPointSample`:从输入点云中裁剪一个含有固定数量点的小块 (patch)。`block_size` 指定了裁剪块的边长,在 S3DIS 上这个数值一般设置为 `1.0`。
+- `NormalizePointsColor`:将输入点的颜色信息归一化,通过将 RGB 值除以 `255` 来实现。
+- 数据增广:
+ - `GlobalRotScaleTrans`:对输入点云进行随机旋转和放缩变换。
+ - `RandomJitterPoints`:通过对每一个点施加不同的噪声向量以实现对点云的随机扰动。
+ - `RandomDropPointsColor`:以 `drop_ratio` 的概率随机将点云的颜色值全部置零。
+
+## 度量指标
+
+通常我们使用平均交并比 (mean Intersection over Union, mIoU) 作为 ScanNet 语义分割任务的度量指标。
+具体而言,我们先计算所有类别的 IoU,然后取平均值作为 mIoU。
+更多实现细节请参考 [seg_eval.py](https://github.com/open-mmlab/mmdetection3d/blob/master/mmdet3d/core/evaluation/seg_eval.py)。
+
+正如在 `提取 S3DIS 数据` 一节中所提及的,S3DIS 通常在 5 个区域上进行训练,然后在余下的 1 个区域上进行测试。但是在其他论文中,也有不同的划分方式。
+为了便于灵活划分训练和测试的子集,我们首先定义子数据集 (sub-dataset) 来表示每一个区域,然后根据区域划分对其进行合并,以得到完整的训练集。
+以下是在区域 1、2、3、4、6 上训练并在区域 5 上测试的一个配置文件例子:
+
+```python
+dataset_type = 'S3DISSegDataset'
+data_root = './data/s3dis/'
+class_names = ('ceiling', 'floor', 'wall', 'beam', 'column', 'window', 'door',
+ 'table', 'chair', 'sofa', 'bookcase', 'board', 'clutter')
+train_area = [1, 2, 3, 4, 6]
+test_area = 5
+data = dict(
+ train=dict(
+ type=dataset_type,
+ data_root=data_root,
+ ann_files=[
+ data_root + f's3dis_infos_Area_{i}.pkl' for i in train_area
+ ],
+ pipeline=train_pipeline,
+ classes=class_names,
+ test_mode=False,
+ ignore_index=len(class_names),
+ scene_idxs=[
+ data_root + f'seg_info/Area_{i}_resampled_scene_idxs.npy'
+ for i in train_area
+ ]),
+ val=dict(
+ type=dataset_type,
+ data_root=data_root,
+ ann_files=data_root + f's3dis_infos_Area_{test_area}.pkl',
+ pipeline=test_pipeline,
+ classes=class_names,
+ test_mode=True,
+ ignore_index=len(class_names),
+ scene_idxs=data_root +
+ f'seg_info/Area_{test_area}_resampled_scene_idxs.npy'))
+```
+
+可以看到,我们通过将多个相应路径构成的列表 (list) 输入 `ann_files` 和 `scene_idxs` 以实现训练测试集的划分。
+如果修改训练测试区域的划分,只需要简单修改 `train_area` 和 `test_area` 即可。
diff --git a/docs/zh_cn/datasets/scannet_det.md b/docs/zh_cn/datasets/scannet_det.md
new file mode 100644
index 0000000..cf5c5ae
--- /dev/null
+++ b/docs/zh_cn/datasets/scannet_det.md
@@ -0,0 +1,304 @@
+# 3D 目标检测 Scannet 数据集
+
+## 数据集准备
+
+请参考 ScanNet 的[指南](https://github.com/open-mmlab/mmdetection3d/blob/master/data/scannet/README.md/)以查看总体流程。
+
+### 提取 ScanNet 点云数据
+
+通过提取 ScanNet 数据,我们加载原始点云文件,并生成包括语义标签、实例标签和真实物体包围框在内的相关标注。
+
+```shell
+python batch_load_scannet_data.py
+```
+
+数据处理之前的文件目录结构如下:
+
+```
+mmdetection3d
+├── mmdet3d
+├── tools
+├── configs
+├── data
+│ ├── scannet
+│ │ ├── meta_data
+│ │ ├── scans
+│ │ │ ├── scenexxxx_xx
+│ │ ├── batch_load_scannet_data.py
+│ │ ├── load_scannet_data.py
+│ │ ├── scannet_utils.py
+│ │ ├── README.md
+```
+
+在 `scans` 文件夹下总共有 1201 个训练样本文件夹和 312 个验证样本文件夹,其中存有未处理的点云数据和相关的标注。比如说,在文件夹 `scene0001_01` 下文件是这样组织的:
+
+- `scene0001_01_vh_clean_2.ply`: 存有每个顶点坐标和颜色的网格文件。网格的顶点被直接用作未处理的点云数据。
+- `scene0001_01.aggregation.json`: 包含物体 ID、分割部分 ID、标签的标注文件。
+- `scene0001_01_vh_clean_2.0.010000.segs.json`: 包含分割部分 ID 和顶点的分割标注文件。
+- `scene0001_01.txt`: 包括对齐矩阵等的元文件。
+- `scene0001_01_vh_clean_2.labels.ply`:包含每个顶点类别的标注文件。
+
+通过运行 `python batch_load_scannet_data.py` 来提取 ScanNet 数据。主要步骤包括:
+
+- 从原始文件中提取出点云、实例标签、语义标签和包围框标签文件。
+- 下采样原始点云并过滤掉不合法的类别。
+- 保存处理后的点云数据和相关的标注文件。
+
+`load_scannet_data.py` 中的核心函数 `export` 如下:
+
+```python
+def export(mesh_file,
+ agg_file,
+ seg_file,
+ meta_file,
+ label_map_file,
+ output_file=None,
+ test_mode=False):
+
+ # 标签映射文件:./data/scannet/meta_data/scannetv2-labels.combined.tsv
+ # 该标签映射文件中有多种标签标准,比如 'nyu40id'
+ label_map = scannet_utils.read_label_mapping(
+ label_map_file, label_from='raw_category', label_to='nyu40id')
+ # 加载原始点云数据,特征包括6维:XYZRGB
+ mesh_vertices = scannet_utils.read_mesh_vertices_rgb(mesh_file)
+
+ # 加载场景坐标轴对齐矩阵:一个 4x4 的变换矩阵
+ # 将传感器坐标系下的原始点转化到另一个坐标系下
+ # 该坐标系与房屋的两边平行(也就是与坐标轴平行)
+ lines = open(meta_file).readlines()
+ # 测试集的数据没有对齐矩阵
+ axis_align_matrix = np.eye(4)
+ for line in lines:
+ if 'axisAlignment' in line:
+ axis_align_matrix = [
+ float(x)
+ for x in line.rstrip().strip('axisAlignment = ').split(' ')
+ ]
+ break
+ axis_align_matrix = np.array(axis_align_matrix).reshape((4, 4))
+
+ # 对网格顶点进行全局的对齐
+ pts = np.ones((mesh_vertices.shape[0], 4))
+ # 同种类坐标下的原始点云,每一行的数据是 [x, y, z, 1]
+ pts[:, 0:3] = mesh_vertices[:, 0:3]
+ # 将原始网格顶点转换为对齐后的顶点
+ pts = np.dot(pts, axis_align_matrix.transpose()) # Nx4
+ aligned_mesh_vertices = np.concatenate([pts[:, 0:3], mesh_vertices[:, 3:]],
+ axis=1)
+
+ # 加载语义与实例标签
+ if not test_mode:
+ # 每个物体都有一个语义标签,并且包含几个分割部分
+ object_id_to_segs, label_to_segs = read_aggregation(agg_file)
+ # 很多点属于同一分割部分
+ seg_to_verts, num_verts = read_segmentation(seg_file)
+ label_ids = np.zeros(shape=(num_verts), dtype=np.uint32)
+ object_id_to_label_id = {}
+ for label, segs in label_to_segs.items():
+ label_id = label_map[label]
+ for seg in segs:
+ verts = seg_to_verts[seg]
+ # 每个点都有一个语义标签
+ label_ids[verts] = label_id
+ instance_ids = np.zeros(
+ shape=(num_verts), dtype=np.uint32) # 0:未标注的
+ for object_id, segs in object_id_to_segs.items():
+ for seg in segs:
+ verts = seg_to_verts[seg]
+ # object_id 从 1 开始计数,比如 1,2,3,.,,,.NUM_INSTANCES
+ # 每个点都属于一个物体
+ instance_ids[verts] = object_id
+ if object_id not in object_id_to_label_id:
+ object_id_to_label_id[object_id] = label_ids[verts][0]
+ # 包围框格式为 [x, y, z, dx, dy, dz, label_id]
+ # [x, y, z] 是包围框的重力中心, [dx, dy, dz] 是与坐标轴平行的
+ # [label_id] 是 'nyu40id' 标准下的语义标签
+ # 注意:因为三维包围框是与坐标轴平行的,所以旋转角是 0
+ unaligned_bboxes = extract_bbox(mesh_vertices, object_id_to_segs,
+ object_id_to_label_id, instance_ids)
+ aligned_bboxes = extract_bbox(aligned_mesh_vertices, object_id_to_segs,
+ object_id_to_label_id, instance_ids)
+ ...
+
+ return mesh_vertices, label_ids, instance_ids, unaligned_bboxes, \
+ aligned_bboxes, object_id_to_label_id, axis_align_matrix
+
+```
+
+在从每个场景的扫描文件提取数据后,如果原始点云点数过多,可以将其下采样(比如到 50000 个点),但在三维语义分割任务中,点云不会被下采样。此外,在 `nyu40id` 标准之外的不合法语义标签或者可选的 `DONOT CARE` 类别标签应被过滤。最终,点云文件、语义标签、实例标签和真实物体的集合应被存储于 `.npy` 文件中。
+
+### 提取 ScanNet RGB 色彩数据(可选的)
+
+通过提取 ScanNet RGB 色彩数据,对于每个场景我们加载 RGB 图像与配套 4x4 位姿矩阵、单个 4x4 相机内参矩阵的集合。请注意,这一步是可选的,除非要运行多视图物体检测,否则可以略去这步。
+
+```shell
+python extract_posed_images.py
+```
+
+1201 个训练样本,312 个验证样本和 100 个测试样本中的每一个都包含一个单独的 `.sens` 文件。比如说,对于场景 `0001_01` 我们有 `data/scannet/scans/scene0001_01/0001_01.sens`。对于这个场景所有图像和位姿数据都被提取至 `data/scannet/posed_images/scene0001_01`。具体来说,该文件夹下会有 300 个 xxxxx.jpg 格式的图像数据,300 个 xxxxx.txt 格式的相机位姿数据和一个单独的 `intrinsic.txt` 内参文件。通常来说,一个场景包含数千张图像。默认情况下,我们只会提取其中的 300 张,从而只占用少于 100 Gb 的空间。要想提取更多图像,请使用 `--max-images-per-scene` 参数。
+
+### 创建数据集
+
+```shell
+python tools/create_data.py scannet --root-path ./data/scannet \
+--out-dir ./data/scannet --extra-tag scannet
+```
+
+上述提取的点云文件,语义类别标注文件,和物体实例标注文件被进一步以 `.bin` 格式保存。与此同时 `.pkl` 格式的文件被生成并用于训练和验证。获取数据信息的核心函数 `process_single_scene` 如下:
+
+```python
+def process_single_scene(sample_idx):
+
+ # 分别以 .bin 格式保存点云文件,语义类别标注文件和物体实例标注文件
+ # 获取 info['pts_path'],info['pts_instance_mask_path'] 和 info['pts_semantic_mask_path']
+ ...
+
+ # 获取标注
+ if has_label:
+ annotations = {}
+ # 包围框的形状为 [k, 6 + class]
+ aligned_box_label = self.get_aligned_box_label(sample_idx)
+ unaligned_box_label = self.get_unaligned_box_label(sample_idx)
+ annotations['gt_num'] = aligned_box_label.shape[0]
+ if annotations['gt_num'] != 0:
+ aligned_box = aligned_box_label[:, :-1] # k, 6
+ unaligned_box = unaligned_box_label[:, :-1]
+ classes = aligned_box_label[:, -1] # k
+ annotations['name'] = np.array([
+ self.label2cat[self.cat_ids2class[classes[i]]]
+ for i in range(annotations['gt_num'])
+ ])
+ # 为了向后兼容,默认的参数名赋予了与坐标轴平行的包围框
+ # 我们同时保存了对应的与坐标轴不平行的包围框的信息
+ annotations['location'] = aligned_box[:, :3]
+ annotations['dimensions'] = aligned_box[:, 3:6]
+ annotations['gt_boxes_upright_depth'] = aligned_box
+ annotations['unaligned_location'] = unaligned_box[:, :3]
+ annotations['unaligned_dimensions'] = unaligned_box[:, 3:6]
+ annotations[
+ 'unaligned_gt_boxes_upright_depth'] = unaligned_box
+ annotations['index'] = np.arange(
+ annotations['gt_num'], dtype=np.int32)
+ annotations['class'] = np.array([
+ self.cat_ids2class[classes[i]]
+ for i in range(annotations['gt_num'])
+ ])
+ axis_align_matrix = self.get_axis_align_matrix(sample_idx)
+ annotations['axis_align_matrix'] = axis_align_matrix # 4x4
+ info['annos'] = annotations
+ return info
+```
+
+如上数据处理后,文件目录结构应如下:
+
+```
+scannet
+├── meta_data
+├── batch_load_scannet_data.py
+├── load_scannet_data.py
+├── scannet_utils.py
+├── README.md
+├── scans
+├── scans_test
+├── scannet_instance_data
+├── points
+│ ├── xxxxx.bin
+├── instance_mask
+│ ├── xxxxx.bin
+├── semantic_mask
+│ ├── xxxxx.bin
+├── seg_info
+│ ├── train_label_weight.npy
+│ ├── train_resampled_scene_idxs.npy
+│ ├── val_label_weight.npy
+│ ├── val_resampled_scene_idxs.npy
+├── posed_images
+│ ├── scenexxxx_xx
+│ │ ├── xxxxxx.txt
+│ │ ├── xxxxxx.jpg
+│ │ ├── intrinsic.txt
+├── scannet_infos_train.pkl
+├── scannet_infos_val.pkl
+├── scannet_infos_test.pkl
+```
+
+- `points/xxxxx.bin`:下采样后,未与坐标轴平行(即没有对齐)的点云。因为 ScanNet 3D 检测任务将与坐标轴平行的点云作为输入,而 ScanNet 3D 语义分割任务将对齐前的点云作为输入,我们选择存储对齐前的点云和它们的对齐矩阵。请注意:在 3D 检测的预处理流程 [`GlobalAlignment`](https://github.com/open-mmlab/mmdetection3d/blob/9f0b01caf6aefed861ef4c3eb197c09362d26b32/mmdet3d/datasets/pipelines/transforms_3d.py#L423) 后,点云就都是与坐标轴平行的了。
+- `instance_mask/xxxxx.bin`:每个点的实例标签,值的范围为:\[0, NUM_INSTANCES\],其中 0 表示没有标注。
+- `semantic_mask/xxxxx.bin`:每个点的语义标签,值的范围为:\[1, 40\], 也就是 `nyu40id` 的标准。请注意:在训练流程 `PointSegClassMapping` 中,`nyu40id` 的 ID 会被映射到训练 ID。
+- `posed_images/scenexxxx_xx`:`.jpg` 图像的集合,还包含 `.txt` 格式的 4x4 相机姿态和单个 `.txt` 格式的相机内参矩阵文件。
+- `scannet_infos_train.pkl`:训练集的数据信息,每个场景的具体信息如下:
+ - info\['point_cloud'\]:`{'num_features': 6, 'lidar_idx': sample_idx}`,其中 `sample_idx` 为该场景的索引。
+ - info\['pts_path'\]:`points/xxxxx.bin` 的路径。
+ - info\['pts_instance_mask_path'\]:`instance_mask/xxxxx.bin` 的路径。
+ - info\['pts_semantic_mask_path'\]:`semantic_mask/xxxxx.bin` 的路径。
+ - info\['annos'\]:每个场景的标注。
+ - annotations\['gt_num'\]:真实物体 (ground truth) 的数量。
+ - annotations\['name'\]:所有真实物体的语义类别名称,比如 `chair`(椅子)。
+ - annotations\['location'\]:depth 坐标系下与坐标轴平行的三维包围框的重力中心 (gravity center),形状为 \[K, 3\],其中 K 是真实物体的数量。
+ - annotations\['dimensions'\]:depth 坐标系下与坐标轴平行的三维包围框的大小,形状为 \[K, 3\]。
+ - annotations\['gt_boxes_upright_depth'\]:depth 坐标系下与坐标轴平行的三维包围框 `(x, y, z, x_size, y_size, z_size, yaw)`,形状为 \[K, 6\]。
+ - annotations\['unaligned_location'\]:depth 坐标系下与坐标轴不平行(对齐前)的三维包围框的重力中心。
+ - annotations\['unaligned_dimensions'\]:depth 坐标系下与坐标轴不平行的三维包围框的大小。
+ - annotations\['unaligned_gt_boxes_upright_depth'\]:depth 坐标系下与坐标轴不平行的三维包围框。
+ - annotations\['index'\]:所有真实物体的索引,范围为 \[0, K)。
+ - annotations\['class'\]:所有真实物体类别的标号,范围为 \[0, 18),形状为 \[K, \]。
+- `scannet_infos_val.pkl`:验证集上的数据信息,与 `scannet_infos_train.pkl` 格式完全一致。
+- `scannet_infos_test.pkl`:测试集上的数据信息,与 `scannet_infos_train.pkl` 格式几乎完全一致,除了缺少标注。
+
+## 训练流程
+
+ScanNet 上 3D 物体检测的典型流程如下:
+
+```python
+train_pipeline = [
+ dict(
+ type='LoadPointsFromFile',
+ coord_type='DEPTH',
+ shift_height=True,
+ load_dim=6,
+ use_dim=[0, 1, 2]),
+ dict(
+ type='LoadAnnotations3D',
+ with_bbox_3d=True,
+ with_label_3d=True,
+ with_mask_3d=True,
+ with_seg_3d=True),
+ dict(type='GlobalAlignment', rotation_axis=2),
+ dict(
+ type='PointSegClassMapping',
+ valid_cat_ids=(3, 4, 5, 6, 7, 8, 9, 10, 11, 12, 14, 16, 24, 28, 33, 34,
+ 36, 39),
+ max_cat_id=40),
+ dict(type='PointSample', num_points=40000),
+ dict(
+ type='RandomFlip3D',
+ sync_2d=False,
+ flip_ratio_bev_horizontal=0.5,
+ flip_ratio_bev_vertical=0.5),
+ dict(
+ type='GlobalRotScaleTrans',
+ rot_range=[-0.087266, 0.087266],
+ scale_ratio_range=[1.0, 1.0],
+ shift_height=True),
+ dict(type='DefaultFormatBundle3D', class_names=class_names),
+ dict(
+ type='Collect3D',
+ keys=[
+ 'points', 'gt_bboxes_3d', 'gt_labels_3d', 'pts_semantic_mask',
+ 'pts_instance_mask'
+ ])
+]
+```
+
+- `GlobalAlignment`:输入的点云在施加了坐标轴平行的矩阵后应被转换为与坐标轴平行的形式。
+- `PointSegClassMapping`:训练中,只有合法的类别 ID 才会被映射到类别标签,比如 \[0, 18)。
+- 数据增强:
+ - `PointSample`:下采样输入点云。
+ - `RandomFlip3D`:随机左右或前后翻转点云。
+ - `GlobalRotScaleTrans`: 旋转输入点云,对于 ScanNet 角度通常落入 \[-5, 5\] (度)的范围;并放缩输入点云,对于 ScanNet 比例通常为 1.0(即不做缩放);最后平移输入点云,对于 ScanNet 通常位移量为 0(即不做位移)。
+
+## 评估指标
+
+通常 mAP(全类平均精度)被用于 ScanNet 的检测任务的评估,比如 `mAP@0.25` 和 `mAP@0.5`。具体来说,评估时一个通用的计算 3D 物体检测多个类别的精度和召回率的函数被调用,可以参考 [indoor_eval](https://github.com/open-mmlab/mmdetection3d/blob/master/mmdet3D/core/evaluation/indoor_eval.py)。
+
+与在章节`提取 ScanNet 数据` 中介绍的那样,所有真实物体的三维包围框是与坐标轴平行的,也就是说旋转角为 0。因此,预测包围框的网络接受的包围框旋转角监督也是 0,且在后处理阶段我们使用适用于与坐标轴平行的包围框的非极大值抑制 (NMS) ,该过程不会考虑包围框的旋转。
diff --git a/docs/zh_cn/datasets/scannet_sem_seg.md b/docs/zh_cn/datasets/scannet_sem_seg.md
new file mode 100644
index 0000000..b8c30fe
--- /dev/null
+++ b/docs/zh_cn/datasets/scannet_sem_seg.md
@@ -0,0 +1,137 @@
+# 3D 语义分割 ScanNet 数据集
+
+## 数据集的准备
+
+ScanNet 3D 语义分割数据集的准备和 3D 检测任务的准备很相似,请查看[此文档](https://github.com/open-mmlab/mmdetection3d/blob/master/docs_zh-CN/datasets/scannet_det.md#dataset-preparation)以获取更多细节。
+以下我们只罗列部分 3D 语义分割特有的处理步骤和数据信息。
+
+### 提取 ScanNet 数据
+
+因为 ScanNet 测试集对 3D 语义分割任务提供在线评测的基准,我们也需要下载其测试集并置于 `scannet` 目录下。
+数据预处理前的文件目录结构应如下所示:
+
+```
+mmdetection3d
+├── mmdet3d
+├── tools
+├── configs
+├── data
+│ ├── scannet
+│ │ ├── meta_data
+│ │ ├── scans
+│ │ │ ├── scenexxxx_xx
+│ │ ├── scans_test
+│ │ │ ├── scenexxxx_xx
+│ │ ├── batch_load_scannet_data.py
+│ │ ├── load_scannet_data.py
+│ │ ├── scannet_utils.py
+│ │ ├── README.md
+```
+
+在 `scans_test` 目录下有 100 个测试集 scan 的文件夹,每个文件夹仅包含了原始点云数据和基础的数据元文件。
+例如,在 `scene0707_00` 这一目录下的文件如下所示:
+
+- `scene0707_00_vh_clean_2.ply`:原始网格文件,存储有每个顶点的坐标和颜色。网格的顶点会被选取作为处理后点云中的点。
+- `scene0707_00.txt`:数据的元文件,包含数据采集传感器的参数等信息。注意,与 `scans` 目录下的数据 (训练集和验证集) 不同,测试集 scan 并没有提供用于和坐标轴对齐的变换矩阵 (`axis-aligned matrix`)。
+
+用户可以通过运行 `python batch_load_scannet_data.py` 指令来从原始文件中提取 ScanNet 数据。
+注意,测试集只会保存下点云数据,因为没有提供标注信息。
+
+### 创建数据集
+
+与 3D 检测任务类似,我们通过运行 `python tools/create_data.py scannet --root-path ./data/scannet --out-dir ./data/scannet --extra-tag scannet` 指令即可创建 ScanNet 数据集。
+预处理后的数据目录结构如下所示:
+
+```
+scannet
+├── scannet_utils.py
+├── batch_load_scannet_data.py
+├── load_scannet_data.py
+├── scannet_utils.py
+├── README.md
+├── scans
+├── scans_test
+├── scannet_instance_data
+├── points
+│ ├── xxxxx.bin
+├── instance_mask
+│ ├── xxxxx.bin
+├── semantic_mask
+│ ├── xxxxx.bin
+├── seg_info
+│ ├── train_label_weight.npy
+│ ├── train_resampled_scene_idxs.npy
+│ ├── val_label_weight.npy
+│ ├── val_resampled_scene_idxs.npy
+├── scannet_infos_train.pkl
+├── scannet_infos_val.pkl
+├── scannet_infos_test.pkl
+```
+
+- `seg_info`:为支持语义分割任务所生成的信息文件。
+ - `train_label_weight.npy`:每一语义类别的权重系数。因为 ScanNet 中属于不同类的点的数量相差很大,一个常见的操作是在计算损失时对不同类别进行加权 (label re-weighting) 以得到更好的分割性能。
+ - `train_resampled_scene_idxs.npy`:每一个场景 (房间) 的重采样标签。在训练过程中,我们依据每个场景的点的数量,会对其进行不同次数的重采样,以保证训练数据均衡。
+
+## 训练流程
+
+ScanNet 上 3D 语义分割的一种典型数据载入流程如下所示:
+
+```python
+train_pipeline = [
+ dict(
+ type='LoadPointsFromFile',
+ coord_type='DEPTH',
+ shift_height=False,
+ use_color=True,
+ load_dim=6,
+ use_dim=[0, 1, 2, 3, 4, 5]),
+ dict(
+ type='LoadAnnotations3D',
+ with_bbox_3d=False,
+ with_label_3d=False,
+ with_mask_3d=False,
+ with_seg_3d=True),
+ dict(
+ type='PointSegClassMapping',
+ valid_cat_ids=(1, 2, 3, 4, 5, 6, 7, 8, 9, 10, 11, 12, 14, 16, 24, 28,
+ 33, 34, 36, 39),
+ max_cat_id=40),
+ dict(
+ type='IndoorPatchPointSample',
+ num_points=num_points,
+ block_size=1.5,
+ ignore_index=len(class_names),
+ use_normalized_coord=False,
+ enlarge_size=0.2,
+ min_unique_num=None),
+ dict(type='NormalizePointsColor', color_mean=None),
+ dict(type='DefaultFormatBundle3D', class_names=class_names),
+ dict(type='Collect3D', keys=['points', 'pts_semantic_mask'])
+]
+```
+
+- `PointSegClassMapping`:在训练过程中,只有被使用的类别的序号会被映射到类似 \[0, 20) 范围内的类别标签。其余的类别序号会被转换为 `ignore_index` 所制定的忽略标签,在本例中是 `20`。
+- `IndoorPatchPointSample`:从输入点云中裁剪一个含有固定数量点的小块 (patch)。`block_size` 指定了裁剪块的边长,在 ScanNet 上这个数值一般设置为 `1.5`。
+- `NormalizePointsColor`:将输入点的颜色信息归一化,通过将 RGB 值除以 `255` 来实现。
+
+## 度量指标
+
+通常我们使用平均交并比 (mean Intersection over Union, mIoU) 作为 ScanNet 语义分割任务的度量指标。
+具体而言,我们先计算所有类别的 IoU,然后取平均值作为 mIoU。
+更多实现细节请参考 [seg_eval.py](https://github.com/open-mmlab/mmdetection3d/blob/master/mmdet3d/core/evaluation/seg_eval.py)。
+
+## 在测试集上测试并提交结果
+
+默认情况下,MMDet3D 的代码是在训练集上进行模型训练,然后在验证集上进行模型测试。
+如果你也想在在线基准上测试模型的性能,请在测试命令中加上 `--format-only` 的标记,同时也要将 ScanNet 数据集[配置文件](https://github.com/open-mmlab/mmdetection3d/blob/master/configs/_base_/datasets/scannet_seg-3d-20class.py#L126)中的 `ann_file=data_root + 'scannet_infos_val.pkl'` 改成 `ann_file=data_root + 'scannet_infos_test.pkl'`。
+请记得通过 `txt_prefix` 来指定想要保存测试结果的文件夹名称。
+
+以 PointNet++ (SSG) 在 ScanNet 上的测试为例,你可以运行以下命令来完成测试结果的保存:
+
+```
+./tools/dist_test.sh configs/pointnet2/pointnet2_ssg_16x2_cosine_200e_scannet_seg-3d-20class.py \
+ work_dirs/pointnet2_ssg/latest.pth --format-only \
+ --eval-options txt_prefix=work_dirs/pointnet2_ssg/test_submission
+```
+
+在保存测试结果后,你可以将该文件夹压缩,然后提交到 [ScanNet 在线测试服务器](http://kaldir.vc.in.tum.de/scannet_benchmark/semantic_label_3d)上进行验证。
diff --git a/docs/zh_cn/datasets/sunrgbd_det.md b/docs/zh_cn/datasets/sunrgbd_det.md
new file mode 100644
index 0000000..6fa6e35
--- /dev/null
+++ b/docs/zh_cn/datasets/sunrgbd_det.md
@@ -0,0 +1,346 @@
+# 3D 目标检测 SUN RGB-D 数据集
+
+## 数据集的准备
+
+对于数据集准备的整体流程,请参考 SUN RGB-D 的[指南](https://github.com/open-mmlab/mmdetection3d/blob/master/data/sunrgbd/README.md/)。
+
+### 下载 SUN RGB-D 数据与工具包
+
+在[这里](http://rgbd.cs.princeton.edu/data/)下载 SUN RGB-D 的数据。接下来,将 `SUNRGBD.zip`、`SUNRGBDMeta2DBB_v2.mat`、`SUNRGBDMeta3DBB_v2.mat` 和 `SUNRGBDtoolbox.zip` 移动到 `OFFICIAL_SUNRGBD` 文件夹,并解压文件。
+
+下载完成后,数据处理之前的文件目录结构如下:
+
+```
+sunrgbd
+├── README.md
+├── matlab
+│ ├── extract_rgbd_data_v1.m
+│ ├── extract_rgbd_data_v2.m
+│ ├── extract_split.m
+├── OFFICIAL_SUNRGBD
+│ ├── SUNRGBD
+│ ├── SUNRGBDMeta2DBB_v2.mat
+│ ├── SUNRGBDMeta3DBB_v2.mat
+│ ├── SUNRGBDtoolbox
+```
+
+### 从原始数据中提取 3D 检测所需数据与标注
+
+通过运行如下指令从原始文件中提取出 SUN RGB-D 的标注(这需要您的机器中安装了 MATLAB):
+
+```bash
+matlab -nosplash -nodesktop -r 'extract_split;quit;'
+matlab -nosplash -nodesktop -r 'extract_rgbd_data_v2;quit;'
+matlab -nosplash -nodesktop -r 'extract_rgbd_data_v1;quit;'
+```
+
+主要的步骤包括:
+
+- 提取出训练集和验证集的索引文件;
+- 从原始数据中提取出 3D 检测所需要的数据;
+- 从原始的标注数据中提取并组织检测任务使用的标注数据。
+
+用于从深度图中提取点云数据的 `extract_rgbd_data_v2.m` 的主要部分如下:
+
+```matlab
+data = SUNRGBDMeta(imageId);
+data.depthpath(1:16) = '';
+data.depthpath = strcat('../OFFICIAL_SUNRGBD', data.depthpath);
+data.rgbpath(1:16) = '';
+data.rgbpath = strcat('../OFFICIAL_SUNRGBD', data.rgbpath);
+
+% 从深度图获取点云
+[rgb,points3d,depthInpaint,imsize]=read3dPoints(data);
+rgb(isnan(points3d(:,1)),:) = [];
+points3d(isnan(points3d(:,1)),:) = [];
+points3d_rgb = [points3d, rgb];
+
+% MAT 文件比 TXT 文件小三倍。在 Python 中我们可以使用
+% scipy.io.loadmat('xxx.mat')['points3d_rgb'] 来加载数据
+mat_filename = strcat(num2str(imageId,'%06d'), '.mat');
+txt_filename = strcat(num2str(imageId,'%06d'), '.txt');
+% 保存点云数据
+parsave(strcat(depth_folder, mat_filename), points3d_rgb);
+```
+
+用于提取并组织检测任务标注的 `extract_rgbd_data_v1.m` 的主要部分如下:
+
+```matlab
+% 输出 2D 和 3D 包围框
+data2d = data;
+fid = fopen(strcat(det_label_folder, txt_filename), 'w');
+for j = 1:length(data.groundtruth3DBB)
+ centroid = data.groundtruth3DBB(j).centroid; % 3D 包围框中心
+ classname = data.groundtruth3DBB(j).classname; % 类名
+ orientation = data.groundtruth3DBB(j).orientation; % 3D 包围框方向
+ coeffs = abs(data.groundtruth3DBB(j).coeffs); % 3D 包围框大小
+ box2d = data2d.groundtruth2DBB(j).gtBb2D; % 2D 包围框
+ fprintf(fid, '%s %d %d %d %d %f %f %f %f %f %f %f %f\n', classname, box2d(1), box2d(2), box2d(3), box2d(4), centroid(1), centroid(2), centroid(3), coeffs(1), coeffs(2), coeffs(3), orientation(1), orientation(2));
+end
+fclose(fid);
+```
+
+上面的两个脚本调用了 SUN RGB-D 提供的[工具包](https://rgbd.cs.princeton.edu/data/SUNRGBDtoolbox.zip)中的一些函数,如 `read3dPoints`。
+
+使用上述脚本提取数据后,文件目录结构应如下:
+
+```
+sunrgbd
+├── README.md
+├── matlab
+│ ├── extract_rgbd_data_v1.m
+│ ├── extract_rgbd_data_v2.m
+│ ├── extract_split.m
+├── OFFICIAL_SUNRGBD
+│ ├── SUNRGBD
+│ ├── SUNRGBDMeta2DBB_v2.mat
+│ ├── SUNRGBDMeta3DBB_v2.mat
+│ ├── SUNRGBDtoolbox
+├── sunrgbd_trainval
+│ ├── calib
+│ ├── depth
+│ ├── image
+│ ├── label
+│ ├── label_v1
+│ ├── seg_label
+│ ├── train_data_idx.txt
+│ ├── val_data_idx.txt
+```
+
+在如下每个文件夹下,都有总计 5285 个训练集样本和 5050 个验证集样本:
+
+- `calib`:`.txt` 后缀的相机标定文件。
+- `depth`:`.mat` 后缀的点云文件,包含 xyz 坐标和 rgb 色彩值。
+- `image`:`.jpg` 后缀的二维图像文件。
+- `label`:`.txt` 后缀的用于检测任务的标注数据(版本二)。
+- `label_v1`:`.txt` 后缀的用于检测任务的标注数据(版本一)。
+- `seg_label`:`.txt` 后缀的用于分割任务的标注数据。
+
+目前,我们使用版本一的数据用于训练与测试,因此版本二的标注并未使用。
+
+### 创建数据集
+
+请运行如下指令创建数据集:
+
+```shell
+python tools/create_data.py sunrgbd --root-path ./data/sunrgbd \
+--out-dir ./data/sunrgbd --extra-tag sunrgbd
+```
+
+或者,如果使用 slurm,可以使用如下指令替代:
+
+```
+bash tools/create_data.sh sunrgbd
+```
+
+之前提到的点云数据就会被处理并以 `.bin` 格式重新存储。与此同时,`.pkl` 文件也被生成,用于存储数据标注和元信息。这一步处理中,用于生成 `.pkl` 文件的核心函数 `process_single_scene` 如下:
+
+```python
+def process_single_scene(sample_idx):
+ print(f'{self.split} sample_idx: {sample_idx}')
+ # 将深度图转换为点云并降采样点云
+ SAMPLE_NUM = 50000
+ pc_upright_depth = self.get_depth(sample_idx)
+ pc_upright_depth_subsampled = random_sampling(
+ pc_upright_depth, SAMPLE_NUM)
+
+ info = dict()
+ pc_info = {'num_features': 6, 'lidar_idx': sample_idx}
+ info['point_cloud'] = pc_info
+
+ # 将点云保存为 `.bin` 格式
+ mmcv.mkdir_or_exist(osp.join(self.root_dir, 'points'))
+ pc_upright_depth_subsampled.tofile(
+ osp.join(self.root_dir, 'points', f'{sample_idx:06d}.bin'))
+
+ # 存储点云存储路径
+ info['pts_path'] = osp.join('points', f'{sample_idx:06d}.bin')
+
+ # 存储图像存储路径以及其元信息
+ img_path = osp.join('image', f'{sample_idx:06d}.jpg')
+ image_info = {
+ 'image_idx': sample_idx,
+ 'image_shape': self.get_image_shape(sample_idx),
+ 'image_path': img_path
+ }
+ info['image'] = image_info
+
+ # 保存标定信息
+ K, Rt = self.get_calibration(sample_idx)
+ calib_info = {'K': K, 'Rt': Rt}
+ info['calib'] = calib_info
+
+ # 保存所有数据标注
+ if has_label:
+ obj_list = self.get_label_objects(sample_idx)
+ annotations = {}
+ annotations['gt_num'] = len([
+ obj.classname for obj in obj_list
+ if obj.classname in self.cat2label.keys()
+ ])
+ if annotations['gt_num'] != 0:
+ # 类别名称
+ annotations['name'] = np.array([
+ obj.classname for obj in obj_list
+ if obj.classname in self.cat2label.keys()
+ ])
+ # 二维图像包围框
+ annotations['bbox'] = np.concatenate([
+ obj.box2d.reshape(1, 4) for obj in obj_list
+ if obj.classname in self.cat2label.keys()
+ ], axis=0)
+ # depth 坐标系下的三维包围框中心坐标
+ annotations['location'] = np.concatenate([
+ obj.centroid.reshape(1, 3) for obj in obj_list
+ if obj.classname in self.cat2label.keys()
+ ], axis=0)
+ # depth 坐标系下的三维包围框大小
+ annotations['dimensions'] = 2 * np.array([
+ [obj.l, obj.h, obj.w] for obj in obj_list
+ if obj.classname in self.cat2label.keys()
+ ])
+ # depth 坐标系下的三维包围框旋转角
+ annotations['rotation_y'] = np.array([
+ obj.heading_angle for obj in obj_list
+ if obj.classname in self.cat2label.keys()
+ ])
+ annotations['index'] = np.arange(
+ len(obj_list), dtype=np.int32)
+ # 类别标签(数字)
+ annotations['class'] = np.array([
+ self.cat2label[obj.classname] for obj in obj_list
+ if obj.classname in self.cat2label.keys()
+ ])
+ # depth 坐标系下的三维包围框
+ annotations['gt_boxes_upright_depth'] = np.stack(
+ [
+ obj.box3d for obj in obj_list
+ if obj.classname in self.cat2label.keys()
+ ], axis=0) # (K,8)
+ info['annos'] = annotations
+ return info
+```
+
+如上数据处理后,文件目录结构应如下:
+
+```
+sunrgbd
+├── README.md
+├── matlab
+│ ├── ...
+├── OFFICIAL_SUNRGBD
+│ ├── ...
+├── sunrgbd_trainval
+│ ├── ...
+├── points
+├── sunrgbd_infos_train.pkl
+├── sunrgbd_infos_val.pkl
+```
+
+- `points/0xxxxx.bin`:降采样后的点云数据。
+- `sunrgbd_infos_train.pkl`:训练集数据信息(标注与元信息),每个场景所含数据信息具体如下:
+ - info\['point_cloud'\]:`{'num_features': 6, 'lidar_idx': sample_idx}`,其中 `sample_idx` 为该场景的索引。
+ - info\['pts_path'\]:`points/0xxxxx.bin` 的路径。
+ - info\['image'\]:图像路径与元信息:
+ - image\['image_idx'\]:图像索引。
+ - image\['image_shape'\]:图像张量的形状(即其尺寸)。
+ - image\['image_path'\]:图像路径。
+ - info\['annos'\]:每个场景的标注:
+ - annotations\['gt_num'\]:真实物体 (ground truth) 的数量。
+ - annotations\['name'\]:所有真实物体的语义类别名称,比如 `chair`(椅子)。
+ - annotations\['location'\]:depth 坐标系下三维包围框的重力中心 (gravity center),形状为 \[K, 3\],其中 K 是真实物体的数量。
+ - annotations\['dimensions'\]:depth 坐标系下三维包围框的大小,形状为 \[K, 3\]。
+ - annotations\['rotation_y'\]:depth 坐标系下三维包围框的旋转角,形状为 \[K, \]。
+ - annotations\['gt_boxes_upright_depth'\]:depth 坐标系下三维包围框 `(x, y, z, x_size, y_size, z_size, yaw)`,形状为 \[K, 7\]。
+ - annotations\['bbox'\]:二维包围框 `(x, y, x_size, y_size)`,形状为 \[K, 4\]。
+ - annotations\['index'\]:所有真实物体的索引,范围为 \[0, K)。
+ - annotations\['class'\]:所有真实物体类别的标号,范围为 \[0, 10),形状为 \[K, \]。
+- `sunrgbd_infos_val.pkl`:验证集上的数据信息,与 `sunrgbd_infos_train.pkl` 格式完全一致。
+
+## 训练流程
+
+SUN RGB-D 上纯点云 3D 物体检测的典型流程如下:
+
+```python
+train_pipeline = [
+ dict(
+ type='LoadPointsFromFile',
+ coord_type='DEPTH',
+ shift_height=True,
+ load_dim=6,
+ use_dim=[0, 1, 2]),
+ dict(type='LoadAnnotations3D'),
+ dict(
+ type='RandomFlip3D',
+ sync_2d=False,
+ flip_ratio_bev_horizontal=0.5,
+ ),
+ dict(
+ type='GlobalRotScaleTrans',
+ rot_range=[-0.523599, 0.523599],
+ scale_ratio_range=[0.85, 1.15],
+ shift_height=True),
+ dict(type='PointSample', num_points=20000),
+ dict(type='DefaultFormatBundle3D', class_names=class_names),
+ dict(type='Collect3D', keys=['points', 'gt_bboxes_3d', 'gt_labels_3d'])
+]
+```
+
+点云上的数据增强
+
+- `RandomFlip3D`:随机左右或前后翻转输入点云。
+- `GlobalRotScaleTrans`:旋转输入点云,对于 SUN RGB-D 角度通常落入 \[-30, 30\] (度)的范围;并放缩输入点云,对于 SUN RGB-D 比例通常落入 \[0.85, 1.15\] 的范围;最后平移输入点云,对于 SUN RGB-D 通常位移量为 0(即不做位移)。
+- `PointSample`:降采样输入点云。
+
+SUN RGB-D 上多模态(点云和图像)3D 物体检测的典型流程如下:
+
+```python
+train_pipeline = [
+ dict(
+ type='LoadPointsFromFile',
+ coord_type='DEPTH',
+ shift_height=True,
+ load_dim=6,
+ use_dim=[0, 1, 2]),
+ dict(type='LoadImageFromFile'),
+ dict(type='LoadAnnotations3D'),
+ dict(type='LoadAnnotations', with_bbox=True),
+ dict(type='Resize', img_scale=(1333, 600), keep_ratio=True),
+ dict(type='RandomFlip', flip_ratio=0.0),
+ dict(type='Normalize', **img_norm_cfg),
+ dict(type='Pad', size_divisor=32),
+ dict(
+ type='RandomFlip3D',
+ sync_2d=False,
+ flip_ratio_bev_horizontal=0.5,
+ ),
+ dict(
+ type='GlobalRotScaleTrans',
+ rot_range=[-0.523599, 0.523599],
+ scale_ratio_range=[0.85, 1.15],
+ shift_height=True),
+ dict(type='PointSample', num_points=20000),
+ dict(type='DefaultFormatBundle3D', class_names=class_names),
+ dict(
+ type='Collect3D',
+ keys=[
+ 'img', 'gt_bboxes', 'gt_labels', 'points', 'gt_bboxes_3d',
+ 'gt_labels_3d'
+ ])
+]
+```
+
+图像上的数据增强/归一化
+
+- `Resize`: 改变输入图像的大小, `keep_ratio=True` 意味着图像的比例不改变。
+- `Normalize`: 归一化图像的 RGB 通道。
+- `RandomFlip`: 随机地翻折图像。
+- `Pad`: 扩大图像,默认情况下用零填充图像的边缘。
+
+图像增强和归一化函数的实现取自 [MMDetection](https://github.com/open-mmlab/mmdetection/tree/master/mmdet/datasets/pipelines)。
+
+## 度量指标
+
+与 ScanNet 一样,通常 mAP(全类平均精度)被用于 SUN RGB-D 的检测任务的评估,比如 `mAP@0.25` 和 `mAP@0.5`。具体来说,评估时一个通用的计算 3D 物体检测多个类别的精度和召回率的函数被调用,可以参考 [`indoor_eval.py`](https://github.com/open-mmlab/mmdetection3d/blob/master/mmdet3d/core/evaluation/indoor_eval.py)。
+
+因为 SUN RGB-D 包含有图像数据,所以图像上的物体检测也是可行的。举个例子,在 ImVoteNet 中,我们首先训练了一个图像检测器,并且也使用 mAP 指标,如 `mAP@0.5`,来评估其表现。我们使用 [MMDetection](https://github.com/open-mmlab/mmdetection) 库中的 `eval_map` 函数来计算 mAP。
diff --git a/docs/zh_cn/datasets/waymo_det.md b/docs/zh_cn/datasets/waymo_det.md
new file mode 100644
index 0000000..2c0ff7d
--- /dev/null
+++ b/docs/zh_cn/datasets/waymo_det.md
@@ -0,0 +1,175 @@
+# Waymo 数据集
+
+本文档页包含了关于 MMDetection3D 中 Waymo 数据集用法的教程。
+
+## 数据集准备
+
+在准备 Waymo 数据集之前,如果您之前只安装了 `requirements/build.txt` 和 `requirements/runtime.txt` 中的依赖,请通过运行如下指令额外安装 Waymo 数据集所依赖的官方包:
+
+```
+# tf 2.1.0.
+pip install waymo-open-dataset-tf-2-1-0==1.2.0
+# tf 2.0.0
+# pip install waymo-open-dataset-tf-2-0-0==1.2.0
+# tf 1.15.0
+# pip install waymo-open-dataset-tf-1-15-0==1.2.0
+```
+
+或者
+
+```
+pip install -r requirements/optional.txt
+```
+
+和准备数据集的通用方法一致,我们推荐将数据集根目录软链接至 `$MMDETECTION3D/data`。
+由于原始 Waymo 数据的格式基于 `tfrecord`,我们需要将原始数据进行预处理,以便于训练和测试时使用。我们的方法是将它们转换为 KITTI 格式。
+
+处理之前,文件目录结构组织如下:
+
+```
+mmdetection3d
+├── mmdet3d
+├── tools
+├── configs
+├── data
+│ ├── waymo
+│ │ ├── waymo_format
+│ │ │ ├── training
+│ │ │ ├── validation
+│ │ │ ├── testing
+│ │ │ ├── gt.bin
+│ │ ├── kitti_format
+│ │ │ ├── ImageSets
+
+```
+
+您可以在[这里](https://waymo.com/open/download/)下载 1.2 版本的 Waymo 公开数据集,并在[这里](https://drive.google.com/drive/folders/18BVuF_RYJF0NjZpt8SnfzANiakoRMf0o?usp=sharing)下载其训练/验证/测试集拆分文件。接下来,请将 `tfrecord` 文件放入 `data/waymo/waymo_format/` 下的对应文件夹,并将 txt 格式的数据集拆分文件放入 `data/waymo/kitti_format/ImageSets`。在[这里](https://console.cloud.google.com/storage/browser/waymo_open_dataset_v_1_2_0/validation/ground_truth_objects)下载验证集使用的 bin 格式真实标注 (Ground Truth) 文件并放入 `data/waymo/waymo_format/`。小窍门:您可以使用 `gsutil` 来在命令行下载大规模数据集。您可以将该[工具](https://github.com/RalphMao/Waymo-Dataset-Tool) 作为一个例子来查看更多细节。之后,通过运行如下指令准备 Waymo 数据:
+
+```bash
+python tools/create_data.py waymo --root-path ./data/waymo/ --out-dir ./data/waymo/ --workers 128 --extra-tag waymo
+```
+
+请注意,如果您的本地磁盘没有足够空间保存转换后的数据,您可以将 `--out-dir` 改为其他目录;只要在创建文件夹、准备数据并转换格式后,将数据文件链接到 `data/waymo/kitti_format` 即可。
+
+在数据转换后,文件目录结构应组织如下:
+
+```
+mmdetection3d
+├── mmdet3d
+├── tools
+├── configs
+├── data
+│ ├── waymo
+│ │ ├── waymo_format
+│ │ │ ├── training
+│ │ │ ├── validation
+│ │ │ ├── testing
+│ │ │ ├── gt.bin
+│ │ ├── kitti_format
+│ │ │ ├── ImageSets
+│ │ │ ├── training
+│ │ │ │ ├── calib
+│ │ │ │ ├── image_0
+│ │ │ │ ├── image_1
+│ │ │ │ ├── image_2
+│ │ │ │ ├── image_3
+│ │ │ │ ├── image_4
+│ │ │ │ ├── label_0
+│ │ │ │ ├── label_1
+│ │ │ │ ├── label_2
+│ │ │ │ ├── label_3
+│ │ │ │ ├── label_4
+│ │ │ │ ├── label_all
+│ │ │ │ ├── pose
+│ │ │ │ ├── velodyne
+│ │ │ ├── testing
+│ │ │ │ ├── (the same as training)
+│ │ │ ├── waymo_gt_database
+│ │ │ ├── waymo_infos_trainval.pkl
+│ │ │ ├── waymo_infos_train.pkl
+│ │ │ ├── waymo_infos_val.pkl
+│ │ │ ├── waymo_infos_test.pkl
+│ │ │ ├── waymo_dbinfos_train.pkl
+
+```
+
+因为 Waymo 数据的来源包含数个相机,这里我们将每个相机对应的图像和标签文件分别存储,并将相机位姿 (pose) 文件存储下来以供后续处理连续多帧的点云。我们使用 `{a}{bbb}{ccc}` 的名称编码方式为每帧数据命名,其中 `a` 是不同数据拆分的前缀(`0` 指代训练集,`1` 指代验证集,`2` 指代测试集),`bbb` 是分割部分 (segment) 的索引,而 `ccc` 是帧索引。您可以轻而易举地按照如上命名规则定位到所需的帧。我们将训练和验证所需数据按 KITTI 的方式集合在一起,然后将训练集/验证集/测试集的索引存储在 `ImageSet` 下的文件中。
+
+## 训练
+
+考虑到原始数据集中的数据有很多相似的帧,我们基本上可以主要使用一个子集来训练我们的模型。在我们初步的基线中,我们在每五帧图片中加载一帧。得益于我们的超参数设置和数据增强方案,我们得到了比 Waymo [原论文](https://arxiv.org/pdf/1912.04838.pdf)中更好的性能。请移步 `configs/pointpillars/` 下的 README.md 以查看更多配置和性能相关的细节。我们会尽快发布一个更完整的 Waymo 基准榜单 (benchmark)。
+
+## 评估
+
+为了在 Waymo 数据集上进行检测性能评估,请按照[此处指示](https://github.com/waymo-research/waymo-open-dataset/blob/master/docs/quick_start.md/)构建用于计算评估指标的二进制文件 `compute_detection_metrics_main`,并将它置于 `mmdet3d/core/evaluation/waymo_utils/` 下。您基本上可以按照下方命令安装 `bazel`,然后构建二进制文件:
+
+```shell
+# download the code and enter the base directory
+git clone https://github.com/waymo-research/waymo-open-dataset.git waymo-od
+cd waymo-od
+git checkout remotes/origin/master
+
+# use the Bazel build system
+sudo apt-get install --assume-yes pkg-config zip g++ zlib1g-dev unzip python3 python3-pip
+BAZEL_VERSION=3.1.0
+wget https://github.com/bazelbuild/bazel/releases/download/${BAZEL_VERSION}/bazel-${BAZEL_VERSION}-installer-linux-x86_64.sh
+sudo bash bazel-${BAZEL_VERSION}-installer-linux-x86_64.sh
+sudo apt install build-essential
+
+# configure .bazelrc
+./configure.sh
+# delete previous bazel outputs and reset internal caches
+bazel clean
+
+bazel build waymo_open_dataset/metrics/tools/compute_detection_metrics_main
+cp bazel-bin/waymo_open_dataset/metrics/tools/compute_detection_metrics_main ../mmdetection3d/mmdet3d/core/evaluation/waymo_utils/
+```
+
+接下来,您就可以在 Waymo 上评估您的模型了。如下示例是使用 8 个图形处理器 (GPU) 在 Waymo 上用 Waymo 评价指标评估 PointPillars 模型的情景:
+
+```shell
+./tools/slurm_test.sh ${PARTITION} ${JOB_NAME} configs/pointpillars/hv_pointpillars_secfpn_sbn-2x16_2x_waymo-3d-car.py \
+ checkpoints/hv_pointpillars_secfpn_sbn-2x16_2x_waymo-3d-car_latest.pth --out results/waymo-car/results_eval.pkl \
+ --eval waymo --eval-options 'pklfile_prefix=results/waymo-car/kitti_results' \
+ 'submission_prefix=results/waymo-car/kitti_results'
+```
+
+如果需要生成 bin 文件,应在 `--eval-options` 中给出 `pklfile_prefix`。对于评价指标, `waymo` 是我们推荐的官方评估原型。目前,`kitti` 这一评估选项是从 KITTI 迁移而来的,且每个难度下的评估结果和 KITTI 数据集中定义得到的不尽相同——目前大多数物体被标记为难度 0(日后会修复)。`kitti` 评估选项的不稳定来源于很大的计算量,转换的数据中遮挡 (occlusion) 和截断 (truncation) 的缺失,难度的不同定义方式,以及不同的平均精度 (Average Precision) 计算方式。
+
+**注意**:
+
+1. 有时用 `bazel` 构建 `compute_detection_metrics_main` 的过程中会出现如下错误:`'round' 不是 'std' 的成员` (`'round' is not a member of 'std'`)。我们只需要移除该文件中,`round` 前的 `std::`。
+
+2. 考虑到 Waymo 上评估一次耗时不短,我们建议只在模型训练结束时进行评估。
+
+3. 为了在 CUDA 9 环境使用 TensorFlow,我们建议通过编译 TensorFlow 源码的方式使用。除了官方教程之外,您还可以参考该[链接](https://github.com/SmileTM/Tensorflow2.X-GPU-CUDA9.0)以寻找可能合适的预编译包以及编译源码的实用攻略。
+
+## 测试并提交到官方服务器
+
+如下是一个使用 8 个图形处理器在 Waymo 上测试 PointPillars,生成 bin 文件并提交结果到官方榜单的例子:
+
+```shell
+./tools/slurm_test.sh ${PARTITION} ${JOB_NAME} configs/pointpillars/hv_pointpillars_secfpn_sbn-2x16_2x_waymo-3d-car.py \
+ checkpoints/hv_pointpillars_secfpn_sbn-2x16_2x_waymo-3d-car_latest.pth --out results/waymo-car/results_eval.pkl \
+ --format-only --eval-options 'pklfile_prefix=results/waymo-car/kitti_results' \
+ 'submission_prefix=results/waymo-car/kitti_results'
+```
+
+在生成 bin 文件后,您可以简单地构建二进制文件 `create_submission`,并按照[指示](https://github.com/waymo-research/waymo-open-dataset/blob/master/docs/quick_start.md/) 创建一个提交文件。下面是一些示例:
+
+```shell
+cd ../waymo-od/
+bazel build waymo_open_dataset/metrics/tools/create_submission
+cp bazel-bin/waymo_open_dataset/metrics/tools/create_submission ../mmdetection3d/mmdet3d/core/evaluation/waymo_utils/
+vim waymo_open_dataset/metrics/tools/submission.txtpb # set the metadata information
+cp waymo_open_dataset/metrics/tools/submission.txtpb ../mmdetection3d/mmdet3d/core/evaluation/waymo_utils/
+
+cd ../mmdetection3d
+# suppose the result bin is in `results/waymo-car/submission`
+mmdet3d/core/evaluation/waymo_utils/create_submission --input_filenames='results/waymo-car/kitti_results_test.bin' --output_filename='results/waymo-car/submission/model' --submission_filename='mmdet3d/core/evaluation/waymo_utils/submission.txtpb'
+
+tar cvf results/waymo-car/submission/my_model.tar results/waymo-car/submission/my_model/
+gzip results/waymo-car/submission/my_model.tar
+```
+
+如果想用官方评估服务器评估您在验证集上的结果,您可以使用同样的方法生成提交文件,只需确保您在运行如上指令前更改 `submission.txtpb` 中的字段值即可。
diff --git a/docs/zh_cn/demo.md b/docs/zh_cn/demo.md
new file mode 100644
index 0000000..9fcd07a
--- /dev/null
+++ b/docs/zh_cn/demo.md
@@ -0,0 +1,87 @@
+# 样例
+
+# 介绍
+
+我们提供了多模态/单模态(基于激光雷达/图像)、室内/室外场景的 3D 检测和 3D 语义分割样例的脚本,预训练模型可以从 [Model Zoo](https://github.com/open-mmlab/mmdetection3d/blob/master/docs_zh-CN/model_zoo.md/) 下载。我们也提供了 KITTI、SUN RGB-D、nuScenes 和 ScanNet 数据集的预处理样本数据,你可以根据我们的预处理步骤使用任何其它数据。
+
+## 测试
+
+### 3D 检测
+
+#### 单模态样例
+
+在点云数据上测试 3D 检测器,运行:
+
+```shell
+python demo/pcd_demo.py ${PCD_FILE} ${CONFIG_FILE} ${CHECKPOINT_FILE} [--device ${GPU_ID}] [--score-thr ${SCORE_THR}] [--out-dir ${OUT_DIR}] [--show]
+```
+
+点云和预测 3D 框的可视化结果会被保存在 `${OUT_DIR}/PCD_NAME`,它可以使用 [MeshLab](http://www.meshlab.net/) 打开。注意如果你设置了 `--show`,通过 [Open3D](http://www.open3d.org/) 可以在线显示预测结果。
+
+在 KITTI 数据上测试 [SECOND](https://github.com/open-mmlab/mmdetection3d/tree/master/configs/second) 模型:
+
+```shell
+python demo/pcd_demo.py demo/data/kitti/kitti_000008.bin configs/second/hv_second_secfpn_6x8_80e_kitti-3d-car.py checkpoints/hv_second_secfpn_6x8_80e_kitti-3d-car_20200620_230238-393f000c.pth
+```
+
+在 SUN RGB-D 数据上测试 [VoteNet](https://github.com/open-mmlab/mmdetection3d/tree/master/configs/votenet) 模型:
+
+```shell
+python demo/pcd_demo.py demo/data/sunrgbd/sunrgbd_000017.bin configs/votenet/votenet_16x8_sunrgbd-3d-10class.py checkpoints/votenet_16x8_sunrgbd-3d-10class_20200620_230238-4483c0c0.pth
+```
+
+如果你正在使用的 mmdetection3d 版本 >= 0.6.0,记住转换 VoteNet 的模型权重文件,查看 [README](https://github.com/open-mmlab/mmdetection3d/blob/master/configs/votenet/README.md/) 来获取转换模型权重文件的详细说明。
+
+#### 多模态样例
+
+在多模态数据(通常是点云和图像)上测试 3D 检测器,运行:
+
+```shell
+python demo/multi_modality_demo.py ${PCD_FILE} ${IMAGE_FILE} ${ANNOTATION_FILE} ${CONFIG_FILE} ${CHECKPOINT_FILE} [--device ${GPU_ID}] [--score-thr ${SCORE_THR}] [--out-dir ${OUT_DIR}] [--show]
+```
+
+`ANNOTATION_FILE` 需要提供 3D 到 2D 的仿射矩阵,可视化结果会被保存在 `${OUT_DIR}/PCD_NAME`,其中包括点云、图像、预测的 3D 框以及它们在图像上的投影。
+
+在 KITTI 数据上测试 [MVX-Net](https://github.com/open-mmlab/mmdetection3d/tree/master/configs/mvxnet) 模型:
+
+```shell
+python demo/multi_modality_demo.py demo/data/kitti/kitti_000008.bin demo/data/kitti/kitti_000008.png demo/data/kitti/kitti_000008_infos.pkl configs/mvxnet/dv_mvx-fpn_second_secfpn_adamw_2x8_80e_kitti-3d-3class.py checkpoints/dv_mvx-fpn_second_secfpn_adamw_2x8_80e_kitti-3d-3class_20200621_003904-10140f2d.pth
+```
+
+在 SUN RGB-D 数据上测试 [ImVoteNet](https://github.com/open-mmlab/mmdetection3d/tree/master/configs/imvotenet) 模型:
+
+```shell
+python demo/multi_modality_demo.py demo/data/sunrgbd/sunrgbd_000017.bin demo/data/sunrgbd/sunrgbd_000017.jpg demo/data/sunrgbd/sunrgbd_000017_infos.pkl configs/imvotenet/imvotenet_stage2_16x8_sunrgbd-3d-10class.py checkpoints/imvotenet_stage2_16x8_sunrgbd-3d-10class_20210323_184021-d44dcb66.pth
+```
+
+### 单目 3D 检测
+
+在图像数据上测试单目 3D 检测器,运行:
+
+```shell
+python demo/mono_det_demo.py ${IMAGE_FILE} ${ANNOTATION_FILE} ${CONFIG_FILE} ${CHECKPOINT_FILE} [--device ${GPU_ID}] [--out-dir ${OUT_DIR}] [--show]
+```
+
+`ANNOTATION_FILE` 需要提供 3D 到 2D 的仿射矩阵(相机内参矩阵),可视化结果会被保存在 `${OUT_DIR}/PCD_NAME`,其中包括图像以及预测 3D 框在图像上的投影。
+
+在 nuScenes 数据上测试 [FCOS3D](https://github.com/open-mmlab/mmdetection3d/tree/master/configs/fcos3d) 模型:
+
+```shell
+python demo/mono_det_demo.py demo/data/nuscenes/n015-2018-07-24-11-22-45+0800__CAM_BACK__1532402927637525.jpg demo/data/nuscenes/n015-2018-07-24-11-22-45+0800__CAM_BACK__1532402927637525_mono3d.coco.json configs/fcos3d/fcos3d_r101_caffe_fpn_gn-head_dcn_2x8_1x_nus-mono3d_finetune.py checkpoints/fcos3d_r101_caffe_fpn_gn-head_dcn_2x8_1x_nus-mono3d_finetune_20210717_095645-8d806dc2.pth
+```
+
+### 3D 分割
+
+在点云数据上测试 3D 分割器,运行:
+
+```shell
+python demo/pc_seg_demo.py ${PCD_FILE} ${CONFIG_FILE} ${CHECKPOINT_FILE} [--device ${GPU_ID}] [--out-dir ${OUT_DIR}] [--show]
+```
+
+可视化结果会被保存在 `${OUT_DIR}/PCD_NAME`,其中包括点云以及预测的 3D 分割掩码。
+
+在 ScanNet 数据上测试 [PointNet++ (SSG)](https://github.com/open-mmlab/mmdetection3d/tree/master/configs/pointnet2) 模型:
+
+```shell
+python demo/pc_seg_demo.py demo/data/scannet/scene0000_00.bin configs/pointnet2/pointnet2_ssg_16x2_cosine_200e_scannet_seg-3d-20class.py checkpoints/pointnet2_ssg_16x2_cosine_200e_scannet_seg-3d-20class_20210514_143644-ee73704a.pth
+```
diff --git a/docs/zh_cn/faq.md b/docs/zh_cn/faq.md
new file mode 100644
index 0000000..44a96d2
--- /dev/null
+++ b/docs/zh_cn/faq.md
@@ -0,0 +1,41 @@
+# 常见问题解答
+
+我们列出了一些用户和开发者在开发过程中会遇到的常见问题以及对应的解决方案,如果您发现了任何频繁出现的问题,请随时扩充本列表,非常欢迎您提出的任何解决方案。如果您在环境配置、模型训练等工作中遇到任何的问题,请使用[问题模板](https://github.com/open-mmlab/mmdetection3d/blob/master/.github/ISSUE_TEMPLATE/error-report.md/)来创建相应的 issue,并将所需的所有信息填入到问题模板中,我们会尽快解决您的问题。
+
+## MMCV/MMDet/MMDet3D Installation
+
+- 如果您在 `import open3d` 时遇到下面的问题:
+
+ `OSError: /lib/x86_64-linux-gnu/libm.so.6: version 'GLIBC_2.27' not found`
+
+ 请将 open3d 的版本降级至 0.9.0.0,因为最新版 open3d 需要 'GLIBC_2.27' 文件的支持, Ubuntu 16.04 系统中缺失该文件,且该文件仅存在于 Ubuntu 18.04 及之后的系统中。
+
+- 如果您在 `import pycocotools` 时遇到版本错误的问题,这是由于 nuscenes-devkit 需要安装 pycocotools,然而 mmdet 依赖于 mmpycocotools,当前的解决方案如下所示,我们将会在之后全面支持 pycocotools :
+
+ ```shell
+ pip uninstall pycocotools mmpycocotools
+ pip install mmpycocotools
+ ```
+
+ **注意**: 我们已经在 0.13.0 及之后的版本中全面支持 pycocotools。
+
+- 如果您在导入 pycocotools 相关包时遇到下面的问题:
+
+ `ValueError: numpy.ndarray size changed, may indicate binary incompatibility. Expected 88 from C header, got 80 from PyObject`
+
+ 请将 pycocotools 的版本降级至 2.0.1,这是由于最新版本的 pycocotools 与 numpy \< 1.20.0 不兼容。或者通过下面的方式从源码进行编译来安装最新版本的 pycocotools :
+
+ `pip install -e "git+https://github.com/cocodataset/cocoapi#egg=pycocotools&subdirectory=PythonAPI"`
+
+ 或者
+
+ `pip install -e "git+https://github.com/ppwwyyxx/cocoapi#egg=pycocotools&subdirectory=PythonAPI"`
+
+## 如何标注点云?
+
+MMDetection3D 不支持点云标注。我们提供一些开源的标注工具供参考:
+
+- [SUSTechPOINTS](https://github.com/naurril/SUSTechPOINTS)
+- [LATTE](https://github.com/bernwang/latte)
+
+此外,我们改进了 [LATTE](https://github.com/bernwang/latte) 以便更方便的标注。 更多的细节请参考 [这里](https://arxiv.org/abs/2011.10174)。
diff --git a/docs/zh_cn/getting_started.md b/docs/zh_cn/getting_started.md
new file mode 100644
index 0000000..219e2d5
--- /dev/null
+++ b/docs/zh_cn/getting_started.md
@@ -0,0 +1,320 @@
+# 依赖
+
+- Linux 或者 macOS (实验性支持 Windows)
+- Python 3.6+
+- PyTorch 1.3+
+- CUDA 9.2+ (如果你从源码编译 PyTorch, CUDA 9.0 也是兼容的。)
+- GCC 5+
+- [MMCV](https://mmcv.readthedocs.io/en/latest/#installation)
+
+| MMDetection3D 版本 | MMDetection 版本 | MMSegmentation 版本 | MMCV 版本 |
+| :--------------: | :----------------------: | :---------------------: | :-------------------------: |
+| master | mmdet>=2.24.0, \<=3.0.0 | mmseg>=0.20.0, \<=1.0.0 | mmcv-full>=1.4.8, \<=1.6.0 |
+| v1.0.0rc3 | mmdet>=2.24.0, \<=3.0.0 | mmseg>=0.20.0, \<=1.0.0 | mmcv-full>=1.4.8, \<=1.6.0 |
+| v1.0.0rc2 | mmdet>=2.24.0, \<=3.0.0 | mmseg>=0.20.0, \<=1.0.0 | mmcv-full>=1.4.8, \<=1.6.0 |
+| v1.0.0rc1 | mmdet>=2.19.0, \<=3.0.0 | mmseg>=0.20.0, \<=1.0.0 | mmcv-full>=1.4.8, \<=1.5.0 |
+| v1.0.0rc0 | mmdet>=2.19.0, \<=3.0.0 | mmseg>=0.20.0, \<=1.0.0 | mmcv-full>=1.3.17, \<=1.5.0 |
+| 0.18.1 | mmdet>=2.19.0, \<=3.0.0 | mmseg>=0.20.0, \<=1.0.0 | mmcv-full>=1.3.17, \<=1.5.0 |
+| 0.18.0 | mmdet>=2.19.0, \<=3.0.0 | mmseg>=0.20.0, \<=1.0.0 | mmcv-full>=1.3.17, \<=1.5.0 |
+| 0.17.3 | mmdet>=2.14.0, \<=3.0.0 | mmseg>=0.14.1, \<=1.0.0 | mmcv-full>=1.3.8, \<=1.4.0 |
+| 0.17.2 | mmdet>=2.14.0, \<=3.0.0 | mmseg>=0.14.1, \<=1.0.0 | mmcv-full>=1.3.8, \<=1.4.0 |
+| 0.17.1 | mmdet>=2.14.0, \<=3.0.0 | mmseg>=0.14.1, \<=1.0.0 | mmcv-full>=1.3.8, \<=1.4.0 |
+| 0.17.0 | mmdet>=2.14.0, \<=3.0.0 | mmseg>=0.14.1, \<=1.0.0 | mmcv-full>=1.3.8, \<=1.4.0 |
+| 0.16.0 | mmdet>=2.14.0, \<=3.0.0 | mmseg>=0.14.1, \<=1.0.0 | mmcv-full>=1.3.8, \<=1.4.0 |
+| 0.15.0 | mmdet>=2.14.0, \<=3.0.0 | mmseg>=0.14.1, \<=1.0.0 | mmcv-full>=1.3.8, \<=1.4.0 |
+| 0.14.0 | mmdet>=2.10.0, \<=2.11.0 | mmseg==0.14.0 | mmcv-full>=1.3.1, \<=1.4.0 |
+| 0.13.0 | mmdet>=2.10.0, \<=2.11.0 | Not required | mmcv-full>=1.2.4, \<=1.4.0 |
+| 0.12.0 | mmdet>=2.5.0, \<=2.11.0 | Not required | mmcv-full>=1.2.4, \<=1.4.0 |
+| 0.11.0 | mmdet>=2.5.0, \<=2.11.0 | Not required | mmcv-full>=1.2.4, \<=1.3.0 |
+| 0.10.0 | mmdet>=2.5.0, \<=2.11.0 | Not required | mmcv-full>=1.2.4, \<=1.3.0 |
+| 0.9.0 | mmdet>=2.5.0, \<=2.11.0 | Not required | mmcv-full>=1.2.4, \<=1.3.0 |
+| 0.8.0 | mmdet>=2.5.0, \<=2.11.0 | Not required | mmcv-full>=1.1.5, \<=1.3.0 |
+| 0.7.0 | mmdet>=2.5.0, \<=2.11.0 | Not required | mmcv-full>=1.1.5, \<=1.3.0 |
+| 0.6.0 | mmdet>=2.4.0, \<=2.11.0 | Not required | mmcv-full>=1.1.3, \<=1.2.0 |
+| 0.5.0 | 2.3.0 | Not required | mmcv-full==1.0.5 |
+
+# 安装
+
+## MMdetection3D 安装流程
+
+### 快速安装脚本
+
+如果你已经成功安装 CUDA 11.0,那么你可以使用这个快速安装命令进行 MMDetection3D 的安装。 否则,则参考下一小节的详细安装流程。
+
+```shell
+conda create -n open-mmlab python=3.7 pytorch=1.9 cudatoolkit=11.0 torchvision -c pytorch -y
+conda activate open-mmlab
+pip3 install openmim
+mim install mmcv-full
+mim install mmdet
+mim install mmsegmentation
+git clone https://github.com/open-mmlab/mmdetection3d.git
+cd mmdetection3d
+pip3 install -e .
+```
+
+### 详细安装流程
+
+**a. 使用 conda 新建虚拟环境,并进入该虚拟环境。**
+
+```shell
+conda create -n open-mmlab python=3.7 -y
+conda activate open-mmlab
+```
+
+**b. 基于 [PyTorch 官网](https://pytorch.org/)安装 PyTorch 和 torchvision,例如:**
+
+```shell
+conda install pytorch torchvision -c pytorch
+```
+
+**注意**:需要确保 CUDA 的编译版本和运行版本匹配。可以在 [PyTorch 官网](https://pytorch.org/)查看预编译包所支持的 CUDA 版本。
+
+`例 1` 例如在 `/usr/local/cuda` 下安装了 CUDA 10.1, 并想安装 PyTorch 1.5,则需要安装支持 CUDA 10.1 的预构建 PyTorch:
+
+```shell
+conda install pytorch cudatoolkit=10.1 torchvision -c pytorch
+```
+
+`例 2` 例如在 `/usr/local/cuda` 下安装了 CUDA 9.2, 并想安装 PyTorch 1.3.1,则需要安装支持 CUDA 9.2 的预构建 PyTorch:
+
+```shell
+conda install pytorch=1.3.1 cudatoolkit=9.2 torchvision=0.4.2 -c pytorch
+```
+
+如果不是安装预构建的包,而是从源码中构建 PyTorch,则可以使用更多的 CUDA 版本,例如 CUDA 9.0。
+
+**c. 安装 [MMCV](https://mmcv.readthedocs.io/en/latest/).**
+需要安装 *mmcv-full*,因为 MMDetection3D 依赖 MMDetection 且需要 *mmcv-full* 中基于 CUDA 的程序。
+
+`例` 可以使用下面命令安装预编译版本的 *mmcv-full* :(可使用的版本在[这里](https://mmcv.readthedocs.io/en/latest/#install-with-pip)可以找到)
+
+```shell
+pip install mmcv-full -f https://download.openmmlab.com/mmcv/dist/{cu_version}/{torch_version}/index.html
+```
+
+需要把命令行中的 `{cu_version}` 和 `{torch_version}` 替换成对应的版本。例如:在 CUDA 11 和 PyTorch 1.7.0 的环境下,可以使用下面命令安装最新版本的 MMCV:
+
+```shell
+pip install mmcv-full -f https://download.openmmlab.com/mmcv/dist/cu110/torch1.7.0/index.html
+```
+
+PyTorch 在 1.x.0 和 1.x.1 之间通常是兼容的,故 mmcv-full 只提供 1.x.0 的编译包。如果你的 PyTorch 版本是 1.x.1,你可以放心地安装在 1.x.0 版本编译的 mmcv-full。
+
+```
+# 我们可以忽略 PyTorch 的小版本号
+pip install mmcv-full -f https://download.openmmlab.com/mmcv/dist/cu110/torch1.7/index.html
+```
+
+请参考 [MMCV](https://mmcv.readthedocs.io/en/latest/#installation) 获取不同版本的 MMCV 所兼容的的不同的 PyTorch 和 CUDA 版本。同时,也可以通过以下命令行从源码编译 MMCV:
+
+```shell
+git clone https://github.com/open-mmlab/mmcv.git
+cd mmcv
+MMCV_WITH_OPS=1 pip install -e . # 安装好 mmcv-full
+cd ..
+```
+
+或者,可以直接使用命令行安装:
+
+```shell
+pip install mmcv-full
+```
+
+**d. 安装 [MMDetection](https://github.com/open-mmlab/mmdetection).**
+
+```shell
+pip install mmdet
+```
+
+同时,如果你想修改这部分的代码,也可以通过以下命令从源码编译 MMDetection:
+
+```shell
+git clone https://github.com/open-mmlab/mmdetection.git
+cd mmdetection
+git checkout v2.19.0 # 转到 v2.19.0 分支
+pip install -r requirements/build.txt
+pip install -v -e . # or "python setup.py develop"
+```
+
+**e. 安装 [MMSegmentation](https://github.com/open-mmlab/mmsegmentation).**
+
+```shell
+pip install mmsegmentation
+```
+
+同时,如果你想修改这部分的代码,也可以通过以下命令从源码编译 MMSegmentation:
+
+```shell
+git clone https://github.com/open-mmlab/mmsegmentation.git
+cd mmsegmentation
+git checkout v0.20.0 # switch to v0.20.0 branch
+pip install -e . # or "python setup.py develop"
+```
+
+**f. 克隆 MMDetection3D 代码仓库**
+
+```shell
+git clone https://github.com/open-mmlab/mmdetection3d.git
+cd mmdetection3d
+```
+
+**g. 安装依赖包和 MMDetection3D.**
+
+```shell
+pip install -v -e . # or "python setup.py develop"
+```
+
+**注意:**
+
+1. Git 的 commit id 在步骤 d 将会被写入到版本号当中,例 0.6.0+2e7045c 。版本号将保存在训练的模型里。推荐在每一次执行步骤 d 时,从 github 上获取最新的更新。如果基于 C++/CUDA 的代码被修改了,请执行以下步骤;
+
+ > 重要: 如果你重装了不同版本的 CUDA 或者 PyTorch 的 mmdet,请务必移除 `./build` 文件。
+
+ ```shell
+ pip uninstall mmdet3d
+ rm -rf ./build
+ find . -name "*.so" | xargs rm
+ ```
+
+2. 按照上述说明,MMDetection3D 安装在 `dev` 模式下,因此在本地对代码做的任何修改都会生效,无需重新安装;
+
+3. 如果希望使用 `opencv-python-headless` 而不是 `opencv-python`, 可以在安装 MMCV 之前安装;
+
+4. 一些安装依赖是可以选择的。例如只需要安装最低运行要求的版本,则可以使用 `pip install -v -e .` 命令。如果希望使用可选择的像 `albumentations` 和 `imagecorruptions` 这种依赖项,可以使用 `pip install -r requirements/optional.txt ` 进行手动安装,或者在使用 `pip` 时指定所需的附加功能(例如 `pip install -v -e .[optional]`),支持附加功能的有效键值包括 `all`、`tests`、`build` 以及 `optional` 。
+
+5. 我们的代码目前不能在只有 CPU 的环境(CUDA 不可用)下编译运行。
+
+## 另一种选择:Docker Image
+
+我们提供了 [Dockerfile](https://github.com/open-mmlab/mmdetection3d/blob/master/docker/Dockerfile) 来建立一个镜像。
+
+```shell
+# 基于 PyTorch 1.6, CUDA 10.1 生成 docker 的镜像
+docker build -t mmdetection3d docker/
+```
+
+运行命令:
+
+```shell
+docker run --gpus all --shm-size=8g -it -v {DATA_DIR}:/mmdetection3d/data mmdetection3d
+```
+
+## 从零开始的安装脚本
+
+以下是一个基于 conda 安装 MMdetection3D 的脚本
+
+```shell
+conda create -n open-mmlab python=3.7 -y
+conda activate open-mmlab
+
+# 安装基于环境中默认 CUDA 版本下最新的 PyTorch (通常使用最新版本)
+conda install -c pytorch pytorch torchvision -y
+
+# 安装 mmcv
+pip install mmcv-full
+
+# 安装 mmdetection
+pip install git+https://github.com/open-mmlab/mmdetection.git
+
+# 安装 mmsegmentation
+pip install git+https://github.com/open-mmlab/mmsegmentation.git
+
+# 安装 mmdetection3d
+git clone https://github.com/open-mmlab/mmdetection3d.git
+cd mmdetection3d
+pip install -v -e .
+```
+
+## 使用多版本的 MMDetection3D
+
+训练和测试的脚本已经在 PYTHONPATH 中进行了修改,以确保脚本使用当前目录中的 MMDetection3D。
+
+要使环境中安装默认的 MMDetection3D 而不是当前正在在使用的,可以删除出现在相关脚本中的代码:
+
+```shell
+PYTHONPATH="$(dirname $0)/..":$PYTHONPATH
+```
+
+# 验证
+
+## 通过点云样例程序来验证
+
+我们提供了一些样例脚本去测试单个样本,预训练的模型可以从[模型库](model_zoo.md)中下载. 运行如下命令可以去测试点云场景下一个单模态的 3D 检测算法。
+
+```shell
+python demo/pcd_demo.py ${PCD_FILE} ${CONFIG_FILE} ${CHECKPOINT_FILE} [--device ${GPU_ID}] [--score-thr ${SCORE_THR}] [--out-dir ${OUT_DIR}]
+```
+
+例:
+
+```shell
+python demo/pcd_demo.py demo/data/kitti/kitti_000008.bin configs/second/hv_second_secfpn_6x8_80e_kitti-3d-car.py checkpoints/hv_second_secfpn_6x8_80e_kitti-3d-car_20200620_230238-393f000c.pth
+```
+
+如果你想输入一个 `ply` 格式的文件,你可以使用如下函数将它转换为 `bin` 的文件格式。然后就可以使用转化成 `bin` 格式的文件去运行样例程序。
+
+请注意在使用此脚本前,你需要先安装 `pandas` 和 `plyfile`。 这个函数也可使用在数据预处理当中,为了能够直接训练 `ply data`。
+
+```python
+import numpy as np
+import pandas as pd
+from plyfile import PlyData
+
+def convert_ply(input_path, output_path):
+ plydata = PlyData.read(input_path) # read file
+ data = plydata.elements[0].data # read data
+ data_pd = pd.DataFrame(data) # convert to DataFrame
+ data_np = np.zeros(data_pd.shape, dtype=np.float) # initialize array to store data
+ property_names = data[0].dtype.names # read names of properties
+ for i, name in enumerate(
+ property_names): # read data by property
+ data_np[:, i] = data_pd[name]
+ data_np.astype(np.float32).tofile(output_path)
+```
+
+例:
+
+```python
+convert_ply('./test.ply', './test.bin')
+```
+
+如果你有其他格式的点云文件 (例:`off`, `obj`), 你可以使用 `trimesh` 将它们转化成 `ply`.
+
+```python
+import trimesh
+
+def to_ply(input_path, output_path, original_type):
+ mesh = trimesh.load(input_path, file_type=original_type) # read file
+ mesh.export(output_path, file_type='ply') # convert to ply
+```
+
+例:
+
+```python
+to_ply('./test.obj', './test.ply', 'obj')
+```
+
+更多的关于单/多模态和室内/室外的 3D 检测的样例可以在[此](demo.md)找到.
+
+## 测试点云的高级接口
+
+### 同步接口
+
+这里有一个例子去说明如何构建模型以及测试给出的点云:
+
+```python
+from mmdet3d.apis import init_model, inference_detector
+
+config_file = 'configs/votenet/votenet_8x8_scannet-3d-18class.py'
+checkpoint_file = 'checkpoints/votenet_8x8_scannet-3d-18class_20200620_230238-2cea9c3a.pth'
+
+# 从配置文件和预训练的模型文件中构建模型
+model = init_model(config_file, checkpoint_file, device='cuda:0')
+
+# 测试单个文件并可视化结果
+point_cloud = 'test.bin'
+result, data = inference_detector(model, point_cloud)
+# 可视化结果并且将结果保存到 'results' 文件夹
+model.show_results(data, result, out_dir='results')
+```
diff --git a/docs/zh_cn/index.rst b/docs/zh_cn/index.rst
new file mode 100644
index 0000000..b2ae80d
--- /dev/null
+++ b/docs/zh_cn/index.rst
@@ -0,0 +1,98 @@
+Welcome to MMDetection3D's documentation!
+==========================================
+
+.. toctree::
+ :maxdepth: 1
+ :caption: 开始你的第一步
+
+ getting_started.md
+
+.. toctree::
+ :maxdepth: 1
+ :caption: 演示
+
+ demo.md
+
+.. toctree::
+ :maxdepth: 1
+ :caption: 模型库
+
+ model_zoo.md
+
+.. toctree::
+ :maxdepth: 1
+ :caption: 数据预处理
+
+ data_preparation.md
+
+.. toctree::
+ :maxdepth: 1
+ :caption: 使用现有数据及模型
+
+ 1_exist_data_model.md
+
+.. toctree::
+ :maxdepth: 1
+ :caption: 创建新的数据与模型
+
+ 2_new_data_model.md
+
+.. toctree::
+ :maxdepth: 1
+ :caption: 支持的任务
+
+ supported_tasks/index.rst
+
+.. toctree::
+ :maxdepth: 1
+ :caption: 数据集介绍
+
+ datasets/index.rst
+
+.. toctree::
+ :maxdepth: 1
+ :caption: 教程
+
+ tutorials/index.rst
+
+.. toctree::
+ :maxdepth: 1
+ :caption: 实用工具与脚本
+
+ useful_tools.md
+
+.. toctree::
+ :maxdepth: 1
+ :caption: 说明
+
+ benchmarks.md
+
+.. toctree::
+ :maxdepth: 1
+ :caption: 常见问题
+
+ faq.md
+
+.. toctree::
+ :maxdepth: 1
+ :caption: 兼容性
+
+ compatibility.md
+
+.. toctree::
+ :maxdepth: 1
+ :caption: 接口文档(英文)
+
+ api.rst
+
+.. toctree::
+ :maxdepth: 1
+ :caption: 语言切换
+
+ switch_language.md
+
+Indices and tables
+==================
+
+* :ref:`genindex`
+* :ref:`search`
diff --git a/docs/zh_cn/make.bat b/docs/zh_cn/make.bat
new file mode 100644
index 0000000..922152e
--- /dev/null
+++ b/docs/zh_cn/make.bat
@@ -0,0 +1,35 @@
+@ECHO OFF
+
+pushd %~dp0
+
+REM Command file for Sphinx documentation
+
+if "%SPHINXBUILD%" == "" (
+ set SPHINXBUILD=sphinx-build
+)
+set SOURCEDIR=.
+set BUILDDIR=_build
+
+if "%1" == "" goto help
+
+%SPHINXBUILD% >NUL 2>NUL
+if errorlevel 9009 (
+ echo.
+ echo.The 'sphinx-build' command was not found. Make sure you have Sphinx
+ echo.installed, then set the SPHINXBUILD environment variable to point
+ echo.to the full path of the 'sphinx-build' executable. Alternatively you
+ echo.may add the Sphinx directory to PATH.
+ echo.
+ echo.If you don't have Sphinx installed, grab it from
+ echo.http://sphinx-doc.org/
+ exit /b 1
+)
+
+%SPHINXBUILD% -M %1 %SOURCEDIR% %BUILDDIR% %SPHINXOPTS% %O%
+goto end
+
+:help
+%SPHINXBUILD% -M help %SOURCEDIR% %BUILDDIR% %SPHINXOPTS% %O%
+
+:end
+popd
diff --git a/docs/zh_cn/model_zoo.md b/docs/zh_cn/model_zoo.md
new file mode 100644
index 0000000..48cbc98
--- /dev/null
+++ b/docs/zh_cn/model_zoo.md
@@ -0,0 +1,109 @@
+# 模型库
+
+## 通用设置
+
+- 使用分布式训练;
+- 为了和其他代码库做公平对比,本文展示的是使用 `torch.cuda.max_memory_allocated()` 在 8 个 GPUs 上得到的最大 GPU 显存占用值,需要注意的是,这些显存占用值通常小于 `nvidia-smi` 显示出来的显存占用值;
+- 在模型库中所展示的推理时间是包括网络前向传播和后处理所需的总时间,不包括数据加载所需的时间,模型库中所展示的结果均由 [benchmark.py](https://github.com/open-mmlab/mmdetection/blob/master/tools/analysis_tools/benchmark.py) 脚本文件在 2000 张图像上所计算的平均时间。
+
+## 基准结果
+
+### SECOND
+
+请参考 [SECOND](https://github.com/open-mmlab/mmdetection3d/blob/master/configs/second) 获取更多的细节,我们在 KITTI 和 Waymo 数据集上都给出了相应的基准结果。
+
+### PointPillars
+
+请参考 [PointPillars](https://github.com/open-mmlab/mmdetection3d/blob/master/configs/pointpillars) 获取更多细节,我们在 KITTI 、nuScenes 、Lyft 、Waymo 数据集上给出了相应的基准结果。
+
+### Part-A2
+
+请参考 [Part-A2](https://github.com/open-mmlab/mmdetection3d/blob/master/configs/parta2) 获取更多细节。
+
+### VoteNet
+
+请参考 [VoteNet](https://github.com/open-mmlab/mmdetection3d/blob/master/configs/votenet) 获取更多细节,我们在 ScanNet 和 SUNRGBD 数据集上给出了相应的基准结果。
+
+### Dynamic Voxelization
+
+请参考 [Dynamic Voxelization](https://github.com/open-mmlab/mmdetection3d/blob/master/configs/dynamic_voxelization) 获取更多细节。
+
+### MVXNet
+
+请参考 [MVXNet](https://github.com/open-mmlab/mmdetection3d/blob/master/configs/mvxnet) 获取更多细节。
+
+### RegNetX
+
+请参考 [RegNet](https://github.com/open-mmlab/mmdetection3d/blob/master/configs/regnet) 获取更多细节,我们将 pointpillars 的主干网络替换成 RegNetX,并在 nuScenes 和 Lyft 数据集上给出了相应的基准结果。
+
+### nuImages
+
+我们在 [nuImages 数据集](https://www.nuscenes.org/nuimages) 上也提供基准模型,请参考 [nuImages](https://github.com/open-mmlab/mmdetection3d/blob/master/configs/nuimages) 获取更多细节,我们在该数据集上提供 Mask R-CNN , Cascade Mask R-CNN 和 HTC 的结果。
+
+### H3DNet
+
+请参考 [H3DNet](https://github.com/open-mmlab/mmdetection3d/blob/master/configs/h3dnet) 获取更多细节。
+
+### 3DSSD
+
+请参考 [3DSSD](https://github.com/open-mmlab/mmdetection3d/blob/master/configs/3dssd) 获取更多细节。
+
+### CenterPoint
+
+请参考 [CenterPoint](https://github.com/open-mmlab/mmdetection3d/blob/master/configs/centerpoint) 获取更多细节。
+
+### SSN
+
+请参考 [SSN](https://github.com/open-mmlab/mmdetection3d/blob/master/configs/ssn) 获取更多细节,我们将 pointpillars 中的检测头替换成 SSN 模型中所使用的 ‘shape-aware grouping heads’,并在 nuScenes 和 Lyft 数据集上给出了相应的基准结果。
+
+### ImVoteNet
+
+请参考 [ImVoteNet](https://github.com/open-mmlab/mmdetection3d/blob/master/configs/imvotenet) 获取更多细节,我们在 SUNRGBD 数据集上给出了相应的结果。
+
+### FCOS3D
+
+请参考 [FCOS3D](https://github.com/open-mmlab/mmdetection3d/blob/master/configs/fcos3d) 获取更多细节,我们在 nuScenes 数据集上给出了相应的结果。
+
+### PointNet++
+
+请参考 [PointNet++](https://github.com/open-mmlab/mmdetection3d/blob/master/configs/pointnet2) 获取更多细节,我们在 ScanNet 和 S3DIS 数据集上给出了相应的结果。
+
+### Group-Free-3D
+
+请参考 [Group-Free-3D](https://github.com/open-mmlab/mmdetection3d/blob/master/configs/groupfree3d) 获取更多细节,我们在 ScanNet 数据集上给出了相应的结果。
+
+### ImVoxelNet
+
+请参考 [ImVoxelNet](https://github.com/open-mmlab/mmdetection3d/blob/master/configs/imvoxelnet) 获取更多细节,我们在 KITTI 数据集上给出了相应的结果。
+
+### PAConv
+
+请参考 [PAConv](https://github.com/open-mmlab/mmdetection3d/blob/master/configs/paconv) 获取更多细节,我们在 S3DIS 数据集上给出了相应的结果.
+
+### DGCNN
+
+请参考 [DGCNN](https://github.com/open-mmlab/mmdetection3d/tree/v1.0.0.dev0/configs/dgcnn) 获取更多细节,我们在 S3DIS 数据集上给出了相应的结果.
+
+### SMOKE
+
+请参考 [SMOKE](https://github.com/open-mmlab/mmdetection3d/tree/v1.0.0.dev0/configs/smoke) 获取更多细节,我们在 KITTI 数据集上给出了相应的结果.
+
+### PGD
+
+请参考 [PGD](https://github.com/open-mmlab/mmdetection3d/tree/v1.0.0.dev0/configs/pgd) 获取更多细节,我们在 KITTI 和 nuScenes 数据集上给出了相应的结果.
+
+### PointRCNN
+
+请参考 [PointRCNN](https://github.com/open-mmlab/mmdetection3d/tree/v1.0.0.dev0/configs/point_rcnn) 获取更多细节,我们在 KITTI 数据集上给出了相应的结果.
+
+### MonoFlex
+
+请参考 [MonoFlex](https://github.com/open-mmlab/mmdetection3d/tree/v1.0.0.dev0/configs/monoflex) 获取更多细节,我们在 KITTI 数据集上给出了相应的结果.
+
+### SA-SSD
+
+请参考 [SA-SSD](https://github.com/open-mmlab/mmdetection3d/blob/master/configs/sassd) 获取更多的细节,我们在 KITTI 数据集上给出了相应的基准结果。
+
+### Mixed Precision (FP16) Training
+
+细节请参考 [Mixed Precision (FP16) Training 在 PointPillars 训练的样例](https://github.com/open-mmlab/mmdetection3d/tree/v1.0.0.dev0/configs/pointpillars/hv_pointpillars_fpn_sbn-all_fp16_2x8_2x_nus-3d.py).
diff --git a/docs/zh_cn/stat.py b/docs/zh_cn/stat.py
new file mode 100755
index 0000000..b5f10a8
--- /dev/null
+++ b/docs/zh_cn/stat.py
@@ -0,0 +1,62 @@
+#!/usr/bin/env python
+import functools as func
+import glob
+import re
+from os import path as osp
+
+import numpy as np
+
+url_prefix = 'https://github.com/open-mmlab/mmdetection3d/blob/master/'
+
+files = sorted(glob.glob('../configs/*/README.md'))
+
+stats = []
+titles = []
+num_ckpts = 0
+
+for f in files:
+ url = osp.dirname(f.replace('../', url_prefix))
+
+ with open(f, 'r') as content_file:
+ content = content_file.read()
+
+ title = content.split('\n')[0].replace('#', '').strip()
+ ckpts = set(x.lower().strip()
+ for x in re.findall(r'https?://download.*\.pth', content)
+ if 'mmdetection3d' in x)
+ if len(ckpts) == 0:
+ continue
+
+ _papertype = [x for x in re.findall(r'', content)]
+ assert len(_papertype) > 0
+ papertype = _papertype[0]
+
+ paper = set([(papertype, title)])
+
+ titles.append(title)
+ num_ckpts += len(ckpts)
+ statsmsg = f"""
+\t* [{papertype}] [{title}]({url}) ({len(ckpts)} ckpts)
+"""
+ stats.append((paper, ckpts, statsmsg))
+
+allpapers = func.reduce(lambda a, b: a.union(b), [p for p, _, _ in stats])
+msglist = '\n'.join(x for _, _, x in stats)
+
+papertypes, papercounts = np.unique([t for t, _ in allpapers],
+ return_counts=True)
+countstr = '\n'.join(
+ [f' - {t}: {c}' for t, c in zip(papertypes, papercounts)])
+
+modelzoo = f"""
+\n## Model Zoo Statistics
+
+* Number of papers: {len(set(titles))}
+{countstr}
+
+* Number of checkpoints: {num_ckpts}
+{msglist}
+"""
+
+with open('model_zoo.md', 'a') as f:
+ f.write(modelzoo)
diff --git a/docs/zh_cn/supported_tasks/index.rst b/docs/zh_cn/supported_tasks/index.rst
new file mode 100644
index 0000000..7b30c59
--- /dev/null
+++ b/docs/zh_cn/supported_tasks/index.rst
@@ -0,0 +1,6 @@
+.. toctree::
+ :maxdepth: 2
+
+ lidar_det3d.md
+ vision_det3d.md
+ lidar_sem_seg3d.md
diff --git a/docs/zh_cn/supported_tasks/lidar_det3d.md b/docs/zh_cn/supported_tasks/lidar_det3d.md
new file mode 100644
index 0000000..9294581
--- /dev/null
+++ b/docs/zh_cn/supported_tasks/lidar_det3d.md
@@ -0,0 +1,82 @@
+# 基于 LiDAR 的 3D 检测
+
+基于 LiDAR 的 3D 检测算法是 MMDetection3D 支持的最基础的任务之一。对于给定的算法模型,输入为任意数量的、附有 LiDAR 采集的特征的点,输出为每个感兴趣目标的 3D 矩形框 (Bounding Box) 和类别标签。接下来,我们将以在 KITTI 数据集上训练 PointPillars 为例,介绍如何准备数据,如何在标准 3D 检测基准数据集上训练和测试模型,以及如何可视化并验证结果。
+
+## 数据预处理
+
+最开始,我们需要下载原始数据,并按[文档](https://mmdetection3d.readthedocs.io/zh_CN/latest/data_preparation.html)中介绍的那样,把数据重新整理成标准格式。值得注意的是,对于 KIITI 数据集,我们需要额外的 txt 文件用于数据整理。
+
+由于不同数据集上的原始数据有不同的组织方式,我们通常需要用 .pkl 或者 .json 文件收集有用的数据信息。在准备好原始数据后,我们需要运行脚本 `create_data.py`,为不同的数据集生成数据。如,对于 KITTI 数据集,我们需要执行:
+
+```
+python tools/create_data.py kitti --root-path ./data/kitti --out-dir ./data/kitti --extra-tag kitti
+```
+
+随后,相对目录结构将变成如下形式:
+
+```
+mmdetection3d
+├── mmdet3d
+├── tools
+├── configs
+├── data
+│ ├── kitti
+│ │ ├── ImageSets
+│ │ ├── testing
+│ │ │ ├── calib
+│ │ │ ├── image_2
+│ │ │ ├── velodyne
+│ │ ├── training
+│ │ │ ├── calib
+│ │ │ ├── image_2
+│ │ │ ├── label_2
+│ │ │ ├── velodyne
+│ │ ├── kitti_gt_database
+│ │ ├── kitti_infos_train.pkl
+│ │ ├── kitti_infos_trainval.pkl
+│ │ ├── kitti_infos_val.pkl
+│ │ ├── kitti_infos_test.pkl
+│ │ ├── kitti_dbinfos_train.pkl
+```
+
+## 训练
+
+接着,我们将使用提供的配置文件训练 PointPillars。当你使用不同的 GPU 设置进行训练时,你基本上可以按照这个[教程](https://mmdetection3d.readthedocs.io/zh_CN/latest/1_exist_data_model.html)的示例脚本进行训练。假设我们在一台具有 8 块 GPU 的机器上进行分布式训练:
+
+```
+./tools/dist_train.sh configs/pointpillars/hv_pointpillars_secfpn_6x8_160e_kitti-3d-3class.py 8
+```
+
+注意到,配置文件名字中的 `6x8` 是指训练时是用了 8 块 GPU,每块 GPU 上有 6 个样本。如果你有不同的自定义的设置,那么有时你可能需要调整学习率。可以参考这篇[文献](https://arxiv.org/abs/1706.02677)。
+
+## 定量评估
+
+在训练期间,模型将会根据配置文件中的 `evaluation = dict(interval=xxx)` 设置,被周期性地评估。我们支持不同数据集的官方评估方案。对于 KITTI, 模型的评价指标为平均精度 (mAP, mean average precision)。3 种类型的 mAP 的交并比 (IoU, Intersection over Union) 阈值可以取 0.5/0.7。评估结果将会被打印到终端中,如下所示:
+
+```
+Car AP@0.70, 0.70, 0.70:
+bbox AP:98.1839, 89.7606, 88.7837
+bev AP:89.6905, 87.4570, 85.4865
+3d AP:87.4561, 76.7569, 74.1302
+aos AP:97.70, 88.73, 87.34
+Car AP@0.70, 0.50, 0.50:
+bbox AP:98.1839, 89.7606, 88.7837
+bev AP:98.4400, 90.1218, 89.6270
+3d AP:98.3329, 90.0209, 89.4035
+aos AP:97.70, 88.73, 87.34
+```
+
+评估某个特定的模型权重文件。你可以简单地执行下列的脚本:
+
+```
+./tools/dist_test.sh configs/pointpillars/hv_pointpillars_secfpn_6x8_160e_kitti-3d-3class.py \
+ work_dirs/pointpillars/latest.pth --eval mAP
+```
+
+## 测试与提交
+
+如果你只想在线上基准上进行推理或者测试模型的表现,你只需要把上面评估脚本中的 `--eval mAP` 替换为 `--format-only`。如果需要的话,还可以指定 `pklfile_prefix` 和 `submission_prefix`,如,添加命令行选项 `--eval-options submission_prefix=work_dirs/pointpillars/test_submission`。请确保配置文件中的[测试信息](https://github.com/open-mmlab/mmdetection3d/blob/master/configs/_base_/datasets/kitti-3d-3class.py#L131)与测试集对应,而不是验证集。在生成结果后,你可以压缩文件夹,并上传到 KITTI 的评估服务器上。
+
+## 定性验证
+
+MMDetection3D 还提供了通用的可视化工具,以便于我们可以对训练好的模型的预测结果有一个直观的感受。你可以在命令行中添加 `--eval-options 'show=True' 'out_dir=${SHOW_DIR}'` 选项,在评估过程中在线地可视化检测结果;你也可以使用 `tools/misc/visualize_results.py`, 离线地进行可视化。另外,我们还提供了脚本 `tools/misc/browse_dataset.py`, 可视化数据集而不做推理。更多的细节请参考[可视化文档](https://mmdetection3d.readthedocs.io/zh_CN/latest/useful_tools.html#id2)
diff --git a/docs/zh_cn/supported_tasks/lidar_sem_seg3d.md b/docs/zh_cn/supported_tasks/lidar_sem_seg3d.md
new file mode 100644
index 0000000..7e289c4
--- /dev/null
+++ b/docs/zh_cn/supported_tasks/lidar_sem_seg3d.md
@@ -0,0 +1,78 @@
+# 基于激光雷达的 3D 语义分割
+
+基于激光雷达的 3D 语义分割是 MMDetection3D 支持的最基础的任务之一。它期望给定的模型以激光雷达采集的任意数量的特征点为输入,并预测每个输入点的语义标签。接下来,我们以 ScanNet 数据集上的 PointNet++ (SSG) 为例,展示如何准备数据,在标准的 3D 语义分割基准上训练并测试模型,以及可视化并验证结果。
+
+## 数据准备
+
+首先,我们需要从 ScanNet [官方网站](http://kaldir.vc.in.tum.de/scannet_benchmark/documentation)下载原始数据。
+
+由于不同数据集的原始数据有不同的组织方式,我们通常需要用 pkl 或 json 文件收集有用的数据信息。
+
+因此,在准备好所有的原始数据之后,我们可以遵循 [ScanNet 文档](https://github.com/open-mmlab/mmdetection3d/blob/master/data/scannet/README.md/)中的说明生成数据信息。
+
+随后,相关的目录结构将如下所示:
+
+```
+mmdetection3d
+├── mmdet3d
+├── tools
+├── configs
+├── data
+│ ├── scannet
+│ │ ├── scannet_utils.py
+│ │ ├── batch_load_scannet_data.py
+│ │ ├── load_scannet_data.py
+│ │ ├── scannet_utils.py
+│ │ ├── README.md
+│ │ ├── scans
+│ │ ├── scans_test
+│ │ ├── scannet_instance_data
+│ │ ├── points
+│ │ ├── instance_mask
+│ │ ├── semantic_mask
+│ │ ├── seg_info
+│ │ │ ├── train_label_weight.npy
+│ │ │ ├── train_resampled_scene_idxs.npy
+│ │ │ ├── val_label_weight.npy
+│ │ │ ├── val_resampled_scene_idxs.npy
+│ │ ├── scannet_infos_train.pkl
+│ │ ├── scannet_infos_val.pkl
+│ │ ├── scannet_infos_test.pkl
+```
+
+## 训练
+
+接着,我们将使用提供的配置文件训练 PointNet++ (SSG) 模型。当你使用不同的 GPU 设置进行训练时,你基本上可以按照这个[教程](https://mmdetection3d.readthedocs.io/zh_CN/latest/1_exist_data_model.html#inference-with-existing-models)的示例脚本。假设我们在一台具有 2 块 GPU 的机器上使用分布式训练:
+
+```
+./tools/dist_train.sh configs/pointnet2/pointnet2_ssg_16x2_cosine_200e_scannet_seg-3d-20class.py 2
+```
+
+注意,配置文件名中的 `16x2` 是指训练时用了 2 块 GPU,每块 GPU 上有 16 个样本。如果你的自定义设置不同于此,那么有时候你需要相应的调整学习率。基本规则可以参考[此处](https://arxiv.org/abs/1706.02677)。
+
+## 定量评估
+
+在训练期间,模型权重将会根据配置文件中的 `evaluation = dict(interval=xxx)` 设置被周期性地评估。我们支持不同数据集的官方评估方案。对于 ScanNet,将使用 20 个类别的平均交并比 (mIoU) 对模型进行评估。评估结果将会被打印到终端中,如下所示:
+
+```
++---------+--------+--------+---------+--------+--------+--------+--------+--------+--------+-----------+---------+---------+--------+---------+--------------+----------------+--------+--------+---------+----------------+--------+--------+---------+
+| classes | wall | floor | cabinet | bed | chair | sofa | table | door | window | bookshelf | picture | counter | desk | curtain | refrigerator | showercurtrain | toilet | sink | bathtub | otherfurniture | miou | acc | acc_cls |
++---------+--------+--------+---------+--------+--------+--------+--------+--------+--------+-----------+---------+---------+--------+---------+--------------+----------------+--------+--------+---------+----------------+--------+--------+---------+
+| results | 0.7257 | 0.9373 | 0.4625 | 0.6613 | 0.7707 | 0.5562 | 0.5864 | 0.4010 | 0.4558 | 0.7011 | 0.2500 | 0.4645 | 0.4540 | 0.5399 | 0.2802 | 0.3488 | 0.7359 | 0.4971 | 0.6922 | 0.3681 | 0.5444 | 0.8118 | 0.6695 |
++---------+--------+--------+---------+--------+--------+--------+--------+--------+--------+-----------+---------+---------+--------+---------+--------------+----------------+--------+--------+---------+----------------+--------+--------+---------+
+```
+
+此外,在训练完成后你也可以评估特定的模型权重文件。你可以简单地执行以下脚本:
+
+```
+./tools/dist_test.sh configs/pointnet2/pointnet2_ssg_16x2_cosine_200e_scannet_seg-3d-20class.py \
+ work_dirs/pointnet2_ssg/latest.pth --eval mIoU
+```
+
+## 测试与提交
+
+如果你只想在在线基准上进行推理或测试模型性能,你需要将之前评估脚本中的 `--eval mIoU` 替换成 `--format-only`,并将 ScanNet 数据集[配置文件](https://github.com/open-mmlab/mmdetection3d/blob/master/configs/_base_/datasets/scannet_seg-3d-20class.py#L126)中的 `ann_file=data_root + 'scannet_infos_val.pkl'` 变成 `ann_file=data_root + 'scannet_infos_test.pkl'`。记住将 `txt_prefix` 指定为保存测试结果的目录,例如,添加选项 `--eval-options txt_prefix=work_dirs/pointnet2_ssg/test_submission`。在生成结果后,你可以压缩文件夹并上传至 [ScanNet 评估服务器](http://kaldir.vc.in.tum.de/scannet_benchmark/semantic_label_3d)上。
+
+## 定性评估
+
+MMDetection3D 还提供了通用的可视化工具,以便于我们可以对训练好的模型预测的分割结果有一个直观的感受。你也可以在评估阶段通过设置 `--eval-options 'show=True' 'out_dir=${SHOW_DIR}'` 来在线可视化分割结果,或者使用 `tools/misc/visualize_results.py` 来离线地进行可视化。此外,我们还提供了脚本 `tools/misc/browse_dataset.py` 用于可视化数据集而不做推理。更多的细节请参考[可视化文档](https://mmdetection3d.readthedocs.io/zh_CN/latest/useful_tools.html#visualization)。
diff --git a/docs/zh_cn/supported_tasks/vision_det3d.md b/docs/zh_cn/supported_tasks/vision_det3d.md
new file mode 100644
index 0000000..18c546e
--- /dev/null
+++ b/docs/zh_cn/supported_tasks/vision_det3d.md
@@ -0,0 +1,114 @@
+# 基于视觉的 3D 检测
+
+基于视觉的 3D 检测是指基于纯视觉输入的 3D 检测方法,例如基于单目、双目和多视图图像的 3D 检测。目前,我们只支持单目和多视图的 3D 检测方法。其他方法也应该与我们的框架兼容,并在将来得到支持。
+
+它期望给定的模型以任意数量的图像作为输入,并为每一个感兴趣的目标预测 3D 框及类别标签。以 nuScenes 数据集 FCOS3D 为例,我们将展示如何准备数据,在标准的 3D 检测基准上训练并测试模型,以及可视化并验证结果。
+
+## 数据准备
+
+首先,我们需要下载原始数据并按照[数据准备文档](https://mmdetection3d.readthedocs.io/zh_CN/latest/data_preparation.html)中提供的标准方式重新组织数据。
+
+由于不同数据集的原始数据有不同的组织方式,我们通常需要用 pkl 或 json 文件收集有用的数据信息。因此,在准备好所有的原始数据之后,我们需要运行 `create_data.py` 中提供的脚本来为不同的数据集生成数据信息。例如,对于 nuScenes,我们需要运行如下命令:
+
+```
+python tools/create_data.py nuscenes --root-path ./data/nuscenes --out-dir ./data/nuscenes --extra-tag nuscenes
+```
+
+随后,相关的目录结构将如下所示:
+
+```
+mmdetection3d
+├── mmdet3d
+├── tools
+├── configs
+├── data
+│ ├── nuscenes
+│ │ ├── maps
+│ │ ├── samples
+│ │ ├── sweeps
+│ │ ├── v1.0-test
+| | ├── v1.0-trainval
+│ │ ├── nuscenes_database
+│ │ ├── nuscenes_infos_train.pkl
+│ │ ├── nuscenes_infos_trainval.pkl
+│ │ ├── nuscenes_infos_val.pkl
+│ │ ├── nuscenes_infos_test.pkl
+│ │ ├── nuscenes_dbinfos_train.pkl
+│ │ ├── nuscenes_infos_train_mono3d.coco.json
+│ │ ├── nuscenes_infos_trainval_mono3d.coco.json
+│ │ ├── nuscenes_infos_val_mono3d.coco.json
+│ │ ├── nuscenes_infos_test_mono3d.coco.json
+```
+
+注意,此处的 pkl 文件主要用于使用 LiDAR 数据的方法,json 文件用于 2D 检测/纯视觉的 3D 检测。在 v0.13.0 支持单目 3D 检测之前,json 文件只包含 2D 检测的信息,因此如果你需要最新的信息,请切换到 v0.13.0 之后的分支。
+
+## 训练
+
+接着,我们将使用提供的配置文件训练 FCOS3D。基本的脚本与其他模型一样。当你使用不同的 GPU 设置进行训练时,你基本上可以按照这个[教程](https://mmdetection3d.readthedocs.io/zh_CN/latest/1_exist_data_model.html#inference-with-existing-models)的示例。假设我们在一台具有 8 块 GPU 的机器上使用分布式训练:
+
+```
+./tools/dist_train.sh configs/fcos3d/fcos3d_r101_caffe_fpn_gn-head_dcn_2x8_1x_nus-mono3d.py 8
+```
+
+注意,配置文件名中的 `2x8` 是指训练时用了 8 块 GPU,每块 GPU 上有 2 个数据样本。如果你的自定义设置不同于此,那么有时候你需要相应的调整学习率。基本规则可以参考[此处](https://arxiv.org/abs/1706.02677)。
+
+我们也可以通过运行以下命令微调 FCOS3D,从而达到更好的性能:
+
+```
+./tools/dist_train.sh fcos3d_r101_caffe_fpn_gn-head_dcn_2x8_1x_nus-mono3d_finetune.py 8
+```
+
+通过先前的脚本训练好一个基准模型后,请记得相应的修改[此处](https://github.com/open-mmlab/mmdetection3d/blob/master/configs/fcos3d/fcos3d_r101_caffe_fpn_gn-head_dcn_2x8_1x_nus-mono3d_finetune.py#L8)的路径。
+
+## 定量评估
+
+在训练期间,模型权重文件将会根据配置文件中的 `evaluation = dict(interval=xxx)` 设置被周期性地评估。
+
+我们支持不同数据集的官方评估方案。由于输出格式与基于其他模态的 3D 检测相同,因此评估方法也是一样的。
+
+对于 nuScenes,将使用基于距离的平均精度(mAP)以及 nuScenes 检测分数(NDS)分别对 10 个类别进行评估。评估结果将会被打印到终端中,如下所示:
+
+```
+mAP: 0.3197
+mATE: 0.7595
+mASE: 0.2700
+mAOE: 0.4918
+mAVE: 1.3307
+mAAE: 0.1724
+NDS: 0.3905
+Eval time: 170.8s
+
+Per-class results:
+Object Class AP ATE ASE AOE AVE AAE
+car 0.503 0.577 0.152 0.111 2.096 0.136
+truck 0.223 0.857 0.224 0.220 1.389 0.179
+bus 0.294 0.855 0.204 0.190 2.689 0.283
+trailer 0.081 1.094 0.243 0.553 0.742 0.167
+construction_vehicle 0.058 1.017 0.450 1.019 0.137 0.341
+pedestrian 0.392 0.687 0.284 0.694 0.876 0.158
+motorcycle 0.317 0.737 0.265 0.580 2.033 0.104
+bicycle 0.308 0.704 0.299 0.892 0.683 0.010
+traffic_cone 0.555 0.486 0.309 nan nan nan
+barrier 0.466 0.581 0.269 0.169 nan nan
+```
+
+此外,在训练完成后你也可以评估特定的模型权重文件。你可以简单地执行以下脚本:
+
+```
+./tools/dist_test.sh configs/fcos3d/fcos3d_r101_caffe_fpn_gn-head_dcn_2x8_1x_nus-mono3d.py \
+ work_dirs/fcos3d/latest.pth --eval mAP
+```
+
+## 测试与提交
+
+如果你只想在在线基准上进行推理或测试模型性能,你需要将之前评估脚本中的 `--eval mAP` 替换成 `--format-only`,并在需要的情况下指定 `jsonfile_prefix`,例如,添加选项 `--eval-options jsonfile_prefix=work_dirs/fcos3d/test_submission`。请确保配置文件中的[测试信息](https://github.com/open-mmlab/mmdetection3d/blob/master/configs/_base_/datasets/nus-mono3d.py#L93)由验证集相应地改为测试集。
+
+在生成结果后,你可以压缩文件夹并上传至 nuScenes 3D 检测挑战的 evalAI 评估服务器上。
+
+## 定性评估
+
+MMDetection3D 还提供了通用的可视化工具,以便于我们可以对训练好的模型预测的检测结果有一个直观的感受。你也可以在评估阶段通过设置 `--eval-options 'show=True' 'out_dir=${SHOW_DIR}'` 来在线可视化检测结果,或者使用 `tools/misc/visualize_results.py` 来离线地进行可视化。
+
+此外,我们还提供了脚本 `tools/misc/browse_dataset.py` 用于可视化数据集而不做推理。更多的细节请参考[可视化文档](https://mmdetection3d.readthedocs.io/zh_CN/latest/useful_tools.html#visualization)。
+
+注意,目前我们仅支持纯视觉方法在图像上的可视化。将来我们将集成在前景图以及鸟瞰图(BEV)中的可视化。
diff --git a/docs/zh_cn/switch_language.md b/docs/zh_cn/switch_language.md
new file mode 100644
index 0000000..d33d080
--- /dev/null
+++ b/docs/zh_cn/switch_language.md
@@ -0,0 +1,3 @@
+## English
+
+## 简体中文
diff --git a/docs/zh_cn/tutorials/backends_support.md b/docs/zh_cn/tutorials/backends_support.md
new file mode 100644
index 0000000..bdcaf15
--- /dev/null
+++ b/docs/zh_cn/tutorials/backends_support.md
@@ -0,0 +1,154 @@
+# 教程 7: 后端支持
+
+我们支持不同的文件客户端后端:磁盘、Ceph 和 LMDB 等。下面是修改配置使之从 Ceph 加载和保存数据的示例。
+
+## 从 Ceph 读取数据和标注文件
+
+我们支持从 Ceph 加载数据和生成的标注信息文件(pkl 和 json):
+
+```python
+# set file client backends as Ceph
+file_client_args = dict(
+ backend='petrel',
+ path_mapping=dict({
+ './data/nuscenes/':
+ 's3://openmmlab/datasets/detection3d/nuscenes/', # replace the path with your data path on Ceph
+ 'data/nuscenes/':
+ 's3://openmmlab/datasets/detection3d/nuscenes/' # replace the path with your data path on Ceph
+ }))
+
+db_sampler = dict(
+ data_root=data_root,
+ info_path=data_root + 'kitti_dbinfos_train.pkl',
+ rate=1.0,
+ prepare=dict(filter_by_difficulty=[-1], filter_by_min_points=dict(Car=5)),
+ sample_groups=dict(Car=15),
+ classes=class_names,
+ # set file client for points loader to load training data
+ points_loader=dict(
+ type='LoadPointsFromFile',
+ coord_type='LIDAR',
+ load_dim=4,
+ use_dim=4,
+ file_client_args=file_client_args),
+ # set file client for data base sampler to load db info file
+ file_client_args=file_client_args)
+
+train_pipeline = [
+ # set file client for loading training data
+ dict(type='LoadPointsFromFile', coord_type='LIDAR', load_dim=4, use_dim=4, file_client_args=file_client_args),
+ # set file client for loading training data annotations
+ dict(type='LoadAnnotations3D', with_bbox_3d=True, with_label_3d=True, file_client_args=file_client_args),
+ dict(type='ObjectSample', db_sampler=db_sampler),
+ dict(
+ type='ObjectNoise',
+ num_try=100,
+ translation_std=[0.25, 0.25, 0.25],
+ global_rot_range=[0.0, 0.0],
+ rot_range=[-0.15707963267, 0.15707963267]),
+ dict(type='RandomFlip3D', flip_ratio_bev_horizontal=0.5),
+ dict(
+ type='GlobalRotScaleTrans',
+ rot_range=[-0.78539816, 0.78539816],
+ scale_ratio_range=[0.95, 1.05]),
+ dict(type='PointsRangeFilter', point_cloud_range=point_cloud_range),
+ dict(type='ObjectRangeFilter', point_cloud_range=point_cloud_range),
+ dict(type='PointShuffle'),
+ dict(type='DefaultFormatBundle3D', class_names=class_names),
+ dict(type='Collect3D', keys=['points', 'gt_bboxes_3d', 'gt_labels_3d'])
+]
+test_pipeline = [
+ # set file client for loading validation/testing data
+ dict(type='LoadPointsFromFile', coord_type='LIDAR', load_dim=4, use_dim=4, file_client_args=file_client_args),
+ dict(
+ type='MultiScaleFlipAug3D',
+ img_scale=(1333, 800),
+ pts_scale_ratio=1,
+ flip=False,
+ transforms=[
+ dict(
+ type='GlobalRotScaleTrans',
+ rot_range=[0, 0],
+ scale_ratio_range=[1., 1.],
+ translation_std=[0, 0, 0]),
+ dict(type='RandomFlip3D'),
+ dict(
+ type='PointsRangeFilter', point_cloud_range=point_cloud_range),
+ dict(
+ type='DefaultFormatBundle3D',
+ class_names=class_names,
+ with_label=False),
+ dict(type='Collect3D', keys=['points'])
+ ])
+]
+
+data = dict(
+ # set file client for loading training info files (.pkl)
+ train=dict(
+ type='RepeatDataset',
+ times=2,
+ dataset=dict(pipeline=train_pipeline, classes=class_names, file_client_args=file_client_args)),
+ # set file client for loading validation info files (.pkl)
+ val=dict(pipeline=test_pipeline, classes=class_names,file_client_args=file_client_args),
+ # set file client for loading testing info files (.pkl)
+ test=dict(pipeline=test_pipeline, classes=class_names, file_client_args=file_client_args))
+```
+
+## 从 Ceph 读取预训练模型
+
+```python
+model = dict(
+ pts_backbone=dict(
+ _delete_=True,
+ type='NoStemRegNet',
+ arch='regnetx_1.6gf',
+ init_cfg=dict(
+ type='Pretrained', checkpoint='s3://openmmlab/checkpoints/mmdetection3d/regnetx_1.6gf'), # replace the path with your pretrained model path on Ceph
+ ...
+```
+
+## 从 Ceph 读取模型权重文件
+
+```python
+# replace the path with your checkpoint path on Ceph
+load_from = 's3://openmmlab/checkpoints/mmdetection3d/v0.1.0_models/pointpillars/hv_pointpillars_secfpn_6x8_160e_kitti-3d-car/hv_pointpillars_secfpn_6x8_160e_kitti-3d-car_20200620_230614-77663cd6.pth.pth'
+resume_from = None
+workflow = [('train', 1)]
+```
+
+## 保存模型权重文件至 Ceph
+
+```python
+# checkpoint saving
+# replace the path with your checkpoint saving path on Ceph
+checkpoint_config = dict(interval=1, max_keep_ckpts=2, out_dir='s3://openmmlab/mmdetection3d')
+```
+
+## EvalHook 保存最优模型权重文件至 Ceph
+
+```python
+# replace the path with your checkpoint saving path on Ceph
+evaluation = dict(interval=1, save_best='bbox', out_dir='s3://openmmlab/mmdetection3d')
+```
+
+## 训练日志保存至 Ceph
+
+训练后的训练日志会备份到指定的 Ceph 路径。
+
+```python
+log_config = dict(
+ interval=50,
+ hooks=[
+ dict(type='TextLoggerHook', out_dir='s3://openmmlab/mmdetection3d'),
+ ])
+```
+
+您还可以通过设置 `keep_local = False` 备份到指定的 Ceph 路径后删除本地训练日志。
+
+```python
+log_config = dict(
+ interval=50,
+ hooks=[
+ dict(type='TextLoggerHook', out_dir='s3://openmmlab/mmdetection3d'', keep_local=False),
+ ])
+```
diff --git a/docs/zh_cn/tutorials/config.md b/docs/zh_cn/tutorials/config.md
new file mode 100644
index 0000000..329dd90
--- /dev/null
+++ b/docs/zh_cn/tutorials/config.md
@@ -0,0 +1,527 @@
+# 教程 1: 学习配置文件
+
+我们在配置文件中支持了继承和模块化来方便进行各种实验。
+如果需要检查配置文件,可以通过运行 `python tools/misc/print_config.py /PATH/TO/CONFIG` 来查看完整的配置。
+你也可以传入 `--options xxx.yyy=zzz` 参数来查看更新后的配置。
+
+## 配置文件结构
+
+在 `config/_base_` 文件夹下有 4 个基本组件类型,分别是:数据集 (dataset),模型 (model),训练策略 (schedule) 和运行时的默认设置 (default runtime)。
+通过从上述每个文件夹中选取一个组件进行组合,许多方法如 SECOND、PointPillars、PartA2 和 VoteNet 都能够很容易地构建出来。
+由 `_base_` 下的组件组成的配置,被我们称为 _原始配置 (primitive)_。
+
+对于同一文件夹下的所有配置,推荐**只有一个**对应的 _原始配置_ 文件,所有其他的配置文件都应该继承自这个 _原始配置_ 文件,这样就能保证配置文件的最大继承深度为 3。
+
+为了便于理解,我们建议贡献者继承现有方法。
+例如,如果在 PointPillars 的基础上做了一些修改,用户首先可以通过指定 `_base_ = ../pointpillars/hv_pointpillars_fpn_sbn-all_4x8_2x_nus-3d.py` 来继承基础的 PointPillars 结构,然后修改配置文件中的必要参数以完成继承。
+
+如果你在构建一个与任何现有方法不共享结构的全新方法,可以在 `configs` 文件夹下创建一个新的例如 `xxx_rcnn` 文件夹。
+
+更多细节请参考 [MMCV](https://mmcv.readthedocs.io/en/latest/understand_mmcv/config.html) 文档。
+
+## 配置文件名称风格
+
+我们遵循以下样式来命名配置文件,并建议贡献者遵循相同的风格。
+
+```
+{model}_[model setting]_{backbone}_{neck}_[norm setting]_[misc]_[gpu x batch_per_gpu]_{schedule}_{dataset}
+```
+
+`{xxx}` 是被要求填写的字段而 `[yyy]` 是可选的。
+
+- `{model}`:模型种类,例如 `hv_pointpillars` (Hard Voxelization PointPillars)、`VoteNet` 等。
+- `[model setting]`:某些模型的特殊设定。
+- `{backbone}`: 主干网络种类例如 `regnet-400mf`、`regnet-1.6gf` 等。
+- `{neck}`:模型颈部的种类包括 `fpn`、`secfpn` 等。
+- `[norm_setting]`:如无特殊声明,默认使用 `bn` (Batch Normalization),其他类型可以有 `gn` (Group Normalization)、`sbn` (Synchronized Batch Normalization) 等。
+ `gn-head`/`gn-neck` 表示 GN 仅应用于网络的头部或颈部,而 `gn-all` 表示 GN 用于整个模型,例如主干网络、颈部和头部。
+- `[misc]`:模型中各式各样的设置/插件,例如 `strong-aug` 意味着在训练过程中使用更强的数据增广策略。
+- `[batch_per_gpu x gpu]`:每个 GPU 的样本数和 GPU 数量,默认使用 `4x8`。
+- `{schedule}`:训练方案,选项是 `1x`、`2x`、`20e` 等。
+ `1x` 和 `2x` 分别代表训练 12 和 24 轮。
+ `20e` 在级联模型中使用,表示训练 20 轮。
+ 对于 `1x`/`2x`,初始学习率在第 8/16 和第 11/22 轮衰减 10 倍;对于 `20e`,初始学习率在第 16 和第 19 轮衰减 10 倍。
+- `{dataset}`:数据集,例如 `nus-3d`、`kitti-3d`、`lyft-3d`、`scannet-3d`、`sunrgbd-3d` 等。
+ 当某一数据集存在多种设定时,我们也标记下所使用的类别数量,例如 `kitti-3d-3class` 和 `kitti-3d-car` 分别意味着在 KITTI 的所有三类上和单独车这一类上进行训练。
+
+## 弃用的 train_cfg/test_cfg
+
+遵循 MMDetection 的做法,我们在配置文件中弃用 `train_cfg` 和 `test_cfg`,请在模型配置中指定它们。
+原始的配置结构如下:
+
+```python
+# 已经弃用的形式
+model = dict(
+ type=...,
+ ...
+)
+train_cfg=dict(...)
+test_cfg=dict(...)
+```
+
+迁移后的配置结构如下:
+
+```python
+# 推荐的形式
+model = dict(
+ type=...,
+ ...
+ train_cfg=dict(...),
+ test_cfg=dict(...),
+)
+```
+
+## VoteNet 配置文件示例
+
+```python
+model = dict(
+ type='VoteNet', # 检测器的类型,更多细节请参考 mmdet3d.models.detectors
+ backbone=dict(
+ type='PointNet2SASSG', # 主干网络的类型,更多细节请参考 mmdet3d.models.backbones
+ in_channels=4, # 点云输入通道数
+ num_points=(2048, 1024, 512, 256), # 每个 SA 模块采样的中心点的数量
+ radius=(0.2, 0.4, 0.8, 1.2), # 每个 SA 层的半径
+ num_samples=(64, 32, 16, 16), # 每个 SA 层聚集的点的数量
+ sa_channels=((64, 64, 128), (128, 128, 256), (128, 128, 256),
+ (128, 128, 256)), # SA 模块中每个多层感知器的输出通道数
+ fp_channels=((256, 256), (256, 256)), # FP 模块中每个多层感知器的输出通道数
+ norm_cfg=dict(type='BN2d'), # 归一化层的配置
+ sa_cfg=dict( # 点集抽象 (SA) 模块的配置
+ type='PointSAModule', # SA 模块的类型
+ pool_mod='max', # SA 模块的池化方法 (最大池化或平均池化)
+ use_xyz=True, # 在特征聚合中是否使用 xyz 坐标
+ normalize_xyz=True)), # 在特征聚合中是否使用标准化的 xyz 坐标
+ bbox_head=dict(
+ type='VoteHead', # 检测框头的类型,更多细节请参考 mmdet3d.models.dense_heads
+ num_classes=18, # 分类的类别数量
+ bbox_coder=dict(
+ type='PartialBinBasedBBoxCoder', # 框编码层的类型,更多细节请参考 mmdet3d.core.bbox.coders
+ num_sizes=18, # 尺寸聚类的数量
+ num_dir_bins=1, # 编码方向角的间隔数
+ with_rot=False, # 框是否带有旋转角度
+ mean_sizes=[[0.76966727, 0.8116021, 0.92573744],
+ [1.876858, 1.8425595, 1.1931566],
+ [0.61328, 0.6148609, 0.7182701],
+ [1.3955007, 1.5121545, 0.83443564],
+ [0.97949594, 1.0675149, 0.6329687],
+ [0.531663, 0.5955577, 1.7500148],
+ [0.9624706, 0.72462326, 1.1481868],
+ [0.83221924, 1.0490936, 1.6875663],
+ [0.21132214, 0.4206159, 0.5372846],
+ [1.4440073, 1.8970833, 0.26985747],
+ [1.0294262, 1.4040797, 0.87554324],
+ [1.3766412, 0.65521795, 1.6813129],
+ [0.6650819, 0.71111923, 1.298853],
+ [0.41999173, 0.37906948, 1.7513971],
+ [0.59359556, 0.5912492, 0.73919016],
+ [0.50867593, 0.50656086, 0.30136237],
+ [1.1511526, 1.0546296, 0.49706793],
+ [0.47535285, 0.49249494, 0.5802117]]), # 每一类的平均尺寸,其顺序与类名顺序相同
+ vote_moudule_cfg=dict( # 投票 (vote) 模块的配置,更多细节请参考 mmdet3d.models.model_utils
+ in_channels=256, # 投票模块的输入通道数
+ vote_per_seed=1, # 对于每个种子点生成的投票数
+ gt_per_seed=3, # 每个种子点的真实标签个数
+ conv_channels=(256, 256), # 卷积通道数
+ conv_cfg=dict(type='Conv1d'), # 卷积配置
+ norm_cfg=dict(type='BN1d'), # 归一化层配置
+ norm_feats=True, # 是否标准化特征
+ vote_loss=dict( # 投票分支的损失函数配置
+ type='ChamferDistance', # 投票分支的损失函数类型
+ mode='l1', # 投票分支的损失函数模式
+ reduction='none', # 设置对损失函数输出的聚合方法
+ loss_dst_weight=10.0)), # 投票分支的目标损失权重
+ vote_aggregation_cfg=dict( # 投票聚合分支的配置
+ type='PointSAModule', # 投票聚合模块的类型
+ num_point=256, # 投票聚合分支中 SA 模块的点的数量
+ radius=0.3, # 投票聚合分支中 SA 模块的半径
+ num_sample=16, # 投票聚合分支中 SA 模块的采样点的数量
+ mlp_channels=[256, 128, 128, 128], # 投票聚合分支中 SA 模块的多层感知器的通道数
+ use_xyz=True, # 是否使用 xyz 坐标
+ normalize_xyz=True), # 是否使用标准化后的 xyz 坐标
+ feat_channels=(128, 128), # 特征卷积的通道数
+ conv_cfg=dict(type='Conv1d'), # 卷积的配置
+ norm_cfg=dict(type='BN1d'), # 归一化层的配置
+ objectness_loss=dict( # 物体性 (objectness) 损失函数的配置
+ type='CrossEntropyLoss', # 损失函数类型
+ class_weight=[0.2, 0.8], # 损失函数对每一类的权重
+ reduction='sum', # 设置损失函数输出的聚合方法
+ loss_weight=5.0), # 损失函数权重
+ center_loss=dict( # 中心 (center) 损失函数的配置
+ type='ChamferDistance', # 损失函数类型
+ mode='l2', # 损失函数模式
+ reduction='sum', # 设置损失函数输出的聚合方法
+ loss_src_weight=10.0, # 源损失权重
+ loss_dst_weight=10.0), # 目标损失权重
+ dir_class_loss=dict( # 方向分类损失函数的配置
+ type='CrossEntropyLoss', # 损失函数类型
+ reduction='sum', # 设置损失函数输出的聚合方法
+ loss_weight=1.0), # 损失函数权重
+ dir_res_loss=dict( # 方向残差 (residual) 损失函数的配置
+ type='SmoothL1Loss', # 损失函数类型
+ reduction='sum', # 设置损失函数输出的聚合方法
+ loss_weight=10.0), # 损失函数权重
+ size_class_loss=dict( # 尺寸分类损失函数的配置
+ type='CrossEntropyLoss', # 损失函数类型
+ reduction='sum', # 设置损失函数输出的聚合方法
+ loss_weight=1.0), # 损失函数权重
+ size_res_loss=dict( # 尺寸残差损失函数的配置
+ type='SmoothL1Loss', # 损失函数类型
+ reduction='sum', # 设置损失函数输出的聚合方法
+ loss_weight=3.3333333333333335), # 损失函数权重
+ semantic_loss=dict( # 语义损失函数的配置
+ type='CrossEntropyLoss', # 损失函数类型
+ reduction='sum', # 设置损失函数输出的聚合方法
+ loss_weight=1.0)), # 损失函数权重
+ train_cfg = dict( # VoteNet 训练的超参数配置
+ pos_distance_thr=0.3, # 距离 >= 0.3 阈值的样本将被视为正样本
+ neg_distance_thr=0.6, # 距离 < 0.6 阈值的样本将被视为负样本
+ sample_mod='vote'), # 采样方法的模式
+ test_cfg = dict( # VoteNet 测试的超参数配置
+ sample_mod='seed', # 采样方法的模式
+ nms_thr=0.25, # NMS 中使用的阈值
+ score_thr=0.8, # 剔除框的阈值
+ per_class_proposal=False)) # 是否使用逐类提议框 (proposal)
+dataset_type = 'ScanNetDataset' # 数据集类型
+data_root = './data/scannet/' # 数据路径
+class_names = ('cabinet', 'bed', 'chair', 'sofa', 'table', 'door', 'window',
+ 'bookshelf', 'picture', 'counter', 'desk', 'curtain',
+ 'refrigerator', 'showercurtrain', 'toilet', 'sink', 'bathtub',
+ 'garbagebin') # 类的名称
+train_pipeline = [ # 训练流水线,更多细节请参考 mmdet3d.datasets.pipelines
+ dict(
+ type='LoadPointsFromFile', # 第一个流程,用于读取点,更多细节请参考 mmdet3d.datasets.pipelines.indoor_loading
+ shift_height=True, # 是否使用变换高度
+ load_dim=6, # 读取的点的维度
+ use_dim=[0, 1, 2]), # 使用所读取点的哪些维度
+ dict(
+ type='LoadAnnotations3D', # 第二个流程,用于读取标注,更多细节请参考 mmdet3d.datasets.pipelines.indoor_loading
+ with_bbox_3d=True, # 是否读取 3D 框
+ with_label_3d=True, # 是否读取 3D 框对应的类别标签
+ with_mask_3d=True, # 是否读取 3D 实例分割掩码
+ with_seg_3d=True), # 是否读取 3D 语义分割掩码
+ dict(
+ type='PointSegClassMapping', # 选取有效的类别,更多细节请参考 mmdet3d.datasets.pipelines.point_seg_class_mapping
+ valid_cat_ids=(3, 4, 5, 6, 7, 8, 9, 10, 11, 12, 14, 16, 24, 28, 33, 34,
+ 36, 39), # 所有有效类别的编号
+ max_cat_id=40), # 输入语义分割掩码中可能存在的最大类别编号
+ dict(type='PointSample', # 室内点采样,更多细节请参考 mmdet3d.datasets.pipelines.indoor_sample
+ num_points=40000), # 采样的点的数量
+ dict(type='IndoorFlipData', # 数据增广流程,随机翻转点和 3D 框
+ flip_ratio_yz=0.5, # 沿着 yz 平面被翻转的概率
+ flip_ratio_xz=0.5), # 沿着 xz 平面被翻转的概率
+ dict(
+ type='IndoorGlobalRotScale', # 数据增广流程,旋转并放缩点和 3D 框,更多细节请参考 mmdet3d.datasets.pipelines.indoor_augment
+ shift_height=True, # 读取的点是否有高度这一属性
+ rot_range=[-0.027777777777777776, 0.027777777777777776], # 旋转角范围
+ scale_range=None), # 缩放尺寸范围
+ dict(
+ type='DefaultFormatBundle3D', # 默认格式打包以收集读取的所有数据,更多细节请参考 mmdet3d.datasets.pipelines.formatting
+ class_names=('cabinet', 'bed', 'chair', 'sofa', 'table', 'door',
+ 'window', 'bookshelf', 'picture', 'counter', 'desk',
+ 'curtain', 'refrigerator', 'showercurtrain', 'toilet',
+ 'sink', 'bathtub', 'garbagebin')),
+ dict(
+ type='Collect3D', # 最后一个流程,决定哪些键值对应的数据会被输入给检测器,更多细节请参考 mmdet3d.datasets.pipelines.formatting
+ keys=[
+ 'points', 'gt_bboxes_3d', 'gt_labels_3d', 'pts_semantic_mask',
+ 'pts_instance_mask'
+ ])
+]
+test_pipeline = [ # 测试流水线,更多细节请参考 mmdet3d.datasets.pipelines
+ dict(
+ type='LoadPointsFromFile', # 第一个流程,用于读取点,更多细节请参考 mmdet3d.datasets.pipelines.indoor_loading
+ shift_height=True, # 是否使用变换高度
+ load_dim=6, # 读取的点的维度
+ use_dim=[0, 1, 2]), # 使用所读取点的哪些维度
+ dict(type='PointSample', # 室内点采样,更多细节请参考 mmdet3d.datasets.pipelines.indoor_sample
+ num_points=40000), # 采样的点的数量
+ dict(
+ type='DefaultFormatBundle3D', # 默认格式打包以收集读取的所有数据,更多细节请参考 mmdet3d.datasets.pipelines.formatting
+ class_names=('cabinet', 'bed', 'chair', 'sofa', 'table', 'door',
+ 'window', 'bookshelf', 'picture', 'counter', 'desk',
+ 'curtain', 'refrigerator', 'showercurtrain', 'toilet',
+ 'sink', 'bathtub', 'garbagebin')),
+ dict(type='Collect3D', # 最后一个流程,决定哪些键值对应的数据会被输入给检测器,更多细节请参考 mmdet3d.datasets.pipelines.formatting
+ keys=['points'])
+]
+eval_pipeline = [ # 模型验证或可视化所使用的流水线,更多细节请参考 mmdet3d.datasets.pipelines
+ dict(
+ type='LoadPointsFromFile', # 第一个流程,用于读取点,更多细节请参考 mmdet3d.datasets.pipelines.indoor_loading
+ shift_height=True, # 是否使用变换高度
+ load_dim=6, # 读取的点的维度
+ use_dim=[0, 1, 2]), # 使用所读取点的哪些维度
+ dict(
+ type='DefaultFormatBundle3D', # 默认格式打包以收集读取的所有数据,更多细节请参考 mmdet3d.datasets.pipelines.formatting
+ class_names=('cabinet', 'bed', 'chair', 'sofa', 'table', 'door',
+ 'window', 'bookshelf', 'picture', 'counter', 'desk',
+ 'curtain', 'refrigerator', 'showercurtrain', 'toilet',
+ 'sink', 'bathtub', 'garbagebin')),
+ with_label=False),
+ dict(type='Collect3D', # 最后一个流程,决定哪些键值对应的数据会被输入给检测器,更多细节请参考 mmdet3d.datasets.pipelines.formatting
+ keys=['points'])
+]
+data = dict(
+ samples_per_gpu=8, # 单张 GPU 上的样本数
+ workers_per_gpu=4, # 每张 GPU 上用于读取数据的进程数
+ train=dict( # 训练数据集配置
+ type='RepeatDataset', # 数据集嵌套,更多细节请参考 https://github.com/open-mmlab/mmdetection/blob/master/mmdet/datasets/dataset_wrappers.py
+ times=5, # 重复次数
+ dataset=dict(
+ type='ScanNetDataset', # 数据集类型
+ data_root='./data/scannet/', # 数据路径
+ ann_file='./data/scannet/scannet_infos_train.pkl', # 数据标注文件的路径
+ pipeline=[ # 流水线,这里传入的就是上面创建的训练流水线变量
+ dict(
+ type='LoadPointsFromFile',
+ shift_height=True,
+ load_dim=6,
+ use_dim=[0, 1, 2]),
+ dict(
+ type='LoadAnnotations3D',
+ with_bbox_3d=True,
+ with_label_3d=True,
+ with_mask_3d=True,
+ with_seg_3d=True),
+ dict(
+ type='PointSegClassMapping',
+ valid_cat_ids=(3, 4, 5, 6, 7, 8, 9, 10, 11, 12, 14, 16, 24,
+ 28, 33, 34, 36, 39),
+ max_cat_id=40),
+ dict(type='PointSample', num_points=40000),
+ dict(
+ type='IndoorFlipData',
+ flip_ratio_yz=0.5,
+ flip_ratio_xz=0.5),
+ dict(
+ type='IndoorGlobalRotScale',
+ shift_height=True,
+ rot_range=[-0.027777777777777776, 0.027777777777777776],
+ scale_range=None),
+ dict(
+ type='DefaultFormatBundle3D',
+ class_names=('cabinet', 'bed', 'chair', 'sofa', 'table',
+ 'door', 'window', 'bookshelf', 'picture',
+ 'counter', 'desk', 'curtain', 'refrigerator',
+ 'showercurtrain', 'toilet', 'sink', 'bathtub',
+ 'garbagebin')),
+ dict(
+ type='Collect3D',
+ keys=[
+ 'points', 'gt_bboxes_3d', 'gt_labels_3d',
+ 'pts_semantic_mask', 'pts_instance_mask'
+ ])
+ ],
+ filter_empty_gt=False, # 是否过滤掉空的标签框
+ classes=('cabinet', 'bed', 'chair', 'sofa', 'table', 'door',
+ 'window', 'bookshelf', 'picture', 'counter', 'desk',
+ 'curtain', 'refrigerator', 'showercurtrain', 'toilet',
+ 'sink', 'bathtub', 'garbagebin'))), # 类别名称
+ val=dict( # 验证数据集配置
+ type='ScanNetDataset', # 数据集类型
+ data_root='./data/scannet/', # 数据路径
+ ann_file='./data/scannet/scannet_infos_val.pkl', # 数据标注文件的路径
+ pipeline=[ # 流水线,这里传入的就是上面创建的测试流水线变量
+ dict(
+ type='LoadPointsFromFile',
+ shift_height=True,
+ load_dim=6,
+ use_dim=[0, 1, 2]),
+ dict(type='PointSample', num_points=40000),
+ dict(
+ type='DefaultFormatBundle3D',
+ class_names=('cabinet', 'bed', 'chair', 'sofa', 'table',
+ 'door', 'window', 'bookshelf', 'picture',
+ 'counter', 'desk', 'curtain', 'refrigerator',
+ 'showercurtrain', 'toilet', 'sink', 'bathtub',
+ 'garbagebin')),
+ dict(type='Collect3D', keys=['points'])
+ ],
+ classes=('cabinet', 'bed', 'chair', 'sofa', 'table', 'door', 'window',
+ 'bookshelf', 'picture', 'counter', 'desk', 'curtain',
+ 'refrigerator', 'showercurtrain', 'toilet', 'sink', 'bathtub',
+ 'garbagebin'), # 类别名称
+ test_mode=True), # 是否开启测试模式
+ test=dict( # 测试数据集配置
+ type='ScanNetDataset', # 数据集类型
+ data_root='./data/scannet/', # 数据路径
+ ann_file='./data/scannet/scannet_infos_val.pkl', # 数据标注文件的路径
+ pipeline=[ # 流水线,这里传入的就是上面创建的测试流水线变量
+ dict(
+ type='LoadPointsFromFile',
+ shift_height=True,
+ load_dim=6,
+ use_dim=[0, 1, 2]),
+ dict(type='PointSample', num_points=40000),
+ dict(
+ type='DefaultFormatBundle3D',
+ class_names=('cabinet', 'bed', 'chair', 'sofa', 'table',
+ 'door', 'window', 'bookshelf', 'picture',
+ 'counter', 'desk', 'curtain', 'refrigerator',
+ 'showercurtrain', 'toilet', 'sink', 'bathtub',
+ 'garbagebin')),
+ dict(type='Collect3D', keys=['points'])
+ ],
+ classes=('cabinet', 'bed', 'chair', 'sofa', 'table', 'door', 'window',
+ 'bookshelf', 'picture', 'counter', 'desk', 'curtain',
+ 'refrigerator', 'showercurtrain', 'toilet', 'sink', 'bathtub',
+ 'garbagebin'), # 类别名称
+ test_mode=True)) # 是否开启测试模式
+evaluation = dict(pipeline=[ # 流水线,这里传入的就是上面创建的验证流水线变量
+ dict(
+ type='LoadPointsFromFile',
+ coord_type='DEPTH',
+ shift_height=False,
+ load_dim=6,
+ use_dim=[0, 1, 2]),
+ dict(
+ type='DefaultFormatBundle3D',
+ class_names=('cabinet', 'bed', 'chair', 'sofa', 'table', 'door',
+ 'window', 'bookshelf', 'picture', 'counter', 'desk',
+ 'curtain', 'refrigerator', 'showercurtrain', 'toilet',
+ 'sink', 'bathtub', 'garbagebin'),
+ with_label=False),
+ dict(type='Collect3D', keys=['points'])
+])
+lr = 0.008 # 优化器的学习率
+optimizer = dict( # 构建优化器所使用的配置,我们支持所有 PyTorch 中支持的优化器,并且拥有相同的参数名称
+ type='Adam', # 优化器类型,更多细节请参考 https://github.com/open-mmlab/mmcv/blob/v1.3.7/mmcv/runner/optimizer/default_constructor.py#L12
+ lr=0.008) # 优化器的学习率,用户可以在 PyTorch 文档中查看这些参数的详细使用方法
+optimizer_config = dict( # 构建优化器钩子的配置,更多实现细节可参考 https://github.com/open-mmlab/mmcv/blob/v1.3.7/mmcv/runner/hooks/optimizer.py#L22
+ grad_clip=dict( # 梯度裁剪的配置
+ max_norm=10, # 梯度的最大模长
+ norm_type=2)) # 所使用的 p-范数的类型,可以设置成 'inf' 则指代无穷范数
+lr_config = dict( # 学习率策略配置,用于注册学习率更新的钩子
+ policy='step', # 学习率调整的策略,支持 CosineAnnealing、Cyclic 等,更多支持的种类请参考 https://github.com/open-mmlab/mmcv/blob/v1.3.7/mmcv/runner/hooks/lr_updater.py#L9
+ warmup=None, # Warmup 策略,同时也支持 `exp` 和 `constant`
+ step=[24, 32]) # 学习率衰减的步数
+checkpoint_config = dict( # 设置保存模型权重钩子的配置,具体实现请参考 https://github.com/open-mmlab/mmcv/blob/master/mmcv/runner/hooks/checkpoint.py
+ interval=1) # 保存模型权重的间隔是 1 轮
+log_config = dict( # 用于注册输出记录信息钩子的配置
+ interval=50, # 输出记录信息的间隔
+ hooks=[dict(type='TextLoggerHook'),
+ dict(type='TensorboardLoggerHook')]) # 用于记录训练过程的信息记录机制
+runner = dict(type='EpochBasedRunner', max_epochs=36) # 程序运行器,将会运行 `workflow` `max_epochs` 次
+dist_params = dict(backend='nccl') # 设置分布式训练的配置,通讯端口值也可被设置
+log_level = 'INFO' # 输出记录信息的等级
+find_unused_parameters = True # 是否查找模型中未使用的参数
+work_dir = None # 当前实验存储模型权重和输出信息的路径
+load_from = None # 从指定路径读取一个预训练的模型权重,这将不会继续 (resume) 训练
+resume_from = None # 从一个指定路径读入模型权重并继续训练,这意味着训练轮数、优化器状态等都将被读取
+workflow = [('train', 1)] # 要运行的工作流。[('train', 1)] 意味着只有一个名为 'train' 的工作流,它只会被执行一次。这一工作流依据 `max_epochs` 的值将会训练模型 36 轮。
+gpu_ids = range(0, 1) # 所使用的 GPU 编号
+```
+
+## 常问问题 (FAQ)
+
+### 忽略基础配置文件里的部分内容
+
+有时,您也许会需要通过设置 `_delete_=True` 来忽略基础配置文件里的一些域内容。
+请参照 [mmcv](https://mmcv.readthedocs.io/en/latest/understand_mmcv/config.html#inherit-from-base-config-with-ignored-fields) 来获得一些简单的指导。
+
+例如在 MMDetection3D 中,为了改变如下所示 PointPillars FPN 模块的某些配置:
+
+```python
+model = dict(
+ type='MVXFasterRCNN',
+ pts_voxel_layer=dict(...),
+ pts_voxel_encoder=dict(...),
+ pts_middle_encoder=dict(...),
+ pts_backbone=dict(...),
+ pts_neck=dict(
+ type='FPN',
+ norm_cfg=dict(type='naiveSyncBN2d', eps=1e-3, momentum=0.01),
+ act_cfg=dict(type='ReLU'),
+ in_channels=[64, 128, 256],
+ out_channels=256,
+ start_level=0,
+ num_outs=3),
+ pts_bbox_head=dict(...))
+```
+
+`FPN` 和 `SECONDFPN` 使用不同的关键词来构建。
+
+```python
+_base_ = '../_base_/models/hv_pointpillars_fpn_nus.py'
+model = dict(
+ pts_neck=dict(
+ _delete_=True,
+ type='SECONDFPN',
+ norm_cfg=dict(type='naiveSyncBN2d', eps=1e-3, momentum=0.01),
+ in_channels=[64, 128, 256],
+ upsample_strides=[1, 2, 4],
+ out_channels=[128, 128, 128]),
+ pts_bbox_head=dict(...))
+```
+
+`_delete_=True` 的标识将会使用新的键值覆盖掉 `pts_neck` 中的所有旧键值。
+
+### 使用配置文件里的中间变量
+
+配置文件里会使用一些中间变量,例如数据集中的 `train_pipeline`/`test_pipeline`。
+值得注意的是,当修改子配置文件中的中间变量后,用户还需再次将其传入相应字段。
+例如,我们想在训练和测试中,对 PointPillars 使用多尺度策略 (multi scale strategy),那么 `train_pipeline`/`test_pipeline` 就是我们想要修改的中间变量。
+
+```python
+_base_ = './nus-3d.py'
+train_pipeline = [
+ dict(
+ type='LoadPointsFromFile',
+ load_dim=5,
+ use_dim=5,
+ file_client_args=file_client_args),
+ dict(
+ type='LoadPointsFromMultiSweeps',
+ sweeps_num=10,
+ file_client_args=file_client_args),
+ dict(type='LoadAnnotations3D', with_bbox_3d=True, with_label_3d=True),
+ dict(
+ type='GlobalRotScaleTrans',
+ rot_range=[-0.3925, 0.3925],
+ scale_ratio_range=[0.95, 1.05],
+ translation_std=[0, 0, 0]),
+ dict(type='RandomFlip3D', flip_ratio_bev_horizontal=0.5),
+ dict(type='PointsRangeFilter', point_cloud_range=point_cloud_range),
+ dict(type='ObjectRangeFilter', point_cloud_range=point_cloud_range),
+ dict(type='ObjectNameFilter', classes=class_names),
+ dict(type='PointShuffle'),
+ dict(type='DefaultFormatBundle3D', class_names=class_names),
+ dict(type='Collect3D', keys=['points', 'gt_bboxes_3d', 'gt_labels_3d'])
+]
+test_pipeline = [
+ dict(
+ type='LoadPointsFromFile',
+ load_dim=5,
+ use_dim=5,
+ file_client_args=file_client_args),
+ dict(
+ type='LoadPointsFromMultiSweeps',
+ sweeps_num=10,
+ file_client_args=file_client_args),
+ dict(
+ type='MultiScaleFlipAug3D',
+ img_scale=(1333, 800),
+ pts_scale_ratio=[0.95, 1.0, 1.05],
+ flip=False,
+ transforms=[
+ dict(
+ type='GlobalRotScaleTrans',
+ rot_range=[0, 0],
+ scale_ratio_range=[1., 1.],
+ translation_std=[0, 0, 0]),
+ dict(type='RandomFlip3D'),
+ dict(
+ type='PointsRangeFilter', point_cloud_range=point_cloud_range),
+ dict(
+ type='DefaultFormatBundle3D',
+ class_names=class_names,
+ with_label=False),
+ dict(type='Collect3D', keys=['points'])
+ ])
+]
+data = dict(
+ train=dict(pipeline=train_pipeline),
+ val=dict(pipeline=test_pipeline),
+ test=dict(pipeline=test_pipeline))
+```
+
+这里,我们首先定义了新的 `train_pipeline`/`test_pipeline`,然后将其传入 `data`。
diff --git a/docs/zh_cn/tutorials/coord_sys_tutorial.md b/docs/zh_cn/tutorials/coord_sys_tutorial.md
new file mode 100644
index 0000000..47e03d8
--- /dev/null
+++ b/docs/zh_cn/tutorials/coord_sys_tutorial.md
@@ -0,0 +1,240 @@
+# 教程 6: 坐标系
+
+## 概述
+
+MMDetection3D 使用 3 种不同的坐标系。3D 目标检测领域中不同坐标系的存在是非常有必要的,因为对于各种 3D 数据采集设备来说,如激光雷达、深度相机等,使用的坐标系是不一致的,不同的 3D 数据集也遵循不同的数据格式。早期的工作,比如 SECOND、VoteNet 将原始数据转换为另一种格式,形成了一些后续工作也遵循的约定,使得不同坐标系之间的转换变得更加复杂。
+
+尽管数据集和采集设备多种多样,但是通过总结 3D 目标检测的工作线,我们可以将坐标系大致分为三类:
+
+- 相机坐标系 -- 大多数相机的坐标系,在该坐标系中 y 轴正方向指向地面,x 轴正方向指向右侧,z 轴正方向指向前方。
+ ```
+ 上 z 前
+ | ^
+ | /
+ | /
+ | /
+ |/
+ 左 ------ 0 ------> x 右
+ |
+ |
+ |
+ |
+ v
+ y 下
+ ```
+- 激光雷达坐标系 -- 众多激光雷达的坐标系,在该坐标系中 z 轴负方向指向地面,x 轴正方向指向前方,y 轴正方向指向左侧。
+ ```
+ z 上 x 前
+ ^ ^
+ | /
+ | /
+ | /
+ |/
+ y 左 <------ 0 ------ 右
+ ```
+- 深度坐标系 -- VoteNet、H3DNet 等模型使用的坐标系,在该坐标系中 z 轴负方向指向地面,x 轴正方向指向右侧,y 轴正方向指向前方。
+ ```
+ z 上 y 前
+ ^ ^
+ | /
+ | /
+ | /
+ |/
+ 左 ------ 0 ------> x 右
+ ```
+
+该教程中的坐标系定义实际上**不仅仅是定义三个轴**。对于形如 `` $$`(x, y, z, dx, dy, dz, r)`$$ `` 的框来说,我们的坐标系也定义了如何解释框的尺寸 `` $$`(dx, dy, dz)`$$ `` 和转向角 (yaw) 角度 `` $$`r`$$ ``。
+
+三个坐标系的图示如下:
+
+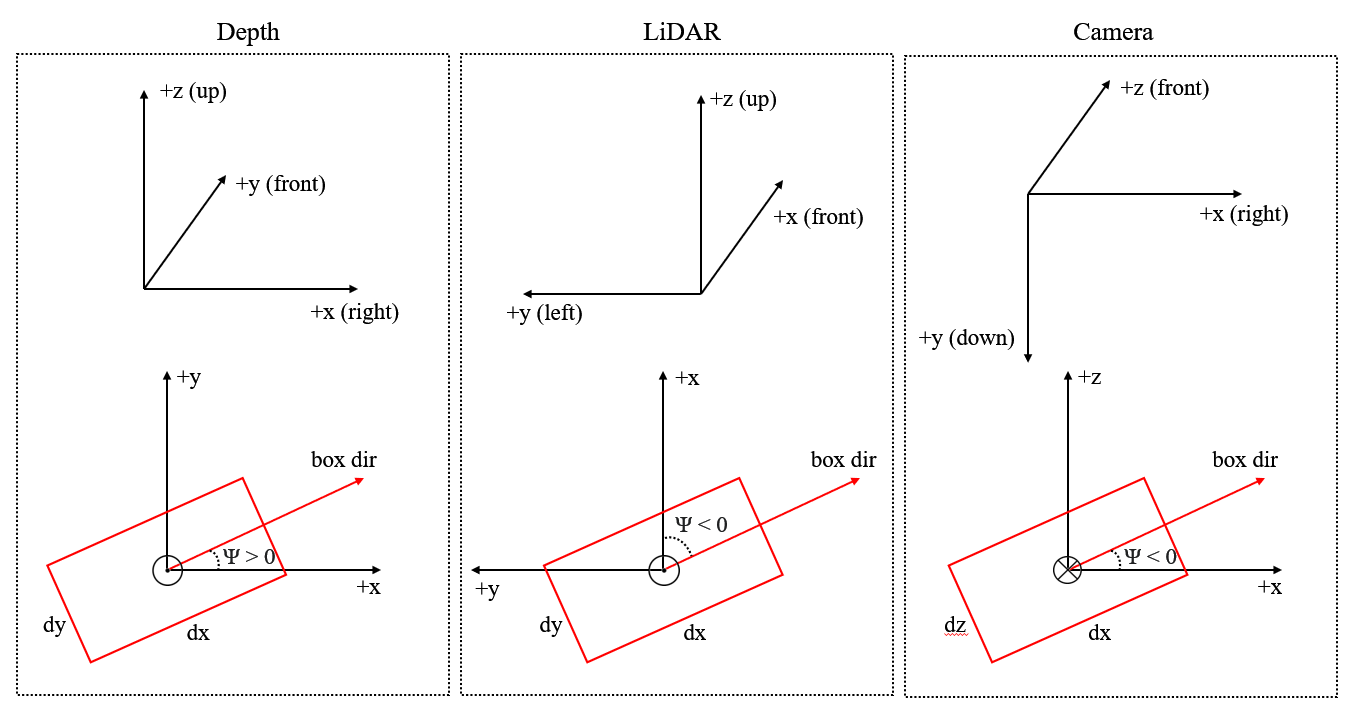
+
+上面三张图是 3D 坐标系,下面三张图是鸟瞰图。
+
+以后我们将坚持使用本教程中定义的三个坐标系。
+
+## 转向角 (yaw) 的定义
+
+请参考[维基百科](https://en.wikipedia.org/wiki/Euler_angles#Tait%E2%80%93Bryan_angles)了解转向角的标准定义。在目标检测中,我们选择一个轴作为重力轴,并在垂直于重力轴的平面 `` $$`\Pi`$$ `` 上选取一个参考方向,那么参考方向的转向角为 0,在 `` $$`\Pi`$$ `` 上的其他方向有非零的转向角,其角度取决于其与参考方向的角度。
+
+目前,对于所有支持的数据集,标注不包括俯仰角 (pitch) 和滚动角 (roll),这意味着我们在预测框和计算框之间的重叠时只需考虑转向角 (yaw)。
+
+在 MMDetection3D 中,所有坐标系都是右手坐标系,这意味着如果从重力轴的负方向(轴的正方向指向人眼)看,转向角 (yaw) 沿着逆时针方向增加。
+
+下图显示,在右手坐标系中,如果我们设定 x 轴正方向为参考方向,那么 y 轴正方向的转向角 (yaw) 为 `` $$`\frac{\pi}{2}`$$ ``。
+
+```
+ z 上 y 前 (yaw=0.5*pi)
+ ^ ^
+ | /
+ | /
+ | /
+ |/
+左 (yaw=pi) ------ 0 ------> x 右 (yaw=0)
+```
+
+对于一个框来说,其转向角 (yaw) 的值等于其方向减去一个参考方向。在 MMDetection3D 的所有三个坐标系中,参考方向总是 x 轴的正方向,而如果一个框的转向角 (yaw) 为 0,则其方向被定义为与 x 轴平行。框的转向角 (yaw) 的定义如下图所示。
+
+```
+ y 前
+ ^ 框的方向 (yaw=0.5*pi)
+ /|\ ^
+ | /|\
+ | ____|____
+ | | | |
+ | | | |
+__|____|____|____|______\ x 右
+ | | | | /
+ | | | |
+ | |____|____|
+ |
+```
+
+## 框尺寸的定义
+
+框尺寸的定义与转向角 (yaw) 的定义是分不开的。在上一节中,我们提到如果一个框的转向角 (yaw) 为 0,它的方向就被定义为与 x 轴平行。那么自然地,一个框对应于 x 轴的尺寸应该是 `` $$`dx`$$ ``。但是,这在某些数据集中并非总是如此(我们稍后会解决这个问题)。
+
+下图展示了 x 轴和 `` $$`dx`$$ ``,y 轴和 `` $$`dy`$$ `` 对应的含义。
+
+```
+y 前
+ ^ 框的方向 (yaw=0.5*pi)
+ /|\ ^
+ | /|\
+ | ____|____
+ | | | |
+ | | | | dx
+__|____|____|____|______\ x 右
+ | | | | /
+ | | | |
+ | |____|____|
+ | dy
+```
+
+注意框的方向总是和 `` $$`dx`$$ `` 边平行。
+
+```
+y 前
+ ^ _________
+ /|\ | | |
+ | | | |
+ | | | | dy
+ | |____|____|____\ 框的方向 (yaw=0)
+ | | | | /
+__|____|____|____|_________\ x 右
+ | | | | /
+ | |____|____|
+ | dx
+ |
+```
+
+## 与支持的数据集的原始坐标系的关系
+
+### KITTI
+
+KITTI 数据集的原始标注是在相机坐标系下的,详见 [get_label_anno](https://github.com/open-mmlab/mmdetection3d/blob/master/tools/data_converter/kitti_data_utils.py)。在 MMDetection3D 中,为了在 KITTI 数据集上训练基于激光雷达的模型,首先将数据从相机坐标系转换到激光雷达坐标,详见 [get_ann_info](https://github.com/open-mmlab/mmdetection3d/blob/master/mmdet3d/datasets/kitti_dataset.py)。对于训练基于视觉的模型,数据保持在相机坐标系不变。
+
+在 SECOND 中,框的激光雷达坐标系定义如下(鸟瞰图):
+
+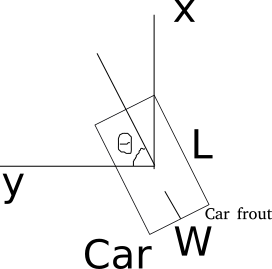
+
+对于每个框来说,尺寸为 `` $$`(w, l, h)`$$ ``,转向角 (yaw) 的参考方向为 y 轴正方向。更多细节请参考[代码库](https://github.com/traveller59/second.pytorch#concepts)。
+
+我们的激光雷达坐标系有两处改变:
+
+- 转向角 (yaw) 被定义为右手而非左手,从而保持一致性;
+- 框的尺寸为 `` $$`(l, w, h)`$$ `` 而非 `` $$`(w, l, h)`$$ ``,由于在 KITTI 数据集中 `` $$`w`$$ `` 对应 `` $$`dy`$$ ``,`` $$`l`$$ `` 对应 `` $$`dx`$$ ``。
+
+### Waymo
+
+我们使用 Waymo 数据集的 KITTI 格式数据。因此,在我们的实现中 KITTI 和 Waymo 也共用相同的坐标系。
+
+### NuScenes
+
+NuScenes 提供了一个评估工具包,其中每个框都被包装成一个 `Box` 实例。`Box` 的坐标系不同于我们的激光雷达坐标系,在 `Box` 坐标系中,前两个表示框尺寸的元素分别对应 `` $$`(dy, dx)`$$ `` 或者 `` $$`(w, l)`$$ ``,和我们的表示方法相反。更多细节请参考 NuScenes [教程](https://github.com/open-mmlab/mmdetection3d/blob/master/docs/zh_cn/datasets/nuscenes_det.md#notes)。
+
+读者可以参考 [NuScenes 开发工具](https://github.com/nutonomy/nuscenes-devkit/tree/master/python-sdk/nuscenes/eval/detection),了解 [NuScenes 框](https://github.com/nutonomy/nuscenes-devkit/blob/2c6a752319f23910d5f55cc995abc547a9e54142/python-sdk/nuscenes/utils/data_classes.py#L457) 的定义和 [NuScenes 评估](https://github.com/nutonomy/nuscenes-devkit/blob/master/python-sdk/nuscenes/eval/detection/evaluate.py)的过程。
+
+### Lyft
+
+就涉及坐标系而言,Lyft 和 NuScenes 共用相同的数据格式。
+
+请参考[官方网站](https://www.kaggle.com/c/3d-object-detection-for-autonomous-vehicles/data)获取更多信息。
+
+### ScanNet
+
+ScanNet 的原始数据不是点云而是网格,需要在我们的深度坐标系下进行采样得到点云数据。对于 ScanNet 检测任务,框的标注是轴对齐的,并且转向角 (yaw) 始终是 0。因此,我们的深度坐标系中转向角 (yaw) 的方向对 ScanNet 没有影响。
+
+### SUN RGB-D
+
+SUN RGB-D 的原始数据不是点云而是 RGB-D 图像。我们通过反投影,可以得到每张图像对应的点云,其在我们的深度坐标系下。但是,数据集的标注并不在我们的系统中,所以需要进行转换。
+
+将原始标注转换为我们的深度坐标系下的标注的转换过程请参考 [sunrgbd_data_utils.py](https://github.com/open-mmlab/mmdetection3d/blob/master/tools/data_converter/sunrgbd_data_utils.py)。
+
+### S3DIS
+
+在我们的实现中,S3DIS 与 ScanNet 共用相同的坐标系。然而 S3DIS 是一个仅限于分割任务的数据集,因此没有标注是坐标系敏感的。
+
+## 例子
+
+### 框(在不同坐标系间)的转换
+
+以相机坐标系和激光雷达坐标系间的转换为例:
+
+首先,对于点和框的中心点,坐标转换前后满足下列关系:
+
+- `` $$`x_{LiDAR}=z_{camera}`$$ ``
+- `` $$`y_{LiDAR}=-x_{camera}`$$ ``
+- `` $$`z_{LiDAR}=-y_{camera}`$$ ``
+
+然后,框的尺寸转换前后满足下列关系:
+
+- `` $$`dx_{LiDAR}=dx_{camera}`$$ ``
+- `` $$`dy_{LiDAR}=dz_{camera}`$$ ``
+- `` $$`dz_{LiDAR}=dy_{camera}`$$ ``
+
+最后,转向角 (yaw) 也应该被转换:
+
+- `` $$`r_{LiDAR}=-\frac{\pi}{2}-r_{camera}`$$ ``
+
+详见[此处](https://github.com/open-mmlab/mmdetection3d/blob/master/mmdet3d/core/bbox/structures/box_3d_mode.py)代码了解更多细节。
+
+### 鸟瞰图
+
+如果 3D 框是 `` $$`(x, y, z, dx, dy, dz, r)`$$ ``,相机坐标系下框的鸟瞰图是 `` $$`(x, z, dx, dz, -r)`$$ ``。转向角 (yaw) 符号取反是因为相机坐标系重力轴的正方向指向地面。
+
+详见[此处](https://github.com/open-mmlab/mmdetection3d/blob/master/mmdet3d/core/bbox/structures/cam_box3d.py)代码了解更多细节。
+
+### 框的旋转
+
+我们将各种框的旋转设定为绕着重力轴逆时针旋转。因此,为了旋转一个 3D 框,我们首先需要计算新的框的中心,然后将旋转角度添加到转向角 (yaw)。
+
+详见[此处](https://github.com/open-mmlab/mmdetection3d/blob/master/mmdet3d/core/bbox/structures/cam_box3d.py)代码了解更多细节。
+
+## 常见问题
+
+#### Q1: 与框相关的算子是否适用于所有坐标系类型?
+
+否。例如,[用于 RoI-Aware Pooling 的算子](https://github.com/open-mmlab/mmcv/blob/master/mmcv/ops/roiaware_pool3d.py)只适用于深度坐标系和激光雷达坐标系下的框。由于如果从上方看,旋转是顺时针的,所以 KITTI 数据集[这里](https://github.com/open-mmlab/mmdetection3d/blob/master/mmdet3d/core/evaluation/kitti_utils)的评估函数仅适用于相机坐标系下的框。
+
+对于每个和框相关的算子,我们注明了其所适用的框类型。
+
+#### Q2: 在每个坐标系中,三个轴是否分别准确地指向右侧、前方和地面?
+
+否。例如在 KITTI 中,从相机坐标系转换为激光雷达坐标系时,我们需要一个校准矩阵。
+
+#### Q3: 框中转向角 (yaw) `` $$`2\pi`$$ `` 的相位差如何影响评估?
+
+对于交并比 (IoU) 计算,转向角 (yaw) 有 `` $$`2\pi`$$ `` 的相位差的两个框是相同的,所以不会影响评估。
+
+对于角度预测评估,例如 NuScenes 中的 NDS 指标和 KITTI 中的 AOS 指标,会先对预测框的角度进行标准化,因此 `` $$`2\pi`$$ `` 的相位差不会改变结果。
+
+#### Q4: 框中转向角 (yaw) `` $$`\pi`$$ `` 的相位差如何影响评估?
+
+对于交并比 (IoU) 计算,转向角 (yaw) 有 `` $$`\pi`$$ `` 的相位差的两个框是相同的,所以不会影响评估。
+
+然而,对于角度预测评估,这会导致完全相反的方向。
+
+考虑一辆汽车,转向角 (yaw) 是汽车前部方向与 x 轴正方向之间的夹角。如果我们将该角度增加 `` $$`\pi`$$ ``,车前部将变成车后部。
+
+对于某些类别,例如障碍物,前后没有区别,因此 `` $$`\pi`$$ `` 的相位差不会对角度预测分数产生影响。
diff --git a/docs/zh_cn/tutorials/customize_dataset.md b/docs/zh_cn/tutorials/customize_dataset.md
new file mode 100644
index 0000000..e425f47
--- /dev/null
+++ b/docs/zh_cn/tutorials/customize_dataset.md
@@ -0,0 +1,358 @@
+# 教程 2: 自定义数据集
+
+## 支持新的数据格式
+
+为了支持新的数据格式,可以通过将新数据转换为现有的数据形式,或者直接将新数据转换为能够被模型直接调用的中间格式。此外,可以通过数据离线转换的方式(在调用脚本进行训练之前完成)或者通过数据在线转换的格式(调用新的数据集并在训练过程中进行数据转换)。在 MMDetection3D 中,对于那些不便于在线读取的数据,我们建议通过离线转换的方法将其转换为 KTIIT 数据集的格式,因此只需要在转换后修改配置文件中的数据标注文件的路径和标注数据所包含类别;对于那些与现有数据格式相似的新数据集,如 Lyft 数据集和 nuScenes 数据集,我们建议直接调用数据转换器和现有的数据集类别信息,在这个过程中,可以考虑通过继承的方式来减少实施数据转换的负担。
+
+### 将新数据的格式转换为现有数据的格式
+
+对于那些不便于在线读取的数据,最简单的方法是将新数据集的格式转换为现有数据集的格式。
+
+通常来说,我们需要一个数据转换器来重新组织原始数据的格式,并将对应的标注格式转换为 KITTI 数据集的风格;当现有数据集与新数据集存在差异时,可以通过定义一个从现有数据集类继承而来的新数据集类来处理具体的差异;最后,用户需要进一步修改配置文件来调用新的数据集。可以参考如何通过将 Waymo 数据集转换为 KITTI 数据集的风格并进一步训练模型的[例子](https://mmdetection3d.readthedocs.io/zh_CN/latest/2_new_data_model.html)。
+
+### 将新数据集的格式转换为一种当前可支持的中间格式
+
+如果不想采用将标注格式转为为现有格式的方式,也可以通过以下的方式来完成新数据集的转换。
+实际上,我们将所支持的所有数据集都转换成 pickle 文件的格式,这些文件整理了所有应用于模型训练和推理的有用的信息。
+
+数据集的标注信息是通过一个字典列表来描述的,每个字典包含对应数据帧的标注信息。
+下面展示了一个基础例子(应用在 KITTI 数据集上),每一帧包含了几项关键字,如 `image`、`point_cloud`、`calib` 和 `annos` 等。只要能够根据这些信息来直接读取到数据,其原始数据的组织方式就可以不同于现有的数据组织方式。通过这种设计,我们提供一种可替代的方案来自定义数据集。
+
+```python
+
+[
+ {'image': {'image_idx': 0, 'image_path': 'training/image_2/000000.png', 'image_shape': array([ 370, 1224], dtype=int32)},
+ 'point_cloud': {'num_features': 4, 'velodyne_path': 'training/velodyne/000000.bin'},
+ 'calib': {'P0': array([[707.0493, 0. , 604.0814, 0. ],
+ [ 0. , 707.0493, 180.5066, 0. ],
+ [ 0. , 0. , 1. , 0. ],
+ [ 0. , 0. , 0. , 1. ]]),
+ 'P1': array([[ 707.0493, 0. , 604.0814, -379.7842],
+ [ 0. , 707.0493, 180.5066, 0. ],
+ [ 0. , 0. , 1. , 0. ],
+ [ 0. , 0. , 0. , 1. ]]),
+ 'P2': array([[ 7.070493e+02, 0.000000e+00, 6.040814e+02, 4.575831e+01],
+ [ 0.000000e+00, 7.070493e+02, 1.805066e+02, -3.454157e-01],
+ [ 0.000000e+00, 0.000000e+00, 1.000000e+00, 4.981016e-03],
+ [ 0.000000e+00, 0.000000e+00, 0.000000e+00, 1.000000e+00]]),
+ 'P3': array([[ 7.070493e+02, 0.000000e+00, 6.040814e+02, -3.341081e+02],
+ [ 0.000000e+00, 7.070493e+02, 1.805066e+02, 2.330660e+00],
+ [ 0.000000e+00, 0.000000e+00, 1.000000e+00, 3.201153e-03],
+ [ 0.000000e+00, 0.000000e+00, 0.000000e+00, 1.000000e+00]]),
+ 'R0_rect': array([[ 0.9999128 , 0.01009263, -0.00851193, 0. ],
+ [-0.01012729, 0.9999406 , -0.00403767, 0. ],
+ [ 0.00847068, 0.00412352, 0.9999556 , 0. ],
+ [ 0. , 0. , 0. , 1. ]]),
+ 'Tr_velo_to_cam': array([[ 0.00692796, -0.9999722 , -0.00275783, -0.02457729],
+ [-0.00116298, 0.00274984, -0.9999955 , -0.06127237],
+ [ 0.9999753 , 0.00693114, -0.0011439 , -0.3321029 ],
+ [ 0. , 0. , 0. , 1. ]]),
+ 'Tr_imu_to_velo': array([[ 9.999976e-01, 7.553071e-04, -2.035826e-03, -8.086759e-01],
+ [-7.854027e-04, 9.998898e-01, -1.482298e-02, 3.195559e-01],
+ [ 2.024406e-03, 1.482454e-02, 9.998881e-01, -7.997231e-01],
+ [ 0.000000e+00, 0.000000e+00, 0.000000e+00, 1.000000e+00]])},
+ 'annos': {'name': array(['Pedestrian'], dtype=' 0
+ loss = torch.abs(pred - target)
+ return loss
+
+@LOSSES.register_module()
+class MyLoss(nn.Module):
+
+ def __init__(self, reduction='mean', loss_weight=1.0):
+ super(MyLoss, self).__init__()
+ self.reduction = reduction
+ self.loss_weight = loss_weight
+
+ def forward(self,
+ pred,
+ target,
+ weight=None,
+ avg_factor=None,
+ reduction_override=None):
+ assert reduction_override in (None, 'none', 'mean', 'sum')
+ reduction = (
+ reduction_override if reduction_override else self.reduction)
+ loss_bbox = self.loss_weight * my_loss(
+ pred, target, weight, reduction=reduction, avg_factor=avg_factor)
+ return loss_bbox
+```
+
+接着,用户需要将 loss 添加到 `mmdet3d/models/losses/__init__.py`:
+
+```python
+from .my_loss import MyLoss, my_loss
+
+```
+
+此外,用户也可以添加以下的代码到配置文件中,从而实现相同的目标。
+
+```python
+custom_imports=dict(
+ imports=['mmdet3d.models.losses.my_loss'])
+```
+
+为了使用该 loss,需要对 `loss_xxx` 域进行修改。
+因为 MyLoss 主要用于检测框的回归,因此需要在对应的 head 中修改 `loss_bbox` 域的值。
+
+```python
+loss_bbox=dict(type='MyLoss', loss_weight=1.0))
+```
diff --git a/docs/zh_cn/tutorials/customize_runtime.md b/docs/zh_cn/tutorials/customize_runtime.md
new file mode 100644
index 0000000..ac9dc98
--- /dev/null
+++ b/docs/zh_cn/tutorials/customize_runtime.md
@@ -0,0 +1,330 @@
+# 教程 5: 自定义运行时配置
+
+## 自定义优化器设置
+
+### 自定义 PyTorch 支持的优化器
+
+我们已经支持使用所有 PyTorch 实现的优化器,且唯一需要修改的地方就是改变配置文件中的 `optimizer` 字段。
+举个例子,如果您想使用 `ADAM` (注意到这样可能会使性能大幅下降),您可以这样修改:
+
+```python
+optimizer = dict(type='Adam', lr=0.0003, weight_decay=0.0001)
+```
+
+为了修改模型的学习率,用户只需要修改优化器配置中的 `lr` 字段。用户可以根据 PyTorch 的 [API 文档](https://pytorch.org/docs/stable/optim.html?highlight=optim#module-torch.optim) 直接设置参数。
+
+### 自定义并实现优化器
+
+#### 1. 定义新的优化器
+
+一个自定义优化器可以按照如下过程定义:
+
+假设您想要添加一个叫 `MyOptimizer` 的,拥有参数 `a`,`b` 和 `c` 的优化器,您需要创建一个叫做 `mmdet3d/core/optimizer` 的目录。
+接下来,应该在目录下某个文件中实现新的优化器,比如 `mmdet3d/core/optimizer/my_optimizer.py`:
+
+```python
+from mmcv.runner.optimizer import OPTIMIZERS
+from torch.optim import Optimizer
+
+
+@OPTIMIZERS.register_module()
+class MyOptimizer(Optimizer):
+
+ def __init__(self, a, b, c)
+
+```
+
+#### 2. 将优化器添加到注册器
+
+为了找到上述定义的优化器模块,该模块首先需要被引入主命名空间。有两种方法实现之:
+
+- 新建 `mmdet3d/core/optimizer/__init__.py` 文件用于引入。
+
+ 新定义的模块应该在 `mmdet3d/core/optimizer/__init__.py` 中被引入,使得注册器可以找到新模块并注册之:
+
+```python
+from .my_optimizer import MyOptimizer
+
+__all__ = ['MyOptimizer']
+
+```
+
+您也需要通过添加如下语句在 `mmdet3d/core/__init__.py` 中引入 `optimizer`:
+
+```python
+from .optimizer import *
+```
+
+或者在配置中使用 `custom_imports` 来人工引入新优化器:
+
+```python
+custom_imports = dict(imports=['mmdet3d.core.optimizer.my_optimizer'], allow_failed_imports=False)
+```
+
+模块 `mmdet3d.core.optimizer.my_optimizer` 会在程序伊始被引入,且 `MyOptimizer` 类在那时会自动被注册。
+注意到只有包含 `MyOptimizer` 类的包应该被引入。
+`mmdet3d.core.optimizer.my_optimizer.MyOptimizer` **不能** 被直接引入。
+
+事实上,用户可以在这种引入的方法中使用完全不同的文件目录结构,只要保证根目录能在 `PYTHONPATH` 中被定位。
+
+#### 3. 在配置文件中指定优化器
+
+接下来您可以在配置文件的 `optimizer` 字段中使用 `MyOptimizer`。
+在配置文件中,优化器在 `optimizer` 字段中以如下方式定义:
+
+```python
+optimizer = dict(type='SGD', lr=0.02, momentum=0.9, weight_decay=0.0001)
+```
+
+为了使用您自己的优化器,该字段可以改为:
+
+```python
+optimizer = dict(type='MyOptimizer', a=a_value, b=b_value, c=c_value)
+```
+
+### 自定义优化器的构造器
+
+部分模型可能会拥有一些参数专属的优化器设置,比如 BatchNorm 层的权重衰减 (weight decay)。
+用户可以通过自定义优化器的构造器来对那些细粒度的参数进行调优。
+
+```python
+from mmcv.utils import build_from_cfg
+
+from mmcv.runner.optimizer import OPTIMIZER_BUILDERS, OPTIMIZERS
+from mmdet.utils import get_root_logger
+from .my_optimizer import MyOptimizer
+
+
+@OPTIMIZER_BUILDERS.register_module()
+class MyOptimizerConstructor(object):
+
+ def __init__(self, optimizer_cfg, paramwise_cfg=None):
+
+ def __call__(self, model):
+
+ return my_optimizer
+
+```
+
+默认优化器构造器在[这里](https://github.com/open-mmlab/mmcv/blob/v1.3.7/mmcv/runner/optimizer/default_constructor.py#L11)实现。这部分代码也可以用作新优化器构造器的模版。
+
+### 额外的设置
+
+没有在优化器部分实现的技巧应该通过优化器构造器或者钩子来实现 (比如逐参数的学习率设置)。我们列举了一些常用的可以稳定训练过程或者加速训练的设置。我们欢迎提供更多类似设置的 PR 和 issue。
+
+- __使用梯度裁剪 (gradient clip) 来稳定训练过程__:
+
+ 一些模型依赖梯度裁剪技术来裁剪训练中的梯度,以稳定训练过程。举例如下:
+
+ ```python
+ optimizer_config = dict(
+ _delete_=True, grad_clip=dict(max_norm=35, norm_type=2))
+ ```
+
+ 如果您的配置继承了一个已经设置了 `optimizer_config` 的基础配置,那么您可能需要 `_delete_=True` 字段来覆盖基础配置中无用的设置。详见配置文件的[说明文档](https://mmdetection.readthedocs.io/zh_CN/latest/tutorials/config.html)。
+
+- __使用动量规划器 (momentum scheduler) 来加速模型收敛__:
+
+ 我们支持用动量规划器来根据学习率更改模型的动量,这样可以使模型更快地收敛。
+ 动量规划器通常和学习率规划器一起使用,比如说,如下配置文件在 3D 检测中被用于加速模型收敛。
+ 更多细节详见 [CyclicLrUpdater](https://github.com/open-mmlab/mmcv/blob/v1.3.7/mmcv/runner/hooks/lr_updater.py#L358) 和 [CyclicMomentumUpdater](https://github.com/open-mmlab/mmcv/blob/v1.3.7/mmcv/runner/hooks/momentum_updater.py#L225) 的实现。
+
+ ```python
+ lr_config = dict(
+ policy='cyclic',
+ target_ratio=(10, 1e-4),
+ cyclic_times=1,
+ step_ratio_up=0.4,
+ )
+ momentum_config = dict(
+ policy='cyclic',
+ target_ratio=(0.85 / 0.95, 1),
+ cyclic_times=1,
+ step_ratio_up=0.4,
+ )
+ ```
+
+## 自定义训练规程
+
+默认情况,我们使用阶梯式学习率衰减的 1 倍训练规程。这会调用 `MMCV` 中的 [`StepLRHook`](https://github.com/open-mmlab/mmcv/blob/v1.3.7/mmcv/runner/hooks/lr_updater.py#L167)。
+我们在[这里](https://github.com/open-mmlab/mmcv/blob/v1.3.7/mmcv/runner/hooks/lr_updater.py)支持很多其他学习率规划方案,比如`余弦退火`和`多项式衰减`规程。下面是一些样例:
+
+- 多项式衰减规程:
+
+ ```python
+ lr_config = dict(policy='poly', power=0.9, min_lr=1e-4, by_epoch=False)
+ ```
+
+- 余弦退火规程:
+
+ ```python
+ lr_config = dict(
+ policy='CosineAnnealing',
+ warmup='linear',
+ warmup_iters=1000,
+ warmup_ratio=1.0 / 10,
+ min_lr_ratio=1e-5)
+ ```
+
+## 自定义工作流
+
+工作流是一个(阶段,epoch 数)的列表,用于指定不同阶段运行顺序和运行的 epoch 数。
+默认情况它被设置为:
+
+```python
+workflow = [('train', 1)]
+```
+
+这意味着,工作流包括训练 1 个 epoch。
+有时候用户可能想要检查一些模型在验证集上的评估指标(比如损失、准确率)。
+在这种情况中,我们可以将工作流设置如下:
+
+```python
+[('train', 1), ('val', 1)]
+```
+
+这样,就是交替地运行 1 个 epoch 进行训练,1 个 epoch 进行验证。
+
+**请注意**:
+
+1. 模型参数在验证期间不会被更新。
+2. 配置文件中,`runner` 里的 `max_epochs` 字段只控制训练 epoch 的数量,而不会影响验证工作流。
+3. `[('train', 1), ('val', 1)]` 和 `[('train', 1)]` 工作流不会改变 `EvalHook` 的行为,这是因为 `EvalHook` 被 `after_train_epoch` 调用,且验证工作流只会影响通过 `after_val_epoch` 调用的钩子。因此,`[('train', 1), ('val', 1)]` 和 `[('train', 1)]` 的唯一区别就是执行器 (runner) 会在每个训练 epoch 之后在验证集上计算损失。
+
+## 自定义钩子
+
+### 自定义并实现钩子
+
+#### 1. 实现一个新钩子
+
+存在一些情况下用户可能需要实现新钩子。在版本 v2.3.0 之后,MMDetection 支持自定义训练过程中的钩子 (#3395)。因此用户可以直接在 mmdet 中,或者在其基于 mmdet 的代码库中实现钩子并通过更改训练配置来使用钩子。
+在 v2.3.0 之前,用户需要更改代码以使得训练开始之前钩子已经注册完毕。
+这里我们给出一个,在 mmdet3d 中创建并使用新钩子的例子。
+
+```python
+from mmcv.runner import HOOKS, Hook
+
+
+@HOOKS.register_module()
+class MyHook(Hook):
+
+ def __init__(self, a, b):
+ pass
+
+ def before_run(self, runner):
+ pass
+
+ def after_run(self, runner):
+ pass
+
+ def before_epoch(self, runner):
+ pass
+
+ def after_epoch(self, runner):
+ pass
+
+ def before_iter(self, runner):
+ pass
+
+ def after_iter(self, runner):
+ pass
+```
+
+取决于钩子的功能,用户需要指定钩子在每个训练阶段时的行为,具体包括如下阶段:`before_run`,`after_run`,`before_epoch`,`after_epoch`,`before_iter`,和 `after_iter`。
+
+#### 2. 注册新钩子
+
+接下来我们需要引入 `MyHook`。假设新钩子位于文件 `mmdet3d/core/utils/my_hook.py` 中,有两种方法可以实现之:
+
+- 更改 `mmdet3d/core/utils/__init__.py` 来引入之:
+
+ 新定义的模块应在 `mmdet3d/core/utils/__init__.py` 中引入,以使得注册器可以找到新模块并注册之:
+
+```python
+from .my_hook import MyHook
+
+__all__ = [..., 'MyHook']
+
+```
+
+或者在配置中使用 `custom_imports` 来人为地引入之
+
+```python
+custom_imports = dict(imports=['mmdet3d.core.utils.my_hook'], allow_failed_imports=False)
+```
+
+#### 3. 更改配置文件
+
+```python
+custom_hooks = [
+ dict(type='MyHook', a=a_value, b=b_value)
+]
+```
+
+您可以将字段 `priority` 设置为 `'NORMAL'` 或者 `'HIGHEST'`,来设置钩子的优先级,如下所示:
+
+```python
+custom_hooks = [
+ dict(type='MyHook', a=a_value, b=b_value, priority='NORMAL')
+]
+```
+
+默认情况,在注册阶段钩子的优先级被设置为 `NORMAL`。
+
+### 使用 MMCV 中实现的钩子
+
+如果钩子已经在 MMCV 中被实现了,您可以直接通过更改配置文件来使用该钩子:
+
+```python
+custom_hooks = [
+ dict(type='MyHook', a=a_value, b=b_value, priority='NORMAL')
+]
+```
+
+### 更改默认的运行时钩子
+
+有一些常用的钩子并没有通过 `custom_hooks` 注册,它们是:
+
+- 日志配置 (log_config)
+- 检查点配置 (checkpoint_config)
+- 评估 (evaluation)
+- 学习率配置 (lr_config)
+- 优化器配置 (optimizer_config)
+- 动量配置 (momentum_config)
+
+在这些钩子中,只有日志钩子拥有 `VERY_LOW` 的优先级,其他钩子的优先级均为 `NORMAL`。
+上述教程已经涉及了如何更改 `optimizer_config`,`momentum_config`,和 `lr_config`。
+下面我们展示如何在 `log_config`,`checkpoint_config`,和 `evaluation` 上做文章。
+
+#### 检查点配置
+
+MMCV 执行器会使用 `checkpoint_config` 来初始化 [`CheckpointHook`](https://github.com/open-mmlab/mmcv/blob/v1.3.7/mmcv/runner/hooks/checkpoint.py#L9)。
+
+```python
+checkpoint_config = dict(interval=1)
+```
+
+用户可以设置 `max_keep_ckpts` 来保存一定少量的检查点,或者用 `save_optimizer` 来决定是否保存优化器的状态。更多参数的细节详见[这里](https://mmcv.readthedocs.io/en/latest/api.html#mmcv.runner.CheckpointHook)。
+
+#### 日志配置
+
+`log_config` 将多个日志钩子封装在一起,并允许设置日志记录间隔。现在 MMCV 支持 `WandbLoggerHook`,`MlflowLoggerHook`,和 `TensorboardLoggerHook`。
+更详细的使用方法请移步 [MMCV 文档](https://mmcv.readthedocs.io/en/latest/api.html#mmcv.runner.LoggerHook)。
+
+```python
+log_config = dict(
+ interval=50,
+ hooks=[
+ dict(type='TextLoggerHook'),
+ dict(type='TensorboardLoggerHook')
+ ])
+```
+
+#### 评估配置
+
+`evaluation` 的配置会被用于初始化 [`EvalHook`](https://github.com/open-mmlab/mmdetection/blob/v2.13.0/mmdet/core/evaluation/eval_hooks.py#L9)。
+除了 `interval` 字段,其他参数,比如 `metric`,会被传递给 `dataset.evaluate()`。
+
+```python
+evaluation = dict(interval=1, metric='bbox')
+```
diff --git a/docs/zh_cn/tutorials/data_pipeline.md b/docs/zh_cn/tutorials/data_pipeline.md
new file mode 100644
index 0000000..a176717
--- /dev/null
+++ b/docs/zh_cn/tutorials/data_pipeline.md
@@ -0,0 +1,190 @@
+# 教程 3: 自定义数据预处理流程
+
+## 数据预处理流程的设计
+
+遵循一般惯例,我们使用 `Dataset` 和 `DataLoader` 来调用多个进程进行数据的加载。`Dataset` 将会返回与模型前向传播的参数所对应的数据项构成的字典。因为目标检测中的数据的尺寸可能无法保持一致(如点云中点的数量、真实标注框的尺寸等),我们在 MMCV 中引入一个 `DataContainer` 类型,来帮助收集和分发不同尺寸的数据。请参考[此处](https://github.com/open-mmlab/mmcv/blob/master/mmcv/parallel/data_container.py)获取更多细节。
+
+数据预处理流程和数据集之间是互相分离的两个部分,通常数据集定义了如何处理标注信息,而数据预处理流程定义了准备数据项字典的所有步骤。数据集预处理流程包含一系列的操作,每个操作将一个字典作为输入,并输出应用于下一个转换的一个新的字典。
+
+我们将在下图中展示一个最经典的数据集预处理流程,其中蓝色框表示预处理流程中的各项操作。随着预处理的进行,每一个操作都会添加新的键值(图中标记为绿色)到输出字典中,或者更新当前存在的键值(图中标记为橙色)。
+
+
+预处理流程中的各项操作主要分为数据加载、预处理、格式化、测试时的数据增强。
+
+接下来将展示一个用于 PointPillars 模型的数据集预处理流程的例子。
+
+```python
+train_pipeline = [
+ dict(
+ type='LoadPointsFromFile',
+ load_dim=5,
+ use_dim=5,
+ file_client_args=file_client_args),
+ dict(
+ type='LoadPointsFromMultiSweeps',
+ sweeps_num=10,
+ file_client_args=file_client_args),
+ dict(type='LoadAnnotations3D', with_bbox_3d=True, with_label_3d=True),
+ dict(
+ type='GlobalRotScaleTrans',
+ rot_range=[-0.3925, 0.3925],
+ scale_ratio_range=[0.95, 1.05],
+ translation_std=[0, 0, 0]),
+ dict(type='RandomFlip3D', flip_ratio_bev_horizontal=0.5),
+ dict(type='PointsRangeFilter', point_cloud_range=point_cloud_range),
+ dict(type='ObjectRangeFilter', point_cloud_range=point_cloud_range),
+ dict(type='ObjectNameFilter', classes=class_names),
+ dict(type='PointShuffle'),
+ dict(type='DefaultFormatBundle3D', class_names=class_names),
+ dict(type='Collect3D', keys=['points', 'gt_bboxes_3d', 'gt_labels_3d'])
+]
+test_pipeline = [
+ dict(
+ type='LoadPointsFromFile',
+ load_dim=5,
+ use_dim=5,
+ file_client_args=file_client_args),
+ dict(
+ type='LoadPointsFromMultiSweeps',
+ sweeps_num=10,
+ file_client_args=file_client_args),
+ dict(
+ type='MultiScaleFlipAug',
+ img_scale=(1333, 800),
+ pts_scale_ratio=1.0,
+ flip=False,
+ pcd_horizontal_flip=False,
+ pcd_vertical_flip=False,
+ transforms=[
+ dict(
+ type='GlobalRotScaleTrans',
+ rot_range=[0, 0],
+ scale_ratio_range=[1., 1.],
+ translation_std=[0, 0, 0]),
+ dict(type='RandomFlip3D'),
+ dict(
+ type='PointsRangeFilter', point_cloud_range=point_cloud_range),
+ dict(
+ type='DefaultFormatBundle3D',
+ class_names=class_names,
+ with_label=False),
+ dict(type='Collect3D', keys=['points'])
+ ])
+]
+```
+
+对于每项操作,我们将列出相关的被添加/更新/移除的字典项。
+
+### 数据加载
+
+`LoadPointsFromFile`
+
+- 添加:points
+
+`LoadPointsFromMultiSweeps`
+
+- 更新:points
+
+`LoadAnnotations3D`
+
+- 添加:gt_bboxes_3d, gt_labels_3d, gt_bboxes, gt_labels, pts_instance_mask, pts_semantic_mask, bbox3d_fields, pts_mask_fields, pts_seg_fields
+
+### 预处理
+
+`GlobalRotScaleTrans`
+
+- 添加:pcd_trans, pcd_rotation, pcd_scale_factor
+- 更新:points, \*bbox3d_fields
+
+`RandomFlip3D`
+
+- 添加:flip, pcd_horizontal_flip, pcd_vertical_flip
+- 更新:points, \*bbox3d_fields
+
+`PointsRangeFilter`
+
+- 更新:points
+
+`ObjectRangeFilter`
+
+- 更新:gt_bboxes_3d, gt_labels_3d
+
+`ObjectNameFilter`
+
+- 更新:gt_bboxes_3d, gt_labels_3d
+
+`PointShuffle`
+
+- 更新:points
+
+`PointsRangeFilter`
+
+- 更新:points
+
+### 格式化
+
+`DefaultFormatBundle3D`
+
+- 更新:points, gt_bboxes_3d, gt_labels_3d, gt_bboxes, gt_labels
+
+`Collect3D`
+
+- 添加:img_meta (由 `meta_keys` 指定的键值构成的 img_meta)
+- 移除:所有除 `keys` 指定的键值以外的其他键值
+
+### 测试时的数据增强
+
+`MultiScaleFlipAug`
+
+- 更新: scale, pcd_scale_factor, flip, flip_direction, pcd_horizontal_flip, pcd_vertical_flip (与这些指定的参数对应的增强后的数据列表)
+
+## 扩展并使用自定义数据集预处理方法
+
+1. 在任意文件中写入新的数据集预处理方法,如 `my_pipeline.py`,该预处理方法的输入和输出均为字典
+
+ ```python
+ from mmdet.datasets import PIPELINES
+
+ @PIPELINES.register_module()
+ class MyTransform:
+
+ def __call__(self, results):
+ results['dummy'] = True
+ return results
+ ```
+
+2. 导入新的预处理方法类
+
+ ```python
+ from .my_pipeline import MyTransform
+ ```
+
+3. 在配置文件中使用该数据集预处理方法
+
+ ```python
+ train_pipeline = [
+ dict(
+ type='LoadPointsFromFile',
+ load_dim=5,
+ use_dim=5,
+ file_client_args=file_client_args),
+ dict(
+ type='LoadPointsFromMultiSweeps',
+ sweeps_num=10,
+ file_client_args=file_client_args),
+ dict(type='LoadAnnotations3D', with_bbox_3d=True, with_label_3d=True),
+ dict(
+ type='GlobalRotScaleTrans',
+ rot_range=[-0.3925, 0.3925],
+ scale_ratio_range=[0.95, 1.05],
+ translation_std=[0, 0, 0]),
+ dict(type='RandomFlip3D', flip_ratio_bev_horizontal=0.5),
+ dict(type='PointsRangeFilter', point_cloud_range=point_cloud_range),
+ dict(type='ObjectRangeFilter', point_cloud_range=point_cloud_range),
+ dict(type='ObjectNameFilter', classes=class_names),
+ dict(type='MyTransform'),
+ dict(type='PointShuffle'),
+ dict(type='DefaultFormatBundle3D', class_names=class_names),
+ dict(type='Collect3D', keys=['points', 'gt_bboxes_3d', 'gt_labels_3d'])
+ ]
+ ```
diff --git a/docs/zh_cn/tutorials/index.rst b/docs/zh_cn/tutorials/index.rst
new file mode 100644
index 0000000..7e3f928
--- /dev/null
+++ b/docs/zh_cn/tutorials/index.rst
@@ -0,0 +1,10 @@
+.. toctree::
+ :maxdepth: 2
+
+ config.md
+ customize_dataset.md
+ data_pipeline.md
+ customize_models.md
+ customize_runtime.md
+ coord_sys_tutorial.md
+ backends_support.md
diff --git a/docs/zh_cn/tutorials/model_deployment.md b/docs/zh_cn/tutorials/model_deployment.md
new file mode 100644
index 0000000..47fdab7
--- /dev/null
+++ b/docs/zh_cn/tutorials/model_deployment.md
@@ -0,0 +1,121 @@
+# 教程 8: MMDet3D 模型部署
+
+为了满足在实际使用过程中遇到的算法模型的速度需求,通常我们会将训练好的模型部署到各种推理后端上。 [MMDeploy](https://github.com/open-mmlab/mmdeploy) 是 OpenMMLab 系列算法库的部署框架,现在 MMDeploy 已经支持了 MMDetection3D,我们可以通过 MMDeploy 将训练好的模型部署到各种推理后端上。
+
+## 准备
+
+### 安装 MMDeploy
+
+```bash
+git clone -b master git@github.com:open-mmlab/mmdeploy.git
+cd mmdeploy
+git submodule update --init --recursive
+```
+
+### 安装推理后端编译自定义算子
+
+根据 MMDeploy 的文档选择安装推理后端并编译自定义算子,目前 MMDet3D 模型支持了的推理后端有 [OnnxRuntime](https://mmdeploy.readthedocs.io/en/latest/backends/onnxruntime.html),[TensorRT](https://mmdeploy.readthedocs.io/en/latest/backends/tensorrt.html),[OpenVINO](https://mmdeploy.readthedocs.io/en/latest/backends/openvino.html)。
+
+## 模型导出
+
+将 MMDet3D 训练好的 Pytorch 模型转换成 ONNX 模型文件和推理后端所需要的模型文件。你可以参考 MMDeploy 的文档 [how_to_convert_model.md](https://github.com/open-mmlab/mmdeploy/blob/master/docs/zh_cn/tutorials/how_to_convert_model.md)。
+
+```bash
+python ./tools/deploy.py \
+ ${DEPLOY_CFG_PATH} \
+ ${MODEL_CFG_PATH} \
+ ${MODEL_CHECKPOINT_PATH} \
+ ${INPUT_IMG} \
+ --test-img ${TEST_IMG} \
+ --work-dir ${WORK_DIR} \
+ --calib-dataset-cfg ${CALIB_DATA_CFG} \
+ --device ${DEVICE} \
+ --log-level INFO \
+ --show \
+ --dump-info
+```
+
+### 参数描述
+
+- `deploy_cfg` : MMDeploy 代码库中用于部署的配置文件路径。
+- `model_cfg` : OpenMMLab 系列代码库中使用的模型配置文件路径。
+- `checkpoint` : OpenMMLab 系列代码库的模型文件路径。
+- `img` : 用于模型转换时使用的点云文件或图像文件路径。
+- `--test-img` : 用于测试模型的图像文件路径。如果没有指定,将设置成 `None`。
+- `--work-dir` : 工作目录,用来保存日志和模型文件。
+- `--calib-dataset-cfg` : 此参数只在 int8 模式下生效,用于校准数据集配置文件。如果没有指定,将被设置成 `None`,并使用模型配置文件中的 'val' 数据集进行校准。
+- `--device` : 用于模型转换的设备。如果没有指定,将被设置成 cpu。
+- `--log-level` : 设置日记的等级,选项包括 `'CRITICAL','FATAL','ERROR','WARN','WARNING','INFO','DEBUG','NOTSET'`。如果没有指定,将被设置成 INFO。
+- `--show` : 是否显示检测的结果。
+- `--dump-info` : 是否输出 SDK 信息。
+
+### 示例
+
+```bash
+cd mmdeploy
+python tools/deploy.py \
+ configs/mmdet3d/voxel-detection/voxel-detection_tensorrt_dynamic-kitti.py \
+ ${$MMDET3D_DIR}/configs/pointpillars/hv_pointpillars_secfpn_6x8_160e_kitti-3d-3class.py \
+ ${$MMDET3D_DIR}/checkpoints/hv_pointpillars_secfpn_6x8_160e_kitti-3d-3class_20200620_230421-aa0f3adb.pth \
+ ${$MMDET3D_DIR}/demo/data/kitti/kitti_000008.bin \
+ --work-dir work-dir \
+ --device cuda:0 \
+ --show
+```
+
+## 模型推理
+
+现在你可以使用推理后端提供的 API 进行模型推理。但是,如果你想立即测试模型怎么办?我们为您准备了一些推理后端的封装。
+
+```python
+from mmdeploy.apis import inference_model
+
+result = inference_model(model_cfg, deploy_cfg, backend_files, img=img, device=device)
+```
+
+`inference_model` 将创建一个推理后端的模块并为你进行推理。推理结果与模型的 OpenMMLab 代码库具有相同的格式。
+
+## 测试模型(可选)
+
+可以测试部署在推理后端上的模型的精度和速度。你可以参考 [how to measure performance of models](https://mmdeploy.readthedocs.io/en/latest/tutorials/how_to_measure_performance_of_models.html)。
+
+```bash
+python tools/test.py \
+ ${DEPLOY_CFG} \
+ ${MODEL_CFG} \
+ --model ${BACKEND_MODEL_FILES} \
+ [--out ${OUTPUT_PKL_FILE}] \
+ [--format-only] \
+ [--metrics ${METRICS}] \
+ [--show] \
+ [--show-dir ${OUTPUT_IMAGE_DIR}] \
+ [--show-score-thr ${SHOW_SCORE_THR}] \
+ --device ${DEVICE} \
+ [--cfg-options ${CFG_OPTIONS}] \
+ [--metric-options ${METRIC_OPTIONS}] \
+ [--log2file work_dirs/output.txt]
+```
+
+### 示例
+
+```bash
+cd mmdeploy
+python tools/test.py \
+ configs/mmdet3d/voxel-detection/voxel-detection_onnxruntime_dynamic.py \
+ ${MMDET3D_DIR}/configs/centerpoint/centerpoint_02pillar_second_secfpn_circlenms_4x8_cyclic_20e_nus.py \
+ --model work-dir/end2end.onnx \
+ --metrics bbox \
+ --device cpu
+```
+
+## 支持模型列表
+
+| Model | TorchScript | OnnxRuntime | TensorRT | NCNN | PPLNN | OpenVINO | Model config |
+| -------------------- | :---------: | :---------: | :------: | :--: | :---: | :------: | -------------------------------------------------------------------------------------- |
+| PointPillars | ? | Y | Y | N | N | Y | [config](https://github.com/open-mmlab/mmdetection3d/blob/master/configs/pointpillars) |
+| CenterPoint (pillar) | ? | Y | Y | N | N | Y | [config](https://github.com/open-mmlab/mmdetection3d/blob/master/configs/centerpoint) |
+
+## 注意
+
+- MMDeploy 的版本需要 >= 0.4.0。
+- 目前 CenterPoint 仅支持了 pillar 版本的。
diff --git a/docs/zh_cn/useful_tools.md b/docs/zh_cn/useful_tools.md
new file mode 100644
index 0000000..8acb5e7
--- /dev/null
+++ b/docs/zh_cn/useful_tools.md
@@ -0,0 +1,286 @@
+我们在 `tools/` 文件夹路径下提供了许多有用的工具。
+
+# 日志分析
+
+给定一个训练的日志文件,您可以绘制出 loss/mAP 曲线。首先需要运行 `pip install seaborn` 安装依赖包。
+
+
+
+```shell
+python tools/analysis_tools/analyze_logs.py plot_curve [--keys ${KEYS}] [--title ${TITLE}] [--legend ${LEGEND}] [--backend ${BACKEND}] [--style ${STYLE}] [--out ${OUT_FILE}] [--mode ${MODE}] [--interval ${INTERVAL}]
+```
+
+**注意**: 如果您想绘制的指标是在验证阶段计算得到的,您需要添加一个标志 `--mode eval` ,如果您每经过一个 `${INTERVAL}` 的间隔进行评估,您需要增加一个参数 `--interval ${INTERVAL}`。
+
+示例:
+
+- 绘制出某次运行的分类 loss。
+
+ ```shell
+ python tools/analysis_tools/analyze_logs.py plot_curve log.json --keys loss_cls --legend loss_cls
+ ```
+
+- 绘制出某次运行的分类和回归 loss,并且保存图片为 pdf 格式。
+
+ ```shell
+ python tools/analysis_tools/analyze_logs.py plot_curve log.json --keys loss_cls loss_bbox --out losses.pdf
+ ```
+
+- 在同一张图片中比较两次运行的 bbox mAP。
+
+ ```shell
+ # 根据 Car_3D_moderate_strict 在 KITTI 上评估 PartA2 和 second。
+ python tools/analysis_tools/analyze_logs.py plot_curve tools/logs/PartA2.log.json tools/logs/second.log.json --keys KITTI/Car_3D_moderate_strict --legend PartA2 second --mode eval --interval 1
+ # 根据 Car_3D_moderate_strict 在 KITTI 上分别对车和 3 类评估 PointPillars。
+ python tools/analysis_tools/analyze_logs.py plot_curve tools/logs/pp-3class.log.json tools/logs/pp.log.json --keys KITTI/Car_3D_moderate_strict --legend pp-3class pp --mode eval --interval 2
+ ```
+
+您也能计算平均训练速度。
+
+```shell
+python tools/analysis_tools/analyze_logs.py cal_train_time log.json [--include-outliers]
+```
+
+预期输出应该如下所示。
+
+```
+-----Analyze train time of work_dirs/some_exp/20190611_192040.log.json-----
+slowest epoch 11, average time is 1.2024
+fastest epoch 1, average time is 1.1909
+time std over epochs is 0.0028
+average iter time: 1.1959 s/iter
+```
+
+
+
+# 可视化
+
+## 结果
+
+为了观察模型的预测结果,您可以运行下面的指令
+
+```bash
+python tools/test.py ${CONFIG_FILE} ${CKPT_PATH} --show --show-dir ${SHOW_DIR}
+```
+
+在运行这个指令后,所有的绘制结果包括输入数据,以及在输入数据基础上可视化的网络输出和真值(例如: 3D 单模态检测任务中的 `***_points.obj` 和 `***_pred.obj`),将会被保存在 `${SHOW_DIR}`。
+
+要在评估期间看见预测结果,您可以运行下面的指令
+
+```bash
+python tools/test.py ${CONFIG_FILE} ${CKPT_PATH} --eval 'mAP' --eval-options 'show=True' 'out_dir=${SHOW_DIR}'
+```
+
+在运行这个指令后,您将会在 `${SHOW_DIR}` 获得输入数据、可视化在输入上的网络输出和真值标签(例如:在多模态检测任务中的`***_points.obj`,`***_pred.obj`,`***_gt.obj`,`***_img.png` 和 `***_pred.png` )。当 `show` 被激活,[Open3D](http://www.open3d.org/) 将会被用来在线可视化结果。当您在没有 GUI 的远程服务器上运行测试的时候,无法进行在线可视化,您可以设定 `show=False` 将输出结果保存在 `{SHOW_DIR}`。
+
+至于离线可视化,您将有两个选择。
+利用 `Open3D` 后端可视化结果,您可以运行下面的指令
+
+```bash
+python tools/misc/visualize_results.py ${CONFIG_FILE} --result ${RESULTS_PATH} --show-dir ${SHOW_DIR}
+```
+
+
+
+或者您可以使用 3D 可视化软件,例如 [MeshLab](http://www.meshlab.net/) 来打开这些在 `${SHOW_DIR}` 目录下的文件,从而查看 3D 检测输出。具体来说,打开 `***_points.obj` 查看输入点云,打开 `***_pred.obj` 查看预测的 3D 边界框。这允许推理和结果生成在远程服务器中完成,用户可以使用 GUI 在他们的主机上打开它们。
+
+**注意**:可视化接口有一些不稳定,我们将计划和 MMDetection 一起重构这一部分。
+
+## 数据集
+
+我们也提供脚本用来可视化数据集,而无需推理。您可以使用 `tools/misc/browse_dataset.py` 来在线显示载入的数据和真值标签,并且保存进磁盘。现在我们支持所有数据集上的单模态 3D 检测和 3D 分割,支持 KITTI 和 SUN RGB-D 数据集上的多模态 3D 检测,同时支持 nuScenes 数据集上的单目 3D 检测。为了浏览 KITTI 数据集,您可以运行下面的指令
+
+```shell
+python tools/misc/browse_dataset.py configs/_base_/datasets/kitti-3d-3class.py --task det --output-dir ${OUTPUT_DIR} --online
+```
+
+**注意**:一旦指定 `--output-dir` ,当按下 open3d 窗口的 `_ESC_`,用户指定的视图图像将被保存。如果您没有显示器,您可以移除 `--online` 标志,从而仅仅保存可视化结果并且进行离线浏览。
+
+为了验证数据的一致性和数据增强的效果,您还可以使用以下命令添加 `--aug` 标志来可视化数据增强后的数据:
+
+```shell
+python tools/misc/browse_dataset.py configs/_base_/datasets/kitti-3d-3class.py --task det --aug --output-dir ${OUTPUT_DIR} --online
+```
+
+如果您还想显示 2D 图像以及投影的 3D 边界框,则需要找到支持多模态数据加载的配置文件,然后将 `--task` 参数更改为 `multi_modality-det`。一个例子如下所示
+
+```shell
+python tools/misc/browse_dataset.py configs/mvxnet/dv_mvx-fpn_second_secfpn_adamw_2x8_80e_kitti-3d-3class.py --task multi_modality-det --output-dir ${OUTPUT_DIR} --online
+```
+
+
+
+您可以简单的使用不同的配置文件,浏览不同的数据集,例如:在 3D 语义分割任务中可视化 ScanNet 数据集
+
+```shell
+python tools/misc/browse_dataset.py configs/_base_/datasets/scannet_seg-3d-20class.py --task seg --output-dir ${OUTPUT_DIR} --online
+```
+
+
+
+在单目 3D 检测任务中浏览 nuScenes 数据集
+
+```shell
+python tools/misc/browse_dataset.py configs/_base_/datasets/nus-mono3d.py --task mono-det --output-dir ${OUTPUT_DIR} --online
+```
+
+
+
+
+
+# 模型部署
+
+**Note**: 此工具仍然处于试验阶段,目前只有 SECOND 支持用 [`TorchServe`](https://pytorch.org/serve/) 部署,我们将会在未来支持更多的模型。
+
+为了使用 [`TorchServe`](https://pytorch.org/serve/) 部署 `MMDetection3D` 模型,您可以遵循以下步骤:
+
+## 1. 将模型从 MMDetection3D 转换到 TorchServe
+
+```shell
+python tools/deployment/mmdet3d2torchserve.py ${CONFIG_FILE} ${CHECKPOINT_FILE} \
+--output-folder ${MODEL_STORE} \
+--model-name ${MODEL_NAME}
+```
+
+**Note**: ${MODEL_STORE} 需要为文件夹的绝对路径。
+
+## 2. 构建 `mmdet3d-serve` 镜像
+
+```shell
+docker build -t mmdet3d-serve:latest docker/serve/
+```
+
+## 3. 运行 `mmdet3d-serve`
+
+查看官网文档来 [使用 docker 运行 TorchServe](https://github.com/pytorch/serve/blob/master/docker/README.md#running-torchserve-in-a-production-docker-environment)。
+
+为了在 GPU 上运行,您需要安装 [nvidia-docker](https://docs.nvidia.com/datacenter/cloud-native/container-toolkit/install-guide.html)。您可以忽略 `--gpus` 参数,从而在 CPU 上运行。
+
+例子:
+
+```shell
+docker run --rm \
+--cpus 8 \
+--gpus device=0 \
+-p8080:8080 -p8081:8081 -p8082:8082 \
+--mount type=bind,source=$MODEL_STORE,target=/home/model-server/model-store \
+mmdet3d-serve:latest
+```
+
+[阅读文档](https://github.com/pytorch/serve/blob/072f5d088cce9bb64b2a18af065886c9b01b317b/docs/rest_api.md/) 关于 Inference (8080), Management (8081) and Metrics (8082) 接口。
+
+## 4. 测试部署
+
+您可以使用 `test_torchserver.py` 进行部署, 同时比较 torchserver 和 pytorch 的结果。
+
+```shell
+python tools/deployment/test_torchserver.py ${IMAGE_FILE} ${CONFIG_FILE} ${CHECKPOINT_FILE} ${MODEL_NAME}
+[--inference-addr ${INFERENCE_ADDR}] [--device ${DEVICE}] [--score-thr ${SCORE_THR}]
+```
+
+例子:
+
+```shell
+python tools/deployment/test_torchserver.py demo/data/kitti/kitti_000008.bin configs/second/hv_second_secfpn_6x8_80e_kitti-3d-car.py checkpoints/hv_second_secfpn_6x8_80e_kitti-3d-car_20200620_230238-393f000c.pth second
+```
+
+
+
+# 模型复杂度
+
+您可以使用 MMDetection 中的 `tools/analysis_tools/get_flops.py` 这个脚本文件,基于 [flops-counter.pytorch](https://github.com/sovrasov/flops-counter.pytorch) 计算一个给定模型的计算量 (FLOPS) 和参数量 (params)。
+
+```shell
+python tools/analysis_tools/get_flops.py ${CONFIG_FILE} [--shape ${INPUT_SHAPE}]
+```
+
+您将会得到如下的结果:
+
+```text
+==============================
+Input shape: (4000, 4)
+Flops: 5.78 GFLOPs
+Params: 953.83 k
+==============================
+```
+
+**注意**: 此工具仍然处于试验阶段,我们不能保证数值是绝对正确的。您可以将结果用于简单的比较,但在写技术文档报告或者论文之前您需要再次确认一下。
+
+1. 计算量 (FLOPs) 和输入形状有关,但是参数量 (params) 则和输入形状无关。默认的输入形状为 (1, 40000, 4)。
+2. 一些运算操作不计入计算量 (FLOPs),比如说像GN和定制的运算操作,详细细节请参考 [`mmcv.cnn.get_model_complexity_info()`](https://github.com/open-mmlab/mmcv/blob/master/mmcv/cnn/utils/flops_counter.py)。
+3. 我们现在仅仅支持单模态输入(点云或者图片)的单阶段模型的计算量 (FLOPs) 计算,我们将会在未来支持两阶段和多模态模型的计算。
+
+
+
+# 模型转换
+
+## RegNet 模型转换到 MMDetection
+
+`tools/model_converters/regnet2mmdet.py` 将 pycls 预训练 RegNet 模型中的键转换为 MMDetection 风格。
+
+```shell
+python tools/model_converters/regnet2mmdet.py ${SRC} ${DST} [-h]
+```
+
+## Detectron ResNet 转换到 Pytorch
+
+MMDetection 中的 `tools/detectron2pytorch.py` 能够把原始的 detectron 中预训练的 ResNet 模型的键转换为 PyTorch 风格。
+
+```shell
+python tools/detectron2pytorch.py ${SRC} ${DST} ${DEPTH} [-h]
+```
+
+## 准备要发布的模型
+
+`tools/model_converters/publish_model.py` 帮助用户准备他们用于发布的模型。
+
+在您上传一个模型到云服务器 (AWS) 之前,您需要做以下几步:
+
+1. 将模型权重转换为 CPU 张量
+2. 删除记录优化器状态 (optimizer states) 的相关信息
+3. 计算检查点 (checkpoint) 文件的哈希编码 (hash id) 并且把哈希编码加到文件名里
+
+```shell
+python tools/model_converters/publish_model.py ${INPUT_FILENAME} ${OUTPUT_FILENAME}
+```
+
+例如,
+
+```shell
+python tools/model_converters/publish_model.py work_dirs/faster_rcnn/latest.pth faster_rcnn_r50_fpn_1x_20190801.pth
+```
+
+最终的输出文件名将会是 `faster_rcnn_r50_fpn_1x_20190801-{hash id}.pth`。
+
+
+
+# 数据集转换
+
+`tools/data_converter/` 包含转换数据集为其他格式的一些工具。其中大多数转换数据集为基于 pickle 的信息文件,比如 KITTI,nuscense 和 lyft。Waymo 转换器被用来重新组织 waymo 原始数据为 KITTI 风格。用户能够参考它们了解我们转换数据格式的方法。将它们修改为 nuImages 转换器等脚本也很方便。
+
+为了转换 nuImages 数据集为 COCO 格式,请使用下面的指令:
+
+```shell
+python -u tools/data_converter/nuimage_converter.py --data-root ${DATA_ROOT} --version ${VERSIONS} \
+ --out-dir ${OUT_DIR} --nproc ${NUM_WORKERS} --extra-tag ${TAG}
+```
+
+- `--data-root`: 数据集的根目录,默认为 `./data/nuimages`。
+- `--version`: 数据集的版本,默认为 `v1.0-mini`。要获取完整数据集,请使用 `--version v1.0-train v1.0-val v1.0-mini`。
+- `--out-dir`: 注释和语义掩码的输出目录,默认为 `./data/nuimages/annotations/`。
+- `--nproc`: 数据准备的进程数,默认为 `4`。由于图片是并行处理的,更大的进程数目能够减少准备时间。
+- `--extra-tag`: 注释的额外标签,默认为 `nuimages`。这可用于将不同时间处理的不同注释分开以供研究。
+
+更多的数据准备细节参考 [doc](https://mmdetection3d.readthedocs.io/zh_CN/latest/data_preparation.html),nuImages 数据集的细节参考 [README](https://github.com/open-mmlab/mmdetection3d/blob/master/configs/nuimages/README.md/)。
+
+
+
+# 其他内容
+
+## 打印完整的配置文件
+
+`tools/misc/print_config.py` 逐字打印整个配置文件,展开所有的导入。
+
+```shell
+python tools/misc/print_config.py ${CONFIG} [-h] [--options ${OPTIONS [OPTIONS...]}]
+```
diff --git a/mmdet3d/__init__.py b/mmdet3d/__init__.py
new file mode 100644
index 0000000..312e9b4
--- /dev/null
+++ b/mmdet3d/__init__.py
@@ -0,0 +1,49 @@
+# Copyright (c) OpenMMLab. All rights reserved.
+import mmcv
+
+import mmdet
+import mmseg
+from .version import __version__, short_version
+
+
+def digit_version(version_str):
+ digit_version = []
+ for x in version_str.split('.'):
+ if x.isdigit():
+ digit_version.append(int(x))
+ elif x.find('rc') != -1:
+ patch_version = x.split('rc')
+ digit_version.append(int(patch_version[0]) - 1)
+ digit_version.append(int(patch_version[1]))
+ return digit_version
+
+
+mmcv_minimum_version = '1.4.8'
+mmcv_maximum_version = '1.6.0'
+mmcv_version = digit_version(mmcv.__version__)
+
+
+assert (mmcv_version >= digit_version(mmcv_minimum_version)
+ and mmcv_version <= digit_version(mmcv_maximum_version)), \
+ f'MMCV=={mmcv.__version__} is used but incompatible. ' \
+ f'Please install mmcv>={mmcv_minimum_version}, <={mmcv_maximum_version}.'
+
+mmdet_minimum_version = '2.24.0'
+mmdet_maximum_version = '3.0.0'
+mmdet_version = digit_version(mmdet.__version__)
+assert (mmdet_version >= digit_version(mmdet_minimum_version)
+ and mmdet_version <= digit_version(mmdet_maximum_version)), \
+ f'MMDET=={mmdet.__version__} is used but incompatible. ' \
+ f'Please install mmdet>={mmdet_minimum_version}, ' \
+ f'<={mmdet_maximum_version}.'
+
+mmseg_minimum_version = '0.20.0'
+mmseg_maximum_version = '1.0.0'
+mmseg_version = digit_version(mmseg.__version__)
+assert (mmseg_version >= digit_version(mmseg_minimum_version)
+ and mmseg_version <= digit_version(mmseg_maximum_version)), \
+ f'MMSEG=={mmseg.__version__} is used but incompatible. ' \
+ f'Please install mmseg>={mmseg_minimum_version}, ' \
+ f'<={mmseg_maximum_version}.'
+
+__all__ = ['__version__', 'short_version']
diff --git a/mmdet3d/apis/__init__.py b/mmdet3d/apis/__init__.py
new file mode 100644
index 0000000..5befc10
--- /dev/null
+++ b/mmdet3d/apis/__init__.py
@@ -0,0 +1,14 @@
+# Copyright (c) OpenMMLab. All rights reserved.
+from .inference import (convert_SyncBN, inference_detector,
+ inference_mono_3d_detector,
+ inference_multi_modality_detector, inference_segmentor,
+ init_model, show_result_meshlab)
+from .test import single_gpu_test
+from .train import init_random_seed, train_model
+
+__all__ = [
+ 'inference_detector', 'init_model', 'single_gpu_test',
+ 'inference_mono_3d_detector', 'show_result_meshlab', 'convert_SyncBN',
+ 'train_model', 'inference_multi_modality_detector', 'inference_segmentor',
+ 'init_random_seed'
+]
diff --git a/mmdet3d/apis/inference.py b/mmdet3d/apis/inference.py
new file mode 100644
index 0000000..1457182
--- /dev/null
+++ b/mmdet3d/apis/inference.py
@@ -0,0 +1,526 @@
+# Copyright (c) OpenMMLab. All rights reserved.
+import re
+from copy import deepcopy
+from os import path as osp
+
+import mmcv
+import numpy as np
+import torch
+from mmcv.parallel import collate, scatter
+from mmcv.runner import load_checkpoint
+
+from mmdet3d.core import (Box3DMode, CameraInstance3DBoxes, Coord3DMode,
+ DepthInstance3DBoxes, LiDARInstance3DBoxes,
+ show_multi_modality_result, show_result,
+ show_seg_result)
+from mmdet3d.core.bbox import get_box_type
+from mmdet3d.datasets.pipelines import Compose
+from mmdet3d.models import build_model
+from mmdet3d.utils import get_root_logger
+
+
+def convert_SyncBN(config):
+ """Convert config's naiveSyncBN to BN.
+
+ Args:
+ config (str or :obj:`mmcv.Config`): Config file path or the config
+ object.
+ """
+ if isinstance(config, dict):
+ for item in config:
+ if item == 'norm_cfg':
+ config[item]['type'] = config[item]['type']. \
+ replace('naiveSyncBN', 'BN')
+ else:
+ convert_SyncBN(config[item])
+
+
+def init_model(config, checkpoint=None, device='cuda:0'):
+ """Initialize a model from config file, which could be a 3D detector or a
+ 3D segmentor.
+
+ Args:
+ config (str or :obj:`mmcv.Config`): Config file path or the config
+ object.
+ checkpoint (str, optional): Checkpoint path. If left as None, the model
+ will not load any weights.
+ device (str): Device to use.
+
+ Returns:
+ nn.Module: The constructed detector.
+ """
+ if isinstance(config, str):
+ config = mmcv.Config.fromfile(config)
+ elif not isinstance(config, mmcv.Config):
+ raise TypeError('config must be a filename or Config object, '
+ f'but got {type(config)}')
+ config.model.pretrained = None
+ convert_SyncBN(config.model)
+ config.model.train_cfg = None
+ model = build_model(config.model, test_cfg=config.get('test_cfg'))
+ if checkpoint is not None:
+ checkpoint = load_checkpoint(model, checkpoint, map_location='cpu')
+ if 'CLASSES' in checkpoint['meta']:
+ model.CLASSES = checkpoint['meta']['CLASSES']
+ else:
+ model.CLASSES = config.class_names
+ if 'PALETTE' in checkpoint['meta']: # 3D Segmentor
+ model.PALETTE = checkpoint['meta']['PALETTE']
+ model.cfg = config # save the config in the model for convenience
+ if device != 'cpu':
+ torch.cuda.set_device(device)
+ else:
+ logger = get_root_logger()
+ logger.warning('Don\'t suggest using CPU device. '
+ 'Some functions are not supported for now.')
+ model.to(device)
+ model.eval()
+ return model
+
+
+def inference_detector(model, pcd):
+ """Inference point cloud with the detector.
+
+ Args:
+ model (nn.Module): The loaded detector.
+ pcd (str): Point cloud files.
+
+ Returns:
+ tuple: Predicted results and data from pipeline.
+ """
+ cfg = model.cfg
+ device = next(model.parameters()).device # model device
+
+ if not isinstance(pcd, str):
+ cfg = cfg.copy()
+ # set loading pipeline type
+ cfg.data.test.pipeline[0].type = 'LoadPointsFromDict'
+
+ # build the data pipeline
+ test_pipeline = deepcopy(cfg.data.test.pipeline)
+ test_pipeline = Compose(test_pipeline)
+ box_type_3d, box_mode_3d = get_box_type(cfg.data.test.box_type_3d)
+
+ if isinstance(pcd, str):
+ # load from point clouds file
+ data = dict(
+ pts_filename=pcd,
+ box_type_3d=box_type_3d,
+ box_mode_3d=box_mode_3d,
+ # for ScanNet demo we need axis_align_matrix
+ ann_info=dict(axis_align_matrix=np.eye(4)),
+ sweeps=[],
+ # set timestamp = 0
+ timestamp=[0],
+ img_fields=[],
+ bbox3d_fields=[],
+ pts_mask_fields=[],
+ pts_seg_fields=[],
+ bbox_fields=[],
+ mask_fields=[],
+ seg_fields=[])
+ else:
+ # load from http
+ data = dict(
+ points=pcd,
+ box_type_3d=box_type_3d,
+ box_mode_3d=box_mode_3d,
+ # for ScanNet demo we need axis_align_matrix
+ ann_info=dict(axis_align_matrix=np.eye(4)),
+ sweeps=[],
+ # set timestamp = 0
+ timestamp=[0],
+ img_fields=[],
+ bbox3d_fields=[],
+ pts_mask_fields=[],
+ pts_seg_fields=[],
+ bbox_fields=[],
+ mask_fields=[],
+ seg_fields=[])
+ data = test_pipeline(data)
+ data = collate([data], samples_per_gpu=1)
+ if next(model.parameters()).is_cuda:
+ # scatter to specified GPU
+ data = scatter(data, [device.index])[0]
+ else:
+ # this is a workaround to avoid the bug of MMDataParallel
+ data['img_metas'] = data['img_metas'][0].data
+ data['points'] = data['points'][0].data
+ # forward the model
+ with torch.no_grad():
+ result = model(return_loss=False, rescale=True, **data)
+ return result, data
+
+
+def inference_multi_modality_detector(model, pcd, image, ann_file):
+ """Inference point cloud with the multi-modality detector.
+
+ Args:
+ model (nn.Module): The loaded detector.
+ pcd (str): Point cloud files.
+ image (str): Image files.
+ ann_file (str): Annotation files.
+
+ Returns:
+ tuple: Predicted results and data from pipeline.
+ """
+ cfg = model.cfg
+ device = next(model.parameters()).device # model device
+ # build the data pipeline
+ test_pipeline = deepcopy(cfg.data.test.pipeline)
+ test_pipeline = Compose(test_pipeline)
+ box_type_3d, box_mode_3d = get_box_type(cfg.data.test.box_type_3d)
+ # get data info containing calib
+ data_infos = mmcv.load(ann_file)
+ image_idx = int(re.findall(r'\d+', image)[-1]) # xxx/sunrgbd_000017.jpg
+ for x in data_infos:
+ if int(x['image']['image_idx']) != image_idx:
+ continue
+ info = x
+ break
+ data = dict(
+ pts_filename=pcd,
+ img_prefix=osp.dirname(image),
+ img_info=dict(filename=osp.basename(image)),
+ box_type_3d=box_type_3d,
+ box_mode_3d=box_mode_3d,
+ img_fields=[],
+ bbox3d_fields=[],
+ pts_mask_fields=[],
+ pts_seg_fields=[],
+ bbox_fields=[],
+ mask_fields=[],
+ seg_fields=[])
+ data = test_pipeline(data)
+
+ # TODO: this code is dataset-specific. Move lidar2img and
+ # depth2img to .pkl annotations in the future.
+ # LiDAR to image conversion
+ if box_mode_3d == Box3DMode.LIDAR:
+ rect = info['calib']['R0_rect'].astype(np.float32)
+ Trv2c = info['calib']['Tr_velo_to_cam'].astype(np.float32)
+ P2 = info['calib']['P2'].astype(np.float32)
+ lidar2img = P2 @ rect @ Trv2c
+ data['img_metas'][0].data['lidar2img'] = lidar2img
+ # Depth to image conversion
+ elif box_mode_3d == Box3DMode.DEPTH:
+ rt_mat = info['calib']['Rt']
+ # follow Coord3DMode.convert_point
+ rt_mat = np.array([[1, 0, 0], [0, 0, -1], [0, 1, 0]
+ ]) @ rt_mat.transpose(1, 0)
+ depth2img = info['calib']['K'] @ rt_mat
+ data['img_metas'][0].data['depth2img'] = depth2img
+
+ data = collate([data], samples_per_gpu=1)
+ if next(model.parameters()).is_cuda:
+ # scatter to specified GPU
+ data = scatter(data, [device.index])[0]
+ else:
+ # this is a workaround to avoid the bug of MMDataParallel
+ data['img_metas'] = data['img_metas'][0].data
+ data['points'] = data['points'][0].data
+ data['img'] = data['img'][0].data
+
+ # forward the model
+ with torch.no_grad():
+ result = model(return_loss=False, rescale=True, **data)
+ return result, data
+
+
+def inference_mono_3d_detector(model, image, ann_file):
+ """Inference image with the monocular 3D detector.
+
+ Args:
+ model (nn.Module): The loaded detector.
+ image (str): Image files.
+ ann_file (str): Annotation files.
+
+ Returns:
+ tuple: Predicted results and data from pipeline.
+ """
+ cfg = model.cfg
+ device = next(model.parameters()).device # model device
+ # build the data pipeline
+ test_pipeline = deepcopy(cfg.data.test.pipeline)
+ test_pipeline = Compose(test_pipeline)
+ box_type_3d, box_mode_3d = get_box_type(cfg.data.test.box_type_3d)
+ # get data info containing calib
+ data_infos = mmcv.load(ann_file)
+ # find the info corresponding to this image
+ for x in data_infos['images']:
+ if osp.basename(x['file_name']) != osp.basename(image):
+ continue
+ img_info = x
+ break
+ data = dict(
+ img_prefix=osp.dirname(image),
+ img_info=dict(filename=osp.basename(image)),
+ box_type_3d=box_type_3d,
+ box_mode_3d=box_mode_3d,
+ img_fields=[],
+ bbox3d_fields=[],
+ pts_mask_fields=[],
+ pts_seg_fields=[],
+ bbox_fields=[],
+ mask_fields=[],
+ seg_fields=[])
+
+ # camera points to image conversion
+ if box_mode_3d == Box3DMode.CAM:
+ data['img_info'].update(dict(cam_intrinsic=img_info['cam_intrinsic']))
+
+ data = test_pipeline(data)
+
+ data = collate([data], samples_per_gpu=1)
+ if next(model.parameters()).is_cuda:
+ # scatter to specified GPU
+ data = scatter(data, [device.index])[0]
+ else:
+ # this is a workaround to avoid the bug of MMDataParallel
+ data['img_metas'] = data['img_metas'][0].data
+ data['img'] = data['img'][0].data
+
+ # forward the model
+ with torch.no_grad():
+ result = model(return_loss=False, rescale=True, **data)
+ return result, data
+
+
+def inference_segmentor(model, pcd):
+ """Inference point cloud with the segmentor.
+
+ Args:
+ model (nn.Module): The loaded segmentor.
+ pcd (str): Point cloud files.
+
+ Returns:
+ tuple: Predicted results and data from pipeline.
+ """
+ cfg = model.cfg
+ device = next(model.parameters()).device # model device
+ # build the data pipeline
+ test_pipeline = deepcopy(cfg.data.test.pipeline)
+ test_pipeline = Compose(test_pipeline)
+ data = dict(
+ pts_filename=pcd,
+ img_fields=[],
+ bbox3d_fields=[],
+ pts_mask_fields=[],
+ pts_seg_fields=[],
+ bbox_fields=[],
+ mask_fields=[],
+ seg_fields=[])
+ data = test_pipeline(data)
+ data = collate([data], samples_per_gpu=1)
+ if next(model.parameters()).is_cuda:
+ # scatter to specified GPU
+ data = scatter(data, [device.index])[0]
+ else:
+ # this is a workaround to avoid the bug of MMDataParallel
+ data['img_metas'] = data['img_metas'][0].data
+ data['points'] = data['points'][0].data
+ # forward the model
+ with torch.no_grad():
+ result = model(return_loss=False, rescale=True, **data)
+ return result, data
+
+
+def show_det_result_meshlab(data,
+ result,
+ out_dir,
+ score_thr=0.0,
+ show=False,
+ snapshot=False):
+ """Show 3D detection result by meshlab."""
+ points = data['points'][0][0].cpu().numpy()
+ pts_filename = data['img_metas'][0][0]['pts_filename']
+ file_name = osp.split(pts_filename)[-1].split('.')[0]
+
+ if 'pts_bbox' in result[0].keys():
+ pred_bboxes = result[0]['pts_bbox']['boxes_3d'].tensor.numpy()
+ pred_scores = result[0]['pts_bbox']['scores_3d'].numpy()
+ else:
+ pred_bboxes = result[0]['boxes_3d'].tensor.numpy()
+ pred_scores = result[0]['scores_3d'].numpy()
+
+ # filter out low score bboxes for visualization
+ if score_thr > 0:
+ inds = pred_scores > score_thr
+ pred_bboxes = pred_bboxes[inds]
+
+ # for now we convert points into depth mode
+ box_mode = data['img_metas'][0][0]['box_mode_3d']
+ if box_mode != Box3DMode.DEPTH:
+ points = Coord3DMode.convert(points, box_mode, Coord3DMode.DEPTH)
+ show_bboxes = Box3DMode.convert(pred_bboxes, box_mode, Box3DMode.DEPTH)
+ else:
+ show_bboxes = deepcopy(pred_bboxes)
+
+ show_result(
+ points,
+ None,
+ show_bboxes,
+ out_dir,
+ file_name,
+ show=show,
+ snapshot=snapshot)
+
+ return file_name
+
+
+def show_seg_result_meshlab(data,
+ result,
+ out_dir,
+ palette,
+ show=False,
+ snapshot=False):
+ """Show 3D segmentation result by meshlab."""
+ points = data['points'][0][0].cpu().numpy()
+ pts_filename = data['img_metas'][0][0]['pts_filename']
+ file_name = osp.split(pts_filename)[-1].split('.')[0]
+
+ pred_seg = result[0]['semantic_mask'].numpy()
+
+ if palette is None:
+ # generate random color map
+ max_idx = pred_seg.max()
+ palette = np.random.randint(0, 256, size=(max_idx + 1, 3))
+ palette = np.array(palette).astype(np.int)
+
+ show_seg_result(
+ points,
+ None,
+ pred_seg,
+ out_dir,
+ file_name,
+ palette=palette,
+ show=show,
+ snapshot=snapshot)
+
+ return file_name
+
+
+def show_proj_det_result_meshlab(data,
+ result,
+ out_dir,
+ score_thr=0.0,
+ show=False,
+ snapshot=False):
+ """Show result of projecting 3D bbox to 2D image by meshlab."""
+ assert 'img' in data.keys(), 'image data is not provided for visualization'
+
+ img_filename = data['img_metas'][0][0]['filename']
+ file_name = osp.split(img_filename)[-1].split('.')[0]
+
+ # read from file because img in data_dict has undergone pipeline transform
+ img = mmcv.imread(img_filename)
+
+ if 'pts_bbox' in result[0].keys():
+ result[0] = result[0]['pts_bbox']
+ elif 'img_bbox' in result[0].keys():
+ result[0] = result[0]['img_bbox']
+ pred_bboxes = result[0]['boxes_3d'].tensor.numpy()
+ pred_scores = result[0]['scores_3d'].numpy()
+
+ # filter out low score bboxes for visualization
+ if score_thr > 0:
+ inds = pred_scores > score_thr
+ pred_bboxes = pred_bboxes[inds]
+
+ box_mode = data['img_metas'][0][0]['box_mode_3d']
+ if box_mode == Box3DMode.LIDAR:
+ if 'lidar2img' not in data['img_metas'][0][0]:
+ raise NotImplementedError(
+ 'LiDAR to image transformation matrix is not provided')
+
+ show_bboxes = LiDARInstance3DBoxes(pred_bboxes, origin=(0.5, 0.5, 0))
+
+ show_multi_modality_result(
+ img,
+ None,
+ show_bboxes,
+ data['img_metas'][0][0]['lidar2img'],
+ out_dir,
+ file_name,
+ box_mode='lidar',
+ show=show)
+ elif box_mode == Box3DMode.DEPTH:
+ show_bboxes = DepthInstance3DBoxes(pred_bboxes, origin=(0.5, 0.5, 0))
+
+ show_multi_modality_result(
+ img,
+ None,
+ show_bboxes,
+ None,
+ out_dir,
+ file_name,
+ box_mode='depth',
+ img_metas=data['img_metas'][0][0],
+ show=show)
+ elif box_mode == Box3DMode.CAM:
+ if 'cam2img' not in data['img_metas'][0][0]:
+ raise NotImplementedError(
+ 'camera intrinsic matrix is not provided')
+
+ show_bboxes = CameraInstance3DBoxes(
+ pred_bboxes, box_dim=pred_bboxes.shape[-1], origin=(0.5, 1.0, 0.5))
+
+ show_multi_modality_result(
+ img,
+ None,
+ show_bboxes,
+ data['img_metas'][0][0]['cam2img'],
+ out_dir,
+ file_name,
+ box_mode='camera',
+ show=show)
+ else:
+ raise NotImplementedError(
+ f'visualization of {box_mode} bbox is not supported')
+
+ return file_name
+
+
+def show_result_meshlab(data,
+ result,
+ out_dir,
+ score_thr=0.0,
+ show=False,
+ snapshot=False,
+ task='det',
+ palette=None):
+ """Show result by meshlab.
+
+ Args:
+ data (dict): Contain data from pipeline.
+ result (dict): Predicted result from model.
+ out_dir (str): Directory to save visualized result.
+ score_thr (float, optional): Minimum score of bboxes to be shown.
+ Default: 0.0
+ show (bool, optional): Visualize the results online. Defaults to False.
+ snapshot (bool, optional): Whether to save the online results.
+ Defaults to False.
+ task (str, optional): Distinguish which task result to visualize.
+ Currently we support 3D detection, multi-modality detection and
+ 3D segmentation. Defaults to 'det'.
+ palette (list[list[int]]] | np.ndarray, optional): The palette
+ of segmentation map. If None is given, random palette will be
+ generated. Defaults to None.
+ """
+ assert task in ['det', 'multi_modality-det', 'seg', 'mono-det'], \
+ f'unsupported visualization task {task}'
+ assert out_dir is not None, 'Expect out_dir, got none.'
+
+ if task in ['det', 'multi_modality-det']:
+ file_name = show_det_result_meshlab(data, result, out_dir, score_thr,
+ show, snapshot)
+
+ if task in ['seg']:
+ file_name = show_seg_result_meshlab(data, result, out_dir, palette,
+ show, snapshot)
+
+ if task in ['multi_modality-det', 'mono-det']:
+ file_name = show_proj_det_result_meshlab(data, result, out_dir,
+ score_thr, show, snapshot)
+
+ return out_dir, file_name
diff --git a/mmdet3d/apis/test.py b/mmdet3d/apis/test.py
new file mode 100644
index 0000000..c0e66c0
--- /dev/null
+++ b/mmdet3d/apis/test.py
@@ -0,0 +1,90 @@
+# Copyright (c) OpenMMLab. All rights reserved.
+from os import path as osp
+
+import mmcv
+import torch
+from mmcv.image import tensor2imgs
+
+from mmdet3d.models import (Base3DDetector, Base3DSegmentor,
+ SingleStageMono3DDetector)
+
+
+def single_gpu_test(model,
+ data_loader,
+ show=False,
+ out_dir=None,
+ show_score_thr=0.3):
+ """Test model with single gpu.
+
+ This method tests model with single gpu and gives the 'show' option.
+ By setting ``show=True``, it saves the visualization results under
+ ``out_dir``.
+
+ Args:
+ model (nn.Module): Model to be tested.
+ data_loader (nn.Dataloader): Pytorch data loader.
+ show (bool, optional): Whether to save viualization results.
+ Default: True.
+ out_dir (str, optional): The path to save visualization results.
+ Default: None.
+
+ Returns:
+ list[dict]: The prediction results.
+ """
+ model.eval()
+ results = []
+ dataset = data_loader.dataset
+ prog_bar = mmcv.ProgressBar(len(dataset))
+ for i, data in enumerate(data_loader):
+ with torch.no_grad():
+ result = model(return_loss=False, rescale=True, **data)
+
+ if show:
+ # Visualize the results of MMDetection3D model
+ # 'show_results' is MMdetection3D visualization API
+ models_3d = (Base3DDetector, Base3DSegmentor,
+ SingleStageMono3DDetector)
+ if isinstance(model.module, models_3d):
+ model.module.show_results(
+ data,
+ result,
+ out_dir=out_dir,
+ show=show,
+ score_thr=show_score_thr)
+ # Visualize the results of MMDetection model
+ # 'show_result' is MMdetection visualization API
+ else:
+ batch_size = len(result)
+ if batch_size == 1 and isinstance(data['img'][0],
+ torch.Tensor):
+ img_tensor = data['img'][0]
+ else:
+ img_tensor = data['img'][0].data[0]
+ img_metas = data['img_metas'][0].data[0]
+ imgs = tensor2imgs(img_tensor, **img_metas[0]['img_norm_cfg'])
+ assert len(imgs) == len(img_metas)
+
+ for i, (img, img_meta) in enumerate(zip(imgs, img_metas)):
+ h, w, _ = img_meta['img_shape']
+ img_show = img[:h, :w, :]
+
+ ori_h, ori_w = img_meta['ori_shape'][:-1]
+ img_show = mmcv.imresize(img_show, (ori_w, ori_h))
+
+ if out_dir:
+ out_file = osp.join(out_dir, img_meta['ori_filename'])
+ else:
+ out_file = None
+
+ model.module.show_result(
+ img_show,
+ result[i],
+ show=show,
+ out_file=out_file,
+ score_thr=show_score_thr)
+ results.extend(result)
+
+ batch_size = len(result)
+ for _ in range(batch_size):
+ prog_bar.update()
+ return results
diff --git a/mmdet3d/apis/train.py b/mmdet3d/apis/train.py
new file mode 100644
index 0000000..4d97026
--- /dev/null
+++ b/mmdet3d/apis/train.py
@@ -0,0 +1,351 @@
+# Copyright (c) OpenMMLab. All rights reserved.
+import random
+import warnings
+
+import numpy as np
+import torch
+from mmcv.parallel import MMDataParallel, MMDistributedDataParallel
+from mmcv.runner import (HOOKS, DistSamplerSeedHook, EpochBasedRunner,
+ Fp16OptimizerHook, OptimizerHook, build_optimizer,
+ build_runner, get_dist_info)
+from mmcv.utils import build_from_cfg
+from torch import distributed as dist
+
+from mmdet3d.datasets import build_dataset
+from mmdet3d.utils import find_latest_checkpoint
+from mmdet.core import DistEvalHook as MMDET_DistEvalHook
+from mmdet.core import EvalHook as MMDET_EvalHook
+from mmdet.datasets import build_dataloader as build_mmdet_dataloader
+from mmdet.datasets import replace_ImageToTensor
+from mmdet.utils import get_root_logger as get_mmdet_root_logger
+from mmseg.core import DistEvalHook as MMSEG_DistEvalHook
+from mmseg.core import EvalHook as MMSEG_EvalHook
+from mmseg.datasets import build_dataloader as build_mmseg_dataloader
+from mmseg.utils import get_root_logger as get_mmseg_root_logger
+
+
+def init_random_seed(seed=None, device='cuda'):
+ """Initialize random seed.
+
+ If the seed is not set, the seed will be automatically randomized,
+ and then broadcast to all processes to prevent some potential bugs.
+ Args:
+ seed (int, optional): The seed. Default to None.
+ device (str, optional): The device where the seed will be put on.
+ Default to 'cuda'.
+ Returns:
+ int: Seed to be used.
+ """
+ if seed is not None:
+ return seed
+
+ # Make sure all ranks share the same random seed to prevent
+ # some potential bugs. Please refer to
+ # https://github.com/open-mmlab/mmdetection/issues/6339
+ rank, world_size = get_dist_info()
+ seed = np.random.randint(2**31)
+ if world_size == 1:
+ return seed
+
+ if rank == 0:
+ random_num = torch.tensor(seed, dtype=torch.int32, device=device)
+ else:
+ random_num = torch.tensor(0, dtype=torch.int32, device=device)
+ dist.broadcast(random_num, src=0)
+ return random_num.item()
+
+
+def set_random_seed(seed, deterministic=False):
+ """Set random seed.
+
+ Args:
+ seed (int): Seed to be used.
+ deterministic (bool): Whether to set the deterministic option for
+ CUDNN backend, i.e., set `torch.backends.cudnn.deterministic`
+ to True and `torch.backends.cudnn.benchmark` to False.
+ Default: False.
+ """
+ random.seed(seed)
+ np.random.seed(seed)
+ torch.manual_seed(seed)
+ torch.cuda.manual_seed_all(seed)
+ if deterministic:
+ torch.backends.cudnn.deterministic = True
+ torch.backends.cudnn.benchmark = False
+
+
+def train_segmentor(model,
+ dataset,
+ cfg,
+ distributed=False,
+ validate=False,
+ timestamp=None,
+ meta=None):
+ """Launch segmentor training."""
+ logger = get_mmseg_root_logger(cfg.log_level)
+
+ # prepare data loaders
+ dataset = dataset if isinstance(dataset, (list, tuple)) else [dataset]
+ data_loaders = [
+ build_mmseg_dataloader(
+ ds,
+ cfg.data.samples_per_gpu,
+ cfg.data.workers_per_gpu,
+ # cfg.gpus will be ignored if distributed
+ len(cfg.gpu_ids),
+ dist=distributed,
+ seed=cfg.seed,
+ drop_last=True) for ds in dataset
+ ]
+
+ # put model on gpus
+ if distributed:
+ find_unused_parameters = cfg.get('find_unused_parameters', False)
+ # Sets the `find_unused_parameters` parameter in
+ # torch.nn.parallel.DistributedDataParallel
+ model = MMDistributedDataParallel(
+ model.cuda(),
+ device_ids=[torch.cuda.current_device()],
+ broadcast_buffers=False,
+ find_unused_parameters=find_unused_parameters)
+ else:
+ model = MMDataParallel(
+ model.cuda(cfg.gpu_ids[0]), device_ids=cfg.gpu_ids)
+
+ # build runner
+ optimizer = build_optimizer(model, cfg.optimizer)
+
+ if cfg.get('runner') is None:
+ cfg.runner = {'type': 'IterBasedRunner', 'max_iters': cfg.total_iters}
+ warnings.warn(
+ 'config is now expected to have a `runner` section, '
+ 'please set `runner` in your config.', UserWarning)
+
+ runner = build_runner(
+ cfg.runner,
+ default_args=dict(
+ model=model,
+ batch_processor=None,
+ optimizer=optimizer,
+ work_dir=cfg.work_dir,
+ logger=logger,
+ meta=meta))
+
+ # register hooks
+ runner.register_training_hooks(cfg.lr_config, cfg.optimizer_config,
+ cfg.checkpoint_config, cfg.log_config,
+ cfg.get('momentum_config', None))
+
+ # an ugly walkaround to make the .log and .log.json filenames the same
+ runner.timestamp = timestamp
+
+ # register eval hooks
+ if validate:
+ val_dataset = build_dataset(cfg.data.val, dict(test_mode=True))
+ val_dataloader = build_mmseg_dataloader(
+ val_dataset,
+ samples_per_gpu=1,
+ workers_per_gpu=cfg.data.workers_per_gpu,
+ dist=distributed,
+ shuffle=False)
+ eval_cfg = cfg.get('evaluation', {})
+ eval_cfg['by_epoch'] = cfg.runner['type'] != 'IterBasedRunner'
+ eval_hook = MMSEG_DistEvalHook if distributed else MMSEG_EvalHook
+ # In this PR (https://github.com/open-mmlab/mmcv/pull/1193), the
+ # priority of IterTimerHook has been modified from 'NORMAL' to 'LOW'.
+ runner.register_hook(
+ eval_hook(val_dataloader, **eval_cfg), priority='LOW')
+
+ # user-defined hooks
+ if cfg.get('custom_hooks', None):
+ custom_hooks = cfg.custom_hooks
+ assert isinstance(custom_hooks, list), \
+ f'custom_hooks expect list type, but got {type(custom_hooks)}'
+ for hook_cfg in cfg.custom_hooks:
+ assert isinstance(hook_cfg, dict), \
+ 'Each item in custom_hooks expects dict type, but got ' \
+ f'{type(hook_cfg)}'
+ hook_cfg = hook_cfg.copy()
+ priority = hook_cfg.pop('priority', 'NORMAL')
+ hook = build_from_cfg(hook_cfg, HOOKS)
+ runner.register_hook(hook, priority=priority)
+
+ if cfg.resume_from:
+ runner.resume(cfg.resume_from)
+ elif cfg.load_from:
+ runner.load_checkpoint(cfg.load_from)
+ runner.run(data_loaders, cfg.workflow)
+
+
+def train_detector(model,
+ dataset,
+ cfg,
+ distributed=False,
+ validate=False,
+ timestamp=None,
+ meta=None):
+ logger = get_mmdet_root_logger(log_level=cfg.log_level)
+
+ # prepare data loaders
+ dataset = dataset if isinstance(dataset, (list, tuple)) else [dataset]
+ if 'imgs_per_gpu' in cfg.data:
+ logger.warning('"imgs_per_gpu" is deprecated in MMDet V2.0. '
+ 'Please use "samples_per_gpu" instead')
+ if 'samples_per_gpu' in cfg.data:
+ logger.warning(
+ f'Got "imgs_per_gpu"={cfg.data.imgs_per_gpu} and '
+ f'"samples_per_gpu"={cfg.data.samples_per_gpu}, "imgs_per_gpu"'
+ f'={cfg.data.imgs_per_gpu} is used in this experiments')
+ else:
+ logger.warning(
+ 'Automatically set "samples_per_gpu"="imgs_per_gpu"='
+ f'{cfg.data.imgs_per_gpu} in this experiments')
+ cfg.data.samples_per_gpu = cfg.data.imgs_per_gpu
+
+ runner_type = 'EpochBasedRunner' if 'runner' not in cfg else cfg.runner[
+ 'type']
+ data_loaders = [
+ build_mmdet_dataloader(
+ ds,
+ cfg.data.samples_per_gpu,
+ cfg.data.workers_per_gpu,
+ # `num_gpus` will be ignored if distributed
+ num_gpus=len(cfg.gpu_ids),
+ dist=distributed,
+ seed=cfg.seed,
+ runner_type=runner_type,
+ persistent_workers=cfg.data.get('persistent_workers', False))
+ for ds in dataset
+ ]
+
+ # put model on gpus
+ if distributed:
+ find_unused_parameters = cfg.get('find_unused_parameters', False)
+ # Sets the `find_unused_parameters` parameter in
+ # torch.nn.parallel.DistributedDataParallel
+ model = MMDistributedDataParallel(
+ model.cuda(),
+ device_ids=[torch.cuda.current_device()],
+ broadcast_buffers=False,
+ find_unused_parameters=find_unused_parameters)
+ else:
+ model = MMDataParallel(
+ model.cuda(cfg.gpu_ids[0]), device_ids=cfg.gpu_ids)
+
+ # build runner
+ optimizer = build_optimizer(model, cfg.optimizer)
+
+ if 'runner' not in cfg:
+ cfg.runner = {
+ 'type': 'EpochBasedRunner',
+ 'max_epochs': cfg.total_epochs
+ }
+ warnings.warn(
+ 'config is now expected to have a `runner` section, '
+ 'please set `runner` in your config.', UserWarning)
+ else:
+ if 'total_epochs' in cfg:
+ assert cfg.total_epochs == cfg.runner.max_epochs
+
+ runner = build_runner(
+ cfg.runner,
+ default_args=dict(
+ model=model,
+ optimizer=optimizer,
+ work_dir=cfg.work_dir,
+ logger=logger,
+ meta=meta))
+
+ # an ugly workaround to make .log and .log.json filenames the same
+ runner.timestamp = timestamp
+
+ # fp16 setting
+ fp16_cfg = cfg.get('fp16', None)
+ if fp16_cfg is not None:
+ optimizer_config = Fp16OptimizerHook(
+ **cfg.optimizer_config, **fp16_cfg, distributed=distributed)
+ elif distributed and 'type' not in cfg.optimizer_config:
+ optimizer_config = OptimizerHook(**cfg.optimizer_config)
+ else:
+ optimizer_config = cfg.optimizer_config
+
+ # register hooks
+ runner.register_training_hooks(
+ cfg.lr_config,
+ optimizer_config,
+ cfg.checkpoint_config,
+ cfg.log_config,
+ cfg.get('momentum_config', None),
+ custom_hooks_config=cfg.get('custom_hooks', None))
+
+ if distributed:
+ if isinstance(runner, EpochBasedRunner):
+ runner.register_hook(DistSamplerSeedHook())
+
+ # register eval hooks
+ if validate:
+ # Support batch_size > 1 in validation
+ val_samples_per_gpu = cfg.data.val.pop('samples_per_gpu', 1)
+ if val_samples_per_gpu > 1:
+ # Replace 'ImageToTensor' to 'DefaultFormatBundle'
+ cfg.data.val.pipeline = replace_ImageToTensor(
+ cfg.data.val.pipeline)
+ val_dataset = build_dataset(cfg.data.val, dict(test_mode=True))
+ val_dataloader = build_mmdet_dataloader(
+ val_dataset,
+ samples_per_gpu=val_samples_per_gpu,
+ workers_per_gpu=cfg.data.workers_per_gpu,
+ dist=distributed,
+ shuffle=False)
+ eval_cfg = cfg.get('evaluation', {})
+ eval_cfg['by_epoch'] = cfg.runner['type'] != 'IterBasedRunner'
+ eval_hook = MMDET_DistEvalHook if distributed else MMDET_EvalHook
+ # In this PR (https://github.com/open-mmlab/mmcv/pull/1193), the
+ # priority of IterTimerHook has been modified from 'NORMAL' to 'LOW'.
+ runner.register_hook(
+ eval_hook(val_dataloader, **eval_cfg), priority='LOW')
+
+ resume_from = None
+ if cfg.resume_from is None and cfg.get('auto_resume'):
+ resume_from = find_latest_checkpoint(cfg.work_dir)
+
+ if resume_from is not None:
+ cfg.resume_from = resume_from
+
+ if cfg.resume_from:
+ runner.resume(cfg.resume_from)
+ elif cfg.load_from:
+ runner.load_checkpoint(cfg.load_from)
+ runner.run(data_loaders, cfg.workflow)
+
+
+def train_model(model,
+ dataset,
+ cfg,
+ distributed=False,
+ validate=False,
+ timestamp=None,
+ meta=None):
+ """A function wrapper for launching model training according to cfg.
+
+ Because we need different eval_hook in runner. Should be deprecated in the
+ future.
+ """
+ if cfg.model.type in ['EncoderDecoder3D']:
+ train_segmentor(
+ model,
+ dataset,
+ cfg,
+ distributed=distributed,
+ validate=validate,
+ timestamp=timestamp,
+ meta=meta)
+ else:
+ train_detector(
+ model,
+ dataset,
+ cfg,
+ distributed=distributed,
+ validate=validate,
+ timestamp=timestamp,
+ meta=meta)
diff --git a/mmdet3d/core/__init__.py b/mmdet3d/core/__init__.py
new file mode 100644
index 0000000..ffb0c1a
--- /dev/null
+++ b/mmdet3d/core/__init__.py
@@ -0,0 +1,9 @@
+# Copyright (c) OpenMMLab. All rights reserved.
+from .anchor import * # noqa: F401, F403
+from .bbox import * # noqa: F401, F403
+from .evaluation import * # noqa: F401, F403
+from .points import * # noqa: F401, F403
+from .post_processing import * # noqa: F401, F403
+from .utils import * # noqa: F401, F403
+from .visualizer import * # noqa: F401, F403
+from .voxel import * # noqa: F401, F403
diff --git a/mmdet3d/core/anchor/__init__.py b/mmdet3d/core/anchor/__init__.py
new file mode 100644
index 0000000..7a34bf5
--- /dev/null
+++ b/mmdet3d/core/anchor/__init__.py
@@ -0,0 +1,10 @@
+# Copyright (c) OpenMMLab. All rights reserved.
+from mmdet.core.anchor import build_prior_generator
+from .anchor_3d_generator import (AlignedAnchor3DRangeGenerator,
+ AlignedAnchor3DRangeGeneratorPerCls,
+ Anchor3DRangeGenerator)
+
+__all__ = [
+ 'AlignedAnchor3DRangeGenerator', 'Anchor3DRangeGenerator',
+ 'build_prior_generator', 'AlignedAnchor3DRangeGeneratorPerCls'
+]
diff --git a/mmdet3d/core/anchor/anchor_3d_generator.py b/mmdet3d/core/anchor/anchor_3d_generator.py
new file mode 100644
index 0000000..e8681b7
--- /dev/null
+++ b/mmdet3d/core/anchor/anchor_3d_generator.py
@@ -0,0 +1,419 @@
+# Copyright (c) OpenMMLab. All rights reserved.
+import mmcv
+import torch
+
+from mmdet.core.anchor import ANCHOR_GENERATORS
+
+
+@ANCHOR_GENERATORS.register_module()
+class Anchor3DRangeGenerator(object):
+ """3D Anchor Generator by range.
+
+ This anchor generator generates anchors by the given range in different
+ feature levels.
+ Due the convention in 3D detection, different anchor sizes are related to
+ different ranges for different categories. However we find this setting
+ does not effect the performance much in some datasets, e.g., nuScenes.
+
+ Args:
+ ranges (list[list[float]]): Ranges of different anchors.
+ The ranges are the same across different feature levels. But may
+ vary for different anchor sizes if size_per_range is True.
+ sizes (list[list[float]], optional): 3D sizes of anchors.
+ Defaults to [[3.9, 1.6, 1.56]].
+ scales (list[int], optional): Scales of anchors in different feature
+ levels. Defaults to [1].
+ rotations (list[float], optional): Rotations of anchors in a feature
+ grid. Defaults to [0, 1.5707963].
+ custom_values (tuple[float], optional): Customized values of that
+ anchor. For example, in nuScenes the anchors have velocities.
+ Defaults to ().
+ reshape_out (bool, optional): Whether to reshape the output into
+ (N x 4). Defaults to True.
+ size_per_range (bool, optional): Whether to use separate ranges for
+ different sizes. If size_per_range is True, the ranges should have
+ the same length as the sizes, if not, it will be duplicated.
+ Defaults to True.
+ """
+
+ def __init__(self,
+ ranges,
+ sizes=[[3.9, 1.6, 1.56]],
+ scales=[1],
+ rotations=[0, 1.5707963],
+ custom_values=(),
+ reshape_out=True,
+ size_per_range=True):
+ assert mmcv.is_list_of(ranges, list)
+ if size_per_range:
+ if len(sizes) != len(ranges):
+ assert len(ranges) == 1
+ ranges = ranges * len(sizes)
+ assert len(ranges) == len(sizes)
+ else:
+ assert len(ranges) == 1
+ assert mmcv.is_list_of(sizes, list)
+ assert isinstance(scales, list)
+
+ self.sizes = sizes
+ self.scales = scales
+ self.ranges = ranges
+ self.rotations = rotations
+ self.custom_values = custom_values
+ self.cached_anchors = None
+ self.reshape_out = reshape_out
+ self.size_per_range = size_per_range
+
+ def __repr__(self):
+ s = self.__class__.__name__ + '('
+ s += f'anchor_range={self.ranges},\n'
+ s += f'scales={self.scales},\n'
+ s += f'sizes={self.sizes},\n'
+ s += f'rotations={self.rotations},\n'
+ s += f'reshape_out={self.reshape_out},\n'
+ s += f'size_per_range={self.size_per_range})'
+ return s
+
+ @property
+ def num_base_anchors(self):
+ """list[int]: Total number of base anchors in a feature grid."""
+ num_rot = len(self.rotations)
+ num_size = torch.tensor(self.sizes).reshape(-1, 3).size(0)
+ return num_rot * num_size
+
+ @property
+ def num_levels(self):
+ """int: Number of feature levels that the generator is applied to."""
+ return len(self.scales)
+
+ def grid_anchors(self, featmap_sizes, device='cuda'):
+ """Generate grid anchors in multiple feature levels.
+
+ Args:
+ featmap_sizes (list[tuple]): List of feature map sizes in
+ multiple feature levels.
+ device (str, optional): Device where the anchors will be put on.
+ Defaults to 'cuda'.
+
+ Returns:
+ list[torch.Tensor]: Anchors in multiple feature levels.
+ The sizes of each tensor should be [N, 4], where
+ N = width * height * num_base_anchors, width and height
+ are the sizes of the corresponding feature level,
+ num_base_anchors is the number of anchors for that level.
+ """
+ assert self.num_levels == len(featmap_sizes)
+ multi_level_anchors = []
+ for i in range(self.num_levels):
+ anchors = self.single_level_grid_anchors(
+ featmap_sizes[i], self.scales[i], device=device)
+ if self.reshape_out:
+ anchors = anchors.reshape(-1, anchors.size(-1))
+ multi_level_anchors.append(anchors)
+ return multi_level_anchors
+
+ def single_level_grid_anchors(self, featmap_size, scale, device='cuda'):
+ """Generate grid anchors of a single level feature map.
+
+ This function is usually called by method ``self.grid_anchors``.
+
+ Args:
+ featmap_size (tuple[int]): Size of the feature map.
+ scale (float): Scale factor of the anchors in the current level.
+ device (str, optional): Device the tensor will be put on.
+ Defaults to 'cuda'.
+
+ Returns:
+ torch.Tensor: Anchors in the overall feature map.
+ """
+ # We reimplement the anchor generator using torch in cuda
+ # torch: 0.6975 s for 1000 times
+ # numpy: 4.3345 s for 1000 times
+ # which is ~5 times faster than the numpy implementation
+ if not self.size_per_range:
+ return self.anchors_single_range(
+ featmap_size,
+ self.ranges[0],
+ scale,
+ self.sizes,
+ self.rotations,
+ device=device)
+
+ mr_anchors = []
+ for anchor_range, anchor_size in zip(self.ranges, self.sizes):
+ mr_anchors.append(
+ self.anchors_single_range(
+ featmap_size,
+ anchor_range,
+ scale,
+ anchor_size,
+ self.rotations,
+ device=device))
+ mr_anchors = torch.cat(mr_anchors, dim=-3)
+ return mr_anchors
+
+ def anchors_single_range(self,
+ feature_size,
+ anchor_range,
+ scale=1,
+ sizes=[[3.9, 1.6, 1.56]],
+ rotations=[0, 1.5707963],
+ device='cuda'):
+ """Generate anchors in a single range.
+
+ Args:
+ feature_size (list[float] | tuple[float]): Feature map size. It is
+ either a list of a tuple of [D, H, W](in order of z, y, and x).
+ anchor_range (torch.Tensor | list[float]): Range of anchors with
+ shape [6]. The order is consistent with that of anchors, i.e.,
+ (x_min, y_min, z_min, x_max, y_max, z_max).
+ scale (float | int, optional): The scale factor of anchors.
+ Defaults to 1.
+ sizes (list[list] | np.ndarray | torch.Tensor, optional):
+ Anchor size with shape [N, 3], in order of x, y, z.
+ Defaults to [[3.9, 1.6, 1.56]].
+ rotations (list[float] | np.ndarray | torch.Tensor, optional):
+ Rotations of anchors in a single feature grid.
+ Defaults to [0, 1.5707963].
+ device (str): Devices that the anchors will be put on.
+ Defaults to 'cuda'.
+
+ Returns:
+ torch.Tensor: Anchors with shape
+ [*feature_size, num_sizes, num_rots, 7].
+ """
+ if len(feature_size) == 2:
+ feature_size = [1, feature_size[0], feature_size[1]]
+ anchor_range = torch.tensor(anchor_range, device=device)
+ z_centers = torch.linspace(
+ anchor_range[2], anchor_range[5], feature_size[0], device=device)
+ y_centers = torch.linspace(
+ anchor_range[1], anchor_range[4], feature_size[1], device=device)
+ x_centers = torch.linspace(
+ anchor_range[0], anchor_range[3], feature_size[2], device=device)
+ sizes = torch.tensor(sizes, device=device).reshape(-1, 3) * scale
+ rotations = torch.tensor(rotations, device=device)
+
+ # torch.meshgrid default behavior is 'id', np's default is 'xy'
+ rets = torch.meshgrid(x_centers, y_centers, z_centers, rotations)
+ # torch.meshgrid returns a tuple rather than list
+ rets = list(rets)
+ tile_shape = [1] * 5
+ tile_shape[-2] = int(sizes.shape[0])
+ for i in range(len(rets)):
+ rets[i] = rets[i].unsqueeze(-2).repeat(tile_shape).unsqueeze(-1)
+
+ sizes = sizes.reshape([1, 1, 1, -1, 1, 3])
+ tile_size_shape = list(rets[0].shape)
+ tile_size_shape[3] = 1
+ sizes = sizes.repeat(tile_size_shape)
+ rets.insert(3, sizes)
+
+ ret = torch.cat(rets, dim=-1).permute([2, 1, 0, 3, 4, 5])
+ # [1, 200, 176, N, 2, 7] for kitti after permute
+
+ if len(self.custom_values) > 0:
+ custom_ndim = len(self.custom_values)
+ custom = ret.new_zeros([*ret.shape[:-1], custom_ndim])
+ # custom[:] = self.custom_values
+ ret = torch.cat([ret, custom], dim=-1)
+ # [1, 200, 176, N, 2, 9] for nus dataset after permute
+ return ret
+
+
+@ANCHOR_GENERATORS.register_module()
+class AlignedAnchor3DRangeGenerator(Anchor3DRangeGenerator):
+ """Aligned 3D Anchor Generator by range.
+
+ This anchor generator uses a different manner to generate the positions
+ of anchors' centers from :class:`Anchor3DRangeGenerator`.
+
+ Note:
+ The `align` means that the anchor's center is aligned with the voxel
+ grid, which is also the feature grid. The previous implementation of
+ :class:`Anchor3DRangeGenerator` does not generate the anchors' center
+ according to the voxel grid. Rather, it generates the center by
+ uniformly distributing the anchors inside the minimum and maximum
+ anchor ranges according to the feature map sizes.
+ However, this makes the anchors center does not match the feature grid.
+ The :class:`AlignedAnchor3DRangeGenerator` add + 1 when using the
+ feature map sizes to obtain the corners of the voxel grid. Then it
+ shifts the coordinates to the center of voxel grid and use the left
+ up corner to distribute anchors.
+
+ Args:
+ anchor_corner (bool, optional): Whether to align with the corner of the
+ voxel grid. By default it is False and the anchor's center will be
+ the same as the corresponding voxel's center, which is also the
+ center of the corresponding greature grid. Defaults to False.
+ """
+
+ def __init__(self, align_corner=False, **kwargs):
+ super(AlignedAnchor3DRangeGenerator, self).__init__(**kwargs)
+ self.align_corner = align_corner
+
+ def anchors_single_range(self,
+ feature_size,
+ anchor_range,
+ scale,
+ sizes=[[3.9, 1.6, 1.56]],
+ rotations=[0, 1.5707963],
+ device='cuda'):
+ """Generate anchors in a single range.
+
+ Args:
+ feature_size (list[float] | tuple[float]): Feature map size. It is
+ either a list of a tuple of [D, H, W](in order of z, y, and x).
+ anchor_range (torch.Tensor | list[float]): Range of anchors with
+ shape [6]. The order is consistent with that of anchors, i.e.,
+ (x_min, y_min, z_min, x_max, y_max, z_max).
+ scale (float | int): The scale factor of anchors.
+ sizes (list[list] | np.ndarray | torch.Tensor, optional):
+ Anchor size with shape [N, 3], in order of x, y, z.
+ Defaults to [[3.9, 1.6, 1.56]].
+ rotations (list[float] | np.ndarray | torch.Tensor, optional):
+ Rotations of anchors in a single feature grid.
+ Defaults to [0, 1.5707963].
+ device (str, optional): Devices that the anchors will be put on.
+ Defaults to 'cuda'.
+
+ Returns:
+ torch.Tensor: Anchors with shape
+ [*feature_size, num_sizes, num_rots, 7].
+ """
+ if len(feature_size) == 2:
+ feature_size = [1, feature_size[0], feature_size[1]]
+ anchor_range = torch.tensor(anchor_range, device=device)
+ z_centers = torch.linspace(
+ anchor_range[2],
+ anchor_range[5],
+ feature_size[0] + 1,
+ device=device)
+ y_centers = torch.linspace(
+ anchor_range[1],
+ anchor_range[4],
+ feature_size[1] + 1,
+ device=device)
+ x_centers = torch.linspace(
+ anchor_range[0],
+ anchor_range[3],
+ feature_size[2] + 1,
+ device=device)
+ sizes = torch.tensor(sizes, device=device).reshape(-1, 3) * scale
+ rotations = torch.tensor(rotations, device=device)
+
+ # shift the anchor center
+ if not self.align_corner:
+ z_shift = (z_centers[1] - z_centers[0]) / 2
+ y_shift = (y_centers[1] - y_centers[0]) / 2
+ x_shift = (x_centers[1] - x_centers[0]) / 2
+ z_centers += z_shift
+ y_centers += y_shift
+ x_centers += x_shift
+
+ # torch.meshgrid default behavior is 'id', np's default is 'xy'
+ rets = torch.meshgrid(x_centers[:feature_size[2]],
+ y_centers[:feature_size[1]],
+ z_centers[:feature_size[0]], rotations)
+
+ # torch.meshgrid returns a tuple rather than list
+ rets = list(rets)
+ tile_shape = [1] * 5
+ tile_shape[-2] = int(sizes.shape[0])
+ for i in range(len(rets)):
+ rets[i] = rets[i].unsqueeze(-2).repeat(tile_shape).unsqueeze(-1)
+
+ sizes = sizes.reshape([1, 1, 1, -1, 1, 3])
+ tile_size_shape = list(rets[0].shape)
+ tile_size_shape[3] = 1
+ sizes = sizes.repeat(tile_size_shape)
+ rets.insert(3, sizes)
+
+ ret = torch.cat(rets, dim=-1).permute([2, 1, 0, 3, 4, 5])
+
+ if len(self.custom_values) > 0:
+ custom_ndim = len(self.custom_values)
+ custom = ret.new_zeros([*ret.shape[:-1], custom_ndim])
+ # TODO: check the support of custom values
+ # custom[:] = self.custom_values
+ ret = torch.cat([ret, custom], dim=-1)
+ return ret
+
+
+@ANCHOR_GENERATORS.register_module()
+class AlignedAnchor3DRangeGeneratorPerCls(AlignedAnchor3DRangeGenerator):
+ """3D Anchor Generator by range for per class.
+
+ This anchor generator generates anchors by the given range for per class.
+ Note that feature maps of different classes may be different.
+
+ Args:
+ kwargs (dict): Arguments are the same as those in
+ :class:`AlignedAnchor3DRangeGenerator`.
+ """
+
+ def __init__(self, **kwargs):
+ super(AlignedAnchor3DRangeGeneratorPerCls, self).__init__(**kwargs)
+ assert len(self.scales) == 1, 'Multi-scale feature map levels are' + \
+ ' not supported currently in this kind of anchor generator.'
+
+ def grid_anchors(self, featmap_sizes, device='cuda'):
+ """Generate grid anchors in multiple feature levels.
+
+ Args:
+ featmap_sizes (list[tuple]): List of feature map sizes for
+ different classes in a single feature level.
+ device (str, optional): Device where the anchors will be put on.
+ Defaults to 'cuda'.
+
+ Returns:
+ list[list[torch.Tensor]]: Anchors in multiple feature levels.
+ Note that in this anchor generator, we currently only
+ support single feature level. The sizes of each tensor
+ should be [num_sizes/ranges*num_rots*featmap_size,
+ box_code_size].
+ """
+ multi_level_anchors = []
+ anchors = self.multi_cls_grid_anchors(
+ featmap_sizes, self.scales[0], device=device)
+ multi_level_anchors.append(anchors)
+ return multi_level_anchors
+
+ def multi_cls_grid_anchors(self, featmap_sizes, scale, device='cuda'):
+ """Generate grid anchors of a single level feature map for multi-class
+ with different feature map sizes.
+
+ This function is usually called by method ``self.grid_anchors``.
+
+ Args:
+ featmap_sizes (list[tuple]): List of feature map sizes for
+ different classes in a single feature level.
+ scale (float): Scale factor of the anchors in the current level.
+ device (str, optional): Device the tensor will be put on.
+ Defaults to 'cuda'.
+
+ Returns:
+ torch.Tensor: Anchors in the overall feature map.
+ """
+ assert len(featmap_sizes) == len(self.sizes) == len(self.ranges), \
+ 'The number of different feature map sizes anchor sizes and ' + \
+ 'ranges should be the same.'
+
+ multi_cls_anchors = []
+ for i in range(len(featmap_sizes)):
+ anchors = self.anchors_single_range(
+ featmap_sizes[i],
+ self.ranges[i],
+ scale,
+ self.sizes[i],
+ self.rotations,
+ device=device)
+ # [*featmap_size, num_sizes/ranges, num_rots, box_code_size]
+ ndim = len(featmap_sizes[i])
+ anchors = anchors.view(*featmap_sizes[i], -1, anchors.size(-1))
+ # [*featmap_size, num_sizes/ranges*num_rots, box_code_size]
+ anchors = anchors.permute(ndim, *range(0, ndim), ndim + 1)
+ # [num_sizes/ranges*num_rots, *featmap_size, box_code_size]
+ multi_cls_anchors.append(anchors.reshape(-1, anchors.size(-1)))
+ # [num_sizes/ranges*num_rots*featmap_size, box_code_size]
+ return multi_cls_anchors
diff --git a/mmdet3d/core/bbox/__init__.py b/mmdet3d/core/bbox/__init__.py
new file mode 100644
index 0000000..8c66630
--- /dev/null
+++ b/mmdet3d/core/bbox/__init__.py
@@ -0,0 +1,30 @@
+# Copyright (c) OpenMMLab. All rights reserved.
+from .assigners import AssignResult, BaseAssigner, MaxIoUAssigner
+from .coders import DeltaXYZWLHRBBoxCoder
+# from .bbox_target import bbox_target
+from .iou_calculators import (AxisAlignedBboxOverlaps3D, BboxOverlaps3D,
+ BboxOverlapsNearest3D,
+ axis_aligned_bbox_overlaps_3d, bbox_overlaps_3d,
+ bbox_overlaps_nearest_3d)
+from .samplers import (BaseSampler, CombinedSampler,
+ InstanceBalancedPosSampler, IoUBalancedNegSampler,
+ PseudoSampler, RandomSampler, SamplingResult)
+from .structures import (BaseInstance3DBoxes, Box3DMode, CameraInstance3DBoxes,
+ Coord3DMode, DepthInstance3DBoxes,
+ LiDARInstance3DBoxes, get_box_type, limit_period,
+ mono_cam_box2vis, points_cam2img, points_img2cam,
+ xywhr2xyxyr)
+from .transforms import bbox3d2result, bbox3d2roi, bbox3d_mapping_back
+
+__all__ = [
+ 'BaseSampler', 'AssignResult', 'BaseAssigner', 'MaxIoUAssigner',
+ 'PseudoSampler', 'RandomSampler', 'InstanceBalancedPosSampler',
+ 'IoUBalancedNegSampler', 'CombinedSampler', 'SamplingResult',
+ 'DeltaXYZWLHRBBoxCoder', 'BboxOverlapsNearest3D', 'BboxOverlaps3D',
+ 'bbox_overlaps_nearest_3d', 'bbox_overlaps_3d',
+ 'AxisAlignedBboxOverlaps3D', 'axis_aligned_bbox_overlaps_3d', 'Box3DMode',
+ 'LiDARInstance3DBoxes', 'CameraInstance3DBoxes', 'bbox3d2roi',
+ 'bbox3d2result', 'DepthInstance3DBoxes', 'BaseInstance3DBoxes',
+ 'bbox3d_mapping_back', 'xywhr2xyxyr', 'limit_period', 'points_cam2img',
+ 'points_img2cam', 'get_box_type', 'Coord3DMode', 'mono_cam_box2vis'
+]
diff --git a/mmdet3d/core/bbox/assigners/__init__.py b/mmdet3d/core/bbox/assigners/__init__.py
new file mode 100644
index 0000000..d149368
--- /dev/null
+++ b/mmdet3d/core/bbox/assigners/__init__.py
@@ -0,0 +1,4 @@
+# Copyright (c) OpenMMLab. All rights reserved.
+from mmdet.core.bbox import AssignResult, BaseAssigner, MaxIoUAssigner
+
+__all__ = ['BaseAssigner', 'MaxIoUAssigner', 'AssignResult']
diff --git a/mmdet3d/core/bbox/box_np_ops.py b/mmdet3d/core/bbox/box_np_ops.py
new file mode 100644
index 0000000..bb52bbb
--- /dev/null
+++ b/mmdet3d/core/bbox/box_np_ops.py
@@ -0,0 +1,827 @@
+# Copyright (c) OpenMMLab. All rights reserved.
+# TODO: clean the functions in this file and move the APIs into box structures
+# in the future
+# NOTICE: All functions in this file are valid for LiDAR or depth boxes only
+# if we use default parameters.
+
+import numba
+import numpy as np
+
+from .structures.utils import limit_period, points_cam2img, rotation_3d_in_axis
+
+
+def camera_to_lidar(points, r_rect, velo2cam):
+ """Convert points in camera coordinate to lidar coordinate.
+
+ Note:
+ This function is for KITTI only.
+
+ Args:
+ points (np.ndarray, shape=[N, 3]): Points in camera coordinate.
+ r_rect (np.ndarray, shape=[4, 4]): Matrix to project points in
+ specific camera coordinate (e.g. CAM2) to CAM0.
+ velo2cam (np.ndarray, shape=[4, 4]): Matrix to project points in
+ camera coordinate to lidar coordinate.
+
+ Returns:
+ np.ndarray, shape=[N, 3]: Points in lidar coordinate.
+ """
+ points_shape = list(points.shape[0:-1])
+ if points.shape[-1] == 3:
+ points = np.concatenate([points, np.ones(points_shape + [1])], axis=-1)
+ lidar_points = points @ np.linalg.inv((r_rect @ velo2cam).T)
+ return lidar_points[..., :3]
+
+
+def box_camera_to_lidar(data, r_rect, velo2cam):
+ """Convert boxes in camera coordinate to lidar coordinate.
+
+ Note:
+ This function is for KITTI only.
+
+ Args:
+ data (np.ndarray, shape=[N, 7]): Boxes in camera coordinate.
+ r_rect (np.ndarray, shape=[4, 4]): Matrix to project points in
+ specific camera coordinate (e.g. CAM2) to CAM0.
+ velo2cam (np.ndarray, shape=[4, 4]): Matrix to project points in
+ camera coordinate to lidar coordinate.
+
+ Returns:
+ np.ndarray, shape=[N, 3]: Boxes in lidar coordinate.
+ """
+ xyz = data[:, 0:3]
+ x_size, y_size, z_size = data[:, 3:4], data[:, 4:5], data[:, 5:6]
+ r = data[:, 6:7]
+ xyz_lidar = camera_to_lidar(xyz, r_rect, velo2cam)
+ # yaw and dims also needs to be converted
+ r_new = -r - np.pi / 2
+ r_new = limit_period(r_new, period=np.pi * 2)
+ return np.concatenate([xyz_lidar, x_size, z_size, y_size, r_new], axis=1)
+
+
+def corners_nd(dims, origin=0.5):
+ """Generate relative box corners based on length per dim and origin point.
+
+ Args:
+ dims (np.ndarray, shape=[N, ndim]): Array of length per dim
+ origin (list or array or float, optional): origin point relate to
+ smallest point. Defaults to 0.5
+
+ Returns:
+ np.ndarray, shape=[N, 2 ** ndim, ndim]: Returned corners.
+ point layout example: (2d) x0y0, x0y1, x1y0, x1y1;
+ (3d) x0y0z0, x0y0z1, x0y1z0, x0y1z1, x1y0z0, x1y0z1, x1y1z0, x1y1z1
+ where x0 < x1, y0 < y1, z0 < z1.
+ """
+ ndim = int(dims.shape[1])
+ corners_norm = np.stack(
+ np.unravel_index(np.arange(2**ndim), [2] * ndim),
+ axis=1).astype(dims.dtype)
+ # now corners_norm has format: (2d) x0y0, x0y1, x1y0, x1y1
+ # (3d) x0y0z0, x0y0z1, x0y1z0, x0y1z1, x1y0z0, x1y0z1, x1y1z0, x1y1z1
+ # so need to convert to a format which is convenient to do other computing.
+ # for 2d boxes, format is clockwise start with minimum point
+ # for 3d boxes, please draw lines by your hand.
+ if ndim == 2:
+ # generate clockwise box corners
+ corners_norm = corners_norm[[0, 1, 3, 2]]
+ elif ndim == 3:
+ corners_norm = corners_norm[[0, 1, 3, 2, 4, 5, 7, 6]]
+ corners_norm = corners_norm - np.array(origin, dtype=dims.dtype)
+ corners = dims.reshape([-1, 1, ndim]) * corners_norm.reshape(
+ [1, 2**ndim, ndim])
+ return corners
+
+
+def center_to_corner_box2d(centers, dims, angles=None, origin=0.5):
+ """Convert kitti locations, dimensions and angles to corners.
+ format: center(xy), dims(xy), angles(counterclockwise when positive)
+
+ Args:
+ centers (np.ndarray): Locations in kitti label file with shape (N, 2).
+ dims (np.ndarray): Dimensions in kitti label file with shape (N, 2).
+ angles (np.ndarray, optional): Rotation_y in kitti label file with
+ shape (N). Defaults to None.
+ origin (list or array or float, optional): origin point relate to
+ smallest point. Defaults to 0.5.
+
+ Returns:
+ np.ndarray: Corners with the shape of (N, 4, 2).
+ """
+ # 'length' in kitti format is in x axis.
+ # xyz(hwl)(kitti label file)<->xyz(lhw)(camera)<->z(-x)(-y)(wlh)(lidar)
+ # center in kitti format is [0.5, 1.0, 0.5] in xyz.
+ corners = corners_nd(dims, origin=origin)
+ # corners: [N, 4, 2]
+ if angles is not None:
+ corners = rotation_3d_in_axis(corners, angles)
+ corners += centers.reshape([-1, 1, 2])
+ return corners
+
+
+@numba.jit(nopython=True)
+def depth_to_points(depth, trunc_pixel):
+ """Convert depth map to points.
+
+ Args:
+ depth (np.array, shape=[H, W]): Depth map which
+ the row of [0~`trunc_pixel`] are truncated.
+ trunc_pixel (int): The number of truncated row.
+
+ Returns:
+ np.ndarray: Points in camera coordinates.
+ """
+ num_pts = np.sum(depth[trunc_pixel:, ] > 0.1)
+ points = np.zeros((num_pts, 3), dtype=depth.dtype)
+ x = np.array([0, 0, 1], dtype=depth.dtype)
+ k = 0
+ for i in range(trunc_pixel, depth.shape[0]):
+ for j in range(depth.shape[1]):
+ if depth[i, j] > 0.1:
+ x = np.array([j, i, 1], dtype=depth.dtype)
+ points[k] = x * depth[i, j]
+ k += 1
+ return points
+
+
+def depth_to_lidar_points(depth, trunc_pixel, P2, r_rect, velo2cam):
+ """Convert depth map to points in lidar coordinate.
+
+ Args:
+ depth (np.array, shape=[H, W]): Depth map which
+ the row of [0~`trunc_pixel`] are truncated.
+ trunc_pixel (int): The number of truncated row.
+ P2 (p.array, shape=[4, 4]): Intrinsics of Camera2.
+ r_rect (np.ndarray, shape=[4, 4]): Matrix to project points in
+ specific camera coordinate (e.g. CAM2) to CAM0.
+ velo2cam (np.ndarray, shape=[4, 4]): Matrix to project points in
+ camera coordinate to lidar coordinate.
+
+ Returns:
+ np.ndarray: Points in lidar coordinates.
+ """
+ pts = depth_to_points(depth, trunc_pixel)
+ points_shape = list(pts.shape[0:-1])
+ points = np.concatenate([pts, np.ones(points_shape + [1])], axis=-1)
+ points = points @ np.linalg.inv(P2.T)
+ lidar_points = camera_to_lidar(points, r_rect, velo2cam)
+ return lidar_points
+
+
+def center_to_corner_box3d(centers,
+ dims,
+ angles=None,
+ origin=(0.5, 1.0, 0.5),
+ axis=1):
+ """Convert kitti locations, dimensions and angles to corners.
+
+ Args:
+ centers (np.ndarray): Locations in kitti label file with shape (N, 3).
+ dims (np.ndarray): Dimensions in kitti label file with shape (N, 3).
+ angles (np.ndarray, optional): Rotation_y in kitti label file with
+ shape (N). Defaults to None.
+ origin (list or array or float, optional): Origin point relate to
+ smallest point. Use (0.5, 1.0, 0.5) in camera and (0.5, 0.5, 0)
+ in lidar. Defaults to (0.5, 1.0, 0.5).
+ axis (int, optional): Rotation axis. 1 for camera and 2 for lidar.
+ Defaults to 1.
+
+ Returns:
+ np.ndarray: Corners with the shape of (N, 8, 3).
+ """
+ # 'length' in kitti format is in x axis.
+ # yzx(hwl)(kitti label file)<->xyz(lhw)(camera)<->z(-x)(-y)(lwh)(lidar)
+ # center in kitti format is [0.5, 1.0, 0.5] in xyz.
+ corners = corners_nd(dims, origin=origin)
+ # corners: [N, 8, 3]
+ if angles is not None:
+ corners = rotation_3d_in_axis(corners, angles, axis=axis)
+ corners += centers.reshape([-1, 1, 3])
+ return corners
+
+
+@numba.jit(nopython=True)
+def box2d_to_corner_jit(boxes):
+ """Convert box2d to corner.
+
+ Args:
+ boxes (np.ndarray, shape=[N, 5]): Boxes2d with rotation.
+
+ Returns:
+ box_corners (np.ndarray, shape=[N, 4, 2]): Box corners.
+ """
+ num_box = boxes.shape[0]
+ corners_norm = np.zeros((4, 2), dtype=boxes.dtype)
+ corners_norm[1, 1] = 1.0
+ corners_norm[2] = 1.0
+ corners_norm[3, 0] = 1.0
+ corners_norm -= np.array([0.5, 0.5], dtype=boxes.dtype)
+ corners = boxes.reshape(num_box, 1, 5)[:, :, 2:4] * corners_norm.reshape(
+ 1, 4, 2)
+ rot_mat_T = np.zeros((2, 2), dtype=boxes.dtype)
+ box_corners = np.zeros((num_box, 4, 2), dtype=boxes.dtype)
+ for i in range(num_box):
+ rot_sin = np.sin(boxes[i, -1])
+ rot_cos = np.cos(boxes[i, -1])
+ rot_mat_T[0, 0] = rot_cos
+ rot_mat_T[0, 1] = rot_sin
+ rot_mat_T[1, 0] = -rot_sin
+ rot_mat_T[1, 1] = rot_cos
+ box_corners[i] = corners[i] @ rot_mat_T + boxes[i, :2]
+ return box_corners
+
+
+@numba.njit
+def corner_to_standup_nd_jit(boxes_corner):
+ """Convert boxes_corner to aligned (min-max) boxes.
+
+ Args:
+ boxes_corner (np.ndarray, shape=[N, 2**dim, dim]): Boxes corners.
+
+ Returns:
+ np.ndarray, shape=[N, dim*2]: Aligned (min-max) boxes.
+ """
+ num_boxes = boxes_corner.shape[0]
+ ndim = boxes_corner.shape[-1]
+ result = np.zeros((num_boxes, ndim * 2), dtype=boxes_corner.dtype)
+ for i in range(num_boxes):
+ for j in range(ndim):
+ result[i, j] = np.min(boxes_corner[i, :, j])
+ for j in range(ndim):
+ result[i, j + ndim] = np.max(boxes_corner[i, :, j])
+ return result
+
+
+@numba.jit(nopython=True)
+def corner_to_surfaces_3d_jit(corners):
+ """Convert 3d box corners from corner function above to surfaces that
+ normal vectors all direct to internal.
+
+ Args:
+ corners (np.ndarray): 3d box corners with the shape of (N, 8, 3).
+
+ Returns:
+ np.ndarray: Surfaces with the shape of (N, 6, 4, 3).
+ """
+ # box_corners: [N, 8, 3], must from corner functions in this module
+ num_boxes = corners.shape[0]
+ surfaces = np.zeros((num_boxes, 6, 4, 3), dtype=corners.dtype)
+ corner_idxes = np.array([
+ 0, 1, 2, 3, 7, 6, 5, 4, 0, 3, 7, 4, 1, 5, 6, 2, 0, 4, 5, 1, 3, 2, 6, 7
+ ]).reshape(6, 4)
+ for i in range(num_boxes):
+ for j in range(6):
+ for k in range(4):
+ surfaces[i, j, k] = corners[i, corner_idxes[j, k]]
+ return surfaces
+
+
+def rotation_points_single_angle(points, angle, axis=0):
+ """Rotate points with a single angle.
+
+ Args:
+ points (np.ndarray, shape=[N, 3]]):
+ angle (np.ndarray, shape=[1]]):
+ axis (int, optional): Axis to rotate at. Defaults to 0.
+
+ Returns:
+ np.ndarray: Rotated points.
+ """
+ # points: [N, 3]
+ rot_sin = np.sin(angle)
+ rot_cos = np.cos(angle)
+ if axis == 1:
+ rot_mat_T = np.array(
+ [[rot_cos, 0, rot_sin], [0, 1, 0], [-rot_sin, 0, rot_cos]],
+ dtype=points.dtype)
+ elif axis == 2 or axis == -1:
+ rot_mat_T = np.array(
+ [[rot_cos, rot_sin, 0], [-rot_sin, rot_cos, 0], [0, 0, 1]],
+ dtype=points.dtype)
+ elif axis == 0:
+ rot_mat_T = np.array(
+ [[1, 0, 0], [0, rot_cos, rot_sin], [0, -rot_sin, rot_cos]],
+ dtype=points.dtype)
+ else:
+ raise ValueError('axis should in range')
+
+ return points @ rot_mat_T, rot_mat_T
+
+
+def box3d_to_bbox(box3d, P2):
+ """Convert box3d in camera coordinates to bbox in image coordinates.
+
+ Args:
+ box3d (np.ndarray, shape=[N, 7]): Boxes in camera coordinate.
+ P2 (np.array, shape=[4, 4]): Intrinsics of Camera2.
+
+ Returns:
+ np.ndarray, shape=[N, 4]: Boxes 2d in image coordinates.
+ """
+ box_corners = center_to_corner_box3d(
+ box3d[:, :3], box3d[:, 3:6], box3d[:, 6], [0.5, 1.0, 0.5], axis=1)
+ box_corners_in_image = points_cam2img(box_corners, P2)
+ # box_corners_in_image: [N, 8, 2]
+ minxy = np.min(box_corners_in_image, axis=1)
+ maxxy = np.max(box_corners_in_image, axis=1)
+ bbox = np.concatenate([minxy, maxxy], axis=1)
+ return bbox
+
+
+def corner_to_surfaces_3d(corners):
+ """convert 3d box corners from corner function above to surfaces that
+ normal vectors all direct to internal.
+
+ Args:
+ corners (np.ndarray): 3D box corners with shape of (N, 8, 3).
+
+ Returns:
+ np.ndarray: Surfaces with the shape of (N, 6, 4, 3).
+ """
+ # box_corners: [N, 8, 3], must from corner functions in this module
+ surfaces = np.array([
+ [corners[:, 0], corners[:, 1], corners[:, 2], corners[:, 3]],
+ [corners[:, 7], corners[:, 6], corners[:, 5], corners[:, 4]],
+ [corners[:, 0], corners[:, 3], corners[:, 7], corners[:, 4]],
+ [corners[:, 1], corners[:, 5], corners[:, 6], corners[:, 2]],
+ [corners[:, 0], corners[:, 4], corners[:, 5], corners[:, 1]],
+ [corners[:, 3], corners[:, 2], corners[:, 6], corners[:, 7]],
+ ]).transpose([2, 0, 1, 3])
+ return surfaces
+
+
+def points_in_rbbox(points, rbbox, z_axis=2, origin=(0.5, 0.5, 0)):
+ """Check points in rotated bbox and return indices.
+
+ Note:
+ This function is for counterclockwise boxes.
+
+ Args:
+ points (np.ndarray, shape=[N, 3+dim]): Points to query.
+ rbbox (np.ndarray, shape=[M, 7]): Boxes3d with rotation.
+ z_axis (int, optional): Indicate which axis is height.
+ Defaults to 2.
+ origin (tuple[int], optional): Indicate the position of
+ box center. Defaults to (0.5, 0.5, 0).
+
+ Returns:
+ np.ndarray, shape=[N, M]: Indices of points in each box.
+ """
+ # TODO: this function is different from PointCloud3D, be careful
+ # when start to use nuscene, check the input
+ rbbox_corners = center_to_corner_box3d(
+ rbbox[:, :3], rbbox[:, 3:6], rbbox[:, 6], origin=origin, axis=z_axis)
+ surfaces = corner_to_surfaces_3d(rbbox_corners)
+ indices = points_in_convex_polygon_3d_jit(points[:, :3], surfaces)
+ return indices
+
+
+def minmax_to_corner_2d(minmax_box):
+ """Convert minmax box to corners2d.
+
+ Args:
+ minmax_box (np.ndarray, shape=[N, dims]): minmax boxes.
+
+ Returns:
+ np.ndarray: 2d corners of boxes
+ """
+ ndim = minmax_box.shape[-1] // 2
+ center = minmax_box[..., :ndim]
+ dims = minmax_box[..., ndim:] - center
+ return center_to_corner_box2d(center, dims, origin=0.0)
+
+
+def create_anchors_3d_range(feature_size,
+ anchor_range,
+ sizes=((3.9, 1.6, 1.56), ),
+ rotations=(0, np.pi / 2),
+ dtype=np.float32):
+ """Create anchors 3d by range.
+
+ Args:
+ feature_size (list[float] | tuple[float]): Feature map size. It is
+ either a list of a tuple of [D, H, W](in order of z, y, and x).
+ anchor_range (torch.Tensor | list[float]): Range of anchors with
+ shape [6]. The order is consistent with that of anchors, i.e.,
+ (x_min, y_min, z_min, x_max, y_max, z_max).
+ sizes (list[list] | np.ndarray | torch.Tensor, optional):
+ Anchor size with shape [N, 3], in order of x, y, z.
+ Defaults to ((3.9, 1.6, 1.56), ).
+ rotations (list[float] | np.ndarray | torch.Tensor, optional):
+ Rotations of anchors in a single feature grid.
+ Defaults to (0, np.pi / 2).
+ dtype (type, optional): Data type. Defaults to np.float32.
+
+ Returns:
+ np.ndarray: Range based anchors with shape of
+ (*feature_size, num_sizes, num_rots, 7).
+ """
+ anchor_range = np.array(anchor_range, dtype)
+ z_centers = np.linspace(
+ anchor_range[2], anchor_range[5], feature_size[0], dtype=dtype)
+ y_centers = np.linspace(
+ anchor_range[1], anchor_range[4], feature_size[1], dtype=dtype)
+ x_centers = np.linspace(
+ anchor_range[0], anchor_range[3], feature_size[2], dtype=dtype)
+ sizes = np.reshape(np.array(sizes, dtype=dtype), [-1, 3])
+ rotations = np.array(rotations, dtype=dtype)
+ rets = np.meshgrid(
+ x_centers, y_centers, z_centers, rotations, indexing='ij')
+ tile_shape = [1] * 5
+ tile_shape[-2] = int(sizes.shape[0])
+ for i in range(len(rets)):
+ rets[i] = np.tile(rets[i][..., np.newaxis, :], tile_shape)
+ rets[i] = rets[i][..., np.newaxis] # for concat
+ sizes = np.reshape(sizes, [1, 1, 1, -1, 1, 3])
+ tile_size_shape = list(rets[0].shape)
+ tile_size_shape[3] = 1
+ sizes = np.tile(sizes, tile_size_shape)
+ rets.insert(3, sizes)
+ ret = np.concatenate(rets, axis=-1)
+ return np.transpose(ret, [2, 1, 0, 3, 4, 5])
+
+
+def center_to_minmax_2d(centers, dims, origin=0.5):
+ """Center to minmax.
+
+ Args:
+ centers (np.ndarray): Center points.
+ dims (np.ndarray): Dimensions.
+ origin (list or array or float, optional): Origin point relate
+ to smallest point. Defaults to 0.5.
+
+ Returns:
+ np.ndarray: Minmax points.
+ """
+ if origin == 0.5:
+ return np.concatenate([centers - dims / 2, centers + dims / 2],
+ axis=-1)
+ corners = center_to_corner_box2d(centers, dims, origin=origin)
+ return corners[:, [0, 2]].reshape([-1, 4])
+
+
+def rbbox2d_to_near_bbox(rbboxes):
+ """convert rotated bbox to nearest 'standing' or 'lying' bbox.
+
+ Args:
+ rbboxes (np.ndarray): Rotated bboxes with shape of
+ (N, 5(x, y, xdim, ydim, rad)).
+
+ Returns:
+ np.ndarray: Bounding boxes with the shape of
+ (N, 4(xmin, ymin, xmax, ymax)).
+ """
+ rots = rbboxes[..., -1]
+ rots_0_pi_div_2 = np.abs(limit_period(rots, 0.5, np.pi))
+ cond = (rots_0_pi_div_2 > np.pi / 4)[..., np.newaxis]
+ bboxes_center = np.where(cond, rbboxes[:, [0, 1, 3, 2]], rbboxes[:, :4])
+ bboxes = center_to_minmax_2d(bboxes_center[:, :2], bboxes_center[:, 2:])
+ return bboxes
+
+
+@numba.jit(nopython=True)
+def iou_jit(boxes, query_boxes, mode='iou', eps=0.0):
+ """Calculate box iou. Note that jit version runs ~10x faster than the
+ box_overlaps function in mmdet3d.core.evaluation.
+
+ Note:
+ This function is for counterclockwise boxes.
+
+ Args:
+ boxes (np.ndarray): Input bounding boxes with shape of (N, 4).
+ query_boxes (np.ndarray): Query boxes with shape of (K, 4).
+ mode (str, optional): IoU mode. Defaults to 'iou'.
+ eps (float, optional): Value added to denominator. Defaults to 0.
+
+ Returns:
+ np.ndarray: Overlap between boxes and query_boxes
+ with the shape of [N, K].
+ """
+ N = boxes.shape[0]
+ K = query_boxes.shape[0]
+ overlaps = np.zeros((N, K), dtype=boxes.dtype)
+ for k in range(K):
+ box_area = ((query_boxes[k, 2] - query_boxes[k, 0] + eps) *
+ (query_boxes[k, 3] - query_boxes[k, 1] + eps))
+ for n in range(N):
+ iw = (
+ min(boxes[n, 2], query_boxes[k, 2]) -
+ max(boxes[n, 0], query_boxes[k, 0]) + eps)
+ if iw > 0:
+ ih = (
+ min(boxes[n, 3], query_boxes[k, 3]) -
+ max(boxes[n, 1], query_boxes[k, 1]) + eps)
+ if ih > 0:
+ if mode == 'iou':
+ ua = ((boxes[n, 2] - boxes[n, 0] + eps) *
+ (boxes[n, 3] - boxes[n, 1] + eps) + box_area -
+ iw * ih)
+ else:
+ ua = ((boxes[n, 2] - boxes[n, 0] + eps) *
+ (boxes[n, 3] - boxes[n, 1] + eps))
+ overlaps[n, k] = iw * ih / ua
+ return overlaps
+
+
+def projection_matrix_to_CRT_kitti(proj):
+ """Split projection matrix of KITTI.
+
+ Note:
+ This function is for KITTI only.
+
+ P = C @ [R|T]
+ C is upper triangular matrix, so we need to inverse CR and use QR
+ stable for all kitti camera projection matrix.
+
+ Args:
+ proj (p.array, shape=[4, 4]): Intrinsics of camera.
+
+ Returns:
+ tuple[np.ndarray]: Splited matrix of C, R and T.
+ """
+
+ CR = proj[0:3, 0:3]
+ CT = proj[0:3, 3]
+ RinvCinv = np.linalg.inv(CR)
+ Rinv, Cinv = np.linalg.qr(RinvCinv)
+ C = np.linalg.inv(Cinv)
+ R = np.linalg.inv(Rinv)
+ T = Cinv @ CT
+ return C, R, T
+
+
+def remove_outside_points(points, rect, Trv2c, P2, image_shape):
+ """Remove points which are outside of image.
+
+ Note:
+ This function is for KITTI only.
+
+ Args:
+ points (np.ndarray, shape=[N, 3+dims]): Total points.
+ rect (np.ndarray, shape=[4, 4]): Matrix to project points in
+ specific camera coordinate (e.g. CAM2) to CAM0.
+ Trv2c (np.ndarray, shape=[4, 4]): Matrix to project points in
+ camera coordinate to lidar coordinate.
+ P2 (p.array, shape=[4, 4]): Intrinsics of Camera2.
+ image_shape (list[int]): Shape of image.
+
+ Returns:
+ np.ndarray, shape=[N, 3+dims]: Filtered points.
+ """
+ # 5x faster than remove_outside_points_v1(2ms vs 10ms)
+ C, R, T = projection_matrix_to_CRT_kitti(P2)
+ image_bbox = [0, 0, image_shape[1], image_shape[0]]
+ frustum = get_frustum(image_bbox, C)
+ frustum -= T
+ frustum = np.linalg.inv(R) @ frustum.T
+ frustum = camera_to_lidar(frustum.T, rect, Trv2c)
+ frustum_surfaces = corner_to_surfaces_3d_jit(frustum[np.newaxis, ...])
+ indices = points_in_convex_polygon_3d_jit(points[:, :3], frustum_surfaces)
+ points = points[indices.reshape([-1])]
+ return points
+
+
+def get_frustum(bbox_image, C, near_clip=0.001, far_clip=100):
+ """Get frustum corners in camera coordinates.
+
+ Args:
+ bbox_image (list[int]): box in image coordinates.
+ C (np.ndarray): Intrinsics.
+ near_clip (float, optional): Nearest distance of frustum.
+ Defaults to 0.001.
+ far_clip (float, optional): Farthest distance of frustum.
+ Defaults to 100.
+
+ Returns:
+ np.ndarray, shape=[8, 3]: coordinates of frustum corners.
+ """
+ fku = C[0, 0]
+ fkv = -C[1, 1]
+ u0v0 = C[0:2, 2]
+ z_points = np.array(
+ [near_clip] * 4 + [far_clip] * 4, dtype=C.dtype)[:, np.newaxis]
+ b = bbox_image
+ box_corners = np.array(
+ [[b[0], b[1]], [b[0], b[3]], [b[2], b[3]], [b[2], b[1]]],
+ dtype=C.dtype)
+ near_box_corners = (box_corners - u0v0) / np.array(
+ [fku / near_clip, -fkv / near_clip], dtype=C.dtype)
+ far_box_corners = (box_corners - u0v0) / np.array(
+ [fku / far_clip, -fkv / far_clip], dtype=C.dtype)
+ ret_xy = np.concatenate([near_box_corners, far_box_corners],
+ axis=0) # [8, 2]
+ ret_xyz = np.concatenate([ret_xy, z_points], axis=1)
+ return ret_xyz
+
+
+def surface_equ_3d(polygon_surfaces):
+ """
+
+ Args:
+ polygon_surfaces (np.ndarray): Polygon surfaces with shape of
+ [num_polygon, max_num_surfaces, max_num_points_of_surface, 3].
+ All surfaces' normal vector must direct to internal.
+ Max_num_points_of_surface must at least 3.
+
+ Returns:
+ tuple: normal vector and its direction.
+ """
+ # return [a, b, c], d in ax+by+cz+d=0
+ # polygon_surfaces: [num_polygon, num_surfaces, num_points_of_polygon, 3]
+ surface_vec = polygon_surfaces[:, :, :2, :] - \
+ polygon_surfaces[:, :, 1:3, :]
+ # normal_vec: [..., 3]
+ normal_vec = np.cross(surface_vec[:, :, 0, :], surface_vec[:, :, 1, :])
+ # print(normal_vec.shape, points[..., 0, :].shape)
+ # d = -np.inner(normal_vec, points[..., 0, :])
+ d = np.einsum('aij, aij->ai', normal_vec, polygon_surfaces[:, :, 0, :])
+ return normal_vec, -d
+
+
+@numba.njit
+def _points_in_convex_polygon_3d_jit(points, polygon_surfaces, normal_vec, d,
+ num_surfaces):
+ """
+ Args:
+ points (np.ndarray): Input points with shape of (num_points, 3).
+ polygon_surfaces (np.ndarray): Polygon surfaces with shape of
+ (num_polygon, max_num_surfaces, max_num_points_of_surface, 3).
+ All surfaces' normal vector must direct to internal.
+ Max_num_points_of_surface must at least 3.
+ normal_vec (np.ndarray): Normal vector of polygon_surfaces.
+ d (int): Directions of normal vector.
+ num_surfaces (np.ndarray): Number of surfaces a polygon contains
+ shape of (num_polygon).
+
+ Returns:
+ np.ndarray: Result matrix with the shape of [num_points, num_polygon].
+ """
+ max_num_surfaces, max_num_points_of_surface = polygon_surfaces.shape[1:3]
+ num_points = points.shape[0]
+ num_polygons = polygon_surfaces.shape[0]
+ ret = np.ones((num_points, num_polygons), dtype=np.bool_)
+ sign = 0.0
+ for i in range(num_points):
+ for j in range(num_polygons):
+ for k in range(max_num_surfaces):
+ if k > num_surfaces[j]:
+ break
+ sign = (
+ points[i, 0] * normal_vec[j, k, 0] +
+ points[i, 1] * normal_vec[j, k, 1] +
+ points[i, 2] * normal_vec[j, k, 2] + d[j, k])
+ if sign >= 0:
+ ret[i, j] = False
+ break
+ return ret
+
+
+def points_in_convex_polygon_3d_jit(points,
+ polygon_surfaces,
+ num_surfaces=None):
+ """Check points is in 3d convex polygons.
+
+ Args:
+ points (np.ndarray): Input points with shape of (num_points, 3).
+ polygon_surfaces (np.ndarray): Polygon surfaces with shape of
+ (num_polygon, max_num_surfaces, max_num_points_of_surface, 3).
+ All surfaces' normal vector must direct to internal.
+ Max_num_points_of_surface must at least 3.
+ num_surfaces (np.ndarray, optional): Number of surfaces a polygon
+ contains shape of (num_polygon). Defaults to None.
+
+ Returns:
+ np.ndarray: Result matrix with the shape of [num_points, num_polygon].
+ """
+ max_num_surfaces, max_num_points_of_surface = polygon_surfaces.shape[1:3]
+ # num_points = points.shape[0]
+ num_polygons = polygon_surfaces.shape[0]
+ if num_surfaces is None:
+ num_surfaces = np.full((num_polygons, ), 9999999, dtype=np.int64)
+ normal_vec, d = surface_equ_3d(polygon_surfaces[:, :, :3, :])
+ # normal_vec: [num_polygon, max_num_surfaces, 3]
+ # d: [num_polygon, max_num_surfaces]
+ return _points_in_convex_polygon_3d_jit(points, polygon_surfaces,
+ normal_vec, d, num_surfaces)
+
+
+@numba.njit
+def points_in_convex_polygon_jit(points, polygon, clockwise=False):
+ """Check points is in 2d convex polygons. True when point in polygon.
+
+ Args:
+ points (np.ndarray): Input points with the shape of [num_points, 2].
+ polygon (np.ndarray): Input polygon with the shape of
+ [num_polygon, num_points_of_polygon, 2].
+ clockwise (bool, optional): Indicate polygon is clockwise. Defaults
+ to True.
+
+ Returns:
+ np.ndarray: Result matrix with the shape of [num_points, num_polygon].
+ """
+ # first convert polygon to directed lines
+ num_points_of_polygon = polygon.shape[1]
+ num_points = points.shape[0]
+ num_polygons = polygon.shape[0]
+ # vec for all the polygons
+ if clockwise:
+ vec1 = polygon - polygon[:,
+ np.array([num_points_of_polygon - 1] + list(
+ range(num_points_of_polygon - 1))), :]
+ else:
+ vec1 = polygon[:,
+ np.array([num_points_of_polygon - 1] +
+ list(range(num_points_of_polygon -
+ 1))), :] - polygon
+ ret = np.zeros((num_points, num_polygons), dtype=np.bool_)
+ success = True
+ cross = 0.0
+ for i in range(num_points):
+ for j in range(num_polygons):
+ success = True
+ for k in range(num_points_of_polygon):
+ vec = vec1[j, k]
+ cross = vec[1] * (polygon[j, k, 0] - points[i, 0])
+ cross -= vec[0] * (polygon[j, k, 1] - points[i, 1])
+ if cross >= 0:
+ success = False
+ break
+ ret[i, j] = success
+ return ret
+
+
+def boxes3d_to_corners3d_lidar(boxes3d, bottom_center=True):
+ """Convert kitti center boxes to corners.
+
+ 7 -------- 4
+ /| /|
+ 6 -------- 5 .
+ | | | |
+ . 3 -------- 0
+ |/ |/
+ 2 -------- 1
+
+ Note:
+ This function is for LiDAR boxes only.
+
+ Args:
+ boxes3d (np.ndarray): Boxes with shape of (N, 7)
+ [x, y, z, x_size, y_size, z_size, ry] in LiDAR coords,
+ see the definition of ry in KITTI dataset.
+ bottom_center (bool, optional): Whether z is on the bottom center
+ of object. Defaults to True.
+
+ Returns:
+ np.ndarray: Box corners with the shape of [N, 8, 3].
+ """
+ boxes_num = boxes3d.shape[0]
+ x_size, y_size, z_size = boxes3d[:, 3], boxes3d[:, 4], boxes3d[:, 5]
+ x_corners = np.array([
+ x_size / 2., -x_size / 2., -x_size / 2., x_size / 2., x_size / 2.,
+ -x_size / 2., -x_size / 2., x_size / 2.
+ ],
+ dtype=np.float32).T
+ y_corners = np.array([
+ -y_size / 2., -y_size / 2., y_size / 2., y_size / 2., -y_size / 2.,
+ -y_size / 2., y_size / 2., y_size / 2.
+ ],
+ dtype=np.float32).T
+ if bottom_center:
+ z_corners = np.zeros((boxes_num, 8), dtype=np.float32)
+ z_corners[:, 4:8] = z_size.reshape(boxes_num, 1).repeat(
+ 4, axis=1) # (N, 8)
+ else:
+ z_corners = np.array([
+ -z_size / 2., -z_size / 2., -z_size / 2., -z_size / 2.,
+ z_size / 2., z_size / 2., z_size / 2., z_size / 2.
+ ],
+ dtype=np.float32).T
+
+ ry = boxes3d[:, 6]
+ zeros, ones = np.zeros(
+ ry.size, dtype=np.float32), np.ones(
+ ry.size, dtype=np.float32)
+ rot_list = np.array([[np.cos(ry), np.sin(ry), zeros],
+ [-np.sin(ry), np.cos(ry), zeros],
+ [zeros, zeros, ones]]) # (3, 3, N)
+ R_list = np.transpose(rot_list, (2, 0, 1)) # (N, 3, 3)
+
+ temp_corners = np.concatenate((x_corners.reshape(
+ -1, 8, 1), y_corners.reshape(-1, 8, 1), z_corners.reshape(-1, 8, 1)),
+ axis=2) # (N, 8, 3)
+ rotated_corners = np.matmul(temp_corners, R_list) # (N, 8, 3)
+ x_corners = rotated_corners[:, :, 0]
+ y_corners = rotated_corners[:, :, 1]
+ z_corners = rotated_corners[:, :, 2]
+
+ x_loc, y_loc, z_loc = boxes3d[:, 0], boxes3d[:, 1], boxes3d[:, 2]
+
+ x = x_loc.reshape(-1, 1) + x_corners.reshape(-1, 8)
+ y = y_loc.reshape(-1, 1) + y_corners.reshape(-1, 8)
+ z = z_loc.reshape(-1, 1) + z_corners.reshape(-1, 8)
+
+ corners = np.concatenate(
+ (x.reshape(-1, 8, 1), y.reshape(-1, 8, 1), z.reshape(-1, 8, 1)),
+ axis=2)
+
+ return corners.astype(np.float32)
diff --git a/mmdet3d/core/bbox/coders/__init__.py b/mmdet3d/core/bbox/coders/__init__.py
new file mode 100644
index 0000000..b306525
--- /dev/null
+++ b/mmdet3d/core/bbox/coders/__init__.py
@@ -0,0 +1,19 @@
+# Copyright (c) OpenMMLab. All rights reserved.
+from mmdet.core.bbox import build_bbox_coder
+from .anchor_free_bbox_coder import AnchorFreeBBoxCoder
+from .centerpoint_bbox_coders import CenterPointBBoxCoder
+from .delta_xyzwhlr_bbox_coder import DeltaXYZWLHRBBoxCoder
+from .fcos3d_bbox_coder import FCOS3DBBoxCoder
+from .groupfree3d_bbox_coder import GroupFree3DBBoxCoder
+from .monoflex_bbox_coder import MonoFlexCoder
+from .partial_bin_based_bbox_coder import PartialBinBasedBBoxCoder
+from .pgd_bbox_coder import PGDBBoxCoder
+from .point_xyzwhlr_bbox_coder import PointXYZWHLRBBoxCoder
+from .smoke_bbox_coder import SMOKECoder
+
+__all__ = [
+ 'build_bbox_coder', 'DeltaXYZWLHRBBoxCoder', 'PartialBinBasedBBoxCoder',
+ 'CenterPointBBoxCoder', 'AnchorFreeBBoxCoder', 'GroupFree3DBBoxCoder',
+ 'PointXYZWHLRBBoxCoder', 'FCOS3DBBoxCoder', 'PGDBBoxCoder', 'SMOKECoder',
+ 'MonoFlexCoder'
+]
diff --git a/mmdet3d/core/bbox/coders/anchor_free_bbox_coder.py b/mmdet3d/core/bbox/coders/anchor_free_bbox_coder.py
new file mode 100644
index 0000000..d64f38b
--- /dev/null
+++ b/mmdet3d/core/bbox/coders/anchor_free_bbox_coder.py
@@ -0,0 +1,130 @@
+# Copyright (c) OpenMMLab. All rights reserved.
+import numpy as np
+import torch
+
+from mmdet.core.bbox.builder import BBOX_CODERS
+from .partial_bin_based_bbox_coder import PartialBinBasedBBoxCoder
+
+
+@BBOX_CODERS.register_module()
+class AnchorFreeBBoxCoder(PartialBinBasedBBoxCoder):
+ """Anchor free bbox coder for 3D boxes.
+
+ Args:
+ num_dir_bins (int): Number of bins to encode direction angle.
+ with_rot (bool): Whether the bbox is with rotation.
+ """
+
+ def __init__(self, num_dir_bins, with_rot=True):
+ super(AnchorFreeBBoxCoder, self).__init__(
+ num_dir_bins, 0, [], with_rot=with_rot)
+ self.num_dir_bins = num_dir_bins
+ self.with_rot = with_rot
+
+ def encode(self, gt_bboxes_3d, gt_labels_3d):
+ """Encode ground truth to prediction targets.
+
+ Args:
+ gt_bboxes_3d (BaseInstance3DBoxes): Ground truth bboxes
+ with shape (n, 7).
+ gt_labels_3d (torch.Tensor): Ground truth classes.
+
+ Returns:
+ tuple: Targets of center, size and direction.
+ """
+ # generate center target
+ center_target = gt_bboxes_3d.gravity_center
+
+ # generate bbox size target
+ size_res_target = gt_bboxes_3d.dims / 2
+
+ # generate dir target
+ box_num = gt_labels_3d.shape[0]
+ if self.with_rot:
+ (dir_class_target,
+ dir_res_target) = self.angle2class(gt_bboxes_3d.yaw)
+ dir_res_target /= (2 * np.pi / self.num_dir_bins)
+ else:
+ dir_class_target = gt_labels_3d.new_zeros(box_num)
+ dir_res_target = gt_bboxes_3d.tensor.new_zeros(box_num)
+
+ return (center_target, size_res_target, dir_class_target,
+ dir_res_target)
+
+ def decode(self, bbox_out):
+ """Decode predicted parts to bbox3d.
+
+ Args:
+ bbox_out (dict): Predictions from model, should contain keys below.
+
+ - center: predicted bottom center of bboxes.
+ - dir_class: predicted bbox direction class.
+ - dir_res: predicted bbox direction residual.
+ - size: predicted bbox size.
+
+ Returns:
+ torch.Tensor: Decoded bbox3d with shape (batch, n, 7).
+ """
+ center = bbox_out['center']
+ batch_size, num_proposal = center.shape[:2]
+
+ # decode heading angle
+ if self.with_rot:
+ dir_class = torch.argmax(bbox_out['dir_class'], -1)
+ dir_res = torch.gather(bbox_out['dir_res'], 2,
+ dir_class.unsqueeze(-1))
+ dir_res.squeeze_(2)
+ dir_angle = self.class2angle(dir_class, dir_res).reshape(
+ batch_size, num_proposal, 1)
+ else:
+ dir_angle = center.new_zeros(batch_size, num_proposal, 1)
+
+ # decode bbox size
+ bbox_size = torch.clamp(bbox_out['size'] * 2, min=0.1)
+
+ bbox3d = torch.cat([center, bbox_size, dir_angle], dim=-1)
+ return bbox3d
+
+ def split_pred(self, cls_preds, reg_preds, base_xyz):
+ """Split predicted features to specific parts.
+
+ Args:
+ cls_preds (torch.Tensor): Class predicted features to split.
+ reg_preds (torch.Tensor): Regression predicted features to split.
+ base_xyz (torch.Tensor): Coordinates of points.
+
+ Returns:
+ dict[str, torch.Tensor]: Split results.
+ """
+ results = {}
+ results['obj_scores'] = cls_preds
+
+ start, end = 0, 0
+ reg_preds_trans = reg_preds.transpose(2, 1)
+
+ # decode center
+ end += 3
+ # (batch_size, num_proposal, 3)
+ results['center_offset'] = reg_preds_trans[..., start:end]
+ results['center'] = base_xyz.detach() + reg_preds_trans[..., start:end]
+ start = end
+
+ # decode center
+ end += 3
+ # (batch_size, num_proposal, 3)
+ results['size'] = reg_preds_trans[..., start:end]
+ start = end
+
+ # decode direction
+ end += self.num_dir_bins
+ results['dir_class'] = reg_preds_trans[..., start:end]
+ start = end
+
+ end += self.num_dir_bins
+ dir_res_norm = reg_preds_trans[..., start:end]
+ start = end
+
+ results['dir_res_norm'] = dir_res_norm
+ results['dir_res'] = dir_res_norm * (2 * np.pi / self.num_dir_bins)
+
+ return results
diff --git a/mmdet3d/core/bbox/coders/centerpoint_bbox_coders.py b/mmdet3d/core/bbox/coders/centerpoint_bbox_coders.py
new file mode 100644
index 0000000..6d43a63
--- /dev/null
+++ b/mmdet3d/core/bbox/coders/centerpoint_bbox_coders.py
@@ -0,0 +1,229 @@
+# Copyright (c) OpenMMLab. All rights reserved.
+import torch
+
+from mmdet.core.bbox import BaseBBoxCoder
+from mmdet.core.bbox.builder import BBOX_CODERS
+
+
+@BBOX_CODERS.register_module()
+class CenterPointBBoxCoder(BaseBBoxCoder):
+ """Bbox coder for CenterPoint.
+
+ Args:
+ pc_range (list[float]): Range of point cloud.
+ out_size_factor (int): Downsample factor of the model.
+ voxel_size (list[float]): Size of voxel.
+ post_center_range (list[float], optional): Limit of the center.
+ Default: None.
+ max_num (int, optional): Max number to be kept. Default: 100.
+ score_threshold (float, optional): Threshold to filter boxes
+ based on score. Default: None.
+ code_size (int, optional): Code size of bboxes. Default: 9
+ """
+
+ def __init__(self,
+ pc_range,
+ out_size_factor,
+ voxel_size,
+ post_center_range=None,
+ max_num=100,
+ score_threshold=None,
+ code_size=9):
+
+ self.pc_range = pc_range
+ self.out_size_factor = out_size_factor
+ self.voxel_size = voxel_size
+ self.post_center_range = post_center_range
+ self.max_num = max_num
+ self.score_threshold = score_threshold
+ self.code_size = code_size
+
+ def _gather_feat(self, feats, inds, feat_masks=None):
+ """Given feats and indexes, returns the gathered feats.
+
+ Args:
+ feats (torch.Tensor): Features to be transposed and gathered
+ with the shape of [B, 2, W, H].
+ inds (torch.Tensor): Indexes with the shape of [B, N].
+ feat_masks (torch.Tensor, optional): Mask of the feats.
+ Default: None.
+
+ Returns:
+ torch.Tensor: Gathered feats.
+ """
+ dim = feats.size(2)
+ inds = inds.unsqueeze(2).expand(inds.size(0), inds.size(1), dim)
+ feats = feats.gather(1, inds)
+ if feat_masks is not None:
+ feat_masks = feat_masks.unsqueeze(2).expand_as(feats)
+ feats = feats[feat_masks]
+ feats = feats.view(-1, dim)
+ return feats
+
+ def _topk(self, scores, K=80):
+ """Get indexes based on scores.
+
+ Args:
+ scores (torch.Tensor): scores with the shape of [B, N, W, H].
+ K (int, optional): Number to be kept. Defaults to 80.
+
+ Returns:
+ tuple[torch.Tensor]
+ torch.Tensor: Selected scores with the shape of [B, K].
+ torch.Tensor: Selected indexes with the shape of [B, K].
+ torch.Tensor: Selected classes with the shape of [B, K].
+ torch.Tensor: Selected y coord with the shape of [B, K].
+ torch.Tensor: Selected x coord with the shape of [B, K].
+ """
+ batch, cat, height, width = scores.size()
+
+ topk_scores, topk_inds = torch.topk(scores.view(batch, cat, -1), K)
+
+ topk_inds = topk_inds % (height * width)
+ topk_ys = (topk_inds.float() /
+ torch.tensor(width, dtype=torch.float)).int().float()
+ topk_xs = (topk_inds % width).int().float()
+
+ topk_score, topk_ind = torch.topk(topk_scores.view(batch, -1), K)
+ topk_clses = (topk_ind / torch.tensor(K, dtype=torch.float)).int()
+ topk_inds = self._gather_feat(topk_inds.view(batch, -1, 1),
+ topk_ind).view(batch, K)
+ topk_ys = self._gather_feat(topk_ys.view(batch, -1, 1),
+ topk_ind).view(batch, K)
+ topk_xs = self._gather_feat(topk_xs.view(batch, -1, 1),
+ topk_ind).view(batch, K)
+
+ return topk_score, topk_inds, topk_clses, topk_ys, topk_xs
+
+ def _transpose_and_gather_feat(self, feat, ind):
+ """Given feats and indexes, returns the transposed and gathered feats.
+
+ Args:
+ feat (torch.Tensor): Features to be transposed and gathered
+ with the shape of [B, 2, W, H].
+ ind (torch.Tensor): Indexes with the shape of [B, N].
+
+ Returns:
+ torch.Tensor: Transposed and gathered feats.
+ """
+ feat = feat.permute(0, 2, 3, 1).contiguous()
+ feat = feat.view(feat.size(0), -1, feat.size(3))
+ feat = self._gather_feat(feat, ind)
+ return feat
+
+ def encode(self):
+ pass
+
+ def decode(self,
+ heat,
+ rot_sine,
+ rot_cosine,
+ hei,
+ dim,
+ vel,
+ reg=None,
+ task_id=-1):
+ """Decode bboxes.
+
+ Args:
+ heat (torch.Tensor): Heatmap with the shape of [B, N, W, H].
+ rot_sine (torch.Tensor): Sine of rotation with the shape of
+ [B, 1, W, H].
+ rot_cosine (torch.Tensor): Cosine of rotation with the shape of
+ [B, 1, W, H].
+ hei (torch.Tensor): Height of the boxes with the shape
+ of [B, 1, W, H].
+ dim (torch.Tensor): Dim of the boxes with the shape of
+ [B, 1, W, H].
+ vel (torch.Tensor): Velocity with the shape of [B, 1, W, H].
+ reg (torch.Tensor, optional): Regression value of the boxes in
+ 2D with the shape of [B, 2, W, H]. Default: None.
+ task_id (int, optional): Index of task. Default: -1.
+
+ Returns:
+ list[dict]: Decoded boxes.
+ """
+ batch, cat, _, _ = heat.size()
+
+ scores, inds, clses, ys, xs = self._topk(heat, K=self.max_num)
+
+ if reg is not None:
+ reg = self._transpose_and_gather_feat(reg, inds)
+ reg = reg.view(batch, self.max_num, 2)
+ xs = xs.view(batch, self.max_num, 1) + reg[:, :, 0:1]
+ ys = ys.view(batch, self.max_num, 1) + reg[:, :, 1:2]
+ else:
+ xs = xs.view(batch, self.max_num, 1) + 0.5
+ ys = ys.view(batch, self.max_num, 1) + 0.5
+
+ # rotation value and direction label
+ rot_sine = self._transpose_and_gather_feat(rot_sine, inds)
+ rot_sine = rot_sine.view(batch, self.max_num, 1)
+
+ rot_cosine = self._transpose_and_gather_feat(rot_cosine, inds)
+ rot_cosine = rot_cosine.view(batch, self.max_num, 1)
+ rot = torch.atan2(rot_sine, rot_cosine)
+
+ # height in the bev
+ hei = self._transpose_and_gather_feat(hei, inds)
+ hei = hei.view(batch, self.max_num, 1)
+
+ # dim of the box
+ dim = self._transpose_and_gather_feat(dim, inds)
+ dim = dim.view(batch, self.max_num, 3)
+
+ # class label
+ clses = clses.view(batch, self.max_num).float()
+ scores = scores.view(batch, self.max_num)
+
+ xs = xs.view(
+ batch, self.max_num,
+ 1) * self.out_size_factor * self.voxel_size[0] + self.pc_range[0]
+ ys = ys.view(
+ batch, self.max_num,
+ 1) * self.out_size_factor * self.voxel_size[1] + self.pc_range[1]
+
+ if vel is None: # KITTI FORMAT
+ final_box_preds = torch.cat([xs, ys, hei, dim, rot], dim=2)
+ else: # exist velocity, nuscene format
+ vel = self._transpose_and_gather_feat(vel, inds)
+ vel = vel.view(batch, self.max_num, 2)
+ final_box_preds = torch.cat([xs, ys, hei, dim, rot, vel], dim=2)
+
+ final_scores = scores
+ final_preds = clses
+
+ # use score threshold
+ if self.score_threshold is not None:
+ thresh_mask = final_scores > self.score_threshold
+
+ if self.post_center_range is not None:
+ self.post_center_range = torch.tensor(
+ self.post_center_range, device=heat.device)
+ mask = (final_box_preds[..., :3] >=
+ self.post_center_range[:3]).all(2)
+ mask &= (final_box_preds[..., :3] <=
+ self.post_center_range[3:]).all(2)
+
+ predictions_dicts = []
+ for i in range(batch):
+ cmask = mask[i, :]
+ if self.score_threshold:
+ cmask &= thresh_mask[i]
+
+ boxes3d = final_box_preds[i, cmask]
+ scores = final_scores[i, cmask]
+ labels = final_preds[i, cmask]
+ predictions_dict = {
+ 'bboxes': boxes3d,
+ 'scores': scores,
+ 'labels': labels
+ }
+
+ predictions_dicts.append(predictions_dict)
+ else:
+ raise NotImplementedError(
+ 'Need to reorganize output as a batch, only '
+ 'support post_center_range is not None for now!')
+
+ return predictions_dicts
diff --git a/mmdet3d/core/bbox/coders/delta_xyzwhlr_bbox_coder.py b/mmdet3d/core/bbox/coders/delta_xyzwhlr_bbox_coder.py
new file mode 100644
index 0000000..931e839
--- /dev/null
+++ b/mmdet3d/core/bbox/coders/delta_xyzwhlr_bbox_coder.py
@@ -0,0 +1,91 @@
+# Copyright (c) OpenMMLab. All rights reserved.
+import torch
+
+from mmdet.core.bbox import BaseBBoxCoder
+from mmdet.core.bbox.builder import BBOX_CODERS
+
+
+@BBOX_CODERS.register_module()
+class DeltaXYZWLHRBBoxCoder(BaseBBoxCoder):
+ """Bbox Coder for 3D boxes.
+
+ Args:
+ code_size (int): The dimension of boxes to be encoded.
+ """
+
+ def __init__(self, code_size=7):
+ super(DeltaXYZWLHRBBoxCoder, self).__init__()
+ self.code_size = code_size
+
+ @staticmethod
+ def encode(src_boxes, dst_boxes):
+ """Get box regression transformation deltas (dx, dy, dz, dx_size,
+ dy_size, dz_size, dr, dv*) that can be used to transform the
+ `src_boxes` into the `target_boxes`.
+
+ Args:
+ src_boxes (torch.Tensor): source boxes, e.g., object proposals.
+ dst_boxes (torch.Tensor): target of the transformation, e.g.,
+ ground-truth boxes.
+
+ Returns:
+ torch.Tensor: Box transformation deltas.
+ """
+ box_ndim = src_boxes.shape[-1]
+ cas, cgs, cts = [], [], []
+ if box_ndim > 7:
+ xa, ya, za, wa, la, ha, ra, *cas = torch.split(
+ src_boxes, 1, dim=-1)
+ xg, yg, zg, wg, lg, hg, rg, *cgs = torch.split(
+ dst_boxes, 1, dim=-1)
+ cts = [g - a for g, a in zip(cgs, cas)]
+ else:
+ xa, ya, za, wa, la, ha, ra = torch.split(src_boxes, 1, dim=-1)
+ xg, yg, zg, wg, lg, hg, rg = torch.split(dst_boxes, 1, dim=-1)
+ za = za + ha / 2
+ zg = zg + hg / 2
+ diagonal = torch.sqrt(la**2 + wa**2)
+ xt = (xg - xa) / diagonal
+ yt = (yg - ya) / diagonal
+ zt = (zg - za) / ha
+ lt = torch.log(lg / la)
+ wt = torch.log(wg / wa)
+ ht = torch.log(hg / ha)
+ rt = rg - ra
+ return torch.cat([xt, yt, zt, wt, lt, ht, rt, *cts], dim=-1)
+
+ @staticmethod
+ def decode(anchors, deltas):
+ """Apply transformation `deltas` (dx, dy, dz, dx_size, dy_size,
+ dz_size, dr, dv*) to `boxes`.
+
+ Args:
+ anchors (torch.Tensor): Parameters of anchors with shape (N, 7).
+ deltas (torch.Tensor): Encoded boxes with shape
+ (N, 7+n) [x, y, z, x_size, y_size, z_size, r, velo*].
+
+ Returns:
+ torch.Tensor: Decoded boxes.
+ """
+ cas, cts = [], []
+ box_ndim = anchors.shape[-1]
+ if box_ndim > 7:
+ xa, ya, za, wa, la, ha, ra, *cas = torch.split(anchors, 1, dim=-1)
+ xt, yt, zt, wt, lt, ht, rt, *cts = torch.split(deltas, 1, dim=-1)
+ else:
+ xa, ya, za, wa, la, ha, ra = torch.split(anchors, 1, dim=-1)
+ xt, yt, zt, wt, lt, ht, rt = torch.split(deltas, 1, dim=-1)
+
+ za = za + ha / 2
+ diagonal = torch.sqrt(la**2 + wa**2)
+ xg = xt * diagonal + xa
+ yg = yt * diagonal + ya
+ zg = zt * ha + za
+
+ lg = torch.exp(lt) * la
+ wg = torch.exp(wt) * wa
+ hg = torch.exp(ht) * ha
+ rg = rt + ra
+ zg = zg - hg / 2
+ cgs = [t + a for t, a in zip(cts, cas)]
+ return torch.cat([xg, yg, zg, wg, lg, hg, rg, *cgs], dim=-1)
diff --git a/mmdet3d/core/bbox/coders/fcos3d_bbox_coder.py b/mmdet3d/core/bbox/coders/fcos3d_bbox_coder.py
new file mode 100644
index 0000000..7cb6b1a
--- /dev/null
+++ b/mmdet3d/core/bbox/coders/fcos3d_bbox_coder.py
@@ -0,0 +1,127 @@
+# Copyright (c) OpenMMLab. All rights reserved.
+import numpy as np
+import torch
+
+from mmdet.core.bbox import BaseBBoxCoder
+from mmdet.core.bbox.builder import BBOX_CODERS
+from ..structures import limit_period
+
+
+@BBOX_CODERS.register_module()
+class FCOS3DBBoxCoder(BaseBBoxCoder):
+ """Bounding box coder for FCOS3D.
+
+ Args:
+ base_depths (tuple[tuple[float]]): Depth references for decode box
+ depth. Defaults to None.
+ base_dims (tuple[tuple[float]]): Dimension references for decode box
+ dimension. Defaults to None.
+ code_size (int): The dimension of boxes to be encoded. Defaults to 7.
+ norm_on_bbox (bool): Whether to apply normalization on the bounding
+ box 2D attributes. Defaults to True.
+ """
+
+ def __init__(self,
+ base_depths=None,
+ base_dims=None,
+ code_size=7,
+ norm_on_bbox=True):
+ super(FCOS3DBBoxCoder, self).__init__()
+ self.base_depths = base_depths
+ self.base_dims = base_dims
+ self.bbox_code_size = code_size
+ self.norm_on_bbox = norm_on_bbox
+
+ def encode(self, gt_bboxes_3d, gt_labels_3d, gt_bboxes, gt_labels):
+ # TODO: refactor the encoder in the FCOS3D and PGD head
+ pass
+
+ def decode(self, bbox, scale, stride, training, cls_score=None):
+ """Decode regressed results into 3D predictions.
+
+ Note that offsets are not transformed to the projected 3D centers.
+
+ Args:
+ bbox (torch.Tensor): Raw bounding box predictions in shape
+ [N, C, H, W].
+ scale (tuple[`Scale`]): Learnable scale parameters.
+ stride (int): Stride for a specific feature level.
+ training (bool): Whether the decoding is in the training
+ procedure.
+ cls_score (torch.Tensor): Classification score map for deciding
+ which base depth or dim is used. Defaults to None.
+
+ Returns:
+ torch.Tensor: Decoded boxes.
+ """
+ # scale the bbox of different level
+ # only apply to offset, depth and size prediction
+ scale_offset, scale_depth, scale_size = scale[0:3]
+
+ clone_bbox = bbox.clone()
+ bbox[:, :2] = scale_offset(clone_bbox[:, :2]).float()
+ bbox[:, 2] = scale_depth(clone_bbox[:, 2]).float()
+ bbox[:, 3:6] = scale_size(clone_bbox[:, 3:6]).float()
+
+ if self.base_depths is None:
+ bbox[:, 2] = bbox[:, 2].exp()
+ elif len(self.base_depths) == 1: # only single prior
+ mean = self.base_depths[0][0]
+ std = self.base_depths[0][1]
+ bbox[:, 2] = mean + bbox.clone()[:, 2] * std
+ else: # multi-class priors
+ assert len(self.base_depths) == cls_score.shape[1], \
+ 'The number of multi-class depth priors should be equal to ' \
+ 'the number of categories.'
+ indices = cls_score.max(dim=1)[1]
+ depth_priors = cls_score.new_tensor(
+ self.base_depths)[indices, :].permute(0, 3, 1, 2)
+ mean = depth_priors[:, 0]
+ std = depth_priors[:, 1]
+ bbox[:, 2] = mean + bbox.clone()[:, 2] * std
+
+ bbox[:, 3:6] = bbox[:, 3:6].exp()
+ if self.base_dims is not None:
+ assert len(self.base_dims) == cls_score.shape[1], \
+ 'The number of anchor sizes should be equal to the number ' \
+ 'of categories.'
+ indices = cls_score.max(dim=1)[1]
+ size_priors = cls_score.new_tensor(
+ self.base_dims)[indices, :].permute(0, 3, 1, 2)
+ bbox[:, 3:6] = size_priors * bbox.clone()[:, 3:6]
+
+ assert self.norm_on_bbox is True, 'Setting norm_on_bbox to False '\
+ 'has not been thoroughly tested for FCOS3D.'
+ if self.norm_on_bbox:
+ if not training:
+ # Note that this line is conducted only when testing
+ bbox[:, :2] *= stride
+
+ return bbox
+
+ @staticmethod
+ def decode_yaw(bbox, centers2d, dir_cls, dir_offset, cam2img):
+ """Decode yaw angle and change it from local to global.i.
+
+ Args:
+ bbox (torch.Tensor): Bounding box predictions in shape
+ [N, C] with yaws to be decoded.
+ centers2d (torch.Tensor): Projected 3D-center on the image planes
+ corresponding to the box predictions.
+ dir_cls (torch.Tensor): Predicted direction classes.
+ dir_offset (float): Direction offset before dividing all the
+ directions into several classes.
+ cam2img (torch.Tensor): Camera intrinsic matrix in shape [4, 4].
+
+ Returns:
+ torch.Tensor: Bounding boxes with decoded yaws.
+ """
+ if bbox.shape[0] > 0:
+ dir_rot = limit_period(bbox[..., 6] - dir_offset, 0, np.pi)
+ bbox[..., 6] = \
+ dir_rot + dir_offset + np.pi * dir_cls.to(bbox.dtype)
+
+ bbox[:, 6] = torch.atan2(centers2d[:, 0] - cam2img[0, 2],
+ cam2img[0, 0]) + bbox[:, 6]
+
+ return bbox
diff --git a/mmdet3d/core/bbox/coders/groupfree3d_bbox_coder.py b/mmdet3d/core/bbox/coders/groupfree3d_bbox_coder.py
new file mode 100644
index 0000000..08d83e9
--- /dev/null
+++ b/mmdet3d/core/bbox/coders/groupfree3d_bbox_coder.py
@@ -0,0 +1,191 @@
+# Copyright (c) OpenMMLab. All rights reserved.
+import numpy as np
+import torch
+
+from mmdet.core.bbox.builder import BBOX_CODERS
+from .partial_bin_based_bbox_coder import PartialBinBasedBBoxCoder
+
+
+@BBOX_CODERS.register_module()
+class GroupFree3DBBoxCoder(PartialBinBasedBBoxCoder):
+ """Modified partial bin based bbox coder for GroupFree3D.
+
+ Args:
+ num_dir_bins (int): Number of bins to encode direction angle.
+ num_sizes (int): Number of size clusters.
+ mean_sizes (list[list[int]]): Mean size of bboxes in each class.
+ with_rot (bool, optional): Whether the bbox is with rotation.
+ Defaults to True.
+ size_cls_agnostic (bool, optional): Whether the predicted size is
+ class-agnostic. Defaults to True.
+ """
+
+ def __init__(self,
+ num_dir_bins,
+ num_sizes,
+ mean_sizes,
+ with_rot=True,
+ size_cls_agnostic=True):
+ super(GroupFree3DBBoxCoder, self).__init__(
+ num_dir_bins=num_dir_bins,
+ num_sizes=num_sizes,
+ mean_sizes=mean_sizes,
+ with_rot=with_rot)
+ self.size_cls_agnostic = size_cls_agnostic
+
+ def encode(self, gt_bboxes_3d, gt_labels_3d):
+ """Encode ground truth to prediction targets.
+
+ Args:
+ gt_bboxes_3d (BaseInstance3DBoxes): Ground truth bboxes
+ with shape (n, 7).
+ gt_labels_3d (torch.Tensor): Ground truth classes.
+
+ Returns:
+ tuple: Targets of center, size and direction.
+ """
+ # generate center target
+ center_target = gt_bboxes_3d.gravity_center
+
+ # generate bbox size target
+ size_target = gt_bboxes_3d.dims
+ size_class_target = gt_labels_3d
+ size_res_target = gt_bboxes_3d.dims - gt_bboxes_3d.tensor.new_tensor(
+ self.mean_sizes)[size_class_target]
+
+ # generate dir target
+ box_num = gt_labels_3d.shape[0]
+ if self.with_rot:
+ (dir_class_target,
+ dir_res_target) = self.angle2class(gt_bboxes_3d.yaw)
+ else:
+ dir_class_target = gt_labels_3d.new_zeros(box_num)
+ dir_res_target = gt_bboxes_3d.tensor.new_zeros(box_num)
+
+ return (center_target, size_target, size_class_target, size_res_target,
+ dir_class_target, dir_res_target)
+
+ def decode(self, bbox_out, prefix=''):
+ """Decode predicted parts to bbox3d.
+
+ Args:
+ bbox_out (dict): Predictions from model, should contain keys below.
+
+ - center: predicted bottom center of bboxes.
+ - dir_class: predicted bbox direction class.
+ - dir_res: predicted bbox direction residual.
+ - size_class: predicted bbox size class.
+ - size_res: predicted bbox size residual.
+ - size: predicted class-agnostic bbox size
+ prefix (str, optional): Decode predictions with specific prefix.
+ Defaults to ''.
+
+ Returns:
+ torch.Tensor: Decoded bbox3d with shape (batch, n, 7).
+ """
+ center = bbox_out[f'{prefix}center']
+ batch_size, num_proposal = center.shape[:2]
+
+ # decode heading angle
+ if self.with_rot:
+ dir_class = torch.argmax(bbox_out[f'{prefix}dir_class'], -1)
+ dir_res = torch.gather(bbox_out[f'{prefix}dir_res'], 2,
+ dir_class.unsqueeze(-1))
+ dir_res.squeeze_(2)
+ dir_angle = self.class2angle(dir_class, dir_res).reshape(
+ batch_size, num_proposal, 1)
+ else:
+ dir_angle = center.new_zeros(batch_size, num_proposal, 1)
+
+ # decode bbox size
+ if self.size_cls_agnostic:
+ bbox_size = bbox_out[f'{prefix}size'].reshape(
+ batch_size, num_proposal, 3)
+ else:
+ size_class = torch.argmax(
+ bbox_out[f'{prefix}size_class'], -1, keepdim=True)
+ size_res = torch.gather(
+ bbox_out[f'{prefix}size_res'], 2,
+ size_class.unsqueeze(-1).repeat(1, 1, 1, 3))
+ mean_sizes = center.new_tensor(self.mean_sizes)
+ size_base = torch.index_select(mean_sizes, 0,
+ size_class.reshape(-1))
+ bbox_size = size_base.reshape(batch_size, num_proposal,
+ -1) + size_res.squeeze(2)
+
+ bbox3d = torch.cat([center, bbox_size, dir_angle], dim=-1)
+ return bbox3d
+
+ def split_pred(self, cls_preds, reg_preds, base_xyz, prefix=''):
+ """Split predicted features to specific parts.
+
+ Args:
+ cls_preds (torch.Tensor): Class predicted features to split.
+ reg_preds (torch.Tensor): Regression predicted features to split.
+ base_xyz (torch.Tensor): Coordinates of points.
+ prefix (str, optional): Decode predictions with specific prefix.
+ Defaults to ''.
+
+ Returns:
+ dict[str, torch.Tensor]: Split results.
+ """
+ results = {}
+ start, end = 0, 0
+
+ cls_preds_trans = cls_preds.transpose(2, 1)
+ reg_preds_trans = reg_preds.transpose(2, 1)
+
+ # decode center
+ end += 3
+ # (batch_size, num_proposal, 3)
+ results[f'{prefix}center_residual'] = \
+ reg_preds_trans[..., start:end].contiguous()
+ results[f'{prefix}center'] = base_xyz + \
+ reg_preds_trans[..., start:end].contiguous()
+ start = end
+
+ # decode direction
+ end += self.num_dir_bins
+ results[f'{prefix}dir_class'] = \
+ reg_preds_trans[..., start:end].contiguous()
+ start = end
+
+ end += self.num_dir_bins
+ dir_res_norm = reg_preds_trans[..., start:end].contiguous()
+ start = end
+
+ results[f'{prefix}dir_res_norm'] = dir_res_norm
+ results[f'{prefix}dir_res'] = dir_res_norm * (
+ np.pi / self.num_dir_bins)
+
+ # decode size
+ if self.size_cls_agnostic:
+ end += 3
+ results[f'{prefix}size'] = \
+ reg_preds_trans[..., start:end].contiguous()
+ else:
+ end += self.num_sizes
+ results[f'{prefix}size_class'] = reg_preds_trans[
+ ..., start:end].contiguous()
+ start = end
+
+ end += self.num_sizes * 3
+ size_res_norm = reg_preds_trans[..., start:end]
+ batch_size, num_proposal = reg_preds_trans.shape[:2]
+ size_res_norm = size_res_norm.view(
+ [batch_size, num_proposal, self.num_sizes, 3])
+ start = end
+
+ results[f'{prefix}size_res_norm'] = size_res_norm.contiguous()
+ mean_sizes = reg_preds.new_tensor(self.mean_sizes)
+ results[f'{prefix}size_res'] = (
+ size_res_norm * mean_sizes.unsqueeze(0).unsqueeze(0))
+
+ # decode objectness score
+ # Group-Free-3D objectness output shape (batch, proposal, 1)
+ results[f'{prefix}obj_scores'] = cls_preds_trans[..., :1].contiguous()
+
+ # decode semantic score
+ results[f'{prefix}sem_scores'] = cls_preds_trans[..., 1:].contiguous()
+
+ return results
diff --git a/mmdet3d/core/bbox/coders/monoflex_bbox_coder.py b/mmdet3d/core/bbox/coders/monoflex_bbox_coder.py
new file mode 100644
index 0000000..e2ada29
--- /dev/null
+++ b/mmdet3d/core/bbox/coders/monoflex_bbox_coder.py
@@ -0,0 +1,515 @@
+# Copyright (c) OpenMMLab. All rights reserved.
+import numpy as np
+import torch
+from torch.nn import functional as F
+
+from mmdet.core.bbox import BaseBBoxCoder
+from mmdet.core.bbox.builder import BBOX_CODERS
+
+
+@BBOX_CODERS.register_module()
+class MonoFlexCoder(BaseBBoxCoder):
+ """Bbox Coder for MonoFlex.
+
+ Args:
+ depth_mode (str): The mode for depth calculation.
+ Available options are "linear", "inv_sigmoid", and "exp".
+ base_depth (tuple[float]): References for decoding box depth.
+ depth_range (list): Depth range of predicted depth.
+ combine_depth (bool): Whether to use combined depth (direct depth
+ and depth from keypoints) or use direct depth only.
+ uncertainty_range (list): Uncertainty range of predicted depth.
+ base_dims (tuple[tuple[float]]): Dimensions mean and std of decode bbox
+ dimensions [l, h, w] for each category.
+ dims_mode (str): The mode for dimension calculation.
+ Available options are "linear" and "exp".
+ multibin (bool): Whether to use multibin representation.
+ num_dir_bins (int): Number of Number of bins to encode
+ direction angle.
+ bin_centers (list[float]): Local yaw centers while using multibin
+ representations.
+ bin_margin (float): Margin of multibin representations.
+ code_size (int): The dimension of boxes to be encoded.
+ eps (float, optional): A value added to the denominator for numerical
+ stability. Default 1e-3.
+ """
+
+ def __init__(self,
+ depth_mode,
+ base_depth,
+ depth_range,
+ combine_depth,
+ uncertainty_range,
+ base_dims,
+ dims_mode,
+ multibin,
+ num_dir_bins,
+ bin_centers,
+ bin_margin,
+ code_size,
+ eps=1e-3):
+ super(MonoFlexCoder, self).__init__()
+
+ # depth related
+ self.depth_mode = depth_mode
+ self.base_depth = base_depth
+ self.depth_range = depth_range
+ self.combine_depth = combine_depth
+ self.uncertainty_range = uncertainty_range
+
+ # dimensions related
+ self.base_dims = base_dims
+ self.dims_mode = dims_mode
+
+ # orientation related
+ self.multibin = multibin
+ self.num_dir_bins = num_dir_bins
+ self.bin_centers = bin_centers
+ self.bin_margin = bin_margin
+
+ # output related
+ self.bbox_code_size = code_size
+ self.eps = eps
+
+ def encode(self, gt_bboxes_3d):
+ """Encode ground truth to prediction targets.
+
+ Args:
+ gt_bboxes_3d (`BaseInstance3DBoxes`): Ground truth 3D bboxes.
+ shape: (N, 7).
+
+ Returns:
+ torch.Tensor: Targets of orientations.
+ """
+ local_yaw = gt_bboxes_3d.local_yaw
+ # encode local yaw (-pi ~ pi) to multibin format
+ encode_local_yaw = local_yaw.new_zeros(
+ [local_yaw.shape[0], self.num_dir_bins * 2])
+ bin_size = 2 * np.pi / self.num_dir_bins
+ margin_size = bin_size * self.bin_margin
+
+ bin_centers = local_yaw.new_tensor(self.bin_centers)
+ range_size = bin_size / 2 + margin_size
+
+ offsets = local_yaw.unsqueeze(1) - bin_centers.unsqueeze(0)
+ offsets[offsets > np.pi] = offsets[offsets > np.pi] - 2 * np.pi
+ offsets[offsets < -np.pi] = offsets[offsets < -np.pi] + 2 * np.pi
+
+ for i in range(self.num_dir_bins):
+ offset = offsets[:, i]
+ inds = abs(offset) < range_size
+ encode_local_yaw[inds, i] = 1
+ encode_local_yaw[inds, i + self.num_dir_bins] = offset[inds]
+
+ orientation_target = encode_local_yaw
+
+ return orientation_target
+
+ def decode(self, bbox, base_centers2d, labels, downsample_ratio, cam2imgs):
+ """Decode bounding box regression into 3D predictions.
+
+ Args:
+ bbox (Tensor): Raw bounding box predictions for each
+ predict center2d point.
+ shape: (N, C)
+ base_centers2d (torch.Tensor): Base centers2d for 3D bboxes.
+ shape: (N, 2).
+ labels (Tensor): Batch predict class label for each predict
+ center2d point.
+ shape: (N, )
+ downsample_ratio (int): The stride of feature map.
+ cam2imgs (Tensor): Batch images' camera intrinsic matrix.
+ shape: kitti (N, 4, 4) nuscenes (N, 3, 3)
+
+ Return:
+ dict: The 3D prediction dict decoded from regression map.
+ the dict has components below:
+ - bboxes2d (torch.Tensor): Decoded [x1, y1, x2, y2] format
+ 2D bboxes.
+ - dimensions (torch.Tensor): Decoded dimensions for each
+ object.
+ - offsets2d (torch.Tenosr): Offsets between base centers2d
+ and real centers2d.
+ - direct_depth (torch.Tensor): Decoded directly regressed
+ depth.
+ - keypoints2d (torch.Tensor): Keypoints of each projected
+ 3D box on image.
+ - keypoints_depth (torch.Tensor): Decoded depth from keypoints.
+ - combined_depth (torch.Tensor): Combined depth using direct
+ depth and keypoints depth with depth uncertainty.
+ - orientations (torch.Tensor): Multibin format orientations
+ (local yaw) for each objects.
+ """
+
+ # 4 dimensions for FCOS style regression
+ pred_bboxes2d = bbox[:, 0:4]
+
+ # change FCOS style to [x1, y1, x2, y2] format for IOU Loss
+ pred_bboxes2d = self.decode_bboxes2d(pred_bboxes2d, base_centers2d)
+
+ # 2 dimensions for projected centers2d offsets
+ pred_offsets2d = bbox[:, 4:6]
+
+ # 3 dimensions for 3D bbox dimensions offsets
+ pred_dimensions_offsets3d = bbox[:, 29:32]
+
+ # the first 8 dimensions are for orientation bin classification
+ # and the second 8 dimensions are for orientation offsets.
+ pred_orientations = torch.cat((bbox[:, 32:40], bbox[:, 40:48]), dim=1)
+
+ # 3 dimensions for the uncertainties of the solved depths from
+ # groups of keypoints
+ pred_keypoints_depth_uncertainty = bbox[:, 26:29]
+
+ # 1 dimension for the uncertainty of directly regressed depth
+ pred_direct_depth_uncertainty = bbox[:, 49:50].squeeze(-1)
+
+ # 2 dimension of offsets x keypoints (8 corners + top/bottom center)
+ pred_keypoints2d = bbox[:, 6:26].reshape(-1, 10, 2)
+
+ # 1 dimension for depth offsets
+ pred_direct_depth_offsets = bbox[:, 48:49].squeeze(-1)
+
+ # decode the pred residual dimensions to real dimensions
+ pred_dimensions = self.decode_dims(labels, pred_dimensions_offsets3d)
+ pred_direct_depth = self.decode_direct_depth(pred_direct_depth_offsets)
+ pred_keypoints_depth = self.keypoints2depth(pred_keypoints2d,
+ pred_dimensions, cam2imgs,
+ downsample_ratio)
+
+ pred_direct_depth_uncertainty = torch.clamp(
+ pred_direct_depth_uncertainty, self.uncertainty_range[0],
+ self.uncertainty_range[1])
+ pred_keypoints_depth_uncertainty = torch.clamp(
+ pred_keypoints_depth_uncertainty, self.uncertainty_range[0],
+ self.uncertainty_range[1])
+
+ if self.combine_depth:
+ pred_depth_uncertainty = torch.cat(
+ (pred_direct_depth_uncertainty.unsqueeze(-1),
+ pred_keypoints_depth_uncertainty),
+ dim=1).exp()
+ pred_depth = torch.cat(
+ (pred_direct_depth.unsqueeze(-1), pred_keypoints_depth), dim=1)
+ pred_combined_depth = \
+ self.combine_depths(pred_depth, pred_depth_uncertainty)
+ else:
+ pred_combined_depth = None
+
+ preds = dict(
+ bboxes2d=pred_bboxes2d,
+ dimensions=pred_dimensions,
+ offsets2d=pred_offsets2d,
+ keypoints2d=pred_keypoints2d,
+ orientations=pred_orientations,
+ direct_depth=pred_direct_depth,
+ keypoints_depth=pred_keypoints_depth,
+ combined_depth=pred_combined_depth,
+ direct_depth_uncertainty=pred_direct_depth_uncertainty,
+ keypoints_depth_uncertainty=pred_keypoints_depth_uncertainty,
+ )
+
+ return preds
+
+ def decode_direct_depth(self, depth_offsets):
+ """Transform depth offset to directly regressed depth.
+
+ Args:
+ depth_offsets (torch.Tensor): Predicted depth offsets.
+ shape: (N, )
+
+ Return:
+ torch.Tensor: Directly regressed depth.
+ shape: (N, )
+ """
+ if self.depth_mode == 'exp':
+ direct_depth = depth_offsets.exp()
+ elif self.depth_mode == 'linear':
+ base_depth = depth_offsets.new_tensor(self.base_depth)
+ direct_depth = depth_offsets * base_depth[1] + base_depth[0]
+ elif self.depth_mode == 'inv_sigmoid':
+ direct_depth = 1 / torch.sigmoid(depth_offsets) - 1
+ else:
+ raise ValueError
+
+ if self.depth_range is not None:
+ direct_depth = torch.clamp(
+ direct_depth, min=self.depth_range[0], max=self.depth_range[1])
+
+ return direct_depth
+
+ def decode_location(self,
+ base_centers2d,
+ offsets2d,
+ depths,
+ cam2imgs,
+ downsample_ratio,
+ pad_mode='default'):
+ """Retrieve object location.
+
+ Args:
+ base_centers2d (torch.Tensor): predicted base centers2d.
+ shape: (N, 2)
+ offsets2d (torch.Tensor): The offsets between real centers2d
+ and base centers2d.
+ shape: (N , 2)
+ depths (torch.Tensor): Depths of objects.
+ shape: (N, )
+ cam2imgs (torch.Tensor): Batch images' camera intrinsic matrix.
+ shape: kitti (N, 4, 4) nuscenes (N, 3, 3)
+ downsample_ratio (int): The stride of feature map.
+ pad_mode (str, optional): Padding mode used in
+ training data augmentation.
+
+ Return:
+ tuple(torch.Tensor): Centers of 3D boxes.
+ shape: (N, 3)
+ """
+ N = cam2imgs.shape[0]
+ # (N, 4, 4)
+ cam2imgs_inv = cam2imgs.inverse()
+ if pad_mode == 'default':
+ centers2d_img = (base_centers2d + offsets2d) * downsample_ratio
+ else:
+ raise NotImplementedError
+ # (N, 3)
+ centers2d_img = \
+ torch.cat((centers2d_img, depths.unsqueeze(-1)), dim=1)
+ # (N, 4, 1)
+ centers2d_extend = \
+ torch.cat((centers2d_img, centers2d_img.new_ones(N, 1)),
+ dim=1).unsqueeze(-1)
+ locations = torch.matmul(cam2imgs_inv, centers2d_extend).squeeze(-1)
+
+ return locations[:, :3]
+
+ def keypoints2depth(self,
+ keypoints2d,
+ dimensions,
+ cam2imgs,
+ downsample_ratio=4,
+ group0_index=[(7, 3), (0, 4)],
+ group1_index=[(2, 6), (1, 5)]):
+ """Decode depth form three groups of keypoints and geometry projection
+ model. 2D keypoints inlucding 8 coreners and top/bottom centers will be
+ divided into three groups which will be used to calculate three depths
+ of object.
+
+ .. code-block:: none
+
+ Group center keypoints:
+
+ + --------------- +
+ /| top center /|
+ / | . / |
+ / | | / |
+ + ---------|----- + +
+ | / | | /
+ | / . | /
+ |/ bottom center |/
+ + --------------- +
+
+ Group 0 keypoints:
+
+ 0
+ + -------------- +
+ /| /|
+ / | / |
+ / | 5/ |
+ + -------------- + +
+ | /3 | /
+ | / | /
+ |/ |/
+ + -------------- + 6
+
+ Group 1 keypoints:
+
+ 4
+ + -------------- +
+ /| /|
+ / | / |
+ / | / |
+ 1 + -------------- + + 7
+ | / | /
+ | / | /
+ |/ |/
+ 2 + -------------- +
+
+
+ Args:
+ keypoints2d (torch.Tensor): Keypoints of objects.
+ 8 vertices + top/bottom center.
+ shape: (N, 10, 2)
+ dimensions (torch.Tensor): Dimensions of objetcts.
+ shape: (N, 3)
+ cam2imgs (torch.Tensor): Batch images' camera intrinsic matrix.
+ shape: kitti (N, 4, 4) nuscenes (N, 3, 3)
+ downsample_ratio (int, opitonal): The stride of feature map.
+ Defaults: 4.
+ group0_index(list[tuple[int]], optional): Keypoints group 0
+ of index to calculate the depth.
+ Defaults: [0, 3, 4, 7].
+ group1_index(list[tuple[int]], optional): Keypoints group 1
+ of index to calculate the depth.
+ Defaults: [1, 2, 5, 6]
+
+ Return:
+ tuple(torch.Tensor): Depth computed from three groups of
+ keypoints (top/bottom, group0, group1)
+ shape: (N, 3)
+ """
+
+ pred_height_3d = dimensions[:, 1].clone()
+ f_u = cam2imgs[:, 0, 0]
+ center_height = keypoints2d[:, -2, 1] - keypoints2d[:, -1, 1]
+ corner_group0_height = keypoints2d[:, group0_index[0], 1] \
+ - keypoints2d[:, group0_index[1], 1]
+ corner_group1_height = keypoints2d[:, group1_index[0], 1] \
+ - keypoints2d[:, group1_index[1], 1]
+ center_depth = f_u * pred_height_3d / (
+ F.relu(center_height) * downsample_ratio + self.eps)
+ corner_group0_depth = (f_u * pred_height_3d).unsqueeze(-1) / (
+ F.relu(corner_group0_height) * downsample_ratio + self.eps)
+ corner_group1_depth = (f_u * pred_height_3d).unsqueeze(-1) / (
+ F.relu(corner_group1_height) * downsample_ratio + self.eps)
+
+ corner_group0_depth = corner_group0_depth.mean(dim=1)
+ corner_group1_depth = corner_group1_depth.mean(dim=1)
+
+ keypoints_depth = torch.stack(
+ (center_depth, corner_group0_depth, corner_group1_depth), dim=1)
+ keypoints_depth = torch.clamp(
+ keypoints_depth, min=self.depth_range[0], max=self.depth_range[1])
+
+ return keypoints_depth
+
+ def decode_dims(self, labels, dims_offset):
+ """Retrieve object dimensions.
+
+ Args:
+ labels (torch.Tensor): Each points' category id.
+ shape: (N, K)
+ dims_offset (torch.Tensor): Dimension offsets.
+ shape: (N, 3)
+
+ Returns:
+ torch.Tensor: Shape (N, 3)
+ """
+
+ if self.dims_mode == 'exp':
+ dims_offset = dims_offset.exp()
+ elif self.dims_mode == 'linear':
+ labels = labels.long()
+ base_dims = dims_offset.new_tensor(self.base_dims)
+ dims_mean = base_dims[:, :3]
+ dims_std = base_dims[:, 3:6]
+ cls_dimension_mean = dims_mean[labels, :]
+ cls_dimension_std = dims_std[labels, :]
+ dimensions = dims_offset * cls_dimension_mean + cls_dimension_std
+ else:
+ raise ValueError
+
+ return dimensions
+
+ def decode_orientation(self, ori_vector, locations):
+ """Retrieve object orientation.
+
+ Args:
+ ori_vector (torch.Tensor): Local orientation vector
+ in [axis_cls, head_cls, sin, cos] format.
+ shape: (N, num_dir_bins * 4)
+ locations (torch.Tensor): Object location.
+ shape: (N, 3)
+
+ Returns:
+ tuple[torch.Tensor]: yaws and local yaws of 3d bboxes.
+ """
+ if self.multibin:
+ pred_bin_cls = ori_vector[:, :self.num_dir_bins * 2].view(
+ -1, self.num_dir_bins, 2)
+ pred_bin_cls = pred_bin_cls.softmax(dim=2)[..., 1]
+ orientations = ori_vector.new_zeros(ori_vector.shape[0])
+ for i in range(self.num_dir_bins):
+ mask_i = (pred_bin_cls.argmax(dim=1) == i)
+ start_bin = self.num_dir_bins * 2 + i * 2
+ end_bin = start_bin + 2
+ pred_bin_offset = ori_vector[mask_i, start_bin:end_bin]
+ orientations[mask_i] = pred_bin_offset[:, 0].atan2(
+ pred_bin_offset[:, 1]) + self.bin_centers[i]
+ else:
+ axis_cls = ori_vector[:, :2].softmax(dim=1)
+ axis_cls = axis_cls[:, 0] < axis_cls[:, 1]
+ head_cls = ori_vector[:, 2:4].softmax(dim=1)
+ head_cls = head_cls[:, 0] < head_cls[:, 1]
+ # cls axis
+ orientations = self.bin_centers[axis_cls + head_cls * 2]
+ sin_cos_offset = F.normalize(ori_vector[:, 4:])
+ orientations += sin_cos_offset[:, 0].atan(sin_cos_offset[:, 1])
+
+ locations = locations.view(-1, 3)
+ rays = locations[:, 0].atan2(locations[:, 2])
+ local_yaws = orientations
+ yaws = local_yaws + rays
+
+ larger_idx = (yaws > np.pi).nonzero(as_tuple=False)
+ small_idx = (yaws < -np.pi).nonzero(as_tuple=False)
+ if len(larger_idx) != 0:
+ yaws[larger_idx] -= 2 * np.pi
+ if len(small_idx) != 0:
+ yaws[small_idx] += 2 * np.pi
+
+ larger_idx = (local_yaws > np.pi).nonzero(as_tuple=False)
+ small_idx = (local_yaws < -np.pi).nonzero(as_tuple=False)
+ if len(larger_idx) != 0:
+ local_yaws[larger_idx] -= 2 * np.pi
+ if len(small_idx) != 0:
+ local_yaws[small_idx] += 2 * np.pi
+
+ return yaws, local_yaws
+
+ def decode_bboxes2d(self, reg_bboxes2d, base_centers2d):
+ """Retrieve [x1, y1, x2, y2] format 2D bboxes.
+
+ Args:
+ reg_bboxes2d (torch.Tensor): Predicted FCOS style
+ 2D bboxes.
+ shape: (N, 4)
+ base_centers2d (torch.Tensor): predicted base centers2d.
+ shape: (N, 2)
+
+ Returns:
+ torch.Tenosr: [x1, y1, x2, y2] format 2D bboxes.
+ """
+ centers_x = base_centers2d[:, 0]
+ centers_y = base_centers2d[:, 1]
+
+ xs_min = centers_x - reg_bboxes2d[..., 0]
+ ys_min = centers_y - reg_bboxes2d[..., 1]
+ xs_max = centers_x + reg_bboxes2d[..., 2]
+ ys_max = centers_y + reg_bboxes2d[..., 3]
+
+ bboxes2d = torch.stack([xs_min, ys_min, xs_max, ys_max], dim=-1)
+
+ return bboxes2d
+
+ def combine_depths(self, depth, depth_uncertainty):
+ """Combine all the prediced depths with depth uncertainty.
+
+ Args:
+ depth (torch.Tensor): Predicted depths of each object.
+ 2D bboxes.
+ shape: (N, 4)
+ depth_uncertainty (torch.Tensor): Depth uncertainty for
+ each depth of each object.
+ shape: (N, 4)
+
+ Returns:
+ torch.Tenosr: combined depth.
+ """
+ uncertainty_weights = 1 / depth_uncertainty
+ uncertainty_weights = \
+ uncertainty_weights / \
+ uncertainty_weights.sum(dim=1, keepdim=True)
+ combined_depth = torch.sum(depth * uncertainty_weights, dim=1)
+
+ return combined_depth
diff --git a/mmdet3d/core/bbox/coders/partial_bin_based_bbox_coder.py b/mmdet3d/core/bbox/coders/partial_bin_based_bbox_coder.py
new file mode 100644
index 0000000..ed8020d
--- /dev/null
+++ b/mmdet3d/core/bbox/coders/partial_bin_based_bbox_coder.py
@@ -0,0 +1,241 @@
+# Copyright (c) OpenMMLab. All rights reserved.
+import numpy as np
+import torch
+
+from mmdet.core.bbox import BaseBBoxCoder
+from mmdet.core.bbox.builder import BBOX_CODERS
+
+
+@BBOX_CODERS.register_module()
+class PartialBinBasedBBoxCoder(BaseBBoxCoder):
+ """Partial bin based bbox coder.
+
+ Args:
+ num_dir_bins (int): Number of bins to encode direction angle.
+ num_sizes (int): Number of size clusters.
+ mean_sizes (list[list[int]]): Mean size of bboxes in each class.
+ with_rot (bool): Whether the bbox is with rotation.
+ """
+
+ def __init__(self, num_dir_bins, num_sizes, mean_sizes, with_rot=True):
+ super(PartialBinBasedBBoxCoder, self).__init__()
+ assert len(mean_sizes) == num_sizes
+ self.num_dir_bins = num_dir_bins
+ self.num_sizes = num_sizes
+ self.mean_sizes = mean_sizes
+ self.with_rot = with_rot
+
+ def encode(self, gt_bboxes_3d, gt_labels_3d):
+ """Encode ground truth to prediction targets.
+
+ Args:
+ gt_bboxes_3d (BaseInstance3DBoxes): Ground truth bboxes
+ with shape (n, 7).
+ gt_labels_3d (torch.Tensor): Ground truth classes.
+
+ Returns:
+ tuple: Targets of center, size and direction.
+ """
+ # generate center target
+ center_target = gt_bboxes_3d.gravity_center
+
+ # generate bbox size target
+ size_class_target = gt_labels_3d
+ size_res_target = gt_bboxes_3d.dims - gt_bboxes_3d.tensor.new_tensor(
+ self.mean_sizes)[size_class_target]
+
+ # generate dir target
+ box_num = gt_labels_3d.shape[0]
+ if self.with_rot:
+ (dir_class_target,
+ dir_res_target) = self.angle2class(gt_bboxes_3d.yaw)
+ else:
+ dir_class_target = gt_labels_3d.new_zeros(box_num)
+ dir_res_target = gt_bboxes_3d.tensor.new_zeros(box_num)
+
+ return (center_target, size_class_target, size_res_target,
+ dir_class_target, dir_res_target)
+
+ def decode(self, bbox_out, suffix=''):
+ """Decode predicted parts to bbox3d.
+
+ Args:
+ bbox_out (dict): Predictions from model, should contain keys below.
+
+ - center: predicted bottom center of bboxes.
+ - dir_class: predicted bbox direction class.
+ - dir_res: predicted bbox direction residual.
+ - size_class: predicted bbox size class.
+ - size_res: predicted bbox size residual.
+ suffix (str): Decode predictions with specific suffix.
+
+ Returns:
+ torch.Tensor: Decoded bbox3d with shape (batch, n, 7).
+ """
+ center = bbox_out['center' + suffix]
+ batch_size, num_proposal = center.shape[:2]
+
+ # decode heading angle
+ if self.with_rot:
+ dir_class = torch.argmax(bbox_out['dir_class' + suffix], -1)
+ dir_res = torch.gather(bbox_out['dir_res' + suffix], 2,
+ dir_class.unsqueeze(-1))
+ dir_res.squeeze_(2)
+ dir_angle = self.class2angle(dir_class, dir_res).reshape(
+ batch_size, num_proposal, 1)
+ else:
+ dir_angle = center.new_zeros(batch_size, num_proposal, 1)
+
+ # decode bbox size
+ size_class = torch.argmax(
+ bbox_out['size_class' + suffix], -1, keepdim=True)
+ size_res = torch.gather(bbox_out['size_res' + suffix], 2,
+ size_class.unsqueeze(-1).repeat(1, 1, 1, 3))
+ mean_sizes = center.new_tensor(self.mean_sizes)
+ size_base = torch.index_select(mean_sizes, 0, size_class.reshape(-1))
+ bbox_size = size_base.reshape(batch_size, num_proposal,
+ -1) + size_res.squeeze(2)
+
+ bbox3d = torch.cat([center, bbox_size, dir_angle], dim=-1)
+ return bbox3d
+
+ def decode_corners(self, center, size_res, size_class):
+ """Decode center, size residuals and class to corners. Only useful for
+ axis-aligned bounding boxes, so angle isn't considered.
+
+ Args:
+ center (torch.Tensor): Shape [B, N, 3]
+ size_res (torch.Tensor): Shape [B, N, 3] or [B, N, C, 3]
+ size_class (torch.Tensor): Shape: [B, N] or [B, N, 1]
+ or [B, N, C, 3]
+
+ Returns:
+ torch.Tensor: Corners with shape [B, N, 6]
+ """
+ if len(size_class.shape) == 2 or size_class.shape[-1] == 1:
+ batch_size, proposal_num = size_class.shape[:2]
+ one_hot_size_class = size_res.new_zeros(
+ (batch_size, proposal_num, self.num_sizes))
+ if len(size_class.shape) == 2:
+ size_class = size_class.unsqueeze(-1)
+ one_hot_size_class.scatter_(2, size_class, 1)
+ one_hot_size_class_expand = one_hot_size_class.unsqueeze(
+ -1).repeat(1, 1, 1, 3).contiguous()
+ else:
+ one_hot_size_class_expand = size_class
+
+ if len(size_res.shape) == 4:
+ size_res = torch.sum(size_res * one_hot_size_class_expand, 2)
+
+ mean_sizes = size_res.new_tensor(self.mean_sizes)
+ mean_sizes = torch.sum(mean_sizes * one_hot_size_class_expand, 2)
+ size_full = (size_res + 1) * mean_sizes
+ size_full = torch.clamp(size_full, 0)
+ half_size_full = size_full / 2
+ corner1 = center - half_size_full
+ corner2 = center + half_size_full
+ corners = torch.cat([corner1, corner2], dim=-1)
+ return corners
+
+ def split_pred(self, cls_preds, reg_preds, base_xyz):
+ """Split predicted features to specific parts.
+
+ Args:
+ cls_preds (torch.Tensor): Class predicted features to split.
+ reg_preds (torch.Tensor): Regression predicted features to split.
+ base_xyz (torch.Tensor): Coordinates of points.
+
+ Returns:
+ dict[str, torch.Tensor]: Split results.
+ """
+ results = {}
+ start, end = 0, 0
+
+ cls_preds_trans = cls_preds.transpose(2, 1)
+ reg_preds_trans = reg_preds.transpose(2, 1)
+
+ # decode center
+ end += 3
+ # (batch_size, num_proposal, 3)
+ results['center'] = base_xyz + \
+ reg_preds_trans[..., start:end].contiguous()
+ start = end
+
+ # decode direction
+ end += self.num_dir_bins
+ results['dir_class'] = reg_preds_trans[..., start:end].contiguous()
+ start = end
+
+ end += self.num_dir_bins
+ dir_res_norm = reg_preds_trans[..., start:end].contiguous()
+ start = end
+
+ results['dir_res_norm'] = dir_res_norm
+ results['dir_res'] = dir_res_norm * (np.pi / self.num_dir_bins)
+
+ # decode size
+ end += self.num_sizes
+ results['size_class'] = reg_preds_trans[..., start:end].contiguous()
+ start = end
+
+ end += self.num_sizes * 3
+ size_res_norm = reg_preds_trans[..., start:end]
+ batch_size, num_proposal = reg_preds_trans.shape[:2]
+ size_res_norm = size_res_norm.view(
+ [batch_size, num_proposal, self.num_sizes, 3])
+ start = end
+
+ results['size_res_norm'] = size_res_norm.contiguous()
+ mean_sizes = reg_preds.new_tensor(self.mean_sizes)
+ results['size_res'] = (
+ size_res_norm * mean_sizes.unsqueeze(0).unsqueeze(0))
+
+ # decode objectness score
+ start = 0
+ end = 2
+ results['obj_scores'] = cls_preds_trans[..., start:end].contiguous()
+ start = end
+
+ # decode semantic score
+ results['sem_scores'] = cls_preds_trans[..., start:].contiguous()
+
+ return results
+
+ def angle2class(self, angle):
+ """Convert continuous angle to a discrete class and a residual.
+
+ Convert continuous angle to a discrete class and a small
+ regression number from class center angle to current angle.
+
+ Args:
+ angle (torch.Tensor): Angle is from 0-2pi (or -pi~pi),
+ class center at 0, 1*(2pi/N), 2*(2pi/N) ... (N-1)*(2pi/N).
+
+ Returns:
+ tuple: Encoded discrete class and residual.
+ """
+ angle = angle % (2 * np.pi)
+ angle_per_class = 2 * np.pi / float(self.num_dir_bins)
+ shifted_angle = (angle + angle_per_class / 2) % (2 * np.pi)
+ angle_cls = shifted_angle // angle_per_class
+ angle_res = shifted_angle - (
+ angle_cls * angle_per_class + angle_per_class / 2)
+ return angle_cls.long(), angle_res
+
+ def class2angle(self, angle_cls, angle_res, limit_period=True):
+ """Inverse function to angle2class.
+
+ Args:
+ angle_cls (torch.Tensor): Angle class to decode.
+ angle_res (torch.Tensor): Angle residual to decode.
+ limit_period (bool): Whether to limit angle to [-pi, pi].
+
+ Returns:
+ torch.Tensor: Angle decoded from angle_cls and angle_res.
+ """
+ angle_per_class = 2 * np.pi / float(self.num_dir_bins)
+ angle_center = angle_cls.float() * angle_per_class
+ angle = angle_center + angle_res
+ if limit_period:
+ angle[angle > np.pi] -= 2 * np.pi
+ return angle
diff --git a/mmdet3d/core/bbox/coders/pgd_bbox_coder.py b/mmdet3d/core/bbox/coders/pgd_bbox_coder.py
new file mode 100644
index 0000000..094ed39
--- /dev/null
+++ b/mmdet3d/core/bbox/coders/pgd_bbox_coder.py
@@ -0,0 +1,128 @@
+# Copyright (c) OpenMMLab. All rights reserved.
+import numpy as np
+import torch
+from torch.nn import functional as F
+
+from mmdet.core.bbox.builder import BBOX_CODERS
+from .fcos3d_bbox_coder import FCOS3DBBoxCoder
+
+
+@BBOX_CODERS.register_module()
+class PGDBBoxCoder(FCOS3DBBoxCoder):
+ """Bounding box coder for PGD."""
+
+ def encode(self, gt_bboxes_3d, gt_labels_3d, gt_bboxes, gt_labels):
+ # TODO: refactor the encoder codes in the FCOS3D and PGD head
+ pass
+
+ def decode_2d(self,
+ bbox,
+ scale,
+ stride,
+ max_regress_range,
+ training,
+ pred_keypoints=False,
+ pred_bbox2d=True):
+ """Decode regressed 2D attributes.
+
+ Args:
+ bbox (torch.Tensor): Raw bounding box predictions in shape
+ [N, C, H, W].
+ scale (tuple[`Scale`]): Learnable scale parameters.
+ stride (int): Stride for a specific feature level.
+ max_regress_range (int): Maximum regression range for a specific
+ feature level.
+ training (bool): Whether the decoding is in the training
+ procedure.
+ pred_keypoints (bool, optional): Whether to predict keypoints.
+ Defaults to False.
+ pred_bbox2d (bool, optional): Whether to predict 2D bounding
+ boxes. Defaults to False.
+
+ Returns:
+ torch.Tensor: Decoded boxes.
+ """
+ clone_bbox = bbox.clone()
+ if pred_keypoints:
+ scale_kpts = scale[3]
+ # 2 dimension of offsets x 8 corners of a 3D bbox
+ bbox[:, self.bbox_code_size:self.bbox_code_size + 16] = \
+ torch.tanh(scale_kpts(clone_bbox[
+ :, self.bbox_code_size:self.bbox_code_size + 16]).float())
+
+ if pred_bbox2d:
+ scale_bbox2d = scale[-1]
+ # The last four dimensions are offsets to four sides of a 2D bbox
+ bbox[:, -4:] = scale_bbox2d(clone_bbox[:, -4:]).float()
+
+ if self.norm_on_bbox:
+ if pred_bbox2d:
+ bbox[:, -4:] = F.relu(bbox.clone()[:, -4:])
+ if not training:
+ if pred_keypoints:
+ bbox[
+ :, self.bbox_code_size:self.bbox_code_size + 16] *= \
+ max_regress_range
+ if pred_bbox2d:
+ bbox[:, -4:] *= stride
+ else:
+ if pred_bbox2d:
+ bbox[:, -4:] = bbox.clone()[:, -4:].exp()
+ return bbox
+
+ def decode_prob_depth(self, depth_cls_preds, depth_range, depth_unit,
+ division, num_depth_cls):
+ """Decode probabilistic depth map.
+
+ Args:
+ depth_cls_preds (torch.Tensor): Depth probabilistic map in shape
+ [..., self.num_depth_cls] (raw output before softmax).
+ depth_range (tuple[float]): Range of depth estimation.
+ depth_unit (int): Unit of depth range division.
+ division (str): Depth division method. Options include 'uniform',
+ 'linear', 'log', 'loguniform'.
+ num_depth_cls (int): Number of depth classes.
+
+ Returns:
+ torch.Tensor: Decoded probabilistic depth estimation.
+ """
+ if division == 'uniform':
+ depth_multiplier = depth_unit * \
+ depth_cls_preds.new_tensor(
+ list(range(num_depth_cls))).reshape([1, -1])
+ prob_depth_preds = (F.softmax(depth_cls_preds.clone(), dim=-1) *
+ depth_multiplier).sum(dim=-1)
+ return prob_depth_preds
+ elif division == 'linear':
+ split_pts = depth_cls_preds.new_tensor(list(
+ range(num_depth_cls))).reshape([1, -1])
+ depth_multiplier = depth_range[0] + (
+ depth_range[1] - depth_range[0]) / \
+ (num_depth_cls * (num_depth_cls - 1)) * \
+ (split_pts * (split_pts+1))
+ prob_depth_preds = (F.softmax(depth_cls_preds.clone(), dim=-1) *
+ depth_multiplier).sum(dim=-1)
+ return prob_depth_preds
+ elif division == 'log':
+ split_pts = depth_cls_preds.new_tensor(list(
+ range(num_depth_cls))).reshape([1, -1])
+ start = max(depth_range[0], 1)
+ end = depth_range[1]
+ depth_multiplier = (np.log(start) +
+ split_pts * np.log(end / start) /
+ (num_depth_cls - 1)).exp()
+ prob_depth_preds = (F.softmax(depth_cls_preds.clone(), dim=-1) *
+ depth_multiplier).sum(dim=-1)
+ return prob_depth_preds
+ elif division == 'loguniform':
+ split_pts = depth_cls_preds.new_tensor(list(
+ range(num_depth_cls))).reshape([1, -1])
+ start = max(depth_range[0], 1)
+ end = depth_range[1]
+ log_multiplier = np.log(start) + \
+ split_pts * np.log(end / start) / (num_depth_cls - 1)
+ prob_depth_preds = (F.softmax(depth_cls_preds.clone(), dim=-1) *
+ log_multiplier).sum(dim=-1).exp()
+ return prob_depth_preds
+ else:
+ raise NotImplementedError
diff --git a/mmdet3d/core/bbox/coders/point_xyzwhlr_bbox_coder.py b/mmdet3d/core/bbox/coders/point_xyzwhlr_bbox_coder.py
new file mode 100644
index 0000000..d246777
--- /dev/null
+++ b/mmdet3d/core/bbox/coders/point_xyzwhlr_bbox_coder.py
@@ -0,0 +1,117 @@
+# Copyright (c) OpenMMLab. All rights reserved.
+import numpy as np
+import torch
+
+from mmdet.core.bbox import BaseBBoxCoder
+from mmdet.core.bbox.builder import BBOX_CODERS
+
+
+@BBOX_CODERS.register_module()
+class PointXYZWHLRBBoxCoder(BaseBBoxCoder):
+ """Point based bbox coder for 3D boxes.
+
+ Args:
+ code_size (int): The dimension of boxes to be encoded.
+ use_mean_size (bool, optional): Whether using anchors based on class.
+ Defaults to True.
+ mean_size (list[list[float]], optional): Mean size of bboxes in
+ each class. Defaults to None.
+ """
+
+ def __init__(self, code_size=7, use_mean_size=True, mean_size=None):
+ super(PointXYZWHLRBBoxCoder, self).__init__()
+ self.code_size = code_size
+ self.use_mean_size = use_mean_size
+ if self.use_mean_size:
+ self.mean_size = torch.from_numpy(np.array(mean_size)).float()
+ assert self.mean_size.min() > 0, \
+ f'The min of mean_size should > 0, however currently it is '\
+ f'{self.mean_size.min()}, please check it in your config.'
+
+ def encode(self, gt_bboxes_3d, points, gt_labels_3d=None):
+ """Encode ground truth to prediction targets.
+
+ Args:
+ gt_bboxes_3d (:obj:`BaseInstance3DBoxes`): Ground truth bboxes
+ with shape (N, 7 + C).
+ points (torch.Tensor): Point cloud with shape (N, 3).
+ gt_labels_3d (torch.Tensor, optional): Ground truth classes.
+ Defaults to None.
+
+ Returns:
+ torch.Tensor: Encoded boxes with shape (N, 8 + C).
+ """
+ gt_bboxes_3d[:, 3:6] = torch.clamp_min(gt_bboxes_3d[:, 3:6], min=1e-5)
+
+ xg, yg, zg, dxg, dyg, dzg, rg, *cgs = torch.split(
+ gt_bboxes_3d, 1, dim=-1)
+ xa, ya, za = torch.split(points, 1, dim=-1)
+
+ if self.use_mean_size:
+ assert gt_labels_3d.max() <= self.mean_size.shape[0] - 1, \
+ f'the max gt label {gt_labels_3d.max()} is bigger than' \
+ f'anchor types {self.mean_size.shape[0] - 1}.'
+ self.mean_size = self.mean_size.to(gt_labels_3d.device)
+ point_anchor_size = self.mean_size[gt_labels_3d]
+ dxa, dya, dza = torch.split(point_anchor_size, 1, dim=-1)
+ diagonal = torch.sqrt(dxa**2 + dya**2)
+ xt = (xg - xa) / diagonal
+ yt = (yg - ya) / diagonal
+ zt = (zg - za) / dza
+ dxt = torch.log(dxg / dxa)
+ dyt = torch.log(dyg / dya)
+ dzt = torch.log(dzg / dza)
+ else:
+ xt = (xg - xa)
+ yt = (yg - ya)
+ zt = (zg - za)
+ dxt = torch.log(dxg)
+ dyt = torch.log(dyg)
+ dzt = torch.log(dzg)
+
+ return torch.cat(
+ [xt, yt, zt, dxt, dyt, dzt,
+ torch.cos(rg),
+ torch.sin(rg), *cgs],
+ dim=-1)
+
+ def decode(self, box_encodings, points, pred_labels_3d=None):
+ """Decode predicted parts and points to bbox3d.
+
+ Args:
+ box_encodings (torch.Tensor): Encoded boxes with shape (N, 8 + C).
+ points (torch.Tensor): Point cloud with shape (N, 3).
+ pred_labels_3d (torch.Tensor): Bbox predicted labels (N, M).
+
+ Returns:
+ torch.Tensor: Decoded boxes with shape (N, 7 + C)
+ """
+ xt, yt, zt, dxt, dyt, dzt, cost, sint, *cts = torch.split(
+ box_encodings, 1, dim=-1)
+ xa, ya, za = torch.split(points, 1, dim=-1)
+
+ if self.use_mean_size:
+ assert pred_labels_3d.max() <= self.mean_size.shape[0] - 1, \
+ f'The max pred label {pred_labels_3d.max()} is bigger than' \
+ f'anchor types {self.mean_size.shape[0] - 1}.'
+ self.mean_size = self.mean_size.to(pred_labels_3d.device)
+ point_anchor_size = self.mean_size[pred_labels_3d]
+ dxa, dya, dza = torch.split(point_anchor_size, 1, dim=-1)
+ diagonal = torch.sqrt(dxa**2 + dya**2)
+ xg = xt * diagonal + xa
+ yg = yt * diagonal + ya
+ zg = zt * dza + za
+
+ dxg = torch.exp(dxt) * dxa
+ dyg = torch.exp(dyt) * dya
+ dzg = torch.exp(dzt) * dza
+ else:
+ xg = xt + xa
+ yg = yt + ya
+ zg = zt + za
+ dxg, dyg, dzg = torch.split(
+ torch.exp(box_encodings[..., 3:6]), 1, dim=-1)
+
+ rg = torch.atan2(sint, cost)
+
+ return torch.cat([xg, yg, zg, dxg, dyg, dzg, rg, *cts], dim=-1)
diff --git a/mmdet3d/core/bbox/coders/smoke_bbox_coder.py b/mmdet3d/core/bbox/coders/smoke_bbox_coder.py
new file mode 100644
index 0000000..134af3a
--- /dev/null
+++ b/mmdet3d/core/bbox/coders/smoke_bbox_coder.py
@@ -0,0 +1,208 @@
+# Copyright (c) OpenMMLab. All rights reserved.
+import numpy as np
+import torch
+
+from mmdet.core.bbox import BaseBBoxCoder
+from mmdet.core.bbox.builder import BBOX_CODERS
+
+
+@BBOX_CODERS.register_module()
+class SMOKECoder(BaseBBoxCoder):
+ """Bbox Coder for SMOKE.
+
+ Args:
+ base_depth (tuple[float]): Depth references for decode box depth.
+ base_dims (tuple[tuple[float]]): Dimension references [l, h, w]
+ for decode box dimension for each category.
+ code_size (int): The dimension of boxes to be encoded.
+ """
+
+ def __init__(self, base_depth, base_dims, code_size):
+ super(SMOKECoder, self).__init__()
+ self.base_depth = base_depth
+ self.base_dims = base_dims
+ self.bbox_code_size = code_size
+
+ def encode(self, locations, dimensions, orientations, input_metas):
+ """Encode CameraInstance3DBoxes by locations, dimensions, orientations.
+
+ Args:
+ locations (Tensor): Center location for 3D boxes.
+ (N, 3)
+ dimensions (Tensor): Dimensions for 3D boxes.
+ shape (N, 3)
+ orientations (Tensor): Orientations for 3D boxes.
+ shape (N, 1)
+ input_metas (list[dict]): Meta information of each image, e.g.,
+ image size, scaling factor, etc.
+
+ Return:
+ :obj:`CameraInstance3DBoxes`: 3D bboxes of batch images,
+ shape (N, bbox_code_size).
+ """
+
+ bboxes = torch.cat((locations, dimensions, orientations), dim=1)
+ assert bboxes.shape[1] == self.bbox_code_size, 'bboxes shape dose not'\
+ 'match the bbox_code_size.'
+ batch_bboxes = input_metas[0]['box_type_3d'](
+ bboxes, box_dim=self.bbox_code_size)
+
+ return batch_bboxes
+
+ def decode(self,
+ reg,
+ points,
+ labels,
+ cam2imgs,
+ trans_mats,
+ locations=None):
+ """Decode regression into locations, dimensions, orientations.
+
+ Args:
+ reg (Tensor): Batch regression for each predict center2d point.
+ shape: (batch * K (max_objs), C)
+ points(Tensor): Batch projected bbox centers on image plane.
+ shape: (batch * K (max_objs) , 2)
+ labels (Tensor): Batch predict class label for each predict
+ center2d point.
+ shape: (batch, K (max_objs))
+ cam2imgs (Tensor): Batch images' camera intrinsic matrix.
+ shape: kitti (batch, 4, 4) nuscenes (batch, 3, 3)
+ trans_mats (Tensor): transformation matrix from original image
+ to feature map.
+ shape: (batch, 3, 3)
+ locations (None | Tensor): if locations is None, this function
+ is used to decode while inference, otherwise, it's used while
+ training using the ground truth 3d bbox locations.
+ shape: (batch * K (max_objs), 3)
+
+ Return:
+ tuple(Tensor): The tuple has components below:
+ - locations (Tensor): Centers of 3D boxes.
+ shape: (batch * K (max_objs), 3)
+ - dimensions (Tensor): Dimensions of 3D boxes.
+ shape: (batch * K (max_objs), 3)
+ - orientations (Tensor): Orientations of 3D
+ boxes.
+ shape: (batch * K (max_objs), 1)
+ """
+ depth_offsets = reg[:, 0]
+ centers2d_offsets = reg[:, 1:3]
+ dimensions_offsets = reg[:, 3:6]
+ orientations = reg[:, 6:8]
+ depths = self._decode_depth(depth_offsets)
+ # get the 3D Bounding box's center location.
+ pred_locations = self._decode_location(points, centers2d_offsets,
+ depths, cam2imgs, trans_mats)
+ pred_dimensions = self._decode_dimension(labels, dimensions_offsets)
+ if locations is None:
+ pred_orientations = self._decode_orientation(
+ orientations, pred_locations)
+ else:
+ pred_orientations = self._decode_orientation(
+ orientations, locations)
+
+ return pred_locations, pred_dimensions, pred_orientations
+
+ def _decode_depth(self, depth_offsets):
+ """Transform depth offset to depth."""
+ base_depth = depth_offsets.new_tensor(self.base_depth)
+ depths = depth_offsets * base_depth[1] + base_depth[0]
+
+ return depths
+
+ def _decode_location(self, points, centers2d_offsets, depths, cam2imgs,
+ trans_mats):
+ """Retrieve objects location in camera coordinate based on projected
+ points.
+
+ Args:
+ points (Tensor): Projected points on feature map in (x, y)
+ shape: (batch * K, 2)
+ centers2d_offset (Tensor): Project points offset in
+ (delta_x, delta_y). shape: (batch * K, 2)
+ depths (Tensor): Object depth z.
+ shape: (batch * K)
+ cam2imgs (Tensor): Batch camera intrinsics matrix.
+ shape: kitti (batch, 4, 4) nuscenes (batch, 3, 3)
+ trans_mats (Tensor): transformation matrix from original image
+ to feature map.
+ shape: (batch, 3, 3)
+ """
+ # number of points
+ N = centers2d_offsets.shape[0]
+ # batch_size
+ N_batch = cam2imgs.shape[0]
+ batch_id = torch.arange(N_batch).unsqueeze(1)
+ obj_id = batch_id.repeat(1, N // N_batch).flatten()
+ trans_mats_inv = trans_mats.inverse()[obj_id]
+ cam2imgs_inv = cam2imgs.inverse()[obj_id]
+ centers2d = points + centers2d_offsets
+ centers2d_extend = torch.cat((centers2d, centers2d.new_ones(N, 1)),
+ dim=1)
+ # expand project points as [N, 3, 1]
+ centers2d_extend = centers2d_extend.unsqueeze(-1)
+ # transform project points back on original image
+ centers2d_img = torch.matmul(trans_mats_inv, centers2d_extend)
+ centers2d_img = centers2d_img * depths.view(N, -1, 1)
+ if cam2imgs.shape[1] == 4:
+ centers2d_img = torch.cat(
+ (centers2d_img, centers2d.new_ones(N, 1, 1)), dim=1)
+ locations = torch.matmul(cam2imgs_inv, centers2d_img).squeeze(2)
+
+ return locations[:, :3]
+
+ def _decode_dimension(self, labels, dims_offset):
+ """Transform dimension offsets to dimension according to its category.
+
+ Args:
+ labels (Tensor): Each points' category id.
+ shape: (N, K)
+ dims_offset (Tensor): Dimension offsets.
+ shape: (N, 3)
+ """
+ labels = labels.flatten().long()
+ base_dims = dims_offset.new_tensor(self.base_dims)
+ dims_select = base_dims[labels, :]
+ dimensions = dims_offset.exp() * dims_select
+
+ return dimensions
+
+ def _decode_orientation(self, ori_vector, locations):
+ """Retrieve object orientation.
+
+ Args:
+ ori_vector (Tensor): Local orientation in [sin, cos] format.
+ shape: (N, 2)
+ locations (Tensor): Object location.
+ shape: (N, 3)
+
+ Return:
+ Tensor: yaw(Orientation). Notice that the yaw's
+ range is [-np.pi, np.pi].
+ shape:(N, 1)
+ """
+ assert len(ori_vector) == len(locations)
+ locations = locations.view(-1, 3)
+ rays = torch.atan(locations[:, 0] / (locations[:, 2] + 1e-7))
+ alphas = torch.atan(ori_vector[:, 0] / (ori_vector[:, 1] + 1e-7))
+
+ # get cosine value positive and negative index.
+ cos_pos_inds = (ori_vector[:, 1] >= 0).nonzero(as_tuple=False)
+ cos_neg_inds = (ori_vector[:, 1] < 0).nonzero(as_tuple=False)
+
+ alphas[cos_pos_inds] -= np.pi / 2
+ alphas[cos_neg_inds] += np.pi / 2
+ # retrieve object rotation y angle.
+ yaws = alphas + rays
+
+ larger_inds = (yaws > np.pi).nonzero(as_tuple=False)
+ small_inds = (yaws < -np.pi).nonzero(as_tuple=False)
+
+ if len(larger_inds) != 0:
+ yaws[larger_inds] -= 2 * np.pi
+ if len(small_inds) != 0:
+ yaws[small_inds] += 2 * np.pi
+
+ yaws = yaws.unsqueeze(-1)
+ return yaws
diff --git a/mmdet3d/core/bbox/iou_calculators/__init__.py b/mmdet3d/core/bbox/iou_calculators/__init__.py
new file mode 100644
index 0000000..d2faf69
--- /dev/null
+++ b/mmdet3d/core/bbox/iou_calculators/__init__.py
@@ -0,0 +1,11 @@
+# Copyright (c) OpenMMLab. All rights reserved.
+from .iou3d_calculator import (AxisAlignedBboxOverlaps3D, BboxOverlaps3D,
+ BboxOverlapsNearest3D,
+ axis_aligned_bbox_overlaps_3d, bbox_overlaps_3d,
+ bbox_overlaps_nearest_3d)
+
+__all__ = [
+ 'BboxOverlapsNearest3D', 'BboxOverlaps3D', 'bbox_overlaps_nearest_3d',
+ 'bbox_overlaps_3d', 'AxisAlignedBboxOverlaps3D',
+ 'axis_aligned_bbox_overlaps_3d'
+]
diff --git a/mmdet3d/core/bbox/iou_calculators/iou3d_calculator.py b/mmdet3d/core/bbox/iou_calculators/iou3d_calculator.py
new file mode 100644
index 0000000..2b1d8ea
--- /dev/null
+++ b/mmdet3d/core/bbox/iou_calculators/iou3d_calculator.py
@@ -0,0 +1,329 @@
+# Copyright (c) OpenMMLab. All rights reserved.
+import torch
+
+from mmdet.core.bbox import bbox_overlaps
+from mmdet.core.bbox.iou_calculators.builder import IOU_CALCULATORS
+from ..structures import get_box_type
+
+
+@IOU_CALCULATORS.register_module()
+class BboxOverlapsNearest3D(object):
+ """Nearest 3D IoU Calculator.
+
+ Note:
+ This IoU calculator first finds the nearest 2D boxes in bird eye view
+ (BEV), and then calculates the 2D IoU using :meth:`bbox_overlaps`.
+
+ Args:
+ coordinate (str): 'camera', 'lidar', or 'depth' coordinate system.
+ """
+
+ def __init__(self, coordinate='lidar'):
+ assert coordinate in ['camera', 'lidar', 'depth']
+ self.coordinate = coordinate
+
+ def __call__(self, bboxes1, bboxes2, mode='iou', is_aligned=False):
+ """Calculate nearest 3D IoU.
+
+ Note:
+ If ``is_aligned`` is ``False``, then it calculates the ious between
+ each bbox of bboxes1 and bboxes2, otherwise it calculates the ious
+ between each aligned pair of bboxes1 and bboxes2.
+
+ Args:
+ bboxes1 (torch.Tensor): shape (N, 7+N)
+ [x, y, z, x_size, y_size, z_size, ry, v].
+ bboxes2 (torch.Tensor): shape (M, 7+N)
+ [x, y, z, x_size, y_size, z_size, ry, v].
+ mode (str): "iou" (intersection over union) or iof
+ (intersection over foreground).
+ is_aligned (bool): Whether the calculation is aligned.
+
+ Return:
+ torch.Tensor: If ``is_aligned`` is ``True``, return ious between
+ bboxes1 and bboxes2 with shape (M, N). If ``is_aligned`` is
+ ``False``, return shape is M.
+ """
+ return bbox_overlaps_nearest_3d(bboxes1, bboxes2, mode, is_aligned,
+ self.coordinate)
+
+ def __repr__(self):
+ """str: Return a string that describes the module."""
+ repr_str = self.__class__.__name__
+ repr_str += f'(coordinate={self.coordinate}'
+ return repr_str
+
+
+@IOU_CALCULATORS.register_module()
+class BboxOverlaps3D(object):
+ """3D IoU Calculator.
+
+ Args:
+ coordinate (str): The coordinate system, valid options are
+ 'camera', 'lidar', and 'depth'.
+ """
+
+ def __init__(self, coordinate):
+ assert coordinate in ['camera', 'lidar', 'depth']
+ self.coordinate = coordinate
+
+ def __call__(self, bboxes1, bboxes2, mode='iou'):
+ """Calculate 3D IoU using cuda implementation.
+
+ Note:
+ This function calculate the IoU of 3D boxes based on their volumes.
+ IoU calculator ``:class:BboxOverlaps3D`` uses this function to
+ calculate the actual 3D IoUs of boxes.
+
+ Args:
+ bboxes1 (torch.Tensor): with shape (N, 7+C),
+ (x, y, z, x_size, y_size, z_size, ry, v*).
+ bboxes2 (torch.Tensor): with shape (M, 7+C),
+ (x, y, z, x_size, y_size, z_size, ry, v*).
+ mode (str): "iou" (intersection over union) or
+ iof (intersection over foreground).
+
+ Return:
+ torch.Tensor: Bbox overlaps results of bboxes1 and bboxes2
+ with shape (M, N) (aligned mode is not supported currently).
+ """
+ return bbox_overlaps_3d(bboxes1, bboxes2, mode, self.coordinate)
+
+ def __repr__(self):
+ """str: return a string that describes the module"""
+ repr_str = self.__class__.__name__
+ repr_str += f'(coordinate={self.coordinate}'
+ return repr_str
+
+
+def bbox_overlaps_nearest_3d(bboxes1,
+ bboxes2,
+ mode='iou',
+ is_aligned=False,
+ coordinate='lidar'):
+ """Calculate nearest 3D IoU.
+
+ Note:
+ This function first finds the nearest 2D boxes in bird eye view
+ (BEV), and then calculates the 2D IoU using :meth:`bbox_overlaps`.
+ This IoU calculator :class:`BboxOverlapsNearest3D` uses this
+ function to calculate IoUs of boxes.
+
+ If ``is_aligned`` is ``False``, then it calculates the ious between
+ each bbox of bboxes1 and bboxes2, otherwise the ious between each
+ aligned pair of bboxes1 and bboxes2.
+
+ Args:
+ bboxes1 (torch.Tensor): with shape (N, 7+C),
+ (x, y, z, x_size, y_size, z_size, ry, v*).
+ bboxes2 (torch.Tensor): with shape (M, 7+C),
+ (x, y, z, x_size, y_size, z_size, ry, v*).
+ mode (str): "iou" (intersection over union) or iof
+ (intersection over foreground).
+ is_aligned (bool): Whether the calculation is aligned
+
+ Return:
+ torch.Tensor: If ``is_aligned`` is ``True``, return ious between
+ bboxes1 and bboxes2 with shape (M, N). If ``is_aligned`` is
+ ``False``, return shape is M.
+ """
+ assert bboxes1.size(-1) == bboxes2.size(-1) >= 7
+
+ box_type, _ = get_box_type(coordinate)
+
+ bboxes1 = box_type(bboxes1, box_dim=bboxes1.shape[-1])
+ bboxes2 = box_type(bboxes2, box_dim=bboxes2.shape[-1])
+
+ # Change the bboxes to bev
+ # box conversion and iou calculation in torch version on CUDA
+ # is 10x faster than that in numpy version
+ bboxes1_bev = bboxes1.nearest_bev
+ bboxes2_bev = bboxes2.nearest_bev
+
+ ret = bbox_overlaps(
+ bboxes1_bev, bboxes2_bev, mode=mode, is_aligned=is_aligned)
+ return ret
+
+
+def bbox_overlaps_3d(bboxes1, bboxes2, mode='iou', coordinate='camera'):
+ """Calculate 3D IoU using cuda implementation.
+
+ Note:
+ This function calculates the IoU of 3D boxes based on their volumes.
+ IoU calculator :class:`BboxOverlaps3D` uses this function to
+ calculate the actual IoUs of boxes.
+
+ Args:
+ bboxes1 (torch.Tensor): with shape (N, 7+C),
+ (x, y, z, x_size, y_size, z_size, ry, v*).
+ bboxes2 (torch.Tensor): with shape (M, 7+C),
+ (x, y, z, x_size, y_size, z_size, ry, v*).
+ mode (str): "iou" (intersection over union) or
+ iof (intersection over foreground).
+ coordinate (str): 'camera' or 'lidar' coordinate system.
+
+ Return:
+ torch.Tensor: Bbox overlaps results of bboxes1 and bboxes2
+ with shape (M, N) (aligned mode is not supported currently).
+ """
+ assert bboxes1.size(-1) == bboxes2.size(-1) >= 7
+
+ box_type, _ = get_box_type(coordinate)
+
+ bboxes1 = box_type(bboxes1, box_dim=bboxes1.shape[-1])
+ bboxes2 = box_type(bboxes2, box_dim=bboxes2.shape[-1])
+
+ return bboxes1.overlaps(bboxes1, bboxes2, mode=mode)
+
+
+@IOU_CALCULATORS.register_module()
+class AxisAlignedBboxOverlaps3D(object):
+ """Axis-aligned 3D Overlaps (IoU) Calculator."""
+
+ def __call__(self, bboxes1, bboxes2, mode='iou', is_aligned=False):
+ """Calculate IoU between 2D bboxes.
+
+ Args:
+ bboxes1 (Tensor): shape (B, m, 6) in
+ format or empty.
+ bboxes2 (Tensor): shape (B, n, 6) in
+ format or empty.
+ B indicates the batch dim, in shape (B1, B2, ..., Bn).
+ If ``is_aligned`` is ``True``, then m and n must be equal.
+ mode (str): "iou" (intersection over union) or "giou" (generalized
+ intersection over union).
+ is_aligned (bool, optional): If True, then m and n must be equal.
+ Defaults to False.
+ Returns:
+ Tensor: shape (m, n) if ``is_aligned`` is False else shape (m,)
+ """
+ assert bboxes1.size(-1) == bboxes2.size(-1) == 6
+ return axis_aligned_bbox_overlaps_3d(bboxes1, bboxes2, mode,
+ is_aligned)
+
+ def __repr__(self):
+ """str: a string describing the module"""
+ repr_str = self.__class__.__name__ + '()'
+ return repr_str
+
+
+def axis_aligned_bbox_overlaps_3d(bboxes1,
+ bboxes2,
+ mode='iou',
+ is_aligned=False,
+ eps=1e-6):
+ """Calculate overlap between two set of axis aligned 3D bboxes. If
+ ``is_aligned`` is ``False``, then calculate the overlaps between each bbox
+ of bboxes1 and bboxes2, otherwise the overlaps between each aligned pair of
+ bboxes1 and bboxes2.
+
+ Args:
+ bboxes1 (Tensor): shape (B, m, 6) in
+ format or empty.
+ bboxes2 (Tensor): shape (B, n, 6) in
+ format or empty.
+ B indicates the batch dim, in shape (B1, B2, ..., Bn).
+ If ``is_aligned`` is ``True``, then m and n must be equal.
+ mode (str): "iou" (intersection over union) or "giou" (generalized
+ intersection over union).
+ is_aligned (bool, optional): If True, then m and n must be equal.
+ Defaults to False.
+ eps (float, optional): A value added to the denominator for numerical
+ stability. Defaults to 1e-6.
+
+ Returns:
+ Tensor: shape (m, n) if ``is_aligned`` is False else shape (m,)
+
+ Example:
+ >>> bboxes1 = torch.FloatTensor([
+ >>> [0, 0, 0, 10, 10, 10],
+ >>> [10, 10, 10, 20, 20, 20],
+ >>> [32, 32, 32, 38, 40, 42],
+ >>> ])
+ >>> bboxes2 = torch.FloatTensor([
+ >>> [0, 0, 0, 10, 20, 20],
+ >>> [0, 10, 10, 10, 19, 20],
+ >>> [10, 10, 10, 20, 20, 20],
+ >>> ])
+ >>> overlaps = axis_aligned_bbox_overlaps_3d(bboxes1, bboxes2)
+ >>> assert overlaps.shape == (3, 3)
+ >>> overlaps = bbox_overlaps(bboxes1, bboxes2, is_aligned=True)
+ >>> assert overlaps.shape == (3, )
+ Example:
+ >>> empty = torch.empty(0, 6)
+ >>> nonempty = torch.FloatTensor([[0, 0, 0, 10, 9, 10]])
+ >>> assert tuple(bbox_overlaps(empty, nonempty).shape) == (0, 1)
+ >>> assert tuple(bbox_overlaps(nonempty, empty).shape) == (1, 0)
+ >>> assert tuple(bbox_overlaps(empty, empty).shape) == (0, 0)
+ """
+
+ assert mode in ['iou', 'giou'], f'Unsupported mode {mode}'
+ # Either the boxes are empty or the length of boxes's last dimension is 6
+ assert (bboxes1.size(-1) == 6 or bboxes1.size(0) == 0)
+ assert (bboxes2.size(-1) == 6 or bboxes2.size(0) == 0)
+
+ # Batch dim must be the same
+ # Batch dim: (B1, B2, ... Bn)
+ assert bboxes1.shape[:-2] == bboxes2.shape[:-2]
+ batch_shape = bboxes1.shape[:-2]
+
+ rows = bboxes1.size(-2)
+ cols = bboxes2.size(-2)
+ if is_aligned:
+ assert rows == cols
+
+ if rows * cols == 0:
+ if is_aligned:
+ return bboxes1.new(batch_shape + (rows, ))
+ else:
+ return bboxes1.new(batch_shape + (rows, cols))
+
+ area1 = (bboxes1[..., 3] -
+ bboxes1[..., 0]) * (bboxes1[..., 4] - bboxes1[..., 1]) * (
+ bboxes1[..., 5] - bboxes1[..., 2])
+ area2 = (bboxes2[..., 3] -
+ bboxes2[..., 0]) * (bboxes2[..., 4] - bboxes2[..., 1]) * (
+ bboxes2[..., 5] - bboxes2[..., 2])
+
+ if is_aligned:
+ lt = torch.max(bboxes1[..., :3], bboxes2[..., :3]) # [B, rows, 3]
+ rb = torch.min(bboxes1[..., 3:], bboxes2[..., 3:]) # [B, rows, 3]
+
+ wh = (rb - lt).clamp(min=0) # [B, rows, 2]
+ overlap = wh[..., 0] * wh[..., 1] * wh[..., 2]
+
+ if mode in ['iou', 'giou']:
+ union = area1 + area2 - overlap
+ else:
+ union = area1
+ if mode == 'giou':
+ enclosed_lt = torch.min(bboxes1[..., :3], bboxes2[..., :3])
+ enclosed_rb = torch.max(bboxes1[..., 3:], bboxes2[..., 3:])
+ else:
+ lt = torch.max(bboxes1[..., :, None, :3],
+ bboxes2[..., None, :, :3]) # [B, rows, cols, 3]
+ rb = torch.min(bboxes1[..., :, None, 3:],
+ bboxes2[..., None, :, 3:]) # [B, rows, cols, 3]
+
+ wh = (rb - lt).clamp(min=0) # [B, rows, cols, 3]
+ overlap = wh[..., 0] * wh[..., 1] * wh[..., 2]
+
+ if mode in ['iou', 'giou']:
+ union = area1[..., None] + area2[..., None, :] - overlap
+ if mode == 'giou':
+ enclosed_lt = torch.min(bboxes1[..., :, None, :3],
+ bboxes2[..., None, :, :3])
+ enclosed_rb = torch.max(bboxes1[..., :, None, 3:],
+ bboxes2[..., None, :, 3:])
+
+ eps = union.new_tensor([eps])
+ union = torch.max(union, eps)
+ ious = overlap / union
+ if mode in ['iou']:
+ return ious
+ # calculate gious
+ enclose_wh = (enclosed_rb - enclosed_lt).clamp(min=0)
+ enclose_area = enclose_wh[..., 0] * enclose_wh[..., 1] * enclose_wh[..., 2]
+ enclose_area = torch.max(enclose_area, eps)
+ gious = ious - (enclose_area - union) / enclose_area
+ return gious
diff --git a/mmdet3d/core/bbox/samplers/__init__.py b/mmdet3d/core/bbox/samplers/__init__.py
new file mode 100644
index 0000000..168780b
--- /dev/null
+++ b/mmdet3d/core/bbox/samplers/__init__.py
@@ -0,0 +1,13 @@
+# Copyright (c) OpenMMLab. All rights reserved.
+from mmdet.core.bbox.samplers import (BaseSampler, CombinedSampler,
+ InstanceBalancedPosSampler,
+ IoUBalancedNegSampler, OHEMSampler,
+ PseudoSampler, RandomSampler,
+ SamplingResult)
+from .iou_neg_piecewise_sampler import IoUNegPiecewiseSampler
+
+__all__ = [
+ 'BaseSampler', 'PseudoSampler', 'RandomSampler',
+ 'InstanceBalancedPosSampler', 'IoUBalancedNegSampler', 'CombinedSampler',
+ 'OHEMSampler', 'SamplingResult', 'IoUNegPiecewiseSampler'
+]
diff --git a/mmdet3d/core/bbox/samplers/iou_neg_piecewise_sampler.py b/mmdet3d/core/bbox/samplers/iou_neg_piecewise_sampler.py
new file mode 100644
index 0000000..cbd8483
--- /dev/null
+++ b/mmdet3d/core/bbox/samplers/iou_neg_piecewise_sampler.py
@@ -0,0 +1,183 @@
+# Copyright (c) OpenMMLab. All rights reserved.
+import torch
+
+from mmdet.core.bbox.builder import BBOX_SAMPLERS
+from . import RandomSampler, SamplingResult
+
+
+@BBOX_SAMPLERS.register_module()
+class IoUNegPiecewiseSampler(RandomSampler):
+ """IoU Piece-wise Sampling.
+
+ Sampling negative proposals according to a list of IoU thresholds.
+ The negative proposals are divided into several pieces according
+ to `neg_iou_piece_thrs`. And the ratio of each piece is indicated
+ by `neg_piece_fractions`.
+
+ Args:
+ num (int): Number of proposals.
+ pos_fraction (float): The fraction of positive proposals.
+ neg_piece_fractions (list): A list contains fractions that indicates
+ the ratio of each piece of total negative samplers.
+ neg_iou_piece_thrs (list): A list contains IoU thresholds that
+ indicate the upper bound of this piece.
+ neg_pos_ub (float): The total ratio to limit the upper bound
+ number of negative samples.
+ add_gt_as_proposals (bool): Whether to add gt as proposals.
+ """
+
+ def __init__(self,
+ num,
+ pos_fraction=None,
+ neg_piece_fractions=None,
+ neg_iou_piece_thrs=None,
+ neg_pos_ub=-1,
+ add_gt_as_proposals=False,
+ return_iou=False):
+ super(IoUNegPiecewiseSampler,
+ self).__init__(num, pos_fraction, neg_pos_ub,
+ add_gt_as_proposals)
+ assert isinstance(neg_piece_fractions, list)
+ assert len(neg_piece_fractions) == len(neg_iou_piece_thrs)
+ self.neg_piece_fractions = neg_piece_fractions
+ self.neg_iou_thr = neg_iou_piece_thrs
+ self.return_iou = return_iou
+ self.neg_piece_num = len(self.neg_piece_fractions)
+
+ def _sample_pos(self, assign_result, num_expected, **kwargs):
+ """Randomly sample some positive samples."""
+ pos_inds = torch.nonzero(assign_result.gt_inds > 0, as_tuple=False)
+ if pos_inds.numel() != 0:
+ pos_inds = pos_inds.squeeze(1)
+ if pos_inds.numel() <= num_expected:
+ return pos_inds
+ else:
+ return self.random_choice(pos_inds, num_expected)
+
+ def _sample_neg(self, assign_result, num_expected, **kwargs):
+ """Randomly sample some negative samples."""
+ neg_inds = torch.nonzero(assign_result.gt_inds == 0, as_tuple=False)
+ if neg_inds.numel() != 0:
+ neg_inds = neg_inds.squeeze(1)
+ if len(neg_inds) <= 0:
+ return neg_inds.squeeze(1)
+ else:
+ neg_inds_choice = neg_inds.new_zeros([0])
+ extend_num = 0
+ max_overlaps = assign_result.max_overlaps[neg_inds]
+
+ for piece_inds in range(self.neg_piece_num):
+ if piece_inds == self.neg_piece_num - 1: # for the last piece
+ piece_expected_num = num_expected - len(neg_inds_choice)
+ min_iou_thr = 0
+ else:
+ # if the numbers of negative samplers in previous
+ # pieces are less than the expected number, extend
+ # the same number in the current piece.
+ piece_expected_num = int(
+ num_expected *
+ self.neg_piece_fractions[piece_inds]) + extend_num
+ min_iou_thr = self.neg_iou_thr[piece_inds + 1]
+ max_iou_thr = self.neg_iou_thr[piece_inds]
+ piece_neg_inds = torch.nonzero(
+ (max_overlaps >= min_iou_thr)
+ & (max_overlaps < max_iou_thr),
+ as_tuple=False).view(-1)
+
+ if len(piece_neg_inds) < piece_expected_num:
+ neg_inds_choice = torch.cat(
+ [neg_inds_choice, neg_inds[piece_neg_inds]], dim=0)
+ extend_num += piece_expected_num - len(piece_neg_inds)
+
+ # for the last piece
+ if piece_inds == self.neg_piece_num - 1:
+ extend_neg_num = num_expected - len(neg_inds_choice)
+ # if the numbers of nagetive samples > 0, we will
+ # randomly select num_expected samples in last piece
+ if piece_neg_inds.numel() > 0:
+ rand_idx = torch.randint(
+ low=0,
+ high=piece_neg_inds.numel(),
+ size=(extend_neg_num, )).long()
+ neg_inds_choice = torch.cat(
+ [neg_inds_choice, piece_neg_inds[rand_idx]],
+ dim=0)
+ # if the numbers of nagetive samples == 0, we will
+ # randomly select num_expected samples in all
+ # previous pieces
+ else:
+ rand_idx = torch.randint(
+ low=0,
+ high=neg_inds_choice.numel(),
+ size=(extend_neg_num, )).long()
+ neg_inds_choice = torch.cat(
+ [neg_inds_choice, neg_inds_choice[rand_idx]],
+ dim=0)
+ else:
+ piece_choice = self.random_choice(piece_neg_inds,
+ piece_expected_num)
+ neg_inds_choice = torch.cat(
+ [neg_inds_choice, neg_inds[piece_choice]], dim=0)
+ extend_num = 0
+ assert len(neg_inds_choice) == num_expected
+ return neg_inds_choice
+
+ def sample(self,
+ assign_result,
+ bboxes,
+ gt_bboxes,
+ gt_labels=None,
+ **kwargs):
+ """Sample positive and negative bboxes.
+
+ This is a simple implementation of bbox sampling given candidates,
+ assigning results and ground truth bboxes.
+
+ Args:
+ assign_result (:obj:`AssignResult`): Bbox assigning results.
+ bboxes (torch.Tensor): Boxes to be sampled from.
+ gt_bboxes (torch.Tensor): Ground truth bboxes.
+ gt_labels (torch.Tensor, optional): Class labels of ground truth
+ bboxes.
+
+ Returns:
+ :obj:`SamplingResult`: Sampling result.
+ """
+ if len(bboxes.shape) < 2:
+ bboxes = bboxes[None, :]
+
+ gt_flags = bboxes.new_zeros((bboxes.shape[0], ), dtype=torch.bool)
+ if self.add_gt_as_proposals and len(gt_bboxes) > 0:
+ if gt_labels is None:
+ raise ValueError(
+ 'gt_labels must be given when add_gt_as_proposals is True')
+ bboxes = torch.cat([gt_bboxes, bboxes], dim=0)
+ assign_result.add_gt_(gt_labels)
+ gt_ones = bboxes.new_ones(gt_bboxes.shape[0], dtype=torch.bool)
+ gt_flags = torch.cat([gt_ones, gt_flags])
+
+ num_expected_pos = int(self.num * self.pos_fraction)
+ pos_inds = self.pos_sampler._sample_pos(
+ assign_result, num_expected_pos, bboxes=bboxes, **kwargs)
+ # We found that sampled indices have duplicated items occasionally.
+ # (may be a bug of PyTorch)
+ pos_inds = pos_inds.unique()
+ num_sampled_pos = pos_inds.numel()
+ num_expected_neg = self.num - num_sampled_pos
+ if self.neg_pos_ub >= 0:
+ _pos = max(1, num_sampled_pos)
+ neg_upper_bound = int(self.neg_pos_ub * _pos)
+ if num_expected_neg > neg_upper_bound:
+ num_expected_neg = neg_upper_bound
+ neg_inds = self.neg_sampler._sample_neg(
+ assign_result, num_expected_neg, bboxes=bboxes, **kwargs)
+
+ sampling_result = SamplingResult(pos_inds, neg_inds, bboxes, gt_bboxes,
+ assign_result, gt_flags)
+ if self.return_iou:
+ # PartA2 needs iou score to regression.
+ sampling_result.iou = assign_result.max_overlaps[torch.cat(
+ [pos_inds, neg_inds])]
+ sampling_result.iou.detach_()
+
+ return sampling_result
diff --git a/mmdet3d/core/bbox/structures/__init__.py b/mmdet3d/core/bbox/structures/__init__.py
new file mode 100644
index 0000000..460035a
--- /dev/null
+++ b/mmdet3d/core/bbox/structures/__init__.py
@@ -0,0 +1,18 @@
+# Copyright (c) OpenMMLab. All rights reserved.
+from .base_box3d import BaseInstance3DBoxes
+from .box_3d_mode import Box3DMode
+from .cam_box3d import CameraInstance3DBoxes
+from .coord_3d_mode import Coord3DMode
+from .depth_box3d import DepthInstance3DBoxes
+from .lidar_box3d import LiDARInstance3DBoxes
+from .utils import (get_box_type, get_proj_mat_by_coord_type, limit_period,
+ mono_cam_box2vis, points_cam2img, points_img2cam,
+ rotation_3d_in_axis, xywhr2xyxyr)
+
+__all__ = [
+ 'Box3DMode', 'BaseInstance3DBoxes', 'LiDARInstance3DBoxes',
+ 'CameraInstance3DBoxes', 'DepthInstance3DBoxes', 'xywhr2xyxyr',
+ 'get_box_type', 'rotation_3d_in_axis', 'limit_period', 'points_cam2img',
+ 'points_img2cam', 'Coord3DMode', 'mono_cam_box2vis',
+ 'get_proj_mat_by_coord_type'
+]
diff --git a/mmdet3d/core/bbox/structures/base_box3d.py b/mmdet3d/core/bbox/structures/base_box3d.py
new file mode 100644
index 0000000..3c74f67
--- /dev/null
+++ b/mmdet3d/core/bbox/structures/base_box3d.py
@@ -0,0 +1,578 @@
+# Copyright (c) OpenMMLab. All rights reserved.
+import warnings
+from abc import abstractmethod
+
+import numpy as np
+import torch
+from mmcv.ops import box_iou_rotated, points_in_boxes_all, points_in_boxes_part
+
+from .utils import limit_period
+
+
+class BaseInstance3DBoxes(object):
+ """Base class for 3D Boxes.
+
+ Note:
+ The box is bottom centered, i.e. the relative position of origin in
+ the box is (0.5, 0.5, 0).
+
+ Args:
+ tensor (torch.Tensor | np.ndarray | list): a N x box_dim matrix.
+ box_dim (int): Number of the dimension of a box.
+ Each row is (x, y, z, x_size, y_size, z_size, yaw).
+ Defaults to 7.
+ with_yaw (bool): Whether the box is with yaw rotation.
+ If False, the value of yaw will be set to 0 as minmax boxes.
+ Defaults to True.
+ origin (tuple[float], optional): Relative position of the box origin.
+ Defaults to (0.5, 0.5, 0). This will guide the box be converted to
+ (0.5, 0.5, 0) mode.
+
+ Attributes:
+ tensor (torch.Tensor): Float matrix of N x box_dim.
+ box_dim (int): Integer indicating the dimension of a box.
+ Each row is (x, y, z, x_size, y_size, z_size, yaw, ...).
+ with_yaw (bool): If True, the value of yaw will be set to 0 as minmax
+ boxes.
+ """
+
+ def __init__(self, tensor, box_dim=7, with_yaw=True, origin=(0.5, 0.5, 0)):
+ if isinstance(tensor, torch.Tensor):
+ device = tensor.device
+ else:
+ device = torch.device('cpu')
+ tensor = torch.as_tensor(tensor, dtype=torch.float32, device=device)
+ if tensor.numel() == 0:
+ # Use reshape, so we don't end up creating a new tensor that
+ # does not depend on the inputs (and consequently confuses jit)
+ tensor = tensor.reshape((0, box_dim)).to(
+ dtype=torch.float32, device=device)
+ assert tensor.dim() == 2 and tensor.size(-1) == box_dim, tensor.size()
+
+ if tensor.shape[-1] == 6:
+ # If the dimension of boxes is 6, we expand box_dim by padding
+ # 0 as a fake yaw and set with_yaw to False.
+ assert box_dim == 6
+ fake_rot = tensor.new_zeros(tensor.shape[0], 1)
+ tensor = torch.cat((tensor, fake_rot), dim=-1)
+ self.box_dim = box_dim + 1
+ self.with_yaw = False
+ else:
+ self.box_dim = box_dim
+ self.with_yaw = with_yaw
+ self.tensor = tensor.clone()
+
+ if origin != (0.5, 0.5, 0):
+ dst = self.tensor.new_tensor((0.5, 0.5, 0))
+ src = self.tensor.new_tensor(origin)
+ self.tensor[:, :3] += self.tensor[:, 3:6] * (dst - src)
+
+ @property
+ def volume(self):
+ """torch.Tensor: A vector with volume of each box."""
+ return self.tensor[:, 3] * self.tensor[:, 4] * self.tensor[:, 5]
+
+ @property
+ def dims(self):
+ """torch.Tensor: Size dimensions of each box in shape (N, 3)."""
+ return self.tensor[:, 3:6]
+
+ @property
+ def yaw(self):
+ """torch.Tensor: A vector with yaw of each box in shape (N, )."""
+ return self.tensor[:, 6]
+
+ @property
+ def height(self):
+ """torch.Tensor: A vector with height of each box in shape (N, )."""
+ return self.tensor[:, 5]
+
+ @property
+ def top_height(self):
+ """torch.Tensor:
+ A vector with the top height of each box in shape (N, )."""
+ return self.bottom_height + self.height
+
+ @property
+ def bottom_height(self):
+ """torch.Tensor:
+ A vector with bottom's height of each box in shape (N, )."""
+ return self.tensor[:, 2]
+
+ @property
+ def center(self):
+ """Calculate the center of all the boxes.
+
+ Note:
+ In MMDetection3D's convention, the bottom center is
+ usually taken as the default center.
+
+ The relative position of the centers in different kinds of
+ boxes are different, e.g., the relative center of a boxes is
+ (0.5, 1.0, 0.5) in camera and (0.5, 0.5, 0) in lidar.
+ It is recommended to use ``bottom_center`` or ``gravity_center``
+ for clearer usage.
+
+ Returns:
+ torch.Tensor: A tensor with center of each box in shape (N, 3).
+ """
+ return self.bottom_center
+
+ @property
+ def bottom_center(self):
+ """torch.Tensor: A tensor with center of each box in shape (N, 3)."""
+ return self.tensor[:, :3]
+
+ @property
+ def gravity_center(self):
+ """torch.Tensor: A tensor with center of each box in shape (N, 3)."""
+ pass
+
+ @property
+ def corners(self):
+ """torch.Tensor:
+ a tensor with 8 corners of each box in shape (N, 8, 3)."""
+ pass
+
+ @property
+ def bev(self):
+ """torch.Tensor: 2D BEV box of each box with rotation
+ in XYWHR format, in shape (N, 5)."""
+ return self.tensor[:, [0, 1, 3, 4, 6]]
+
+ @property
+ def nearest_bev(self):
+ """torch.Tensor: A tensor of 2D BEV box of each box
+ without rotation."""
+ # Obtain BEV boxes with rotation in XYWHR format
+ bev_rotated_boxes = self.bev
+ # convert the rotation to a valid range
+ rotations = bev_rotated_boxes[:, -1]
+ normed_rotations = torch.abs(limit_period(rotations, 0.5, np.pi))
+
+ # find the center of boxes
+ conditions = (normed_rotations > np.pi / 4)[..., None]
+ bboxes_xywh = torch.where(conditions, bev_rotated_boxes[:,
+ [0, 1, 3, 2]],
+ bev_rotated_boxes[:, :4])
+
+ centers = bboxes_xywh[:, :2]
+ dims = bboxes_xywh[:, 2:]
+ bev_boxes = torch.cat([centers - dims / 2, centers + dims / 2], dim=-1)
+ return bev_boxes
+
+ def in_range_bev(self, box_range):
+ """Check whether the boxes are in the given range.
+
+ Args:
+ box_range (list | torch.Tensor): the range of box
+ (x_min, y_min, x_max, y_max)
+
+ Note:
+ The original implementation of SECOND checks whether boxes in
+ a range by checking whether the points are in a convex
+ polygon, we reduce the burden for simpler cases.
+
+ Returns:
+ torch.Tensor: Whether each box is inside the reference range.
+ """
+ in_range_flags = ((self.bev[:, 0] > box_range[0])
+ & (self.bev[:, 1] > box_range[1])
+ & (self.bev[:, 0] < box_range[2])
+ & (self.bev[:, 1] < box_range[3]))
+ return in_range_flags
+
+ @abstractmethod
+ def rotate(self, angle, points=None):
+ """Rotate boxes with points (optional) with the given angle or rotation
+ matrix.
+
+ Args:
+ angle (float | torch.Tensor | np.ndarray):
+ Rotation angle or rotation matrix.
+ points (torch.Tensor | numpy.ndarray |
+ :obj:`BasePoints`, optional):
+ Points to rotate. Defaults to None.
+ """
+ pass
+
+ @abstractmethod
+ def flip(self, bev_direction='horizontal'):
+ """Flip the boxes in BEV along given BEV direction.
+
+ Args:
+ bev_direction (str, optional): Direction by which to flip.
+ Can be chosen from 'horizontal' and 'vertical'.
+ Defaults to 'horizontal'.
+ """
+ pass
+
+ def translate(self, trans_vector):
+ """Translate boxes with the given translation vector.
+
+ Args:
+ trans_vector (torch.Tensor): Translation vector of size (1, 3).
+ """
+ if not isinstance(trans_vector, torch.Tensor):
+ trans_vector = self.tensor.new_tensor(trans_vector)
+ self.tensor[:, :3] += trans_vector
+
+ def in_range_3d(self, box_range):
+ """Check whether the boxes are in the given range.
+
+ Args:
+ box_range (list | torch.Tensor): The range of box
+ (x_min, y_min, z_min, x_max, y_max, z_max)
+
+ Note:
+ In the original implementation of SECOND, checking whether
+ a box in the range checks whether the points are in a convex
+ polygon, we try to reduce the burden for simpler cases.
+
+ Returns:
+ torch.Tensor: A binary vector indicating whether each box is
+ inside the reference range.
+ """
+ in_range_flags = ((self.tensor[:, 0] > box_range[0])
+ & (self.tensor[:, 1] > box_range[1])
+ & (self.tensor[:, 2] > box_range[2])
+ & (self.tensor[:, 0] < box_range[3])
+ & (self.tensor[:, 1] < box_range[4])
+ & (self.tensor[:, 2] < box_range[5]))
+ return in_range_flags
+
+ @abstractmethod
+ def convert_to(self, dst, rt_mat=None):
+ """Convert self to ``dst`` mode.
+
+ Args:
+ dst (:obj:`Box3DMode`): The target Box mode.
+ rt_mat (np.ndarray | torch.Tensor, optional): The rotation and
+ translation matrix between different coordinates.
+ Defaults to None.
+ The conversion from `src` coordinates to `dst` coordinates
+ usually comes along the change of sensors, e.g., from camera
+ to LiDAR. This requires a transformation matrix.
+
+ Returns:
+ :obj:`BaseInstance3DBoxes`: The converted box of the same type
+ in the `dst` mode.
+ """
+ pass
+
+ def scale(self, scale_factor):
+ """Scale the box with horizontal and vertical scaling factors.
+
+ Args:
+ scale_factors (float): Scale factors to scale the boxes.
+ """
+ self.tensor[:, :6] *= scale_factor
+ self.tensor[:, 7:] *= scale_factor # velocity
+
+ def limit_yaw(self, offset=0.5, period=np.pi):
+ """Limit the yaw to a given period and offset.
+
+ Args:
+ offset (float, optional): The offset of the yaw. Defaults to 0.5.
+ period (float, optional): The expected period. Defaults to np.pi.
+ """
+ self.tensor[:, 6] = limit_period(self.tensor[:, 6], offset, period)
+
+ def nonempty(self, threshold=0.0):
+ """Find boxes that are non-empty.
+
+ A box is considered empty,
+ if either of its side is no larger than threshold.
+
+ Args:
+ threshold (float, optional): The threshold of minimal sizes.
+ Defaults to 0.0.
+
+ Returns:
+ torch.Tensor: A binary vector which represents whether each
+ box is empty (False) or non-empty (True).
+ """
+ box = self.tensor
+ size_x = box[..., 3]
+ size_y = box[..., 4]
+ size_z = box[..., 5]
+ keep = ((size_x > threshold)
+ & (size_y > threshold) & (size_z > threshold))
+ return keep
+
+ def __getitem__(self, item):
+ """
+ Note:
+ The following usage are allowed:
+ 1. `new_boxes = boxes[3]`:
+ return a `Boxes` that contains only one box.
+ 2. `new_boxes = boxes[2:10]`:
+ return a slice of boxes.
+ 3. `new_boxes = boxes[vector]`:
+ where vector is a torch.BoolTensor with `length = len(boxes)`.
+ Nonzero elements in the vector will be selected.
+ Note that the returned Boxes might share storage with this Boxes,
+ subject to Pytorch's indexing semantics.
+
+ Returns:
+ :obj:`BaseInstance3DBoxes`: A new object of
+ :class:`BaseInstance3DBoxes` after indexing.
+ """
+ original_type = type(self)
+ if isinstance(item, int):
+ return original_type(
+ self.tensor[item].view(1, -1),
+ box_dim=self.box_dim,
+ with_yaw=self.with_yaw)
+ b = self.tensor[item]
+ assert b.dim() == 2, \
+ f'Indexing on Boxes with {item} failed to return a matrix!'
+ return original_type(b, box_dim=self.box_dim, with_yaw=self.with_yaw)
+
+ def __len__(self):
+ """int: Number of boxes in the current object."""
+ return self.tensor.shape[0]
+
+ def __repr__(self):
+ """str: Return a strings that describes the object."""
+ return self.__class__.__name__ + '(\n ' + str(self.tensor) + ')'
+
+ @classmethod
+ def cat(cls, boxes_list):
+ """Concatenate a list of Boxes into a single Boxes.
+
+ Args:
+ boxes_list (list[:obj:`BaseInstance3DBoxes`]): List of boxes.
+
+ Returns:
+ :obj:`BaseInstance3DBoxes`: The concatenated Boxes.
+ """
+ assert isinstance(boxes_list, (list, tuple))
+ if len(boxes_list) == 0:
+ return cls(torch.empty(0))
+ assert all(isinstance(box, cls) for box in boxes_list)
+
+ # use torch.cat (v.s. layers.cat)
+ # so the returned boxes never share storage with input
+ cat_boxes = cls(
+ torch.cat([b.tensor for b in boxes_list], dim=0),
+ box_dim=boxes_list[0].tensor.shape[1],
+ with_yaw=boxes_list[0].with_yaw)
+ return cat_boxes
+
+ def to(self, device):
+ """Convert current boxes to a specific device.
+
+ Args:
+ device (str | :obj:`torch.device`): The name of the device.
+
+ Returns:
+ :obj:`BaseInstance3DBoxes`: A new boxes object on the
+ specific device.
+ """
+ original_type = type(self)
+ return original_type(
+ self.tensor.to(device),
+ box_dim=self.box_dim,
+ with_yaw=self.with_yaw)
+
+ def clone(self):
+ """Clone the Boxes.
+
+ Returns:
+ :obj:`BaseInstance3DBoxes`: Box object with the same properties
+ as self.
+ """
+ original_type = type(self)
+ return original_type(
+ self.tensor.clone(), box_dim=self.box_dim, with_yaw=self.with_yaw)
+
+ @property
+ def device(self):
+ """str: The device of the boxes are on."""
+ return self.tensor.device
+
+ def __iter__(self):
+ """Yield a box as a Tensor of shape (4,) at a time.
+
+ Returns:
+ torch.Tensor: A box of shape (4,).
+ """
+ yield from self.tensor
+
+ @classmethod
+ def height_overlaps(cls, boxes1, boxes2, mode='iou'):
+ """Calculate height overlaps of two boxes.
+
+ Note:
+ This function calculates the height overlaps between boxes1 and
+ boxes2, boxes1 and boxes2 should be in the same type.
+
+ Args:
+ boxes1 (:obj:`BaseInstance3DBoxes`): Boxes 1 contain N boxes.
+ boxes2 (:obj:`BaseInstance3DBoxes`): Boxes 2 contain M boxes.
+ mode (str, optional): Mode of IoU calculation. Defaults to 'iou'.
+
+ Returns:
+ torch.Tensor: Calculated iou of boxes.
+ """
+ assert isinstance(boxes1, BaseInstance3DBoxes)
+ assert isinstance(boxes2, BaseInstance3DBoxes)
+ assert type(boxes1) == type(boxes2), '"boxes1" and "boxes2" should' \
+ f'be in the same type, got {type(boxes1)} and {type(boxes2)}.'
+
+ boxes1_top_height = boxes1.top_height.view(-1, 1)
+ boxes1_bottom_height = boxes1.bottom_height.view(-1, 1)
+ boxes2_top_height = boxes2.top_height.view(1, -1)
+ boxes2_bottom_height = boxes2.bottom_height.view(1, -1)
+
+ heighest_of_bottom = torch.max(boxes1_bottom_height,
+ boxes2_bottom_height)
+ lowest_of_top = torch.min(boxes1_top_height, boxes2_top_height)
+ overlaps_h = torch.clamp(lowest_of_top - heighest_of_bottom, min=0)
+ return overlaps_h
+
+ @classmethod
+ def overlaps(cls, boxes1, boxes2, mode='iou'):
+ """Calculate 3D overlaps of two boxes.
+
+ Note:
+ This function calculates the overlaps between ``boxes1`` and
+ ``boxes2``, ``boxes1`` and ``boxes2`` should be in the same type.
+
+ Args:
+ boxes1 (:obj:`BaseInstance3DBoxes`): Boxes 1 contain N boxes.
+ boxes2 (:obj:`BaseInstance3DBoxes`): Boxes 2 contain M boxes.
+ mode (str, optional): Mode of iou calculation. Defaults to 'iou'.
+
+ Returns:
+ torch.Tensor: Calculated 3D overlaps of the boxes.
+ """
+ assert isinstance(boxes1, BaseInstance3DBoxes)
+ assert isinstance(boxes2, BaseInstance3DBoxes)
+ assert type(boxes1) == type(boxes2), '"boxes1" and "boxes2" should' \
+ f'be in the same type, got {type(boxes1)} and {type(boxes2)}.'
+
+ assert mode in ['iou', 'iof']
+
+ rows = len(boxes1)
+ cols = len(boxes2)
+ if rows * cols == 0:
+ return boxes1.tensor.new(rows, cols)
+
+ # height overlap
+ overlaps_h = cls.height_overlaps(boxes1, boxes2)
+
+ # bev overlap
+ iou2d = box_iou_rotated(boxes1.bev, boxes2.bev)
+ areas1 = (boxes1.bev[:, 2] * boxes1.bev[:, 3]).unsqueeze(1).expand(
+ rows, cols)
+ areas2 = (boxes2.bev[:, 2] * boxes2.bev[:, 3]).unsqueeze(0).expand(
+ rows, cols)
+ overlaps_bev = iou2d * (areas1 + areas2) / (1 + iou2d)
+
+ # 3d overlaps
+ overlaps_3d = overlaps_bev.to(boxes1.device) * overlaps_h
+
+ volume1 = boxes1.volume.view(-1, 1)
+ volume2 = boxes2.volume.view(1, -1)
+
+ if mode == 'iou':
+ # the clamp func is used to avoid division of 0
+ iou3d = overlaps_3d / torch.clamp(
+ volume1 + volume2 - overlaps_3d, min=1e-8)
+ else:
+ iou3d = overlaps_3d / torch.clamp(volume1, min=1e-8)
+
+ return iou3d
+
+ def new_box(self, data):
+ """Create a new box object with data.
+
+ The new box and its tensor has the similar properties
+ as self and self.tensor, respectively.
+
+ Args:
+ data (torch.Tensor | numpy.array | list): Data to be copied.
+
+ Returns:
+ :obj:`BaseInstance3DBoxes`: A new bbox object with ``data``,
+ the object's other properties are similar to ``self``.
+ """
+ new_tensor = self.tensor.new_tensor(data) \
+ if not isinstance(data, torch.Tensor) else data.to(self.device)
+ original_type = type(self)
+ return original_type(
+ new_tensor, box_dim=self.box_dim, with_yaw=self.with_yaw)
+
+ def points_in_boxes_part(self, points, boxes_override=None):
+ """Find the box in which each point is.
+
+ Args:
+ points (torch.Tensor): Points in shape (1, M, 3) or (M, 3),
+ 3 dimensions are (x, y, z) in LiDAR or depth coordinate.
+ boxes_override (torch.Tensor, optional): Boxes to override
+ `self.tensor`. Defaults to None.
+
+ Returns:
+ torch.Tensor: The index of the first box that each point
+ is in, in shape (M, ). Default value is -1
+ (if the point is not enclosed by any box).
+
+ Note:
+ If a point is enclosed by multiple boxes, the index of the
+ first box will be returned.
+ """
+ if boxes_override is not None:
+ boxes = boxes_override
+ else:
+ boxes = self.tensor
+ if points.dim() == 2:
+ points = points.unsqueeze(0)
+ box_idx = points_in_boxes_part(points,
+ boxes.unsqueeze(0).to(
+ points.device)).squeeze(0)
+ return box_idx
+
+ def points_in_boxes_all(self, points, boxes_override=None):
+ """Find all boxes in which each point is.
+
+ Args:
+ points (torch.Tensor): Points in shape (1, M, 3) or (M, 3),
+ 3 dimensions are (x, y, z) in LiDAR or depth coordinate.
+ boxes_override (torch.Tensor, optional): Boxes to override
+ `self.tensor`. Defaults to None.
+
+ Returns:
+ torch.Tensor: A tensor indicating whether a point is in a box,
+ in shape (M, T). T is the number of boxes. Denote this
+ tensor as A, if the m^th point is in the t^th box, then
+ `A[m, t] == 1`, elsewise `A[m, t] == 0`.
+ """
+ if boxes_override is not None:
+ boxes = boxes_override
+ else:
+ boxes = self.tensor
+
+ points_clone = points.clone()[..., :3]
+ if points_clone.dim() == 2:
+ points_clone = points_clone.unsqueeze(0)
+ else:
+ assert points_clone.dim() == 3 and points_clone.shape[0] == 1
+
+ boxes = boxes.to(points_clone.device).unsqueeze(0)
+ box_idxs_of_pts = points_in_boxes_all(points_clone, boxes)
+
+ return box_idxs_of_pts.squeeze(0)
+
+ def points_in_boxes(self, points, boxes_override=None):
+ warnings.warn('DeprecationWarning: points_in_boxes is a '
+ 'deprecated method, please consider using '
+ 'points_in_boxes_part.')
+ return self.points_in_boxes_part(points, boxes_override)
+
+ def points_in_boxes_batch(self, points, boxes_override=None):
+ warnings.warn('DeprecationWarning: points_in_boxes_batch is a '
+ 'deprecated method, please consider using '
+ 'points_in_boxes_all.')
+ return self.points_in_boxes_all(points, boxes_override)
diff --git a/mmdet3d/core/bbox/structures/box_3d_mode.py b/mmdet3d/core/bbox/structures/box_3d_mode.py
new file mode 100644
index 0000000..3048b0a
--- /dev/null
+++ b/mmdet3d/core/bbox/structures/box_3d_mode.py
@@ -0,0 +1,197 @@
+# Copyright (c) OpenMMLab. All rights reserved.
+from enum import IntEnum, unique
+
+import numpy as np
+import torch
+
+from .base_box3d import BaseInstance3DBoxes
+from .cam_box3d import CameraInstance3DBoxes
+from .depth_box3d import DepthInstance3DBoxes
+from .lidar_box3d import LiDARInstance3DBoxes
+from .utils import limit_period
+
+
+@unique
+class Box3DMode(IntEnum):
+ r"""Enum of different ways to represent a box.
+
+ Coordinates in LiDAR:
+
+ .. code-block:: none
+
+ up z
+ ^ x front
+ | /
+ | /
+ left y <------ 0
+
+ The relative coordinate of bottom center in a LiDAR box is (0.5, 0.5, 0),
+ and the yaw is around the z axis, thus the rotation axis=2.
+
+ Coordinates in camera:
+
+ .. code-block:: none
+
+ z front
+ /
+ /
+ 0 ------> x right
+ |
+ |
+ v
+ down y
+
+ The relative coordinate of bottom center in a CAM box is [0.5, 1.0, 0.5],
+ and the yaw is around the y axis, thus the rotation axis=1.
+
+ Coordinates in Depth mode:
+
+ .. code-block:: none
+
+ up z
+ ^ y front
+ | /
+ | /
+ 0 ------> x right
+
+ The relative coordinate of bottom center in a DEPTH box is (0.5, 0.5, 0),
+ and the yaw is around the z axis, thus the rotation axis=2.
+ """
+
+ LIDAR = 0
+ CAM = 1
+ DEPTH = 2
+
+ @staticmethod
+ def convert(box, src, dst, rt_mat=None, with_yaw=True):
+ """Convert boxes from `src` mode to `dst` mode.
+
+ Args:
+ box (tuple | list | np.ndarray |
+ torch.Tensor | :obj:`BaseInstance3DBoxes`):
+ Can be a k-tuple, k-list or an Nxk array/tensor, where k = 7.
+ src (:obj:`Box3DMode`): The src Box mode.
+ dst (:obj:`Box3DMode`): The target Box mode.
+ rt_mat (np.ndarray | torch.Tensor, optional): The rotation and
+ translation matrix between different coordinates.
+ Defaults to None.
+ The conversion from `src` coordinates to `dst` coordinates
+ usually comes along the change of sensors, e.g., from camera
+ to LiDAR. This requires a transformation matrix.
+ with_yaw (bool, optional): If `box` is an instance of
+ :obj:`BaseInstance3DBoxes`, whether or not it has a yaw angle.
+ Defaults to True.
+
+ Returns:
+ (tuple | list | np.ndarray | torch.Tensor |
+ :obj:`BaseInstance3DBoxes`):
+ The converted box of the same type.
+ """
+ if src == dst:
+ return box
+
+ is_numpy = isinstance(box, np.ndarray)
+ is_Instance3DBoxes = isinstance(box, BaseInstance3DBoxes)
+ single_box = isinstance(box, (list, tuple))
+ if single_box:
+ assert len(box) >= 7, (
+ 'Box3DMode.convert takes either a k-tuple/list or '
+ 'an Nxk array/tensor, where k >= 7')
+ arr = torch.tensor(box)[None, :]
+ else:
+ # avoid modifying the input box
+ if is_numpy:
+ arr = torch.from_numpy(np.asarray(box)).clone()
+ elif is_Instance3DBoxes:
+ arr = box.tensor.clone()
+ else:
+ arr = box.clone()
+
+ if is_Instance3DBoxes:
+ with_yaw = box.with_yaw
+
+ # convert box from `src` mode to `dst` mode.
+ x_size, y_size, z_size = arr[..., 3:4], arr[..., 4:5], arr[..., 5:6]
+ if with_yaw:
+ yaw = arr[..., 6:7]
+ if src == Box3DMode.LIDAR and dst == Box3DMode.CAM:
+ if rt_mat is None:
+ rt_mat = arr.new_tensor([[0, -1, 0], [0, 0, -1], [1, 0, 0]])
+ xyz_size = torch.cat([x_size, z_size, y_size], dim=-1)
+ if with_yaw:
+ yaw = -yaw - np.pi / 2
+ yaw = limit_period(yaw, period=np.pi * 2)
+ elif src == Box3DMode.CAM and dst == Box3DMode.LIDAR:
+ if rt_mat is None:
+ rt_mat = arr.new_tensor([[0, 0, 1], [-1, 0, 0], [0, -1, 0]])
+ xyz_size = torch.cat([x_size, z_size, y_size], dim=-1)
+ if with_yaw:
+ yaw = -yaw - np.pi / 2
+ yaw = limit_period(yaw, period=np.pi * 2)
+ elif src == Box3DMode.DEPTH and dst == Box3DMode.CAM:
+ if rt_mat is None:
+ rt_mat = arr.new_tensor([[1, 0, 0], [0, 0, -1], [0, 1, 0]])
+ xyz_size = torch.cat([x_size, z_size, y_size], dim=-1)
+ if with_yaw:
+ yaw = -yaw
+ elif src == Box3DMode.CAM and dst == Box3DMode.DEPTH:
+ if rt_mat is None:
+ rt_mat = arr.new_tensor([[1, 0, 0], [0, 0, 1], [0, -1, 0]])
+ xyz_size = torch.cat([x_size, z_size, y_size], dim=-1)
+ if with_yaw:
+ yaw = -yaw
+ elif src == Box3DMode.LIDAR and dst == Box3DMode.DEPTH:
+ if rt_mat is None:
+ rt_mat = arr.new_tensor([[0, -1, 0], [1, 0, 0], [0, 0, 1]])
+ xyz_size = torch.cat([x_size, y_size, z_size], dim=-1)
+ if with_yaw:
+ yaw = yaw + np.pi / 2
+ yaw = limit_period(yaw, period=np.pi * 2)
+ elif src == Box3DMode.DEPTH and dst == Box3DMode.LIDAR:
+ if rt_mat is None:
+ rt_mat = arr.new_tensor([[0, 1, 0], [-1, 0, 0], [0, 0, 1]])
+ xyz_size = torch.cat([x_size, y_size, z_size], dim=-1)
+ if with_yaw:
+ yaw = yaw - np.pi / 2
+ yaw = limit_period(yaw, period=np.pi * 2)
+ else:
+ raise NotImplementedError(
+ f'Conversion from Box3DMode {src} to {dst} '
+ 'is not supported yet')
+
+ if not isinstance(rt_mat, torch.Tensor):
+ rt_mat = arr.new_tensor(rt_mat)
+ if rt_mat.size(1) == 4:
+ extended_xyz = torch.cat(
+ [arr[..., :3], arr.new_ones(arr.size(0), 1)], dim=-1)
+ xyz = extended_xyz @ rt_mat.t()
+ else:
+ xyz = arr[..., :3] @ rt_mat.t()
+
+ if with_yaw:
+ remains = arr[..., 7:]
+ arr = torch.cat([xyz[..., :3], xyz_size, yaw, remains], dim=-1)
+ else:
+ remains = arr[..., 6:]
+ arr = torch.cat([xyz[..., :3], xyz_size, remains], dim=-1)
+
+ # convert arr to the original type
+ original_type = type(box)
+ if single_box:
+ return original_type(arr.flatten().tolist())
+ if is_numpy:
+ return arr.numpy()
+ elif is_Instance3DBoxes:
+ if dst == Box3DMode.CAM:
+ target_type = CameraInstance3DBoxes
+ elif dst == Box3DMode.LIDAR:
+ target_type = LiDARInstance3DBoxes
+ elif dst == Box3DMode.DEPTH:
+ target_type = DepthInstance3DBoxes
+ else:
+ raise NotImplementedError(
+ f'Conversion to {dst} through {original_type}'
+ ' is not supported yet')
+ return target_type(arr, box_dim=arr.size(-1), with_yaw=with_yaw)
+ else:
+ return arr
diff --git a/mmdet3d/core/bbox/structures/cam_box3d.py b/mmdet3d/core/bbox/structures/cam_box3d.py
new file mode 100644
index 0000000..b708613
--- /dev/null
+++ b/mmdet3d/core/bbox/structures/cam_box3d.py
@@ -0,0 +1,354 @@
+# Copyright (c) OpenMMLab. All rights reserved.
+import numpy as np
+import torch
+
+from ...points import BasePoints
+from .base_box3d import BaseInstance3DBoxes
+from .utils import rotation_3d_in_axis, yaw2local
+
+
+class CameraInstance3DBoxes(BaseInstance3DBoxes):
+ """3D boxes of instances in CAM coordinates.
+
+ Coordinates in camera:
+
+ .. code-block:: none
+
+ z front (yaw=-0.5*pi)
+ /
+ /
+ 0 ------> x right (yaw=0)
+ |
+ |
+ v
+ down y
+
+ The relative coordinate of bottom center in a CAM box is (0.5, 1.0, 0.5),
+ and the yaw is around the y axis, thus the rotation axis=1.
+ The yaw is 0 at the positive direction of x axis, and decreases from
+ the positive direction of x to the positive direction of z.
+
+ Attributes:
+ tensor (torch.Tensor): Float matrix in shape (N, box_dim).
+ box_dim (int): Integer indicating the dimension of a box
+ Each row is (x, y, z, x_size, y_size, z_size, yaw, ...).
+ with_yaw (bool): If True, the value of yaw will be set to 0 as
+ axis-aligned boxes tightly enclosing the original boxes.
+ """
+ YAW_AXIS = 1
+
+ def __init__(self,
+ tensor,
+ box_dim=7,
+ with_yaw=True,
+ origin=(0.5, 1.0, 0.5)):
+ if isinstance(tensor, torch.Tensor):
+ device = tensor.device
+ else:
+ device = torch.device('cpu')
+ tensor = torch.as_tensor(tensor, dtype=torch.float32, device=device)
+ if tensor.numel() == 0:
+ # Use reshape, so we don't end up creating a new tensor that
+ # does not depend on the inputs (and consequently confuses jit)
+ tensor = tensor.reshape((0, box_dim)).to(
+ dtype=torch.float32, device=device)
+ assert tensor.dim() == 2 and tensor.size(-1) == box_dim, tensor.size()
+
+ if tensor.shape[-1] == 6:
+ # If the dimension of boxes is 6, we expand box_dim by padding
+ # 0 as a fake yaw and set with_yaw to False.
+ assert box_dim == 6
+ fake_rot = tensor.new_zeros(tensor.shape[0], 1)
+ tensor = torch.cat((tensor, fake_rot), dim=-1)
+ self.box_dim = box_dim + 1
+ self.with_yaw = False
+ else:
+ self.box_dim = box_dim
+ self.with_yaw = with_yaw
+ self.tensor = tensor.clone()
+
+ if origin != (0.5, 1.0, 0.5):
+ dst = self.tensor.new_tensor((0.5, 1.0, 0.5))
+ src = self.tensor.new_tensor(origin)
+ self.tensor[:, :3] += self.tensor[:, 3:6] * (dst - src)
+
+ @property
+ def height(self):
+ """torch.Tensor: A vector with height of each box in shape (N, )."""
+ return self.tensor[:, 4]
+
+ @property
+ def top_height(self):
+ """torch.Tensor:
+ A vector with the top height of each box in shape (N, )."""
+ # the positive direction is down rather than up
+ return self.bottom_height - self.height
+
+ @property
+ def bottom_height(self):
+ """torch.Tensor:
+ A vector with bottom's height of each box in shape (N, )."""
+ return self.tensor[:, 1]
+
+ @property
+ def local_yaw(self):
+ """torch.Tensor:
+ A vector with local yaw of each box in shape (N, ).
+ local_yaw equals to alpha in kitti, which is commonly
+ used in monocular 3D object detection task, so only
+ :obj:`CameraInstance3DBoxes` has the property.
+ """
+ yaw = self.yaw
+ loc = self.gravity_center
+ local_yaw = yaw2local(yaw, loc)
+
+ return local_yaw
+
+ @property
+ def gravity_center(self):
+ """torch.Tensor: A tensor with center of each box in shape (N, 3)."""
+ bottom_center = self.bottom_center
+ gravity_center = torch.zeros_like(bottom_center)
+ gravity_center[:, [0, 2]] = bottom_center[:, [0, 2]]
+ gravity_center[:, 1] = bottom_center[:, 1] - self.tensor[:, 4] * 0.5
+ return gravity_center
+
+ @property
+ def corners(self):
+ """torch.Tensor: Coordinates of corners of all the boxes in
+ shape (N, 8, 3).
+
+ Convert the boxes to in clockwise order, in the form of
+ (x0y0z0, x0y0z1, x0y1z1, x0y1z0, x1y0z0, x1y0z1, x1y1z1, x1y1z0)
+
+ .. code-block:: none
+
+ front z
+ /
+ /
+ (x0, y0, z1) + ----------- + (x1, y0, z1)
+ /| / |
+ / | / |
+ (x0, y0, z0) + ----------- + + (x1, y1, z1)
+ | / . | /
+ | / origin | /
+ (x0, y1, z0) + ----------- + -------> x right
+ | (x1, y1, z0)
+ |
+ v
+ down y
+ """
+ if self.tensor.numel() == 0:
+ return torch.empty([0, 8, 3], device=self.tensor.device)
+
+ dims = self.dims
+ corners_norm = torch.from_numpy(
+ np.stack(np.unravel_index(np.arange(8), [2] * 3), axis=1)).to(
+ device=dims.device, dtype=dims.dtype)
+
+ corners_norm = corners_norm[[0, 1, 3, 2, 4, 5, 7, 6]]
+ # use relative origin [0.5, 1, 0.5]
+ corners_norm = corners_norm - dims.new_tensor([0.5, 1, 0.5])
+ corners = dims.view([-1, 1, 3]) * corners_norm.reshape([1, 8, 3])
+
+ corners = rotation_3d_in_axis(
+ corners, self.tensor[:, 6], axis=self.YAW_AXIS)
+ corners += self.tensor[:, :3].view(-1, 1, 3)
+ return corners
+
+ @property
+ def bev(self):
+ """torch.Tensor: 2D BEV box of each box with rotation
+ in XYWHR format, in shape (N, 5)."""
+ bev = self.tensor[:, [0, 2, 3, 5, 6]].clone()
+ # positive direction of the gravity axis
+ # in cam coord system points to the earth
+ # so the bev yaw angle needs to be reversed
+ bev[:, -1] = -bev[:, -1]
+ return bev
+
+ def rotate(self, angle, points=None):
+ """Rotate boxes with points (optional) with the given angle or rotation
+ matrix.
+
+ Args:
+ angle (float | torch.Tensor | np.ndarray):
+ Rotation angle or rotation matrix.
+ points (torch.Tensor | np.ndarray | :obj:`BasePoints`, optional):
+ Points to rotate. Defaults to None.
+
+ Returns:
+ tuple or None: When ``points`` is None, the function returns
+ None, otherwise it returns the rotated points and the
+ rotation matrix ``rot_mat_T``.
+ """
+ if not isinstance(angle, torch.Tensor):
+ angle = self.tensor.new_tensor(angle)
+
+ assert angle.shape == torch.Size([3, 3]) or angle.numel() == 1, \
+ f'invalid rotation angle shape {angle.shape}'
+
+ if angle.numel() == 1:
+ self.tensor[:, 0:3], rot_mat_T = rotation_3d_in_axis(
+ self.tensor[:, 0:3],
+ angle,
+ axis=self.YAW_AXIS,
+ return_mat=True)
+ else:
+ rot_mat_T = angle
+ rot_sin = rot_mat_T[2, 0]
+ rot_cos = rot_mat_T[0, 0]
+ angle = np.arctan2(rot_sin, rot_cos)
+ self.tensor[:, 0:3] = self.tensor[:, 0:3] @ rot_mat_T
+
+ self.tensor[:, 6] += angle
+
+ if points is not None:
+ if isinstance(points, torch.Tensor):
+ points[:, :3] = points[:, :3] @ rot_mat_T
+ elif isinstance(points, np.ndarray):
+ rot_mat_T = rot_mat_T.cpu().numpy()
+ points[:, :3] = np.dot(points[:, :3], rot_mat_T)
+ elif isinstance(points, BasePoints):
+ points.rotate(rot_mat_T)
+ else:
+ raise ValueError
+ return points, rot_mat_T
+
+ def flip(self, bev_direction='horizontal', points=None):
+ """Flip the boxes in BEV along given BEV direction.
+
+ In CAM coordinates, it flips the x (horizontal) or z (vertical) axis.
+
+ Args:
+ bev_direction (str): Flip direction (horizontal or vertical).
+ points (torch.Tensor | np.ndarray | :obj:`BasePoints`, optional):
+ Points to flip. Defaults to None.
+
+ Returns:
+ torch.Tensor, numpy.ndarray or None: Flipped points.
+ """
+ assert bev_direction in ('horizontal', 'vertical')
+ if bev_direction == 'horizontal':
+ self.tensor[:, 0::7] = -self.tensor[:, 0::7]
+ if self.with_yaw:
+ self.tensor[:, 6] = -self.tensor[:, 6] + np.pi
+ elif bev_direction == 'vertical':
+ self.tensor[:, 2::7] = -self.tensor[:, 2::7]
+ if self.with_yaw:
+ self.tensor[:, 6] = -self.tensor[:, 6]
+
+ if points is not None:
+ assert isinstance(points, (torch.Tensor, np.ndarray, BasePoints))
+ if isinstance(points, (torch.Tensor, np.ndarray)):
+ if bev_direction == 'horizontal':
+ points[:, 0] = -points[:, 0]
+ elif bev_direction == 'vertical':
+ points[:, 2] = -points[:, 2]
+ elif isinstance(points, BasePoints):
+ points.flip(bev_direction)
+ return points
+
+ @classmethod
+ def height_overlaps(cls, boxes1, boxes2, mode='iou'):
+ """Calculate height overlaps of two boxes.
+
+ This function calculates the height overlaps between ``boxes1`` and
+ ``boxes2``, where ``boxes1`` and ``boxes2`` should be in the same type.
+
+ Args:
+ boxes1 (:obj:`CameraInstance3DBoxes`): Boxes 1 contain N boxes.
+ boxes2 (:obj:`CameraInstance3DBoxes`): Boxes 2 contain M boxes.
+ mode (str, optional): Mode of iou calculation. Defaults to 'iou'.
+
+ Returns:
+ torch.Tensor: Calculated iou of boxes' heights.
+ """
+ assert isinstance(boxes1, CameraInstance3DBoxes)
+ assert isinstance(boxes2, CameraInstance3DBoxes)
+
+ boxes1_top_height = boxes1.top_height.view(-1, 1)
+ boxes1_bottom_height = boxes1.bottom_height.view(-1, 1)
+ boxes2_top_height = boxes2.top_height.view(1, -1)
+ boxes2_bottom_height = boxes2.bottom_height.view(1, -1)
+
+ # positive direction of the gravity axis
+ # in cam coord system points to the earth
+ heighest_of_bottom = torch.min(boxes1_bottom_height,
+ boxes2_bottom_height)
+ lowest_of_top = torch.max(boxes1_top_height, boxes2_top_height)
+ overlaps_h = torch.clamp(heighest_of_bottom - lowest_of_top, min=0)
+ return overlaps_h
+
+ def convert_to(self, dst, rt_mat=None):
+ """Convert self to ``dst`` mode.
+
+ Args:
+ dst (:obj:`Box3DMode`): The target Box mode.
+ rt_mat (np.ndarray | torch.Tensor, optional): The rotation and
+ translation matrix between different coordinates.
+ Defaults to None.
+ The conversion from ``src`` coordinates to ``dst`` coordinates
+ usually comes along the change of sensors, e.g., from camera
+ to LiDAR. This requires a transformation matrix.
+
+ Returns:
+ :obj:`BaseInstance3DBoxes`:
+ The converted box of the same type in the ``dst`` mode.
+ """
+ from .box_3d_mode import Box3DMode
+ return Box3DMode.convert(
+ box=self, src=Box3DMode.CAM, dst=dst, rt_mat=rt_mat)
+
+ def points_in_boxes_part(self, points, boxes_override=None):
+ """Find the box in which each point is.
+
+ Args:
+ points (torch.Tensor): Points in shape (1, M, 3) or (M, 3),
+ 3 dimensions are (x, y, z) in LiDAR or depth coordinate.
+ boxes_override (torch.Tensor, optional): Boxes to override
+ `self.tensor `. Defaults to None.
+
+ Returns:
+ torch.Tensor: The index of the box in which
+ each point is, in shape (M, ). Default value is -1
+ (if the point is not enclosed by any box).
+ """
+ from .coord_3d_mode import Coord3DMode
+
+ points_lidar = Coord3DMode.convert(points, Coord3DMode.CAM,
+ Coord3DMode.LIDAR)
+ if boxes_override is not None:
+ boxes_lidar = boxes_override
+ else:
+ boxes_lidar = Coord3DMode.convert(self.tensor, Coord3DMode.CAM,
+ Coord3DMode.LIDAR)
+
+ box_idx = super().points_in_boxes_part(points_lidar, boxes_lidar)
+ return box_idx
+
+ def points_in_boxes_all(self, points, boxes_override=None):
+ """Find all boxes in which each point is.
+
+ Args:
+ points (torch.Tensor): Points in shape (1, M, 3) or (M, 3),
+ 3 dimensions are (x, y, z) in LiDAR or depth coordinate.
+ boxes_override (torch.Tensor, optional): Boxes to override
+ `self.tensor `. Defaults to None.
+
+ Returns:
+ torch.Tensor: The index of all boxes in which each point is,
+ in shape (B, M, T).
+ """
+ from .coord_3d_mode import Coord3DMode
+
+ points_lidar = Coord3DMode.convert(points, Coord3DMode.CAM,
+ Coord3DMode.LIDAR)
+ if boxes_override is not None:
+ boxes_lidar = boxes_override
+ else:
+ boxes_lidar = Coord3DMode.convert(self.tensor, Coord3DMode.CAM,
+ Coord3DMode.LIDAR)
+
+ box_idx = super().points_in_boxes_all(points_lidar, boxes_lidar)
+ return box_idx
diff --git a/mmdet3d/core/bbox/structures/coord_3d_mode.py b/mmdet3d/core/bbox/structures/coord_3d_mode.py
new file mode 100644
index 0000000..6309b65
--- /dev/null
+++ b/mmdet3d/core/bbox/structures/coord_3d_mode.py
@@ -0,0 +1,234 @@
+# Copyright (c) OpenMMLab. All rights reserved.
+from enum import IntEnum, unique
+
+import numpy as np
+import torch
+
+from ...points import BasePoints, CameraPoints, DepthPoints, LiDARPoints
+from .base_box3d import BaseInstance3DBoxes
+from .box_3d_mode import Box3DMode
+
+
+@unique
+class Coord3DMode(IntEnum):
+ r"""Enum of different ways to represent a box
+ and point cloud.
+
+ Coordinates in LiDAR:
+
+ .. code-block:: none
+
+ up z
+ ^ x front
+ | /
+ | /
+ left y <------ 0
+
+ The relative coordinate of bottom center in a LiDAR box is (0.5, 0.5, 0),
+ and the yaw is around the z axis, thus the rotation axis=2.
+
+ Coordinates in camera:
+
+ .. code-block:: none
+
+ z front
+ /
+ /
+ 0 ------> x right
+ |
+ |
+ v
+ down y
+
+ The relative coordinate of bottom center in a CAM box is [0.5, 1.0, 0.5],
+ and the yaw is around the y axis, thus the rotation axis=1.
+
+ Coordinates in Depth mode:
+
+ .. code-block:: none
+
+ up z
+ ^ y front
+ | /
+ | /
+ 0 ------> x right
+
+ The relative coordinate of bottom center in a DEPTH box is (0.5, 0.5, 0),
+ and the yaw is around the z axis, thus the rotation axis=2.
+ """
+
+ LIDAR = 0
+ CAM = 1
+ DEPTH = 2
+
+ @staticmethod
+ def convert(input, src, dst, rt_mat=None, with_yaw=True, is_point=True):
+ """Convert boxes or points from `src` mode to `dst` mode.
+
+ Args:
+ input (tuple | list | np.ndarray | torch.Tensor |
+ :obj:`BaseInstance3DBoxes` | :obj:`BasePoints`):
+ Can be a k-tuple, k-list or an Nxk array/tensor, where k = 7.
+ src (:obj:`Box3DMode` | :obj:`Coord3DMode`): The source mode.
+ dst (:obj:`Box3DMode` | :obj:`Coord3DMode`): The target mode.
+ rt_mat (np.ndarray | torch.Tensor, optional): The rotation and
+ translation matrix between different coordinates.
+ Defaults to None.
+ The conversion from `src` coordinates to `dst` coordinates
+ usually comes along the change of sensors, e.g., from camera
+ to LiDAR. This requires a transformation matrix.
+ with_yaw (bool): If `box` is an instance of
+ :obj:`BaseInstance3DBoxes`, whether or not it has a yaw angle.
+ Defaults to True.
+ is_point (bool): If `input` is neither an instance of
+ :obj:`BaseInstance3DBoxes` nor an instance of
+ :obj:`BasePoints`, whether or not it is point data.
+ Defaults to True.
+
+ Returns:
+ (tuple | list | np.ndarray | torch.Tensor |
+ :obj:`BaseInstance3DBoxes` | :obj:`BasePoints`):
+ The converted box of the same type.
+ """
+ if isinstance(input, BaseInstance3DBoxes):
+ return Coord3DMode.convert_box(
+ input, src, dst, rt_mat=rt_mat, with_yaw=with_yaw)
+ elif isinstance(input, BasePoints):
+ return Coord3DMode.convert_point(input, src, dst, rt_mat=rt_mat)
+ elif isinstance(input, (tuple, list, np.ndarray, torch.Tensor)):
+ if is_point:
+ return Coord3DMode.convert_point(
+ input, src, dst, rt_mat=rt_mat)
+ else:
+ return Coord3DMode.convert_box(
+ input, src, dst, rt_mat=rt_mat, with_yaw=with_yaw)
+ else:
+ raise NotImplementedError
+
+ @staticmethod
+ def convert_box(box, src, dst, rt_mat=None, with_yaw=True):
+ """Convert boxes from `src` mode to `dst` mode.
+
+ Args:
+ box (tuple | list | np.ndarray |
+ torch.Tensor | :obj:`BaseInstance3DBoxes`):
+ Can be a k-tuple, k-list or an Nxk array/tensor, where k = 7.
+ src (:obj:`Box3DMode`): The src Box mode.
+ dst (:obj:`Box3DMode`): The target Box mode.
+ rt_mat (np.ndarray | torch.Tensor, optional): The rotation and
+ translation matrix between different coordinates.
+ Defaults to None.
+ The conversion from `src` coordinates to `dst` coordinates
+ usually comes along the change of sensors, e.g., from camera
+ to LiDAR. This requires a transformation matrix.
+ with_yaw (bool): If `box` is an instance of
+ :obj:`BaseInstance3DBoxes`, whether or not it has a yaw angle.
+ Defaults to True.
+
+ Returns:
+ (tuple | list | np.ndarray | torch.Tensor |
+ :obj:`BaseInstance3DBoxes`):
+ The converted box of the same type.
+ """
+ return Box3DMode.convert(box, src, dst, rt_mat=rt_mat)
+
+ @staticmethod
+ def convert_point(point, src, dst, rt_mat=None):
+ """Convert points from `src` mode to `dst` mode.
+
+ Args:
+ point (tuple | list | np.ndarray |
+ torch.Tensor | :obj:`BasePoints`):
+ Can be a k-tuple, k-list or an Nxk array/tensor.
+ src (:obj:`CoordMode`): The src Point mode.
+ dst (:obj:`CoordMode`): The target Point mode.
+ rt_mat (np.ndarray | torch.Tensor, optional): The rotation and
+ translation matrix between different coordinates.
+ Defaults to None.
+ The conversion from `src` coordinates to `dst` coordinates
+ usually comes along the change of sensors, e.g., from camera
+ to LiDAR. This requires a transformation matrix.
+
+ Returns:
+ (tuple | list | np.ndarray | torch.Tensor | :obj:`BasePoints`):
+ The converted point of the same type.
+ """
+ if src == dst:
+ return point
+
+ is_numpy = isinstance(point, np.ndarray)
+ is_InstancePoints = isinstance(point, BasePoints)
+ single_point = isinstance(point, (list, tuple))
+ if single_point:
+ assert len(point) >= 3, (
+ 'CoordMode.convert takes either a k-tuple/list or '
+ 'an Nxk array/tensor, where k >= 3')
+ arr = torch.tensor(point)[None, :]
+ else:
+ # avoid modifying the input point
+ if is_numpy:
+ arr = torch.from_numpy(np.asarray(point)).clone()
+ elif is_InstancePoints:
+ arr = point.tensor.clone()
+ else:
+ arr = point.clone()
+
+ # convert point from `src` mode to `dst` mode.
+ if src == Coord3DMode.LIDAR and dst == Coord3DMode.CAM:
+ if rt_mat is None:
+ rt_mat = arr.new_tensor([[0, -1, 0], [0, 0, -1], [1, 0, 0]])
+ elif src == Coord3DMode.CAM and dst == Coord3DMode.LIDAR:
+ if rt_mat is None:
+ rt_mat = arr.new_tensor([[0, 0, 1], [-1, 0, 0], [0, -1, 0]])
+ elif src == Coord3DMode.DEPTH and dst == Coord3DMode.CAM:
+ if rt_mat is None:
+ rt_mat = arr.new_tensor([[1, 0, 0], [0, 0, -1], [0, 1, 0]])
+ elif src == Coord3DMode.CAM and dst == Coord3DMode.DEPTH:
+ if rt_mat is None:
+ rt_mat = arr.new_tensor([[1, 0, 0], [0, 0, 1], [0, -1, 0]])
+ elif src == Coord3DMode.LIDAR and dst == Coord3DMode.DEPTH:
+ if rt_mat is None:
+ rt_mat = arr.new_tensor([[0, -1, 0], [1, 0, 0], [0, 0, 1]])
+ elif src == Coord3DMode.DEPTH and dst == Coord3DMode.LIDAR:
+ if rt_mat is None:
+ rt_mat = arr.new_tensor([[0, 1, 0], [-1, 0, 0], [0, 0, 1]])
+ else:
+ raise NotImplementedError(
+ f'Conversion from Coord3DMode {src} to {dst} '
+ 'is not supported yet')
+
+ if not isinstance(rt_mat, torch.Tensor):
+ rt_mat = arr.new_tensor(rt_mat)
+ if rt_mat.size(1) == 4:
+ extended_xyz = torch.cat(
+ [arr[..., :3], arr.new_ones(arr.size(0), 1)], dim=-1)
+ xyz = extended_xyz @ rt_mat.t()
+ else:
+ xyz = arr[..., :3] @ rt_mat.t()
+
+ remains = arr[..., 3:]
+ arr = torch.cat([xyz[..., :3], remains], dim=-1)
+
+ # convert arr to the original type
+ original_type = type(point)
+ if single_point:
+ return original_type(arr.flatten().tolist())
+ if is_numpy:
+ return arr.numpy()
+ elif is_InstancePoints:
+ if dst == Coord3DMode.CAM:
+ target_type = CameraPoints
+ elif dst == Coord3DMode.LIDAR:
+ target_type = LiDARPoints
+ elif dst == Coord3DMode.DEPTH:
+ target_type = DepthPoints
+ else:
+ raise NotImplementedError(
+ f'Conversion to {dst} through {original_type}'
+ ' is not supported yet')
+ return target_type(
+ arr,
+ points_dim=arr.size(-1),
+ attribute_dims=point.attribute_dims)
+ else:
+ return arr
diff --git a/mmdet3d/core/bbox/structures/depth_box3d.py b/mmdet3d/core/bbox/structures/depth_box3d.py
new file mode 100644
index 0000000..dd9278b
--- /dev/null
+++ b/mmdet3d/core/bbox/structures/depth_box3d.py
@@ -0,0 +1,270 @@
+# Copyright (c) OpenMMLab. All rights reserved.
+import numpy as np
+import torch
+
+from mmdet3d.core.points import BasePoints
+from .base_box3d import BaseInstance3DBoxes
+from .utils import rotation_3d_in_axis
+
+
+class DepthInstance3DBoxes(BaseInstance3DBoxes):
+ """3D boxes of instances in Depth coordinates.
+
+ Coordinates in Depth:
+
+ .. code-block:: none
+
+ up z y front (yaw=-0.5*pi)
+ ^ ^
+ | /
+ | /
+ 0 ------> x right (yaw=0)
+
+ The relative coordinate of bottom center in a Depth box is (0.5, 0.5, 0),
+ and the yaw is around the z axis, thus the rotation axis=2.
+ The yaw is 0 at the positive direction of x axis, and decreases from
+ the positive direction of x to the positive direction of y.
+ Also note that rotation of DepthInstance3DBoxes is counterclockwise,
+ which is reverse to the definition of the yaw angle (clockwise).
+
+ A refactor is ongoing to make the three coordinate systems
+ easier to understand and convert between each other.
+
+ Attributes:
+ tensor (torch.Tensor): Float matrix of N x box_dim.
+ box_dim (int): Integer indicates the dimension of a box
+ Each row is (x, y, z, x_size, y_size, z_size, yaw, ...).
+ with_yaw (bool): If True, the value of yaw will be set to 0 as minmax
+ boxes.
+ """
+ YAW_AXIS = 2
+
+ @property
+ def gravity_center(self):
+ """torch.Tensor: A tensor with center of each box in shape (N, 3)."""
+ bottom_center = self.bottom_center
+ gravity_center = torch.zeros_like(bottom_center)
+ gravity_center[:, :2] = bottom_center[:, :2]
+ gravity_center[:, 2] = bottom_center[:, 2] + self.tensor[:, 5] * 0.5
+ return gravity_center
+
+ @property
+ def corners(self):
+ """torch.Tensor: Coordinates of corners of all the boxes
+ in shape (N, 8, 3).
+
+ Convert the boxes to corners in clockwise order, in form of
+ ``(x0y0z0, x0y0z1, x0y1z1, x0y1z0, x1y0z0, x1y0z1, x1y1z1, x1y1z0)``
+
+ .. code-block:: none
+
+ up z
+ front y ^
+ / |
+ / |
+ (x0, y1, z1) + ----------- + (x1, y1, z1)
+ /| / |
+ / | / |
+ (x0, y0, z1) + ----------- + + (x1, y1, z0)
+ | / . | /
+ | / origin | /
+ (x0, y0, z0) + ----------- + --------> right x
+ (x1, y0, z0)
+ """
+ if self.tensor.numel() == 0:
+ return torch.empty([0, 8, 3], device=self.tensor.device)
+
+ dims = self.dims
+ corners_norm = torch.from_numpy(
+ np.stack(np.unravel_index(np.arange(8), [2] * 3), axis=1)).to(
+ device=dims.device, dtype=dims.dtype)
+
+ corners_norm = corners_norm[[0, 1, 3, 2, 4, 5, 7, 6]]
+ # use relative origin (0.5, 0.5, 0)
+ corners_norm = corners_norm - dims.new_tensor([0.5, 0.5, 0])
+ corners = dims.view([-1, 1, 3]) * corners_norm.reshape([1, 8, 3])
+
+ # rotate around z axis
+ corners = rotation_3d_in_axis(
+ corners, self.tensor[:, 6], axis=self.YAW_AXIS)
+ corners += self.tensor[:, :3].view(-1, 1, 3)
+ return corners
+
+ def rotate(self, angle, points=None):
+ """Rotate boxes with points (optional) with the given angle or rotation
+ matrix.
+
+ Args:
+ angle (float | torch.Tensor | np.ndarray):
+ Rotation angle or rotation matrix.
+ points (torch.Tensor | np.ndarray | :obj:`BasePoints`, optional):
+ Points to rotate. Defaults to None.
+
+ Returns:
+ tuple or None: When ``points`` is None, the function returns
+ None, otherwise it returns the rotated points and the
+ rotation matrix ``rot_mat_T``.
+ """
+ if not isinstance(angle, torch.Tensor):
+ angle = self.tensor.new_tensor(angle)
+
+ assert angle.shape == torch.Size([3, 3]) or angle.numel() == 1, \
+ f'invalid rotation angle shape {angle.shape}'
+
+ if angle.numel() == 1:
+ self.tensor[:, 0:3], rot_mat_T = rotation_3d_in_axis(
+ self.tensor[:, 0:3],
+ angle,
+ axis=self.YAW_AXIS,
+ return_mat=True)
+ else:
+ rot_mat_T = angle
+ rot_sin = rot_mat_T[0, 1]
+ rot_cos = rot_mat_T[0, 0]
+ angle = np.arctan2(rot_sin, rot_cos)
+ self.tensor[:, 0:3] = self.tensor[:, 0:3] @ rot_mat_T
+
+ if self.with_yaw:
+ self.tensor[:, 6] += angle
+ else:
+ # for axis-aligned boxes, we take the new
+ # enclosing axis-aligned boxes after rotation
+ corners_rot = self.corners @ rot_mat_T
+ new_x_size = corners_rot[..., 0].max(
+ dim=1, keepdim=True)[0] - corners_rot[..., 0].min(
+ dim=1, keepdim=True)[0]
+ new_y_size = corners_rot[..., 1].max(
+ dim=1, keepdim=True)[0] - corners_rot[..., 1].min(
+ dim=1, keepdim=True)[0]
+ self.tensor[:, 3:5] = torch.cat((new_x_size, new_y_size), dim=-1)
+
+ if points is not None:
+ if isinstance(points, torch.Tensor):
+ points[:, :3] = points[:, :3] @ rot_mat_T
+ elif isinstance(points, np.ndarray):
+ rot_mat_T = rot_mat_T.cpu().numpy()
+ points[:, :3] = np.dot(points[:, :3], rot_mat_T)
+ elif isinstance(points, BasePoints):
+ points.rotate(rot_mat_T)
+ else:
+ raise ValueError
+ return points, rot_mat_T
+
+ def flip(self, bev_direction='horizontal', points=None):
+ """Flip the boxes in BEV along given BEV direction.
+
+ In Depth coordinates, it flips x (horizontal) or y (vertical) axis.
+
+ Args:
+ bev_direction (str, optional): Flip direction
+ (horizontal or vertical). Defaults to 'horizontal'.
+ points (torch.Tensor | np.ndarray | :obj:`BasePoints`, optional):
+ Points to flip. Defaults to None.
+
+ Returns:
+ torch.Tensor, numpy.ndarray or None: Flipped points.
+ """
+ assert bev_direction in ('horizontal', 'vertical')
+ if bev_direction == 'horizontal':
+ self.tensor[:, 0::7] = -self.tensor[:, 0::7]
+ if self.with_yaw:
+ self.tensor[:, 6] = -self.tensor[:, 6] + np.pi
+ elif bev_direction == 'vertical':
+ self.tensor[:, 1::7] = -self.tensor[:, 1::7]
+ if self.with_yaw:
+ self.tensor[:, 6] = -self.tensor[:, 6]
+
+ if points is not None:
+ assert isinstance(points, (torch.Tensor, np.ndarray, BasePoints))
+ if isinstance(points, (torch.Tensor, np.ndarray)):
+ if bev_direction == 'horizontal':
+ points[:, 0] = -points[:, 0]
+ elif bev_direction == 'vertical':
+ points[:, 1] = -points[:, 1]
+ elif isinstance(points, BasePoints):
+ points.flip(bev_direction)
+ return points
+
+ def convert_to(self, dst, rt_mat=None):
+ """Convert self to ``dst`` mode.
+
+ Args:
+ dst (:obj:`Box3DMode`): The target Box mode.
+ rt_mat (np.ndarray | torch.Tensor, optional): The rotation and
+ translation matrix between different coordinates.
+ Defaults to None.
+ The conversion from ``src`` coordinates to ``dst`` coordinates
+ usually comes along the change of sensors, e.g., from camera
+ to LiDAR. This requires a transformation matrix.
+
+ Returns:
+ :obj:`DepthInstance3DBoxes`:
+ The converted box of the same type in the ``dst`` mode.
+ """
+ from .box_3d_mode import Box3DMode
+ return Box3DMode.convert(
+ box=self, src=Box3DMode.DEPTH, dst=dst, rt_mat=rt_mat)
+
+ def enlarged_box(self, extra_width):
+ """Enlarge the length, width and height boxes.
+
+ Args:
+ extra_width (float | torch.Tensor): Extra width to enlarge the box.
+
+ Returns:
+ :obj:`DepthInstance3DBoxes`: Enlarged boxes.
+ """
+ enlarged_boxes = self.tensor.clone()
+ enlarged_boxes[:, 3:6] += extra_width * 2
+ # bottom center z minus extra_width
+ enlarged_boxes[:, 2] -= extra_width
+ return self.new_box(enlarged_boxes)
+
+ def get_surface_line_center(self):
+ """Compute surface and line center of bounding boxes.
+
+ Returns:
+ torch.Tensor: Surface and line center of bounding boxes.
+ """
+ obj_size = self.dims
+ center = self.gravity_center.view(-1, 1, 3)
+ batch_size = center.shape[0]
+
+ rot_sin = torch.sin(-self.yaw)
+ rot_cos = torch.cos(-self.yaw)
+ rot_mat_T = self.yaw.new_zeros(tuple(list(self.yaw.shape) + [3, 3]))
+ rot_mat_T[..., 0, 0] = rot_cos
+ rot_mat_T[..., 0, 1] = -rot_sin
+ rot_mat_T[..., 1, 0] = rot_sin
+ rot_mat_T[..., 1, 1] = rot_cos
+ rot_mat_T[..., 2, 2] = 1
+
+ # Get the object surface center
+ offset = obj_size.new_tensor([[0, 0, 1], [0, 0, -1], [0, 1, 0],
+ [0, -1, 0], [1, 0, 0], [-1, 0, 0]])
+ offset = offset.view(1, 6, 3) / 2
+ surface_3d = (offset *
+ obj_size.view(batch_size, 1, 3).repeat(1, 6, 1)).reshape(
+ -1, 3)
+
+ # Get the object line center
+ offset = obj_size.new_tensor([[1, 0, 1], [-1, 0, 1], [0, 1, 1],
+ [0, -1, 1], [1, 0, -1], [-1, 0, -1],
+ [0, 1, -1], [0, -1, -1], [1, 1, 0],
+ [1, -1, 0], [-1, 1, 0], [-1, -1, 0]])
+ offset = offset.view(1, 12, 3) / 2
+
+ line_3d = (offset *
+ obj_size.view(batch_size, 1, 3).repeat(1, 12, 1)).reshape(
+ -1, 3)
+
+ surface_rot = rot_mat_T.repeat(6, 1, 1)
+ surface_3d = torch.matmul(surface_3d.unsqueeze(-2),
+ surface_rot).squeeze(-2)
+ surface_center = center.repeat(1, 6, 1).reshape(-1, 3) + surface_3d
+
+ line_rot = rot_mat_T.repeat(12, 1, 1)
+ line_3d = torch.matmul(line_3d.unsqueeze(-2), line_rot).squeeze(-2)
+ line_center = center.repeat(1, 12, 1).reshape(-1, 3) + line_3d
+
+ return surface_center, line_center
diff --git a/mmdet3d/core/bbox/structures/lidar_box3d.py b/mmdet3d/core/bbox/structures/lidar_box3d.py
new file mode 100644
index 0000000..706a6c0
--- /dev/null
+++ b/mmdet3d/core/bbox/structures/lidar_box3d.py
@@ -0,0 +1,210 @@
+# Copyright (c) OpenMMLab. All rights reserved.
+import numpy as np
+import torch
+
+from mmdet3d.core.points import BasePoints
+from .base_box3d import BaseInstance3DBoxes
+from .utils import rotation_3d_in_axis
+
+
+class LiDARInstance3DBoxes(BaseInstance3DBoxes):
+ """3D boxes of instances in LIDAR coordinates.
+
+ Coordinates in LiDAR:
+
+ .. code-block:: none
+
+ up z x front (yaw=0)
+ ^ ^
+ | /
+ | /
+ (yaw=0.5*pi) left y <------ 0
+
+ The relative coordinate of bottom center in a LiDAR box is (0.5, 0.5, 0),
+ and the yaw is around the z axis, thus the rotation axis=2.
+ The yaw is 0 at the positive direction of x axis, and increases from
+ the positive direction of x to the positive direction of y.
+
+ A refactor is ongoing to make the three coordinate systems
+ easier to understand and convert between each other.
+
+ Attributes:
+ tensor (torch.Tensor): Float matrix of N x box_dim.
+ box_dim (int): Integer indicating the dimension of a box.
+ Each row is (x, y, z, x_size, y_size, z_size, yaw, ...).
+ with_yaw (bool): If True, the value of yaw will be set to 0 as minmax
+ boxes.
+ """
+ YAW_AXIS = 2
+
+ @property
+ def gravity_center(self):
+ """torch.Tensor: A tensor with center of each box in shape (N, 3)."""
+ bottom_center = self.bottom_center
+ gravity_center = torch.zeros_like(bottom_center)
+ gravity_center[:, :2] = bottom_center[:, :2]
+ gravity_center[:, 2] = bottom_center[:, 2] + self.tensor[:, 5] * 0.5
+ return gravity_center
+
+ @property
+ def corners(self):
+ """torch.Tensor: Coordinates of corners of all the boxes
+ in shape (N, 8, 3).
+
+ Convert the boxes to corners in clockwise order, in form of
+ ``(x0y0z0, x0y0z1, x0y1z1, x0y1z0, x1y0z0, x1y0z1, x1y1z1, x1y1z0)``
+
+ .. code-block:: none
+
+ up z
+ front x ^
+ / |
+ / |
+ (x1, y0, z1) + ----------- + (x1, y1, z1)
+ /| / |
+ / | / |
+ (x0, y0, z1) + ----------- + + (x1, y1, z0)
+ | / . | /
+ | / origin | /
+ left y<-------- + ----------- + (x0, y1, z0)
+ (x0, y0, z0)
+ """
+ if self.tensor.numel() == 0:
+ return torch.empty([0, 8, 3], device=self.tensor.device)
+
+ dims = self.dims
+ corners_norm = torch.from_numpy(
+ np.stack(np.unravel_index(np.arange(8), [2] * 3), axis=1)).to(
+ device=dims.device, dtype=dims.dtype)
+
+ corners_norm = corners_norm[[0, 1, 3, 2, 4, 5, 7, 6]]
+ # use relative origin [0.5, 0.5, 0]
+ corners_norm = corners_norm - dims.new_tensor([0.5, 0.5, 0])
+ corners = dims.view([-1, 1, 3]) * corners_norm.reshape([1, 8, 3])
+
+ # rotate around z axis
+ corners = rotation_3d_in_axis(
+ corners, self.tensor[:, 6], axis=self.YAW_AXIS)
+ corners += self.tensor[:, :3].view(-1, 1, 3)
+ return corners
+
+ def rotate(self, angle, points=None):
+ """Rotate boxes with points (optional) with the given angle or rotation
+ matrix.
+
+ Args:
+ angles (float | torch.Tensor | np.ndarray):
+ Rotation angle or rotation matrix.
+ points (torch.Tensor | np.ndarray | :obj:`BasePoints`, optional):
+ Points to rotate. Defaults to None.
+
+ Returns:
+ tuple or None: When ``points`` is None, the function returns
+ None, otherwise it returns the rotated points and the
+ rotation matrix ``rot_mat_T``.
+ """
+ if not isinstance(angle, torch.Tensor):
+ angle = self.tensor.new_tensor(angle)
+
+ assert angle.shape == torch.Size([3, 3]) or angle.numel() == 1, \
+ f'invalid rotation angle shape {angle.shape}'
+
+ if angle.numel() == 1:
+ self.tensor[:, 0:3], rot_mat_T = rotation_3d_in_axis(
+ self.tensor[:, 0:3],
+ angle,
+ axis=self.YAW_AXIS,
+ return_mat=True)
+ else:
+ rot_mat_T = angle
+ rot_sin = rot_mat_T[0, 1]
+ rot_cos = rot_mat_T[0, 0]
+ angle = np.arctan2(rot_sin, rot_cos)
+ self.tensor[:, 0:3] = self.tensor[:, 0:3] @ rot_mat_T
+
+ self.tensor[:, 6] += angle
+
+ if self.tensor.shape[1] == 9:
+ # rotate velo vector
+ self.tensor[:, 7:9] = self.tensor[:, 7:9] @ rot_mat_T[:2, :2]
+
+ if points is not None:
+ if isinstance(points, torch.Tensor):
+ points[:, :3] = points[:, :3] @ rot_mat_T
+ elif isinstance(points, np.ndarray):
+ rot_mat_T = rot_mat_T.cpu().numpy()
+ points[:, :3] = np.dot(points[:, :3], rot_mat_T)
+ elif isinstance(points, BasePoints):
+ points.rotate(rot_mat_T)
+ else:
+ raise ValueError
+ return points, rot_mat_T
+
+ def flip(self, bev_direction='horizontal', points=None):
+ """Flip the boxes in BEV along given BEV direction.
+
+ In LIDAR coordinates, it flips the y (horizontal) or x (vertical) axis.
+
+ Args:
+ bev_direction (str): Flip direction (horizontal or vertical).
+ points (torch.Tensor | np.ndarray | :obj:`BasePoints`, optional):
+ Points to flip. Defaults to None.
+
+ Returns:
+ torch.Tensor, numpy.ndarray or None: Flipped points.
+ """
+ assert bev_direction in ('horizontal', 'vertical')
+ if bev_direction == 'horizontal':
+ self.tensor[:, 1::7] = -self.tensor[:, 1::7]
+ if self.with_yaw:
+ self.tensor[:, 6] = -self.tensor[:, 6]
+ elif bev_direction == 'vertical':
+ self.tensor[:, 0::7] = -self.tensor[:, 0::7]
+ if self.with_yaw:
+ self.tensor[:, 6] = -self.tensor[:, 6] + np.pi
+
+ if points is not None:
+ assert isinstance(points, (torch.Tensor, np.ndarray, BasePoints))
+ if isinstance(points, (torch.Tensor, np.ndarray)):
+ if bev_direction == 'horizontal':
+ points[:, 1] = -points[:, 1]
+ elif bev_direction == 'vertical':
+ points[:, 0] = -points[:, 0]
+ elif isinstance(points, BasePoints):
+ points.flip(bev_direction)
+ return points
+
+ def convert_to(self, dst, rt_mat=None):
+ """Convert self to ``dst`` mode.
+
+ Args:
+ dst (:obj:`Box3DMode`): the target Box mode
+ rt_mat (np.ndarray | torch.Tensor, optional): The rotation and
+ translation matrix between different coordinates.
+ Defaults to None.
+ The conversion from ``src`` coordinates to ``dst`` coordinates
+ usually comes along the change of sensors, e.g., from camera
+ to LiDAR. This requires a transformation matrix.
+
+ Returns:
+ :obj:`BaseInstance3DBoxes`:
+ The converted box of the same type in the ``dst`` mode.
+ """
+ from .box_3d_mode import Box3DMode
+ return Box3DMode.convert(
+ box=self, src=Box3DMode.LIDAR, dst=dst, rt_mat=rt_mat)
+
+ def enlarged_box(self, extra_width):
+ """Enlarge the length, width and height boxes.
+
+ Args:
+ extra_width (float | torch.Tensor): Extra width to enlarge the box.
+
+ Returns:
+ :obj:`LiDARInstance3DBoxes`: Enlarged boxes.
+ """
+ enlarged_boxes = self.tensor.clone()
+ enlarged_boxes[:, 3:6] += extra_width * 2
+ # bottom center z minus extra_width
+ enlarged_boxes[:, 2] -= extra_width
+ return self.new_box(enlarged_boxes)
diff --git a/mmdet3d/core/bbox/structures/utils.py b/mmdet3d/core/bbox/structures/utils.py
new file mode 100644
index 0000000..82a4c25
--- /dev/null
+++ b/mmdet3d/core/bbox/structures/utils.py
@@ -0,0 +1,335 @@
+# Copyright (c) OpenMMLab. All rights reserved.
+from logging import warning
+
+import numpy as np
+import torch
+
+from mmdet3d.core.utils import array_converter
+
+
+@array_converter(apply_to=('val', ))
+def limit_period(val, offset=0.5, period=np.pi):
+ """Limit the value into a period for periodic function.
+
+ Args:
+ val (torch.Tensor | np.ndarray): The value to be converted.
+ offset (float, optional): Offset to set the value range.
+ Defaults to 0.5.
+ period ([type], optional): Period of the value. Defaults to np.pi.
+
+ Returns:
+ (torch.Tensor | np.ndarray): Value in the range of
+ [-offset * period, (1-offset) * period]
+ """
+ limited_val = val - torch.floor(val / period + offset) * period
+ return limited_val
+
+
+@array_converter(apply_to=('points', 'angles'))
+def rotation_3d_in_axis(points,
+ angles,
+ axis=0,
+ return_mat=False,
+ clockwise=False):
+ """Rotate points by angles according to axis.
+
+ Args:
+ points (np.ndarray | torch.Tensor | list | tuple ):
+ Points of shape (N, M, 3).
+ angles (np.ndarray | torch.Tensor | list | tuple | float):
+ Vector of angles in shape (N,)
+ axis (int, optional): The axis to be rotated. Defaults to 0.
+ return_mat: Whether or not return the rotation matrix (transposed).
+ Defaults to False.
+ clockwise: Whether the rotation is clockwise. Defaults to False.
+
+ Raises:
+ ValueError: when the axis is not in range [0, 1, 2], it will
+ raise value error.
+
+ Returns:
+ (torch.Tensor | np.ndarray): Rotated points in shape (N, M, 3).
+ """
+ batch_free = len(points.shape) == 2
+ if batch_free:
+ points = points[None]
+
+ if isinstance(angles, float) or len(angles.shape) == 0:
+ angles = torch.full(points.shape[:1], angles)
+
+ assert len(points.shape) == 3 and len(angles.shape) == 1 \
+ and points.shape[0] == angles.shape[0], f'Incorrect shape of points ' \
+ f'angles: {points.shape}, {angles.shape}'
+
+ assert points.shape[-1] in [2, 3], \
+ f'Points size should be 2 or 3 instead of {points.shape[-1]}'
+
+ rot_sin = torch.sin(angles)
+ rot_cos = torch.cos(angles)
+ ones = torch.ones_like(rot_cos)
+ zeros = torch.zeros_like(rot_cos)
+
+ if points.shape[-1] == 3:
+ if axis == 1 or axis == -2:
+ rot_mat_T = torch.stack([
+ torch.stack([rot_cos, zeros, -rot_sin]),
+ torch.stack([zeros, ones, zeros]),
+ torch.stack([rot_sin, zeros, rot_cos])
+ ])
+ elif axis == 2 or axis == -1:
+ rot_mat_T = torch.stack([
+ torch.stack([rot_cos, rot_sin, zeros]),
+ torch.stack([-rot_sin, rot_cos, zeros]),
+ torch.stack([zeros, zeros, ones])
+ ])
+ elif axis == 0 or axis == -3:
+ rot_mat_T = torch.stack([
+ torch.stack([ones, zeros, zeros]),
+ torch.stack([zeros, rot_cos, rot_sin]),
+ torch.stack([zeros, -rot_sin, rot_cos])
+ ])
+ else:
+ raise ValueError(f'axis should in range '
+ f'[-3, -2, -1, 0, 1, 2], got {axis}')
+ else:
+ rot_mat_T = torch.stack([
+ torch.stack([rot_cos, rot_sin]),
+ torch.stack([-rot_sin, rot_cos])
+ ])
+
+ if clockwise:
+ rot_mat_T = rot_mat_T.transpose(0, 1)
+
+ if points.shape[0] == 0:
+ points_new = points
+ else:
+ points_new = torch.einsum('aij,jka->aik', points, rot_mat_T)
+
+ if batch_free:
+ points_new = points_new.squeeze(0)
+
+ if return_mat:
+ rot_mat_T = torch.einsum('jka->ajk', rot_mat_T)
+ if batch_free:
+ rot_mat_T = rot_mat_T.squeeze(0)
+ return points_new, rot_mat_T
+ else:
+ return points_new
+
+
+@array_converter(apply_to=('boxes_xywhr', ))
+def xywhr2xyxyr(boxes_xywhr):
+ """Convert a rotated boxes in XYWHR format to XYXYR format.
+
+ Args:
+ boxes_xywhr (torch.Tensor | np.ndarray): Rotated boxes in XYWHR format.
+
+ Returns:
+ (torch.Tensor | np.ndarray): Converted boxes in XYXYR format.
+ """
+ boxes = torch.zeros_like(boxes_xywhr)
+ half_w = boxes_xywhr[..., 2] / 2
+ half_h = boxes_xywhr[..., 3] / 2
+
+ boxes[..., 0] = boxes_xywhr[..., 0] - half_w
+ boxes[..., 1] = boxes_xywhr[..., 1] - half_h
+ boxes[..., 2] = boxes_xywhr[..., 0] + half_w
+ boxes[..., 3] = boxes_xywhr[..., 1] + half_h
+ boxes[..., 4] = boxes_xywhr[..., 4]
+ return boxes
+
+
+def get_box_type(box_type):
+ """Get the type and mode of box structure.
+
+ Args:
+ box_type (str): The type of box structure.
+ The valid value are "LiDAR", "Camera", or "Depth".
+
+ Raises:
+ ValueError: A ValueError is raised when `box_type`
+ does not belong to the three valid types.
+
+ Returns:
+ tuple: Box type and box mode.
+ """
+ from .box_3d_mode import (Box3DMode, CameraInstance3DBoxes,
+ DepthInstance3DBoxes, LiDARInstance3DBoxes)
+ box_type_lower = box_type.lower()
+ if box_type_lower == 'lidar':
+ box_type_3d = LiDARInstance3DBoxes
+ box_mode_3d = Box3DMode.LIDAR
+ elif box_type_lower == 'camera':
+ box_type_3d = CameraInstance3DBoxes
+ box_mode_3d = Box3DMode.CAM
+ elif box_type_lower == 'depth':
+ box_type_3d = DepthInstance3DBoxes
+ box_mode_3d = Box3DMode.DEPTH
+ else:
+ raise ValueError('Only "box_type" of "camera", "lidar", "depth"'
+ f' are supported, got {box_type}')
+
+ return box_type_3d, box_mode_3d
+
+
+@array_converter(apply_to=('points_3d', 'proj_mat'))
+def points_cam2img(points_3d, proj_mat, with_depth=False):
+ """Project points in camera coordinates to image coordinates.
+
+ Args:
+ points_3d (torch.Tensor | np.ndarray): Points in shape (N, 3)
+ proj_mat (torch.Tensor | np.ndarray):
+ Transformation matrix between coordinates.
+ with_depth (bool, optional): Whether to keep depth in the output.
+ Defaults to False.
+
+ Returns:
+ (torch.Tensor | np.ndarray): Points in image coordinates,
+ with shape [N, 2] if `with_depth=False`, else [N, 3].
+ """
+ points_shape = list(points_3d.shape)
+ points_shape[-1] = 1
+
+ assert len(proj_mat.shape) == 2, 'The dimension of the projection'\
+ f' matrix should be 2 instead of {len(proj_mat.shape)}.'
+ d1, d2 = proj_mat.shape[:2]
+ assert (d1 == 3 and d2 == 3) or (d1 == 3 and d2 == 4) or (
+ d1 == 4 and d2 == 4), 'The shape of the projection matrix'\
+ f' ({d1}*{d2}) is not supported.'
+ if d1 == 3:
+ proj_mat_expanded = torch.eye(
+ 4, device=proj_mat.device, dtype=proj_mat.dtype)
+ proj_mat_expanded[:d1, :d2] = proj_mat
+ proj_mat = proj_mat_expanded
+
+ # previous implementation use new_zeros, new_one yields better results
+ points_4 = torch.cat([points_3d, points_3d.new_ones(points_shape)], dim=-1)
+
+ point_2d = points_4 @ proj_mat.T
+ point_2d_res = point_2d[..., :2] / point_2d[..., 2:3]
+
+ if with_depth:
+ point_2d_res = torch.cat([point_2d_res, point_2d[..., 2:3]], dim=-1)
+
+ return point_2d_res
+
+
+@array_converter(apply_to=('points', 'cam2img'))
+def points_img2cam(points, cam2img):
+ """Project points in image coordinates to camera coordinates.
+
+ Args:
+ points (torch.Tensor): 2.5D points in 2D images, [N, 3],
+ 3 corresponds with x, y in the image and depth.
+ cam2img (torch.Tensor): Camera intrinsic matrix. The shape can be
+ [3, 3], [3, 4] or [4, 4].
+
+ Returns:
+ torch.Tensor: points in 3D space. [N, 3],
+ 3 corresponds with x, y, z in 3D space.
+ """
+ assert cam2img.shape[0] <= 4
+ assert cam2img.shape[1] <= 4
+ assert points.shape[1] == 3
+
+ xys = points[:, :2]
+ depths = points[:, 2].view(-1, 1)
+ unnormed_xys = torch.cat([xys * depths, depths], dim=1)
+
+ pad_cam2img = torch.eye(4, dtype=xys.dtype, device=xys.device)
+ pad_cam2img[:cam2img.shape[0], :cam2img.shape[1]] = cam2img
+ inv_pad_cam2img = torch.inverse(pad_cam2img).transpose(0, 1)
+
+ # Do operation in homogeneous coordinates.
+ num_points = unnormed_xys.shape[0]
+ homo_xys = torch.cat([unnormed_xys, xys.new_ones((num_points, 1))], dim=1)
+ points3D = torch.mm(homo_xys, inv_pad_cam2img)[:, :3]
+
+ return points3D
+
+
+def mono_cam_box2vis(cam_box):
+ """This is a post-processing function on the bboxes from Mono-3D task. If
+ we want to perform projection visualization, we need to:
+
+ 1. rotate the box along x-axis for np.pi / 2 (roll)
+ 2. change orientation from local yaw to global yaw
+ 3. convert yaw by (np.pi / 2 - yaw)
+
+ After applying this function, we can project and draw it on 2D images.
+
+ Args:
+ cam_box (:obj:`CameraInstance3DBoxes`): 3D bbox in camera coordinate
+ system before conversion. Could be gt bbox loaded from dataset
+ or network prediction output.
+
+ Returns:
+ :obj:`CameraInstance3DBoxes`: Box after conversion.
+ """
+ warning.warn('DeprecationWarning: The hack of yaw and dimension in the '
+ 'monocular 3D detection on nuScenes has been removed. The '
+ 'function mono_cam_box2vis will be deprecated.')
+ from . import CameraInstance3DBoxes
+ assert isinstance(cam_box, CameraInstance3DBoxes), \
+ 'input bbox should be CameraInstance3DBoxes!'
+
+ loc = cam_box.gravity_center
+ dim = cam_box.dims
+ yaw = cam_box.yaw
+ feats = cam_box.tensor[:, 7:]
+ # rotate along x-axis for np.pi / 2
+ # see also here: https://github.com/open-mmlab/mmdetection3d/blob/master/mmdet3d/datasets/nuscenes_mono_dataset.py#L557 # noqa
+ dim[:, [1, 2]] = dim[:, [2, 1]]
+ # change local yaw to global yaw for visualization
+ # refer to https://github.com/open-mmlab/mmdetection3d/blob/master/mmdet3d/datasets/nuscenes_mono_dataset.py#L164-L166 # noqa
+ yaw += torch.atan2(loc[:, 0], loc[:, 2])
+ # convert yaw by (-yaw - np.pi / 2)
+ # this is because mono 3D box class such as `NuScenesBox` has different
+ # definition of rotation with our `CameraInstance3DBoxes`
+ yaw = -yaw - np.pi / 2
+ cam_box = torch.cat([loc, dim, yaw[:, None], feats], dim=1)
+ cam_box = CameraInstance3DBoxes(
+ cam_box, box_dim=cam_box.shape[-1], origin=(0.5, 0.5, 0.5))
+
+ return cam_box
+
+
+def get_proj_mat_by_coord_type(img_meta, coord_type):
+ """Obtain image features using points.
+
+ Args:
+ img_meta (dict): Meta info.
+ coord_type (str): 'DEPTH' or 'CAMERA' or 'LIDAR'.
+ Can be case-insensitive.
+
+ Returns:
+ torch.Tensor: transformation matrix.
+ """
+ coord_type = coord_type.upper()
+ mapping = {'LIDAR': 'lidar2img', 'DEPTH': 'depth2img', 'CAMERA': 'cam2img'}
+ assert coord_type in mapping.keys()
+ return img_meta[mapping[coord_type]]
+
+
+def yaw2local(yaw, loc):
+ """Transform global yaw to local yaw (alpha in kitti) in camera
+ coordinates, ranges from -pi to pi.
+
+ Args:
+ yaw (torch.Tensor): A vector with local yaw of each box.
+ shape: (N, )
+ loc (torch.Tensor): gravity center of each box.
+ shape: (N, 3)
+
+ Returns:
+ torch.Tensor: local yaw (alpha in kitti).
+ """
+ local_yaw = yaw - torch.atan2(loc[:, 0], loc[:, 2])
+ larger_idx = (local_yaw > np.pi).nonzero(as_tuple=False)
+ small_idx = (local_yaw < -np.pi).nonzero(as_tuple=False)
+ if len(larger_idx) != 0:
+ local_yaw[larger_idx] -= 2 * np.pi
+ if len(small_idx) != 0:
+ local_yaw[small_idx] += 2 * np.pi
+
+ return local_yaw
diff --git a/mmdet3d/core/bbox/transforms.py b/mmdet3d/core/bbox/transforms.py
new file mode 100644
index 0000000..8a2eb90
--- /dev/null
+++ b/mmdet3d/core/bbox/transforms.py
@@ -0,0 +1,76 @@
+# Copyright (c) OpenMMLab. All rights reserved.
+import torch
+
+
+def bbox3d_mapping_back(bboxes, scale_factor, flip_horizontal, flip_vertical):
+ """Map bboxes from testing scale to original image scale.
+
+ Args:
+ bboxes (:obj:`BaseInstance3DBoxes`): Boxes to be mapped back.
+ scale_factor (float): Scale factor.
+ flip_horizontal (bool): Whether to flip horizontally.
+ flip_vertical (bool): Whether to flip vertically.
+
+ Returns:
+ :obj:`BaseInstance3DBoxes`: Boxes mapped back.
+ """
+ new_bboxes = bboxes.clone()
+ if flip_horizontal:
+ new_bboxes.flip('horizontal')
+ if flip_vertical:
+ new_bboxes.flip('vertical')
+ new_bboxes.scale(1 / scale_factor)
+
+ return new_bboxes
+
+
+def bbox3d2roi(bbox_list):
+ """Convert a list of bounding boxes to roi format.
+
+ Args:
+ bbox_list (list[torch.Tensor]): A list of bounding boxes
+ corresponding to a batch of images.
+
+ Returns:
+ torch.Tensor: Region of interests in shape (n, c), where
+ the channels are in order of [batch_ind, x, y ...].
+ """
+ rois_list = []
+ for img_id, bboxes in enumerate(bbox_list):
+ if bboxes.size(0) > 0:
+ img_inds = bboxes.new_full((bboxes.size(0), 1), img_id)
+ rois = torch.cat([img_inds, bboxes], dim=-1)
+ else:
+ rois = torch.zeros_like(bboxes)
+ rois_list.append(rois)
+ rois = torch.cat(rois_list, 0)
+ return rois
+
+
+def bbox3d2result(bboxes, scores, labels, attrs=None):
+ """Convert detection results to a list of numpy arrays.
+
+ Args:
+ bboxes (torch.Tensor): Bounding boxes with shape (N, 5).
+ labels (torch.Tensor): Labels with shape (N, ).
+ scores (torch.Tensor): Scores with shape (N, ).
+ attrs (torch.Tensor, optional): Attributes with shape (N, ).
+ Defaults to None.
+
+ Returns:
+ dict[str, torch.Tensor]: Bounding box results in cpu mode.
+
+ - boxes_3d (torch.Tensor): 3D boxes.
+ - scores (torch.Tensor): Prediction scores.
+ - labels_3d (torch.Tensor): Box labels.
+ - attrs_3d (torch.Tensor, optional): Box attributes.
+ """
+ result_dict = dict(
+ boxes_3d=bboxes.to('cpu'),
+ scores_3d=scores.cpu(),
+ labels_3d=labels.cpu())
+
+ if attrs is not None:
+ result_dict['attrs_3d'] = attrs.cpu()
+
+ return result_dict
diff --git a/mmdet3d/core/evaluation/__init__.py b/mmdet3d/core/evaluation/__init__.py
new file mode 100644
index 0000000..f20ca3e
--- /dev/null
+++ b/mmdet3d/core/evaluation/__init__.py
@@ -0,0 +1,12 @@
+# Copyright (c) OpenMMLab. All rights reserved.
+from .indoor_eval import indoor_eval
+from .instance_seg_eval import instance_seg_eval
+from .instance_seg_eval_v2 import instance_seg_eval_v2
+from .kitti_utils import kitti_eval, kitti_eval_coco_style
+from .lyft_eval import lyft_eval
+from .seg_eval import seg_eval
+
+__all__ = [
+ 'kitti_eval_coco_style', 'kitti_eval', 'indoor_eval', 'lyft_eval',
+ 'seg_eval', 'instance_seg_eval', 'instance_seg_eval_v2'
+]
diff --git a/mmdet3d/core/evaluation/indoor_eval.py b/mmdet3d/core/evaluation/indoor_eval.py
new file mode 100644
index 0000000..2ff9877
--- /dev/null
+++ b/mmdet3d/core/evaluation/indoor_eval.py
@@ -0,0 +1,309 @@
+# Copyright (c) OpenMMLab. All rights reserved.
+import numpy as np
+import torch
+from mmcv.utils import print_log
+from terminaltables import AsciiTable
+
+
+def average_precision(recalls, precisions, mode='area'):
+ """Calculate average precision (for single or multiple scales).
+
+ Args:
+ recalls (np.ndarray): Recalls with shape of (num_scales, num_dets)
+ or (num_dets, ).
+ precisions (np.ndarray): Precisions with shape of
+ (num_scales, num_dets) or (num_dets, ).
+ mode (str): 'area' or '11points', 'area' means calculating the area
+ under precision-recall curve, '11points' means calculating
+ the average precision of recalls at [0, 0.1, ..., 1]
+
+ Returns:
+ float or np.ndarray: Calculated average precision.
+ """
+ if recalls.ndim == 1:
+ recalls = recalls[np.newaxis, :]
+ precisions = precisions[np.newaxis, :]
+
+ assert recalls.shape == precisions.shape
+ assert recalls.ndim == 2
+
+ num_scales = recalls.shape[0]
+ ap = np.zeros(num_scales, dtype=np.float32)
+ if mode == 'area':
+ zeros = np.zeros((num_scales, 1), dtype=recalls.dtype)
+ ones = np.ones((num_scales, 1), dtype=recalls.dtype)
+ mrec = np.hstack((zeros, recalls, ones))
+ mpre = np.hstack((zeros, precisions, zeros))
+ for i in range(mpre.shape[1] - 1, 0, -1):
+ mpre[:, i - 1] = np.maximum(mpre[:, i - 1], mpre[:, i])
+ for i in range(num_scales):
+ ind = np.where(mrec[i, 1:] != mrec[i, :-1])[0]
+ ap[i] = np.sum(
+ (mrec[i, ind + 1] - mrec[i, ind]) * mpre[i, ind + 1])
+ elif mode == '11points':
+ for i in range(num_scales):
+ for thr in np.arange(0, 1 + 1e-3, 0.1):
+ precs = precisions[i, recalls[i, :] >= thr]
+ prec = precs.max() if precs.size > 0 else 0
+ ap[i] += prec
+ ap /= 11
+ else:
+ raise ValueError(
+ 'Unrecognized mode, only "area" and "11points" are supported')
+ return ap
+
+
+def eval_det_cls(pred, gt, iou_thr=None):
+ """Generic functions to compute precision/recall for object detection for a
+ single class.
+
+ Args:
+ pred (dict): Predictions mapping from image id to bounding boxes
+ and scores.
+ gt (dict): Ground truths mapping from image id to bounding boxes.
+ iou_thr (list[float]): A list of iou thresholds.
+
+ Return:
+ tuple (np.ndarray, np.ndarray, float): Recalls, precisions and
+ average precision.
+ """
+
+ # {img_id: {'bbox': box structure, 'det': matched list}}
+ class_recs = {}
+ npos = 0
+ for img_id in gt.keys():
+ cur_gt_num = len(gt[img_id])
+ if cur_gt_num != 0:
+ gt_cur = torch.zeros([cur_gt_num, 7], dtype=torch.float32)
+ for i in range(cur_gt_num):
+ gt_cur[i] = gt[img_id][i].tensor
+ bbox = gt[img_id][0].new_box(gt_cur)
+ else:
+ bbox = gt[img_id]
+ det = [[False] * len(bbox) for i in iou_thr]
+ npos += len(bbox)
+ class_recs[img_id] = {'bbox': bbox, 'det': det}
+
+ # construct dets
+ image_ids = []
+ confidence = []
+ ious = []
+ for img_id in pred.keys():
+ cur_num = len(pred[img_id])
+ if cur_num == 0:
+ continue
+ pred_cur = torch.zeros((cur_num, 7), dtype=torch.float32)
+ box_idx = 0
+ for box, score in pred[img_id]:
+ image_ids.append(img_id)
+ confidence.append(score)
+ pred_cur[box_idx] = box.tensor
+ box_idx += 1
+ pred_cur = box.new_box(pred_cur)
+ gt_cur = class_recs[img_id]['bbox']
+ if len(gt_cur) > 0:
+ # calculate iou in each image
+ iou_cur = pred_cur.overlaps(pred_cur, gt_cur)
+ for i in range(cur_num):
+ ious.append(iou_cur[i])
+ else:
+ for i in range(cur_num):
+ ious.append(np.zeros(1))
+
+ confidence = np.array(confidence)
+
+ # sort by confidence
+ sorted_ind = np.argsort(-confidence)
+ image_ids = [image_ids[x] for x in sorted_ind]
+ ious = [ious[x] for x in sorted_ind]
+
+ # go down dets and mark TPs and FPs
+ nd = len(image_ids)
+ tp_thr = [np.zeros(nd) for i in iou_thr]
+ fp_thr = [np.zeros(nd) for i in iou_thr]
+ for d in range(nd):
+ R = class_recs[image_ids[d]]
+ iou_max = -np.inf
+ BBGT = R['bbox']
+ cur_iou = ious[d]
+
+ if len(BBGT) > 0:
+ # compute overlaps
+ for j in range(len(BBGT)):
+ # iou = get_iou_main(get_iou_func, (bb, BBGT[j,...]))
+ iou = cur_iou[j]
+ if iou > iou_max:
+ iou_max = iou
+ jmax = j
+
+ for iou_idx, thresh in enumerate(iou_thr):
+ if iou_max > thresh:
+ if not R['det'][iou_idx][jmax]:
+ tp_thr[iou_idx][d] = 1.
+ R['det'][iou_idx][jmax] = 1
+ else:
+ fp_thr[iou_idx][d] = 1.
+ else:
+ fp_thr[iou_idx][d] = 1.
+
+ ret = []
+ for iou_idx, thresh in enumerate(iou_thr):
+ # compute precision recall
+ fp = np.cumsum(fp_thr[iou_idx])
+ tp = np.cumsum(tp_thr[iou_idx])
+ recall = tp / float(npos)
+ # avoid divide by zero in case the first detection matches a difficult
+ # ground truth
+ precision = tp / np.maximum(tp + fp, np.finfo(np.float64).eps)
+ ap = average_precision(recall, precision)
+ ret.append((recall, precision, ap))
+
+ return ret
+
+
+def eval_map_recall(pred, gt, ovthresh=None):
+ """Evaluate mAP and recall.
+
+ Generic functions to compute precision/recall for object detection
+ for multiple classes.
+
+ Args:
+ pred (dict): Information of detection results,
+ which maps class_id and predictions.
+ gt (dict): Information of ground truths, which maps class_id and
+ ground truths.
+ ovthresh (list[float], optional): iou threshold. Default: None.
+
+ Return:
+ tuple[dict]: dict results of recall, AP, and precision for all classes.
+ """
+
+ ret_values = {}
+ for classname in gt.keys():
+ if classname in pred:
+ ret_values[classname] = eval_det_cls(pred[classname],
+ gt[classname], ovthresh)
+ recall = [{} for i in ovthresh]
+ precision = [{} for i in ovthresh]
+ ap = [{} for i in ovthresh]
+
+ for label in gt.keys():
+ for iou_idx, thresh in enumerate(ovthresh):
+ if label in pred:
+ recall[iou_idx][label], precision[iou_idx][label], ap[iou_idx][
+ label] = ret_values[label][iou_idx]
+ else:
+ recall[iou_idx][label] = np.zeros(1)
+ precision[iou_idx][label] = np.zeros(1)
+ ap[iou_idx][label] = np.zeros(1)
+
+ return recall, precision, ap
+
+
+def indoor_eval(gt_annos,
+ dt_annos,
+ metric,
+ label2cat,
+ logger=None,
+ box_type_3d=None,
+ box_mode_3d=None):
+ """Indoor Evaluation.
+
+ Evaluate the result of the detection.
+
+ Args:
+ gt_annos (list[dict]): Ground truth annotations.
+ dt_annos (list[dict]): Detection annotations. the dict
+ includes the following keys
+
+ - labels_3d (torch.Tensor): Labels of boxes.
+ - boxes_3d (:obj:`BaseInstance3DBoxes`):
+ 3D bounding boxes in Depth coordinate.
+ - scores_3d (torch.Tensor): Scores of boxes.
+ metric (list[float]): IoU thresholds for computing average precisions.
+ label2cat (dict): Map from label to category.
+ logger (logging.Logger | str, optional): The way to print the mAP
+ summary. See `mmdet.utils.print_log()` for details. Default: None.
+
+ Return:
+ dict[str, float]: Dict of results.
+ """
+ assert len(dt_annos) == len(gt_annos)
+ pred = {} # map {class_id: pred}
+ gt = {} # map {class_id: gt}
+ for img_id in range(len(dt_annos)):
+ # parse detected annotations
+ det_anno = dt_annos[img_id]
+ for i in range(len(det_anno['labels_3d'])):
+ label = det_anno['labels_3d'].numpy()[i]
+ bbox = det_anno['boxes_3d'].convert_to(box_mode_3d)[i]
+ score = det_anno['scores_3d'].numpy()[i]
+ if label not in pred:
+ pred[int(label)] = {}
+ if img_id not in pred[label]:
+ pred[int(label)][img_id] = []
+ if label not in gt:
+ gt[int(label)] = {}
+ if img_id not in gt[label]:
+ gt[int(label)][img_id] = []
+ pred[int(label)][img_id].append((bbox, score))
+
+ # parse gt annotations
+ gt_anno = gt_annos[img_id]
+ if gt_anno['gt_num'] != 0:
+ gt_boxes = box_type_3d(
+ gt_anno['gt_boxes_upright_depth'],
+ box_dim=gt_anno['gt_boxes_upright_depth'].shape[-1],
+ origin=(0.5, 0.5, 0.5)).convert_to(box_mode_3d)
+ labels_3d = gt_anno['class']
+ else:
+ gt_boxes = box_type_3d(np.array([], dtype=np.float32))
+ labels_3d = np.array([], dtype=np.int64)
+
+ for i in range(len(labels_3d)):
+ label = labels_3d[i]
+ bbox = gt_boxes[i]
+ if label not in gt:
+ gt[label] = {}
+ if img_id not in gt[label]:
+ gt[label][img_id] = []
+ gt[label][img_id].append(bbox)
+
+ rec, prec, ap = eval_map_recall(pred, gt, metric)
+ ret_dict = dict()
+ header = ['classes']
+ table_columns = [[label2cat[label]
+ for label in ap[0].keys()] + ['Overall']]
+
+ for i, iou_thresh in enumerate(metric):
+ header.append(f'AP_{iou_thresh:.2f}')
+ header.append(f'AR_{iou_thresh:.2f}')
+ rec_list = []
+ for label in ap[i].keys():
+ ret_dict[f'{label2cat[label]}_AP_{iou_thresh:.2f}'] = float(
+ ap[i][label][0])
+ ret_dict[f'mAP_{iou_thresh:.2f}'] = float(
+ np.mean(list(ap[i].values())))
+
+ table_columns.append(list(map(float, list(ap[i].values()))))
+ table_columns[-1] += [ret_dict[f'mAP_{iou_thresh:.2f}']]
+ table_columns[-1] = [f'{x:.4f}' for x in table_columns[-1]]
+
+ for label in rec[i].keys():
+ ret_dict[f'{label2cat[label]}_rec_{iou_thresh:.2f}'] = float(
+ rec[i][label][-1])
+ rec_list.append(rec[i][label][-1])
+ ret_dict[f'mAR_{iou_thresh:.2f}'] = float(np.mean(rec_list))
+
+ table_columns.append(list(map(float, rec_list)))
+ table_columns[-1] += [ret_dict[f'mAR_{iou_thresh:.2f}']]
+ table_columns[-1] = [f'{x:.4f}' for x in table_columns[-1]]
+
+ table_data = [header]
+ table_rows = list(zip(*table_columns))
+ table_data += table_rows
+ table = AsciiTable(table_data)
+ table.inner_footing_row_border = True
+ print_log('\n' + table.table, logger=logger)
+
+ return ret_dict
diff --git a/mmdet3d/core/evaluation/instance_seg_eval.py b/mmdet3d/core/evaluation/instance_seg_eval.py
new file mode 100644
index 0000000..31f5110
--- /dev/null
+++ b/mmdet3d/core/evaluation/instance_seg_eval.py
@@ -0,0 +1,128 @@
+# Copyright (c) OpenMMLab. All rights reserved.
+import numpy as np
+from mmcv.utils import print_log
+from terminaltables import AsciiTable
+
+from .scannet_utils.evaluate_semantic_instance import scannet_eval
+
+
+def aggregate_predictions(masks, labels, scores, valid_class_ids):
+ """Maps predictions to ScanNet evaluator format.
+
+ Args:
+ masks (list[torch.Tensor]): Per scene predicted instance masks.
+ labels (list[torch.Tensor]): Per scene predicted instance labels.
+ scores (list[torch.Tensor]): Per scene predicted instance scores.
+ valid_class_ids (tuple[int]): Ids of valid categories.
+
+ Returns:
+ list[dict]: Per scene aggregated predictions.
+ """
+ infos = []
+ for id, (mask, label, score) in enumerate(zip(masks, labels, scores)):
+ mask = mask.clone().numpy()
+ label = label.clone().numpy()
+ score = score.clone().numpy()
+ info = dict()
+ n_instances = mask.max() + 1
+ for i in range(n_instances):
+ # match pred_instance['filename'] from assign_instances_for_scan
+ file_name = f'{id}_{i}'
+ info[file_name] = dict()
+ info[file_name]['mask'] = (mask == i).astype(np.int)
+ info[file_name]['label_id'] = valid_class_ids[label[i]]
+ info[file_name]['conf'] = score[i]
+ infos.append(info)
+ return infos
+
+
+def rename_gt(gt_semantic_masks, gt_instance_masks, valid_class_ids):
+ """Maps gt instance and semantic masks to instance masks for ScanNet
+ evaluator.
+
+ Args:
+ gt_semantic_masks (list[torch.Tensor]): Per scene gt semantic masks.
+ gt_instance_masks (list[torch.Tensor]): Per scene gt instance masks.
+ valid_class_ids (tuple[int]): Ids of valid categories.
+
+ Returns:
+ list[np.array]: Per scene instance masks.
+ """
+ renamed_instance_masks = []
+ for semantic_mask, instance_mask in zip(gt_semantic_masks,
+ gt_instance_masks):
+ semantic_mask = semantic_mask.clone().numpy()
+ instance_mask = instance_mask.clone().numpy()
+ unique = np.unique(instance_mask)
+ assert len(unique) < 1000
+ for i in unique:
+ semantic_instance = semantic_mask[instance_mask == i]
+ semantic_unique = np.unique(semantic_instance)
+ assert len(semantic_unique) == 1
+ if semantic_unique[0] < len(valid_class_ids):
+ instance_mask[
+ instance_mask ==
+ i] = 1000 * valid_class_ids[semantic_unique[0]] + i
+ renamed_instance_masks.append(instance_mask)
+ return renamed_instance_masks
+
+
+def instance_seg_eval(gt_semantic_masks,
+ gt_instance_masks,
+ pred_instance_masks,
+ pred_instance_labels,
+ pred_instance_scores,
+ valid_class_ids,
+ class_labels,
+ options=None,
+ logger=None):
+ """Instance Segmentation Evaluation.
+
+ Evaluate the result of the instance segmentation.
+
+ Args:
+ gt_semantic_masks (list[torch.Tensor]): Ground truth semantic masks.
+ gt_instance_masks (list[torch.Tensor]): Ground truth instance masks.
+ pred_instance_masks (list[torch.Tensor]): Predicted instance masks.
+ pred_instance_labels (list[torch.Tensor]): Predicted instance labels.
+ pred_instance_scores (list[torch.Tensor]): Predicted instance labels.
+ valid_class_ids (tuple[int]): Ids of valid categories.
+ class_labels (tuple[str]): Names of valid categories.
+ options (dict, optional): Additional options. Keys may contain:
+ `overlaps`, `min_region_sizes`, `distance_threshes`,
+ `distance_confs`. Default: None.
+ logger (logging.Logger | str, optional): The way to print the mAP
+ summary. See `mmdet.utils.print_log()` for details. Default: None.
+
+ Returns:
+ dict[str, float]: Dict of results.
+ """
+ assert len(valid_class_ids) == len(class_labels)
+ id_to_label = {
+ valid_class_ids[i]: class_labels[i]
+ for i in range(len(valid_class_ids))
+ }
+ preds = aggregate_predictions(
+ masks=pred_instance_masks,
+ labels=pred_instance_labels,
+ scores=pred_instance_scores,
+ valid_class_ids=valid_class_ids)
+ gts = rename_gt(gt_semantic_masks, gt_instance_masks, valid_class_ids)
+ metrics = scannet_eval(
+ preds=preds,
+ gts=gts,
+ options=options,
+ valid_class_ids=valid_class_ids,
+ class_labels=class_labels,
+ id_to_label=id_to_label)
+ header = ['classes', 'AP_0.25', 'AP_0.50', 'AP']
+ rows = []
+ for label, data in metrics['classes'].items():
+ aps = [data['ap25%'], data['ap50%'], data['ap']]
+ rows.append([label] + [f'{ap:.4f}' for ap in aps])
+ aps = metrics['all_ap_25%'], metrics['all_ap_50%'], metrics['all_ap']
+ footer = ['Overall'] + [f'{ap:.4f}' for ap in aps]
+ table = AsciiTable([header] + rows + [footer])
+ table.inner_footing_row_border = True
+ print_log('\n' + table.table, logger=logger)
+ return metrics
diff --git a/mmdet3d/core/evaluation/instance_seg_eval_v2.py b/mmdet3d/core/evaluation/instance_seg_eval_v2.py
new file mode 100644
index 0000000..420d904
--- /dev/null
+++ b/mmdet3d/core/evaluation/instance_seg_eval_v2.py
@@ -0,0 +1,128 @@
+# Copyright (c) OpenMMLab. All rights reserved.
+import numpy as np
+from mmcv.utils import print_log
+from terminaltables import AsciiTable
+
+from .scannet_utils.evaluate_semantic_instance import scannet_eval
+
+
+def aggregate_predictions(masks, labels, scores, valid_class_ids):
+ """Maps predictions to ScanNet evaluator format.
+
+ Args:
+ masks (list[torch.Tensor]): Per scene predicted instance masks.
+ Recommented dtype is torch.bool.
+ labels (list[torch.Tensor]): Per scene predicted instance labels.
+ scores (list[torch.Tensor]): Per scene predicted instance scores.
+ valid_class_ids (tuple[int]): Ids of valid categories.
+
+ Returns:
+ list[dict]: Per scene aggregated predictions.
+ """
+ infos = []
+ for id, (mask, label, score) in enumerate(zip(masks, labels, scores)):
+ mask = mask.numpy()
+ label = label.numpy()
+ score = score.numpy()
+ info = dict()
+ for i in range(mask.shape[0]):
+ # match pred_instance['filename'] from assign_instances_for_scan
+ file_name = f'{id}_{i}'
+ info[file_name] = dict()
+ info[file_name]['mask'] = mask[i]
+ info[file_name]['label_id'] = valid_class_ids[label[i]]
+ info[file_name]['conf'] = score[i]
+ infos.append(info)
+ return infos
+
+
+def rename_gt(gt_semantic_masks, gt_instance_masks, valid_class_ids):
+ """Maps gt instance and semantic masks to instance masks for ScanNet
+ evaluator.
+
+ Args:
+ gt_semantic_masks (list[torch.Tensor]): Per scene gt semantic masks.
+ gt_instance_masks (list[torch.Tensor]): Per scene gt instance masks.
+ valid_class_ids (tuple[int]): Ids of valid categories.
+
+ Returns:
+ list[np.array]: Per scene instance masks.
+ """
+ renamed_instance_masks = []
+ for semantic_mask, instance_mask in zip(gt_semantic_masks,
+ gt_instance_masks):
+ semantic_mask = semantic_mask.numpy()
+ instance_mask = instance_mask.numpy()
+ unique = np.unique(instance_mask)
+ assert len(unique) < 1000
+ for i in unique:
+ semantic_instance = semantic_mask[instance_mask == i]
+ semantic_unique = np.unique(semantic_instance)
+ assert len(semantic_unique) == 1
+ if semantic_unique[0] in valid_class_ids:
+ instance_mask[
+ instance_mask ==
+ i] = 1000 * semantic_unique[0] + i
+ renamed_instance_masks.append(instance_mask)
+ return renamed_instance_masks
+
+
+def instance_seg_eval_v2(gt_semantic_masks,
+ gt_instance_masks,
+ pred_instance_masks,
+ pred_instance_labels,
+ pred_instance_scores,
+ valid_class_ids,
+ class_labels,
+ options=None,
+ logger=None):
+ """Instance Segmentation Evaluation.
+
+ Evaluate the result of the instance segmentation.
+
+ Args:
+ gt_semantic_masks (list[torch.Tensor]): Ground truth semantic masks.
+ gt_instance_masks (list[torch.Tensor]): Ground truth instance masks.
+ pred_instance_masks (list[torch.Tensor]): Predicted instance masks.
+ pred_instance_labels (list[torch.Tensor]): Predicted instance labels.
+ pred_instance_scores (list[torch.Tensor]): Predicted instance labels.
+ valid_class_ids (tuple[int]): Ids of valid categories.
+ class_labels (tuple[str]): Names of valid categories.
+ options (dict, optional): Additional options. Keys may contain:
+ `overlaps`, `min_region_sizes`, `distance_threshes`,
+ `distance_confs`. Default: None.
+ logger (logging.Logger | str, optional): The way to print the mAP
+ summary. See `mmdet.utils.print_log()` for details. Default: None.
+
+ Returns:
+ dict[str, float]: Dict of results.
+ """
+ assert len(valid_class_ids) == len(class_labels)
+ id_to_label = {
+ valid_class_ids[i]: class_labels[i]
+ for i in range(len(valid_class_ids))
+ }
+ preds = aggregate_predictions(
+ masks=pred_instance_masks,
+ labels=pred_instance_labels,
+ scores=pred_instance_scores,
+ valid_class_ids=valid_class_ids)
+ gts = rename_gt(gt_semantic_masks, gt_instance_masks, valid_class_ids)
+ metrics = scannet_eval(
+ preds=preds,
+ gts=gts,
+ options=options,
+ valid_class_ids=valid_class_ids,
+ class_labels=class_labels,
+ id_to_label=id_to_label)
+ header = ['classes', 'AP_0.25', 'AP_0.50', 'AP']
+ rows = []
+ for label, data in metrics['classes'].items():
+ aps = [data['ap25%'], data['ap50%'], data['ap']]
+ rows.append([label] + [f'{ap:.4f}' for ap in aps])
+ aps = metrics['all_ap_25%'], metrics['all_ap_50%'], metrics['all_ap']
+ footer = ['Overall'] + [f'{ap:.4f}' for ap in aps]
+ table = AsciiTable([header] + rows + [footer])
+ table.inner_footing_row_border = True
+ print_log('\n' + table.table, logger=logger)
+ return metrics
diff --git a/mmdet3d/core/evaluation/kitti_utils/__init__.py b/mmdet3d/core/evaluation/kitti_utils/__init__.py
new file mode 100644
index 0000000..23c1cdf
--- /dev/null
+++ b/mmdet3d/core/evaluation/kitti_utils/__init__.py
@@ -0,0 +1,4 @@
+# Copyright (c) OpenMMLab. All rights reserved.
+from .eval import kitti_eval, kitti_eval_coco_style
+
+__all__ = ['kitti_eval', 'kitti_eval_coco_style']
diff --git a/mmdet3d/core/evaluation/kitti_utils/eval.py b/mmdet3d/core/evaluation/kitti_utils/eval.py
new file mode 100644
index 0000000..f8408df
--- /dev/null
+++ b/mmdet3d/core/evaluation/kitti_utils/eval.py
@@ -0,0 +1,950 @@
+# Copyright (c) OpenMMLab. All rights reserved.
+import gc
+import io as sysio
+
+import numba
+import numpy as np
+
+
+@numba.jit
+def get_thresholds(scores: np.ndarray, num_gt, num_sample_pts=41):
+ scores.sort()
+ scores = scores[::-1]
+ current_recall = 0
+ thresholds = []
+ for i, score in enumerate(scores):
+ l_recall = (i + 1) / num_gt
+ if i < (len(scores) - 1):
+ r_recall = (i + 2) / num_gt
+ else:
+ r_recall = l_recall
+ if (((r_recall - current_recall) < (current_recall - l_recall))
+ and (i < (len(scores) - 1))):
+ continue
+ # recall = l_recall
+ thresholds.append(score)
+ current_recall += 1 / (num_sample_pts - 1.0)
+ return thresholds
+
+
+def clean_data(gt_anno, dt_anno, current_class, difficulty):
+ CLASS_NAMES = ['car', 'pedestrian', 'cyclist']
+ MIN_HEIGHT = [40, 25, 25]
+ MAX_OCCLUSION = [0, 1, 2]
+ MAX_TRUNCATION = [0.15, 0.3, 0.5]
+ dc_bboxes, ignored_gt, ignored_dt = [], [], []
+ current_cls_name = CLASS_NAMES[current_class].lower()
+ num_gt = len(gt_anno['name'])
+ num_dt = len(dt_anno['name'])
+ num_valid_gt = 0
+ for i in range(num_gt):
+ bbox = gt_anno['bbox'][i]
+ gt_name = gt_anno['name'][i].lower()
+ height = bbox[3] - bbox[1]
+ valid_class = -1
+ if (gt_name == current_cls_name):
+ valid_class = 1
+ elif (current_cls_name == 'Pedestrian'.lower()
+ and 'Person_sitting'.lower() == gt_name):
+ valid_class = 0
+ elif (current_cls_name == 'Car'.lower() and 'Van'.lower() == gt_name):
+ valid_class = 0
+ else:
+ valid_class = -1
+ ignore = False
+ if ((gt_anno['occluded'][i] > MAX_OCCLUSION[difficulty])
+ or (gt_anno['truncated'][i] > MAX_TRUNCATION[difficulty])
+ or (height <= MIN_HEIGHT[difficulty])):
+ ignore = True
+ if valid_class == 1 and not ignore:
+ ignored_gt.append(0)
+ num_valid_gt += 1
+ elif (valid_class == 0 or (ignore and (valid_class == 1))):
+ ignored_gt.append(1)
+ else:
+ ignored_gt.append(-1)
+ # for i in range(num_gt):
+ if gt_anno['name'][i] == 'DontCare':
+ dc_bboxes.append(gt_anno['bbox'][i])
+ for i in range(num_dt):
+ if (dt_anno['name'][i].lower() == current_cls_name):
+ valid_class = 1
+ else:
+ valid_class = -1
+ height = abs(dt_anno['bbox'][i, 3] - dt_anno['bbox'][i, 1])
+ if height < MIN_HEIGHT[difficulty]:
+ ignored_dt.append(1)
+ elif valid_class == 1:
+ ignored_dt.append(0)
+ else:
+ ignored_dt.append(-1)
+
+ return num_valid_gt, ignored_gt, ignored_dt, dc_bboxes
+
+
+@numba.jit(nopython=True)
+def image_box_overlap(boxes, query_boxes, criterion=-1):
+ N = boxes.shape[0]
+ K = query_boxes.shape[0]
+ overlaps = np.zeros((N, K), dtype=boxes.dtype)
+ for k in range(K):
+ qbox_area = ((query_boxes[k, 2] - query_boxes[k, 0]) *
+ (query_boxes[k, 3] - query_boxes[k, 1]))
+ for n in range(N):
+ iw = (
+ min(boxes[n, 2], query_boxes[k, 2]) -
+ max(boxes[n, 0], query_boxes[k, 0]))
+ if iw > 0:
+ ih = (
+ min(boxes[n, 3], query_boxes[k, 3]) -
+ max(boxes[n, 1], query_boxes[k, 1]))
+ if ih > 0:
+ if criterion == -1:
+ ua = ((boxes[n, 2] - boxes[n, 0]) *
+ (boxes[n, 3] - boxes[n, 1]) + qbox_area -
+ iw * ih)
+ elif criterion == 0:
+ ua = ((boxes[n, 2] - boxes[n, 0]) *
+ (boxes[n, 3] - boxes[n, 1]))
+ elif criterion == 1:
+ ua = qbox_area
+ else:
+ ua = 1.0
+ overlaps[n, k] = iw * ih / ua
+ return overlaps
+
+
+def bev_box_overlap(boxes, qboxes, criterion=-1):
+ from .rotate_iou import rotate_iou_gpu_eval
+ riou = rotate_iou_gpu_eval(boxes, qboxes, criterion)
+ return riou
+
+
+@numba.jit(nopython=True, parallel=True)
+def d3_box_overlap_kernel(boxes, qboxes, rinc, criterion=-1):
+ # ONLY support overlap in CAMERA, not lidar.
+ # TODO: change to use prange for parallel mode, should check the difference
+ N, K = boxes.shape[0], qboxes.shape[0]
+ for i in numba.prange(N):
+ for j in numba.prange(K):
+ if rinc[i, j] > 0:
+ # iw = (min(boxes[i, 1] + boxes[i, 4], qboxes[j, 1] +
+ # qboxes[j, 4]) - max(boxes[i, 1], qboxes[j, 1]))
+ iw = (
+ min(boxes[i, 1], qboxes[j, 1]) -
+ max(boxes[i, 1] - boxes[i, 4],
+ qboxes[j, 1] - qboxes[j, 4]))
+
+ if iw > 0:
+ area1 = boxes[i, 3] * boxes[i, 4] * boxes[i, 5]
+ area2 = qboxes[j, 3] * qboxes[j, 4] * qboxes[j, 5]
+ inc = iw * rinc[i, j]
+ if criterion == -1:
+ ua = (area1 + area2 - inc)
+ elif criterion == 0:
+ ua = area1
+ elif criterion == 1:
+ ua = area2
+ else:
+ ua = inc
+ rinc[i, j] = inc / ua
+ else:
+ rinc[i, j] = 0.0
+
+
+def d3_box_overlap(boxes, qboxes, criterion=-1):
+ from .rotate_iou import rotate_iou_gpu_eval
+ rinc = rotate_iou_gpu_eval(boxes[:, [0, 2, 3, 5, 6]],
+ qboxes[:, [0, 2, 3, 5, 6]], 2)
+ d3_box_overlap_kernel(boxes, qboxes, rinc, criterion)
+ return rinc
+
+
+@numba.jit(nopython=True)
+def compute_statistics_jit(overlaps,
+ gt_datas,
+ dt_datas,
+ ignored_gt,
+ ignored_det,
+ dc_bboxes,
+ metric,
+ min_overlap,
+ thresh=0,
+ compute_fp=False,
+ compute_aos=False):
+
+ det_size = dt_datas.shape[0]
+ gt_size = gt_datas.shape[0]
+ dt_scores = dt_datas[:, -1]
+ dt_alphas = dt_datas[:, 4]
+ gt_alphas = gt_datas[:, 4]
+ dt_bboxes = dt_datas[:, :4]
+ # gt_bboxes = gt_datas[:, :4]
+
+ assigned_detection = [False] * det_size
+ ignored_threshold = [False] * det_size
+ if compute_fp:
+ for i in range(det_size):
+ if (dt_scores[i] < thresh):
+ ignored_threshold[i] = True
+ NO_DETECTION = -10000000
+ tp, fp, fn, similarity = 0, 0, 0, 0
+ # thresholds = [0.0]
+ # delta = [0.0]
+ thresholds = np.zeros((gt_size, ))
+ thresh_idx = 0
+ delta = np.zeros((gt_size, ))
+ delta_idx = 0
+ for i in range(gt_size):
+ if ignored_gt[i] == -1:
+ continue
+ det_idx = -1
+ valid_detection = NO_DETECTION
+ max_overlap = 0
+ assigned_ignored_det = False
+
+ for j in range(det_size):
+ if (ignored_det[j] == -1):
+ continue
+ if (assigned_detection[j]):
+ continue
+ if (ignored_threshold[j]):
+ continue
+ overlap = overlaps[j, i]
+ dt_score = dt_scores[j]
+ if (not compute_fp and (overlap > min_overlap)
+ and dt_score > valid_detection):
+ det_idx = j
+ valid_detection = dt_score
+ elif (compute_fp and (overlap > min_overlap)
+ and (overlap > max_overlap or assigned_ignored_det)
+ and ignored_det[j] == 0):
+ max_overlap = overlap
+ det_idx = j
+ valid_detection = 1
+ assigned_ignored_det = False
+ elif (compute_fp and (overlap > min_overlap)
+ and (valid_detection == NO_DETECTION)
+ and ignored_det[j] == 1):
+ det_idx = j
+ valid_detection = 1
+ assigned_ignored_det = True
+
+ if (valid_detection == NO_DETECTION) and ignored_gt[i] == 0:
+ fn += 1
+ elif ((valid_detection != NO_DETECTION)
+ and (ignored_gt[i] == 1 or ignored_det[det_idx] == 1)):
+ assigned_detection[det_idx] = True
+ elif valid_detection != NO_DETECTION:
+ tp += 1
+ # thresholds.append(dt_scores[det_idx])
+ thresholds[thresh_idx] = dt_scores[det_idx]
+ thresh_idx += 1
+ if compute_aos:
+ # delta.append(gt_alphas[i] - dt_alphas[det_idx])
+ delta[delta_idx] = gt_alphas[i] - dt_alphas[det_idx]
+ delta_idx += 1
+
+ assigned_detection[det_idx] = True
+ if compute_fp:
+ for i in range(det_size):
+ if (not (assigned_detection[i] or ignored_det[i] == -1
+ or ignored_det[i] == 1 or ignored_threshold[i])):
+ fp += 1
+ nstuff = 0
+ if metric == 0:
+ overlaps_dt_dc = image_box_overlap(dt_bboxes, dc_bboxes, 0)
+ for i in range(dc_bboxes.shape[0]):
+ for j in range(det_size):
+ if (assigned_detection[j]):
+ continue
+ if (ignored_det[j] == -1 or ignored_det[j] == 1):
+ continue
+ if (ignored_threshold[j]):
+ continue
+ if overlaps_dt_dc[j, i] > min_overlap:
+ assigned_detection[j] = True
+ nstuff += 1
+ fp -= nstuff
+ if compute_aos:
+ tmp = np.zeros((fp + delta_idx, ))
+ # tmp = [0] * fp
+ for i in range(delta_idx):
+ tmp[i + fp] = (1.0 + np.cos(delta[i])) / 2.0
+ # tmp.append((1.0 + np.cos(delta[i])) / 2.0)
+ # assert len(tmp) == fp + tp
+ # assert len(delta) == tp
+ if tp > 0 or fp > 0:
+ similarity = np.sum(tmp)
+ else:
+ similarity = -1
+ return tp, fp, fn, similarity, thresholds[:thresh_idx]
+
+
+def get_split_parts(num, num_part):
+ same_part = num // num_part
+ remain_num = num % num_part
+ if remain_num == 0:
+ return [same_part] * num_part
+ else:
+ return [same_part] * num_part + [remain_num]
+
+
+@numba.jit(nopython=True)
+def fused_compute_statistics(overlaps,
+ pr,
+ gt_nums,
+ dt_nums,
+ dc_nums,
+ gt_datas,
+ dt_datas,
+ dontcares,
+ ignored_gts,
+ ignored_dets,
+ metric,
+ min_overlap,
+ thresholds,
+ compute_aos=False):
+ gt_num = 0
+ dt_num = 0
+ dc_num = 0
+ for i in range(gt_nums.shape[0]):
+ for t, thresh in enumerate(thresholds):
+ overlap = overlaps[dt_num:dt_num + dt_nums[i],
+ gt_num:gt_num + gt_nums[i]]
+
+ gt_data = gt_datas[gt_num:gt_num + gt_nums[i]]
+ dt_data = dt_datas[dt_num:dt_num + dt_nums[i]]
+ ignored_gt = ignored_gts[gt_num:gt_num + gt_nums[i]]
+ ignored_det = ignored_dets[dt_num:dt_num + dt_nums[i]]
+ dontcare = dontcares[dc_num:dc_num + dc_nums[i]]
+ tp, fp, fn, similarity, _ = compute_statistics_jit(
+ overlap,
+ gt_data,
+ dt_data,
+ ignored_gt,
+ ignored_det,
+ dontcare,
+ metric,
+ min_overlap=min_overlap,
+ thresh=thresh,
+ compute_fp=True,
+ compute_aos=compute_aos)
+ pr[t, 0] += tp
+ pr[t, 1] += fp
+ pr[t, 2] += fn
+ if similarity != -1:
+ pr[t, 3] += similarity
+ gt_num += gt_nums[i]
+ dt_num += dt_nums[i]
+ dc_num += dc_nums[i]
+
+
+def calculate_iou_partly(gt_annos, dt_annos, metric, num_parts=50):
+ """Fast iou algorithm. this function can be used independently to do result
+ analysis. Must be used in CAMERA coordinate system.
+
+ Args:
+ gt_annos (dict): Must from get_label_annos() in kitti_common.py.
+ dt_annos (dict): Must from get_label_annos() in kitti_common.py.
+ metric (int): Eval type. 0: bbox, 1: bev, 2: 3d.
+ num_parts (int): A parameter for fast calculate algorithm.
+ """
+ assert len(gt_annos) == len(dt_annos)
+ total_dt_num = np.stack([len(a['name']) for a in dt_annos], 0)
+ total_gt_num = np.stack([len(a['name']) for a in gt_annos], 0)
+ num_examples = len(gt_annos)
+ split_parts = get_split_parts(num_examples, num_parts)
+ parted_overlaps = []
+ example_idx = 0
+
+ for num_part in split_parts:
+ gt_annos_part = gt_annos[example_idx:example_idx + num_part]
+ dt_annos_part = dt_annos[example_idx:example_idx + num_part]
+ if metric == 0:
+ gt_boxes = np.concatenate([a['bbox'] for a in gt_annos_part], 0)
+ dt_boxes = np.concatenate([a['bbox'] for a in dt_annos_part], 0)
+ overlap_part = image_box_overlap(gt_boxes, dt_boxes)
+ elif metric == 1:
+ loc = np.concatenate(
+ [a['location'][:, [0, 2]] for a in gt_annos_part], 0)
+ dims = np.concatenate(
+ [a['dimensions'][:, [0, 2]] for a in gt_annos_part], 0)
+ rots = np.concatenate([a['rotation_y'] for a in gt_annos_part], 0)
+ gt_boxes = np.concatenate([loc, dims, rots[..., np.newaxis]],
+ axis=1)
+ loc = np.concatenate(
+ [a['location'][:, [0, 2]] for a in dt_annos_part], 0)
+ dims = np.concatenate(
+ [a['dimensions'][:, [0, 2]] for a in dt_annos_part], 0)
+ rots = np.concatenate([a['rotation_y'] for a in dt_annos_part], 0)
+ dt_boxes = np.concatenate([loc, dims, rots[..., np.newaxis]],
+ axis=1)
+ overlap_part = bev_box_overlap(gt_boxes,
+ dt_boxes).astype(np.float64)
+ elif metric == 2:
+ loc = np.concatenate([a['location'] for a in gt_annos_part], 0)
+ dims = np.concatenate([a['dimensions'] for a in gt_annos_part], 0)
+ rots = np.concatenate([a['rotation_y'] for a in gt_annos_part], 0)
+ gt_boxes = np.concatenate([loc, dims, rots[..., np.newaxis]],
+ axis=1)
+ loc = np.concatenate([a['location'] for a in dt_annos_part], 0)
+ dims = np.concatenate([a['dimensions'] for a in dt_annos_part], 0)
+ rots = np.concatenate([a['rotation_y'] for a in dt_annos_part], 0)
+ dt_boxes = np.concatenate([loc, dims, rots[..., np.newaxis]],
+ axis=1)
+ overlap_part = d3_box_overlap(gt_boxes,
+ dt_boxes).astype(np.float64)
+ else:
+ raise ValueError('unknown metric')
+ parted_overlaps.append(overlap_part)
+ example_idx += num_part
+ overlaps = []
+ example_idx = 0
+ for j, num_part in enumerate(split_parts):
+ gt_annos_part = gt_annos[example_idx:example_idx + num_part]
+ dt_annos_part = dt_annos[example_idx:example_idx + num_part]
+ gt_num_idx, dt_num_idx = 0, 0
+ for i in range(num_part):
+ gt_box_num = total_gt_num[example_idx + i]
+ dt_box_num = total_dt_num[example_idx + i]
+ overlaps.append(
+ parted_overlaps[j][gt_num_idx:gt_num_idx + gt_box_num,
+ dt_num_idx:dt_num_idx + dt_box_num])
+ gt_num_idx += gt_box_num
+ dt_num_idx += dt_box_num
+ example_idx += num_part
+
+ return overlaps, parted_overlaps, total_gt_num, total_dt_num
+
+
+def _prepare_data(gt_annos, dt_annos, current_class, difficulty):
+ gt_datas_list = []
+ dt_datas_list = []
+ total_dc_num = []
+ ignored_gts, ignored_dets, dontcares = [], [], []
+ total_num_valid_gt = 0
+ for i in range(len(gt_annos)):
+ rets = clean_data(gt_annos[i], dt_annos[i], current_class, difficulty)
+ num_valid_gt, ignored_gt, ignored_det, dc_bboxes = rets
+ ignored_gts.append(np.array(ignored_gt, dtype=np.int64))
+ ignored_dets.append(np.array(ignored_det, dtype=np.int64))
+ if len(dc_bboxes) == 0:
+ dc_bboxes = np.zeros((0, 4)).astype(np.float64)
+ else:
+ dc_bboxes = np.stack(dc_bboxes, 0).astype(np.float64)
+ total_dc_num.append(dc_bboxes.shape[0])
+ dontcares.append(dc_bboxes)
+ total_num_valid_gt += num_valid_gt
+ gt_datas = np.concatenate(
+ [gt_annos[i]['bbox'], gt_annos[i]['alpha'][..., np.newaxis]], 1)
+ dt_datas = np.concatenate([
+ dt_annos[i]['bbox'], dt_annos[i]['alpha'][..., np.newaxis],
+ dt_annos[i]['score'][..., np.newaxis]
+ ], 1)
+ gt_datas_list.append(gt_datas)
+ dt_datas_list.append(dt_datas)
+ total_dc_num = np.stack(total_dc_num, axis=0)
+ return (gt_datas_list, dt_datas_list, ignored_gts, ignored_dets, dontcares,
+ total_dc_num, total_num_valid_gt)
+
+
+def eval_class(gt_annos,
+ dt_annos,
+ current_classes,
+ difficultys,
+ metric,
+ min_overlaps,
+ compute_aos=False,
+ num_parts=200):
+ """Kitti eval. support 2d/bev/3d/aos eval. support 0.5:0.05:0.95 coco AP.
+
+ Args:
+ gt_annos (dict): Must from get_label_annos() in kitti_common.py.
+ dt_annos (dict): Must from get_label_annos() in kitti_common.py.
+ current_classes (list[int]): 0: car, 1: pedestrian, 2: cyclist.
+ difficultys (list[int]): Eval difficulty, 0: easy, 1: normal, 2: hard
+ metric (int): Eval type. 0: bbox, 1: bev, 2: 3d
+ min_overlaps (float): Min overlap. format:
+ [num_overlap, metric, class].
+ num_parts (int): A parameter for fast calculate algorithm
+
+ Returns:
+ dict[str, np.ndarray]: recall, precision and aos
+ """
+ assert len(gt_annos) == len(dt_annos)
+ num_examples = len(gt_annos)
+ if num_examples < num_parts:
+ num_parts = num_examples
+ split_parts = get_split_parts(num_examples, num_parts)
+
+ rets = calculate_iou_partly(dt_annos, gt_annos, metric, num_parts)
+ overlaps, parted_overlaps, total_dt_num, total_gt_num = rets
+ N_SAMPLE_PTS = 41
+ num_minoverlap = len(min_overlaps)
+ num_class = len(current_classes)
+ num_difficulty = len(difficultys)
+ precision = np.zeros(
+ [num_class, num_difficulty, num_minoverlap, N_SAMPLE_PTS])
+ recall = np.zeros(
+ [num_class, num_difficulty, num_minoverlap, N_SAMPLE_PTS])
+ aos = np.zeros([num_class, num_difficulty, num_minoverlap, N_SAMPLE_PTS])
+ for m, current_class in enumerate(current_classes):
+ for idx_l, difficulty in enumerate(difficultys):
+ rets = _prepare_data(gt_annos, dt_annos, current_class, difficulty)
+ (gt_datas_list, dt_datas_list, ignored_gts, ignored_dets,
+ dontcares, total_dc_num, total_num_valid_gt) = rets
+ for k, min_overlap in enumerate(min_overlaps[:, metric, m]):
+ thresholdss = []
+ for i in range(len(gt_annos)):
+ rets = compute_statistics_jit(
+ overlaps[i],
+ gt_datas_list[i],
+ dt_datas_list[i],
+ ignored_gts[i],
+ ignored_dets[i],
+ dontcares[i],
+ metric,
+ min_overlap=min_overlap,
+ thresh=0.0,
+ compute_fp=False)
+ tp, fp, fn, similarity, thresholds = rets
+ thresholdss += thresholds.tolist()
+ thresholdss = np.array(thresholdss)
+ thresholds = get_thresholds(thresholdss, total_num_valid_gt)
+ thresholds = np.array(thresholds)
+ pr = np.zeros([len(thresholds), 4])
+ idx = 0
+ for j, num_part in enumerate(split_parts):
+ gt_datas_part = np.concatenate(
+ gt_datas_list[idx:idx + num_part], 0)
+ dt_datas_part = np.concatenate(
+ dt_datas_list[idx:idx + num_part], 0)
+ dc_datas_part = np.concatenate(
+ dontcares[idx:idx + num_part], 0)
+ ignored_dets_part = np.concatenate(
+ ignored_dets[idx:idx + num_part], 0)
+ ignored_gts_part = np.concatenate(
+ ignored_gts[idx:idx + num_part], 0)
+ fused_compute_statistics(
+ parted_overlaps[j],
+ pr,
+ total_gt_num[idx:idx + num_part],
+ total_dt_num[idx:idx + num_part],
+ total_dc_num[idx:idx + num_part],
+ gt_datas_part,
+ dt_datas_part,
+ dc_datas_part,
+ ignored_gts_part,
+ ignored_dets_part,
+ metric,
+ min_overlap=min_overlap,
+ thresholds=thresholds,
+ compute_aos=compute_aos)
+ idx += num_part
+ for i in range(len(thresholds)):
+ recall[m, idx_l, k, i] = pr[i, 0] / (pr[i, 0] + pr[i, 2])
+ precision[m, idx_l, k, i] = pr[i, 0] / (
+ pr[i, 0] + pr[i, 1])
+ if compute_aos:
+ aos[m, idx_l, k, i] = pr[i, 3] / (pr[i, 0] + pr[i, 1])
+ for i in range(len(thresholds)):
+ precision[m, idx_l, k, i] = np.max(
+ precision[m, idx_l, k, i:], axis=-1)
+ recall[m, idx_l, k, i] = np.max(
+ recall[m, idx_l, k, i:], axis=-1)
+ if compute_aos:
+ aos[m, idx_l, k, i] = np.max(
+ aos[m, idx_l, k, i:], axis=-1)
+ ret_dict = {
+ 'recall': recall,
+ 'precision': precision,
+ 'orientation': aos,
+ }
+
+ # clean temp variables
+ del overlaps
+ del parted_overlaps
+
+ gc.collect()
+ return ret_dict
+
+
+def get_mAP11(prec):
+ sums = 0
+ for i in range(0, prec.shape[-1], 4):
+ sums = sums + prec[..., i]
+ return sums / 11 * 100
+
+
+def get_mAP40(prec):
+ sums = 0
+ for i in range(1, prec.shape[-1]):
+ sums = sums + prec[..., i]
+ return sums / 40 * 100
+
+
+def print_str(value, *arg, sstream=None):
+ if sstream is None:
+ sstream = sysio.StringIO()
+ sstream.truncate(0)
+ sstream.seek(0)
+ print(value, *arg, file=sstream)
+ return sstream.getvalue()
+
+
+def do_eval(gt_annos,
+ dt_annos,
+ current_classes,
+ min_overlaps,
+ eval_types=['bbox', 'bev', '3d']):
+ # min_overlaps: [num_minoverlap, metric, num_class]
+ difficultys = [0, 1, 2]
+ mAP11_bbox = None
+ mAP11_aos = None
+ mAP40_bbox = None
+ mAP40_aos = None
+ if 'bbox' in eval_types:
+ ret = eval_class(
+ gt_annos,
+ dt_annos,
+ current_classes,
+ difficultys,
+ 0,
+ min_overlaps,
+ compute_aos=('aos' in eval_types))
+ # ret: [num_class, num_diff, num_minoverlap, num_sample_points]
+ mAP11_bbox = get_mAP11(ret['precision'])
+ mAP40_bbox = get_mAP40(ret['precision'])
+ if 'aos' in eval_types:
+ mAP11_aos = get_mAP11(ret['orientation'])
+ mAP40_aos = get_mAP40(ret['orientation'])
+
+ mAP11_bev = None
+ mAP40_bev = None
+ if 'bev' in eval_types:
+ ret = eval_class(gt_annos, dt_annos, current_classes, difficultys, 1,
+ min_overlaps)
+ mAP11_bev = get_mAP11(ret['precision'])
+ mAP40_bev = get_mAP40(ret['precision'])
+
+ mAP11_3d = None
+ mAP40_3d = None
+ if '3d' in eval_types:
+ ret = eval_class(gt_annos, dt_annos, current_classes, difficultys, 2,
+ min_overlaps)
+ mAP11_3d = get_mAP11(ret['precision'])
+ mAP40_3d = get_mAP40(ret['precision'])
+ return (mAP11_bbox, mAP11_bev, mAP11_3d, mAP11_aos, mAP40_bbox, mAP40_bev,
+ mAP40_3d, mAP40_aos)
+
+
+def do_coco_style_eval(gt_annos, dt_annos, current_classes, overlap_ranges,
+ compute_aos):
+ # overlap_ranges: [range, metric, num_class]
+ min_overlaps = np.zeros([10, *overlap_ranges.shape[1:]])
+ for i in range(overlap_ranges.shape[1]):
+ for j in range(overlap_ranges.shape[2]):
+ min_overlaps[:, i, j] = np.linspace(*overlap_ranges[:, i, j])
+ mAP_bbox, mAP_bev, mAP_3d, mAP_aos, _, _, \
+ _, _ = do_eval(gt_annos, dt_annos,
+ current_classes, min_overlaps,
+ compute_aos)
+ # ret: [num_class, num_diff, num_minoverlap]
+ mAP_bbox = mAP_bbox.mean(-1)
+ mAP_bev = mAP_bev.mean(-1)
+ mAP_3d = mAP_3d.mean(-1)
+ if mAP_aos is not None:
+ mAP_aos = mAP_aos.mean(-1)
+ return mAP_bbox, mAP_bev, mAP_3d, mAP_aos
+
+
+def kitti_eval(gt_annos,
+ dt_annos,
+ current_classes,
+ eval_types=['bbox', 'bev', '3d']):
+ """KITTI evaluation.
+
+ Args:
+ gt_annos (list[dict]): Contain gt information of each sample.
+ dt_annos (list[dict]): Contain detected information of each sample.
+ current_classes (list[str]): Classes to evaluation.
+ eval_types (list[str], optional): Types to eval.
+ Defaults to ['bbox', 'bev', '3d'].
+
+ Returns:
+ tuple: String and dict of evaluation results.
+ """
+ assert len(eval_types) > 0, 'must contain at least one evaluation type'
+ if 'aos' in eval_types:
+ assert 'bbox' in eval_types, 'must evaluate bbox when evaluating aos'
+ overlap_0_7 = np.array([[0.7, 0.5, 0.5, 0.7,
+ 0.5], [0.7, 0.5, 0.5, 0.7, 0.5],
+ [0.7, 0.5, 0.5, 0.7, 0.5]])
+ overlap_0_5 = np.array([[0.7, 0.5, 0.5, 0.7, 0.5],
+ [0.5, 0.25, 0.25, 0.5, 0.25],
+ [0.5, 0.25, 0.25, 0.5, 0.25]])
+ min_overlaps = np.stack([overlap_0_7, overlap_0_5], axis=0) # [2, 3, 5]
+ class_to_name = {
+ 0: 'Car',
+ 1: 'Pedestrian',
+ 2: 'Cyclist',
+ 3: 'Van',
+ 4: 'Person_sitting',
+ }
+ name_to_class = {v: n for n, v in class_to_name.items()}
+ if not isinstance(current_classes, (list, tuple)):
+ current_classes = [current_classes]
+ current_classes_int = []
+ for curcls in current_classes:
+ if isinstance(curcls, str):
+ current_classes_int.append(name_to_class[curcls])
+ else:
+ current_classes_int.append(curcls)
+ current_classes = current_classes_int
+ min_overlaps = min_overlaps[:, :, current_classes]
+ result = ''
+ # check whether alpha is valid
+ compute_aos = False
+ pred_alpha = False
+ valid_alpha_gt = False
+ for anno in dt_annos:
+ mask = (anno['alpha'] != -10)
+ if anno['alpha'][mask].shape[0] != 0:
+ pred_alpha = True
+ break
+ for anno in gt_annos:
+ if anno['alpha'][0] != -10:
+ valid_alpha_gt = True
+ break
+ compute_aos = (pred_alpha and valid_alpha_gt)
+ if compute_aos:
+ eval_types.append('aos')
+
+ mAP11_bbox, mAP11_bev, mAP11_3d, mAP11_aos, mAP40_bbox, mAP40_bev, \
+ mAP40_3d, mAP40_aos = do_eval(gt_annos, dt_annos,
+ current_classes, min_overlaps,
+ eval_types)
+
+ ret_dict = {}
+ difficulty = ['easy', 'moderate', 'hard']
+
+ # calculate AP11
+ result += '\n----------- AP11 Results ------------\n\n'
+ for j, curcls in enumerate(current_classes):
+ # mAP threshold array: [num_minoverlap, metric, class]
+ # mAP result: [num_class, num_diff, num_minoverlap]
+ curcls_name = class_to_name[curcls]
+ for i in range(min_overlaps.shape[0]):
+ # prepare results for print
+ result += ('{} AP11@{:.2f}, {:.2f}, {:.2f}:\n'.format(
+ curcls_name, *min_overlaps[i, :, j]))
+ if mAP11_bbox is not None:
+ result += 'bbox AP11:{:.4f}, {:.4f}, {:.4f}\n'.format(
+ *mAP11_bbox[j, :, i])
+ if mAP11_bev is not None:
+ result += 'bev AP11:{:.4f}, {:.4f}, {:.4f}\n'.format(
+ *mAP11_bev[j, :, i])
+ if mAP11_3d is not None:
+ result += '3d AP11:{:.4f}, {:.4f}, {:.4f}\n'.format(
+ *mAP11_3d[j, :, i])
+ if compute_aos:
+ result += 'aos AP11:{:.2f}, {:.2f}, {:.2f}\n'.format(
+ *mAP11_aos[j, :, i])
+
+ # prepare results for logger
+ for idx in range(3):
+ if i == 0:
+ postfix = f'{difficulty[idx]}_strict'
+ else:
+ postfix = f'{difficulty[idx]}_loose'
+ prefix = f'KITTI/{curcls_name}'
+ if mAP11_3d is not None:
+ ret_dict[f'{prefix}_3D_AP11_{postfix}'] =\
+ mAP11_3d[j, idx, i]
+ if mAP11_bev is not None:
+ ret_dict[f'{prefix}_BEV_AP11_{postfix}'] =\
+ mAP11_bev[j, idx, i]
+ if mAP11_bbox is not None:
+ ret_dict[f'{prefix}_2D_AP11_{postfix}'] =\
+ mAP11_bbox[j, idx, i]
+
+ # calculate mAP11 over all classes if there are multiple classes
+ if len(current_classes) > 1:
+ # prepare results for print
+ result += ('\nOverall AP11@{}, {}, {}:\n'.format(*difficulty))
+ if mAP11_bbox is not None:
+ mAP11_bbox = mAP11_bbox.mean(axis=0)
+ result += 'bbox AP11:{:.4f}, {:.4f}, {:.4f}\n'.format(
+ *mAP11_bbox[:, 0])
+ if mAP11_bev is not None:
+ mAP11_bev = mAP11_bev.mean(axis=0)
+ result += 'bev AP11:{:.4f}, {:.4f}, {:.4f}\n'.format(
+ *mAP11_bev[:, 0])
+ if mAP11_3d is not None:
+ mAP11_3d = mAP11_3d.mean(axis=0)
+ result += '3d AP11:{:.4f}, {:.4f}, {:.4f}\n'.format(*mAP11_3d[:,
+ 0])
+ if compute_aos:
+ mAP11_aos = mAP11_aos.mean(axis=0)
+ result += 'aos AP11:{:.2f}, {:.2f}, {:.2f}\n'.format(
+ *mAP11_aos[:, 0])
+
+ # prepare results for logger
+ for idx in range(3):
+ postfix = f'{difficulty[idx]}'
+ if mAP11_3d is not None:
+ ret_dict[f'KITTI/Overall_3D_AP11_{postfix}'] = mAP11_3d[idx, 0]
+ if mAP11_bev is not None:
+ ret_dict[f'KITTI/Overall_BEV_AP11_{postfix}'] =\
+ mAP11_bev[idx, 0]
+ if mAP11_bbox is not None:
+ ret_dict[f'KITTI/Overall_2D_AP11_{postfix}'] =\
+ mAP11_bbox[idx, 0]
+
+ # Calculate AP40
+ result += '\n----------- AP40 Results ------------\n\n'
+ for j, curcls in enumerate(current_classes):
+ # mAP threshold array: [num_minoverlap, metric, class]
+ # mAP result: [num_class, num_diff, num_minoverlap]
+ curcls_name = class_to_name[curcls]
+ for i in range(min_overlaps.shape[0]):
+ # prepare results for print
+ result += ('{} AP40@{:.2f}, {:.2f}, {:.2f}:\n'.format(
+ curcls_name, *min_overlaps[i, :, j]))
+ if mAP40_bbox is not None:
+ result += 'bbox AP40:{:.4f}, {:.4f}, {:.4f}\n'.format(
+ *mAP40_bbox[j, :, i])
+ if mAP40_bev is not None:
+ result += 'bev AP40:{:.4f}, {:.4f}, {:.4f}\n'.format(
+ *mAP40_bev[j, :, i])
+ if mAP40_3d is not None:
+ result += '3d AP40:{:.4f}, {:.4f}, {:.4f}\n'.format(
+ *mAP40_3d[j, :, i])
+ if compute_aos:
+ result += 'aos AP40:{:.2f}, {:.2f}, {:.2f}\n'.format(
+ *mAP40_aos[j, :, i])
+
+ # prepare results for logger
+ for idx in range(3):
+ if i == 0:
+ postfix = f'{difficulty[idx]}_strict'
+ else:
+ postfix = f'{difficulty[idx]}_loose'
+ prefix = f'KITTI/{curcls_name}'
+ if mAP40_3d is not None:
+ ret_dict[f'{prefix}_3D_AP40_{postfix}'] =\
+ mAP40_3d[j, idx, i]
+ if mAP40_bev is not None:
+ ret_dict[f'{prefix}_BEV_AP40_{postfix}'] =\
+ mAP40_bev[j, idx, i]
+ if mAP40_bbox is not None:
+ ret_dict[f'{prefix}_2D_AP40_{postfix}'] =\
+ mAP40_bbox[j, idx, i]
+
+ # calculate mAP40 over all classes if there are multiple classes
+ if len(current_classes) > 1:
+ # prepare results for print
+ result += ('\nOverall AP40@{}, {}, {}:\n'.format(*difficulty))
+ if mAP40_bbox is not None:
+ mAP40_bbox = mAP40_bbox.mean(axis=0)
+ result += 'bbox AP40:{:.4f}, {:.4f}, {:.4f}\n'.format(
+ *mAP40_bbox[:, 0])
+ if mAP40_bev is not None:
+ mAP40_bev = mAP40_bev.mean(axis=0)
+ result += 'bev AP40:{:.4f}, {:.4f}, {:.4f}\n'.format(
+ *mAP40_bev[:, 0])
+ if mAP40_3d is not None:
+ mAP40_3d = mAP40_3d.mean(axis=0)
+ result += '3d AP40:{:.4f}, {:.4f}, {:.4f}\n'.format(*mAP40_3d[:,
+ 0])
+ if compute_aos:
+ mAP40_aos = mAP40_aos.mean(axis=0)
+ result += 'aos AP40:{:.2f}, {:.2f}, {:.2f}\n'.format(
+ *mAP40_aos[:, 0])
+
+ # prepare results for logger
+ for idx in range(3):
+ postfix = f'{difficulty[idx]}'
+ if mAP40_3d is not None:
+ ret_dict[f'KITTI/Overall_3D_AP40_{postfix}'] = mAP40_3d[idx, 0]
+ if mAP40_bev is not None:
+ ret_dict[f'KITTI/Overall_BEV_AP40_{postfix}'] =\
+ mAP40_bev[idx, 0]
+ if mAP40_bbox is not None:
+ ret_dict[f'KITTI/Overall_2D_AP40_{postfix}'] =\
+ mAP40_bbox[idx, 0]
+
+ return result, ret_dict
+
+
+def kitti_eval_coco_style(gt_annos, dt_annos, current_classes):
+ """coco style evaluation of kitti.
+
+ Args:
+ gt_annos (list[dict]): Contain gt information of each sample.
+ dt_annos (list[dict]): Contain detected information of each sample.
+ current_classes (list[str]): Classes to evaluation.
+
+ Returns:
+ string: Evaluation results.
+ """
+ class_to_name = {
+ 0: 'Car',
+ 1: 'Pedestrian',
+ 2: 'Cyclist',
+ 3: 'Van',
+ 4: 'Person_sitting',
+ }
+ class_to_range = {
+ 0: [0.5, 0.95, 10],
+ 1: [0.25, 0.7, 10],
+ 2: [0.25, 0.7, 10],
+ 3: [0.5, 0.95, 10],
+ 4: [0.25, 0.7, 10],
+ }
+ name_to_class = {v: n for n, v in class_to_name.items()}
+ if not isinstance(current_classes, (list, tuple)):
+ current_classes = [current_classes]
+ current_classes_int = []
+ for curcls in current_classes:
+ if isinstance(curcls, str):
+ current_classes_int.append(name_to_class[curcls])
+ else:
+ current_classes_int.append(curcls)
+ current_classes = current_classes_int
+ overlap_ranges = np.zeros([3, 3, len(current_classes)])
+ for i, curcls in enumerate(current_classes):
+ overlap_ranges[:, :, i] = np.array(class_to_range[curcls])[:,
+ np.newaxis]
+ result = ''
+ # check whether alpha is valid
+ compute_aos = False
+ for anno in dt_annos:
+ if anno['alpha'].shape[0] != 0:
+ if anno['alpha'][0] != -10:
+ compute_aos = True
+ break
+ mAPbbox, mAPbev, mAP3d, mAPaos = do_coco_style_eval(
+ gt_annos, dt_annos, current_classes, overlap_ranges, compute_aos)
+ for j, curcls in enumerate(current_classes):
+ # mAP threshold array: [num_minoverlap, metric, class]
+ # mAP result: [num_class, num_diff, num_minoverlap]
+ o_range = np.array(class_to_range[curcls])[[0, 2, 1]]
+ o_range[1] = (o_range[2] - o_range[0]) / (o_range[1] - 1)
+ result += print_str((f'{class_to_name[curcls]} '
+ 'coco AP@{:.2f}:{:.2f}:{:.2f}:'.format(*o_range)))
+ result += print_str((f'bbox AP:{mAPbbox[j, 0]:.2f}, '
+ f'{mAPbbox[j, 1]:.2f}, '
+ f'{mAPbbox[j, 2]:.2f}'))
+ result += print_str((f'bev AP:{mAPbev[j, 0]:.2f}, '
+ f'{mAPbev[j, 1]:.2f}, '
+ f'{mAPbev[j, 2]:.2f}'))
+ result += print_str((f'3d AP:{mAP3d[j, 0]:.2f}, '
+ f'{mAP3d[j, 1]:.2f}, '
+ f'{mAP3d[j, 2]:.2f}'))
+ if compute_aos:
+ result += print_str((f'aos AP:{mAPaos[j, 0]:.2f}, '
+ f'{mAPaos[j, 1]:.2f}, '
+ f'{mAPaos[j, 2]:.2f}'))
+ return result
diff --git a/mmdet3d/core/evaluation/kitti_utils/rotate_iou.py b/mmdet3d/core/evaluation/kitti_utils/rotate_iou.py
new file mode 100644
index 0000000..9ed75bf
--- /dev/null
+++ b/mmdet3d/core/evaluation/kitti_utils/rotate_iou.py
@@ -0,0 +1,379 @@
+# Copyright (c) OpenMMLab. All rights reserved.
+#####################
+# Based on https://github.com/hongzhenwang/RRPN-revise
+# Licensed under The MIT License
+# Author: yanyan, scrin@foxmail.com
+#####################
+import math
+
+import numba
+import numpy as np
+from numba import cuda
+
+
+@numba.jit(nopython=True)
+def div_up(m, n):
+ return m // n + (m % n > 0)
+
+
+@cuda.jit(device=True, inline=True)
+def trangle_area(a, b, c):
+ return ((a[0] - c[0]) * (b[1] - c[1]) - (a[1] - c[1]) *
+ (b[0] - c[0])) / 2.0
+
+
+@cuda.jit(device=True, inline=True)
+def area(int_pts, num_of_inter):
+ area_val = 0.0
+ for i in range(num_of_inter - 2):
+ area_val += abs(
+ trangle_area(int_pts[:2], int_pts[2 * i + 2:2 * i + 4],
+ int_pts[2 * i + 4:2 * i + 6]))
+ return area_val
+
+
+@cuda.jit(device=True, inline=True)
+def sort_vertex_in_convex_polygon(int_pts, num_of_inter):
+ if num_of_inter > 0:
+ center = cuda.local.array((2, ), dtype=numba.float32)
+ center[:] = 0.0
+ for i in range(num_of_inter):
+ center[0] += int_pts[2 * i]
+ center[1] += int_pts[2 * i + 1]
+ center[0] /= num_of_inter
+ center[1] /= num_of_inter
+ v = cuda.local.array((2, ), dtype=numba.float32)
+ vs = cuda.local.array((16, ), dtype=numba.float32)
+ for i in range(num_of_inter):
+ v[0] = int_pts[2 * i] - center[0]
+ v[1] = int_pts[2 * i + 1] - center[1]
+ d = math.sqrt(v[0] * v[0] + v[1] * v[1])
+ v[0] = v[0] / d
+ v[1] = v[1] / d
+ if v[1] < 0:
+ v[0] = -2 - v[0]
+ vs[i] = v[0]
+ j = 0
+ temp = 0
+ for i in range(1, num_of_inter):
+ if vs[i - 1] > vs[i]:
+ temp = vs[i]
+ tx = int_pts[2 * i]
+ ty = int_pts[2 * i + 1]
+ j = i
+ while j > 0 and vs[j - 1] > temp:
+ vs[j] = vs[j - 1]
+ int_pts[j * 2] = int_pts[j * 2 - 2]
+ int_pts[j * 2 + 1] = int_pts[j * 2 - 1]
+ j -= 1
+
+ vs[j] = temp
+ int_pts[j * 2] = tx
+ int_pts[j * 2 + 1] = ty
+
+
+@cuda.jit(device=True, inline=True)
+def line_segment_intersection(pts1, pts2, i, j, temp_pts):
+ A = cuda.local.array((2, ), dtype=numba.float32)
+ B = cuda.local.array((2, ), dtype=numba.float32)
+ C = cuda.local.array((2, ), dtype=numba.float32)
+ D = cuda.local.array((2, ), dtype=numba.float32)
+
+ A[0] = pts1[2 * i]
+ A[1] = pts1[2 * i + 1]
+
+ B[0] = pts1[2 * ((i + 1) % 4)]
+ B[1] = pts1[2 * ((i + 1) % 4) + 1]
+
+ C[0] = pts2[2 * j]
+ C[1] = pts2[2 * j + 1]
+
+ D[0] = pts2[2 * ((j + 1) % 4)]
+ D[1] = pts2[2 * ((j + 1) % 4) + 1]
+ BA0 = B[0] - A[0]
+ BA1 = B[1] - A[1]
+ DA0 = D[0] - A[0]
+ CA0 = C[0] - A[0]
+ DA1 = D[1] - A[1]
+ CA1 = C[1] - A[1]
+ acd = DA1 * CA0 > CA1 * DA0
+ bcd = (D[1] - B[1]) * (C[0] - B[0]) > (C[1] - B[1]) * (D[0] - B[0])
+ if acd != bcd:
+ abc = CA1 * BA0 > BA1 * CA0
+ abd = DA1 * BA0 > BA1 * DA0
+ if abc != abd:
+ DC0 = D[0] - C[0]
+ DC1 = D[1] - C[1]
+ ABBA = A[0] * B[1] - B[0] * A[1]
+ CDDC = C[0] * D[1] - D[0] * C[1]
+ DH = BA1 * DC0 - BA0 * DC1
+ Dx = ABBA * DC0 - BA0 * CDDC
+ Dy = ABBA * DC1 - BA1 * CDDC
+ temp_pts[0] = Dx / DH
+ temp_pts[1] = Dy / DH
+ return True
+ return False
+
+
+@cuda.jit(device=True, inline=True)
+def line_segment_intersection_v1(pts1, pts2, i, j, temp_pts):
+ a = cuda.local.array((2, ), dtype=numba.float32)
+ b = cuda.local.array((2, ), dtype=numba.float32)
+ c = cuda.local.array((2, ), dtype=numba.float32)
+ d = cuda.local.array((2, ), dtype=numba.float32)
+
+ a[0] = pts1[2 * i]
+ a[1] = pts1[2 * i + 1]
+
+ b[0] = pts1[2 * ((i + 1) % 4)]
+ b[1] = pts1[2 * ((i + 1) % 4) + 1]
+
+ c[0] = pts2[2 * j]
+ c[1] = pts2[2 * j + 1]
+
+ d[0] = pts2[2 * ((j + 1) % 4)]
+ d[1] = pts2[2 * ((j + 1) % 4) + 1]
+
+ area_abc = trangle_area(a, b, c)
+ area_abd = trangle_area(a, b, d)
+
+ if area_abc * area_abd >= 0:
+ return False
+
+ area_cda = trangle_area(c, d, a)
+ area_cdb = area_cda + area_abc - area_abd
+
+ if area_cda * area_cdb >= 0:
+ return False
+ t = area_cda / (area_abd - area_abc)
+
+ dx = t * (b[0] - a[0])
+ dy = t * (b[1] - a[1])
+ temp_pts[0] = a[0] + dx
+ temp_pts[1] = a[1] + dy
+ return True
+
+
+@cuda.jit(device=True, inline=True)
+def point_in_quadrilateral(pt_x, pt_y, corners):
+ ab0 = corners[2] - corners[0]
+ ab1 = corners[3] - corners[1]
+
+ ad0 = corners[6] - corners[0]
+ ad1 = corners[7] - corners[1]
+
+ ap0 = pt_x - corners[0]
+ ap1 = pt_y - corners[1]
+
+ abab = ab0 * ab0 + ab1 * ab1
+ abap = ab0 * ap0 + ab1 * ap1
+ adad = ad0 * ad0 + ad1 * ad1
+ adap = ad0 * ap0 + ad1 * ap1
+
+ return abab >= abap and abap >= 0 and adad >= adap and adap >= 0
+
+
+@cuda.jit(device=True, inline=True)
+def quadrilateral_intersection(pts1, pts2, int_pts):
+ num_of_inter = 0
+ for i in range(4):
+ if point_in_quadrilateral(pts1[2 * i], pts1[2 * i + 1], pts2):
+ int_pts[num_of_inter * 2] = pts1[2 * i]
+ int_pts[num_of_inter * 2 + 1] = pts1[2 * i + 1]
+ num_of_inter += 1
+ if point_in_quadrilateral(pts2[2 * i], pts2[2 * i + 1], pts1):
+ int_pts[num_of_inter * 2] = pts2[2 * i]
+ int_pts[num_of_inter * 2 + 1] = pts2[2 * i + 1]
+ num_of_inter += 1
+ temp_pts = cuda.local.array((2, ), dtype=numba.float32)
+ for i in range(4):
+ for j in range(4):
+ has_pts = line_segment_intersection(pts1, pts2, i, j, temp_pts)
+ if has_pts:
+ int_pts[num_of_inter * 2] = temp_pts[0]
+ int_pts[num_of_inter * 2 + 1] = temp_pts[1]
+ num_of_inter += 1
+
+ return num_of_inter
+
+
+@cuda.jit(device=True, inline=True)
+def rbbox_to_corners(corners, rbbox):
+ # generate clockwise corners and rotate it clockwise
+ angle = rbbox[4]
+ a_cos = math.cos(angle)
+ a_sin = math.sin(angle)
+ center_x = rbbox[0]
+ center_y = rbbox[1]
+ x_d = rbbox[2]
+ y_d = rbbox[3]
+ corners_x = cuda.local.array((4, ), dtype=numba.float32)
+ corners_y = cuda.local.array((4, ), dtype=numba.float32)
+ corners_x[0] = -x_d / 2
+ corners_x[1] = -x_d / 2
+ corners_x[2] = x_d / 2
+ corners_x[3] = x_d / 2
+ corners_y[0] = -y_d / 2
+ corners_y[1] = y_d / 2
+ corners_y[2] = y_d / 2
+ corners_y[3] = -y_d / 2
+ for i in range(4):
+ corners[2 * i] = a_cos * corners_x[i] + a_sin * corners_y[i] + center_x
+ corners[2 * i +
+ 1] = -a_sin * corners_x[i] + a_cos * corners_y[i] + center_y
+
+
+@cuda.jit(device=True, inline=True)
+def inter(rbbox1, rbbox2):
+ """Compute intersection of two rotated boxes.
+
+ Args:
+ rbox1 (np.ndarray, shape=[5]): Rotated 2d box.
+ rbox2 (np.ndarray, shape=[5]): Rotated 2d box.
+
+ Returns:
+ float: Intersection of two rotated boxes.
+ """
+ corners1 = cuda.local.array((8, ), dtype=numba.float32)
+ corners2 = cuda.local.array((8, ), dtype=numba.float32)
+ intersection_corners = cuda.local.array((16, ), dtype=numba.float32)
+
+ rbbox_to_corners(corners1, rbbox1)
+ rbbox_to_corners(corners2, rbbox2)
+
+ num_intersection = quadrilateral_intersection(corners1, corners2,
+ intersection_corners)
+ sort_vertex_in_convex_polygon(intersection_corners, num_intersection)
+ # print(intersection_corners.reshape([-1, 2])[:num_intersection])
+
+ return area(intersection_corners, num_intersection)
+
+
+@cuda.jit(device=True, inline=True)
+def devRotateIoUEval(rbox1, rbox2, criterion=-1):
+ """Compute rotated iou on device.
+
+ Args:
+ rbox1 (np.ndarray, shape=[5]): Rotated 2d box.
+ rbox2 (np.ndarray, shape=[5]): Rotated 2d box.
+ criterion (int, optional): Indicate different type of iou.
+ -1 indicate `area_inter / (area1 + area2 - area_inter)`,
+ 0 indicate `area_inter / area1`,
+ 1 indicate `area_inter / area2`.
+
+ Returns:
+ float: iou between two input boxes.
+ """
+ area1 = rbox1[2] * rbox1[3]
+ area2 = rbox2[2] * rbox2[3]
+ area_inter = inter(rbox1, rbox2)
+ if criterion == -1:
+ return area_inter / (area1 + area2 - area_inter)
+ elif criterion == 0:
+ return area_inter / area1
+ elif criterion == 1:
+ return area_inter / area2
+ else:
+ return area_inter
+
+
+@cuda.jit(
+ '(int64, int64, float32[:], float32[:], float32[:], int32)',
+ fastmath=False)
+def rotate_iou_kernel_eval(N,
+ K,
+ dev_boxes,
+ dev_query_boxes,
+ dev_iou,
+ criterion=-1):
+ """Kernel of computing rotated IoU. This function is for bev boxes in
+ camera coordinate system ONLY (the rotation is clockwise).
+
+ Args:
+ N (int): The number of boxes.
+ K (int): The number of query boxes.
+ dev_boxes (np.ndarray): Boxes on device.
+ dev_query_boxes (np.ndarray): Query boxes on device.
+ dev_iou (np.ndarray): Computed iou to return.
+ criterion (int, optional): Indicate different type of iou.
+ -1 indicate `area_inter / (area1 + area2 - area_inter)`,
+ 0 indicate `area_inter / area1`,
+ 1 indicate `area_inter / area2`.
+ """
+ threadsPerBlock = 8 * 8
+ row_start = cuda.blockIdx.x
+ col_start = cuda.blockIdx.y
+ tx = cuda.threadIdx.x
+ row_size = min(N - row_start * threadsPerBlock, threadsPerBlock)
+ col_size = min(K - col_start * threadsPerBlock, threadsPerBlock)
+ block_boxes = cuda.shared.array(shape=(64 * 5, ), dtype=numba.float32)
+ block_qboxes = cuda.shared.array(shape=(64 * 5, ), dtype=numba.float32)
+
+ dev_query_box_idx = threadsPerBlock * col_start + tx
+ dev_box_idx = threadsPerBlock * row_start + tx
+ if (tx < col_size):
+ block_qboxes[tx * 5 + 0] = dev_query_boxes[dev_query_box_idx * 5 + 0]
+ block_qboxes[tx * 5 + 1] = dev_query_boxes[dev_query_box_idx * 5 + 1]
+ block_qboxes[tx * 5 + 2] = dev_query_boxes[dev_query_box_idx * 5 + 2]
+ block_qboxes[tx * 5 + 3] = dev_query_boxes[dev_query_box_idx * 5 + 3]
+ block_qboxes[tx * 5 + 4] = dev_query_boxes[dev_query_box_idx * 5 + 4]
+ if (tx < row_size):
+ block_boxes[tx * 5 + 0] = dev_boxes[dev_box_idx * 5 + 0]
+ block_boxes[tx * 5 + 1] = dev_boxes[dev_box_idx * 5 + 1]
+ block_boxes[tx * 5 + 2] = dev_boxes[dev_box_idx * 5 + 2]
+ block_boxes[tx * 5 + 3] = dev_boxes[dev_box_idx * 5 + 3]
+ block_boxes[tx * 5 + 4] = dev_boxes[dev_box_idx * 5 + 4]
+ cuda.syncthreads()
+ if tx < row_size:
+ for i in range(col_size):
+ offset = (
+ row_start * threadsPerBlock * K + col_start * threadsPerBlock +
+ tx * K + i)
+ dev_iou[offset] = devRotateIoUEval(block_qboxes[i * 5:i * 5 + 5],
+ block_boxes[tx * 5:tx * 5 + 5],
+ criterion)
+
+
+def rotate_iou_gpu_eval(boxes, query_boxes, criterion=-1, device_id=0):
+ """Rotated box iou running in gpu. 500x faster than cpu version (take 5ms
+ in one example with numba.cuda code). convert from [this project](
+ https://github.com/hongzhenwang/RRPN-revise/tree/master/lib/rotation).
+
+ This function is for bev boxes in camera coordinate system ONLY
+ (the rotation is clockwise).
+
+ Args:
+ boxes (torch.Tensor): rbboxes. format: centers, dims,
+ angles(clockwise when positive) with the shape of [N, 5].
+ query_boxes (torch.FloatTensor, shape=(K, 5)):
+ rbboxes to compute iou with boxes.
+ device_id (int, optional): Defaults to 0. Device to use.
+ criterion (int, optional): Indicate different type of iou.
+ -1 indicate `area_inter / (area1 + area2 - area_inter)`,
+ 0 indicate `area_inter / area1`,
+ 1 indicate `area_inter / area2`.
+
+ Returns:
+ np.ndarray: IoU results.
+ """
+ boxes = boxes.astype(np.float32)
+ query_boxes = query_boxes.astype(np.float32)
+ N = boxes.shape[0]
+ K = query_boxes.shape[0]
+ iou = np.zeros((N, K), dtype=np.float32)
+ if N == 0 or K == 0:
+ return iou
+ threadsPerBlock = 8 * 8
+ cuda.select_device(device_id)
+ blockspergrid = (div_up(N, threadsPerBlock), div_up(K, threadsPerBlock))
+
+ stream = cuda.stream()
+ with stream.auto_synchronize():
+ boxes_dev = cuda.to_device(boxes.reshape([-1]), stream)
+ query_boxes_dev = cuda.to_device(query_boxes.reshape([-1]), stream)
+ iou_dev = cuda.to_device(iou.reshape([-1]), stream)
+ rotate_iou_kernel_eval[blockspergrid, threadsPerBlock,
+ stream](N, K, boxes_dev, query_boxes_dev,
+ iou_dev, criterion)
+ iou_dev.copy_to_host(iou.reshape([-1]), stream=stream)
+ return iou.astype(boxes.dtype)
diff --git a/mmdet3d/core/evaluation/lyft_eval.py b/mmdet3d/core/evaluation/lyft_eval.py
new file mode 100644
index 0000000..47c5cd6
--- /dev/null
+++ b/mmdet3d/core/evaluation/lyft_eval.py
@@ -0,0 +1,285 @@
+# Copyright (c) OpenMMLab. All rights reserved.
+from os import path as osp
+
+import mmcv
+import numpy as np
+from lyft_dataset_sdk.eval.detection.mAP_evaluation import (Box3D, get_ap,
+ get_class_names,
+ get_ious,
+ group_by_key,
+ wrap_in_box)
+from mmcv.utils import print_log
+from terminaltables import AsciiTable
+
+
+def load_lyft_gts(lyft, data_root, eval_split, logger=None):
+ """Loads ground truth boxes from database.
+
+ Args:
+ lyft (:obj:`LyftDataset`): Lyft class in the sdk.
+ data_root (str): Root of data for reading splits.
+ eval_split (str): Name of the split for evaluation.
+ logger (logging.Logger | str, optional): Logger used for printing
+ related information during evaluation. Default: None.
+
+ Returns:
+ list[dict]: List of annotation dictionaries.
+ """
+ split_scenes = mmcv.list_from_file(
+ osp.join(data_root, f'{eval_split}.txt'))
+
+ # Read out all sample_tokens in DB.
+ sample_tokens_all = [s['token'] for s in lyft.sample]
+ assert len(sample_tokens_all) > 0, 'Error: Database has no samples!'
+
+ if eval_split == 'test':
+ # Check that you aren't trying to cheat :)
+ assert len(lyft.sample_annotation) > 0, \
+ 'Error: You are trying to evaluate on the test set \
+ but you do not have the annotations!'
+
+ sample_tokens = []
+ for sample_token in sample_tokens_all:
+ scene_token = lyft.get('sample', sample_token)['scene_token']
+ scene_record = lyft.get('scene', scene_token)
+ if scene_record['name'] in split_scenes:
+ sample_tokens.append(sample_token)
+
+ all_annotations = []
+
+ print_log('Loading ground truth annotations...', logger=logger)
+ # Load annotations and filter predictions and annotations.
+ for sample_token in mmcv.track_iter_progress(sample_tokens):
+ sample = lyft.get('sample', sample_token)
+ sample_annotation_tokens = sample['anns']
+ for sample_annotation_token in sample_annotation_tokens:
+ # Get label name in detection task and filter unused labels.
+ sample_annotation = \
+ lyft.get('sample_annotation', sample_annotation_token)
+ detection_name = sample_annotation['category_name']
+ if detection_name is None:
+ continue
+ annotation = {
+ 'sample_token': sample_token,
+ 'translation': sample_annotation['translation'],
+ 'size': sample_annotation['size'],
+ 'rotation': sample_annotation['rotation'],
+ 'name': detection_name,
+ }
+ all_annotations.append(annotation)
+
+ return all_annotations
+
+
+def load_lyft_predictions(res_path):
+ """Load Lyft predictions from json file.
+
+ Args:
+ res_path (str): Path of result json file recording detections.
+
+ Returns:
+ list[dict]: List of prediction dictionaries.
+ """
+ predictions = mmcv.load(res_path)
+ predictions = predictions['results']
+ all_preds = []
+ for sample_token in predictions.keys():
+ all_preds.extend(predictions[sample_token])
+ return all_preds
+
+
+def lyft_eval(lyft, data_root, res_path, eval_set, output_dir, logger=None):
+ """Evaluation API for Lyft dataset.
+
+ Args:
+ lyft (:obj:`LyftDataset`): Lyft class in the sdk.
+ data_root (str): Root of data for reading splits.
+ res_path (str): Path of result json file recording detections.
+ eval_set (str): Name of the split for evaluation.
+ output_dir (str): Output directory for output json files.
+ logger (logging.Logger | str, optional): Logger used for printing
+ related information during evaluation. Default: None.
+
+ Returns:
+ dict[str, float]: The evaluation results.
+ """
+ # evaluate by lyft metrics
+ gts = load_lyft_gts(lyft, data_root, eval_set, logger)
+ predictions = load_lyft_predictions(res_path)
+
+ class_names = get_class_names(gts)
+ print('Calculating mAP@0.5:0.95...')
+
+ iou_thresholds = [0.5, 0.55, 0.6, 0.65, 0.7, 0.75, 0.8, 0.85, 0.9, 0.95]
+ metrics = {}
+ average_precisions = \
+ get_classwise_aps(gts, predictions, class_names, iou_thresholds)
+ APs_data = [['IOU', 0.5, 0.55, 0.6, 0.65, 0.7, 0.75, 0.8, 0.85, 0.9, 0.95]]
+
+ mAPs = np.mean(average_precisions, axis=0)
+ mAPs_cate = np.mean(average_precisions, axis=1)
+ final_mAP = np.mean(mAPs)
+
+ metrics['average_precisions'] = average_precisions.tolist()
+ metrics['mAPs'] = mAPs.tolist()
+ metrics['Final mAP'] = float(final_mAP)
+ metrics['class_names'] = class_names
+ metrics['mAPs_cate'] = mAPs_cate.tolist()
+
+ APs_data = [['class', 'mAP@0.5:0.95']]
+ for i in range(len(class_names)):
+ row = [class_names[i], round(mAPs_cate[i], 3)]
+ APs_data.append(row)
+ APs_data.append(['Overall', round(final_mAP, 3)])
+ APs_table = AsciiTable(APs_data, title='mAPs@0.5:0.95')
+ APs_table.inner_footing_row_border = True
+ print_log(APs_table.table, logger=logger)
+
+ res_path = osp.join(output_dir, 'lyft_metrics.json')
+ mmcv.dump(metrics, res_path)
+ return metrics
+
+
+def get_classwise_aps(gt, predictions, class_names, iou_thresholds):
+ """Returns an array with an average precision per class.
+
+ Note: Ground truth and predictions should have the following format.
+
+ .. code-block::
+
+ gt = [{
+ 'sample_token': '0f0e3ce89d2324d8b45aa55a7b4f8207
+ fbb039a550991a5149214f98cec136ac',
+ 'translation': [974.2811881299899, 1714.6815014457964,
+ -23.689857123368846],
+ 'size': [1.796, 4.488, 1.664],
+ 'rotation': [0.14882026466054782, 0, 0, 0.9888642620837121],
+ 'name': 'car'
+ }]
+
+ predictions = [{
+ 'sample_token': '0f0e3ce89d2324d8b45aa55a7b4f8207
+ fbb039a550991a5149214f98cec136ac',
+ 'translation': [971.8343488872263, 1713.6816097857359,
+ -25.82534357061308],
+ 'size': [2.519726579986132, 7.810161372666739, 3.483438286096803],
+ 'rotation': [0.10913582721095375, 0.04099572636992043,
+ 0.01927712319721745, 1.029328402625659],
+ 'name': 'car',
+ 'score': 0.3077029437237213
+ }]
+
+ Args:
+ gt (list[dict]): list of dictionaries in the format described below.
+ predictions (list[dict]): list of dictionaries in the format
+ described below.
+ class_names (list[str]): list of the class names.
+ iou_thresholds (list[float]): IOU thresholds used to calculate
+ TP / FN
+
+ Returns:
+ np.ndarray: an array with an average precision per class.
+ """
+ assert all([0 <= iou_th <= 1 for iou_th in iou_thresholds])
+
+ gt_by_class_name = group_by_key(gt, 'name')
+ pred_by_class_name = group_by_key(predictions, 'name')
+
+ average_precisions = np.zeros((len(class_names), len(iou_thresholds)))
+
+ for class_id, class_name in enumerate(class_names):
+ if class_name in pred_by_class_name:
+ recalls, precisions, average_precision = get_single_class_aps(
+ gt_by_class_name[class_name], pred_by_class_name[class_name],
+ iou_thresholds)
+ average_precisions[class_id, :] = average_precision
+
+ return average_precisions
+
+
+def get_single_class_aps(gt, predictions, iou_thresholds):
+ """Compute recall and precision for all iou thresholds. Adapted from
+ LyftDatasetDevkit.
+
+ Args:
+ gt (list[dict]): list of dictionaries in the format described above.
+ predictions (list[dict]): list of dictionaries in the format
+ described below.
+ iou_thresholds (list[float]): IOU thresholds used to calculate
+ TP / FN
+
+ Returns:
+ tuple[np.ndarray]: Returns (recalls, precisions, average precisions)
+ for each class.
+ """
+ num_gts = len(gt)
+ image_gts = group_by_key(gt, 'sample_token')
+ image_gts = wrap_in_box(image_gts)
+
+ sample_gt_checked = {
+ sample_token: np.zeros((len(boxes), len(iou_thresholds)))
+ for sample_token, boxes in image_gts.items()
+ }
+
+ predictions = sorted(predictions, key=lambda x: x['score'], reverse=True)
+
+ # go down dets and mark TPs and FPs
+ num_predictions = len(predictions)
+ tps = np.zeros((num_predictions, len(iou_thresholds)))
+ fps = np.zeros((num_predictions, len(iou_thresholds)))
+
+ for prediction_index, prediction in enumerate(predictions):
+ predicted_box = Box3D(**prediction)
+
+ sample_token = prediction['sample_token']
+
+ max_overlap = -np.inf
+ jmax = -1
+
+ if sample_token in image_gts:
+ gt_boxes = image_gts[sample_token]
+ # gt_boxes per sample
+ gt_checked = sample_gt_checked[sample_token]
+ # gt flags per sample
+ else:
+ gt_boxes = []
+ gt_checked = None
+
+ if len(gt_boxes) > 0:
+ overlaps = get_ious(gt_boxes, predicted_box)
+
+ max_overlap = np.max(overlaps)
+
+ jmax = np.argmax(overlaps)
+
+ for i, iou_threshold in enumerate(iou_thresholds):
+ if max_overlap > iou_threshold:
+ if gt_checked[jmax, i] == 0:
+ tps[prediction_index, i] = 1.0
+ gt_checked[jmax, i] = 1
+ else:
+ fps[prediction_index, i] = 1.0
+ else:
+ fps[prediction_index, i] = 1.0
+
+ # compute precision recall
+ fps = np.cumsum(fps, axis=0)
+ tps = np.cumsum(tps, axis=0)
+
+ recalls = tps / float(num_gts)
+ # avoid divide by zero in case the first detection
+ # matches a difficult ground truth
+ precisions = tps / np.maximum(tps + fps, np.finfo(np.float64).eps)
+
+ aps = []
+ for i in range(len(iou_thresholds)):
+ recall = recalls[:, i]
+ precision = precisions[:, i]
+ assert np.all(0 <= recall) & np.all(recall <= 1)
+ assert np.all(0 <= precision) & np.all(precision <= 1)
+ ap = get_ap(recall, precision)
+ aps.append(ap)
+
+ aps = np.array(aps)
+
+ return recalls, precisions, aps
diff --git a/mmdet3d/core/evaluation/scannet_utils/__init__.py b/mmdet3d/core/evaluation/scannet_utils/__init__.py
new file mode 100644
index 0000000..c98ea83
--- /dev/null
+++ b/mmdet3d/core/evaluation/scannet_utils/__init__.py
@@ -0,0 +1,4 @@
+# Copyright (c) OpenMMLab. All rights reserved.
+from .evaluate_semantic_instance import evaluate_matches, scannet_eval
+
+__all__ = ['scannet_eval', 'evaluate_matches']
diff --git a/mmdet3d/core/evaluation/scannet_utils/evaluate_semantic_instance.py b/mmdet3d/core/evaluation/scannet_utils/evaluate_semantic_instance.py
new file mode 100644
index 0000000..e4b9439
--- /dev/null
+++ b/mmdet3d/core/evaluation/scannet_utils/evaluate_semantic_instance.py
@@ -0,0 +1,347 @@
+# Copyright (c) OpenMMLab. All rights reserved.
+# adapted from https://github.com/ScanNet/ScanNet/blob/master/BenchmarkScripts/3d_evaluation/evaluate_semantic_instance.py # noqa
+from copy import deepcopy
+
+import numpy as np
+
+from . import util_3d
+
+
+def evaluate_matches(matches, class_labels, options):
+ """Evaluate instance segmentation from matched gt and predicted instances
+ for all scenes.
+
+ Args:
+ matches (dict): Contains gt2pred and pred2gt infos for every scene.
+ class_labels (tuple[str]): Class names.
+ options (dict): ScanNet evaluator options. See get_options.
+
+ Returns:
+ np.array: Average precision scores for all thresholds and categories.
+ """
+ overlaps = options['overlaps']
+ min_region_sizes = [options['min_region_sizes'][0]]
+ dist_threshes = [options['distance_threshes'][0]]
+ dist_confs = [options['distance_confs'][0]]
+
+ # results: class x overlap
+ ap = np.zeros((len(dist_threshes), len(class_labels), len(overlaps)),
+ np.float)
+ for di, (min_region_size, distance_thresh, distance_conf) in enumerate(
+ zip(min_region_sizes, dist_threshes, dist_confs)):
+ for oi, overlap_th in enumerate(overlaps):
+ pred_visited = {}
+ for m in matches:
+ for label_name in class_labels:
+ for p in matches[m]['pred'][label_name]:
+ if 'filename' in p:
+ pred_visited[p['filename']] = False
+ for li, label_name in enumerate(class_labels):
+ y_true = np.empty(0)
+ y_score = np.empty(0)
+ hard_false_negatives = 0
+ has_gt = False
+ has_pred = False
+ for m in matches:
+ pred_instances = matches[m]['pred'][label_name]
+ gt_instances = matches[m]['gt'][label_name]
+ # filter groups in ground truth
+ gt_instances = [
+ gt for gt in gt_instances
+ if gt['instance_id'] >= 1000 and gt['vert_count'] >=
+ min_region_size and gt['med_dist'] <= distance_thresh
+ and gt['dist_conf'] >= distance_conf
+ ]
+ if gt_instances:
+ has_gt = True
+ if pred_instances:
+ has_pred = True
+
+ cur_true = np.ones(len(gt_instances))
+ cur_score = np.ones(len(gt_instances)) * (-float('inf'))
+ cur_match = np.zeros(len(gt_instances), dtype=np.bool)
+ # collect matches
+ for (gti, gt) in enumerate(gt_instances):
+ found_match = False
+ for pred in gt['matched_pred']:
+ # greedy assignments
+ if pred_visited[pred['filename']]:
+ continue
+ overlap = float(pred['intersection']) / (
+ gt['vert_count'] + pred['vert_count'] -
+ pred['intersection'])
+ if overlap > overlap_th:
+ confidence = pred['confidence']
+ # if already have a prediction for this gt,
+ # the prediction with the lower score is automatically a false positive # noqa
+ if cur_match[gti]:
+ max_score = max(cur_score[gti], confidence)
+ min_score = min(cur_score[gti], confidence)
+ cur_score[gti] = max_score
+ # append false positive
+ cur_true = np.append(cur_true, 0)
+ cur_score = np.append(cur_score, min_score)
+ cur_match = np.append(cur_match, True)
+ # otherwise set score
+ else:
+ found_match = True
+ cur_match[gti] = True
+ cur_score[gti] = confidence
+ pred_visited[pred['filename']] = True
+ if not found_match:
+ hard_false_negatives += 1
+ # remove non-matched ground truth instances
+ cur_true = cur_true[cur_match]
+ cur_score = cur_score[cur_match]
+
+ # collect non-matched predictions as false positive
+ for pred in pred_instances:
+ found_gt = False
+ for gt in pred['matched_gt']:
+ overlap = float(gt['intersection']) / (
+ gt['vert_count'] + pred['vert_count'] -
+ gt['intersection'])
+ if overlap > overlap_th:
+ found_gt = True
+ break
+ if not found_gt:
+ num_ignore = pred['void_intersection']
+ for gt in pred['matched_gt']:
+ # group?
+ if gt['instance_id'] < 1000:
+ num_ignore += gt['intersection']
+ # small ground truth instances
+ if gt['vert_count'] < min_region_size or gt[
+ 'med_dist'] > distance_thresh or gt[
+ 'dist_conf'] < distance_conf:
+ num_ignore += gt['intersection']
+ proportion_ignore = float(
+ num_ignore) / pred['vert_count']
+ # if not ignored append false positive
+ if proportion_ignore <= overlap_th:
+ cur_true = np.append(cur_true, 0)
+ confidence = pred['confidence']
+ cur_score = np.append(cur_score, confidence)
+
+ # append to overall results
+ y_true = np.append(y_true, cur_true)
+ y_score = np.append(y_score, cur_score)
+
+ # compute average precision
+ if has_gt and has_pred:
+ # compute precision recall curve first
+
+ # sorting and cumsum
+ score_arg_sort = np.argsort(y_score)
+ y_score_sorted = y_score[score_arg_sort]
+ y_true_sorted = y_true[score_arg_sort]
+ y_true_sorted_cumsum = np.cumsum(y_true_sorted)
+
+ # unique thresholds
+ (thresholds, unique_indices) = np.unique(
+ y_score_sorted, return_index=True)
+ num_prec_recall = len(unique_indices) + 1
+
+ # prepare precision recall
+ num_examples = len(y_score_sorted)
+ # follow https://github.com/ScanNet/ScanNet/pull/26 ? # noqa
+ num_true_examples = y_true_sorted_cumsum[-1] if len(
+ y_true_sorted_cumsum) > 0 else 0
+ precision = np.zeros(num_prec_recall)
+ recall = np.zeros(num_prec_recall)
+
+ # deal with the first point
+ y_true_sorted_cumsum = np.append(y_true_sorted_cumsum, 0)
+ # deal with remaining
+ for idx_res, idx_scores in enumerate(unique_indices):
+ cumsum = y_true_sorted_cumsum[idx_scores - 1]
+ tp = num_true_examples - cumsum
+ fp = num_examples - idx_scores - tp
+ fn = cumsum + hard_false_negatives
+ p = float(tp) / (tp + fp)
+ r = float(tp) / (tp + fn)
+ precision[idx_res] = p
+ recall[idx_res] = r
+
+ # first point in curve is artificial
+ precision[-1] = 1.
+ recall[-1] = 0.
+
+ # compute average of precision-recall curve
+ recall_for_conv = np.copy(recall)
+ recall_for_conv = np.append(recall_for_conv[0],
+ recall_for_conv)
+ recall_for_conv = np.append(recall_for_conv, 0.)
+
+ stepWidths = np.convolve(recall_for_conv, [-0.5, 0, 0.5],
+ 'valid')
+ # integrate is now simply a dot product
+ ap_current = np.dot(precision, stepWidths)
+
+ elif has_gt:
+ ap_current = 0.0
+ else:
+ ap_current = float('nan')
+ ap[di, li, oi] = ap_current
+ return ap
+
+
+def compute_averages(aps, options, class_labels):
+ """Averages AP scores for all categories.
+
+ Args:
+ aps (np.array): AP scores for all thresholds and categories.
+ options (dict): ScanNet evaluator options. See get_options.
+ class_labels (tuple[str]): Class names.
+
+ Returns:
+ dict: Overall and per-category AP scores.
+ """
+ d_inf = 0
+ o50 = np.where(np.isclose(options['overlaps'], 0.5))
+ o25 = np.where(np.isclose(options['overlaps'], 0.25))
+ o_all_but25 = np.where(
+ np.logical_not(np.isclose(options['overlaps'], 0.25)))
+ avg_dict = {}
+ avg_dict['all_ap'] = np.nanmean(aps[d_inf, :, o_all_but25])
+ avg_dict['all_ap_50%'] = np.nanmean(aps[d_inf, :, o50])
+ avg_dict['all_ap_25%'] = np.nanmean(aps[d_inf, :, o25])
+ avg_dict['classes'] = {}
+ for (li, label_name) in enumerate(class_labels):
+ avg_dict['classes'][label_name] = {}
+ avg_dict['classes'][label_name]['ap'] = np.average(aps[d_inf, li,
+ o_all_but25])
+ avg_dict['classes'][label_name]['ap50%'] = np.average(aps[d_inf, li,
+ o50])
+ avg_dict['classes'][label_name]['ap25%'] = np.average(aps[d_inf, li,
+ o25])
+ return avg_dict
+
+
+def assign_instances_for_scan(pred_info, gt_ids, options, valid_class_ids,
+ class_labels, id_to_label):
+ """Assign gt and predicted instances for a single scene.
+
+ Args:
+ pred_info (dict): Predicted masks, labels and scores.
+ gt_ids (np.array): Ground truth instance masks.
+ options (dict): ScanNet evaluator options. See get_options.
+ valid_class_ids (tuple[int]): Ids of valid categories.
+ class_labels (tuple[str]): Class names.
+ id_to_label (dict[int, str]): Mapping of valid class id to class label.
+
+ Returns:
+ dict: Per class assigned gt to predicted instances.
+ dict: Per class assigned predicted to gt instances.
+ """
+ # get gt instances
+ gt_instances = util_3d.get_instances(gt_ids, valid_class_ids, class_labels,
+ id_to_label)
+ # associate
+ gt2pred = deepcopy(gt_instances)
+ for label in gt2pred:
+ for gt in gt2pred[label]:
+ gt['matched_pred'] = []
+ pred2gt = {}
+ for label in class_labels:
+ pred2gt[label] = []
+ num_pred_instances = 0
+ # mask of void labels in the ground truth
+ bool_void = np.logical_not(np.in1d(gt_ids // 1000, valid_class_ids))
+ # go through all prediction masks
+ for pred_mask_file in pred_info:
+ label_id = int(pred_info[pred_mask_file]['label_id'])
+ conf = pred_info[pred_mask_file]['conf']
+ if not label_id in id_to_label: # noqa E713
+ continue
+ label_name = id_to_label[label_id]
+ # read the mask
+ pred_mask = pred_info[pred_mask_file]['mask']
+ if len(pred_mask) != len(gt_ids):
+ raise ValueError('len(pred_mask) != len(gt_ids)')
+ # convert to binary
+ pred_mask = np.not_equal(pred_mask, 0)
+ num = np.count_nonzero(pred_mask)
+ if num < options['min_region_sizes'][0]:
+ continue # skip if empty
+
+ pred_instance = {}
+ pred_instance['filename'] = pred_mask_file
+ pred_instance['pred_id'] = num_pred_instances
+ pred_instance['label_id'] = label_id
+ pred_instance['vert_count'] = num
+ pred_instance['confidence'] = conf
+ pred_instance['void_intersection'] = np.count_nonzero(
+ np.logical_and(bool_void, pred_mask))
+
+ # matched gt instances
+ matched_gt = []
+ # go through all gt instances with matching label
+ for (gt_num, gt_inst) in enumerate(gt2pred[label_name]):
+ intersection = np.count_nonzero(
+ np.logical_and(gt_ids == gt_inst['instance_id'], pred_mask))
+ if intersection > 0:
+ gt_copy = gt_inst.copy()
+ pred_copy = pred_instance.copy()
+ gt_copy['intersection'] = intersection
+ pred_copy['intersection'] = intersection
+ matched_gt.append(gt_copy)
+ gt2pred[label_name][gt_num]['matched_pred'].append(pred_copy)
+ pred_instance['matched_gt'] = matched_gt
+ num_pred_instances += 1
+ pred2gt[label_name].append(pred_instance)
+
+ return gt2pred, pred2gt
+
+
+def scannet_eval(preds, gts, options, valid_class_ids, class_labels,
+ id_to_label):
+ """Evaluate instance segmentation in ScanNet protocol.
+
+ Args:
+ preds (list[dict]): Per scene predictions of mask, label and
+ confidence.
+ gts (list[np.array]): Per scene ground truth instance masks.
+ options (dict): ScanNet evaluator options. See get_options.
+ valid_class_ids (tuple[int]): Ids of valid categories.
+ class_labels (tuple[str]): Class names.
+ id_to_label (dict[int, str]): Mapping of valid class id to class label.
+
+ Returns:
+ dict: Overall and per-category AP scores.
+ """
+ options = get_options(options)
+ matches = {}
+ for i, (pred, gt) in enumerate(zip(preds, gts)):
+ matches_key = i
+ # assign gt to predictions
+ gt2pred, pred2gt = assign_instances_for_scan(pred, gt, options,
+ valid_class_ids,
+ class_labels, id_to_label)
+ matches[matches_key] = {}
+ matches[matches_key]['gt'] = gt2pred
+ matches[matches_key]['pred'] = pred2gt
+
+ ap_scores = evaluate_matches(matches, class_labels, options)
+ avgs = compute_averages(ap_scores, options, class_labels)
+ return avgs
+
+
+def get_options(options=None):
+ """Set ScanNet evaluator options.
+
+ Args:
+ options (dict, optional): Not default options. Default: None.
+
+ Returns:
+ dict: Updated options with all 4 keys.
+ """
+ assert options is None or isinstance(options, dict)
+ _options = dict(
+ overlaps=np.append(np.arange(0.5, 0.95, 0.05), 0.25),
+ min_region_sizes=np.array([100]),
+ distance_threshes=np.array([float('inf')]),
+ distance_confs=np.array([-float('inf')]))
+ if options is not None:
+ _options.update(options)
+ return _options
diff --git a/mmdet3d/core/evaluation/scannet_utils/util_3d.py b/mmdet3d/core/evaluation/scannet_utils/util_3d.py
new file mode 100644
index 0000000..527d341
--- /dev/null
+++ b/mmdet3d/core/evaluation/scannet_utils/util_3d.py
@@ -0,0 +1,84 @@
+# Copyright (c) OpenMMLab. All rights reserved.
+# adapted from https://github.com/ScanNet/ScanNet/blob/master/BenchmarkScripts/util_3d.py # noqa
+import json
+
+import numpy as np
+
+
+class Instance:
+ """Single instance for ScanNet evaluator.
+
+ Args:
+ mesh_vert_instances (np.array): Instance ids for each point.
+ instance_id: Id of single instance.
+ """
+ instance_id = 0
+ label_id = 0
+ vert_count = 0
+ med_dist = -1
+ dist_conf = 0.0
+
+ def __init__(self, mesh_vert_instances, instance_id):
+ if instance_id == -1:
+ return
+ self.instance_id = int(instance_id)
+ self.label_id = int(self.get_label_id(instance_id))
+ self.vert_count = int(
+ self.get_instance_verts(mesh_vert_instances, instance_id))
+
+ @staticmethod
+ def get_label_id(instance_id):
+ return int(instance_id // 1000)
+
+ @staticmethod
+ def get_instance_verts(mesh_vert_instances, instance_id):
+ return (mesh_vert_instances == instance_id).sum()
+
+ def to_json(self):
+ return json.dumps(
+ self, default=lambda o: o.__dict__, sort_keys=True, indent=4)
+
+ def to_dict(self):
+ dict = {}
+ dict['instance_id'] = self.instance_id
+ dict['label_id'] = self.label_id
+ dict['vert_count'] = self.vert_count
+ dict['med_dist'] = self.med_dist
+ dict['dist_conf'] = self.dist_conf
+ return dict
+
+ def from_json(self, data):
+ self.instance_id = int(data['instance_id'])
+ self.label_id = int(data['label_id'])
+ self.vert_count = int(data['vert_count'])
+ if 'med_dist' in data:
+ self.med_dist = float(data['med_dist'])
+ self.dist_conf = float(data['dist_conf'])
+
+ def __str__(self):
+ return '(' + str(self.instance_id) + ')'
+
+
+def get_instances(ids, class_ids, class_labels, id2label):
+ """Transform gt instance mask to Instance objects.
+
+ Args:
+ ids (np.array): Instance ids for each point.
+ class_ids: (tuple[int]): Ids of valid categories.
+ class_labels (tuple[str]): Class names.
+ id2label: (dict[int, str]): Mapping of valid class id to class label.
+
+ Returns:
+ dict [str, list]: Instance objects grouped by class label.
+ """
+ instances = {}
+ for label in class_labels:
+ instances[label] = []
+ instance_ids = np.unique(ids)
+ for id in instance_ids:
+ if id == 0:
+ continue
+ inst = Instance(ids, id)
+ if inst.label_id in class_ids:
+ instances[id2label[inst.label_id]].append(inst.to_dict())
+ return instances
diff --git a/mmdet3d/core/evaluation/seg_eval.py b/mmdet3d/core/evaluation/seg_eval.py
new file mode 100644
index 0000000..4a3166d
--- /dev/null
+++ b/mmdet3d/core/evaluation/seg_eval.py
@@ -0,0 +1,131 @@
+# Copyright (c) OpenMMLab. All rights reserved.
+import numpy as np
+from mmcv.utils import print_log
+from terminaltables import AsciiTable
+
+
+def fast_hist(preds, labels, num_classes):
+ """Compute the confusion matrix for every batch.
+
+ Args:
+ preds (np.ndarray): Prediction labels of points with shape of
+ (num_points, ).
+ labels (np.ndarray): Ground truth labels of points with shape of
+ (num_points, ).
+ num_classes (int): number of classes
+
+ Returns:
+ np.ndarray: Calculated confusion matrix.
+ """
+
+ k = (labels >= 0) & (labels < num_classes)
+ bin_count = np.bincount(
+ num_classes * labels[k].astype(int) + preds[k],
+ minlength=num_classes**2)
+ return bin_count[:num_classes**2].reshape(num_classes, num_classes)
+
+
+def per_class_iou(hist):
+ """Compute the per class iou.
+
+ Args:
+ hist(np.ndarray): Overall confusion martix
+ (num_classes, num_classes ).
+
+ Returns:
+ np.ndarray: Calculated per class iou
+ """
+
+ return np.diag(hist) / (hist.sum(1) + hist.sum(0) - np.diag(hist))
+
+
+def get_acc(hist):
+ """Compute the overall accuracy.
+
+ Args:
+ hist(np.ndarray): Overall confusion martix
+ (num_classes, num_classes ).
+
+ Returns:
+ float: Calculated overall acc
+ """
+
+ return np.diag(hist).sum() / hist.sum()
+
+
+def get_acc_cls(hist):
+ """Compute the class average accuracy.
+
+ Args:
+ hist(np.ndarray): Overall confusion martix
+ (num_classes, num_classes ).
+
+ Returns:
+ float: Calculated class average acc
+ """
+
+ return np.nanmean(np.diag(hist) / hist.sum(axis=1))
+
+
+def seg_eval(gt_labels, seg_preds, label2cat, ignore_index, logger=None):
+ """Semantic Segmentation Evaluation.
+
+ Evaluate the result of the Semantic Segmentation.
+
+ Args:
+ gt_labels (list[torch.Tensor]): Ground truth labels.
+ seg_preds (list[torch.Tensor]): Predictions.
+ label2cat (dict): Map from label to category name.
+ ignore_index (int): Index that will be ignored in evaluation.
+ logger (logging.Logger | str, optional): The way to print the mAP
+ summary. See `mmdet.utils.print_log()` for details. Default: None.
+
+ Returns:
+ dict[str, float]: Dict of results.
+ """
+ assert len(seg_preds) == len(gt_labels)
+ num_classes = len(label2cat)
+
+ hist_list = []
+ for i in range(len(gt_labels)):
+ gt_seg = gt_labels[i].clone().numpy().astype(np.int)
+ pred_seg = seg_preds[i].clone().numpy().astype(np.int)
+
+ # filter out ignored points
+ pred_seg[gt_seg == ignore_index] = -1
+ gt_seg[gt_seg == ignore_index] = -1
+
+ # calculate one instance result
+ hist_list.append(fast_hist(pred_seg, gt_seg, num_classes))
+
+ iou = per_class_iou(sum(hist_list))
+ miou = np.nanmean(iou)
+ acc = get_acc(sum(hist_list))
+ acc_cls = get_acc_cls(sum(hist_list))
+
+ header = ['classes']
+ for i in range(len(label2cat)):
+ header.append(label2cat[i])
+ header.extend(['miou', 'acc', 'acc_cls'])
+
+ ret_dict = dict()
+ table_columns = [['results']]
+ for i in range(len(label2cat)):
+ ret_dict[label2cat[i]] = float(iou[i])
+ table_columns.append([f'{iou[i]:.4f}'])
+ ret_dict['miou'] = float(miou)
+ ret_dict['acc'] = float(acc)
+ ret_dict['acc_cls'] = float(acc_cls)
+
+ table_columns.append([f'{miou:.4f}'])
+ table_columns.append([f'{acc:.4f}'])
+ table_columns.append([f'{acc_cls:.4f}'])
+
+ table_data = [header]
+ table_rows = list(zip(*table_columns))
+ table_data += table_rows
+ table = AsciiTable(table_data)
+ table.inner_footing_row_border = True
+ print_log('\n' + table.table, logger=logger)
+
+ return ret_dict
diff --git a/mmdet3d/core/evaluation/waymo_utils/__init__.py b/mmdet3d/core/evaluation/waymo_utils/__init__.py
new file mode 100644
index 0000000..72d3a9b
--- /dev/null
+++ b/mmdet3d/core/evaluation/waymo_utils/__init__.py
@@ -0,0 +1,4 @@
+# Copyright (c) OpenMMLab. All rights reserved.
+from .prediction_kitti_to_waymo import KITTI2Waymo
+
+__all__ = ['KITTI2Waymo']
diff --git a/mmdet3d/core/evaluation/waymo_utils/prediction_kitti_to_waymo.py b/mmdet3d/core/evaluation/waymo_utils/prediction_kitti_to_waymo.py
new file mode 100644
index 0000000..205c24c
--- /dev/null
+++ b/mmdet3d/core/evaluation/waymo_utils/prediction_kitti_to_waymo.py
@@ -0,0 +1,263 @@
+# Copyright (c) OpenMMLab. All rights reserved.
+r"""Adapted from `Waymo to KITTI converter
+ `_.
+"""
+
+try:
+ from waymo_open_dataset import dataset_pb2 as open_dataset
+except ImportError:
+ raise ImportError(
+ 'Please run "pip install waymo-open-dataset-tf-2-1-0==1.2.0" '
+ 'to install the official devkit first.')
+
+from glob import glob
+from os.path import join
+
+import mmcv
+import numpy as np
+import tensorflow as tf
+from waymo_open_dataset import label_pb2
+from waymo_open_dataset.protos import metrics_pb2
+
+
+class KITTI2Waymo(object):
+ """KITTI predictions to Waymo converter.
+
+ This class serves as the converter to change predictions from KITTI to
+ Waymo format.
+
+ Args:
+ kitti_result_files (list[dict]): Predictions in KITTI format.
+ waymo_tfrecords_dir (str): Directory to load waymo raw data.
+ waymo_results_save_dir (str): Directory to save converted predictions
+ in waymo format (.bin files).
+ waymo_results_final_path (str): Path to save combined
+ predictions in waymo format (.bin file), like 'a/b/c.bin'.
+ prefix (str): Prefix of filename. In general, 0 for training, 1 for
+ validation and 2 for testing.
+ workers (str): Number of parallel processes.
+ """
+
+ def __init__(self,
+ kitti_result_files,
+ waymo_tfrecords_dir,
+ waymo_results_save_dir,
+ waymo_results_final_path,
+ prefix,
+ workers=64):
+
+ self.kitti_result_files = kitti_result_files
+ self.waymo_tfrecords_dir = waymo_tfrecords_dir
+ self.waymo_results_save_dir = waymo_results_save_dir
+ self.waymo_results_final_path = waymo_results_final_path
+ self.prefix = prefix
+ self.workers = int(workers)
+ self.name2idx = {}
+ for idx, result in enumerate(kitti_result_files):
+ if len(result['sample_idx']) > 0:
+ self.name2idx[str(result['sample_idx'][0])] = idx
+
+ # turn on eager execution for older tensorflow versions
+ if int(tf.__version__.split('.')[0]) < 2:
+ tf.enable_eager_execution()
+
+ self.k2w_cls_map = {
+ 'Car': label_pb2.Label.TYPE_VEHICLE,
+ 'Pedestrian': label_pb2.Label.TYPE_PEDESTRIAN,
+ 'Sign': label_pb2.Label.TYPE_SIGN,
+ 'Cyclist': label_pb2.Label.TYPE_CYCLIST,
+ }
+
+ self.T_ref_to_front_cam = np.array([[0.0, 0.0, 1.0, 0.0],
+ [-1.0, 0.0, 0.0, 0.0],
+ [0.0, -1.0, 0.0, 0.0],
+ [0.0, 0.0, 0.0, 1.0]])
+
+ self.get_file_names()
+ self.create_folder()
+
+ def get_file_names(self):
+ """Get file names of waymo raw data."""
+ self.waymo_tfrecord_pathnames = sorted(
+ glob(join(self.waymo_tfrecords_dir, '*.tfrecord')))
+ print(len(self.waymo_tfrecord_pathnames), 'tfrecords found.')
+
+ def create_folder(self):
+ """Create folder for data conversion."""
+ mmcv.mkdir_or_exist(self.waymo_results_save_dir)
+
+ def parse_objects(self, kitti_result, T_k2w, context_name,
+ frame_timestamp_micros):
+ """Parse one prediction with several instances in kitti format and
+ convert them to `Object` proto.
+
+ Args:
+ kitti_result (dict): Predictions in kitti format.
+
+ - name (np.ndarray): Class labels of predictions.
+ - dimensions (np.ndarray): Height, width, length of boxes.
+ - location (np.ndarray): Bottom center of boxes (x, y, z).
+ - rotation_y (np.ndarray): Orientation of boxes.
+ - score (np.ndarray): Scores of predictions.
+ T_k2w (np.ndarray): Transformation matrix from kitti to waymo.
+ context_name (str): Context name of the frame.
+ frame_timestamp_micros (int): Frame timestamp.
+
+ Returns:
+ :obj:`Object`: Predictions in waymo dataset Object proto.
+ """
+
+ def parse_one_object(instance_idx):
+ """Parse one instance in kitti format and convert them to `Object`
+ proto.
+
+ Args:
+ instance_idx (int): Index of the instance to be converted.
+
+ Returns:
+ :obj:`Object`: Predicted instance in waymo dataset
+ Object proto.
+ """
+ cls = kitti_result['name'][instance_idx]
+ length = round(kitti_result['dimensions'][instance_idx, 0], 4)
+ height = round(kitti_result['dimensions'][instance_idx, 1], 4)
+ width = round(kitti_result['dimensions'][instance_idx, 2], 4)
+ x = round(kitti_result['location'][instance_idx, 0], 4)
+ y = round(kitti_result['location'][instance_idx, 1], 4)
+ z = round(kitti_result['location'][instance_idx, 2], 4)
+ rotation_y = round(kitti_result['rotation_y'][instance_idx], 4)
+ score = round(kitti_result['score'][instance_idx], 4)
+
+ # y: downwards; move box origin from bottom center (kitti) to
+ # true center (waymo)
+ y -= height / 2
+ # frame transformation: kitti -> waymo
+ x, y, z = self.transform(T_k2w, x, y, z)
+
+ # different conventions
+ heading = -(rotation_y + np.pi / 2)
+ while heading < -np.pi:
+ heading += 2 * np.pi
+ while heading > np.pi:
+ heading -= 2 * np.pi
+
+ box = label_pb2.Label.Box()
+ box.center_x = x
+ box.center_y = y
+ box.center_z = z
+ box.length = length
+ box.width = width
+ box.height = height
+ box.heading = heading
+
+ o = metrics_pb2.Object()
+ o.object.box.CopyFrom(box)
+ o.object.type = self.k2w_cls_map[cls]
+ o.score = score
+
+ o.context_name = context_name
+ o.frame_timestamp_micros = frame_timestamp_micros
+
+ return o
+
+ objects = metrics_pb2.Objects()
+
+ for instance_idx in range(len(kitti_result['name'])):
+ o = parse_one_object(instance_idx)
+ objects.objects.append(o)
+
+ return objects
+
+ def convert_one(self, file_idx):
+ """Convert action for single file.
+
+ Args:
+ file_idx (int): Index of the file to be converted.
+ """
+ file_pathname = self.waymo_tfrecord_pathnames[file_idx]
+ file_data = tf.data.TFRecordDataset(file_pathname, compression_type='')
+
+ for frame_num, frame_data in enumerate(file_data):
+ frame = open_dataset.Frame()
+ frame.ParseFromString(bytearray(frame_data.numpy()))
+
+ filename = f'{self.prefix}{file_idx:03d}{frame_num:03d}'
+
+ for camera in frame.context.camera_calibrations:
+ # FRONT = 1, see dataset.proto for details
+ if camera.name == 1:
+ T_front_cam_to_vehicle = np.array(
+ camera.extrinsic.transform).reshape(4, 4)
+
+ T_k2w = T_front_cam_to_vehicle @ self.T_ref_to_front_cam
+
+ context_name = frame.context.name
+ frame_timestamp_micros = frame.timestamp_micros
+
+ if filename in self.name2idx:
+ kitti_result = \
+ self.kitti_result_files[self.name2idx[filename]]
+ objects = self.parse_objects(kitti_result, T_k2w, context_name,
+ frame_timestamp_micros)
+ else:
+ print(filename, 'not found.')
+ objects = metrics_pb2.Objects()
+
+ with open(
+ join(self.waymo_results_save_dir, f'{filename}.bin'),
+ 'wb') as f:
+ f.write(objects.SerializeToString())
+
+ def convert(self):
+ """Convert action."""
+ print('Start converting ...')
+ mmcv.track_parallel_progress(self.convert_one, range(len(self)),
+ self.workers)
+ print('\nFinished ...')
+
+ # combine all files into one .bin
+ pathnames = sorted(glob(join(self.waymo_results_save_dir, '*.bin')))
+ combined = self.combine(pathnames)
+
+ with open(self.waymo_results_final_path, 'wb') as f:
+ f.write(combined.SerializeToString())
+
+ def __len__(self):
+ """Length of the filename list."""
+ return len(self.waymo_tfrecord_pathnames)
+
+ def transform(self, T, x, y, z):
+ """Transform the coordinates with matrix T.
+
+ Args:
+ T (np.ndarray): Transformation matrix.
+ x(float): Coordinate in x axis.
+ y(float): Coordinate in y axis.
+ z(float): Coordinate in z axis.
+
+ Returns:
+ list: Coordinates after transformation.
+ """
+ pt_bef = np.array([x, y, z, 1.0]).reshape(4, 1)
+ pt_aft = np.matmul(T, pt_bef)
+ return pt_aft[:3].flatten().tolist()
+
+ def combine(self, pathnames):
+ """Combine predictions in waymo format for each sample together.
+
+ Args:
+ pathnames (str): Paths to save predictions.
+
+ Returns:
+ :obj:`Objects`: Combined predictions in Objects proto.
+ """
+ combined = metrics_pb2.Objects()
+
+ for pathname in pathnames:
+ objects = metrics_pb2.Objects()
+ with open(pathname, 'rb') as f:
+ objects.ParseFromString(f.read())
+ for o in objects.objects:
+ combined.objects.append(o)
+
+ return combined
diff --git a/mmdet3d/core/points/__init__.py b/mmdet3d/core/points/__init__.py
new file mode 100644
index 0000000..73d2d83
--- /dev/null
+++ b/mmdet3d/core/points/__init__.py
@@ -0,0 +1,30 @@
+# Copyright (c) OpenMMLab. All rights reserved.
+from .base_points import BasePoints
+from .cam_points import CameraPoints
+from .depth_points import DepthPoints
+from .lidar_points import LiDARPoints
+
+__all__ = ['BasePoints', 'CameraPoints', 'DepthPoints', 'LiDARPoints']
+
+
+def get_points_type(points_type):
+ """Get the class of points according to coordinate type.
+
+ Args:
+ points_type (str): The type of points coordinate.
+ The valid value are "CAMERA", "LIDAR", or "DEPTH".
+
+ Returns:
+ class: Points type.
+ """
+ if points_type == 'CAMERA':
+ points_cls = CameraPoints
+ elif points_type == 'LIDAR':
+ points_cls = LiDARPoints
+ elif points_type == 'DEPTH':
+ points_cls = DepthPoints
+ else:
+ raise ValueError('Only "points_type" of "CAMERA", "LIDAR", or "DEPTH"'
+ f' are supported, got {points_type}')
+
+ return points_cls
diff --git a/mmdet3d/core/points/base_points.py b/mmdet3d/core/points/base_points.py
new file mode 100644
index 0000000..929fa21
--- /dev/null
+++ b/mmdet3d/core/points/base_points.py
@@ -0,0 +1,440 @@
+# Copyright (c) OpenMMLab. All rights reserved.
+import warnings
+from abc import abstractmethod
+
+import numpy as np
+import torch
+
+from ..bbox.structures.utils import rotation_3d_in_axis
+
+
+class BasePoints(object):
+ """Base class for Points.
+
+ Args:
+ tensor (torch.Tensor | np.ndarray | list): a N x points_dim matrix.
+ points_dim (int, optional): Number of the dimension of a point.
+ Each row is (x, y, z). Defaults to 3.
+ attribute_dims (dict, optional): Dictionary to indicate the
+ meaning of extra dimension. Defaults to None.
+
+ Attributes:
+ tensor (torch.Tensor): Float matrix of N x points_dim.
+ points_dim (int): Integer indicating the dimension of a point.
+ Each row is (x, y, z, ...).
+ attribute_dims (bool): Dictionary to indicate the meaning of extra
+ dimension. Defaults to None.
+ rotation_axis (int): Default rotation axis for points rotation.
+ """
+
+ def __init__(self, tensor, points_dim=3, attribute_dims=None):
+ if isinstance(tensor, torch.Tensor):
+ device = tensor.device
+ else:
+ device = torch.device('cpu')
+ tensor = torch.as_tensor(tensor, dtype=torch.float32, device=device)
+ if tensor.numel() == 0:
+ # Use reshape, so we don't end up creating a new tensor that
+ # does not depend on the inputs (and consequently confuses jit)
+ tensor = tensor.reshape((0, points_dim)).to(
+ dtype=torch.float32, device=device)
+ assert tensor.dim() == 2 and tensor.size(-1) == \
+ points_dim, tensor.size()
+
+ self.tensor = tensor
+ self.points_dim = points_dim
+ self.attribute_dims = attribute_dims
+ self.rotation_axis = 0
+
+ @property
+ def coord(self):
+ """torch.Tensor: Coordinates of each point in shape (N, 3)."""
+ return self.tensor[:, :3]
+
+ @coord.setter
+ def coord(self, tensor):
+ """Set the coordinates of each point."""
+ try:
+ tensor = tensor.reshape(self.shape[0], 3)
+ except (RuntimeError, ValueError): # for torch.Tensor and np.ndarray
+ raise ValueError(f'got unexpected shape {tensor.shape}')
+ if not isinstance(tensor, torch.Tensor):
+ tensor = self.tensor.new_tensor(tensor)
+ self.tensor[:, :3] = tensor
+
+ @property
+ def height(self):
+ """torch.Tensor:
+ A vector with height of each point in shape (N, 1), or None."""
+ if self.attribute_dims is not None and \
+ 'height' in self.attribute_dims.keys():
+ return self.tensor[:, self.attribute_dims['height']]
+ else:
+ return None
+
+ @height.setter
+ def height(self, tensor):
+ """Set the height of each point."""
+ try:
+ tensor = tensor.reshape(self.shape[0])
+ except (RuntimeError, ValueError): # for torch.Tensor and np.ndarray
+ raise ValueError(f'got unexpected shape {tensor.shape}')
+ if not isinstance(tensor, torch.Tensor):
+ tensor = self.tensor.new_tensor(tensor)
+ if self.attribute_dims is not None and \
+ 'height' in self.attribute_dims.keys():
+ self.tensor[:, self.attribute_dims['height']] = tensor
+ else:
+ # add height attribute
+ if self.attribute_dims is None:
+ self.attribute_dims = dict()
+ attr_dim = self.shape[1]
+ self.tensor = torch.cat([self.tensor, tensor.unsqueeze(1)], dim=1)
+ self.attribute_dims.update(dict(height=attr_dim))
+ self.points_dim += 1
+
+ @property
+ def color(self):
+ """torch.Tensor:
+ A vector with color of each point in shape (N, 3), or None."""
+ if self.attribute_dims is not None and \
+ 'color' in self.attribute_dims.keys():
+ return self.tensor[:, self.attribute_dims['color']]
+ else:
+ return None
+
+ @color.setter
+ def color(self, tensor):
+ """Set the color of each point."""
+ try:
+ tensor = tensor.reshape(self.shape[0], 3)
+ except (RuntimeError, ValueError): # for torch.Tensor and np.ndarray
+ raise ValueError(f'got unexpected shape {tensor.shape}')
+ if tensor.max() >= 256 or tensor.min() < 0:
+ warnings.warn('point got color value beyond [0, 255]')
+ if not isinstance(tensor, torch.Tensor):
+ tensor = self.tensor.new_tensor(tensor)
+ if self.attribute_dims is not None and \
+ 'color' in self.attribute_dims.keys():
+ self.tensor[:, self.attribute_dims['color']] = tensor
+ else:
+ # add color attribute
+ if self.attribute_dims is None:
+ self.attribute_dims = dict()
+ attr_dim = self.shape[1]
+ self.tensor = torch.cat([self.tensor, tensor], dim=1)
+ self.attribute_dims.update(
+ dict(color=[attr_dim, attr_dim + 1, attr_dim + 2]))
+ self.points_dim += 3
+
+ @property
+ def shape(self):
+ """torch.Shape: Shape of points."""
+ return self.tensor.shape
+
+ def shuffle(self):
+ """Shuffle the points.
+
+ Returns:
+ torch.Tensor: The shuffled index.
+ """
+ idx = torch.randperm(self.__len__(), device=self.tensor.device)
+ self.tensor = self.tensor[idx]
+ return idx
+
+ def rotate(self, rotation, axis=None):
+ """Rotate points with the given rotation matrix or angle.
+
+ Args:
+ rotation (float | np.ndarray | torch.Tensor): Rotation matrix
+ or angle.
+ axis (int, optional): Axis to rotate at. Defaults to None.
+ """
+ if not isinstance(rotation, torch.Tensor):
+ rotation = self.tensor.new_tensor(rotation)
+ assert rotation.shape == torch.Size([3, 3]) or \
+ rotation.numel() == 1, f'invalid rotation shape {rotation.shape}'
+
+ if axis is None:
+ axis = self.rotation_axis
+
+ if rotation.numel() == 1:
+ rotated_points, rot_mat_T = rotation_3d_in_axis(
+ self.tensor[:, :3][None], rotation, axis=axis, return_mat=True)
+ self.tensor[:, :3] = rotated_points.squeeze(0)
+ rot_mat_T = rot_mat_T.squeeze(0)
+ else:
+ # rotation.numel() == 9
+ self.tensor[:, :3] = self.tensor[:, :3] @ rotation
+ rot_mat_T = rotation
+
+ return rot_mat_T
+
+ @abstractmethod
+ def flip(self, bev_direction='horizontal'):
+ """Flip the points along given BEV direction.
+
+ Args:
+ bev_direction (str): Flip direction (horizontal or vertical).
+ """
+ pass
+
+ def translate(self, trans_vector):
+ """Translate points with the given translation vector.
+
+ Args:
+ trans_vector (np.ndarray, torch.Tensor): Translation
+ vector of size 3 or nx3.
+ """
+ if not isinstance(trans_vector, torch.Tensor):
+ trans_vector = self.tensor.new_tensor(trans_vector)
+ trans_vector = trans_vector.squeeze(0)
+ if trans_vector.dim() == 1:
+ assert trans_vector.shape[0] == 3
+ elif trans_vector.dim() == 2:
+ assert trans_vector.shape[0] == self.tensor.shape[0] and \
+ trans_vector.shape[1] == 3
+ else:
+ raise NotImplementedError(
+ f'Unsupported translation vector of shape {trans_vector.shape}'
+ )
+ self.tensor[:, :3] += trans_vector
+
+ def in_range_3d(self, point_range):
+ """Check whether the points are in the given range.
+
+ Args:
+ point_range (list | torch.Tensor): The range of point
+ (x_min, y_min, z_min, x_max, y_max, z_max)
+
+ Note:
+ In the original implementation of SECOND, checking whether
+ a box in the range checks whether the points are in a convex
+ polygon, we try to reduce the burden for simpler cases.
+
+ Returns:
+ torch.Tensor: A binary vector indicating whether each point is
+ inside the reference range.
+ """
+ in_range_flags = ((self.tensor[:, 0] > point_range[0])
+ & (self.tensor[:, 1] > point_range[1])
+ & (self.tensor[:, 2] > point_range[2])
+ & (self.tensor[:, 0] < point_range[3])
+ & (self.tensor[:, 1] < point_range[4])
+ & (self.tensor[:, 2] < point_range[5]))
+ return in_range_flags
+
+ @property
+ def bev(self):
+ """torch.Tensor: BEV of the points in shape (N, 2)."""
+ return self.tensor[:, [0, 1]]
+
+ def in_range_bev(self, point_range):
+ """Check whether the points are in the given range.
+
+ Args:
+ point_range (list | torch.Tensor): The range of point
+ in order of (x_min, y_min, x_max, y_max).
+
+ Returns:
+ torch.Tensor: Indicating whether each point is inside
+ the reference range.
+ """
+ in_range_flags = ((self.bev[:, 0] > point_range[0])
+ & (self.bev[:, 1] > point_range[1])
+ & (self.bev[:, 0] < point_range[2])
+ & (self.bev[:, 1] < point_range[3]))
+ return in_range_flags
+
+ @abstractmethod
+ def convert_to(self, dst, rt_mat=None):
+ """Convert self to ``dst`` mode.
+
+ Args:
+ dst (:obj:`CoordMode`): The target Box mode.
+ rt_mat (np.ndarray | torch.Tensor, optional): The rotation and
+ translation matrix between different coordinates.
+ Defaults to None.
+ The conversion from `src` coordinates to `dst` coordinates
+ usually comes along the change of sensors, e.g., from camera
+ to LiDAR. This requires a transformation matrix.
+
+ Returns:
+ :obj:`BasePoints`: The converted box of the same type
+ in the `dst` mode.
+ """
+ pass
+
+ def scale(self, scale_factor):
+ """Scale the points with horizontal and vertical scaling factors.
+
+ Args:
+ scale_factors (float): Scale factors to scale the points.
+ """
+ self.tensor[:, :3] *= scale_factor
+
+ def __getitem__(self, item):
+ """
+ Note:
+ The following usage are allowed:
+ 1. `new_points = points[3]`:
+ return a `Points` that contains only one point.
+ 2. `new_points = points[2:10]`:
+ return a slice of points.
+ 3. `new_points = points[vector]`:
+ where vector is a torch.BoolTensor with `length = len(points)`.
+ Nonzero elements in the vector will be selected.
+ 4. `new_points = points[3:11, vector]`:
+ return a slice of points and attribute dims.
+ 5. `new_points = points[4:12, 2]`:
+ return a slice of points with single attribute.
+ Note that the returned Points might share storage with this Points,
+ subject to Pytorch's indexing semantics.
+
+ Returns:
+ :obj:`BasePoints`: A new object of
+ :class:`BasePoints` after indexing.
+ """
+ original_type = type(self)
+ if isinstance(item, int):
+ return original_type(
+ self.tensor[item].view(1, -1),
+ points_dim=self.points_dim,
+ attribute_dims=self.attribute_dims)
+ elif isinstance(item, tuple) and len(item) == 2:
+ if isinstance(item[1], slice):
+ start = 0 if item[1].start is None else item[1].start
+ stop = self.tensor.shape[1] if \
+ item[1].stop is None else item[1].stop
+ step = 1 if item[1].step is None else item[1].step
+ item = list(item)
+ item[1] = list(range(start, stop, step))
+ item = tuple(item)
+ elif isinstance(item[1], int):
+ item = list(item)
+ item[1] = [item[1]]
+ item = tuple(item)
+ p = self.tensor[item[0], item[1]]
+
+ keep_dims = list(
+ set(item[1]).intersection(set(range(3, self.tensor.shape[1]))))
+ if self.attribute_dims is not None:
+ attribute_dims = self.attribute_dims.copy()
+ for key in self.attribute_dims.keys():
+ cur_attribute_dims = attribute_dims[key]
+ if isinstance(cur_attribute_dims, int):
+ cur_attribute_dims = [cur_attribute_dims]
+ intersect_attr = list(
+ set(cur_attribute_dims).intersection(set(keep_dims)))
+ if len(intersect_attr) == 1:
+ attribute_dims[key] = intersect_attr[0]
+ elif len(intersect_attr) > 1:
+ attribute_dims[key] = intersect_attr
+ else:
+ attribute_dims.pop(key)
+ else:
+ attribute_dims = None
+ elif isinstance(item, (slice, np.ndarray, torch.Tensor)):
+ p = self.tensor[item]
+ attribute_dims = self.attribute_dims
+ else:
+ raise NotImplementedError(f'Invalid slice {item}!')
+
+ assert p.dim() == 2, \
+ f'Indexing on Points with {item} failed to return a matrix!'
+ return original_type(
+ p, points_dim=p.shape[1], attribute_dims=attribute_dims)
+
+ def __len__(self):
+ """int: Number of points in the current object."""
+ return self.tensor.shape[0]
+
+ def __repr__(self):
+ """str: Return a strings that describes the object."""
+ return self.__class__.__name__ + '(\n ' + str(self.tensor) + ')'
+
+ @classmethod
+ def cat(cls, points_list):
+ """Concatenate a list of Points into a single Points.
+
+ Args:
+ points_list (list[:obj:`BasePoints`]): List of points.
+
+ Returns:
+ :obj:`BasePoints`: The concatenated Points.
+ """
+ assert isinstance(points_list, (list, tuple))
+ if len(points_list) == 0:
+ return cls(torch.empty(0))
+ assert all(isinstance(points, cls) for points in points_list)
+
+ # use torch.cat (v.s. layers.cat)
+ # so the returned points never share storage with input
+ cat_points = cls(
+ torch.cat([p.tensor for p in points_list], dim=0),
+ points_dim=points_list[0].tensor.shape[1],
+ attribute_dims=points_list[0].attribute_dims)
+ return cat_points
+
+ def to(self, device):
+ """Convert current points to a specific device.
+
+ Args:
+ device (str | :obj:`torch.device`): The name of the device.
+
+ Returns:
+ :obj:`BasePoints`: A new boxes object on the
+ specific device.
+ """
+ original_type = type(self)
+ return original_type(
+ self.tensor.to(device),
+ points_dim=self.points_dim,
+ attribute_dims=self.attribute_dims)
+
+ def clone(self):
+ """Clone the Points.
+
+ Returns:
+ :obj:`BasePoints`: Box object with the same properties
+ as self.
+ """
+ original_type = type(self)
+ return original_type(
+ self.tensor.clone(),
+ points_dim=self.points_dim,
+ attribute_dims=self.attribute_dims)
+
+ @property
+ def device(self):
+ """str: The device of the points are on."""
+ return self.tensor.device
+
+ def __iter__(self):
+ """Yield a point as a Tensor of shape (4,) at a time.
+
+ Returns:
+ torch.Tensor: A point of shape (4,).
+ """
+ yield from self.tensor
+
+ def new_point(self, data):
+ """Create a new point object with data.
+
+ The new point and its tensor has the similar properties
+ as self and self.tensor, respectively.
+
+ Args:
+ data (torch.Tensor | numpy.array | list): Data to be copied.
+
+ Returns:
+ :obj:`BasePoints`: A new point object with ``data``,
+ the object's other properties are similar to ``self``.
+ """
+ new_tensor = self.tensor.new_tensor(data) \
+ if not isinstance(data, torch.Tensor) else data.to(self.device)
+ original_type = type(self)
+ return original_type(
+ new_tensor,
+ points_dim=self.points_dim,
+ attribute_dims=self.attribute_dims)
diff --git a/mmdet3d/core/points/cam_points.py b/mmdet3d/core/points/cam_points.py
new file mode 100644
index 0000000..a57c3db
--- /dev/null
+++ b/mmdet3d/core/points/cam_points.py
@@ -0,0 +1,63 @@
+# Copyright (c) OpenMMLab. All rights reserved.
+from .base_points import BasePoints
+
+
+class CameraPoints(BasePoints):
+ """Points of instances in CAM coordinates.
+
+ Args:
+ tensor (torch.Tensor | np.ndarray | list): a N x points_dim matrix.
+ points_dim (int, optional): Number of the dimension of a point.
+ Each row is (x, y, z). Defaults to 3.
+ attribute_dims (dict, optional): Dictionary to indicate the
+ meaning of extra dimension. Defaults to None.
+
+ Attributes:
+ tensor (torch.Tensor): Float matrix of N x points_dim.
+ points_dim (int): Integer indicating the dimension of a point.
+ Each row is (x, y, z, ...).
+ attribute_dims (bool): Dictionary to indicate the meaning of extra
+ dimension. Defaults to None.
+ rotation_axis (int): Default rotation axis for points rotation.
+ """
+
+ def __init__(self, tensor, points_dim=3, attribute_dims=None):
+ super(CameraPoints, self).__init__(
+ tensor, points_dim=points_dim, attribute_dims=attribute_dims)
+ self.rotation_axis = 1
+
+ def flip(self, bev_direction='horizontal'):
+ """Flip the points along given BEV direction.
+
+ Args:
+ bev_direction (str): Flip direction (horizontal or vertical).
+ """
+ if bev_direction == 'horizontal':
+ self.tensor[:, 0] = -self.tensor[:, 0]
+ elif bev_direction == 'vertical':
+ self.tensor[:, 2] = -self.tensor[:, 2]
+
+ @property
+ def bev(self):
+ """torch.Tensor: BEV of the points in shape (N, 2)."""
+ return self.tensor[:, [0, 2]]
+
+ def convert_to(self, dst, rt_mat=None):
+ """Convert self to ``dst`` mode.
+
+ Args:
+ dst (:obj:`CoordMode`): The target Point mode.
+ rt_mat (np.ndarray | torch.Tensor, optional): The rotation and
+ translation matrix between different coordinates.
+ Defaults to None.
+ The conversion from `src` coordinates to `dst` coordinates
+ usually comes along the change of sensors, e.g., from camera
+ to LiDAR. This requires a transformation matrix.
+
+ Returns:
+ :obj:`BasePoints`: The converted point of the same type
+ in the `dst` mode.
+ """
+ from mmdet3d.core.bbox import Coord3DMode
+ return Coord3DMode.convert_point(
+ point=self, src=Coord3DMode.CAM, dst=dst, rt_mat=rt_mat)
diff --git a/mmdet3d/core/points/depth_points.py b/mmdet3d/core/points/depth_points.py
new file mode 100644
index 0000000..2d9221f
--- /dev/null
+++ b/mmdet3d/core/points/depth_points.py
@@ -0,0 +1,58 @@
+# Copyright (c) OpenMMLab. All rights reserved.
+from .base_points import BasePoints
+
+
+class DepthPoints(BasePoints):
+ """Points of instances in DEPTH coordinates.
+
+ Args:
+ tensor (torch.Tensor | np.ndarray | list): a N x points_dim matrix.
+ points_dim (int, optional): Number of the dimension of a point.
+ Each row is (x, y, z). Defaults to 3.
+ attribute_dims (dict, optional): Dictionary to indicate the
+ meaning of extra dimension. Defaults to None.
+
+ Attributes:
+ tensor (torch.Tensor): Float matrix of N x points_dim.
+ points_dim (int): Integer indicating the dimension of a point.
+ Each row is (x, y, z, ...).
+ attribute_dims (bool): Dictionary to indicate the meaning of extra
+ dimension. Defaults to None.
+ rotation_axis (int): Default rotation axis for points rotation.
+ """
+
+ def __init__(self, tensor, points_dim=3, attribute_dims=None):
+ super(DepthPoints, self).__init__(
+ tensor, points_dim=points_dim, attribute_dims=attribute_dims)
+ self.rotation_axis = 2
+
+ def flip(self, bev_direction='horizontal'):
+ """Flip the points along given BEV direction.
+
+ Args:
+ bev_direction (str): Flip direction (horizontal or vertical).
+ """
+ if bev_direction == 'horizontal':
+ self.tensor[:, 0] = -self.tensor[:, 0]
+ elif bev_direction == 'vertical':
+ self.tensor[:, 1] = -self.tensor[:, 1]
+
+ def convert_to(self, dst, rt_mat=None):
+ """Convert self to ``dst`` mode.
+
+ Args:
+ dst (:obj:`CoordMode`): The target Point mode.
+ rt_mat (np.ndarray | torch.Tensor, optional): The rotation and
+ translation matrix between different coordinates.
+ Defaults to None.
+ The conversion from `src` coordinates to `dst` coordinates
+ usually comes along the change of sensors, e.g., from camera
+ to LiDAR. This requires a transformation matrix.
+
+ Returns:
+ :obj:`BasePoints`: The converted point of the same type
+ in the `dst` mode.
+ """
+ from mmdet3d.core.bbox import Coord3DMode
+ return Coord3DMode.convert_point(
+ point=self, src=Coord3DMode.DEPTH, dst=dst, rt_mat=rt_mat)
diff --git a/mmdet3d/core/points/lidar_points.py b/mmdet3d/core/points/lidar_points.py
new file mode 100644
index 0000000..ff4f57a
--- /dev/null
+++ b/mmdet3d/core/points/lidar_points.py
@@ -0,0 +1,58 @@
+# Copyright (c) OpenMMLab. All rights reserved.
+from .base_points import BasePoints
+
+
+class LiDARPoints(BasePoints):
+ """Points of instances in LIDAR coordinates.
+
+ Args:
+ tensor (torch.Tensor | np.ndarray | list): a N x points_dim matrix.
+ points_dim (int, optional): Number of the dimension of a point.
+ Each row is (x, y, z). Defaults to 3.
+ attribute_dims (dict, optional): Dictionary to indicate the
+ meaning of extra dimension. Defaults to None.
+
+ Attributes:
+ tensor (torch.Tensor): Float matrix of N x points_dim.
+ points_dim (int): Integer indicating the dimension of a point.
+ Each row is (x, y, z, ...).
+ attribute_dims (bool): Dictionary to indicate the meaning of extra
+ dimension. Defaults to None.
+ rotation_axis (int): Default rotation axis for points rotation.
+ """
+
+ def __init__(self, tensor, points_dim=3, attribute_dims=None):
+ super(LiDARPoints, self).__init__(
+ tensor, points_dim=points_dim, attribute_dims=attribute_dims)
+ self.rotation_axis = 2
+
+ def flip(self, bev_direction='horizontal'):
+ """Flip the points along given BEV direction.
+
+ Args:
+ bev_direction (str): Flip direction (horizontal or vertical).
+ """
+ if bev_direction == 'horizontal':
+ self.tensor[:, 1] = -self.tensor[:, 1]
+ elif bev_direction == 'vertical':
+ self.tensor[:, 0] = -self.tensor[:, 0]
+
+ def convert_to(self, dst, rt_mat=None):
+ """Convert self to ``dst`` mode.
+
+ Args:
+ dst (:obj:`CoordMode`): The target Point mode.
+ rt_mat (np.ndarray | torch.Tensor, optional): The rotation and
+ translation matrix between different coordinates.
+ Defaults to None.
+ The conversion from `src` coordinates to `dst` coordinates
+ usually comes along the change of sensors, e.g., from camera
+ to LiDAR. This requires a transformation matrix.
+
+ Returns:
+ :obj:`BasePoints`: The converted point of the same type
+ in the `dst` mode.
+ """
+ from mmdet3d.core.bbox import Coord3DMode
+ return Coord3DMode.convert_point(
+ point=self, src=Coord3DMode.LIDAR, dst=dst, rt_mat=rt_mat)
diff --git a/mmdet3d/core/post_processing/__init__.py b/mmdet3d/core/post_processing/__init__.py
new file mode 100644
index 0000000..2fb534e
--- /dev/null
+++ b/mmdet3d/core/post_processing/__init__.py
@@ -0,0 +1,14 @@
+# Copyright (c) OpenMMLab. All rights reserved.
+from mmdet.core.post_processing import (merge_aug_bboxes, merge_aug_masks,
+ merge_aug_proposals, merge_aug_scores,
+ multiclass_nms)
+from .box3d_nms import (aligned_3d_nms, box3d_multiclass_nms, circle_nms,
+ nms_bev, nms_normal_bev)
+from .merge_augs import merge_aug_bboxes_3d
+
+__all__ = [
+ 'multiclass_nms', 'merge_aug_proposals', 'merge_aug_bboxes',
+ 'merge_aug_scores', 'merge_aug_masks', 'box3d_multiclass_nms',
+ 'aligned_3d_nms', 'merge_aug_bboxes_3d', 'circle_nms', 'nms_bev',
+ 'nms_normal_bev'
+]
diff --git a/mmdet3d/core/post_processing/box3d_nms.py b/mmdet3d/core/post_processing/box3d_nms.py
new file mode 100644
index 0000000..2d42085
--- /dev/null
+++ b/mmdet3d/core/post_processing/box3d_nms.py
@@ -0,0 +1,288 @@
+# Copyright (c) OpenMMLab. All rights reserved.
+import numba
+import numpy as np
+import torch
+from mmcv.ops import nms, nms_rotated
+
+
+def box3d_multiclass_nms(mlvl_bboxes,
+ mlvl_bboxes_for_nms,
+ mlvl_scores,
+ score_thr,
+ max_num,
+ cfg,
+ mlvl_dir_scores=None,
+ mlvl_attr_scores=None,
+ mlvl_bboxes2d=None):
+ """Multi-class NMS for 3D boxes. The IoU used for NMS is defined as the 2D
+ IoU between BEV boxes.
+
+ Args:
+ mlvl_bboxes (torch.Tensor): Multi-level boxes with shape (N, M).
+ M is the dimensions of boxes.
+ mlvl_bboxes_for_nms (torch.Tensor): Multi-level boxes with shape
+ (N, 5) ([x1, y1, x2, y2, ry]). N is the number of boxes.
+ The coordinate system of the BEV boxes is counterclockwise.
+ mlvl_scores (torch.Tensor): Multi-level boxes with shape
+ (N, C + 1). N is the number of boxes. C is the number of classes.
+ score_thr (float): Score threshold to filter boxes with low
+ confidence.
+ max_num (int): Maximum number of boxes will be kept.
+ cfg (dict): Configuration dict of NMS.
+ mlvl_dir_scores (torch.Tensor, optional): Multi-level scores
+ of direction classifier. Defaults to None.
+ mlvl_attr_scores (torch.Tensor, optional): Multi-level scores
+ of attribute classifier. Defaults to None.
+ mlvl_bboxes2d (torch.Tensor, optional): Multi-level 2D bounding
+ boxes. Defaults to None.
+
+ Returns:
+ tuple[torch.Tensor]: Return results after nms, including 3D
+ bounding boxes, scores, labels, direction scores, attribute
+ scores (optional) and 2D bounding boxes (optional).
+ """
+ # do multi class nms
+ # the fg class id range: [0, num_classes-1]
+ num_classes = mlvl_scores.shape[1] - 1
+ bboxes = []
+ scores = []
+ labels = []
+ dir_scores = []
+ attr_scores = []
+ bboxes2d = []
+ for i in range(0, num_classes):
+ # get bboxes and scores of this class
+ cls_inds = mlvl_scores[:, i] > score_thr
+ if not cls_inds.any():
+ continue
+
+ _scores = mlvl_scores[cls_inds, i]
+ _bboxes_for_nms = mlvl_bboxes_for_nms[cls_inds, :]
+
+ if cfg.use_rotate_nms:
+ nms_func = nms_bev
+ else:
+ nms_func = nms_normal_bev
+
+ selected = nms_func(_bboxes_for_nms, _scores, cfg.nms_thr)
+ _mlvl_bboxes = mlvl_bboxes[cls_inds, :]
+ bboxes.append(_mlvl_bboxes[selected])
+ scores.append(_scores[selected])
+ cls_label = mlvl_bboxes.new_full((len(selected), ),
+ i,
+ dtype=torch.long)
+ labels.append(cls_label)
+
+ if mlvl_dir_scores is not None:
+ _mlvl_dir_scores = mlvl_dir_scores[cls_inds]
+ dir_scores.append(_mlvl_dir_scores[selected])
+ if mlvl_attr_scores is not None:
+ _mlvl_attr_scores = mlvl_attr_scores[cls_inds]
+ attr_scores.append(_mlvl_attr_scores[selected])
+ if mlvl_bboxes2d is not None:
+ _mlvl_bboxes2d = mlvl_bboxes2d[cls_inds]
+ bboxes2d.append(_mlvl_bboxes2d[selected])
+
+ if bboxes:
+ bboxes = torch.cat(bboxes, dim=0)
+ scores = torch.cat(scores, dim=0)
+ labels = torch.cat(labels, dim=0)
+ if mlvl_dir_scores is not None:
+ dir_scores = torch.cat(dir_scores, dim=0)
+ if mlvl_attr_scores is not None:
+ attr_scores = torch.cat(attr_scores, dim=0)
+ if mlvl_bboxes2d is not None:
+ bboxes2d = torch.cat(bboxes2d, dim=0)
+ if bboxes.shape[0] > max_num:
+ _, inds = scores.sort(descending=True)
+ inds = inds[:max_num]
+ bboxes = bboxes[inds, :]
+ labels = labels[inds]
+ scores = scores[inds]
+ if mlvl_dir_scores is not None:
+ dir_scores = dir_scores[inds]
+ if mlvl_attr_scores is not None:
+ attr_scores = attr_scores[inds]
+ if mlvl_bboxes2d is not None:
+ bboxes2d = bboxes2d[inds]
+ else:
+ bboxes = mlvl_scores.new_zeros((0, mlvl_bboxes.size(-1)))
+ scores = mlvl_scores.new_zeros((0, ))
+ labels = mlvl_scores.new_zeros((0, ), dtype=torch.long)
+ if mlvl_dir_scores is not None:
+ dir_scores = mlvl_scores.new_zeros((0, ))
+ if mlvl_attr_scores is not None:
+ attr_scores = mlvl_scores.new_zeros((0, ))
+ if mlvl_bboxes2d is not None:
+ bboxes2d = mlvl_scores.new_zeros((0, 4))
+
+ results = (bboxes, scores, labels)
+
+ if mlvl_dir_scores is not None:
+ results = results + (dir_scores, )
+ if mlvl_attr_scores is not None:
+ results = results + (attr_scores, )
+ if mlvl_bboxes2d is not None:
+ results = results + (bboxes2d, )
+
+ return results
+
+
+def aligned_3d_nms(boxes, scores, classes, thresh):
+ """3D NMS for aligned boxes.
+
+ Args:
+ boxes (torch.Tensor): Aligned box with shape [n, 6].
+ scores (torch.Tensor): Scores of each box.
+ classes (torch.Tensor): Class of each box.
+ thresh (float): IoU threshold for nms.
+
+ Returns:
+ torch.Tensor: Indices of selected boxes.
+ """
+ x1 = boxes[:, 0]
+ y1 = boxes[:, 1]
+ z1 = boxes[:, 2]
+ x2 = boxes[:, 3]
+ y2 = boxes[:, 4]
+ z2 = boxes[:, 5]
+ area = (x2 - x1) * (y2 - y1) * (z2 - z1)
+ zero = boxes.new_zeros(1, )
+
+ score_sorted = torch.argsort(scores)
+ pick = []
+ while (score_sorted.shape[0] != 0):
+ last = score_sorted.shape[0]
+ i = score_sorted[-1]
+ pick.append(i)
+
+ xx1 = torch.max(x1[i], x1[score_sorted[:last - 1]])
+ yy1 = torch.max(y1[i], y1[score_sorted[:last - 1]])
+ zz1 = torch.max(z1[i], z1[score_sorted[:last - 1]])
+ xx2 = torch.min(x2[i], x2[score_sorted[:last - 1]])
+ yy2 = torch.min(y2[i], y2[score_sorted[:last - 1]])
+ zz2 = torch.min(z2[i], z2[score_sorted[:last - 1]])
+ classes1 = classes[i]
+ classes2 = classes[score_sorted[:last - 1]]
+ inter_l = torch.max(zero, xx2 - xx1)
+ inter_w = torch.max(zero, yy2 - yy1)
+ inter_h = torch.max(zero, zz2 - zz1)
+
+ inter = inter_l * inter_w * inter_h
+ iou = inter / (area[i] + area[score_sorted[:last - 1]] - inter)
+ iou = iou * (classes1 == classes2).float()
+ score_sorted = score_sorted[torch.nonzero(
+ iou <= thresh, as_tuple=False).flatten()]
+
+ indices = boxes.new_tensor(pick, dtype=torch.long)
+ return indices
+
+
+@numba.jit(nopython=True)
+def circle_nms(dets, thresh, post_max_size=83):
+ """Circular NMS.
+
+ An object is only counted as positive if no other center
+ with a higher confidence exists within a radius r using a
+ bird-eye view distance metric.
+
+ Args:
+ dets (torch.Tensor): Detection results with the shape of [N, 3].
+ thresh (float): Value of threshold.
+ post_max_size (int, optional): Max number of prediction to be kept.
+ Defaults to 83.
+
+ Returns:
+ torch.Tensor: Indexes of the detections to be kept.
+ """
+ x1 = dets[:, 0]
+ y1 = dets[:, 1]
+ scores = dets[:, 2]
+ order = scores.argsort()[::-1].astype(np.int32) # highest->lowest
+ ndets = dets.shape[0]
+ suppressed = np.zeros((ndets), dtype=np.int32)
+ keep = []
+ for _i in range(ndets):
+ i = order[_i] # start with highest score box
+ if suppressed[
+ i] == 1: # if any box have enough iou with this, remove it
+ continue
+ keep.append(i)
+ for _j in range(_i + 1, ndets):
+ j = order[_j]
+ if suppressed[j] == 1:
+ continue
+ # calculate center distance between i and j box
+ dist = (x1[i] - x1[j])**2 + (y1[i] - y1[j])**2
+
+ # ovr = inter / areas[j]
+ if dist <= thresh:
+ suppressed[j] = 1
+
+ if post_max_size < len(keep):
+ return keep[:post_max_size]
+
+ return keep
+
+
+# This function duplicates functionality of mmcv.ops.iou_3d.nms_bev
+# from mmcv<=1.5, but using cuda ops from mmcv.ops.nms.nms_rotated.
+# Nms api will be unified in mmdetection3d one day.
+def nms_bev(boxes, scores, thresh, pre_max_size=None, post_max_size=None):
+ """NMS function GPU implementation (for BEV boxes). The overlap of two
+ boxes for IoU calculation is defined as the exact overlapping area of the
+ two boxes. In this function, one can also set ``pre_max_size`` and
+ ``post_max_size``.
+
+ Args:
+ boxes (torch.Tensor): Input boxes with the shape of [N, 5]
+ ([x1, y1, x2, y2, ry]).
+ scores (torch.Tensor): Scores of boxes with the shape of [N].
+ thresh (float): Overlap threshold of NMS.
+ pre_max_size (int, optional): Max size of boxes before NMS.
+ Default: None.
+ post_max_size (int, optional): Max size of boxes after NMS.
+ Default: None.
+
+ Returns:
+ torch.Tensor: Indexes after NMS.
+ """
+ assert boxes.size(1) == 5, 'Input boxes shape should be [N, 5]'
+ order = scores.sort(0, descending=True)[1]
+ if pre_max_size is not None:
+ order = order[:pre_max_size]
+ boxes = boxes[order].contiguous()
+ scores = scores[order]
+
+ # xyxyr -> back to xywhr
+ # note: better skip this step before nms_bev call in the future
+ boxes = torch.stack(
+ ((boxes[:, 0] + boxes[:, 2]) / 2, (boxes[:, 1] + boxes[:, 3]) / 2,
+ boxes[:, 2] - boxes[:, 0], boxes[:, 3] - boxes[:, 1], boxes[:, 4]),
+ dim=-1)
+
+ keep = nms_rotated(boxes, scores, thresh)[1]
+ keep = order[keep]
+ if post_max_size is not None:
+ keep = keep[:post_max_size]
+ return keep
+
+
+# This function duplicates functionality of mmcv.ops.iou_3d.nms_normal_bev
+# from mmcv<=1.5, but using cuda ops from mmcv.ops.nms.nms.
+# Nms api will be unified in mmdetection3d one day.
+def nms_normal_bev(boxes, scores, thresh):
+ """Normal NMS function GPU implementation (for BEV boxes). The overlap of
+ two boxes for IoU calculation is defined as the exact overlapping area of
+ the two boxes WITH their yaw angle set to 0.
+
+ Args:
+ boxes (torch.Tensor): Input boxes with shape (N, 5).
+ scores (torch.Tensor): Scores of predicted boxes with shape (N).
+ thresh (float): Overlap threshold of NMS.
+
+ Returns:
+ torch.Tensor: Remaining indices with scores in descending order.
+ """
+ assert boxes.shape[1] == 5, 'Input boxes shape should be [N, 5]'
+ return nms(boxes[:, :-1], scores, thresh)[1]
diff --git a/mmdet3d/core/post_processing/merge_augs.py b/mmdet3d/core/post_processing/merge_augs.py
new file mode 100644
index 0000000..0e20dcd
--- /dev/null
+++ b/mmdet3d/core/post_processing/merge_augs.py
@@ -0,0 +1,92 @@
+# Copyright (c) OpenMMLab. All rights reserved.
+import torch
+
+from mmdet3d.core.post_processing import nms_bev, nms_normal_bev
+from ..bbox import bbox3d2result, bbox3d_mapping_back, xywhr2xyxyr
+
+
+def merge_aug_bboxes_3d(aug_results, img_metas, test_cfg):
+ """Merge augmented detection 3D bboxes and scores.
+
+ Args:
+ aug_results (list[dict]): The dict of detection results.
+ The dict contains the following keys
+
+ - boxes_3d (:obj:`BaseInstance3DBoxes`): Detection bbox.
+ - scores_3d (torch.Tensor): Detection scores.
+ - labels_3d (torch.Tensor): Predicted box labels.
+ img_metas (list[dict]): Meta information of each sample.
+ test_cfg (dict): Test config.
+
+ Returns:
+ dict: Bounding boxes results in cpu mode, containing merged results.
+
+ - boxes_3d (:obj:`BaseInstance3DBoxes`): Merged detection bbox.
+ - scores_3d (torch.Tensor): Merged detection scores.
+ - labels_3d (torch.Tensor): Merged predicted box labels.
+ """
+
+ assert len(aug_results) == len(img_metas), \
+ '"aug_results" should have the same length as "img_metas", got len(' \
+ f'aug_results)={len(aug_results)} and len(img_metas)={len(img_metas)}'
+
+ recovered_bboxes = []
+ recovered_scores = []
+ recovered_labels = []
+
+ for bboxes, img_info in zip(aug_results, img_metas):
+ scale_factor = img_info[0]['pcd_scale_factor']
+ pcd_horizontal_flip = img_info[0]['pcd_horizontal_flip']
+ pcd_vertical_flip = img_info[0]['pcd_vertical_flip']
+ recovered_scores.append(bboxes['scores_3d'])
+ recovered_labels.append(bboxes['labels_3d'])
+ bboxes = bbox3d_mapping_back(bboxes['boxes_3d'], scale_factor,
+ pcd_horizontal_flip, pcd_vertical_flip)
+ recovered_bboxes.append(bboxes)
+
+ aug_bboxes = recovered_bboxes[0].cat(recovered_bboxes)
+ aug_bboxes_for_nms = xywhr2xyxyr(aug_bboxes.bev)
+ aug_scores = torch.cat(recovered_scores, dim=0)
+ aug_labels = torch.cat(recovered_labels, dim=0)
+
+ # TODO: use a more elegent way to deal with nms
+ if test_cfg.use_rotate_nms:
+ nms_func = nms_bev
+ else:
+ nms_func = nms_normal_bev
+
+ merged_bboxes = []
+ merged_scores = []
+ merged_labels = []
+
+ # Apply multi-class nms when merge bboxes
+ if len(aug_labels) == 0:
+ return bbox3d2result(aug_bboxes, aug_scores, aug_labels)
+
+ for class_id in range(torch.max(aug_labels).item() + 1):
+ class_inds = (aug_labels == class_id)
+ bboxes_i = aug_bboxes[class_inds]
+ bboxes_nms_i = aug_bboxes_for_nms[class_inds, :]
+ scores_i = aug_scores[class_inds]
+ labels_i = aug_labels[class_inds]
+ if len(bboxes_nms_i) == 0:
+ continue
+ selected = nms_func(bboxes_nms_i, scores_i, test_cfg.nms_thr)
+
+ merged_bboxes.append(bboxes_i[selected, :])
+ merged_scores.append(scores_i[selected])
+ merged_labels.append(labels_i[selected])
+
+ merged_bboxes = merged_bboxes[0].cat(merged_bboxes)
+ merged_scores = torch.cat(merged_scores, dim=0)
+ merged_labels = torch.cat(merged_labels, dim=0)
+
+ _, order = merged_scores.sort(0, descending=True)
+ num = min(test_cfg.max_num, len(aug_bboxes))
+ order = order[:num]
+
+ merged_bboxes = merged_bboxes[order]
+ merged_scores = merged_scores[order]
+ merged_labels = merged_labels[order]
+
+ return bbox3d2result(merged_bboxes, merged_scores, merged_labels)
diff --git a/mmdet3d/core/utils/__init__.py b/mmdet3d/core/utils/__init__.py
new file mode 100644
index 0000000..b2a8dec
--- /dev/null
+++ b/mmdet3d/core/utils/__init__.py
@@ -0,0 +1,10 @@
+# Copyright (c) OpenMMLab. All rights reserved.
+from .array_converter import ArrayConverter, array_converter
+from .gaussian import (draw_heatmap_gaussian, ellip_gaussian2D, gaussian_2d,
+ gaussian_radius, get_ellip_gaussian_2D)
+
+__all__ = [
+ 'gaussian_2d', 'gaussian_radius', 'draw_heatmap_gaussian',
+ 'ArrayConverter', 'array_converter', 'ellip_gaussian2D',
+ 'get_ellip_gaussian_2D'
+]
diff --git a/mmdet3d/core/utils/array_converter.py b/mmdet3d/core/utils/array_converter.py
new file mode 100644
index 0000000..a555aa6
--- /dev/null
+++ b/mmdet3d/core/utils/array_converter.py
@@ -0,0 +1,324 @@
+# Copyright (c) OpenMMLab. All rights reserved.
+import functools
+from inspect import getfullargspec
+
+import numpy as np
+import torch
+
+
+def array_converter(to_torch=True,
+ apply_to=tuple(),
+ template_arg_name_=None,
+ recover=True):
+ """Wrapper function for data-type agnostic processing.
+
+ First converts input arrays to PyTorch tensors or NumPy ndarrays
+ for middle calculation, then convert output to original data-type if
+ `recover=True`.
+
+ Args:
+ to_torch (Bool, optional): Whether convert to PyTorch tensors
+ for middle calculation. Defaults to True.
+ apply_to (tuple[str], optional): The arguments to which we apply
+ data-type conversion. Defaults to an empty tuple.
+ template_arg_name_ (str, optional): Argument serving as the template (
+ return arrays should have the same dtype and device
+ as the template). Defaults to None. If None, we will use the
+ first argument in `apply_to` as the template argument.
+ recover (Bool, optional): Whether or not recover the wrapped function
+ outputs to the `template_arg_name_` type. Defaults to True.
+
+ Raises:
+ ValueError: When template_arg_name_ is not among all args, or
+ when apply_to contains an arg which is not among all args,
+ a ValueError will be raised. When the template argument or
+ an argument to convert is a list or tuple, and cannot be
+ converted to a NumPy array, a ValueError will be raised.
+ TypeError: When the type of the template argument or
+ an argument to convert does not belong to the above range,
+ or the contents of such an list-or-tuple-type argument
+ do not share the same data type, a TypeError is raised.
+
+ Returns:
+ (function): wrapped function.
+
+ Example:
+ >>> import torch
+ >>> import numpy as np
+ >>>
+ >>> # Use torch addition for a + b,
+ >>> # and convert return values to the type of a
+ >>> @array_converter(apply_to=('a', 'b'))
+ >>> def simple_add(a, b):
+ >>> return a + b
+ >>>
+ >>> a = np.array([1.1])
+ >>> b = np.array([2.2])
+ >>> simple_add(a, b)
+ >>>
+ >>> # Use numpy addition for a + b,
+ >>> # and convert return values to the type of b
+ >>> @array_converter(to_torch=False, apply_to=('a', 'b'),
+ >>> template_arg_name_='b')
+ >>> def simple_add(a, b):
+ >>> return a + b
+ >>>
+ >>> simple_add()
+ >>>
+ >>> # Use torch funcs for floor(a) if flag=True else ceil(a),
+ >>> # and return the torch tensor
+ >>> @array_converter(apply_to=('a',), recover=False)
+ >>> def floor_or_ceil(a, flag=True):
+ >>> return torch.floor(a) if flag else torch.ceil(a)
+ >>>
+ >>> floor_or_ceil(a, flag=False)
+ """
+
+ def array_converter_wrapper(func):
+ """Outer wrapper for the function."""
+
+ @functools.wraps(func)
+ def new_func(*args, **kwargs):
+ """Inner wrapper for the arguments."""
+ if len(apply_to) == 0:
+ return func(*args, **kwargs)
+
+ func_name = func.__name__
+
+ arg_spec = getfullargspec(func)
+
+ arg_names = arg_spec.args
+ arg_num = len(arg_names)
+ default_arg_values = arg_spec.defaults
+ if default_arg_values is None:
+ default_arg_values = []
+ no_default_arg_num = len(arg_names) - len(default_arg_values)
+
+ kwonly_arg_names = arg_spec.kwonlyargs
+ kwonly_default_arg_values = arg_spec.kwonlydefaults
+ if kwonly_default_arg_values is None:
+ kwonly_default_arg_values = {}
+
+ all_arg_names = arg_names + kwonly_arg_names
+
+ # in case there are args in the form of *args
+ if len(args) > arg_num:
+ named_args = args[:arg_num]
+ nameless_args = args[arg_num:]
+ else:
+ named_args = args
+ nameless_args = []
+
+ # template argument data type is used for all array-like arguments
+ if template_arg_name_ is None:
+ template_arg_name = apply_to[0]
+ else:
+ template_arg_name = template_arg_name_
+
+ if template_arg_name not in all_arg_names:
+ raise ValueError(f'{template_arg_name} is not among the '
+ f'argument list of function {func_name}')
+
+ # inspect apply_to
+ for arg_to_apply in apply_to:
+ if arg_to_apply not in all_arg_names:
+ raise ValueError(f'{arg_to_apply} is not '
+ f'an argument of {func_name}')
+
+ new_args = []
+ new_kwargs = {}
+
+ converter = ArrayConverter()
+ target_type = torch.Tensor if to_torch else np.ndarray
+
+ # non-keyword arguments
+ for i, arg_value in enumerate(named_args):
+ if arg_names[i] in apply_to:
+ new_args.append(
+ converter.convert(
+ input_array=arg_value, target_type=target_type))
+ else:
+ new_args.append(arg_value)
+
+ if arg_names[i] == template_arg_name:
+ template_arg_value = arg_value
+
+ kwonly_default_arg_values.update(kwargs)
+ kwargs = kwonly_default_arg_values
+
+ # keyword arguments and non-keyword arguments using default value
+ for i in range(len(named_args), len(all_arg_names)):
+ arg_name = all_arg_names[i]
+ if arg_name in kwargs:
+ if arg_name in apply_to:
+ new_kwargs[arg_name] = converter.convert(
+ input_array=kwargs[arg_name],
+ target_type=target_type)
+ else:
+ new_kwargs[arg_name] = kwargs[arg_name]
+ else:
+ default_value = default_arg_values[i - no_default_arg_num]
+ if arg_name in apply_to:
+ new_kwargs[arg_name] = converter.convert(
+ input_array=default_value, target_type=target_type)
+ else:
+ new_kwargs[arg_name] = default_value
+ if arg_name == template_arg_name:
+ template_arg_value = kwargs[arg_name]
+
+ # add nameless args provided by *args (if exists)
+ new_args += nameless_args
+
+ return_values = func(*new_args, **new_kwargs)
+ converter.set_template(template_arg_value)
+
+ def recursive_recover(input_data):
+ if isinstance(input_data, (tuple, list)):
+ new_data = []
+ for item in input_data:
+ new_data.append(recursive_recover(item))
+ return tuple(new_data) if isinstance(input_data,
+ tuple) else new_data
+ elif isinstance(input_data, dict):
+ new_data = {}
+ for k, v in input_data.items():
+ new_data[k] = recursive_recover(v)
+ return new_data
+ elif isinstance(input_data, (torch.Tensor, np.ndarray)):
+ return converter.recover(input_data)
+ else:
+ return input_data
+
+ if recover:
+ return recursive_recover(return_values)
+ else:
+ return return_values
+
+ return new_func
+
+ return array_converter_wrapper
+
+
+class ArrayConverter:
+
+ SUPPORTED_NON_ARRAY_TYPES = (int, float, np.int8, np.int16, np.int32,
+ np.int64, np.uint8, np.uint16, np.uint32,
+ np.uint64, np.float16, np.float32, np.float64)
+
+ def __init__(self, template_array=None):
+ if template_array is not None:
+ self.set_template(template_array)
+
+ def set_template(self, array):
+ """Set template array.
+
+ Args:
+ array (tuple | list | int | float | np.ndarray | torch.Tensor):
+ Template array.
+
+ Raises:
+ ValueError: If input is list or tuple and cannot be converted to
+ to a NumPy array, a ValueError is raised.
+ TypeError: If input type does not belong to the above range,
+ or the contents of a list or tuple do not share the
+ same data type, a TypeError is raised.
+ """
+ self.array_type = type(array)
+ self.is_num = False
+ self.device = 'cpu'
+
+ if isinstance(array, np.ndarray):
+ self.dtype = array.dtype
+ elif isinstance(array, torch.Tensor):
+ self.dtype = array.dtype
+ self.device = array.device
+ elif isinstance(array, (list, tuple)):
+ try:
+ array = np.array(array)
+ if array.dtype not in self.SUPPORTED_NON_ARRAY_TYPES:
+ raise TypeError
+ self.dtype = array.dtype
+ except (ValueError, TypeError):
+ print(f'The following list cannot be converted to'
+ f' a numpy array of supported dtype:\n{array}')
+ raise
+ elif isinstance(array, self.SUPPORTED_NON_ARRAY_TYPES):
+ self.array_type = np.ndarray
+ self.is_num = True
+ self.dtype = np.dtype(type(array))
+ else:
+ raise TypeError(f'Template type {self.array_type}'
+ f' is not supported.')
+
+ def convert(self, input_array, target_type=None, target_array=None):
+ """Convert input array to target data type.
+
+ Args:
+ input_array (tuple | list | np.ndarray |
+ torch.Tensor | int | float ):
+ Input array. Defaults to None.
+ target_type ( | ,
+ optional):
+ Type to which input array is converted. Defaults to None.
+ target_array (np.ndarray | torch.Tensor, optional):
+ Template array to which input array is converted.
+ Defaults to None.
+
+ Raises:
+ ValueError: If input is list or tuple and cannot be converted to
+ to a NumPy array, a ValueError is raised.
+ TypeError: If input type does not belong to the above range,
+ or the contents of a list or tuple do not share the
+ same data type, a TypeError is raised.
+ """
+ if isinstance(input_array, (list, tuple)):
+ try:
+ input_array = np.array(input_array)
+ if input_array.dtype not in self.SUPPORTED_NON_ARRAY_TYPES:
+ raise TypeError
+ except (ValueError, TypeError):
+ print(f'The input cannot be converted to'
+ f' a single-type numpy array:\n{input_array}')
+ raise
+ elif isinstance(input_array, self.SUPPORTED_NON_ARRAY_TYPES):
+ input_array = np.array(input_array)
+ array_type = type(input_array)
+ assert target_type is not None or target_array is not None, \
+ 'must specify a target'
+ if target_type is not None:
+ assert target_type in (np.ndarray, torch.Tensor), \
+ 'invalid target type'
+ if target_type == array_type:
+ return input_array
+ elif target_type == np.ndarray:
+ # default dtype is float32
+ converted_array = input_array.cpu().numpy().astype(np.float32)
+ else:
+ # default dtype is float32, device is 'cpu'
+ converted_array = torch.tensor(
+ input_array, dtype=torch.float32)
+ else:
+ assert isinstance(target_array, (np.ndarray, torch.Tensor)), \
+ 'invalid target array type'
+ if isinstance(target_array, array_type):
+ return input_array
+ elif isinstance(target_array, np.ndarray):
+ converted_array = input_array.cpu().numpy().astype(
+ target_array.dtype)
+ else:
+ converted_array = target_array.new_tensor(input_array)
+ return converted_array
+
+ def recover(self, input_array):
+ assert isinstance(input_array, (np.ndarray, torch.Tensor)), \
+ 'invalid input array type'
+ if isinstance(input_array, self.array_type):
+ return input_array
+ elif isinstance(input_array, torch.Tensor):
+ converted_array = input_array.cpu().numpy().astype(self.dtype)
+ else:
+ converted_array = torch.tensor(
+ input_array, dtype=self.dtype, device=self.device)
+ if self.is_num:
+ converted_array = converted_array.item()
+ return converted_array
diff --git a/mmdet3d/core/utils/gaussian.py b/mmdet3d/core/utils/gaussian.py
new file mode 100644
index 0000000..66ccbd9
--- /dev/null
+++ b/mmdet3d/core/utils/gaussian.py
@@ -0,0 +1,158 @@
+# Copyright (c) OpenMMLab. All rights reserved.
+import numpy as np
+import torch
+
+
+def gaussian_2d(shape, sigma=1):
+ """Generate gaussian map.
+
+ Args:
+ shape (list[int]): Shape of the map.
+ sigma (float, optional): Sigma to generate gaussian map.
+ Defaults to 1.
+
+ Returns:
+ np.ndarray: Generated gaussian map.
+ """
+ m, n = [(ss - 1.) / 2. for ss in shape]
+ y, x = np.ogrid[-m:m + 1, -n:n + 1]
+
+ h = np.exp(-(x * x + y * y) / (2 * sigma * sigma))
+ h[h < np.finfo(h.dtype).eps * h.max()] = 0
+ return h
+
+
+def draw_heatmap_gaussian(heatmap, center, radius, k=1):
+ """Get gaussian masked heatmap.
+
+ Args:
+ heatmap (torch.Tensor): Heatmap to be masked.
+ center (torch.Tensor): Center coord of the heatmap.
+ radius (int): Radius of gaussian.
+ K (int, optional): Multiple of masked_gaussian. Defaults to 1.
+
+ Returns:
+ torch.Tensor: Masked heatmap.
+ """
+ diameter = 2 * radius + 1
+ gaussian = gaussian_2d((diameter, diameter), sigma=diameter / 6)
+
+ x, y = int(center[0]), int(center[1])
+
+ height, width = heatmap.shape[0:2]
+
+ left, right = min(x, radius), min(width - x, radius + 1)
+ top, bottom = min(y, radius), min(height - y, radius + 1)
+
+ masked_heatmap = heatmap[y - top:y + bottom, x - left:x + right]
+ masked_gaussian = torch.from_numpy(
+ gaussian[radius - top:radius + bottom,
+ radius - left:radius + right]).to(heatmap.device,
+ torch.float32)
+ if min(masked_gaussian.shape) > 0 and min(masked_heatmap.shape) > 0:
+ torch.max(masked_heatmap, masked_gaussian * k, out=masked_heatmap)
+ return heatmap
+
+
+def gaussian_radius(det_size, min_overlap=0.5):
+ """Get radius of gaussian.
+
+ Args:
+ det_size (tuple[torch.Tensor]): Size of the detection result.
+ min_overlap (float, optional): Gaussian_overlap. Defaults to 0.5.
+
+ Returns:
+ torch.Tensor: Computed radius.
+ """
+ height, width = det_size
+
+ a1 = 1
+ b1 = (height + width)
+ c1 = width * height * (1 - min_overlap) / (1 + min_overlap)
+ sq1 = torch.sqrt(b1**2 - 4 * a1 * c1)
+ r1 = (b1 + sq1) / 2
+
+ a2 = 4
+ b2 = 2 * (height + width)
+ c2 = (1 - min_overlap) * width * height
+ sq2 = torch.sqrt(b2**2 - 4 * a2 * c2)
+ r2 = (b2 + sq2) / 2
+
+ a3 = 4 * min_overlap
+ b3 = -2 * min_overlap * (height + width)
+ c3 = (min_overlap - 1) * width * height
+ sq3 = torch.sqrt(b3**2 - 4 * a3 * c3)
+ r3 = (b3 + sq3) / 2
+ return min(r1, r2, r3)
+
+
+def get_ellip_gaussian_2D(heatmap, center, radius_x, radius_y, k=1):
+ """Generate 2D ellipse gaussian heatmap.
+
+ Args:
+ heatmap (Tensor): Input heatmap, the gaussian kernel will cover on
+ it and maintain the max value.
+ center (list[int]): Coord of gaussian kernel's center.
+ radius_x (int): X-axis radius of gaussian kernel.
+ radius_y (int): Y-axis radius of gaussian kernel.
+ k (int, optional): Coefficient of gaussian kernel. Default: 1.
+
+ Returns:
+ out_heatmap (Tensor): Updated heatmap covered by gaussian kernel.
+ """
+ diameter_x, diameter_y = 2 * radius_x + 1, 2 * radius_y + 1
+ gaussian_kernel = ellip_gaussian2D((radius_x, radius_y),
+ sigma_x=diameter_x / 6,
+ sigma_y=diameter_y / 6,
+ dtype=heatmap.dtype,
+ device=heatmap.device)
+
+ x, y = int(center[0]), int(center[1])
+ height, width = heatmap.shape[0:2]
+
+ left, right = min(x, radius_x), min(width - x, radius_x + 1)
+ top, bottom = min(y, radius_y), min(height - y, radius_y + 1)
+
+ masked_heatmap = heatmap[y - top:y + bottom, x - left:x + right]
+ masked_gaussian = gaussian_kernel[radius_y - top:radius_y + bottom,
+ radius_x - left:radius_x + right]
+ out_heatmap = heatmap
+ torch.max(
+ masked_heatmap,
+ masked_gaussian * k,
+ out=out_heatmap[y - top:y + bottom, x - left:x + right])
+
+ return out_heatmap
+
+
+def ellip_gaussian2D(radius,
+ sigma_x,
+ sigma_y,
+ dtype=torch.float32,
+ device='cpu'):
+ """Generate 2D ellipse gaussian kernel.
+
+ Args:
+ radius (tuple(int)): Ellipse radius (radius_x, radius_y) of gaussian
+ kernel.
+ sigma_x (int): X-axis sigma of gaussian function.
+ sigma_y (int): Y-axis sigma of gaussian function.
+ dtype (torch.dtype, optional): Dtype of gaussian tensor.
+ Default: torch.float32.
+ device (str, optional): Device of gaussian tensor.
+ Default: 'cpu'.
+
+ Returns:
+ h (Tensor): Gaussian kernel with a
+ ``(2 * radius_y + 1) * (2 * radius_x + 1)`` shape.
+ """
+ x = torch.arange(
+ -radius[0], radius[0] + 1, dtype=dtype, device=device).view(1, -1)
+ y = torch.arange(
+ -radius[1], radius[1] + 1, dtype=dtype, device=device).view(-1, 1)
+
+ h = (-(x * x) / (2 * sigma_x * sigma_x) - (y * y) /
+ (2 * sigma_y * sigma_y)).exp()
+ h[h < torch.finfo(h.dtype).eps * h.max()] = 0
+
+ return h
diff --git a/mmdet3d/core/visualizer/__init__.py b/mmdet3d/core/visualizer/__init__.py
new file mode 100644
index 0000000..bbf1e60
--- /dev/null
+++ b/mmdet3d/core/visualizer/__init__.py
@@ -0,0 +1,5 @@
+# Copyright (c) OpenMMLab. All rights reserved.
+from .show_result import (show_multi_modality_result, show_result,
+ show_seg_result)
+
+__all__ = ['show_result', 'show_seg_result', 'show_multi_modality_result']
diff --git a/mmdet3d/core/visualizer/image_vis.py b/mmdet3d/core/visualizer/image_vis.py
new file mode 100644
index 0000000..7ac765c
--- /dev/null
+++ b/mmdet3d/core/visualizer/image_vis.py
@@ -0,0 +1,206 @@
+# Copyright (c) OpenMMLab. All rights reserved.
+import copy
+
+import cv2
+import numpy as np
+import torch
+from matplotlib import pyplot as plt
+
+
+def project_pts_on_img(points,
+ raw_img,
+ lidar2img_rt,
+ max_distance=70,
+ thickness=-1):
+ """Project the 3D points cloud on 2D image.
+
+ Args:
+ points (numpy.array): 3D points cloud (x, y, z) to visualize.
+ raw_img (numpy.array): The numpy array of image.
+ lidar2img_rt (numpy.array, shape=[4, 4]): The projection matrix
+ according to the camera intrinsic parameters.
+ max_distance (float, optional): the max distance of the points cloud.
+ Default: 70.
+ thickness (int, optional): The thickness of 2D points. Default: -1.
+ """
+ img = raw_img.copy()
+ num_points = points.shape[0]
+ pts_4d = np.concatenate([points[:, :3], np.ones((num_points, 1))], axis=-1)
+ pts_2d = pts_4d @ lidar2img_rt.T
+
+ # cam_points is Tensor of Nx4 whose last column is 1
+ # transform camera coordinate to image coordinate
+ pts_2d[:, 2] = np.clip(pts_2d[:, 2], a_min=1e-5, a_max=99999)
+ pts_2d[:, 0] /= pts_2d[:, 2]
+ pts_2d[:, 1] /= pts_2d[:, 2]
+
+ fov_inds = ((pts_2d[:, 0] < img.shape[1])
+ & (pts_2d[:, 0] >= 0)
+ & (pts_2d[:, 1] < img.shape[0])
+ & (pts_2d[:, 1] >= 0))
+
+ imgfov_pts_2d = pts_2d[fov_inds, :3] # u, v, d
+
+ cmap = plt.cm.get_cmap('hsv', 256)
+ cmap = np.array([cmap(i) for i in range(256)])[:, :3] * 255
+ for i in range(imgfov_pts_2d.shape[0]):
+ depth = imgfov_pts_2d[i, 2]
+ color = cmap[np.clip(int(max_distance * 10 / depth), 0, 255), :]
+ cv2.circle(
+ img,
+ center=(int(np.round(imgfov_pts_2d[i, 0])),
+ int(np.round(imgfov_pts_2d[i, 1]))),
+ radius=1,
+ color=tuple(color),
+ thickness=thickness,
+ )
+ cv2.imshow('project_pts_img', img.astype(np.uint8))
+ cv2.waitKey(100)
+
+
+def plot_rect3d_on_img(img,
+ num_rects,
+ rect_corners,
+ color=(0, 255, 0),
+ thickness=1):
+ """Plot the boundary lines of 3D rectangular on 2D images.
+
+ Args:
+ img (numpy.array): The numpy array of image.
+ num_rects (int): Number of 3D rectangulars.
+ rect_corners (numpy.array): Coordinates of the corners of 3D
+ rectangulars. Should be in the shape of [num_rect, 8, 2].
+ color (tuple[int], optional): The color to draw bboxes.
+ Default: (0, 255, 0).
+ thickness (int, optional): The thickness of bboxes. Default: 1.
+ """
+ line_indices = ((0, 1), (0, 3), (0, 4), (1, 2), (1, 5), (3, 2), (3, 7),
+ (4, 5), (4, 7), (2, 6), (5, 6), (6, 7))
+ for i in range(num_rects):
+ corners = rect_corners[i].astype(np.int)
+ for start, end in line_indices:
+ cv2.line(img, (corners[start, 0], corners[start, 1]),
+ (corners[end, 0], corners[end, 1]), color, thickness,
+ cv2.LINE_AA)
+
+ return img.astype(np.uint8)
+
+
+def draw_lidar_bbox3d_on_img(bboxes3d,
+ raw_img,
+ lidar2img_rt,
+ img_metas,
+ color=(0, 255, 0),
+ thickness=1):
+ """Project the 3D bbox on 2D plane and draw on input image.
+
+ Args:
+ bboxes3d (:obj:`LiDARInstance3DBoxes`):
+ 3d bbox in lidar coordinate system to visualize.
+ raw_img (numpy.array): The numpy array of image.
+ lidar2img_rt (numpy.array, shape=[4, 4]): The projection matrix
+ according to the camera intrinsic parameters.
+ img_metas (dict): Useless here.
+ color (tuple[int], optional): The color to draw bboxes.
+ Default: (0, 255, 0).
+ thickness (int, optional): The thickness of bboxes. Default: 1.
+ """
+ img = raw_img.copy()
+ corners_3d = bboxes3d.corners
+ num_bbox = corners_3d.shape[0]
+ pts_4d = np.concatenate(
+ [corners_3d.reshape(-1, 3),
+ np.ones((num_bbox * 8, 1))], axis=-1)
+ lidar2img_rt = copy.deepcopy(lidar2img_rt).reshape(4, 4)
+ if isinstance(lidar2img_rt, torch.Tensor):
+ lidar2img_rt = lidar2img_rt.cpu().numpy()
+ pts_2d = pts_4d @ lidar2img_rt.T
+
+ pts_2d[:, 2] = np.clip(pts_2d[:, 2], a_min=1e-5, a_max=1e5)
+ pts_2d[:, 0] /= pts_2d[:, 2]
+ pts_2d[:, 1] /= pts_2d[:, 2]
+ imgfov_pts_2d = pts_2d[..., :2].reshape(num_bbox, 8, 2)
+
+ return plot_rect3d_on_img(img, num_bbox, imgfov_pts_2d, color, thickness)
+
+
+# TODO: remove third parameter in all functions here in favour of img_metas
+def draw_depth_bbox3d_on_img(bboxes3d,
+ raw_img,
+ calibs,
+ img_metas,
+ color=(0, 255, 0),
+ thickness=1):
+ """Project the 3D bbox on 2D plane and draw on input image.
+
+ Args:
+ bboxes3d (:obj:`DepthInstance3DBoxes`, shape=[M, 7]):
+ 3d bbox in depth coordinate system to visualize.
+ raw_img (numpy.array): The numpy array of image.
+ calibs (dict): Camera calibration information, Rt and K.
+ img_metas (dict): Used in coordinates transformation.
+ color (tuple[int], optional): The color to draw bboxes.
+ Default: (0, 255, 0).
+ thickness (int, optional): The thickness of bboxes. Default: 1.
+ """
+ from mmdet3d.core.bbox import points_cam2img
+ from mmdet3d.models import apply_3d_transformation
+
+ img = raw_img.copy()
+ img_metas = copy.deepcopy(img_metas)
+ corners_3d = bboxes3d.corners
+ num_bbox = corners_3d.shape[0]
+ points_3d = corners_3d.reshape(-1, 3)
+
+ # first reverse the data transformations
+ xyz_depth = apply_3d_transformation(
+ points_3d, 'DEPTH', img_metas, reverse=True)
+
+ # project to 2d to get image coords (uv)
+ uv_origin = points_cam2img(xyz_depth,
+ xyz_depth.new_tensor(img_metas['depth2img']))
+ uv_origin = (uv_origin - 1).round()
+ imgfov_pts_2d = uv_origin[..., :2].reshape(num_bbox, 8, 2).numpy()
+
+ return plot_rect3d_on_img(img, num_bbox, imgfov_pts_2d, color, thickness)
+
+
+def draw_camera_bbox3d_on_img(bboxes3d,
+ raw_img,
+ cam2img,
+ img_metas,
+ color=(0, 255, 0),
+ thickness=1):
+ """Project the 3D bbox on 2D plane and draw on input image.
+
+ Args:
+ bboxes3d (:obj:`CameraInstance3DBoxes`, shape=[M, 7]):
+ 3d bbox in camera coordinate system to visualize.
+ raw_img (numpy.array): The numpy array of image.
+ cam2img (dict): Camera intrinsic matrix,
+ denoted as `K` in depth bbox coordinate system.
+ img_metas (dict): Useless here.
+ color (tuple[int], optional): The color to draw bboxes.
+ Default: (0, 255, 0).
+ thickness (int, optional): The thickness of bboxes. Default: 1.
+ """
+ from mmdet3d.core.bbox import points_cam2img
+
+ img = raw_img.copy()
+ cam2img = copy.deepcopy(cam2img)
+ corners_3d = bboxes3d.corners
+ num_bbox = corners_3d.shape[0]
+ points_3d = corners_3d.reshape(-1, 3)
+ if not isinstance(cam2img, torch.Tensor):
+ cam2img = torch.from_numpy(np.array(cam2img))
+
+ assert (cam2img.shape == torch.Size([3, 3])
+ or cam2img.shape == torch.Size([4, 4]))
+ cam2img = cam2img.float().cpu()
+
+ # project to 2d to get image coords (uv)
+ uv_origin = points_cam2img(points_3d, cam2img)
+ uv_origin = (uv_origin - 1).round()
+ imgfov_pts_2d = uv_origin[..., :2].reshape(num_bbox, 8, 2).numpy()
+
+ return plot_rect3d_on_img(img, num_bbox, imgfov_pts_2d, color, thickness)
diff --git a/mmdet3d/core/visualizer/open3d_vis.py b/mmdet3d/core/visualizer/open3d_vis.py
new file mode 100644
index 0000000..c63b6ec
--- /dev/null
+++ b/mmdet3d/core/visualizer/open3d_vis.py
@@ -0,0 +1,460 @@
+# Copyright (c) OpenMMLab. All rights reserved.
+import copy
+
+import numpy as np
+import torch
+
+try:
+ import open3d as o3d
+ from open3d import geometry
+except ImportError:
+ raise ImportError(
+ 'Please run "pip install open3d" to install open3d first.')
+
+
+def _draw_points(points,
+ vis,
+ points_size=2,
+ point_color=(0.5, 0.5, 0.5),
+ mode='xyz'):
+ """Draw points on visualizer.
+
+ Args:
+ points (numpy.array | torch.tensor, shape=[N, 3+C]):
+ points to visualize.
+ vis (:obj:`open3d.visualization.Visualizer`): open3d visualizer.
+ points_size (int, optional): the size of points to show on visualizer.
+ Default: 2.
+ point_color (tuple[float], optional): the color of points.
+ Default: (0.5, 0.5, 0.5).
+ mode (str, optional): indicate type of the input points,
+ available mode ['xyz', 'xyzrgb']. Default: 'xyz'.
+
+ Returns:
+ tuple: points, color of each point.
+ """
+ vis.get_render_option().point_size = points_size # set points size
+ if isinstance(points, torch.Tensor):
+ points = points.cpu().numpy()
+
+ points = points.copy()
+ pcd = geometry.PointCloud()
+ if mode == 'xyz':
+ pcd.points = o3d.utility.Vector3dVector(points[:, :3])
+ points_colors = np.tile(np.array(point_color), (points.shape[0], 1))
+ elif mode == 'xyzrgb':
+ pcd.points = o3d.utility.Vector3dVector(points[:, :3])
+ points_colors = points[:, 3:6]
+ # normalize to [0, 1] for open3d drawing
+ if not ((points_colors >= 0.0) & (points_colors <= 1.0)).all():
+ points_colors /= 255.0
+ else:
+ raise NotImplementedError
+
+ pcd.colors = o3d.utility.Vector3dVector(points_colors)
+ vis.add_geometry(pcd)
+
+ return pcd, points_colors
+
+
+def _draw_bboxes(bbox3d,
+ vis,
+ points_colors,
+ pcd=None,
+ bbox_color=(0, 1, 0),
+ points_in_box_color=(1, 0, 0),
+ rot_axis=2,
+ center_mode='lidar_bottom',
+ mode='xyz'):
+ """Draw bbox on visualizer and change the color of points inside bbox3d.
+
+ Args:
+ bbox3d (numpy.array | torch.tensor, shape=[M, 7]):
+ 3d bbox (x, y, z, x_size, y_size, z_size, yaw) to visualize.
+ vis (:obj:`open3d.visualization.Visualizer`): open3d visualizer.
+ points_colors (numpy.array): color of each points.
+ pcd (:obj:`open3d.geometry.PointCloud`, optional): point cloud.
+ Default: None.
+ bbox_color (tuple[float], optional): the color of bbox.
+ Default: (0, 1, 0).
+ points_in_box_color (tuple[float], optional):
+ the color of points inside bbox3d. Default: (1, 0, 0).
+ rot_axis (int, optional): rotation axis of bbox. Default: 2.
+ center_mode (bool, optional): indicate the center of bbox is
+ bottom center or gravity center. available mode
+ ['lidar_bottom', 'camera_bottom']. Default: 'lidar_bottom'.
+ mode (str, optional): indicate type of the input points,
+ available mode ['xyz', 'xyzrgb']. Default: 'xyz'.
+ """
+ if isinstance(bbox3d, torch.Tensor):
+ bbox3d = bbox3d.cpu().numpy()
+ bbox3d = bbox3d.copy()
+
+ in_box_color = np.array(points_in_box_color)
+ for i in range(len(bbox3d)):
+ center = bbox3d[i, 0:3]
+ dim = bbox3d[i, 3:6]
+ yaw = np.zeros(3)
+ yaw[rot_axis] = bbox3d[i, 6]
+ rot_mat = geometry.get_rotation_matrix_from_xyz(yaw)
+
+ if center_mode == 'lidar_bottom':
+ center[rot_axis] += dim[
+ rot_axis] / 2 # bottom center to gravity center
+ elif center_mode == 'camera_bottom':
+ center[rot_axis] -= dim[
+ rot_axis] / 2 # bottom center to gravity center
+ box3d = geometry.OrientedBoundingBox(center, rot_mat, dim)
+
+ line_set = geometry.LineSet.create_from_oriented_bounding_box(box3d)
+ line_set.paint_uniform_color(bbox_color)
+ # draw bboxes on visualizer
+ vis.add_geometry(line_set)
+
+ # change the color of points which are in box
+ if pcd is not None and mode == 'xyz':
+ indices = box3d.get_point_indices_within_bounding_box(pcd.points)
+ points_colors[indices] = in_box_color
+
+ # update points colors
+ if pcd is not None:
+ pcd.colors = o3d.utility.Vector3dVector(points_colors)
+ vis.update_geometry(pcd)
+
+
+def show_pts_boxes(points,
+ bbox3d=None,
+ show=True,
+ save_path=None,
+ points_size=2,
+ point_color=(0.5, 0.5, 0.5),
+ bbox_color=(0, 1, 0),
+ points_in_box_color=(1, 0, 0),
+ rot_axis=2,
+ center_mode='lidar_bottom',
+ mode='xyz'):
+ """Draw bbox and points on visualizer.
+
+ Args:
+ points (numpy.array | torch.tensor, shape=[N, 3+C]):
+ points to visualize.
+ bbox3d (numpy.array | torch.tensor, shape=[M, 7], optional):
+ 3D bbox (x, y, z, x_size, y_size, z_size, yaw) to visualize.
+ Defaults to None.
+ show (bool, optional): whether to show the visualization results.
+ Default: True.
+ save_path (str, optional): path to save visualized results.
+ Default: None.
+ points_size (int, optional): the size of points to show on visualizer.
+ Default: 2.
+ point_color (tuple[float], optional): the color of points.
+ Default: (0.5, 0.5, 0.5).
+ bbox_color (tuple[float], optional): the color of bbox.
+ Default: (0, 1, 0).
+ points_in_box_color (tuple[float], optional):
+ the color of points which are in bbox3d. Default: (1, 0, 0).
+ rot_axis (int, optional): rotation axis of bbox. Default: 2.
+ center_mode (bool, optional): indicate the center of bbox is bottom
+ center or gravity center. available mode
+ ['lidar_bottom', 'camera_bottom']. Default: 'lidar_bottom'.
+ mode (str, optional): indicate type of the input points, available
+ mode ['xyz', 'xyzrgb']. Default: 'xyz'.
+ """
+ # TODO: support score and class info
+ assert 0 <= rot_axis <= 2
+
+ # init visualizer
+ vis = o3d.visualization.Visualizer()
+ vis.create_window()
+ mesh_frame = geometry.TriangleMesh.create_coordinate_frame(
+ size=1, origin=[0, 0, 0]) # create coordinate frame
+ vis.add_geometry(mesh_frame)
+
+ # draw points
+ pcd, points_colors = _draw_points(points, vis, points_size, point_color,
+ mode)
+
+ # draw boxes
+ if bbox3d is not None:
+ _draw_bboxes(bbox3d, vis, points_colors, pcd, bbox_color,
+ points_in_box_color, rot_axis, center_mode, mode)
+
+ if show:
+ vis.run()
+
+ if save_path is not None:
+ vis.capture_screen_image(save_path)
+
+ vis.destroy_window()
+
+
+def _draw_bboxes_ind(bbox3d,
+ vis,
+ indices,
+ points_colors,
+ pcd=None,
+ bbox_color=(0, 1, 0),
+ points_in_box_color=(1, 0, 0),
+ rot_axis=2,
+ center_mode='lidar_bottom',
+ mode='xyz'):
+ """Draw bbox on visualizer and change the color or points inside bbox3d
+ with indices.
+
+ Args:
+ bbox3d (numpy.array | torch.tensor, shape=[M, 7]):
+ 3d bbox (x, y, z, x_size, y_size, z_size, yaw) to visualize.
+ vis (:obj:`open3d.visualization.Visualizer`): open3d visualizer.
+ indices (numpy.array | torch.tensor, shape=[N, M]):
+ indicate which bbox3d that each point lies in.
+ points_colors (numpy.array): color of each points.
+ pcd (:obj:`open3d.geometry.PointCloud`, optional): point cloud.
+ Default: None.
+ bbox_color (tuple[float], optional): the color of bbox.
+ Default: (0, 1, 0).
+ points_in_box_color (tuple[float], optional):
+ the color of points which are in bbox3d. Default: (1, 0, 0).
+ rot_axis (int, optional): rotation axis of bbox. Default: 2.
+ center_mode (bool, optional): indicate the center of bbox is
+ bottom center or gravity center. available mode
+ ['lidar_bottom', 'camera_bottom']. Default: 'lidar_bottom'.
+ mode (str, optional): indicate type of the input points,
+ available mode ['xyz', 'xyzrgb']. Default: 'xyz'.
+ """
+ if isinstance(bbox3d, torch.Tensor):
+ bbox3d = bbox3d.cpu().numpy()
+ if isinstance(indices, torch.Tensor):
+ indices = indices.cpu().numpy()
+ bbox3d = bbox3d.copy()
+
+ in_box_color = np.array(points_in_box_color)
+ for i in range(len(bbox3d)):
+ center = bbox3d[i, 0:3]
+ dim = bbox3d[i, 3:6]
+ yaw = np.zeros(3)
+ # TODO: fix problem of current coordinate system
+ # dim[0], dim[1] = dim[1], dim[0] # for current coordinate
+ # yaw[rot_axis] = -(bbox3d[i, 6] - 0.5 * np.pi)
+ yaw[rot_axis] = -bbox3d[i, 6]
+ rot_mat = geometry.get_rotation_matrix_from_xyz(yaw)
+ if center_mode == 'lidar_bottom':
+ center[rot_axis] += dim[
+ rot_axis] / 2 # bottom center to gravity center
+ elif center_mode == 'camera_bottom':
+ center[rot_axis] -= dim[
+ rot_axis] / 2 # bottom center to gravity center
+ box3d = geometry.OrientedBoundingBox(center, rot_mat, dim)
+
+ line_set = geometry.LineSet.create_from_oriented_bounding_box(box3d)
+ line_set.paint_uniform_color(bbox_color)
+ # draw bboxes on visualizer
+ vis.add_geometry(line_set)
+
+ # change the color of points which are in box
+ if pcd is not None and mode == 'xyz':
+ points_colors[indices[:, i].astype(np.bool)] = in_box_color
+
+ # update points colors
+ if pcd is not None:
+ pcd.colors = o3d.utility.Vector3dVector(points_colors)
+ vis.update_geometry(pcd)
+
+
+def show_pts_index_boxes(points,
+ bbox3d=None,
+ show=True,
+ indices=None,
+ save_path=None,
+ points_size=2,
+ point_color=(0.5, 0.5, 0.5),
+ bbox_color=(0, 1, 0),
+ points_in_box_color=(1, 0, 0),
+ rot_axis=2,
+ center_mode='lidar_bottom',
+ mode='xyz'):
+ """Draw bbox and points on visualizer with indices that indicate which
+ bbox3d that each point lies in.
+
+ Args:
+ points (numpy.array | torch.tensor, shape=[N, 3+C]):
+ points to visualize.
+ bbox3d (numpy.array | torch.tensor, shape=[M, 7]):
+ 3D bbox (x, y, z, x_size, y_size, z_size, yaw) to visualize.
+ Defaults to None.
+ show (bool, optional): whether to show the visualization results.
+ Default: True.
+ indices (numpy.array | torch.tensor, shape=[N, M], optional):
+ indicate which bbox3d that each point lies in. Default: None.
+ save_path (str, optional): path to save visualized results.
+ Default: None.
+ points_size (int, optional): the size of points to show on visualizer.
+ Default: 2.
+ point_color (tuple[float], optional): the color of points.
+ Default: (0.5, 0.5, 0.5).
+ bbox_color (tuple[float], optional): the color of bbox.
+ Default: (0, 1, 0).
+ points_in_box_color (tuple[float], optional):
+ the color of points which are in bbox3d. Default: (1, 0, 0).
+ rot_axis (int, optional): rotation axis of bbox. Default: 2.
+ center_mode (bool, optional): indicate the center of bbox is
+ bottom center or gravity center. available mode
+ ['lidar_bottom', 'camera_bottom']. Default: 'lidar_bottom'.
+ mode (str, optional): indicate type of the input points,
+ available mode ['xyz', 'xyzrgb']. Default: 'xyz'.
+ """
+ # TODO: support score and class info
+ assert 0 <= rot_axis <= 2
+
+ # init visualizer
+ vis = o3d.visualization.Visualizer()
+ vis.create_window()
+ mesh_frame = geometry.TriangleMesh.create_coordinate_frame(
+ size=1, origin=[0, 0, 0]) # create coordinate frame
+ vis.add_geometry(mesh_frame)
+
+ # draw points
+ pcd, points_colors = _draw_points(points, vis, points_size, point_color,
+ mode)
+
+ # draw boxes
+ if bbox3d is not None:
+ _draw_bboxes_ind(bbox3d, vis, indices, points_colors, pcd, bbox_color,
+ points_in_box_color, rot_axis, center_mode, mode)
+
+ if show:
+ vis.run()
+
+ if save_path is not None:
+ vis.capture_screen_image(save_path)
+
+ vis.destroy_window()
+
+
+class Visualizer(object):
+ r"""Online visualizer implemented with Open3d.
+
+ Args:
+ points (numpy.array, shape=[N, 3+C]): Points to visualize. The Points
+ cloud is in mode of Coord3DMode.DEPTH (please refer to
+ core.structures.coord_3d_mode).
+ bbox3d (numpy.array, shape=[M, 7], optional): 3D bbox
+ (x, y, z, x_size, y_size, z_size, yaw) to visualize.
+ The 3D bbox is in mode of Box3DMode.DEPTH with
+ gravity_center (please refer to core.structures.box_3d_mode).
+ Default: None.
+ save_path (str, optional): path to save visualized results.
+ Default: None.
+ points_size (int, optional): the size of points to show on visualizer.
+ Default: 2.
+ point_color (tuple[float], optional): the color of points.
+ Default: (0.5, 0.5, 0.5).
+ bbox_color (tuple[float], optional): the color of bbox.
+ Default: (0, 1, 0).
+ points_in_box_color (tuple[float], optional):
+ the color of points which are in bbox3d. Default: (1, 0, 0).
+ rot_axis (int, optional): rotation axis of bbox. Default: 2.
+ center_mode (bool, optional): indicate the center of bbox is
+ bottom center or gravity center. available mode
+ ['lidar_bottom', 'camera_bottom']. Default: 'lidar_bottom'.
+ mode (str, optional): indicate type of the input points,
+ available mode ['xyz', 'xyzrgb']. Default: 'xyz'.
+ """
+
+ def __init__(self,
+ points,
+ bbox3d=None,
+ save_path=None,
+ points_size=2,
+ point_color=(0.5, 0.5, 0.5),
+ bbox_color=(0, 1, 0),
+ points_in_box_color=(1, 0, 0),
+ rot_axis=2,
+ center_mode='lidar_bottom',
+ mode='xyz'):
+ super(Visualizer, self).__init__()
+ assert 0 <= rot_axis <= 2
+
+ # init visualizer
+ self.o3d_visualizer = o3d.visualization.Visualizer()
+ self.o3d_visualizer.create_window()
+ mesh_frame = geometry.TriangleMesh.create_coordinate_frame(
+ size=1, origin=[0, 0, 0]) # create coordinate frame
+ self.o3d_visualizer.add_geometry(mesh_frame)
+
+ self.points_size = points_size
+ self.point_color = point_color
+ self.bbox_color = bbox_color
+ self.points_in_box_color = points_in_box_color
+ self.rot_axis = rot_axis
+ self.center_mode = center_mode
+ self.mode = mode
+ self.seg_num = 0
+
+ # draw points
+ if points is not None:
+ self.pcd, self.points_colors = _draw_points(
+ points, self.o3d_visualizer, points_size, point_color, mode)
+
+ # draw boxes
+ if bbox3d is not None:
+ _draw_bboxes(bbox3d, self.o3d_visualizer, self.points_colors,
+ self.pcd, bbox_color, points_in_box_color, rot_axis,
+ center_mode, mode)
+
+ def add_bboxes(self, bbox3d, bbox_color=None, points_in_box_color=None):
+ """Add bounding box to visualizer.
+
+ Args:
+ bbox3d (numpy.array, shape=[M, 7]):
+ 3D bbox (x, y, z, x_size, y_size, z_size, yaw)
+ to be visualized. The 3d bbox is in mode of
+ Box3DMode.DEPTH with gravity_center (please refer to
+ core.structures.box_3d_mode).
+ bbox_color (tuple[float]): the color of bbox. Default: None.
+ points_in_box_color (tuple[float]): the color of points which
+ are in bbox3d. Default: None.
+ """
+ if bbox_color is None:
+ bbox_color = self.bbox_color
+ if points_in_box_color is None:
+ points_in_box_color = self.points_in_box_color
+ _draw_bboxes(bbox3d, self.o3d_visualizer, self.points_colors, self.pcd,
+ bbox_color, points_in_box_color, self.rot_axis,
+ self.center_mode, self.mode)
+
+ def add_seg_mask(self, seg_mask_colors):
+ """Add segmentation mask to visualizer via per-point colorization.
+
+ Args:
+ seg_mask_colors (numpy.array, shape=[N, 6]):
+ The segmentation mask whose first 3 dims are point coordinates
+ and last 3 dims are converted colors.
+ """
+ # we can't draw the colors on existing points
+ # in case gt and pred mask would overlap
+ # instead we set a large offset along x-axis for each seg mask
+ self.seg_num += 1
+ offset = (np.array(self.pcd.points).max(0) -
+ np.array(self.pcd.points).min(0))[0] * 1.2 * self.seg_num
+ mesh_frame = geometry.TriangleMesh.create_coordinate_frame(
+ size=1, origin=[offset, 0, 0]) # create coordinate frame for seg
+ self.o3d_visualizer.add_geometry(mesh_frame)
+ seg_points = copy.deepcopy(seg_mask_colors)
+ seg_points[:, 0] += offset
+ _draw_points(
+ seg_points, self.o3d_visualizer, self.points_size, mode='xyzrgb')
+
+ def show(self, save_path=None):
+ """Visualize the points cloud.
+
+ Args:
+ save_path (str, optional): path to save image. Default: None.
+ """
+
+ self.o3d_visualizer.run()
+
+ if save_path is not None:
+ self.o3d_visualizer.capture_screen_image(save_path)
+
+ self.o3d_visualizer.destroy_window()
+ return
diff --git a/mmdet3d/core/visualizer/show_result.py b/mmdet3d/core/visualizer/show_result.py
new file mode 100644
index 0000000..aa732cf
--- /dev/null
+++ b/mmdet3d/core/visualizer/show_result.py
@@ -0,0 +1,291 @@
+# Copyright (c) OpenMMLab. All rights reserved.
+from os import path as osp
+
+import mmcv
+import numpy as np
+import trimesh
+
+from .image_vis import (draw_camera_bbox3d_on_img, draw_depth_bbox3d_on_img,
+ draw_lidar_bbox3d_on_img)
+
+
+def _write_obj(points, out_filename):
+ """Write points into ``obj`` format for meshlab visualization.
+
+ Args:
+ points (np.ndarray): Points in shape (N, dim).
+ out_filename (str): Filename to be saved.
+ """
+ N = points.shape[0]
+ fout = open(out_filename, 'w')
+ for i in range(N):
+ if points.shape[1] == 6:
+ c = points[i, 3:].astype(int)
+ fout.write(
+ 'v %f %f %f %d %d %d\n' %
+ (points[i, 0], points[i, 1], points[i, 2], c[0], c[1], c[2]))
+
+ else:
+ fout.write('v %f %f %f\n' %
+ (points[i, 0], points[i, 1], points[i, 2]))
+ fout.close()
+
+
+def _write_oriented_bbox(scene_bbox, out_filename):
+ """Export oriented (around Z axis) scene bbox to meshes.
+
+ Args:
+ scene_bbox(list[ndarray] or ndarray): xyz pos of center and
+ 3 lengths (x_size, y_size, z_size) and heading angle around Z axis.
+ Y forward, X right, Z upward. heading angle of positive X is 0,
+ heading angle of positive Y is 90 degrees.
+ out_filename(str): Filename.
+ """
+
+ def heading2rotmat(heading_angle):
+ rotmat = np.zeros((3, 3))
+ rotmat[2, 2] = 1
+ cosval = np.cos(heading_angle)
+ sinval = np.sin(heading_angle)
+ rotmat[0:2, 0:2] = np.array([[cosval, -sinval], [sinval, cosval]])
+ return rotmat
+
+ def convert_oriented_box_to_trimesh_fmt(box):
+ ctr = box[:3]
+ lengths = box[3:6]
+ trns = np.eye(4)
+ trns[0:3, 3] = ctr
+ trns[3, 3] = 1.0
+ trns[0:3, 0:3] = heading2rotmat(box[6])
+ box_trimesh_fmt = trimesh.creation.box(lengths, trns)
+ return box_trimesh_fmt
+
+ if len(scene_bbox) == 0:
+ scene_bbox = np.zeros((1, 7))
+ scene = trimesh.scene.Scene()
+ for box in scene_bbox:
+ scene.add_geometry(convert_oriented_box_to_trimesh_fmt(box))
+
+ mesh_list = trimesh.util.concatenate(scene.dump())
+ # save to obj file
+ trimesh.io.export.export_mesh(mesh_list, out_filename, file_type='obj')
+
+ return
+
+
+def show_result(points,
+ gt_bboxes,
+ pred_bboxes,
+ out_dir,
+ filename,
+ show=False,
+ snapshot=False,
+ pred_labels=None):
+ """Convert results into format that is directly readable for meshlab.
+
+ Args:
+ points (np.ndarray): Points.
+ gt_bboxes (np.ndarray): Ground truth boxes.
+ pred_bboxes (np.ndarray): Predicted boxes.
+ out_dir (str): Path of output directory
+ filename (str): Filename of the current frame.
+ show (bool, optional): Visualize the results online. Defaults to False.
+ snapshot (bool, optional): Whether to save the online results.
+ Defaults to False.
+ pred_labels (np.ndarray, optional): Predicted labels of boxes.
+ Defaults to None.
+ """
+ result_path = osp.join(out_dir, filename)
+ mmcv.mkdir_or_exist(result_path)
+
+ if show:
+ from .open3d_vis import Visualizer
+
+ vis = Visualizer(points)
+ if pred_bboxes is not None:
+ if pred_labels is None:
+ vis.add_bboxes(bbox3d=pred_bboxes)
+ else:
+ palette = np.random.randint(
+ 0, 255, size=(pred_labels.max() + 1, 3)) / 256
+ labelDict = {}
+ for j in range(len(pred_labels)):
+ i = int(pred_labels[j].numpy())
+ if labelDict.get(i) is None:
+ labelDict[i] = []
+ labelDict[i].append(pred_bboxes[j])
+ for i in labelDict:
+ vis.add_bboxes(
+ bbox3d=np.array(labelDict[i]),
+ bbox_color=palette[i],
+ points_in_box_color=palette[i])
+
+ if gt_bboxes is not None:
+ vis.add_bboxes(bbox3d=gt_bboxes, bbox_color=(0, 0, 1))
+ show_path = osp.join(result_path,
+ f'{filename}_online.png') if snapshot else None
+ vis.show(show_path)
+
+ if points is not None:
+ _write_obj(points, osp.join(result_path, f'{filename}_points.obj'))
+
+ if gt_bboxes is not None:
+ # bottom center to gravity center
+ gt_bboxes[..., 2] += gt_bboxes[..., 5] / 2
+
+ _write_oriented_bbox(gt_bboxes,
+ osp.join(result_path, f'{filename}_gt.obj'))
+
+ if pred_bboxes is not None:
+ # bottom center to gravity center
+ pred_bboxes[..., 2] += pred_bboxes[..., 5] / 2
+
+ _write_oriented_bbox(pred_bboxes,
+ osp.join(result_path, f'{filename}_pred.obj'))
+
+
+def show_seg_result(points,
+ gt_seg,
+ pred_seg,
+ out_dir,
+ filename,
+ palette,
+ ignore_index=None,
+ show=False,
+ snapshot=False):
+ """Convert results into format that is directly readable for meshlab.
+
+ Args:
+ points (np.ndarray): Points.
+ gt_seg (np.ndarray): Ground truth segmentation mask.
+ pred_seg (np.ndarray): Predicted segmentation mask.
+ out_dir (str): Path of output directory
+ filename (str): Filename of the current frame.
+ palette (np.ndarray): Mapping between class labels and colors.
+ ignore_index (int, optional): The label index to be ignored, e.g.
+ unannotated points. Defaults to None.
+ show (bool, optional): Visualize the results online. Defaults to False.
+ snapshot (bool, optional): Whether to save the online results.
+ Defaults to False.
+ """
+ # we need 3D coordinates to visualize segmentation mask
+ if gt_seg is not None or pred_seg is not None:
+ assert points is not None, \
+ '3D coordinates are required for segmentation visualization'
+
+ # filter out ignored points
+ if gt_seg is not None and ignore_index is not None:
+ if points is not None:
+ points = points[gt_seg != ignore_index]
+ if pred_seg is not None:
+ pred_seg = pred_seg[gt_seg != ignore_index]
+ gt_seg = gt_seg[gt_seg != ignore_index]
+
+ if gt_seg is not None:
+ gt_seg_color = palette[gt_seg]
+ gt_seg_color = np.concatenate([points[:, :3], gt_seg_color], axis=1)
+ if pred_seg is not None:
+ pred_seg_color = palette[pred_seg]
+ pred_seg_color = np.concatenate([points[:, :3], pred_seg_color],
+ axis=1)
+
+ result_path = osp.join(out_dir, filename)
+ mmcv.mkdir_or_exist(result_path)
+
+ # online visualization of segmentation mask
+ # we show three masks in a row, scene_points, gt_mask, pred_mask
+ if show:
+ from .open3d_vis import Visualizer
+ mode = 'xyzrgb' if points.shape[1] == 6 else 'xyz'
+ vis = Visualizer(points, mode=mode)
+ if gt_seg is not None:
+ vis.add_seg_mask(gt_seg_color)
+ if pred_seg is not None:
+ vis.add_seg_mask(pred_seg_color)
+ show_path = osp.join(result_path,
+ f'{filename}_online.png') if snapshot else None
+ vis.show(show_path)
+
+ if points is not None:
+ _write_obj(points, osp.join(result_path, f'{filename}_points.obj'))
+
+ if gt_seg is not None:
+ _write_obj(gt_seg_color, osp.join(result_path, f'{filename}_gt.obj'))
+
+ if pred_seg is not None:
+ _write_obj(pred_seg_color, osp.join(result_path,
+ f'{filename}_pred.obj'))
+
+
+def show_multi_modality_result(img,
+ gt_bboxes,
+ pred_bboxes,
+ proj_mat,
+ out_dir,
+ filename,
+ box_mode='lidar',
+ img_metas=None,
+ show=False,
+ gt_bbox_color=(61, 102, 255),
+ pred_bbox_color=(241, 101, 72)):
+ """Convert multi-modality detection results into 2D results.
+
+ Project the predicted 3D bbox to 2D image plane and visualize them.
+
+ Args:
+ img (np.ndarray): The numpy array of image in cv2 fashion.
+ gt_bboxes (:obj:`BaseInstance3DBoxes`): Ground truth boxes.
+ pred_bboxes (:obj:`BaseInstance3DBoxes`): Predicted boxes.
+ proj_mat (numpy.array, shape=[4, 4]): The projection matrix
+ according to the camera intrinsic parameters.
+ out_dir (str): Path of output directory.
+ filename (str): Filename of the current frame.
+ box_mode (str, optional): Coordinate system the boxes are in.
+ Should be one of 'depth', 'lidar' and 'camera'.
+ Defaults to 'lidar'.
+ img_metas (dict, optional): Used in projecting depth bbox.
+ Defaults to None.
+ show (bool, optional): Visualize the results online. Defaults to False.
+ gt_bbox_color (str or tuple(int), optional): Color of bbox lines.
+ The tuple of color should be in BGR order. Default: (255, 102, 61).
+ pred_bbox_color (str or tuple(int), optional): Color of bbox lines.
+ The tuple of color should be in BGR order. Default: (72, 101, 241).
+ """
+ if box_mode == 'depth':
+ draw_bbox = draw_depth_bbox3d_on_img
+ elif box_mode == 'lidar':
+ draw_bbox = draw_lidar_bbox3d_on_img
+ elif box_mode == 'camera':
+ draw_bbox = draw_camera_bbox3d_on_img
+ else:
+ raise NotImplementedError(f'unsupported box mode {box_mode}')
+
+ result_path = osp.join(out_dir, filename)
+ mmcv.mkdir_or_exist(result_path)
+
+ if show:
+ show_img = img.copy()
+ if gt_bboxes is not None:
+ show_img = draw_bbox(
+ gt_bboxes, show_img, proj_mat, img_metas, color=gt_bbox_color)
+ if pred_bboxes is not None:
+ show_img = draw_bbox(
+ pred_bboxes,
+ show_img,
+ proj_mat,
+ img_metas,
+ color=pred_bbox_color)
+ mmcv.imshow(show_img, win_name='project_bbox3d_img', wait_time=0)
+
+ if img is not None:
+ mmcv.imwrite(img, osp.join(result_path, f'{filename}_img.png'))
+
+ if gt_bboxes is not None:
+ gt_img = draw_bbox(
+ gt_bboxes, img, proj_mat, img_metas, color=gt_bbox_color)
+ mmcv.imwrite(gt_img, osp.join(result_path, f'{filename}_gt.png'))
+
+ if pred_bboxes is not None:
+ pred_img = draw_bbox(
+ pred_bboxes, img, proj_mat, img_metas, color=pred_bbox_color)
+ mmcv.imwrite(pred_img, osp.join(result_path, f'{filename}_pred.png'))
diff --git a/mmdet3d/core/voxel/__init__.py b/mmdet3d/core/voxel/__init__.py
new file mode 100644
index 0000000..8d69543
--- /dev/null
+++ b/mmdet3d/core/voxel/__init__.py
@@ -0,0 +1,5 @@
+# Copyright (c) OpenMMLab. All rights reserved.
+from .builder import build_voxel_generator
+from .voxel_generator import VoxelGenerator
+
+__all__ = ['build_voxel_generator', 'VoxelGenerator']
diff --git a/mmdet3d/core/voxel/builder.py b/mmdet3d/core/voxel/builder.py
new file mode 100644
index 0000000..bc663ee
--- /dev/null
+++ b/mmdet3d/core/voxel/builder.py
@@ -0,0 +1,16 @@
+# Copyright (c) OpenMMLab. All rights reserved.
+import mmcv
+
+from . import voxel_generator
+
+
+def build_voxel_generator(cfg, **kwargs):
+ """Builder of voxel generator."""
+ if isinstance(cfg, voxel_generator.VoxelGenerator):
+ return cfg
+ elif isinstance(cfg, dict):
+ return mmcv.runner.obj_from_dict(
+ cfg, voxel_generator, default_args=kwargs)
+ else:
+ raise TypeError('Invalid type {} for building a sampler'.format(
+ type(cfg)))
diff --git a/mmdet3d/core/voxel/voxel_generator.py b/mmdet3d/core/voxel/voxel_generator.py
new file mode 100644
index 0000000..404f2cd
--- /dev/null
+++ b/mmdet3d/core/voxel/voxel_generator.py
@@ -0,0 +1,280 @@
+# Copyright (c) OpenMMLab. All rights reserved.
+import numba
+import numpy as np
+
+
+class VoxelGenerator(object):
+ """Voxel generator in numpy implementation.
+
+ Args:
+ voxel_size (list[float]): Size of a single voxel
+ point_cloud_range (list[float]): Range of points
+ max_num_points (int): Maximum number of points in a single voxel
+ max_voxels (int, optional): Maximum number of voxels.
+ Defaults to 20000.
+ """
+
+ def __init__(self,
+ voxel_size,
+ point_cloud_range,
+ max_num_points,
+ max_voxels=20000):
+
+ point_cloud_range = np.array(point_cloud_range, dtype=np.float32)
+ # [0, -40, -3, 70.4, 40, 1]
+ voxel_size = np.array(voxel_size, dtype=np.float32)
+ grid_size = (point_cloud_range[3:] -
+ point_cloud_range[:3]) / voxel_size
+ grid_size = np.round(grid_size).astype(np.int64)
+
+ self._voxel_size = voxel_size
+ self._point_cloud_range = point_cloud_range
+ self._max_num_points = max_num_points
+ self._max_voxels = max_voxels
+ self._grid_size = grid_size
+
+ def generate(self, points):
+ """Generate voxels given points."""
+ return points_to_voxel(points, self._voxel_size,
+ self._point_cloud_range, self._max_num_points,
+ True, self._max_voxels)
+
+ @property
+ def voxel_size(self):
+ """list[float]: Size of a single voxel."""
+ return self._voxel_size
+
+ @property
+ def max_num_points_per_voxel(self):
+ """int: Maximum number of points per voxel."""
+ return self._max_num_points
+
+ @property
+ def point_cloud_range(self):
+ """list[float]: Range of point cloud."""
+ return self._point_cloud_range
+
+ @property
+ def grid_size(self):
+ """np.ndarray: The size of grids."""
+ return self._grid_size
+
+ def __repr__(self):
+ """str: Return a string that describes the module."""
+ repr_str = self.__class__.__name__
+ indent = ' ' * (len(repr_str) + 1)
+ repr_str += f'(voxel_size={self._voxel_size},\n'
+ repr_str += indent + 'point_cloud_range='
+ repr_str += f'{self._point_cloud_range.tolist()},\n'
+ repr_str += indent + f'max_num_points={self._max_num_points},\n'
+ repr_str += indent + f'max_voxels={self._max_voxels},\n'
+ repr_str += indent + f'grid_size={self._grid_size.tolist()}'
+ repr_str += ')'
+ return repr_str
+
+
+def points_to_voxel(points,
+ voxel_size,
+ coors_range,
+ max_points=35,
+ reverse_index=True,
+ max_voxels=20000):
+ """convert kitti points(N, >=3) to voxels.
+
+ Args:
+ points (np.ndarray): [N, ndim]. points[:, :3] contain xyz points and
+ points[:, 3:] contain other information such as reflectivity.
+ voxel_size (list, tuple, np.ndarray): [3] xyz, indicate voxel size
+ coors_range (list[float | tuple[float] | ndarray]): Voxel range.
+ format: xyzxyz, minmax
+ max_points (int): Indicate maximum points contained in a voxel.
+ reverse_index (bool): Whether return reversed coordinates.
+ if points has xyz format and reverse_index is True, output
+ coordinates will be zyx format, but points in features always
+ xyz format.
+ max_voxels (int): Maximum number of voxels this function creates.
+ For second, 20000 is a good choice. Points should be shuffled for
+ randomness before this function because max_voxels drops points.
+
+ Returns:
+ tuple[np.ndarray]:
+ voxels: [M, max_points, ndim] float tensor. only contain points.
+ coordinates: [M, 3] int32 tensor.
+ num_points_per_voxel: [M] int32 tensor.
+ """
+ if not isinstance(voxel_size, np.ndarray):
+ voxel_size = np.array(voxel_size, dtype=points.dtype)
+ if not isinstance(coors_range, np.ndarray):
+ coors_range = np.array(coors_range, dtype=points.dtype)
+ voxelmap_shape = (coors_range[3:] - coors_range[:3]) / voxel_size
+ voxelmap_shape = tuple(np.round(voxelmap_shape).astype(np.int32).tolist())
+ if reverse_index:
+ voxelmap_shape = voxelmap_shape[::-1]
+ # don't create large array in jit(nopython=True) code.
+ num_points_per_voxel = np.zeros(shape=(max_voxels, ), dtype=np.int32)
+ coor_to_voxelidx = -np.ones(shape=voxelmap_shape, dtype=np.int32)
+ voxels = np.zeros(
+ shape=(max_voxels, max_points, points.shape[-1]), dtype=points.dtype)
+ coors = np.zeros(shape=(max_voxels, 3), dtype=np.int32)
+ if reverse_index:
+ voxel_num = _points_to_voxel_reverse_kernel(
+ points, voxel_size, coors_range, num_points_per_voxel,
+ coor_to_voxelidx, voxels, coors, max_points, max_voxels)
+
+ else:
+ voxel_num = _points_to_voxel_kernel(points, voxel_size, coors_range,
+ num_points_per_voxel,
+ coor_to_voxelidx, voxels, coors,
+ max_points, max_voxels)
+
+ coors = coors[:voxel_num]
+ voxels = voxels[:voxel_num]
+ num_points_per_voxel = num_points_per_voxel[:voxel_num]
+
+ return voxels, coors, num_points_per_voxel
+
+
+@numba.jit(nopython=True)
+def _points_to_voxel_reverse_kernel(points,
+ voxel_size,
+ coors_range,
+ num_points_per_voxel,
+ coor_to_voxelidx,
+ voxels,
+ coors,
+ max_points=35,
+ max_voxels=20000):
+ """convert kitti points(N, >=3) to voxels.
+
+ Args:
+ points (np.ndarray): [N, ndim]. points[:, :3] contain xyz points and
+ points[:, 3:] contain other information such as reflectivity.
+ voxel_size (list, tuple, np.ndarray): [3] xyz, indicate voxel size
+ coors_range (list[float | tuple[float] | ndarray]): Range of voxels.
+ format: xyzxyz, minmax
+ num_points_per_voxel (int): Number of points per voxel.
+ coor_to_voxel_idx (np.ndarray): A voxel grid of shape (D, H, W),
+ which has the same shape as the complete voxel map. It indicates
+ the index of each corresponding voxel.
+ voxels (np.ndarray): Created empty voxels.
+ coors (np.ndarray): Created coordinates of each voxel.
+ max_points (int): Indicate maximum points contained in a voxel.
+ max_voxels (int): Maximum number of voxels this function create.
+ for second, 20000 is a good choice. Points should be shuffled for
+ randomness before this function because max_voxels drops points.
+
+ Returns:
+ tuple[np.ndarray]:
+ voxels: Shape [M, max_points, ndim], only contain points.
+ coordinates: Shape [M, 3].
+ num_points_per_voxel: Shape [M].
+ """
+ # put all computations to one loop.
+ # we shouldn't create large array in main jit code, otherwise
+ # reduce performance
+ N = points.shape[0]
+ # ndim = points.shape[1] - 1
+ ndim = 3
+ ndim_minus_1 = ndim - 1
+ grid_size = (coors_range[3:] - coors_range[:3]) / voxel_size
+ # np.round(grid_size)
+ # grid_size = np.round(grid_size).astype(np.int64)(np.int32)
+ grid_size = np.round(grid_size, 0, grid_size).astype(np.int32)
+ coor = np.zeros(shape=(3, ), dtype=np.int32)
+ voxel_num = 0
+ failed = False
+ for i in range(N):
+ failed = False
+ for j in range(ndim):
+ c = np.floor((points[i, j] - coors_range[j]) / voxel_size[j])
+ if c < 0 or c >= grid_size[j]:
+ failed = True
+ break
+ coor[ndim_minus_1 - j] = c
+ if failed:
+ continue
+ voxelidx = coor_to_voxelidx[coor[0], coor[1], coor[2]]
+ if voxelidx == -1:
+ voxelidx = voxel_num
+ if voxel_num >= max_voxels:
+ continue
+ voxel_num += 1
+ coor_to_voxelidx[coor[0], coor[1], coor[2]] = voxelidx
+ coors[voxelidx] = coor
+ num = num_points_per_voxel[voxelidx]
+ if num < max_points:
+ voxels[voxelidx, num] = points[i]
+ num_points_per_voxel[voxelidx] += 1
+ return voxel_num
+
+
+@numba.jit(nopython=True)
+def _points_to_voxel_kernel(points,
+ voxel_size,
+ coors_range,
+ num_points_per_voxel,
+ coor_to_voxelidx,
+ voxels,
+ coors,
+ max_points=35,
+ max_voxels=20000):
+ """convert kitti points(N, >=3) to voxels.
+
+ Args:
+ points (np.ndarray): [N, ndim]. points[:, :3] contain xyz points and
+ points[:, 3:] contain other information such as reflectivity.
+ voxel_size (list, tuple, np.ndarray): [3] xyz, indicate voxel size.
+ coors_range (list[float | tuple[float] | ndarray]): Range of voxels.
+ format: xyzxyz, minmax
+ num_points_per_voxel (int): Number of points per voxel.
+ coor_to_voxel_idx (np.ndarray): A voxel grid of shape (D, H, W),
+ which has the same shape as the complete voxel map. It indicates
+ the index of each corresponding voxel.
+ voxels (np.ndarray): Created empty voxels.
+ coors (np.ndarray): Created coordinates of each voxel.
+ max_points (int): Indicate maximum points contained in a voxel.
+ max_voxels (int): Maximum number of voxels this function create.
+ for second, 20000 is a good choice. Points should be shuffled for
+ randomness before this function because max_voxels drops points.
+
+ Returns:
+ tuple[np.ndarray]:
+ voxels: Shape [M, max_points, ndim], only contain points.
+ coordinates: Shape [M, 3].
+ num_points_per_voxel: Shape [M].
+ """
+ N = points.shape[0]
+ # ndim = points.shape[1] - 1
+ ndim = 3
+ grid_size = (coors_range[3:] - coors_range[:3]) / voxel_size
+ # grid_size = np.round(grid_size).astype(np.int64)(np.int32)
+ grid_size = np.round(grid_size, 0, grid_size).astype(np.int32)
+
+ # lower_bound = coors_range[:3]
+ # upper_bound = coors_range[3:]
+ coor = np.zeros(shape=(3, ), dtype=np.int32)
+ voxel_num = 0
+ failed = False
+ for i in range(N):
+ failed = False
+ for j in range(ndim):
+ c = np.floor((points[i, j] - coors_range[j]) / voxel_size[j])
+ if c < 0 or c >= grid_size[j]:
+ failed = True
+ break
+ coor[j] = c
+ if failed:
+ continue
+ voxelidx = coor_to_voxelidx[coor[0], coor[1], coor[2]]
+ if voxelidx == -1:
+ voxelidx = voxel_num
+ if voxel_num >= max_voxels:
+ continue
+ voxel_num += 1
+ coor_to_voxelidx[coor[0], coor[1], coor[2]] = voxelidx
+ coors[voxelidx] = coor
+ num = num_points_per_voxel[voxelidx]
+ if num < max_points:
+ voxels[voxelidx, num] = points[i]
+ num_points_per_voxel[voxelidx] += 1
+ return voxel_num
diff --git a/mmdet3d/datasets/__init__.py b/mmdet3d/datasets/__init__.py
new file mode 100644
index 0000000..c0c6534
--- /dev/null
+++ b/mmdet3d/datasets/__init__.py
@@ -0,0 +1,45 @@
+# Copyright (c) OpenMMLab. All rights reserved.
+from mmdet.datasets.builder import build_dataloader
+from .builder import DATASETS, PIPELINES, build_dataset
+from .custom_3d import Custom3DDataset
+from .custom_3d_seg import Custom3DSegDataset
+from .kitti_dataset import KittiDataset
+from .kitti_mono_dataset import KittiMonoDataset
+from .lyft_dataset import LyftDataset
+from .nuscenes_dataset import NuScenesDataset
+from .nuscenes_mono_dataset import NuScenesMonoDataset
+# yapf: disable
+from .pipelines import (AffineResize, BackgroundPointsFilter, GlobalAlignment,
+ GlobalRotScaleTrans, IndoorPatchPointSample,
+ IndoorPointSample, LoadAnnotations3D,
+ LoadPointsFromDict, LoadPointsFromFile,
+ LoadPointsFromMultiSweeps, NormalizePointsColor,
+ ObjectNameFilter, ObjectNoise, ObjectRangeFilter,
+ ObjectSample, PointSample, PointShuffle,
+ PointsRangeFilter, RandomDropPointsColor, RandomFlip3D,
+ RandomJitterPoints, RandomShiftScale,
+ VoxelBasedPointSampler)
+# yapf: enable
+from .s3dis_dataset import S3DISDataset, S3DISSegDataset, S3DISInstanceSegDataset
+from .scannet_dataset import (ScanNetDataset, ScanNetInstanceSegDataset,
+ ScanNetSegDataset, ScanNetInstanceSegV2Dataset)
+from .semantickitti_dataset import SemanticKITTIDataset
+from .sunrgbd_dataset import SUNRGBDDataset
+from .utils import get_loading_pipeline
+from .waymo_dataset import WaymoDataset
+
+__all__ = [
+ 'KittiDataset', 'KittiMonoDataset', 'build_dataloader', 'DATASETS',
+ 'build_dataset', 'NuScenesDataset', 'NuScenesMonoDataset', 'LyftDataset',
+ 'ObjectSample', 'RandomFlip3D', 'ObjectNoise', 'GlobalRotScaleTrans',
+ 'PointShuffle', 'ObjectRangeFilter', 'PointsRangeFilter',
+ 'LoadPointsFromFile', 'S3DISSegDataset', 'S3DISDataset',
+ 'NormalizePointsColor', 'IndoorPatchPointSample', 'IndoorPointSample',
+ 'PointSample', 'LoadAnnotations3D', 'GlobalAlignment', 'SUNRGBDDataset',
+ 'ScanNetDataset', 'ScanNetSegDataset', 'ScanNetInstanceSegDataset',
+ 'SemanticKITTIDataset', 'Custom3DDataset', 'Custom3DSegDataset',
+ 'LoadPointsFromMultiSweeps', 'WaymoDataset', 'BackgroundPointsFilter',
+ 'VoxelBasedPointSampler', 'get_loading_pipeline', 'RandomDropPointsColor',
+ 'RandomJitterPoints', 'ObjectNameFilter', 'AffineResize',
+ 'RandomShiftScale', 'LoadPointsFromDict', 'PIPELINES'
+]
diff --git a/mmdet3d/datasets/builder.py b/mmdet3d/datasets/builder.py
new file mode 100644
index 0000000..157f640
--- /dev/null
+++ b/mmdet3d/datasets/builder.py
@@ -0,0 +1,47 @@
+# Copyright (c) OpenMMLab. All rights reserved.
+import platform
+
+from mmcv.utils import Registry, build_from_cfg
+
+from mmdet.datasets import DATASETS as MMDET_DATASETS
+from mmdet.datasets.builder import _concat_dataset
+
+if platform.system() != 'Windows':
+ # https://github.com/pytorch/pytorch/issues/973
+ import resource
+ rlimit = resource.getrlimit(resource.RLIMIT_NOFILE)
+ base_soft_limit = rlimit[0]
+ hard_limit = rlimit[1]
+ soft_limit = min(max(4096, base_soft_limit), hard_limit)
+ resource.setrlimit(resource.RLIMIT_NOFILE, (soft_limit, hard_limit))
+
+OBJECTSAMPLERS = Registry('Object sampler')
+DATASETS = Registry('dataset')
+PIPELINES = Registry('pipeline')
+
+
+def build_dataset(cfg, default_args=None):
+ from mmdet3d.datasets.dataset_wrappers import CBGSDataset
+ from mmdet.datasets.dataset_wrappers import (ClassBalancedDataset,
+ ConcatDataset, RepeatDataset)
+ if isinstance(cfg, (list, tuple)):
+ dataset = ConcatDataset([build_dataset(c, default_args) for c in cfg])
+ elif cfg['type'] == 'ConcatDataset':
+ dataset = ConcatDataset(
+ [build_dataset(c, default_args) for c in cfg['datasets']],
+ cfg.get('separate_eval', True))
+ elif cfg['type'] == 'RepeatDataset':
+ dataset = RepeatDataset(
+ build_dataset(cfg['dataset'], default_args), cfg['times'])
+ elif cfg['type'] == 'ClassBalancedDataset':
+ dataset = ClassBalancedDataset(
+ build_dataset(cfg['dataset'], default_args), cfg['oversample_thr'])
+ elif cfg['type'] == 'CBGSDataset':
+ dataset = CBGSDataset(build_dataset(cfg['dataset'], default_args))
+ elif isinstance(cfg.get('ann_file'), (list, tuple)):
+ dataset = _concat_dataset(cfg, default_args)
+ elif cfg['type'] in DATASETS._module_dict.keys():
+ dataset = build_from_cfg(cfg, DATASETS, default_args)
+ else:
+ dataset = build_from_cfg(cfg, MMDET_DATASETS, default_args)
+ return dataset
diff --git a/mmdet3d/datasets/custom_3d.py b/mmdet3d/datasets/custom_3d.py
new file mode 100644
index 0000000..9c6e351
--- /dev/null
+++ b/mmdet3d/datasets/custom_3d.py
@@ -0,0 +1,448 @@
+# Copyright (c) OpenMMLab. All rights reserved.
+import tempfile
+import warnings
+from os import path as osp
+
+import mmcv
+import numpy as np
+from torch.utils.data import Dataset
+
+from ..core.bbox import get_box_type
+from .builder import DATASETS
+from .pipelines import Compose
+from .utils import extract_result_dict, get_loading_pipeline
+
+
+@DATASETS.register_module()
+class Custom3DDataset(Dataset):
+ """Customized 3D dataset.
+
+ This is the base dataset of SUNRGB-D, ScanNet, nuScenes, and KITTI
+ dataset.
+
+ .. code-block:: none
+
+ [
+ {'sample_idx':
+ 'lidar_points': {'lidar_path': velodyne_path,
+ ....
+ },
+ 'annos': {'box_type_3d': (str) 'LiDAR/Camera/Depth'
+ 'gt_bboxes_3d': (n, 7)
+ 'gt_names': [list]
+ ....
+ }
+ 'calib': { .....}
+ 'images': { .....}
+ }
+ ]
+
+ Args:
+ data_root (str): Path of dataset root.
+ ann_file (str): Path of annotation file.
+ pipeline (list[dict], optional): Pipeline used for data processing.
+ Defaults to None.
+ classes (tuple[str], optional): Classes used in the dataset.
+ Defaults to None.
+ modality (dict, optional): Modality to specify the sensor data used
+ as input. Defaults to None.
+ box_type_3d (str, optional): Type of 3D box of this dataset.
+ Based on the `box_type_3d`, the dataset will encapsulate the box
+ to its original format then converted them to `box_type_3d`.
+ Defaults to 'LiDAR'. Available options includes
+
+ - 'LiDAR': Box in LiDAR coordinates.
+ - 'Depth': Box in depth coordinates, usually for indoor dataset.
+ - 'Camera': Box in camera coordinates.
+ filter_empty_gt (bool, optional): Whether to filter empty GT.
+ Defaults to True.
+ test_mode (bool, optional): Whether the dataset is in test mode.
+ Defaults to False.
+ """
+
+ def __init__(self,
+ data_root,
+ ann_file,
+ pipeline=None,
+ classes=None,
+ modality=None,
+ box_type_3d='LiDAR',
+ filter_empty_gt=True,
+ test_mode=False,
+ file_client_args=dict(backend='disk')):
+ super().__init__()
+ self.data_root = data_root
+ self.ann_file = ann_file
+ self.test_mode = test_mode
+ self.modality = modality
+ self.filter_empty_gt = filter_empty_gt
+ self.box_type_3d, self.box_mode_3d = get_box_type(box_type_3d)
+
+ self.CLASSES = self.get_classes(classes)
+ self.file_client = mmcv.FileClient(**file_client_args)
+ self.cat2id = {name: i for i, name in enumerate(self.CLASSES)}
+
+ # load annotations
+ if hasattr(self.file_client, 'get_local_path'):
+ with self.file_client.get_local_path(self.ann_file) as local_path:
+ self.data_infos = self.load_annotations(open(local_path, 'rb'))
+ else:
+ warnings.warn(
+ 'The used MMCV version does not have get_local_path. '
+ f'We treat the {self.ann_file} as local paths and it '
+ 'might cause errors if the path is not a local path. '
+ 'Please use MMCV>= 1.3.16 if you meet errors.')
+ self.data_infos = self.load_annotations(self.ann_file)
+
+ # process pipeline
+ if pipeline is not None:
+ self.pipeline = Compose(pipeline)
+
+ # set group flag for the samplers
+ if not self.test_mode:
+ self._set_group_flag()
+
+ def load_annotations(self, ann_file):
+ """Load annotations from ann_file.
+
+ Args:
+ ann_file (str): Path of the annotation file.
+
+ Returns:
+ list[dict]: List of annotations.
+ """
+ # loading data from a file-like object needs file format
+ return mmcv.load(ann_file, file_format='pkl')
+
+ def get_data_info(self, index):
+ """Get data info according to the given index.
+
+ Args:
+ index (int): Index of the sample data to get.
+
+ Returns:
+ dict: Data information that will be passed to the data
+ preprocessing pipelines. It includes the following keys:
+
+ - sample_idx (str): Sample index.
+ - pts_filename (str): Filename of point clouds.
+ - file_name (str): Filename of point clouds.
+ - ann_info (dict): Annotation info.
+ """
+ info = self.data_infos[index]
+ sample_idx = info['sample_idx']
+ pts_filename = osp.join(self.data_root,
+ info['lidar_points']['lidar_path'])
+
+ input_dict = dict(
+ pts_filename=pts_filename,
+ sample_idx=sample_idx,
+ file_name=pts_filename)
+
+ if not self.test_mode:
+ annos = self.get_ann_info(index)
+ input_dict['ann_info'] = annos
+ if self.filter_empty_gt and ~(annos['gt_labels_3d'] != -1).any():
+ return None
+ return input_dict
+
+ def get_ann_info(self, index):
+ """Get annotation info according to the given index.
+
+ Args:
+ index (int): Index of the annotation data to get.
+
+ Returns:
+ dict: Annotation information consists of the following keys:
+
+ - gt_bboxes_3d (:obj:`LiDARInstance3DBoxes`):
+ 3D ground truth bboxes
+ - gt_labels_3d (np.ndarray): Labels of ground truths.
+ - gt_names (list[str]): Class names of ground truths.
+ """
+ info = self.data_infos[index]
+ gt_bboxes_3d = info['annos']['gt_bboxes_3d']
+ gt_names_3d = info['annos']['gt_names']
+ gt_labels_3d = []
+ for cat in gt_names_3d:
+ if cat in self.CLASSES:
+ gt_labels_3d.append(self.CLASSES.index(cat))
+ else:
+ gt_labels_3d.append(-1)
+ gt_labels_3d = np.array(gt_labels_3d)
+
+ # Obtain original box 3d type in info file
+ ori_box_type_3d = info['annos']['box_type_3d']
+ ori_box_type_3d, _ = get_box_type(ori_box_type_3d)
+
+ # turn original box type to target box type
+ gt_bboxes_3d = ori_box_type_3d(
+ gt_bboxes_3d,
+ box_dim=gt_bboxes_3d.shape[-1],
+ origin=(0.5, 0.5, 0.5)).convert_to(self.box_mode_3d)
+
+ anns_results = dict(
+ gt_bboxes_3d=gt_bboxes_3d,
+ gt_labels_3d=gt_labels_3d,
+ gt_names=gt_names_3d)
+ return anns_results
+
+ def pre_pipeline(self, results):
+ """Initialization before data preparation.
+
+ Args:
+ results (dict): Dict before data preprocessing.
+
+ - img_fields (list): Image fields.
+ - bbox3d_fields (list): 3D bounding boxes fields.
+ - pts_mask_fields (list): Mask fields of points.
+ - pts_seg_fields (list): Mask fields of point segments.
+ - bbox_fields (list): Fields of bounding boxes.
+ - mask_fields (list): Fields of masks.
+ - seg_fields (list): Segment fields.
+ - box_type_3d (str): 3D box type.
+ - box_mode_3d (str): 3D box mode.
+ """
+ results['img_fields'] = []
+ results['bbox3d_fields'] = []
+ results['pts_mask_fields'] = []
+ results['pts_seg_fields'] = []
+ results['bbox_fields'] = []
+ results['mask_fields'] = []
+ results['seg_fields'] = []
+ results['box_type_3d'] = self.box_type_3d
+ results['box_mode_3d'] = self.box_mode_3d
+
+ def prepare_train_data(self, index):
+ """Training data preparation.
+
+ Args:
+ index (int): Index for accessing the target data.
+
+ Returns:
+ dict: Training data dict of the corresponding index.
+ """
+ input_dict = self.get_data_info(index)
+ if input_dict is None:
+ return None
+ self.pre_pipeline(input_dict)
+ example = self.pipeline(input_dict)
+ if self.filter_empty_gt and \
+ (example is None or
+ ~(example['gt_labels_3d']._data != -1).any()):
+ return None
+ return example
+
+ def prepare_test_data(self, index):
+ """Prepare data for testing.
+
+ Args:
+ index (int): Index for accessing the target data.
+
+ Returns:
+ dict: Testing data dict of the corresponding index.
+ """
+ input_dict = self.get_data_info(index)
+ self.pre_pipeline(input_dict)
+ example = self.pipeline(input_dict)
+ return example
+
+ @classmethod
+ def get_classes(cls, classes=None):
+ """Get class names of current dataset.
+
+ Args:
+ classes (Sequence[str] | str): If classes is None, use
+ default CLASSES defined by builtin dataset. If classes is a
+ string, take it as a file name. The file contains the name of
+ classes where each line contains one class name. If classes is
+ a tuple or list, override the CLASSES defined by the dataset.
+
+ Return:
+ list[str]: A list of class names.
+ """
+ if classes is None:
+ return cls.CLASSES
+
+ if isinstance(classes, str):
+ # take it as a file path
+ class_names = mmcv.list_from_file(classes)
+ elif isinstance(classes, (tuple, list)):
+ class_names = classes
+ else:
+ raise ValueError(f'Unsupported type {type(classes)} of classes.')
+
+ return class_names
+
+ def format_results(self,
+ outputs,
+ pklfile_prefix=None,
+ submission_prefix=None):
+ """Format the results to pkl file.
+
+ Args:
+ outputs (list[dict]): Testing results of the dataset.
+ pklfile_prefix (str): The prefix of pkl files. It includes
+ the file path and the prefix of filename, e.g., "a/b/prefix".
+ If not specified, a temp file will be created. Default: None.
+
+ Returns:
+ tuple: (outputs, tmp_dir), outputs is the detection results,
+ tmp_dir is the temporal directory created for saving json
+ files when ``jsonfile_prefix`` is not specified.
+ """
+ if pklfile_prefix is None:
+ tmp_dir = tempfile.TemporaryDirectory()
+ pklfile_prefix = osp.join(tmp_dir.name, 'results')
+ out = f'{pklfile_prefix}.pkl'
+ mmcv.dump(outputs, out)
+ return outputs, tmp_dir
+
+ def evaluate(self,
+ results,
+ metric=None,
+ iou_thr=(0.25, 0.5),
+ logger=None,
+ show=False,
+ out_dir=None,
+ pipeline=None):
+ """Evaluate.
+
+ Evaluation in indoor protocol.
+
+ Args:
+ results (list[dict]): List of results.
+ metric (str | list[str], optional): Metrics to be evaluated.
+ Defaults to None.
+ iou_thr (list[float]): AP IoU thresholds. Defaults to (0.25, 0.5).
+ logger (logging.Logger | str, optional): Logger used for printing
+ related information during evaluation. Defaults to None.
+ show (bool, optional): Whether to visualize.
+ Default: False.
+ out_dir (str, optional): Path to save the visualization results.
+ Default: None.
+ pipeline (list[dict], optional): raw data loading for showing.
+ Default: None.
+
+ Returns:
+ dict: Evaluation results.
+ """
+ from mmdet3d.core.evaluation import indoor_eval
+ assert isinstance(
+ results, list), f'Expect results to be list, got {type(results)}.'
+ assert len(results) > 0, 'Expect length of results > 0.'
+ assert len(results) == len(self.data_infos)
+ assert isinstance(
+ results[0], dict
+ ), f'Expect elements in results to be dict, got {type(results[0])}.'
+ gt_annos = [info['annos'] for info in self.data_infos]
+ label2cat = {i: cat_id for i, cat_id in enumerate(self.CLASSES)}
+ ret_dict = indoor_eval(
+ gt_annos,
+ results,
+ iou_thr,
+ label2cat,
+ logger=logger,
+ box_type_3d=self.box_type_3d,
+ box_mode_3d=self.box_mode_3d)
+ if show:
+ self.show(results, out_dir, pipeline=pipeline)
+
+ return ret_dict
+
+ def _build_default_pipeline(self):
+ """Build the default pipeline for this dataset."""
+ raise NotImplementedError('_build_default_pipeline is not implemented '
+ f'for dataset {self.__class__.__name__}')
+
+ def _get_pipeline(self, pipeline):
+ """Get data loading pipeline in self.show/evaluate function.
+
+ Args:
+ pipeline (list[dict]): Input pipeline. If None is given,
+ get from self.pipeline.
+ """
+ if pipeline is None:
+ if not hasattr(self, 'pipeline') or self.pipeline is None:
+ warnings.warn(
+ 'Use default pipeline for data loading, this may cause '
+ 'errors when data is on ceph')
+ return self._build_default_pipeline()
+ loading_pipeline = get_loading_pipeline(self.pipeline.transforms)
+ return Compose(loading_pipeline)
+ return Compose(pipeline)
+
+ def _extract_data(self, index, pipeline, key, load_annos=False):
+ """Load data using input pipeline and extract data according to key.
+
+ Args:
+ index (int): Index for accessing the target data.
+ pipeline (:obj:`Compose`): Composed data loading pipeline.
+ key (str | list[str]): One single or a list of data key.
+ load_annos (bool): Whether to load data annotations.
+ If True, need to set self.test_mode as False before loading.
+
+ Returns:
+ np.ndarray | torch.Tensor | list[np.ndarray | torch.Tensor]:
+ A single or a list of loaded data.
+ """
+ assert pipeline is not None, 'data loading pipeline is not provided'
+ # when we want to load ground-truth via pipeline (e.g. bbox, seg mask)
+ # we need to set self.test_mode as False so that we have 'annos'
+ if load_annos:
+ original_test_mode = self.test_mode
+ self.test_mode = False
+ input_dict = self.get_data_info(index)
+ self.pre_pipeline(input_dict)
+ example = pipeline(input_dict)
+
+ # extract data items according to keys
+ if isinstance(key, str):
+ data = extract_result_dict(example, key)
+ else:
+ data = [extract_result_dict(example, k) for k in key]
+ if load_annos:
+ self.test_mode = original_test_mode
+
+ return data
+
+ def __len__(self):
+ """Return the length of data infos.
+
+ Returns:
+ int: Length of data infos.
+ """
+ return len(self.data_infos)
+
+ def _rand_another(self, idx):
+ """Randomly get another item with the same flag.
+
+ Returns:
+ int: Another index of item with the same flag.
+ """
+ pool = np.where(self.flag == self.flag[idx])[0]
+ return np.random.choice(pool)
+
+ def __getitem__(self, idx):
+ """Get item from infos according to the given index.
+
+ Returns:
+ dict: Data dictionary of the corresponding index.
+ """
+ if self.test_mode:
+ return self.prepare_test_data(idx)
+ while True:
+ data = self.prepare_train_data(idx)
+ if data is None:
+ idx = self._rand_another(idx)
+ continue
+ return data
+
+ def _set_group_flag(self):
+ """Set flag according to image aspect ratio.
+
+ Images with aspect ratio greater than 1 will be set as group 1,
+ otherwise group 0. In 3D datasets, they are all the same, thus are all
+ zeros.
+ """
+ self.flag = np.zeros(len(self), dtype=np.uint8)
diff --git a/mmdet3d/datasets/custom_3d_seg.py b/mmdet3d/datasets/custom_3d_seg.py
new file mode 100644
index 0000000..e123611
--- /dev/null
+++ b/mmdet3d/datasets/custom_3d_seg.py
@@ -0,0 +1,465 @@
+# Copyright (c) OpenMMLab. All rights reserved.
+import tempfile
+import warnings
+from os import path as osp
+
+import mmcv
+import numpy as np
+from torch.utils.data import Dataset
+
+from mmseg.datasets import DATASETS as SEG_DATASETS
+from .builder import DATASETS
+from .pipelines import Compose
+from .utils import extract_result_dict, get_loading_pipeline
+
+
+@DATASETS.register_module()
+@SEG_DATASETS.register_module()
+class Custom3DSegDataset(Dataset):
+ """Customized 3D dataset for semantic segmentation task.
+
+ This is the base dataset of ScanNet and S3DIS dataset.
+
+ Args:
+ data_root (str): Path of dataset root.
+ ann_file (str): Path of annotation file.
+ pipeline (list[dict], optional): Pipeline used for data processing.
+ Defaults to None.
+ classes (tuple[str], optional): Classes used in the dataset.
+ Defaults to None.
+ palette (list[list[int]], optional): The palette of segmentation map.
+ Defaults to None.
+ modality (dict, optional): Modality to specify the sensor data used
+ as input. Defaults to None.
+ test_mode (bool, optional): Whether the dataset is in test mode.
+ Defaults to False.
+ ignore_index (int, optional): The label index to be ignored, e.g.
+ unannotated points. If None is given, set to len(self.CLASSES) to
+ be consistent with PointSegClassMapping function in pipeline.
+ Defaults to None.
+ scene_idxs (np.ndarray | str, optional): Precomputed index to load
+ data. For scenes with many points, we may sample it several times.
+ Defaults to None.
+ """
+ # names of all classes data used for the task
+ CLASSES = None
+
+ # class_ids used for training
+ VALID_CLASS_IDS = None
+
+ # all possible class_ids in loaded segmentation mask
+ ALL_CLASS_IDS = None
+
+ # official color for visualization
+ PALETTE = None
+
+ def __init__(self,
+ data_root,
+ ann_file,
+ pipeline=None,
+ classes=None,
+ palette=None,
+ modality=None,
+ test_mode=False,
+ ignore_index=None,
+ scene_idxs=None,
+ file_client_args=dict(backend='disk')):
+ super().__init__()
+ self.data_root = data_root
+ self.ann_file = ann_file
+ self.test_mode = test_mode
+ self.modality = modality
+ self.file_client = mmcv.FileClient(**file_client_args)
+
+ # load annotations
+ if hasattr(self.file_client, 'get_local_path'):
+ with self.file_client.get_local_path(self.ann_file) as local_path:
+ self.data_infos = self.load_annotations(open(local_path, 'rb'))
+ else:
+ warnings.warn(
+ 'The used MMCV version does not have get_local_path. '
+ f'We treat the {self.ann_file} as local paths and it '
+ 'might cause errors if the path is not a local path. '
+ 'Please use MMCV>= 1.3.16 if you meet errors.')
+ self.data_infos = self.load_annotations(self.ann_file)
+
+ if pipeline is not None:
+ self.pipeline = Compose(pipeline)
+
+ self.ignore_index = len(self.CLASSES) if \
+ ignore_index is None else ignore_index
+
+ self.scene_idxs = self.get_scene_idxs(scene_idxs)
+ self.CLASSES, self.PALETTE = \
+ self.get_classes_and_palette(classes, palette)
+
+ # set group flag for the sampler
+ if not self.test_mode:
+ self._set_group_flag()
+
+ def load_annotations(self, ann_file):
+ """Load annotations from ann_file.
+
+ Args:
+ ann_file (str): Path of the annotation file.
+
+ Returns:
+ list[dict]: List of annotations.
+ """
+ # loading data from a file-like object needs file format
+ return mmcv.load(ann_file, file_format='pkl')
+
+ def get_data_info(self, index):
+ """Get data info according to the given index.
+
+ Args:
+ index (int): Index of the sample data to get.
+
+ Returns:
+ dict: Data information that will be passed to the data
+ preprocessing pipelines. It includes the following keys:
+
+ - sample_idx (str): Sample index.
+ - pts_filename (str): Filename of point clouds.
+ - file_name (str): Filename of point clouds.
+ - ann_info (dict): Annotation info.
+ """
+ info = self.data_infos[index]
+ sample_idx = info['point_cloud']['lidar_idx']
+ pts_filename = osp.join(self.data_root, info['pts_path'])
+
+ input_dict = dict(
+ pts_filename=pts_filename,
+ sample_idx=sample_idx,
+ file_name=pts_filename)
+
+ if not self.test_mode:
+ annos = self.get_ann_info(index)
+ input_dict['ann_info'] = annos
+ return input_dict
+
+ def pre_pipeline(self, results):
+ """Initialization before data preparation.
+
+ Args:
+ results (dict): Dict before data preprocessing.
+
+ - img_fields (list): Image fields.
+ - pts_mask_fields (list): Mask fields of points.
+ - pts_seg_fields (list): Mask fields of point segments.
+ - mask_fields (list): Fields of masks.
+ - seg_fields (list): Segment fields.
+ """
+ results['img_fields'] = []
+ results['pts_mask_fields'] = []
+ results['pts_seg_fields'] = []
+ results['mask_fields'] = []
+ results['seg_fields'] = []
+ results['bbox3d_fields'] = []
+
+ def prepare_train_data(self, index):
+ """Training data preparation.
+
+ Args:
+ index (int): Index for accessing the target data.
+
+ Returns:
+ dict: Training data dict of the corresponding index.
+ """
+ input_dict = self.get_data_info(index)
+ if input_dict is None:
+ return None
+ self.pre_pipeline(input_dict)
+ example = self.pipeline(input_dict)
+ return example
+
+ def prepare_test_data(self, index):
+ """Prepare data for testing.
+
+ Args:
+ index (int): Index for accessing the target data.
+
+ Returns:
+ dict: Testing data dict of the corresponding index.
+ """
+ input_dict = self.get_data_info(index)
+ self.pre_pipeline(input_dict)
+ example = self.pipeline(input_dict)
+ return example
+
+ def get_classes_and_palette(self, classes=None, palette=None):
+ """Get class names of current dataset.
+
+ This function is taken from MMSegmentation.
+
+ Args:
+ classes (Sequence[str] | str): If classes is None, use
+ default CLASSES defined by builtin dataset. If classes is a
+ string, take it as a file name. The file contains the name of
+ classes where each line contains one class name. If classes is
+ a tuple or list, override the CLASSES defined by the dataset.
+ Defaults to None.
+ palette (Sequence[Sequence[int]]] | np.ndarray):
+ The palette of segmentation map. If None is given, random
+ palette will be generated. Defaults to None.
+ """
+ if classes is None:
+ self.custom_classes = False
+ # map id in the loaded mask to label used for training
+ self.label_map = {
+ cls_id: self.ignore_index
+ for cls_id in self.ALL_CLASS_IDS
+ }
+ self.label_map.update(
+ {cls_id: i
+ for i, cls_id in enumerate(self.VALID_CLASS_IDS)})
+ # map label to category name
+ self.label2cat = {
+ i: cat_name
+ for i, cat_name in enumerate(self.CLASSES)
+ }
+ return self.CLASSES, self.PALETTE
+
+ self.custom_classes = True
+ if isinstance(classes, str):
+ # take it as a file path
+ class_names = mmcv.list_from_file(classes)
+ elif isinstance(classes, (tuple, list)):
+ class_names = classes
+ else:
+ raise ValueError(f'Unsupported type {type(classes)} of classes.')
+
+ if self.CLASSES:
+ if not set(class_names).issubset(self.CLASSES):
+ raise ValueError('classes is not a subset of CLASSES.')
+
+ # update valid_class_ids
+ self.VALID_CLASS_IDS = [
+ self.VALID_CLASS_IDS[self.CLASSES.index(cls_name)]
+ for cls_name in class_names
+ ]
+
+ # dictionary, its keys are the old label ids and its values
+ # are the new label ids.
+ # used for changing pixel labels in load_annotations.
+ self.label_map = {
+ cls_id: self.ignore_index
+ for cls_id in self.ALL_CLASS_IDS
+ }
+ self.label_map.update(
+ {cls_id: i
+ for i, cls_id in enumerate(self.VALID_CLASS_IDS)})
+ self.label2cat = {
+ i: cat_name
+ for i, cat_name in enumerate(class_names)
+ }
+
+ # modify palette for visualization
+ palette = [
+ self.PALETTE[self.CLASSES.index(cls_name)]
+ for cls_name in class_names
+ ]
+
+ return class_names, palette
+
+ def get_scene_idxs(self, scene_idxs):
+ """Compute scene_idxs for data sampling.
+
+ We sample more times for scenes with more points.
+ """
+ if self.test_mode:
+ # when testing, we load one whole scene every time
+ return np.arange(len(self.data_infos)).astype(np.int32)
+
+ # we may need to re-sample different scenes according to scene_idxs
+ # this is necessary for indoor scene segmentation such as ScanNet
+ if scene_idxs is None:
+ scene_idxs = np.arange(len(self.data_infos))
+ if isinstance(scene_idxs, str):
+ with self.file_client.get_local_path(scene_idxs) as local_path:
+ scene_idxs = np.load(local_path)
+ else:
+ scene_idxs = np.array(scene_idxs)
+
+ return scene_idxs.astype(np.int32)
+
+ def format_results(self,
+ outputs,
+ pklfile_prefix=None,
+ submission_prefix=None):
+ """Format the results to pkl file.
+
+ Args:
+ outputs (list[dict]): Testing results of the dataset.
+ pklfile_prefix (str): The prefix of pkl files. It includes
+ the file path and the prefix of filename, e.g., "a/b/prefix".
+ If not specified, a temp file will be created. Default: None.
+
+ Returns:
+ tuple: (outputs, tmp_dir), outputs is the detection results,
+ tmp_dir is the temporal directory created for saving json
+ files when ``jsonfile_prefix`` is not specified.
+ """
+ if pklfile_prefix is None:
+ tmp_dir = tempfile.TemporaryDirectory()
+ pklfile_prefix = osp.join(tmp_dir.name, 'results')
+ out = f'{pklfile_prefix}.pkl'
+ mmcv.dump(outputs, out)
+ return outputs, tmp_dir
+
+ def evaluate(self,
+ results,
+ metric=None,
+ logger=None,
+ show=False,
+ out_dir=None,
+ pipeline=None):
+ """Evaluate.
+
+ Evaluation in semantic segmentation protocol.
+
+ Args:
+ results (list[dict]): List of results.
+ metric (str | list[str]): Metrics to be evaluated.
+ logger (logging.Logger | str, optional): Logger used for printing
+ related information during evaluation. Defaults to None.
+ show (bool, optional): Whether to visualize.
+ Defaults to False.
+ out_dir (str, optional): Path to save the visualization results.
+ Defaults to None.
+ pipeline (list[dict], optional): raw data loading for showing.
+ Default: None.
+
+ Returns:
+ dict: Evaluation results.
+ """
+ from mmdet3d.core.evaluation import seg_eval
+ assert isinstance(
+ results, list), f'Expect results to be list, got {type(results)}.'
+ assert len(results) > 0, 'Expect length of results > 0.'
+ assert len(results) == len(self.data_infos)
+ assert isinstance(
+ results[0], dict
+ ), f'Expect elements in results to be dict, got {type(results[0])}.'
+
+ load_pipeline = self._get_pipeline(pipeline)
+ pred_sem_masks = [result['semantic_mask'] for result in results]
+ gt_sem_masks = [
+ self._extract_data(
+ i, load_pipeline, 'pts_semantic_mask', load_annos=True)
+ for i in range(len(self.data_infos))
+ ]
+ ret_dict = seg_eval(
+ gt_sem_masks,
+ pred_sem_masks,
+ self.label2cat,
+ self.ignore_index,
+ logger=logger)
+
+ if show:
+ self.show(pred_sem_masks, out_dir, pipeline=pipeline)
+
+ return ret_dict
+
+ def _rand_another(self, idx):
+ """Randomly get another item with the same flag.
+
+ Returns:
+ int: Another index of item with the same flag.
+ """
+ pool = np.where(self.flag == self.flag[idx])[0]
+ return np.random.choice(pool)
+
+ def _build_default_pipeline(self):
+ """Build the default pipeline for this dataset."""
+ raise NotImplementedError('_build_default_pipeline is not implemented '
+ f'for dataset {self.__class__.__name__}')
+
+ def _get_pipeline(self, pipeline):
+ """Get data loading pipeline in self.show/evaluate function.
+
+ Args:
+ pipeline (list[dict]): Input pipeline. If None is given,
+ get from self.pipeline.
+ """
+ if pipeline is None:
+ if not hasattr(self, 'pipeline') or self.pipeline is None:
+ warnings.warn(
+ 'Use default pipeline for data loading, this may cause '
+ 'errors when data is on ceph')
+ return self._build_default_pipeline()
+ loading_pipeline = get_loading_pipeline(self.pipeline.transforms)
+ return Compose(loading_pipeline)
+ return Compose(pipeline)
+
+ def _extract_data(self, index, pipeline, key, load_annos=False):
+ """Load data using input pipeline and extract data according to key.
+
+ Args:
+ index (int): Index for accessing the target data.
+ pipeline (:obj:`Compose`): Composed data loading pipeline.
+ key (str | list[str]): One single or a list of data key.
+ load_annos (bool): Whether to load data annotations.
+ If True, need to set self.test_mode as False before loading.
+
+ Returns:
+ np.ndarray | torch.Tensor | list[np.ndarray | torch.Tensor]:
+ A single or a list of loaded data.
+ """
+ assert pipeline is not None, 'data loading pipeline is not provided'
+ # when we want to load ground-truth via pipeline (e.g. bbox, seg mask)
+ # we need to set self.test_mode as False so that we have 'annos'
+ if load_annos:
+ original_test_mode = self.test_mode
+ self.test_mode = False
+ input_dict = self.get_data_info(index)
+ self.pre_pipeline(input_dict)
+ example = pipeline(input_dict)
+
+ # extract data items according to keys
+ if isinstance(key, str):
+ data = extract_result_dict(example, key)
+ else:
+ data = [extract_result_dict(example, k) for k in key]
+ if load_annos:
+ self.test_mode = original_test_mode
+
+ return data
+
+ def __len__(self):
+ """Return the length of scene_idxs.
+
+ Returns:
+ int: Length of data infos.
+ """
+ return len(self.scene_idxs)
+
+ def __getitem__(self, idx):
+ """Get item from infos according to the given index.
+
+ In indoor scene segmentation task, each scene contains millions of
+ points. However, we only sample less than 10k points within a patch
+ each time. Therefore, we use `scene_idxs` to re-sample different rooms.
+
+ Returns:
+ dict: Data dictionary of the corresponding index.
+ """
+ scene_idx = self.scene_idxs[idx] # map to scene idx
+ if self.test_mode:
+ return self.prepare_test_data(scene_idx)
+ while True:
+ data = self.prepare_train_data(scene_idx)
+ if data is None:
+ idx = self._rand_another(idx)
+ scene_idx = self.scene_idxs[idx] # map to scene idx
+ continue
+ return data
+
+ def _set_group_flag(self):
+ """Set flag according to image aspect ratio.
+
+ Images with aspect ratio greater than 1 will be set as group 1,
+ otherwise group 0. In 3D datasets, they are all the same, thus are all
+ zeros.
+ """
+ self.flag = np.zeros(len(self), dtype=np.uint8)
diff --git a/mmdet3d/datasets/dataset_wrappers.py b/mmdet3d/datasets/dataset_wrappers.py
new file mode 100644
index 0000000..2ae3327
--- /dev/null
+++ b/mmdet3d/datasets/dataset_wrappers.py
@@ -0,0 +1,76 @@
+# Copyright (c) OpenMMLab. All rights reserved.
+import numpy as np
+
+from .builder import DATASETS
+
+
+@DATASETS.register_module()
+class CBGSDataset(object):
+ """A wrapper of class sampled dataset with ann_file path. Implementation of
+ paper `Class-balanced Grouping and Sampling for Point Cloud 3D Object
+ Detection `_.
+
+ Balance the number of scenes under different classes.
+
+ Args:
+ dataset (:obj:`CustomDataset`): The dataset to be class sampled.
+ """
+
+ def __init__(self, dataset):
+ self.dataset = dataset
+ self.CLASSES = dataset.CLASSES
+ self.cat2id = {name: i for i, name in enumerate(self.CLASSES)}
+ self.sample_indices = self._get_sample_indices()
+ # self.dataset.data_infos = self.data_infos
+ if hasattr(self.dataset, 'flag'):
+ self.flag = np.array(
+ [self.dataset.flag[ind] for ind in self.sample_indices],
+ dtype=np.uint8)
+
+ def _get_sample_indices(self):
+ """Load annotations from ann_file.
+
+ Args:
+ ann_file (str): Path of the annotation file.
+
+ Returns:
+ list[dict]: List of annotations after class sampling.
+ """
+ class_sample_idxs = {cat_id: [] for cat_id in self.cat2id.values()}
+ for idx in range(len(self.dataset)):
+ sample_cat_ids = self.dataset.get_cat_ids(idx)
+ for cat_id in sample_cat_ids:
+ class_sample_idxs[cat_id].append(idx)
+ duplicated_samples = sum(
+ [len(v) for _, v in class_sample_idxs.items()])
+ class_distribution = {
+ k: len(v) / duplicated_samples
+ for k, v in class_sample_idxs.items()
+ }
+
+ sample_indices = []
+
+ frac = 1.0 / len(self.CLASSES)
+ ratios = [frac / v for v in class_distribution.values()]
+ for cls_inds, ratio in zip(list(class_sample_idxs.values()), ratios):
+ sample_indices += np.random.choice(cls_inds,
+ int(len(cls_inds) *
+ ratio)).tolist()
+ return sample_indices
+
+ def __getitem__(self, idx):
+ """Get item from infos according to the given index.
+
+ Returns:
+ dict: Data dictionary of the corresponding index.
+ """
+ ori_idx = self.sample_indices[idx]
+ return self.dataset[ori_idx]
+
+ def __len__(self):
+ """Return the length of data infos.
+
+ Returns:
+ int: Length of data infos.
+ """
+ return len(self.sample_indices)
diff --git a/mmdet3d/datasets/kitti2d_dataset.py b/mmdet3d/datasets/kitti2d_dataset.py
new file mode 100644
index 0000000..a943932
--- /dev/null
+++ b/mmdet3d/datasets/kitti2d_dataset.py
@@ -0,0 +1,241 @@
+# Copyright (c) OpenMMLab. All rights reserved.
+import mmcv
+import numpy as np
+
+from mmdet.datasets import CustomDataset
+from .builder import DATASETS
+
+
+@DATASETS.register_module()
+class Kitti2DDataset(CustomDataset):
+ r"""KITTI 2D Dataset.
+
+ This class serves as the API for experiments on the `KITTI Dataset
+ `_.
+
+ Args:
+ data_root (str): Path of dataset root.
+ ann_file (str): Path of annotation file.
+ pipeline (list[dict], optional): Pipeline used for data processing.
+ Defaults to None.
+ classes (tuple[str], optional): Classes used in the dataset.
+ Defaults to None.
+ modality (dict, optional): Modality to specify the sensor data used
+ as input. Defaults to None.
+ box_type_3d (str, optional): Type of 3D box of this dataset.
+ Based on the `box_type_3d`, the dataset will encapsulate the box
+ to its original format then converted them to `box_type_3d`.
+ Defaults to 'LiDAR'. Available options includes
+
+ - 'LiDAR': Box in LiDAR coordinates.
+ - 'Depth': Box in depth coordinates, usually for indoor dataset.
+ - 'Camera': Box in camera coordinates.
+ filter_empty_gt (bool, optional): Whether to filter empty GT.
+ Defaults to True.
+ test_mode (bool, optional): Whether the dataset is in test mode.
+ Defaults to False.
+ """
+
+ CLASSES = ('car', 'pedestrian', 'cyclist')
+ """
+ Annotation format:
+ [
+ {
+ 'image': {
+ 'image_idx': 0,
+ 'image_path': 'training/image_2/000000.png',
+ 'image_shape': array([ 370, 1224], dtype=int32)
+ },
+ 'point_cloud': {
+ 'num_features': 4,
+ 'velodyne_path': 'training/velodyne/000000.bin'
+ },
+ 'calib': {
+ 'P0': (4, 4),
+ 'P1': (4, 4),
+ 'P2': (4, 4),
+ 'P3': (4, 4),
+ 'R0_rect':4x4 np.array,
+ 'Tr_velo_to_cam': 4x4 np.array,
+ 'Tr_imu_to_velo': 4x4 np.array
+ },
+ 'annos': {
+ 'name': (n),
+ 'truncated': (n),
+ 'occluded': (n),
+ 'alpha': (n),
+ 'bbox': (n, 4),
+ 'dimensions': (n, 3),
+ 'location': (n, 3),
+ 'rotation_y': (n),
+ 'score': (n),
+ 'index': array([0], dtype=int32),
+ 'group_ids': array([0], dtype=int32),
+ 'difficulty': array([0], dtype=int32),
+ 'num_points_in_gt': (n),
+ }
+ }
+ ]
+ """
+
+ def load_annotations(self, ann_file):
+ """Load annotations from ann_file.
+
+ Args:
+ ann_file (str): Path of the annotation file.
+
+ Returns:
+ list[dict]: List of annotations.
+ """
+ self.data_infos = mmcv.load(ann_file)
+ self.cat2label = {
+ cat_name: i
+ for i, cat_name in enumerate(self.CLASSES)
+ }
+ return self.data_infos
+
+ def _filter_imgs(self, min_size=32):
+ """Filter images without ground truths."""
+ valid_inds = []
+ for i, img_info in enumerate(self.data_infos):
+ if len(img_info['annos']['name']) > 0:
+ valid_inds.append(i)
+ return valid_inds
+
+ def get_ann_info(self, index):
+ """Get annotation info according to the given index.
+
+ Args:
+ index (int): Index of the annotation data to get.
+
+ Returns:
+ dict: Annotation information consists of the following keys:
+
+ - bboxes (np.ndarray): Ground truth bboxes.
+ - labels (np.ndarray): Labels of ground truths.
+ """
+ # Use index to get the annos, thus the evalhook could also use this api
+ info = self.data_infos[index]
+ annos = info['annos']
+ gt_names = annos['name']
+ gt_bboxes = annos['bbox']
+ difficulty = annos['difficulty']
+
+ # remove classes that is not needed
+ selected = self.keep_arrays_by_name(gt_names, self.CLASSES)
+ gt_bboxes = gt_bboxes[selected]
+ gt_names = gt_names[selected]
+ difficulty = difficulty[selected]
+ gt_labels = np.array([self.cat2label[n] for n in gt_names])
+
+ anns_results = dict(
+ bboxes=gt_bboxes.astype(np.float32),
+ labels=gt_labels,
+ )
+ return anns_results
+
+ def prepare_train_img(self, idx):
+ """Training image preparation.
+
+ Args:
+ index (int): Index for accessing the target image data.
+
+ Returns:
+ dict: Training image data dict after preprocessing
+ corresponding to the index.
+ """
+ img_raw_info = self.data_infos[idx]['image']
+ img_info = dict(filename=img_raw_info['image_path'])
+ ann_info = self.get_ann_info(idx)
+ if len(ann_info['bboxes']) == 0:
+ return None
+ results = dict(img_info=img_info, ann_info=ann_info)
+ if self.proposals is not None:
+ results['proposals'] = self.proposals[idx]
+ self.pre_pipeline(results)
+ return self.pipeline(results)
+
+ def prepare_test_img(self, idx):
+ """Prepare data for testing.
+
+ Args:
+ index (int): Index for accessing the target image data.
+
+ Returns:
+ dict: Testing image data dict after preprocessing
+ corresponding to the index.
+ """
+ img_raw_info = self.data_infos[idx]['image']
+ img_info = dict(filename=img_raw_info['image_path'])
+ results = dict(img_info=img_info)
+ if self.proposals is not None:
+ results['proposals'] = self.proposals[idx]
+ self.pre_pipeline(results)
+ return self.pipeline(results)
+
+ def drop_arrays_by_name(self, gt_names, used_classes):
+ """Drop irrelevant ground truths by name.
+
+ Args:
+ gt_names (list[str]): Names of ground truths.
+ used_classes (list[str]): Classes of interest.
+
+ Returns:
+ np.ndarray: Indices of ground truths that will be dropped.
+ """
+ inds = [i for i, x in enumerate(gt_names) if x not in used_classes]
+ inds = np.array(inds, dtype=np.int64)
+ return inds
+
+ def keep_arrays_by_name(self, gt_names, used_classes):
+ """Keep useful ground truths by name.
+
+ Args:
+ gt_names (list[str]): Names of ground truths.
+ used_classes (list[str]): Classes of interest.
+
+ Returns:
+ np.ndarray: Indices of ground truths that will be keeped.
+ """
+ inds = [i for i, x in enumerate(gt_names) if x in used_classes]
+ inds = np.array(inds, dtype=np.int64)
+ return inds
+
+ def reformat_bbox(self, outputs, out=None):
+ """Reformat bounding boxes to KITTI 2D styles.
+
+ Args:
+ outputs (list[np.ndarray]): List of arrays storing the inferenced
+ bounding boxes and scores.
+ out (str, optional): The prefix of output file.
+ Default: None.
+
+ Returns:
+ list[dict]: A list of dictionaries with the kitti 2D format.
+ """
+ from mmdet3d.core.bbox.transforms import bbox2result_kitti2d
+ sample_idx = [info['image']['image_idx'] for info in self.data_infos]
+ result_files = bbox2result_kitti2d(outputs, self.CLASSES, sample_idx,
+ out)
+ return result_files
+
+ def evaluate(self, result_files, eval_types=None):
+ """Evaluation in KITTI protocol.
+
+ Args:
+ result_files (str): Path of result files.
+ eval_types (str, optional): Types of evaluation. Default: None.
+ KITTI dataset only support 'bbox' evaluation type.
+
+ Returns:
+ tuple (str, dict): Average precision results in str format
+ and average precision results in dict format.
+ """
+ from mmdet3d.core.evaluation import kitti_eval
+ eval_types = ['bbox'] if not eval_types else eval_types
+ assert eval_types in ('bbox', ['bbox'
+ ]), 'KITTI data set only evaluate bbox'
+ gt_annos = [info['annos'] for info in self.data_infos]
+ ap_result_str, ap_dict = kitti_eval(
+ gt_annos, result_files, self.CLASSES, eval_types=['bbox'])
+ return ap_result_str, ap_dict
diff --git a/mmdet3d/datasets/kitti_dataset.py b/mmdet3d/datasets/kitti_dataset.py
new file mode 100644
index 0000000..4802538
--- /dev/null
+++ b/mmdet3d/datasets/kitti_dataset.py
@@ -0,0 +1,773 @@
+# Copyright (c) OpenMMLab. All rights reserved.
+import copy
+import os
+import tempfile
+from os import path as osp
+
+import mmcv
+import numpy as np
+import torch
+from mmcv.utils import print_log
+
+from ..core import show_multi_modality_result, show_result
+from ..core.bbox import (Box3DMode, CameraInstance3DBoxes, Coord3DMode,
+ LiDARInstance3DBoxes, points_cam2img)
+from .builder import DATASETS
+from .custom_3d import Custom3DDataset
+from .pipelines import Compose
+
+
+@DATASETS.register_module()
+class KittiDataset(Custom3DDataset):
+ r"""KITTI Dataset.
+
+ This class serves as the API for experiments on the `KITTI Dataset
+ `_.
+
+ Args:
+ data_root (str): Path of dataset root.
+ ann_file (str): Path of annotation file.
+ split (str): Split of input data.
+ pts_prefix (str, optional): Prefix of points files.
+ Defaults to 'velodyne'.
+ pipeline (list[dict], optional): Pipeline used for data processing.
+ Defaults to None.
+ classes (tuple[str], optional): Classes used in the dataset.
+ Defaults to None.
+ modality (dict, optional): Modality to specify the sensor data used
+ as input. Defaults to None.
+ box_type_3d (str, optional): Type of 3D box of this dataset.
+ Based on the `box_type_3d`, the dataset will encapsulate the box
+ to its original format then converted them to `box_type_3d`.
+ Defaults to 'LiDAR' in this dataset. Available options includes
+
+ - 'LiDAR': Box in LiDAR coordinates.
+ - 'Depth': Box in depth coordinates, usually for indoor dataset.
+ - 'Camera': Box in camera coordinates.
+ filter_empty_gt (bool, optional): Whether to filter empty GT.
+ Defaults to True.
+ test_mode (bool, optional): Whether the dataset is in test mode.
+ Defaults to False.
+ pcd_limit_range (list, optional): The range of point cloud used to
+ filter invalid predicted boxes.
+ Default: [0, -40, -3, 70.4, 40, 0.0].
+ """
+ CLASSES = ('car', 'pedestrian', 'cyclist')
+
+ def __init__(self,
+ data_root,
+ ann_file,
+ split,
+ pts_prefix='velodyne',
+ pipeline=None,
+ classes=None,
+ modality=None,
+ box_type_3d='LiDAR',
+ filter_empty_gt=True,
+ test_mode=False,
+ pcd_limit_range=[0, -40, -3, 70.4, 40, 0.0],
+ **kwargs):
+ super().__init__(
+ data_root=data_root,
+ ann_file=ann_file,
+ pipeline=pipeline,
+ classes=classes,
+ modality=modality,
+ box_type_3d=box_type_3d,
+ filter_empty_gt=filter_empty_gt,
+ test_mode=test_mode,
+ **kwargs)
+
+ self.split = split
+ self.root_split = os.path.join(self.data_root, split)
+ assert self.modality is not None
+ self.pcd_limit_range = pcd_limit_range
+ self.pts_prefix = pts_prefix
+
+ def _get_pts_filename(self, idx):
+ """Get point cloud filename according to the given index.
+
+ Args:
+ index (int): Index of the point cloud file to get.
+
+ Returns:
+ str: Name of the point cloud file.
+ """
+ pts_filename = osp.join(self.root_split, self.pts_prefix,
+ f'{idx:06d}.bin')
+ return pts_filename
+
+ def get_data_info(self, index):
+ """Get data info according to the given index.
+
+ Args:
+ index (int): Index of the sample data to get.
+
+ Returns:
+ dict: Data information that will be passed to the data
+ preprocessing pipelines. It includes the following keys:
+
+ - sample_idx (str): Sample index.
+ - pts_filename (str): Filename of point clouds.
+ - img_prefix (str): Prefix of image files.
+ - img_info (dict): Image info.
+ - lidar2img (list[np.ndarray], optional): Transformations
+ from lidar to different cameras.
+ - ann_info (dict): Annotation info.
+ """
+ info = self.data_infos[index]
+ sample_idx = info['image']['image_idx']
+ img_filename = os.path.join(self.data_root,
+ info['image']['image_path'])
+
+ # TODO: consider use torch.Tensor only
+ rect = info['calib']['R0_rect'].astype(np.float32)
+ Trv2c = info['calib']['Tr_velo_to_cam'].astype(np.float32)
+ P2 = info['calib']['P2'].astype(np.float32)
+ lidar2img = P2 @ rect @ Trv2c
+
+ pts_filename = self._get_pts_filename(sample_idx)
+ input_dict = dict(
+ sample_idx=sample_idx,
+ pts_filename=pts_filename,
+ img_prefix=None,
+ img_info=dict(filename=img_filename),
+ lidar2img=lidar2img)
+
+ if not self.test_mode:
+ annos = self.get_ann_info(index)
+ input_dict['ann_info'] = annos
+
+ return input_dict
+
+ def get_ann_info(self, index):
+ """Get annotation info according to the given index.
+
+ Args:
+ index (int): Index of the annotation data to get.
+
+ Returns:
+ dict: annotation information consists of the following keys:
+
+ - gt_bboxes_3d (:obj:`LiDARInstance3DBoxes`):
+ 3D ground truth bboxes.
+ - gt_labels_3d (np.ndarray): Labels of ground truths.
+ - gt_bboxes (np.ndarray): 2D ground truth bboxes.
+ - gt_labels (np.ndarray): Labels of ground truths.
+ - gt_names (list[str]): Class names of ground truths.
+ - difficulty (int): Difficulty defined by KITTI.
+ 0, 1, 2 represent xxxxx respectively.
+ """
+ # Use index to get the annos, thus the evalhook could also use this api
+ info = self.data_infos[index]
+ rect = info['calib']['R0_rect'].astype(np.float32)
+ Trv2c = info['calib']['Tr_velo_to_cam'].astype(np.float32)
+
+ if 'plane' in info:
+ # convert ground plane to velodyne coordinates
+ reverse = np.linalg.inv(rect @ Trv2c)
+
+ (plane_norm_cam,
+ plane_off_cam) = (info['plane'][:3],
+ -info['plane'][:3] * info['plane'][3])
+ plane_norm_lidar = \
+ (reverse[:3, :3] @ plane_norm_cam[:, None])[:, 0]
+ plane_off_lidar = (
+ reverse[:3, :3] @ plane_off_cam[:, None][:, 0] +
+ reverse[:3, 3])
+ plane_lidar = np.zeros_like(plane_norm_lidar, shape=(4, ))
+ plane_lidar[:3] = plane_norm_lidar
+ plane_lidar[3] = -plane_norm_lidar.T @ plane_off_lidar
+ else:
+ plane_lidar = None
+
+ difficulty = info['annos']['difficulty']
+ annos = info['annos']
+ # we need other objects to avoid collision when sample
+ annos = self.remove_dontcare(annos)
+ loc = annos['location']
+ dims = annos['dimensions']
+ rots = annos['rotation_y']
+ gt_names = annos['name']
+ gt_bboxes_3d = np.concatenate([loc, dims, rots[..., np.newaxis]],
+ axis=1).astype(np.float32)
+
+ # convert gt_bboxes_3d to velodyne coordinates
+ gt_bboxes_3d = CameraInstance3DBoxes(gt_bboxes_3d).convert_to(
+ self.box_mode_3d, np.linalg.inv(rect @ Trv2c))
+ gt_bboxes = annos['bbox']
+
+ selected = self.drop_arrays_by_name(gt_names, ['DontCare'])
+ gt_bboxes = gt_bboxes[selected].astype('float32')
+ gt_names = gt_names[selected]
+
+ gt_labels = []
+ for cat in gt_names:
+ if cat in self.CLASSES:
+ gt_labels.append(self.CLASSES.index(cat))
+ else:
+ gt_labels.append(-1)
+ gt_labels = np.array(gt_labels).astype(np.int64)
+ gt_labels_3d = copy.deepcopy(gt_labels)
+
+ anns_results = dict(
+ gt_bboxes_3d=gt_bboxes_3d,
+ gt_labels_3d=gt_labels_3d,
+ bboxes=gt_bboxes,
+ labels=gt_labels,
+ gt_names=gt_names,
+ plane=plane_lidar,
+ difficulty=difficulty)
+ return anns_results
+
+ def drop_arrays_by_name(self, gt_names, used_classes):
+ """Drop irrelevant ground truths by name.
+
+ Args:
+ gt_names (list[str]): Names of ground truths.
+ used_classes (list[str]): Classes of interest.
+
+ Returns:
+ np.ndarray: Indices of ground truths that will be dropped.
+ """
+ inds = [i for i, x in enumerate(gt_names) if x not in used_classes]
+ inds = np.array(inds, dtype=np.int64)
+ return inds
+
+ def keep_arrays_by_name(self, gt_names, used_classes):
+ """Keep useful ground truths by name.
+
+ Args:
+ gt_names (list[str]): Names of ground truths.
+ used_classes (list[str]): Classes of interest.
+
+ Returns:
+ np.ndarray: Indices of ground truths that will be keeped.
+ """
+ inds = [i for i, x in enumerate(gt_names) if x in used_classes]
+ inds = np.array(inds, dtype=np.int64)
+ return inds
+
+ def remove_dontcare(self, ann_info):
+ """Remove annotations that do not need to be cared.
+
+ Args:
+ ann_info (dict): Dict of annotation infos. The ``'DontCare'``
+ annotations will be removed according to ann_file['name'].
+
+ Returns:
+ dict: Annotations after filtering.
+ """
+ img_filtered_annotations = {}
+ relevant_annotation_indices = [
+ i for i, x in enumerate(ann_info['name']) if x != 'DontCare'
+ ]
+ for key in ann_info.keys():
+ img_filtered_annotations[key] = (
+ ann_info[key][relevant_annotation_indices])
+ return img_filtered_annotations
+
+ def format_results(self,
+ outputs,
+ pklfile_prefix=None,
+ submission_prefix=None):
+ """Format the results to pkl file.
+
+ Args:
+ outputs (list[dict]): Testing results of the dataset.
+ pklfile_prefix (str): The prefix of pkl files. It includes
+ the file path and the prefix of filename, e.g., "a/b/prefix".
+ If not specified, a temp file will be created. Default: None.
+ submission_prefix (str): The prefix of submitted files. It
+ includes the file path and the prefix of filename, e.g.,
+ "a/b/prefix". If not specified, a temp file will be created.
+ Default: None.
+
+ Returns:
+ tuple: (result_files, tmp_dir), result_files is a dict containing
+ the json filepaths, tmp_dir is the temporal directory created
+ for saving json files when jsonfile_prefix is not specified.
+ """
+ if pklfile_prefix is None:
+ tmp_dir = tempfile.TemporaryDirectory()
+ pklfile_prefix = osp.join(tmp_dir.name, 'results')
+ else:
+ tmp_dir = None
+
+ if not isinstance(outputs[0], dict):
+ result_files = self.bbox2result_kitti2d(outputs, self.CLASSES,
+ pklfile_prefix,
+ submission_prefix)
+ elif 'pts_bbox' in outputs[0] or 'img_bbox' in outputs[0]:
+ result_files = dict()
+ for name in outputs[0]:
+ results_ = [out[name] for out in outputs]
+ pklfile_prefix_ = pklfile_prefix + name
+ if submission_prefix is not None:
+ submission_prefix_ = submission_prefix + name
+ else:
+ submission_prefix_ = None
+ if 'img' in name:
+ result_files = self.bbox2result_kitti2d(
+ results_, self.CLASSES, pklfile_prefix_,
+ submission_prefix_)
+ else:
+ result_files_ = self.bbox2result_kitti(
+ results_, self.CLASSES, pklfile_prefix_,
+ submission_prefix_)
+ result_files[name] = result_files_
+ else:
+ result_files = self.bbox2result_kitti(outputs, self.CLASSES,
+ pklfile_prefix,
+ submission_prefix)
+ return result_files, tmp_dir
+
+ def evaluate(self,
+ results,
+ metric=None,
+ logger=None,
+ pklfile_prefix=None,
+ submission_prefix=None,
+ show=False,
+ out_dir=None,
+ pipeline=None):
+ """Evaluation in KITTI protocol.
+
+ Args:
+ results (list[dict]): Testing results of the dataset.
+ metric (str | list[str], optional): Metrics to be evaluated.
+ Default: None.
+ logger (logging.Logger | str, optional): Logger used for printing
+ related information during evaluation. Default: None.
+ pklfile_prefix (str, optional): The prefix of pkl files, including
+ the file path and the prefix of filename, e.g., "a/b/prefix".
+ If not specified, a temp file will be created. Default: None.
+ submission_prefix (str, optional): The prefix of submission data.
+ If not specified, the submission data will not be generated.
+ Default: None.
+ show (bool, optional): Whether to visualize.
+ Default: False.
+ out_dir (str, optional): Path to save the visualization results.
+ Default: None.
+ pipeline (list[dict], optional): raw data loading for showing.
+ Default: None.
+
+ Returns:
+ dict[str, float]: Results of each evaluation metric.
+ """
+ result_files, tmp_dir = self.format_results(results, pklfile_prefix)
+ from mmdet3d.core.evaluation import kitti_eval
+ gt_annos = [info['annos'] for info in self.data_infos]
+
+ if isinstance(result_files, dict):
+ ap_dict = dict()
+ for name, result_files_ in result_files.items():
+ eval_types = ['bbox', 'bev', '3d']
+ if 'img' in name:
+ eval_types = ['bbox']
+ ap_result_str, ap_dict_ = kitti_eval(
+ gt_annos,
+ result_files_,
+ self.CLASSES,
+ eval_types=eval_types)
+ for ap_type, ap in ap_dict_.items():
+ ap_dict[f'{name}/{ap_type}'] = float('{:.4f}'.format(ap))
+
+ print_log(
+ f'Results of {name}:\n' + ap_result_str, logger=logger)
+
+ else:
+ if metric == 'img_bbox':
+ ap_result_str, ap_dict = kitti_eval(
+ gt_annos, result_files, self.CLASSES, eval_types=['bbox'])
+ else:
+ ap_result_str, ap_dict = kitti_eval(gt_annos, result_files,
+ self.CLASSES)
+ print_log('\n' + ap_result_str, logger=logger)
+
+ if tmp_dir is not None:
+ tmp_dir.cleanup()
+ if show or out_dir:
+ self.show(results, out_dir, show=show, pipeline=pipeline)
+ return ap_dict
+
+ def bbox2result_kitti(self,
+ net_outputs,
+ class_names,
+ pklfile_prefix=None,
+ submission_prefix=None):
+ """Convert 3D detection results to kitti format for evaluation and test
+ submission.
+
+ Args:
+ net_outputs (list[np.ndarray]): List of array storing the
+ inferenced bounding boxes and scores.
+ class_names (list[String]): A list of class names.
+ pklfile_prefix (str): The prefix of pkl file.
+ submission_prefix (str): The prefix of submission file.
+
+ Returns:
+ list[dict]: A list of dictionaries with the kitti format.
+ """
+ assert len(net_outputs) == len(self.data_infos), \
+ 'invalid list length of network outputs'
+ if submission_prefix is not None:
+ mmcv.mkdir_or_exist(submission_prefix)
+
+ det_annos = []
+ print('\nConverting prediction to KITTI format')
+ for idx, pred_dicts in enumerate(
+ mmcv.track_iter_progress(net_outputs)):
+ annos = []
+ info = self.data_infos[idx]
+ sample_idx = info['image']['image_idx']
+ image_shape = info['image']['image_shape'][:2]
+ box_dict = self.convert_valid_bboxes(pred_dicts, info)
+ anno = {
+ 'name': [],
+ 'truncated': [],
+ 'occluded': [],
+ 'alpha': [],
+ 'bbox': [],
+ 'dimensions': [],
+ 'location': [],
+ 'rotation_y': [],
+ 'score': []
+ }
+ if len(box_dict['bbox']) > 0:
+ box_2d_preds = box_dict['bbox']
+ box_preds = box_dict['box3d_camera']
+ scores = box_dict['scores']
+ box_preds_lidar = box_dict['box3d_lidar']
+ label_preds = box_dict['label_preds']
+
+ for box, box_lidar, bbox, score, label in zip(
+ box_preds, box_preds_lidar, box_2d_preds, scores,
+ label_preds):
+ bbox[2:] = np.minimum(bbox[2:], image_shape[::-1])
+ bbox[:2] = np.maximum(bbox[:2], [0, 0])
+ anno['name'].append(class_names[int(label)])
+ anno['truncated'].append(0.0)
+ anno['occluded'].append(0)
+ anno['alpha'].append(
+ -np.arctan2(-box_lidar[1], box_lidar[0]) + box[6])
+ anno['bbox'].append(bbox)
+ anno['dimensions'].append(box[3:6])
+ anno['location'].append(box[:3])
+ anno['rotation_y'].append(box[6])
+ anno['score'].append(score)
+
+ anno = {k: np.stack(v) for k, v in anno.items()}
+ annos.append(anno)
+ else:
+ anno = {
+ 'name': np.array([]),
+ 'truncated': np.array([]),
+ 'occluded': np.array([]),
+ 'alpha': np.array([]),
+ 'bbox': np.zeros([0, 4]),
+ 'dimensions': np.zeros([0, 3]),
+ 'location': np.zeros([0, 3]),
+ 'rotation_y': np.array([]),
+ 'score': np.array([]),
+ }
+ annos.append(anno)
+
+ if submission_prefix is not None:
+ curr_file = f'{submission_prefix}/{sample_idx:06d}.txt'
+ with open(curr_file, 'w') as f:
+ bbox = anno['bbox']
+ loc = anno['location']
+ dims = anno['dimensions'] # lhw -> hwl
+
+ for idx in range(len(bbox)):
+ print(
+ '{} -1 -1 {:.4f} {:.4f} {:.4f} {:.4f} '
+ '{:.4f} {:.4f} {:.4f} '
+ '{:.4f} {:.4f} {:.4f} {:.4f} {:.4f} {:.4f}'.format(
+ anno['name'][idx], anno['alpha'][idx],
+ bbox[idx][0], bbox[idx][1], bbox[idx][2],
+ bbox[idx][3], dims[idx][1], dims[idx][2],
+ dims[idx][0], loc[idx][0], loc[idx][1],
+ loc[idx][2], anno['rotation_y'][idx],
+ anno['score'][idx]),
+ file=f)
+
+ annos[-1]['sample_idx'] = np.array(
+ [sample_idx] * len(annos[-1]['score']), dtype=np.int64)
+
+ det_annos += annos
+
+ if pklfile_prefix is not None:
+ if not pklfile_prefix.endswith(('.pkl', '.pickle')):
+ out = f'{pklfile_prefix}.pkl'
+ mmcv.dump(det_annos, out)
+ print(f'Result is saved to {out}.')
+
+ return det_annos
+
+ def bbox2result_kitti2d(self,
+ net_outputs,
+ class_names,
+ pklfile_prefix=None,
+ submission_prefix=None):
+ """Convert 2D detection results to kitti format for evaluation and test
+ submission.
+
+ Args:
+ net_outputs (list[np.ndarray]): List of array storing the
+ inferenced bounding boxes and scores.
+ class_names (list[String]): A list of class names.
+ pklfile_prefix (str): The prefix of pkl file.
+ submission_prefix (str): The prefix of submission file.
+
+ Returns:
+ list[dict]: A list of dictionaries have the kitti format
+ """
+ assert len(net_outputs) == len(self.data_infos), \
+ 'invalid list length of network outputs'
+ det_annos = []
+ print('\nConverting prediction to KITTI format')
+ for i, bboxes_per_sample in enumerate(
+ mmcv.track_iter_progress(net_outputs)):
+ annos = []
+ anno = dict(
+ name=[],
+ truncated=[],
+ occluded=[],
+ alpha=[],
+ bbox=[],
+ dimensions=[],
+ location=[],
+ rotation_y=[],
+ score=[])
+ sample_idx = self.data_infos[i]['image']['image_idx']
+
+ num_example = 0
+ for label in range(len(bboxes_per_sample)):
+ bbox = bboxes_per_sample[label]
+ for i in range(bbox.shape[0]):
+ anno['name'].append(class_names[int(label)])
+ anno['truncated'].append(0.0)
+ anno['occluded'].append(0)
+ anno['alpha'].append(0.0)
+ anno['bbox'].append(bbox[i, :4])
+ # set dimensions (height, width, length) to zero
+ anno['dimensions'].append(
+ np.zeros(shape=[3], dtype=np.float32))
+ # set the 3D translation to (-1000, -1000, -1000)
+ anno['location'].append(
+ np.ones(shape=[3], dtype=np.float32) * (-1000.0))
+ anno['rotation_y'].append(0.0)
+ anno['score'].append(bbox[i, 4])
+ num_example += 1
+
+ if num_example == 0:
+ annos.append(
+ dict(
+ name=np.array([]),
+ truncated=np.array([]),
+ occluded=np.array([]),
+ alpha=np.array([]),
+ bbox=np.zeros([0, 4]),
+ dimensions=np.zeros([0, 3]),
+ location=np.zeros([0, 3]),
+ rotation_y=np.array([]),
+ score=np.array([]),
+ ))
+ else:
+ anno = {k: np.stack(v) for k, v in anno.items()}
+ annos.append(anno)
+
+ annos[-1]['sample_idx'] = np.array(
+ [sample_idx] * num_example, dtype=np.int64)
+ det_annos += annos
+
+ if pklfile_prefix is not None:
+ # save file in pkl format
+ pklfile_path = (
+ pklfile_prefix[:-4] if pklfile_prefix.endswith(
+ ('.pkl', '.pickle')) else pklfile_prefix)
+ mmcv.dump(det_annos, pklfile_path)
+
+ if submission_prefix is not None:
+ # save file in submission format
+ mmcv.mkdir_or_exist(submission_prefix)
+ print(f'Saving KITTI submission to {submission_prefix}')
+ for i, anno in enumerate(det_annos):
+ sample_idx = self.data_infos[i]['image']['image_idx']
+ cur_det_file = f'{submission_prefix}/{sample_idx:06d}.txt'
+ with open(cur_det_file, 'w') as f:
+ bbox = anno['bbox']
+ loc = anno['location']
+ dims = anno['dimensions'][::-1] # lhw -> hwl
+ for idx in range(len(bbox)):
+ print(
+ '{} -1 -1 {:4f} {:4f} {:4f} {:4f} {:4f} {:4f} '
+ '{:4f} {:4f} {:4f} {:4f} {:4f} {:4f} {:4f}'.format(
+ anno['name'][idx],
+ anno['alpha'][idx],
+ *bbox[idx], # 4 float
+ *dims[idx], # 3 float
+ *loc[idx], # 3 float
+ anno['rotation_y'][idx],
+ anno['score'][idx]),
+ file=f,
+ )
+ print(f'Result is saved to {submission_prefix}')
+
+ return det_annos
+
+ def convert_valid_bboxes(self, box_dict, info):
+ """Convert the predicted boxes into valid ones.
+
+ Args:
+ box_dict (dict): Box dictionaries to be converted.
+
+ - boxes_3d (:obj:`LiDARInstance3DBoxes`): 3D bounding boxes.
+ - scores_3d (torch.Tensor): Scores of boxes.
+ - labels_3d (torch.Tensor): Class labels of boxes.
+ info (dict): Data info.
+
+ Returns:
+ dict: Valid predicted boxes.
+
+ - bbox (np.ndarray): 2D bounding boxes.
+ - box3d_camera (np.ndarray): 3D bounding boxes in
+ camera coordinate.
+ - box3d_lidar (np.ndarray): 3D bounding boxes in
+ LiDAR coordinate.
+ - scores (np.ndarray): Scores of boxes.
+ - label_preds (np.ndarray): Class label predictions.
+ - sample_idx (int): Sample index.
+ """
+ # TODO: refactor this function
+ box_preds = box_dict['boxes_3d']
+ scores = box_dict['scores_3d']
+ labels = box_dict['labels_3d']
+ sample_idx = info['image']['image_idx']
+ box_preds.limit_yaw(offset=0.5, period=np.pi * 2)
+
+ if len(box_preds) == 0:
+ return dict(
+ bbox=np.zeros([0, 4]),
+ box3d_camera=np.zeros([0, 7]),
+ box3d_lidar=np.zeros([0, 7]),
+ scores=np.zeros([0]),
+ label_preds=np.zeros([0, 4]),
+ sample_idx=sample_idx)
+
+ rect = info['calib']['R0_rect'].astype(np.float32)
+ Trv2c = info['calib']['Tr_velo_to_cam'].astype(np.float32)
+ P2 = info['calib']['P2'].astype(np.float32)
+ img_shape = info['image']['image_shape']
+ P2 = box_preds.tensor.new_tensor(P2)
+
+ box_preds_camera = box_preds.convert_to(Box3DMode.CAM, rect @ Trv2c)
+
+ box_corners = box_preds_camera.corners
+ box_corners_in_image = points_cam2img(box_corners, P2)
+ # box_corners_in_image: [N, 8, 2]
+ minxy = torch.min(box_corners_in_image, dim=1)[0]
+ maxxy = torch.max(box_corners_in_image, dim=1)[0]
+ box_2d_preds = torch.cat([minxy, maxxy], dim=1)
+ # Post-processing
+ # check box_preds_camera
+ image_shape = box_preds.tensor.new_tensor(img_shape)
+ valid_cam_inds = ((box_2d_preds[:, 0] < image_shape[1]) &
+ (box_2d_preds[:, 1] < image_shape[0]) &
+ (box_2d_preds[:, 2] > 0) & (box_2d_preds[:, 3] > 0))
+ # check box_preds
+ limit_range = box_preds.tensor.new_tensor(self.pcd_limit_range)
+ valid_pcd_inds = ((box_preds.center > limit_range[:3]) &
+ (box_preds.center < limit_range[3:]))
+ valid_inds = valid_cam_inds & valid_pcd_inds.all(-1)
+
+ if valid_inds.sum() > 0:
+ return dict(
+ bbox=box_2d_preds[valid_inds, :].numpy(),
+ box3d_camera=box_preds_camera[valid_inds].tensor.numpy(),
+ box3d_lidar=box_preds[valid_inds].tensor.numpy(),
+ scores=scores[valid_inds].numpy(),
+ label_preds=labels[valid_inds].numpy(),
+ sample_idx=sample_idx)
+ else:
+ return dict(
+ bbox=np.zeros([0, 4]),
+ box3d_camera=np.zeros([0, 7]),
+ box3d_lidar=np.zeros([0, 7]),
+ scores=np.zeros([0]),
+ label_preds=np.zeros([0, 4]),
+ sample_idx=sample_idx)
+
+ def _build_default_pipeline(self):
+ """Build the default pipeline for this dataset."""
+ pipeline = [
+ dict(
+ type='LoadPointsFromFile',
+ coord_type='LIDAR',
+ load_dim=4,
+ use_dim=4,
+ file_client_args=dict(backend='disk')),
+ dict(
+ type='DefaultFormatBundle3D',
+ class_names=self.CLASSES,
+ with_label=False),
+ dict(type='Collect3D', keys=['points'])
+ ]
+ if self.modality['use_camera']:
+ pipeline.insert(0, dict(type='LoadImageFromFile'))
+ return Compose(pipeline)
+
+ def show(self, results, out_dir, show=True, pipeline=None):
+ """Results visualization.
+
+ Args:
+ results (list[dict]): List of bounding boxes results.
+ out_dir (str): Output directory of visualization result.
+ show (bool): Whether to visualize the results online.
+ Default: False.
+ pipeline (list[dict], optional): raw data loading for showing.
+ Default: None.
+ """
+ assert out_dir is not None, 'Expect out_dir, got none.'
+ pipeline = self._get_pipeline(pipeline)
+ for i, result in enumerate(results):
+ if 'pts_bbox' in result.keys():
+ result = result['pts_bbox']
+ data_info = self.data_infos[i]
+ pts_path = data_info['point_cloud']['velodyne_path']
+ file_name = osp.split(pts_path)[-1].split('.')[0]
+ points, img_metas, img = self._extract_data(
+ i, pipeline, ['points', 'img_metas', 'img'])
+ points = points.numpy()
+ # for now we convert points into depth mode
+ points = Coord3DMode.convert_point(points, Coord3DMode.LIDAR,
+ Coord3DMode.DEPTH)
+ gt_bboxes = self.get_ann_info(i)['gt_bboxes_3d'].tensor.numpy()
+ show_gt_bboxes = Box3DMode.convert(gt_bboxes, Box3DMode.LIDAR,
+ Box3DMode.DEPTH)
+ pred_bboxes = result['boxes_3d'].tensor.numpy()
+ show_pred_bboxes = Box3DMode.convert(pred_bboxes, Box3DMode.LIDAR,
+ Box3DMode.DEPTH)
+ show_result(points, show_gt_bboxes, show_pred_bboxes, out_dir,
+ file_name, show)
+
+ # multi-modality visualization
+ if self.modality['use_camera'] and 'lidar2img' in img_metas.keys():
+ img = img.numpy()
+ # need to transpose channel to first dim
+ img = img.transpose(1, 2, 0)
+ show_pred_bboxes = LiDARInstance3DBoxes(
+ pred_bboxes, origin=(0.5, 0.5, 0))
+ show_gt_bboxes = LiDARInstance3DBoxes(
+ gt_bboxes, origin=(0.5, 0.5, 0))
+ show_multi_modality_result(
+ img,
+ show_gt_bboxes,
+ show_pred_bboxes,
+ img_metas['lidar2img'],
+ out_dir,
+ file_name,
+ box_mode='lidar',
+ show=show)
diff --git a/mmdet3d/datasets/kitti_mono_dataset.py b/mmdet3d/datasets/kitti_mono_dataset.py
new file mode 100644
index 0000000..c669b0a
--- /dev/null
+++ b/mmdet3d/datasets/kitti_mono_dataset.py
@@ -0,0 +1,569 @@
+# Copyright (c) OpenMMLab. All rights reserved.
+import copy
+import tempfile
+from os import path as osp
+
+import mmcv
+import numpy as np
+import torch
+from mmcv.utils import print_log
+
+from ..core.bbox import Box3DMode, CameraInstance3DBoxes, points_cam2img
+from .builder import DATASETS
+from .nuscenes_mono_dataset import NuScenesMonoDataset
+
+
+@DATASETS.register_module()
+class KittiMonoDataset(NuScenesMonoDataset):
+ """Monocular 3D detection on KITTI Dataset.
+
+ Args:
+ data_root (str): Path of dataset root.
+ info_file (str): Path of info file.
+ load_interval (int, optional): Interval of loading the dataset. It is
+ used to uniformly sample the dataset. Defaults to 1.
+ with_velocity (bool, optional): Whether include velocity prediction
+ into the experiments. Defaults to False.
+ eval_version (str, optional): Configuration version of evaluation.
+ Defaults to None.
+ version (str, optional): Dataset version. Defaults to None.
+ kwargs (dict): Other arguments are the same of NuScenesMonoDataset.
+ """
+
+ CLASSES = ('Pedestrian', 'Cyclist', 'Car')
+
+ def __init__(self,
+ data_root,
+ info_file,
+ ann_file,
+ pipeline,
+ load_interval=1,
+ with_velocity=False,
+ eval_version=None,
+ version=None,
+ **kwargs):
+ super().__init__(
+ data_root=data_root,
+ ann_file=ann_file,
+ pipeline=pipeline,
+ load_interval=load_interval,
+ with_velocity=with_velocity,
+ eval_version=eval_version,
+ version=version,
+ **kwargs)
+ self.anno_infos = mmcv.load(info_file)
+ self.bbox_code_size = 7
+
+ def _parse_ann_info(self, img_info, ann_info):
+ """Parse bbox and mask annotation.
+
+ Args:
+ ann_info (list[dict]): Annotation info of an image.
+ with_mask (bool): Whether to parse mask annotations.
+
+ Returns:
+ dict: A dict containing the following keys: bboxes, bboxes_ignore,
+ labels, masks, seg_map. "masks" are raw annotations and not
+ decoded into binary masks.
+ """
+ gt_bboxes = []
+ gt_labels = []
+ gt_bboxes_ignore = []
+ gt_masks_ann = []
+ gt_bboxes_cam3d = []
+ centers2d = []
+ depths = []
+ for i, ann in enumerate(ann_info):
+ if ann.get('ignore', False):
+ continue
+ x1, y1, w, h = ann['bbox']
+ inter_w = max(0, min(x1 + w, img_info['width']) - max(x1, 0))
+ inter_h = max(0, min(y1 + h, img_info['height']) - max(y1, 0))
+ if inter_w * inter_h == 0:
+ continue
+ if ann['area'] <= 0 or w < 1 or h < 1:
+ continue
+ if ann['category_id'] not in self.cat_ids:
+ continue
+ bbox = [x1, y1, x1 + w, y1 + h]
+ if ann.get('iscrowd', False):
+ gt_bboxes_ignore.append(bbox)
+ else:
+ gt_bboxes.append(bbox)
+ gt_labels.append(self.cat2label[ann['category_id']])
+ gt_masks_ann.append(ann.get('segmentation', None))
+ # 3D annotations in camera coordinates
+ bbox_cam3d = np.array(ann['bbox_cam3d']).reshape(-1, )
+ gt_bboxes_cam3d.append(bbox_cam3d)
+ # 2.5D annotations in camera coordinates
+ center2d = ann['center2d'][:2]
+ depth = ann['center2d'][2]
+ centers2d.append(center2d)
+ depths.append(depth)
+
+ if gt_bboxes:
+ gt_bboxes = np.array(gt_bboxes, dtype=np.float32)
+ gt_labels = np.array(gt_labels, dtype=np.int64)
+ else:
+ gt_bboxes = np.zeros((0, 4), dtype=np.float32)
+ gt_labels = np.array([], dtype=np.int64)
+
+ if gt_bboxes_cam3d:
+ gt_bboxes_cam3d = np.array(gt_bboxes_cam3d, dtype=np.float32)
+ centers2d = np.array(centers2d, dtype=np.float32)
+ depths = np.array(depths, dtype=np.float32)
+ else:
+ gt_bboxes_cam3d = np.zeros((0, self.bbox_code_size),
+ dtype=np.float32)
+ centers2d = np.zeros((0, 2), dtype=np.float32)
+ depths = np.zeros((0), dtype=np.float32)
+
+ gt_bboxes_cam3d = CameraInstance3DBoxes(
+ gt_bboxes_cam3d,
+ box_dim=gt_bboxes_cam3d.shape[-1],
+ origin=(0.5, 0.5, 0.5))
+ gt_labels_3d = copy.deepcopy(gt_labels)
+
+ if gt_bboxes_ignore:
+ gt_bboxes_ignore = np.array(gt_bboxes_ignore, dtype=np.float32)
+ else:
+ gt_bboxes_ignore = np.zeros((0, 4), dtype=np.float32)
+
+ seg_map = img_info['filename'].replace('jpg', 'png')
+
+ ann = dict(
+ bboxes=gt_bboxes,
+ labels=gt_labels,
+ gt_bboxes_3d=gt_bboxes_cam3d,
+ gt_labels_3d=gt_labels_3d,
+ centers2d=centers2d,
+ depths=depths,
+ bboxes_ignore=gt_bboxes_ignore,
+ masks=gt_masks_ann,
+ seg_map=seg_map)
+
+ return ann
+
+ def format_results(self,
+ outputs,
+ pklfile_prefix=None,
+ submission_prefix=None):
+ """Format the results to pkl file.
+
+ Args:
+ outputs (list[dict]): Testing results of the dataset.
+ pklfile_prefix (str): The prefix of pkl files. It includes
+ the file path and the prefix of filename, e.g., "a/b/prefix".
+ If not specified, a temp file will be created. Default: None.
+ submission_prefix (str): The prefix of submitted files. It
+ includes the file path and the prefix of filename, e.g.,
+ "a/b/prefix". If not specified, a temp file will be created.
+ Default: None.
+
+ Returns:
+ tuple: (result_files, tmp_dir), result_files is a dict containing
+ the json filepaths, tmp_dir is the temporal directory created
+ for saving json files when jsonfile_prefix is not specified.
+ """
+ if pklfile_prefix is None:
+ tmp_dir = tempfile.TemporaryDirectory()
+ pklfile_prefix = osp.join(tmp_dir.name, 'results')
+ else:
+ tmp_dir = None
+
+ if not isinstance(outputs[0], dict):
+ result_files = self.bbox2result_kitti2d(outputs, self.CLASSES,
+ pklfile_prefix,
+ submission_prefix)
+ elif 'pts_bbox' in outputs[0] or 'img_bbox' in outputs[0] or \
+ 'img_bbox2d' in outputs[0]:
+ result_files = dict()
+ for name in outputs[0]:
+ results_ = [out[name] for out in outputs]
+ pklfile_prefix_ = pklfile_prefix + name
+ if submission_prefix is not None:
+ submission_prefix_ = submission_prefix + name
+ else:
+ submission_prefix_ = None
+ if '2d' in name:
+ result_files_ = self.bbox2result_kitti2d(
+ results_, self.CLASSES, pklfile_prefix_,
+ submission_prefix_)
+ else:
+ result_files_ = self.bbox2result_kitti(
+ results_, self.CLASSES, pklfile_prefix_,
+ submission_prefix_)
+ result_files[name] = result_files_
+ else:
+ result_files = self.bbox2result_kitti(outputs, self.CLASSES,
+ pklfile_prefix,
+ submission_prefix)
+ return result_files, tmp_dir
+
+ def evaluate(self,
+ results,
+ metric=None,
+ logger=None,
+ pklfile_prefix=None,
+ submission_prefix=None,
+ show=False,
+ out_dir=None,
+ pipeline=None):
+ """Evaluation in KITTI protocol.
+
+ Args:
+ results (list[dict]): Testing results of the dataset.
+ metric (str | list[str], optional): Metrics to be evaluated.
+ Defaults to None.
+ logger (logging.Logger | str, optional): Logger used for printing
+ related information during evaluation. Default: None.
+ pklfile_prefix (str, optional): The prefix of pkl files, including
+ the file path and the prefix of filename, e.g., "a/b/prefix".
+ If not specified, a temp file will be created. Default: None.
+ submission_prefix (str, optional): The prefix of submission data.
+ If not specified, the submission data will not be generated.
+ show (bool, optional): Whether to visualize.
+ Default: False.
+ out_dir (str, optional): Path to save the visualization results.
+ Default: None.
+ pipeline (list[dict], optional): raw data loading for showing.
+ Default: None.
+
+ Returns:
+ dict[str, float]: Results of each evaluation metric.
+ """
+ result_files, tmp_dir = self.format_results(results, pklfile_prefix)
+ from mmdet3d.core.evaluation import kitti_eval
+ gt_annos = [info['annos'] for info in self.anno_infos]
+
+ if isinstance(result_files, dict):
+ ap_dict = dict()
+ for name, result_files_ in result_files.items():
+ eval_types = ['bbox', 'bev', '3d']
+ if '2d' in name:
+ eval_types = ['bbox']
+ ap_result_str, ap_dict_ = kitti_eval(
+ gt_annos,
+ result_files_,
+ self.CLASSES,
+ eval_types=eval_types)
+ for ap_type, ap in ap_dict_.items():
+ ap_dict[f'{name}/{ap_type}'] = float('{:.4f}'.format(ap))
+
+ print_log(
+ f'Results of {name}:\n' + ap_result_str, logger=logger)
+
+ else:
+ if metric == 'img_bbox2d':
+ ap_result_str, ap_dict = kitti_eval(
+ gt_annos, result_files, self.CLASSES, eval_types=['bbox'])
+ else:
+ ap_result_str, ap_dict = kitti_eval(gt_annos, result_files,
+ self.CLASSES)
+ print_log('\n' + ap_result_str, logger=logger)
+
+ if tmp_dir is not None:
+ tmp_dir.cleanup()
+ if show or out_dir:
+ self.show(results, out_dir, show=show, pipeline=pipeline)
+ return ap_dict
+
+ def bbox2result_kitti(self,
+ net_outputs,
+ class_names,
+ pklfile_prefix=None,
+ submission_prefix=None):
+ """Convert 3D detection results to kitti format for evaluation and test
+ submission.
+
+ Args:
+ net_outputs (list[np.ndarray]): List of array storing the
+ inferenced bounding boxes and scores.
+ class_names (list[String]): A list of class names.
+ pklfile_prefix (str): The prefix of pkl file.
+ submission_prefix (str): The prefix of submission file.
+
+ Returns:
+ list[dict]: A list of dictionaries with the kitti format.
+ """
+ assert len(net_outputs) == len(self.anno_infos)
+ if submission_prefix is not None:
+ mmcv.mkdir_or_exist(submission_prefix)
+
+ det_annos = []
+ print('\nConverting prediction to KITTI format')
+ for idx, pred_dicts in enumerate(
+ mmcv.track_iter_progress(net_outputs)):
+ annos = []
+ info = self.anno_infos[idx]
+ sample_idx = info['image']['image_idx']
+ image_shape = info['image']['image_shape'][:2]
+
+ box_dict = self.convert_valid_bboxes(pred_dicts, info)
+ anno = {
+ 'name': [],
+ 'truncated': [],
+ 'occluded': [],
+ 'alpha': [],
+ 'bbox': [],
+ 'dimensions': [],
+ 'location': [],
+ 'rotation_y': [],
+ 'score': []
+ }
+ if len(box_dict['bbox']) > 0:
+ box_2d_preds = box_dict['bbox']
+ box_preds = box_dict['box3d_camera']
+ scores = box_dict['scores']
+ box_preds_lidar = box_dict['box3d_lidar']
+ label_preds = box_dict['label_preds']
+
+ for box, box_lidar, bbox, score, label in zip(
+ box_preds, box_preds_lidar, box_2d_preds, scores,
+ label_preds):
+ bbox[2:] = np.minimum(bbox[2:], image_shape[::-1])
+ bbox[:2] = np.maximum(bbox[:2], [0, 0])
+ anno['name'].append(class_names[int(label)])
+ anno['truncated'].append(0.0)
+ anno['occluded'].append(0)
+ anno['alpha'].append(-np.arctan2(box[0], box[2]) + box[6])
+ anno['bbox'].append(bbox)
+ anno['dimensions'].append(box[3:6])
+ anno['location'].append(box[:3])
+ anno['rotation_y'].append(box[6])
+ anno['score'].append(score)
+
+ anno = {k: np.stack(v) for k, v in anno.items()}
+ annos.append(anno)
+
+ else:
+ anno = {
+ 'name': np.array([]),
+ 'truncated': np.array([]),
+ 'occluded': np.array([]),
+ 'alpha': np.array([]),
+ 'bbox': np.zeros([0, 4]),
+ 'dimensions': np.zeros([0, 3]),
+ 'location': np.zeros([0, 3]),
+ 'rotation_y': np.array([]),
+ 'score': np.array([]),
+ }
+ annos.append(anno)
+
+ if submission_prefix is not None:
+ curr_file = f'{submission_prefix}/{sample_idx:06d}.txt'
+ with open(curr_file, 'w') as f:
+ bbox = anno['bbox']
+ loc = anno['location']
+ dims = anno['dimensions'] # lhw -> hwl
+
+ for idx in range(len(bbox)):
+ print(
+ '{} -1 -1 {:.4f} {:.4f} {:.4f} {:.4f} '
+ '{:.4f} {:.4f} {:.4f} '
+ '{:.4f} {:.4f} {:.4f} {:.4f} {:.4f} {:.4f}'.format(
+ anno['name'][idx], anno['alpha'][idx],
+ bbox[idx][0], bbox[idx][1], bbox[idx][2],
+ bbox[idx][3], dims[idx][1], dims[idx][2],
+ dims[idx][0], loc[idx][0], loc[idx][1],
+ loc[idx][2], anno['rotation_y'][idx],
+ anno['score'][idx]),
+ file=f)
+
+ annos[-1]['sample_idx'] = np.array(
+ [sample_idx] * len(annos[-1]['score']), dtype=np.int64)
+
+ det_annos += annos
+
+ if pklfile_prefix is not None:
+ if not pklfile_prefix.endswith(('.pkl', '.pickle')):
+ out = f'{pklfile_prefix}.pkl'
+ mmcv.dump(det_annos, out)
+ print('Result is saved to %s' % out)
+
+ return det_annos
+
+ def bbox2result_kitti2d(self,
+ net_outputs,
+ class_names,
+ pklfile_prefix=None,
+ submission_prefix=None):
+ """Convert 2D detection results to kitti format for evaluation and test
+ submission.
+
+ Args:
+ net_outputs (list[np.ndarray]): List of array storing the
+ inferenced bounding boxes and scores.
+ class_names (list[String]): A list of class names.
+ pklfile_prefix (str): The prefix of pkl file.
+ submission_prefix (str): The prefix of submission file.
+
+ Returns:
+ list[dict]: A list of dictionaries have the kitti format
+ """
+ assert len(net_outputs) == len(self.anno_infos)
+
+ det_annos = []
+ print('\nConverting prediction to KITTI format')
+ for i, bboxes_per_sample in enumerate(
+ mmcv.track_iter_progress(net_outputs)):
+ annos = []
+ anno = dict(
+ name=[],
+ truncated=[],
+ occluded=[],
+ alpha=[],
+ bbox=[],
+ dimensions=[],
+ location=[],
+ rotation_y=[],
+ score=[])
+ sample_idx = self.anno_infos[i]['image']['image_idx']
+
+ num_example = 0
+ for label in range(len(bboxes_per_sample)):
+ bbox = bboxes_per_sample[label]
+ for i in range(bbox.shape[0]):
+ anno['name'].append(class_names[int(label)])
+ anno['truncated'].append(0.0)
+ anno['occluded'].append(0)
+ anno['alpha'].append(-10)
+ anno['bbox'].append(bbox[i, :4])
+ # set dimensions (height, width, length) to zero
+ anno['dimensions'].append(
+ np.zeros(shape=[3], dtype=np.float32))
+ # set the 3D translation to (-1000, -1000, -1000)
+ anno['location'].append(
+ np.ones(shape=[3], dtype=np.float32) * (-1000.0))
+ anno['rotation_y'].append(0.0)
+ anno['score'].append(bbox[i, 4])
+ num_example += 1
+
+ if num_example == 0:
+ annos.append(
+ dict(
+ name=np.array([]),
+ truncated=np.array([]),
+ occluded=np.array([]),
+ alpha=np.array([]),
+ bbox=np.zeros([0, 4]),
+ dimensions=np.zeros([0, 3]),
+ location=np.zeros([0, 3]),
+ rotation_y=np.array([]),
+ score=np.array([]),
+ ))
+ else:
+ anno = {k: np.stack(v) for k, v in anno.items()}
+ annos.append(anno)
+
+ annos[-1]['sample_idx'] = np.array(
+ [sample_idx] * num_example, dtype=np.int64)
+ det_annos += annos
+
+ if pklfile_prefix is not None:
+ if not pklfile_prefix.endswith(('.pkl', '.pickle')):
+ out = f'{pklfile_prefix}.pkl'
+ mmcv.dump(det_annos, out)
+ print('Result is saved to %s' % out)
+
+ if submission_prefix is not None:
+ # save file in submission format
+ mmcv.mkdir_or_exist(submission_prefix)
+ print(f'Saving KITTI submission to {submission_prefix}')
+ for i, anno in enumerate(det_annos):
+ sample_idx = self.anno_infos[i]['image']['image_idx']
+ cur_det_file = f'{submission_prefix}/{sample_idx:06d}.txt'
+ with open(cur_det_file, 'w') as f:
+ bbox = anno['bbox']
+ loc = anno['location']
+ dims = anno['dimensions'][::-1] # lhw -> hwl
+ for idx in range(len(bbox)):
+ print(
+ '{} -1 -1 {:4f} {:4f} {:4f} {:4f} {:4f} {:4f} '
+ '{:4f} {:4f} {:4f} {:4f} {:4f} {:4f} {:4f}'.format(
+ anno['name'][idx],
+ anno['alpha'][idx],
+ *bbox[idx], # 4 float
+ *dims[idx], # 3 float
+ *loc[idx], # 3 float
+ anno['rotation_y'][idx],
+ anno['score'][idx]),
+ file=f,
+ )
+ print(f'Result is saved to {submission_prefix}')
+
+ return det_annos
+
+ def convert_valid_bboxes(self, box_dict, info):
+ """Convert the predicted boxes into valid ones.
+
+ Args:
+ box_dict (dict): Box dictionaries to be converted.
+ - boxes_3d (:obj:`CameraInstance3DBoxes`): 3D bounding boxes.
+ - scores_3d (torch.Tensor): Scores of boxes.
+ - labels_3d (torch.Tensor): Class labels of boxes.
+ info (dict): Data info.
+
+ Returns:
+ dict: Valid predicted boxes.
+ - bbox (np.ndarray): 2D bounding boxes.
+ - box3d_camera (np.ndarray): 3D bounding boxes in
+ camera coordinate.
+ - scores (np.ndarray): Scores of boxes.
+ - label_preds (np.ndarray): Class label predictions.
+ - sample_idx (int): Sample index.
+ """
+ box_preds = box_dict['boxes_3d']
+ scores = box_dict['scores_3d']
+ labels = box_dict['labels_3d']
+ sample_idx = info['image']['image_idx']
+
+ if len(box_preds) == 0:
+ return dict(
+ bbox=np.zeros([0, 4]),
+ box3d_camera=np.zeros([0, 7]),
+ scores=np.zeros([0]),
+ label_preds=np.zeros([0, 4]),
+ sample_idx=sample_idx)
+
+ rect = info['calib']['R0_rect'].astype(np.float32)
+ Trv2c = info['calib']['Tr_velo_to_cam'].astype(np.float32)
+ P2 = info['calib']['P2'].astype(np.float32)
+ img_shape = info['image']['image_shape']
+ P2 = box_preds.tensor.new_tensor(P2)
+
+ box_preds_camera = box_preds
+ box_preds_lidar = box_preds.convert_to(Box3DMode.LIDAR,
+ np.linalg.inv(rect @ Trv2c))
+
+ box_corners = box_preds_camera.corners
+ box_corners_in_image = points_cam2img(box_corners, P2)
+ # box_corners_in_image: [N, 8, 2]
+ minxy = torch.min(box_corners_in_image, dim=1)[0]
+ maxxy = torch.max(box_corners_in_image, dim=1)[0]
+ box_2d_preds = torch.cat([minxy, maxxy], dim=1)
+ # Post-processing
+ # check box_preds_camera
+ image_shape = box_preds.tensor.new_tensor(img_shape)
+ valid_cam_inds = ((box_2d_preds[:, 0] < image_shape[1]) &
+ (box_2d_preds[:, 1] < image_shape[0]) &
+ (box_2d_preds[:, 2] > 0) & (box_2d_preds[:, 3] > 0))
+ # check box_preds
+ valid_inds = valid_cam_inds
+
+ if valid_inds.sum() > 0:
+ return dict(
+ bbox=box_2d_preds[valid_inds, :].numpy(),
+ box3d_camera=box_preds_camera[valid_inds].tensor.numpy(),
+ box3d_lidar=box_preds_lidar[valid_inds].tensor.numpy(),
+ scores=scores[valid_inds].numpy(),
+ label_preds=labels[valid_inds].numpy(),
+ sample_idx=sample_idx)
+ else:
+ return dict(
+ bbox=np.zeros([0, 4]),
+ box3d_camera=np.zeros([0, 7]),
+ box3d_lidar=np.zeros([0, 7]),
+ scores=np.zeros([0]),
+ label_preds=np.zeros([0, 4]),
+ sample_idx=sample_idx)
diff --git a/mmdet3d/datasets/lyft_dataset.py b/mmdet3d/datasets/lyft_dataset.py
new file mode 100644
index 0000000..031d86a
--- /dev/null
+++ b/mmdet3d/datasets/lyft_dataset.py
@@ -0,0 +1,567 @@
+# Copyright (c) OpenMMLab. All rights reserved.
+import os
+import tempfile
+from os import path as osp
+
+import mmcv
+import numpy as np
+import pandas as pd
+from lyft_dataset_sdk.lyftdataset import LyftDataset as Lyft
+from lyft_dataset_sdk.utils.data_classes import Box as LyftBox
+from pyquaternion import Quaternion
+
+from mmdet3d.core.evaluation.lyft_eval import lyft_eval
+from ..core import show_result
+from ..core.bbox import Box3DMode, Coord3DMode, LiDARInstance3DBoxes
+from .builder import DATASETS
+from .custom_3d import Custom3DDataset
+from .pipelines import Compose
+
+
+@DATASETS.register_module()
+class LyftDataset(Custom3DDataset):
+ r"""Lyft Dataset.
+
+ This class serves as the API for experiments on the Lyft Dataset.
+
+ Please refer to
+ ``_
+ for data downloading.
+
+ Args:
+ ann_file (str): Path of annotation file.
+ pipeline (list[dict], optional): Pipeline used for data processing.
+ Defaults to None.
+ data_root (str): Path of dataset root.
+ classes (tuple[str], optional): Classes used in the dataset.
+ Defaults to None.
+ load_interval (int, optional): Interval of loading the dataset. It is
+ used to uniformly sample the dataset. Defaults to 1.
+ modality (dict, optional): Modality to specify the sensor data used
+ as input. Defaults to None.
+ box_type_3d (str, optional): Type of 3D box of this dataset.
+ Based on the `box_type_3d`, the dataset will encapsulate the box
+ to its original format then converted them to `box_type_3d`.
+ Defaults to 'LiDAR' in this dataset. Available options includes
+
+ - 'LiDAR': Box in LiDAR coordinates.
+ - 'Depth': Box in depth coordinates, usually for indoor dataset.
+ - 'Camera': Box in camera coordinates.
+ filter_empty_gt (bool, optional): Whether to filter empty GT.
+ Defaults to True.
+ test_mode (bool, optional): Whether the dataset is in test mode.
+ Defaults to False.
+ """ # noqa: E501
+ NameMapping = {
+ 'bicycle': 'bicycle',
+ 'bus': 'bus',
+ 'car': 'car',
+ 'emergency_vehicle': 'emergency_vehicle',
+ 'motorcycle': 'motorcycle',
+ 'other_vehicle': 'other_vehicle',
+ 'pedestrian': 'pedestrian',
+ 'truck': 'truck',
+ 'animal': 'animal'
+ }
+ DefaultAttribute = {
+ 'car': 'is_stationary',
+ 'truck': 'is_stationary',
+ 'bus': 'is_stationary',
+ 'emergency_vehicle': 'is_stationary',
+ 'other_vehicle': 'is_stationary',
+ 'motorcycle': 'is_stationary',
+ 'bicycle': 'is_stationary',
+ 'pedestrian': 'is_stationary',
+ 'animal': 'is_stationary'
+ }
+ CLASSES = ('car', 'truck', 'bus', 'emergency_vehicle', 'other_vehicle',
+ 'motorcycle', 'bicycle', 'pedestrian', 'animal')
+
+ def __init__(self,
+ ann_file,
+ pipeline=None,
+ data_root=None,
+ classes=None,
+ load_interval=1,
+ modality=None,
+ box_type_3d='LiDAR',
+ filter_empty_gt=True,
+ test_mode=False,
+ **kwargs):
+ self.load_interval = load_interval
+ super().__init__(
+ data_root=data_root,
+ ann_file=ann_file,
+ pipeline=pipeline,
+ classes=classes,
+ modality=modality,
+ box_type_3d=box_type_3d,
+ filter_empty_gt=filter_empty_gt,
+ test_mode=test_mode,
+ **kwargs)
+
+ if self.modality is None:
+ self.modality = dict(
+ use_camera=False,
+ use_lidar=True,
+ use_radar=False,
+ use_map=False,
+ use_external=False,
+ )
+
+ def load_annotations(self, ann_file):
+ """Load annotations from ann_file.
+
+ Args:
+ ann_file (str): Path of the annotation file.
+
+ Returns:
+ list[dict]: List of annotations sorted by timestamps.
+ """
+ # loading data from a file-like object needs file format
+ data = mmcv.load(ann_file, file_format='pkl')
+ data_infos = list(sorted(data['infos'], key=lambda e: e['timestamp']))
+ data_infos = data_infos[::self.load_interval]
+ self.metadata = data['metadata']
+ self.version = self.metadata['version']
+ return data_infos
+
+ def get_data_info(self, index):
+ """Get data info according to the given index.
+
+ Args:
+ index (int): Index of the sample data to get.
+
+ Returns:
+ dict: Data information that will be passed to the data
+ preprocessing pipelines. It includes the following keys:
+
+ - sample_idx (str): sample index
+ - pts_filename (str): filename of point clouds
+ - sweeps (list[dict]): infos of sweeps
+ - timestamp (float): sample timestamp
+ - img_filename (str, optional): image filename
+ - lidar2img (list[np.ndarray], optional): transformations
+ from lidar to different cameras
+ - ann_info (dict): annotation info
+ """
+ info = self.data_infos[index]
+
+ # standard protocol modified from SECOND.Pytorch
+ input_dict = dict(
+ sample_idx=info['token'],
+ pts_filename=info['lidar_path'],
+ sweeps=info['sweeps'],
+ timestamp=info['timestamp'] / 1e6,
+ )
+
+ if self.modality['use_camera']:
+ image_paths = []
+ lidar2img_rts = []
+ for cam_type, cam_info in info['cams'].items():
+ image_paths.append(cam_info['data_path'])
+ # obtain lidar to image transformation matrix
+ lidar2cam_r = np.linalg.inv(cam_info['sensor2lidar_rotation'])
+ lidar2cam_t = cam_info[
+ 'sensor2lidar_translation'] @ lidar2cam_r.T
+ lidar2cam_rt = np.eye(4)
+ lidar2cam_rt[:3, :3] = lidar2cam_r.T
+ lidar2cam_rt[3, :3] = -lidar2cam_t
+ intrinsic = cam_info['cam_intrinsic']
+ viewpad = np.eye(4)
+ viewpad[:intrinsic.shape[0], :intrinsic.shape[1]] = intrinsic
+ lidar2img_rt = (viewpad @ lidar2cam_rt.T)
+ lidar2img_rts.append(lidar2img_rt)
+
+ input_dict.update(
+ dict(
+ img_filename=image_paths,
+ lidar2img=lidar2img_rts,
+ ))
+
+ if not self.test_mode:
+ annos = self.get_ann_info(index)
+ input_dict['ann_info'] = annos
+
+ return input_dict
+
+ def get_ann_info(self, index):
+ """Get annotation info according to the given index.
+
+ Args:
+ index (int): Index of the annotation data to get.
+
+ Returns:
+ dict: Annotation information consists of the following keys:
+
+ - gt_bboxes_3d (:obj:`LiDARInstance3DBoxes`):
+ 3D ground truth bboxes.
+ - gt_labels_3d (np.ndarray): Labels of ground truths.
+ - gt_names (list[str]): Class names of ground truths.
+ """
+ info = self.data_infos[index]
+ gt_bboxes_3d = info['gt_boxes']
+ gt_names_3d = info['gt_names']
+ gt_labels_3d = []
+ for cat in gt_names_3d:
+ if cat in self.CLASSES:
+ gt_labels_3d.append(self.CLASSES.index(cat))
+ else:
+ gt_labels_3d.append(-1)
+ gt_labels_3d = np.array(gt_labels_3d)
+
+ if 'gt_shape' in info:
+ gt_shape = info['gt_shape']
+ gt_bboxes_3d = np.concatenate([gt_bboxes_3d, gt_shape], axis=-1)
+
+ # the lyft box center is [0.5, 0.5, 0.5], we change it to be
+ # the same as KITTI (0.5, 0.5, 0)
+ gt_bboxes_3d = LiDARInstance3DBoxes(
+ gt_bboxes_3d,
+ box_dim=gt_bboxes_3d.shape[-1],
+ origin=(0.5, 0.5, 0.5)).convert_to(self.box_mode_3d)
+
+ anns_results = dict(
+ gt_bboxes_3d=gt_bboxes_3d,
+ gt_labels_3d=gt_labels_3d,
+ )
+ return anns_results
+
+ def _format_bbox(self, results, jsonfile_prefix=None):
+ """Convert the results to the standard format.
+
+ Args:
+ results (list[dict]): Testing results of the dataset.
+ jsonfile_prefix (str): The prefix of the output jsonfile.
+ You can specify the output directory/filename by
+ modifying the jsonfile_prefix. Default: None.
+
+ Returns:
+ str: Path of the output json file.
+ """
+ lyft_annos = {}
+ mapped_class_names = self.CLASSES
+
+ print('Start to convert detection format...')
+ for sample_id, det in enumerate(mmcv.track_iter_progress(results)):
+ annos = []
+ boxes = output_to_lyft_box(det)
+ sample_token = self.data_infos[sample_id]['token']
+ boxes = lidar_lyft_box_to_global(self.data_infos[sample_id], boxes)
+ for i, box in enumerate(boxes):
+ name = mapped_class_names[box.label]
+ lyft_anno = dict(
+ sample_token=sample_token,
+ translation=box.center.tolist(),
+ size=box.wlh.tolist(),
+ rotation=box.orientation.elements.tolist(),
+ name=name,
+ score=box.score)
+ annos.append(lyft_anno)
+ lyft_annos[sample_token] = annos
+ lyft_submissions = {
+ 'meta': self.modality,
+ 'results': lyft_annos,
+ }
+
+ mmcv.mkdir_or_exist(jsonfile_prefix)
+ res_path = osp.join(jsonfile_prefix, 'results_lyft.json')
+ print('Results writes to', res_path)
+ mmcv.dump(lyft_submissions, res_path)
+ return res_path
+
+ def _evaluate_single(self,
+ result_path,
+ logger=None,
+ metric='bbox',
+ result_name='pts_bbox'):
+ """Evaluation for a single model in Lyft protocol.
+
+ Args:
+ result_path (str): Path of the result file.
+ logger (logging.Logger | str, optional): Logger used for printing
+ related information during evaluation. Default: None.
+ metric (str, optional): Metric name used for evaluation.
+ Default: 'bbox'.
+ result_name (str, optional): Result name in the metric prefix.
+ Default: 'pts_bbox'.
+
+ Returns:
+ dict: Dictionary of evaluation details.
+ """
+
+ output_dir = osp.join(*osp.split(result_path)[:-1])
+ lyft = Lyft(
+ data_path=osp.join(self.data_root, self.version),
+ json_path=osp.join(self.data_root, self.version, self.version),
+ verbose=True)
+ eval_set_map = {
+ 'v1.01-train': 'val',
+ }
+ metrics = lyft_eval(lyft, self.data_root, result_path,
+ eval_set_map[self.version], output_dir, logger)
+
+ # record metrics
+ detail = dict()
+ metric_prefix = f'{result_name}_Lyft'
+
+ for i, name in enumerate(metrics['class_names']):
+ AP = float(metrics['mAPs_cate'][i])
+ detail[f'{metric_prefix}/{name}_AP'] = AP
+
+ detail[f'{metric_prefix}/mAP'] = metrics['Final mAP']
+ return detail
+
+ def format_results(self, results, jsonfile_prefix=None, csv_savepath=None):
+ """Format the results to json (standard format for COCO evaluation).
+
+ Args:
+ results (list[dict]): Testing results of the dataset.
+ jsonfile_prefix (str): The prefix of json files. It includes
+ the file path and the prefix of filename, e.g., "a/b/prefix".
+ If not specified, a temp file will be created. Default: None.
+ csv_savepath (str): The path for saving csv files.
+ It includes the file path and the csv filename,
+ e.g., "a/b/filename.csv". If not specified,
+ the result will not be converted to csv file.
+
+ Returns:
+ tuple: Returns (result_files, tmp_dir), where `result_files` is a
+ dict containing the json filepaths, `tmp_dir` is the temporal
+ directory created for saving json files when
+ `jsonfile_prefix` is not specified.
+ """
+ assert isinstance(results, list), 'results must be a list'
+ assert len(results) == len(self), (
+ 'The length of results is not equal to the dataset len: {} != {}'.
+ format(len(results), len(self)))
+
+ if jsonfile_prefix is None:
+ tmp_dir = tempfile.TemporaryDirectory()
+ jsonfile_prefix = osp.join(tmp_dir.name, 'results')
+ else:
+ tmp_dir = None
+
+ # currently the output prediction results could be in two formats
+ # 1. list of dict('boxes_3d': ..., 'scores_3d': ..., 'labels_3d': ...)
+ # 2. list of dict('pts_bbox' or 'img_bbox':
+ # dict('boxes_3d': ..., 'scores_3d': ..., 'labels_3d': ...))
+ # this is a workaround to enable evaluation of both formats on Lyft
+ # refer to https://github.com/open-mmlab/mmdetection3d/issues/449
+ if not ('pts_bbox' in results[0] or 'img_bbox' in results[0]):
+ result_files = self._format_bbox(results, jsonfile_prefix)
+ else:
+ # should take the inner dict out of 'pts_bbox' or 'img_bbox' dict
+ result_files = dict()
+ for name in results[0]:
+ print(f'\nFormating bboxes of {name}')
+ results_ = [out[name] for out in results]
+ tmp_file_ = osp.join(jsonfile_prefix, name)
+ result_files.update(
+ {name: self._format_bbox(results_, tmp_file_)})
+ if csv_savepath is not None:
+ self.json2csv(result_files['pts_bbox'], csv_savepath)
+ return result_files, tmp_dir
+
+ def evaluate(self,
+ results,
+ metric='bbox',
+ logger=None,
+ jsonfile_prefix=None,
+ csv_savepath=None,
+ result_names=['pts_bbox'],
+ show=False,
+ out_dir=None,
+ pipeline=None):
+ """Evaluation in Lyft protocol.
+
+ Args:
+ results (list[dict]): Testing results of the dataset.
+ metric (str | list[str], optional): Metrics to be evaluated.
+ Default: 'bbox'.
+ logger (logging.Logger | str, optional): Logger used for printing
+ related information during evaluation. Default: None.
+ jsonfile_prefix (str, optional): The prefix of json files including
+ the file path and the prefix of filename, e.g., "a/b/prefix".
+ If not specified, a temp file will be created. Default: None.
+ csv_savepath (str, optional): The path for saving csv files.
+ It includes the file path and the csv filename,
+ e.g., "a/b/filename.csv". If not specified,
+ the result will not be converted to csv file.
+ result_names (list[str], optional): Result names in the
+ metric prefix. Default: ['pts_bbox'].
+ show (bool, optional): Whether to visualize.
+ Default: False.
+ out_dir (str, optional): Path to save the visualization results.
+ Default: None.
+ pipeline (list[dict], optional): raw data loading for showing.
+ Default: None.
+
+ Returns:
+ dict[str, float]: Evaluation results.
+ """
+ result_files, tmp_dir = self.format_results(results, jsonfile_prefix,
+ csv_savepath)
+
+ if isinstance(result_files, dict):
+ results_dict = dict()
+ for name in result_names:
+ print(f'Evaluating bboxes of {name}')
+ ret_dict = self._evaluate_single(result_files[name])
+ results_dict.update(ret_dict)
+ elif isinstance(result_files, str):
+ results_dict = self._evaluate_single(result_files)
+
+ if tmp_dir is not None:
+ tmp_dir.cleanup()
+
+ if show or out_dir:
+ self.show(results, out_dir, show=show, pipeline=pipeline)
+ return results_dict
+
+ def _build_default_pipeline(self):
+ """Build the default pipeline for this dataset."""
+ pipeline = [
+ dict(
+ type='LoadPointsFromFile',
+ coord_type='LIDAR',
+ load_dim=5,
+ use_dim=5,
+ file_client_args=dict(backend='disk')),
+ dict(
+ type='LoadPointsFromMultiSweeps',
+ sweeps_num=10,
+ file_client_args=dict(backend='disk')),
+ dict(
+ type='DefaultFormatBundle3D',
+ class_names=self.CLASSES,
+ with_label=False),
+ dict(type='Collect3D', keys=['points'])
+ ]
+ return Compose(pipeline)
+
+ def show(self, results, out_dir, show=False, pipeline=None):
+ """Results visualization.
+
+ Args:
+ results (list[dict]): List of bounding boxes results.
+ out_dir (str): Output directory of visualization result.
+ show (bool): Whether to visualize the results online.
+ Default: False.
+ pipeline (list[dict], optional): raw data loading for showing.
+ Default: None.
+ """
+ assert out_dir is not None, 'Expect out_dir, got none.'
+ pipeline = self._get_pipeline(pipeline)
+ for i, result in enumerate(results):
+ if 'pts_bbox' in result.keys():
+ result = result['pts_bbox']
+ data_info = self.data_infos[i]
+ pts_path = data_info['lidar_path']
+ file_name = osp.split(pts_path)[-1].split('.')[0]
+ points = self._extract_data(i, pipeline, 'points').numpy()
+ points = Coord3DMode.convert_point(points, Coord3DMode.LIDAR,
+ Coord3DMode.DEPTH)
+ inds = result['scores_3d'] > 0.1
+ gt_bboxes = self.get_ann_info(i)['gt_bboxes_3d'].tensor.numpy()
+ show_gt_bboxes = Box3DMode.convert(gt_bboxes, Box3DMode.LIDAR,
+ Box3DMode.DEPTH)
+ pred_bboxes = result['boxes_3d'][inds].tensor.numpy()
+ show_pred_bboxes = Box3DMode.convert(pred_bboxes, Box3DMode.LIDAR,
+ Box3DMode.DEPTH)
+ show_result(points, show_gt_bboxes, show_pred_bboxes, out_dir,
+ file_name, show)
+
+ def json2csv(self, json_path, csv_savepath):
+ """Convert the json file to csv format for submission.
+
+ Args:
+ json_path (str): Path of the result json file.
+ csv_savepath (str): Path to save the csv file.
+ """
+ results = mmcv.load(json_path)['results']
+ sample_list_path = osp.join(self.data_root, 'sample_submission.csv')
+ data = pd.read_csv(sample_list_path)
+ Id_list = list(data['Id'])
+ pred_list = list(data['PredictionString'])
+ cnt = 0
+ print('Converting the json to csv...')
+ for token in results.keys():
+ cnt += 1
+ predictions = results[token]
+ prediction_str = ''
+ for i in range(len(predictions)):
+ prediction_str += \
+ str(predictions[i]['score']) + ' ' + \
+ str(predictions[i]['translation'][0]) + ' ' + \
+ str(predictions[i]['translation'][1]) + ' ' + \
+ str(predictions[i]['translation'][2]) + ' ' + \
+ str(predictions[i]['size'][0]) + ' ' + \
+ str(predictions[i]['size'][1]) + ' ' + \
+ str(predictions[i]['size'][2]) + ' ' + \
+ str(Quaternion(list(predictions[i]['rotation']))
+ .yaw_pitch_roll[0]) + ' ' + \
+ predictions[i]['name'] + ' '
+ prediction_str = prediction_str[:-1]
+ idx = Id_list.index(token)
+ pred_list[idx] = prediction_str
+ df = pd.DataFrame({'Id': Id_list, 'PredictionString': pred_list})
+ mmcv.mkdir_or_exist(os.path.dirname(csv_savepath))
+ df.to_csv(csv_savepath, index=False)
+
+
+def output_to_lyft_box(detection):
+ """Convert the output to the box class in the Lyft.
+
+ Args:
+ detection (dict): Detection results.
+
+ Returns:
+ list[:obj:`LyftBox`]: List of standard LyftBoxes.
+ """
+ box3d = detection['boxes_3d']
+ scores = detection['scores_3d'].numpy()
+ labels = detection['labels_3d'].numpy()
+
+ box_gravity_center = box3d.gravity_center.numpy()
+ box_dims = box3d.dims.numpy()
+ box_yaw = box3d.yaw.numpy()
+
+ # our LiDAR coordinate system -> Lyft box coordinate system
+ lyft_box_dims = box_dims[:, [1, 0, 2]]
+
+ box_list = []
+ for i in range(len(box3d)):
+ quat = Quaternion(axis=[0, 0, 1], radians=box_yaw[i])
+ box = LyftBox(
+ box_gravity_center[i],
+ lyft_box_dims[i],
+ quat,
+ label=labels[i],
+ score=scores[i])
+ box_list.append(box)
+ return box_list
+
+
+def lidar_lyft_box_to_global(info, boxes):
+ """Convert the box from ego to global coordinate.
+
+ Args:
+ info (dict): Info for a specific sample data, including the
+ calibration information.
+ boxes (list[:obj:`LyftBox`]): List of predicted LyftBoxes.
+
+ Returns:
+ list: List of standard LyftBoxes in the global
+ coordinate.
+ """
+ box_list = []
+ for box in boxes:
+ # Move box to ego vehicle coord system
+ box.rotate(Quaternion(info['lidar2ego_rotation']))
+ box.translate(np.array(info['lidar2ego_translation']))
+ # Move box to global coord system
+ box.rotate(Quaternion(info['ego2global_rotation']))
+ box.translate(np.array(info['ego2global_translation']))
+ box_list.append(box)
+ return box_list
diff --git a/mmdet3d/datasets/nuscenes_dataset.py b/mmdet3d/datasets/nuscenes_dataset.py
new file mode 100644
index 0000000..1ca8265
--- /dev/null
+++ b/mmdet3d/datasets/nuscenes_dataset.py
@@ -0,0 +1,654 @@
+# Copyright (c) OpenMMLab. All rights reserved.
+import tempfile
+from os import path as osp
+
+import mmcv
+import numpy as np
+import pyquaternion
+from nuscenes.utils.data_classes import Box as NuScenesBox
+
+from ..core import show_result
+from ..core.bbox import Box3DMode, Coord3DMode, LiDARInstance3DBoxes
+from .builder import DATASETS
+from .custom_3d import Custom3DDataset
+from .pipelines import Compose
+
+
+@DATASETS.register_module()
+class NuScenesDataset(Custom3DDataset):
+ r"""NuScenes Dataset.
+
+ This class serves as the API for experiments on the NuScenes Dataset.
+
+ Please refer to `NuScenes Dataset `_
+ for data downloading.
+
+ Args:
+ ann_file (str): Path of annotation file.
+ pipeline (list[dict], optional): Pipeline used for data processing.
+ Defaults to None.
+ data_root (str): Path of dataset root.
+ classes (tuple[str], optional): Classes used in the dataset.
+ Defaults to None.
+ load_interval (int, optional): Interval of loading the dataset. It is
+ used to uniformly sample the dataset. Defaults to 1.
+ with_velocity (bool, optional): Whether include velocity prediction
+ into the experiments. Defaults to True.
+ modality (dict, optional): Modality to specify the sensor data used
+ as input. Defaults to None.
+ box_type_3d (str, optional): Type of 3D box of this dataset.
+ Based on the `box_type_3d`, the dataset will encapsulate the box
+ to its original format then converted them to `box_type_3d`.
+ Defaults to 'LiDAR' in this dataset. Available options includes.
+ - 'LiDAR': Box in LiDAR coordinates.
+ - 'Depth': Box in depth coordinates, usually for indoor dataset.
+ - 'Camera': Box in camera coordinates.
+ filter_empty_gt (bool, optional): Whether to filter empty GT.
+ Defaults to True.
+ test_mode (bool, optional): Whether the dataset is in test mode.
+ Defaults to False.
+ eval_version (bool, optional): Configuration version of evaluation.
+ Defaults to 'detection_cvpr_2019'.
+ use_valid_flag (bool, optional): Whether to use `use_valid_flag` key
+ in the info file as mask to filter gt_boxes and gt_names.
+ Defaults to False.
+ """
+ NameMapping = {
+ 'movable_object.barrier': 'barrier',
+ 'vehicle.bicycle': 'bicycle',
+ 'vehicle.bus.bendy': 'bus',
+ 'vehicle.bus.rigid': 'bus',
+ 'vehicle.car': 'car',
+ 'vehicle.construction': 'construction_vehicle',
+ 'vehicle.motorcycle': 'motorcycle',
+ 'human.pedestrian.adult': 'pedestrian',
+ 'human.pedestrian.child': 'pedestrian',
+ 'human.pedestrian.construction_worker': 'pedestrian',
+ 'human.pedestrian.police_officer': 'pedestrian',
+ 'movable_object.trafficcone': 'traffic_cone',
+ 'vehicle.trailer': 'trailer',
+ 'vehicle.truck': 'truck'
+ }
+ DefaultAttribute = {
+ 'car': 'vehicle.parked',
+ 'pedestrian': 'pedestrian.moving',
+ 'trailer': 'vehicle.parked',
+ 'truck': 'vehicle.parked',
+ 'bus': 'vehicle.moving',
+ 'motorcycle': 'cycle.without_rider',
+ 'construction_vehicle': 'vehicle.parked',
+ 'bicycle': 'cycle.without_rider',
+ 'barrier': '',
+ 'traffic_cone': '',
+ }
+ AttrMapping = {
+ 'cycle.with_rider': 0,
+ 'cycle.without_rider': 1,
+ 'pedestrian.moving': 2,
+ 'pedestrian.standing': 3,
+ 'pedestrian.sitting_lying_down': 4,
+ 'vehicle.moving': 5,
+ 'vehicle.parked': 6,
+ 'vehicle.stopped': 7,
+ }
+ AttrMapping_rev = [
+ 'cycle.with_rider',
+ 'cycle.without_rider',
+ 'pedestrian.moving',
+ 'pedestrian.standing',
+ 'pedestrian.sitting_lying_down',
+ 'vehicle.moving',
+ 'vehicle.parked',
+ 'vehicle.stopped',
+ ]
+ # https://github.com/nutonomy/nuscenes-devkit/blob/57889ff20678577025326cfc24e57424a829be0a/python-sdk/nuscenes/eval/detection/evaluate.py#L222 # noqa
+ ErrNameMapping = {
+ 'trans_err': 'mATE',
+ 'scale_err': 'mASE',
+ 'orient_err': 'mAOE',
+ 'vel_err': 'mAVE',
+ 'attr_err': 'mAAE'
+ }
+ CLASSES = ('car', 'truck', 'trailer', 'bus', 'construction_vehicle',
+ 'bicycle', 'motorcycle', 'pedestrian', 'traffic_cone',
+ 'barrier')
+
+ def __init__(self,
+ ann_file,
+ pipeline=None,
+ data_root=None,
+ classes=None,
+ load_interval=1,
+ with_velocity=True,
+ modality=None,
+ box_type_3d='LiDAR',
+ filter_empty_gt=True,
+ test_mode=False,
+ eval_version='detection_cvpr_2019',
+ use_valid_flag=False):
+ self.load_interval = load_interval
+ self.use_valid_flag = use_valid_flag
+ super().__init__(
+ data_root=data_root,
+ ann_file=ann_file,
+ pipeline=pipeline,
+ classes=classes,
+ modality=modality,
+ box_type_3d=box_type_3d,
+ filter_empty_gt=filter_empty_gt,
+ test_mode=test_mode)
+
+ self.with_velocity = with_velocity
+ self.eval_version = eval_version
+ from nuscenes.eval.detection.config import config_factory
+ self.eval_detection_configs = config_factory(self.eval_version)
+ if self.modality is None:
+ self.modality = dict(
+ use_camera=False,
+ use_lidar=True,
+ use_radar=False,
+ use_map=False,
+ use_external=False,
+ )
+
+ def get_cat_ids(self, idx):
+ """Get category distribution of single scene.
+
+ Args:
+ idx (int): Index of the data_info.
+
+ Returns:
+ dict[list]: for each category, if the current scene
+ contains such boxes, store a list containing idx,
+ otherwise, store empty list.
+ """
+ info = self.data_infos[idx]
+ if self.use_valid_flag:
+ mask = info['valid_flag']
+ gt_names = set(info['gt_names'][mask])
+ else:
+ gt_names = set(info['gt_names'])
+
+ cat_ids = []
+ for name in gt_names:
+ if name in self.CLASSES:
+ cat_ids.append(self.cat2id[name])
+ return cat_ids
+
+ def load_annotations(self, ann_file):
+ """Load annotations from ann_file.
+
+ Args:
+ ann_file (str): Path of the annotation file.
+
+ Returns:
+ list[dict]: List of annotations sorted by timestamps.
+ """
+ data = mmcv.load(ann_file, file_format='pkl')
+ data_infos = list(sorted(data['infos'], key=lambda e: e['timestamp']))
+ data_infos = data_infos[::self.load_interval]
+ self.metadata = data['metadata']
+ self.version = self.metadata['version']
+ return data_infos
+
+ def get_data_info(self, index):
+ """Get data info according to the given index.
+
+ Args:
+ index (int): Index of the sample data to get.
+
+ Returns:
+ dict: Data information that will be passed to the data
+ preprocessing pipelines. It includes the following keys:
+
+ - sample_idx (str): Sample index.
+ - pts_filename (str): Filename of point clouds.
+ - sweeps (list[dict]): Infos of sweeps.
+ - timestamp (float): Sample timestamp.
+ - img_filename (str, optional): Image filename.
+ - lidar2img (list[np.ndarray], optional): Transformations
+ from lidar to different cameras.
+ - ann_info (dict): Annotation info.
+ """
+ info = self.data_infos[index]
+ # standard protocol modified from SECOND.Pytorch
+ input_dict = dict(
+ sample_idx=info['token'],
+ pts_filename=info['lidar_path'],
+ sweeps=info['sweeps'],
+ timestamp=info['timestamp'] / 1e6,
+ )
+
+ if self.modality['use_camera']:
+ image_paths = []
+ lidar2img_rts = []
+ for cam_type, cam_info in info['cams'].items():
+ image_paths.append(cam_info['data_path'])
+ # obtain lidar to image transformation matrix
+ lidar2cam_r = np.linalg.inv(cam_info['sensor2lidar_rotation'])
+ lidar2cam_t = cam_info[
+ 'sensor2lidar_translation'] @ lidar2cam_r.T
+ lidar2cam_rt = np.eye(4)
+ lidar2cam_rt[:3, :3] = lidar2cam_r.T
+ lidar2cam_rt[3, :3] = -lidar2cam_t
+ intrinsic = cam_info['cam_intrinsic']
+ viewpad = np.eye(4)
+ viewpad[:intrinsic.shape[0], :intrinsic.shape[1]] = intrinsic
+ lidar2img_rt = (viewpad @ lidar2cam_rt.T)
+ lidar2img_rts.append(lidar2img_rt)
+
+ input_dict.update(
+ dict(
+ img_filename=image_paths,
+ lidar2img=lidar2img_rts,
+ ))
+
+ if not self.test_mode:
+ annos = self.get_ann_info(index)
+ input_dict['ann_info'] = annos
+
+ return input_dict
+
+ def get_ann_info(self, index):
+ """Get annotation info according to the given index.
+
+ Args:
+ index (int): Index of the annotation data to get.
+
+ Returns:
+ dict: Annotation information consists of the following keys:
+
+ - gt_bboxes_3d (:obj:`LiDARInstance3DBoxes`):
+ 3D ground truth bboxes
+ - gt_labels_3d (np.ndarray): Labels of ground truths.
+ - gt_names (list[str]): Class names of ground truths.
+ """
+ info = self.data_infos[index]
+ # filter out bbox containing no points
+ if self.use_valid_flag:
+ mask = info['valid_flag']
+ else:
+ mask = info['num_lidar_pts'] > 0
+ gt_bboxes_3d = info['gt_boxes'][mask]
+ gt_names_3d = info['gt_names'][mask]
+ gt_labels_3d = []
+ for cat in gt_names_3d:
+ if cat in self.CLASSES:
+ gt_labels_3d.append(self.CLASSES.index(cat))
+ else:
+ gt_labels_3d.append(-1)
+ gt_labels_3d = np.array(gt_labels_3d)
+
+ if self.with_velocity:
+ gt_velocity = info['gt_velocity'][mask]
+ nan_mask = np.isnan(gt_velocity[:, 0])
+ gt_velocity[nan_mask] = [0.0, 0.0]
+ gt_bboxes_3d = np.concatenate([gt_bboxes_3d, gt_velocity], axis=-1)
+
+ # the nuscenes box center is [0.5, 0.5, 0.5], we change it to be
+ # the same as KITTI (0.5, 0.5, 0)
+ gt_bboxes_3d = LiDARInstance3DBoxes(
+ gt_bboxes_3d,
+ box_dim=gt_bboxes_3d.shape[-1],
+ origin=(0.5, 0.5, 0.5)).convert_to(self.box_mode_3d)
+
+ anns_results = dict(
+ gt_bboxes_3d=gt_bboxes_3d,
+ gt_labels_3d=gt_labels_3d,
+ gt_names=gt_names_3d)
+ return anns_results
+
+ def _format_bbox(self, results, jsonfile_prefix=None):
+ """Convert the results to the standard format.
+
+ Args:
+ results (list[dict]): Testing results of the dataset.
+ jsonfile_prefix (str): The prefix of the output jsonfile.
+ You can specify the output directory/filename by
+ modifying the jsonfile_prefix. Default: None.
+
+ Returns:
+ str: Path of the output json file.
+ """
+ nusc_annos = {}
+ mapped_class_names = self.CLASSES
+
+ print('Start to convert detection format...')
+ for sample_id, det in enumerate(mmcv.track_iter_progress(results)):
+ annos = []
+ boxes = output_to_nusc_box(det)
+ sample_token = self.data_infos[sample_id]['token']
+ boxes = lidar_nusc_box_to_global(self.data_infos[sample_id], boxes,
+ mapped_class_names,
+ self.eval_detection_configs,
+ self.eval_version)
+ for i, box in enumerate(boxes):
+ name = mapped_class_names[box.label]
+ if np.sqrt(box.velocity[0]**2 + box.velocity[1]**2) > 0.2:
+ if name in [
+ 'car',
+ 'construction_vehicle',
+ 'bus',
+ 'truck',
+ 'trailer',
+ ]:
+ attr = 'vehicle.moving'
+ elif name in ['bicycle', 'motorcycle']:
+ attr = 'cycle.with_rider'
+ else:
+ attr = NuScenesDataset.DefaultAttribute[name]
+ else:
+ if name in ['pedestrian']:
+ attr = 'pedestrian.standing'
+ elif name in ['bus']:
+ attr = 'vehicle.stopped'
+ else:
+ attr = NuScenesDataset.DefaultAttribute[name]
+
+ nusc_anno = dict(
+ sample_token=sample_token,
+ translation=box.center.tolist(),
+ size=box.wlh.tolist(),
+ rotation=box.orientation.elements.tolist(),
+ velocity=box.velocity[:2].tolist(),
+ detection_name=name,
+ detection_score=box.score,
+ attribute_name=attr)
+ annos.append(nusc_anno)
+ nusc_annos[sample_token] = annos
+ nusc_submissions = {
+ 'meta': self.modality,
+ 'results': nusc_annos,
+ }
+
+ mmcv.mkdir_or_exist(jsonfile_prefix)
+ res_path = osp.join(jsonfile_prefix, 'results_nusc.json')
+ print('Results writes to', res_path)
+ mmcv.dump(nusc_submissions, res_path)
+ return res_path
+
+ def _evaluate_single(self,
+ result_path,
+ logger=None,
+ metric='bbox',
+ result_name='pts_bbox'):
+ """Evaluation for a single model in nuScenes protocol.
+
+ Args:
+ result_path (str): Path of the result file.
+ logger (logging.Logger | str, optional): Logger used for printing
+ related information during evaluation. Default: None.
+ metric (str, optional): Metric name used for evaluation.
+ Default: 'bbox'.
+ result_name (str, optional): Result name in the metric prefix.
+ Default: 'pts_bbox'.
+
+ Returns:
+ dict: Dictionary of evaluation details.
+ """
+ from nuscenes import NuScenes
+ from nuscenes.eval.detection.evaluate import NuScenesEval
+
+ output_dir = osp.join(*osp.split(result_path)[:-1])
+ nusc = NuScenes(
+ version=self.version, dataroot=self.data_root, verbose=False)
+ eval_set_map = {
+ 'v1.0-mini': 'mini_val',
+ 'v1.0-trainval': 'val',
+ }
+ nusc_eval = NuScenesEval(
+ nusc,
+ config=self.eval_detection_configs,
+ result_path=result_path,
+ eval_set=eval_set_map[self.version],
+ output_dir=output_dir,
+ verbose=False)
+ nusc_eval.main(render_curves=False)
+
+ # record metrics
+ metrics = mmcv.load(osp.join(output_dir, 'metrics_summary.json'))
+ detail = dict()
+ metric_prefix = f'{result_name}_NuScenes'
+ for name in self.CLASSES:
+ for k, v in metrics['label_aps'][name].items():
+ val = float('{:.4f}'.format(v))
+ detail['{}/{}_AP_dist_{}'.format(metric_prefix, name, k)] = val
+ for k, v in metrics['label_tp_errors'][name].items():
+ val = float('{:.4f}'.format(v))
+ detail['{}/{}_{}'.format(metric_prefix, name, k)] = val
+ for k, v in metrics['tp_errors'].items():
+ val = float('{:.4f}'.format(v))
+ detail['{}/{}'.format(metric_prefix,
+ self.ErrNameMapping[k])] = val
+
+ detail['{}/NDS'.format(metric_prefix)] = metrics['nd_score']
+ detail['{}/mAP'.format(metric_prefix)] = metrics['mean_ap']
+ return detail
+
+ def format_results(self, results, jsonfile_prefix=None):
+ """Format the results to json (standard format for COCO evaluation).
+
+ Args:
+ results (list[dict]): Testing results of the dataset.
+ jsonfile_prefix (str): The prefix of json files. It includes
+ the file path and the prefix of filename, e.g., "a/b/prefix".
+ If not specified, a temp file will be created. Default: None.
+
+ Returns:
+ tuple: Returns (result_files, tmp_dir), where `result_files` is a
+ dict containing the json filepaths, `tmp_dir` is the temporal
+ directory created for saving json files when
+ `jsonfile_prefix` is not specified.
+ """
+ assert isinstance(results, list), 'results must be a list'
+ assert len(results) == len(self), (
+ 'The length of results is not equal to the dataset len: {} != {}'.
+ format(len(results), len(self)))
+
+ if jsonfile_prefix is None:
+ tmp_dir = tempfile.TemporaryDirectory()
+ jsonfile_prefix = osp.join(tmp_dir.name, 'results')
+ else:
+ tmp_dir = None
+
+ # currently the output prediction results could be in two formats
+ # 1. list of dict('boxes_3d': ..., 'scores_3d': ..., 'labels_3d': ...)
+ # 2. list of dict('pts_bbox' or 'img_bbox':
+ # dict('boxes_3d': ..., 'scores_3d': ..., 'labels_3d': ...))
+ # this is a workaround to enable evaluation of both formats on nuScenes
+ # refer to https://github.com/open-mmlab/mmdetection3d/issues/449
+ if not ('pts_bbox' in results[0] or 'img_bbox' in results[0]):
+ result_files = self._format_bbox(results, jsonfile_prefix)
+ else:
+ # should take the inner dict out of 'pts_bbox' or 'img_bbox' dict
+ result_files = dict()
+ for name in results[0]:
+ print(f'\nFormating bboxes of {name}')
+ results_ = [out[name] for out in results]
+ tmp_file_ = osp.join(jsonfile_prefix, name)
+ result_files.update(
+ {name: self._format_bbox(results_, tmp_file_)})
+ return result_files, tmp_dir
+
+ def evaluate(self,
+ results,
+ metric='bbox',
+ logger=None,
+ jsonfile_prefix=None,
+ result_names=['pts_bbox'],
+ show=False,
+ out_dir=None,
+ pipeline=None):
+ """Evaluation in nuScenes protocol.
+
+ Args:
+ results (list[dict]): Testing results of the dataset.
+ metric (str | list[str], optional): Metrics to be evaluated.
+ Default: 'bbox'.
+ logger (logging.Logger | str, optional): Logger used for printing
+ related information during evaluation. Default: None.
+ jsonfile_prefix (str, optional): The prefix of json files including
+ the file path and the prefix of filename, e.g., "a/b/prefix".
+ If not specified, a temp file will be created. Default: None.
+ show (bool, optional): Whether to visualize.
+ Default: False.
+ out_dir (str, optional): Path to save the visualization results.
+ Default: None.
+ pipeline (list[dict], optional): raw data loading for showing.
+ Default: None.
+
+ Returns:
+ dict[str, float]: Results of each evaluation metric.
+ """
+ result_files, tmp_dir = self.format_results(results, jsonfile_prefix)
+
+ if isinstance(result_files, dict):
+ results_dict = dict()
+ for name in result_names:
+ print('Evaluating bboxes of {}'.format(name))
+ ret_dict = self._evaluate_single(result_files[name])
+ results_dict.update(ret_dict)
+ elif isinstance(result_files, str):
+ results_dict = self._evaluate_single(result_files)
+
+ if tmp_dir is not None:
+ tmp_dir.cleanup()
+
+ if show or out_dir:
+ self.show(results, out_dir, show=show, pipeline=pipeline)
+ return results_dict
+
+ def _build_default_pipeline(self):
+ """Build the default pipeline for this dataset."""
+ pipeline = [
+ dict(
+ type='LoadPointsFromFile',
+ coord_type='LIDAR',
+ load_dim=5,
+ use_dim=5,
+ file_client_args=dict(backend='disk')),
+ dict(
+ type='LoadPointsFromMultiSweeps',
+ sweeps_num=10,
+ file_client_args=dict(backend='disk')),
+ dict(
+ type='DefaultFormatBundle3D',
+ class_names=self.CLASSES,
+ with_label=False),
+ dict(type='Collect3D', keys=['points'])
+ ]
+ return Compose(pipeline)
+
+ def show(self, results, out_dir, show=False, pipeline=None):
+ """Results visualization.
+
+ Args:
+ results (list[dict]): List of bounding boxes results.
+ out_dir (str): Output directory of visualization result.
+ show (bool): Whether to visualize the results online.
+ Default: False.
+ pipeline (list[dict], optional): raw data loading for showing.
+ Default: None.
+ """
+ assert out_dir is not None, 'Expect out_dir, got none.'
+ pipeline = self._get_pipeline(pipeline)
+ for i, result in enumerate(results):
+ if 'pts_bbox' in result.keys():
+ result = result['pts_bbox']
+ data_info = self.data_infos[i]
+ pts_path = data_info['lidar_path']
+ file_name = osp.split(pts_path)[-1].split('.')[0]
+ points = self._extract_data(i, pipeline, 'points').numpy()
+ # for now we convert points into depth mode
+ points = Coord3DMode.convert_point(points, Coord3DMode.LIDAR,
+ Coord3DMode.DEPTH)
+ inds = result['scores_3d'] > 0.1
+ gt_bboxes = self.get_ann_info(i)['gt_bboxes_3d'].tensor.numpy()
+ show_gt_bboxes = Box3DMode.convert(gt_bboxes, Box3DMode.LIDAR,
+ Box3DMode.DEPTH)
+ pred_bboxes = result['boxes_3d'][inds].tensor.numpy()
+ show_pred_bboxes = Box3DMode.convert(pred_bboxes, Box3DMode.LIDAR,
+ Box3DMode.DEPTH)
+ show_result(points, show_gt_bboxes, show_pred_bboxes, out_dir,
+ file_name, show)
+
+
+def output_to_nusc_box(detection):
+ """Convert the output to the box class in the nuScenes.
+
+ Args:
+ detection (dict): Detection results.
+
+ - boxes_3d (:obj:`BaseInstance3DBoxes`): Detection bbox.
+ - scores_3d (torch.Tensor): Detection scores.
+ - labels_3d (torch.Tensor): Predicted box labels.
+
+ Returns:
+ list[:obj:`NuScenesBox`]: List of standard NuScenesBoxes.
+ """
+ box3d = detection['boxes_3d']
+ scores = detection['scores_3d'].numpy()
+ labels = detection['labels_3d'].numpy()
+
+ box_gravity_center = box3d.gravity_center.numpy()
+ box_dims = box3d.dims.numpy()
+ box_yaw = box3d.yaw.numpy()
+
+ # our LiDAR coordinate system -> nuScenes box coordinate system
+ nus_box_dims = box_dims[:, [1, 0, 2]]
+
+ box_list = []
+ for i in range(len(box3d)):
+ quat = pyquaternion.Quaternion(axis=[0, 0, 1], radians=box_yaw[i])
+ velocity = (*box3d.tensor[i, 7:9], 0.0)
+ # velo_val = np.linalg.norm(box3d[i, 7:9])
+ # velo_ori = box3d[i, 6]
+ # velocity = (
+ # velo_val * np.cos(velo_ori), velo_val * np.sin(velo_ori), 0.0)
+ box = NuScenesBox(
+ box_gravity_center[i],
+ nus_box_dims[i],
+ quat,
+ label=labels[i],
+ score=scores[i],
+ velocity=velocity)
+ box_list.append(box)
+ return box_list
+
+
+def lidar_nusc_box_to_global(info,
+ boxes,
+ classes,
+ eval_configs,
+ eval_version='detection_cvpr_2019'):
+ """Convert the box from ego to global coordinate.
+
+ Args:
+ info (dict): Info for a specific sample data, including the
+ calibration information.
+ boxes (list[:obj:`NuScenesBox`]): List of predicted NuScenesBoxes.
+ classes (list[str]): Mapped classes in the evaluation.
+ eval_configs (object): Evaluation configuration object.
+ eval_version (str, optional): Evaluation version.
+ Default: 'detection_cvpr_2019'
+
+ Returns:
+ list: List of standard NuScenesBoxes in the global
+ coordinate.
+ """
+ box_list = []
+ for box in boxes:
+ # Move box to ego vehicle coord system
+ box.rotate(pyquaternion.Quaternion(info['lidar2ego_rotation']))
+ box.translate(np.array(info['lidar2ego_translation']))
+ # filter det in ego.
+ cls_range_map = eval_configs.class_range
+ radius = np.linalg.norm(box.center[:2], 2)
+ det_range = cls_range_map[classes[box.label]]
+ if radius > det_range:
+ continue
+ # Move box to global coord system
+ box.rotate(pyquaternion.Quaternion(info['ego2global_rotation']))
+ box.translate(np.array(info['ego2global_translation']))
+ box_list.append(box)
+ return box_list
diff --git a/mmdet3d/datasets/nuscenes_mono_dataset.py b/mmdet3d/datasets/nuscenes_mono_dataset.py
new file mode 100644
index 0000000..c3eb8f1
--- /dev/null
+++ b/mmdet3d/datasets/nuscenes_mono_dataset.py
@@ -0,0 +1,840 @@
+# Copyright (c) OpenMMLab. All rights reserved.
+import copy
+import tempfile
+import warnings
+from os import path as osp
+
+import mmcv
+import numpy as np
+import pyquaternion
+import torch
+from nuscenes.utils.data_classes import Box as NuScenesBox
+
+from mmdet3d.core import bbox3d2result, box3d_multiclass_nms, xywhr2xyxyr
+from mmdet.datasets import CocoDataset
+from ..core import show_multi_modality_result
+from ..core.bbox import CameraInstance3DBoxes, get_box_type
+from .builder import DATASETS
+from .pipelines import Compose
+from .utils import extract_result_dict, get_loading_pipeline
+
+
+@DATASETS.register_module()
+class NuScenesMonoDataset(CocoDataset):
+ r"""Monocular 3D detection on NuScenes Dataset.
+
+ This class serves as the API for experiments on the NuScenes Dataset.
+
+ Please refer to `NuScenes Dataset `_
+ for data downloading.
+
+ Args:
+ ann_file (str): Path of annotation file.
+ data_root (str): Path of dataset root.
+ load_interval (int, optional): Interval of loading the dataset. It is
+ used to uniformly sample the dataset. Defaults to 1.
+ with_velocity (bool, optional): Whether include velocity prediction
+ into the experiments. Defaults to True.
+ modality (dict, optional): Modality to specify the sensor data used
+ as input. Defaults to None.
+ box_type_3d (str, optional): Type of 3D box of this dataset.
+ Based on the `box_type_3d`, the dataset will encapsulate the box
+ to its original format then converted them to `box_type_3d`.
+ Defaults to 'Camera' in this class. Available options includes.
+ - 'LiDAR': Box in LiDAR coordinates.
+ - 'Depth': Box in depth coordinates, usually for indoor dataset.
+ - 'Camera': Box in camera coordinates.
+ eval_version (str, optional): Configuration version of evaluation.
+ Defaults to 'detection_cvpr_2019'.
+ use_valid_flag (bool, optional): Whether to use `use_valid_flag` key
+ in the info file as mask to filter gt_boxes and gt_names.
+ Defaults to False.
+ version (str, optional): Dataset version. Defaults to 'v1.0-trainval'.
+ """
+ CLASSES = ('car', 'truck', 'trailer', 'bus', 'construction_vehicle',
+ 'bicycle', 'motorcycle', 'pedestrian', 'traffic_cone',
+ 'barrier')
+ DefaultAttribute = {
+ 'car': 'vehicle.parked',
+ 'pedestrian': 'pedestrian.moving',
+ 'trailer': 'vehicle.parked',
+ 'truck': 'vehicle.parked',
+ 'bus': 'vehicle.moving',
+ 'motorcycle': 'cycle.without_rider',
+ 'construction_vehicle': 'vehicle.parked',
+ 'bicycle': 'cycle.without_rider',
+ 'barrier': '',
+ 'traffic_cone': '',
+ }
+ # https://github.com/nutonomy/nuscenes-devkit/blob/57889ff20678577025326cfc24e57424a829be0a/python-sdk/nuscenes/eval/detection/evaluate.py#L222 # noqa
+ ErrNameMapping = {
+ 'trans_err': 'mATE',
+ 'scale_err': 'mASE',
+ 'orient_err': 'mAOE',
+ 'vel_err': 'mAVE',
+ 'attr_err': 'mAAE'
+ }
+
+ def __init__(self,
+ data_root,
+ ann_file,
+ pipeline,
+ load_interval=1,
+ with_velocity=True,
+ modality=None,
+ box_type_3d='Camera',
+ eval_version='detection_cvpr_2019',
+ use_valid_flag=False,
+ version='v1.0-trainval',
+ classes=None,
+ img_prefix='',
+ seg_prefix=None,
+ proposal_file=None,
+ test_mode=False,
+ filter_empty_gt=True,
+ file_client_args=dict(backend='disk')):
+ self.ann_file = ann_file
+ self.data_root = data_root
+ self.img_prefix = img_prefix
+ self.seg_prefix = seg_prefix
+ self.proposal_file = proposal_file
+ self.test_mode = test_mode
+ self.filter_empty_gt = filter_empty_gt
+ self.CLASSES = self.get_classes(classes)
+ self.file_client = mmcv.FileClient(**file_client_args)
+
+ # load annotations (and proposals)
+ with self.file_client.get_local_path(self.ann_file) as local_path:
+ self.data_infos = self.load_annotations(local_path)
+
+ if self.proposal_file is not None:
+ with self.file_client.get_local_path(
+ self.proposal_file) as local_path:
+ self.proposals = self.load_proposals(local_path)
+ else:
+ self.proposals = None
+
+ # filter images too small and containing no annotations
+ if not test_mode:
+ valid_inds = self._filter_imgs()
+ self.data_infos = [self.data_infos[i] for i in valid_inds]
+ if self.proposals is not None:
+ self.proposals = [self.proposals[i] for i in valid_inds]
+ # set group flag for the sampler
+ self._set_group_flag()
+
+ # processing pipeline
+ self.pipeline = Compose(pipeline)
+
+ self.load_interval = load_interval
+ self.with_velocity = with_velocity
+ self.modality = modality
+ self.box_type_3d, self.box_mode_3d = get_box_type(box_type_3d)
+ self.eval_version = eval_version
+ self.use_valid_flag = use_valid_flag
+ self.bbox_code_size = 9
+ self.version = version
+ if self.eval_version is not None:
+ from nuscenes.eval.detection.config import config_factory
+ self.eval_detection_configs = config_factory(self.eval_version)
+ if self.modality is None:
+ self.modality = dict(
+ use_camera=True,
+ use_lidar=False,
+ use_radar=False,
+ use_map=False,
+ use_external=False)
+
+ def pre_pipeline(self, results):
+ """Initialization before data preparation.
+
+ Args:
+ results (dict): Dict before data preprocessing.
+
+ - img_fields (list): Image fields.
+ - bbox3d_fields (list): 3D bounding boxes fields.
+ - pts_mask_fields (list): Mask fields of points.
+ - pts_seg_fields (list): Mask fields of point segments.
+ - bbox_fields (list): Fields of bounding boxes.
+ - mask_fields (list): Fields of masks.
+ - seg_fields (list): Segment fields.
+ - box_type_3d (str): 3D box type.
+ - box_mode_3d (str): 3D box mode.
+ """
+ results['img_prefix'] = self.img_prefix
+ results['seg_prefix'] = self.seg_prefix
+ results['proposal_file'] = self.proposal_file
+ results['img_fields'] = []
+ results['bbox3d_fields'] = []
+ results['pts_mask_fields'] = []
+ results['pts_seg_fields'] = []
+ results['bbox_fields'] = []
+ results['mask_fields'] = []
+ results['seg_fields'] = []
+ results['box_type_3d'] = self.box_type_3d
+ results['box_mode_3d'] = self.box_mode_3d
+
+ def _parse_ann_info(self, img_info, ann_info):
+ """Parse bbox annotation.
+
+ Args:
+ img_info (list[dict]): Image info.
+ ann_info (list[dict]): Annotation info of an image.
+
+ Returns:
+ dict: A dict containing the following keys: bboxes, labels,
+ gt_bboxes_3d, gt_labels_3d, attr_labels, centers2d,
+ depths, bboxes_ignore, masks, seg_map
+ """
+ gt_bboxes = []
+ gt_labels = []
+ attr_labels = []
+ gt_bboxes_ignore = []
+ gt_masks_ann = []
+ gt_bboxes_cam3d = []
+ centers2d = []
+ depths = []
+ for i, ann in enumerate(ann_info):
+ if ann.get('ignore', False):
+ continue
+ x1, y1, w, h = ann['bbox']
+ inter_w = max(0, min(x1 + w, img_info['width']) - max(x1, 0))
+ inter_h = max(0, min(y1 + h, img_info['height']) - max(y1, 0))
+ if inter_w * inter_h == 0:
+ continue
+ if ann['area'] <= 0 or w < 1 or h < 1:
+ continue
+ if ann['category_id'] not in self.cat_ids:
+ continue
+ bbox = [x1, y1, x1 + w, y1 + h]
+ if ann.get('iscrowd', False):
+ gt_bboxes_ignore.append(bbox)
+ else:
+ gt_bboxes.append(bbox)
+ gt_labels.append(self.cat2label[ann['category_id']])
+ attr_labels.append(ann['attribute_id'])
+ gt_masks_ann.append(ann.get('segmentation', None))
+ # 3D annotations in camera coordinates
+ bbox_cam3d = np.array(ann['bbox_cam3d']).reshape(1, -1)
+ velo_cam3d = np.array(ann['velo_cam3d']).reshape(1, 2)
+ nan_mask = np.isnan(velo_cam3d[:, 0])
+ velo_cam3d[nan_mask] = [0.0, 0.0]
+ bbox_cam3d = np.concatenate([bbox_cam3d, velo_cam3d], axis=-1)
+ gt_bboxes_cam3d.append(bbox_cam3d.squeeze())
+ # 2.5D annotations in camera coordinates
+ center2d = ann['center2d'][:2]
+ depth = ann['center2d'][2]
+ centers2d.append(center2d)
+ depths.append(depth)
+
+ if gt_bboxes:
+ gt_bboxes = np.array(gt_bboxes, dtype=np.float32)
+ gt_labels = np.array(gt_labels, dtype=np.int64)
+ attr_labels = np.array(attr_labels, dtype=np.int64)
+ else:
+ gt_bboxes = np.zeros((0, 4), dtype=np.float32)
+ gt_labels = np.array([], dtype=np.int64)
+ attr_labels = np.array([], dtype=np.int64)
+
+ if gt_bboxes_cam3d:
+ gt_bboxes_cam3d = np.array(gt_bboxes_cam3d, dtype=np.float32)
+ centers2d = np.array(centers2d, dtype=np.float32)
+ depths = np.array(depths, dtype=np.float32)
+ else:
+ gt_bboxes_cam3d = np.zeros((0, self.bbox_code_size),
+ dtype=np.float32)
+ centers2d = np.zeros((0, 2), dtype=np.float32)
+ depths = np.zeros((0), dtype=np.float32)
+
+ gt_bboxes_cam3d = CameraInstance3DBoxes(
+ gt_bboxes_cam3d,
+ box_dim=gt_bboxes_cam3d.shape[-1],
+ origin=(0.5, 0.5, 0.5))
+ gt_labels_3d = copy.deepcopy(gt_labels)
+
+ if gt_bboxes_ignore:
+ gt_bboxes_ignore = np.array(gt_bboxes_ignore, dtype=np.float32)
+ else:
+ gt_bboxes_ignore = np.zeros((0, 4), dtype=np.float32)
+
+ seg_map = img_info['filename'].replace('jpg', 'png')
+
+ ann = dict(
+ bboxes=gt_bboxes,
+ labels=gt_labels,
+ gt_bboxes_3d=gt_bboxes_cam3d,
+ gt_labels_3d=gt_labels_3d,
+ attr_labels=attr_labels,
+ centers2d=centers2d,
+ depths=depths,
+ bboxes_ignore=gt_bboxes_ignore,
+ masks=gt_masks_ann,
+ seg_map=seg_map)
+
+ return ann
+
+ def get_attr_name(self, attr_idx, label_name):
+ """Get attribute from predicted index.
+
+ This is a workaround to predict attribute when the predicted velocity
+ is not reliable. We map the predicted attribute index to the one
+ in the attribute set. If it is consistent with the category, we will
+ keep it. Otherwise, we will use the default attribute.
+
+ Args:
+ attr_idx (int): Attribute index.
+ label_name (str): Predicted category name.
+
+ Returns:
+ str: Predicted attribute name.
+ """
+ # TODO: Simplify the variable name
+ AttrMapping_rev2 = [
+ 'cycle.with_rider', 'cycle.without_rider', 'pedestrian.moving',
+ 'pedestrian.standing', 'pedestrian.sitting_lying_down',
+ 'vehicle.moving', 'vehicle.parked', 'vehicle.stopped', 'None'
+ ]
+ if label_name == 'car' or label_name == 'bus' \
+ or label_name == 'truck' or label_name == 'trailer' \
+ or label_name == 'construction_vehicle':
+ if AttrMapping_rev2[attr_idx] == 'vehicle.moving' or \
+ AttrMapping_rev2[attr_idx] == 'vehicle.parked' or \
+ AttrMapping_rev2[attr_idx] == 'vehicle.stopped':
+ return AttrMapping_rev2[attr_idx]
+ else:
+ return NuScenesMonoDataset.DefaultAttribute[label_name]
+ elif label_name == 'pedestrian':
+ if AttrMapping_rev2[attr_idx] == 'pedestrian.moving' or \
+ AttrMapping_rev2[attr_idx] == 'pedestrian.standing' or \
+ AttrMapping_rev2[attr_idx] == \
+ 'pedestrian.sitting_lying_down':
+ return AttrMapping_rev2[attr_idx]
+ else:
+ return NuScenesMonoDataset.DefaultAttribute[label_name]
+ elif label_name == 'bicycle' or label_name == 'motorcycle':
+ if AttrMapping_rev2[attr_idx] == 'cycle.with_rider' or \
+ AttrMapping_rev2[attr_idx] == 'cycle.without_rider':
+ return AttrMapping_rev2[attr_idx]
+ else:
+ return NuScenesMonoDataset.DefaultAttribute[label_name]
+ else:
+ return NuScenesMonoDataset.DefaultAttribute[label_name]
+
+ def _format_bbox(self, results, jsonfile_prefix=None):
+ """Convert the results to the standard format.
+
+ Args:
+ results (list[dict]): Testing results of the dataset.
+ jsonfile_prefix (str): The prefix of the output jsonfile.
+ You can specify the output directory/filename by
+ modifying the jsonfile_prefix. Default: None.
+
+ Returns:
+ str: Path of the output json file.
+ """
+ nusc_annos = {}
+ mapped_class_names = self.CLASSES
+
+ print('Start to convert detection format...')
+
+ CAM_NUM = 6
+
+ for sample_id, det in enumerate(mmcv.track_iter_progress(results)):
+
+ if sample_id % CAM_NUM == 0:
+ boxes_per_frame = []
+ attrs_per_frame = []
+
+ # need to merge results from images of the same sample
+ annos = []
+ boxes, attrs = output_to_nusc_box(det)
+ sample_token = self.data_infos[sample_id]['token']
+ boxes, attrs = cam_nusc_box_to_global(self.data_infos[sample_id],
+ boxes, attrs,
+ mapped_class_names,
+ self.eval_detection_configs,
+ self.eval_version)
+
+ boxes_per_frame.extend(boxes)
+ attrs_per_frame.extend(attrs)
+ # Remove redundant predictions caused by overlap of images
+ if (sample_id + 1) % CAM_NUM != 0:
+ continue
+ boxes = global_nusc_box_to_cam(
+ self.data_infos[sample_id + 1 - CAM_NUM], boxes_per_frame,
+ mapped_class_names, self.eval_detection_configs,
+ self.eval_version)
+ cam_boxes3d, scores, labels = nusc_box_to_cam_box3d(boxes)
+ # box nms 3d over 6 images in a frame
+ # TODO: move this global setting into config
+ nms_cfg = dict(
+ use_rotate_nms=True,
+ nms_across_levels=False,
+ nms_pre=4096,
+ nms_thr=0.05,
+ score_thr=0.01,
+ min_bbox_size=0,
+ max_per_frame=500)
+ from mmcv import Config
+ nms_cfg = Config(nms_cfg)
+ cam_boxes3d_for_nms = xywhr2xyxyr(cam_boxes3d.bev)
+ boxes3d = cam_boxes3d.tensor
+ # generate attr scores from attr labels
+ attrs = labels.new_tensor([attr for attr in attrs_per_frame])
+ boxes3d, scores, labels, attrs = box3d_multiclass_nms(
+ boxes3d,
+ cam_boxes3d_for_nms,
+ scores,
+ nms_cfg.score_thr,
+ nms_cfg.max_per_frame,
+ nms_cfg,
+ mlvl_attr_scores=attrs)
+ cam_boxes3d = CameraInstance3DBoxes(boxes3d, box_dim=9)
+ det = bbox3d2result(cam_boxes3d, scores, labels, attrs)
+ boxes, attrs = output_to_nusc_box(det)
+ boxes, attrs = cam_nusc_box_to_global(
+ self.data_infos[sample_id + 1 - CAM_NUM], boxes, attrs,
+ mapped_class_names, self.eval_detection_configs,
+ self.eval_version)
+
+ for i, box in enumerate(boxes):
+ name = mapped_class_names[box.label]
+ attr = self.get_attr_name(attrs[i], name)
+ nusc_anno = dict(
+ sample_token=sample_token,
+ translation=box.center.tolist(),
+ size=box.wlh.tolist(),
+ rotation=box.orientation.elements.tolist(),
+ velocity=box.velocity[:2].tolist(),
+ detection_name=name,
+ detection_score=box.score,
+ attribute_name=attr)
+ annos.append(nusc_anno)
+ # other views results of the same frame should be concatenated
+ if sample_token in nusc_annos:
+ nusc_annos[sample_token].extend(annos)
+ else:
+ nusc_annos[sample_token] = annos
+
+ nusc_submissions = {
+ 'meta': self.modality,
+ 'results': nusc_annos,
+ }
+
+ mmcv.mkdir_or_exist(jsonfile_prefix)
+ res_path = osp.join(jsonfile_prefix, 'results_nusc.json')
+ print('Results writes to', res_path)
+ mmcv.dump(nusc_submissions, res_path)
+ return res_path
+
+ def _evaluate_single(self,
+ result_path,
+ logger=None,
+ metric='bbox',
+ result_name='img_bbox'):
+ """Evaluation for a single model in nuScenes protocol.
+
+ Args:
+ result_path (str): Path of the result file.
+ logger (logging.Logger | str, optional): Logger used for printing
+ related information during evaluation. Default: None.
+ metric (str, optional): Metric name used for evaluation.
+ Default: 'bbox'.
+ result_name (str, optional): Result name in the metric prefix.
+ Default: 'img_bbox'.
+
+ Returns:
+ dict: Dictionary of evaluation details.
+ """
+ from nuscenes import NuScenes
+ from nuscenes.eval.detection.evaluate import NuScenesEval
+
+ output_dir = osp.join(*osp.split(result_path)[:-1])
+ nusc = NuScenes(
+ version=self.version, dataroot=self.data_root, verbose=False)
+ eval_set_map = {
+ 'v1.0-mini': 'mini_val',
+ 'v1.0-trainval': 'val',
+ }
+ nusc_eval = NuScenesEval(
+ nusc,
+ config=self.eval_detection_configs,
+ result_path=result_path,
+ eval_set=eval_set_map[self.version],
+ output_dir=output_dir,
+ verbose=False)
+ nusc_eval.main(render_curves=True)
+
+ # record metrics
+ metrics = mmcv.load(osp.join(output_dir, 'metrics_summary.json'))
+ detail = dict()
+ metric_prefix = f'{result_name}_NuScenes'
+ for name in self.CLASSES:
+ for k, v in metrics['label_aps'][name].items():
+ val = float('{:.4f}'.format(v))
+ detail['{}/{}_AP_dist_{}'.format(metric_prefix, name, k)] = val
+ for k, v in metrics['label_tp_errors'][name].items():
+ val = float('{:.4f}'.format(v))
+ detail['{}/{}_{}'.format(metric_prefix, name, k)] = val
+ for k, v in metrics['tp_errors'].items():
+ val = float('{:.4f}'.format(v))
+ detail['{}/{}'.format(metric_prefix,
+ self.ErrNameMapping[k])] = val
+
+ detail['{}/NDS'.format(metric_prefix)] = metrics['nd_score']
+ detail['{}/mAP'.format(metric_prefix)] = metrics['mean_ap']
+ return detail
+
+ def format_results(self, results, jsonfile_prefix=None, **kwargs):
+ """Format the results to json (standard format for COCO evaluation).
+
+ Args:
+ results (list[tuple | numpy.ndarray]): Testing results of the
+ dataset.
+ jsonfile_prefix (str): The prefix of json files. It includes
+ the file path and the prefix of filename, e.g., "a/b/prefix".
+ If not specified, a temp file will be created. Default: None.
+
+ Returns:
+ tuple: (result_files, tmp_dir), result_files is a dict containing
+ the json filepaths, tmp_dir is the temporal directory created
+ for saving json files when jsonfile_prefix is not specified.
+ """
+ assert isinstance(results, list), 'results must be a list'
+ assert len(results) == len(self), (
+ 'The length of results is not equal to the dataset len: {} != {}'.
+ format(len(results), len(self)))
+
+ if jsonfile_prefix is None:
+ tmp_dir = tempfile.TemporaryDirectory()
+ jsonfile_prefix = osp.join(tmp_dir.name, 'results')
+ else:
+ tmp_dir = None
+
+ # currently the output prediction results could be in two formats
+ # 1. list of dict('boxes_3d': ..., 'scores_3d': ..., 'labels_3d': ...)
+ # 2. list of dict('pts_bbox' or 'img_bbox':
+ # dict('boxes_3d': ..., 'scores_3d': ..., 'labels_3d': ...))
+ # this is a workaround to enable evaluation of both formats on nuScenes
+ # refer to https://github.com/open-mmlab/mmdetection3d/issues/449
+ if not ('pts_bbox' in results[0] or 'img_bbox' in results[0]):
+ result_files = self._format_bbox(results, jsonfile_prefix)
+ else:
+ # should take the inner dict out of 'pts_bbox' or 'img_bbox' dict
+ result_files = dict()
+ for name in results[0]:
+ # not evaluate 2D predictions on nuScenes
+ if '2d' in name:
+ continue
+ print(f'\nFormating bboxes of {name}')
+ results_ = [out[name] for out in results]
+ tmp_file_ = osp.join(jsonfile_prefix, name)
+ result_files.update(
+ {name: self._format_bbox(results_, tmp_file_)})
+
+ return result_files, tmp_dir
+
+ def evaluate(self,
+ results,
+ metric='bbox',
+ logger=None,
+ jsonfile_prefix=None,
+ result_names=['img_bbox'],
+ show=False,
+ out_dir=None,
+ pipeline=None):
+ """Evaluation in nuScenes protocol.
+
+ Args:
+ results (list[dict]): Testing results of the dataset.
+ metric (str | list[str], optional): Metrics to be evaluated.
+ Default: 'bbox'.
+ logger (logging.Logger | str, optional): Logger used for printing
+ related information during evaluation. Default: None.
+ jsonfile_prefix (str): The prefix of json files. It includes
+ the file path and the prefix of filename, e.g., "a/b/prefix".
+ If not specified, a temp file will be created. Default: None.
+ result_names (list[str], optional): Result names in the
+ metric prefix. Default: ['img_bbox'].
+ show (bool, optional): Whether to visualize.
+ Default: False.
+ out_dir (str, optional): Path to save the visualization results.
+ Default: None.
+ pipeline (list[dict], optional): raw data loading for showing.
+ Default: None.
+
+ Returns:
+ dict[str, float]: Results of each evaluation metric.
+ """
+
+ result_files, tmp_dir = self.format_results(results, jsonfile_prefix)
+
+ if isinstance(result_files, dict):
+ results_dict = dict()
+ for name in result_names:
+ print('Evaluating bboxes of {}'.format(name))
+ ret_dict = self._evaluate_single(result_files[name])
+ results_dict.update(ret_dict)
+ elif isinstance(result_files, str):
+ results_dict = self._evaluate_single(result_files)
+
+ if tmp_dir is not None:
+ tmp_dir.cleanup()
+
+ if show or out_dir:
+ self.show(results, out_dir, pipeline=pipeline)
+ return results_dict
+
+ def _extract_data(self, index, pipeline, key, load_annos=False):
+ """Load data using input pipeline and extract data according to key.
+
+ Args:
+ index (int): Index for accessing the target data.
+ pipeline (:obj:`Compose`): Composed data loading pipeline.
+ key (str | list[str]): One single or a list of data key.
+ load_annos (bool): Whether to load data annotations.
+ If True, need to set self.test_mode as False before loading.
+
+ Returns:
+ np.ndarray | torch.Tensor | list[np.ndarray | torch.Tensor]:
+ A single or a list of loaded data.
+ """
+ assert pipeline is not None, 'data loading pipeline is not provided'
+ img_info = self.data_infos[index]
+ input_dict = dict(img_info=img_info)
+
+ if load_annos:
+ ann_info = self.get_ann_info(index)
+ input_dict.update(dict(ann_info=ann_info))
+
+ self.pre_pipeline(input_dict)
+ example = pipeline(input_dict)
+
+ # extract data items according to keys
+ if isinstance(key, str):
+ data = extract_result_dict(example, key)
+ else:
+ data = [extract_result_dict(example, k) for k in key]
+
+ return data
+
+ def _get_pipeline(self, pipeline):
+ """Get data loading pipeline in self.show/evaluate function.
+
+ Args:
+ pipeline (list[dict]): Input pipeline. If None is given,
+ get from self.pipeline.
+ """
+ if pipeline is None:
+ if not hasattr(self, 'pipeline') or self.pipeline is None:
+ warnings.warn(
+ 'Use default pipeline for data loading, this may cause '
+ 'errors when data is on ceph')
+ return self._build_default_pipeline()
+ loading_pipeline = get_loading_pipeline(self.pipeline.transforms)
+ return Compose(loading_pipeline)
+ return Compose(pipeline)
+
+ def _build_default_pipeline(self):
+ """Build the default pipeline for this dataset."""
+ pipeline = [
+ dict(type='LoadImageFromFileMono3D'),
+ dict(
+ type='DefaultFormatBundle3D',
+ class_names=self.CLASSES,
+ with_label=False),
+ dict(type='Collect3D', keys=['img'])
+ ]
+ return Compose(pipeline)
+
+ def show(self, results, out_dir, show=False, pipeline=None):
+ """Results visualization.
+
+ Args:
+ results (list[dict]): List of bounding boxes results.
+ out_dir (str): Output directory of visualization result.
+ show (bool): Whether to visualize the results online.
+ Default: False.
+ pipeline (list[dict], optional): raw data loading for showing.
+ Default: None.
+ """
+ assert out_dir is not None, 'Expect out_dir, got none.'
+ pipeline = self._get_pipeline(pipeline)
+ for i, result in enumerate(results):
+ if 'img_bbox' in result.keys():
+ result = result['img_bbox']
+ data_info = self.data_infos[i]
+ img_path = data_info['file_name']
+ file_name = osp.split(img_path)[-1].split('.')[0]
+ img, img_metas = self._extract_data(i, pipeline,
+ ['img', 'img_metas'])
+ # need to transpose channel to first dim
+ img = img.numpy().transpose(1, 2, 0)
+ gt_bboxes = self.get_ann_info(i)['gt_bboxes_3d']
+ pred_bboxes = result['boxes_3d']
+ show_multi_modality_result(
+ img,
+ gt_bboxes,
+ pred_bboxes,
+ img_metas['cam2img'],
+ out_dir,
+ file_name,
+ box_mode='camera',
+ show=show)
+
+
+def output_to_nusc_box(detection):
+ """Convert the output to the box class in the nuScenes.
+
+ Args:
+ detection (dict): Detection results.
+
+ - boxes_3d (:obj:`BaseInstance3DBoxes`): Detection bbox.
+ - scores_3d (torch.Tensor): Detection scores.
+ - labels_3d (torch.Tensor): Predicted box labels.
+ - attrs_3d (torch.Tensor, optional): Predicted attributes.
+
+ Returns:
+ list[:obj:`NuScenesBox`]: List of standard NuScenesBoxes.
+ """
+ box3d = detection['boxes_3d']
+ scores = detection['scores_3d'].numpy()
+ labels = detection['labels_3d'].numpy()
+ attrs = None
+ if 'attrs_3d' in detection:
+ attrs = detection['attrs_3d'].numpy()
+
+ box_gravity_center = box3d.gravity_center.numpy()
+ box_dims = box3d.dims.numpy()
+ box_yaw = box3d.yaw.numpy()
+
+ # convert the dim/rot to nuscbox convention
+ box_dims[:, [0, 1, 2]] = box_dims[:, [2, 0, 1]]
+ box_yaw = -box_yaw
+
+ box_list = []
+ for i in range(len(box3d)):
+ q1 = pyquaternion.Quaternion(axis=[0, 0, 1], radians=box_yaw[i])
+ q2 = pyquaternion.Quaternion(axis=[1, 0, 0], radians=np.pi / 2)
+ quat = q2 * q1
+ velocity = (box3d.tensor[i, 7], 0.0, box3d.tensor[i, 8])
+ box = NuScenesBox(
+ box_gravity_center[i],
+ box_dims[i],
+ quat,
+ label=labels[i],
+ score=scores[i],
+ velocity=velocity)
+ box_list.append(box)
+ return box_list, attrs
+
+
+def cam_nusc_box_to_global(info,
+ boxes,
+ attrs,
+ classes,
+ eval_configs,
+ eval_version='detection_cvpr_2019'):
+ """Convert the box from camera to global coordinate.
+
+ Args:
+ info (dict): Info for a specific sample data, including the
+ calibration information.
+ boxes (list[:obj:`NuScenesBox`]): List of predicted NuScenesBoxes.
+ classes (list[str]): Mapped classes in the evaluation.
+ eval_configs (object): Evaluation configuration object.
+ eval_version (str, optional): Evaluation version.
+ Default: 'detection_cvpr_2019'
+
+ Returns:
+ list: List of standard NuScenesBoxes in the global
+ coordinate.
+ """
+ box_list = []
+ attr_list = []
+ for (box, attr) in zip(boxes, attrs):
+ # Move box to ego vehicle coord system
+ box.rotate(pyquaternion.Quaternion(info['cam2ego_rotation']))
+ box.translate(np.array(info['cam2ego_translation']))
+ # filter det in ego.
+ cls_range_map = eval_configs.class_range
+ radius = np.linalg.norm(box.center[:2], 2)
+ det_range = cls_range_map[classes[box.label]]
+ if radius > det_range:
+ continue
+ # Move box to global coord system
+ box.rotate(pyquaternion.Quaternion(info['ego2global_rotation']))
+ box.translate(np.array(info['ego2global_translation']))
+ box_list.append(box)
+ attr_list.append(attr)
+ return box_list, attr_list
+
+
+def global_nusc_box_to_cam(info,
+ boxes,
+ classes,
+ eval_configs,
+ eval_version='detection_cvpr_2019'):
+ """Convert the box from global to camera coordinate.
+
+ Args:
+ info (dict): Info for a specific sample data, including the
+ calibration information.
+ boxes (list[:obj:`NuScenesBox`]): List of predicted NuScenesBoxes.
+ classes (list[str]): Mapped classes in the evaluation.
+ eval_configs (object): Evaluation configuration object.
+ eval_version (str, optional): Evaluation version.
+ Default: 'detection_cvpr_2019'
+
+ Returns:
+ list: List of standard NuScenesBoxes in the global
+ coordinate.
+ """
+ box_list = []
+ for box in boxes:
+ # Move box to ego vehicle coord system
+ box.translate(-np.array(info['ego2global_translation']))
+ box.rotate(
+ pyquaternion.Quaternion(info['ego2global_rotation']).inverse)
+ # filter det in ego.
+ cls_range_map = eval_configs.class_range
+ radius = np.linalg.norm(box.center[:2], 2)
+ det_range = cls_range_map[classes[box.label]]
+ if radius > det_range:
+ continue
+ # Move box to camera coord system
+ box.translate(-np.array(info['cam2ego_translation']))
+ box.rotate(pyquaternion.Quaternion(info['cam2ego_rotation']).inverse)
+ box_list.append(box)
+ return box_list
+
+
+def nusc_box_to_cam_box3d(boxes):
+ """Convert boxes from :obj:`NuScenesBox` to :obj:`CameraInstance3DBoxes`.
+
+ Args:
+ boxes (list[:obj:`NuScenesBox`]): List of predicted NuScenesBoxes.
+
+ Returns:
+ tuple (:obj:`CameraInstance3DBoxes` | torch.Tensor | torch.Tensor):
+ Converted 3D bounding boxes, scores and labels.
+ """
+ locs = torch.Tensor([b.center for b in boxes]).view(-1, 3)
+ dims = torch.Tensor([b.wlh for b in boxes]).view(-1, 3)
+ rots = torch.Tensor([b.orientation.yaw_pitch_roll[0]
+ for b in boxes]).view(-1, 1)
+ velocity = torch.Tensor([b.velocity[0::2] for b in boxes]).view(-1, 2)
+
+ # convert nusbox to cambox convention
+ dims[:, [0, 1, 2]] = dims[:, [1, 2, 0]]
+ rots = -rots
+
+ boxes_3d = torch.cat([locs, dims, rots, velocity], dim=1).cuda()
+ cam_boxes3d = CameraInstance3DBoxes(
+ boxes_3d, box_dim=9, origin=(0.5, 0.5, 0.5))
+ scores = torch.Tensor([b.score for b in boxes]).cuda()
+ labels = torch.LongTensor([b.label for b in boxes]).cuda()
+ nms_scores = scores.new_zeros(scores.shape[0], 10 + 1)
+ indices = labels.new_tensor(list(range(scores.shape[0])))
+ nms_scores[indices, labels] = scores
+ return cam_boxes3d, nms_scores, labels
diff --git a/mmdet3d/datasets/pipelines/__init__.py b/mmdet3d/datasets/pipelines/__init__.py
new file mode 100644
index 0000000..7e7d2c6
--- /dev/null
+++ b/mmdet3d/datasets/pipelines/__init__.py
@@ -0,0 +1,32 @@
+# Copyright (c) OpenMMLab. All rights reserved.
+from .compose import Compose
+from .dbsampler import DataBaseSampler
+from .formating import Collect3D, DefaultFormatBundle, DefaultFormatBundle3D
+from .loading import (LoadAnnotations3D, LoadImageFromFileMono3D,
+ LoadMultiViewImageFromFiles, LoadPointsFromDict,
+ LoadPointsFromFile, LoadPointsFromMultiSweeps,
+ NormalizePointsColor, PointSegClassMapping)
+from .test_time_aug import MultiScaleFlipAug3D
+# yapf: disable
+from .transforms_3d import (AffineResize, BackgroundPointsFilter,
+ GlobalAlignment, GlobalRotScaleTrans,
+ IndoorPatchPointSample, IndoorPointSample,
+ ObjectNameFilter, ObjectNoise, ObjectRangeFilter,
+ ObjectSample, PointSample, PointShuffle,
+ PointsRangeFilter, RandomDropPointsColor,
+ RandomFlip3D, RandomJitterPoints, RandomShiftScale,
+ VoxelBasedPointSampler)
+
+__all__ = [
+ 'ObjectSample', 'RandomFlip3D', 'ObjectNoise', 'GlobalRotScaleTrans',
+ 'PointShuffle', 'ObjectRangeFilter', 'PointsRangeFilter', 'Collect3D',
+ 'Compose', 'LoadMultiViewImageFromFiles', 'LoadPointsFromFile',
+ 'DefaultFormatBundle', 'DefaultFormatBundle3D', 'DataBaseSampler',
+ 'NormalizePointsColor', 'LoadAnnotations3D', 'IndoorPointSample',
+ 'PointSample', 'PointSegClassMapping', 'MultiScaleFlipAug3D',
+ 'LoadPointsFromMultiSweeps', 'BackgroundPointsFilter',
+ 'VoxelBasedPointSampler', 'GlobalAlignment', 'IndoorPatchPointSample',
+ 'LoadImageFromFileMono3D', 'ObjectNameFilter', 'RandomDropPointsColor',
+ 'RandomJitterPoints', 'AffineResize', 'RandomShiftScale',
+ 'LoadPointsFromDict'
+]
diff --git a/mmdet3d/datasets/pipelines/compose.py b/mmdet3d/datasets/pipelines/compose.py
new file mode 100644
index 0000000..9ab25d9
--- /dev/null
+++ b/mmdet3d/datasets/pipelines/compose.py
@@ -0,0 +1,60 @@
+# Copyright (c) OpenMMLab. All rights reserved.
+import collections
+
+from mmcv.utils import build_from_cfg
+
+from mmdet.datasets.builder import PIPELINES as MMDET_PIPELINES
+from ..builder import PIPELINES
+
+
+@PIPELINES.register_module()
+class Compose:
+ """Compose multiple transforms sequentially. The pipeline registry of
+ mmdet3d separates with mmdet, however, sometimes we may need to use mmdet's
+ pipeline. So the class is rewritten to be able to use pipelines from both
+ mmdet3d and mmdet.
+
+ Args:
+ transforms (Sequence[dict | callable]): Sequence of transform object or
+ config dict to be composed.
+ """
+
+ def __init__(self, transforms):
+ assert isinstance(transforms, collections.abc.Sequence)
+ self.transforms = []
+ for transform in transforms:
+ if isinstance(transform, dict):
+ _, key = PIPELINES.split_scope_key(transform['type'])
+ if key in PIPELINES._module_dict.keys():
+ transform = build_from_cfg(transform, PIPELINES)
+ else:
+ transform = build_from_cfg(transform, MMDET_PIPELINES)
+ self.transforms.append(transform)
+ elif callable(transform):
+ self.transforms.append(transform)
+ else:
+ raise TypeError('transform must be callable or a dict')
+
+ def __call__(self, data):
+ """Call function to apply transforms sequentially.
+
+ Args:
+ data (dict): A result dict contains the data to transform.
+
+ Returns:
+ dict: Transformed data.
+ """
+
+ for t in self.transforms:
+ data = t(data)
+ if data is None:
+ return None
+ return data
+
+ def __repr__(self):
+ format_string = self.__class__.__name__ + '('
+ for t in self.transforms:
+ format_string += '\n'
+ format_string += f' {t}'
+ format_string += '\n)'
+ return format_string
diff --git a/mmdet3d/datasets/pipelines/data_augment_utils.py b/mmdet3d/datasets/pipelines/data_augment_utils.py
new file mode 100644
index 0000000..21be3c0
--- /dev/null
+++ b/mmdet3d/datasets/pipelines/data_augment_utils.py
@@ -0,0 +1,411 @@
+# Copyright (c) OpenMMLab. All rights reserved.
+import warnings
+
+import numba
+import numpy as np
+from numba.core.errors import NumbaPerformanceWarning
+
+from mmdet3d.core.bbox import box_np_ops
+
+warnings.filterwarnings('ignore', category=NumbaPerformanceWarning)
+
+
+@numba.njit
+def _rotation_box2d_jit_(corners, angle, rot_mat_T):
+ """Rotate 2D boxes.
+
+ Args:
+ corners (np.ndarray): Corners of boxes.
+ angle (float): Rotation angle.
+ rot_mat_T (np.ndarray): Transposed rotation matrix.
+ """
+ rot_sin = np.sin(angle)
+ rot_cos = np.cos(angle)
+ rot_mat_T[0, 0] = rot_cos
+ rot_mat_T[0, 1] = rot_sin
+ rot_mat_T[1, 0] = -rot_sin
+ rot_mat_T[1, 1] = rot_cos
+ corners[:] = corners @ rot_mat_T
+
+
+@numba.jit(nopython=True)
+def box_collision_test(boxes, qboxes, clockwise=True):
+ """Box collision test.
+
+ Args:
+ boxes (np.ndarray): Corners of current boxes.
+ qboxes (np.ndarray): Boxes to be avoid colliding.
+ clockwise (bool, optional): Whether the corners are in
+ clockwise order. Default: True.
+ """
+ N = boxes.shape[0]
+ K = qboxes.shape[0]
+ ret = np.zeros((N, K), dtype=np.bool_)
+ slices = np.array([1, 2, 3, 0])
+ lines_boxes = np.stack((boxes, boxes[:, slices, :]),
+ axis=2) # [N, 4, 2(line), 2(xy)]
+ lines_qboxes = np.stack((qboxes, qboxes[:, slices, :]), axis=2)
+ # vec = np.zeros((2,), dtype=boxes.dtype)
+ boxes_standup = box_np_ops.corner_to_standup_nd_jit(boxes)
+ qboxes_standup = box_np_ops.corner_to_standup_nd_jit(qboxes)
+ for i in range(N):
+ for j in range(K):
+ # calculate standup first
+ iw = (
+ min(boxes_standup[i, 2], qboxes_standup[j, 2]) -
+ max(boxes_standup[i, 0], qboxes_standup[j, 0]))
+ if iw > 0:
+ ih = (
+ min(boxes_standup[i, 3], qboxes_standup[j, 3]) -
+ max(boxes_standup[i, 1], qboxes_standup[j, 1]))
+ if ih > 0:
+ for k in range(4):
+ for box_l in range(4):
+ A = lines_boxes[i, k, 0]
+ B = lines_boxes[i, k, 1]
+ C = lines_qboxes[j, box_l, 0]
+ D = lines_qboxes[j, box_l, 1]
+ acd = (D[1] - A[1]) * (C[0] -
+ A[0]) > (C[1] - A[1]) * (
+ D[0] - A[0])
+ bcd = (D[1] - B[1]) * (C[0] -
+ B[0]) > (C[1] - B[1]) * (
+ D[0] - B[0])
+ if acd != bcd:
+ abc = (C[1] - A[1]) * (B[0] - A[0]) > (
+ B[1] - A[1]) * (
+ C[0] - A[0])
+ abd = (D[1] - A[1]) * (B[0] - A[0]) > (
+ B[1] - A[1]) * (
+ D[0] - A[0])
+ if abc != abd:
+ ret[i, j] = True # collision.
+ break
+ if ret[i, j] is True:
+ break
+ if ret[i, j] is False:
+ # now check complete overlap.
+ # box overlap qbox:
+ box_overlap_qbox = True
+ for box_l in range(4): # point l in qboxes
+ for k in range(4): # corner k in boxes
+ vec = boxes[i, k] - boxes[i, (k + 1) % 4]
+ if clockwise:
+ vec = -vec
+ cross = vec[1] * (
+ boxes[i, k, 0] - qboxes[j, box_l, 0])
+ cross -= vec[0] * (
+ boxes[i, k, 1] - qboxes[j, box_l, 1])
+ if cross >= 0:
+ box_overlap_qbox = False
+ break
+ if box_overlap_qbox is False:
+ break
+
+ if box_overlap_qbox is False:
+ qbox_overlap_box = True
+ for box_l in range(4): # point box_l in boxes
+ for k in range(4): # corner k in qboxes
+ vec = qboxes[j, k] - qboxes[j, (k + 1) % 4]
+ if clockwise:
+ vec = -vec
+ cross = vec[1] * (
+ qboxes[j, k, 0] - boxes[i, box_l, 0])
+ cross -= vec[0] * (
+ qboxes[j, k, 1] - boxes[i, box_l, 1])
+ if cross >= 0: #
+ qbox_overlap_box = False
+ break
+ if qbox_overlap_box is False:
+ break
+ if qbox_overlap_box:
+ ret[i, j] = True # collision.
+ else:
+ ret[i, j] = True # collision.
+ return ret
+
+
+@numba.njit
+def noise_per_box(boxes, valid_mask, loc_noises, rot_noises):
+ """Add noise to every box (only on the horizontal plane).
+
+ Args:
+ boxes (np.ndarray): Input boxes with shape (N, 5).
+ valid_mask (np.ndarray): Mask to indicate which boxes are valid
+ with shape (N).
+ loc_noises (np.ndarray): Location noises with shape (N, M, 3).
+ rot_noises (np.ndarray): Rotation noises with shape (N, M).
+
+ Returns:
+ np.ndarray: Mask to indicate whether the noise is
+ added successfully (pass the collision test).
+ """
+ num_boxes = boxes.shape[0]
+ num_tests = loc_noises.shape[1]
+ box_corners = box_np_ops.box2d_to_corner_jit(boxes)
+ current_corners = np.zeros((4, 2), dtype=boxes.dtype)
+ rot_mat_T = np.zeros((2, 2), dtype=boxes.dtype)
+ success_mask = -np.ones((num_boxes, ), dtype=np.int64)
+ # print(valid_mask)
+ for i in range(num_boxes):
+ if valid_mask[i]:
+ for j in range(num_tests):
+ current_corners[:] = box_corners[i]
+ current_corners -= boxes[i, :2]
+ _rotation_box2d_jit_(current_corners, rot_noises[i, j],
+ rot_mat_T)
+ current_corners += boxes[i, :2] + loc_noises[i, j, :2]
+ coll_mat = box_collision_test(
+ current_corners.reshape(1, 4, 2), box_corners)
+ coll_mat[0, i] = False
+ # print(coll_mat)
+ if not coll_mat.any():
+ success_mask[i] = j
+ box_corners[i] = current_corners
+ break
+ return success_mask
+
+
+@numba.njit
+def noise_per_box_v2_(boxes, valid_mask, loc_noises, rot_noises,
+ global_rot_noises):
+ """Add noise to every box (only on the horizontal plane). Version 2 used
+ when enable global rotations.
+
+ Args:
+ boxes (np.ndarray): Input boxes with shape (N, 5).
+ valid_mask (np.ndarray): Mask to indicate which boxes are valid
+ with shape (N).
+ loc_noises (np.ndarray): Location noises with shape (N, M, 3).
+ rot_noises (np.ndarray): Rotation noises with shape (N, M).
+
+ Returns:
+ np.ndarray: Mask to indicate whether the noise is
+ added successfully (pass the collision test).
+ """
+ num_boxes = boxes.shape[0]
+ num_tests = loc_noises.shape[1]
+ box_corners = box_np_ops.box2d_to_corner_jit(boxes)
+ current_corners = np.zeros((4, 2), dtype=boxes.dtype)
+ current_box = np.zeros((1, 5), dtype=boxes.dtype)
+ rot_mat_T = np.zeros((2, 2), dtype=boxes.dtype)
+ dst_pos = np.zeros((2, ), dtype=boxes.dtype)
+ success_mask = -np.ones((num_boxes, ), dtype=np.int64)
+ corners_norm = np.zeros((4, 2), dtype=boxes.dtype)
+ corners_norm[1, 1] = 1.0
+ corners_norm[2] = 1.0
+ corners_norm[3, 0] = 1.0
+ corners_norm -= np.array([0.5, 0.5], dtype=boxes.dtype)
+ corners_norm = corners_norm.reshape(4, 2)
+ for i in range(num_boxes):
+ if valid_mask[i]:
+ for j in range(num_tests):
+ current_box[0, :] = boxes[i]
+ current_radius = np.sqrt(boxes[i, 0]**2 + boxes[i, 1]**2)
+ current_grot = np.arctan2(boxes[i, 0], boxes[i, 1])
+ dst_grot = current_grot + global_rot_noises[i, j]
+ dst_pos[0] = current_radius * np.sin(dst_grot)
+ dst_pos[1] = current_radius * np.cos(dst_grot)
+ current_box[0, :2] = dst_pos
+ current_box[0, -1] += (dst_grot - current_grot)
+
+ rot_sin = np.sin(current_box[0, -1])
+ rot_cos = np.cos(current_box[0, -1])
+ rot_mat_T[0, 0] = rot_cos
+ rot_mat_T[0, 1] = rot_sin
+ rot_mat_T[1, 0] = -rot_sin
+ rot_mat_T[1, 1] = rot_cos
+ current_corners[:] = current_box[
+ 0, 2:4] * corners_norm @ rot_mat_T + current_box[0, :2]
+ current_corners -= current_box[0, :2]
+ _rotation_box2d_jit_(current_corners, rot_noises[i, j],
+ rot_mat_T)
+ current_corners += current_box[0, :2] + loc_noises[i, j, :2]
+ coll_mat = box_collision_test(
+ current_corners.reshape(1, 4, 2), box_corners)
+ coll_mat[0, i] = False
+ if not coll_mat.any():
+ success_mask[i] = j
+ box_corners[i] = current_corners
+ loc_noises[i, j, :2] += (dst_pos - boxes[i, :2])
+ rot_noises[i, j] += (dst_grot - current_grot)
+ break
+ return success_mask
+
+
+def _select_transform(transform, indices):
+ """Select transform.
+
+ Args:
+ transform (np.ndarray): Transforms to select from.
+ indices (np.ndarray): Mask to indicate which transform to select.
+
+ Returns:
+ np.ndarray: Selected transforms.
+ """
+ result = np.zeros((transform.shape[0], *transform.shape[2:]),
+ dtype=transform.dtype)
+ for i in range(transform.shape[0]):
+ if indices[i] != -1:
+ result[i] = transform[i, indices[i]]
+ return result
+
+
+@numba.njit
+def _rotation_matrix_3d_(rot_mat_T, angle, axis):
+ """Get the 3D rotation matrix.
+
+ Args:
+ rot_mat_T (np.ndarray): Transposed rotation matrix.
+ angle (float): Rotation angle.
+ axis (int): Rotation axis.
+ """
+ rot_sin = np.sin(angle)
+ rot_cos = np.cos(angle)
+ rot_mat_T[:] = np.eye(3)
+ if axis == 1:
+ rot_mat_T[0, 0] = rot_cos
+ rot_mat_T[0, 2] = rot_sin
+ rot_mat_T[2, 0] = -rot_sin
+ rot_mat_T[2, 2] = rot_cos
+ elif axis == 2 or axis == -1:
+ rot_mat_T[0, 0] = rot_cos
+ rot_mat_T[0, 1] = rot_sin
+ rot_mat_T[1, 0] = -rot_sin
+ rot_mat_T[1, 1] = rot_cos
+ elif axis == 0:
+ rot_mat_T[1, 1] = rot_cos
+ rot_mat_T[1, 2] = rot_sin
+ rot_mat_T[2, 1] = -rot_sin
+ rot_mat_T[2, 2] = rot_cos
+
+
+@numba.njit
+def points_transform_(points, centers, point_masks, loc_transform,
+ rot_transform, valid_mask):
+ """Apply transforms to points and box centers.
+
+ Args:
+ points (np.ndarray): Input points.
+ centers (np.ndarray): Input box centers.
+ point_masks (np.ndarray): Mask to indicate which points need
+ to be transformed.
+ loc_transform (np.ndarray): Location transform to be applied.
+ rot_transform (np.ndarray): Rotation transform to be applied.
+ valid_mask (np.ndarray): Mask to indicate which boxes are valid.
+ """
+ num_box = centers.shape[0]
+ num_points = points.shape[0]
+ rot_mat_T = np.zeros((num_box, 3, 3), dtype=points.dtype)
+ for i in range(num_box):
+ _rotation_matrix_3d_(rot_mat_T[i], rot_transform[i], 2)
+ for i in range(num_points):
+ for j in range(num_box):
+ if valid_mask[j]:
+ if point_masks[i, j] == 1:
+ points[i, :3] -= centers[j, :3]
+ points[i:i + 1, :3] = points[i:i + 1, :3] @ rot_mat_T[j]
+ points[i, :3] += centers[j, :3]
+ points[i, :3] += loc_transform[j]
+ break # only apply first box's transform
+
+
+@numba.njit
+def box3d_transform_(boxes, loc_transform, rot_transform, valid_mask):
+ """Transform 3D boxes.
+
+ Args:
+ boxes (np.ndarray): 3D boxes to be transformed.
+ loc_transform (np.ndarray): Location transform to be applied.
+ rot_transform (np.ndarray): Rotation transform to be applied.
+ valid_mask (np.ndarray): Mask to indicate which boxes are valid.
+ """
+ num_box = boxes.shape[0]
+ for i in range(num_box):
+ if valid_mask[i]:
+ boxes[i, :3] += loc_transform[i]
+ boxes[i, 6] += rot_transform[i]
+
+
+def noise_per_object_v3_(gt_boxes,
+ points=None,
+ valid_mask=None,
+ rotation_perturb=np.pi / 4,
+ center_noise_std=1.0,
+ global_random_rot_range=np.pi / 4,
+ num_try=100):
+ """Random rotate or remove each groundtruth independently. use kitti viewer
+ to test this function points_transform_
+
+ Args:
+ gt_boxes (np.ndarray): Ground truth boxes with shape (N, 7).
+ points (np.ndarray, optional): Input point cloud with
+ shape (M, 4). Default: None.
+ valid_mask (np.ndarray, optional): Mask to indicate which
+ boxes are valid. Default: None.
+ rotation_perturb (float, optional): Rotation perturbation.
+ Default: pi / 4.
+ center_noise_std (float, optional): Center noise standard deviation.
+ Default: 1.0.
+ global_random_rot_range (float, optional): Global random rotation
+ range. Default: pi/4.
+ num_try (int, optional): Number of try. Default: 100.
+ """
+ num_boxes = gt_boxes.shape[0]
+ if not isinstance(rotation_perturb, (list, tuple, np.ndarray)):
+ rotation_perturb = [-rotation_perturb, rotation_perturb]
+ if not isinstance(global_random_rot_range, (list, tuple, np.ndarray)):
+ global_random_rot_range = [
+ -global_random_rot_range, global_random_rot_range
+ ]
+ enable_grot = np.abs(global_random_rot_range[0] -
+ global_random_rot_range[1]) >= 1e-3
+
+ if not isinstance(center_noise_std, (list, tuple, np.ndarray)):
+ center_noise_std = [
+ center_noise_std, center_noise_std, center_noise_std
+ ]
+ if valid_mask is None:
+ valid_mask = np.ones((num_boxes, ), dtype=np.bool_)
+ center_noise_std = np.array(center_noise_std, dtype=gt_boxes.dtype)
+
+ loc_noises = np.random.normal(
+ scale=center_noise_std, size=[num_boxes, num_try, 3])
+ rot_noises = np.random.uniform(
+ rotation_perturb[0], rotation_perturb[1], size=[num_boxes, num_try])
+ gt_grots = np.arctan2(gt_boxes[:, 0], gt_boxes[:, 1])
+ grot_lowers = global_random_rot_range[0] - gt_grots
+ grot_uppers = global_random_rot_range[1] - gt_grots
+ global_rot_noises = np.random.uniform(
+ grot_lowers[..., np.newaxis],
+ grot_uppers[..., np.newaxis],
+ size=[num_boxes, num_try])
+
+ origin = (0.5, 0.5, 0)
+ gt_box_corners = box_np_ops.center_to_corner_box3d(
+ gt_boxes[:, :3],
+ gt_boxes[:, 3:6],
+ gt_boxes[:, 6],
+ origin=origin,
+ axis=2)
+
+ # TODO: rewrite this noise box function?
+ if not enable_grot:
+ selected_noise = noise_per_box(gt_boxes[:, [0, 1, 3, 4, 6]],
+ valid_mask, loc_noises, rot_noises)
+ else:
+ selected_noise = noise_per_box_v2_(gt_boxes[:, [0, 1, 3, 4, 6]],
+ valid_mask, loc_noises, rot_noises,
+ global_rot_noises)
+
+ loc_transforms = _select_transform(loc_noises, selected_noise)
+ rot_transforms = _select_transform(rot_noises, selected_noise)
+ surfaces = box_np_ops.corner_to_surfaces_3d_jit(gt_box_corners)
+ if points is not None:
+ # TODO: replace this points_in_convex function by my tools?
+ point_masks = box_np_ops.points_in_convex_polygon_3d_jit(
+ points[:, :3], surfaces)
+ points_transform_(points, gt_boxes[:, :3], point_masks, loc_transforms,
+ rot_transforms, valid_mask)
+
+ box3d_transform_(gt_boxes, loc_transforms, rot_transforms, valid_mask)
diff --git a/mmdet3d/datasets/pipelines/dbsampler.py b/mmdet3d/datasets/pipelines/dbsampler.py
new file mode 100644
index 0000000..ef82c88
--- /dev/null
+++ b/mmdet3d/datasets/pipelines/dbsampler.py
@@ -0,0 +1,340 @@
+# Copyright (c) OpenMMLab. All rights reserved.
+import copy
+import os
+import warnings
+
+import mmcv
+import numpy as np
+
+from mmdet3d.core.bbox import box_np_ops
+from mmdet3d.datasets.pipelines import data_augment_utils
+from ..builder import OBJECTSAMPLERS, PIPELINES
+
+
+class BatchSampler:
+ """Class for sampling specific category of ground truths.
+
+ Args:
+ sample_list (list[dict]): List of samples.
+ name (str, optional): The category of samples. Default: None.
+ epoch (int, optional): Sampling epoch. Default: None.
+ shuffle (bool, optional): Whether to shuffle indices. Default: False.
+ drop_reminder (bool, optional): Drop reminder. Default: False.
+ """
+
+ def __init__(self,
+ sampled_list,
+ name=None,
+ epoch=None,
+ shuffle=True,
+ drop_reminder=False):
+ self._sampled_list = sampled_list
+ self._indices = np.arange(len(sampled_list))
+ if shuffle:
+ np.random.shuffle(self._indices)
+ self._idx = 0
+ self._example_num = len(sampled_list)
+ self._name = name
+ self._shuffle = shuffle
+ self._epoch = epoch
+ self._epoch_counter = 0
+ self._drop_reminder = drop_reminder
+
+ def _sample(self, num):
+ """Sample specific number of ground truths and return indices.
+
+ Args:
+ num (int): Sampled number.
+
+ Returns:
+ list[int]: Indices of sampled ground truths.
+ """
+ if self._idx + num >= self._example_num:
+ ret = self._indices[self._idx:].copy()
+ self._reset()
+ else:
+ ret = self._indices[self._idx:self._idx + num]
+ self._idx += num
+ return ret
+
+ def _reset(self):
+ """Reset the index of batchsampler to zero."""
+ assert self._name is not None
+ # print("reset", self._name)
+ if self._shuffle:
+ np.random.shuffle(self._indices)
+ self._idx = 0
+
+ def sample(self, num):
+ """Sample specific number of ground truths.
+
+ Args:
+ num (int): Sampled number.
+
+ Returns:
+ list[dict]: Sampled ground truths.
+ """
+ indices = self._sample(num)
+ return [self._sampled_list[i] for i in indices]
+
+
+@OBJECTSAMPLERS.register_module()
+class DataBaseSampler(object):
+ """Class for sampling data from the ground truth database.
+
+ Args:
+ info_path (str): Path of groundtruth database info.
+ data_root (str): Path of groundtruth database.
+ rate (float): Rate of actual sampled over maximum sampled number.
+ prepare (dict): Name of preparation functions and the input value.
+ sample_groups (dict): Sampled classes and numbers.
+ classes (list[str], optional): List of classes. Default: None.
+ points_loader(dict, optional): Config of points loader. Default:
+ dict(type='LoadPointsFromFile', load_dim=4, use_dim=[0,1,2,3])
+ """
+
+ def __init__(self,
+ info_path,
+ data_root,
+ rate,
+ prepare,
+ sample_groups,
+ classes=None,
+ points_loader=dict(
+ type='LoadPointsFromFile',
+ coord_type='LIDAR',
+ load_dim=4,
+ use_dim=[0, 1, 2, 3]),
+ file_client_args=dict(backend='disk')):
+ super().__init__()
+ self.data_root = data_root
+ self.info_path = info_path
+ self.rate = rate
+ self.prepare = prepare
+ self.classes = classes
+ self.cat2label = {name: i for i, name in enumerate(classes)}
+ self.label2cat = {i: name for i, name in enumerate(classes)}
+ self.points_loader = mmcv.build_from_cfg(points_loader, PIPELINES)
+ self.file_client = mmcv.FileClient(**file_client_args)
+
+ # load data base infos
+ if hasattr(self.file_client, 'get_local_path'):
+ with self.file_client.get_local_path(info_path) as local_path:
+ # loading data from a file-like object needs file format
+ db_infos = mmcv.load(open(local_path, 'rb'), file_format='pkl')
+ else:
+ warnings.warn(
+ 'The used MMCV version does not have get_local_path. '
+ f'We treat the {info_path} as local paths and it '
+ 'might cause errors if the path is not a local path. '
+ 'Please use MMCV>= 1.3.16 if you meet errors.')
+ db_infos = mmcv.load(info_path)
+
+ # filter database infos
+ from mmdet3d.utils import get_root_logger
+ logger = get_root_logger()
+ for k, v in db_infos.items():
+ logger.info(f'load {len(v)} {k} database infos')
+ for prep_func, val in prepare.items():
+ db_infos = getattr(self, prep_func)(db_infos, val)
+ logger.info('After filter database:')
+ for k, v in db_infos.items():
+ logger.info(f'load {len(v)} {k} database infos')
+
+ self.db_infos = db_infos
+
+ # load sample groups
+ # TODO: more elegant way to load sample groups
+ self.sample_groups = []
+ for name, num in sample_groups.items():
+ self.sample_groups.append({name: int(num)})
+
+ self.group_db_infos = self.db_infos # just use db_infos
+ self.sample_classes = []
+ self.sample_max_nums = []
+ for group_info in self.sample_groups:
+ self.sample_classes += list(group_info.keys())
+ self.sample_max_nums += list(group_info.values())
+
+ self.sampler_dict = {}
+ for k, v in self.group_db_infos.items():
+ self.sampler_dict[k] = BatchSampler(v, k, shuffle=True)
+ # TODO: No group_sampling currently
+
+ @staticmethod
+ def filter_by_difficulty(db_infos, removed_difficulty):
+ """Filter ground truths by difficulties.
+
+ Args:
+ db_infos (dict): Info of groundtruth database.
+ removed_difficulty (list): Difficulties that are not qualified.
+
+ Returns:
+ dict: Info of database after filtering.
+ """
+ new_db_infos = {}
+ for key, dinfos in db_infos.items():
+ new_db_infos[key] = [
+ info for info in dinfos
+ if info['difficulty'] not in removed_difficulty
+ ]
+ return new_db_infos
+
+ @staticmethod
+ def filter_by_min_points(db_infos, min_gt_points_dict):
+ """Filter ground truths by number of points in the bbox.
+
+ Args:
+ db_infos (dict): Info of groundtruth database.
+ min_gt_points_dict (dict): Different number of minimum points
+ needed for different categories of ground truths.
+
+ Returns:
+ dict: Info of database after filtering.
+ """
+ for name, min_num in min_gt_points_dict.items():
+ min_num = int(min_num)
+ if min_num > 0:
+ filtered_infos = []
+ for info in db_infos[name]:
+ if info['num_points_in_gt'] >= min_num:
+ filtered_infos.append(info)
+ db_infos[name] = filtered_infos
+ return db_infos
+
+ def sample_all(self, gt_bboxes, gt_labels, img=None, ground_plane=None):
+ """Sampling all categories of bboxes.
+
+ Args:
+ gt_bboxes (np.ndarray): Ground truth bounding boxes.
+ gt_labels (np.ndarray): Ground truth labels of boxes.
+
+ Returns:
+ dict: Dict of sampled 'pseudo ground truths'.
+
+ - gt_labels_3d (np.ndarray): ground truths labels
+ of sampled objects.
+ - gt_bboxes_3d (:obj:`BaseInstance3DBoxes`):
+ sampled ground truth 3D bounding boxes
+ - points (np.ndarray): sampled points
+ - group_ids (np.ndarray): ids of sampled ground truths
+ """
+ sampled_num_dict = {}
+ sample_num_per_class = []
+ for class_name, max_sample_num in zip(self.sample_classes,
+ self.sample_max_nums):
+ class_label = self.cat2label[class_name]
+ # sampled_num = int(max_sample_num -
+ # np.sum([n == class_name for n in gt_names]))
+ sampled_num = int(max_sample_num -
+ np.sum([n == class_label for n in gt_labels]))
+ sampled_num = np.round(self.rate * sampled_num).astype(np.int64)
+ sampled_num_dict[class_name] = sampled_num
+ sample_num_per_class.append(sampled_num)
+
+ sampled = []
+ sampled_gt_bboxes = []
+ avoid_coll_boxes = gt_bboxes
+
+ for class_name, sampled_num in zip(self.sample_classes,
+ sample_num_per_class):
+ if sampled_num > 0:
+ sampled_cls = self.sample_class_v2(class_name, sampled_num,
+ avoid_coll_boxes)
+
+ sampled += sampled_cls
+ if len(sampled_cls) > 0:
+ if len(sampled_cls) == 1:
+ sampled_gt_box = sampled_cls[0]['box3d_lidar'][
+ np.newaxis, ...]
+ else:
+ sampled_gt_box = np.stack(
+ [s['box3d_lidar'] for s in sampled_cls], axis=0)
+
+ sampled_gt_bboxes += [sampled_gt_box]
+ avoid_coll_boxes = np.concatenate(
+ [avoid_coll_boxes, sampled_gt_box], axis=0)
+
+ ret = None
+ if len(sampled) > 0:
+ sampled_gt_bboxes = np.concatenate(sampled_gt_bboxes, axis=0)
+ # center = sampled_gt_bboxes[:, 0:3]
+
+ # num_sampled = len(sampled)
+ s_points_list = []
+ count = 0
+ for info in sampled:
+ file_path = os.path.join(
+ self.data_root,
+ info['path']) if self.data_root else info['path']
+ results = dict(pts_filename=file_path)
+ s_points = self.points_loader(results)['points']
+ s_points.translate(info['box3d_lidar'][:3])
+
+ count += 1
+
+ s_points_list.append(s_points)
+
+ gt_labels = np.array([self.cat2label[s['name']] for s in sampled],
+ dtype=np.long)
+
+ if ground_plane is not None:
+ xyz = sampled_gt_bboxes[:, :3]
+ dz = (ground_plane[:3][None, :] *
+ xyz).sum(-1) + ground_plane[3]
+ sampled_gt_bboxes[:, 2] -= dz
+ for i, s_points in enumerate(s_points_list):
+ s_points.tensor[:, 2].sub_(dz[i])
+
+ ret = {
+ 'gt_labels_3d':
+ gt_labels,
+ 'gt_bboxes_3d':
+ sampled_gt_bboxes,
+ 'points':
+ s_points_list[0].cat(s_points_list),
+ 'group_ids':
+ np.arange(gt_bboxes.shape[0],
+ gt_bboxes.shape[0] + len(sampled))
+ }
+
+ return ret
+
+ def sample_class_v2(self, name, num, gt_bboxes):
+ """Sampling specific categories of bounding boxes.
+
+ Args:
+ name (str): Class of objects to be sampled.
+ num (int): Number of sampled bboxes.
+ gt_bboxes (np.ndarray): Ground truth boxes.
+
+ Returns:
+ list[dict]: Valid samples after collision test.
+ """
+ sampled = self.sampler_dict[name].sample(num)
+ sampled = copy.deepcopy(sampled)
+ num_gt = gt_bboxes.shape[0]
+ num_sampled = len(sampled)
+ gt_bboxes_bv = box_np_ops.center_to_corner_box2d(
+ gt_bboxes[:, 0:2], gt_bboxes[:, 3:5], gt_bboxes[:, 6])
+
+ sp_boxes = np.stack([i['box3d_lidar'] for i in sampled], axis=0)
+ boxes = np.concatenate([gt_bboxes, sp_boxes], axis=0).copy()
+
+ sp_boxes_new = boxes[gt_bboxes.shape[0]:]
+ sp_boxes_bv = box_np_ops.center_to_corner_box2d(
+ sp_boxes_new[:, 0:2], sp_boxes_new[:, 3:5], sp_boxes_new[:, 6])
+
+ total_bv = np.concatenate([gt_bboxes_bv, sp_boxes_bv], axis=0)
+ coll_mat = data_augment_utils.box_collision_test(total_bv, total_bv)
+ diag = np.arange(total_bv.shape[0])
+ coll_mat[diag, diag] = False
+
+ valid_samples = []
+ for i in range(num_gt, num_gt + num_sampled):
+ if coll_mat[i].any():
+ coll_mat[i] = False
+ coll_mat[:, i] = False
+ else:
+ valid_samples.append(sampled[i - num_gt])
+ return valid_samples
diff --git a/mmdet3d/datasets/pipelines/formating.py b/mmdet3d/datasets/pipelines/formating.py
new file mode 100644
index 0000000..78cb0fd
--- /dev/null
+++ b/mmdet3d/datasets/pipelines/formating.py
@@ -0,0 +1,266 @@
+# Copyright (c) OpenMMLab. All rights reserved.
+import numpy as np
+from mmcv.parallel import DataContainer as DC
+
+from mmdet3d.core.bbox import BaseInstance3DBoxes
+from mmdet3d.core.points import BasePoints
+from mmdet.datasets.pipelines import to_tensor
+from ..builder import PIPELINES
+
+
+@PIPELINES.register_module()
+class DefaultFormatBundle(object):
+ """Default formatting bundle.
+
+ It simplifies the pipeline of formatting common fields, including "img",
+ "proposals", "gt_bboxes", "gt_labels", "gt_masks" and "gt_semantic_seg".
+ These fields are formatted as follows.
+
+ - img: (1)transpose, (2)to tensor, (3)to DataContainer (stack=True)
+ - proposals: (1)to tensor, (2)to DataContainer
+ - gt_bboxes: (1)to tensor, (2)to DataContainer
+ - gt_bboxes_ignore: (1)to tensor, (2)to DataContainer
+ - gt_labels: (1)to tensor, (2)to DataContainer
+ - gt_masks: (1)to tensor, (2)to DataContainer (cpu_only=True)
+ - gt_semantic_seg: (1)unsqueeze dim-0 (2)to tensor,
+ (3)to DataContainer (stack=True)
+ """
+
+ def __init__(self, ):
+ return
+
+ def __call__(self, results):
+ """Call function to transform and format common fields in results.
+
+ Args:
+ results (dict): Result dict contains the data to convert.
+
+ Returns:
+ dict: The result dict contains the data that is formatted with
+ default bundle.
+ """
+ if 'img' in results:
+ if isinstance(results['img'], list):
+ # process multiple imgs in single frame
+ imgs = [img.transpose(2, 0, 1) for img in results['img']]
+ imgs = np.ascontiguousarray(np.stack(imgs, axis=0))
+ results['img'] = DC(to_tensor(imgs), stack=True)
+ else:
+ img = np.ascontiguousarray(results['img'].transpose(2, 0, 1))
+ results['img'] = DC(to_tensor(img), stack=True)
+ for key in [
+ 'proposals', 'gt_bboxes', 'gt_bboxes_ignore', 'gt_labels',
+ 'gt_labels_3d', 'attr_labels', 'pts_instance_mask',
+ 'pts_semantic_mask', 'centers2d', 'depths'
+ ]:
+ if key not in results:
+ continue
+ if isinstance(results[key], list):
+ results[key] = DC([to_tensor(res) for res in results[key]])
+ else:
+ results[key] = DC(to_tensor(results[key]))
+ if 'gt_bboxes_3d' in results:
+ if isinstance(results['gt_bboxes_3d'], BaseInstance3DBoxes):
+ results['gt_bboxes_3d'] = DC(
+ results['gt_bboxes_3d'], cpu_only=True)
+ else:
+ results['gt_bboxes_3d'] = DC(
+ to_tensor(results['gt_bboxes_3d']))
+
+ if 'gt_masks' in results:
+ results['gt_masks'] = DC(results['gt_masks'], cpu_only=True)
+ if 'gt_semantic_seg' in results:
+ results['gt_semantic_seg'] = DC(
+ to_tensor(results['gt_semantic_seg'][None, ...]), stack=True)
+
+ return results
+
+ def __repr__(self):
+ return self.__class__.__name__
+
+
+@PIPELINES.register_module()
+class Collect3D(object):
+ """Collect data from the loader relevant to the specific task.
+
+ This is usually the last stage of the data loader pipeline. Typically keys
+ is set to some subset of "img", "proposals", "gt_bboxes",
+ "gt_bboxes_ignore", "gt_labels", and/or "gt_masks".
+
+ The "img_meta" item is always populated. The contents of the "img_meta"
+ dictionary depends on "meta_keys". By default this includes:
+
+ - 'img_shape': shape of the image input to the network as a tuple
+ (h, w, c). Note that images may be zero padded on the
+ bottom/right if the batch tensor is larger than this shape.
+ - 'scale_factor': a float indicating the preprocessing scale
+ - 'flip': a boolean indicating if image flip transform was used
+ - 'filename': path to the image file
+ - 'ori_shape': original shape of the image as a tuple (h, w, c)
+ - 'pad_shape': image shape after padding
+ - 'lidar2img': transform from lidar to image
+ - 'depth2img': transform from depth to image
+ - 'cam2img': transform from camera to image
+ - 'pcd_horizontal_flip': a boolean indicating if point cloud is
+ flipped horizontally
+ - 'pcd_vertical_flip': a boolean indicating if point cloud is
+ flipped vertically
+ - 'box_mode_3d': 3D box mode
+ - 'box_type_3d': 3D box type
+ - 'img_norm_cfg': a dict of normalization information:
+ - mean: per channel mean subtraction
+ - std: per channel std divisor
+ - to_rgb: bool indicating if bgr was converted to rgb
+ - 'pcd_trans': point cloud transformations
+ - 'sample_idx': sample index
+ - 'pcd_scale_factor': point cloud scale factor
+ - 'pcd_rotation': rotation applied to point cloud
+ - 'pts_filename': path to point cloud file.
+
+ Args:
+ keys (Sequence[str]): Keys of results to be collected in ``data``.
+ meta_keys (Sequence[str], optional): Meta keys to be converted to
+ ``mmcv.DataContainer`` and collected in ``data[img_metas]``.
+ Default: ('filename', 'ori_shape', 'img_shape', 'lidar2img',
+ 'depth2img', 'cam2img', 'pad_shape', 'scale_factor', 'flip',
+ 'pcd_horizontal_flip', 'pcd_vertical_flip', 'box_mode_3d',
+ 'box_type_3d', 'img_norm_cfg', 'pcd_trans',
+ 'sample_idx', 'pcd_scale_factor', 'pcd_rotation', 'pts_filename')
+ """
+
+ def __init__(
+ self,
+ keys,
+ meta_keys=('filename', 'ori_shape', 'img_shape', 'lidar2img',
+ 'depth2img', 'cam2img', 'pad_shape', 'scale_factor', 'flip',
+ 'pcd_horizontal_flip', 'pcd_vertical_flip', 'box_mode_3d',
+ 'box_type_3d', 'img_norm_cfg', 'pcd_trans', 'sample_idx',
+ 'pcd_scale_factor', 'pcd_rotation', 'pcd_rotation_angle',
+ 'pts_filename', 'transformation_3d_flow', 'trans_mat',
+ 'affine_aug', 'inner_center')):
+ self.keys = keys
+ self.meta_keys = meta_keys
+
+ def __call__(self, results):
+ """Call function to collect keys in results. The keys in ``meta_keys``
+ will be converted to :obj:`mmcv.DataContainer`.
+
+ Args:
+ results (dict): Result dict contains the data to collect.
+
+ Returns:
+ dict: The result dict contains the following keys
+ - keys in ``self.keys``
+ - ``img_metas``
+ """
+ data = {}
+ img_metas = {}
+ for key in self.meta_keys:
+ if key in results:
+ img_metas[key] = results[key]
+
+ data['img_metas'] = DC(img_metas, cpu_only=True)
+ for key in self.keys:
+ data[key] = results[key]
+ return data
+
+ def __repr__(self):
+ """str: Return a string that describes the module."""
+ return self.__class__.__name__ + \
+ f'(keys={self.keys}, meta_keys={self.meta_keys})'
+
+
+@PIPELINES.register_module()
+class DefaultFormatBundle3D(DefaultFormatBundle):
+ """Default formatting bundle.
+
+ It simplifies the pipeline of formatting common fields for voxels,
+ including "proposals", "gt_bboxes", "gt_labels", "gt_masks" and
+ "gt_semantic_seg".
+ These fields are formatted as follows.
+
+ - img: (1)transpose, (2)to tensor, (3)to DataContainer (stack=True)
+ - proposals: (1)to tensor, (2)to DataContainer
+ - gt_bboxes: (1)to tensor, (2)to DataContainer
+ - gt_bboxes_ignore: (1)to tensor, (2)to DataContainer
+ - gt_labels: (1)to tensor, (2)to DataContainer
+ """
+
+ def __init__(self, class_names, with_gt=True, with_label=True):
+ super(DefaultFormatBundle3D, self).__init__()
+ self.class_names = class_names
+ self.with_gt = with_gt
+ self.with_label = with_label
+
+ def __call__(self, results):
+ """Call function to transform and format common fields in results.
+
+ Args:
+ results (dict): Result dict contains the data to convert.
+
+ Returns:
+ dict: The result dict contains the data that is formatted with
+ default bundle.
+ """
+ # Format 3D data
+ if 'points' in results:
+ assert isinstance(results['points'], BasePoints)
+ results['points'] = DC(results['points'].tensor)
+
+ for key in ['voxels', 'coors', 'voxel_centers', 'num_points']:
+ if key not in results:
+ continue
+ results[key] = DC(to_tensor(results[key]), stack=False)
+
+ if self.with_gt:
+ # Clean GT bboxes in the final
+ if 'gt_bboxes_3d_mask' in results:
+ gt_bboxes_3d_mask = results['gt_bboxes_3d_mask']
+ results['gt_bboxes_3d'] = results['gt_bboxes_3d'][
+ gt_bboxes_3d_mask]
+ if 'gt_names_3d' in results:
+ results['gt_names_3d'] = results['gt_names_3d'][
+ gt_bboxes_3d_mask]
+ if 'centers2d' in results:
+ results['centers2d'] = results['centers2d'][
+ gt_bboxes_3d_mask]
+ if 'depths' in results:
+ results['depths'] = results['depths'][gt_bboxes_3d_mask]
+ if 'gt_bboxes_mask' in results:
+ gt_bboxes_mask = results['gt_bboxes_mask']
+ if 'gt_bboxes' in results:
+ results['gt_bboxes'] = results['gt_bboxes'][gt_bboxes_mask]
+ results['gt_names'] = results['gt_names'][gt_bboxes_mask]
+ if self.with_label:
+ if 'gt_names' in results and len(results['gt_names']) == 0:
+ results['gt_labels'] = np.array([], dtype=np.int64)
+ results['attr_labels'] = np.array([], dtype=np.int64)
+ elif 'gt_names' in results and isinstance(
+ results['gt_names'][0], list):
+ # gt_labels might be a list of list in multi-view setting
+ results['gt_labels'] = [
+ np.array([self.class_names.index(n) for n in res],
+ dtype=np.int64) for res in results['gt_names']
+ ]
+ elif 'gt_names' in results:
+ results['gt_labels'] = np.array([
+ self.class_names.index(n) for n in results['gt_names']
+ ],
+ dtype=np.int64)
+ # we still assume one pipeline for one frame LiDAR
+ # thus, the 3D name is list[string]
+ if 'gt_names_3d' in results:
+ results['gt_labels_3d'] = np.array([
+ self.class_names.index(n)
+ for n in results['gt_names_3d']
+ ],
+ dtype=np.int64)
+ results = super(DefaultFormatBundle3D, self).__call__(results)
+ return results
+
+ def __repr__(self):
+ """str: Return a string that describes the module."""
+ repr_str = self.__class__.__name__
+ repr_str += f'(class_names={self.class_names}, '
+ repr_str += f'with_gt={self.with_gt}, with_label={self.with_label})'
+ return repr_str
diff --git a/mmdet3d/datasets/pipelines/loading.py b/mmdet3d/datasets/pipelines/loading.py
new file mode 100644
index 0000000..e651d3e
--- /dev/null
+++ b/mmdet3d/datasets/pipelines/loading.py
@@ -0,0 +1,750 @@
+# Copyright (c) OpenMMLab. All rights reserved.
+import mmcv
+import numpy as np
+
+from mmdet3d.core.points import BasePoints, get_points_type
+from mmdet.datasets.pipelines import LoadAnnotations, LoadImageFromFile
+from ..builder import PIPELINES
+
+
+@PIPELINES.register_module()
+class LoadMultiViewImageFromFiles(object):
+ """Load multi channel images from a list of separate channel files.
+
+ Expects results['img_filename'] to be a list of filenames.
+
+ Args:
+ to_float32 (bool, optional): Whether to convert the img to float32.
+ Defaults to False.
+ color_type (str, optional): Color type of the file.
+ Defaults to 'unchanged'.
+ """
+
+ def __init__(self, to_float32=False, color_type='unchanged'):
+ self.to_float32 = to_float32
+ self.color_type = color_type
+
+ def __call__(self, results):
+ """Call function to load multi-view image from files.
+
+ Args:
+ results (dict): Result dict containing multi-view image filenames.
+
+ Returns:
+ dict: The result dict containing the multi-view image data.
+ Added keys and values are described below.
+
+ - filename (str): Multi-view image filenames.
+ - img (np.ndarray): Multi-view image arrays.
+ - img_shape (tuple[int]): Shape of multi-view image arrays.
+ - ori_shape (tuple[int]): Shape of original image arrays.
+ - pad_shape (tuple[int]): Shape of padded image arrays.
+ - scale_factor (float): Scale factor.
+ - img_norm_cfg (dict): Normalization configuration of images.
+ """
+ filename = results['img_filename']
+ # img is of shape (h, w, c, num_views)
+ img = np.stack(
+ [mmcv.imread(name, self.color_type) for name in filename], axis=-1)
+ if self.to_float32:
+ img = img.astype(np.float32)
+ results['filename'] = filename
+ # unravel to list, see `DefaultFormatBundle` in formatting.py
+ # which will transpose each image separately and then stack into array
+ results['img'] = [img[..., i] for i in range(img.shape[-1])]
+ results['img_shape'] = img.shape
+ results['ori_shape'] = img.shape
+ # Set initial values for default meta_keys
+ results['pad_shape'] = img.shape
+ results['scale_factor'] = 1.0
+ num_channels = 1 if len(img.shape) < 3 else img.shape[2]
+ results['img_norm_cfg'] = dict(
+ mean=np.zeros(num_channels, dtype=np.float32),
+ std=np.ones(num_channels, dtype=np.float32),
+ to_rgb=False)
+ return results
+
+ def __repr__(self):
+ """str: Return a string that describes the module."""
+ repr_str = self.__class__.__name__
+ repr_str += f'(to_float32={self.to_float32}, '
+ repr_str += f"color_type='{self.color_type}')"
+ return repr_str
+
+
+@PIPELINES.register_module()
+class LoadImageFromFileMono3D(LoadImageFromFile):
+ """Load an image from file in monocular 3D object detection. Compared to 2D
+ detection, additional camera parameters need to be loaded.
+
+ Args:
+ kwargs (dict): Arguments are the same as those in
+ :class:`LoadImageFromFile`.
+ """
+
+ def __call__(self, results):
+ """Call functions to load image and get image meta information.
+
+ Args:
+ results (dict): Result dict from :obj:`mmdet.CustomDataset`.
+
+ Returns:
+ dict: The dict contains loaded image and meta information.
+ """
+ super().__call__(results)
+ results['cam2img'] = results['img_info']['cam_intrinsic']
+ return results
+
+
+@PIPELINES.register_module()
+class LoadPointsFromMultiSweeps(object):
+ """Load points from multiple sweeps.
+
+ This is usually used for nuScenes dataset to utilize previous sweeps.
+
+ Args:
+ sweeps_num (int, optional): Number of sweeps. Defaults to 10.
+ load_dim (int, optional): Dimension number of the loaded points.
+ Defaults to 5.
+ use_dim (list[int], optional): Which dimension to use.
+ Defaults to [0, 1, 2, 4].
+ file_client_args (dict, optional): Config dict of file clients,
+ refer to
+ https://github.com/open-mmlab/mmcv/blob/master/mmcv/fileio/file_client.py
+ for more details. Defaults to dict(backend='disk').
+ pad_empty_sweeps (bool, optional): Whether to repeat keyframe when
+ sweeps is empty. Defaults to False.
+ remove_close (bool, optional): Whether to remove close points.
+ Defaults to False.
+ test_mode (bool, optional): If `test_mode=True`, it will not
+ randomly sample sweeps but select the nearest N frames.
+ Defaults to False.
+ """
+
+ def __init__(self,
+ sweeps_num=10,
+ load_dim=5,
+ use_dim=[0, 1, 2, 4],
+ file_client_args=dict(backend='disk'),
+ pad_empty_sweeps=False,
+ remove_close=False,
+ test_mode=False):
+ self.load_dim = load_dim
+ self.sweeps_num = sweeps_num
+ self.use_dim = use_dim
+ self.file_client_args = file_client_args.copy()
+ self.file_client = None
+ self.pad_empty_sweeps = pad_empty_sweeps
+ self.remove_close = remove_close
+ self.test_mode = test_mode
+
+ def _load_points(self, pts_filename):
+ """Private function to load point clouds data.
+
+ Args:
+ pts_filename (str): Filename of point clouds data.
+
+ Returns:
+ np.ndarray: An array containing point clouds data.
+ """
+ if self.file_client is None:
+ self.file_client = mmcv.FileClient(**self.file_client_args)
+ try:
+ pts_bytes = self.file_client.get(pts_filename)
+ points = np.frombuffer(pts_bytes, dtype=np.float32)
+ except ConnectionError:
+ mmcv.check_file_exist(pts_filename)
+ if pts_filename.endswith('.npy'):
+ points = np.load(pts_filename)
+ else:
+ points = np.fromfile(pts_filename, dtype=np.float32)
+ return points
+
+ def _remove_close(self, points, radius=1.0):
+ """Removes point too close within a certain radius from origin.
+
+ Args:
+ points (np.ndarray | :obj:`BasePoints`): Sweep points.
+ radius (float, optional): Radius below which points are removed.
+ Defaults to 1.0.
+
+ Returns:
+ np.ndarray: Points after removing.
+ """
+ if isinstance(points, np.ndarray):
+ points_numpy = points
+ elif isinstance(points, BasePoints):
+ points_numpy = points.tensor.numpy()
+ else:
+ raise NotImplementedError
+ x_filt = np.abs(points_numpy[:, 0]) < radius
+ y_filt = np.abs(points_numpy[:, 1]) < radius
+ not_close = np.logical_not(np.logical_and(x_filt, y_filt))
+ return points[not_close]
+
+ def __call__(self, results):
+ """Call function to load multi-sweep point clouds from files.
+
+ Args:
+ results (dict): Result dict containing multi-sweep point cloud
+ filenames.
+
+ Returns:
+ dict: The result dict containing the multi-sweep points data.
+ Added key and value are described below.
+
+ - points (np.ndarray | :obj:`BasePoints`): Multi-sweep point
+ cloud arrays.
+ """
+ points = results['points']
+ points.tensor[:, 4] = 0
+ sweep_points_list = [points]
+ ts = results['timestamp']
+ if self.pad_empty_sweeps and len(results['sweeps']) == 0:
+ for i in range(self.sweeps_num):
+ if self.remove_close:
+ sweep_points_list.append(self._remove_close(points))
+ else:
+ sweep_points_list.append(points)
+ else:
+ if len(results['sweeps']) <= self.sweeps_num:
+ choices = np.arange(len(results['sweeps']))
+ elif self.test_mode:
+ choices = np.arange(self.sweeps_num)
+ else:
+ choices = np.random.choice(
+ len(results['sweeps']), self.sweeps_num, replace=False)
+ for idx in choices:
+ sweep = results['sweeps'][idx]
+ points_sweep = self._load_points(sweep['data_path'])
+ points_sweep = np.copy(points_sweep).reshape(-1, self.load_dim)
+ if self.remove_close:
+ points_sweep = self._remove_close(points_sweep)
+ sweep_ts = sweep['timestamp'] / 1e6
+ points_sweep[:, :3] = points_sweep[:, :3] @ sweep[
+ 'sensor2lidar_rotation'].T
+ points_sweep[:, :3] += sweep['sensor2lidar_translation']
+ points_sweep[:, 4] = ts - sweep_ts
+ points_sweep = points.new_point(points_sweep)
+ sweep_points_list.append(points_sweep)
+
+ points = points.cat(sweep_points_list)
+ points = points[:, self.use_dim]
+ results['points'] = points
+ return results
+
+ def __repr__(self):
+ """str: Return a string that describes the module."""
+ return f'{self.__class__.__name__}(sweeps_num={self.sweeps_num})'
+
+
+@PIPELINES.register_module()
+class PointSegClassMapping(object):
+ """Map original semantic class to valid category ids.
+
+ Map valid classes as 0~len(valid_cat_ids)-1 and
+ others as len(valid_cat_ids).
+
+ Args:
+ valid_cat_ids (tuple[int]): A tuple of valid category.
+ max_cat_id (int, optional): The max possible cat_id in input
+ segmentation mask. Defaults to 40.
+ """
+
+ def __init__(self, valid_cat_ids, max_cat_id=40):
+ assert max_cat_id >= np.max(valid_cat_ids), \
+ 'max_cat_id should be greater than maximum id in valid_cat_ids'
+
+ self.valid_cat_ids = valid_cat_ids
+ self.max_cat_id = int(max_cat_id)
+
+ # build cat_id to class index mapping
+ neg_cls = len(valid_cat_ids)
+ self.cat_id2class = np.ones(
+ self.max_cat_id + 1, dtype=np.int) * neg_cls
+ for cls_idx, cat_id in enumerate(valid_cat_ids):
+ self.cat_id2class[cat_id] = cls_idx
+
+ def __call__(self, results):
+ """Call function to map original semantic class to valid category ids.
+
+ Args:
+ results (dict): Result dict containing point semantic masks.
+
+ Returns:
+ dict: The result dict containing the mapped category ids.
+ Updated key and value are described below.
+
+ - pts_semantic_mask (np.ndarray): Mapped semantic masks.
+ """
+ assert 'pts_semantic_mask' in results
+ pts_semantic_mask = results['pts_semantic_mask']
+
+ converted_pts_sem_mask = self.cat_id2class[pts_semantic_mask]
+
+ results['pts_semantic_mask'] = converted_pts_sem_mask
+ return results
+
+ def __repr__(self):
+ """str: Return a string that describes the module."""
+ repr_str = self.__class__.__name__
+ repr_str += f'(valid_cat_ids={self.valid_cat_ids}, '
+ repr_str += f'max_cat_id={self.max_cat_id})'
+ return repr_str
+
+
+@PIPELINES.register_module()
+class PointSegClassMappingV2(object):
+ """Map original semantic class to valid category ids.
+
+ Map valid classes as 0~len(valid_cat_ids)-1 and
+ others as len(valid_cat_ids).
+
+ Args:
+ valid_cat_ids (tuple[int]): A tuple of valid category.
+ max_cat_id (int, optional): The max possible cat_id in input
+ segmentation mask. Defaults to 40.
+ """
+
+ def __init__(self, valid_cat_ids, max_cat_id=40):
+ assert max_cat_id >= np.max(valid_cat_ids), \
+ 'max_cat_id should be greater than maximum id in valid_cat_ids'
+
+ self.valid_cat_ids = valid_cat_ids
+ self.max_cat_id = int(max_cat_id)
+
+ # build cat_id to class index mapping
+ self.cat_id2class = -np.ones(
+ self.max_cat_id + 1, dtype=np.int)
+ for cls_idx, cat_id in enumerate(valid_cat_ids):
+ self.cat_id2class[cat_id] = cls_idx
+
+ def __call__(self, results):
+ """Call function to map original semantic class to valid category ids.
+
+ Args:
+ results (dict): Result dict containing point semantic masks.
+
+ Returns:
+ dict: The result dict containing the mapped category ids.
+ Updated key and value are described below.
+
+ - pts_semantic_mask (np.ndarray): Mapped semantic masks.
+ - pts_instance_mask (np.ndarray): Mapped instance masks.
+ """
+ assert 'pts_semantic_mask' in results
+ pts_semantic_mask = results['pts_semantic_mask']
+ converted_pts_sem_mask = self.cat_id2class[pts_semantic_mask]
+
+ mask = converted_pts_sem_mask >= 0
+ pts_instance_mask = results['pts_instance_mask']
+ instance_ids = np.unique(pts_instance_mask[mask])
+ assert len(instance_ids) == len(results['gt_bboxes_3d'])
+ mapping = -np.ones(
+ pts_instance_mask.max() + 1, dtype=np.int)
+ for i, instance_id in enumerate(instance_ids):
+ mapping[instance_id] = i
+ converted_pts_instance_mask = mapping[pts_instance_mask]
+
+ results['pts_semantic_mask'] = converted_pts_sem_mask
+ results['pts_instance_mask'] = converted_pts_instance_mask
+ return results
+
+ def __repr__(self):
+ """str: Return a string that describes the module."""
+ repr_str = self.__class__.__name__
+ repr_str += f'(valid_cat_ids={self.valid_cat_ids}, '
+ repr_str += f'max_cat_id={self.max_cat_id})'
+ return repr_str
+
+
+@PIPELINES.register_module()
+class NormalizePointsColor(object):
+ """Normalize color of points.
+
+ Args:
+ color_mean (list[float]): Mean color of the point cloud.
+ """
+
+ def __init__(self, color_mean):
+ self.color_mean = color_mean
+
+ def __call__(self, results):
+ """Call function to normalize color of points.
+
+ Args:
+ results (dict): Result dict containing point clouds data.
+
+ Returns:
+ dict: The result dict containing the normalized points.
+ Updated key and value are described below.
+
+ - points (:obj:`BasePoints`): Points after color normalization.
+ """
+ points = results['points']
+ assert points.attribute_dims is not None and \
+ 'color' in points.attribute_dims.keys(), \
+ 'Expect points have color attribute'
+ if self.color_mean is not None:
+ points.color = points.color - \
+ points.color.new_tensor(self.color_mean)
+ points.color = points.color / 255.0
+ results['points'] = points
+ return results
+
+ def __repr__(self):
+ """str: Return a string that describes the module."""
+ repr_str = self.__class__.__name__
+ repr_str += f'(color_mean={self.color_mean})'
+ return repr_str
+
+
+@PIPELINES.register_module()
+class LoadPointsFromFile(object):
+ """Load Points From File.
+
+ Load points from file.
+
+ Args:
+ coord_type (str): The type of coordinates of points cloud.
+ Available options includes:
+ - 'LIDAR': Points in LiDAR coordinates.
+ - 'DEPTH': Points in depth coordinates, usually for indoor dataset.
+ - 'CAMERA': Points in camera coordinates.
+ load_dim (int, optional): The dimension of the loaded points.
+ Defaults to 6.
+ use_dim (list[int], optional): Which dimensions of the points to use.
+ Defaults to [0, 1, 2]. For KITTI dataset, set use_dim=4
+ or use_dim=[0, 1, 2, 3] to use the intensity dimension.
+ shift_height (bool, optional): Whether to use shifted height.
+ Defaults to False.
+ use_color (bool, optional): Whether to use color features.
+ Defaults to False.
+ file_client_args (dict, optional): Config dict of file clients,
+ refer to
+ https://github.com/open-mmlab/mmcv/blob/master/mmcv/fileio/file_client.py
+ for more details. Defaults to dict(backend='disk').
+ """
+
+ def __init__(self,
+ coord_type,
+ load_dim=6,
+ use_dim=[0, 1, 2],
+ shift_height=False,
+ use_color=False,
+ file_client_args=dict(backend='disk')):
+ self.shift_height = shift_height
+ self.use_color = use_color
+ if isinstance(use_dim, int):
+ use_dim = list(range(use_dim))
+ assert max(use_dim) < load_dim, \
+ f'Expect all used dimensions < {load_dim}, got {use_dim}'
+ assert coord_type in ['CAMERA', 'LIDAR', 'DEPTH']
+
+ self.coord_type = coord_type
+ self.load_dim = load_dim
+ self.use_dim = use_dim
+ self.file_client_args = file_client_args.copy()
+ self.file_client = None
+
+ def _load_points(self, pts_filename):
+ """Private function to load point clouds data.
+
+ Args:
+ pts_filename (str): Filename of point clouds data.
+
+ Returns:
+ np.ndarray: An array containing point clouds data.
+ """
+ if self.file_client is None:
+ self.file_client = mmcv.FileClient(**self.file_client_args)
+ try:
+ pts_bytes = self.file_client.get(pts_filename)
+ points = np.frombuffer(pts_bytes, dtype=np.float32)
+ except ConnectionError:
+ mmcv.check_file_exist(pts_filename)
+ if pts_filename.endswith('.npy'):
+ points = np.load(pts_filename)
+ else:
+ points = np.fromfile(pts_filename, dtype=np.float32)
+
+ return points
+
+ def __call__(self, results):
+ """Call function to load points data from file.
+
+ Args:
+ results (dict): Result dict containing point clouds data.
+
+ Returns:
+ dict: The result dict containing the point clouds data.
+ Added key and value are described below.
+
+ - points (:obj:`BasePoints`): Point clouds data.
+ """
+ pts_filename = results['pts_filename']
+ points = self._load_points(pts_filename)
+ points = points.reshape(-1, self.load_dim)
+ points = points[:, self.use_dim]
+ attribute_dims = None
+
+ if self.shift_height:
+ floor_height = np.percentile(points[:, 2], 0.99)
+ height = points[:, 2] - floor_height
+ points = np.concatenate(
+ [points[:, :3],
+ np.expand_dims(height, 1), points[:, 3:]], 1)
+ attribute_dims = dict(height=3)
+
+ if self.use_color:
+ assert len(self.use_dim) >= 6
+ if attribute_dims is None:
+ attribute_dims = dict()
+ attribute_dims.update(
+ dict(color=[
+ points.shape[1] - 3,
+ points.shape[1] - 2,
+ points.shape[1] - 1,
+ ]))
+
+ points_class = get_points_type(self.coord_type)
+ points = points_class(
+ points, points_dim=points.shape[-1], attribute_dims=attribute_dims)
+ results['points'] = points
+
+ return results
+
+ def __repr__(self):
+ """str: Return a string that describes the module."""
+ repr_str = self.__class__.__name__ + '('
+ repr_str += f'shift_height={self.shift_height}, '
+ repr_str += f'use_color={self.use_color}, '
+ repr_str += f'file_client_args={self.file_client_args}, '
+ repr_str += f'load_dim={self.load_dim}, '
+ repr_str += f'use_dim={self.use_dim})'
+ return repr_str
+
+
+@PIPELINES.register_module()
+class LoadPointsFromDict(LoadPointsFromFile):
+ """Load Points From Dict."""
+
+ def __call__(self, results):
+ assert 'points' in results
+ return results
+
+
+@PIPELINES.register_module()
+class LoadAnnotations3D(LoadAnnotations):
+ """Load Annotations3D.
+
+ Load instance mask and semantic mask of points and
+ encapsulate the items into related fields.
+
+ Args:
+ with_bbox_3d (bool, optional): Whether to load 3D boxes.
+ Defaults to True.
+ with_label_3d (bool, optional): Whether to load 3D labels.
+ Defaults to True.
+ with_attr_label (bool, optional): Whether to load attribute label.
+ Defaults to False.
+ with_mask_3d (bool, optional): Whether to load 3D instance masks.
+ for points. Defaults to False.
+ with_seg_3d (bool, optional): Whether to load 3D semantic masks.
+ for points. Defaults to False.
+ with_bbox (bool, optional): Whether to load 2D boxes.
+ Defaults to False.
+ with_label (bool, optional): Whether to load 2D labels.
+ Defaults to False.
+ with_mask (bool, optional): Whether to load 2D instance masks.
+ Defaults to False.
+ with_seg (bool, optional): Whether to load 2D semantic masks.
+ Defaults to False.
+ with_bbox_depth (bool, optional): Whether to load 2.5D boxes.
+ Defaults to False.
+ poly2mask (bool, optional): Whether to convert polygon annotations
+ to bitmasks. Defaults to True.
+ seg_3d_dtype (dtype, optional): Dtype of 3D semantic masks.
+ Defaults to int64
+ file_client_args (dict): Config dict of file clients, refer to
+ https://github.com/open-mmlab/mmcv/blob/master/mmcv/fileio/file_client.py
+ for more details.
+ """
+
+ def __init__(self,
+ with_bbox_3d=True,
+ with_label_3d=True,
+ with_attr_label=False,
+ with_mask_3d=False,
+ with_seg_3d=False,
+ with_bbox=False,
+ with_label=False,
+ with_mask=False,
+ with_seg=False,
+ with_bbox_depth=False,
+ poly2mask=True,
+ seg_3d_dtype=np.int64,
+ file_client_args=dict(backend='disk')):
+ super().__init__(
+ with_bbox,
+ with_label,
+ with_mask,
+ with_seg,
+ poly2mask,
+ file_client_args=file_client_args)
+ self.with_bbox_3d = with_bbox_3d
+ self.with_bbox_depth = with_bbox_depth
+ self.with_label_3d = with_label_3d
+ self.with_attr_label = with_attr_label
+ self.with_mask_3d = with_mask_3d
+ self.with_seg_3d = with_seg_3d
+ self.seg_3d_dtype = seg_3d_dtype
+
+ def _load_bboxes_3d(self, results):
+ """Private function to load 3D bounding box annotations.
+
+ Args:
+ results (dict): Result dict from :obj:`mmdet3d.CustomDataset`.
+
+ Returns:
+ dict: The dict containing loaded 3D bounding box annotations.
+ """
+ results['gt_bboxes_3d'] = results['ann_info']['gt_bboxes_3d']
+ results['bbox3d_fields'].append('gt_bboxes_3d')
+ return results
+
+ def _load_bboxes_depth(self, results):
+ """Private function to load 2.5D bounding box annotations.
+
+ Args:
+ results (dict): Result dict from :obj:`mmdet3d.CustomDataset`.
+
+ Returns:
+ dict: The dict containing loaded 2.5D bounding box annotations.
+ """
+ results['centers2d'] = results['ann_info']['centers2d']
+ results['depths'] = results['ann_info']['depths']
+ return results
+
+ def _load_labels_3d(self, results):
+ """Private function to load label annotations.
+
+ Args:
+ results (dict): Result dict from :obj:`mmdet3d.CustomDataset`.
+
+ Returns:
+ dict: The dict containing loaded label annotations.
+ """
+ results['gt_labels_3d'] = results['ann_info']['gt_labels_3d']
+ return results
+
+ def _load_attr_labels(self, results):
+ """Private function to load label annotations.
+
+ Args:
+ results (dict): Result dict from :obj:`mmdet3d.CustomDataset`.
+
+ Returns:
+ dict: The dict containing loaded label annotations.
+ """
+ results['attr_labels'] = results['ann_info']['attr_labels']
+ return results
+
+ def _load_masks_3d(self, results):
+ """Private function to load 3D mask annotations.
+
+ Args:
+ results (dict): Result dict from :obj:`mmdet3d.CustomDataset`.
+
+ Returns:
+ dict: The dict containing loaded 3D mask annotations.
+ """
+ pts_instance_mask_path = results['ann_info']['pts_instance_mask_path']
+
+ if self.file_client is None:
+ self.file_client = mmcv.FileClient(**self.file_client_args)
+ try:
+ mask_bytes = self.file_client.get(pts_instance_mask_path)
+ pts_instance_mask = np.frombuffer(mask_bytes, dtype=np.int64)
+ except ConnectionError:
+ mmcv.check_file_exist(pts_instance_mask_path)
+ pts_instance_mask = np.fromfile(
+ pts_instance_mask_path, dtype=np.int64)
+
+ results['pts_instance_mask'] = pts_instance_mask
+ results['pts_mask_fields'].append('pts_instance_mask')
+ return results
+
+ def _load_semantic_seg_3d(self, results):
+ """Private function to load 3D semantic segmentation annotations.
+
+ Args:
+ results (dict): Result dict from :obj:`mmdet3d.CustomDataset`.
+
+ Returns:
+ dict: The dict containing the semantic segmentation annotations.
+ """
+ pts_semantic_mask_path = results['ann_info']['pts_semantic_mask_path']
+
+ if self.file_client is None:
+ self.file_client = mmcv.FileClient(**self.file_client_args)
+ try:
+ mask_bytes = self.file_client.get(pts_semantic_mask_path)
+ # add .copy() to fix read-only bug
+ pts_semantic_mask = np.frombuffer(
+ mask_bytes, dtype=self.seg_3d_dtype).copy()
+ except ConnectionError:
+ mmcv.check_file_exist(pts_semantic_mask_path)
+ pts_semantic_mask = np.fromfile(
+ pts_semantic_mask_path, dtype=np.int64)
+
+ results['pts_semantic_mask'] = pts_semantic_mask
+ results['pts_seg_fields'].append('pts_semantic_mask')
+ return results
+
+ def __call__(self, results):
+ """Call function to load multiple types annotations.
+
+ Args:
+ results (dict): Result dict from :obj:`mmdet3d.CustomDataset`.
+
+ Returns:
+ dict: The dict containing loaded 3D bounding box, label, mask and
+ semantic segmentation annotations.
+ """
+ results = super().__call__(results)
+ if self.with_bbox_3d:
+ results = self._load_bboxes_3d(results)
+ if results is None:
+ return None
+ if self.with_bbox_depth:
+ results = self._load_bboxes_depth(results)
+ if results is None:
+ return None
+ if self.with_label_3d:
+ results = self._load_labels_3d(results)
+ if self.with_attr_label:
+ results = self._load_attr_labels(results)
+ if self.with_mask_3d:
+ results = self._load_masks_3d(results)
+ if self.with_seg_3d:
+ results = self._load_semantic_seg_3d(results)
+
+ return results
+
+ def __repr__(self):
+ """str: Return a string that describes the module."""
+ indent_str = ' '
+ repr_str = self.__class__.__name__ + '(\n'
+ repr_str += f'{indent_str}with_bbox_3d={self.with_bbox_3d}, '
+ repr_str += f'{indent_str}with_label_3d={self.with_label_3d}, '
+ repr_str += f'{indent_str}with_attr_label={self.with_attr_label}, '
+ repr_str += f'{indent_str}with_mask_3d={self.with_mask_3d}, '
+ repr_str += f'{indent_str}with_seg_3d={self.with_seg_3d}, '
+ repr_str += f'{indent_str}with_bbox={self.with_bbox}, '
+ repr_str += f'{indent_str}with_label={self.with_label}, '
+ repr_str += f'{indent_str}with_mask={self.with_mask}, '
+ repr_str += f'{indent_str}with_seg={self.with_seg}, '
+ repr_str += f'{indent_str}with_bbox_depth={self.with_bbox_depth}, '
+ repr_str += f'{indent_str}poly2mask={self.poly2mask})'
+ return repr_str
diff --git a/mmdet3d/datasets/pipelines/test_time_aug.py b/mmdet3d/datasets/pipelines/test_time_aug.py
new file mode 100644
index 0000000..d53f110
--- /dev/null
+++ b/mmdet3d/datasets/pipelines/test_time_aug.py
@@ -0,0 +1,229 @@
+# Copyright (c) OpenMMLab. All rights reserved.
+import warnings
+from copy import deepcopy
+
+import mmcv
+
+from ..builder import PIPELINES
+from .compose import Compose
+
+
+@PIPELINES.register_module()
+class MultiScaleFlipAug:
+ """Test-time augmentation with multiple scales and flipping. An example
+ configuration is as followed:
+
+ .. code-block::
+ img_scale=[(1333, 400), (1333, 800)],
+ flip=True,
+ transforms=[
+ dict(type='Resize', keep_ratio=True),
+ dict(type='RandomFlip'),
+ dict(type='Normalize', **img_norm_cfg),
+ dict(type='Pad', size_divisor=32),
+ dict(type='ImageToTensor', keys=['img']),
+ dict(type='Collect', keys=['img']),
+ ]
+ After MultiScaleFLipAug with above configuration, the results are wrapped
+ into lists of the same length as followed:
+ .. code-block::
+ dict(
+ img=[...],
+ img_shape=[...],
+ scale=[(1333, 400), (1333, 400), (1333, 800), (1333, 800)]
+ flip=[False, True, False, True]
+ ...
+ )
+ Args:
+ transforms (list[dict]): Transforms to apply in each augmentation.
+ img_scale (tuple | list[tuple] | None): Images scales for resizing.
+ scale_factor (float | list[float] | None): Scale factors for resizing.
+ flip (bool): Whether apply flip augmentation. Default: False.
+ flip_direction (str | list[str]): Flip augmentation directions,
+ options are "horizontal", "vertical" and "diagonal". If
+ flip_direction is a list, multiple flip augmentations will be
+ applied. It has no effect when flip == False. Default:
+ "horizontal".
+ """
+
+ def __init__(self,
+ transforms,
+ img_scale=None,
+ scale_factor=None,
+ flip=False,
+ flip_direction='horizontal'):
+ self.transforms = Compose(transforms)
+ assert (img_scale is None) ^ (scale_factor is None), (
+ 'Must have but only one variable can be set')
+ if img_scale is not None:
+ self.img_scale = img_scale if isinstance(img_scale,
+ list) else [img_scale]
+ self.scale_key = 'scale'
+ assert mmcv.is_list_of(self.img_scale, tuple)
+ else:
+ self.img_scale = scale_factor if isinstance(
+ scale_factor, list) else [scale_factor]
+ self.scale_key = 'scale_factor'
+
+ self.flip = flip
+ self.flip_direction = flip_direction if isinstance(
+ flip_direction, list) else [flip_direction]
+ assert mmcv.is_list_of(self.flip_direction, str)
+ if not self.flip and self.flip_direction != ['horizontal']:
+ warnings.warn(
+ 'flip_direction has no effect when flip is set to False')
+ if (self.flip
+ and not any([t['type'] == 'RandomFlip' for t in transforms])):
+ warnings.warn(
+ 'flip has no effect when RandomFlip is not in transforms')
+
+ def __call__(self, results):
+ """Call function to apply test time augment transforms on results.
+
+ Args:
+ results (dict): Result dict contains the data to transform.
+ Returns:
+ dict[str: list]: The augmented data, where each value is wrapped
+ into a list.
+ """
+
+ aug_data = []
+ flip_args = [(False, None)]
+ if self.flip:
+ flip_args += [(True, direction)
+ for direction in self.flip_direction]
+ for scale in self.img_scale:
+ for flip, direction in flip_args:
+ _results = results.copy()
+ _results[self.scale_key] = scale
+ _results['flip'] = flip
+ _results['flip_direction'] = direction
+ data = self.transforms(_results)
+ aug_data.append(data)
+ # list of dict to dict of list
+ aug_data_dict = {key: [] for key in aug_data[0]}
+ for data in aug_data:
+ for key, val in data.items():
+ aug_data_dict[key].append(val)
+ return aug_data_dict
+
+ def __repr__(self):
+ repr_str = self.__class__.__name__
+ repr_str += f'(transforms={self.transforms}, '
+ repr_str += f'img_scale={self.img_scale}, flip={self.flip}, '
+ repr_str += f'flip_direction={self.flip_direction})'
+ return repr_str
+
+
+@PIPELINES.register_module()
+class MultiScaleFlipAug3D(object):
+ """Test-time augmentation with multiple scales and flipping.
+
+ Args:
+ transforms (list[dict]): Transforms to apply in each augmentation.
+ img_scale (tuple | list[tuple]: Images scales for resizing.
+ pts_scale_ratio (float | list[float]): Points scale ratios for
+ resizing.
+ flip (bool, optional): Whether apply flip augmentation.
+ Defaults to False.
+ flip_direction (str | list[str], optional): Flip augmentation
+ directions for images, options are "horizontal" and "vertical".
+ If flip_direction is list, multiple flip augmentations will
+ be applied. It has no effect when ``flip == False``.
+ Defaults to "horizontal".
+ pcd_horizontal_flip (bool, optional): Whether apply horizontal
+ flip augmentation to point cloud. Defaults to True.
+ Note that it works only when 'flip' is turned on.
+ pcd_vertical_flip (bool, optional): Whether apply vertical flip
+ augmentation to point cloud. Defaults to True.
+ Note that it works only when 'flip' is turned on.
+ """
+
+ def __init__(self,
+ transforms,
+ img_scale,
+ pts_scale_ratio,
+ flip=False,
+ flip_direction='horizontal',
+ pcd_horizontal_flip=False,
+ pcd_vertical_flip=False):
+ self.transforms = Compose(transforms)
+ self.img_scale = img_scale if isinstance(img_scale,
+ list) else [img_scale]
+ self.pts_scale_ratio = pts_scale_ratio \
+ if isinstance(pts_scale_ratio, list) else[float(pts_scale_ratio)]
+
+ assert mmcv.is_list_of(self.img_scale, tuple)
+ assert mmcv.is_list_of(self.pts_scale_ratio, float)
+
+ self.flip = flip
+ self.pcd_horizontal_flip = pcd_horizontal_flip
+ self.pcd_vertical_flip = pcd_vertical_flip
+
+ self.flip_direction = flip_direction if isinstance(
+ flip_direction, list) else [flip_direction]
+ assert mmcv.is_list_of(self.flip_direction, str)
+ if not self.flip and self.flip_direction != ['horizontal']:
+ warnings.warn(
+ 'flip_direction has no effect when flip is set to False')
+ if (self.flip and not any([(t['type'] == 'RandomFlip3D'
+ or t['type'] == 'RandomFlip')
+ for t in transforms])):
+ warnings.warn(
+ 'flip has no effect when RandomFlip is not in transforms')
+
+ def __call__(self, results):
+ """Call function to augment common fields in results.
+
+ Args:
+ results (dict): Result dict contains the data to augment.
+
+ Returns:
+ dict: The result dict contains the data that is augmented with
+ different scales and flips.
+ """
+ aug_data = []
+
+ # modified from `flip_aug = [False, True] if self.flip else [False]`
+ # to reduce unnecessary scenes when using double flip augmentation
+ # during test time
+ flip_aug = [True] if self.flip else [False]
+ pcd_horizontal_flip_aug = [False, True] \
+ if self.flip and self.pcd_horizontal_flip else [False]
+ pcd_vertical_flip_aug = [False, True] \
+ if self.flip and self.pcd_vertical_flip else [False]
+ for scale in self.img_scale:
+ for pts_scale_ratio in self.pts_scale_ratio:
+ for flip in flip_aug:
+ for pcd_horizontal_flip in pcd_horizontal_flip_aug:
+ for pcd_vertical_flip in pcd_vertical_flip_aug:
+ for direction in self.flip_direction:
+ # results.copy will cause bug
+ # since it is shallow copy
+ _results = deepcopy(results)
+ _results['scale'] = scale
+ _results['flip'] = flip
+ _results['pcd_scale_factor'] = \
+ pts_scale_ratio
+ _results['flip_direction'] = direction
+ _results['pcd_horizontal_flip'] = \
+ pcd_horizontal_flip
+ _results['pcd_vertical_flip'] = \
+ pcd_vertical_flip
+ data = self.transforms(_results)
+ aug_data.append(data)
+ # list of dict to dict of list
+ aug_data_dict = {key: [] for key in aug_data[0]}
+ for data in aug_data:
+ for key, val in data.items():
+ aug_data_dict[key].append(val)
+ return aug_data_dict
+
+ def __repr__(self):
+ """str: Return a string that describes the module."""
+ repr_str = self.__class__.__name__
+ repr_str += f'(transforms={self.transforms}, '
+ repr_str += f'img_scale={self.img_scale}, flip={self.flip}, '
+ repr_str += f'pts_scale_ratio={self.pts_scale_ratio}, '
+ repr_str += f'flip_direction={self.flip_direction})'
+ return repr_str
diff --git a/mmdet3d/datasets/pipelines/transforms_3d.py b/mmdet3d/datasets/pipelines/transforms_3d.py
new file mode 100644
index 0000000..ec26af8
--- /dev/null
+++ b/mmdet3d/datasets/pipelines/transforms_3d.py
@@ -0,0 +1,1853 @@
+# Copyright (c) OpenMMLab. All rights reserved.
+import random
+import warnings
+
+import cv2
+import numpy as np
+import torch
+
+from mmcv import is_tuple_of
+from mmcv.utils import build_from_cfg
+
+from mmdet3d.core import VoxelGenerator
+from mmdet3d.core.bbox import (CameraInstance3DBoxes, DepthInstance3DBoxes,
+ LiDARInstance3DBoxes, box_np_ops)
+from mmdet.datasets.pipelines import RandomFlip
+from ..builder import OBJECTSAMPLERS, PIPELINES
+from .data_augment_utils import noise_per_object_v3_
+import scipy
+
+@PIPELINES.register_module()
+class RandomDropPointsColor(object):
+ r"""Randomly set the color of points to all zeros.
+
+ Once this transform is executed, all the points' color will be dropped.
+ Refer to `PAConv `_ for more details.
+
+ Args:
+ drop_ratio (float, optional): The probability of dropping point colors.
+ Defaults to 0.2.
+ """
+
+ def __init__(self, drop_ratio=0.2):
+ assert isinstance(drop_ratio, (int, float)) and 0 <= drop_ratio <= 1, \
+ f'invalid drop_ratio value {drop_ratio}'
+ self.drop_ratio = drop_ratio
+
+ def __call__(self, input_dict):
+ """Call function to drop point colors.
+
+ Args:
+ input_dict (dict): Result dict from loading pipeline.
+
+ Returns:
+ dict: Results after color dropping,
+ 'points' key is updated in the result dict.
+ """
+ points = input_dict['points']
+ assert points.attribute_dims is not None and \
+ 'color' in points.attribute_dims, \
+ 'Expect points have color attribute'
+
+ # this if-expression is a bit strange
+ # `RandomDropPointsColor` is used in training 3D segmentor PAConv
+ # we discovered in our experiments that, using
+ # `if np.random.rand() > 1.0 - self.drop_ratio` consistently leads to
+ # better results than using `if np.random.rand() < self.drop_ratio`
+ # so we keep this hack in our codebase
+ if np.random.rand() > 1.0 - self.drop_ratio:
+ points.color = points.color * 0.0
+ return input_dict
+
+ def __repr__(self):
+ """str: Return a string that describes the module."""
+ repr_str = self.__class__.__name__
+ repr_str += f'(drop_ratio={self.drop_ratio})'
+ return repr_str
+
+
+@PIPELINES.register_module()
+class RandomFlip3D(RandomFlip):
+ """Flip the points & bbox.
+
+ If the input dict contains the key "flip", then the flag will be used,
+ otherwise it will be randomly decided by a ratio specified in the init
+ method.
+
+ Args:
+ sync_2d (bool, optional): Whether to apply flip according to the 2D
+ images. If True, it will apply the same flip as that to 2D images.
+ If False, it will decide whether to flip randomly and independently
+ to that of 2D images. Defaults to True.
+ flip_ratio_bev_horizontal (float, optional): The flipping probability
+ in horizontal direction. Defaults to 0.0.
+ flip_ratio_bev_vertical (float, optional): The flipping probability
+ in vertical direction. Defaults to 0.0.
+ """
+
+ def __init__(self,
+ sync_2d=True,
+ flip_ratio_bev_horizontal=0.0,
+ flip_ratio_bev_vertical=0.0,
+ **kwargs):
+ super(RandomFlip3D, self).__init__(
+ flip_ratio=flip_ratio_bev_horizontal, **kwargs)
+ self.sync_2d = sync_2d
+ self.flip_ratio_bev_vertical = flip_ratio_bev_vertical
+ if flip_ratio_bev_horizontal is not None:
+ assert isinstance(
+ flip_ratio_bev_horizontal,
+ (int, float)) and 0 <= flip_ratio_bev_horizontal <= 1
+ if flip_ratio_bev_vertical is not None:
+ assert isinstance(
+ flip_ratio_bev_vertical,
+ (int, float)) and 0 <= flip_ratio_bev_vertical <= 1
+
+ def random_flip_data_3d(self, input_dict, direction='horizontal'):
+ """Flip 3D data randomly.
+
+ Args:
+ input_dict (dict): Result dict from loading pipeline.
+ direction (str, optional): Flip direction.
+ Default: 'horizontal'.
+
+ Returns:
+ dict: Flipped results, 'points', 'bbox3d_fields' keys are
+ updated in the result dict.
+ """
+ assert direction in ['horizontal', 'vertical']
+ # for semantic segmentation task, only points will be flipped.
+ if 'bbox3d_fields' not in input_dict:
+ input_dict['points'].flip(direction)
+ return
+ if len(input_dict['bbox3d_fields']) == 0: # test mode
+ input_dict['bbox3d_fields'].append('empty_box3d')
+ input_dict['empty_box3d'] = input_dict['box_type_3d'](
+ np.array([], dtype=np.float32))
+ assert len(input_dict['bbox3d_fields']) == 1
+ for key in input_dict['bbox3d_fields']:
+ if 'points' in input_dict:
+ input_dict['points'] = input_dict[key].flip(
+ direction, points=input_dict['points'])
+ else:
+ input_dict[key].flip(direction)
+ if 'centers2d' in input_dict:
+ assert self.sync_2d is True and direction == 'horizontal', \
+ 'Only support sync_2d=True and horizontal flip with images'
+ w = input_dict['ori_shape'][1]
+ input_dict['centers2d'][..., 0] = \
+ w - input_dict['centers2d'][..., 0]
+ # need to modify the horizontal position of camera center
+ # along u-axis in the image (flip like centers2d)
+ # ['cam2img'][0][2] = c_u
+ # see more details and examples at
+ # https://github.com/open-mmlab/mmdetection3d/pull/744
+ input_dict['cam2img'][0][2] = w - input_dict['cam2img'][0][2]
+
+ def __call__(self, input_dict):
+ """Call function to flip points, values in the ``bbox3d_fields`` and
+ also flip 2D image and its annotations.
+
+ Args:
+ input_dict (dict): Result dict from loading pipeline.
+
+ Returns:
+ dict: Flipped results, 'flip', 'flip_direction',
+ 'pcd_horizontal_flip' and 'pcd_vertical_flip' keys are added
+ into result dict.
+ """
+ # flip 2D image and its annotations
+ super(RandomFlip3D, self).__call__(input_dict)
+
+ if self.sync_2d:
+ input_dict['pcd_horizontal_flip'] = input_dict['flip']
+ input_dict['pcd_vertical_flip'] = False
+ else:
+ if 'pcd_horizontal_flip' not in input_dict:
+ flip_horizontal = True if np.random.rand(
+ ) < self.flip_ratio else False
+ input_dict['pcd_horizontal_flip'] = flip_horizontal
+ if 'pcd_vertical_flip' not in input_dict:
+ flip_vertical = True if np.random.rand(
+ ) < self.flip_ratio_bev_vertical else False
+ input_dict['pcd_vertical_flip'] = flip_vertical
+
+ if 'transformation_3d_flow' not in input_dict:
+ input_dict['transformation_3d_flow'] = []
+
+ if input_dict['pcd_horizontal_flip']:
+ self.random_flip_data_3d(input_dict, 'horizontal')
+ input_dict['transformation_3d_flow'].extend(['HF'])
+ if input_dict['pcd_vertical_flip']:
+ self.random_flip_data_3d(input_dict, 'vertical')
+ input_dict['transformation_3d_flow'].extend(['VF'])
+ return input_dict
+
+ def __repr__(self):
+ """str: Return a string that describes the module."""
+ repr_str = self.__class__.__name__
+ repr_str += f'(sync_2d={self.sync_2d},'
+ repr_str += f' flip_ratio_bev_vertical={self.flip_ratio_bev_vertical})'
+ return repr_str
+
+
+@PIPELINES.register_module()
+class RandomJitterPoints(object):
+ """Randomly jitter point coordinates.
+
+ Different from the global translation in ``GlobalRotScaleTrans``, here we
+ apply different noises to each point in a scene.
+
+ Args:
+ jitter_std (list[float]): The standard deviation of jittering noise.
+ This applies random noise to all points in a 3D scene, which is
+ sampled from a gaussian distribution whose standard deviation is
+ set by ``jitter_std``. Defaults to [0.01, 0.01, 0.01]
+ clip_range (list[float]): Clip the randomly generated jitter
+ noise into this range. If None is given, don't perform clipping.
+ Defaults to [-0.05, 0.05]
+
+ Note:
+ This transform should only be used in point cloud segmentation tasks
+ because we don't transform ground-truth bboxes accordingly.
+ For similar transform in detection task, please refer to `ObjectNoise`.
+ """
+
+ def __init__(self,
+ jitter_std=[0.01, 0.01, 0.01],
+ clip_range=[-0.05, 0.05]):
+ seq_types = (list, tuple, np.ndarray)
+ if not isinstance(jitter_std, seq_types):
+ assert isinstance(jitter_std, (int, float)), \
+ f'unsupported jitter_std type {type(jitter_std)}'
+ jitter_std = [jitter_std, jitter_std, jitter_std]
+ self.jitter_std = jitter_std
+
+ if clip_range is not None:
+ if not isinstance(clip_range, seq_types):
+ assert isinstance(clip_range, (int, float)), \
+ f'unsupported clip_range type {type(clip_range)}'
+ clip_range = [-clip_range, clip_range]
+ self.clip_range = clip_range
+
+ def __call__(self, input_dict):
+ """Call function to jitter all the points in the scene.
+
+ Args:
+ input_dict (dict): Result dict from loading pipeline.
+
+ Returns:
+ dict: Results after adding noise to each point,
+ 'points' key is updated in the result dict.
+ """
+ points = input_dict['points']
+ jitter_std = np.array(self.jitter_std, dtype=np.float32)
+ jitter_noise = \
+ np.random.randn(points.shape[0], 3) * jitter_std[None, :]
+ if self.clip_range is not None:
+ jitter_noise = np.clip(jitter_noise, self.clip_range[0],
+ self.clip_range[1])
+
+ points.translate(jitter_noise)
+ return input_dict
+
+ def __repr__(self):
+ """str: Return a string that describes the module."""
+ repr_str = self.__class__.__name__
+ repr_str += f'(jitter_std={self.jitter_std},'
+ repr_str += f' clip_range={self.clip_range})'
+ return repr_str
+
+
+@PIPELINES.register_module()
+class ObjectSample(object):
+ """Sample GT objects to the data.
+
+ Args:
+ db_sampler (dict): Config dict of the database sampler.
+ sample_2d (bool): Whether to also paste 2D image patch to the images
+ This should be true when applying multi-modality cut-and-paste.
+ Defaults to False.
+ use_ground_plane (bool): Whether to use gound plane to adjust the
+ 3D labels.
+ """
+
+ def __init__(self, db_sampler, sample_2d=False, use_ground_plane=False):
+ self.sampler_cfg = db_sampler
+ self.sample_2d = sample_2d
+ if 'type' not in db_sampler.keys():
+ db_sampler['type'] = 'DataBaseSampler'
+ self.db_sampler = build_from_cfg(db_sampler, OBJECTSAMPLERS)
+ self.use_ground_plane = use_ground_plane
+
+ @staticmethod
+ def remove_points_in_boxes(points, boxes):
+ """Remove the points in the sampled bounding boxes.
+
+ Args:
+ points (:obj:`BasePoints`): Input point cloud array.
+ boxes (np.ndarray): Sampled ground truth boxes.
+
+ Returns:
+ np.ndarray: Points with those in the boxes removed.
+ """
+ masks = box_np_ops.points_in_rbbox(points.coord.numpy(), boxes)
+ points = points[np.logical_not(masks.any(-1))]
+ return points
+
+ def __call__(self, input_dict):
+ """Call function to sample ground truth objects to the data.
+
+ Args:
+ input_dict (dict): Result dict from loading pipeline.
+
+ Returns:
+ dict: Results after object sampling augmentation,
+ 'points', 'gt_bboxes_3d', 'gt_labels_3d' keys are updated
+ in the result dict.
+ """
+ gt_bboxes_3d = input_dict['gt_bboxes_3d']
+ gt_labels_3d = input_dict['gt_labels_3d']
+
+ if self.use_ground_plane and 'plane' in input_dict['ann_info']:
+ ground_plane = input_dict['ann_info']['plane']
+ input_dict['plane'] = ground_plane
+ else:
+ ground_plane = None
+ # change to float for blending operation
+ points = input_dict['points']
+ if self.sample_2d:
+ img = input_dict['img']
+ gt_bboxes_2d = input_dict['gt_bboxes']
+ # Assume for now 3D & 2D bboxes are the same
+ sampled_dict = self.db_sampler.sample_all(
+ gt_bboxes_3d.tensor.numpy(),
+ gt_labels_3d,
+ gt_bboxes_2d=gt_bboxes_2d,
+ img=img)
+ else:
+ sampled_dict = self.db_sampler.sample_all(
+ gt_bboxes_3d.tensor.numpy(),
+ gt_labels_3d,
+ img=None,
+ ground_plane=ground_plane)
+
+ if sampled_dict is not None:
+ sampled_gt_bboxes_3d = sampled_dict['gt_bboxes_3d']
+ sampled_points = sampled_dict['points']
+ sampled_gt_labels = sampled_dict['gt_labels_3d']
+
+ gt_labels_3d = np.concatenate([gt_labels_3d, sampled_gt_labels],
+ axis=0)
+ gt_bboxes_3d = gt_bboxes_3d.new_box(
+ np.concatenate(
+ [gt_bboxes_3d.tensor.numpy(), sampled_gt_bboxes_3d]))
+
+ points = self.remove_points_in_boxes(points, sampled_gt_bboxes_3d)
+ # check the points dimension
+ points = points.cat([sampled_points, points])
+
+ if self.sample_2d:
+ sampled_gt_bboxes_2d = sampled_dict['gt_bboxes_2d']
+ gt_bboxes_2d = np.concatenate(
+ [gt_bboxes_2d, sampled_gt_bboxes_2d]).astype(np.float32)
+
+ input_dict['gt_bboxes'] = gt_bboxes_2d
+ input_dict['img'] = sampled_dict['img']
+
+ input_dict['gt_bboxes_3d'] = gt_bboxes_3d
+ input_dict['gt_labels_3d'] = gt_labels_3d.astype(np.int64)
+ input_dict['points'] = points
+
+ return input_dict
+
+ def __repr__(self):
+ """str: Return a string that describes the module."""
+ repr_str = self.__class__.__name__
+ repr_str += f' sample_2d={self.sample_2d},'
+ repr_str += f' data_root={self.sampler_cfg.data_root},'
+ repr_str += f' info_path={self.sampler_cfg.info_path},'
+ repr_str += f' rate={self.sampler_cfg.rate},'
+ repr_str += f' prepare={self.sampler_cfg.prepare},'
+ repr_str += f' classes={self.sampler_cfg.classes},'
+ repr_str += f' sample_groups={self.sampler_cfg.sample_groups}'
+ return repr_str
+
+
+@PIPELINES.register_module()
+class ObjectNoise(object):
+ """Apply noise to each GT objects in the scene.
+
+ Args:
+ translation_std (list[float], optional): Standard deviation of the
+ distribution where translation noise are sampled from.
+ Defaults to [0.25, 0.25, 0.25].
+ global_rot_range (list[float], optional): Global rotation to the scene.
+ Defaults to [0.0, 0.0].
+ rot_range (list[float], optional): Object rotation range.
+ Defaults to [-0.15707963267, 0.15707963267].
+ num_try (int, optional): Number of times to try if the noise applied is
+ invalid. Defaults to 100.
+ """
+
+ def __init__(self,
+ translation_std=[0.25, 0.25, 0.25],
+ global_rot_range=[0.0, 0.0],
+ rot_range=[-0.15707963267, 0.15707963267],
+ num_try=100):
+ self.translation_std = translation_std
+ self.global_rot_range = global_rot_range
+ self.rot_range = rot_range
+ self.num_try = num_try
+
+ def __call__(self, input_dict):
+ """Call function to apply noise to each ground truth in the scene.
+
+ Args:
+ input_dict (dict): Result dict from loading pipeline.
+
+ Returns:
+ dict: Results after adding noise to each object,
+ 'points', 'gt_bboxes_3d' keys are updated in the result dict.
+ """
+ gt_bboxes_3d = input_dict['gt_bboxes_3d']
+ points = input_dict['points']
+
+ # TODO: this is inplace operation
+ numpy_box = gt_bboxes_3d.tensor.numpy()
+ numpy_points = points.tensor.numpy()
+
+ noise_per_object_v3_(
+ numpy_box,
+ numpy_points,
+ rotation_perturb=self.rot_range,
+ center_noise_std=self.translation_std,
+ global_random_rot_range=self.global_rot_range,
+ num_try=self.num_try)
+
+ input_dict['gt_bboxes_3d'] = gt_bboxes_3d.new_box(numpy_box)
+ input_dict['points'] = points.new_point(numpy_points)
+ return input_dict
+
+ def __repr__(self):
+ """str: Return a string that describes the module."""
+ repr_str = self.__class__.__name__
+ repr_str += f'(num_try={self.num_try},'
+ repr_str += f' translation_std={self.translation_std},'
+ repr_str += f' global_rot_range={self.global_rot_range},'
+ repr_str += f' rot_range={self.rot_range})'
+ return repr_str
+
+
+@PIPELINES.register_module()
+class GlobalAlignment(object):
+ """Apply global alignment to 3D scene points by rotation and translation.
+
+ Args:
+ rotation_axis (int): Rotation axis for points and bboxes rotation.
+
+ Note:
+ We do not record the applied rotation and translation as in
+ GlobalRotScaleTrans. Because usually, we do not need to reverse
+ the alignment step.
+ For example, ScanNet 3D detection task uses aligned ground-truth
+ bounding boxes for evaluation.
+ """
+
+ def __init__(self, rotation_axis):
+ self.rotation_axis = rotation_axis
+
+ def _trans_points(self, input_dict, trans_factor):
+ """Private function to translate points.
+
+ Args:
+ input_dict (dict): Result dict from loading pipeline.
+ trans_factor (np.ndarray): Translation vector to be applied.
+
+ Returns:
+ dict: Results after translation, 'points' is updated in the dict.
+ """
+ input_dict['points'].translate(trans_factor)
+
+ def _rot_points(self, input_dict, rot_mat):
+ """Private function to rotate bounding boxes and points.
+
+ Args:
+ input_dict (dict): Result dict from loading pipeline.
+ rot_mat (np.ndarray): Rotation matrix to be applied.
+
+ Returns:
+ dict: Results after rotation, 'points' is updated in the dict.
+ """
+ # input should be rot_mat_T so I transpose it here
+ input_dict['points'].rotate(rot_mat.T)
+
+ def _check_rot_mat(self, rot_mat):
+ """Check if rotation matrix is valid for self.rotation_axis.
+
+ Args:
+ rot_mat (np.ndarray): Rotation matrix to be applied.
+ """
+ is_valid = np.allclose(np.linalg.det(rot_mat), 1.0)
+ valid_array = np.zeros(3)
+ valid_array[self.rotation_axis] = 1.0
+ is_valid &= (rot_mat[self.rotation_axis, :] == valid_array).all()
+ is_valid &= (rot_mat[:, self.rotation_axis] == valid_array).all()
+ assert is_valid, f'invalid rotation matrix {rot_mat}'
+
+ def __call__(self, input_dict):
+ """Call function to shuffle points.
+
+ Args:
+ input_dict (dict): Result dict from loading pipeline.
+
+ Returns:
+ dict: Results after global alignment, 'points' and keys in
+ input_dict['bbox3d_fields'] are updated in the result dict.
+ """
+ assert 'axis_align_matrix' in input_dict['ann_info'].keys(), \
+ 'axis_align_matrix is not provided in GlobalAlignment'
+
+ axis_align_matrix = input_dict['ann_info']['axis_align_matrix']
+ assert axis_align_matrix.shape == (4, 4), \
+ f'invalid shape {axis_align_matrix.shape} for axis_align_matrix'
+ rot_mat = axis_align_matrix[:3, :3]
+ trans_vec = axis_align_matrix[:3, -1]
+
+ self._check_rot_mat(rot_mat)
+ self._rot_points(input_dict, rot_mat)
+ self._trans_points(input_dict, trans_vec)
+
+ return input_dict
+
+ def __repr__(self):
+ repr_str = self.__class__.__name__
+ repr_str += f'(rotation_axis={self.rotation_axis})'
+ return repr_str
+
+
+@PIPELINES.register_module()
+class GlobalRotScaleTrans(object):
+ """Apply global rotation, scaling and translation to a 3D scene.
+
+ Args:
+ rot_range (list[float], optional): Range of rotation angle.
+ Defaults to [-0.78539816, 0.78539816] (close to [-pi/4, pi/4]).
+ scale_ratio_range (list[float], optional): Range of scale ratio.
+ Defaults to [0.95, 1.05].
+ translation_std (list[float], optional): The standard deviation of
+ translation noise applied to a scene, which
+ is sampled from a gaussian distribution whose standard deviation
+ is set by ``translation_std``. Defaults to [0, 0, 0]
+ shift_height (bool, optional): Whether to shift height.
+ (the fourth dimension of indoor points) when scaling.
+ Defaults to False.
+ """
+
+ def __init__(self,
+ rot_range_z=[-0.78539816, 0.78539816],
+ rot_range_x_y=[-0.1308, 0.1308],
+ scale_ratio_range=[0.95, 1.05],
+ translation_std=[0, 0, 0],
+ shift_height=False):
+ seq_types = (list, tuple, np.ndarray)
+
+ self.rot_range_z = rot_range_z
+ self.rot_range_x_y = rot_range_x_y
+ self.prev_cropped_scene = {}
+
+ assert isinstance(scale_ratio_range, seq_types), \
+ f'unsupported scale_ratio_range type {type(scale_ratio_range)}'
+ self.scale_ratio_range = scale_ratio_range
+
+ if not isinstance(translation_std, seq_types):
+ assert isinstance(translation_std, (int, float)), \
+ f'unsupported translation_std type {type(translation_std)}'
+ translation_std = [
+ translation_std, translation_std, translation_std
+ ]
+ assert all([std >= 0 for std in translation_std]), \
+ 'translation_std should be positive'
+ self.translation_std = translation_std
+ self.shift_height = shift_height
+
+ def _trans_bbox_points(self, input_dict):
+ """Private function to translate bounding boxes and points.
+
+ Args:
+ input_dict (dict): Result dict from loading pipeline.
+
+ Returns:
+ dict: Results after translation, 'points', 'pcd_trans'
+ and keys in input_dict['bbox3d_fields'] are updated
+ in the result dict.
+ """
+ translation_std = np.array(self.translation_std, dtype=np.float32)
+ trans_factor = np.random.normal(scale=translation_std, size=3).T
+
+ input_dict['points'].translate(trans_factor)
+ input_dict['pcd_trans'] = trans_factor
+ for key in input_dict['bbox3d_fields']:
+ input_dict[key].translate(trans_factor)
+
+ def _rot_bbox_points(self, input_dict):
+ """Private function to rotate bounding boxes and points.
+
+ Args:
+ input_dict (dict): Result dict from loading pipeline.
+
+ Returns:
+ dict: Results after rotation, 'points', 'pcd_rotation'
+ and keys in input_dict['bbox3d_fields'] are updated
+ in the result dict.
+ """
+
+ # elastic
+ coords = input_dict['points'].tensor[:, :3].numpy()
+ coords = self.elastic(coords, 6, 40.)
+ coords = self.elastic(coords, 20, 160.)
+ input_dict['points'].tensor[:, :3] = torch.tensor(coords)
+
+ # crop
+ if len(self.prev_cropped_scene) == 0:
+ self.crop(input_dict, self.prev_cropped_scene)
+ else:
+ tmp_prev_cropped_scene = {}
+ self.crop(input_dict, tmp_prev_cropped_scene)
+ self.crop(input_dict, input_dict)
+ self.cat(input_dict, self.prev_cropped_scene)
+ self.prev_cropped_scene = tmp_prev_cropped_scene
+
+ #rotation
+ noise_rotation_z = np.random.uniform(self.rot_range_z[0], self.rot_range_z[1])
+ noise_rotation_x = np.random.uniform(self.rot_range_x_y[0], self.rot_range_x_y[1])
+ noise_rotation_y = np.random.uniform(self.rot_range_x_y[0], self.rot_range_x_y[1])
+
+ rot_mat_T_z = input_dict['points'].rotate(noise_rotation_z, axis=2)
+ rot_mat_T_x = input_dict['points'].rotate(noise_rotation_x, axis=0) #todo: is axis=0 x?
+ rot_mat_T_y = input_dict['points'].rotate(noise_rotation_y, axis=1) #todo: similarly
+ input_dict['pcd_rotation'] = rot_mat_T_z @ rot_mat_T_x @ rot_mat_T_y
+ input_dict['pcd_rotation_angle'] = noise_rotation_z
+
+ #calculate new bboxes
+ pts_instance_mask = torch.tensor(input_dict['pts_instance_mask'])
+ pts_instance_mask[pts_instance_mask == -1] = torch.max(pts_instance_mask) + 1
+ pts_instance_mask_one_hot = torch.nn.functional.one_hot(pts_instance_mask)[
+ :, :-1
+ ]
+
+ points = input_dict['points'][:, :3].tensor
+ points_for_max = points.unsqueeze(1).expand(points.shape[0], pts_instance_mask_one_hot.shape[1], points.shape[1]).clone()
+ points_for_min = points.unsqueeze(1).expand(points.shape[0], pts_instance_mask_one_hot.shape[1], points.shape[1]).clone()
+ points_for_max[~pts_instance_mask_one_hot.bool()] = float('-inf')
+ points_for_min[~pts_instance_mask_one_hot.bool()] = float('inf')
+ bboxes_max = points_for_max.max(axis=0)[0]
+ bboxes_min = points_for_min.min(axis=0)[0]
+ bboxes_sizes = bboxes_max - bboxes_min
+ bboxes_centers = (bboxes_max + bboxes_min) / 2
+ bboxes = torch.hstack((bboxes_centers, bboxes_sizes, torch.zeros_like(bboxes_sizes[:, :1])))
+
+ input_dict["gt_bboxes_3d"] = input_dict["gt_bboxes_3d"].__class__(bboxes, with_yaw=False, origin=(.5, .5, .5))
+
+ pts_semantic_mask = torch.tensor(input_dict['pts_semantic_mask'])
+ pts_semantic_mask_expand = pts_semantic_mask.unsqueeze(1).expand(pts_semantic_mask.shape[0], pts_instance_mask_one_hot.shape[1]).clone()
+ pts_semantic_mask_expand[~pts_instance_mask_one_hot.bool()] = -1
+ assert pts_semantic_mask_expand.max(axis=0)[0].shape[0] != 0
+ input_dict['gt_labels_3d'] = pts_semantic_mask_expand.max(axis=0)[0].numpy()
+
+ def elastic(self, x, gran, mag):
+ blur0 = np.ones((3, 1, 1)).astype('float32') / 3
+ blur1 = np.ones((1, 3, 1)).astype('float32') / 3
+ blur2 = np.ones((1, 1, 3)).astype('float32') / 3
+
+ bb = np.abs(x).max(0).astype(np.int32) // gran + 3
+ noise = [np.random.randn(bb[0], bb[1], bb[2]).astype('float32') for _ in range(3)]
+ noise = [scipy.ndimage.filters.convolve(n, blur0, mode='constant', cval=0) for n in noise]
+ noise = [scipy.ndimage.filters.convolve(n, blur1, mode='constant', cval=0) for n in noise]
+ noise = [scipy.ndimage.filters.convolve(n, blur2, mode='constant', cval=0) for n in noise]
+ noise = [scipy.ndimage.filters.convolve(n, blur0, mode='constant', cval=0) for n in noise]
+ noise = [scipy.ndimage.filters.convolve(n, blur1, mode='constant', cval=0) for n in noise]
+ noise = [scipy.ndimage.filters.convolve(n, blur2, mode='constant', cval=0) for n in noise]
+ ax = [np.linspace(-(b - 1) * gran, (b - 1) * gran, b) for b in bb]
+ interp = [
+ scipy.interpolate.RegularGridInterpolator(ax, n, bounds_error=0, fill_value=0)
+ for n in noise
+ ]
+
+ def g(x_):
+ return np.hstack([i(x_)[:, None] for i in interp])
+
+ return x + g(x) * mag
+
+ def cat(self, input_dict, prev_cropped_scene):
+ if input_dict['points'].tensor.shape[0] == 100000: #todo: why 100000?
+ return
+ min_x_cropped = prev_cropped_scene['points'].tensor.min(axis=0)[0][0]
+ max_x_src = input_dict['points'].tensor.max(axis=0)[0][0]
+ prev_cropped_scene['points'].tensor[:, 0] = prev_cropped_scene['points'].tensor[:, 0] - min_x_cropped + max_x_src
+
+ min_y_z_cropped = prev_cropped_scene['points'].tensor.min(axis=0)[0][1:3]
+ min_y_z_src = input_dict['points'].tensor.min(axis=0)[0][1:3]
+ prev_cropped_scene['points'].tensor[:, 1:3] = prev_cropped_scene['points'].tensor[:, 1:3] - min_y_z_cropped + min_y_z_src
+
+ input_dict['points'].tensor = torch.cat((input_dict['points'].tensor, prev_cropped_scene['points'].tensor))
+ input_dict['pts_semantic_mask'] = np.hstack((input_dict['pts_semantic_mask'], prev_cropped_scene['pts_semantic_mask']))
+ cropped_mask = prev_cropped_scene['pts_instance_mask']
+ cropped_mask[cropped_mask != -1] += input_dict['pts_instance_mask'].max() + 1
+ input_dict['pts_instance_mask'] = np.hstack((input_dict['pts_instance_mask'], cropped_mask))
+
+ def crop(self, input_dict, output_dict):
+ coords = input_dict['points'].tensor[:, :3].numpy()
+ new_idxs = self.get_cropped_idxs(coords)
+ if new_idxs.sum() == 0:
+ return
+
+ pts_instance_mask = torch.tensor(input_dict['pts_instance_mask'])
+ inst_idxs = torch.unique(pts_instance_mask)[1:] # because the first elem - -1
+ pts_instance_mask[pts_instance_mask == -1] = torch.max(pts_instance_mask) + 1
+ pts_instance_mask_one_hot = torch.nn.functional.one_hot(pts_instance_mask)[
+ :, :-1
+ ]
+ num_pts_per_inst_src = pts_instance_mask_one_hot.sum(axis=0)
+
+ pts_instance_mask = torch.cat((torch.tensor(input_dict['pts_instance_mask'][new_idxs]), inst_idxs))
+ idxs_sum = (pts_instance_mask == -1).sum()
+ pts_instance_mask[pts_instance_mask == -1] = torch.max(pts_instance_mask) + 1
+ pts_instance_mask_one_hot = torch.nn.functional.one_hot(pts_instance_mask)
+ if idxs_sum > 0:
+ pts_instance_mask_one_hot = pts_instance_mask_one_hot[:, :-1]
+
+ pts_instance_mask_one_hot = pts_instance_mask_one_hot[:-len(inst_idxs), :]
+
+ num_pts_per_inst = pts_instance_mask_one_hot.sum(axis=0)
+
+ good_insts = num_pts_per_inst / num_pts_per_inst_src > 0.3
+
+ if good_insts.sum() == 0:
+ return
+
+ pts_instance_mask_one_hot = pts_instance_mask_one_hot[:, good_insts]
+ idxs, insts = torch.where(pts_instance_mask_one_hot)
+
+ output_dict['points'] = input_dict['points'][new_idxs]
+ output_dict['pts_semantic_mask'] = input_dict['pts_semantic_mask'][new_idxs]
+ new_pts_instance_mask = torch.zeros(output_dict['points'].shape[0], dtype=torch.long) - 1
+ new_pts_instance_mask[idxs] = insts
+ output_dict['pts_instance_mask'] = new_pts_instance_mask.numpy()
+
+ def get_cropped_idxs(self, pts):
+ max_borders = pts.max(0)
+ min_borders = pts.min(0)
+
+ room_range = max_borders - min_borders
+
+ shift = 0.5 * room_range
+
+ new_max_borders = max_borders + shift
+ new_min_borders = min_borders + shift
+
+ new_idxs = (pts[:, 0] > new_min_borders[0]) * (pts[:, 0] < new_max_borders[0])
+ return new_idxs
+
+ def _scale_bbox_points(self, input_dict):
+ """Private function to scale bounding boxes and points.
+
+ Args:
+ input_dict (dict): Result dict from loading pipeline.
+
+ Returns:
+ dict: Results after scaling, 'points'and keys in
+ input_dict['bbox3d_fields'] are updated in the result dict.
+ """
+ scale = input_dict['pcd_scale_factor']
+ points = input_dict['points']
+ points.scale(scale)
+ if self.shift_height:
+ assert 'height' in points.attribute_dims.keys(), \
+ 'setting shift_height=True but points have no height attribute'
+ points.tensor[:, points.attribute_dims['height']] *= scale
+ input_dict['points'] = points
+
+ for key in input_dict['bbox3d_fields']:
+ input_dict[key].scale(scale)
+
+ def _random_scale(self, input_dict):
+ """Private function to randomly set the scale factor.
+
+ Args:
+ input_dict (dict): Result dict from loading pipeline.
+
+ Returns:
+ dict: Results after scaling, 'pcd_scale_factor' are updated
+ in the result dict.
+ """
+ scale_factor = np.random.uniform(self.scale_ratio_range[0],
+ self.scale_ratio_range[1])
+ input_dict['pcd_scale_factor'] = scale_factor
+
+ def __call__(self, input_dict):
+ """Private function to rotate, scale and translate bounding boxes and
+ points.
+
+ Args:
+ input_dict (dict): Result dict from loading pipeline.
+
+ Returns:
+ dict: Results after scaling, 'points', 'pcd_rotation',
+ 'pcd_scale_factor', 'pcd_trans' and keys in
+ input_dict['bbox3d_fields'] are updated in the result dict.
+ """
+ if 'transformation_3d_flow' not in input_dict:
+ input_dict['transformation_3d_flow'] = []
+
+ self._rot_bbox_points(input_dict)
+
+ if 'pcd_scale_factor' not in input_dict:
+ self._random_scale(input_dict)
+ self._scale_bbox_points(input_dict)
+
+ self._trans_bbox_points(input_dict)
+
+ input_dict['transformation_3d_flow'].extend(['R', 'S', 'T'])
+ return input_dict
+
+ def __repr__(self):
+ """str: Return a string that describes the module."""
+ repr_str = self.__class__.__name__
+ repr_str += f'(rot_range={self.rot_range},'
+ repr_str += f' scale_ratio_range={self.scale_ratio_range},'
+ repr_str += f' translation_std={self.translation_std},'
+ repr_str += f' shift_height={self.shift_height})'
+ return repr_str
+
+
+@PIPELINES.register_module()
+class PointShuffle(object):
+ """Shuffle input points."""
+
+ def __call__(self, input_dict):
+ """Call function to shuffle points.
+
+ Args:
+ input_dict (dict): Result dict from loading pipeline.
+
+ Returns:
+ dict: Results after filtering, 'points', 'pts_instance_mask'
+ and 'pts_semantic_mask' keys are updated in the result dict.
+ """
+ idx = input_dict['points'].shuffle()
+ idx = idx.numpy()
+
+ pts_instance_mask = input_dict.get('pts_instance_mask', None)
+ pts_semantic_mask = input_dict.get('pts_semantic_mask', None)
+
+ if pts_instance_mask is not None:
+ input_dict['pts_instance_mask'] = pts_instance_mask[idx]
+
+ if pts_semantic_mask is not None:
+ input_dict['pts_semantic_mask'] = pts_semantic_mask[idx]
+
+ return input_dict
+
+ def __repr__(self):
+ return self.__class__.__name__
+
+
+@PIPELINES.register_module()
+class ObjectRangeFilter(object):
+ """Filter objects by the range.
+
+ Args:
+ point_cloud_range (list[float]): Point cloud range.
+ """
+
+ def __init__(self, point_cloud_range):
+ self.pcd_range = np.array(point_cloud_range, dtype=np.float32)
+
+ def __call__(self, input_dict):
+ """Call function to filter objects by the range.
+
+ Args:
+ input_dict (dict): Result dict from loading pipeline.
+
+ Returns:
+ dict: Results after filtering, 'gt_bboxes_3d', 'gt_labels_3d'
+ keys are updated in the result dict.
+ """
+ # Check points instance type and initialise bev_range
+ if isinstance(input_dict['gt_bboxes_3d'],
+ (LiDARInstance3DBoxes, DepthInstance3DBoxes)):
+ bev_range = self.pcd_range[[0, 1, 3, 4]]
+ elif isinstance(input_dict['gt_bboxes_3d'], CameraInstance3DBoxes):
+ bev_range = self.pcd_range[[0, 2, 3, 5]]
+
+ gt_bboxes_3d = input_dict['gt_bboxes_3d']
+ gt_labels_3d = input_dict['gt_labels_3d']
+ mask = gt_bboxes_3d.in_range_bev(bev_range)
+ gt_bboxes_3d = gt_bboxes_3d[mask]
+ # mask is a torch tensor but gt_labels_3d is still numpy array
+ # using mask to index gt_labels_3d will cause bug when
+ # len(gt_labels_3d) == 1, where mask=1 will be interpreted
+ # as gt_labels_3d[1] and cause out of index error
+ gt_labels_3d = gt_labels_3d[mask.numpy().astype(np.bool)]
+
+ # limit rad to [-pi, pi]
+ gt_bboxes_3d.limit_yaw(offset=0.5, period=2 * np.pi)
+ input_dict['gt_bboxes_3d'] = gt_bboxes_3d
+ input_dict['gt_labels_3d'] = gt_labels_3d
+
+ return input_dict
+
+ def __repr__(self):
+ """str: Return a string that describes the module."""
+ repr_str = self.__class__.__name__
+ repr_str += f'(point_cloud_range={self.pcd_range.tolist()})'
+ return repr_str
+
+
+@PIPELINES.register_module()
+class PointsRangeFilter(object):
+ """Filter points by the range.
+
+ Args:
+ point_cloud_range (list[float]): Point cloud range.
+ """
+
+ def __init__(self, point_cloud_range):
+ self.pcd_range = np.array(point_cloud_range, dtype=np.float32)
+
+ def __call__(self, input_dict):
+ """Call function to filter points by the range.
+
+ Args:
+ input_dict (dict): Result dict from loading pipeline.
+
+ Returns:
+ dict: Results after filtering, 'points', 'pts_instance_mask'
+ and 'pts_semantic_mask' keys are updated in the result dict.
+ """
+ points = input_dict['points']
+ points_mask = points.in_range_3d(self.pcd_range)
+ clean_points = points[points_mask]
+ input_dict['points'] = clean_points
+ points_mask = points_mask.numpy()
+
+ pts_instance_mask = input_dict.get('pts_instance_mask', None)
+ pts_semantic_mask = input_dict.get('pts_semantic_mask', None)
+
+ if pts_instance_mask is not None:
+ input_dict['pts_instance_mask'] = pts_instance_mask[points_mask]
+
+ if pts_semantic_mask is not None:
+ input_dict['pts_semantic_mask'] = pts_semantic_mask[points_mask]
+
+ return input_dict
+
+ def __repr__(self):
+ """str: Return a string that describes the module."""
+ repr_str = self.__class__.__name__
+ repr_str += f'(point_cloud_range={self.pcd_range.tolist()})'
+ return repr_str
+
+
+@PIPELINES.register_module()
+class ObjectNameFilter(object):
+ """Filter GT objects by their names.
+
+ Args:
+ classes (list[str]): List of class names to be kept for training.
+ """
+
+ def __init__(self, classes):
+ self.classes = classes
+ self.labels = list(range(len(self.classes)))
+
+ def __call__(self, input_dict):
+ """Call function to filter objects by their names.
+
+ Args:
+ input_dict (dict): Result dict from loading pipeline.
+
+ Returns:
+ dict: Results after filtering, 'gt_bboxes_3d', 'gt_labels_3d'
+ keys are updated in the result dict.
+ """
+ gt_labels_3d = input_dict['gt_labels_3d']
+ gt_bboxes_mask = np.array([n in self.labels for n in gt_labels_3d],
+ dtype=np.bool_)
+ input_dict['gt_bboxes_3d'] = input_dict['gt_bboxes_3d'][gt_bboxes_mask]
+ input_dict['gt_labels_3d'] = input_dict['gt_labels_3d'][gt_bboxes_mask]
+
+ return input_dict
+
+ def __repr__(self):
+ """str: Return a string that describes the module."""
+ repr_str = self.__class__.__name__
+ repr_str += f'(classes={self.classes})'
+ return repr_str
+
+
+@PIPELINES.register_module()
+class PointSample(object):
+ """Point sample.
+
+ Sampling data to a certain number.
+
+ Args:
+ num_points (int): Number of points to be sampled.
+ sample_range (float, optional): The range where to sample points.
+ If not None, the points with depth larger than `sample_range` are
+ prior to be sampled. Defaults to None.
+ replace (bool, optional): Whether the sampling is with or without
+ replacement. Defaults to False.
+ """
+
+ def __init__(self, num_points, sample_range=None, replace=False):
+ self.num_points = num_points
+ self.sample_range = sample_range
+ self.replace = replace
+
+ def _points_random_sampling(self,
+ points,
+ num_samples,
+ sample_range=None,
+ replace=False,
+ return_choices=False):
+ """Points random sampling.
+
+ Sample points to a certain number.
+
+ Args:
+ points (np.ndarray | :obj:`BasePoints`): 3D Points.
+ num_samples (int): Number of samples to be sampled.
+ sample_range (float, optional): Indicating the range where the
+ points will be sampled. Defaults to None.
+ replace (bool, optional): Sampling with or without replacement.
+ Defaults to None.
+ return_choices (bool, optional): Whether return choice.
+ Defaults to False.
+ Returns:
+ tuple[np.ndarray] | np.ndarray:
+ - points (np.ndarray | :obj:`BasePoints`): 3D Points.
+ - choices (np.ndarray, optional): The generated random samples.
+ """
+ if not replace:
+ replace = (points.shape[0] < num_samples)
+ point_range = range(len(points))
+ if sample_range is not None and not replace:
+ # Only sampling the near points when len(points) >= num_samples
+ dist = np.linalg.norm(points.tensor, axis=1)
+ far_inds = np.where(dist >= sample_range)[0]
+ near_inds = np.where(dist < sample_range)[0]
+ # in case there are too many far points
+ if len(far_inds) > num_samples:
+ far_inds = np.random.choice(
+ far_inds, num_samples, replace=False)
+ point_range = near_inds
+ num_samples -= len(far_inds)
+ choices = np.random.choice(point_range, num_samples, replace=replace)
+ if sample_range is not None and not replace:
+ choices = np.concatenate((far_inds, choices))
+ # Shuffle points after sampling
+ np.random.shuffle(choices)
+ if return_choices:
+ return points[choices], choices
+ else:
+ return points[choices]
+
+ def __call__(self, results):
+ """Call function to sample points to in indoor scenes.
+
+ Args:
+ input_dict (dict): Result dict from loading pipeline.
+ Returns:
+ dict: Results after sampling, 'points', 'pts_instance_mask'
+ and 'pts_semantic_mask' keys are updated in the result dict.
+ """
+ points = results['points']
+ points, choices = self._points_random_sampling(
+ points,
+ self.num_points,
+ self.sample_range,
+ self.replace,
+ return_choices=True)
+ results['points'] = points
+
+ pts_instance_mask = results.get('pts_instance_mask', None)
+ pts_semantic_mask = results.get('pts_semantic_mask', None)
+
+ if pts_instance_mask is not None:
+ pts_instance_mask = pts_instance_mask[choices]
+ results['pts_instance_mask'] = pts_instance_mask
+
+ if pts_semantic_mask is not None:
+ pts_semantic_mask = pts_semantic_mask[choices]
+ results['pts_semantic_mask'] = pts_semantic_mask
+
+ return results
+
+ def __repr__(self):
+ """str: Return a string that describes the module."""
+ repr_str = self.__class__.__name__
+ repr_str += f'(num_points={self.num_points},'
+ repr_str += f' sample_range={self.sample_range},'
+ repr_str += f' replace={self.replace})'
+
+ return repr_str
+
+
+@PIPELINES.register_module()
+class IndoorPointSample(PointSample):
+ """Indoor point sample.
+
+ Sampling data to a certain number.
+ NOTE: IndoorPointSample is deprecated in favor of PointSample
+
+ Args:
+ num_points (int): Number of points to be sampled.
+ """
+
+ def __init__(self, *args, **kwargs):
+ warnings.warn(
+ 'IndoorPointSample is deprecated in favor of PointSample')
+ super(IndoorPointSample, self).__init__(*args, **kwargs)
+
+
+@PIPELINES.register_module()
+class IndoorPatchPointSample(object):
+ r"""Indoor point sample within a patch. Modified from `PointNet++ `_.
+
+ Sampling data to a certain number for semantic segmentation.
+
+ Args:
+ num_points (int): Number of points to be sampled.
+ block_size (float, optional): Size of a block to sample points from.
+ Defaults to 1.5.
+ sample_rate (float, optional): Stride used in sliding patch generation.
+ This parameter is unused in `IndoorPatchPointSample` and thus has
+ been deprecated. We plan to remove it in the future.
+ Defaults to None.
+ ignore_index (int, optional): Label index that won't be used for the
+ segmentation task. This is set in PointSegClassMapping as neg_cls.
+ If not None, will be used as a patch selection criterion.
+ Defaults to None.
+ use_normalized_coord (bool, optional): Whether to use normalized xyz as
+ additional features. Defaults to False.
+ num_try (int, optional): Number of times to try if the patch selected
+ is invalid. Defaults to 10.
+ enlarge_size (float, optional): Enlarge the sampled patch to
+ [-block_size / 2 - enlarge_size, block_size / 2 + enlarge_size] as
+ an augmentation. If None, set it as 0. Defaults to 0.2.
+ min_unique_num (int, optional): Minimum number of unique points
+ the sampled patch should contain. If None, use PointNet++'s method
+ to judge uniqueness. Defaults to None.
+ eps (float, optional): A value added to patch boundary to guarantee
+ points coverage. Defaults to 1e-2.
+
+ Note:
+ This transform should only be used in the training process of point
+ cloud segmentation tasks. For the sliding patch generation and
+ inference process in testing, please refer to the `slide_inference`
+ function of `EncoderDecoder3D` class.
+ """
+
+ def __init__(self,
+ num_points,
+ block_size=1.5,
+ sample_rate=None,
+ ignore_index=None,
+ use_normalized_coord=False,
+ num_try=10,
+ enlarge_size=0.2,
+ min_unique_num=None,
+ eps=1e-2):
+ self.num_points = num_points
+ self.block_size = block_size
+ self.ignore_index = ignore_index
+ self.use_normalized_coord = use_normalized_coord
+ self.num_try = num_try
+ self.enlarge_size = enlarge_size if enlarge_size is not None else 0.0
+ self.min_unique_num = min_unique_num
+ self.eps = eps
+
+ if sample_rate is not None:
+ warnings.warn(
+ "'sample_rate' has been deprecated and will be removed in "
+ 'the future. Please remove them from your code.')
+
+ def _input_generation(self, coords, patch_center, coord_max, attributes,
+ attribute_dims, point_type):
+ """Generating model input.
+
+ Generate input by subtracting patch center and adding additional
+ features. Currently support colors and normalized xyz as features.
+
+ Args:
+ coords (np.ndarray): Sampled 3D Points.
+ patch_center (np.ndarray): Center coordinate of the selected patch.
+ coord_max (np.ndarray): Max coordinate of all 3D Points.
+ attributes (np.ndarray): features of input points.
+ attribute_dims (dict): Dictionary to indicate the meaning of extra
+ dimension.
+ point_type (type): class of input points inherited from BasePoints.
+
+ Returns:
+ :obj:`BasePoints`: The generated input data.
+ """
+ # subtract patch center, the z dimension is not centered
+ centered_coords = coords.copy()
+ centered_coords[:, 0] -= patch_center[0]
+ centered_coords[:, 1] -= patch_center[1]
+
+ if self.use_normalized_coord:
+ normalized_coord = coords / coord_max
+ attributes = np.concatenate([attributes, normalized_coord], axis=1)
+ if attribute_dims is None:
+ attribute_dims = dict()
+ attribute_dims.update(
+ dict(normalized_coord=[
+ attributes.shape[1], attributes.shape[1] +
+ 1, attributes.shape[1] + 2
+ ]))
+
+ points = np.concatenate([centered_coords, attributes], axis=1)
+ points = point_type(
+ points, points_dim=points.shape[1], attribute_dims=attribute_dims)
+
+ return points
+
+ def _patch_points_sampling(self, points, sem_mask):
+ """Patch points sampling.
+
+ First sample a valid patch.
+ Then sample points within that patch to a certain number.
+
+ Args:
+ points (:obj:`BasePoints`): 3D Points.
+ sem_mask (np.ndarray): semantic segmentation mask for input points.
+
+ Returns:
+ tuple[:obj:`BasePoints`, np.ndarray] | :obj:`BasePoints`:
+
+ - points (:obj:`BasePoints`): 3D Points.
+ - choices (np.ndarray): The generated random samples.
+ """
+ coords = points.coord.numpy()
+ attributes = points.tensor[:, 3:].numpy()
+ attribute_dims = points.attribute_dims
+ point_type = type(points)
+
+ coord_max = np.amax(coords, axis=0)
+ coord_min = np.amin(coords, axis=0)
+
+ for _ in range(self.num_try):
+ # random sample a point as patch center
+ cur_center = coords[np.random.choice(coords.shape[0])]
+
+ # boundary of a patch, which would be enlarged by
+ # `self.enlarge_size` as an augmentation
+ cur_max = cur_center + np.array(
+ [self.block_size / 2.0, self.block_size / 2.0, 0.0])
+ cur_min = cur_center - np.array(
+ [self.block_size / 2.0, self.block_size / 2.0, 0.0])
+ cur_max[2] = coord_max[2]
+ cur_min[2] = coord_min[2]
+ cur_choice = np.sum(
+ (coords >= (cur_min - self.enlarge_size)) *
+ (coords <= (cur_max + self.enlarge_size)),
+ axis=1) == 3
+
+ if not cur_choice.any(): # no points in this patch
+ continue
+
+ cur_coords = coords[cur_choice, :]
+ cur_sem_mask = sem_mask[cur_choice]
+ point_idxs = np.where(cur_choice)[0]
+ mask = np.sum(
+ (cur_coords >= (cur_min - self.eps)) * (cur_coords <=
+ (cur_max + self.eps)),
+ axis=1) == 3
+
+ # two criteria for patch sampling, adopted from PointNet++
+ # 1. selected patch should contain enough unique points
+ if self.min_unique_num is None:
+ # use PointNet++'s method as default
+ # [31, 31, 62] are just some big values used to transform
+ # coords from 3d array to 1d and then check their uniqueness
+ # this is used in all the ScanNet code following PointNet++
+ vidx = np.ceil(
+ (cur_coords[mask, :] - cur_min) / (cur_max - cur_min) *
+ np.array([31.0, 31.0, 62.0]))
+ vidx = np.unique(vidx[:, 0] * 31.0 * 62.0 + vidx[:, 1] * 62.0 +
+ vidx[:, 2])
+ flag1 = len(vidx) / 31.0 / 31.0 / 62.0 >= 0.02
+ else:
+ # if `min_unique_num` is provided, directly compare with it
+ flag1 = mask.sum() >= self.min_unique_num
+
+ # 2. selected patch should contain enough annotated points
+ if self.ignore_index is None:
+ flag2 = True
+ else:
+ flag2 = np.sum(cur_sem_mask != self.ignore_index) / \
+ len(cur_sem_mask) >= 0.7
+
+ if flag1 and flag2:
+ break
+
+ # sample idx to `self.num_points`
+ if point_idxs.size >= self.num_points:
+ # no duplicate in sub-sampling
+ choices = np.random.choice(
+ point_idxs, self.num_points, replace=False)
+ else:
+ # do not use random choice here to avoid some points not counted
+ dup = np.random.choice(point_idxs.size,
+ self.num_points - point_idxs.size)
+ idx_dup = np.concatenate(
+ [np.arange(point_idxs.size),
+ np.array(dup)], 0)
+ choices = point_idxs[idx_dup]
+
+ # construct model input
+ points = self._input_generation(coords[choices], cur_center, coord_max,
+ attributes[choices], attribute_dims,
+ point_type)
+
+ return points, choices
+
+ def __call__(self, results):
+ """Call function to sample points to in indoor scenes.
+
+ Args:
+ input_dict (dict): Result dict from loading pipeline.
+
+ Returns:
+ dict: Results after sampling, 'points', 'pts_instance_mask'
+ and 'pts_semantic_mask' keys are updated in the result dict.
+ """
+ points = results['points']
+
+ assert 'pts_semantic_mask' in results.keys(), \
+ 'semantic mask should be provided in training and evaluation'
+ pts_semantic_mask = results['pts_semantic_mask']
+
+ points, choices = self._patch_points_sampling(points,
+ pts_semantic_mask)
+
+ results['points'] = points
+ results['pts_semantic_mask'] = pts_semantic_mask[choices]
+ pts_instance_mask = results.get('pts_instance_mask', None)
+ if pts_instance_mask is not None:
+ results['pts_instance_mask'] = pts_instance_mask[choices]
+
+ return results
+
+ def __repr__(self):
+ """str: Return a string that describes the module."""
+ repr_str = self.__class__.__name__
+ repr_str += f'(num_points={self.num_points},'
+ repr_str += f' block_size={self.block_size},'
+ repr_str += f' ignore_index={self.ignore_index},'
+ repr_str += f' use_normalized_coord={self.use_normalized_coord},'
+ repr_str += f' num_try={self.num_try},'
+ repr_str += f' enlarge_size={self.enlarge_size},'
+ repr_str += f' min_unique_num={self.min_unique_num},'
+ repr_str += f' eps={self.eps})'
+ return repr_str
+
+
+@PIPELINES.register_module()
+class BackgroundPointsFilter(object):
+ """Filter background points near the bounding box.
+
+ Args:
+ bbox_enlarge_range (tuple[float], float): Bbox enlarge range.
+ """
+
+ def __init__(self, bbox_enlarge_range):
+ assert (is_tuple_of(bbox_enlarge_range, float)
+ and len(bbox_enlarge_range) == 3) \
+ or isinstance(bbox_enlarge_range, float), \
+ f'Invalid arguments bbox_enlarge_range {bbox_enlarge_range}'
+
+ if isinstance(bbox_enlarge_range, float):
+ bbox_enlarge_range = [bbox_enlarge_range] * 3
+ self.bbox_enlarge_range = np.array(
+ bbox_enlarge_range, dtype=np.float32)[np.newaxis, :]
+
+ def __call__(self, input_dict):
+ """Call function to filter points by the range.
+
+ Args:
+ input_dict (dict): Result dict from loading pipeline.
+
+ Returns:
+ dict: Results after filtering, 'points', 'pts_instance_mask'
+ and 'pts_semantic_mask' keys are updated in the result dict.
+ """
+ points = input_dict['points']
+ gt_bboxes_3d = input_dict['gt_bboxes_3d']
+
+ # avoid groundtruth being modified
+ gt_bboxes_3d_np = gt_bboxes_3d.tensor.clone().numpy()
+ gt_bboxes_3d_np[:, :3] = gt_bboxes_3d.gravity_center.clone().numpy()
+
+ enlarged_gt_bboxes_3d = gt_bboxes_3d_np.copy()
+ enlarged_gt_bboxes_3d[:, 3:6] += self.bbox_enlarge_range
+ points_numpy = points.tensor.clone().numpy()
+ foreground_masks = box_np_ops.points_in_rbbox(
+ points_numpy, gt_bboxes_3d_np, origin=(0.5, 0.5, 0.5))
+ enlarge_foreground_masks = box_np_ops.points_in_rbbox(
+ points_numpy, enlarged_gt_bboxes_3d, origin=(0.5, 0.5, 0.5))
+ foreground_masks = foreground_masks.max(1)
+ enlarge_foreground_masks = enlarge_foreground_masks.max(1)
+ valid_masks = ~np.logical_and(~foreground_masks,
+ enlarge_foreground_masks)
+
+ input_dict['points'] = points[valid_masks]
+ pts_instance_mask = input_dict.get('pts_instance_mask', None)
+ if pts_instance_mask is not None:
+ input_dict['pts_instance_mask'] = pts_instance_mask[valid_masks]
+
+ pts_semantic_mask = input_dict.get('pts_semantic_mask', None)
+ if pts_semantic_mask is not None:
+ input_dict['pts_semantic_mask'] = pts_semantic_mask[valid_masks]
+ return input_dict
+
+ def __repr__(self):
+ """str: Return a string that describes the module."""
+ repr_str = self.__class__.__name__
+ repr_str += f'(bbox_enlarge_range={self.bbox_enlarge_range.tolist()})'
+ return repr_str
+
+
+@PIPELINES.register_module()
+class VoxelBasedPointSampler(object):
+ """Voxel based point sampler.
+
+ Apply voxel sampling to multiple sweep points.
+
+ Args:
+ cur_sweep_cfg (dict): Config for sampling current points.
+ prev_sweep_cfg (dict): Config for sampling previous points.
+ time_dim (int): Index that indicate the time dimension
+ for input points.
+ """
+
+ def __init__(self, cur_sweep_cfg, prev_sweep_cfg=None, time_dim=3):
+ self.cur_voxel_generator = VoxelGenerator(**cur_sweep_cfg)
+ self.cur_voxel_num = self.cur_voxel_generator._max_voxels
+ self.time_dim = time_dim
+ if prev_sweep_cfg is not None:
+ assert prev_sweep_cfg['max_num_points'] == \
+ cur_sweep_cfg['max_num_points']
+ self.prev_voxel_generator = VoxelGenerator(**prev_sweep_cfg)
+ self.prev_voxel_num = self.prev_voxel_generator._max_voxels
+ else:
+ self.prev_voxel_generator = None
+ self.prev_voxel_num = 0
+
+ def _sample_points(self, points, sampler, point_dim):
+ """Sample points for each points subset.
+
+ Args:
+ points (np.ndarray): Points subset to be sampled.
+ sampler (VoxelGenerator): Voxel based sampler for
+ each points subset.
+ point_dim (int): The dimension of each points
+
+ Returns:
+ np.ndarray: Sampled points.
+ """
+ voxels, coors, num_points_per_voxel = sampler.generate(points)
+ if voxels.shape[0] < sampler._max_voxels:
+ padding_points = np.zeros([
+ sampler._max_voxels - voxels.shape[0], sampler._max_num_points,
+ point_dim
+ ],
+ dtype=points.dtype)
+ padding_points[:] = voxels[0]
+ sample_points = np.concatenate([voxels, padding_points], axis=0)
+ else:
+ sample_points = voxels
+
+ return sample_points
+
+ def __call__(self, results):
+ """Call function to sample points from multiple sweeps.
+
+ Args:
+ input_dict (dict): Result dict from loading pipeline.
+
+ Returns:
+ dict: Results after sampling, 'points', 'pts_instance_mask'
+ and 'pts_semantic_mask' keys are updated in the result dict.
+ """
+ points = results['points']
+ original_dim = points.shape[1]
+
+ # TODO: process instance and semantic mask while _max_num_points
+ # is larger than 1
+ # Extend points with seg and mask fields
+ map_fields2dim = []
+ start_dim = original_dim
+ points_numpy = points.tensor.numpy()
+ extra_channel = [points_numpy]
+ for idx, key in enumerate(results['pts_mask_fields']):
+ map_fields2dim.append((key, idx + start_dim))
+ extra_channel.append(results[key][..., None])
+
+ start_dim += len(results['pts_mask_fields'])
+ for idx, key in enumerate(results['pts_seg_fields']):
+ map_fields2dim.append((key, idx + start_dim))
+ extra_channel.append(results[key][..., None])
+
+ points_numpy = np.concatenate(extra_channel, axis=-1)
+
+ # Split points into two part, current sweep points and
+ # previous sweeps points.
+ # TODO: support different sampling methods for next sweeps points
+ # and previous sweeps points.
+ cur_points_flag = (points_numpy[:, self.time_dim] == 0)
+ cur_sweep_points = points_numpy[cur_points_flag]
+ prev_sweeps_points = points_numpy[~cur_points_flag]
+ if prev_sweeps_points.shape[0] == 0:
+ prev_sweeps_points = cur_sweep_points
+
+ # Shuffle points before sampling
+ np.random.shuffle(cur_sweep_points)
+ np.random.shuffle(prev_sweeps_points)
+
+ cur_sweep_points = self._sample_points(cur_sweep_points,
+ self.cur_voxel_generator,
+ points_numpy.shape[1])
+ if self.prev_voxel_generator is not None:
+ prev_sweeps_points = self._sample_points(prev_sweeps_points,
+ self.prev_voxel_generator,
+ points_numpy.shape[1])
+
+ points_numpy = np.concatenate(
+ [cur_sweep_points, prev_sweeps_points], 0)
+ else:
+ points_numpy = cur_sweep_points
+
+ if self.cur_voxel_generator._max_num_points == 1:
+ points_numpy = points_numpy.squeeze(1)
+ results['points'] = points.new_point(points_numpy[..., :original_dim])
+
+ # Restore the corresponding seg and mask fields
+ for key, dim_index in map_fields2dim:
+ results[key] = points_numpy[..., dim_index]
+
+ return results
+
+ def __repr__(self):
+ """str: Return a string that describes the module."""
+
+ def _auto_indent(repr_str, indent):
+ repr_str = repr_str.split('\n')
+ repr_str = [' ' * indent + t + '\n' for t in repr_str]
+ repr_str = ''.join(repr_str)[:-1]
+ return repr_str
+
+ repr_str = self.__class__.__name__
+ indent = 4
+ repr_str += '(\n'
+ repr_str += ' ' * indent + f'num_cur_sweep={self.cur_voxel_num},\n'
+ repr_str += ' ' * indent + f'num_prev_sweep={self.prev_voxel_num},\n'
+ repr_str += ' ' * indent + f'time_dim={self.time_dim},\n'
+ repr_str += ' ' * indent + 'cur_voxel_generator=\n'
+ repr_str += f'{_auto_indent(repr(self.cur_voxel_generator), 8)},\n'
+ repr_str += ' ' * indent + 'prev_voxel_generator=\n'
+ repr_str += f'{_auto_indent(repr(self.prev_voxel_generator), 8)})'
+ return repr_str
+
+
+@PIPELINES.register_module()
+class AffineResize(object):
+ """Get the affine transform matrices to the target size.
+
+ Different from :class:`RandomAffine` in MMDetection, this class can
+ calculate the affine transform matrices while resizing the input image
+ to a fixed size. The affine transform matrices include: 1) matrix
+ transforming original image to the network input image size. 2) matrix
+ transforming original image to the network output feature map size.
+
+ Args:
+ img_scale (tuple): Images scales for resizing.
+ down_ratio (int): The down ratio of feature map.
+ Actually the arg should be >= 1.
+ bbox_clip_border (bool, optional): Whether clip the objects
+ outside the border of the image. Defaults to True.
+ """
+
+ def __init__(self, img_scale, down_ratio, bbox_clip_border=True):
+
+ self.img_scale = img_scale
+ self.down_ratio = down_ratio
+ self.bbox_clip_border = bbox_clip_border
+
+ def __call__(self, results):
+ """Call function to do affine transform to input image and labels.
+
+ Args:
+ results (dict): Result dict from loading pipeline.
+
+ Returns:
+ dict: Results after affine resize, 'affine_aug', 'trans_mat'
+ keys are added in the result dict.
+ """
+ # The results have gone through RandomShiftScale before AffineResize
+ if 'center' not in results:
+ img = results['img']
+ height, width = img.shape[:2]
+ center = np.array([width / 2, height / 2], dtype=np.float32)
+ size = np.array([width, height], dtype=np.float32)
+ results['affine_aug'] = False
+ else:
+ # The results did not go through RandomShiftScale before
+ # AffineResize
+ img = results['img']
+ center = results['center']
+ size = results['size']
+
+ trans_affine = self._get_transform_matrix(center, size, self.img_scale)
+
+ img = cv2.warpAffine(img, trans_affine[:2, :], self.img_scale)
+
+ if isinstance(self.down_ratio, tuple):
+ trans_mat = [
+ self._get_transform_matrix(
+ center, size,
+ (self.img_scale[0] // ratio, self.img_scale[1] // ratio))
+ for ratio in self.down_ratio
+ ] # (3, 3)
+ else:
+ trans_mat = self._get_transform_matrix(
+ center, size, (self.img_scale[0] // self.down_ratio,
+ self.img_scale[1] // self.down_ratio))
+
+ results['img'] = img
+ results['img_shape'] = img.shape
+ results['pad_shape'] = img.shape
+ results['trans_mat'] = trans_mat
+
+ self._affine_bboxes(results, trans_affine)
+
+ if 'centers2d' in results:
+ centers2d = self._affine_transform(results['centers2d'],
+ trans_affine)
+ valid_index = (centers2d[:, 0] >
+ 0) & (centers2d[:, 0] <
+ self.img_scale[0]) & (centers2d[:, 1] > 0) & (
+ centers2d[:, 1] < self.img_scale[1])
+ results['centers2d'] = centers2d[valid_index]
+
+ for key in results.get('bbox_fields', []):
+ if key in ['gt_bboxes']:
+ results[key] = results[key][valid_index]
+ if 'gt_labels' in results:
+ results['gt_labels'] = results['gt_labels'][
+ valid_index]
+ if 'gt_masks' in results:
+ raise NotImplementedError(
+ 'AffineResize only supports bbox.')
+
+ for key in results.get('bbox3d_fields', []):
+ if key in ['gt_bboxes_3d']:
+ results[key].tensor = results[key].tensor[valid_index]
+ if 'gt_labels_3d' in results:
+ results['gt_labels_3d'] = results['gt_labels_3d'][
+ valid_index]
+
+ results['depths'] = results['depths'][valid_index]
+
+ return results
+
+ def _affine_bboxes(self, results, matrix):
+ """Affine transform bboxes to input image.
+
+ Args:
+ results (dict): Result dict from loading pipeline.
+ matrix (np.ndarray): Matrix transforming original
+ image to the network input image size.
+ shape: (3, 3)
+ """
+
+ for key in results.get('bbox_fields', []):
+ bboxes = results[key]
+ bboxes[:, :2] = self._affine_transform(bboxes[:, :2], matrix)
+ bboxes[:, 2:] = self._affine_transform(bboxes[:, 2:], matrix)
+ if self.bbox_clip_border:
+ bboxes[:,
+ [0, 2]] = bboxes[:,
+ [0, 2]].clip(0, self.img_scale[0] - 1)
+ bboxes[:,
+ [1, 3]] = bboxes[:,
+ [1, 3]].clip(0, self.img_scale[1] - 1)
+ results[key] = bboxes
+
+ def _affine_transform(self, points, matrix):
+ """Affine transform bbox points to input image.
+
+ Args:
+ points (np.ndarray): Points to be transformed.
+ shape: (N, 2)
+ matrix (np.ndarray): Affine transform matrix.
+ shape: (3, 3)
+
+ Returns:
+ np.ndarray: Transformed points.
+ """
+ num_points = points.shape[0]
+ hom_points_2d = np.concatenate((points, np.ones((num_points, 1))),
+ axis=1)
+ hom_points_2d = hom_points_2d.T
+ affined_points = np.matmul(matrix, hom_points_2d).T
+ return affined_points[:, :2]
+
+ def _get_transform_matrix(self, center, scale, output_scale):
+ """Get affine transform matrix.
+
+ Args:
+ center (tuple): Center of current image.
+ scale (tuple): Scale of current image.
+ output_scale (tuple[float]): The transform target image scales.
+
+ Returns:
+ np.ndarray: Affine transform matrix.
+ """
+ # TODO: further add rot and shift here.
+ src_w = scale[0]
+ dst_w = output_scale[0]
+ dst_h = output_scale[1]
+
+ src_dir = np.array([0, src_w * -0.5])
+ dst_dir = np.array([0, dst_w * -0.5])
+
+ src = np.zeros((3, 2), dtype=np.float32)
+ dst = np.zeros((3, 2), dtype=np.float32)
+ src[0, :] = center
+ src[1, :] = center + src_dir
+ dst[0, :] = np.array([dst_w * 0.5, dst_h * 0.5])
+ dst[1, :] = np.array([dst_w * 0.5, dst_h * 0.5]) + dst_dir
+
+ src[2, :] = self._get_ref_point(src[0, :], src[1, :])
+ dst[2, :] = self._get_ref_point(dst[0, :], dst[1, :])
+
+ get_matrix = cv2.getAffineTransform(src, dst)
+
+ matrix = np.concatenate((get_matrix, [[0., 0., 1.]]))
+
+ return matrix.astype(np.float32)
+
+ def _get_ref_point(self, ref_point1, ref_point2):
+ """Get reference point to calculate affine transform matrix.
+
+ While using opencv to calculate the affine matrix, we need at least
+ three corresponding points separately on original image and target
+ image. Here we use two points to get the the third reference point.
+ """
+ d = ref_point1 - ref_point2
+ ref_point3 = ref_point2 + np.array([-d[1], d[0]])
+ return ref_point3
+
+ def __repr__(self):
+ repr_str = self.__class__.__name__
+ repr_str += f'(img_scale={self.img_scale}, '
+ repr_str += f'down_ratio={self.down_ratio}) '
+ return repr_str
+
+
+@PIPELINES.register_module()
+class RandomShiftScale(object):
+ """Random shift scale.
+
+ Different from the normal shift and scale function, it doesn't
+ directly shift or scale image. It can record the shift and scale
+ infos into loading pipelines. It's designed to be used with
+ AffineResize together.
+
+ Args:
+ shift_scale (tuple[float]): Shift and scale range.
+ aug_prob (float): The shifting and scaling probability.
+ """
+
+ def __init__(self, shift_scale, aug_prob):
+
+ self.shift_scale = shift_scale
+ self.aug_prob = aug_prob
+
+ def __call__(self, results):
+ """Call function to record random shift and scale infos.
+
+ Args:
+ results (dict): Result dict from loading pipeline.
+
+ Returns:
+ dict: Results after random shift and scale, 'center', 'size'
+ and 'affine_aug' keys are added in the result dict.
+ """
+ img = results['img']
+
+ height, width = img.shape[:2]
+
+ center = np.array([width / 2, height / 2], dtype=np.float32)
+ size = np.array([width, height], dtype=np.float32)
+
+ if random.random() < self.aug_prob:
+ shift, scale = self.shift_scale[0], self.shift_scale[1]
+ shift_ranges = np.arange(-shift, shift + 0.1, 0.1)
+ center[0] += size[0] * random.choice(shift_ranges)
+ center[1] += size[1] * random.choice(shift_ranges)
+ scale_ranges = np.arange(1 - scale, 1 + scale + 0.1, 0.1)
+ size *= random.choice(scale_ranges)
+ results['affine_aug'] = True
+ else:
+ results['affine_aug'] = False
+
+ results['center'] = center
+ results['size'] = size
+
+ return results
+
+ def __repr__(self):
+ repr_str = self.__class__.__name__
+ repr_str += f'(shift_scale={self.shift_scale}, '
+ repr_str += f'aug_prob={self.aug_prob}) '
+ return repr_str
+
+
+from scipy.spatial.distance import cdist
+
+
+@PIPELINES.register_module()
+class AddInnerCenter:
+ def __init__(self, n_samples):
+ self.n_samples = n_samples
+
+ def __call__(self, results):
+ inner_centers = []
+ box = results['gt_bboxes_3d']
+ for i in range(len(box)):
+ mask = results['pts_instance_mask'] == i
+ assert mask.sum() > 0
+ points = results['points'].tensor.numpy()[mask, :3]
+ points = np.random.permutation(points)[:self.n_samples]
+ distances = cdist(points, points)
+ best = distances.sum(axis=1).argmin()
+ # for mass center use:
+ # = results['points'].tensor.numpy()[mask, :3].mean(axis=0)
+ cx, cy, cz = points[best]
+ box = results['gt_bboxes_3d']
+ x, y, z = box.gravity_center.numpy()[i]
+ w, l, h = box.tensor.numpy()[i, 3:6]
+ assert x - w / 2 - .01 < cx < x + w / 2 + .01
+ assert y - l / 2 - .01 < cy < y + l / 2 + .01
+ assert z - h / 2 - .01 < cz < z + h / 2 + .01
+ inner_centers.append([cx, cy, cz])
+ results['inner_center'] = np.array(inner_centers).astype(np.float32)
+ return results
diff --git a/mmdet3d/datasets/s3dis_dataset.py b/mmdet3d/datasets/s3dis_dataset.py
new file mode 100644
index 0000000..883a52d
--- /dev/null
+++ b/mmdet3d/datasets/s3dis_dataset.py
@@ -0,0 +1,568 @@
+# Copyright (c) OpenMMLab. All rights reserved.
+from os import path as osp
+
+import numpy as np
+
+from mmdet3d.core import show_seg_result, instance_seg_eval_v2
+from mmdet3d.core.bbox import DepthInstance3DBoxes
+from mmseg.datasets import DATASETS as SEG_DATASETS
+from .builder import DATASETS
+from .custom_3d import Custom3DDataset
+from .custom_3d_seg import Custom3DSegDataset
+from .pipelines import Compose
+
+
+@DATASETS.register_module()
+class S3DISDataset(Custom3DDataset):
+ r"""S3DIS Dataset for Detection Task.
+
+ This class is the inner dataset for S3DIS. Since S3DIS has 6 areas, we
+ often train on 5 of them and test on the remaining one. The one for
+ test is Area_5 as suggested in `GSDN `_.
+ To concatenate 5 areas during training
+ `mmdet.datasets.dataset_wrappers.ConcatDataset` should be used.
+
+ Args:
+ data_root (str): Path of dataset root.
+ ann_file (str): Path of annotation file.
+ pipeline (list[dict], optional): Pipeline used for data processing.
+ Defaults to None.
+ classes (tuple[str], optional): Classes used in the dataset.
+ Defaults to None.
+ modality (dict, optional): Modality to specify the sensor data used
+ as input. Defaults to None.
+ box_type_3d (str, optional): Type of 3D box of this dataset.
+ Based on the `box_type_3d`, the dataset will encapsulate the box
+ to its original format then converted them to `box_type_3d`.
+ Defaults to 'Depth' in this dataset. Available options includes
+
+ - 'LiDAR': Box in LiDAR coordinates.
+ - 'Depth': Box in depth coordinates, usually for indoor dataset.
+ - 'Camera': Box in camera coordinates.
+ filter_empty_gt (bool, optional): Whether to filter empty GT.
+ Defaults to True.
+ test_mode (bool, optional): Whether the dataset is in test mode.
+ Defaults to False.
+ """
+ CLASSES = ('table', 'chair', 'sofa', 'bookcase', 'board')
+
+ def __init__(self,
+ data_root,
+ ann_file,
+ pipeline=None,
+ classes=None,
+ modality=None,
+ box_type_3d='Depth',
+ filter_empty_gt=True,
+ test_mode=False,
+ *kwargs):
+ super().__init__(
+ data_root=data_root,
+ ann_file=ann_file,
+ pipeline=pipeline,
+ classes=classes,
+ modality=modality,
+ box_type_3d=box_type_3d,
+ filter_empty_gt=filter_empty_gt,
+ test_mode=test_mode,
+ *kwargs)
+
+ def get_ann_info(self, index):
+ """Get annotation info according to the given index.
+
+ Args:
+ index (int): Index of the annotation data to get.
+
+ Returns:
+ dict: annotation information consists of the following keys:
+
+ - gt_bboxes_3d (:obj:`DepthInstance3DBoxes`):
+ 3D ground truth bboxes
+ - gt_labels_3d (np.ndarray): Labels of ground truths.
+ - pts_instance_mask_path (str): Path of instance masks.
+ - pts_semantic_mask_path (str): Path of semantic masks.
+ """
+ # Use index to get the annos, thus the evalhook could also use this api
+ info = self.data_infos[index]
+ if info['annos']['gt_num'] != 0:
+ gt_bboxes_3d = info['annos']['gt_boxes_upright_depth'].astype(
+ np.float32) # k, 6
+ gt_labels_3d = info['annos']['class'].astype(np.int64)
+ else:
+ gt_bboxes_3d = np.zeros((0, 6), dtype=np.float32)
+ gt_labels_3d = np.zeros((0, ), dtype=np.int64)
+
+ # to target box structure
+ gt_bboxes_3d = DepthInstance3DBoxes(
+ gt_bboxes_3d,
+ box_dim=gt_bboxes_3d.shape[-1],
+ with_yaw=False,
+ origin=(0.5, 0.5, 0.5)).convert_to(self.box_mode_3d)
+
+ pts_instance_mask_path = osp.join(self.data_root,
+ info['pts_instance_mask_path'])
+ pts_semantic_mask_path = osp.join(self.data_root,
+ info['pts_semantic_mask_path'])
+
+ anns_results = dict(
+ gt_bboxes_3d=gt_bboxes_3d,
+ gt_labels_3d=gt_labels_3d,
+ pts_instance_mask_path=pts_instance_mask_path,
+ pts_semantic_mask_path=pts_semantic_mask_path)
+ return anns_results
+
+ def get_data_info(self, index):
+ """Get data info according to the given index.
+
+ Args:
+ index (int): Index of the sample data to get.
+
+ Returns:
+ dict: Data information that will be passed to the data
+ preprocessing pipelines. It includes the following keys:
+
+ - pts_filename (str): Filename of point clouds.
+ - file_name (str): Filename of point clouds.
+ - ann_info (dict): Annotation info.
+ """
+ info = self.data_infos[index]
+ pts_filename = osp.join(self.data_root, info['pts_path'])
+ input_dict = dict(pts_filename=pts_filename)
+
+ if not self.test_mode:
+ annos = self.get_ann_info(index)
+ input_dict['ann_info'] = annos
+ if self.filter_empty_gt and ~(annos['gt_labels_3d'] != -1).any():
+ return None
+ return input_dict
+
+ def _build_default_pipeline(self):
+ """Build the default pipeline for this dataset."""
+ pipeline = [
+ dict(
+ type='LoadPointsFromFile',
+ coord_type='DEPTH',
+ shift_height=False,
+ load_dim=6,
+ use_dim=[0, 1, 2, 3, 4, 5]),
+ dict(
+ type='DefaultFormatBundle3D',
+ class_names=self.CLASSES,
+ with_label=False),
+ dict(type='Collect3D', keys=['points'])
+ ]
+ return Compose(pipeline)
+
+
+class _S3DISSegDataset(Custom3DSegDataset):
+ r"""S3DIS Dataset for Semantic Segmentation Task.
+
+ This class is the inner dataset for S3DIS. Since S3DIS has 6 areas, we
+ often train on 5 of them and test on the remaining one.
+ However, there is not a fixed train-test split of S3DIS. People often test
+ on Area_5 as suggested by `SEGCloud `_.
+ But many papers also report the average results of 6-fold cross validation
+ over the 6 areas (e.g. `DGCNN `_).
+ Therefore, we use an inner dataset for one area, and further use a dataset
+ wrapper to concat all the provided data in different areas.
+
+ Args:
+ data_root (str): Path of dataset root.
+ ann_file (str): Path of annotation file.
+ pipeline (list[dict], optional): Pipeline used for data processing.
+ Defaults to None.
+ classes (tuple[str], optional): Classes used in the dataset.
+ Defaults to None.
+ palette (list[list[int]], optional): The palette of segmentation map.
+ Defaults to None.
+ modality (dict, optional): Modality to specify the sensor data used
+ as input. Defaults to None.
+ test_mode (bool, optional): Whether the dataset is in test mode.
+ Defaults to False.
+ ignore_index (int, optional): The label index to be ignored, e.g.
+ unannotated points. If None is given, set to len(self.CLASSES).
+ Defaults to None.
+ scene_idxs (np.ndarray | str, optional): Precomputed index to load
+ data. For scenes with many points, we may sample it several times.
+ Defaults to None.
+ """
+ CLASSES = ('ceiling', 'floor', 'wall', 'beam', 'column', 'window', 'door',
+ 'table', 'chair', 'sofa', 'bookcase', 'board', 'clutter')
+
+ VALID_CLASS_IDS = tuple(range(13))
+
+ ALL_CLASS_IDS = tuple(range(14)) # possibly with 'stair' class
+
+ PALETTE = [[0, 255, 0], [0, 0, 255], [0, 255, 255], [255, 255, 0],
+ [255, 0, 255], [100, 100, 255], [200, 200, 100],
+ [170, 120, 200], [255, 0, 0], [200, 100, 100], [10, 200, 100],
+ [200, 200, 200], [50, 50, 50]]
+
+ def __init__(self,
+ data_root,
+ ann_file,
+ pipeline=None,
+ classes=None,
+ palette=None,
+ modality=None,
+ test_mode=False,
+ ignore_index=None,
+ scene_idxs=None,
+ **kwargs):
+
+ super().__init__(
+ data_root=data_root,
+ ann_file=ann_file,
+ pipeline=pipeline,
+ classes=classes,
+ palette=palette,
+ modality=modality,
+ test_mode=test_mode,
+ ignore_index=ignore_index,
+ scene_idxs=scene_idxs,
+ **kwargs)
+
+ def get_ann_info(self, index):
+ """Get annotation info according to the given index.
+
+ Args:
+ index (int): Index of the annotation data to get.
+
+ Returns:
+ dict: annotation information consists of the following keys:
+
+ - pts_semantic_mask_path (str): Path of semantic masks.
+ """
+ # Use index to get the annos, thus the evalhook could also use this api
+ info = self.data_infos[index]
+
+ pts_semantic_mask_path = osp.join(self.data_root,
+ info['pts_semantic_mask_path'])
+
+ anns_results = dict(pts_semantic_mask_path=pts_semantic_mask_path)
+ return anns_results
+
+ def _build_default_pipeline(self):
+ """Build the default pipeline for this dataset."""
+ pipeline = [
+ dict(
+ type='LoadPointsFromFile',
+ coord_type='DEPTH',
+ shift_height=False,
+ use_color=True,
+ load_dim=6,
+ use_dim=[0, 1, 2, 3, 4, 5]),
+ dict(
+ type='LoadAnnotations3D',
+ with_bbox_3d=False,
+ with_label_3d=False,
+ with_mask_3d=False,
+ with_seg_3d=True),
+ dict(
+ type='PointSegClassMapping',
+ valid_cat_ids=self.VALID_CLASS_IDS,
+ max_cat_id=np.max(self.ALL_CLASS_IDS)),
+ dict(
+ type='DefaultFormatBundle3D',
+ with_label=False,
+ class_names=self.CLASSES),
+ dict(type='Collect3D', keys=['points', 'pts_semantic_mask'])
+ ]
+ return Compose(pipeline)
+
+ def show(self, results, out_dir, show=True, pipeline=None):
+ """Results visualization.
+
+ Args:
+ results (list[dict]): List of bounding boxes results.
+ out_dir (str): Output directory of visualization result.
+ show (bool): Visualize the results online.
+ pipeline (list[dict], optional): raw data loading for showing.
+ Default: None.
+ """
+ assert out_dir is not None, 'Expect out_dir, got none.'
+ pipeline = self._get_pipeline(pipeline)
+ for i, result in enumerate(results):
+ data_info = self.data_infos[i]
+ pts_path = data_info['pts_path']
+ file_name = osp.split(pts_path)[-1].split('.')[0]
+ points, gt_sem_mask = self._extract_data(
+ i, pipeline, ['points', 'pts_semantic_mask'], load_annos=True)
+ points = points.numpy()
+ pred_sem_mask = result['semantic_mask'].numpy()
+ show_seg_result(points, gt_sem_mask,
+ pred_sem_mask, out_dir, file_name,
+ np.array(self.PALETTE), self.ignore_index, show)
+
+ def get_scene_idxs(self, scene_idxs):
+ """Compute scene_idxs for data sampling.
+
+ We sample more times for scenes with more points.
+ """
+ # when testing, we load one whole scene every time
+ if not self.test_mode and scene_idxs is None:
+ raise NotImplementedError(
+ 'please provide re-sampled scene indexes for training')
+
+ return super().get_scene_idxs(scene_idxs)
+
+
+@DATASETS.register_module()
+@SEG_DATASETS.register_module()
+class S3DISSegDataset(_S3DISSegDataset):
+ r"""S3DIS Dataset for Semantic Segmentation Task.
+
+ This class serves as the API for experiments on the S3DIS Dataset.
+ It wraps the provided datasets of different areas.
+ We don't use `mmdet.datasets.dataset_wrappers.ConcatDataset` because we
+ need to concat the `scene_idxs` of different areas.
+
+ Please refer to the `google form `_ for
+ data downloading.
+
+ Args:
+ data_root (str): Path of dataset root.
+ ann_files (list[str]): Path of several annotation files.
+ pipeline (list[dict], optional): Pipeline used for data processing.
+ Defaults to None.
+ classes (tuple[str], optional): Classes used in the dataset.
+ Defaults to None.
+ palette (list[list[int]], optional): The palette of segmentation map.
+ Defaults to None.
+ modality (dict, optional): Modality to specify the sensor data used
+ as input. Defaults to None.
+ test_mode (bool, optional): Whether the dataset is in test mode.
+ Defaults to False.
+ ignore_index (int, optional): The label index to be ignored, e.g.
+ unannotated points. If None is given, set to len(self.CLASSES).
+ Defaults to None.
+ scene_idxs (list[np.ndarray] | list[str], optional): Precomputed index
+ to load data. For scenes with many points, we may sample it several
+ times. Defaults to None.
+ """
+
+ def __init__(self,
+ data_root,
+ ann_files,
+ pipeline=None,
+ classes=None,
+ palette=None,
+ modality=None,
+ test_mode=False,
+ ignore_index=None,
+ scene_idxs=None,
+ **kwargs):
+
+ # make sure that ann_files and scene_idxs have same length
+ ann_files = self._check_ann_files(ann_files)
+ scene_idxs = self._check_scene_idxs(scene_idxs, len(ann_files))
+
+ # initialize some attributes as datasets[0]
+ super().__init__(
+ data_root=data_root,
+ ann_file=ann_files[0],
+ pipeline=pipeline,
+ classes=classes,
+ palette=palette,
+ modality=modality,
+ test_mode=test_mode,
+ ignore_index=ignore_index,
+ scene_idxs=scene_idxs[0],
+ **kwargs)
+
+ datasets = [
+ _S3DISSegDataset(
+ data_root=data_root,
+ ann_file=ann_files[i],
+ pipeline=pipeline,
+ classes=classes,
+ palette=palette,
+ modality=modality,
+ test_mode=test_mode,
+ ignore_index=ignore_index,
+ scene_idxs=scene_idxs[i],
+ **kwargs) for i in range(len(ann_files))
+ ]
+
+ # data_infos and scene_idxs need to be concat
+ self.concat_data_infos([dst.data_infos for dst in datasets])
+ self.concat_scene_idxs([dst.scene_idxs for dst in datasets])
+
+ # set group flag for the sampler
+ if not self.test_mode:
+ self._set_group_flag()
+
+ def concat_data_infos(self, data_infos):
+ """Concat data_infos from several datasets to form self.data_infos.
+
+ Args:
+ data_infos (list[list[dict]])
+ """
+ self.data_infos = [
+ info for one_data_infos in data_infos for info in one_data_infos
+ ]
+
+ def concat_scene_idxs(self, scene_idxs):
+ """Concat scene_idxs from several datasets to form self.scene_idxs.
+
+ Needs to manually add offset to scene_idxs[1, 2, ...].
+
+ Args:
+ scene_idxs (list[np.ndarray])
+ """
+ self.scene_idxs = np.array([], dtype=np.int32)
+ offset = 0
+ for one_scene_idxs in scene_idxs:
+ self.scene_idxs = np.concatenate(
+ [self.scene_idxs, one_scene_idxs + offset]).astype(np.int32)
+ offset = np.unique(self.scene_idxs).max() + 1
+
+ @staticmethod
+ def _duplicate_to_list(x, num):
+ """Repeat x `num` times to form a list."""
+ return [x for _ in range(num)]
+
+ def _check_ann_files(self, ann_file):
+ """Make ann_files as list/tuple."""
+ # ann_file could be str
+ if not isinstance(ann_file, (list, tuple)):
+ ann_file = self._duplicate_to_list(ann_file, 1)
+ return ann_file
+
+ def _check_scene_idxs(self, scene_idx, num):
+ """Make scene_idxs as list/tuple."""
+ if scene_idx is None:
+ return self._duplicate_to_list(scene_idx, num)
+ # scene_idx could be str, np.ndarray, list or tuple
+ if isinstance(scene_idx, str): # str
+ return self._duplicate_to_list(scene_idx, num)
+ if isinstance(scene_idx[0], str): # list of str
+ return scene_idx
+ if isinstance(scene_idx[0], (list, tuple, np.ndarray)): # list of idx
+ return scene_idx
+ # single idx
+ return self._duplicate_to_list(scene_idx, num)
+
+
+@DATASETS.register_module()
+class S3DISInstanceSegDataset(S3DISDataset):
+ VALID_CLASS_IDS = (7, 8, 9, 10, 11)
+
+ def _build_default_pipeline(self):
+ """Build the default pipeline for this dataset."""
+ pipeline = [
+ dict(
+ type='LoadPointsFromFile',
+ coord_type='DEPTH',
+ shift_height=False,
+ use_color=True,
+ load_dim=6,
+ use_dim=[0, 1, 2, 3, 4, 5]),
+ dict(
+ type='LoadAnnotations3D',
+ with_bbox_3d=False,
+ with_label_3d=False,
+ with_mask_3d=True,
+ with_seg_3d=True),
+ dict(
+ type='DefaultFormatBundle3D',
+ with_label=False,
+ class_names=self.CLASSES),
+ dict(
+ type='Collect3D',
+ keys=['points', 'pts_semantic_mask', 'pts_instance_mask'])
+ ]
+ return Compose(pipeline)
+
+ def evaluate(self,
+ results,
+ metric=None,
+ options=None,
+ logger=None,
+ show=False,
+ out_dir=None,
+ pipeline=None):
+ """Evaluation in instance segmentation protocol.
+
+ Args:
+ results (list[dict]): List of results.
+ metric (str | list[str]): Metrics to be evaluated.
+ options (dict, optional): options for instance_seg_eval.
+ logger (logging.Logger | None | str): Logger used for printing
+ related information during evaluation. Defaults to None.
+ show (bool, optional): Whether to visualize.
+ Defaults to False.
+ out_dir (str, optional): Path to save the visualization results.
+ Defaults to None.
+ pipeline (list[dict], optional): raw data loading for showing.
+ Default: None.
+
+ Returns:
+ dict: Evaluation results.
+ """
+ assert isinstance(
+ results, list), f'Expect results to be list, got {type(results)}.'
+ assert len(results) > 0, 'Expect length of results > 0.'
+ assert len(results) == len(self.data_infos)
+ assert isinstance(
+ results[0], dict
+ ), f'Expect elements in results to be dict, got {type(results[0])}.'
+
+ load_pipeline = self._build_default_pipeline()
+ pred_instance_masks = [result['instance_mask'] for result in results]
+ pred_instance_labels = [result['instance_label'] for result in results]
+ pred_instance_scores = [result['instance_score'] for result in results]
+ gt_semantic_masks, gt_instance_masks = zip(*[
+ self._extract_data(
+ index=i,
+ pipeline=load_pipeline,
+ key=['pts_semantic_mask', 'pts_instance_mask'],
+ load_annos=True) for i in range(len(self.data_infos))
+ ])
+ ret_dict = instance_seg_eval_v2(
+ gt_semantic_masks,
+ gt_instance_masks,
+ pred_instance_masks,
+ pred_instance_labels,
+ pred_instance_scores,
+ valid_class_ids=self.VALID_CLASS_IDS,
+ class_labels=self.CLASSES,
+ options=options,
+ logger=logger)
+
+ if show:
+ self.show(results, out_dir)
+
+ return ret_dict
+
+ def show(self, results, out_dir, show=True, pipeline=None):
+ assert out_dir is not None, 'Expect out_dir, got none.'
+ load_pipeline = self._build_default_pipeline()
+ for i, result in enumerate(results):
+ data_info = self.data_infos[i]
+ pts_path = data_info['pts_path']
+ file_name = osp.split(pts_path)[-1].split('.')[0]
+ points, gt_instance_mask, gt_sem_mask = self._extract_data(
+ i, load_pipeline, ['points', 'pts_instance_mask', 'pts_semantic_mask'], load_annos=True)
+ points = points.numpy()
+ gt_inst_mask_final = np.zeros_like(gt_instance_mask)
+ for cls_idx in self.VALID_CLASS_IDS:
+ mask = gt_sem_mask == cls_idx
+ gt_inst_mask_final += mask.numpy()
+ gt_instance_mask[gt_inst_mask_final == 0] = -1
+
+ pred_instance_masks = result['instance_mask']
+ pred_instance_scores = result['instance_score']
+
+ pred_instance_masks_sort = pred_instance_masks[pred_instance_scores.argsort()]
+ pred_instance_masks_label = pred_instance_masks_sort[0].long() - 1
+ for i in range(1, pred_instance_masks_sort.shape[0]):
+ pred_instance_masks_label[pred_instance_masks_sort[i].bool()] = i
+
+ palette = np.random.random((max(max(pred_instance_masks_label) + 2, max(gt_instance_mask) + 2), 3)) * 255
+ palette[-1] = 255
+
+ show_seg_result(points, gt_instance_mask,
+ pred_instance_masks_label, out_dir, file_name,
+ palette)
\ No newline at end of file
diff --git a/mmdet3d/datasets/scannet_dataset.py b/mmdet3d/datasets/scannet_dataset.py
new file mode 100644
index 0000000..e18ddb7
--- /dev/null
+++ b/mmdet3d/datasets/scannet_dataset.py
@@ -0,0 +1,749 @@
+# Copyright (c) OpenMMLab. All rights reserved.
+import tempfile
+import warnings
+from os import path as osp
+
+import numpy as np
+
+from mmdet3d.core import (
+ instance_seg_eval, instance_seg_eval_v2, show_result, show_seg_result)
+from mmdet3d.core.bbox import DepthInstance3DBoxes
+from mmseg.datasets import DATASETS as SEG_DATASETS
+from .builder import DATASETS
+from .custom_3d import Custom3DDataset
+from .custom_3d_seg import Custom3DSegDataset
+from .pipelines import Compose
+
+
+@DATASETS.register_module()
+class ScanNetDataset(Custom3DDataset):
+ r"""ScanNet Dataset for Detection Task.
+
+ This class serves as the API for experiments on the ScanNet Dataset.
+
+ Please refer to the `github repo `_
+ for data downloading.
+
+ Args:
+ data_root (str): Path of dataset root.
+ ann_file (str): Path of annotation file.
+ pipeline (list[dict], optional): Pipeline used for data processing.
+ Defaults to None.
+ classes (tuple[str], optional): Classes used in the dataset.
+ Defaults to None.
+ modality (dict, optional): Modality to specify the sensor data used
+ as input. Defaults to None.
+ box_type_3d (str, optional): Type of 3D box of this dataset.
+ Based on the `box_type_3d`, the dataset will encapsulate the box
+ to its original format then converted them to `box_type_3d`.
+ Defaults to 'Depth' in this dataset. Available options includes
+
+ - 'LiDAR': Box in LiDAR coordinates.
+ - 'Depth': Box in depth coordinates, usually for indoor dataset.
+ - 'Camera': Box in camera coordinates.
+ filter_empty_gt (bool, optional): Whether to filter empty GT.
+ Defaults to True.
+ test_mode (bool, optional): Whether the dataset is in test mode.
+ Defaults to False.
+ """
+ CLASSES = ('cabinet', 'bed', 'chair', 'sofa', 'table', 'door', 'window',
+ 'bookshelf', 'picture', 'counter', 'desk', 'curtain',
+ 'refrigerator', 'showercurtrain', 'toilet', 'sink', 'bathtub',
+ 'garbagebin')
+
+ def __init__(self,
+ data_root,
+ ann_file,
+ pipeline=None,
+ classes=None,
+ modality=dict(use_camera=False, use_depth=True),
+ box_type_3d='Depth',
+ filter_empty_gt=True,
+ test_mode=False,
+ **kwargs):
+ super().__init__(
+ data_root=data_root,
+ ann_file=ann_file,
+ pipeline=pipeline,
+ classes=classes,
+ modality=modality,
+ box_type_3d=box_type_3d,
+ filter_empty_gt=filter_empty_gt,
+ test_mode=test_mode,
+ **kwargs)
+ assert 'use_camera' in self.modality and \
+ 'use_depth' in self.modality
+ assert self.modality['use_camera'] or self.modality['use_depth']
+
+ def get_data_info(self, index):
+ """Get data info according to the given index.
+
+ Args:
+ index (int): Index of the sample data to get.
+
+ Returns:
+ dict: Data information that will be passed to the data
+ preprocessing pipelines. It includes the following keys:
+
+ - sample_idx (str): Sample index.
+ - pts_filename (str): Filename of point clouds.
+ - file_name (str): Filename of point clouds.
+ - img_prefix (str, optional): Prefix of image files.
+ - img_info (dict, optional): Image info.
+ - ann_info (dict): Annotation info.
+ """
+ info = self.data_infos[index]
+ sample_idx = info['point_cloud']['lidar_idx']
+ pts_filename = osp.join(self.data_root, info['pts_path'])
+ input_dict = dict(sample_idx=sample_idx)
+
+ if self.modality['use_depth']:
+ input_dict['pts_filename'] = pts_filename
+ input_dict['file_name'] = pts_filename
+
+ if self.modality['use_camera']:
+ img_info = []
+ for img_path in info['img_paths']:
+ img_info.append(
+ dict(filename=osp.join(self.data_root, img_path)))
+ intrinsic = info['intrinsics']
+ axis_align_matrix = self._get_axis_align_matrix(info)
+ depth2img = []
+ for extrinsic in info['extrinsics']:
+ depth2img.append(
+ intrinsic @ np.linalg.inv(axis_align_matrix @ extrinsic))
+
+ input_dict['img_prefix'] = None
+ input_dict['img_info'] = img_info
+ input_dict['depth2img'] = depth2img
+
+ if not self.test_mode:
+ annos = self.get_ann_info(index)
+ input_dict['ann_info'] = annos
+ if self.filter_empty_gt and ~(annos['gt_labels_3d'] != -1).any():
+ return None
+ return input_dict
+
+ def get_ann_info(self, index):
+ """Get annotation info according to the given index.
+
+ Args:
+ index (int): Index of the annotation data to get.
+
+ Returns:
+ dict: annotation information consists of the following keys:
+
+ - gt_bboxes_3d (:obj:`DepthInstance3DBoxes`):
+ 3D ground truth bboxes
+ - gt_labels_3d (np.ndarray): Labels of ground truths.
+ - pts_instance_mask_path (str): Path of instance masks.
+ - pts_semantic_mask_path (str): Path of semantic masks.
+ - axis_align_matrix (np.ndarray): Transformation matrix for
+ global scene alignment.
+ """
+ # Use index to get the annos, thus the evalhook could also use this api
+ info = self.data_infos[index]
+ if info['annos']['gt_num'] != 0:
+ gt_bboxes_3d = info['annos']['gt_boxes_upright_depth'].astype(
+ np.float32) # k, 6
+ gt_labels_3d = info['annos']['class'].astype(np.int64)
+ else:
+ gt_bboxes_3d = np.zeros((0, 6), dtype=np.float32)
+ gt_labels_3d = np.zeros((0, ), dtype=np.int64)
+
+ # to target box structure
+ gt_bboxes_3d = DepthInstance3DBoxes(
+ gt_bboxes_3d,
+ box_dim=gt_bboxes_3d.shape[-1],
+ with_yaw=False,
+ origin=(0.5, 0.5, 0.5)).convert_to(self.box_mode_3d)
+
+ pts_instance_mask_path = osp.join(self.data_root,
+ info['pts_instance_mask_path'])
+ pts_semantic_mask_path = osp.join(self.data_root,
+ info['pts_semantic_mask_path'])
+
+ axis_align_matrix = self._get_axis_align_matrix(info)
+
+ anns_results = dict(
+ gt_bboxes_3d=gt_bboxes_3d,
+ gt_labels_3d=gt_labels_3d,
+ pts_instance_mask_path=pts_instance_mask_path,
+ pts_semantic_mask_path=pts_semantic_mask_path,
+ axis_align_matrix=axis_align_matrix)
+ return anns_results
+
+ def prepare_test_data(self, index):
+ """Prepare data for testing.
+
+ We should take axis_align_matrix from self.data_infos since we need
+ to align point clouds.
+
+ Args:
+ index (int): Index for accessing the target data.
+
+ Returns:
+ dict: Testing data dict of the corresponding index.
+ """
+ input_dict = self.get_data_info(index)
+ # take the axis_align_matrix from data_infos
+ input_dict['ann_info'] = dict(
+ axis_align_matrix=self._get_axis_align_matrix(
+ self.data_infos[index]))
+ self.pre_pipeline(input_dict)
+ example = self.pipeline(input_dict)
+ return example
+
+ @staticmethod
+ def _get_axis_align_matrix(info):
+ """Get axis_align_matrix from info. If not exist, return identity mat.
+
+ Args:
+ info (dict): one data info term.
+
+ Returns:
+ np.ndarray: 4x4 transformation matrix.
+ """
+ if 'annos' not in info.keys():
+ return np.eye(4).astype(np.float32)
+
+ if 'axis_align_matrix' in info['annos'].keys():
+ return info['annos']['axis_align_matrix'].astype(np.float32)
+ else:
+ warnings.warn(
+ 'axis_align_matrix is not found in ScanNet data info, please '
+ 'use new pre-process scripts to re-generate ScanNet data')
+ return np.eye(4).astype(np.float32)
+
+ def _build_default_pipeline(self):
+ """Build the default pipeline for this dataset."""
+ pipeline = [
+ dict(
+ type='LoadPointsFromFile',
+ coord_type='DEPTH',
+ shift_height=False,
+ load_dim=6,
+ use_dim=[0, 1, 2]),
+ dict(type='GlobalAlignment', rotation_axis=2),
+ dict(
+ type='DefaultFormatBundle3D',
+ class_names=self.CLASSES,
+ with_label=False),
+ dict(type='Collect3D', keys=['points'])
+ ]
+ return Compose(pipeline)
+
+ def show(self, results, out_dir, show=True, pipeline=None):
+ """Results visualization.
+
+ Args:
+ results (list[dict]): List of bounding boxes results.
+ out_dir (str): Output directory of visualization result.
+ show (bool): Visualize the results online.
+ pipeline (list[dict], optional): raw data loading for showing.
+ Default: None.
+ """
+ assert out_dir is not None, 'Expect out_dir, got none.'
+ pipeline = self._get_pipeline(pipeline)
+ for i, result in enumerate(results):
+ data_info = self.data_infos[i]
+ pts_path = data_info['pts_path']
+ file_name = osp.split(pts_path)[-1].split('.')[0]
+ points = self._extract_data(i, pipeline, 'points').numpy()
+ gt_bboxes = self.get_ann_info(i)['gt_bboxes_3d'].tensor.numpy()
+ pred_bboxes = result['boxes_3d'].tensor.numpy()
+ show_result(points, gt_bboxes, pred_bboxes, out_dir, file_name,
+ show)
+
+
+@DATASETS.register_module()
+@SEG_DATASETS.register_module()
+class ScanNetSegDataset(Custom3DSegDataset):
+ r"""ScanNet Dataset for Semantic Segmentation Task.
+
+ This class serves as the API for experiments on the ScanNet Dataset.
+
+ Please refer to the `github repo `_
+ for data downloading.
+
+ Args:
+ data_root (str): Path of dataset root.
+ ann_file (str): Path of annotation file.
+ pipeline (list[dict], optional): Pipeline used for data processing.
+ Defaults to None.
+ classes (tuple[str], optional): Classes used in the dataset.
+ Defaults to None.
+ palette (list[list[int]], optional): The palette of segmentation map.
+ Defaults to None.
+ modality (dict, optional): Modality to specify the sensor data used
+ as input. Defaults to None.
+ test_mode (bool, optional): Whether the dataset is in test mode.
+ Defaults to False.
+ ignore_index (int, optional): The label index to be ignored, e.g.
+ unannotated points. If None is given, set to len(self.CLASSES).
+ Defaults to None.
+ scene_idxs (np.ndarray | str, optional): Precomputed index to load
+ data. For scenes with many points, we may sample it several times.
+ Defaults to None.
+ """
+ CLASSES = ('wall', 'floor', 'cabinet', 'bed', 'chair', 'sofa', 'table',
+ 'door', 'window', 'bookshelf', 'picture', 'counter', 'desk',
+ 'curtain', 'refrigerator', 'showercurtrain', 'toilet', 'sink',
+ 'bathtub', 'otherfurniture')
+
+ VALID_CLASS_IDS = (1, 2, 3, 4, 5, 6, 7, 8, 9, 10, 11, 12, 14, 16, 24, 28,
+ 33, 34, 36, 39)
+
+ ALL_CLASS_IDS = tuple(range(41))
+
+ PALETTE = [
+ [174, 199, 232],
+ [152, 223, 138],
+ [31, 119, 180],
+ [255, 187, 120],
+ [188, 189, 34],
+ [140, 86, 75],
+ [255, 152, 150],
+ [214, 39, 40],
+ [197, 176, 213],
+ [148, 103, 189],
+ [196, 156, 148],
+ [23, 190, 207],
+ [247, 182, 210],
+ [219, 219, 141],
+ [255, 127, 14],
+ [158, 218, 229],
+ [44, 160, 44],
+ [112, 128, 144],
+ [227, 119, 194],
+ [82, 84, 163],
+ ]
+
+ def __init__(self,
+ data_root,
+ ann_file,
+ pipeline=None,
+ classes=None,
+ palette=None,
+ modality=None,
+ test_mode=False,
+ ignore_index=None,
+ scene_idxs=None,
+ **kwargs):
+
+ super().__init__(
+ data_root=data_root,
+ ann_file=ann_file,
+ pipeline=pipeline,
+ classes=classes,
+ palette=palette,
+ modality=modality,
+ test_mode=test_mode,
+ ignore_index=ignore_index,
+ scene_idxs=scene_idxs,
+ **kwargs)
+
+ def get_ann_info(self, index):
+ """Get annotation info according to the given index.
+
+ Args:
+ index (int): Index of the annotation data to get.
+
+ Returns:
+ dict: annotation information consists of the following keys:
+
+ - pts_semantic_mask_path (str): Path of semantic masks.
+ """
+ # Use index to get the annos, thus the evalhook could also use this api
+ info = self.data_infos[index]
+
+ pts_semantic_mask_path = osp.join(self.data_root,
+ info['pts_semantic_mask_path'])
+
+ anns_results = dict(pts_semantic_mask_path=pts_semantic_mask_path)
+ return anns_results
+
+ def _build_default_pipeline(self):
+ """Build the default pipeline for this dataset."""
+ pipeline = [
+ dict(
+ type='LoadPointsFromFile',
+ coord_type='DEPTH',
+ shift_height=False,
+ use_color=True,
+ load_dim=6,
+ use_dim=[0, 1, 2, 3, 4, 5]),
+ dict(
+ type='LoadAnnotations3D',
+ with_bbox_3d=False,
+ with_label_3d=False,
+ with_mask_3d=False,
+ with_seg_3d=True),
+ dict(
+ type='PointSegClassMapping',
+ valid_cat_ids=self.VALID_CLASS_IDS,
+ max_cat_id=np.max(self.ALL_CLASS_IDS)),
+ dict(
+ type='DefaultFormatBundle3D',
+ with_label=False,
+ class_names=self.CLASSES),
+ dict(type='Collect3D', keys=['points', 'pts_semantic_mask'])
+ ]
+ return Compose(pipeline)
+
+ def show(self, results, out_dir, show=True, pipeline=None):
+ """Results visualization.
+
+ Args:
+ results (list[dict]): List of bounding boxes results.
+ out_dir (str): Output directory of visualization result.
+ show (bool): Visualize the results online.
+ pipeline (list[dict], optional): raw data loading for showing.
+ Default: None.
+ """
+ assert out_dir is not None, 'Expect out_dir, got none.'
+ pipeline = self._get_pipeline(pipeline)
+ for i, result in enumerate(results):
+ data_info = self.data_infos[i]
+ pts_path = data_info['pts_path']
+ file_name = osp.split(pts_path)[-1].split('.')[0]
+ points, gt_sem_mask = self._extract_data(
+ i, pipeline, ['points', 'pts_semantic_mask'], load_annos=True)
+ points = points.numpy()
+ pred_sem_mask = result['semantic_mask'].numpy()
+ show_seg_result(points, gt_sem_mask,
+ pred_sem_mask, out_dir, file_name,
+ np.array(self.PALETTE), self.ignore_index, show)
+
+ def get_scene_idxs(self, scene_idxs):
+ """Compute scene_idxs for data sampling.
+
+ We sample more times for scenes with more points.
+ """
+ # when testing, we load one whole scene every time
+ if not self.test_mode and scene_idxs is None:
+ raise NotImplementedError(
+ 'please provide re-sampled scene indexes for training')
+
+ return super().get_scene_idxs(scene_idxs)
+
+ def format_results(self, results, txtfile_prefix=None):
+ r"""Format the results to txt file. Refer to `ScanNet documentation
+ `_.
+
+ Args:
+ outputs (list[dict]): Testing results of the dataset.
+ txtfile_prefix (str): The prefix of saved files. It includes
+ the file path and the prefix of filename, e.g., "a/b/prefix".
+ If not specified, a temp file will be created. Default: None.
+
+ Returns:
+ tuple: (outputs, tmp_dir), outputs is the detection results,
+ tmp_dir is the temporal directory created for saving submission
+ files when ``submission_prefix`` is not specified.
+ """
+ import mmcv
+
+ if txtfile_prefix is None:
+ tmp_dir = tempfile.TemporaryDirectory()
+ txtfile_prefix = osp.join(tmp_dir.name, 'results')
+ else:
+ tmp_dir = None
+ mmcv.mkdir_or_exist(txtfile_prefix)
+
+ # need to map network output to original label idx
+ pred2label = np.zeros(len(self.VALID_CLASS_IDS)).astype(np.int)
+ for original_label, output_idx in self.label_map.items():
+ if output_idx != self.ignore_index:
+ pred2label[output_idx] = original_label
+
+ outputs = []
+ for i, result in enumerate(results):
+ info = self.data_infos[i]
+ sample_idx = info['point_cloud']['lidar_idx']
+ pred_sem_mask = result['semantic_mask'].numpy().astype(np.int)
+ pred_label = pred2label[pred_sem_mask]
+ curr_file = f'{txtfile_prefix}/{sample_idx}.txt'
+ np.savetxt(curr_file, pred_label, fmt='%d')
+ outputs.append(dict(seg_mask=pred_label))
+
+ return outputs, tmp_dir
+
+
+@DATASETS.register_module()
+@SEG_DATASETS.register_module()
+class ScanNetInstanceSegDataset(Custom3DSegDataset):
+ CLASSES = ('cabinet', 'bed', 'chair', 'sofa', 'table', 'door', 'window',
+ 'bookshelf', 'picture', 'counter', 'desk', 'curtain',
+ 'refrigerator', 'showercurtrain', 'toilet', 'sink', 'bathtub',
+ 'garbagebin')
+
+ VALID_CLASS_IDS = (3, 4, 5, 6, 7, 8, 9, 10, 11, 12, 14, 16, 24, 28, 33, 34,
+ 36, 39)
+
+ ALL_CLASS_IDS = tuple(range(41))
+
+ def get_ann_info(self, index):
+ """Get annotation info according to the given index.
+
+ Args:
+ index (int): Index of the annotation data to get.
+
+ Returns:
+ dict: annotation information consists of the following keys:
+ - pts_semantic_mask_path (str): Path of semantic masks.
+ - pts_instance_mask_path (str): Path of instance masks.
+ """
+ # Use index to get the annos, thus the evalhook could also use this api
+ info = self.data_infos[index]
+
+ pts_instance_mask_path = osp.join(self.data_root,
+ info['pts_instance_mask_path'])
+ pts_semantic_mask_path = osp.join(self.data_root,
+ info['pts_semantic_mask_path'])
+
+ anns_results = dict(
+ pts_instance_mask_path=pts_instance_mask_path,
+ pts_semantic_mask_path=pts_semantic_mask_path)
+ return anns_results
+
+ def get_classes_and_palette(self, classes=None, palette=None):
+ """Get class names of current dataset. Palette is simply ignored for
+ instance segmentation.
+
+ Args:
+ classes (Sequence[str] | str | None): If classes is None, use
+ default CLASSES defined by builtin dataset. If classes is a
+ string, take it as a file name. The file contains the name of
+ classes where each line contains one class name. If classes is
+ a tuple or list, override the CLASSES defined by the dataset.
+ Defaults to None.
+ palette (Sequence[Sequence[int]]] | np.ndarray | None):
+ The palette of segmentation map. If None is given, random
+ palette will be generated. Defaults to None.
+ """
+ if classes is not None:
+ return classes, None
+ return self.CLASSES, None
+
+ def _build_default_pipeline(self):
+ """Build the default pipeline for this dataset."""
+ pipeline = [
+ dict(
+ type='LoadPointsFromFile',
+ coord_type='DEPTH',
+ shift_height=False,
+ use_color=True,
+ load_dim=6,
+ use_dim=[0, 1, 2, 3, 4, 5]),
+ dict(
+ type='LoadAnnotations3D',
+ with_bbox_3d=False,
+ with_label_3d=False,
+ with_mask_3d=True,
+ with_seg_3d=True),
+ dict(
+ type='PointSegClassMapping',
+ valid_cat_ids=self.VALID_CLASS_IDS,
+ max_cat_id=40),
+ dict(
+ type='DefaultFormatBundle3D',
+ with_label=False,
+ class_names=self.CLASSES),
+ dict(
+ type='Collect3D',
+ keys=['points', 'pts_semantic_mask', 'pts_instance_mask'])
+ ]
+ return Compose(pipeline)
+
+ def evaluate(self,
+ results,
+ metric=None,
+ options=None,
+ logger=None,
+ show=False,
+ out_dir=None,
+ pipeline=None):
+ """Evaluation in instance segmentation protocol.
+
+ Args:
+ results (list[dict]): List of results.
+ metric (str | list[str]): Metrics to be evaluated.
+ options (dict, optional): options for instance_seg_eval.
+ logger (logging.Logger | None | str): Logger used for printing
+ related information during evaluation. Defaults to None.
+ show (bool, optional): Whether to visualize.
+ Defaults to False.
+ out_dir (str, optional): Path to save the visualization results.
+ Defaults to None.
+ pipeline (list[dict], optional): raw data loading for showing.
+ Default: None.
+
+ Returns:
+ dict: Evaluation results.
+ """
+ assert isinstance(
+ results, list), f'Expect results to be list, got {type(results)}.'
+ assert len(results) > 0, 'Expect length of results > 0.'
+ assert len(results) == len(self.data_infos)
+ assert isinstance(
+ results[0], dict
+ ), f'Expect elements in results to be dict, got {type(results[0])}.'
+
+ load_pipeline = self._get_pipeline(pipeline)
+ pred_instance_masks = [result['instance_mask'] for result in results]
+ pred_instance_labels = [result['instance_label'] for result in results]
+ pred_instance_scores = [result['instance_score'] for result in results]
+ gt_semantic_masks, gt_instance_masks = zip(*[
+ self._extract_data(
+ index=i,
+ pipeline=load_pipeline,
+ key=['pts_semantic_mask', 'pts_instance_mask'],
+ load_annos=True) for i in range(len(self.data_infos))
+ ])
+ ret_dict = instance_seg_eval(
+ gt_semantic_masks,
+ gt_instance_masks,
+ pred_instance_masks,
+ pred_instance_labels,
+ pred_instance_scores,
+ valid_class_ids=self.VALID_CLASS_IDS,
+ class_labels=self.CLASSES,
+ options=options,
+ logger=logger)
+
+ if show:
+ raise NotImplementedError('show is not implemented for now')
+
+ return ret_dict
+
+@DATASETS.register_module()
+class ScanNetInstanceSegV2Dataset(ScanNetDataset):
+ VALID_CLASS_IDS = (3, 4, 5, 6, 7, 8, 9, 10, 11, 12, 14, 16, 24, 28,
+ 33, 34, 36, 39)
+
+ def _build_default_pipeline(self):
+ """Build the default pipeline for this dataset."""
+ pipeline = [
+ dict(
+ type='LoadPointsFromFile',
+ coord_type='DEPTH',
+ shift_height=False,
+ use_color=True,
+ load_dim=6,
+ use_dim=[0, 1, 2, 3, 4, 5]),
+ dict(
+ type='LoadAnnotations3D',
+ with_bbox_3d=False,
+ with_label_3d=False,
+ with_mask_3d=True,
+ with_seg_3d=True),
+ dict(
+ type='DefaultFormatBundle3D',
+ with_label=False,
+ class_names=self.CLASSES),
+ dict(
+ type='Collect3D',
+ keys=['points', 'pts_semantic_mask', 'pts_instance_mask'])
+ ]
+ return Compose(pipeline)
+
+ def evaluate(self,
+ results,
+ metric=None,
+ options=None,
+ logger=None,
+ show=False,
+ out_dir=None,
+ pipeline=None):
+ """Evaluation in instance segmentation protocol.
+
+ Args:
+ results (list[dict]): List of results.
+ metric (str | list[str]): Metrics to be evaluated.
+ options (dict, optional): options for instance_seg_eval.
+ logger (logging.Logger | None | str): Logger used for printing
+ related information during evaluation. Defaults to None.
+ show (bool, optional): Whether to visualize.
+ Defaults to False.
+ out_dir (str, optional): Path to save the visualization results.
+ Defaults to None.
+ pipeline (list[dict], optional): raw data loading for showing.
+ Default: None.
+
+ Returns:
+ dict: Evaluation results.
+ """
+ assert isinstance(
+ results, list), f'Expect results to be list, got {type(results)}.'
+ assert len(results) > 0, 'Expect length of results > 0.'
+ assert len(results) == len(self.data_infos)
+ assert isinstance(
+ results[0], dict
+ ), f'Expect elements in results to be dict, got {type(results[0])}.'
+
+ load_pipeline = self._build_default_pipeline()
+ pred_instance_masks = [result['instance_mask'] for result in results]
+ pred_instance_labels = [result['instance_label'] for result in results]
+ pred_instance_scores = [result['instance_score'] for result in results]
+ gt_semantic_masks, gt_instance_masks = zip(*[
+ self._extract_data(
+ index=i,
+ pipeline=load_pipeline,
+ key=['pts_semantic_mask', 'pts_instance_mask'],
+ load_annos=True) for i in range(len(self.data_infos))
+ ])
+ ret_dict = instance_seg_eval_v2(
+ gt_semantic_masks,
+ gt_instance_masks,
+ pred_instance_masks,
+ pred_instance_labels,
+ pred_instance_scores,
+ valid_class_ids=self.VALID_CLASS_IDS,
+ class_labels=self.CLASSES,
+ options=options,
+ logger=logger)
+
+ if show:
+ self.show(results, out_dir)
+
+ return ret_dict
+
+ def show(self, results, out_dir, show=True, pipeline=None):
+ assert out_dir is not None, 'Expect out_dir, got none.'
+ load_pipeline = self._build_default_pipeline()
+ for i, result in enumerate(results):
+ data_info = self.data_infos[i]
+ pts_path = data_info['pts_path']
+ file_name = osp.split(pts_path)[-1].split('.')[0]
+ points, gt_instance_mask, gt_sem_mask = self._extract_data(
+ i, load_pipeline, ['points', 'pts_instance_mask', 'pts_semantic_mask'], load_annos=True)
+ points = points.numpy()
+ gt_inst_mask_final = np.zeros_like(gt_instance_mask)
+ for cls_idx in self.VALID_CLASS_IDS:
+ mask = gt_sem_mask == cls_idx
+ gt_inst_mask_final += mask.numpy()
+ gt_instance_mask[gt_inst_mask_final == 0] = -1
+
+ pred_instance_masks = result['instance_mask']
+ pred_instance_scores = result['instance_score']
+
+ pred_instance_masks_sort = pred_instance_masks[pred_instance_scores.argsort()]
+ pred_instance_masks_label = pred_instance_masks_sort[0].long() - 1
+ for i in range(1, pred_instance_masks_sort.shape[0]):
+ pred_instance_masks_label[pred_instance_masks_sort[i].bool()] = i
+
+ palette = np.random.random((max(max(pred_instance_masks_label) + 2, max(gt_instance_mask) + 2), 3)) * 255
+ palette[-1] = 255
+
+ show_seg_result(points, gt_instance_mask,
+ pred_instance_masks_label, out_dir, file_name,
+ palette)
+
+@DATASETS.register_module()
+class ScanNet200InstanceSegDataset(ScanNetInstanceSegV2Dataset):
+ VALID_CLASS_IDS = (
+ 2, 4, 5, 6, 7, 8, 9, 10, 11, 13, 14, 15, 16, 17, 18, 19, 21, 22, 23, 24, 26, 27, 28, 29, 31, 32, 33, 34, 35, 36, 38, 39, 40, 41, 42, 44, 45, 46, 47, 48, 49, 50, 51, 52, 54, 55, 56, 57, 58, 59, 62, 63, 64, 65, 66, 67, 68, 69, 70, 71,
+ 72, 73, 74, 75, 76, 77, 78, 79, 80, 82, 84, 86, 87, 88, 89, 90, 93, 95, 96, 97, 98, 99, 100, 101, 102, 103, 104, 105, 106, 107, 110, 112, 115, 116, 118, 120, 121, 122, 125, 128, 130, 131, 132, 134, 136, 138, 139, 140, 141, 145, 148, 154,
+ 155, 156, 157, 159, 161, 163, 165, 166, 168, 169, 170, 177, 180, 185, 188, 191, 193, 195, 202, 208, 213, 214, 221, 229, 230, 232, 233, 242, 250, 261, 264, 276, 283, 286, 300, 304, 312, 323, 325, 331, 342, 356, 370, 392, 395, 399, 408, 417,
+ 488, 540, 562, 570, 572, 581, 609, 748, 776, 1156, 1163, 1164, 1165, 1166, 1167, 1168, 1169, 1170, 1171, 1172, 1173, 1174, 1175, 1176, 1178, 1179, 1180, 1181, 1182, 1183, 1184, 1185, 1186, 1187, 1188, 1189, 1190, 1191)
diff --git a/mmdet3d/datasets/semantickitti_dataset.py b/mmdet3d/datasets/semantickitti_dataset.py
new file mode 100644
index 0000000..03afbe0
--- /dev/null
+++ b/mmdet3d/datasets/semantickitti_dataset.py
@@ -0,0 +1,110 @@
+# Copyright (c) OpenMMLab. All rights reserved.
+from os import path as osp
+
+from .builder import DATASETS
+from .custom_3d import Custom3DDataset
+
+
+@DATASETS.register_module()
+class SemanticKITTIDataset(Custom3DDataset):
+ r"""SemanticKITTI Dataset.
+
+ This class serves as the API for experiments on the SemanticKITTI Dataset
+ Please refer to `_
+ for data downloading
+
+ Args:
+ data_root (str): Path of dataset root.
+ ann_file (str): Path of annotation file.
+ pipeline (list[dict], optional): Pipeline used for data processing.
+ Defaults to None.
+ classes (tuple[str], optional): Classes used in the dataset.
+ Defaults to None.
+ modality (dict, optional): Modality to specify the sensor data used
+ as input. Defaults to None.
+ box_type_3d (str, optional): NO 3D box for this dataset.
+ You can choose any type
+ Based on the `box_type_3d`, the dataset will encapsulate the box
+ to its original format then converted them to `box_type_3d`.
+ Defaults to 'LiDAR' in this dataset. Available options includes
+
+ - 'LiDAR': Box in LiDAR coordinates.
+ - 'Depth': Box in depth coordinates, usually for indoor dataset.
+ - 'Camera': Box in camera coordinates.
+ filter_empty_gt (bool, optional): Whether to filter empty GT.
+ Defaults to True.
+ test_mode (bool, optional): Whether the dataset is in test mode.
+ Defaults to False.
+ """
+ CLASSES = ('unlabeled', 'car', 'bicycle', 'motorcycle', 'truck', 'bus',
+ 'person', 'bicyclist', 'motorcyclist', 'road', 'parking',
+ 'sidewalk', 'other-ground', 'building', 'fence', 'vegetation',
+ 'trunck', 'terrian', 'pole', 'traffic-sign')
+
+ def __init__(self,
+ data_root,
+ ann_file,
+ pipeline=None,
+ classes=None,
+ modality=None,
+ box_type_3d='Lidar',
+ filter_empty_gt=False,
+ test_mode=False):
+ super().__init__(
+ data_root=data_root,
+ ann_file=ann_file,
+ pipeline=pipeline,
+ classes=classes,
+ modality=modality,
+ box_type_3d=box_type_3d,
+ filter_empty_gt=filter_empty_gt,
+ test_mode=test_mode)
+
+ def get_data_info(self, index):
+ """Get data info according to the given index.
+ Args:
+ index (int): Index of the sample data to get.
+
+ Returns:
+ dict: Data information that will be passed to the data
+ preprocessing pipelines. It includes the following keys:
+ - sample_idx (str): Sample index.
+ - pts_filename (str): Filename of point clouds.
+ - file_name (str): Filename of point clouds.
+ - ann_info (dict): Annotation info.
+ """
+ info = self.data_infos[index]
+ sample_idx = info['point_cloud']['lidar_idx']
+ pts_filename = osp.join(self.data_root, info['pts_path'])
+
+ input_dict = dict(
+ pts_filename=pts_filename,
+ sample_idx=sample_idx,
+ file_name=pts_filename)
+
+ if not self.test_mode:
+ annos = self.get_ann_info(index)
+ input_dict['ann_info'] = annos
+ if self.filter_empty_gt and ~(annos['gt_labels_3d'] != -1).any():
+ return None
+ return input_dict
+
+ def get_ann_info(self, index):
+ """Get annotation info according to the given index.
+
+ Args:
+ index (int): Index of the annotation data to get.
+
+ Returns:
+ dict: annotation information consists of the following keys:
+
+ - pts_semantic_mask_path (str): Path of semantic masks.
+ """
+ # Use index to get the annos, thus the evalhook could also use this api
+ info = self.data_infos[index]
+
+ pts_semantic_mask_path = osp.join(self.data_root,
+ info['pts_semantic_mask_path'])
+
+ anns_results = dict(pts_semantic_mask_path=pts_semantic_mask_path)
+ return anns_results
diff --git a/mmdet3d/datasets/sunrgbd_dataset.py b/mmdet3d/datasets/sunrgbd_dataset.py
new file mode 100644
index 0000000..623ab88
--- /dev/null
+++ b/mmdet3d/datasets/sunrgbd_dataset.py
@@ -0,0 +1,280 @@
+# Copyright (c) OpenMMLab. All rights reserved.
+from collections import OrderedDict
+from os import path as osp
+
+import numpy as np
+
+from mmdet3d.core import show_multi_modality_result, show_result
+from mmdet3d.core.bbox import DepthInstance3DBoxes
+from mmdet.core import eval_map
+from .builder import DATASETS
+from .custom_3d import Custom3DDataset
+from .pipelines import Compose
+
+
+@DATASETS.register_module()
+class SUNRGBDDataset(Custom3DDataset):
+ r"""SUNRGBD Dataset.
+
+ This class serves as the API for experiments on the SUNRGBD Dataset.
+
+ See the `download page `_
+ for data downloading.
+
+ Args:
+ data_root (str): Path of dataset root.
+ ann_file (str): Path of annotation file.
+ pipeline (list[dict], optional): Pipeline used for data processing.
+ Defaults to None.
+ classes (tuple[str], optional): Classes used in the dataset.
+ Defaults to None.
+ modality (dict, optional): Modality to specify the sensor data used
+ as input. Defaults to None.
+ box_type_3d (str, optional): Type of 3D box of this dataset.
+ Based on the `box_type_3d`, the dataset will encapsulate the box
+ to its original format then converted them to `box_type_3d`.
+ Defaults to 'Depth' in this dataset. Available options includes
+
+ - 'LiDAR': Box in LiDAR coordinates.
+ - 'Depth': Box in depth coordinates, usually for indoor dataset.
+ - 'Camera': Box in camera coordinates.
+ filter_empty_gt (bool, optional): Whether to filter empty GT.
+ Defaults to True.
+ test_mode (bool, optional): Whether the dataset is in test mode.
+ Defaults to False.
+ """
+ CLASSES = ('bed', 'table', 'sofa', 'chair', 'toilet', 'desk', 'dresser',
+ 'night_stand', 'bookshelf', 'bathtub')
+
+ def __init__(self,
+ data_root,
+ ann_file,
+ pipeline=None,
+ classes=None,
+ modality=dict(use_camera=True, use_lidar=True),
+ box_type_3d='Depth',
+ filter_empty_gt=True,
+ test_mode=False,
+ **kwargs):
+ super().__init__(
+ data_root=data_root,
+ ann_file=ann_file,
+ pipeline=pipeline,
+ classes=classes,
+ modality=modality,
+ box_type_3d=box_type_3d,
+ filter_empty_gt=filter_empty_gt,
+ test_mode=test_mode,
+ **kwargs)
+ assert 'use_camera' in self.modality and \
+ 'use_lidar' in self.modality
+ assert self.modality['use_camera'] or self.modality['use_lidar']
+
+ def get_data_info(self, index):
+ """Get data info according to the given index.
+
+ Args:
+ index (int): Index of the sample data to get.
+
+ Returns:
+ dict: Data information that will be passed to the data
+ preprocessing pipelines. It includes the following keys:
+
+ - sample_idx (str): Sample index.
+ - pts_filename (str, optional): Filename of point clouds.
+ - file_name (str, optional): Filename of point clouds.
+ - img_prefix (str, optional): Prefix of image files.
+ - img_info (dict, optional): Image info.
+ - calib (dict, optional): Camera calibration info.
+ - ann_info (dict): Annotation info.
+ """
+ info = self.data_infos[index]
+ sample_idx = info['point_cloud']['lidar_idx']
+ assert info['point_cloud']['lidar_idx'] == info['image']['image_idx']
+ input_dict = dict(sample_idx=sample_idx)
+
+ if self.modality['use_lidar']:
+ pts_filename = osp.join(self.data_root, info['pts_path'])
+ input_dict['pts_filename'] = pts_filename
+ input_dict['file_name'] = pts_filename
+
+ if self.modality['use_camera']:
+ img_filename = osp.join(
+ osp.join(self.data_root, 'sunrgbd_trainval'),
+ info['image']['image_path'])
+ input_dict['img_prefix'] = None
+ input_dict['img_info'] = dict(filename=img_filename)
+ calib = info['calib']
+ rt_mat = calib['Rt']
+ # follow Coord3DMode.convert_point
+ rt_mat = np.array([[1, 0, 0], [0, 0, -1], [0, 1, 0]
+ ]) @ rt_mat.transpose(1, 0)
+ depth2img = calib['K'] @ rt_mat
+ input_dict['depth2img'] = depth2img
+
+ if not self.test_mode:
+ annos = self.get_ann_info(index)
+ input_dict['ann_info'] = annos
+ if self.filter_empty_gt and len(annos['gt_bboxes_3d']) == 0:
+ return None
+ return input_dict
+
+ def get_ann_info(self, index):
+ """Get annotation info according to the given index.
+
+ Args:
+ index (int): Index of the annotation data to get.
+
+ Returns:
+ dict: annotation information consists of the following keys:
+
+ - gt_bboxes_3d (:obj:`DepthInstance3DBoxes`):
+ 3D ground truth bboxes
+ - gt_labels_3d (np.ndarray): Labels of ground truths.
+ - pts_instance_mask_path (str): Path of instance masks.
+ - pts_semantic_mask_path (str): Path of semantic masks.
+ """
+ # Use index to get the annos, thus the evalhook could also use this api
+ info = self.data_infos[index]
+ if info['annos']['gt_num'] != 0:
+ gt_bboxes_3d = info['annos']['gt_boxes_upright_depth'].astype(
+ np.float32) # k, 6
+ gt_labels_3d = info['annos']['class'].astype(np.int64)
+ else:
+ gt_bboxes_3d = np.zeros((0, 7), dtype=np.float32)
+ gt_labels_3d = np.zeros((0, ), dtype=np.int64)
+
+ # to target box structure
+ gt_bboxes_3d = DepthInstance3DBoxes(
+ gt_bboxes_3d, origin=(0.5, 0.5, 0.5)).convert_to(self.box_mode_3d)
+
+ anns_results = dict(
+ gt_bboxes_3d=gt_bboxes_3d, gt_labels_3d=gt_labels_3d)
+
+ if self.modality['use_camera']:
+ if info['annos']['gt_num'] != 0:
+ gt_bboxes_2d = info['annos']['bbox'].astype(np.float32)
+ else:
+ gt_bboxes_2d = np.zeros((0, 4), dtype=np.float32)
+ anns_results['bboxes'] = gt_bboxes_2d
+ anns_results['labels'] = gt_labels_3d
+
+ return anns_results
+
+ def _build_default_pipeline(self):
+ """Build the default pipeline for this dataset."""
+ pipeline = [
+ dict(
+ type='LoadPointsFromFile',
+ coord_type='DEPTH',
+ shift_height=False,
+ load_dim=6,
+ use_dim=[0, 1, 2]),
+ dict(
+ type='DefaultFormatBundle3D',
+ class_names=self.CLASSES,
+ with_label=False),
+ dict(type='Collect3D', keys=['points'])
+ ]
+ if self.modality['use_camera']:
+ pipeline.insert(0, dict(type='LoadImageFromFile'))
+ return Compose(pipeline)
+
+ def show(self, results, out_dir, show=True, pipeline=None):
+ """Results visualization.
+
+ Args:
+ results (list[dict]): List of bounding boxes results.
+ out_dir (str): Output directory of visualization result.
+ show (bool): Visualize the results online.
+ pipeline (list[dict], optional): raw data loading for showing.
+ Default: None.
+ """
+ assert out_dir is not None, 'Expect out_dir, got none.'
+ pipeline = self._get_pipeline(pipeline)
+ for i, result in enumerate(results):
+ data_info = self.data_infos[i]
+ pts_path = data_info['pts_path']
+ file_name = osp.split(pts_path)[-1].split('.')[0]
+ points, img_metas, img = self._extract_data(
+ i, pipeline, ['points', 'img_metas', 'img'])
+ # scale colors to [0, 255]
+ points = points.numpy()
+ points[:, 3:] *= 255
+
+ gt_bboxes = self.get_ann_info(i)['gt_bboxes_3d'].tensor.numpy()
+ pred_bboxes = result['boxes_3d'].tensor.numpy()
+ show_result(points, gt_bboxes.copy(), pred_bboxes.copy(), out_dir,
+ file_name, show)
+
+ # multi-modality visualization
+ if self.modality['use_camera']:
+ img = img.numpy()
+ # need to transpose channel to first dim
+ img = img.transpose(1, 2, 0)
+ pred_bboxes = DepthInstance3DBoxes(
+ pred_bboxes, origin=(0.5, 0.5, 0))
+ gt_bboxes = DepthInstance3DBoxes(
+ gt_bboxes, origin=(0.5, 0.5, 0))
+ show_multi_modality_result(
+ img,
+ gt_bboxes,
+ pred_bboxes,
+ None,
+ out_dir,
+ file_name,
+ box_mode='depth',
+ img_metas=img_metas,
+ show=show)
+
+ def evaluate(self,
+ results,
+ metric=None,
+ iou_thr=(0.25, 0.5),
+ iou_thr_2d=(0.5, ),
+ logger=None,
+ show=False,
+ out_dir=None,
+ pipeline=None):
+ """Evaluate.
+
+ Evaluation in indoor protocol.
+
+ Args:
+ results (list[dict]): List of results.
+ metric (str | list[str], optional): Metrics to be evaluated.
+ Default: None.
+ iou_thr (list[float], optional): AP IoU thresholds for 3D
+ evaluation. Default: (0.25, 0.5).
+ iou_thr_2d (list[float], optional): AP IoU thresholds for 2D
+ evaluation. Default: (0.5, ).
+ show (bool, optional): Whether to visualize.
+ Default: False.
+ out_dir (str, optional): Path to save the visualization results.
+ Default: None.
+ pipeline (list[dict], optional): raw data loading for showing.
+ Default: None.
+
+ Returns:
+ dict: Evaluation results.
+ """
+ # evaluate 3D detection performance
+ if isinstance(results[0], dict):
+ return super().evaluate(results, metric, iou_thr, logger, show,
+ out_dir, pipeline)
+ # evaluate 2D detection performance
+ else:
+ eval_results = OrderedDict()
+ annotations = [self.get_ann_info(i) for i in range(len(self))]
+ iou_thr_2d = (iou_thr_2d) if isinstance(iou_thr_2d,
+ float) else iou_thr_2d
+ for iou_thr_2d_single in iou_thr_2d:
+ mean_ap, _ = eval_map(
+ results,
+ annotations,
+ scale_ranges=None,
+ iou_thr=iou_thr_2d_single,
+ dataset=self.CLASSES,
+ logger=logger)
+ eval_results['mAP_' + str(iou_thr_2d_single)] = mean_ap
+ return eval_results
diff --git a/mmdet3d/datasets/utils.py b/mmdet3d/datasets/utils.py
new file mode 100644
index 0000000..e9cfda1
--- /dev/null
+++ b/mmdet3d/datasets/utils.py
@@ -0,0 +1,140 @@
+# Copyright (c) OpenMMLab. All rights reserved.
+import mmcv
+
+# yapf: disable
+from mmdet3d.datasets.pipelines import (Collect3D, DefaultFormatBundle3D,
+ LoadAnnotations3D,
+ LoadImageFromFileMono3D,
+ LoadMultiViewImageFromFiles,
+ LoadPointsFromFile,
+ LoadPointsFromMultiSweeps,
+ MultiScaleFlipAug3D,
+ PointSegClassMapping)
+from mmdet.datasets.pipelines import LoadImageFromFile, MultiScaleFlipAug
+# yapf: enable
+from .builder import PIPELINES
+
+
+def is_loading_function(transform):
+ """Judge whether a transform function is a loading function.
+
+ Note: `MultiScaleFlipAug3D` is a wrapper for multiple pipeline functions,
+ so we need to search if its inner transforms contain any loading function.
+
+ Args:
+ transform (dict | :obj:`Pipeline`): A transform config or a function.
+
+ Returns:
+ bool: Whether it is a loading function. None means can't judge.
+ When transform is `MultiScaleFlipAug3D`, we return None.
+ """
+ # TODO: use more elegant way to distinguish loading modules
+ loading_functions = (LoadImageFromFile, LoadPointsFromFile,
+ LoadAnnotations3D, LoadMultiViewImageFromFiles,
+ LoadPointsFromMultiSweeps, DefaultFormatBundle3D,
+ Collect3D, LoadImageFromFileMono3D,
+ PointSegClassMapping)
+ if isinstance(transform, dict):
+ obj_cls = PIPELINES.get(transform['type'])
+ if obj_cls is None:
+ return False
+ if obj_cls in loading_functions:
+ return True
+ if obj_cls in (MultiScaleFlipAug3D, MultiScaleFlipAug):
+ return None
+ elif callable(transform):
+ if isinstance(transform, loading_functions):
+ return True
+ if isinstance(transform, (MultiScaleFlipAug3D, MultiScaleFlipAug)):
+ return None
+ return False
+
+
+def get_loading_pipeline(pipeline):
+ """Only keep loading image, points and annotations related configuration.
+
+ Args:
+ pipeline (list[dict] | list[:obj:`Pipeline`]):
+ Data pipeline configs or list of pipeline functions.
+
+ Returns:
+ list[dict] | list[:obj:`Pipeline`]): The new pipeline list with only
+ keep loading image, points and annotations related configuration.
+
+ Examples:
+ >>> pipelines = [
+ ... dict(type='LoadPointsFromFile',
+ ... coord_type='LIDAR', load_dim=4, use_dim=4),
+ ... dict(type='LoadImageFromFile'),
+ ... dict(type='LoadAnnotations3D',
+ ... with_bbox=True, with_label_3d=True),
+ ... dict(type='Resize',
+ ... img_scale=[(640, 192), (2560, 768)], keep_ratio=True),
+ ... dict(type='RandomFlip3D', flip_ratio_bev_horizontal=0.5),
+ ... dict(type='PointsRangeFilter',
+ ... point_cloud_range=point_cloud_range),
+ ... dict(type='ObjectRangeFilter',
+ ... point_cloud_range=point_cloud_range),
+ ... dict(type='PointShuffle'),
+ ... dict(type='Normalize', **img_norm_cfg),
+ ... dict(type='Pad', size_divisor=32),
+ ... dict(type='DefaultFormatBundle3D', class_names=class_names),
+ ... dict(type='Collect3D',
+ ... keys=['points', 'img', 'gt_bboxes_3d', 'gt_labels_3d'])
+ ... ]
+ >>> expected_pipelines = [
+ ... dict(type='LoadPointsFromFile',
+ ... coord_type='LIDAR', load_dim=4, use_dim=4),
+ ... dict(type='LoadImageFromFile'),
+ ... dict(type='LoadAnnotations3D',
+ ... with_bbox=True, with_label_3d=True),
+ ... dict(type='DefaultFormatBundle3D', class_names=class_names),
+ ... dict(type='Collect3D',
+ ... keys=['points', 'img', 'gt_bboxes_3d', 'gt_labels_3d'])
+ ... ]
+ >>> assert expected_pipelines == \
+ ... get_loading_pipeline(pipelines)
+ """
+ loading_pipeline = []
+ for transform in pipeline:
+ is_loading = is_loading_function(transform)
+ if is_loading is None: # MultiScaleFlipAug3D
+ # extract its inner pipeline
+ if isinstance(transform, dict):
+ inner_pipeline = transform.get('transforms', [])
+ else:
+ inner_pipeline = transform.transforms.transforms
+ loading_pipeline.extend(get_loading_pipeline(inner_pipeline))
+ elif is_loading:
+ loading_pipeline.append(transform)
+ assert len(loading_pipeline) > 0, \
+ 'The data pipeline in your config file must include ' \
+ 'loading step.'
+ return loading_pipeline
+
+
+def extract_result_dict(results, key):
+ """Extract and return the data corresponding to key in result dict.
+
+ ``results`` is a dict output from `pipeline(input_dict)`, which is the
+ loaded data from ``Dataset`` class.
+ The data terms inside may be wrapped in list, tuple and DataContainer, so
+ this function essentially extracts data from these wrappers.
+
+ Args:
+ results (dict): Data loaded using pipeline.
+ key (str): Key of the desired data.
+
+ Returns:
+ np.ndarray | torch.Tensor: Data term.
+ """
+ if key not in results.keys():
+ return None
+ # results[key] may be data or list[data] or tuple[data]
+ # data may be wrapped inside DataContainer
+ data = results[key]
+ if isinstance(data, (list, tuple)):
+ data = data[0]
+ if isinstance(data, mmcv.parallel.DataContainer):
+ data = data._data
+ return data
diff --git a/mmdet3d/datasets/waymo_dataset.py b/mmdet3d/datasets/waymo_dataset.py
new file mode 100644
index 0000000..6e204df
--- /dev/null
+++ b/mmdet3d/datasets/waymo_dataset.py
@@ -0,0 +1,549 @@
+# Copyright (c) OpenMMLab. All rights reserved.
+import os
+import tempfile
+from os import path as osp
+
+import mmcv
+import numpy as np
+import torch
+from mmcv.utils import print_log
+
+from ..core.bbox import Box3DMode, points_cam2img
+from .builder import DATASETS
+from .kitti_dataset import KittiDataset
+
+
+@DATASETS.register_module()
+class WaymoDataset(KittiDataset):
+ """Waymo Dataset.
+
+ This class serves as the API for experiments on the Waymo Dataset.
+
+ Please refer to ``_for data downloading.
+ It is recommended to symlink the dataset root to $MMDETECTION3D/data and
+ organize them as the doc shows.
+
+ Args:
+ data_root (str): Path of dataset root.
+ ann_file (str): Path of annotation file.
+ split (str): Split of input data.
+ pts_prefix (str, optional): Prefix of points files.
+ Defaults to 'velodyne'.
+ pipeline (list[dict], optional): Pipeline used for data processing.
+ Defaults to None.
+ classes (tuple[str], optional): Classes used in the dataset.
+ Defaults to None.
+ modality (dict, optional): Modality to specify the sensor data used
+ as input. Defaults to None.
+ box_type_3d (str, optional): Type of 3D box of this dataset.
+ Based on the `box_type_3d`, the dataset will encapsulate the box
+ to its original format then converted them to `box_type_3d`.
+ Defaults to 'LiDAR' in this dataset. Available options includes
+
+ - 'LiDAR': box in LiDAR coordinates
+ - 'Depth': box in depth coordinates, usually for indoor dataset
+ - 'Camera': box in camera coordinates
+ filter_empty_gt (bool, optional): Whether to filter empty GT.
+ Defaults to True.
+ test_mode (bool, optional): Whether the dataset is in test mode.
+ Defaults to False.
+ pcd_limit_range (list(float), optional): The range of point cloud used
+ to filter invalid predicted boxes.
+ Default: [-85, -85, -5, 85, 85, 5].
+ """
+
+ CLASSES = ('Car', 'Cyclist', 'Pedestrian')
+
+ def __init__(self,
+ data_root,
+ ann_file,
+ split,
+ pts_prefix='velodyne',
+ pipeline=None,
+ classes=None,
+ modality=None,
+ box_type_3d='LiDAR',
+ filter_empty_gt=True,
+ test_mode=False,
+ load_interval=1,
+ pcd_limit_range=[-85, -85, -5, 85, 85, 5],
+ **kwargs):
+ super().__init__(
+ data_root=data_root,
+ ann_file=ann_file,
+ split=split,
+ pts_prefix=pts_prefix,
+ pipeline=pipeline,
+ classes=classes,
+ modality=modality,
+ box_type_3d=box_type_3d,
+ filter_empty_gt=filter_empty_gt,
+ test_mode=test_mode,
+ pcd_limit_range=pcd_limit_range,
+ **kwargs)
+
+ # to load a subset, just set the load_interval in the dataset config
+ self.data_infos = self.data_infos[::load_interval]
+ if hasattr(self, 'flag'):
+ self.flag = self.flag[::load_interval]
+
+ def _get_pts_filename(self, idx):
+ pts_filename = osp.join(self.root_split, self.pts_prefix,
+ f'{idx:07d}.bin')
+ return pts_filename
+
+ def get_data_info(self, index):
+ """Get data info according to the given index.
+
+ Args:
+ index (int): Index of the sample data to get.
+
+ Returns:
+ dict: Standard input_dict consists of the
+ data information.
+
+ - sample_idx (str): sample index
+ - pts_filename (str): filename of point clouds
+ - img_prefix (str): prefix of image files
+ - img_info (dict): image info
+ - lidar2img (list[np.ndarray], optional): transformations from
+ lidar to different cameras
+ - ann_info (dict): annotation info
+ """
+ info = self.data_infos[index]
+ sample_idx = info['image']['image_idx']
+ img_filename = os.path.join(self.data_root,
+ info['image']['image_path'])
+
+ # TODO: consider use torch.Tensor only
+ rect = info['calib']['R0_rect'].astype(np.float32)
+ Trv2c = info['calib']['Tr_velo_to_cam'].astype(np.float32)
+ P0 = info['calib']['P0'].astype(np.float32)
+ lidar2img = P0 @ rect @ Trv2c
+
+ pts_filename = self._get_pts_filename(sample_idx)
+ input_dict = dict(
+ sample_idx=sample_idx,
+ pts_filename=pts_filename,
+ img_prefix=None,
+ img_info=dict(filename=img_filename),
+ lidar2img=lidar2img)
+
+ if not self.test_mode:
+ annos = self.get_ann_info(index)
+ input_dict['ann_info'] = annos
+
+ return input_dict
+
+ def format_results(self,
+ outputs,
+ pklfile_prefix=None,
+ submission_prefix=None,
+ data_format='waymo'):
+ """Format the results to pkl file.
+
+ Args:
+ outputs (list[dict]): Testing results of the dataset.
+ pklfile_prefix (str): The prefix of pkl files. It includes
+ the file path and the prefix of filename, e.g., "a/b/prefix".
+ If not specified, a temp file will be created. Default: None.
+ submission_prefix (str): The prefix of submitted files. It
+ includes the file path and the prefix of filename, e.g.,
+ "a/b/prefix". If not specified, a temp file will be created.
+ Default: None.
+ data_format (str, optional): Output data format.
+ Default: 'waymo'. Another supported choice is 'kitti'.
+
+ Returns:
+ tuple: (result_files, tmp_dir), result_files is a dict containing
+ the json filepaths, tmp_dir is the temporal directory created
+ for saving json files when jsonfile_prefix is not specified.
+ """
+ if pklfile_prefix is None:
+ tmp_dir = tempfile.TemporaryDirectory()
+ pklfile_prefix = osp.join(tmp_dir.name, 'results')
+ else:
+ tmp_dir = None
+
+ assert ('waymo' in data_format or 'kitti' in data_format), \
+ f'invalid data_format {data_format}'
+
+ if (not isinstance(outputs[0], dict)) or 'img_bbox' in outputs[0]:
+ raise TypeError('Not supported type for reformat results.')
+ elif 'pts_bbox' in outputs[0]:
+ result_files = dict()
+ for name in outputs[0]:
+ results_ = [out[name] for out in outputs]
+ pklfile_prefix_ = pklfile_prefix + name
+ if submission_prefix is not None:
+ submission_prefix_ = f'{submission_prefix}_{name}'
+ else:
+ submission_prefix_ = None
+ result_files_ = self.bbox2result_kitti(results_, self.CLASSES,
+ pklfile_prefix_,
+ submission_prefix_)
+ result_files[name] = result_files_
+ else:
+ result_files = self.bbox2result_kitti(outputs, self.CLASSES,
+ pklfile_prefix,
+ submission_prefix)
+ if 'waymo' in data_format:
+ from ..core.evaluation.waymo_utils.prediction_kitti_to_waymo import \
+ KITTI2Waymo # noqa
+ waymo_root = osp.join(
+ self.data_root.split('kitti_format')[0], 'waymo_format')
+ if self.split == 'training':
+ waymo_tfrecords_dir = osp.join(waymo_root, 'validation')
+ prefix = '1'
+ elif self.split == 'testing':
+ waymo_tfrecords_dir = osp.join(waymo_root, 'testing')
+ prefix = '2'
+ else:
+ raise ValueError('Not supported split value.')
+ save_tmp_dir = tempfile.TemporaryDirectory()
+ waymo_results_save_dir = save_tmp_dir.name
+ waymo_results_final_path = f'{pklfile_prefix}.bin'
+ if 'pts_bbox' in result_files:
+ converter = KITTI2Waymo(result_files['pts_bbox'],
+ waymo_tfrecords_dir,
+ waymo_results_save_dir,
+ waymo_results_final_path, prefix)
+ else:
+ converter = KITTI2Waymo(result_files, waymo_tfrecords_dir,
+ waymo_results_save_dir,
+ waymo_results_final_path, prefix)
+ converter.convert()
+ save_tmp_dir.cleanup()
+
+ return result_files, tmp_dir
+
+ def evaluate(self,
+ results,
+ metric='waymo',
+ logger=None,
+ pklfile_prefix=None,
+ submission_prefix=None,
+ show=False,
+ out_dir=None,
+ pipeline=None):
+ """Evaluation in KITTI protocol.
+
+ Args:
+ results (list[dict]): Testing results of the dataset.
+ metric (str | list[str], optional): Metrics to be evaluated.
+ Default: 'waymo'. Another supported metric is 'kitti'.
+ logger (logging.Logger | str, optional): Logger used for printing
+ related information during evaluation. Default: None.
+ pklfile_prefix (str, optional): The prefix of pkl files including
+ the file path and the prefix of filename, e.g., "a/b/prefix".
+ If not specified, a temp file will be created. Default: None.
+ submission_prefix (str, optional): The prefix of submission data.
+ If not specified, the submission data will not be generated.
+ show (bool, optional): Whether to visualize.
+ Default: False.
+ out_dir (str, optional): Path to save the visualization results.
+ Default: None.
+ pipeline (list[dict], optional): raw data loading for showing.
+ Default: None.
+
+ Returns:
+ dict[str: float]: results of each evaluation metric
+ """
+ assert ('waymo' in metric or 'kitti' in metric), \
+ f'invalid metric {metric}'
+ if 'kitti' in metric:
+ result_files, tmp_dir = self.format_results(
+ results,
+ pklfile_prefix,
+ submission_prefix,
+ data_format='kitti')
+ from mmdet3d.core.evaluation import kitti_eval
+ gt_annos = [info['annos'] for info in self.data_infos]
+
+ if isinstance(result_files, dict):
+ ap_dict = dict()
+ for name, result_files_ in result_files.items():
+ eval_types = ['bev', '3d']
+ ap_result_str, ap_dict_ = kitti_eval(
+ gt_annos,
+ result_files_,
+ self.CLASSES,
+ eval_types=eval_types)
+ for ap_type, ap in ap_dict_.items():
+ ap_dict[f'{name}/{ap_type}'] = float(
+ '{:.4f}'.format(ap))
+
+ print_log(
+ f'Results of {name}:\n' + ap_result_str, logger=logger)
+
+ else:
+ ap_result_str, ap_dict = kitti_eval(
+ gt_annos,
+ result_files,
+ self.CLASSES,
+ eval_types=['bev', '3d'])
+ print_log('\n' + ap_result_str, logger=logger)
+ if 'waymo' in metric:
+ waymo_root = osp.join(
+ self.data_root.split('kitti_format')[0], 'waymo_format')
+ if pklfile_prefix is None:
+ eval_tmp_dir = tempfile.TemporaryDirectory()
+ pklfile_prefix = osp.join(eval_tmp_dir.name, 'results')
+ else:
+ eval_tmp_dir = None
+ result_files, tmp_dir = self.format_results(
+ results,
+ pklfile_prefix,
+ submission_prefix,
+ data_format='waymo')
+ import subprocess
+ ret_bytes = subprocess.check_output(
+ 'mmdet3d/core/evaluation/waymo_utils/' +
+ f'compute_detection_metrics_main {pklfile_prefix}.bin ' +
+ f'{waymo_root}/gt.bin',
+ shell=True)
+ ret_texts = ret_bytes.decode('utf-8')
+ print_log(ret_texts)
+ # parse the text to get ap_dict
+ ap_dict = {
+ 'Vehicle/L1 mAP': 0,
+ 'Vehicle/L1 mAPH': 0,
+ 'Vehicle/L2 mAP': 0,
+ 'Vehicle/L2 mAPH': 0,
+ 'Pedestrian/L1 mAP': 0,
+ 'Pedestrian/L1 mAPH': 0,
+ 'Pedestrian/L2 mAP': 0,
+ 'Pedestrian/L2 mAPH': 0,
+ 'Sign/L1 mAP': 0,
+ 'Sign/L1 mAPH': 0,
+ 'Sign/L2 mAP': 0,
+ 'Sign/L2 mAPH': 0,
+ 'Cyclist/L1 mAP': 0,
+ 'Cyclist/L1 mAPH': 0,
+ 'Cyclist/L2 mAP': 0,
+ 'Cyclist/L2 mAPH': 0,
+ 'Overall/L1 mAP': 0,
+ 'Overall/L1 mAPH': 0,
+ 'Overall/L2 mAP': 0,
+ 'Overall/L2 mAPH': 0
+ }
+ mAP_splits = ret_texts.split('mAP ')
+ mAPH_splits = ret_texts.split('mAPH ')
+ for idx, key in enumerate(ap_dict.keys()):
+ split_idx = int(idx / 2) + 1
+ if idx % 2 == 0: # mAP
+ ap_dict[key] = float(mAP_splits[split_idx].split(']')[0])
+ else: # mAPH
+ ap_dict[key] = float(mAPH_splits[split_idx].split(']')[0])
+ ap_dict['Overall/L1 mAP'] = \
+ (ap_dict['Vehicle/L1 mAP'] + ap_dict['Pedestrian/L1 mAP'] +
+ ap_dict['Cyclist/L1 mAP']) / 3
+ ap_dict['Overall/L1 mAPH'] = \
+ (ap_dict['Vehicle/L1 mAPH'] + ap_dict['Pedestrian/L1 mAPH'] +
+ ap_dict['Cyclist/L1 mAPH']) / 3
+ ap_dict['Overall/L2 mAP'] = \
+ (ap_dict['Vehicle/L2 mAP'] + ap_dict['Pedestrian/L2 mAP'] +
+ ap_dict['Cyclist/L2 mAP']) / 3
+ ap_dict['Overall/L2 mAPH'] = \
+ (ap_dict['Vehicle/L2 mAPH'] + ap_dict['Pedestrian/L2 mAPH'] +
+ ap_dict['Cyclist/L2 mAPH']) / 3
+ if eval_tmp_dir is not None:
+ eval_tmp_dir.cleanup()
+
+ if tmp_dir is not None:
+ tmp_dir.cleanup()
+
+ if show or out_dir:
+ self.show(results, out_dir, show=show, pipeline=pipeline)
+ return ap_dict
+
+ def bbox2result_kitti(self,
+ net_outputs,
+ class_names,
+ pklfile_prefix=None,
+ submission_prefix=None):
+ """Convert results to kitti format for evaluation and test submission.
+
+ Args:
+ net_outputs (List[np.ndarray]): list of array storing the
+ bbox and score
+ class_nanes (List[String]): A list of class names
+ pklfile_prefix (str): The prefix of pkl file.
+ submission_prefix (str): The prefix of submission file.
+
+ Returns:
+ List[dict]: A list of dict have the kitti 3d format
+ """
+ assert len(net_outputs) == len(self.data_infos), \
+ 'invalid list length of network outputs'
+ if submission_prefix is not None:
+ mmcv.mkdir_or_exist(submission_prefix)
+
+ det_annos = []
+ print('\nConverting prediction to KITTI format')
+ for idx, pred_dicts in enumerate(
+ mmcv.track_iter_progress(net_outputs)):
+ annos = []
+ info = self.data_infos[idx]
+ sample_idx = info['image']['image_idx']
+ image_shape = info['image']['image_shape'][:2]
+
+ box_dict = self.convert_valid_bboxes(pred_dicts, info)
+ if len(box_dict['bbox']) > 0:
+ box_2d_preds = box_dict['bbox']
+ box_preds = box_dict['box3d_camera']
+ scores = box_dict['scores']
+ box_preds_lidar = box_dict['box3d_lidar']
+ label_preds = box_dict['label_preds']
+
+ anno = {
+ 'name': [],
+ 'truncated': [],
+ 'occluded': [],
+ 'alpha': [],
+ 'bbox': [],
+ 'dimensions': [],
+ 'location': [],
+ 'rotation_y': [],
+ 'score': []
+ }
+
+ for box, box_lidar, bbox, score, label in zip(
+ box_preds, box_preds_lidar, box_2d_preds, scores,
+ label_preds):
+ bbox[2:] = np.minimum(bbox[2:], image_shape[::-1])
+ bbox[:2] = np.maximum(bbox[:2], [0, 0])
+ anno['name'].append(class_names[int(label)])
+ anno['truncated'].append(0.0)
+ anno['occluded'].append(0)
+ anno['alpha'].append(
+ -np.arctan2(-box_lidar[1], box_lidar[0]) + box[6])
+ anno['bbox'].append(bbox)
+ anno['dimensions'].append(box[3:6])
+ anno['location'].append(box[:3])
+ anno['rotation_y'].append(box[6])
+ anno['score'].append(score)
+
+ anno = {k: np.stack(v) for k, v in anno.items()}
+ annos.append(anno)
+
+ if submission_prefix is not None:
+ curr_file = f'{submission_prefix}/{sample_idx:07d}.txt'
+ with open(curr_file, 'w') as f:
+ bbox = anno['bbox']
+ loc = anno['location']
+ dims = anno['dimensions'] # lhw -> hwl
+
+ for idx in range(len(bbox)):
+ print(
+ '{} -1 -1 {:.4f} {:.4f} {:.4f} {:.4f} '
+ '{:.4f} {:.4f} {:.4f} '
+ '{:.4f} {:.4f} {:.4f} {:.4f} {:.4f} {:.4f}'.
+ format(anno['name'][idx], anno['alpha'][idx],
+ bbox[idx][0], bbox[idx][1],
+ bbox[idx][2], bbox[idx][3],
+ dims[idx][1], dims[idx][2],
+ dims[idx][0], loc[idx][0], loc[idx][1],
+ loc[idx][2], anno['rotation_y'][idx],
+ anno['score'][idx]),
+ file=f)
+ else:
+ annos.append({
+ 'name': np.array([]),
+ 'truncated': np.array([]),
+ 'occluded': np.array([]),
+ 'alpha': np.array([]),
+ 'bbox': np.zeros([0, 4]),
+ 'dimensions': np.zeros([0, 3]),
+ 'location': np.zeros([0, 3]),
+ 'rotation_y': np.array([]),
+ 'score': np.array([]),
+ })
+ annos[-1]['sample_idx'] = np.array(
+ [sample_idx] * len(annos[-1]['score']), dtype=np.int64)
+
+ det_annos += annos
+
+ if pklfile_prefix is not None:
+ if not pklfile_prefix.endswith(('.pkl', '.pickle')):
+ out = f'{pklfile_prefix}.pkl'
+ mmcv.dump(det_annos, out)
+ print(f'Result is saved to {out}.')
+
+ return det_annos
+
+ def convert_valid_bboxes(self, box_dict, info):
+ """Convert the boxes into valid format.
+
+ Args:
+ box_dict (dict): Bounding boxes to be converted.
+
+ - boxes_3d (:obj:``LiDARInstance3DBoxes``): 3D bounding boxes.
+ - scores_3d (np.ndarray): Scores of predicted boxes.
+ - labels_3d (np.ndarray): Class labels of predicted boxes.
+ info (dict): Dataset information dictionary.
+
+ Returns:
+ dict: Valid boxes after conversion.
+
+ - bbox (np.ndarray): 2D bounding boxes (in camera 0).
+ - box3d_camera (np.ndarray): 3D boxes in camera coordinates.
+ - box3d_lidar (np.ndarray): 3D boxes in lidar coordinates.
+ - scores (np.ndarray): Scores of predicted boxes.
+ - label_preds (np.ndarray): Class labels of predicted boxes.
+ - sample_idx (np.ndarray): Sample index.
+ """
+ # TODO: refactor this function
+ box_preds = box_dict['boxes_3d']
+ scores = box_dict['scores_3d']
+ labels = box_dict['labels_3d']
+ sample_idx = info['image']['image_idx']
+ box_preds.limit_yaw(offset=0.5, period=np.pi * 2)
+
+ if len(box_preds) == 0:
+ return dict(
+ bbox=np.zeros([0, 4]),
+ box3d_camera=np.zeros([0, 7]),
+ box3d_lidar=np.zeros([0, 7]),
+ scores=np.zeros([0]),
+ label_preds=np.zeros([0, 4]),
+ sample_idx=sample_idx)
+
+ rect = info['calib']['R0_rect'].astype(np.float32)
+ Trv2c = info['calib']['Tr_velo_to_cam'].astype(np.float32)
+ P0 = info['calib']['P0'].astype(np.float32)
+ P0 = box_preds.tensor.new_tensor(P0)
+
+ box_preds_camera = box_preds.convert_to(Box3DMode.CAM, rect @ Trv2c)
+
+ box_corners = box_preds_camera.corners
+ box_corners_in_image = points_cam2img(box_corners, P0)
+ # box_corners_in_image: [N, 8, 2]
+ minxy = torch.min(box_corners_in_image, dim=1)[0]
+ maxxy = torch.max(box_corners_in_image, dim=1)[0]
+ box_2d_preds = torch.cat([minxy, maxxy], dim=1)
+ # Post-processing
+ # check box_preds
+ limit_range = box_preds.tensor.new_tensor(self.pcd_limit_range)
+ valid_pcd_inds = ((box_preds.center > limit_range[:3]) &
+ (box_preds.center < limit_range[3:]))
+ valid_inds = valid_pcd_inds.all(-1)
+
+ if valid_inds.sum() > 0:
+ return dict(
+ bbox=box_2d_preds[valid_inds, :].numpy(),
+ box3d_camera=box_preds_camera[valid_inds].tensor.numpy(),
+ box3d_lidar=box_preds[valid_inds].tensor.numpy(),
+ scores=scores[valid_inds].numpy(),
+ label_preds=labels[valid_inds].numpy(),
+ sample_idx=sample_idx,
+ )
+ else:
+ return dict(
+ bbox=np.zeros([0, 4]),
+ box3d_camera=np.zeros([0, 7]),
+ box3d_lidar=np.zeros([0, 7]),
+ scores=np.zeros([0]),
+ label_preds=np.zeros([0, 4]),
+ sample_idx=sample_idx,
+ )
diff --git a/mmdet3d/models/__init__.py b/mmdet3d/models/__init__.py
new file mode 100644
index 0000000..7c7e8fc
--- /dev/null
+++ b/mmdet3d/models/__init__.py
@@ -0,0 +1,29 @@
+# Copyright (c) OpenMMLab. All rights reserved.
+from .backbones import * # noqa: F401,F403
+from .builder import (BACKBONES, DETECTORS, FUSION_LAYERS, HEADS, LOSSES,
+ MIDDLE_ENCODERS, NECKS, ROI_EXTRACTORS, SEGMENTORS,
+ SHARED_HEADS, VOXEL_ENCODERS, build_backbone,
+ build_detector, build_fusion_layer, build_head,
+ build_loss, build_middle_encoder, build_model,
+ build_neck, build_roi_extractor, build_shared_head,
+ build_voxel_encoder)
+from .decode_heads import * # noqa: F401,F403
+from .dense_heads import * # noqa: F401,F403
+from .detectors import * # noqa: F401,F403
+from .fusion_layers import * # noqa: F401,F403
+from .losses import * # noqa: F401,F403
+from .middle_encoders import * # noqa: F401,F403
+from .model_utils import * # noqa: F401,F403
+from .necks import * # noqa: F401,F403
+from .roi_heads import * # noqa: F401,F403
+from .segmentors import * # noqa: F401,F403
+from .voxel_encoders import * # noqa: F401,F403
+
+__all__ = [
+ 'BACKBONES', 'NECKS', 'ROI_EXTRACTORS', 'SHARED_HEADS', 'HEADS', 'LOSSES',
+ 'DETECTORS', 'SEGMENTORS', 'VOXEL_ENCODERS', 'MIDDLE_ENCODERS',
+ 'FUSION_LAYERS', 'build_backbone', 'build_neck', 'build_roi_extractor',
+ 'build_shared_head', 'build_head', 'build_loss', 'build_detector',
+ 'build_fusion_layer', 'build_model', 'build_middle_encoder',
+ 'build_voxel_encoder'
+]
diff --git a/mmdet3d/models/backbones/__init__.py b/mmdet3d/models/backbones/__init__.py
new file mode 100644
index 0000000..79a4b78
--- /dev/null
+++ b/mmdet3d/models/backbones/__init__.py
@@ -0,0 +1,18 @@
+# Copyright (c) OpenMMLab. All rights reserved.
+from mmdet.models.backbones import SSDVGG, HRNet, ResNet, ResNetV1d, ResNeXt
+from .dgcnn import DGCNNBackbone
+from .dla import DLANet
+from .mink_resnet import MinkResNet
+from .multi_backbone import MultiBackbone
+from .nostem_regnet import NoStemRegNet
+from .pointnet2_sa_msg import PointNet2SAMSG
+from .pointnet2_sa_ssg import PointNet2SASSG
+from .second import SECOND
+from .mink_unet import CustomUNet, MinkUNet14A, MinkUNet14B, MinkUNet14C, MinkUNet14D
+
+__all__ = [
+ 'ResNet', 'ResNetV1d', 'ResNeXt', 'SSDVGG', 'HRNet', 'NoStemRegNet',
+ 'SECOND', 'DGCNNBackbone', 'PointNet2SASSG', 'PointNet2SAMSG',
+ 'MultiBackbone', 'DLANet', 'CustomUNet', 'MinkResNet', 'MinkUNet14A', 'MinkUNet14B',
+ 'MinkUNet14C', 'MinkUNet14D'
+]
diff --git a/mmdet3d/models/backbones/base_pointnet.py b/mmdet3d/models/backbones/base_pointnet.py
new file mode 100644
index 0000000..31439e6
--- /dev/null
+++ b/mmdet3d/models/backbones/base_pointnet.py
@@ -0,0 +1,39 @@
+# Copyright (c) OpenMMLab. All rights reserved.
+import warnings
+from abc import ABCMeta
+
+from mmcv.runner import BaseModule
+
+
+class BasePointNet(BaseModule, metaclass=ABCMeta):
+ """Base class for PointNet."""
+
+ def __init__(self, init_cfg=None, pretrained=None):
+ super(BasePointNet, self).__init__(init_cfg)
+ self.fp16_enabled = False
+ assert not (init_cfg and pretrained), \
+ 'init_cfg and pretrained cannot be setting at the same time'
+ if isinstance(pretrained, str):
+ warnings.warn('DeprecationWarning: pretrained is a deprecated, '
+ 'please use "init_cfg" instead')
+ self.init_cfg = dict(type='Pretrained', checkpoint=pretrained)
+
+ @staticmethod
+ def _split_point_feats(points):
+ """Split coordinates and features of input points.
+
+ Args:
+ points (torch.Tensor): Point coordinates with features,
+ with shape (B, N, 3 + input_feature_dim).
+
+ Returns:
+ torch.Tensor: Coordinates of input points.
+ torch.Tensor: Features of input points.
+ """
+ xyz = points[..., 0:3].contiguous()
+ if points.size(-1) > 3:
+ features = points[..., 3:].transpose(1, 2).contiguous()
+ else:
+ features = None
+
+ return xyz, features
diff --git a/mmdet3d/models/backbones/dgcnn.py b/mmdet3d/models/backbones/dgcnn.py
new file mode 100644
index 0000000..20e82d9
--- /dev/null
+++ b/mmdet3d/models/backbones/dgcnn.py
@@ -0,0 +1,98 @@
+# Copyright (c) OpenMMLab. All rights reserved.
+from mmcv.runner import BaseModule, auto_fp16
+from torch import nn as nn
+
+from mmdet3d.ops import DGCNNFAModule, DGCNNGFModule
+from ..builder import BACKBONES
+
+
+@BACKBONES.register_module()
+class DGCNNBackbone(BaseModule):
+ """Backbone network for DGCNN.
+
+ Args:
+ in_channels (int): Input channels of point cloud.
+ num_samples (tuple[int], optional): The number of samples for knn or
+ ball query in each graph feature (GF) module.
+ Defaults to (20, 20, 20).
+ knn_modes (tuple[str], optional): Mode of KNN of each knn module.
+ Defaults to ('D-KNN', 'F-KNN', 'F-KNN').
+ radius (tuple[float], optional): Sampling radii of each GF module.
+ Defaults to (None, None, None).
+ gf_channels (tuple[tuple[int]], optional): Out channels of each mlp in
+ GF module. Defaults to ((64, 64), (64, 64), (64, )).
+ fa_channels (tuple[int], optional): Out channels of each mlp in FA
+ module. Defaults to (1024, ).
+ act_cfg (dict, optional): Config of activation layer.
+ Defaults to dict(type='ReLU').
+ init_cfg (dict, optional): Initialization config.
+ Defaults to None.
+ """
+
+ def __init__(self,
+ in_channels,
+ num_samples=(20, 20, 20),
+ knn_modes=('D-KNN', 'F-KNN', 'F-KNN'),
+ radius=(None, None, None),
+ gf_channels=((64, 64), (64, 64), (64, )),
+ fa_channels=(1024, ),
+ act_cfg=dict(type='ReLU'),
+ init_cfg=None):
+ super().__init__(init_cfg=init_cfg)
+ self.num_gf = len(gf_channels)
+
+ assert len(num_samples) == len(knn_modes) == len(radius) == len(
+ gf_channels), 'Num_samples, knn_modes, radius and gf_channels \
+ should have the same length.'
+
+ self.GF_modules = nn.ModuleList()
+ gf_in_channel = in_channels * 2
+ skip_channel_list = [gf_in_channel] # input channel list
+
+ for gf_index in range(self.num_gf):
+ cur_gf_mlps = list(gf_channels[gf_index])
+ cur_gf_mlps = [gf_in_channel] + cur_gf_mlps
+ gf_out_channel = cur_gf_mlps[-1]
+
+ self.GF_modules.append(
+ DGCNNGFModule(
+ mlp_channels=cur_gf_mlps,
+ num_sample=num_samples[gf_index],
+ knn_mode=knn_modes[gf_index],
+ radius=radius[gf_index],
+ act_cfg=act_cfg))
+ skip_channel_list.append(gf_out_channel)
+ gf_in_channel = gf_out_channel * 2
+
+ fa_in_channel = sum(skip_channel_list[1:])
+ cur_fa_mlps = list(fa_channels)
+ cur_fa_mlps = [fa_in_channel] + cur_fa_mlps
+
+ self.FA_module = DGCNNFAModule(
+ mlp_channels=cur_fa_mlps, act_cfg=act_cfg)
+
+ @auto_fp16(apply_to=('points', ))
+ def forward(self, points):
+ """Forward pass.
+
+ Args:
+ points (torch.Tensor): point coordinates with features,
+ with shape (B, N, in_channels).
+
+ Returns:
+ dict[str, list[torch.Tensor]]: Outputs after graph feature (GF) and
+ feature aggregation (FA) modules.
+
+ - gf_points (list[torch.Tensor]): Outputs after each GF module.
+ - fa_points (torch.Tensor): Outputs after FA module.
+ """
+ gf_points = [points]
+
+ for i in range(self.num_gf):
+ cur_points = self.GF_modules[i](gf_points[i])
+ gf_points.append(cur_points)
+
+ fa_points = self.FA_module(gf_points)
+
+ out = dict(gf_points=gf_points, fa_points=fa_points)
+ return out
diff --git a/mmdet3d/models/backbones/dla.py b/mmdet3d/models/backbones/dla.py
new file mode 100644
index 0000000..a547909
--- /dev/null
+++ b/mmdet3d/models/backbones/dla.py
@@ -0,0 +1,446 @@
+# Copyright (c) OpenMMLab. All rights reserved.
+import warnings
+
+import torch
+from mmcv.cnn import build_conv_layer, build_norm_layer
+from mmcv.runner import BaseModule
+from torch import nn
+
+from ..builder import BACKBONES
+
+
+def dla_build_norm_layer(cfg, num_features):
+ """Build normalization layer specially designed for DLANet.
+
+ Args:
+ cfg (dict): The norm layer config, which should contain:
+
+ - type (str): Layer type.
+ - layer args: Args needed to instantiate a norm layer.
+ - requires_grad (bool, optional): Whether stop gradient updates.
+ num_features (int): Number of input channels.
+
+
+ Returns:
+ Function: Build normalization layer in mmcv.
+ """
+ cfg_ = cfg.copy()
+ if cfg_['type'] == 'GN':
+ if num_features % 32 == 0:
+ return build_norm_layer(cfg_, num_features)
+ else:
+ assert 'num_groups' in cfg_
+ cfg_['num_groups'] = cfg_['num_groups'] // 2
+ return build_norm_layer(cfg_, num_features)
+ else:
+ return build_norm_layer(cfg_, num_features)
+
+
+class BasicBlock(BaseModule):
+ """BasicBlock in DLANet.
+
+ Args:
+ in_channels (int): Input feature channel.
+ out_channels (int): Output feature channel.
+ norm_cfg (dict): Dictionary to construct and config
+ norm layer.
+ conv_cfg (dict): Dictionary to construct and config
+ conv layer.
+ stride (int, optional): Conv stride. Default: 1.
+ dilation (int, optional): Conv dilation. Default: 1.
+ init_cfg (dict, optional): Initialization config.
+ Default: None.
+ """
+
+ def __init__(self,
+ in_channels,
+ out_channels,
+ norm_cfg,
+ conv_cfg,
+ stride=1,
+ dilation=1,
+ init_cfg=None):
+ super(BasicBlock, self).__init__(init_cfg)
+ self.conv1 = build_conv_layer(
+ conv_cfg,
+ in_channels,
+ out_channels,
+ 3,
+ stride=stride,
+ padding=dilation,
+ dilation=dilation,
+ bias=False)
+ self.norm1 = dla_build_norm_layer(norm_cfg, out_channels)[1]
+ self.relu = nn.ReLU(inplace=True)
+ self.conv2 = build_conv_layer(
+ conv_cfg,
+ out_channels,
+ out_channels,
+ 3,
+ stride=1,
+ padding=dilation,
+ dilation=dilation,
+ bias=False)
+ self.norm2 = dla_build_norm_layer(norm_cfg, out_channels)[1]
+ self.stride = stride
+
+ def forward(self, x, identity=None):
+ """Forward function."""
+
+ if identity is None:
+ identity = x
+ out = self.conv1(x)
+ out = self.norm1(out)
+ out = self.relu(out)
+ out = self.conv2(out)
+ out = self.norm2(out)
+ out += identity
+ out = self.relu(out)
+
+ return out
+
+
+class Root(BaseModule):
+ """Root in DLANet.
+
+ Args:
+ in_channels (int): Input feature channel.
+ out_channels (int): Output feature channel.
+ norm_cfg (dict): Dictionary to construct and config
+ norm layer.
+ conv_cfg (dict): Dictionary to construct and config
+ conv layer.
+ kernel_size (int): Size of convolution kernel.
+ add_identity (bool): Whether to add identity in root.
+ init_cfg (dict, optional): Initialization config.
+ Default: None.
+ """
+
+ def __init__(self,
+ in_channels,
+ out_channels,
+ norm_cfg,
+ conv_cfg,
+ kernel_size,
+ add_identity,
+ init_cfg=None):
+ super(Root, self).__init__(init_cfg)
+ self.conv = build_conv_layer(
+ conv_cfg,
+ in_channels,
+ out_channels,
+ 1,
+ stride=1,
+ padding=(kernel_size - 1) // 2,
+ bias=False)
+ self.norm = dla_build_norm_layer(norm_cfg, out_channels)[1]
+ self.relu = nn.ReLU(inplace=True)
+ self.add_identity = add_identity
+
+ def forward(self, feat_list):
+ """Forward function.
+
+ Args:
+ feat_list (list[torch.Tensor]): Output features from
+ multiple layers.
+ """
+ children = feat_list
+ x = self.conv(torch.cat(feat_list, 1))
+ x = self.norm(x)
+ if self.add_identity:
+ x += children[0]
+ x = self.relu(x)
+
+ return x
+
+
+class Tree(BaseModule):
+ """Tree in DLANet.
+
+ Args:
+ levels (int): The level of the tree.
+ block (nn.Module): The block module in tree.
+ in_channels: Input feature channel.
+ out_channels: Output feature channel.
+ norm_cfg (dict): Dictionary to construct and config
+ norm layer.
+ conv_cfg (dict): Dictionary to construct and config
+ conv layer.
+ stride (int, optional): Convolution stride.
+ Default: 1.
+ level_root (bool, optional): whether belongs to the
+ root layer.
+ root_dim (int, optional): Root input feature channel.
+ root_kernel_size (int, optional): Size of root
+ convolution kernel. Default: 1.
+ dilation (int, optional): Conv dilation. Default: 1.
+ add_identity (bool, optional): Whether to add
+ identity in root. Default: False.
+ init_cfg (dict, optional): Initialization config.
+ Default: None.
+ """
+
+ def __init__(self,
+ levels,
+ block,
+ in_channels,
+ out_channels,
+ norm_cfg,
+ conv_cfg,
+ stride=1,
+ level_root=False,
+ root_dim=None,
+ root_kernel_size=1,
+ dilation=1,
+ add_identity=False,
+ init_cfg=None):
+ super(Tree, self).__init__(init_cfg)
+ if root_dim is None:
+ root_dim = 2 * out_channels
+ if level_root:
+ root_dim += in_channels
+ if levels == 1:
+ self.root = Root(root_dim, out_channels, norm_cfg, conv_cfg,
+ root_kernel_size, add_identity)
+ self.tree1 = block(
+ in_channels,
+ out_channels,
+ norm_cfg,
+ conv_cfg,
+ stride,
+ dilation=dilation)
+ self.tree2 = block(
+ out_channels,
+ out_channels,
+ norm_cfg,
+ conv_cfg,
+ 1,
+ dilation=dilation)
+ else:
+ self.tree1 = Tree(
+ levels - 1,
+ block,
+ in_channels,
+ out_channels,
+ norm_cfg,
+ conv_cfg,
+ stride,
+ root_dim=None,
+ root_kernel_size=root_kernel_size,
+ dilation=dilation,
+ add_identity=add_identity)
+ self.tree2 = Tree(
+ levels - 1,
+ block,
+ out_channels,
+ out_channels,
+ norm_cfg,
+ conv_cfg,
+ root_dim=root_dim + out_channels,
+ root_kernel_size=root_kernel_size,
+ dilation=dilation,
+ add_identity=add_identity)
+ self.level_root = level_root
+ self.root_dim = root_dim
+ self.downsample = None
+ self.project = None
+ self.levels = levels
+ if stride > 1:
+ self.downsample = nn.MaxPool2d(stride, stride=stride)
+ if in_channels != out_channels:
+ self.project = nn.Sequential(
+ build_conv_layer(
+ conv_cfg,
+ in_channels,
+ out_channels,
+ 1,
+ stride=1,
+ bias=False),
+ dla_build_norm_layer(norm_cfg, out_channels)[1])
+
+ def forward(self, x, identity=None, children=None):
+ children = [] if children is None else children
+ bottom = self.downsample(x) if self.downsample else x
+ identity = self.project(bottom) if self.project else bottom
+ if self.level_root:
+ children.append(bottom)
+ x1 = self.tree1(x, identity)
+ if self.levels == 1:
+ x2 = self.tree2(x1)
+ feat_list = [x2, x1] + children
+ x = self.root(feat_list)
+ else:
+ children.append(x1)
+ x = self.tree2(x1, children=children)
+ return x
+
+
+@BACKBONES.register_module()
+class DLANet(BaseModule):
+ r"""`DLA backbone `_.
+
+ Args:
+ depth (int): Depth of DLA. Default: 34.
+ in_channels (int, optional): Number of input image channels.
+ Default: 3.
+ norm_cfg (dict, optional): Dictionary to construct and config
+ norm layer. Default: None.
+ conv_cfg (dict, optional): Dictionary to construct and config
+ conv layer. Default: None.
+ layer_with_level_root (list[bool], optional): Whether to apply
+ level_root in each DLA layer, this is only used for
+ tree levels. Default: (False, True, True, True).
+ with_identity_root (bool, optional): Whether to add identity
+ in root layer. Default: False.
+ pretrained (str, optional): model pretrained path.
+ Default: None.
+ init_cfg (dict or list[dict], optional): Initialization
+ config dict. Default: None
+ """
+ arch_settings = {
+ 34: (BasicBlock, (1, 1, 1, 2, 2, 1), (16, 32, 64, 128, 256, 512)),
+ }
+
+ def __init__(self,
+ depth,
+ in_channels=3,
+ out_indices=(0, 1, 2, 3, 4, 5),
+ frozen_stages=-1,
+ norm_cfg=None,
+ conv_cfg=None,
+ layer_with_level_root=(False, True, True, True),
+ with_identity_root=False,
+ pretrained=None,
+ init_cfg=None):
+ super(DLANet, self).__init__(init_cfg)
+ if depth not in self.arch_settings:
+ raise KeyError(f'invalida depth {depth} for DLA')
+
+ assert not (init_cfg and pretrained), \
+ 'init_cfg and pretrained cannot be setting at the same time'
+ if isinstance(pretrained, str):
+ warnings.warn('DeprecationWarning: pretrained is a deprecated, '
+ 'please use "init_cfg" instead')
+ self.init_cfg = dict(type='Pretrained', checkpoint=pretrained)
+ elif pretrained is None:
+ if init_cfg is None:
+ self.init_cfg = [
+ dict(type='Kaiming', layer='Conv2d'),
+ dict(
+ type='Constant',
+ val=1,
+ layer=['_BatchNorm', 'GroupNorm'])
+ ]
+
+ block, levels, channels = self.arch_settings[depth]
+ self.channels = channels
+ self.num_levels = len(levels)
+ self.frozen_stages = frozen_stages
+ self.out_indices = out_indices
+ assert max(out_indices) < self.num_levels
+ self.base_layer = nn.Sequential(
+ build_conv_layer(
+ conv_cfg,
+ in_channels,
+ channels[0],
+ 7,
+ stride=1,
+ padding=3,
+ bias=False),
+ dla_build_norm_layer(norm_cfg, channels[0])[1],
+ nn.ReLU(inplace=True))
+
+ # DLANet first uses two conv layers then uses several
+ # Tree layers
+ for i in range(2):
+ level_layer = self._make_conv_level(
+ channels[0],
+ channels[i],
+ levels[i],
+ norm_cfg,
+ conv_cfg,
+ stride=i + 1)
+ layer_name = f'level{i}'
+ self.add_module(layer_name, level_layer)
+
+ for i in range(2, self.num_levels):
+ dla_layer = Tree(
+ levels[i],
+ block,
+ channels[i - 1],
+ channels[i],
+ norm_cfg,
+ conv_cfg,
+ 2,
+ level_root=layer_with_level_root[i - 2],
+ add_identity=with_identity_root)
+ layer_name = f'level{i}'
+ self.add_module(layer_name, dla_layer)
+
+ self._freeze_stages()
+
+ def _make_conv_level(self,
+ in_channels,
+ out_channels,
+ num_convs,
+ norm_cfg,
+ conv_cfg,
+ stride=1,
+ dilation=1):
+ """Conv modules.
+
+ Args:
+ in_channels (int): Input feature channel.
+ out_channels (int): Output feature channel.
+ num_convs (int): Number of Conv module.
+ norm_cfg (dict): Dictionary to construct and config
+ norm layer.
+ conv_cfg (dict): Dictionary to construct and config
+ conv layer.
+ stride (int, optional): Conv stride. Default: 1.
+ dilation (int, optional): Conv dilation. Default: 1.
+ """
+ modules = []
+ for i in range(num_convs):
+ modules.extend([
+ build_conv_layer(
+ conv_cfg,
+ in_channels,
+ out_channels,
+ 3,
+ stride=stride if i == 0 else 1,
+ padding=dilation,
+ bias=False,
+ dilation=dilation),
+ dla_build_norm_layer(norm_cfg, out_channels)[1],
+ nn.ReLU(inplace=True)
+ ])
+ in_channels = out_channels
+ return nn.Sequential(*modules)
+
+ def _freeze_stages(self):
+ if self.frozen_stages >= 0:
+ self.base_layer.eval()
+ for param in self.base_layer.parameters():
+ param.requires_grad = False
+
+ for i in range(2):
+ m = getattr(self, f'level{i}')
+ m.eval()
+ for param in m.parameters():
+ param.requires_grad = False
+
+ for i in range(1, self.frozen_stages + 1):
+ m = getattr(self, f'level{i+1}')
+ m.eval()
+ for param in m.parameters():
+ param.requires_grad = False
+
+ def forward(self, x):
+ outs = []
+ x = self.base_layer(x)
+ for i in range(self.num_levels):
+ x = getattr(self, 'level{}'.format(i))(x)
+ if i in self.out_indices:
+ outs.append(x)
+ return tuple(outs)
diff --git a/mmdet3d/models/backbones/mink_resnet.py b/mmdet3d/models/backbones/mink_resnet.py
new file mode 100644
index 0000000..1b63102
--- /dev/null
+++ b/mmdet3d/models/backbones/mink_resnet.py
@@ -0,0 +1,126 @@
+# Copyright (c) OpenMMLab. All rights reserved.
+# Follow https://github.com/NVIDIA/MinkowskiEngine/blob/master/examples/resnet.py # noqa
+# and mmcv.cnn.ResNet
+try:
+ import MinkowskiEngine as ME
+ from MinkowskiEngine.modules.resnet_block import BasicBlock, Bottleneck
+except ImportError:
+ import warnings
+ warnings.warn(
+ 'Please follow `getting_started.md` to install MinkowskiEngine.`')
+ # blocks are used in the static part of MinkResNet
+ BasicBlock, Bottleneck = None, None
+
+import torch.nn as nn
+
+from mmdet3d.models.builder import BACKBONES
+
+
+@BACKBONES.register_module()
+class MinkResNet(nn.Module):
+ r"""Minkowski ResNet backbone. See `4D Spatio-Temporal ConvNets
+ `_ for more details.
+
+ Args:
+ depth (int): Depth of resnet, from {18, 34, 50, 101, 152}.
+ in_channels (ont): Number of input channels, 3 for RGB.
+ num_stages (int, optional): Resnet stages. Default: 4.
+ pool (bool, optional): Add max pooling after first conv if True.
+ Default: True.
+ """
+ arch_settings = {
+ 18: (BasicBlock, (2, 2, 2, 2)),
+ 34: (BasicBlock, (3, 4, 6, 3)),
+ 50: (Bottleneck, (3, 4, 6, 3)),
+ 101: (Bottleneck, (3, 4, 23, 3)),
+ 152: (Bottleneck, (3, 8, 36, 3))
+ }
+
+ def __init__(self,
+ depth,
+ in_channels,
+ num_stages=4,
+ pool=True,
+ norm='instance',
+ return_stem=False,
+ stride=2):
+ super(MinkResNet, self).__init__()
+ if depth not in self.arch_settings:
+ raise KeyError(f'invalid depth {depth} for resnet')
+ assert 4 >= num_stages >= 1
+ block, stage_blocks = self.arch_settings[depth]
+ stage_blocks = stage_blocks[:num_stages]
+ self.num_stages = num_stages
+ self.pool = pool
+ self.return_stem = return_stem
+ self.inplanes = 64
+ self.conv1 = ME.MinkowskiConvolution(
+ in_channels, self.inplanes, kernel_size=3, stride=stride, dimension=3)
+ norm1 = ME.MinkowskiInstanceNorm if norm == 'instance' \
+ else ME.MinkowskiBatchNorm
+ self.norm1 = norm1(self.inplanes)
+ self.relu = ME.MinkowskiReLU(inplace=True)
+ if self.pool:
+ self.maxpool = ME.MinkowskiMaxPooling(
+ kernel_size=2, stride=2, dimension=3)
+
+ for i, num_blocks in enumerate(stage_blocks):
+ setattr(
+ self, f'layer{i + 1}',
+ self._make_layer(block, 64 * 2**i, stage_blocks[i], stride=2))
+
+ def init_weights(self):
+ for m in self.modules():
+ if isinstance(m, ME.MinkowskiConvolution):
+ ME.utils.kaiming_normal_(
+ m.kernel, mode='fan_out', nonlinearity='relu')
+
+ if isinstance(m, ME.MinkowskiBatchNorm):
+ nn.init.constant_(m.bn.weight, 1)
+ nn.init.constant_(m.bn.bias, 0)
+
+ def _make_layer(self, block, planes, blocks, stride):
+ downsample = None
+ if stride != 1 or self.inplanes != planes * block.expansion:
+ downsample = nn.Sequential(
+ ME.MinkowskiConvolution(
+ self.inplanes,
+ planes * block.expansion,
+ kernel_size=1,
+ stride=stride,
+ dimension=3),
+ ME.MinkowskiBatchNorm(planes * block.expansion))
+ layers = []
+ layers.append(
+ block(
+ self.inplanes,
+ planes,
+ stride=stride,
+ downsample=downsample,
+ dimension=3))
+ self.inplanes = planes * block.expansion
+ for i in range(1, blocks):
+ layers.append(block(self.inplanes, planes, stride=1, dimension=3))
+ return nn.Sequential(*layers)
+
+ def forward(self, x):
+ """Forward pass of ResNet.
+
+ Args:
+ x (ME.SparseTensor): Input sparse tensor.
+
+ Returns:
+ list[ME.SparseTensor]: Output sparse tensors.
+ """
+ outs = []
+ x = self.conv1(x)
+ x = self.norm1(x)
+ x = self.relu(x)
+ if self.return_stem:
+ outs.append(x)
+ if self.pool:
+ x = self.maxpool(x)
+ for i in range(self.num_stages):
+ x = getattr(self, f'layer{i + 1}')(x)
+ outs.append(x)
+ return outs
diff --git a/mmdet3d/models/backbones/mink_unet.py b/mmdet3d/models/backbones/mink_unet.py
new file mode 100644
index 0000000..22453cc
--- /dev/null
+++ b/mmdet3d/models/backbones/mink_unet.py
@@ -0,0 +1,469 @@
+# Copyright (c) Chris Choy (chrischoy@ai.stanford.edu).
+#
+# Permission is hereby granted, free of charge, to any person obtaining a copy of
+# this software and associated documentation files (the "Software"), to deal in
+# the Software without restriction, including without limitation the rights to
+# use, copy, modify, merge, publish, distribute, sublicense, and/or sell copies
+# of the Software, and to permit persons to whom the Software is furnished to do
+# so, subject to the following conditions:
+#
+# The above copyright notice and this permission notice shall be included in all
+# copies or substantial portions of the Software.
+#
+# THE SOFTWARE IS PROVIDED "AS IS", WITHOUT WARRANTY OF ANY KIND, EXPRESS OR
+# IMPLIED, INCLUDING BUT NOT LIMITED TO THE WARRANTIES OF MERCHANTABILITY,
+# FITNESS FOR A PARTICULAR PURPOSE AND NONINFRINGEMENT. IN NO EVENT SHALL THE
+# AUTHORS OR COPYRIGHT HOLDERS BE LIABLE FOR ANY CLAIM, DAMAGES OR OTHER
+# LIABILITY, WHETHER IN AN ACTION OF CONTRACT, TORT OR OTHERWISE, ARISING FROM,
+# OUT OF OR IN CONNECTION WITH THE SOFTWARE OR THE USE OR OTHER DEALINGS IN THE
+# SOFTWARE.
+#
+# Please cite "4D Spatio-Temporal ConvNets: Minkowski Convolutional Neural
+# Networks", CVPR'19 (https://arxiv.org/abs/1904.08755) if you use any part
+# of the code.
+import torch.nn as nn
+import torch
+import MinkowskiEngine as ME
+import MinkowskiEngine.MinkowskiFunctional as MF
+
+from MinkowskiEngine.modules.resnet_block import BasicBlock, Bottleneck
+from mmdet3d.models.builder import BACKBONES
+
+class ResNetBase(nn.Module):
+ BLOCK = None
+ LAYERS = ()
+ INIT_DIM = 64
+ PLANES = (64, 128, 256, 512)
+
+ def __init__(self, in_channels, out_channels, D=3):
+ nn.Module.__init__(self)
+ self.D = D
+ assert self.BLOCK is not None
+
+ self.network_initialization(in_channels, out_channels, D)
+ self.weight_initialization()
+
+ def network_initialization(self, in_channels, out_channels, D):
+
+ self.inplanes = self.INIT_DIM
+ self.conv1 = nn.Sequential(
+ ME.MinkowskiConvolution(
+ in_channels, self.inplanes, kernel_size=3, stride=2, dimension=D
+ ),
+ ME.MinkowskiInstanceNorm(self.inplanes),
+ ME.MinkowskiReLU(inplace=True),
+ ME.MinkowskiMaxPooling(kernel_size=2, stride=2, dimension=D),
+ )
+
+ self.layer1 = self._make_layer(
+ self.BLOCK, self.PLANES[0], self.LAYERS[0], stride=2
+ )
+ self.layer2 = self._make_layer(
+ self.BLOCK, self.PLANES[1], self.LAYERS[1], stride=2
+ )
+ self.layer3 = self._make_layer(
+ self.BLOCK, self.PLANES[2], self.LAYERS[2], stride=2
+ )
+ self.layer4 = self._make_layer(
+ self.BLOCK, self.PLANES[3], self.LAYERS[3], stride=2
+ )
+
+ self.conv5 = nn.Sequential(
+ ME.MinkowskiDropout(),
+ ME.MinkowskiConvolution(
+ self.inplanes, self.inplanes, kernel_size=3, stride=3, dimension=D
+ ),
+ ME.MinkowskiInstanceNorm(self.inplanes),
+ ME.MinkowskiGELU(),
+ )
+
+ self.glob_pool = ME.MinkowskiGlobalMaxPooling()
+
+ self.final = ME.MinkowskiLinear(self.inplanes, out_channels, bias=True)
+
+ def weight_initialization(self):
+ for m in self.modules():
+ if isinstance(m, ME.MinkowskiConvolution):
+ ME.utils.kaiming_normal_(m.kernel, mode="fan_out", nonlinearity="relu")
+
+ if isinstance(m, ME.MinkowskiBatchNorm):
+ nn.init.constant_(m.bn.weight, 1)
+ nn.init.constant_(m.bn.bias, 0)
+
+ def _make_layer(self, block, planes, blocks, stride=1, dilation=1, bn_momentum=0.1):
+ downsample = None
+ if stride != 1 or self.inplanes != planes * block.expansion:
+ downsample = nn.Sequential(
+ ME.MinkowskiConvolution(
+ self.inplanes,
+ planes * block.expansion,
+ kernel_size=1,
+ stride=stride,
+ dimension=self.D,
+ ),
+ ME.MinkowskiBatchNorm(planes * block.expansion),
+ )
+ layers = []
+ layers.append(
+ block(
+ self.inplanes,
+ planes,
+ stride=stride,
+ dilation=dilation,
+ downsample=downsample,
+ dimension=self.D,
+ )
+ )
+ self.inplanes = planes * block.expansion
+ for i in range(1, blocks):
+ layers.append(
+ block(
+ self.inplanes, planes, stride=1, dilation=dilation, dimension=self.D
+ )
+ )
+
+ return nn.Sequential(*layers)
+
+ def forward(self, x: ME.SparseTensor):
+ x = self.conv1(x)
+ x = self.layer1(x)
+ x = self.layer2(x)
+ x = self.layer3(x)
+ x = self.layer4(x)
+ x = self.conv5(x)
+ x = self.glob_pool(x)
+ return self.final(x)
+
+class MinkUNetBase(ResNetBase):
+ BLOCK = None
+ PLANES = None
+ DILATIONS = (1, 1, 1, 1, 1, 1, 1, 1)
+ LAYERS = (2, 2, 2, 2, 2, 2, 2, 2)
+ PLANES = (32, 64, 128, 256, 256, 128, 96, 96)
+ INIT_DIM = 32
+ OUT_TENSOR_STRIDE = 1
+
+ # To use the model, must call initialize_coords before forward pass.
+ # Once data is processed, call clear to reset the model before calling
+ # initialize_coords
+ def __init__(self, in_channels, out_channels, D=3):
+ ResNetBase.__init__(self, in_channels, out_channels, D)
+
+ def network_initialization(self, in_channels, out_channels, D):
+ # Output of the first conv concated to conv6
+ self.inplanes = self.INIT_DIM
+ self.conv0p1s1 = ME.MinkowskiConvolution(
+ in_channels, self.inplanes, kernel_size=5, dimension=D)
+
+ self.bn0 = ME.MinkowskiBatchNorm(self.inplanes)
+
+ self.conv1p1s2 = ME.MinkowskiConvolution(
+ self.inplanes, self.inplanes, kernel_size=2, stride=2, dimension=D)
+ self.bn1 = ME.MinkowskiBatchNorm(self.inplanes)
+
+ self.block1 = self._make_layer(self.BLOCK, self.PLANES[0],
+ self.LAYERS[0])
+
+ self.conv2p2s2 = ME.MinkowskiConvolution(
+ self.inplanes, self.inplanes, kernel_size=2, stride=2, dimension=D)
+ self.bn2 = ME.MinkowskiBatchNorm(self.inplanes)
+
+ self.block2 = self._make_layer(self.BLOCK, self.PLANES[1],
+ self.LAYERS[1])
+
+ self.conv3p4s2 = ME.MinkowskiConvolution(
+ self.inplanes, self.inplanes, kernel_size=2, stride=2, dimension=D)
+
+ self.bn3 = ME.MinkowskiBatchNorm(self.inplanes)
+ self.block3 = self._make_layer(self.BLOCK, self.PLANES[2],
+ self.LAYERS[2])
+
+ self.conv4p8s2 = ME.MinkowskiConvolution(
+ self.inplanes, self.inplanes, kernel_size=2, stride=2, dimension=D)
+ self.bn4 = ME.MinkowskiBatchNorm(self.inplanes)
+ self.block4 = self._make_layer(self.BLOCK, self.PLANES[3],
+ self.LAYERS[3])
+
+ self.convtr4p16s2 = ME.MinkowskiConvolutionTranspose(
+ self.inplanes, self.PLANES[4], kernel_size=2, stride=2, dimension=D)
+ self.bntr4 = ME.MinkowskiBatchNorm(self.PLANES[4])
+
+ self.inplanes = self.PLANES[4] + self.PLANES[2] * self.BLOCK.expansion
+ self.block5 = self._make_layer(self.BLOCK, self.PLANES[4],
+ self.LAYERS[4])
+ self.convtr5p8s2 = ME.MinkowskiConvolutionTranspose(
+ self.inplanes, self.PLANES[5], kernel_size=2, stride=2, dimension=D)
+ self.bntr5 = ME.MinkowskiBatchNorm(self.PLANES[5])
+
+ self.inplanes = self.PLANES[5] + self.PLANES[1] * self.BLOCK.expansion
+ self.block6 = self._make_layer(self.BLOCK, self.PLANES[5],
+ self.LAYERS[5])
+ self.convtr6p4s2 = ME.MinkowskiConvolutionTranspose(
+ self.inplanes, self.PLANES[6], kernel_size=2, stride=2, dimension=D)
+ self.bntr6 = ME.MinkowskiBatchNorm(self.PLANES[6])
+
+ self.inplanes = self.PLANES[6] + self.PLANES[0] * self.BLOCK.expansion
+ self.block7 = self._make_layer(self.BLOCK, self.PLANES[6],
+ self.LAYERS[6])
+ self.convtr7p2s2 = ME.MinkowskiConvolutionTranspose(
+ self.inplanes, self.PLANES[7], kernel_size=2, stride=2, dimension=D)
+ self.bntr7 = ME.MinkowskiBatchNorm(self.PLANES[7])
+
+ self.inplanes = self.PLANES[7] + self.INIT_DIM
+ self.block8 = self._make_layer(self.BLOCK, self.PLANES[7],
+ self.LAYERS[7])
+
+ self.final = ME.MinkowskiConvolution(
+ self.PLANES[7] * self.BLOCK.expansion,
+ out_channels,
+ kernel_size=1,
+ bias=True,
+ dimension=D)
+ self.relu = ME.MinkowskiReLU(inplace=True)
+
+ def forward(self, x):
+ out = self.conv0p1s1(x)
+ out = self.bn0(out)
+ out_p1 = self.relu(out)
+
+ out = self.conv1p1s2(out_p1)
+ out = self.bn1(out)
+ out = self.relu(out)
+ out_b1p2 = self.block1(out)
+
+ out = self.conv2p2s2(out_b1p2)
+ out = self.bn2(out)
+ out = self.relu(out)
+ out_b2p4 = self.block2(out)
+
+ out = self.conv3p4s2(out_b2p4)
+ out = self.bn3(out)
+ out = self.relu(out)
+ out_b3p8 = self.block3(out)
+
+ # tensor_stride=16
+ out = self.conv4p8s2(out_b3p8)
+ out = self.bn4(out)
+ out = self.relu(out)
+ out = self.block4(out)
+
+ # tensor_stride=8
+ out = self.convtr4p16s2(out)
+ out = self.bntr4(out)
+ out = self.relu(out)
+
+ out = ME.cat(out, out_b3p8)
+ out = self.block5(out)
+
+ # tensor_stride=4
+ out = self.convtr5p8s2(out)
+ out = self.bntr5(out)
+ out = self.relu(out)
+
+ out = ME.cat(out, out_b2p4)
+ out = self.block6(out)
+
+ # tensor_stride=2
+ out = self.convtr6p4s2(out)
+ out = self.bntr6(out)
+ out = self.relu(out)
+
+ out = ME.cat(out, out_b1p2)
+ out = self.block7(out)
+
+ # tensor_stride=1
+ out = self.convtr7p2s2(out)
+ out = self.bntr7(out)
+ out = self.relu(out)
+
+ out = ME.cat(out, out_p1)
+ out = self.block8(out)
+
+ return self.final(out)
+
+@BACKBONES.register_module()
+class MinkUNet14(MinkUNetBase):
+ BLOCK = BasicBlock
+ LAYERS = (1, 1, 1, 1, 1, 1, 1, 1)
+
+@BACKBONES.register_module()
+class MinkUNet18(MinkUNetBase):
+ BLOCK = BasicBlock
+ LAYERS = (2, 2, 2, 2, 2, 2, 2, 2)
+
+@BACKBONES.register_module()
+class MinkUNet34(MinkUNetBase):
+ BLOCK = BasicBlock
+ LAYERS = (2, 3, 4, 6, 2, 2, 2, 2)
+
+@BACKBONES.register_module()
+class MinkUNet50(MinkUNetBase):
+ BLOCK = Bottleneck
+ LAYERS = (2, 3, 4, 6, 2, 2, 2, 2)
+
+@BACKBONES.register_module()
+class MinkUNet101(MinkUNetBase):
+ BLOCK = Bottleneck
+ LAYERS = (2, 3, 4, 23, 2, 2, 2, 2)
+
+@BACKBONES.register_module()
+class MinkUNet14A(MinkUNet14):
+ PLANES = (32, 64, 128, 256, 128, 128, 96, 96)
+
+@BACKBONES.register_module()
+class MinkUNet14B(MinkUNet14):
+ PLANES = (32, 64, 128, 256, 128, 128, 128, 128)
+
+@BACKBONES.register_module()
+class MinkUNet14C(MinkUNet14):
+ PLANES = (32, 64, 128, 256, 192, 192, 128, 128)
+
+@BACKBONES.register_module()
+class MinkUNet14D(MinkUNet14):
+ PLANES = (32, 64, 128, 256, 384, 384, 384, 384)
+
+@BACKBONES.register_module()
+class MinkUNet18A(MinkUNet18):
+ PLANES = (32, 64, 128, 256, 128, 128, 96, 96)
+
+@BACKBONES.register_module()
+class MinkUNet18B(MinkUNet18):
+ PLANES = (32, 64, 128, 256, 128, 128, 128, 128)
+
+@BACKBONES.register_module()
+class MinkUNet18D(MinkUNet18):
+ PLANES = (32, 64, 128, 256, 384, 384, 384, 384)
+
+@BACKBONES.register_module()
+class MinkUNet34A(MinkUNet34):
+ PLANES = (32, 64, 128, 256, 256, 128, 64, 64)
+
+@BACKBONES.register_module()
+class MinkUNet34B(MinkUNet34):
+ PLANES = (32, 64, 128, 256, 256, 128, 64, 32)
+
+@BACKBONES.register_module()
+class MinkUNet34C(MinkUNet34):
+ PLANES = (32, 64, 128, 256, 256, 128, 96, 96)
+
+@BACKBONES.register_module()
+class CustomUNet(ME.MinkowskiNetwork):
+ def __init__(self, in_channels, out_channels, D):
+ super(CustomUNet, self).__init__(D)
+ self.block1 = torch.nn.Sequential(
+ ME.MinkowskiConvolution(
+ in_channels=in_channels,
+ out_channels=64,
+ kernel_size=3,
+ stride=2,
+ dimension=D),
+ ME.MinkowskiBatchNorm(64))
+
+ self.block2 = torch.nn.Sequential(
+ ME.MinkowskiConvolution(
+ in_channels=64,
+ out_channels=64,
+ kernel_size=3,
+ stride=2,
+ dimension=D),
+ ME.MinkowskiBatchNorm(64))
+
+ self.block3 = torch.nn.Sequential(
+ ME.MinkowskiConvolution(
+ in_channels=64,
+ out_channels=96,
+ kernel_size=3,
+ stride=2,
+ dimension=D),
+ ME.MinkowskiBatchNorm(96))
+
+ self.block4 = torch.nn.Sequential(
+ ME.MinkowskiConvolution(
+ in_channels=96,
+ out_channels=128,
+ kernel_size=3,
+ stride=2,
+ dimension=D),
+ ME.MinkowskiBatchNorm(128))
+
+ self.block5 = torch.nn.Sequential(
+ ME.MinkowskiConvolution(
+ in_channels=128,
+ out_channels=128,
+ kernel_size=10,
+ stride=1,
+ dimension=D),
+ ME.MinkowskiBatchNorm(128))
+
+ self.block4_tr = torch.nn.Sequential(
+ ME.MinkowskiConvolutionTranspose(
+ in_channels=128,
+ out_channels=96,
+ kernel_size=3,
+ stride=2,
+ dimension=D),
+ ME.MinkowskiBatchNorm(96))
+
+ self.block3_tr = torch.nn.Sequential(
+ ME.MinkowskiConvolutionTranspose(
+ in_channels=192,
+ out_channels=64,
+ kernel_size=3,
+ stride=2,
+ dimension=D),
+ ME.MinkowskiBatchNorm(64))
+
+ self.block2_tr = torch.nn.Sequential(
+ ME.MinkowskiConvolutionTranspose(
+ in_channels=128,
+ out_channels=32,
+ kernel_size=3,
+ stride=2,
+ dimension=D),
+ ME.MinkowskiBatchNorm(32))
+
+ self.block1_tr = torch.nn.Sequential(
+ ME.MinkowskiConvolutionTranspose(
+ in_channels=96,
+ out_channels=32,
+ kernel_size=3,
+ stride=2,
+ dimension=D),
+ ME.MinkowskiBatchNorm(32))
+
+ self.conv1_tr = ME.MinkowskiConvolution(
+ in_channels=32,
+ out_channels=out_channels,
+ kernel_size=1,
+ stride=1,
+ dimension=D)
+
+ def forward(self, x):
+ out_s2 = self.block1(x)
+ out = MF.relu(out_s2)
+
+ out_s4 = self.block2(out)
+ out = MF.relu(out_s4)
+
+ out_s8 = self.block3(out)
+ out = MF.relu(out_s8)
+
+ out_s16 = self.block4(out)
+ out = MF.relu(out_s16)
+
+ out1_s16 = self.block5(out)
+ out = MF.relu(out1_s16)
+
+
+ out = MF.relu(self.block4_tr(out))
+ out = ME.cat(out, out_s8)
+
+ out = MF.relu(self.block3_tr(out))
+ out = ME.cat(out, out_s4)
+
+ out = MF.relu(self.block2_tr(out))
+ out = ME.cat(out, out_s2)
+
+ out = MF.relu(self.block1_tr(out))
+
+ return self.conv1_tr(out)
diff --git a/mmdet3d/models/backbones/multi_backbone.py b/mmdet3d/models/backbones/multi_backbone.py
new file mode 100644
index 0000000..ed04ecd
--- /dev/null
+++ b/mmdet3d/models/backbones/multi_backbone.py
@@ -0,0 +1,127 @@
+# Copyright (c) OpenMMLab. All rights reserved.
+import copy
+import warnings
+
+import torch
+from mmcv.cnn import ConvModule
+from mmcv.runner import BaseModule, auto_fp16
+from torch import nn as nn
+
+from ..builder import BACKBONES, build_backbone
+
+
+@BACKBONES.register_module()
+class MultiBackbone(BaseModule):
+ """MultiBackbone with different configs.
+
+ Args:
+ num_streams (int): The number of backbones.
+ backbones (list or dict): A list of backbone configs.
+ aggregation_mlp_channels (list[int]): Specify the mlp layers
+ for feature aggregation.
+ conv_cfg (dict): Config dict of convolutional layers.
+ norm_cfg (dict): Config dict of normalization layers.
+ act_cfg (dict): Config dict of activation layers.
+ suffixes (list): A list of suffixes to rename the return dict
+ for each backbone.
+ """
+
+ def __init__(self,
+ num_streams,
+ backbones,
+ aggregation_mlp_channels=None,
+ conv_cfg=dict(type='Conv1d'),
+ norm_cfg=dict(type='BN1d', eps=1e-5, momentum=0.01),
+ act_cfg=dict(type='ReLU'),
+ suffixes=('net0', 'net1'),
+ init_cfg=None,
+ pretrained=None,
+ **kwargs):
+ super().__init__(init_cfg=init_cfg)
+ assert isinstance(backbones, dict) or isinstance(backbones, list)
+ if isinstance(backbones, dict):
+ backbones_list = []
+ for ind in range(num_streams):
+ backbones_list.append(copy.deepcopy(backbones))
+ backbones = backbones_list
+
+ assert len(backbones) == num_streams
+ assert len(suffixes) == num_streams
+
+ self.backbone_list = nn.ModuleList()
+ # Rename the ret_dict with different suffixs.
+ self.suffixes = suffixes
+
+ out_channels = 0
+
+ for backbone_cfg in backbones:
+ out_channels += backbone_cfg['fp_channels'][-1][-1]
+ self.backbone_list.append(build_backbone(backbone_cfg))
+
+ # Feature aggregation layers
+ if aggregation_mlp_channels is None:
+ aggregation_mlp_channels = [
+ out_channels, out_channels // 2,
+ out_channels // len(self.backbone_list)
+ ]
+ else:
+ aggregation_mlp_channels.insert(0, out_channels)
+
+ self.aggregation_layers = nn.Sequential()
+ for i in range(len(aggregation_mlp_channels) - 1):
+ self.aggregation_layers.add_module(
+ f'layer{i}',
+ ConvModule(
+ aggregation_mlp_channels[i],
+ aggregation_mlp_channels[i + 1],
+ 1,
+ padding=0,
+ conv_cfg=conv_cfg,
+ norm_cfg=norm_cfg,
+ act_cfg=act_cfg,
+ bias=True,
+ inplace=True))
+
+ assert not (init_cfg and pretrained), \
+ 'init_cfg and pretrained cannot be setting at the same time'
+ if isinstance(pretrained, str):
+ warnings.warn('DeprecationWarning: pretrained is a deprecated, '
+ 'please use "init_cfg" instead')
+ self.init_cfg = dict(type='Pretrained', checkpoint=pretrained)
+
+ @auto_fp16()
+ def forward(self, points):
+ """Forward pass.
+
+ Args:
+ points (torch.Tensor): point coordinates with features,
+ with shape (B, N, 3 + input_feature_dim).
+
+ Returns:
+ dict[str, list[torch.Tensor]]: Outputs from multiple backbones.
+
+ - fp_xyz[suffix] (list[torch.Tensor]): The coordinates of
+ each fp features.
+ - fp_features[suffix] (list[torch.Tensor]): The features
+ from each Feature Propagate Layers.
+ - fp_indices[suffix] (list[torch.Tensor]): Indices of the
+ input points.
+ - hd_feature (torch.Tensor): The aggregation feature
+ from multiple backbones.
+ """
+ ret = {}
+ fp_features = []
+ for ind in range(len(self.backbone_list)):
+ cur_ret = self.backbone_list[ind](points)
+ cur_suffix = self.suffixes[ind]
+ fp_features.append(cur_ret['fp_features'][-1])
+ if cur_suffix != '':
+ for k in cur_ret.keys():
+ cur_ret[k + '_' + cur_suffix] = cur_ret.pop(k)
+ ret.update(cur_ret)
+
+ # Combine the features here
+ hd_feature = torch.cat(fp_features, dim=1)
+ hd_feature = self.aggregation_layers(hd_feature)
+ ret['hd_feature'] = hd_feature
+ return ret
diff --git a/mmdet3d/models/backbones/nostem_regnet.py b/mmdet3d/models/backbones/nostem_regnet.py
new file mode 100644
index 0000000..3090508
--- /dev/null
+++ b/mmdet3d/models/backbones/nostem_regnet.py
@@ -0,0 +1,84 @@
+# Copyright (c) OpenMMLab. All rights reserved.
+from mmdet.models.backbones import RegNet
+from ..builder import BACKBONES
+
+
+@BACKBONES.register_module()
+class NoStemRegNet(RegNet):
+ """RegNet backbone without Stem for 3D detection.
+
+ More details can be found in `paper `_ .
+
+ Args:
+ arch (dict): The parameter of RegNets.
+ - w0 (int): Initial width.
+ - wa (float): Slope of width.
+ - wm (float): Quantization parameter to quantize the width.
+ - depth (int): Depth of the backbone.
+ - group_w (int): Width of group.
+ - bot_mul (float): Bottleneck ratio, i.e. expansion of bottleneck.
+ strides (Sequence[int]): Strides of the first block of each stage.
+ base_channels (int): Base channels after stem layer.
+ in_channels (int): Number of input image channels. Normally 3.
+ dilations (Sequence[int]): Dilation of each stage.
+ out_indices (Sequence[int]): Output from which stages.
+ style (str): `pytorch` or `caffe`. If set to "pytorch", the stride-two
+ layer is the 3x3 conv layer, otherwise the stride-two layer is
+ the first 1x1 conv layer.
+ frozen_stages (int): Stages to be frozen (all param fixed). -1 means
+ not freezing any parameters.
+ norm_cfg (dict): Dictionary to construct and config norm layer.
+ norm_eval (bool): Whether to set norm layers to eval mode, namely,
+ freeze running stats (mean and var). Note: Effect on Batch Norm
+ and its variants only.
+ with_cp (bool): Use checkpoint or not. Using checkpoint will save some
+ memory while slowing down the training speed.
+ zero_init_residual (bool): Whether to use zero init for last norm layer
+ in resblocks to let them behave as identity.
+
+ Example:
+ >>> from mmdet3d.models import NoStemRegNet
+ >>> import torch
+ >>> self = NoStemRegNet(
+ arch=dict(
+ w0=88,
+ wa=26.31,
+ wm=2.25,
+ group_w=48,
+ depth=25,
+ bot_mul=1.0))
+ >>> self.eval()
+ >>> inputs = torch.rand(1, 64, 16, 16)
+ >>> level_outputs = self.forward(inputs)
+ >>> for level_out in level_outputs:
+ ... print(tuple(level_out.shape))
+ (1, 96, 8, 8)
+ (1, 192, 4, 4)
+ (1, 432, 2, 2)
+ (1, 1008, 1, 1)
+ """
+
+ def __init__(self, arch, init_cfg=None, **kwargs):
+ super(NoStemRegNet, self).__init__(arch, init_cfg=init_cfg, **kwargs)
+
+ def _make_stem_layer(self, in_channels, base_channels):
+ """Override the original function that do not initialize a stem layer
+ since 3D detector's voxel encoder works like a stem layer."""
+ return
+
+ def forward(self, x):
+ """Forward function of backbone.
+
+ Args:
+ x (torch.Tensor): Features in shape (N, C, H, W).
+
+ Returns:
+ tuple[torch.Tensor]: Multi-scale features.
+ """
+ outs = []
+ for i, layer_name in enumerate(self.res_layers):
+ res_layer = getattr(self, layer_name)
+ x = res_layer(x)
+ if i in self.out_indices:
+ outs.append(x)
+ return tuple(outs)
diff --git a/mmdet3d/models/backbones/pointnet2_sa_msg.py b/mmdet3d/models/backbones/pointnet2_sa_msg.py
new file mode 100644
index 0000000..f6b1e47
--- /dev/null
+++ b/mmdet3d/models/backbones/pointnet2_sa_msg.py
@@ -0,0 +1,175 @@
+# Copyright (c) OpenMMLab. All rights reserved.
+import torch
+from mmcv.cnn import ConvModule
+from mmcv.runner import auto_fp16
+from torch import nn as nn
+
+from mmdet3d.ops import build_sa_module
+from ..builder import BACKBONES
+from .base_pointnet import BasePointNet
+
+
+@BACKBONES.register_module()
+class PointNet2SAMSG(BasePointNet):
+ """PointNet2 with Multi-scale grouping.
+
+ Args:
+ in_channels (int): Input channels of point cloud.
+ num_points (tuple[int]): The number of points which each SA
+ module samples.
+ radii (tuple[float]): Sampling radii of each SA module.
+ num_samples (tuple[int]): The number of samples for ball
+ query in each SA module.
+ sa_channels (tuple[tuple[int]]): Out channels of each mlp in SA module.
+ aggregation_channels (tuple[int]): Out channels of aggregation
+ multi-scale grouping features.
+ fps_mods (tuple[int]): Mod of FPS for each SA module.
+ fps_sample_range_lists (tuple[tuple[int]]): The number of sampling
+ points which each SA module samples.
+ dilated_group (tuple[bool]): Whether to use dilated ball query for
+ out_indices (Sequence[int]): Output from which stages.
+ norm_cfg (dict): Config of normalization layer.
+ sa_cfg (dict): Config of set abstraction module, which may contain
+ the following keys and values:
+
+ - pool_mod (str): Pool method ('max' or 'avg') for SA modules.
+ - use_xyz (bool): Whether to use xyz as a part of features.
+ - normalize_xyz (bool): Whether to normalize xyz with radii in
+ each SA module.
+ """
+
+ def __init__(self,
+ in_channels,
+ num_points=(2048, 1024, 512, 256),
+ radii=((0.2, 0.4, 0.8), (0.4, 0.8, 1.6), (1.6, 3.2, 4.8)),
+ num_samples=((32, 32, 64), (32, 32, 64), (32, 32, 32)),
+ sa_channels=(((16, 16, 32), (16, 16, 32), (32, 32, 64)),
+ ((64, 64, 128), (64, 64, 128), (64, 96, 128)),
+ ((128, 128, 256), (128, 192, 256), (128, 256,
+ 256))),
+ aggregation_channels=(64, 128, 256),
+ fps_mods=(('D-FPS'), ('FS'), ('F-FPS', 'D-FPS')),
+ fps_sample_range_lists=((-1), (-1), (512, -1)),
+ dilated_group=(True, True, True),
+ out_indices=(2, ),
+ norm_cfg=dict(type='BN2d'),
+ sa_cfg=dict(
+ type='PointSAModuleMSG',
+ pool_mod='max',
+ use_xyz=True,
+ normalize_xyz=False),
+ init_cfg=None):
+ super().__init__(init_cfg=init_cfg)
+ self.num_sa = len(sa_channels)
+ self.out_indices = out_indices
+ assert max(out_indices) < self.num_sa
+ assert len(num_points) == len(radii) == len(num_samples) == len(
+ sa_channels)
+ if aggregation_channels is not None:
+ assert len(sa_channels) == len(aggregation_channels)
+ else:
+ aggregation_channels = [None] * len(sa_channels)
+
+ self.SA_modules = nn.ModuleList()
+ self.aggregation_mlps = nn.ModuleList()
+ sa_in_channel = in_channels - 3 # number of channels without xyz
+ skip_channel_list = [sa_in_channel]
+
+ for sa_index in range(self.num_sa):
+ cur_sa_mlps = list(sa_channels[sa_index])
+ sa_out_channel = 0
+ for radius_index in range(len(radii[sa_index])):
+ cur_sa_mlps[radius_index] = [sa_in_channel] + list(
+ cur_sa_mlps[radius_index])
+ sa_out_channel += cur_sa_mlps[radius_index][-1]
+
+ if isinstance(fps_mods[sa_index], tuple):
+ cur_fps_mod = list(fps_mods[sa_index])
+ else:
+ cur_fps_mod = list([fps_mods[sa_index]])
+
+ if isinstance(fps_sample_range_lists[sa_index], tuple):
+ cur_fps_sample_range_list = list(
+ fps_sample_range_lists[sa_index])
+ else:
+ cur_fps_sample_range_list = list(
+ [fps_sample_range_lists[sa_index]])
+
+ self.SA_modules.append(
+ build_sa_module(
+ num_point=num_points[sa_index],
+ radii=radii[sa_index],
+ sample_nums=num_samples[sa_index],
+ mlp_channels=cur_sa_mlps,
+ fps_mod=cur_fps_mod,
+ fps_sample_range_list=cur_fps_sample_range_list,
+ dilated_group=dilated_group[sa_index],
+ norm_cfg=norm_cfg,
+ cfg=sa_cfg,
+ bias=True))
+ skip_channel_list.append(sa_out_channel)
+
+ cur_aggregation_channel = aggregation_channels[sa_index]
+ if cur_aggregation_channel is None:
+ self.aggregation_mlps.append(None)
+ sa_in_channel = sa_out_channel
+ else:
+ self.aggregation_mlps.append(
+ ConvModule(
+ sa_out_channel,
+ cur_aggregation_channel,
+ conv_cfg=dict(type='Conv1d'),
+ norm_cfg=dict(type='BN1d'),
+ kernel_size=1,
+ bias=True))
+ sa_in_channel = cur_aggregation_channel
+
+ @auto_fp16(apply_to=('points', ))
+ def forward(self, points):
+ """Forward pass.
+
+ Args:
+ points (torch.Tensor): point coordinates with features,
+ with shape (B, N, 3 + input_feature_dim).
+
+ Returns:
+ dict[str, torch.Tensor]: Outputs of the last SA module.
+
+ - sa_xyz (torch.Tensor): The coordinates of sa features.
+ - sa_features (torch.Tensor): The features from the
+ last Set Aggregation Layers.
+ - sa_indices (torch.Tensor): Indices of the
+ input points.
+ """
+ xyz, features = self._split_point_feats(points)
+
+ batch, num_points = xyz.shape[:2]
+ indices = xyz.new_tensor(range(num_points)).unsqueeze(0).repeat(
+ batch, 1).long()
+
+ sa_xyz = [xyz]
+ sa_features = [features]
+ sa_indices = [indices]
+
+ out_sa_xyz = [xyz]
+ out_sa_features = [features]
+ out_sa_indices = [indices]
+
+ for i in range(self.num_sa):
+ cur_xyz, cur_features, cur_indices = self.SA_modules[i](
+ sa_xyz[i], sa_features[i])
+ if self.aggregation_mlps[i] is not None:
+ cur_features = self.aggregation_mlps[i](cur_features)
+ sa_xyz.append(cur_xyz)
+ sa_features.append(cur_features)
+ sa_indices.append(
+ torch.gather(sa_indices[-1], 1, cur_indices.long()))
+ if i in self.out_indices:
+ out_sa_xyz.append(sa_xyz[-1])
+ out_sa_features.append(sa_features[-1])
+ out_sa_indices.append(sa_indices[-1])
+
+ return dict(
+ sa_xyz=out_sa_xyz,
+ sa_features=out_sa_features,
+ sa_indices=out_sa_indices)
diff --git a/mmdet3d/models/backbones/pointnet2_sa_ssg.py b/mmdet3d/models/backbones/pointnet2_sa_ssg.py
new file mode 100644
index 0000000..c7b4152
--- /dev/null
+++ b/mmdet3d/models/backbones/pointnet2_sa_ssg.py
@@ -0,0 +1,143 @@
+# Copyright (c) OpenMMLab. All rights reserved.
+import torch
+from mmcv.runner import auto_fp16
+from torch import nn as nn
+
+from mmdet3d.ops import PointFPModule, build_sa_module
+from ..builder import BACKBONES
+from .base_pointnet import BasePointNet
+
+
+@BACKBONES.register_module()
+class PointNet2SASSG(BasePointNet):
+ """PointNet2 with Single-scale grouping.
+
+ Args:
+ in_channels (int): Input channels of point cloud.
+ num_points (tuple[int]): The number of points which each SA
+ module samples.
+ radius (tuple[float]): Sampling radii of each SA module.
+ num_samples (tuple[int]): The number of samples for ball
+ query in each SA module.
+ sa_channels (tuple[tuple[int]]): Out channels of each mlp in SA module.
+ fp_channels (tuple[tuple[int]]): Out channels of each mlp in FP module.
+ norm_cfg (dict): Config of normalization layer.
+ sa_cfg (dict): Config of set abstraction module, which may contain
+ the following keys and values:
+
+ - pool_mod (str): Pool method ('max' or 'avg') for SA modules.
+ - use_xyz (bool): Whether to use xyz as a part of features.
+ - normalize_xyz (bool): Whether to normalize xyz with radii in
+ each SA module.
+ """
+
+ def __init__(self,
+ in_channels,
+ num_points=(2048, 1024, 512, 256),
+ radius=(0.2, 0.4, 0.8, 1.2),
+ num_samples=(64, 32, 16, 16),
+ sa_channels=((64, 64, 128), (128, 128, 256), (128, 128, 256),
+ (128, 128, 256)),
+ fp_channels=((256, 256), (256, 256)),
+ norm_cfg=dict(type='BN2d'),
+ sa_cfg=dict(
+ type='PointSAModule',
+ pool_mod='max',
+ use_xyz=True,
+ normalize_xyz=True),
+ init_cfg=None):
+ super().__init__(init_cfg=init_cfg)
+ self.num_sa = len(sa_channels)
+ self.num_fp = len(fp_channels)
+
+ assert len(num_points) == len(radius) == len(num_samples) == len(
+ sa_channels)
+ assert len(sa_channels) >= len(fp_channels)
+
+ self.SA_modules = nn.ModuleList()
+ sa_in_channel = in_channels - 3 # number of channels without xyz
+ skip_channel_list = [sa_in_channel]
+
+ for sa_index in range(self.num_sa):
+ cur_sa_mlps = list(sa_channels[sa_index])
+ cur_sa_mlps = [sa_in_channel] + cur_sa_mlps
+ sa_out_channel = cur_sa_mlps[-1]
+
+ self.SA_modules.append(
+ build_sa_module(
+ num_point=num_points[sa_index],
+ radius=radius[sa_index],
+ num_sample=num_samples[sa_index],
+ mlp_channels=cur_sa_mlps,
+ norm_cfg=norm_cfg,
+ cfg=sa_cfg))
+ skip_channel_list.append(sa_out_channel)
+ sa_in_channel = sa_out_channel
+
+ self.FP_modules = nn.ModuleList()
+
+ fp_source_channel = skip_channel_list.pop()
+ fp_target_channel = skip_channel_list.pop()
+ for fp_index in range(len(fp_channels)):
+ cur_fp_mlps = list(fp_channels[fp_index])
+ cur_fp_mlps = [fp_source_channel + fp_target_channel] + cur_fp_mlps
+ self.FP_modules.append(PointFPModule(mlp_channels=cur_fp_mlps))
+ if fp_index != len(fp_channels) - 1:
+ fp_source_channel = cur_fp_mlps[-1]
+ fp_target_channel = skip_channel_list.pop()
+
+ @auto_fp16(apply_to=('points', ))
+ def forward(self, points):
+ """Forward pass.
+
+ Args:
+ points (torch.Tensor): point coordinates with features,
+ with shape (B, N, 3 + input_feature_dim).
+
+ Returns:
+ dict[str, list[torch.Tensor]]: Outputs after SA and FP modules.
+
+ - fp_xyz (list[torch.Tensor]): The coordinates of
+ each fp features.
+ - fp_features (list[torch.Tensor]): The features
+ from each Feature Propagate Layers.
+ - fp_indices (list[torch.Tensor]): Indices of the
+ input points.
+ """
+ xyz, features = self._split_point_feats(points)
+
+ batch, num_points = xyz.shape[:2]
+ indices = xyz.new_tensor(range(num_points)).unsqueeze(0).repeat(
+ batch, 1).long()
+
+ sa_xyz = [xyz]
+ sa_features = [features]
+ sa_indices = [indices]
+
+ for i in range(self.num_sa):
+ cur_xyz, cur_features, cur_indices = self.SA_modules[i](
+ sa_xyz[i], sa_features[i])
+ sa_xyz.append(cur_xyz)
+ sa_features.append(cur_features)
+ sa_indices.append(
+ torch.gather(sa_indices[-1], 1, cur_indices.long()))
+
+ fp_xyz = [sa_xyz[-1]]
+ fp_features = [sa_features[-1]]
+ fp_indices = [sa_indices[-1]]
+
+ for i in range(self.num_fp):
+ fp_features.append(self.FP_modules[i](
+ sa_xyz[self.num_sa - i - 1], sa_xyz[self.num_sa - i],
+ sa_features[self.num_sa - i - 1], fp_features[-1]))
+ fp_xyz.append(sa_xyz[self.num_sa - i - 1])
+ fp_indices.append(sa_indices[self.num_sa - i - 1])
+
+ ret = dict(
+ fp_xyz=fp_xyz,
+ fp_features=fp_features,
+ fp_indices=fp_indices,
+ sa_xyz=sa_xyz,
+ sa_features=sa_features,
+ sa_indices=sa_indices)
+ return ret
diff --git a/mmdet3d/models/backbones/second.py b/mmdet3d/models/backbones/second.py
new file mode 100644
index 0000000..680dbbe
--- /dev/null
+++ b/mmdet3d/models/backbones/second.py
@@ -0,0 +1,91 @@
+# Copyright (c) OpenMMLab. All rights reserved.
+import warnings
+
+from mmcv.cnn import build_conv_layer, build_norm_layer
+from mmcv.runner import BaseModule
+from torch import nn as nn
+
+from ..builder import BACKBONES
+
+
+@BACKBONES.register_module()
+class SECOND(BaseModule):
+ """Backbone network for SECOND/PointPillars/PartA2/MVXNet.
+
+ Args:
+ in_channels (int): Input channels.
+ out_channels (list[int]): Output channels for multi-scale feature maps.
+ layer_nums (list[int]): Number of layers in each stage.
+ layer_strides (list[int]): Strides of each stage.
+ norm_cfg (dict): Config dict of normalization layers.
+ conv_cfg (dict): Config dict of convolutional layers.
+ """
+
+ def __init__(self,
+ in_channels=128,
+ out_channels=[128, 128, 256],
+ layer_nums=[3, 5, 5],
+ layer_strides=[2, 2, 2],
+ norm_cfg=dict(type='BN', eps=1e-3, momentum=0.01),
+ conv_cfg=dict(type='Conv2d', bias=False),
+ init_cfg=None,
+ pretrained=None):
+ super(SECOND, self).__init__(init_cfg=init_cfg)
+ assert len(layer_strides) == len(layer_nums)
+ assert len(out_channels) == len(layer_nums)
+
+ in_filters = [in_channels, *out_channels[:-1]]
+ # note that when stride > 1, conv2d with same padding isn't
+ # equal to pad-conv2d. we should use pad-conv2d.
+ blocks = []
+ for i, layer_num in enumerate(layer_nums):
+ block = [
+ build_conv_layer(
+ conv_cfg,
+ in_filters[i],
+ out_channels[i],
+ 3,
+ stride=layer_strides[i],
+ padding=1),
+ build_norm_layer(norm_cfg, out_channels[i])[1],
+ nn.ReLU(inplace=True),
+ ]
+ for j in range(layer_num):
+ block.append(
+ build_conv_layer(
+ conv_cfg,
+ out_channels[i],
+ out_channels[i],
+ 3,
+ padding=1))
+ block.append(build_norm_layer(norm_cfg, out_channels[i])[1])
+ block.append(nn.ReLU(inplace=True))
+
+ block = nn.Sequential(*block)
+ blocks.append(block)
+
+ self.blocks = nn.ModuleList(blocks)
+
+ assert not (init_cfg and pretrained), \
+ 'init_cfg and pretrained cannot be setting at the same time'
+ if isinstance(pretrained, str):
+ warnings.warn('DeprecationWarning: pretrained is a deprecated, '
+ 'please use "init_cfg" instead')
+ self.init_cfg = dict(type='Pretrained', checkpoint=pretrained)
+ else:
+ self.init_cfg = dict(type='Kaiming', layer='Conv2d')
+
+ def forward(self, x):
+ """Forward function.
+
+ Args:
+ x (torch.Tensor): Input with shape (N, C, H, W).
+
+ Returns:
+ tuple[torch.Tensor]: Multi-scale features.
+ """
+ outs = []
+ for i in range(len(self.blocks)):
+ x = self.blocks[i](x)
+ outs.append(x)
+ return tuple(outs)
diff --git a/mmdet3d/models/builder.py b/mmdet3d/models/builder.py
new file mode 100644
index 0000000..fb8b8c2
--- /dev/null
+++ b/mmdet3d/models/builder.py
@@ -0,0 +1,137 @@
+# Copyright (c) OpenMMLab. All rights reserved.
+import warnings
+
+from mmcv.cnn import MODELS as MMCV_MODELS
+from mmcv.utils import Registry
+
+from mmdet.models.builder import BACKBONES as MMDET_BACKBONES
+from mmdet.models.builder import DETECTORS as MMDET_DETECTORS
+from mmdet.models.builder import HEADS as MMDET_HEADS
+from mmdet.models.builder import LOSSES as MMDET_LOSSES
+from mmdet.models.builder import NECKS as MMDET_NECKS
+from mmdet.models.builder import ROI_EXTRACTORS as MMDET_ROI_EXTRACTORS
+from mmdet.models.builder import SHARED_HEADS as MMDET_SHARED_HEADS
+from mmseg.models.builder import LOSSES as MMSEG_LOSSES
+
+MODELS = Registry('models', parent=MMCV_MODELS)
+
+BACKBONES = MODELS
+NECKS = MODELS
+ROI_EXTRACTORS = MODELS
+SHARED_HEADS = MODELS
+HEADS = MODELS
+LOSSES = MODELS
+DETECTORS = MODELS
+VOXEL_ENCODERS = MODELS
+MIDDLE_ENCODERS = MODELS
+FUSION_LAYERS = MODELS
+SEGMENTORS = MODELS
+
+
+def build_backbone(cfg):
+ """Build backbone."""
+ if cfg['type'] in BACKBONES._module_dict.keys():
+ return BACKBONES.build(cfg)
+ else:
+ return MMDET_BACKBONES.build(cfg)
+
+
+def build_neck(cfg):
+ """Build neck."""
+ if cfg['type'] in NECKS._module_dict.keys():
+ return NECKS.build(cfg)
+ else:
+ return MMDET_NECKS.build(cfg)
+
+
+def build_roi_extractor(cfg):
+ """Build RoI feature extractor."""
+ if cfg['type'] in ROI_EXTRACTORS._module_dict.keys():
+ return ROI_EXTRACTORS.build(cfg)
+ else:
+ return MMDET_ROI_EXTRACTORS.build(cfg)
+
+
+def build_shared_head(cfg):
+ """Build shared head of detector."""
+ if cfg['type'] in SHARED_HEADS._module_dict.keys():
+ return SHARED_HEADS.build(cfg)
+ else:
+ return MMDET_SHARED_HEADS.build(cfg)
+
+
+def build_head(cfg):
+ """Build head."""
+ if cfg['type'] in HEADS._module_dict.keys():
+ return HEADS.build(cfg)
+ else:
+ return MMDET_HEADS.build(cfg)
+
+
+def build_loss(cfg):
+ """Build loss function."""
+ if cfg['type'] in LOSSES._module_dict.keys():
+ return LOSSES.build(cfg)
+ elif cfg['type'] in MMDET_LOSSES._module_dict.keys():
+ return MMDET_LOSSES.build(cfg)
+ else:
+ return MMSEG_LOSSES.build(cfg)
+
+
+def build_detector(cfg, train_cfg=None, test_cfg=None):
+ """Build detector."""
+ if train_cfg is not None or test_cfg is not None:
+ warnings.warn(
+ 'train_cfg and test_cfg is deprecated, '
+ 'please specify them in model', UserWarning)
+ assert cfg.get('train_cfg') is None or train_cfg is None, \
+ 'train_cfg specified in both outer field and model field '
+ assert cfg.get('test_cfg') is None or test_cfg is None, \
+ 'test_cfg specified in both outer field and model field '
+ if cfg['type'] in DETECTORS._module_dict.keys():
+ return DETECTORS.build(
+ cfg, default_args=dict(train_cfg=train_cfg, test_cfg=test_cfg))
+ else:
+ return MMDET_DETECTORS.build(
+ cfg, default_args=dict(train_cfg=train_cfg, test_cfg=test_cfg))
+
+
+def build_segmentor(cfg, train_cfg=None, test_cfg=None):
+ """Build segmentor."""
+ if train_cfg is not None or test_cfg is not None:
+ warnings.warn(
+ 'train_cfg and test_cfg is deprecated, '
+ 'please specify them in model', UserWarning)
+ assert cfg.get('train_cfg') is None or train_cfg is None, \
+ 'train_cfg specified in both outer field and model field '
+ assert cfg.get('test_cfg') is None or test_cfg is None, \
+ 'test_cfg specified in both outer field and model field '
+ return SEGMENTORS.build(
+ cfg, default_args=dict(train_cfg=train_cfg, test_cfg=test_cfg))
+
+
+def build_model(cfg, train_cfg=None, test_cfg=None):
+ """A function warpper for building 3D detector or segmentor according to
+ cfg.
+
+ Should be deprecated in the future.
+ """
+ if cfg.type in ['EncoderDecoder3D']:
+ return build_segmentor(cfg, train_cfg=train_cfg, test_cfg=test_cfg)
+ else:
+ return build_detector(cfg, train_cfg=train_cfg, test_cfg=test_cfg)
+
+
+def build_voxel_encoder(cfg):
+ """Build voxel encoder."""
+ return VOXEL_ENCODERS.build(cfg)
+
+
+def build_middle_encoder(cfg):
+ """Build middle level encoder."""
+ return MIDDLE_ENCODERS.build(cfg)
+
+
+def build_fusion_layer(cfg):
+ """Build fusion layer."""
+ return FUSION_LAYERS.build(cfg)
diff --git a/mmdet3d/models/decode_heads/__init__.py b/mmdet3d/models/decode_heads/__init__.py
new file mode 100644
index 0000000..da7bd30
--- /dev/null
+++ b/mmdet3d/models/decode_heads/__init__.py
@@ -0,0 +1,7 @@
+# Copyright (c) OpenMMLab. All rights reserved.
+from .dgcnn_head import DGCNNHead
+from .paconv_head import PAConvHead
+from .pointnet2_head import PointNet2Head
+from .td3d_instance_head import TD3DInstanceHead
+
+__all__ = ['PointNet2Head', 'DGCNNHead', 'PAConvHead']
diff --git a/mmdet3d/models/decode_heads/decode_head.py b/mmdet3d/models/decode_heads/decode_head.py
new file mode 100644
index 0000000..6ccbfe0
--- /dev/null
+++ b/mmdet3d/models/decode_heads/decode_head.py
@@ -0,0 +1,123 @@
+# Copyright (c) OpenMMLab. All rights reserved.
+from abc import ABCMeta, abstractmethod
+
+from mmcv.cnn import normal_init
+from mmcv.runner import BaseModule, auto_fp16, force_fp32
+from torch import nn as nn
+
+from mmseg.models.builder import build_loss
+
+
+class Base3DDecodeHead(BaseModule, metaclass=ABCMeta):
+ """Base class for BaseDecodeHead.
+
+ Args:
+ channels (int): Channels after modules, before conv_seg.
+ num_classes (int): Number of classes.
+ dropout_ratio (float, optional): Ratio of dropout layer. Default: 0.5.
+ conv_cfg (dict, optional): Config of conv layers.
+ Default: dict(type='Conv1d').
+ norm_cfg (dict, optional): Config of norm layers.
+ Default: dict(type='BN1d').
+ act_cfg (dict, optional): Config of activation layers.
+ Default: dict(type='ReLU').
+ loss_decode (dict, optional): Config of decode loss.
+ Default: dict(type='CrossEntropyLoss').
+ ignore_index (int, optional): The label index to be ignored.
+ When using masked BCE loss, ignore_index should be set to None.
+ Default: 255.
+ """
+
+ def __init__(self,
+ channels,
+ num_classes,
+ dropout_ratio=0.5,
+ conv_cfg=dict(type='Conv1d'),
+ norm_cfg=dict(type='BN1d'),
+ act_cfg=dict(type='ReLU'),
+ loss_decode=dict(
+ type='CrossEntropyLoss',
+ use_sigmoid=False,
+ class_weight=None,
+ loss_weight=1.0),
+ ignore_index=255,
+ init_cfg=None):
+ super(Base3DDecodeHead, self).__init__(init_cfg=init_cfg)
+ self.channels = channels
+ self.num_classes = num_classes
+ self.dropout_ratio = dropout_ratio
+ self.conv_cfg = conv_cfg
+ self.norm_cfg = norm_cfg
+ self.act_cfg = act_cfg
+ self.loss_decode = build_loss(loss_decode)
+ self.ignore_index = ignore_index
+
+ self.conv_seg = nn.Conv1d(channels, num_classes, kernel_size=1)
+ if dropout_ratio > 0:
+ self.dropout = nn.Dropout(dropout_ratio)
+ else:
+ self.dropout = None
+ self.fp16_enabled = False
+
+ def init_weights(self):
+ """Initialize weights of classification layer."""
+ super().init_weights()
+ normal_init(self.conv_seg, mean=0, std=0.01)
+
+ @auto_fp16()
+ @abstractmethod
+ def forward(self, inputs):
+ """Placeholder of forward function."""
+ pass
+
+ def forward_train(self, inputs, img_metas, pts_semantic_mask, train_cfg):
+ """Forward function for training.
+
+ Args:
+ inputs (list[torch.Tensor]): List of multi-level point features.
+ img_metas (list[dict]): Meta information of each sample.
+ pts_semantic_mask (torch.Tensor): Semantic segmentation masks
+ used if the architecture supports semantic segmentation task.
+ train_cfg (dict): The training config.
+
+ Returns:
+ dict[str, Tensor]: a dictionary of loss components
+ """
+ seg_logits = self.forward(inputs)
+ losses = self.losses(seg_logits, pts_semantic_mask)
+ return losses
+
+ def forward_test(self, inputs, img_metas, test_cfg):
+ """Forward function for testing.
+
+ Args:
+ inputs (list[Tensor]): List of multi-level point features.
+ img_metas (list[dict]): Meta information of each sample.
+ test_cfg (dict): The testing config.
+
+ Returns:
+ Tensor: Output segmentation map.
+ """
+ return self.forward(inputs)
+
+ def cls_seg(self, feat):
+ """Classify each points."""
+ if self.dropout is not None:
+ feat = self.dropout(feat)
+ output = self.conv_seg(feat)
+ return output
+
+ @force_fp32(apply_to=('seg_logit', ))
+ def losses(self, seg_logit, seg_label):
+ """Compute semantic segmentation loss.
+
+ Args:
+ seg_logit (torch.Tensor): Predicted per-point segmentation logits
+ of shape [B, num_classes, N].
+ seg_label (torch.Tensor): Ground-truth segmentation label of
+ shape [B, N].
+ """
+ loss = dict()
+ loss['loss_sem_seg'] = self.loss_decode(
+ seg_logit, seg_label, ignore_index=self.ignore_index)
+ return loss
diff --git a/mmdet3d/models/decode_heads/dgcnn_head.py b/mmdet3d/models/decode_heads/dgcnn_head.py
new file mode 100644
index 0000000..1249b3d
--- /dev/null
+++ b/mmdet3d/models/decode_heads/dgcnn_head.py
@@ -0,0 +1,67 @@
+# Copyright (c) OpenMMLab. All rights reserved.
+from mmcv.cnn.bricks import ConvModule
+
+from mmdet3d.ops import DGCNNFPModule
+from ..builder import HEADS
+from .decode_head import Base3DDecodeHead
+
+
+@HEADS.register_module()
+class DGCNNHead(Base3DDecodeHead):
+ r"""DGCNN decoder head.
+
+ Decoder head used in `DGCNN `_.
+ Refer to the
+ `reimplementation code `_.
+
+ Args:
+ fp_channels (tuple[int], optional): Tuple of mlp channels in feature
+ propagation (FP) modules. Defaults to (1216, 512).
+ """
+
+ def __init__(self, fp_channels=(1216, 512), **kwargs):
+ super(DGCNNHead, self).__init__(**kwargs)
+
+ self.FP_module = DGCNNFPModule(
+ mlp_channels=fp_channels, act_cfg=self.act_cfg)
+
+ # https://github.com/charlesq34/pointnet2/blob/master/models/pointnet2_sem_seg.py#L40
+ self.pre_seg_conv = ConvModule(
+ fp_channels[-1],
+ self.channels,
+ kernel_size=1,
+ bias=False,
+ conv_cfg=self.conv_cfg,
+ norm_cfg=self.norm_cfg,
+ act_cfg=self.act_cfg)
+
+ def _extract_input(self, feat_dict):
+ """Extract inputs from features dictionary.
+
+ Args:
+ feat_dict (dict): Feature dict from backbone.
+
+ Returns:
+ torch.Tensor: points for decoder.
+ """
+ fa_points = feat_dict['fa_points']
+
+ return fa_points
+
+ def forward(self, feat_dict):
+ """Forward pass.
+
+ Args:
+ feat_dict (dict): Feature dict from backbone.
+
+ Returns:
+ torch.Tensor: Segmentation map of shape [B, num_classes, N].
+ """
+ fa_points = self._extract_input(feat_dict)
+
+ fp_points = self.FP_module(fa_points)
+ fp_points = fp_points.transpose(1, 2).contiguous()
+ output = self.pre_seg_conv(fp_points)
+ output = self.cls_seg(output)
+
+ return output
diff --git a/mmdet3d/models/decode_heads/paconv_head.py b/mmdet3d/models/decode_heads/paconv_head.py
new file mode 100644
index 0000000..63cc3fd
--- /dev/null
+++ b/mmdet3d/models/decode_heads/paconv_head.py
@@ -0,0 +1,63 @@
+# Copyright (c) OpenMMLab. All rights reserved.
+from mmcv.cnn.bricks import ConvModule
+
+from ..builder import HEADS
+from .pointnet2_head import PointNet2Head
+
+
+@HEADS.register_module()
+class PAConvHead(PointNet2Head):
+ r"""PAConv decoder head.
+
+ Decoder head used in `PAConv `_.
+ Refer to the `official code `_.
+
+ Args:
+ fp_channels (tuple[tuple[int]]): Tuple of mlp channels in FP modules.
+ fp_norm_cfg (dict): Config of norm layers used in FP modules.
+ Default: dict(type='BN2d').
+ """
+
+ def __init__(self,
+ fp_channels=((768, 256, 256), (384, 256, 256),
+ (320, 256, 128), (128 + 6, 128, 128, 128)),
+ fp_norm_cfg=dict(type='BN2d'),
+ **kwargs):
+ super(PAConvHead, self).__init__(fp_channels, fp_norm_cfg, **kwargs)
+
+ # https://github.com/CVMI-Lab/PAConv/blob/main/scene_seg/model/pointnet2/pointnet2_paconv_seg.py#L53
+ # PointNet++'s decoder conv has bias while PAConv's doesn't have
+ # so we need to rebuild it here
+ self.pre_seg_conv = ConvModule(
+ fp_channels[-1][-1],
+ self.channels,
+ kernel_size=1,
+ bias=False,
+ conv_cfg=self.conv_cfg,
+ norm_cfg=self.norm_cfg,
+ act_cfg=self.act_cfg)
+
+ def forward(self, feat_dict):
+ """Forward pass.
+
+ Args:
+ feat_dict (dict): Feature dict from backbone.
+
+ Returns:
+ torch.Tensor: Segmentation map of shape [B, num_classes, N].
+ """
+ sa_xyz, sa_features = self._extract_input(feat_dict)
+
+ # PointNet++ doesn't use the first level of `sa_features` as input
+ # while PAConv inputs it through skip-connection
+ fp_feature = sa_features[-1]
+
+ for i in range(self.num_fp):
+ # consume the points in a bottom-up manner
+ fp_feature = self.FP_modules[i](sa_xyz[-(i + 2)], sa_xyz[-(i + 1)],
+ sa_features[-(i + 2)], fp_feature)
+
+ output = self.pre_seg_conv(fp_feature)
+ output = self.cls_seg(output)
+
+ return output
diff --git a/mmdet3d/models/decode_heads/pointnet2_head.py b/mmdet3d/models/decode_heads/pointnet2_head.py
new file mode 100644
index 0000000..28b677e
--- /dev/null
+++ b/mmdet3d/models/decode_heads/pointnet2_head.py
@@ -0,0 +1,85 @@
+# Copyright (c) OpenMMLab. All rights reserved.
+from mmcv.cnn.bricks import ConvModule
+from torch import nn as nn
+
+from mmdet3d.ops import PointFPModule
+from ..builder import HEADS
+from .decode_head import Base3DDecodeHead
+
+
+@HEADS.register_module()
+class PointNet2Head(Base3DDecodeHead):
+ r"""PointNet2 decoder head.
+
+ Decoder head used in `PointNet++ `_.
+ Refer to the `official code `_.
+
+ Args:
+ fp_channels (tuple[tuple[int]]): Tuple of mlp channels in FP modules.
+ fp_norm_cfg (dict): Config of norm layers used in FP modules.
+ Default: dict(type='BN2d').
+ """
+
+ def __init__(self,
+ fp_channels=((768, 256, 256), (384, 256, 256),
+ (320, 256, 128), (128, 128, 128, 128)),
+ fp_norm_cfg=dict(type='BN2d'),
+ **kwargs):
+ super(PointNet2Head, self).__init__(**kwargs)
+
+ self.num_fp = len(fp_channels)
+ self.FP_modules = nn.ModuleList()
+ for cur_fp_mlps in fp_channels:
+ self.FP_modules.append(
+ PointFPModule(mlp_channels=cur_fp_mlps, norm_cfg=fp_norm_cfg))
+
+ # https://github.com/charlesq34/pointnet2/blob/master/models/pointnet2_sem_seg.py#L40
+ self.pre_seg_conv = ConvModule(
+ fp_channels[-1][-1],
+ self.channels,
+ kernel_size=1,
+ bias=True,
+ conv_cfg=self.conv_cfg,
+ norm_cfg=self.norm_cfg,
+ act_cfg=self.act_cfg)
+
+ def _extract_input(self, feat_dict):
+ """Extract inputs from features dictionary.
+
+ Args:
+ feat_dict (dict): Feature dict from backbone.
+
+ Returns:
+ list[torch.Tensor]: Coordinates of multiple levels of points.
+ list[torch.Tensor]: Features of multiple levels of points.
+ """
+ sa_xyz = feat_dict['sa_xyz']
+ sa_features = feat_dict['sa_features']
+ assert len(sa_xyz) == len(sa_features)
+
+ return sa_xyz, sa_features
+
+ def forward(self, feat_dict):
+ """Forward pass.
+
+ Args:
+ feat_dict (dict): Feature dict from backbone.
+
+ Returns:
+ torch.Tensor: Segmentation map of shape [B, num_classes, N].
+ """
+ sa_xyz, sa_features = self._extract_input(feat_dict)
+
+ # https://github.com/charlesq34/pointnet2/blob/master/models/pointnet2_sem_seg.py#L24
+ sa_features[0] = None
+
+ fp_feature = sa_features[-1]
+
+ for i in range(self.num_fp):
+ # consume the points in a bottom-up manner
+ fp_feature = self.FP_modules[i](sa_xyz[-(i + 2)], sa_xyz[-(i + 1)],
+ sa_features[-(i + 2)], fp_feature)
+ output = self.pre_seg_conv(fp_feature)
+ output = self.cls_seg(output)
+
+ return output
diff --git a/mmdet3d/models/decode_heads/td3d_instance_head.py b/mmdet3d/models/decode_heads/td3d_instance_head.py
new file mode 100644
index 0000000..fadbf6e
--- /dev/null
+++ b/mmdet3d/models/decode_heads/td3d_instance_head.py
@@ -0,0 +1,619 @@
+try:
+ import MinkowskiEngine as ME
+except ImportError:
+ # Please follow getting_started.md to install MinkowskiEngine.
+ pass
+
+import torch
+from torch import nn
+import torch.nn.functional as F
+
+from mmcv.runner import BaseModule
+from mmcv.cnn import Scale, bias_init_with_prob
+from mmdet.core.bbox.builder import build_assigner
+from mmdet3d.models.builder import HEADS, build_backbone, build_loss
+from mmcv.ops import nms3d, nms3d_normal
+
+from mmdet.core.bbox.builder import BBOX_ASSIGNERS
+from mmdet3d.models.builder import (ROI_EXTRACTORS, build_roi_extractor)
+from mmdet3d.models.dense_heads.ngfc_head import get_face_distances
+
+@ROI_EXTRACTORS.register_module()
+class Mink3DRoIExtractor:
+ def __init__(self, voxel_size, padding, min_pts_threshold):
+ # min_pts_threshold: minimal number of points per roi
+ self.voxel_size = voxel_size
+ self.padding = padding
+ self.min_pts_threshold = min_pts_threshold
+
+ # per scene and per level
+ def _extract_single(self, coordinates, features, voxel_size, rois, scores, labels):
+ # coordinates: of shape (n_points, 3)
+ # features: of shape (n_points, c)
+ # voxel_size: float
+ # rois: of shape (n_rois, 7)
+ # -> new indices of shape n_new_points
+ # -> new coordinates of shape (n_new_points, 3)
+ # -> new features of shape (n_new_points, c + 3)
+ # -> new rois of shape (n_new_rois, 7)
+ # -> new scores of shape (n_new_rois)
+ # -> new labels of shape (n_new_rois)
+ n_points = len(coordinates)
+ n_boxes = len(rois)
+ if n_boxes == 0:
+ return (coordinates.new_zeros(0),
+ coordinates.new_zeros((0, 3)),
+ features.new_zeros((0, features.shape[1])),
+ features.new_zeros((0, 7)),
+ features.new_zeros(0),
+ coordinates.new_zeros(0))
+ points = coordinates * self.voxel_size
+ points = points.unsqueeze(1).expand(n_points, n_boxes, 3)
+ rois = rois.unsqueeze(0).expand(n_points, n_boxes, 7)
+ face_distances = get_face_distances(points, rois)
+ inside_condition = face_distances.min(dim=-1).values > 0
+ min_pts_condition = inside_condition.sum(dim=0) > self.min_pts_threshold
+ inside_condition = inside_condition[:, min_pts_condition]
+ rois = rois[0, min_pts_condition]
+ scores = scores[min_pts_condition]
+ labels = labels[min_pts_condition]
+ nonzero = torch.nonzero(inside_condition)
+ new_coordinates = coordinates[nonzero[:, 0]]
+ return nonzero[:, 1], new_coordinates, features[nonzero[:, 0]], rois, scores, labels
+
+ def extract(self, tensors, levels, rois, scores, labels):
+ # tensors: list[SparseTensor] of len n_levels
+ # levels: list[Tensor] of len batch_size;
+ # each of shape n_rois_i
+ # rois: list[BaseInstance3DBoxes] of len batch_size;
+ # each of len n_rois_i
+ # -> list[SparseTensor] of len n_levels
+ # -> list[Tensor] of len n_levels;
+ # contains scene id for each extracted roi
+ # -> list[list[BaseInstance3DBoxes]] of len n_levels;
+ # each of len batch_size; just splitted rois
+ # per level and per scene
+ box_type = rois[0].__class__
+ with_yaw = rois[0].with_yaw
+ for i, roi in enumerate(rois):
+ rois[i] = torch.cat((
+ roi.gravity_center,
+ roi.tensor[:, 3:6] + self.padding,
+ roi.tensor[:, 6:]), dim=1)
+
+ new_tensors, new_ids, new_rois, new_scores, new_labels = [], [], [], [], []
+ for level, x in enumerate(tensors):
+ voxel_size = self.voxel_size * x.tensor_stride[0]
+ new_coordinates, new_features, new_roi, new_score, new_label, ids = [], [], [], [], [], []
+ n_rois = 0
+ for i, (coordinates, features) in enumerate(
+ zip(*x.decomposed_coordinates_and_features)):
+ roi = rois[i][levels[i] == level]
+ score = scores[i][levels[i] == level]
+ label = labels[i][levels[i] == level]
+ new_index, new_coordinate, new_feature, roi, score, label = self._extract_single(
+ coordinates, features, voxel_size, roi, score, label)
+ new_index = new_index + n_rois
+ n_rois += len(roi)
+ new_coordinate = torch.cat((
+ new_index.unsqueeze(1), new_coordinate), dim=1)
+ new_coordinates.append(new_coordinate)
+ new_features.append(new_feature)
+ ids += [i] * len(roi)
+ roi = torch.cat((roi[:, :3],
+ roi[:, 3:6] - self.padding,
+ roi[:, 6:]), dim=1)
+ new_roi.append(box_type(roi, with_yaw=with_yaw, origin=(.5, .5, .5)))
+ new_score.append(score)
+ new_label.append(label)
+ new_tensors.append(ME.SparseTensor(
+ torch.cat(new_features),
+ torch.cat(new_coordinates).float(),
+ tensor_stride=x.tensor_stride))
+ new_ids.append(x.coordinates.new_tensor(ids))
+ new_rois.append(new_roi)
+ new_scores.append(new_score)
+ new_labels.append(new_label)
+
+ return new_tensors, new_ids, new_rois, new_scores, new_labels
+
+@BBOX_ASSIGNERS.register_module()
+class MaxIoU3DAssigner:
+ def __init__(self, threshold):
+ # threshold: for positive IoU
+ self.threshold = threshold
+
+ def assign(self, rois, gt_bboxes):
+ # rois: BaseInstance3DBoxes
+ # gt_bboxes: BaseInstance3DBoxes
+ # -> object id or -1 for each point
+ ious = rois.overlaps(rois, gt_bboxes.to(rois.device))
+ values, indices = ious.max(dim=1)
+ indices = torch.where(values > self.threshold, indices, -1)
+ return indices
+
+@HEADS.register_module()
+class TD3DInstanceHead(BaseModule):
+ def __init__(self,
+ n_classes,
+ in_channels,
+ n_levels,
+ unet,
+ n_reg_outs,
+ voxel_size,
+ padding,
+ first_assigner,
+ second_assigner,
+ roi_extractor,
+ reg_loss=dict(type='SmoothL1Loss'),
+ bbox_loss=dict(type='AxisAlignedIoULoss', mode="diou"),
+ cls_loss=dict(type='FocalLoss'),
+ inst_loss=build_loss(dict(type='CrossEntropyLoss', use_sigmoid=True)),
+ train_cfg=None,
+ test_cfg=None):
+ super(TD3DInstanceHead, self).__init__()
+ self.voxel_size = voxel_size
+ self.unet = build_backbone(unet)
+ self.first_assigner = build_assigner(first_assigner)
+ self.second_assigner = build_assigner(second_assigner)
+ self.roi_extractor = build_roi_extractor(roi_extractor)
+ self.reg_loss = build_loss(reg_loss)
+ self.bbox_loss = build_loss(bbox_loss)
+ self.cls_loss = build_loss(cls_loss)
+ self.inst_loss = inst_loss
+ self.train_cfg = train_cfg
+ self.test_cfg = test_cfg
+ self.padding = padding
+ self.n_classes = n_classes
+ self._init_layers(n_classes, in_channels, n_levels, n_reg_outs)
+
+ def _init_layers(self, n_classes, in_channels, n_levels, n_reg_outs):
+ self.reg_conv = ME.MinkowskiConvolution(
+ in_channels, n_reg_outs, kernel_size=1, bias=True, dimension=3)
+ self.cls_conv = ME.MinkowskiConvolution(
+ in_channels, n_classes, kernel_size=1, bias=True, dimension=3)
+
+
+ def init_weights(self):
+ nn.init.normal_(self.reg_conv.kernel, std=0.01)
+ nn.init.normal_(self.cls_conv.kernel, std=0.01)
+ nn.init.constant_(self.cls_conv.bias, bias_init_with_prob(0.01))
+
+ # per level
+ def _forward_first_single(self, x):
+ reg_pred = torch.exp(self.reg_conv(x).features)
+ cls_pred = self.cls_conv(x).features
+
+ reg_preds, cls_preds, locations = [], [], []
+ for permutation in x.decomposition_permutations:
+ reg_preds.append(reg_pred[permutation])
+ cls_preds.append(cls_pred[permutation])
+ locations.append(x.coordinates[permutation][:, 1:] * self.voxel_size)
+ return reg_preds, cls_preds, locations
+
+ def _forward_first(self, x):
+ reg_preds, cls_preds, locations = [], [], []
+ for i in range(len(x)):
+ reg_pred, cls_pred, point = self._forward_first_single(x[i])
+ reg_preds.append(reg_pred)
+ cls_preds.append(cls_pred)
+ locations.append(point)
+ return reg_preds, cls_preds, locations
+
+ def _forward_second(self, x, targets, bbox_list):
+ rois = [b[0] for b in bbox_list]
+ scores = [b[1] for b in bbox_list]
+ labels = [b[2] for b in bbox_list]
+ levels = [torch.zeros(len(b[0])) for b in bbox_list]
+
+ feats_with_targets = ME.SparseTensor(torch.cat((x.features, targets), axis=1), x.coordinates)
+ tensors, ids, rois, scores, labels = self.roi_extractor.extract([feats_with_targets], levels, rois, scores, labels)
+ if tensors[0].features.shape[0] == 0:
+ return (targets.new_zeros((0, 1)),
+ targets.new_zeros((0, 1)),
+ targets.new_zeros(0),
+ targets.new_zeros(0),
+ [targets.new_zeros((0, 7)) for i in range(len(bbox_list))],
+ [targets.new_zeros(0) for i in range(len(bbox_list))],
+ [targets.new_zeros(0) for i in range(len(bbox_list))])
+
+ feats = ME.SparseTensor(tensors[0].features[:, :-2], tensors[0].coordinates)
+ targets = tensors[0].features[:, -2:]
+
+ preds = self.unet(feats).features
+ return preds, targets, feats.coordinates[:, 0].long(), ids[0], rois[0], scores[0], labels[0]
+
+
+ @staticmethod
+ def _bbox_to_loss(bbox):
+ """Transform box to the axis-aligned or rotated iou loss format.
+ Args:
+ bbox (Tensor): 3D box of shape (N, 6) or (N, 7).
+ Returns:
+ Tensor: Transformed 3D box of shape (N, 6) or (N, 7).
+ """
+ # rotated iou loss accepts (x, y, z, w, h, l, heading)
+ if bbox.shape[-1] != 6:
+ return bbox
+
+ # axis-aligned case: x, y, z, w, h, l -> x1, y1, z1, x2, y2, z2
+ return torch.stack(
+ (bbox[..., 0] - bbox[..., 3] / 2, bbox[..., 1] - bbox[..., 4] / 2,
+ bbox[..., 2] - bbox[..., 5] / 2, bbox[..., 0] + bbox[..., 3] / 2,
+ bbox[..., 1] + bbox[..., 4] / 2, bbox[..., 2] + bbox[..., 5] / 2),
+ dim=-1)
+
+ @staticmethod
+ def _bbox_pred_to_bbox(points, bbox_pred):
+ """Transform predicted bbox parameters to bbox.
+ Args:
+ points (Tensor): Final locations of shape (N, 3)
+ bbox_pred (Tensor): Predicted bbox parameters of shape (N, 6)
+ or (N, 8).
+ Returns:
+ Tensor: Transformed 3D box of shape (N, 6) or (N, 7).
+ """
+ if bbox_pred.shape[0] == 0:
+ return bbox_pred
+
+ x_center = points[:, 0] + (bbox_pred[:, 1] - bbox_pred[:, 0]) / 2
+ y_center = points[:, 1] + (bbox_pred[:, 3] - bbox_pred[:, 2]) / 2
+ z_center = points[:, 2] + (bbox_pred[:, 5] - bbox_pred[:, 4]) / 2
+
+ # dx_min, dx_max, dy_min, dy_max, dz_min, dz_max -> x, y, z, w, l, h
+ base_bbox = torch.stack([
+ x_center,
+ y_center,
+ z_center,
+ bbox_pred[:, 0] + bbox_pred[:, 1],
+ bbox_pred[:, 2] + bbox_pred[:, 3],
+ bbox_pred[:, 4] + bbox_pred[:, 5],
+ ], -1)
+
+ # axis-aligned case
+ if bbox_pred.shape[1] == 6:
+ return base_bbox
+
+ # rotated case: ..., sin(2a)ln(q), cos(2a)ln(q)
+ scale = bbox_pred[:, 0] + bbox_pred[:, 1] + \
+ bbox_pred[:, 2] + bbox_pred[:, 3]
+ q = torch.exp(
+ torch.sqrt(
+ torch.pow(bbox_pred[:, 6], 2) + torch.pow(bbox_pred[:, 7], 2)))
+ alpha = 0.5 * torch.atan2(bbox_pred[:, 6], bbox_pred[:, 7])
+ return torch.stack(
+ (x_center, y_center, z_center, scale / (1 + q), scale /
+ (1 + q) * q, bbox_pred[:, 5] + bbox_pred[:, 4], alpha),
+ dim=-1)
+
+ # per scene
+ def _loss_first_single(self,
+ bbox_preds,
+ cls_preds,
+ points,
+ gt_bboxes,
+ gt_labels,
+ img_meta):
+
+ assigned_ids = self.first_assigner.assign(points, gt_bboxes, img_meta)
+ bbox_preds = torch.cat(bbox_preds)
+ cls_preds = torch.cat(cls_preds)
+ points = torch.cat(points)
+
+ # cls loss
+ n_classes = cls_preds.shape[1]
+ pos_mask = assigned_ids >= 0
+ cls_targets = torch.where(pos_mask, gt_labels[assigned_ids], n_classes)
+ avg_factor = max(pos_mask.sum(), 1)
+ cls_loss = self.cls_loss(cls_preds, cls_targets, avg_factor=avg_factor)
+
+ # bbox loss
+ pos_bbox_preds = bbox_preds[pos_mask]
+ if pos_mask.sum() > 0:
+ pos_points = points[pos_mask]
+ pos_bbox_preds = bbox_preds[pos_mask]
+ bbox_targets = torch.cat((gt_bboxes.gravity_center, gt_bboxes.tensor[:, 3:]), dim=1)
+ pos_bbox_targets = bbox_targets.to(points.device)[assigned_ids][pos_mask]
+ pos_bbox_targets = torch.cat((
+ pos_bbox_targets[:, :3],
+ pos_bbox_targets[:, 3:6] + self.padding,
+ pos_bbox_targets[:, 6:]), dim=1)
+ if pos_bbox_preds.shape[1] == 6:
+ pos_bbox_targets = pos_bbox_targets[:, :6]
+ bbox_loss = self.bbox_loss(
+ self._bbox_to_loss(
+ self._bbox_pred_to_bbox(pos_points, pos_bbox_preds)),
+ self._bbox_to_loss(pos_bbox_targets))
+ else:
+ bbox_loss = pos_bbox_preds.sum()
+ return bbox_loss, cls_loss
+
+ def _loss_first(self, bbox_preds, cls_preds, points,
+ gt_bboxes, gt_labels, img_metas):
+ bbox_losses, cls_losses = [], []
+ for i in range(len(img_metas)):
+ bbox_loss, cls_loss = self._loss_first_single(
+ bbox_preds=[x[i] for x in bbox_preds],
+ cls_preds=[x[i] for x in cls_preds],
+ points=[x[i] for x in points],
+ img_meta=img_metas[i],
+ gt_bboxes=gt_bboxes[i],
+ gt_labels=gt_labels[i])
+ bbox_losses.append(bbox_loss)
+ cls_losses.append(cls_loss)
+ return dict(bbox_loss=torch.mean(torch.stack(bbox_losses)),
+ cls_loss=torch.mean(torch.stack(cls_losses)))
+
+ def _loss_second(self, cls_preds, targets, v2r, r2scene, rois, gt_idxs,
+ gt_bboxes, gt_labels, img_metas):
+ v2scene = r2scene[v2r]
+ inst_losses = []
+ for i in range(len(img_metas)):
+ inst_loss = self._loss_second_single(
+ cls_preds=cls_preds[v2scene == i],
+ targets=targets[v2scene == i],
+ v2r=v2r[v2scene == i],
+ rois=rois[i],
+ gt_idxs=gt_idxs[i],
+ gt_bboxes=gt_bboxes[i],
+ gt_labels=gt_labels[i],
+ img_meta=img_metas[i])
+ inst_losses.append(inst_loss)
+ return dict(inst_loss=torch.mean(torch.stack(inst_losses)))
+
+ def _loss_second_single(self, cls_preds, targets, v2r, rois, gt_idxs, gt_bboxes, gt_labels, img_meta):
+ if len(rois) == 0 or cls_preds.shape[0] == 0:
+ return cls_preds.sum().float()
+ v2r = v2r - v2r.min()
+ assert len(torch.unique(v2r)) == len(rois)
+ assert torch.all(torch.unique(v2r) == torch.arange(0, v2r.max() + 1).to(v2r.device))
+ assert torch.max(gt_idxs) < len(gt_bboxes)
+
+ v2bbox = gt_idxs[v2r.long()]
+ assert torch.unique(v2bbox)[0] != -1
+ inst_targets = targets[:, 0]
+ seg_targets = targets[:, 1]
+
+ seg_preds = cls_preds[:, :-1]
+ inst_preds = cls_preds[:, -1]
+
+ labels = v2bbox == inst_targets
+
+ seg_targets[seg_targets == -1] = self.n_classes
+ seg_loss = self.cls_loss(seg_preds, seg_targets.long())
+
+ inst_loss = self.inst_loss(inst_preds, labels)
+ return inst_loss + seg_loss
+
+ def forward_train(self,
+ x,
+ targets,
+ points,
+ gt_bboxes,
+ gt_labels,
+ pts_semantic_mask,
+ pts_instance_mask,
+ img_metas):
+ #first stage
+ bbox_preds, cls_preds, locations = self._forward_first(x[1:])
+ losses = self._loss_first(bbox_preds, cls_preds, locations,
+ gt_bboxes, gt_labels, img_metas)
+ #second stage
+ bbox_list = self._get_bboxes_train(bbox_preds, cls_preds, locations, gt_bboxes, img_metas)
+ assigned_bbox_list = []
+ for i in range(len(bbox_list)):
+ assigned_ids = self.second_assigner.assign(bbox_list[i][0], gt_bboxes[i])
+ gt_idxs = bbox_list[i][2]
+ gt_idxs[gt_idxs != assigned_ids] = -1
+
+ boxes = bbox_list[i][0][gt_idxs != -1]
+ scores = bbox_list[i][1][gt_idxs != -1]
+ gt_idxs = gt_idxs[gt_idxs != -1]
+
+ if len(boxes) != 0:
+ gt_idxs_one_hot = torch.nn.functional.one_hot(gt_idxs)
+ mask, idxs = torch.topk(gt_idxs_one_hot, min(self.train_cfg.num_rois, len(boxes)), 0)
+ sampled_boxes = img_metas[i]['box_type_3d'](boxes.tensor[idxs].view(-1, 7), with_yaw=gt_bboxes[i].with_yaw)
+ sampled_scores = scores[idxs].view(-1)
+ sampled_gt_idxs = gt_idxs[idxs].view(-1)
+ mask = mask.view(-1).bool()
+ assigned_bbox_list.append((sampled_boxes[mask],
+ sampled_scores[mask],
+ sampled_gt_idxs[mask]))
+ else:
+ assigned_bbox_list.append((boxes,
+ scores,
+ gt_idxs))
+
+ cls_preds, targets, v2r, r2scene, rois, scores, gt_idxs = self._forward_second(x[0], targets, assigned_bbox_list)
+ losses.update(self._loss_second(cls_preds, targets, v2r, r2scene, rois, gt_idxs,
+ gt_bboxes, gt_labels, img_metas))
+
+ return losses
+
+ # per scene
+ def _get_instances_single(self, cls_preds, idxs, v2r, scores, labels, inverse_mapping):
+ if scores.shape[0] == 0:
+ return (inverse_mapping.new_zeros((1, len(inverse_mapping)), dtype=torch.bool),
+ inverse_mapping.new_tensor([0], dtype=torch.long),
+ inverse_mapping.new_tensor([0], dtype=torch.float32))
+ v2r = v2r - v2r.min()
+ assert len(torch.unique(v2r)) == scores.shape[0]
+ assert torch.all(torch.unique(v2r) == torch.arange(0, v2r.max() + 1).to(v2r.device))
+
+ cls_preds = cls_preds.sigmoid()
+ binary_cls_preds = cls_preds > self.test_cfg.binary_score_thr
+ v2r_one_hot = torch.nn.functional.one_hot(v2r).bool()
+ n_rois = v2r_one_hot.shape[1]
+ # todo: why convert from float to long here? can it be long or even int32 before this function?
+ idxs_expand = idxs.unsqueeze(-1).expand(idxs.shape[0], n_rois).long()
+ # todo: can we not convert to ofloat here?
+ binary_cls_preds_expand = binary_cls_preds.unsqueeze(-1).expand(binary_cls_preds.shape[0], n_rois)
+ cls_preds[cls_preds <= self.test_cfg.binary_score_thr] = 0
+ cls_preds_expand = cls_preds.unsqueeze(-1).expand(cls_preds.shape[0], n_rois)
+ idxs_expand[~v2r_one_hot] = inverse_mapping.max() + 1
+
+ # toso: idxs is float. can these tensors be constructed with .new_zeros(..., dtype=bool) ?
+ voxels_masks = idxs.new_zeros(inverse_mapping.max() + 2, n_rois, dtype=bool)
+ voxels_preds = idxs.new_zeros(inverse_mapping.max() + 2, n_rois)
+ voxels_preds = voxels_preds.scatter_(0, idxs_expand, cls_preds_expand)[:-1, :]
+ # todo: is it ok that binary_cls_preds_expand is float?
+ voxels_masks = voxels_masks.scatter_(0, idxs_expand, binary_cls_preds_expand)[:-1, :]
+ scores = scores * voxels_preds.sum(axis=0) / voxels_masks.sum(axis=0)
+ points_masks = voxels_masks[inverse_mapping].T.bool()
+ return points_masks, labels, scores
+
+ def _get_bboxes_single_train(self, bbox_preds, cls_preds, locations, gt_bboxes, img_meta):
+ assigned_ids = self.first_assigner.assign(locations, gt_bboxes, img_meta)
+ scores = torch.cat(cls_preds).sigmoid()
+ bbox_preds = torch.cat(bbox_preds)
+ locations = torch.cat(locations)
+
+ pos_mask = assigned_ids >= 0
+ scores = scores[pos_mask]
+ bbox_preds = bbox_preds[pos_mask]
+ locations = locations[pos_mask]
+ assigned_ids = assigned_ids[pos_mask]
+
+ max_scores, _ = scores.max(dim=1)
+ boxes = self._bbox_pred_to_bbox(locations, bbox_preds)
+ boxes = torch.cat((
+ boxes[:, :3],
+ boxes[:, 3:6] - self.padding,
+ boxes.new_zeros(boxes.shape[0], 1)), dim=1)
+ boxes = img_meta['box_type_3d'](boxes,
+ with_yaw=False,
+ origin=(.5, .5, .5))
+ return boxes, max_scores, assigned_ids
+
+ def _get_instances(self, cls_preds, idxs, v2r, r2scene, scores, labels, inverse_mapping, img_metas):
+ v2scene = r2scene[v2r]
+ results = []
+ for i in range(len(img_metas)):
+ result = self._get_instances_single(
+ cls_preds=cls_preds[v2scene == i],
+ idxs=idxs[v2scene == i],
+ v2r=v2r[v2scene == i],
+ scores=scores[i],
+ labels=labels[i],
+ inverse_mapping=inverse_mapping)
+ results.append(result)
+ return results
+
+ def _get_bboxes_train(self, bbox_preds, cls_preds, locations, gt_bboxes, img_metas):
+ results = []
+ for i in range(len(img_metas)):
+ result = self._get_bboxes_single_train(
+ bbox_preds=[x[i] for x in bbox_preds],
+ cls_preds=[x[i] for x in cls_preds],
+ locations=[x[i] for x in locations],
+ gt_bboxes=gt_bboxes[i],
+ img_meta=img_metas[i])
+ results.append(result)
+ return results
+
+ def _get_bboxes_single_test(self, bbox_preds, cls_preds, locations, cfg, img_meta):
+ scores = torch.cat(cls_preds).sigmoid()
+ bbox_preds = torch.cat(bbox_preds)
+ locations = torch.cat(locations)
+ max_scores, _ = scores.max(dim=1)
+
+ if len(scores) > cfg.nms_pre > 0:
+ _, ids = max_scores.topk(cfg.nms_pre)
+ bbox_preds = bbox_preds[ids]
+ scores = scores[ids]
+ locations = locations[ids]
+
+ boxes = self._bbox_pred_to_bbox(locations, bbox_preds)
+ boxes = torch.cat((
+ boxes[:, :3],
+ boxes[:, 3:6] - self.padding,
+ boxes[:, 6:]), dim=1)
+ boxes, scores, labels = self._nms(boxes, scores, cfg, img_meta)
+ return boxes, scores, labels
+
+ def _get_bboxes_test(self, bbox_preds, cls_preds, locations, cfg, img_metas):
+ results = []
+ for i in range(len(img_metas)):
+ result = self._get_bboxes_single_test(
+ bbox_preds=[x[i] for x in bbox_preds],
+ cls_preds=[x[i] for x in cls_preds],
+ locations=[x[i] for x in locations],
+ cfg=cfg,
+ img_meta=img_metas[i])
+ results.append(result)
+ return results
+
+ def forward_test(self, x, points, img_metas):
+ #first stage
+ bbox_preds, cls_preds, locations = self._forward_first(x[1:])
+ bbox_list = self._get_bboxes_test(bbox_preds, cls_preds, locations, self.test_cfg, img_metas)
+ #second stage
+ inverse_mapping = points.inverse_mapping(x[0].coordinate_map_key).long()
+ src_idxs = torch.arange(0, x[0].features.shape[0]).to(inverse_mapping.device)
+ src_idxs = src_idxs.unsqueeze(1).expand(src_idxs.shape[0], 2)
+ cls_preds, idxs, v2r, r2scene, rois, scores, labels = self._forward_second(x[0], src_idxs, bbox_list)
+ return self._get_instances(cls_preds[:, -1], idxs[:, 0], v2r, r2scene, scores, labels, inverse_mapping, img_metas)
+
+
+ def _nms(self, bboxes, scores, cfg, img_meta):
+ """Multi-class nms for a single scene.
+ Args:
+ bboxes (Tensor): Predicted boxes of shape (N_boxes, 6) or
+ (N_boxes, 7).
+ scores (Tensor): Predicted scores of shape (N_boxes, N_classes).
+ img_meta (dict): Scene meta data.
+ Returns:
+ Tensor: Predicted bboxes.
+ Tensor: Predicted scores.
+ Tensor: Predicted labels.
+ """
+ n_classes = scores.shape[1]
+ yaw_flag = bboxes.shape[1] == 7
+ nms_bboxes, nms_scores, nms_labels = [], [], []
+ for i in range(n_classes):
+ ids = scores[:, i] > cfg.score_thr
+ if not ids.any():
+ continue
+
+ class_scores = scores[ids, i]
+ class_bboxes = bboxes[ids]
+ if yaw_flag:
+ nms_function = nms3d
+ else:
+ class_bboxes = torch.cat(
+ (class_bboxes, torch.zeros_like(class_bboxes[:, :1])),
+ dim=1)
+ nms_function = nms3d_normal
+
+ nms_ids = nms_function(class_bboxes, class_scores,
+ cfg.iou_thr)
+ nms_bboxes.append(class_bboxes[nms_ids])
+ nms_scores.append(class_scores[nms_ids])
+ nms_labels.append(
+ bboxes.new_full(
+ class_scores[nms_ids].shape, i, dtype=torch.long))
+
+ if len(nms_bboxes):
+ nms_bboxes = torch.cat(nms_bboxes, dim=0)
+ nms_scores = torch.cat(nms_scores, dim=0)
+ nms_labels = torch.cat(nms_labels, dim=0)
+ else:
+ nms_bboxes = bboxes.new_zeros((0, bboxes.shape[1]))
+ nms_scores = bboxes.new_zeros((0, ))
+ nms_labels = bboxes.new_zeros((0, ))
+
+ if yaw_flag:
+ box_dim = 7
+ with_yaw = True
+ else:
+ box_dim = 6
+ with_yaw = False
+ nms_bboxes = nms_bboxes[:, :6]
+ nms_bboxes = img_meta['box_type_3d'](
+ nms_bboxes,
+ box_dim=box_dim,
+ with_yaw=with_yaw,
+ origin=(.5, .5, .5))
+
+ return nms_bboxes, nms_scores, nms_labels
\ No newline at end of file
diff --git a/mmdet3d/models/dense_heads/__init__.py b/mmdet3d/models/dense_heads/__init__.py
new file mode 100644
index 0000000..48a4541
--- /dev/null
+++ b/mmdet3d/models/dense_heads/__init__.py
@@ -0,0 +1,28 @@
+# Copyright (c) OpenMMLab. All rights reserved.
+from .anchor3d_head import Anchor3DHead
+from .anchor_free_mono3d_head import AnchorFreeMono3DHead
+from .base_conv_bbox_head import BaseConvBboxHead
+from .base_mono3d_dense_head import BaseMono3DDenseHead
+from .centerpoint_head import CenterHead
+from .fcaf3d_head import FCAF3DHead
+from .fcos_mono3d_head import FCOSMono3DHead
+from .free_anchor3d_head import FreeAnchor3DHead
+from .groupfree3d_head import GroupFree3DHead
+from .monoflex_head import MonoFlexHead
+from .ngfc_head import NgfcOffsetHead, NgfcHead
+from .ngfc_head_v2 import NgfcV2Head
+from .parta2_rpn_head import PartA2RPNHead
+from .pgd_head import PGDHead
+from .point_rpn_head import PointRPNHead
+from .shape_aware_head import ShapeAwareHead
+from .smoke_mono3d_head import SMOKEMono3DHead
+from .ssd_3d_head import SSD3DHead
+from .vote_head import VoteHead
+
+__all__ = [
+ 'Anchor3DHead', 'FreeAnchor3DHead', 'PartA2RPNHead', 'VoteHead',
+ 'SSD3DHead', 'BaseConvBboxHead', 'CenterHead', 'ShapeAwareHead',
+ 'BaseMono3DDenseHead', 'AnchorFreeMono3DHead', 'FCOSMono3DHead',
+ 'GroupFree3DHead', 'PointRPNHead', 'SMOKEMono3DHead', 'PGDHead',
+ 'MonoFlexHead', 'FCAF3DHead', 'NgfcOffsetHead', 'NgfcHead'
+]
diff --git a/mmdet3d/models/dense_heads/anchor3d_head.py b/mmdet3d/models/dense_heads/anchor3d_head.py
new file mode 100644
index 0000000..b747264
--- /dev/null
+++ b/mmdet3d/models/dense_heads/anchor3d_head.py
@@ -0,0 +1,516 @@
+# Copyright (c) OpenMMLab. All rights reserved.
+import numpy as np
+import torch
+from mmcv.runner import BaseModule, force_fp32
+from torch import nn as nn
+
+from mmdet3d.core import (PseudoSampler, box3d_multiclass_nms, limit_period,
+ xywhr2xyxyr)
+from mmdet.core import (build_assigner, build_bbox_coder,
+ build_prior_generator, build_sampler, multi_apply)
+from ..builder import HEADS, build_loss
+from .train_mixins import AnchorTrainMixin
+
+
+@HEADS.register_module()
+class Anchor3DHead(BaseModule, AnchorTrainMixin):
+ """Anchor head for SECOND/PointPillars/MVXNet/PartA2.
+
+ Args:
+ num_classes (int): Number of classes.
+ in_channels (int): Number of channels in the input feature map.
+ train_cfg (dict): Train configs.
+ test_cfg (dict): Test configs.
+ feat_channels (int): Number of channels of the feature map.
+ use_direction_classifier (bool): Whether to add a direction classifier.
+ anchor_generator(dict): Config dict of anchor generator.
+ assigner_per_size (bool): Whether to do assignment for each separate
+ anchor size.
+ assign_per_class (bool): Whether to do assignment for each class.
+ diff_rad_by_sin (bool): Whether to change the difference into sin
+ difference for box regression loss.
+ dir_offset (float | int): The offset of BEV rotation angles.
+ (TODO: may be moved into box coder)
+ dir_limit_offset (float | int): The limited range of BEV
+ rotation angles. (TODO: may be moved into box coder)
+ bbox_coder (dict): Config dict of box coders.
+ loss_cls (dict): Config of classification loss.
+ loss_bbox (dict): Config of localization loss.
+ loss_dir (dict): Config of direction classifier loss.
+ """
+
+ def __init__(self,
+ num_classes,
+ in_channels,
+ train_cfg,
+ test_cfg,
+ feat_channels=256,
+ use_direction_classifier=True,
+ anchor_generator=dict(
+ type='Anchor3DRangeGenerator',
+ range=[0, -39.68, -1.78, 69.12, 39.68, -1.78],
+ strides=[2],
+ sizes=[[3.9, 1.6, 1.56]],
+ rotations=[0, 1.57],
+ custom_values=[],
+ reshape_out=False),
+ assigner_per_size=False,
+ assign_per_class=False,
+ diff_rad_by_sin=True,
+ dir_offset=-np.pi / 2,
+ dir_limit_offset=0,
+ bbox_coder=dict(type='DeltaXYZWLHRBBoxCoder'),
+ loss_cls=dict(
+ type='CrossEntropyLoss',
+ use_sigmoid=True,
+ loss_weight=1.0),
+ loss_bbox=dict(
+ type='SmoothL1Loss', beta=1.0 / 9.0, loss_weight=2.0),
+ loss_dir=dict(type='CrossEntropyLoss', loss_weight=0.2),
+ init_cfg=None):
+ super().__init__(init_cfg=init_cfg)
+ self.in_channels = in_channels
+ self.num_classes = num_classes
+ self.feat_channels = feat_channels
+ self.diff_rad_by_sin = diff_rad_by_sin
+ self.use_direction_classifier = use_direction_classifier
+ self.train_cfg = train_cfg
+ self.test_cfg = test_cfg
+ self.assigner_per_size = assigner_per_size
+ self.assign_per_class = assign_per_class
+ self.dir_offset = dir_offset
+ self.dir_limit_offset = dir_limit_offset
+ import warnings
+ warnings.warn(
+ 'dir_offset and dir_limit_offset will be depressed and be '
+ 'incorporated into box coder in the future')
+ self.fp16_enabled = False
+
+ # build anchor generator
+ self.anchor_generator = build_prior_generator(anchor_generator)
+ # In 3D detection, the anchor stride is connected with anchor size
+ self.num_anchors = self.anchor_generator.num_base_anchors
+ # build box coder
+ self.bbox_coder = build_bbox_coder(bbox_coder)
+ self.box_code_size = self.bbox_coder.code_size
+
+ # build loss function
+ self.use_sigmoid_cls = loss_cls.get('use_sigmoid', False)
+ self.sampling = loss_cls['type'] not in ['FocalLoss', 'GHMC']
+ if not self.use_sigmoid_cls:
+ self.num_classes += 1
+ self.loss_cls = build_loss(loss_cls)
+ self.loss_bbox = build_loss(loss_bbox)
+ self.loss_dir = build_loss(loss_dir)
+ self.fp16_enabled = False
+
+ self._init_layers()
+ self._init_assigner_sampler()
+
+ if init_cfg is None:
+ self.init_cfg = dict(
+ type='Normal',
+ layer='Conv2d',
+ std=0.01,
+ override=dict(
+ type='Normal', name='conv_cls', std=0.01, bias_prob=0.01))
+
+ def _init_assigner_sampler(self):
+ """Initialize the target assigner and sampler of the head."""
+ if self.train_cfg is None:
+ return
+
+ if self.sampling:
+ self.bbox_sampler = build_sampler(self.train_cfg.sampler)
+ else:
+ self.bbox_sampler = PseudoSampler()
+ if isinstance(self.train_cfg.assigner, dict):
+ self.bbox_assigner = build_assigner(self.train_cfg.assigner)
+ elif isinstance(self.train_cfg.assigner, list):
+ self.bbox_assigner = [
+ build_assigner(res) for res in self.train_cfg.assigner
+ ]
+
+ def _init_layers(self):
+ """Initialize neural network layers of the head."""
+ self.cls_out_channels = self.num_anchors * self.num_classes
+ self.conv_cls = nn.Conv2d(self.feat_channels, self.cls_out_channels, 1)
+ self.conv_reg = nn.Conv2d(self.feat_channels,
+ self.num_anchors * self.box_code_size, 1)
+ if self.use_direction_classifier:
+ self.conv_dir_cls = nn.Conv2d(self.feat_channels,
+ self.num_anchors * 2, 1)
+
+ def forward_single(self, x):
+ """Forward function on a single-scale feature map.
+
+ Args:
+ x (torch.Tensor): Input features.
+
+ Returns:
+ tuple[torch.Tensor]: Contain score of each class, bbox
+ regression and direction classification predictions.
+ """
+ cls_score = self.conv_cls(x)
+ bbox_pred = self.conv_reg(x)
+ dir_cls_preds = None
+ if self.use_direction_classifier:
+ dir_cls_preds = self.conv_dir_cls(x)
+ return cls_score, bbox_pred, dir_cls_preds
+
+ def forward(self, feats):
+ """Forward pass.
+
+ Args:
+ feats (list[torch.Tensor]): Multi-level features, e.g.,
+ features produced by FPN.
+
+ Returns:
+ tuple[list[torch.Tensor]]: Multi-level class score, bbox
+ and direction predictions.
+ """
+ return multi_apply(self.forward_single, feats)
+
+ def get_anchors(self, featmap_sizes, input_metas, device='cuda'):
+ """Get anchors according to feature map sizes.
+
+ Args:
+ featmap_sizes (list[tuple]): Multi-level feature map sizes.
+ input_metas (list[dict]): contain pcd and img's meta info.
+ device (str): device of current module.
+
+ Returns:
+ list[list[torch.Tensor]]: Anchors of each image, valid flags
+ of each image.
+ """
+ num_imgs = len(input_metas)
+ # since feature map sizes of all images are the same, we only compute
+ # anchors for one time
+ multi_level_anchors = self.anchor_generator.grid_anchors(
+ featmap_sizes, device=device)
+ anchor_list = [multi_level_anchors for _ in range(num_imgs)]
+ return anchor_list
+
+ def loss_single(self, cls_score, bbox_pred, dir_cls_preds, labels,
+ label_weights, bbox_targets, bbox_weights, dir_targets,
+ dir_weights, num_total_samples):
+ """Calculate loss of Single-level results.
+
+ Args:
+ cls_score (torch.Tensor): Class score in single-level.
+ bbox_pred (torch.Tensor): Bbox prediction in single-level.
+ dir_cls_preds (torch.Tensor): Predictions of direction class
+ in single-level.
+ labels (torch.Tensor): Labels of class.
+ label_weights (torch.Tensor): Weights of class loss.
+ bbox_targets (torch.Tensor): Targets of bbox predictions.
+ bbox_weights (torch.Tensor): Weights of bbox loss.
+ dir_targets (torch.Tensor): Targets of direction predictions.
+ dir_weights (torch.Tensor): Weights of direction loss.
+ num_total_samples (int): The number of valid samples.
+
+ Returns:
+ tuple[torch.Tensor]: Losses of class, bbox
+ and direction, respectively.
+ """
+ # classification loss
+ if num_total_samples is None:
+ num_total_samples = int(cls_score.shape[0])
+ labels = labels.reshape(-1)
+ label_weights = label_weights.reshape(-1)
+ cls_score = cls_score.permute(0, 2, 3, 1).reshape(-1, self.num_classes)
+ assert labels.max().item() <= self.num_classes
+ loss_cls = self.loss_cls(
+ cls_score, labels, label_weights, avg_factor=num_total_samples)
+
+ # regression loss
+ bbox_pred = bbox_pred.permute(0, 2, 3,
+ 1).reshape(-1, self.box_code_size)
+ bbox_targets = bbox_targets.reshape(-1, self.box_code_size)
+ bbox_weights = bbox_weights.reshape(-1, self.box_code_size)
+
+ bg_class_ind = self.num_classes
+ pos_inds = ((labels >= 0)
+ & (labels < bg_class_ind)).nonzero(
+ as_tuple=False).reshape(-1)
+ num_pos = len(pos_inds)
+
+ pos_bbox_pred = bbox_pred[pos_inds]
+ pos_bbox_targets = bbox_targets[pos_inds]
+ pos_bbox_weights = bbox_weights[pos_inds]
+
+ # dir loss
+ if self.use_direction_classifier:
+ dir_cls_preds = dir_cls_preds.permute(0, 2, 3, 1).reshape(-1, 2)
+ dir_targets = dir_targets.reshape(-1)
+ dir_weights = dir_weights.reshape(-1)
+ pos_dir_cls_preds = dir_cls_preds[pos_inds]
+ pos_dir_targets = dir_targets[pos_inds]
+ pos_dir_weights = dir_weights[pos_inds]
+
+ if num_pos > 0:
+ code_weight = self.train_cfg.get('code_weight', None)
+ if code_weight:
+ pos_bbox_weights = pos_bbox_weights * bbox_weights.new_tensor(
+ code_weight)
+ if self.diff_rad_by_sin:
+ pos_bbox_pred, pos_bbox_targets = self.add_sin_difference(
+ pos_bbox_pred, pos_bbox_targets)
+ loss_bbox = self.loss_bbox(
+ pos_bbox_pred,
+ pos_bbox_targets,
+ pos_bbox_weights,
+ avg_factor=num_total_samples)
+
+ # direction classification loss
+ loss_dir = None
+ if self.use_direction_classifier:
+ loss_dir = self.loss_dir(
+ pos_dir_cls_preds,
+ pos_dir_targets,
+ pos_dir_weights,
+ avg_factor=num_total_samples)
+ else:
+ loss_bbox = pos_bbox_pred.sum()
+ if self.use_direction_classifier:
+ loss_dir = pos_dir_cls_preds.sum()
+
+ return loss_cls, loss_bbox, loss_dir
+
+ @staticmethod
+ def add_sin_difference(boxes1, boxes2):
+ """Convert the rotation difference to difference in sine function.
+
+ Args:
+ boxes1 (torch.Tensor): Original Boxes in shape (NxC), where C>=7
+ and the 7th dimension is rotation dimension.
+ boxes2 (torch.Tensor): Target boxes in shape (NxC), where C>=7 and
+ the 7th dimension is rotation dimension.
+
+ Returns:
+ tuple[torch.Tensor]: ``boxes1`` and ``boxes2`` whose 7th
+ dimensions are changed.
+ """
+ rad_pred_encoding = torch.sin(boxes1[..., 6:7]) * torch.cos(
+ boxes2[..., 6:7])
+ rad_tg_encoding = torch.cos(boxes1[..., 6:7]) * torch.sin(boxes2[...,
+ 6:7])
+ boxes1 = torch.cat(
+ [boxes1[..., :6], rad_pred_encoding, boxes1[..., 7:]], dim=-1)
+ boxes2 = torch.cat([boxes2[..., :6], rad_tg_encoding, boxes2[..., 7:]],
+ dim=-1)
+ return boxes1, boxes2
+
+ @force_fp32(apply_to=('cls_scores', 'bbox_preds', 'dir_cls_preds'))
+ def loss(self,
+ cls_scores,
+ bbox_preds,
+ dir_cls_preds,
+ gt_bboxes,
+ gt_labels,
+ input_metas,
+ gt_bboxes_ignore=None):
+ """Calculate losses.
+
+ Args:
+ cls_scores (list[torch.Tensor]): Multi-level class scores.
+ bbox_preds (list[torch.Tensor]): Multi-level bbox predictions.
+ dir_cls_preds (list[torch.Tensor]): Multi-level direction
+ class predictions.
+ gt_bboxes (list[:obj:`BaseInstance3DBoxes`]): Gt bboxes
+ of each sample.
+ gt_labels (list[torch.Tensor]): Gt labels of each sample.
+ input_metas (list[dict]): Contain pcd and img's meta info.
+ gt_bboxes_ignore (list[torch.Tensor]): Specify
+ which bounding boxes to ignore.
+
+ Returns:
+ dict[str, list[torch.Tensor]]: Classification, bbox, and
+ direction losses of each level.
+
+ - loss_cls (list[torch.Tensor]): Classification losses.
+ - loss_bbox (list[torch.Tensor]): Box regression losses.
+ - loss_dir (list[torch.Tensor]): Direction classification
+ losses.
+ """
+ featmap_sizes = [featmap.size()[-2:] for featmap in cls_scores]
+ assert len(featmap_sizes) == self.anchor_generator.num_levels
+ device = cls_scores[0].device
+ anchor_list = self.get_anchors(
+ featmap_sizes, input_metas, device=device)
+ label_channels = self.cls_out_channels if self.use_sigmoid_cls else 1
+ cls_reg_targets = self.anchor_target_3d(
+ anchor_list,
+ gt_bboxes,
+ input_metas,
+ gt_bboxes_ignore_list=gt_bboxes_ignore,
+ gt_labels_list=gt_labels,
+ num_classes=self.num_classes,
+ label_channels=label_channels,
+ sampling=self.sampling)
+
+ if cls_reg_targets is None:
+ return None
+ (labels_list, label_weights_list, bbox_targets_list, bbox_weights_list,
+ dir_targets_list, dir_weights_list, num_total_pos,
+ num_total_neg) = cls_reg_targets
+ num_total_samples = (
+ num_total_pos + num_total_neg if self.sampling else num_total_pos)
+
+ # num_total_samples = None
+ losses_cls, losses_bbox, losses_dir = multi_apply(
+ self.loss_single,
+ cls_scores,
+ bbox_preds,
+ dir_cls_preds,
+ labels_list,
+ label_weights_list,
+ bbox_targets_list,
+ bbox_weights_list,
+ dir_targets_list,
+ dir_weights_list,
+ num_total_samples=num_total_samples)
+ return dict(
+ loss_cls=losses_cls, loss_bbox=losses_bbox, loss_dir=losses_dir)
+
+ def get_bboxes(self,
+ cls_scores,
+ bbox_preds,
+ dir_cls_preds,
+ input_metas,
+ cfg=None,
+ rescale=False):
+ """Get bboxes of anchor head.
+
+ Args:
+ cls_scores (list[torch.Tensor]): Multi-level class scores.
+ bbox_preds (list[torch.Tensor]): Multi-level bbox predictions.
+ dir_cls_preds (list[torch.Tensor]): Multi-level direction
+ class predictions.
+ input_metas (list[dict]): Contain pcd and img's meta info.
+ cfg (:obj:`ConfigDict`): Training or testing config.
+ rescale (list[torch.Tensor]): Whether th rescale bbox.
+
+ Returns:
+ list[tuple]: Prediction resultes of batches.
+ """
+ assert len(cls_scores) == len(bbox_preds)
+ assert len(cls_scores) == len(dir_cls_preds)
+ num_levels = len(cls_scores)
+ featmap_sizes = [cls_scores[i].shape[-2:] for i in range(num_levels)]
+ device = cls_scores[0].device
+ mlvl_anchors = self.anchor_generator.grid_anchors(
+ featmap_sizes, device=device)
+ mlvl_anchors = [
+ anchor.reshape(-1, self.box_code_size) for anchor in mlvl_anchors
+ ]
+
+ result_list = []
+ for img_id in range(len(input_metas)):
+ cls_score_list = [
+ cls_scores[i][img_id].detach() for i in range(num_levels)
+ ]
+ bbox_pred_list = [
+ bbox_preds[i][img_id].detach() for i in range(num_levels)
+ ]
+ dir_cls_pred_list = [
+ dir_cls_preds[i][img_id].detach() for i in range(num_levels)
+ ]
+
+ input_meta = input_metas[img_id]
+ proposals = self.get_bboxes_single(cls_score_list, bbox_pred_list,
+ dir_cls_pred_list, mlvl_anchors,
+ input_meta, cfg, rescale)
+ result_list.append(proposals)
+ return result_list
+
+ def get_bboxes_single(self,
+ cls_scores,
+ bbox_preds,
+ dir_cls_preds,
+ mlvl_anchors,
+ input_meta,
+ cfg=None,
+ rescale=False):
+ """Get bboxes of single branch.
+
+ Args:
+ cls_scores (torch.Tensor): Class score in single batch.
+ bbox_preds (torch.Tensor): Bbox prediction in single batch.
+ dir_cls_preds (torch.Tensor): Predictions of direction class
+ in single batch.
+ mlvl_anchors (List[torch.Tensor]): Multi-level anchors
+ in single batch.
+ input_meta (list[dict]): Contain pcd and img's meta info.
+ cfg (:obj:`ConfigDict`): Training or testing config.
+ rescale (list[torch.Tensor]): whether th rescale bbox.
+
+ Returns:
+ tuple: Contain predictions of single batch.
+
+ - bboxes (:obj:`BaseInstance3DBoxes`): Predicted 3d bboxes.
+ - scores (torch.Tensor): Class score of each bbox.
+ - labels (torch.Tensor): Label of each bbox.
+ """
+ cfg = self.test_cfg if cfg is None else cfg
+ assert len(cls_scores) == len(bbox_preds) == len(mlvl_anchors)
+ mlvl_bboxes = []
+ mlvl_scores = []
+ mlvl_dir_scores = []
+ for cls_score, bbox_pred, dir_cls_pred, anchors in zip(
+ cls_scores, bbox_preds, dir_cls_preds, mlvl_anchors):
+ assert cls_score.size()[-2:] == bbox_pred.size()[-2:]
+ assert cls_score.size()[-2:] == dir_cls_pred.size()[-2:]
+ dir_cls_pred = dir_cls_pred.permute(1, 2, 0).reshape(-1, 2)
+ dir_cls_score = torch.max(dir_cls_pred, dim=-1)[1]
+
+ cls_score = cls_score.permute(1, 2,
+ 0).reshape(-1, self.num_classes)
+ if self.use_sigmoid_cls:
+ scores = cls_score.sigmoid()
+ else:
+ scores = cls_score.softmax(-1)
+ bbox_pred = bbox_pred.permute(1, 2,
+ 0).reshape(-1, self.box_code_size)
+
+ nms_pre = cfg.get('nms_pre', -1)
+ if nms_pre > 0 and scores.shape[0] > nms_pre:
+ if self.use_sigmoid_cls:
+ max_scores, _ = scores.max(dim=1)
+ else:
+ max_scores, _ = scores[:, :-1].max(dim=1)
+ _, topk_inds = max_scores.topk(nms_pre)
+ anchors = anchors[topk_inds, :]
+ bbox_pred = bbox_pred[topk_inds, :]
+ scores = scores[topk_inds, :]
+ dir_cls_score = dir_cls_score[topk_inds]
+
+ bboxes = self.bbox_coder.decode(anchors, bbox_pred)
+ mlvl_bboxes.append(bboxes)
+ mlvl_scores.append(scores)
+ mlvl_dir_scores.append(dir_cls_score)
+
+ mlvl_bboxes = torch.cat(mlvl_bboxes)
+ mlvl_bboxes_for_nms = xywhr2xyxyr(input_meta['box_type_3d'](
+ mlvl_bboxes, box_dim=self.box_code_size).bev)
+ mlvl_scores = torch.cat(mlvl_scores)
+ mlvl_dir_scores = torch.cat(mlvl_dir_scores)
+
+ if self.use_sigmoid_cls:
+ # Add a dummy background class to the front when using sigmoid
+ padding = mlvl_scores.new_zeros(mlvl_scores.shape[0], 1)
+ mlvl_scores = torch.cat([mlvl_scores, padding], dim=1)
+
+ score_thr = cfg.get('score_thr', 0)
+ results = box3d_multiclass_nms(mlvl_bboxes, mlvl_bboxes_for_nms,
+ mlvl_scores, score_thr, cfg.max_num,
+ cfg, mlvl_dir_scores)
+ bboxes, scores, labels, dir_scores = results
+ if bboxes.shape[0] > 0:
+ dir_rot = limit_period(bboxes[..., 6] - self.dir_offset,
+ self.dir_limit_offset, np.pi)
+ bboxes[..., 6] = (
+ dir_rot + self.dir_offset +
+ np.pi * dir_scores.to(bboxes.dtype))
+ bboxes = input_meta['box_type_3d'](bboxes, box_dim=self.box_code_size)
+ return bboxes, scores, labels
diff --git a/mmdet3d/models/dense_heads/anchor_free_mono3d_head.py b/mmdet3d/models/dense_heads/anchor_free_mono3d_head.py
new file mode 100644
index 0000000..e9b27d0
--- /dev/null
+++ b/mmdet3d/models/dense_heads/anchor_free_mono3d_head.py
@@ -0,0 +1,534 @@
+# Copyright (c) OpenMMLab. All rights reserved.
+from abc import abstractmethod
+
+import torch
+from mmcv.cnn import ConvModule, bias_init_with_prob, normal_init
+from mmcv.runner import force_fp32
+from torch import nn as nn
+
+from mmdet.core import multi_apply
+from ..builder import HEADS, build_loss
+from .base_mono3d_dense_head import BaseMono3DDenseHead
+
+
+@HEADS.register_module()
+class AnchorFreeMono3DHead(BaseMono3DDenseHead):
+ """Anchor-free head for monocular 3D object detection.
+
+ Args:
+ num_classes (int): Number of categories excluding the background
+ category.
+ in_channels (int): Number of channels in the input feature map.
+ feat_channels (int, optional): Number of hidden channels.
+ Used in child classes. Defaults to 256.
+ stacked_convs (int, optional): Number of stacking convs of the head.
+ strides (tuple, optional): Downsample factor of each feature map.
+ dcn_on_last_conv (bool, optional): If true, use dcn in the last
+ layer of towers. Default: False.
+ conv_bias (bool | str, optional): If specified as `auto`, it will be
+ decided by the norm_cfg. Bias of conv will be set as True
+ if `norm_cfg` is None, otherwise False. Default: 'auto'.
+ background_label (int, optional): Label ID of background,
+ set as 0 for RPN and num_classes for other heads.
+ It will automatically set as `num_classes` if None is given.
+ use_direction_classifier (bool, optional):
+ Whether to add a direction classifier.
+ diff_rad_by_sin (bool, optional): Whether to change the difference
+ into sin difference for box regression loss. Defaults to True.
+ dir_offset (float, optional): Parameter used in direction
+ classification. Defaults to 0.
+ dir_limit_offset (float, optional): Parameter used in direction
+ classification. Defaults to 0.
+ loss_cls (dict, optional): Config of classification loss.
+ loss_bbox (dict, optional): Config of localization loss.
+ loss_dir (dict, optional): Config of direction classifier loss.
+ loss_attr (dict, optional): Config of attribute classifier loss,
+ which is only active when `pred_attrs=True`.
+ bbox_code_size (int, optional): Dimensions of predicted bounding boxes.
+ pred_attrs (bool, optional): Whether to predict attributes.
+ Defaults to False.
+ num_attrs (int, optional): The number of attributes to be predicted.
+ Default: 9.
+ pred_velo (bool, optional): Whether to predict velocity.
+ Defaults to False.
+ pred_bbox2d (bool, optional): Whether to predict 2D boxes.
+ Defaults to False.
+ group_reg_dims (tuple[int], optional): The dimension of each regression
+ target group. Default: (2, 1, 3, 1, 2).
+ cls_branch (tuple[int], optional): Channels for classification branch.
+ Default: (128, 64).
+ reg_branch (tuple[tuple], optional): Channels for regression branch.
+ Default: (
+ (128, 64), # offset
+ (128, 64), # depth
+ (64, ), # size
+ (64, ), # rot
+ () # velo
+ ),
+ dir_branch (tuple[int], optional): Channels for direction
+ classification branch. Default: (64, ).
+ attr_branch (tuple[int], optional): Channels for classification branch.
+ Default: (64, ).
+ conv_cfg (dict, optional): Config dict for convolution layer.
+ Default: None.
+ norm_cfg (dict, optional): Config dict for normalization layer.
+ Default: None.
+ train_cfg (dict, optional): Training config of anchor head.
+ test_cfg (dict, optional): Testing config of anchor head.
+ """ # noqa: W605
+
+ _version = 1
+
+ def __init__(
+ self,
+ num_classes,
+ in_channels,
+ feat_channels=256,
+ stacked_convs=4,
+ strides=(4, 8, 16, 32, 64),
+ dcn_on_last_conv=False,
+ conv_bias='auto',
+ background_label=None,
+ use_direction_classifier=True,
+ diff_rad_by_sin=True,
+ dir_offset=0,
+ dir_limit_offset=0,
+ loss_cls=dict(
+ type='FocalLoss',
+ use_sigmoid=True,
+ gamma=2.0,
+ alpha=0.25,
+ loss_weight=1.0),
+ loss_bbox=dict(
+ type='SmoothL1Loss', beta=1.0 / 9.0, loss_weight=1.0),
+ loss_dir=dict(
+ type='CrossEntropyLoss', use_sigmoid=False, loss_weight=1.0),
+ loss_attr=dict(
+ type='CrossEntropyLoss', use_sigmoid=False, loss_weight=1.0),
+ bbox_code_size=9, # For nuscenes
+ pred_attrs=False,
+ num_attrs=9, # For nuscenes
+ pred_velo=False,
+ pred_bbox2d=False,
+ group_reg_dims=(2, 1, 3, 1, 2), # offset, depth, size, rot, velo,
+ cls_branch=(128, 64),
+ reg_branch=(
+ (128, 64), # offset
+ (128, 64), # depth
+ (64, ), # size
+ (64, ), # rot
+ () # velo
+ ),
+ dir_branch=(64, ),
+ attr_branch=(64, ),
+ conv_cfg=None,
+ norm_cfg=None,
+ train_cfg=None,
+ test_cfg=None,
+ init_cfg=None):
+ super(AnchorFreeMono3DHead, self).__init__(init_cfg=init_cfg)
+ self.num_classes = num_classes
+ self.cls_out_channels = num_classes
+ self.in_channels = in_channels
+ self.feat_channels = feat_channels
+ self.stacked_convs = stacked_convs
+ self.strides = strides
+ self.dcn_on_last_conv = dcn_on_last_conv
+ assert conv_bias == 'auto' or isinstance(conv_bias, bool)
+ self.conv_bias = conv_bias
+ self.use_direction_classifier = use_direction_classifier
+ self.diff_rad_by_sin = diff_rad_by_sin
+ self.dir_offset = dir_offset
+ self.dir_limit_offset = dir_limit_offset
+ self.loss_cls = build_loss(loss_cls)
+ self.loss_bbox = build_loss(loss_bbox)
+ self.loss_dir = build_loss(loss_dir)
+ self.bbox_code_size = bbox_code_size
+ self.group_reg_dims = list(group_reg_dims)
+ self.cls_branch = cls_branch
+ self.reg_branch = reg_branch
+ assert len(reg_branch) == len(group_reg_dims), 'The number of '\
+ 'element in reg_branch and group_reg_dims should be the same.'
+ self.pred_velo = pred_velo
+ self.pred_bbox2d = pred_bbox2d
+ self.out_channels = []
+ for reg_branch_channels in reg_branch:
+ if len(reg_branch_channels) > 0:
+ self.out_channels.append(reg_branch_channels[-1])
+ else:
+ self.out_channels.append(-1)
+ self.dir_branch = dir_branch
+ self.train_cfg = train_cfg
+ self.test_cfg = test_cfg
+ self.conv_cfg = conv_cfg
+ self.norm_cfg = norm_cfg
+ self.fp16_enabled = False
+ self.background_label = (
+ num_classes if background_label is None else background_label)
+ # background_label should be either 0 or num_classes
+ assert (self.background_label == 0
+ or self.background_label == num_classes)
+ self.pred_attrs = pred_attrs
+ self.attr_background_label = -1
+ self.num_attrs = num_attrs
+ if self.pred_attrs:
+ self.attr_background_label = num_attrs
+ self.loss_attr = build_loss(loss_attr)
+ self.attr_branch = attr_branch
+
+ self._init_layers()
+
+ def _init_layers(self):
+ """Initialize layers of the head."""
+ self._init_cls_convs()
+ self._init_reg_convs()
+ self._init_predictor()
+
+ def _init_cls_convs(self):
+ """Initialize classification conv layers of the head."""
+ self.cls_convs = nn.ModuleList()
+ for i in range(self.stacked_convs):
+ chn = self.in_channels if i == 0 else self.feat_channels
+ if self.dcn_on_last_conv and i == self.stacked_convs - 1:
+ conv_cfg = dict(type='DCNv2')
+ else:
+ conv_cfg = self.conv_cfg
+ self.cls_convs.append(
+ ConvModule(
+ chn,
+ self.feat_channels,
+ 3,
+ stride=1,
+ padding=1,
+ conv_cfg=conv_cfg,
+ norm_cfg=self.norm_cfg,
+ bias=self.conv_bias))
+
+ def _init_reg_convs(self):
+ """Initialize bbox regression conv layers of the head."""
+ self.reg_convs = nn.ModuleList()
+ for i in range(self.stacked_convs):
+ chn = self.in_channels if i == 0 else self.feat_channels
+ if self.dcn_on_last_conv and i == self.stacked_convs - 1:
+ conv_cfg = dict(type='DCNv2')
+ else:
+ conv_cfg = self.conv_cfg
+ self.reg_convs.append(
+ ConvModule(
+ chn,
+ self.feat_channels,
+ 3,
+ stride=1,
+ padding=1,
+ conv_cfg=conv_cfg,
+ norm_cfg=self.norm_cfg,
+ bias=self.conv_bias))
+
+ def _init_branch(self, conv_channels=(64), conv_strides=(1)):
+ """Initialize conv layers as a prediction branch."""
+ conv_before_pred = nn.ModuleList()
+ if isinstance(conv_channels, int):
+ conv_channels = [self.feat_channels] + [conv_channels]
+ conv_strides = [conv_strides]
+ else:
+ conv_channels = [self.feat_channels] + list(conv_channels)
+ conv_strides = list(conv_strides)
+ for i in range(len(conv_strides)):
+ conv_before_pred.append(
+ ConvModule(
+ conv_channels[i],
+ conv_channels[i + 1],
+ 3,
+ stride=conv_strides[i],
+ padding=1,
+ conv_cfg=self.conv_cfg,
+ norm_cfg=self.norm_cfg,
+ bias=self.conv_bias))
+
+ return conv_before_pred
+
+ def _init_predictor(self):
+ """Initialize predictor layers of the head."""
+ self.conv_cls_prev = self._init_branch(
+ conv_channels=self.cls_branch,
+ conv_strides=(1, ) * len(self.cls_branch))
+ self.conv_cls = nn.Conv2d(self.cls_branch[-1], self.cls_out_channels,
+ 1)
+ self.conv_reg_prevs = nn.ModuleList()
+ self.conv_regs = nn.ModuleList()
+ for i in range(len(self.group_reg_dims)):
+ reg_dim = self.group_reg_dims[i]
+ reg_branch_channels = self.reg_branch[i]
+ out_channel = self.out_channels[i]
+ if len(reg_branch_channels) > 0:
+ self.conv_reg_prevs.append(
+ self._init_branch(
+ conv_channels=reg_branch_channels,
+ conv_strides=(1, ) * len(reg_branch_channels)))
+ self.conv_regs.append(nn.Conv2d(out_channel, reg_dim, 1))
+ else:
+ self.conv_reg_prevs.append(None)
+ self.conv_regs.append(
+ nn.Conv2d(self.feat_channels, reg_dim, 1))
+ if self.use_direction_classifier:
+ self.conv_dir_cls_prev = self._init_branch(
+ conv_channels=self.dir_branch,
+ conv_strides=(1, ) * len(self.dir_branch))
+ self.conv_dir_cls = nn.Conv2d(self.dir_branch[-1], 2, 1)
+ if self.pred_attrs:
+ self.conv_attr_prev = self._init_branch(
+ conv_channels=self.attr_branch,
+ conv_strides=(1, ) * len(self.attr_branch))
+ self.conv_attr = nn.Conv2d(self.attr_branch[-1], self.num_attrs, 1)
+
+ def init_weights(self):
+ """Initialize weights of the head.
+
+ We currently still use the customized defined init_weights because the
+ default init of DCN triggered by the init_cfg will init
+ conv_offset.weight, which mistakenly affects the training stability.
+ """
+ for modules in [self.cls_convs, self.reg_convs, self.conv_cls_prev]:
+ for m in modules:
+ if isinstance(m.conv, nn.Conv2d):
+ normal_init(m.conv, std=0.01)
+ for conv_reg_prev in self.conv_reg_prevs:
+ if conv_reg_prev is None:
+ continue
+ for m in conv_reg_prev:
+ if isinstance(m.conv, nn.Conv2d):
+ normal_init(m.conv, std=0.01)
+ if self.use_direction_classifier:
+ for m in self.conv_dir_cls_prev:
+ if isinstance(m.conv, nn.Conv2d):
+ normal_init(m.conv, std=0.01)
+ if self.pred_attrs:
+ for m in self.conv_attr_prev:
+ if isinstance(m.conv, nn.Conv2d):
+ normal_init(m.conv, std=0.01)
+ bias_cls = bias_init_with_prob(0.01)
+ normal_init(self.conv_cls, std=0.01, bias=bias_cls)
+ for conv_reg in self.conv_regs:
+ normal_init(conv_reg, std=0.01)
+ if self.use_direction_classifier:
+ normal_init(self.conv_dir_cls, std=0.01, bias=bias_cls)
+ if self.pred_attrs:
+ normal_init(self.conv_attr, std=0.01, bias=bias_cls)
+
+ def forward(self, feats):
+ """Forward features from the upstream network.
+
+ Args:
+ feats (tuple[Tensor]): Features from the upstream network, each is
+ a 4D-tensor.
+
+ Returns:
+ tuple: Usually contain classification scores, bbox predictions,
+ and direction class predictions.
+ cls_scores (list[Tensor]): Box scores for each scale level,
+ each is a 4D-tensor, the channel number is
+ num_points * num_classes.
+ bbox_preds (list[Tensor]): Box energies / deltas for each scale
+ level, each is a 4D-tensor, the channel number is
+ num_points * bbox_code_size.
+ dir_cls_preds (list[Tensor]): Box scores for direction class
+ predictions on each scale level, each is a 4D-tensor,
+ the channel number is num_points * 2. (bin = 2)
+ attr_preds (list[Tensor]): Attribute scores for each scale
+ level, each is a 4D-tensor, the channel number is
+ num_points * num_attrs.
+ """
+ return multi_apply(self.forward_single, feats)[:5]
+
+ def forward_single(self, x):
+ """Forward features of a single scale level.
+
+ Args:
+ x (Tensor): FPN feature maps of the specified stride.
+
+ Returns:
+ tuple: Scores for each class, bbox predictions, direction class,
+ and attributes, features after classification and regression
+ conv layers, some models needs these features like FCOS.
+ """
+ cls_feat = x
+ reg_feat = x
+
+ for cls_layer in self.cls_convs:
+ cls_feat = cls_layer(cls_feat)
+ # clone the cls_feat for reusing the feature map afterwards
+ clone_cls_feat = cls_feat.clone()
+ for conv_cls_prev_layer in self.conv_cls_prev:
+ clone_cls_feat = conv_cls_prev_layer(clone_cls_feat)
+ cls_score = self.conv_cls(clone_cls_feat)
+
+ for reg_layer in self.reg_convs:
+ reg_feat = reg_layer(reg_feat)
+ bbox_pred = []
+ for i in range(len(self.group_reg_dims)):
+ # clone the reg_feat for reusing the feature map afterwards
+ clone_reg_feat = reg_feat.clone()
+ if len(self.reg_branch[i]) > 0:
+ for conv_reg_prev_layer in self.conv_reg_prevs[i]:
+ clone_reg_feat = conv_reg_prev_layer(clone_reg_feat)
+ bbox_pred.append(self.conv_regs[i](clone_reg_feat))
+ bbox_pred = torch.cat(bbox_pred, dim=1)
+
+ dir_cls_pred = None
+ if self.use_direction_classifier:
+ clone_reg_feat = reg_feat.clone()
+ for conv_dir_cls_prev_layer in self.conv_dir_cls_prev:
+ clone_reg_feat = conv_dir_cls_prev_layer(clone_reg_feat)
+ dir_cls_pred = self.conv_dir_cls(clone_reg_feat)
+
+ attr_pred = None
+ if self.pred_attrs:
+ # clone the cls_feat for reusing the feature map afterwards
+ clone_cls_feat = cls_feat.clone()
+ for conv_attr_prev_layer in self.conv_attr_prev:
+ clone_cls_feat = conv_attr_prev_layer(clone_cls_feat)
+ attr_pred = self.conv_attr(clone_cls_feat)
+
+ return cls_score, bbox_pred, dir_cls_pred, attr_pred, cls_feat, \
+ reg_feat
+
+ @abstractmethod
+ @force_fp32(apply_to=('cls_scores', 'bbox_preds', 'dir_cls_preds'))
+ def loss(self,
+ cls_scores,
+ bbox_preds,
+ dir_cls_preds,
+ attr_preds,
+ gt_bboxes,
+ gt_labels,
+ gt_bboxes_3d,
+ gt_labels_3d,
+ centers2d,
+ depths,
+ attr_labels,
+ img_metas,
+ gt_bboxes_ignore=None):
+ """Compute loss of the head.
+
+ Args:
+ cls_scores (list[Tensor]): Box scores for each scale level,
+ each is a 4D-tensor, the channel number is
+ num_points * num_classes.
+ bbox_preds (list[Tensor]): Box energies / deltas for each scale
+ level, each is a 4D-tensor, the channel number is
+ num_points * bbox_code_size.
+ dir_cls_preds (list[Tensor]): Box scores for direction class
+ predictions on each scale level, each is a 4D-tensor,
+ the channel number is num_points * 2. (bin = 2)
+ attr_preds (list[Tensor]): Box scores for each scale level,
+ each is a 4D-tensor, the channel number is
+ num_points * num_attrs.
+ gt_bboxes (list[Tensor]): Ground truth bboxes for each image with
+ shape (num_gts, 4) in [tl_x, tl_y, br_x, br_y] format.
+ gt_labels (list[Tensor]): class indices corresponding to each box
+ gt_bboxes_3d (list[Tensor]): 3D Ground truth bboxes for each
+ image with shape (num_gts, bbox_code_size).
+ gt_labels_3d (list[Tensor]): 3D class indices of each box.
+ centers2d (list[Tensor]): Projected 3D centers onto 2D images.
+ depths (list[Tensor]): Depth of projected centers on 2D images.
+ attr_labels (list[Tensor], optional): Attribute indices
+ corresponding to each box
+ img_metas (list[dict]): Meta information of each image, e.g.,
+ image size, scaling factor, etc.
+ gt_bboxes_ignore (list[Tensor]): specify which bounding
+ boxes can be ignored when computing the loss.
+ """
+
+ raise NotImplementedError
+
+ @abstractmethod
+ @force_fp32(apply_to=('cls_scores', 'bbox_preds', 'dir_cls_preds'))
+ def get_bboxes(self,
+ cls_scores,
+ bbox_preds,
+ dir_cls_preds,
+ attr_preds,
+ img_metas,
+ cfg=None,
+ rescale=None):
+ """Transform network output for a batch into bbox predictions.
+
+ Args:
+ cls_scores (list[Tensor]): Box scores for each scale level
+ Has shape (N, num_points * num_classes, H, W)
+ bbox_preds (list[Tensor]): Box energies / deltas for each scale
+ level with shape (N, num_points * bbox_code_size, H, W)
+ dir_cls_preds (list[Tensor]): Box scores for direction class
+ predictions on each scale level, each is a 4D-tensor,
+ the channel number is num_points * 2. (bin = 2)
+ attr_preds (list[Tensor]): Attribute scores for each scale level
+ Has shape (N, num_points * num_attrs, H, W)
+ img_metas (list[dict]): Meta information of each image, e.g.,
+ image size, scaling factor, etc.
+ cfg (mmcv.Config): Test / postprocessing configuration,
+ if None, test_cfg would be used
+ rescale (bool): If True, return boxes in original image space
+ """
+
+ raise NotImplementedError
+
+ @abstractmethod
+ def get_targets(self, points, gt_bboxes_list, gt_labels_list,
+ gt_bboxes_3d_list, gt_labels_3d_list, centers2d_list,
+ depths_list, attr_labels_list):
+ """Compute regression, classification and centerss targets for points
+ in multiple images.
+
+ Args:
+ points (list[Tensor]): Points of each fpn level, each has shape
+ (num_points, 2).
+ gt_bboxes_list (list[Tensor]): Ground truth bboxes of each image,
+ each has shape (num_gt, 4).
+ gt_labels_list (list[Tensor]): Ground truth labels of each box,
+ each has shape (num_gt,).
+ gt_bboxes_3d_list (list[Tensor]): 3D Ground truth bboxes of each
+ image, each has shape (num_gt, bbox_code_size).
+ gt_labels_3d_list (list[Tensor]): 3D Ground truth labels of each
+ box, each has shape (num_gt,).
+ centers2d_list (list[Tensor]): Projected 3D centers onto 2D image,
+ each has shape (num_gt, 2).
+ depths_list (list[Tensor]): Depth of projected 3D centers onto 2D
+ image, each has shape (num_gt, 1).
+ attr_labels_list (list[Tensor]): Attribute labels of each box,
+ each has shape (num_gt,).
+ """
+ raise NotImplementedError
+
+ def _get_points_single(self,
+ featmap_size,
+ stride,
+ dtype,
+ device,
+ flatten=False):
+ """Get points of a single scale level."""
+ h, w = featmap_size
+ x_range = torch.arange(w, dtype=dtype, device=device)
+ y_range = torch.arange(h, dtype=dtype, device=device)
+ y, x = torch.meshgrid(y_range, x_range)
+ if flatten:
+ y = y.flatten()
+ x = x.flatten()
+ return y, x
+
+ def get_points(self, featmap_sizes, dtype, device, flatten=False):
+ """Get points according to feature map sizes.
+
+ Args:
+ featmap_sizes (list[tuple]): Multi-level feature map sizes.
+ dtype (torch.dtype): Type of points.
+ device (torch.device): Device of points.
+
+ Returns:
+ tuple: points of each image.
+ """
+ mlvl_points = []
+ for i in range(len(featmap_sizes)):
+ mlvl_points.append(
+ self._get_points_single(featmap_sizes[i], self.strides[i],
+ dtype, device, flatten))
+ return mlvl_points
diff --git a/mmdet3d/models/dense_heads/base_conv_bbox_head.py b/mmdet3d/models/dense_heads/base_conv_bbox_head.py
new file mode 100644
index 0000000..ec5eaa6
--- /dev/null
+++ b/mmdet3d/models/dense_heads/base_conv_bbox_head.py
@@ -0,0 +1,131 @@
+# Copyright (c) OpenMMLab. All rights reserved.
+from mmcv.cnn import ConvModule
+from mmcv.cnn.bricks import build_conv_layer
+from mmcv.runner import BaseModule
+from torch import nn as nn
+
+from ..builder import HEADS
+
+
+@HEADS.register_module()
+class BaseConvBboxHead(BaseModule):
+ r"""More general bbox head, with shared conv layers and two optional
+ separated branches.
+
+ .. code-block:: none
+
+ /-> cls convs -> cls_score
+ shared convs
+ \-> reg convs -> bbox_pred
+ """
+
+ def __init__(self,
+ in_channels=0,
+ shared_conv_channels=(),
+ cls_conv_channels=(),
+ num_cls_out_channels=0,
+ reg_conv_channels=(),
+ num_reg_out_channels=0,
+ conv_cfg=dict(type='Conv1d'),
+ norm_cfg=dict(type='BN1d'),
+ act_cfg=dict(type='ReLU'),
+ bias='auto',
+ init_cfg=None,
+ *args,
+ **kwargs):
+ super(BaseConvBboxHead, self).__init__(
+ init_cfg=init_cfg, *args, **kwargs)
+ assert in_channels > 0
+ assert num_cls_out_channels > 0
+ assert num_reg_out_channels > 0
+ self.in_channels = in_channels
+ self.shared_conv_channels = shared_conv_channels
+ self.cls_conv_channels = cls_conv_channels
+ self.num_cls_out_channels = num_cls_out_channels
+ self.reg_conv_channels = reg_conv_channels
+ self.num_reg_out_channels = num_reg_out_channels
+ self.conv_cfg = conv_cfg
+ self.norm_cfg = norm_cfg
+ self.act_cfg = act_cfg
+ self.bias = bias
+
+ # add shared convs
+ if len(self.shared_conv_channels) > 0:
+ self.shared_convs = self._add_conv_branch(
+ self.in_channels, self.shared_conv_channels)
+ out_channels = self.shared_conv_channels[-1]
+ else:
+ out_channels = self.in_channels
+
+ # add cls specific branch
+ prev_channel = out_channels
+ if len(self.cls_conv_channels) > 0:
+ self.cls_convs = self._add_conv_branch(prev_channel,
+ self.cls_conv_channels)
+ prev_channel = self.cls_conv_channels[-1]
+
+ self.conv_cls = build_conv_layer(
+ conv_cfg,
+ in_channels=prev_channel,
+ out_channels=num_cls_out_channels,
+ kernel_size=1)
+ # add reg specific branch
+ prev_channel = out_channels
+ if len(self.reg_conv_channels) > 0:
+ self.reg_convs = self._add_conv_branch(prev_channel,
+ self.reg_conv_channels)
+ prev_channel = self.reg_conv_channels[-1]
+
+ self.conv_reg = build_conv_layer(
+ conv_cfg,
+ in_channels=prev_channel,
+ out_channels=num_reg_out_channels,
+ kernel_size=1)
+
+ def _add_conv_branch(self, in_channels, conv_channels):
+ """Add shared or separable branch."""
+ conv_spec = [in_channels] + list(conv_channels)
+ # add branch specific conv layers
+ conv_layers = nn.Sequential()
+ for i in range(len(conv_spec) - 1):
+ conv_layers.add_module(
+ f'layer{i}',
+ ConvModule(
+ conv_spec[i],
+ conv_spec[i + 1],
+ kernel_size=1,
+ padding=0,
+ conv_cfg=self.conv_cfg,
+ norm_cfg=self.norm_cfg,
+ act_cfg=self.act_cfg,
+ bias=self.bias,
+ inplace=True))
+ return conv_layers
+
+ def forward(self, feats):
+ """Forward.
+
+ Args:
+ feats (Tensor): Input features
+
+ Returns:
+ Tensor: Class scores predictions
+ Tensor: Regression predictions
+ """
+ # shared part
+ if len(self.shared_conv_channels) > 0:
+ x = self.shared_convs(feats)
+
+ # separate branches
+ x_cls = x
+ x_reg = x
+
+ if len(self.cls_conv_channels) > 0:
+ x_cls = self.cls_convs(x_cls)
+ cls_score = self.conv_cls(x_cls)
+
+ if len(self.reg_conv_channels) > 0:
+ x_reg = self.reg_convs(x_reg)
+ bbox_pred = self.conv_reg(x_reg)
+
+ return cls_score, bbox_pred
diff --git a/mmdet3d/models/dense_heads/base_mono3d_dense_head.py b/mmdet3d/models/dense_heads/base_mono3d_dense_head.py
new file mode 100644
index 0000000..2444473
--- /dev/null
+++ b/mmdet3d/models/dense_heads/base_mono3d_dense_head.py
@@ -0,0 +1,78 @@
+# Copyright (c) OpenMMLab. All rights reserved.
+from abc import ABCMeta, abstractmethod
+
+from mmcv.runner import BaseModule
+
+
+class BaseMono3DDenseHead(BaseModule, metaclass=ABCMeta):
+ """Base class for Monocular 3D DenseHeads."""
+
+ def __init__(self, init_cfg=None):
+ super(BaseMono3DDenseHead, self).__init__(init_cfg=init_cfg)
+
+ @abstractmethod
+ def loss(self, **kwargs):
+ """Compute losses of the head."""
+ pass
+
+ @abstractmethod
+ def get_bboxes(self, **kwargs):
+ """Transform network output for a batch into bbox predictions."""
+ pass
+
+ def forward_train(self,
+ x,
+ img_metas,
+ gt_bboxes,
+ gt_labels=None,
+ gt_bboxes_3d=None,
+ gt_labels_3d=None,
+ centers2d=None,
+ depths=None,
+ attr_labels=None,
+ gt_bboxes_ignore=None,
+ proposal_cfg=None,
+ **kwargs):
+ """
+ Args:
+ x (list[Tensor]): Features from FPN.
+ img_metas (list[dict]): Meta information of each image, e.g.,
+ image size, scaling factor, etc.
+ gt_bboxes (list[Tensor]): Ground truth bboxes of the image,
+ shape (num_gts, 4).
+ gt_labels (list[Tensor]): Ground truth labels of each box,
+ shape (num_gts,).
+ gt_bboxes_3d (list[Tensor]): 3D ground truth bboxes of the image,
+ shape (num_gts, self.bbox_code_size).
+ gt_labels_3d (list[Tensor]): 3D ground truth labels of each box,
+ shape (num_gts,).
+ centers2d (list[Tensor]): Projected 3D center of each box,
+ shape (num_gts, 2).
+ depths (list[Tensor]): Depth of projected 3D center of each box,
+ shape (num_gts,).
+ attr_labels (list[Tensor]): Attribute labels of each box,
+ shape (num_gts,).
+ gt_bboxes_ignore (list[Tensor]): Ground truth bboxes to be
+ ignored, shape (num_ignored_gts, 4).
+ proposal_cfg (mmcv.Config): Test / postprocessing configuration,
+ if None, test_cfg would be used
+
+ Returns:
+ tuple:
+ losses: (dict[str, Tensor]): A dictionary of loss components.
+ proposal_list (list[Tensor]): Proposals of each image.
+ """
+ outs = self(x)
+ if gt_labels is None:
+ loss_inputs = outs + (gt_bboxes, gt_bboxes_3d, centers2d, depths,
+ attr_labels, img_metas)
+ else:
+ loss_inputs = outs + (gt_bboxes, gt_labels, gt_bboxes_3d,
+ gt_labels_3d, centers2d, depths, attr_labels,
+ img_metas)
+ losses = self.loss(*loss_inputs, gt_bboxes_ignore=gt_bboxes_ignore)
+ if proposal_cfg is None:
+ return losses
+ else:
+ proposal_list = self.get_bboxes(*outs, img_metas, cfg=proposal_cfg)
+ return losses, proposal_list
diff --git a/mmdet3d/models/dense_heads/centerpoint_head.py b/mmdet3d/models/dense_heads/centerpoint_head.py
new file mode 100644
index 0000000..2cf758b
--- /dev/null
+++ b/mmdet3d/models/dense_heads/centerpoint_head.py
@@ -0,0 +1,830 @@
+# Copyright (c) OpenMMLab. All rights reserved.
+import copy
+
+import torch
+from mmcv.cnn import ConvModule, build_conv_layer
+from mmcv.runner import BaseModule, force_fp32
+from torch import nn
+
+from mmdet3d.core import (circle_nms, draw_heatmap_gaussian, gaussian_radius,
+ xywhr2xyxyr)
+from mmdet3d.core.post_processing import nms_bev
+from mmdet3d.models import builder
+from mmdet3d.models.utils import clip_sigmoid
+from mmdet.core import build_bbox_coder, multi_apply
+from ..builder import HEADS, build_loss
+
+
+@HEADS.register_module()
+class SeparateHead(BaseModule):
+ """SeparateHead for CenterHead.
+
+ Args:
+ in_channels (int): Input channels for conv_layer.
+ heads (dict): Conv information.
+ head_conv (int, optional): Output channels.
+ Default: 64.
+ final_kernel (int, optional): Kernel size for the last conv layer.
+ Default: 1.
+ init_bias (float, optional): Initial bias. Default: -2.19.
+ conv_cfg (dict, optional): Config of conv layer.
+ Default: dict(type='Conv2d')
+ norm_cfg (dict, optional): Config of norm layer.
+ Default: dict(type='BN2d').
+ bias (str, optional): Type of bias. Default: 'auto'.
+ """
+
+ def __init__(self,
+ in_channels,
+ heads,
+ head_conv=64,
+ final_kernel=1,
+ init_bias=-2.19,
+ conv_cfg=dict(type='Conv2d'),
+ norm_cfg=dict(type='BN2d'),
+ bias='auto',
+ init_cfg=None,
+ **kwargs):
+ assert init_cfg is None, 'To prevent abnormal initialization ' \
+ 'behavior, init_cfg is not allowed to be set'
+ super(SeparateHead, self).__init__(init_cfg=init_cfg)
+ self.heads = heads
+ self.init_bias = init_bias
+ for head in self.heads:
+ classes, num_conv = self.heads[head]
+
+ conv_layers = []
+ c_in = in_channels
+ for i in range(num_conv - 1):
+ conv_layers.append(
+ ConvModule(
+ c_in,
+ head_conv,
+ kernel_size=final_kernel,
+ stride=1,
+ padding=final_kernel // 2,
+ bias=bias,
+ conv_cfg=conv_cfg,
+ norm_cfg=norm_cfg))
+ c_in = head_conv
+
+ conv_layers.append(
+ build_conv_layer(
+ conv_cfg,
+ head_conv,
+ classes,
+ kernel_size=final_kernel,
+ stride=1,
+ padding=final_kernel // 2,
+ bias=True))
+ conv_layers = nn.Sequential(*conv_layers)
+
+ self.__setattr__(head, conv_layers)
+
+ if init_cfg is None:
+ self.init_cfg = dict(type='Kaiming', layer='Conv2d')
+
+ def init_weights(self):
+ """Initialize weights."""
+ super().init_weights()
+ for head in self.heads:
+ if head == 'heatmap':
+ self.__getattr__(head)[-1].bias.data.fill_(self.init_bias)
+
+ def forward(self, x):
+ """Forward function for SepHead.
+
+ Args:
+ x (torch.Tensor): Input feature map with the shape of
+ [B, 512, 128, 128].
+
+ Returns:
+ dict[str: torch.Tensor]: contains the following keys:
+
+ -reg (torch.Tensor): 2D regression value with the
+ shape of [B, 2, H, W].
+ -height (torch.Tensor): Height value with the
+ shape of [B, 1, H, W].
+ -dim (torch.Tensor): Size value with the shape
+ of [B, 3, H, W].
+ -rot (torch.Tensor): Rotation value with the
+ shape of [B, 2, H, W].
+ -vel (torch.Tensor): Velocity value with the
+ shape of [B, 2, H, W].
+ -heatmap (torch.Tensor): Heatmap with the shape of
+ [B, N, H, W].
+ """
+ ret_dict = dict()
+ for head in self.heads:
+ ret_dict[head] = self.__getattr__(head)(x)
+
+ return ret_dict
+
+
+@HEADS.register_module()
+class DCNSeparateHead(BaseModule):
+ r"""DCNSeparateHead for CenterHead.
+
+ .. code-block:: none
+ /-----> DCN for heatmap task -----> heatmap task.
+ feature
+ \-----> DCN for regression tasks -----> regression tasks
+
+ Args:
+ in_channels (int): Input channels for conv_layer.
+ num_cls (int): Number of classes.
+ heads (dict): Conv information.
+ dcn_config (dict): Config of dcn layer.
+ head_conv (int, optional): Output channels.
+ Default: 64.
+ final_kernel (int, optional): Kernel size for the last conv
+ layer. Default: 1.
+ init_bias (float, optional): Initial bias. Default: -2.19.
+ conv_cfg (dict, optional): Config of conv layer.
+ Default: dict(type='Conv2d')
+ norm_cfg (dict, optional): Config of norm layer.
+ Default: dict(type='BN2d').
+ bias (str, optional): Type of bias. Default: 'auto'.
+ """ # noqa: W605
+
+ def __init__(self,
+ in_channels,
+ num_cls,
+ heads,
+ dcn_config,
+ head_conv=64,
+ final_kernel=1,
+ init_bias=-2.19,
+ conv_cfg=dict(type='Conv2d'),
+ norm_cfg=dict(type='BN2d'),
+ bias='auto',
+ init_cfg=None,
+ **kwargs):
+ assert init_cfg is None, 'To prevent abnormal initialization ' \
+ 'behavior, init_cfg is not allowed to be set'
+ super(DCNSeparateHead, self).__init__(init_cfg=init_cfg)
+ if 'heatmap' in heads:
+ heads.pop('heatmap')
+ # feature adaptation with dcn
+ # use separate features for classification / regression
+ self.feature_adapt_cls = build_conv_layer(dcn_config)
+
+ self.feature_adapt_reg = build_conv_layer(dcn_config)
+
+ # heatmap prediction head
+ cls_head = [
+ ConvModule(
+ in_channels,
+ head_conv,
+ kernel_size=3,
+ padding=1,
+ conv_cfg=conv_cfg,
+ bias=bias,
+ norm_cfg=norm_cfg),
+ build_conv_layer(
+ conv_cfg,
+ head_conv,
+ num_cls,
+ kernel_size=3,
+ stride=1,
+ padding=1,
+ bias=bias)
+ ]
+ self.cls_head = nn.Sequential(*cls_head)
+ self.init_bias = init_bias
+ # other regression target
+ self.task_head = SeparateHead(
+ in_channels,
+ heads,
+ head_conv=head_conv,
+ final_kernel=final_kernel,
+ bias=bias)
+ if init_cfg is None:
+ self.init_cfg = dict(type='Kaiming', layer='Conv2d')
+
+ def init_weights(self):
+ """Initialize weights."""
+ super().init_weights()
+ self.cls_head[-1].bias.data.fill_(self.init_bias)
+
+ def forward(self, x):
+ """Forward function for DCNSepHead.
+
+ Args:
+ x (torch.Tensor): Input feature map with the shape of
+ [B, 512, 128, 128].
+
+ Returns:
+ dict[str: torch.Tensor]: contains the following keys:
+
+ -reg (torch.Tensor): 2D regression value with the
+ shape of [B, 2, H, W].
+ -height (torch.Tensor): Height value with the
+ shape of [B, 1, H, W].
+ -dim (torch.Tensor): Size value with the shape
+ of [B, 3, H, W].
+ -rot (torch.Tensor): Rotation value with the
+ shape of [B, 2, H, W].
+ -vel (torch.Tensor): Velocity value with the
+ shape of [B, 2, H, W].
+ -heatmap (torch.Tensor): Heatmap with the shape of
+ [B, N, H, W].
+ """
+ center_feat = self.feature_adapt_cls(x)
+ reg_feat = self.feature_adapt_reg(x)
+
+ cls_score = self.cls_head(center_feat)
+ ret = self.task_head(reg_feat)
+ ret['heatmap'] = cls_score
+
+ return ret
+
+
+@HEADS.register_module()
+class CenterHead(BaseModule):
+ """CenterHead for CenterPoint.
+
+ Args:
+ in_channels (list[int] | int, optional): Channels of the input
+ feature map. Default: [128].
+ tasks (list[dict], optional): Task information including class number
+ and class names. Default: None.
+ train_cfg (dict, optional): Train-time configs. Default: None.
+ test_cfg (dict, optional): Test-time configs. Default: None.
+ bbox_coder (dict, optional): Bbox coder configs. Default: None.
+ common_heads (dict, optional): Conv information for common heads.
+ Default: dict().
+ loss_cls (dict, optional): Config of classification loss function.
+ Default: dict(type='GaussianFocalLoss', reduction='mean').
+ loss_bbox (dict, optional): Config of regression loss function.
+ Default: dict(type='L1Loss', reduction='none').
+ separate_head (dict, optional): Config of separate head. Default: dict(
+ type='SeparateHead', init_bias=-2.19, final_kernel=3)
+ share_conv_channel (int, optional): Output channels for share_conv
+ layer. Default: 64.
+ num_heatmap_convs (int, optional): Number of conv layers for heatmap
+ conv layer. Default: 2.
+ conv_cfg (dict, optional): Config of conv layer.
+ Default: dict(type='Conv2d')
+ norm_cfg (dict, optional): Config of norm layer.
+ Default: dict(type='BN2d').
+ bias (str, optional): Type of bias. Default: 'auto'.
+ """
+
+ def __init__(self,
+ in_channels=[128],
+ tasks=None,
+ train_cfg=None,
+ test_cfg=None,
+ bbox_coder=None,
+ common_heads=dict(),
+ loss_cls=dict(type='GaussianFocalLoss', reduction='mean'),
+ loss_bbox=dict(
+ type='L1Loss', reduction='none', loss_weight=0.25),
+ separate_head=dict(
+ type='SeparateHead', init_bias=-2.19, final_kernel=3),
+ share_conv_channel=64,
+ num_heatmap_convs=2,
+ conv_cfg=dict(type='Conv2d'),
+ norm_cfg=dict(type='BN2d'),
+ bias='auto',
+ norm_bbox=True,
+ init_cfg=None):
+ assert init_cfg is None, 'To prevent abnormal initialization ' \
+ 'behavior, init_cfg is not allowed to be set'
+ super(CenterHead, self).__init__(init_cfg=init_cfg)
+
+ num_classes = [len(t['class_names']) for t in tasks]
+ self.class_names = [t['class_names'] for t in tasks]
+ self.train_cfg = train_cfg
+ self.test_cfg = test_cfg
+ self.in_channels = in_channels
+ self.num_classes = num_classes
+ self.norm_bbox = norm_bbox
+
+ self.loss_cls = build_loss(loss_cls)
+ self.loss_bbox = build_loss(loss_bbox)
+ self.bbox_coder = build_bbox_coder(bbox_coder)
+ self.num_anchor_per_locs = [n for n in num_classes]
+ self.fp16_enabled = False
+
+ # a shared convolution
+ self.shared_conv = ConvModule(
+ in_channels,
+ share_conv_channel,
+ kernel_size=3,
+ padding=1,
+ conv_cfg=conv_cfg,
+ norm_cfg=norm_cfg,
+ bias=bias)
+
+ self.task_heads = nn.ModuleList()
+
+ for num_cls in num_classes:
+ heads = copy.deepcopy(common_heads)
+ heads.update(dict(heatmap=(num_cls, num_heatmap_convs)))
+ separate_head.update(
+ in_channels=share_conv_channel, heads=heads, num_cls=num_cls)
+ self.task_heads.append(builder.build_head(separate_head))
+
+ def forward_single(self, x):
+ """Forward function for CenterPoint.
+
+ Args:
+ x (torch.Tensor): Input feature map with the shape of
+ [B, 512, 128, 128].
+
+ Returns:
+ list[dict]: Output results for tasks.
+ """
+ ret_dicts = []
+
+ x = self.shared_conv(x)
+
+ for task in self.task_heads:
+ ret_dicts.append(task(x))
+
+ return ret_dicts
+
+ def forward(self, feats):
+ """Forward pass.
+
+ Args:
+ feats (list[torch.Tensor]): Multi-level features, e.g.,
+ features produced by FPN.
+
+ Returns:
+ tuple(list[dict]): Output results for tasks.
+ """
+ return multi_apply(self.forward_single, feats)
+
+ def _gather_feat(self, feat, ind, mask=None):
+ """Gather feature map.
+
+ Given feature map and index, return indexed feature map.
+
+ Args:
+ feat (torch.tensor): Feature map with the shape of [B, H*W, 10].
+ ind (torch.Tensor): Index of the ground truth boxes with the
+ shape of [B, max_obj].
+ mask (torch.Tensor, optional): Mask of the feature map with the
+ shape of [B, max_obj]. Default: None.
+
+ Returns:
+ torch.Tensor: Feature map after gathering with the shape
+ of [B, max_obj, 10].
+ """
+ dim = feat.size(2)
+ ind = ind.unsqueeze(2).expand(ind.size(0), ind.size(1), dim)
+ feat = feat.gather(1, ind)
+ if mask is not None:
+ mask = mask.unsqueeze(2).expand_as(feat)
+ feat = feat[mask]
+ feat = feat.view(-1, dim)
+ return feat
+
+ def get_targets(self, gt_bboxes_3d, gt_labels_3d):
+ """Generate targets.
+
+ How each output is transformed:
+
+ Each nested list is transposed so that all same-index elements in
+ each sub-list (1, ..., N) become the new sub-lists.
+ [ [a0, a1, a2, ... ], [b0, b1, b2, ... ], ... ]
+ ==> [ [a0, b0, ... ], [a1, b1, ... ], [a2, b2, ... ] ]
+
+ The new transposed nested list is converted into a list of N
+ tensors generated by concatenating tensors in the new sub-lists.
+ [ tensor0, tensor1, tensor2, ... ]
+
+ Args:
+ gt_bboxes_3d (list[:obj:`LiDARInstance3DBoxes`]): Ground
+ truth gt boxes.
+ gt_labels_3d (list[torch.Tensor]): Labels of boxes.
+
+ Returns:
+ Returns:
+ tuple[list[torch.Tensor]]: Tuple of target including
+ the following results in order.
+
+ - list[torch.Tensor]: Heatmap scores.
+ - list[torch.Tensor]: Ground truth boxes.
+ - list[torch.Tensor]: Indexes indicating the
+ position of the valid boxes.
+ - list[torch.Tensor]: Masks indicating which
+ boxes are valid.
+ """
+ heatmaps, anno_boxes, inds, masks = multi_apply(
+ self.get_targets_single, gt_bboxes_3d, gt_labels_3d)
+ # Transpose heatmaps
+ heatmaps = list(map(list, zip(*heatmaps)))
+ heatmaps = [torch.stack(hms_) for hms_ in heatmaps]
+ # Transpose anno_boxes
+ anno_boxes = list(map(list, zip(*anno_boxes)))
+ anno_boxes = [torch.stack(anno_boxes_) for anno_boxes_ in anno_boxes]
+ # Transpose inds
+ inds = list(map(list, zip(*inds)))
+ inds = [torch.stack(inds_) for inds_ in inds]
+ # Transpose inds
+ masks = list(map(list, zip(*masks)))
+ masks = [torch.stack(masks_) for masks_ in masks]
+ return heatmaps, anno_boxes, inds, masks
+
+ def get_targets_single(self, gt_bboxes_3d, gt_labels_3d):
+ """Generate training targets for a single sample.
+
+ Args:
+ gt_bboxes_3d (:obj:`LiDARInstance3DBoxes`): Ground truth gt boxes.
+ gt_labels_3d (torch.Tensor): Labels of boxes.
+
+ Returns:
+ tuple[list[torch.Tensor]]: Tuple of target including
+ the following results in order.
+
+ - list[torch.Tensor]: Heatmap scores.
+ - list[torch.Tensor]: Ground truth boxes.
+ - list[torch.Tensor]: Indexes indicating the position
+ of the valid boxes.
+ - list[torch.Tensor]: Masks indicating which boxes
+ are valid.
+ """
+ device = gt_labels_3d.device
+ gt_bboxes_3d = torch.cat(
+ (gt_bboxes_3d.gravity_center, gt_bboxes_3d.tensor[:, 3:]),
+ dim=1).to(device)
+ max_objs = self.train_cfg['max_objs'] * self.train_cfg['dense_reg']
+ grid_size = torch.tensor(self.train_cfg['grid_size'])
+ pc_range = torch.tensor(self.train_cfg['point_cloud_range'])
+ voxel_size = torch.tensor(self.train_cfg['voxel_size'])
+
+ feature_map_size = grid_size[:2] // self.train_cfg['out_size_factor']
+
+ # reorganize the gt_dict by tasks
+ task_masks = []
+ flag = 0
+ for class_name in self.class_names:
+ task_masks.append([
+ torch.where(gt_labels_3d == class_name.index(i) + flag)
+ for i in class_name
+ ])
+ flag += len(class_name)
+
+ task_boxes = []
+ task_classes = []
+ flag2 = 0
+ for idx, mask in enumerate(task_masks):
+ task_box = []
+ task_class = []
+ for m in mask:
+ task_box.append(gt_bboxes_3d[m])
+ # 0 is background for each task, so we need to add 1 here.
+ task_class.append(gt_labels_3d[m] + 1 - flag2)
+ task_boxes.append(torch.cat(task_box, axis=0).to(device))
+ task_classes.append(torch.cat(task_class).long().to(device))
+ flag2 += len(mask)
+ draw_gaussian = draw_heatmap_gaussian
+ heatmaps, anno_boxes, inds, masks = [], [], [], []
+
+ for idx, task_head in enumerate(self.task_heads):
+ heatmap = gt_bboxes_3d.new_zeros(
+ (len(self.class_names[idx]), feature_map_size[1],
+ feature_map_size[0]))
+
+ anno_box = gt_bboxes_3d.new_zeros((max_objs, 10),
+ dtype=torch.float32)
+
+ ind = gt_labels_3d.new_zeros((max_objs), dtype=torch.int64)
+ mask = gt_bboxes_3d.new_zeros((max_objs), dtype=torch.uint8)
+
+ num_objs = min(task_boxes[idx].shape[0], max_objs)
+
+ for k in range(num_objs):
+ cls_id = task_classes[idx][k] - 1
+
+ width = task_boxes[idx][k][3]
+ length = task_boxes[idx][k][4]
+ width = width / voxel_size[0] / self.train_cfg[
+ 'out_size_factor']
+ length = length / voxel_size[1] / self.train_cfg[
+ 'out_size_factor']
+
+ if width > 0 and length > 0:
+ radius = gaussian_radius(
+ (length, width),
+ min_overlap=self.train_cfg['gaussian_overlap'])
+ radius = max(self.train_cfg['min_radius'], int(radius))
+
+ # be really careful for the coordinate system of
+ # your box annotation.
+ x, y, z = task_boxes[idx][k][0], task_boxes[idx][k][
+ 1], task_boxes[idx][k][2]
+
+ coor_x = (
+ x - pc_range[0]
+ ) / voxel_size[0] / self.train_cfg['out_size_factor']
+ coor_y = (
+ y - pc_range[1]
+ ) / voxel_size[1] / self.train_cfg['out_size_factor']
+
+ center = torch.tensor([coor_x, coor_y],
+ dtype=torch.float32,
+ device=device)
+ center_int = center.to(torch.int32)
+
+ # throw out not in range objects to avoid out of array
+ # area when creating the heatmap
+ if not (0 <= center_int[0] < feature_map_size[0]
+ and 0 <= center_int[1] < feature_map_size[1]):
+ continue
+
+ draw_gaussian(heatmap[cls_id], center_int, radius)
+
+ new_idx = k
+ x, y = center_int[0], center_int[1]
+
+ assert (y * feature_map_size[0] + x <
+ feature_map_size[0] * feature_map_size[1])
+
+ ind[new_idx] = y * feature_map_size[0] + x
+ mask[new_idx] = 1
+ # TODO: support other outdoor dataset
+ vx, vy = task_boxes[idx][k][7:]
+ rot = task_boxes[idx][k][6]
+ box_dim = task_boxes[idx][k][3:6]
+ if self.norm_bbox:
+ box_dim = box_dim.log()
+ anno_box[new_idx] = torch.cat([
+ center - torch.tensor([x, y], device=device),
+ z.unsqueeze(0), box_dim,
+ torch.sin(rot).unsqueeze(0),
+ torch.cos(rot).unsqueeze(0),
+ vx.unsqueeze(0),
+ vy.unsqueeze(0)
+ ])
+
+ heatmaps.append(heatmap)
+ anno_boxes.append(anno_box)
+ masks.append(mask)
+ inds.append(ind)
+ return heatmaps, anno_boxes, inds, masks
+
+ @force_fp32(apply_to=('preds_dicts'))
+ def loss(self, gt_bboxes_3d, gt_labels_3d, preds_dicts, **kwargs):
+ """Loss function for CenterHead.
+
+ Args:
+ gt_bboxes_3d (list[:obj:`LiDARInstance3DBoxes`]): Ground
+ truth gt boxes.
+ gt_labels_3d (list[torch.Tensor]): Labels of boxes.
+ preds_dicts (dict): Output of forward function.
+
+ Returns:
+ dict[str:torch.Tensor]: Loss of heatmap and bbox of each task.
+ """
+ heatmaps, anno_boxes, inds, masks = self.get_targets(
+ gt_bboxes_3d, gt_labels_3d)
+ loss_dict = dict()
+ for task_id, preds_dict in enumerate(preds_dicts):
+ # heatmap focal loss
+ preds_dict[0]['heatmap'] = clip_sigmoid(preds_dict[0]['heatmap'])
+ num_pos = heatmaps[task_id].eq(1).float().sum().item()
+ loss_heatmap = self.loss_cls(
+ preds_dict[0]['heatmap'],
+ heatmaps[task_id],
+ avg_factor=max(num_pos, 1))
+ target_box = anno_boxes[task_id]
+ # reconstruct the anno_box from multiple reg heads
+ preds_dict[0]['anno_box'] = torch.cat(
+ (preds_dict[0]['reg'], preds_dict[0]['height'],
+ preds_dict[0]['dim'], preds_dict[0]['rot'],
+ preds_dict[0]['vel']),
+ dim=1)
+
+ # Regression loss for dimension, offset, height, rotation
+ ind = inds[task_id]
+ num = masks[task_id].float().sum()
+ pred = preds_dict[0]['anno_box'].permute(0, 2, 3, 1).contiguous()
+ pred = pred.view(pred.size(0), -1, pred.size(3))
+ pred = self._gather_feat(pred, ind)
+ mask = masks[task_id].unsqueeze(2).expand_as(target_box).float()
+ isnotnan = (~torch.isnan(target_box)).float()
+ mask *= isnotnan
+
+ code_weights = self.train_cfg.get('code_weights', None)
+ bbox_weights = mask * mask.new_tensor(code_weights)
+ loss_bbox = self.loss_bbox(
+ pred, target_box, bbox_weights, avg_factor=(num + 1e-4))
+ loss_dict[f'task{task_id}.loss_heatmap'] = loss_heatmap
+ loss_dict[f'task{task_id}.loss_bbox'] = loss_bbox
+ return loss_dict
+
+ def get_bboxes(self, preds_dicts, img_metas, img=None, rescale=False):
+ """Generate bboxes from bbox head predictions.
+
+ Args:
+ preds_dicts (tuple[list[dict]]): Prediction results.
+ img_metas (list[dict]): Point cloud and image's meta info.
+
+ Returns:
+ list[dict]: Decoded bbox, scores and labels after nms.
+ """
+ rets = []
+ for task_id, preds_dict in enumerate(preds_dicts):
+ num_class_with_bg = self.num_classes[task_id]
+ batch_size = preds_dict[0]['heatmap'].shape[0]
+ batch_heatmap = preds_dict[0]['heatmap'].sigmoid()
+
+ batch_reg = preds_dict[0]['reg']
+ batch_hei = preds_dict[0]['height']
+
+ if self.norm_bbox:
+ batch_dim = torch.exp(preds_dict[0]['dim'])
+ else:
+ batch_dim = preds_dict[0]['dim']
+
+ batch_rots = preds_dict[0]['rot'][:, 0].unsqueeze(1)
+ batch_rotc = preds_dict[0]['rot'][:, 1].unsqueeze(1)
+
+ if 'vel' in preds_dict[0]:
+ batch_vel = preds_dict[0]['vel']
+ else:
+ batch_vel = None
+ temp = self.bbox_coder.decode(
+ batch_heatmap,
+ batch_rots,
+ batch_rotc,
+ batch_hei,
+ batch_dim,
+ batch_vel,
+ reg=batch_reg,
+ task_id=task_id)
+ assert self.test_cfg['nms_type'] in ['circle', 'rotate']
+ batch_reg_preds = [box['bboxes'] for box in temp]
+ batch_cls_preds = [box['scores'] for box in temp]
+ batch_cls_labels = [box['labels'] for box in temp]
+ if self.test_cfg['nms_type'] == 'circle':
+ ret_task = []
+ for i in range(batch_size):
+ boxes3d = temp[i]['bboxes']
+ scores = temp[i]['scores']
+ labels = temp[i]['labels']
+ centers = boxes3d[:, [0, 1]]
+ boxes = torch.cat([centers, scores.view(-1, 1)], dim=1)
+ keep = torch.tensor(
+ circle_nms(
+ boxes.detach().cpu().numpy(),
+ self.test_cfg['min_radius'][task_id],
+ post_max_size=self.test_cfg['post_max_size']),
+ dtype=torch.long,
+ device=boxes.device)
+
+ boxes3d = boxes3d[keep]
+ scores = scores[keep]
+ labels = labels[keep]
+ ret = dict(bboxes=boxes3d, scores=scores, labels=labels)
+ ret_task.append(ret)
+ rets.append(ret_task)
+ else:
+ rets.append(
+ self.get_task_detections(num_class_with_bg,
+ batch_cls_preds, batch_reg_preds,
+ batch_cls_labels, img_metas))
+
+ # Merge branches results
+ num_samples = len(rets[0])
+
+ ret_list = []
+ for i in range(num_samples):
+ for k in rets[0][i].keys():
+ if k == 'bboxes':
+ bboxes = torch.cat([ret[i][k] for ret in rets])
+ bboxes[:, 2] = bboxes[:, 2] - bboxes[:, 5] * 0.5
+ bboxes = img_metas[i]['box_type_3d'](
+ bboxes, self.bbox_coder.code_size)
+ elif k == 'scores':
+ scores = torch.cat([ret[i][k] for ret in rets])
+ elif k == 'labels':
+ flag = 0
+ for j, num_class in enumerate(self.num_classes):
+ rets[j][i][k] += flag
+ flag += num_class
+ labels = torch.cat([ret[i][k].int() for ret in rets])
+ ret_list.append([bboxes, scores, labels])
+ return ret_list
+
+ def get_task_detections(self, num_class_with_bg, batch_cls_preds,
+ batch_reg_preds, batch_cls_labels, img_metas):
+ """Rotate nms for each task.
+
+ Args:
+ num_class_with_bg (int): Number of classes for the current task.
+ batch_cls_preds (list[torch.Tensor]): Prediction score with the
+ shape of [N].
+ batch_reg_preds (list[torch.Tensor]): Prediction bbox with the
+ shape of [N, 9].
+ batch_cls_labels (list[torch.Tensor]): Prediction label with the
+ shape of [N].
+ img_metas (list[dict]): Meta information of each sample.
+
+ Returns:
+ list[dict[str: torch.Tensor]]: contains the following keys:
+
+ -bboxes (torch.Tensor): Prediction bboxes after nms with the
+ shape of [N, 9].
+ -scores (torch.Tensor): Prediction scores after nms with the
+ shape of [N].
+ -labels (torch.Tensor): Prediction labels after nms with the
+ shape of [N].
+ """
+ predictions_dicts = []
+ post_center_range = self.test_cfg['post_center_limit_range']
+ if len(post_center_range) > 0:
+ post_center_range = torch.tensor(
+ post_center_range,
+ dtype=batch_reg_preds[0].dtype,
+ device=batch_reg_preds[0].device)
+
+ for i, (box_preds, cls_preds, cls_labels) in enumerate(
+ zip(batch_reg_preds, batch_cls_preds, batch_cls_labels)):
+
+ # Apply NMS in bird eye view
+
+ # get the highest score per prediction, then apply nms
+ # to remove overlapped box.
+ if num_class_with_bg == 1:
+ top_scores = cls_preds.squeeze(-1)
+ top_labels = torch.zeros(
+ cls_preds.shape[0],
+ device=cls_preds.device,
+ dtype=torch.long)
+
+ else:
+ top_labels = cls_labels.long()
+ top_scores = cls_preds.squeeze(-1)
+
+ if self.test_cfg['score_threshold'] > 0.0:
+ thresh = torch.tensor(
+ [self.test_cfg['score_threshold']],
+ device=cls_preds.device).type_as(cls_preds)
+ top_scores_keep = top_scores >= thresh
+ top_scores = top_scores.masked_select(top_scores_keep)
+
+ if top_scores.shape[0] != 0:
+ if self.test_cfg['score_threshold'] > 0.0:
+ box_preds = box_preds[top_scores_keep]
+ top_labels = top_labels[top_scores_keep]
+
+ boxes_for_nms = xywhr2xyxyr(img_metas[i]['box_type_3d'](
+ box_preds[:, :], self.bbox_coder.code_size).bev)
+ # the nms in 3d detection just remove overlap boxes.
+
+ selected = nms_bev(
+ boxes_for_nms,
+ top_scores,
+ thresh=self.test_cfg['nms_thr'],
+ pre_max_size=self.test_cfg['pre_max_size'],
+ post_max_size=self.test_cfg['post_max_size'])
+ else:
+ selected = []
+
+ # if selected is not None:
+ selected_boxes = box_preds[selected]
+ selected_labels = top_labels[selected]
+ selected_scores = top_scores[selected]
+
+ # finally generate predictions.
+ if selected_boxes.shape[0] != 0:
+ box_preds = selected_boxes
+ scores = selected_scores
+ label_preds = selected_labels
+ final_box_preds = box_preds
+ final_scores = scores
+ final_labels = label_preds
+ if post_center_range is not None:
+ mask = (final_box_preds[:, :3] >=
+ post_center_range[:3]).all(1)
+ mask &= (final_box_preds[:, :3] <=
+ post_center_range[3:]).all(1)
+ predictions_dict = dict(
+ bboxes=final_box_preds[mask],
+ scores=final_scores[mask],
+ labels=final_labels[mask])
+ else:
+ predictions_dict = dict(
+ bboxes=final_box_preds,
+ scores=final_scores,
+ labels=final_labels)
+ else:
+ dtype = batch_reg_preds[0].dtype
+ device = batch_reg_preds[0].device
+ predictions_dict = dict(
+ bboxes=torch.zeros([0, self.bbox_coder.code_size],
+ dtype=dtype,
+ device=device),
+ scores=torch.zeros([0], dtype=dtype, device=device),
+ labels=torch.zeros([0],
+ dtype=top_labels.dtype,
+ device=device))
+
+ predictions_dicts.append(predictions_dict)
+ return predictions_dicts
diff --git a/mmdet3d/models/dense_heads/fcaf3d_head.py b/mmdet3d/models/dense_heads/fcaf3d_head.py
new file mode 100644
index 0000000..f666709
--- /dev/null
+++ b/mmdet3d/models/dense_heads/fcaf3d_head.py
@@ -0,0 +1,682 @@
+# Copyright (c) OpenMMLab. All rights reserved.
+# Adapted from https://github.com/SamsungLabs/fcaf3d/blob/master/mmdet3d/models/dense_heads/fcaf3d_neck_with_head.py # noqa
+try:
+ import MinkowskiEngine as ME
+except ImportError:
+ import warnings
+ warnings.warn(
+ 'Please follow `getting_started.md` to install MinkowskiEngine.`')
+
+import torch
+from mmcv.cnn import Scale, bias_init_with_prob
+from mmcv.ops import nms3d, nms3d_normal
+from mmcv.runner.base_module import BaseModule
+from torch import nn
+
+from mmdet3d.core.bbox.structures import rotation_3d_in_axis
+from mmdet3d.models import HEADS, build_loss
+from mmdet.core import reduce_mean
+
+
+@HEADS.register_module()
+class FCAF3DHead(BaseModule):
+ """Bbox head of `FCAF3D `_. Actually here
+ we store both the sparse 3D FPN and a head. The neck and the head can not
+ be simply separated as pruning score on the i-th level of FPN requires
+ classification scores from i+1-th level of the head.
+
+ Args:
+ n_classes (int): Number of classes.
+ in_channels (tuple[int]): Number of channels in input tensors.
+ out_channels (int): Number of channels in the neck output tensors.
+ n_reg_outs (int): Number of regression layer channels.
+ voxel_size (float): Voxel size in meters.
+ pts_prune_threshold (int): Pruning threshold on each feature level.
+ pts_assign_threshold (int): Min number of location per box to
+ be assigned with.
+ pts_center_threshold (int): Max number of locations per box to
+ be assigned with.
+ center_loss (dict, optional): Config of centerness loss.
+ bbox_loss (dict, optional): Config of bbox loss.
+ cls_loss (dict, optional): Config of classification loss.
+ train_cfg (dict, optional): Config for train stage. Defaults to None.
+ test_cfg (dict, optional): Config for test stage. Defaults to None.
+ init_cfg (dict, optional): Config for weight initialization.
+ Defaults to None.
+ """
+
+ def __init__(self,
+ n_classes,
+ in_channels,
+ out_channels,
+ n_reg_outs,
+ voxel_size,
+ pts_prune_threshold,
+ pts_assign_threshold,
+ pts_center_threshold,
+ center_loss=dict(type='CrossEntropyLoss', use_sigmoid=True),
+ bbox_loss=dict(type='AxisAlignedIoULoss'),
+ cls_loss=dict(type='FocalLoss'),
+ train_cfg=None,
+ test_cfg=None,
+ init_cfg=None):
+ super(FCAF3DHead, self).__init__(init_cfg)
+ self.voxel_size = voxel_size
+ self.pts_prune_threshold = pts_prune_threshold
+ self.pts_assign_threshold = pts_assign_threshold
+ self.pts_center_threshold = pts_center_threshold
+ self.center_loss = build_loss(center_loss)
+ self.bbox_loss = build_loss(bbox_loss)
+ self.cls_loss = build_loss(cls_loss)
+ self.train_cfg = train_cfg
+ self.test_cfg = test_cfg
+ self._init_layers(in_channels, out_channels, n_reg_outs, n_classes)
+
+ @staticmethod
+ def _make_block(in_channels, out_channels):
+ """Construct Conv-Norm-Act block.
+
+ Args:
+ in_channels (int): Number of input channels.
+ out_channels (int): Number of output channels.
+
+ Returns:
+ torch.nn.Module: With corresponding layers.
+ """
+ return nn.Sequential(
+ ME.MinkowskiConvolution(
+ in_channels, out_channels, kernel_size=3, dimension=3),
+ ME.MinkowskiBatchNorm(out_channels), ME.MinkowskiELU())
+
+ @staticmethod
+ def _make_up_block(in_channels, out_channels):
+ """Construct DeConv-Norm-Act-Conv-Norm-Act block.
+
+ Args:
+ in_channels (int): Number of input channels.
+ out_channels (int): Number of output channels.
+
+ Returns:
+ torch.nn.Module: With corresponding layers.
+ """
+ return nn.Sequential(
+ ME.MinkowskiGenerativeConvolutionTranspose(
+ in_channels,
+ out_channels,
+ kernel_size=2,
+ stride=2,
+ dimension=3), ME.MinkowskiBatchNorm(out_channels),
+ ME.MinkowskiELU(),
+ ME.MinkowskiConvolution(
+ out_channels, out_channels, kernel_size=3, dimension=3),
+ ME.MinkowskiBatchNorm(out_channels), ME.MinkowskiELU())
+
+ def _init_layers(self, in_channels, out_channels, n_reg_outs, n_classes):
+ """Initialize layers.
+
+ Args:
+ in_channels (tuple[int]): Number of channels in input tensors.
+ out_channels (int): Number of channels in the neck output tensors.
+ n_reg_outs (int): Number of regression layer channels.
+ n_classes (int): Number of classes.
+ """
+ # neck layers
+ self.pruning = ME.MinkowskiPruning()
+ for i in range(len(in_channels)):
+ if i > 0:
+ self.__setattr__(
+ f'up_block_{i}',
+ self._make_up_block(in_channels[i], in_channels[i - 1]))
+ self.__setattr__(f'out_block_{i}',
+ self._make_block(in_channels[i], out_channels))
+
+ # head layers
+ self.center_conv = ME.MinkowskiConvolution(
+ out_channels, 1, kernel_size=1, dimension=3)
+ self.reg_conv = ME.MinkowskiConvolution(
+ out_channels, n_reg_outs, kernel_size=1, dimension=3)
+ self.cls_conv = ME.MinkowskiConvolution(
+ out_channels, n_classes, kernel_size=1, bias=True, dimension=3)
+ self.scales = nn.ModuleList(
+ [Scale(1.) for _ in range(len(in_channels))])
+
+ def init_weights(self):
+ """Initialize weights."""
+ nn.init.normal_(self.center_conv.kernel, std=.01)
+ nn.init.normal_(self.reg_conv.kernel, std=.01)
+ nn.init.normal_(self.cls_conv.kernel, std=.01)
+ nn.init.constant_(self.cls_conv.bias, bias_init_with_prob(.01))
+
+ def forward(self, x):
+ """Forward pass.
+
+ Args:
+ x (list[Tensor]): Features from the backbone.
+
+ Returns:
+ list[list[Tensor]]: Predictions of the head.
+ """
+ center_preds, bbox_preds, cls_preds, points = [], [], [], []
+ inputs = x
+ x = inputs[-1]
+ prune_score = None
+ for i in range(len(inputs) - 1, -1, -1):
+ if i < len(inputs) - 1:
+ x = self.__getattr__(f'up_block_{i + 1}')(x)
+ x = inputs[i] + x
+ x = self._prune(x, prune_score)
+
+ out = self.__getattr__(f'out_block_{i}')(x)
+ center_pred, bbox_pred, cls_pred, point, prune_score = \
+ self._forward_single(out, self.scales[i])
+ center_preds.append(center_pred)
+ bbox_preds.append(bbox_pred)
+ cls_preds.append(cls_pred)
+ points.append(point)
+ return center_preds[::-1], bbox_preds[::-1], cls_preds[::-1], \
+ points[::-1]
+
+ def forward_train(self, x, gt_bboxes, gt_labels, img_metas):
+ """Forward pass of the train stage.
+
+ Args:
+ x (list[SparseTensor]): Features from the backbone.
+ gt_bboxes (list[:obj:`BaseInstance3DBoxes`]): Ground truth
+ bboxes of each sample.
+ gt_labels(list[torch.Tensor]): Labels of each sample.
+ img_metas (list[dict]): Contains scene meta info for each sample.
+
+ Returns:
+ dict: Centerness, bbox and classification loss values.
+ """
+ center_preds, bbox_preds, cls_preds, points = self(x)
+ return self._loss(center_preds, bbox_preds, cls_preds, points,
+ gt_bboxes, gt_labels, img_metas)
+
+ def forward_test(self, x, img_metas):
+ """Forward pass of the test stage.
+
+ Args:
+ x (list[SparseTensor]): Features from the backbone.
+ img_metas (list[dict]): Contains scene meta info for each sample.
+
+ Returns:
+ list[list[Tensor]]: bboxes, scores and labels for each sample.
+ """
+ center_preds, bbox_preds, cls_preds, points = self(x)
+ return self._get_bboxes(center_preds, bbox_preds, cls_preds, points,
+ img_metas)
+
+ def _prune(self, x, scores):
+ """Prunes the tensor by score thresholding.
+
+ Args:
+ x (SparseTensor): Tensor to be pruned.
+ scores (SparseTensor): Scores for thresholding.
+
+ Returns:
+ SparseTensor: Pruned tensor.
+ """
+ with torch.no_grad():
+ coordinates = x.C.float()
+ interpolated_scores = scores.features_at_coordinates(coordinates)
+ prune_mask = interpolated_scores.new_zeros(
+ (len(interpolated_scores)), dtype=torch.bool)
+ for permutation in x.decomposition_permutations:
+ score = interpolated_scores[permutation]
+ mask = score.new_zeros((len(score)), dtype=torch.bool)
+ topk = min(len(score), self.pts_prune_threshold)
+ ids = torch.topk(score.squeeze(1), topk, sorted=False).indices
+ mask[ids] = True
+ prune_mask[permutation[mask]] = True
+ x = self.pruning(x, prune_mask)
+ return x
+
+ def _forward_single(self, x, scale):
+ """Forward pass per level.
+
+ Args:
+ x (SparseTensor): Per level neck output tensor.
+ scale (mmcv.cnn.Scale): Per level multiplication weight.
+
+ Returns:
+ tuple[Tensor]: Per level head predictions.
+ """
+ center_pred = self.center_conv(x).features
+ scores = self.cls_conv(x)
+ cls_pred = scores.features
+ prune_scores = ME.SparseTensor(
+ scores.features.max(dim=1, keepdim=True).values,
+ coordinate_map_key=scores.coordinate_map_key,
+ coordinate_manager=scores.coordinate_manager)
+ reg_final = self.reg_conv(x).features
+ reg_distance = torch.exp(scale(reg_final[:, :6]))
+ reg_angle = reg_final[:, 6:]
+ bbox_pred = torch.cat((reg_distance, reg_angle), dim=1)
+
+ center_preds, bbox_preds, cls_preds, points = [], [], [], []
+ for permutation in x.decomposition_permutations:
+ center_preds.append(center_pred[permutation])
+ bbox_preds.append(bbox_pred[permutation])
+ cls_preds.append(cls_pred[permutation])
+
+ points = x.decomposed_coordinates
+ for i in range(len(points)):
+ points[i] = points[i] * self.voxel_size
+
+ return center_preds, bbox_preds, cls_preds, points, prune_scores
+
+ def _loss_single(self, center_preds, bbox_preds, cls_preds, points,
+ gt_bboxes, gt_labels, img_meta):
+ """Per scene loss function.
+
+ Args:
+ center_preds (list[Tensor]): Centerness predictions for all levels.
+ bbox_preds (list[Tensor]): Bbox predictions for all levels.
+ cls_preds (list[Tensor]): Classification predictions for all
+ levels.
+ points (list[Tensor]): Final location coordinates for all levels.
+ gt_bboxes (BaseInstance3DBoxes): Ground truth boxes.
+ gt_labels (Tensor): Ground truth labels.
+ img_meta (dict): Scene meta info.
+
+ Returns:
+ tuple[Tensor]: Centerness, bbox, and classification loss values.
+ """
+ center_targets, bbox_targets, cls_targets = self._get_targets(
+ points, gt_bboxes, gt_labels)
+
+ center_preds = torch.cat(center_preds)
+ bbox_preds = torch.cat(bbox_preds)
+ cls_preds = torch.cat(cls_preds)
+ points = torch.cat(points)
+
+ # cls loss
+ pos_inds = torch.nonzero(cls_targets >= 0).squeeze(1)
+ n_pos = points.new_tensor(len(pos_inds))
+ n_pos = max(reduce_mean(n_pos), 1.)
+ cls_loss = self.cls_loss(cls_preds, cls_targets, avg_factor=n_pos)
+
+ # bbox and centerness losses
+ pos_center_preds = center_preds[pos_inds]
+ pos_bbox_preds = bbox_preds[pos_inds]
+ pos_center_targets = center_targets[pos_inds].unsqueeze(1)
+ pos_bbox_targets = bbox_targets[pos_inds]
+ # reduce_mean is outside if / else block to prevent deadlock
+ center_denorm = max(
+ reduce_mean(pos_center_targets.sum().detach()), 1e-6)
+ if len(pos_inds) > 0:
+ pos_points = points[pos_inds]
+ center_loss = self.center_loss(
+ pos_center_preds, pos_center_targets, avg_factor=n_pos)
+ bbox_loss = self.bbox_loss(
+ self._bbox_to_loss(
+ self._bbox_pred_to_bbox(pos_points, pos_bbox_preds)),
+ self._bbox_to_loss(pos_bbox_targets),
+ weight=pos_center_targets.squeeze(1),
+ avg_factor=center_denorm)
+ else:
+ center_loss = pos_center_preds.sum()
+ bbox_loss = pos_bbox_preds.sum()
+ return center_loss, bbox_loss, cls_loss
+
+ def _loss(self, center_preds, bbox_preds, cls_preds, points, gt_bboxes,
+ gt_labels, img_metas):
+ """Per scene loss function.
+
+ Args:
+ center_preds (list[list[Tensor]]): Centerness predictions for
+ all scenes.
+ bbox_preds (list[list[Tensor]]): Bbox predictions for all scenes.
+ cls_preds (list[list[Tensor]]): Classification predictions for all
+ scenes.
+ points (list[list[Tensor]]): Final location coordinates for all
+ scenes.
+ gt_bboxes (list[BaseInstance3DBoxes]): Ground truth boxes for all
+ scenes.
+ gt_labels (list[Tensor]): Ground truth labels for all scenes.
+ img_metas (list[dict]): Meta infos for all scenes.
+
+ Returns:
+ dict: Centerness, bbox, and classification loss values.
+ """
+ center_losses, bbox_losses, cls_losses = [], [], []
+ for i in range(len(img_metas)):
+ center_loss, bbox_loss, cls_loss = self._loss_single(
+ center_preds=[x[i] for x in center_preds],
+ bbox_preds=[x[i] for x in bbox_preds],
+ cls_preds=[x[i] for x in cls_preds],
+ points=[x[i] for x in points],
+ img_meta=img_metas[i],
+ gt_bboxes=gt_bboxes[i],
+ gt_labels=gt_labels[i])
+ center_losses.append(center_loss)
+ bbox_losses.append(bbox_loss)
+ cls_losses.append(cls_loss)
+ return dict(
+ center_loss=torch.mean(torch.stack(center_losses)),
+ bbox_loss=torch.mean(torch.stack(bbox_losses)),
+ cls_loss=torch.mean(torch.stack(cls_losses)))
+
+ def _get_bboxes_single(self, center_preds, bbox_preds, cls_preds, points,
+ img_meta):
+ """Generate boxes for a single scene.
+
+ Args:
+ center_preds (list[Tensor]): Centerness predictions for all levels.
+ bbox_preds (list[Tensor]): Bbox predictions for all levels.
+ cls_preds (list[Tensor]): Classification predictions for all
+ levels.
+ points (list[Tensor]): Final location coordinates for all levels.
+ img_meta (dict): Scene meta info.
+
+ Returns:
+ tuple[Tensor]: Predicted bounding boxes, scores and labels.
+ """
+ mlvl_bboxes, mlvl_scores = [], []
+ for center_pred, bbox_pred, cls_pred, point in zip(
+ center_preds, bbox_preds, cls_preds, points):
+ scores = cls_pred.sigmoid() * center_pred.sigmoid()
+ max_scores, _ = scores.max(dim=1)
+
+ if len(scores) > self.test_cfg.nms_pre > 0:
+ _, ids = max_scores.topk(self.test_cfg.nms_pre)
+ bbox_pred = bbox_pred[ids]
+ scores = scores[ids]
+ point = point[ids]
+
+ bboxes = self._bbox_pred_to_bbox(point, bbox_pred)
+ mlvl_bboxes.append(bboxes)
+ mlvl_scores.append(scores)
+
+ bboxes = torch.cat(mlvl_bboxes)
+ scores = torch.cat(mlvl_scores)
+ bboxes, scores, labels = self._single_scene_multiclass_nms(
+ bboxes, scores, img_meta)
+ return bboxes, scores, labels
+
+ def _get_bboxes(self, center_preds, bbox_preds, cls_preds, points,
+ img_metas):
+ """Generate boxes for all scenes.
+
+ Args:
+ center_preds (list[list[Tensor]]): Centerness predictions for
+ all scenes.
+ bbox_preds (list[list[Tensor]]): Bbox predictions for all scenes.
+ cls_preds (list[list[Tensor]]): Classification predictions for all
+ scenes.
+ points (list[list[Tensor]]): Final location coordinates for all
+ scenes.
+ img_metas (list[dict]): Meta infos for all scenes.
+
+ Returns:
+ list[tuple[Tensor]]: Predicted bboxes, scores, and labels for
+ all scenes.
+ """
+ results = []
+ for i in range(len(img_metas)):
+ result = self._get_bboxes_single(
+ center_preds=[x[i] for x in center_preds],
+ bbox_preds=[x[i] for x in bbox_preds],
+ cls_preds=[x[i] for x in cls_preds],
+ points=[x[i] for x in points],
+ img_meta=img_metas[i])
+ results.append(result)
+ return results
+
+ @staticmethod
+ def _bbox_to_loss(bbox):
+ """Transform box to the axis-aligned or rotated iou loss format.
+
+ Args:
+ bbox (Tensor): 3D box of shape (N, 6) or (N, 7).
+
+ Returns:
+ Tensor: Transformed 3D box of shape (N, 6) or (N, 7).
+ """
+ # rotated iou loss accepts (x, y, z, w, h, l, heading)
+ if bbox.shape[-1] != 6:
+ return bbox
+
+ # axis-aligned case: x, y, z, w, h, l -> x1, y1, z1, x2, y2, z2
+ return torch.stack(
+ (bbox[..., 0] - bbox[..., 3] / 2, bbox[..., 1] - bbox[..., 4] / 2,
+ bbox[..., 2] - bbox[..., 5] / 2, bbox[..., 0] + bbox[..., 3] / 2,
+ bbox[..., 1] + bbox[..., 4] / 2, bbox[..., 2] + bbox[..., 5] / 2),
+ dim=-1)
+
+ @staticmethod
+ def _bbox_pred_to_bbox(points, bbox_pred):
+ """Transform predicted bbox parameters to bbox.
+
+ Args:
+ points (Tensor): Final locations of shape (N, 3)
+ bbox_pred (Tensor): Predicted bbox parameters of shape (N, 6)
+ or (N, 8).
+
+ Returns:
+ Tensor: Transformed 3D box of shape (N, 6) or (N, 7).
+ """
+ if bbox_pred.shape[0] == 0:
+ return bbox_pred
+
+ x_center = points[:, 0] + (bbox_pred[:, 1] - bbox_pred[:, 0]) / 2
+ y_center = points[:, 1] + (bbox_pred[:, 3] - bbox_pred[:, 2]) / 2
+ z_center = points[:, 2] + (bbox_pred[:, 5] - bbox_pred[:, 4]) / 2
+
+ # dx_min, dx_max, dy_min, dy_max, dz_min, dz_max -> x, y, z, w, l, h
+ base_bbox = torch.stack([
+ x_center,
+ y_center,
+ z_center,
+ bbox_pred[:, 0] + bbox_pred[:, 1],
+ bbox_pred[:, 2] + bbox_pred[:, 3],
+ bbox_pred[:, 4] + bbox_pred[:, 5],
+ ], -1)
+
+ # axis-aligned case
+ if bbox_pred.shape[1] == 6:
+ return base_bbox
+
+ # rotated case: ..., sin(2a)ln(q), cos(2a)ln(q)
+ scale = bbox_pred[:, 0] + bbox_pred[:, 1] + \
+ bbox_pred[:, 2] + bbox_pred[:, 3]
+ q = torch.exp(
+ torch.sqrt(
+ torch.pow(bbox_pred[:, 6], 2) + torch.pow(bbox_pred[:, 7], 2)))
+ alpha = 0.5 * torch.atan2(bbox_pred[:, 6], bbox_pred[:, 7])
+ return torch.stack(
+ (x_center, y_center, z_center, scale / (1 + q), scale /
+ (1 + q) * q, bbox_pred[:, 5] + bbox_pred[:, 4], alpha),
+ dim=-1)
+
+ @staticmethod
+ def _get_face_distances(points, boxes):
+ """Calculate distances from point to box faces.
+
+ Args:
+ points (Tensor): Final locations of shape (N_points, N_boxes, 3).
+ boxes (Tensor): 3D boxes of shape (N_points, N_boxes, 7)
+
+ Returns:
+ Tensor: Face distances of shape (N_points, N_boxes, 6),
+ (dx_min, dx_max, dy_min, dy_max, dz_min, dz_max).
+ """
+ shift = torch.stack(
+ (points[..., 0] - boxes[..., 0], points[..., 1] - boxes[..., 1],
+ points[..., 2] - boxes[..., 2]),
+ dim=-1).permute(1, 0, 2)
+ shift = rotation_3d_in_axis(
+ shift, -boxes[0, :, 6], axis=2).permute(1, 0, 2)
+ centers = boxes[..., :3] + shift
+ dx_min = centers[..., 0] - boxes[..., 0] + boxes[..., 3] / 2
+ dx_max = boxes[..., 0] + boxes[..., 3] / 2 - centers[..., 0]
+ dy_min = centers[..., 1] - boxes[..., 1] + boxes[..., 4] / 2
+ dy_max = boxes[..., 1] + boxes[..., 4] / 2 - centers[..., 1]
+ dz_min = centers[..., 2] - boxes[..., 2] + boxes[..., 5] / 2
+ dz_max = boxes[..., 2] + boxes[..., 5] / 2 - centers[..., 2]
+ return torch.stack((dx_min, dx_max, dy_min, dy_max, dz_min, dz_max),
+ dim=-1)
+
+ @staticmethod
+ def _get_centerness(face_distances):
+ """Compute point centerness w.r.t containing box.
+
+ Args:
+ face_distances (Tensor): Face distances of shape (B, N, 6),
+ (dx_min, dx_max, dy_min, dy_max, dz_min, dz_max).
+
+ Returns:
+ Tensor: Centerness of shape (B, N).
+ """
+ x_dims = face_distances[..., [0, 1]]
+ y_dims = face_distances[..., [2, 3]]
+ z_dims = face_distances[..., [4, 5]]
+ centerness_targets = x_dims.min(dim=-1)[0] / x_dims.max(dim=-1)[0] * \
+ y_dims.min(dim=-1)[0] / y_dims.max(dim=-1)[0] * \
+ z_dims.min(dim=-1)[0] / z_dims.max(dim=-1)[0]
+ return torch.sqrt(centerness_targets)
+
+ @torch.no_grad()
+ def _get_targets(self, points, gt_bboxes, gt_labels):
+ """Compute targets for final locations for a single scene.
+
+ Args:
+ points (list[Tensor]): Final locations for all levels.
+ gt_bboxes (BaseInstance3DBoxes): Ground truth boxes.
+ gt_labels (Tensor): Ground truth labels.
+
+ Returns:
+ Tensor: Centerness targets for all locations.
+ Tensor: Bbox targets for all locations.
+ Tensor: Classification targets for all locations.
+ """
+ float_max = points[0].new_tensor(1e8)
+ n_levels = len(points)
+ levels = torch.cat([
+ points[i].new_tensor(i).expand(len(points[i]))
+ for i in range(len(points))
+ ])
+ points = torch.cat(points)
+ gt_bboxes = gt_bboxes.to(points.device)
+ n_points = len(points)
+ n_boxes = len(gt_bboxes)
+ volumes = gt_bboxes.volume.unsqueeze(0).expand(n_points, n_boxes)
+
+ # condition 1: point inside box
+ boxes = torch.cat((gt_bboxes.gravity_center, gt_bboxes.tensor[:, 3:]),
+ dim=1)
+ boxes = boxes.expand(n_points, n_boxes, 7)
+ points = points.unsqueeze(1).expand(n_points, n_boxes, 3)
+ face_distances = self._get_face_distances(points, boxes)
+ inside_box_condition = face_distances.min(dim=-1).values > 0
+
+ # condition 2: positive points per level >= limit
+ # calculate positive points per scale
+ n_pos_points_per_level = []
+ for i in range(n_levels):
+ n_pos_points_per_level.append(
+ torch.sum(inside_box_condition[levels == i], dim=0))
+ # find best level
+ n_pos_points_per_level = torch.stack(n_pos_points_per_level, dim=0)
+ lower_limit_mask = n_pos_points_per_level < self.pts_assign_threshold
+ lower_index = torch.argmax(lower_limit_mask.int(), dim=0) - 1
+ lower_index = torch.where(lower_index < 0, 0, lower_index)
+ all_upper_limit_mask = torch.all(
+ torch.logical_not(lower_limit_mask), dim=0)
+ best_level = torch.where(all_upper_limit_mask, n_levels - 1,
+ lower_index)
+ # keep only points with best level
+ best_level = best_level.expand(n_points, n_boxes)
+ levels = torch.unsqueeze(levels, 1).expand(n_points, n_boxes)
+ level_condition = best_level == levels
+
+ # condition 3: limit topk points per box by centerness
+ centerness = self._get_centerness(face_distances)
+ centerness = torch.where(inside_box_condition, centerness,
+ torch.ones_like(centerness) * -1)
+ centerness = torch.where(level_condition, centerness,
+ torch.ones_like(centerness) * -1)
+ top_centerness = torch.topk(
+ centerness,
+ min(self.pts_center_threshold + 1, len(centerness)),
+ dim=0).values[-1]
+ topk_condition = centerness > top_centerness.unsqueeze(0)
+
+ # condition 4: min volume box per point
+ volumes = torch.where(inside_box_condition, volumes, float_max)
+ volumes = torch.where(level_condition, volumes, float_max)
+ volumes = torch.where(topk_condition, volumes, float_max)
+ min_volumes, min_inds = volumes.min(dim=1)
+
+ center_targets = centerness[torch.arange(n_points), min_inds]
+ bbox_targets = boxes[torch.arange(n_points), min_inds]
+ if not gt_bboxes.with_yaw:
+ bbox_targets = bbox_targets[:, :-1]
+ cls_targets = gt_labels[min_inds]
+ cls_targets = torch.where(min_volumes == float_max, -1, cls_targets)
+ return center_targets, bbox_targets, cls_targets
+
+ def _single_scene_multiclass_nms(self, bboxes, scores, img_meta):
+ """Multi-class nms for a single scene.
+
+ Args:
+ bboxes (Tensor): Predicted boxes of shape (N_boxes, 6) or
+ (N_boxes, 7).
+ scores (Tensor): Predicted scores of shape (N_boxes, N_classes).
+ img_meta (dict): Scene meta data.
+
+ Returns:
+ Tensor: Predicted bboxes.
+ Tensor: Predicted scores.
+ Tensor: Predicted labels.
+ """
+ n_classes = scores.shape[1]
+ yaw_flag = bboxes.shape[1] == 7
+ nms_bboxes, nms_scores, nms_labels = [], [], []
+ for i in range(n_classes):
+ ids = scores[:, i] > self.test_cfg.score_thr
+ if not ids.any():
+ continue
+
+ class_scores = scores[ids, i]
+ class_bboxes = bboxes[ids]
+ if yaw_flag:
+ nms_function = nms3d
+ else:
+ class_bboxes = torch.cat(
+ (class_bboxes, torch.zeros_like(class_bboxes[:, :1])),
+ dim=1)
+ nms_function = nms3d_normal
+
+ nms_ids = nms_function(class_bboxes, class_scores,
+ self.test_cfg.iou_thr)
+ nms_bboxes.append(class_bboxes[nms_ids])
+ nms_scores.append(class_scores[nms_ids])
+ nms_labels.append(
+ bboxes.new_full(
+ class_scores[nms_ids].shape, i, dtype=torch.long))
+
+ if len(nms_bboxes):
+ nms_bboxes = torch.cat(nms_bboxes, dim=0)
+ nms_scores = torch.cat(nms_scores, dim=0)
+ nms_labels = torch.cat(nms_labels, dim=0)
+ else:
+ nms_bboxes = bboxes.new_zeros((0, bboxes.shape[1]))
+ nms_scores = bboxes.new_zeros((0, ))
+ nms_labels = bboxes.new_zeros((0, ))
+
+ if yaw_flag:
+ box_dim = 7
+ with_yaw = True
+ else:
+ box_dim = 6
+ with_yaw = False
+ nms_bboxes = nms_bboxes[:, :6]
+ nms_bboxes = img_meta['box_type_3d'](
+ nms_bboxes,
+ box_dim=box_dim,
+ with_yaw=with_yaw,
+ origin=(.5, .5, .5))
+
+ return nms_bboxes, nms_scores, nms_labels
diff --git a/mmdet3d/models/dense_heads/fcos_mono3d_head.py b/mmdet3d/models/dense_heads/fcos_mono3d_head.py
new file mode 100644
index 0000000..d0aa29f
--- /dev/null
+++ b/mmdet3d/models/dense_heads/fcos_mono3d_head.py
@@ -0,0 +1,956 @@
+# Copyright (c) OpenMMLab. All rights reserved.
+from logging import warning
+
+import numpy as np
+import torch
+from mmcv.cnn import Scale, normal_init
+from mmcv.runner import force_fp32
+from torch import nn as nn
+
+from mmdet3d.core import (box3d_multiclass_nms, limit_period, points_img2cam,
+ xywhr2xyxyr)
+from mmdet.core import multi_apply
+from mmdet.core.bbox.builder import build_bbox_coder
+from ..builder import HEADS, build_loss
+from .anchor_free_mono3d_head import AnchorFreeMono3DHead
+
+INF = 1e8
+
+
+@HEADS.register_module()
+class FCOSMono3DHead(AnchorFreeMono3DHead):
+ """Anchor-free head used in FCOS3D.
+
+ Args:
+ num_classes (int): Number of categories excluding the background
+ category.
+ in_channels (int): Number of channels in the input feature map.
+ regress_ranges (tuple[tuple[int, int]], optional): Regress range of multiple
+ level points.
+ center_sampling (bool, optional): If true, use center sampling. Default: True.
+ center_sample_radius (float, optional): Radius of center sampling. Default: 1.5.
+ norm_on_bbox (bool, optional): If true, normalize the regression targets
+ with FPN strides. Default: True.
+ centerness_on_reg (bool, optional): If true, position centerness on the
+ regress branch. Please refer to https://github.com/tianzhi0549/FCOS/issues/89#issuecomment-516877042.
+ Default: True.
+ centerness_alpha (int, optional): Parameter used to adjust the intensity
+ attenuation from the center to the periphery. Default: 2.5.
+ loss_cls (dict, optional): Config of classification loss.
+ loss_bbox (dict, optional): Config of localization loss.
+ loss_dir (dict, optional): Config of direction classification loss.
+ loss_attr (dict, optional): Config of attribute classification loss.
+ loss_centerness (dict, optional): Config of centerness loss.
+ norm_cfg (dict, optional): dictionary to construct and config norm layer.
+ Default: norm_cfg=dict(type='GN', num_groups=32, requires_grad=True).
+ centerness_branch (tuple[int], optional): Channels for centerness branch.
+ Default: (64, ).
+ """ # noqa: E501
+
+ def __init__(self,
+ regress_ranges=((-1, 48), (48, 96), (96, 192), (192, 384),
+ (384, INF)),
+ center_sampling=True,
+ center_sample_radius=1.5,
+ norm_on_bbox=True,
+ centerness_on_reg=True,
+ centerness_alpha=2.5,
+ loss_cls=dict(
+ type='FocalLoss',
+ use_sigmoid=True,
+ gamma=2.0,
+ alpha=0.25,
+ loss_weight=1.0),
+ loss_bbox=dict(
+ type='SmoothL1Loss', beta=1.0 / 9.0, loss_weight=1.0),
+ loss_dir=dict(
+ type='CrossEntropyLoss',
+ use_sigmoid=False,
+ loss_weight=1.0),
+ loss_attr=dict(
+ type='CrossEntropyLoss',
+ use_sigmoid=False,
+ loss_weight=1.0),
+ loss_centerness=dict(
+ type='CrossEntropyLoss',
+ use_sigmoid=True,
+ loss_weight=1.0),
+ bbox_coder=dict(type='FCOS3DBBoxCoder', code_size=9),
+ norm_cfg=dict(type='GN', num_groups=32, requires_grad=True),
+ centerness_branch=(64, ),
+ init_cfg=None,
+ **kwargs):
+ self.regress_ranges = regress_ranges
+ self.center_sampling = center_sampling
+ self.center_sample_radius = center_sample_radius
+ self.norm_on_bbox = norm_on_bbox
+ self.centerness_on_reg = centerness_on_reg
+ self.centerness_alpha = centerness_alpha
+ self.centerness_branch = centerness_branch
+ super().__init__(
+ loss_cls=loss_cls,
+ loss_bbox=loss_bbox,
+ loss_dir=loss_dir,
+ loss_attr=loss_attr,
+ norm_cfg=norm_cfg,
+ init_cfg=init_cfg,
+ **kwargs)
+ self.loss_centerness = build_loss(loss_centerness)
+ bbox_coder['code_size'] = self.bbox_code_size
+ self.bbox_coder = build_bbox_coder(bbox_coder)
+
+ def _init_layers(self):
+ """Initialize layers of the head."""
+ super()._init_layers()
+ self.conv_centerness_prev = self._init_branch(
+ conv_channels=self.centerness_branch,
+ conv_strides=(1, ) * len(self.centerness_branch))
+ self.conv_centerness = nn.Conv2d(self.centerness_branch[-1], 1, 1)
+ self.scale_dim = 3 # only for offset, depth and size regression
+ self.scales = nn.ModuleList([
+ nn.ModuleList([Scale(1.0) for _ in range(self.scale_dim)])
+ for _ in self.strides
+ ])
+
+ def init_weights(self):
+ """Initialize weights of the head.
+
+ We currently still use the customized init_weights because the default
+ init of DCN triggered by the init_cfg will init conv_offset.weight,
+ which mistakenly affects the training stability.
+ """
+ super().init_weights()
+ for m in self.conv_centerness_prev:
+ if isinstance(m.conv, nn.Conv2d):
+ normal_init(m.conv, std=0.01)
+ normal_init(self.conv_centerness, std=0.01)
+
+ def forward(self, feats):
+ """Forward features from the upstream network.
+
+ Args:
+ feats (tuple[Tensor]): Features from the upstream network, each is
+ a 4D-tensor.
+
+ Returns:
+ tuple:
+ cls_scores (list[Tensor]): Box scores for each scale level,
+ each is a 4D-tensor, the channel number is
+ num_points * num_classes.
+ bbox_preds (list[Tensor]): Box energies / deltas for each scale
+ level, each is a 4D-tensor, the channel number is
+ num_points * bbox_code_size.
+ dir_cls_preds (list[Tensor]): Box scores for direction class
+ predictions on each scale level, each is a 4D-tensor,
+ the channel number is num_points * 2. (bin = 2).
+ attr_preds (list[Tensor]): Attribute scores for each scale
+ level, each is a 4D-tensor, the channel number is
+ num_points * num_attrs.
+ centernesses (list[Tensor]): Centerness for each scale level,
+ each is a 4D-tensor, the channel number is num_points * 1.
+ """
+ # Note: we use [:5] to filter feats and only return predictions
+ return multi_apply(self.forward_single, feats, self.scales,
+ self.strides)[:5]
+
+ def forward_single(self, x, scale, stride):
+ """Forward features of a single scale level.
+
+ Args:
+ x (Tensor): FPN feature maps of the specified stride.
+ scale (:obj: `mmcv.cnn.Scale`): Learnable scale module to resize
+ the bbox prediction.
+ stride (int): The corresponding stride for feature maps, only
+ used to normalize the bbox prediction when self.norm_on_bbox
+ is True.
+
+ Returns:
+ tuple: scores for each class, bbox and direction class
+ predictions, centerness predictions of input feature maps.
+ """
+ cls_score, bbox_pred, dir_cls_pred, attr_pred, cls_feat, reg_feat = \
+ super().forward_single(x)
+
+ if self.centerness_on_reg:
+ clone_reg_feat = reg_feat.clone()
+ for conv_centerness_prev_layer in self.conv_centerness_prev:
+ clone_reg_feat = conv_centerness_prev_layer(clone_reg_feat)
+ centerness = self.conv_centerness(clone_reg_feat)
+ else:
+ clone_cls_feat = cls_feat.clone()
+ for conv_centerness_prev_layer in self.conv_centerness_prev:
+ clone_cls_feat = conv_centerness_prev_layer(clone_cls_feat)
+ centerness = self.conv_centerness(clone_cls_feat)
+
+ bbox_pred = self.bbox_coder.decode(bbox_pred, scale, stride,
+ self.training, cls_score)
+
+ return cls_score, bbox_pred, dir_cls_pred, attr_pred, centerness, \
+ cls_feat, reg_feat
+
+ @staticmethod
+ def add_sin_difference(boxes1, boxes2):
+ """Convert the rotation difference to difference in sine function.
+
+ Args:
+ boxes1 (torch.Tensor): Original Boxes in shape (NxC), where C>=7
+ and the 7th dimension is rotation dimension.
+ boxes2 (torch.Tensor): Target boxes in shape (NxC), where C>=7 and
+ the 7th dimension is rotation dimension.
+
+ Returns:
+ tuple[torch.Tensor]: ``boxes1`` and ``boxes2`` whose 7th
+ dimensions are changed.
+ """
+ rad_pred_encoding = torch.sin(boxes1[..., 6:7]) * torch.cos(
+ boxes2[..., 6:7])
+ rad_tg_encoding = torch.cos(boxes1[..., 6:7]) * torch.sin(boxes2[...,
+ 6:7])
+ boxes1 = torch.cat(
+ [boxes1[..., :6], rad_pred_encoding, boxes1[..., 7:]], dim=-1)
+ boxes2 = torch.cat([boxes2[..., :6], rad_tg_encoding, boxes2[..., 7:]],
+ dim=-1)
+ return boxes1, boxes2
+
+ @staticmethod
+ def get_direction_target(reg_targets,
+ dir_offset=0,
+ dir_limit_offset=0.0,
+ num_bins=2,
+ one_hot=True):
+ """Encode direction to 0 ~ num_bins-1.
+
+ Args:
+ reg_targets (torch.Tensor): Bbox regression targets.
+ dir_offset (int, optional): Direction offset. Default to 0.
+ dir_limit_offset (float, optional): Offset to set the direction
+ range. Default to 0.0.
+ num_bins (int, optional): Number of bins to divide 2*PI.
+ Default to 2.
+ one_hot (bool, optional): Whether to encode as one hot.
+ Default to True.
+
+ Returns:
+ torch.Tensor: Encoded direction targets.
+ """
+ rot_gt = reg_targets[..., 6]
+ offset_rot = limit_period(rot_gt - dir_offset, dir_limit_offset,
+ 2 * np.pi)
+ dir_cls_targets = torch.floor(offset_rot /
+ (2 * np.pi / num_bins)).long()
+ dir_cls_targets = torch.clamp(dir_cls_targets, min=0, max=num_bins - 1)
+ if one_hot:
+ dir_targets = torch.zeros(
+ *list(dir_cls_targets.shape),
+ num_bins,
+ dtype=reg_targets.dtype,
+ device=dir_cls_targets.device)
+ dir_targets.scatter_(dir_cls_targets.unsqueeze(dim=-1).long(), 1.0)
+ dir_cls_targets = dir_targets
+ return dir_cls_targets
+
+ @force_fp32(
+ apply_to=('cls_scores', 'bbox_preds', 'dir_cls_preds', 'attr_preds',
+ 'centernesses'))
+ def loss(self,
+ cls_scores,
+ bbox_preds,
+ dir_cls_preds,
+ attr_preds,
+ centernesses,
+ gt_bboxes,
+ gt_labels,
+ gt_bboxes_3d,
+ gt_labels_3d,
+ centers2d,
+ depths,
+ attr_labels,
+ img_metas,
+ gt_bboxes_ignore=None):
+ """Compute loss of the head.
+
+ Args:
+ cls_scores (list[Tensor]): Box scores for each scale level,
+ each is a 4D-tensor, the channel number is
+ num_points * num_classes.
+ bbox_preds (list[Tensor]): Box energies / deltas for each scale
+ level, each is a 4D-tensor, the channel number is
+ num_points * bbox_code_size.
+ dir_cls_preds (list[Tensor]): Box scores for direction class
+ predictions on each scale level, each is a 4D-tensor,
+ the channel number is num_points * 2. (bin = 2)
+ attr_preds (list[Tensor]): Attribute scores for each scale level,
+ each is a 4D-tensor, the channel number is
+ num_points * num_attrs.
+ centernesses (list[Tensor]): Centerness for each scale level, each
+ is a 4D-tensor, the channel number is num_points * 1.
+ gt_bboxes (list[Tensor]): Ground truth bboxes for each image with
+ shape (num_gts, 4) in [tl_x, tl_y, br_x, br_y] format.
+ gt_labels (list[Tensor]): class indices corresponding to each box
+ gt_bboxes_3d (list[Tensor]): 3D boxes ground truth with shape of
+ (num_gts, code_size).
+ gt_labels_3d (list[Tensor]): same as gt_labels
+ centers2d (list[Tensor]): 2D centers on the image with shape of
+ (num_gts, 2).
+ depths (list[Tensor]): Depth ground truth with shape of
+ (num_gts, ).
+ attr_labels (list[Tensor]): Attributes indices of each box.
+ img_metas (list[dict]): Meta information of each image, e.g.,
+ image size, scaling factor, etc.
+ gt_bboxes_ignore (list[Tensor]): specify which bounding
+ boxes can be ignored when computing the loss.
+
+ Returns:
+ dict[str, Tensor]: A dictionary of loss components.
+ """
+ assert len(cls_scores) == len(bbox_preds) == len(centernesses) == len(
+ attr_preds)
+ featmap_sizes = [featmap.size()[-2:] for featmap in cls_scores]
+ all_level_points = self.get_points(featmap_sizes, bbox_preds[0].dtype,
+ bbox_preds[0].device)
+ labels_3d, bbox_targets_3d, centerness_targets, attr_targets = \
+ self.get_targets(
+ all_level_points, gt_bboxes, gt_labels, gt_bboxes_3d,
+ gt_labels_3d, centers2d, depths, attr_labels)
+
+ num_imgs = cls_scores[0].size(0)
+ # flatten cls_scores, bbox_preds, dir_cls_preds and centerness
+ flatten_cls_scores = [
+ cls_score.permute(0, 2, 3, 1).reshape(-1, self.cls_out_channels)
+ for cls_score in cls_scores
+ ]
+ flatten_bbox_preds = [
+ bbox_pred.permute(0, 2, 3, 1).reshape(-1, sum(self.group_reg_dims))
+ for bbox_pred in bbox_preds
+ ]
+ flatten_dir_cls_preds = [
+ dir_cls_pred.permute(0, 2, 3, 1).reshape(-1, 2)
+ for dir_cls_pred in dir_cls_preds
+ ]
+ flatten_centerness = [
+ centerness.permute(0, 2, 3, 1).reshape(-1)
+ for centerness in centernesses
+ ]
+ flatten_cls_scores = torch.cat(flatten_cls_scores)
+ flatten_bbox_preds = torch.cat(flatten_bbox_preds)
+ flatten_dir_cls_preds = torch.cat(flatten_dir_cls_preds)
+ flatten_centerness = torch.cat(flatten_centerness)
+ flatten_labels_3d = torch.cat(labels_3d)
+ flatten_bbox_targets_3d = torch.cat(bbox_targets_3d)
+ flatten_centerness_targets = torch.cat(centerness_targets)
+
+ # FG cat_id: [0, num_classes -1], BG cat_id: num_classes
+ bg_class_ind = self.num_classes
+ pos_inds = ((flatten_labels_3d >= 0)
+ & (flatten_labels_3d < bg_class_ind)).nonzero().reshape(-1)
+ num_pos = len(pos_inds)
+
+ loss_cls = self.loss_cls(
+ flatten_cls_scores,
+ flatten_labels_3d,
+ avg_factor=num_pos + num_imgs) # avoid num_pos is 0
+
+ pos_bbox_preds = flatten_bbox_preds[pos_inds]
+ pos_dir_cls_preds = flatten_dir_cls_preds[pos_inds]
+ pos_centerness = flatten_centerness[pos_inds]
+
+ if self.pred_attrs:
+ flatten_attr_preds = [
+ attr_pred.permute(0, 2, 3, 1).reshape(-1, self.num_attrs)
+ for attr_pred in attr_preds
+ ]
+ flatten_attr_preds = torch.cat(flatten_attr_preds)
+ flatten_attr_targets = torch.cat(attr_targets)
+ pos_attr_preds = flatten_attr_preds[pos_inds]
+
+ if num_pos > 0:
+ pos_bbox_targets_3d = flatten_bbox_targets_3d[pos_inds]
+ pos_centerness_targets = flatten_centerness_targets[pos_inds]
+ if self.pred_attrs:
+ pos_attr_targets = flatten_attr_targets[pos_inds]
+ bbox_weights = pos_centerness_targets.new_ones(
+ len(pos_centerness_targets), sum(self.group_reg_dims))
+ equal_weights = pos_centerness_targets.new_ones(
+ pos_centerness_targets.shape)
+
+ code_weight = self.train_cfg.get('code_weight', None)
+ if code_weight:
+ assert len(code_weight) == sum(self.group_reg_dims)
+ bbox_weights = bbox_weights * bbox_weights.new_tensor(
+ code_weight)
+
+ if self.use_direction_classifier:
+ pos_dir_cls_targets = self.get_direction_target(
+ pos_bbox_targets_3d,
+ self.dir_offset,
+ self.dir_limit_offset,
+ one_hot=False)
+
+ if self.diff_rad_by_sin:
+ pos_bbox_preds, pos_bbox_targets_3d = self.add_sin_difference(
+ pos_bbox_preds, pos_bbox_targets_3d)
+
+ loss_offset = self.loss_bbox(
+ pos_bbox_preds[:, :2],
+ pos_bbox_targets_3d[:, :2],
+ weight=bbox_weights[:, :2],
+ avg_factor=equal_weights.sum())
+ loss_depth = self.loss_bbox(
+ pos_bbox_preds[:, 2],
+ pos_bbox_targets_3d[:, 2],
+ weight=bbox_weights[:, 2],
+ avg_factor=equal_weights.sum())
+ loss_size = self.loss_bbox(
+ pos_bbox_preds[:, 3:6],
+ pos_bbox_targets_3d[:, 3:6],
+ weight=bbox_weights[:, 3:6],
+ avg_factor=equal_weights.sum())
+ loss_rotsin = self.loss_bbox(
+ pos_bbox_preds[:, 6],
+ pos_bbox_targets_3d[:, 6],
+ weight=bbox_weights[:, 6],
+ avg_factor=equal_weights.sum())
+ loss_velo = None
+ if self.pred_velo:
+ loss_velo = self.loss_bbox(
+ pos_bbox_preds[:, 7:9],
+ pos_bbox_targets_3d[:, 7:9],
+ weight=bbox_weights[:, 7:9],
+ avg_factor=equal_weights.sum())
+
+ loss_centerness = self.loss_centerness(pos_centerness,
+ pos_centerness_targets)
+
+ # direction classification loss
+ loss_dir = None
+ # TODO: add more check for use_direction_classifier
+ if self.use_direction_classifier:
+ loss_dir = self.loss_dir(
+ pos_dir_cls_preds,
+ pos_dir_cls_targets,
+ equal_weights,
+ avg_factor=equal_weights.sum())
+
+ # attribute classification loss
+ loss_attr = None
+ if self.pred_attrs:
+ loss_attr = self.loss_attr(
+ pos_attr_preds,
+ pos_attr_targets,
+ pos_centerness_targets,
+ avg_factor=pos_centerness_targets.sum())
+
+ else:
+ # need absolute due to possible negative delta x/y
+ loss_offset = pos_bbox_preds[:, :2].sum()
+ loss_depth = pos_bbox_preds[:, 2].sum()
+ loss_size = pos_bbox_preds[:, 3:6].sum()
+ loss_rotsin = pos_bbox_preds[:, 6].sum()
+ loss_velo = None
+ if self.pred_velo:
+ loss_velo = pos_bbox_preds[:, 7:9].sum()
+ loss_centerness = pos_centerness.sum()
+ loss_dir = None
+ if self.use_direction_classifier:
+ loss_dir = pos_dir_cls_preds.sum()
+ loss_attr = None
+ if self.pred_attrs:
+ loss_attr = pos_attr_preds.sum()
+
+ loss_dict = dict(
+ loss_cls=loss_cls,
+ loss_offset=loss_offset,
+ loss_depth=loss_depth,
+ loss_size=loss_size,
+ loss_rotsin=loss_rotsin,
+ loss_centerness=loss_centerness)
+
+ if loss_velo is not None:
+ loss_dict['loss_velo'] = loss_velo
+
+ if loss_dir is not None:
+ loss_dict['loss_dir'] = loss_dir
+
+ if loss_attr is not None:
+ loss_dict['loss_attr'] = loss_attr
+
+ return loss_dict
+
+ @force_fp32(
+ apply_to=('cls_scores', 'bbox_preds', 'dir_cls_preds', 'attr_preds',
+ 'centernesses'))
+ def get_bboxes(self,
+ cls_scores,
+ bbox_preds,
+ dir_cls_preds,
+ attr_preds,
+ centernesses,
+ img_metas,
+ cfg=None,
+ rescale=None):
+ """Transform network output for a batch into bbox predictions.
+
+ Args:
+ cls_scores (list[Tensor]): Box scores for each scale level
+ Has shape (N, num_points * num_classes, H, W)
+ bbox_preds (list[Tensor]): Box energies / deltas for each scale
+ level with shape (N, num_points * 4, H, W)
+ dir_cls_preds (list[Tensor]): Box scores for direction class
+ predictions on each scale level, each is a 4D-tensor,
+ the channel number is num_points * 2. (bin = 2)
+ attr_preds (list[Tensor]): Attribute scores for each scale level
+ Has shape (N, num_points * num_attrs, H, W)
+ centernesses (list[Tensor]): Centerness for each scale level with
+ shape (N, num_points * 1, H, W)
+ img_metas (list[dict]): Meta information of each image, e.g.,
+ image size, scaling factor, etc.
+ cfg (mmcv.Config): Test / postprocessing configuration,
+ if None, test_cfg would be used
+ rescale (bool): If True, return boxes in original image space
+
+ Returns:
+ list[tuple[Tensor, Tensor]]: Each item in result_list is 2-tuple.
+ The first item is an (n, 5) tensor, where the first 4 columns
+ are bounding box positions (tl_x, tl_y, br_x, br_y) and the
+ 5-th column is a score between 0 and 1. The second item is a
+ (n,) tensor where each item is the predicted class label of
+ the corresponding box.
+ """
+ assert len(cls_scores) == len(bbox_preds) == len(dir_cls_preds) == \
+ len(centernesses) == len(attr_preds)
+ num_levels = len(cls_scores)
+
+ featmap_sizes = [featmap.size()[-2:] for featmap in cls_scores]
+ mlvl_points = self.get_points(featmap_sizes, bbox_preds[0].dtype,
+ bbox_preds[0].device)
+ result_list = []
+ for img_id in range(len(img_metas)):
+ cls_score_list = [
+ cls_scores[i][img_id].detach() for i in range(num_levels)
+ ]
+ bbox_pred_list = [
+ bbox_preds[i][img_id].detach() for i in range(num_levels)
+ ]
+ if self.use_direction_classifier:
+ dir_cls_pred_list = [
+ dir_cls_preds[i][img_id].detach()
+ for i in range(num_levels)
+ ]
+ else:
+ dir_cls_pred_list = [
+ cls_scores[i][img_id].new_full(
+ [2, *cls_scores[i][img_id].shape[1:]], 0).detach()
+ for i in range(num_levels)
+ ]
+ if self.pred_attrs:
+ attr_pred_list = [
+ attr_preds[i][img_id].detach() for i in range(num_levels)
+ ]
+ else:
+ attr_pred_list = [
+ cls_scores[i][img_id].new_full(
+ [self.num_attrs, *cls_scores[i][img_id].shape[1:]],
+ self.attr_background_label).detach()
+ for i in range(num_levels)
+ ]
+ centerness_pred_list = [
+ centernesses[i][img_id].detach() for i in range(num_levels)
+ ]
+ input_meta = img_metas[img_id]
+ det_bboxes = self._get_bboxes_single(
+ cls_score_list, bbox_pred_list, dir_cls_pred_list,
+ attr_pred_list, centerness_pred_list, mlvl_points, input_meta,
+ cfg, rescale)
+ result_list.append(det_bboxes)
+ return result_list
+
+ def _get_bboxes_single(self,
+ cls_scores,
+ bbox_preds,
+ dir_cls_preds,
+ attr_preds,
+ centernesses,
+ mlvl_points,
+ input_meta,
+ cfg,
+ rescale=False):
+ """Transform outputs for a single batch item into bbox predictions.
+
+ Args:
+ cls_scores (list[Tensor]): Box scores for a single scale level
+ Has shape (num_points * num_classes, H, W).
+ bbox_preds (list[Tensor]): Box energies / deltas for a single scale
+ level with shape (num_points * bbox_code_size, H, W).
+ dir_cls_preds (list[Tensor]): Box scores for direction class
+ predictions on a single scale level with shape
+ (num_points * 2, H, W)
+ attr_preds (list[Tensor]): Attribute scores for each scale level
+ Has shape (N, num_points * num_attrs, H, W)
+ centernesses (list[Tensor]): Centerness for a single scale level
+ with shape (num_points, H, W).
+ mlvl_points (list[Tensor]): Box reference for a single scale level
+ with shape (num_total_points, 2).
+ input_meta (dict): Metadata of input image.
+ cfg (mmcv.Config): Test / postprocessing configuration,
+ if None, test_cfg would be used.
+ rescale (bool): If True, return boxes in original image space.
+
+ Returns:
+ tuples[Tensor]: Predicted 3D boxes, scores, labels and attributes.
+ """
+ view = np.array(input_meta['cam2img'])
+ scale_factor = input_meta['scale_factor']
+ cfg = self.test_cfg if cfg is None else cfg
+ assert len(cls_scores) == len(bbox_preds) == len(mlvl_points)
+ mlvl_centers2d = []
+ mlvl_bboxes = []
+ mlvl_scores = []
+ mlvl_dir_scores = []
+ mlvl_attr_scores = []
+ mlvl_centerness = []
+
+ for cls_score, bbox_pred, dir_cls_pred, attr_pred, centerness, \
+ points in zip(cls_scores, bbox_preds, dir_cls_preds,
+ attr_preds, centernesses, mlvl_points):
+ assert cls_score.size()[-2:] == bbox_pred.size()[-2:]
+ scores = cls_score.permute(1, 2, 0).reshape(
+ -1, self.cls_out_channels).sigmoid()
+ dir_cls_pred = dir_cls_pred.permute(1, 2, 0).reshape(-1, 2)
+ dir_cls_score = torch.max(dir_cls_pred, dim=-1)[1]
+ attr_pred = attr_pred.permute(1, 2, 0).reshape(-1, self.num_attrs)
+ attr_score = torch.max(attr_pred, dim=-1)[1]
+ centerness = centerness.permute(1, 2, 0).reshape(-1).sigmoid()
+
+ bbox_pred = bbox_pred.permute(1, 2,
+ 0).reshape(-1,
+ sum(self.group_reg_dims))
+ bbox_pred = bbox_pred[:, :self.bbox_code_size]
+ nms_pre = cfg.get('nms_pre', -1)
+ if nms_pre > 0 and scores.shape[0] > nms_pre:
+ max_scores, _ = (scores * centerness[:, None]).max(dim=1)
+ _, topk_inds = max_scores.topk(nms_pre)
+ points = points[topk_inds, :]
+ bbox_pred = bbox_pred[topk_inds, :]
+ scores = scores[topk_inds, :]
+ dir_cls_pred = dir_cls_pred[topk_inds, :]
+ centerness = centerness[topk_inds]
+ dir_cls_score = dir_cls_score[topk_inds]
+ attr_score = attr_score[topk_inds]
+ # change the offset to actual center predictions
+ bbox_pred[:, :2] = points - bbox_pred[:, :2]
+ if rescale:
+ bbox_pred[:, :2] /= bbox_pred[:, :2].new_tensor(scale_factor)
+ pred_center2d = bbox_pred[:, :3].clone()
+ bbox_pred[:, :3] = points_img2cam(bbox_pred[:, :3], view)
+ mlvl_centers2d.append(pred_center2d)
+ mlvl_bboxes.append(bbox_pred)
+ mlvl_scores.append(scores)
+ mlvl_dir_scores.append(dir_cls_score)
+ mlvl_attr_scores.append(attr_score)
+ mlvl_centerness.append(centerness)
+
+ mlvl_centers2d = torch.cat(mlvl_centers2d)
+ mlvl_bboxes = torch.cat(mlvl_bboxes)
+ mlvl_dir_scores = torch.cat(mlvl_dir_scores)
+
+ # change local yaw to global yaw for 3D nms
+ cam2img = mlvl_centers2d.new_zeros((4, 4))
+ cam2img[:view.shape[0], :view.shape[1]] = \
+ mlvl_centers2d.new_tensor(view)
+ mlvl_bboxes = self.bbox_coder.decode_yaw(mlvl_bboxes, mlvl_centers2d,
+ mlvl_dir_scores,
+ self.dir_offset, cam2img)
+
+ mlvl_bboxes_for_nms = xywhr2xyxyr(input_meta['box_type_3d'](
+ mlvl_bboxes, box_dim=self.bbox_code_size,
+ origin=(0.5, 0.5, 0.5)).bev)
+
+ mlvl_scores = torch.cat(mlvl_scores)
+ padding = mlvl_scores.new_zeros(mlvl_scores.shape[0], 1)
+ # remind that we set FG labels to [0, num_class-1] since mmdet v2.0
+ # BG cat_id: num_class
+ mlvl_scores = torch.cat([mlvl_scores, padding], dim=1)
+ mlvl_attr_scores = torch.cat(mlvl_attr_scores)
+ mlvl_centerness = torch.cat(mlvl_centerness)
+ # no scale_factors in box3d_multiclass_nms
+ # Then we multiply it from outside
+ mlvl_nms_scores = mlvl_scores * mlvl_centerness[:, None]
+ results = box3d_multiclass_nms(mlvl_bboxes, mlvl_bboxes_for_nms,
+ mlvl_nms_scores, cfg.score_thr,
+ cfg.max_per_img, cfg, mlvl_dir_scores,
+ mlvl_attr_scores)
+ bboxes, scores, labels, dir_scores, attrs = results
+ attrs = attrs.to(labels.dtype) # change data type to int
+ bboxes = input_meta['box_type_3d'](
+ bboxes, box_dim=self.bbox_code_size, origin=(0.5, 0.5, 0.5))
+ # Note that the predictions use origin (0.5, 0.5, 0.5)
+ # Due to the ground truth centers2d are the gravity center of objects
+ # v0.10.0 fix inplace operation to the input tensor of cam_box3d
+ # So here we also need to add origin=(0.5, 0.5, 0.5)
+ if not self.pred_attrs:
+ attrs = None
+
+ return bboxes, scores, labels, attrs
+
+ @staticmethod
+ def pts2Dto3D(points, view):
+ """
+ Args:
+ points (torch.Tensor): points in 2D images, [N, 3],
+ 3 corresponds with x, y in the image and depth.
+ view (np.ndarray): camera intrinsic, [3, 3]
+
+ Returns:
+ torch.Tensor: points in 3D space. [N, 3],
+ 3 corresponds with x, y, z in 3D space.
+ """
+ warning.warn('DeprecationWarning: This static method has been moved '
+ 'out of this class to mmdet3d/core. The function '
+ 'pts2Dto3D will be deprecated.')
+
+ assert view.shape[0] <= 4
+ assert view.shape[1] <= 4
+ assert points.shape[1] == 3
+
+ points2D = points[:, :2]
+ depths = points[:, 2].view(-1, 1)
+ unnorm_points2D = torch.cat([points2D * depths, depths], dim=1)
+
+ viewpad = torch.eye(4, dtype=points2D.dtype, device=points2D.device)
+ viewpad[:view.shape[0], :view.shape[1]] = points2D.new_tensor(view)
+ inv_viewpad = torch.inverse(viewpad).transpose(0, 1)
+
+ # Do operation in homogeneous coordinates.
+ nbr_points = unnorm_points2D.shape[0]
+ homo_points2D = torch.cat(
+ [unnorm_points2D,
+ points2D.new_ones((nbr_points, 1))], dim=1)
+ points3D = torch.mm(homo_points2D, inv_viewpad)[:, :3]
+
+ return points3D
+
+ def _get_points_single(self,
+ featmap_size,
+ stride,
+ dtype,
+ device,
+ flatten=False):
+ """Get points according to feature map sizes."""
+ y, x = super()._get_points_single(featmap_size, stride, dtype, device)
+ points = torch.stack((x.reshape(-1) * stride, y.reshape(-1) * stride),
+ dim=-1) + stride // 2
+ return points
+
+ def get_targets(self, points, gt_bboxes_list, gt_labels_list,
+ gt_bboxes_3d_list, gt_labels_3d_list, centers2d_list,
+ depths_list, attr_labels_list):
+ """Compute regression, classification and centerss targets for points
+ in multiple images.
+
+ Args:
+ points (list[Tensor]): Points of each fpn level, each has shape
+ (num_points, 2).
+ gt_bboxes_list (list[Tensor]): Ground truth bboxes of each image,
+ each has shape (num_gt, 4).
+ gt_labels_list (list[Tensor]): Ground truth labels of each box,
+ each has shape (num_gt,).
+ gt_bboxes_3d_list (list[Tensor]): 3D Ground truth bboxes of each
+ image, each has shape (num_gt, bbox_code_size).
+ gt_labels_3d_list (list[Tensor]): 3D Ground truth labels of each
+ box, each has shape (num_gt,).
+ centers2d_list (list[Tensor]): Projected 3D centers onto 2D image,
+ each has shape (num_gt, 2).
+ depths_list (list[Tensor]): Depth of projected 3D centers onto 2D
+ image, each has shape (num_gt, 1).
+ attr_labels_list (list[Tensor]): Attribute labels of each box,
+ each has shape (num_gt,).
+
+ Returns:
+ tuple:
+ concat_lvl_labels (list[Tensor]): Labels of each level.
+ concat_lvl_bbox_targets (list[Tensor]): BBox targets of each
+ level.
+ """
+ assert len(points) == len(self.regress_ranges)
+ num_levels = len(points)
+ # expand regress ranges to align with points
+ expanded_regress_ranges = [
+ points[i].new_tensor(self.regress_ranges[i])[None].expand_as(
+ points[i]) for i in range(num_levels)
+ ]
+ # concat all levels points and regress ranges
+ concat_regress_ranges = torch.cat(expanded_regress_ranges, dim=0)
+ concat_points = torch.cat(points, dim=0)
+
+ # the number of points per img, per lvl
+ num_points = [center.size(0) for center in points]
+
+ if attr_labels_list is None:
+ attr_labels_list = [
+ gt_labels.new_full(gt_labels.shape, self.attr_background_label)
+ for gt_labels in gt_labels_list
+ ]
+
+ # get labels and bbox_targets of each image
+ _, _, labels_3d_list, bbox_targets_3d_list, centerness_targets_list, \
+ attr_targets_list = multi_apply(
+ self._get_target_single,
+ gt_bboxes_list,
+ gt_labels_list,
+ gt_bboxes_3d_list,
+ gt_labels_3d_list,
+ centers2d_list,
+ depths_list,
+ attr_labels_list,
+ points=concat_points,
+ regress_ranges=concat_regress_ranges,
+ num_points_per_lvl=num_points)
+
+ # split to per img, per level
+ labels_3d_list = [
+ labels_3d.split(num_points, 0) for labels_3d in labels_3d_list
+ ]
+ bbox_targets_3d_list = [
+ bbox_targets_3d.split(num_points, 0)
+ for bbox_targets_3d in bbox_targets_3d_list
+ ]
+ centerness_targets_list = [
+ centerness_targets.split(num_points, 0)
+ for centerness_targets in centerness_targets_list
+ ]
+ attr_targets_list = [
+ attr_targets.split(num_points, 0)
+ for attr_targets in attr_targets_list
+ ]
+
+ # concat per level image
+ concat_lvl_labels_3d = []
+ concat_lvl_bbox_targets_3d = []
+ concat_lvl_centerness_targets = []
+ concat_lvl_attr_targets = []
+ for i in range(num_levels):
+ concat_lvl_labels_3d.append(
+ torch.cat([labels[i] for labels in labels_3d_list]))
+ concat_lvl_centerness_targets.append(
+ torch.cat([
+ centerness_targets[i]
+ for centerness_targets in centerness_targets_list
+ ]))
+ bbox_targets_3d = torch.cat([
+ bbox_targets_3d[i] for bbox_targets_3d in bbox_targets_3d_list
+ ])
+ concat_lvl_attr_targets.append(
+ torch.cat(
+ [attr_targets[i] for attr_targets in attr_targets_list]))
+ if self.norm_on_bbox:
+ bbox_targets_3d[:, :
+ 2] = bbox_targets_3d[:, :2] / self.strides[i]
+ concat_lvl_bbox_targets_3d.append(bbox_targets_3d)
+ return concat_lvl_labels_3d, concat_lvl_bbox_targets_3d, \
+ concat_lvl_centerness_targets, concat_lvl_attr_targets
+
+ def _get_target_single(self, gt_bboxes, gt_labels, gt_bboxes_3d,
+ gt_labels_3d, centers2d, depths, attr_labels,
+ points, regress_ranges, num_points_per_lvl):
+ """Compute regression and classification targets for a single image."""
+ num_points = points.size(0)
+ num_gts = gt_labels.size(0)
+ if not isinstance(gt_bboxes_3d, torch.Tensor):
+ gt_bboxes_3d = gt_bboxes_3d.tensor.to(gt_bboxes.device)
+ if num_gts == 0:
+ return gt_labels.new_full((num_points,), self.background_label), \
+ gt_bboxes.new_zeros((num_points, 4)), \
+ gt_labels_3d.new_full(
+ (num_points,), self.background_label), \
+ gt_bboxes_3d.new_zeros((num_points, self.bbox_code_size)), \
+ gt_bboxes_3d.new_zeros((num_points,)), \
+ attr_labels.new_full(
+ (num_points,), self.attr_background_label)
+
+ # change orientation to local yaw
+ gt_bboxes_3d[..., 6] = -torch.atan2(
+ gt_bboxes_3d[..., 0], gt_bboxes_3d[..., 2]) + gt_bboxes_3d[..., 6]
+
+ areas = (gt_bboxes[:, 2] - gt_bboxes[:, 0]) * (
+ gt_bboxes[:, 3] - gt_bboxes[:, 1])
+ areas = areas[None].repeat(num_points, 1)
+ regress_ranges = regress_ranges[:, None, :].expand(
+ num_points, num_gts, 2)
+ gt_bboxes = gt_bboxes[None].expand(num_points, num_gts, 4)
+ centers2d = centers2d[None].expand(num_points, num_gts, 2)
+ gt_bboxes_3d = gt_bboxes_3d[None].expand(num_points, num_gts,
+ self.bbox_code_size)
+ depths = depths[None, :, None].expand(num_points, num_gts, 1)
+ xs, ys = points[:, 0], points[:, 1]
+ xs = xs[:, None].expand(num_points, num_gts)
+ ys = ys[:, None].expand(num_points, num_gts)
+
+ delta_xs = (xs - centers2d[..., 0])[..., None]
+ delta_ys = (ys - centers2d[..., 1])[..., None]
+ bbox_targets_3d = torch.cat(
+ (delta_xs, delta_ys, depths, gt_bboxes_3d[..., 3:]), dim=-1)
+
+ left = xs - gt_bboxes[..., 0]
+ right = gt_bboxes[..., 2] - xs
+ top = ys - gt_bboxes[..., 1]
+ bottom = gt_bboxes[..., 3] - ys
+ bbox_targets = torch.stack((left, top, right, bottom), -1)
+
+ assert self.center_sampling is True, 'Setting center_sampling to '\
+ 'False has not been implemented for FCOS3D.'
+ # condition1: inside a `center bbox`
+ radius = self.center_sample_radius
+ center_xs = centers2d[..., 0]
+ center_ys = centers2d[..., 1]
+ center_gts = torch.zeros_like(gt_bboxes)
+ stride = center_xs.new_zeros(center_xs.shape)
+
+ # project the points on current lvl back to the `original` sizes
+ lvl_begin = 0
+ for lvl_idx, num_points_lvl in enumerate(num_points_per_lvl):
+ lvl_end = lvl_begin + num_points_lvl
+ stride[lvl_begin:lvl_end] = self.strides[lvl_idx] * radius
+ lvl_begin = lvl_end
+
+ center_gts[..., 0] = center_xs - stride
+ center_gts[..., 1] = center_ys - stride
+ center_gts[..., 2] = center_xs + stride
+ center_gts[..., 3] = center_ys + stride
+
+ cb_dist_left = xs - center_gts[..., 0]
+ cb_dist_right = center_gts[..., 2] - xs
+ cb_dist_top = ys - center_gts[..., 1]
+ cb_dist_bottom = center_gts[..., 3] - ys
+ center_bbox = torch.stack(
+ (cb_dist_left, cb_dist_top, cb_dist_right, cb_dist_bottom), -1)
+ inside_gt_bbox_mask = center_bbox.min(-1)[0] > 0
+
+ # condition2: limit the regression range for each location
+ max_regress_distance = bbox_targets.max(-1)[0]
+ inside_regress_range = (
+ (max_regress_distance >= regress_ranges[..., 0])
+ & (max_regress_distance <= regress_ranges[..., 1]))
+
+ # center-based criterion to deal with ambiguity
+ dists = torch.sqrt(torch.sum(bbox_targets_3d[..., :2]**2, dim=-1))
+ dists[inside_gt_bbox_mask == 0] = INF
+ dists[inside_regress_range == 0] = INF
+ min_dist, min_dist_inds = dists.min(dim=1)
+
+ labels = gt_labels[min_dist_inds]
+ labels_3d = gt_labels_3d[min_dist_inds]
+ attr_labels = attr_labels[min_dist_inds]
+ labels[min_dist == INF] = self.background_label # set as BG
+ labels_3d[min_dist == INF] = self.background_label # set as BG
+ attr_labels[min_dist == INF] = self.attr_background_label
+
+ bbox_targets = bbox_targets[range(num_points), min_dist_inds]
+ bbox_targets_3d = bbox_targets_3d[range(num_points), min_dist_inds]
+ relative_dists = torch.sqrt(
+ torch.sum(bbox_targets_3d[..., :2]**2,
+ dim=-1)) / (1.414 * stride[:, 0])
+ # [N, 1] / [N, 1]
+ centerness_targets = torch.exp(-self.centerness_alpha * relative_dists)
+
+ return labels, bbox_targets, labels_3d, bbox_targets_3d, \
+ centerness_targets, attr_labels
diff --git a/mmdet3d/models/dense_heads/free_anchor3d_head.py b/mmdet3d/models/dense_heads/free_anchor3d_head.py
new file mode 100644
index 0000000..a56f2c7
--- /dev/null
+++ b/mmdet3d/models/dense_heads/free_anchor3d_head.py
@@ -0,0 +1,285 @@
+# Copyright (c) OpenMMLab. All rights reserved.
+import torch
+from mmcv.runner import force_fp32
+from torch.nn import functional as F
+
+from mmdet3d.core.bbox import bbox_overlaps_nearest_3d
+from ..builder import HEADS
+from .anchor3d_head import Anchor3DHead
+from .train_mixins import get_direction_target
+
+
+@HEADS.register_module()
+class FreeAnchor3DHead(Anchor3DHead):
+ r"""`FreeAnchor `_ head for 3D detection.
+
+ Note:
+ This implementation is directly modified from the `mmdet implementation
+ `_.
+ We find it also works on 3D detection with minor modification, i.e.,
+ different hyper-parameters and a additional direction classifier.
+
+ Args:
+ pre_anchor_topk (int): Number of boxes that be token in each bag.
+ bbox_thr (float): The threshold of the saturated linear function. It is
+ usually the same with the IoU threshold used in NMS.
+ gamma (float): Gamma parameter in focal loss.
+ alpha (float): Alpha parameter in focal loss.
+ kwargs (dict): Other arguments are the same as those in :class:`Anchor3DHead`.
+ """ # noqa: E501
+
+ def __init__(self,
+ pre_anchor_topk=50,
+ bbox_thr=0.6,
+ gamma=2.0,
+ alpha=0.5,
+ init_cfg=None,
+ **kwargs):
+ super().__init__(init_cfg=init_cfg, **kwargs)
+ self.pre_anchor_topk = pre_anchor_topk
+ self.bbox_thr = bbox_thr
+ self.gamma = gamma
+ self.alpha = alpha
+
+ @force_fp32(apply_to=('cls_scores', 'bbox_preds', 'dir_cls_preds'))
+ def loss(self,
+ cls_scores,
+ bbox_preds,
+ dir_cls_preds,
+ gt_bboxes,
+ gt_labels,
+ input_metas,
+ gt_bboxes_ignore=None):
+ """Calculate loss of FreeAnchor head.
+
+ Args:
+ cls_scores (list[torch.Tensor]): Classification scores of
+ different samples.
+ bbox_preds (list[torch.Tensor]): Box predictions of
+ different samples
+ dir_cls_preds (list[torch.Tensor]): Direction predictions of
+ different samples
+ gt_bboxes (list[:obj:`BaseInstance3DBoxes`]): Ground truth boxes.
+ gt_labels (list[torch.Tensor]): Ground truth labels.
+ input_metas (list[dict]): List of input meta information.
+ gt_bboxes_ignore (list[:obj:`BaseInstance3DBoxes`], optional):
+ Ground truth boxes that should be ignored. Defaults to None.
+
+ Returns:
+ dict[str, torch.Tensor]: Loss items.
+
+ - positive_bag_loss (torch.Tensor): Loss of positive samples.
+ - negative_bag_loss (torch.Tensor): Loss of negative samples.
+ """
+ featmap_sizes = [featmap.size()[-2:] for featmap in cls_scores]
+ assert len(featmap_sizes) == self.anchor_generator.num_levels
+
+ anchor_list = self.get_anchors(featmap_sizes, input_metas)
+ anchors = [torch.cat(anchor) for anchor in anchor_list]
+
+ # concatenate each level
+ cls_scores = [
+ cls_score.permute(0, 2, 3, 1).reshape(
+ cls_score.size(0), -1, self.num_classes)
+ for cls_score in cls_scores
+ ]
+ bbox_preds = [
+ bbox_pred.permute(0, 2, 3, 1).reshape(
+ bbox_pred.size(0), -1, self.box_code_size)
+ for bbox_pred in bbox_preds
+ ]
+ dir_cls_preds = [
+ dir_cls_pred.permute(0, 2, 3,
+ 1).reshape(dir_cls_pred.size(0), -1, 2)
+ for dir_cls_pred in dir_cls_preds
+ ]
+
+ cls_scores = torch.cat(cls_scores, dim=1)
+ bbox_preds = torch.cat(bbox_preds, dim=1)
+ dir_cls_preds = torch.cat(dir_cls_preds, dim=1)
+
+ cls_prob = torch.sigmoid(cls_scores)
+ box_prob = []
+ num_pos = 0
+ positive_losses = []
+ for _, (anchors_, gt_labels_, gt_bboxes_, cls_prob_, bbox_preds_,
+ dir_cls_preds_) in enumerate(
+ zip(anchors, gt_labels, gt_bboxes, cls_prob, bbox_preds,
+ dir_cls_preds)):
+
+ gt_bboxes_ = gt_bboxes_.tensor.to(anchors_.device)
+
+ with torch.no_grad():
+ # box_localization: a_{j}^{loc}, shape: [j, 4]
+ pred_boxes = self.bbox_coder.decode(anchors_, bbox_preds_)
+
+ # object_box_iou: IoU_{ij}^{loc}, shape: [i, j]
+ object_box_iou = bbox_overlaps_nearest_3d(
+ gt_bboxes_, pred_boxes)
+
+ # object_box_prob: P{a_{j} -> b_{i}}, shape: [i, j]
+ t1 = self.bbox_thr
+ t2 = object_box_iou.max(
+ dim=1, keepdim=True).values.clamp(min=t1 + 1e-6)
+ object_box_prob = ((object_box_iou - t1) / (t2 - t1)).clamp(
+ min=0, max=1)
+
+ # object_cls_box_prob: P{a_{j} -> b_{i}}, shape: [i, c, j]
+ num_obj = gt_labels_.size(0)
+ indices = torch.stack(
+ [torch.arange(num_obj).type_as(gt_labels_), gt_labels_],
+ dim=0)
+
+ object_cls_box_prob = torch.sparse_coo_tensor(
+ indices, object_box_prob)
+
+ # image_box_iou: P{a_{j} \in A_{+}}, shape: [c, j]
+ """
+ from "start" to "end" implement:
+ image_box_iou = torch.sparse.max(object_cls_box_prob,
+ dim=0).t()
+
+ """
+ # start
+ box_cls_prob = torch.sparse.sum(
+ object_cls_box_prob, dim=0).to_dense()
+
+ indices = torch.nonzero(box_cls_prob, as_tuple=False).t_()
+ if indices.numel() == 0:
+ image_box_prob = torch.zeros(
+ anchors_.size(0),
+ self.num_classes).type_as(object_box_prob)
+ else:
+ nonzero_box_prob = torch.where(
+ (gt_labels_.unsqueeze(dim=-1) == indices[0]),
+ object_box_prob[:, indices[1]],
+ torch.tensor(
+ [0]).type_as(object_box_prob)).max(dim=0).values
+
+ # upmap to shape [j, c]
+ image_box_prob = torch.sparse_coo_tensor(
+ indices.flip([0]),
+ nonzero_box_prob,
+ size=(anchors_.size(0), self.num_classes)).to_dense()
+ # end
+
+ box_prob.append(image_box_prob)
+
+ # construct bags for objects
+ match_quality_matrix = bbox_overlaps_nearest_3d(
+ gt_bboxes_, anchors_)
+ _, matched = torch.topk(
+ match_quality_matrix,
+ self.pre_anchor_topk,
+ dim=1,
+ sorted=False)
+ del match_quality_matrix
+
+ # matched_cls_prob: P_{ij}^{cls}
+ matched_cls_prob = torch.gather(
+ cls_prob_[matched], 2,
+ gt_labels_.view(-1, 1, 1).repeat(1, self.pre_anchor_topk,
+ 1)).squeeze(2)
+
+ # matched_box_prob: P_{ij}^{loc}
+ matched_anchors = anchors_[matched]
+ matched_object_targets = self.bbox_coder.encode(
+ matched_anchors,
+ gt_bboxes_.unsqueeze(dim=1).expand_as(matched_anchors))
+
+ # direction classification loss
+ loss_dir = None
+ if self.use_direction_classifier:
+ # also calculate direction prob: P_{ij}^{dir}
+ matched_dir_targets = get_direction_target(
+ matched_anchors,
+ matched_object_targets,
+ self.dir_offset,
+ self.dir_limit_offset,
+ one_hot=False)
+ loss_dir = self.loss_dir(
+ dir_cls_preds_[matched].transpose(-2, -1),
+ matched_dir_targets,
+ reduction_override='none')
+
+ # generate bbox weights
+ if self.diff_rad_by_sin:
+ bbox_preds_[matched], matched_object_targets = \
+ self.add_sin_difference(
+ bbox_preds_[matched], matched_object_targets)
+ bbox_weights = matched_anchors.new_ones(matched_anchors.size())
+ # Use pop is not right, check performance
+ code_weight = self.train_cfg.get('code_weight', None)
+ if code_weight:
+ bbox_weights = bbox_weights * bbox_weights.new_tensor(
+ code_weight)
+ loss_bbox = self.loss_bbox(
+ bbox_preds_[matched],
+ matched_object_targets,
+ bbox_weights,
+ reduction_override='none').sum(-1)
+
+ if loss_dir is not None:
+ loss_bbox += loss_dir
+ matched_box_prob = torch.exp(-loss_bbox)
+
+ # positive_losses: {-log( Mean-max(P_{ij}^{cls} * P_{ij}^{loc}) )}
+ num_pos += len(gt_bboxes_)
+ positive_losses.append(
+ self.positive_bag_loss(matched_cls_prob, matched_box_prob))
+
+ positive_loss = torch.cat(positive_losses).sum() / max(1, num_pos)
+
+ # box_prob: P{a_{j} \in A_{+}}
+ box_prob = torch.stack(box_prob, dim=0)
+
+ # negative_loss:
+ # \sum_{j}{ FL((1 - P{a_{j} \in A_{+}}) * (1 - P_{j}^{bg})) } / n||B||
+ negative_loss = self.negative_bag_loss(cls_prob, box_prob).sum() / max(
+ 1, num_pos * self.pre_anchor_topk)
+
+ losses = {
+ 'positive_bag_loss': positive_loss,
+ 'negative_bag_loss': negative_loss
+ }
+ return losses
+
+ def positive_bag_loss(self, matched_cls_prob, matched_box_prob):
+ """Generate positive bag loss.
+
+ Args:
+ matched_cls_prob (torch.Tensor): Classification probability
+ of matched positive samples.
+ matched_box_prob (torch.Tensor): Bounding box probability
+ of matched positive samples.
+
+ Returns:
+ torch.Tensor: Loss of positive samples.
+ """
+ # bag_prob = Mean-max(matched_prob)
+ matched_prob = matched_cls_prob * matched_box_prob
+ weight = 1 / torch.clamp(1 - matched_prob, 1e-12, None)
+ weight /= weight.sum(dim=1).unsqueeze(dim=-1)
+ bag_prob = (weight * matched_prob).sum(dim=1)
+ # positive_bag_loss = -self.alpha * log(bag_prob)
+ bag_prob = bag_prob.clamp(0, 1) # to avoid bug of BCE, check
+ return self.alpha * F.binary_cross_entropy(
+ bag_prob, torch.ones_like(bag_prob), reduction='none')
+
+ def negative_bag_loss(self, cls_prob, box_prob):
+ """Generate negative bag loss.
+
+ Args:
+ cls_prob (torch.Tensor): Classification probability
+ of negative samples.
+ box_prob (torch.Tensor): Bounding box probability
+ of negative samples.
+
+ Returns:
+ torch.Tensor: Loss of negative samples.
+ """
+ prob = cls_prob * (1 - box_prob)
+ prob = prob.clamp(0, 1) # to avoid bug of BCE, check
+ negative_bag_loss = prob**self.gamma * F.binary_cross_entropy(
+ prob, torch.zeros_like(prob), reduction='none')
+ return (1 - self.alpha) * negative_bag_loss
diff --git a/mmdet3d/models/dense_heads/groupfree3d_head.py b/mmdet3d/models/dense_heads/groupfree3d_head.py
new file mode 100644
index 0000000..b76cb05
--- /dev/null
+++ b/mmdet3d/models/dense_heads/groupfree3d_head.py
@@ -0,0 +1,994 @@
+# Copyright (c) OpenMMLab. All rights reserved.
+import copy
+
+import numpy as np
+import torch
+from mmcv import ConfigDict
+from mmcv.cnn import ConvModule, xavier_init
+from mmcv.cnn.bricks.transformer import (build_positional_encoding,
+ build_transformer_layer)
+from mmcv.ops import PointsSampler as Points_Sampler
+from mmcv.ops import gather_points
+from mmcv.runner import BaseModule, force_fp32
+from torch import nn as nn
+from torch.nn import functional as F
+
+from mmdet3d.core.post_processing import aligned_3d_nms
+from mmdet.core import build_bbox_coder, multi_apply
+from ..builder import HEADS, build_loss
+from .base_conv_bbox_head import BaseConvBboxHead
+
+EPS = 1e-6
+
+
+class PointsObjClsModule(BaseModule):
+ """object candidate point prediction from seed point features.
+
+ Args:
+ in_channel (int): number of channels of seed point features.
+ num_convs (int, optional): number of conv layers.
+ Default: 3.
+ conv_cfg (dict, optional): Config of convolution.
+ Default: dict(type='Conv1d').
+ norm_cfg (dict, optional): Config of normalization.
+ Default: dict(type='BN1d').
+ act_cfg (dict, optional): Config of activation.
+ Default: dict(type='ReLU').
+ """
+
+ def __init__(self,
+ in_channel,
+ num_convs=3,
+ conv_cfg=dict(type='Conv1d'),
+ norm_cfg=dict(type='BN1d'),
+ act_cfg=dict(type='ReLU'),
+ init_cfg=None):
+ super().__init__(init_cfg=init_cfg)
+ conv_channels = [in_channel for _ in range(num_convs - 1)]
+ conv_channels.append(1)
+
+ self.mlp = nn.Sequential()
+ prev_channels = in_channel
+ for i in range(num_convs):
+ self.mlp.add_module(
+ f'layer{i}',
+ ConvModule(
+ prev_channels,
+ conv_channels[i],
+ 1,
+ padding=0,
+ conv_cfg=conv_cfg,
+ norm_cfg=norm_cfg if i < num_convs - 1 else None,
+ act_cfg=act_cfg if i < num_convs - 1 else None,
+ bias=True,
+ inplace=True))
+ prev_channels = conv_channels[i]
+
+ def forward(self, seed_features):
+ """Forward pass.
+
+ Args:
+ seed_features (torch.Tensor): seed features, dims:
+ (batch_size, feature_dim, num_seed)
+
+ Returns:
+ torch.Tensor: objectness logits, dim:
+ (batch_size, 1, num_seed)
+ """
+ return self.mlp(seed_features)
+
+
+class GeneralSamplingModule(nn.Module):
+ """Sampling Points.
+
+ Sampling points with given index.
+ """
+
+ def forward(self, xyz, features, sample_inds):
+ """Forward pass.
+
+ Args:
+ xyz: (B, N, 3) the coordinates of the features.
+ features (Tensor): (B, C, N) features to sample.
+ sample_inds (Tensor): (B, M) the given index,
+ where M is the number of points.
+
+ Returns:
+ Tensor: (B, M, 3) coordinates of sampled features
+ Tensor: (B, C, M) the sampled features.
+ Tensor: (B, M) the given index.
+ """
+ xyz_t = xyz.transpose(1, 2).contiguous()
+ new_xyz = gather_points(xyz_t, sample_inds).transpose(1,
+ 2).contiguous()
+ new_features = gather_points(features, sample_inds).contiguous()
+
+ return new_xyz, new_features, sample_inds
+
+
+@HEADS.register_module()
+class GroupFree3DHead(BaseModule):
+ r"""Bbox head of `Group-Free 3D `_.
+
+ Args:
+ num_classes (int): The number of class.
+ in_channels (int): The dims of input features from backbone.
+ bbox_coder (:obj:`BaseBBoxCoder`): Bbox coder for encoding and
+ decoding boxes.
+ num_decoder_layers (int): The number of transformer decoder layers.
+ transformerlayers (dict): Config for transformer decoder.
+ train_cfg (dict): Config for training.
+ test_cfg (dict): Config for testing.
+ num_proposal (int): The number of initial sampling candidates.
+ pred_layer_cfg (dict): Config of classfication and regression
+ prediction layers.
+ size_cls_agnostic (bool): Whether the predicted size is class-agnostic.
+ gt_per_seed (int): the number of candidate instance each point belongs
+ to.
+ sampling_objectness_loss (dict): Config of initial sampling
+ objectness loss.
+ objectness_loss (dict): Config of objectness loss.
+ center_loss (dict): Config of center loss.
+ dir_class_loss (dict): Config of direction classification loss.
+ dir_res_loss (dict): Config of direction residual regression loss.
+ size_class_loss (dict): Config of size classification loss.
+ size_res_loss (dict): Config of size residual regression loss.
+ size_reg_loss (dict): Config of class-agnostic size regression loss.
+ semantic_loss (dict): Config of point-wise semantic segmentation loss.
+ """
+
+ def __init__(self,
+ num_classes,
+ in_channels,
+ bbox_coder,
+ num_decoder_layers,
+ transformerlayers,
+ decoder_self_posembeds=dict(
+ type='ConvBNPositionalEncoding',
+ input_channel=6,
+ num_pos_feats=288),
+ decoder_cross_posembeds=dict(
+ type='ConvBNPositionalEncoding',
+ input_channel=3,
+ num_pos_feats=288),
+ train_cfg=None,
+ test_cfg=None,
+ num_proposal=128,
+ pred_layer_cfg=None,
+ size_cls_agnostic=True,
+ gt_per_seed=3,
+ sampling_objectness_loss=None,
+ objectness_loss=None,
+ center_loss=None,
+ dir_class_loss=None,
+ dir_res_loss=None,
+ size_class_loss=None,
+ size_res_loss=None,
+ size_reg_loss=None,
+ semantic_loss=None,
+ init_cfg=None):
+ super(GroupFree3DHead, self).__init__(init_cfg=init_cfg)
+ self.num_classes = num_classes
+ self.train_cfg = train_cfg
+ self.test_cfg = test_cfg
+ self.num_proposal = num_proposal
+ self.in_channels = in_channels
+ self.num_decoder_layers = num_decoder_layers
+ self.size_cls_agnostic = size_cls_agnostic
+ self.gt_per_seed = gt_per_seed
+
+ # Transformer decoder layers
+ if isinstance(transformerlayers, ConfigDict):
+ transformerlayers = [
+ copy.deepcopy(transformerlayers)
+ for _ in range(num_decoder_layers)
+ ]
+ else:
+ assert isinstance(transformerlayers, list) and \
+ len(transformerlayers) == num_decoder_layers
+ self.decoder_layers = nn.ModuleList()
+ for i in range(self.num_decoder_layers):
+ self.decoder_layers.append(
+ build_transformer_layer(transformerlayers[i]))
+ self.embed_dims = self.decoder_layers[0].embed_dims
+ assert self.embed_dims == decoder_self_posembeds['num_pos_feats']
+ assert self.embed_dims == decoder_cross_posembeds['num_pos_feats']
+
+ # bbox_coder
+ self.bbox_coder = build_bbox_coder(bbox_coder)
+ self.num_sizes = self.bbox_coder.num_sizes
+ self.num_dir_bins = self.bbox_coder.num_dir_bins
+
+ # Initial object candidate sampling
+ self.gsample_module = GeneralSamplingModule()
+ self.fps_module = Points_Sampler([self.num_proposal])
+ self.points_obj_cls = PointsObjClsModule(self.in_channels)
+
+ self.fp16_enabled = False
+
+ # initial candidate prediction
+ self.conv_pred = BaseConvBboxHead(
+ **pred_layer_cfg,
+ num_cls_out_channels=self._get_cls_out_channels(),
+ num_reg_out_channels=self._get_reg_out_channels())
+
+ # query proj and key proj
+ self.decoder_query_proj = nn.Conv1d(
+ self.embed_dims, self.embed_dims, kernel_size=1)
+ self.decoder_key_proj = nn.Conv1d(
+ self.embed_dims, self.embed_dims, kernel_size=1)
+
+ # query position embed
+ self.decoder_self_posembeds = nn.ModuleList()
+ for _ in range(self.num_decoder_layers):
+ self.decoder_self_posembeds.append(
+ build_positional_encoding(decoder_self_posembeds))
+ # key position embed
+ self.decoder_cross_posembeds = nn.ModuleList()
+ for _ in range(self.num_decoder_layers):
+ self.decoder_cross_posembeds.append(
+ build_positional_encoding(decoder_cross_posembeds))
+
+ # Prediction Head
+ self.prediction_heads = nn.ModuleList()
+ for i in range(self.num_decoder_layers):
+ self.prediction_heads.append(
+ BaseConvBboxHead(
+ **pred_layer_cfg,
+ num_cls_out_channels=self._get_cls_out_channels(),
+ num_reg_out_channels=self._get_reg_out_channels()))
+
+ self.sampling_objectness_loss = build_loss(sampling_objectness_loss)
+ self.objectness_loss = build_loss(objectness_loss)
+ self.center_loss = build_loss(center_loss)
+ self.dir_res_loss = build_loss(dir_res_loss)
+ self.dir_class_loss = build_loss(dir_class_loss)
+ self.semantic_loss = build_loss(semantic_loss)
+ if self.size_cls_agnostic:
+ self.size_reg_loss = build_loss(size_reg_loss)
+ else:
+ self.size_res_loss = build_loss(size_res_loss)
+ self.size_class_loss = build_loss(size_class_loss)
+
+ def init_weights(self):
+ """Initialize weights of transformer decoder in GroupFree3DHead."""
+ # initialize transformer
+ for m in self.decoder_layers.parameters():
+ if m.dim() > 1:
+ xavier_init(m, distribution='uniform')
+ for m in self.decoder_self_posembeds.parameters():
+ if m.dim() > 1:
+ xavier_init(m, distribution='uniform')
+ for m in self.decoder_cross_posembeds.parameters():
+ if m.dim() > 1:
+ xavier_init(m, distribution='uniform')
+
+ def _get_cls_out_channels(self):
+ """Return the channel number of classification outputs."""
+ # Class numbers (k) + objectness (1)
+ return self.num_classes + 1
+
+ def _get_reg_out_channels(self):
+ """Return the channel number of regression outputs."""
+ # center residual (3),
+ # heading class+residual (num_dir_bins*2),
+ # size class+residual(num_sizes*4 or 3)
+ if self.size_cls_agnostic:
+ return 6 + self.num_dir_bins * 2
+ else:
+ return 3 + self.num_dir_bins * 2 + self.num_sizes * 4
+
+ def _extract_input(self, feat_dict):
+ """Extract inputs from features dictionary.
+
+ Args:
+ feat_dict (dict): Feature dict from backbone.
+
+ Returns:
+ torch.Tensor: Coordinates of input points.
+ torch.Tensor: Features of input points.
+ torch.Tensor: Indices of input points.
+ """
+
+ seed_points = feat_dict['fp_xyz'][-1]
+ seed_features = feat_dict['fp_features'][-1]
+ seed_indices = feat_dict['fp_indices'][-1]
+
+ return seed_points, seed_features, seed_indices
+
+ def forward(self, feat_dict, sample_mod):
+ """Forward pass.
+
+ Note:
+ The forward of GroupFree3DHead is divided into 2 steps:
+
+ 1. Initial object candidates sampling.
+ 2. Iterative object box prediction by transformer decoder.
+
+ Args:
+ feat_dict (dict): Feature dict from backbone.
+ sample_mod (str): sample mode for initial candidates sampling.
+
+ Returns:
+ results (dict): Predictions of GroupFree3D head.
+ """
+ assert sample_mod in ['fps', 'kps']
+
+ seed_xyz, seed_features, seed_indices = self._extract_input(feat_dict)
+
+ results = dict(
+ seed_points=seed_xyz,
+ seed_features=seed_features,
+ seed_indices=seed_indices)
+
+ # 1. Initial object candidates sampling.
+ if sample_mod == 'fps':
+ sample_inds = self.fps_module(seed_xyz, seed_features)
+ elif sample_mod == 'kps':
+ points_obj_cls_logits = self.points_obj_cls(
+ seed_features) # (batch_size, 1, num_seed)
+ points_obj_cls_scores = points_obj_cls_logits.sigmoid().squeeze(1)
+ sample_inds = torch.topk(points_obj_cls_scores,
+ self.num_proposal)[1].int()
+ results['seeds_obj_cls_logits'] = points_obj_cls_logits
+ else:
+ raise NotImplementedError(
+ f'Sample mode {sample_mod} is not supported!')
+
+ candidate_xyz, candidate_features, sample_inds = self.gsample_module(
+ seed_xyz, seed_features, sample_inds)
+
+ results['query_points_xyz'] = candidate_xyz # (B, M, 3)
+ results['query_points_feature'] = candidate_features # (B, C, M)
+ results['query_points_sample_inds'] = sample_inds.long() # (B, M)
+
+ prefix = 'proposal.'
+ cls_predictions, reg_predictions = self.conv_pred(candidate_features)
+ decode_res = self.bbox_coder.split_pred(cls_predictions,
+ reg_predictions, candidate_xyz,
+ prefix)
+
+ results.update(decode_res)
+ bbox3d = self.bbox_coder.decode(results, prefix)
+
+ # 2. Iterative object box prediction by transformer decoder.
+ base_bbox3d = bbox3d[:, :, :6].detach().clone()
+
+ query = self.decoder_query_proj(candidate_features).permute(2, 0, 1)
+ key = self.decoder_key_proj(seed_features).permute(2, 0, 1)
+ value = key
+
+ # transformer decoder
+ results['num_decoder_layers'] = 0
+ for i in range(self.num_decoder_layers):
+ prefix = f's{i}.'
+
+ query_pos = self.decoder_self_posembeds[i](base_bbox3d).permute(
+ 2, 0, 1)
+ key_pos = self.decoder_cross_posembeds[i](seed_xyz).permute(
+ 2, 0, 1)
+
+ query = self.decoder_layers[i](
+ query, key, value, query_pos=query_pos,
+ key_pos=key_pos).permute(1, 2, 0)
+
+ results[f'{prefix}query'] = query
+
+ cls_predictions, reg_predictions = self.prediction_heads[i](query)
+ decode_res = self.bbox_coder.split_pred(cls_predictions,
+ reg_predictions,
+ candidate_xyz, prefix)
+ # TODO: should save bbox3d instead of decode_res?
+ results.update(decode_res)
+
+ bbox3d = self.bbox_coder.decode(results, prefix)
+ results[f'{prefix}bbox3d'] = bbox3d
+ base_bbox3d = bbox3d[:, :, :6].detach().clone()
+ query = query.permute(2, 0, 1)
+
+ results['num_decoder_layers'] += 1
+
+ return results
+
+ @force_fp32(apply_to=('bbox_preds', ))
+ def loss(self,
+ bbox_preds,
+ points,
+ gt_bboxes_3d,
+ gt_labels_3d,
+ pts_semantic_mask=None,
+ pts_instance_mask=None,
+ img_metas=None,
+ gt_bboxes_ignore=None,
+ ret_target=False):
+ """Compute loss.
+
+ Args:
+ bbox_preds (dict): Predictions from forward of vote head.
+ points (list[torch.Tensor]): Input points.
+ gt_bboxes_3d (list[:obj:`BaseInstance3DBoxes`]): Ground truth
+ bboxes of each sample.
+ gt_labels_3d (list[torch.Tensor]): Labels of each sample.
+ pts_semantic_mask (list[torch.Tensor]): Point-wise
+ semantic mask.
+ pts_instance_mask (list[torch.Tensor]): Point-wise
+ instance mask.
+ img_metas (list[dict]): Contain pcd and img's meta info.
+ gt_bboxes_ignore (list[torch.Tensor]): Specify
+ which bounding.
+ ret_target (Bool): Return targets or not.
+
+ Returns:
+ dict: Losses of GroupFree3D.
+ """
+ targets = self.get_targets(points, gt_bboxes_3d, gt_labels_3d,
+ pts_semantic_mask, pts_instance_mask,
+ bbox_preds)
+ (sampling_targets, sampling_weights, assigned_size_targets,
+ size_class_targets, size_res_targets, dir_class_targets,
+ dir_res_targets, center_targets, assigned_center_targets,
+ mask_targets, valid_gt_masks, objectness_targets, objectness_weights,
+ box_loss_weights, valid_gt_weights) = targets
+
+ batch_size, proposal_num = size_class_targets.shape[:2]
+
+ losses = dict()
+
+ # calculate objectness classification loss
+ sampling_obj_score = bbox_preds['seeds_obj_cls_logits'].reshape(-1, 1)
+ sampling_objectness_loss = self.sampling_objectness_loss(
+ sampling_obj_score,
+ 1 - sampling_targets.reshape(-1),
+ sampling_weights.reshape(-1),
+ avg_factor=batch_size)
+ losses['sampling_objectness_loss'] = sampling_objectness_loss
+
+ prefixes = ['proposal.'] + [
+ f's{i}.' for i in range(bbox_preds['num_decoder_layers'])
+ ]
+ num_stages = len(prefixes)
+ for prefix in prefixes:
+
+ # calculate objectness loss
+ obj_score = bbox_preds[f'{prefix}obj_scores'].transpose(2, 1)
+ objectness_loss = self.objectness_loss(
+ obj_score.reshape(-1, 1),
+ 1 - objectness_targets.reshape(-1),
+ objectness_weights.reshape(-1),
+ avg_factor=batch_size)
+ losses[f'{prefix}objectness_loss'] = objectness_loss / num_stages
+
+ # calculate center loss
+ box_loss_weights_expand = box_loss_weights.unsqueeze(-1).expand(
+ -1, -1, 3)
+ center_loss = self.center_loss(
+ bbox_preds[f'{prefix}center'],
+ assigned_center_targets,
+ weight=box_loss_weights_expand)
+ losses[f'{prefix}center_loss'] = center_loss / num_stages
+
+ # calculate direction class loss
+ dir_class_loss = self.dir_class_loss(
+ bbox_preds[f'{prefix}dir_class'].transpose(2, 1),
+ dir_class_targets,
+ weight=box_loss_weights)
+ losses[f'{prefix}dir_class_loss'] = dir_class_loss / num_stages
+
+ # calculate direction residual loss
+ heading_label_one_hot = size_class_targets.new_zeros(
+ (batch_size, proposal_num, self.num_dir_bins))
+ heading_label_one_hot.scatter_(2, dir_class_targets.unsqueeze(-1),
+ 1)
+ dir_res_norm = torch.sum(
+ bbox_preds[f'{prefix}dir_res_norm'] * heading_label_one_hot,
+ -1)
+ dir_res_loss = self.dir_res_loss(
+ dir_res_norm, dir_res_targets, weight=box_loss_weights)
+ losses[f'{prefix}dir_res_loss'] = dir_res_loss / num_stages
+
+ if self.size_cls_agnostic:
+ # calculate class-agnostic size loss
+ size_reg_loss = self.size_reg_loss(
+ bbox_preds[f'{prefix}size'],
+ assigned_size_targets,
+ weight=box_loss_weights_expand)
+ losses[f'{prefix}size_reg_loss'] = size_reg_loss / num_stages
+
+ else:
+ # calculate size class loss
+ size_class_loss = self.size_class_loss(
+ bbox_preds[f'{prefix}size_class'].transpose(2, 1),
+ size_class_targets,
+ weight=box_loss_weights)
+ losses[
+ f'{prefix}size_class_loss'] = size_class_loss / num_stages
+
+ # calculate size residual loss
+ one_hot_size_targets = size_class_targets.new_zeros(
+ (batch_size, proposal_num, self.num_sizes))
+ one_hot_size_targets.scatter_(2,
+ size_class_targets.unsqueeze(-1),
+ 1)
+ one_hot_size_targets_expand = one_hot_size_targets.unsqueeze(
+ -1).expand(-1, -1, -1, 3).contiguous()
+ size_residual_norm = torch.sum(
+ bbox_preds[f'{prefix}size_res_norm'] *
+ one_hot_size_targets_expand, 2)
+ box_loss_weights_expand = box_loss_weights.unsqueeze(
+ -1).expand(-1, -1, 3)
+ size_res_loss = self.size_res_loss(
+ size_residual_norm,
+ size_res_targets,
+ weight=box_loss_weights_expand)
+ losses[f'{prefix}size_res_loss'] = size_res_loss / num_stages
+
+ # calculate semantic loss
+ semantic_loss = self.semantic_loss(
+ bbox_preds[f'{prefix}sem_scores'].transpose(2, 1),
+ mask_targets,
+ weight=box_loss_weights)
+ losses[f'{prefix}semantic_loss'] = semantic_loss / num_stages
+
+ if ret_target:
+ losses['targets'] = targets
+
+ return losses
+
+ def get_targets(self,
+ points,
+ gt_bboxes_3d,
+ gt_labels_3d,
+ pts_semantic_mask=None,
+ pts_instance_mask=None,
+ bbox_preds=None,
+ max_gt_num=64):
+ """Generate targets of GroupFree3D head.
+
+ Args:
+ points (list[torch.Tensor]): Points of each batch.
+ gt_bboxes_3d (list[:obj:`BaseInstance3DBoxes`]): Ground truth
+ bboxes of each batch.
+ gt_labels_3d (list[torch.Tensor]): Labels of each batch.
+ pts_semantic_mask (list[torch.Tensor]): Point-wise semantic
+ label of each batch.
+ pts_instance_mask (list[torch.Tensor]): Point-wise instance
+ label of each batch.
+ bbox_preds (torch.Tensor): Bounding box predictions of vote head.
+ max_gt_num (int): Max number of GTs for single batch.
+
+ Returns:
+ tuple[torch.Tensor]: Targets of GroupFree3D head.
+ """
+ # find empty example
+ valid_gt_masks = list()
+ gt_num = list()
+ for index in range(len(gt_labels_3d)):
+ if len(gt_labels_3d[index]) == 0:
+ fake_box = gt_bboxes_3d[index].tensor.new_zeros(
+ 1, gt_bboxes_3d[index].tensor.shape[-1])
+ gt_bboxes_3d[index] = gt_bboxes_3d[index].new_box(fake_box)
+ gt_labels_3d[index] = gt_labels_3d[index].new_zeros(1)
+ valid_gt_masks.append(gt_labels_3d[index].new_zeros(1))
+ gt_num.append(1)
+ else:
+ valid_gt_masks.append(gt_labels_3d[index].new_ones(
+ gt_labels_3d[index].shape))
+ gt_num.append(gt_labels_3d[index].shape[0])
+ # max_gt_num = max(gt_num)
+
+ max_gt_nums = [max_gt_num for _ in range(len(gt_labels_3d))]
+
+ if pts_semantic_mask is None:
+ pts_semantic_mask = [None for i in range(len(gt_labels_3d))]
+ pts_instance_mask = [None for i in range(len(gt_labels_3d))]
+
+ seed_points = [
+ bbox_preds['seed_points'][i] for i in range(len(gt_labels_3d))
+ ]
+
+ seed_indices = [
+ bbox_preds['seed_indices'][i] for i in range(len(gt_labels_3d))
+ ]
+
+ candidate_indices = [
+ bbox_preds['query_points_sample_inds'][i]
+ for i in range(len(gt_labels_3d))
+ ]
+
+ (sampling_targets, assigned_size_targets, size_class_targets,
+ size_res_targets, dir_class_targets, dir_res_targets, center_targets,
+ assigned_center_targets, mask_targets, objectness_targets,
+ objectness_masks) = multi_apply(self.get_targets_single, points,
+ gt_bboxes_3d, gt_labels_3d,
+ pts_semantic_mask, pts_instance_mask,
+ max_gt_nums, seed_points,
+ seed_indices, candidate_indices)
+
+ # pad targets as original code of GroupFree3D.
+ for index in range(len(gt_labels_3d)):
+ pad_num = max_gt_num - gt_labels_3d[index].shape[0]
+ valid_gt_masks[index] = F.pad(valid_gt_masks[index], (0, pad_num))
+
+ sampling_targets = torch.stack(sampling_targets)
+ sampling_weights = (sampling_targets >= 0).float()
+ sampling_normalizer = sampling_weights.sum(dim=1, keepdim=True).float()
+ sampling_weights /= sampling_normalizer.clamp(min=1.0)
+
+ assigned_size_targets = torch.stack(assigned_size_targets)
+ center_targets = torch.stack(center_targets)
+ valid_gt_masks = torch.stack(valid_gt_masks)
+
+ assigned_center_targets = torch.stack(assigned_center_targets)
+ objectness_targets = torch.stack(objectness_targets)
+
+ objectness_weights = torch.stack(objectness_masks)
+ cls_normalizer = objectness_weights.sum(dim=1, keepdim=True).float()
+ objectness_weights /= cls_normalizer.clamp(min=1.0)
+
+ box_loss_weights = objectness_targets.float() / (
+ objectness_targets.sum().float() + EPS)
+
+ valid_gt_weights = valid_gt_masks.float() / (
+ valid_gt_masks.sum().float() + EPS)
+
+ dir_class_targets = torch.stack(dir_class_targets)
+ dir_res_targets = torch.stack(dir_res_targets)
+ size_class_targets = torch.stack(size_class_targets)
+ size_res_targets = torch.stack(size_res_targets)
+ mask_targets = torch.stack(mask_targets)
+
+ return (sampling_targets, sampling_weights, assigned_size_targets,
+ size_class_targets, size_res_targets, dir_class_targets,
+ dir_res_targets, center_targets, assigned_center_targets,
+ mask_targets, valid_gt_masks, objectness_targets,
+ objectness_weights, box_loss_weights, valid_gt_weights)
+
+ def get_targets_single(self,
+ points,
+ gt_bboxes_3d,
+ gt_labels_3d,
+ pts_semantic_mask=None,
+ pts_instance_mask=None,
+ max_gt_nums=None,
+ seed_points=None,
+ seed_indices=None,
+ candidate_indices=None,
+ seed_points_obj_topk=4):
+ """Generate targets of GroupFree3D head for single batch.
+
+ Args:
+ points (torch.Tensor): Points of each batch.
+ gt_bboxes_3d (:obj:`BaseInstance3DBoxes`): Ground truth
+ boxes of each batch.
+ gt_labels_3d (torch.Tensor): Labels of each batch.
+ pts_semantic_mask (torch.Tensor): Point-wise semantic
+ label of each batch.
+ pts_instance_mask (torch.Tensor): Point-wise instance
+ label of each batch.
+ max_gt_nums (int): Max number of GTs for single batch.
+ seed_points (torch.Tensor): Coordinates of seed points.
+ seed_indices (torch.Tensor): Indices of seed points.
+ candidate_indices (torch.Tensor): Indices of object candidates.
+ seed_points_obj_topk (int): k value of k-Closest Points Sampling.
+
+ Returns:
+ tuple[torch.Tensor]: Targets of GroupFree3D head.
+ """
+
+ assert self.bbox_coder.with_rot or pts_semantic_mask is not None
+
+ gt_bboxes_3d = gt_bboxes_3d.to(points.device)
+
+ # generate center, dir, size target
+ (center_targets, size_targets, size_class_targets, size_res_targets,
+ dir_class_targets,
+ dir_res_targets) = self.bbox_coder.encode(gt_bboxes_3d, gt_labels_3d)
+
+ # pad targets as original code of GroupFree3D
+ pad_num = max_gt_nums - gt_labels_3d.shape[0]
+ box_label_mask = points.new_zeros([max_gt_nums])
+ box_label_mask[:gt_labels_3d.shape[0]] = 1
+
+ gt_bboxes_pad = F.pad(gt_bboxes_3d.tensor, (0, 0, 0, pad_num))
+ gt_bboxes_pad[gt_labels_3d.shape[0]:, 0:3] += 1000
+ gt_bboxes_3d = gt_bboxes_3d.new_box(gt_bboxes_pad)
+
+ gt_labels_3d = F.pad(gt_labels_3d, (0, pad_num))
+
+ center_targets = F.pad(center_targets, (0, 0, 0, pad_num), value=1000)
+ size_targets = F.pad(size_targets, (0, 0, 0, pad_num))
+ size_class_targets = F.pad(size_class_targets, (0, pad_num))
+ size_res_targets = F.pad(size_res_targets, (0, 0, 0, pad_num))
+ dir_class_targets = F.pad(dir_class_targets, (0, pad_num))
+ dir_res_targets = F.pad(dir_res_targets, (0, pad_num))
+
+ # 0. generate pts_instance_label and pts_obj_mask
+ num_points = points.shape[0]
+ pts_obj_mask = points.new_zeros([num_points], dtype=torch.long)
+ pts_instance_label = points.new_zeros([num_points],
+ dtype=torch.long) - 1
+
+ if self.bbox_coder.with_rot:
+ vote_targets = points.new_zeros([num_points, 4 * self.gt_per_seed])
+ vote_target_idx = points.new_zeros([num_points], dtype=torch.long)
+ box_indices_all = gt_bboxes_3d.points_in_boxes_part(points)
+ for i in range(gt_labels_3d.shape[0]):
+ box_indices = box_indices_all[:, i]
+ indices = torch.nonzero(
+ box_indices, as_tuple=False).squeeze(-1)
+ selected_points = points[indices]
+ pts_obj_mask[indices] = 1
+ vote_targets_tmp = vote_targets[indices]
+ votes = gt_bboxes_3d.gravity_center[i].unsqueeze(
+ 0) - selected_points[:, :3]
+
+ for j in range(self.gt_per_seed):
+ column_indices = torch.nonzero(
+ vote_target_idx[indices] == j,
+ as_tuple=False).squeeze(-1)
+ vote_targets_tmp[column_indices,
+ int(j * 3):int(j * 3 +
+ 3)] = votes[column_indices]
+ vote_targets_tmp[column_indices,
+ j + 3 * self.gt_per_seed] = i
+ if j == 0:
+ vote_targets_tmp[
+ column_indices, :3 *
+ self.gt_per_seed] = votes[column_indices].repeat(
+ 1, self.gt_per_seed)
+ vote_targets_tmp[column_indices,
+ 3 * self.gt_per_seed:] = i
+
+ vote_targets[indices] = vote_targets_tmp
+ vote_target_idx[indices] = torch.clamp(
+ vote_target_idx[indices] + 1, max=2)
+
+ dist = points.new_zeros([num_points, self.gt_per_seed]) + 1000
+ for j in range(self.gt_per_seed):
+ dist[:, j] = (vote_targets[:, 3 * j:3 * j + 3]**2).sum(-1)
+
+ instance_indices = torch.argmin(
+ dist, dim=-1).unsqueeze(-1) + 3 * self.gt_per_seed
+ instance_lable = torch.gather(vote_targets, 1,
+ instance_indices).squeeze(-1)
+ pts_instance_label = instance_lable.long()
+ pts_instance_label[pts_obj_mask == 0] = -1
+
+ elif pts_semantic_mask is not None:
+ for i in torch.unique(pts_instance_mask):
+ indices = torch.nonzero(
+ pts_instance_mask == i, as_tuple=False).squeeze(-1)
+
+ if pts_semantic_mask[indices[0]] < self.num_classes:
+ selected_points = points[indices, :3]
+ center = 0.5 * (
+ selected_points.min(0)[0] + selected_points.max(0)[0])
+
+ delta_xyz = center - center_targets
+ instance_lable = torch.argmin((delta_xyz**2).sum(-1))
+ pts_instance_label[indices] = instance_lable
+ pts_obj_mask[indices] = 1
+
+ else:
+ raise NotImplementedError
+
+ # 1. generate objectness targets in sampling head
+ gt_num = gt_labels_3d.shape[0]
+ num_seed = seed_points.shape[0]
+ num_candidate = candidate_indices.shape[0]
+
+ object_assignment = torch.gather(pts_instance_label, 0, seed_indices)
+ # set background points to the last gt bbox as original code
+ object_assignment[object_assignment < 0] = gt_num - 1
+ object_assignment_one_hot = gt_bboxes_3d.tensor.new_zeros(
+ (num_seed, gt_num))
+ object_assignment_one_hot.scatter_(1, object_assignment.unsqueeze(-1),
+ 1) # (num_seed, gt_num)
+
+ delta_xyz = seed_points.unsqueeze(
+ 1) - gt_bboxes_3d.gravity_center.unsqueeze(
+ 0) # (num_seed, gt_num, 3)
+ delta_xyz = delta_xyz / (gt_bboxes_3d.dims.unsqueeze(0) + EPS)
+
+ new_dist = torch.sum(delta_xyz**2, dim=-1)
+ euclidean_dist1 = torch.sqrt(new_dist + EPS)
+ euclidean_dist1 = euclidean_dist1 * object_assignment_one_hot + 100 * (
+ 1 - object_assignment_one_hot)
+ # (gt_num, num_seed)
+ euclidean_dist1 = euclidean_dist1.permute(1, 0)
+
+ # gt_num x topk
+ topk_inds = torch.topk(
+ euclidean_dist1,
+ seed_points_obj_topk,
+ largest=False)[1] * box_label_mask[:, None] + \
+ (box_label_mask[:, None] - 1)
+ topk_inds = topk_inds.long()
+ topk_inds = topk_inds.view(-1).contiguous()
+
+ sampling_targets = torch.zeros(
+ num_seed + 1, dtype=torch.long).to(points.device)
+ sampling_targets[topk_inds] = 1
+ sampling_targets = sampling_targets[:num_seed]
+ # pts_instance_label
+ objectness_label_mask = torch.gather(pts_instance_label, 0,
+ seed_indices) # num_seed
+ sampling_targets[objectness_label_mask < 0] = 0
+
+ # 2. objectness target
+ seed_obj_gt = torch.gather(pts_obj_mask, 0, seed_indices) # num_seed
+ objectness_targets = torch.gather(seed_obj_gt, 0,
+ candidate_indices) # num_candidate
+
+ # 3. box target
+ seed_instance_label = torch.gather(pts_instance_label, 0,
+ seed_indices) # num_seed
+ query_points_instance_label = torch.gather(
+ seed_instance_label, 0, candidate_indices) # num_candidate
+
+ # Set assignment
+ # (num_candidate, ) with values in 0,1,...,gt_num-1
+ assignment = query_points_instance_label
+ # set background points to the last gt bbox as original code
+ assignment[assignment < 0] = gt_num - 1
+ assignment_expand = assignment.unsqueeze(1).expand(-1, 3)
+
+ assigned_center_targets = center_targets[assignment]
+ assigned_size_targets = size_targets[assignment]
+
+ dir_class_targets = dir_class_targets[assignment]
+ dir_res_targets = dir_res_targets[assignment]
+ dir_res_targets /= (np.pi / self.num_dir_bins)
+
+ size_class_targets = size_class_targets[assignment]
+ size_res_targets = \
+ torch.gather(size_res_targets, 0, assignment_expand)
+ one_hot_size_targets = gt_bboxes_3d.tensor.new_zeros(
+ (num_candidate, self.num_sizes))
+ one_hot_size_targets.scatter_(1, size_class_targets.unsqueeze(-1), 1)
+ one_hot_size_targets = one_hot_size_targets.unsqueeze(-1).expand(
+ -1, -1, 3) # (num_candidate,num_size_cluster,3)
+ mean_sizes = size_res_targets.new_tensor(
+ self.bbox_coder.mean_sizes).unsqueeze(0)
+ pos_mean_sizes = torch.sum(one_hot_size_targets * mean_sizes, 1)
+ size_res_targets /= pos_mean_sizes
+
+ mask_targets = gt_labels_3d[assignment].long()
+
+ objectness_masks = points.new_ones((num_candidate))
+
+ return (sampling_targets, assigned_size_targets, size_class_targets,
+ size_res_targets, dir_class_targets, dir_res_targets,
+ center_targets, assigned_center_targets, mask_targets,
+ objectness_targets, objectness_masks)
+
+ def get_bboxes(self,
+ points,
+ bbox_preds,
+ input_metas,
+ rescale=False,
+ use_nms=True):
+ """Generate bboxes from GroupFree3D head predictions.
+
+ Args:
+ points (torch.Tensor): Input points.
+ bbox_preds (dict): Predictions from GroupFree3D head.
+ input_metas (list[dict]): Point cloud and image's meta info.
+ rescale (bool): Whether to rescale bboxes.
+ use_nms (bool): Whether to apply NMS, skip nms postprocessing
+ while using GroupFree3D head in rpn stage.
+
+ Returns:
+ list[tuple[torch.Tensor]]: Bounding boxes, scores and labels.
+ """
+ # support multi-stage predictions
+ assert self.test_cfg['prediction_stages'] in \
+ ['last', 'all', 'last_three']
+
+ prefixes = list()
+ if self.test_cfg['prediction_stages'] == 'last':
+ prefixes = [f's{self.num_decoder_layers - 1}.']
+ elif self.test_cfg['prediction_stages'] == 'all':
+ prefixes = ['proposal.'] + \
+ [f's{i}.' for i in range(self.num_decoder_layers)]
+ elif self.test_cfg['prediction_stages'] == 'last_three':
+ prefixes = [
+ f's{i}.' for i in range(self.num_decoder_layers -
+ 3, self.num_decoder_layers)
+ ]
+ else:
+ raise NotImplementedError
+
+ obj_scores = list()
+ sem_scores = list()
+ bbox3d = list()
+ for prefix in prefixes:
+ # decode boxes
+ obj_score = bbox_preds[f'{prefix}obj_scores'][..., -1].sigmoid()
+ sem_score = bbox_preds[f'{prefix}sem_scores'].softmax(-1)
+ bbox = self.bbox_coder.decode(bbox_preds, prefix)
+ obj_scores.append(obj_score)
+ sem_scores.append(sem_score)
+ bbox3d.append(bbox)
+
+ obj_scores = torch.cat(obj_scores, dim=1)
+ sem_scores = torch.cat(sem_scores, dim=1)
+ bbox3d = torch.cat(bbox3d, dim=1)
+
+ if use_nms:
+ batch_size = bbox3d.shape[0]
+ results = list()
+ for b in range(batch_size):
+ bbox_selected, score_selected, labels = \
+ self.multiclass_nms_single(obj_scores[b], sem_scores[b],
+ bbox3d[b], points[b, ..., :3],
+ input_metas[b])
+ bbox = input_metas[b]['box_type_3d'](
+ bbox_selected,
+ box_dim=bbox_selected.shape[-1],
+ with_yaw=self.bbox_coder.with_rot)
+ results.append((bbox, score_selected, labels))
+
+ return results
+ else:
+ return bbox3d
+
+ def multiclass_nms_single(self, obj_scores, sem_scores, bbox, points,
+ input_meta):
+ """Multi-class nms in single batch.
+
+ Args:
+ obj_scores (torch.Tensor): Objectness score of bounding boxes.
+ sem_scores (torch.Tensor): semantic class score of bounding boxes.
+ bbox (torch.Tensor): Predicted bounding boxes.
+ points (torch.Tensor): Input points.
+ input_meta (dict): Point cloud and image's meta info.
+
+ Returns:
+ tuple[torch.Tensor]: Bounding boxes, scores and labels.
+ """
+ bbox = input_meta['box_type_3d'](
+ bbox,
+ box_dim=bbox.shape[-1],
+ with_yaw=self.bbox_coder.with_rot,
+ origin=(0.5, 0.5, 0.5))
+ box_indices = bbox.points_in_boxes_all(points)
+
+ corner3d = bbox.corners
+ minmax_box3d = corner3d.new(torch.Size((corner3d.shape[0], 6)))
+ minmax_box3d[:, :3] = torch.min(corner3d, dim=1)[0]
+ minmax_box3d[:, 3:] = torch.max(corner3d, dim=1)[0]
+
+ nonempty_box_mask = box_indices.T.sum(1) > 5
+
+ bbox_classes = torch.argmax(sem_scores, -1)
+ nms_selected = aligned_3d_nms(minmax_box3d[nonempty_box_mask],
+ obj_scores[nonempty_box_mask],
+ bbox_classes[nonempty_box_mask],
+ self.test_cfg.nms_thr)
+
+ # filter empty boxes and boxes with low score
+ scores_mask = (obj_scores > self.test_cfg.score_thr)
+ nonempty_box_inds = torch.nonzero(
+ nonempty_box_mask, as_tuple=False).flatten()
+ nonempty_mask = torch.zeros_like(bbox_classes).scatter(
+ 0, nonempty_box_inds[nms_selected], 1)
+ selected = (nonempty_mask.bool() & scores_mask.bool())
+
+ if self.test_cfg.per_class_proposal:
+ bbox_selected, score_selected, labels = [], [], []
+ for k in range(sem_scores.shape[-1]):
+ bbox_selected.append(bbox[selected].tensor)
+ score_selected.append(obj_scores[selected] *
+ sem_scores[selected][:, k])
+ labels.append(
+ torch.zeros_like(bbox_classes[selected]).fill_(k))
+ bbox_selected = torch.cat(bbox_selected, 0)
+ score_selected = torch.cat(score_selected, 0)
+ labels = torch.cat(labels, 0)
+ else:
+ bbox_selected = bbox[selected].tensor
+ score_selected = obj_scores[selected]
+ labels = bbox_classes[selected]
+
+ return bbox_selected, score_selected, labels
diff --git a/mmdet3d/models/dense_heads/monoflex_head.py b/mmdet3d/models/dense_heads/monoflex_head.py
new file mode 100644
index 0000000..2253c75
--- /dev/null
+++ b/mmdet3d/models/dense_heads/monoflex_head.py
@@ -0,0 +1,771 @@
+# Copyright (c) OpenMMLab. All rights reserved.
+import torch
+from mmcv.cnn import xavier_init
+from torch import nn as nn
+
+from mmdet3d.core.utils import get_ellip_gaussian_2D
+from mmdet3d.models.model_utils import EdgeFusionModule
+from mmdet3d.models.utils import (filter_outside_objs, get_edge_indices,
+ get_keypoints, handle_proj_objs)
+from mmdet.core import multi_apply
+from mmdet.core.bbox.builder import build_bbox_coder
+from mmdet.models.utils import gaussian_radius, gen_gaussian_target
+from mmdet.models.utils.gaussian_target import (get_local_maximum,
+ get_topk_from_heatmap,
+ transpose_and_gather_feat)
+from ..builder import HEADS, build_loss
+from .anchor_free_mono3d_head import AnchorFreeMono3DHead
+
+
+@HEADS.register_module()
+class MonoFlexHead(AnchorFreeMono3DHead):
+ r"""MonoFlex head used in `MonoFlex `_
+
+ .. code-block:: none
+
+ / --> 3 x 3 conv --> 1 x 1 conv --> [edge fusion] --> cls
+ |
+ | --> 3 x 3 conv --> 1 x 1 conv --> 2d bbox
+ |
+ | --> 3 x 3 conv --> 1 x 1 conv --> [edge fusion] --> 2d offsets
+ |
+ | --> 3 x 3 conv --> 1 x 1 conv --> keypoints offsets
+ |
+ | --> 3 x 3 conv --> 1 x 1 conv --> keypoints uncertainty
+ feature
+ | --> 3 x 3 conv --> 1 x 1 conv --> keypoints uncertainty
+ |
+ | --> 3 x 3 conv --> 1 x 1 conv --> 3d dimensions
+ |
+ | |--- 1 x 1 conv --> ori cls
+ | --> 3 x 3 conv --|
+ | |--- 1 x 1 conv --> ori offsets
+ |
+ | --> 3 x 3 conv --> 1 x 1 conv --> depth
+ |
+ \ --> 3 x 3 conv --> 1 x 1 conv --> depth uncertainty
+
+ Args:
+ use_edge_fusion (bool): Whether to use edge fusion module while
+ feature extraction.
+ edge_fusion_inds (list[tuple]): Indices of feature to use edge fusion.
+ edge_heatmap_ratio (float): Ratio of generating target heatmap.
+ filter_outside_objs (bool, optional): Whether to filter the
+ outside objects. Default: True.
+ loss_cls (dict, optional): Config of classification loss.
+ Default: loss_cls=dict(type='GaussionFocalLoss', loss_weight=1.0).
+ loss_bbox (dict, optional): Config of localization loss.
+ Default: loss_bbox=dict(type='IOULoss', loss_weight=10.0).
+ loss_dir (dict, optional): Config of direction classification loss.
+ Default: dict(type='MultibinLoss', loss_weight=0.1).
+ loss_keypoints (dict, optional): Config of keypoints loss.
+ Default: dict(type='L1Loss', loss_weight=0.1).
+ loss_dims: (dict, optional): Config of dimensions loss.
+ Default: dict(type='L1Loss', loss_weight=0.1).
+ loss_offsets2d: (dict, optional): Config of offsets2d loss.
+ Default: dict(type='L1Loss', loss_weight=0.1).
+ loss_direct_depth: (dict, optional): Config of directly regression depth loss.
+ Default: dict(type='L1Loss', loss_weight=0.1).
+ loss_keypoints_depth: (dict, optional): Config of keypoints decoded depth loss.
+ Default: dict(type='L1Loss', loss_weight=0.1).
+ loss_combined_depth: (dict, optional): Config of combined depth loss.
+ Default: dict(type='L1Loss', loss_weight=0.1).
+ loss_attr (dict, optional): Config of attribute classification loss.
+ In MonoFlex, Default: None.
+ bbox_coder (dict, optional): Bbox coder for encoding and decoding boxes.
+ Default: dict(type='MonoFlexCoder', code_size=7).
+ norm_cfg (dict, optional): Dictionary to construct and config norm layer.
+ Default: norm_cfg=dict(type='GN', num_groups=32, requires_grad=True).
+ init_cfg (dict): Initialization config dict. Default: None.
+ """ # noqa: E501
+
+ def __init__(self,
+ num_classes,
+ in_channels,
+ use_edge_fusion,
+ edge_fusion_inds,
+ edge_heatmap_ratio,
+ filter_outside_objs=True,
+ loss_cls=dict(type='GaussianFocalLoss', loss_weight=1.0),
+ loss_bbox=dict(type='IoULoss', loss_weight=0.1),
+ loss_dir=dict(type='MultiBinLoss', loss_weight=0.1),
+ loss_keypoints=dict(type='L1Loss', loss_weight=0.1),
+ loss_dims=dict(type='L1Loss', loss_weight=0.1),
+ loss_offsets2d=dict(type='L1Loss', loss_weight=0.1),
+ loss_direct_depth=dict(type='L1Loss', loss_weight=0.1),
+ loss_keypoints_depth=dict(type='L1Loss', loss_weight=0.1),
+ loss_combined_depth=dict(type='L1Loss', loss_weight=0.1),
+ loss_attr=None,
+ bbox_coder=dict(type='MonoFlexCoder', code_size=7),
+ norm_cfg=dict(type='BN'),
+ init_cfg=None,
+ init_bias=-2.19,
+ **kwargs):
+ self.use_edge_fusion = use_edge_fusion
+ self.edge_fusion_inds = edge_fusion_inds
+ super().__init__(
+ num_classes,
+ in_channels,
+ loss_cls=loss_cls,
+ loss_bbox=loss_bbox,
+ loss_dir=loss_dir,
+ loss_attr=loss_attr,
+ norm_cfg=norm_cfg,
+ init_cfg=init_cfg,
+ **kwargs)
+ self.filter_outside_objs = filter_outside_objs
+ self.edge_heatmap_ratio = edge_heatmap_ratio
+ self.init_bias = init_bias
+ self.loss_dir = build_loss(loss_dir)
+ self.loss_keypoints = build_loss(loss_keypoints)
+ self.loss_dims = build_loss(loss_dims)
+ self.loss_offsets2d = build_loss(loss_offsets2d)
+ self.loss_direct_depth = build_loss(loss_direct_depth)
+ self.loss_keypoints_depth = build_loss(loss_keypoints_depth)
+ self.loss_combined_depth = build_loss(loss_combined_depth)
+ self.bbox_coder = build_bbox_coder(bbox_coder)
+
+ def _init_edge_module(self):
+ """Initialize edge fusion module for feature extraction."""
+ self.edge_fuse_cls = EdgeFusionModule(self.num_classes, 256)
+ for i in range(len(self.edge_fusion_inds)):
+ reg_inds, out_inds = self.edge_fusion_inds[i]
+ out_channels = self.group_reg_dims[reg_inds][out_inds]
+ fusion_layer = EdgeFusionModule(out_channels, 256)
+ layer_name = f'edge_fuse_reg_{reg_inds}_{out_inds}'
+ self.add_module(layer_name, fusion_layer)
+
+ def init_weights(self):
+ """Initialize weights."""
+ super().init_weights()
+ self.conv_cls.bias.data.fill_(self.init_bias)
+ xavier_init(self.conv_regs[4][0], gain=0.01)
+ xavier_init(self.conv_regs[7][0], gain=0.01)
+ for m in self.conv_regs.modules():
+ if isinstance(m, nn.Conv2d):
+ if m.bias is not None:
+ nn.init.constant_(m.bias, 0)
+
+ def _init_predictor(self):
+ """Initialize predictor layers of the head."""
+ self.conv_cls_prev = self._init_branch(
+ conv_channels=self.cls_branch,
+ conv_strides=(1, ) * len(self.cls_branch))
+ self.conv_cls = nn.Conv2d(self.cls_branch[-1], self.cls_out_channels,
+ 1)
+ # init regression head
+ self.conv_reg_prevs = nn.ModuleList()
+ # init output head
+ self.conv_regs = nn.ModuleList()
+ # group_reg_dims:
+ # ((4, ), (2, ), (20, ), (3, ), (3, ), (8, 8), (1, ), (1, ))
+ for i in range(len(self.group_reg_dims)):
+ reg_dims = self.group_reg_dims[i]
+ reg_branch_channels = self.reg_branch[i]
+ out_channel = self.out_channels[i]
+ reg_list = nn.ModuleList()
+ if len(reg_branch_channels) > 0:
+ self.conv_reg_prevs.append(
+ self._init_branch(
+ conv_channels=reg_branch_channels,
+ conv_strides=(1, ) * len(reg_branch_channels)))
+ for reg_dim in reg_dims:
+ reg_list.append(nn.Conv2d(out_channel, reg_dim, 1))
+ self.conv_regs.append(reg_list)
+ else:
+ self.conv_reg_prevs.append(None)
+ for reg_dim in reg_dims:
+ reg_list.append(nn.Conv2d(self.feat_channels, reg_dim, 1))
+ self.conv_regs.append(reg_list)
+
+ def _init_layers(self):
+ """Initialize layers of the head."""
+ self._init_predictor()
+ if self.use_edge_fusion:
+ self._init_edge_module()
+
+ def forward_train(self, x, input_metas, gt_bboxes, gt_labels, gt_bboxes_3d,
+ gt_labels_3d, centers2d, depths, attr_labels,
+ gt_bboxes_ignore, proposal_cfg, **kwargs):
+ """
+ Args:
+ x (list[Tensor]): Features from FPN.
+ input_metas (list[dict]): Meta information of each image, e.g.,
+ image size, scaling factor, etc.
+ gt_bboxes (list[Tensor]): Ground truth bboxes of the image,
+ shape (num_gts, 4).
+ gt_labels (list[Tensor]): Ground truth labels of each box,
+ shape (num_gts,).
+ gt_bboxes_3d (list[Tensor]): 3D ground truth bboxes of the image,
+ shape (num_gts, self.bbox_code_size).
+ gt_labels_3d (list[Tensor]): 3D ground truth labels of each box,
+ shape (num_gts,).
+ centers2d (list[Tensor]): Projected 3D center of each box,
+ shape (num_gts, 2).
+ depths (list[Tensor]): Depth of projected 3D center of each box,
+ shape (num_gts,).
+ attr_labels (list[Tensor]): Attribute labels of each box,
+ shape (num_gts,).
+ gt_bboxes_ignore (list[Tensor]): Ground truth bboxes to be
+ ignored, shape (num_ignored_gts, 4).
+ proposal_cfg (mmcv.Config): Test / postprocessing configuration,
+ if None, test_cfg would be used
+ Returns:
+ tuple:
+ losses: (dict[str, Tensor]): A dictionary of loss components.
+ proposal_list (list[Tensor]): Proposals of each image.
+ """
+ outs = self(x, input_metas)
+ if gt_labels is None:
+ loss_inputs = outs + (gt_bboxes, gt_bboxes_3d, centers2d, depths,
+ attr_labels, input_metas)
+ else:
+ loss_inputs = outs + (gt_bboxes, gt_labels, gt_bboxes_3d,
+ gt_labels_3d, centers2d, depths, attr_labels,
+ input_metas)
+ losses = self.loss(*loss_inputs, gt_bboxes_ignore=gt_bboxes_ignore)
+ if proposal_cfg is None:
+ return losses
+ else:
+ proposal_list = self.get_bboxes(
+ *outs, input_metas, cfg=proposal_cfg)
+ return losses, proposal_list
+
+ def forward(self, feats, input_metas):
+ """Forward features from the upstream network.
+
+ Args:
+ feats (list[Tensor]): Features from the upstream network, each is
+ a 4D-tensor.
+ input_metas (list[dict]): Meta information of each image, e.g.,
+ image size, scaling factor, etc.
+
+ Returns:
+ tuple:
+ cls_scores (list[Tensor]): Box scores for each scale level,
+ each is a 4D-tensor, the channel number is
+ num_points * num_classes.
+ bbox_preds (list[Tensor]): Box energies / deltas for each scale
+ level, each is a 4D-tensor, the channel number is
+ num_points * bbox_code_size.
+ """
+ mlvl_input_metas = [input_metas for i in range(len(feats))]
+ return multi_apply(self.forward_single, feats, mlvl_input_metas)
+
+ def forward_single(self, x, input_metas):
+ """Forward features of a single scale level.
+
+ Args:
+ x (Tensor): Feature maps from a specific FPN feature level.
+ input_metas (list[dict]): Meta information of each image, e.g.,
+ image size, scaling factor, etc.
+
+ Returns:
+ tuple: Scores for each class, bbox predictions.
+ """
+ img_h, img_w = input_metas[0]['pad_shape'][:2]
+ batch_size, _, feat_h, feat_w = x.shape
+ downsample_ratio = img_h / feat_h
+
+ for conv_cls_prev_layer in self.conv_cls_prev:
+ cls_feat = conv_cls_prev_layer(x)
+ out_cls = self.conv_cls(cls_feat)
+
+ if self.use_edge_fusion:
+ # calculate the edge indices for the batch data
+ edge_indices_list = get_edge_indices(
+ input_metas, downsample_ratio, device=x.device)
+ edge_lens = [
+ edge_indices.shape[0] for edge_indices in edge_indices_list
+ ]
+ max_edge_len = max(edge_lens)
+ edge_indices = x.new_zeros((batch_size, max_edge_len, 2),
+ dtype=torch.long)
+ for i in range(batch_size):
+ edge_indices[i, :edge_lens[i]] = edge_indices_list[i]
+ # cls feature map edge fusion
+ out_cls = self.edge_fuse_cls(cls_feat, out_cls, edge_indices,
+ edge_lens, feat_h, feat_w)
+
+ bbox_pred = []
+
+ for i in range(len(self.group_reg_dims)):
+ reg_feat = x.clone()
+ # feature regression head
+ if len(self.reg_branch[i]) > 0:
+ for conv_reg_prev_layer in self.conv_reg_prevs[i]:
+ reg_feat = conv_reg_prev_layer(reg_feat)
+
+ for j, conv_reg in enumerate(self.conv_regs[i]):
+ out_reg = conv_reg(reg_feat)
+ # Use Edge Fusion Module
+ if self.use_edge_fusion and (i, j) in self.edge_fusion_inds:
+ # reg feature map edge fusion
+ out_reg = getattr(self, 'edge_fuse_reg_{}_{}'.format(
+ i, j))(reg_feat, out_reg, edge_indices, edge_lens,
+ feat_h, feat_w)
+ bbox_pred.append(out_reg)
+
+ bbox_pred = torch.cat(bbox_pred, dim=1)
+ cls_score = out_cls.sigmoid() # turn to 0-1
+ cls_score = cls_score.clamp(min=1e-4, max=1 - 1e-4)
+
+ return cls_score, bbox_pred
+
+ def get_bboxes(self, cls_scores, bbox_preds, input_metas):
+ """Generate bboxes from bbox head predictions.
+
+ Args:
+ cls_scores (list[Tensor]): Box scores for each scale level.
+ bbox_preds (list[Tensor]): Box regression for each scale.
+ input_metas (list[dict]): Meta information of each image, e.g.,
+ image size, scaling factor, etc.
+ rescale (bool): If True, return boxes in original image space.
+ Returns:
+ list[tuple[:obj:`CameraInstance3DBoxes`, Tensor, Tensor, None]]:
+ Each item in result_list is 4-tuple.
+ """
+ assert len(cls_scores) == len(bbox_preds) == 1
+ cam2imgs = torch.stack([
+ cls_scores[0].new_tensor(input_meta['cam2img'])
+ for input_meta in input_metas
+ ])
+ batch_bboxes, batch_scores, batch_topk_labels = self.decode_heatmap(
+ cls_scores[0],
+ bbox_preds[0],
+ input_metas,
+ cam2imgs=cam2imgs,
+ topk=100,
+ kernel=3)
+
+ result_list = []
+ for img_id in range(len(input_metas)):
+
+ bboxes = batch_bboxes[img_id]
+ scores = batch_scores[img_id]
+ labels = batch_topk_labels[img_id]
+
+ keep_idx = scores > 0.25
+ bboxes = bboxes[keep_idx]
+ scores = scores[keep_idx]
+ labels = labels[keep_idx]
+
+ bboxes = input_metas[img_id]['box_type_3d'](
+ bboxes, box_dim=self.bbox_code_size, origin=(0.5, 0.5, 0.5))
+ attrs = None
+ result_list.append((bboxes, scores, labels, attrs))
+
+ return result_list
+
+ def decode_heatmap(self,
+ cls_score,
+ reg_pred,
+ input_metas,
+ cam2imgs,
+ topk=100,
+ kernel=3):
+ """Transform outputs into detections raw bbox predictions.
+
+ Args:
+ class_score (Tensor): Center predict heatmap,
+ shape (B, num_classes, H, W).
+ reg_pred (Tensor): Box regression map.
+ shape (B, channel, H , W).
+ input_metas (List[dict]): Meta information of each image, e.g.,
+ image size, scaling factor, etc.
+ cam2imgs (Tensor): Camera intrinsic matrix.
+ shape (N, 4, 4)
+ topk (int, optional): Get top k center keypoints from heatmap.
+ Default 100.
+ kernel (int, optional): Max pooling kernel for extract local
+ maximum pixels. Default 3.
+
+ Returns:
+ tuple[torch.Tensor]: Decoded output of SMOKEHead, containing
+ the following Tensors:
+ - batch_bboxes (Tensor): Coords of each 3D box.
+ shape (B, k, 7)
+ - batch_scores (Tensor): Scores of each 3D box.
+ shape (B, k)
+ - batch_topk_labels (Tensor): Categories of each 3D box.
+ shape (B, k)
+ """
+ img_h, img_w = input_metas[0]['pad_shape'][:2]
+ batch_size, _, feat_h, feat_w = cls_score.shape
+
+ downsample_ratio = img_h / feat_h
+ center_heatmap_pred = get_local_maximum(cls_score, kernel=kernel)
+
+ *batch_dets, topk_ys, topk_xs = get_topk_from_heatmap(
+ center_heatmap_pred, k=topk)
+ batch_scores, batch_index, batch_topk_labels = batch_dets
+
+ regression = transpose_and_gather_feat(reg_pred, batch_index)
+ regression = regression.view(-1, 8)
+
+ pred_base_centers2d = torch.cat(
+ [topk_xs.view(-1, 1),
+ topk_ys.view(-1, 1).float()], dim=1)
+ preds = self.bbox_coder.decode(regression, batch_topk_labels,
+ downsample_ratio, cam2imgs)
+ pred_locations = self.bbox_coder.decode_location(
+ pred_base_centers2d, preds['offsets2d'], preds['combined_depth'],
+ cam2imgs, downsample_ratio)
+ pred_yaws = self.bbox_coder.decode_orientation(
+ preds['orientations']).unsqueeze(-1)
+ pred_dims = preds['dimensions']
+ batch_bboxes = torch.cat((pred_locations, pred_dims, pred_yaws), dim=1)
+ batch_bboxes = batch_bboxes.view(batch_size, -1, self.bbox_code_size)
+ return batch_bboxes, batch_scores, batch_topk_labels
+
+ def get_predictions(self, pred_reg, labels3d, centers2d, reg_mask,
+ batch_indices, input_metas, downsample_ratio):
+ """Prepare predictions for computing loss.
+
+ Args:
+ pred_reg (Tensor): Box regression map.
+ shape (B, channel, H , W).
+ labels3d (Tensor): Labels of each 3D box.
+ shape (B * max_objs, )
+ centers2d (Tensor): Coords of each projected 3D box
+ center on image. shape (N, 2)
+ reg_mask (Tensor): Indexes of the existence of the 3D box.
+ shape (B * max_objs, )
+ batch_indices (Tenosr): Batch indices of the 3D box.
+ shape (N, 3)
+ input_metas (list[dict]): Meta information of each image,
+ e.g., image size, scaling factor, etc.
+ downsample_ratio (int): The stride of feature map.
+
+ Returns:
+ dict: The predictions for computing loss.
+ """
+ batch, channel = pred_reg.shape[0], pred_reg.shape[1]
+ w = pred_reg.shape[3]
+ cam2imgs = torch.stack([
+ centers2d.new_tensor(input_meta['cam2img'])
+ for input_meta in input_metas
+ ])
+ # (batch_size, 4, 4) -> (N, 4, 4)
+ cam2imgs = cam2imgs[batch_indices, :, :]
+ centers2d_inds = centers2d[:, 1] * w + centers2d[:, 0]
+ centers2d_inds = centers2d_inds.view(batch, -1)
+ pred_regression = transpose_and_gather_feat(pred_reg, centers2d_inds)
+ pred_regression_pois = pred_regression.view(-1, channel)[reg_mask]
+ preds = self.bbox_coder.decode(pred_regression_pois, labels3d,
+ downsample_ratio, cam2imgs)
+
+ return preds
+
+ def get_targets(self, gt_bboxes_list, gt_labels_list, gt_bboxes_3d_list,
+ gt_labels_3d_list, centers2d_list, depths_list, feat_shape,
+ img_shape, input_metas):
+ """Get training targets for batch images.
+``
+ Args:
+ gt_bboxes_list (list[Tensor]): Ground truth bboxes of each
+ image, shape (num_gt, 4).
+ gt_labels_list (list[Tensor]): Ground truth labels of each
+ box, shape (num_gt,).
+ gt_bboxes_3d_list (list[:obj:`CameraInstance3DBoxes`]): 3D
+ Ground truth bboxes of each image,
+ shape (num_gt, bbox_code_size).
+ gt_labels_3d_list (list[Tensor]): 3D Ground truth labels of
+ each box, shape (num_gt,).
+ centers2d_list (list[Tensor]): Projected 3D centers onto 2D
+ image, shape (num_gt, 2).
+ depths_list (list[Tensor]): Depth of projected 3D centers onto 2D
+ image, each has shape (num_gt, 1).
+ feat_shape (tuple[int]): Feature map shape with value,
+ shape (B, _, H, W).
+ img_shape (tuple[int]): Image shape in [h, w] format.
+ input_metas (list[dict]): Meta information of each image, e.g.,
+ image size, scaling factor, etc.
+
+ Returns:
+ tuple[Tensor, dict]: The Tensor value is the targets of
+ center heatmap, the dict has components below:
+ - base_centers2d_target (Tensor): Coords of each projected 3D box
+ center on image. shape (B * max_objs, 2), [dtype: int]
+ - labels3d (Tensor): Labels of each 3D box.
+ shape (N, )
+ - reg_mask (Tensor): Mask of the existence of the 3D box.
+ shape (B * max_objs, )
+ - batch_indices (Tensor): Batch id of the 3D box.
+ shape (N, )
+ - depth_target (Tensor): Depth target of each 3D box.
+ shape (N, )
+ - keypoints2d_target (Tensor): Keypoints of each projected 3D box
+ on image. shape (N, 10, 2)
+ - keypoints_mask (Tensor): Keypoints mask of each projected 3D
+ box on image. shape (N, 10)
+ - keypoints_depth_mask (Tensor): Depths decoded from keypoints
+ of each 3D box. shape (N, 3)
+ - orientations_target (Tensor): Orientation (encoded local yaw)
+ target of each 3D box. shape (N, )
+ - offsets2d_target (Tensor): Offsets target of each projected
+ 3D box. shape (N, 2)
+ - dimensions_target (Tensor): Dimensions target of each 3D box.
+ shape (N, 3)
+ - downsample_ratio (int): The stride of feature map.
+ """
+
+ img_h, img_w = img_shape[:2]
+ batch_size, _, feat_h, feat_w = feat_shape
+
+ width_ratio = float(feat_w / img_w) # 1/4
+ height_ratio = float(feat_h / img_h) # 1/4
+
+ assert width_ratio == height_ratio
+
+ # Whether to filter the objects which are not in FOV.
+ if self.filter_outside_objs:
+ filter_outside_objs(gt_bboxes_list, gt_labels_list,
+ gt_bboxes_3d_list, gt_labels_3d_list,
+ centers2d_list, input_metas)
+
+ # transform centers2d to base centers2d for regression and
+ # heatmap generation.
+ # centers2d = int(base_centers2d) + offsets2d
+ base_centers2d_list, offsets2d_list, trunc_mask_list = \
+ handle_proj_objs(centers2d_list, gt_bboxes_list, input_metas)
+
+ keypoints2d_list, keypoints_mask_list, keypoints_depth_mask_list = \
+ get_keypoints(gt_bboxes_3d_list, centers2d_list, input_metas)
+
+ center_heatmap_target = gt_bboxes_list[-1].new_zeros(
+ [batch_size, self.num_classes, feat_h, feat_w])
+
+ for batch_id in range(batch_size):
+ # project gt_bboxes from input image to feat map
+ gt_bboxes = gt_bboxes_list[batch_id] * width_ratio
+ gt_labels = gt_labels_list[batch_id]
+
+ # project base centers2d from input image to feat map
+ gt_base_centers2d = base_centers2d_list[batch_id] * width_ratio
+ trunc_masks = trunc_mask_list[batch_id]
+
+ for j, base_center2d in enumerate(gt_base_centers2d):
+ if trunc_masks[j]:
+ # for outside objects, generate ellipse heatmap
+ base_center2d_x_int, base_center2d_y_int = \
+ base_center2d.int()
+ scale_box_w = min(base_center2d_x_int - gt_bboxes[j][0],
+ gt_bboxes[j][2] - base_center2d_x_int)
+ scale_box_h = min(base_center2d_y_int - gt_bboxes[j][1],
+ gt_bboxes[j][3] - base_center2d_y_int)
+ radius_x = scale_box_w * self.edge_heatmap_ratio
+ radius_y = scale_box_h * self.edge_heatmap_ratio
+ radius_x, radius_y = max(0, int(radius_x)), max(
+ 0, int(radius_y))
+ assert min(radius_x, radius_y) == 0
+ ind = gt_labels[j]
+ get_ellip_gaussian_2D(
+ center_heatmap_target[batch_id, ind],
+ [base_center2d_x_int, base_center2d_y_int], radius_x,
+ radius_y)
+ else:
+ base_center2d_x_int, base_center2d_y_int = \
+ base_center2d.int()
+ scale_box_h = (gt_bboxes[j][3] - gt_bboxes[j][1])
+ scale_box_w = (gt_bboxes[j][2] - gt_bboxes[j][0])
+ radius = gaussian_radius([scale_box_h, scale_box_w],
+ min_overlap=0.7)
+ radius = max(0, int(radius))
+ ind = gt_labels[j]
+ gen_gaussian_target(
+ center_heatmap_target[batch_id, ind],
+ [base_center2d_x_int, base_center2d_y_int], radius)
+
+ avg_factor = max(1, center_heatmap_target.eq(1).sum())
+ num_ctrs = [centers2d.shape[0] for centers2d in centers2d_list]
+ max_objs = max(num_ctrs)
+ batch_indices = [
+ centers2d_list[0].new_full((num_ctrs[i], ), i)
+ for i in range(batch_size)
+ ]
+ batch_indices = torch.cat(batch_indices, dim=0)
+ reg_mask = torch.zeros(
+ (batch_size, max_objs),
+ dtype=torch.bool).to(base_centers2d_list[0].device)
+ gt_bboxes_3d = input_metas['box_type_3d'].cat(gt_bboxes_3d_list)
+ gt_bboxes_3d = gt_bboxes_3d.to(base_centers2d_list[0].device)
+
+ # encode original local yaw to multibin format
+ orienations_target = self.bbox_coder.encode(gt_bboxes_3d)
+
+ batch_base_centers2d = base_centers2d_list[0].new_zeros(
+ (batch_size, max_objs, 2))
+
+ for i in range(batch_size):
+ reg_mask[i, :num_ctrs[i]] = 1
+ batch_base_centers2d[i, :num_ctrs[i]] = base_centers2d_list[i]
+
+ flatten_reg_mask = reg_mask.flatten()
+
+ # transform base centers2d from input scale to output scale
+ batch_base_centers2d = batch_base_centers2d.view(-1, 2) * width_ratio
+
+ dimensions_target = gt_bboxes_3d.tensor[:, 3:6]
+ labels_3d = torch.cat(gt_labels_3d_list)
+ keypoints2d_target = torch.cat(keypoints2d_list)
+ keypoints_mask = torch.cat(keypoints_mask_list)
+ keypoints_depth_mask = torch.cat(keypoints_depth_mask_list)
+ offsets2d_target = torch.cat(offsets2d_list)
+ bboxes2d = torch.cat(gt_bboxes_list)
+
+ # transform FCOS style bbox into [x1, y1, x2, y2] format.
+ bboxes2d_target = torch.cat([bboxes2d[:, 0:2] * -1, bboxes2d[:, 2:]],
+ dim=-1)
+ depths = torch.cat(depths_list)
+
+ target_labels = dict(
+ base_centers2d_target=batch_base_centers2d.int(),
+ labels3d=labels_3d,
+ reg_mask=flatten_reg_mask,
+ batch_indices=batch_indices,
+ bboxes2d_target=bboxes2d_target,
+ depth_target=depths,
+ keypoints2d_target=keypoints2d_target,
+ keypoints_mask=keypoints_mask,
+ keypoints_depth_mask=keypoints_depth_mask,
+ orienations_target=orienations_target,
+ offsets2d_target=offsets2d_target,
+ dimensions_target=dimensions_target,
+ downsample_ratio=1 / width_ratio)
+
+ return center_heatmap_target, avg_factor, target_labels
+
+ def loss(self,
+ cls_scores,
+ bbox_preds,
+ gt_bboxes,
+ gt_labels,
+ gt_bboxes_3d,
+ gt_labels_3d,
+ centers2d,
+ depths,
+ attr_labels,
+ input_metas,
+ gt_bboxes_ignore=None):
+ """Compute loss of the head.
+
+ Args:
+ cls_scores (list[Tensor]): Box scores for each scale level.
+ shape (num_gt, 4).
+ bbox_preds (list[Tensor]): Box dims is a 4D-tensor, the channel
+ number is bbox_code_size.
+ shape (B, 7, H, W).
+ gt_bboxes (list[Tensor]): Ground truth bboxes for each image.
+ shape (num_gts, 4) in [tl_x, tl_y, br_x, br_y] format.
+ gt_labels (list[Tensor]): Class indices corresponding to each box.
+ shape (num_gts, ).
+ gt_bboxes_3d (list[:obj:`CameraInstance3DBoxes`]): 3D boxes ground
+ truth. it is the flipped gt_bboxes
+ gt_labels_3d (list[Tensor]): Same as gt_labels.
+ centers2d (list[Tensor]): 2D centers on the image.
+ shape (num_gts, 2).
+ depths (list[Tensor]): Depth ground truth.
+ shape (num_gts, ).
+ attr_labels (list[Tensor]): Attributes indices of each box.
+ In kitti it's None.
+ input_metas (list[dict]): Meta information of each image, e.g.,
+ image size, scaling factor, etc.
+ gt_bboxes_ignore (None | list[Tensor]): Specify which bounding
+ boxes can be ignored when computing the loss.
+ Default: None.
+
+ Returns:
+ dict[str, Tensor]: A dictionary of loss components.
+ """
+ assert len(cls_scores) == len(bbox_preds) == 1
+ assert attr_labels is None
+ assert gt_bboxes_ignore is None
+ center2d_heatmap = cls_scores[0]
+ pred_reg = bbox_preds[0]
+
+ center2d_heatmap_target, avg_factor, target_labels = \
+ self.get_targets(gt_bboxes, gt_labels, gt_bboxes_3d,
+ gt_labels_3d, centers2d, depths,
+ center2d_heatmap.shape,
+ input_metas[0]['pad_shape'],
+ input_metas)
+
+ preds = self.get_predictions(
+ pred_reg=pred_reg,
+ labels3d=target_labels['labels3d'],
+ centers2d=target_labels['base_centers2d_target'],
+ reg_mask=target_labels['reg_mask'],
+ batch_indices=target_labels['batch_indices'],
+ input_metas=input_metas,
+ downsample_ratio=target_labels['downsample_ratio'])
+
+ # heatmap loss
+ loss_cls = self.loss_cls(
+ center2d_heatmap, center2d_heatmap_target, avg_factor=avg_factor)
+
+ # bbox2d regression loss
+ loss_bbox = self.loss_bbox(preds['bboxes2d'],
+ target_labels['bboxes2d_target'])
+
+ # keypoints loss, the keypoints in predictions and target are all
+ # local coordinates. Check the mask dtype should be bool, not int
+ # or float to ensure the indexing is bool index
+ keypoints2d_mask = target_labels['keypoints2d_mask']
+ loss_keypoints = self.loss_keypoints(
+ preds['keypoints2d'][keypoints2d_mask],
+ target_labels['keypoints2d_target'][keypoints2d_mask])
+
+ # orientations loss
+ loss_dir = self.loss_dir(preds['orientations'],
+ target_labels['orientations_target'])
+
+ # dimensions loss
+ loss_dims = self.loss_dims(preds['dimensions'],
+ target_labels['dimensions_target'])
+
+ # offsets for center heatmap
+ loss_offsets2d = self.loss_offsets2d(preds['offsets2d'],
+ target_labels['offsets2d_target'])
+
+ # directly regressed depth loss with direct depth uncertainty loss
+ direct_depth_weights = torch.exp(-preds['direct_depth_uncertainty'])
+ loss_weight_1 = self.loss_direct_depth.loss_weight
+ loss_direct_depth = self.loss_direct_depth(
+ preds['direct_depth'], target_labels['depth_target'],
+ direct_depth_weights)
+ loss_uncertainty_1 =\
+ preds['direct_depth_uncertainty'] * loss_weight_1
+ loss_direct_depth = loss_direct_depth + loss_uncertainty_1.mean()
+
+ # keypoints decoded depth loss with keypoints depth uncertainty loss
+ depth_mask = target_labels['keypoints_depth_mask']
+ depth_target = target_labels['depth_target'].unsqueeze(-1).repeat(1, 3)
+ valid_keypoints_depth_uncertainty = preds[
+ 'keypoints_depth_uncertainty'][depth_mask]
+ valid_keypoints_depth_weights = torch.exp(
+ -valid_keypoints_depth_uncertainty)
+ loss_keypoints_depth = self.loss_keypoint_depth(
+ preds['keypoints_depth'][depth_mask], depth_target[depth_mask],
+ valid_keypoints_depth_weights)
+ loss_weight_2 = self.loss_keypoints_depth.loss_weight
+ loss_uncertainty_2 =\
+ valid_keypoints_depth_uncertainty * loss_weight_2
+ loss_keypoints_depth = loss_keypoints_depth + loss_uncertainty_2.mean()
+
+ # combined depth loss for optimiaze the uncertainty
+ loss_combined_depth = self.loss_combined_depth(
+ preds['combined_depth'], target_labels['depth_target'])
+
+ loss_dict = dict(
+ loss_cls=loss_cls,
+ loss_bbox=loss_bbox,
+ loss_keypoints=loss_keypoints,
+ loss_dir=loss_dir,
+ loss_dims=loss_dims,
+ loss_offsets2d=loss_offsets2d,
+ loss_direct_depth=loss_direct_depth,
+ loss_keypoints_depth=loss_keypoints_depth,
+ loss_combined_depth=loss_combined_depth)
+
+ return loss_dict
diff --git a/mmdet3d/models/dense_heads/ngfc_head.py b/mmdet3d/models/dense_heads/ngfc_head.py
new file mode 100644
index 0000000..22619a5
--- /dev/null
+++ b/mmdet3d/models/dense_heads/ngfc_head.py
@@ -0,0 +1,508 @@
+try:
+ import MinkowskiEngine as ME
+except ImportError:
+ import warnings
+ warnings.warn(
+ 'Please follow `getting_started.md` to install MinkowskiEngine.`')
+
+import torch
+from torch import nn
+
+from mmcv.runner import BaseModule
+from mmcv.cnn import Scale, bias_init_with_prob
+from mmcv.ops import nms3d, nms3d_normal
+from mmdet.core.bbox.builder import BBOX_ASSIGNERS, build_assigner
+from mmdet3d.models.builder import HEADS, build_loss
+from mmdet3d.core.bbox.structures import rotation_3d_in_axis
+
+
+@HEADS.register_module()
+class NgfcOffsetHead(BaseModule):
+ def __init__(self,
+ n_classes,
+ in_channels,
+ voxel_size,
+ cls_threshold,
+ assigner,
+ bbox_loss=dict(type='L1Loss'),
+ cls_loss=dict(type='FocalLoss')):
+ super(NgfcOffsetHead, self).__init__()
+ self.voxel_size = voxel_size
+ self.cls_threshold = cls_threshold
+ self.assigner = build_assigner(assigner)
+ self.bbox_loss = build_loss(bbox_loss)
+ self.cls_loss = build_loss(cls_loss)
+ self._init_layers(n_classes, in_channels)
+
+ def _init_layers(self, n_classes, in_channels):
+ self.bbox_conv = ME.MinkowskiConvolution(
+ in_channels, 3, kernel_size=1, bias=True, dimension=3)
+ self.cls_conv = ME.MinkowskiConvolution(
+ in_channels, n_classes, kernel_size=1, bias=True, dimension=3)
+ self.conv = nn.Conv1d(in_channels, in_channels, 1)
+
+ def init_weights(self):
+ nn.init.normal_(self.bbox_conv.kernel, std=.01)
+ nn.init.normal_(self.cls_conv.kernel, std=.01)
+ nn.init.constant_(self.cls_conv.bias, bias_init_with_prob(.01))
+
+ def forward(self, x):
+ # -> bbox_preds, cls_preds, points, sampled and shifted tensor
+ bbox_pred = self.bbox_conv(x)
+ cls_pred = self.cls_conv(x)
+
+ bbox_preds, cls_preds, points = [], [], []
+ for permutation in x.decomposition_permutations:
+ bbox_preds.append(bbox_pred.features[permutation])
+ cls_preds.append(cls_pred.features[permutation])
+ points.append(x.coordinates[permutation][:, 1:] * self.voxel_size)
+
+ mask = cls_pred.features.max(dim=1).values.sigmoid() > self.cls_threshold
+ coordinates = x.coordinates[mask]
+ features = x.features[mask]
+ shifts = bbox_pred.features[mask]
+ new_coordinates = torch.cat((
+ coordinates[:, :1],
+ ((coordinates[:, 1:] * self.voxel_size
+ + shifts) / self.voxel_size).round()), dim=1) # todo: .int() ?
+
+
+ if features.shape[0] > 0:
+ features = self.conv(features.unsqueeze(2))[:, :, 0]
+ new_coordinates = torch.cat((x.coordinates, new_coordinates))
+ features = torch.cat((x.features, features))
+
+ # SparseTensor with initial voxel size and stride = 1
+ x = ME.SparseTensor(
+ coordinates=new_coordinates,
+ features=features,
+ quantization_mode=ME.SparseTensorQuantizationMode.UNWEIGHTED_AVERAGE)
+ return bbox_preds, cls_preds, points, x
+
+ # per scene
+ def _loss_single(self,
+ bbox_preds,
+ cls_preds,
+ points,
+ gt_bboxes,
+ gt_labels,
+ img_meta):
+ assigned_ids = self.assigner.assign([points], gt_bboxes)
+
+ # cls loss
+ n_classes = cls_preds.shape[1]
+ pos_mask = assigned_ids >= 0
+ cls_targets = torch.where(pos_mask, gt_labels[assigned_ids], n_classes)
+ avg_factor = max(pos_mask.sum(), 1)
+ cls_loss = self.cls_loss(cls_preds, cls_targets, avg_factor=avg_factor)
+
+ # bbox loss
+ pos_bbox_preds = bbox_preds[pos_mask]
+ if pos_mask.sum() > 0:
+ pos_points = points[pos_mask]
+ pos_bbox_preds = bbox_preds[pos_mask]
+ bbox_targets = gt_bboxes.gravity_center.to(points.device)
+ pos_bbox_targets = bbox_targets[assigned_ids][pos_mask] - pos_points
+ bbox_loss = self.bbox_loss(pos_bbox_preds, pos_bbox_targets,
+ avg_factor=pos_bbox_targets.abs().sum())
+ else:
+ bbox_loss = pos_bbox_preds.sum()
+ return bbox_loss, cls_loss
+
+ def _loss(self, bbox_preds, cls_preds, points,
+ gt_bboxes, gt_labels, img_metas):
+ bbox_losses, cls_losses = [], []
+ for i in range(len(img_metas)):
+ bbox_loss, cls_loss = self._loss_single(
+ bbox_preds=bbox_preds[i],
+ cls_preds=cls_preds[i],
+ points=points[i],
+ img_meta=img_metas[i],
+ gt_bboxes=gt_bboxes[i],
+ gt_labels=gt_labels[i])
+ bbox_losses.append(bbox_loss)
+ cls_losses.append(cls_loss)
+ return dict(
+ offset_loss=torch.mean(torch.stack(bbox_losses)),
+ obj_loss=torch.mean(torch.stack(cls_losses)))
+
+ def forward_train(self, x, gt_bboxes, gt_labels, img_metas):
+ bbox_preds, cls_preds, points, x = self(x)
+ return x, self._loss(bbox_preds, cls_preds, points,
+ gt_bboxes, gt_labels, img_metas)
+
+ def forward_test(self, x, img_metas):
+ _, _, _, x = self(x)
+ return x
+
+
+@HEADS.register_module()
+class NgfcHead(BaseModule):
+ def __init__(self,
+ n_classes,
+ in_channels,
+ n_levels,
+ n_reg_outs,
+ padding,
+ voxel_size,
+ assigner,
+ bbox_loss=dict(type='AxisAlignedIoULoss'),
+ cls_loss=dict(type='FocalLoss'),
+ train_cfg=None,
+ test_cfg=None):
+ super(NgfcHead, self).__init__()
+ self.padding = padding
+ self.voxel_size = voxel_size
+ self.assigner = build_assigner(assigner)
+ self.bbox_loss = build_loss(bbox_loss)
+ self.cls_loss = build_loss(cls_loss)
+ self.train_cfg = train_cfg
+ self.test_cfg = test_cfg
+ self._init_layers(n_classes, in_channels, n_levels, n_reg_outs)
+
+ def _init_layers(self, n_classes, in_channels, n_levels, n_reg_outs):
+ for i in range(n_levels):
+ self.__setattr__(f'scale_{i}', Scale(1.))
+ self.bbox_conv = ME.MinkowskiConvolution(
+ in_channels, n_reg_outs, kernel_size=1, bias=True, dimension=3)
+ self.cls_conv = ME.MinkowskiConvolution(
+ in_channels, n_classes, kernel_size=1, bias=True, dimension=3)
+
+ def init_weights(self):
+ nn.init.normal_(self.bbox_conv.kernel, std=.01)
+ nn.init.normal_(self.cls_conv.kernel, std=.01)
+ nn.init.constant_(self.cls_conv.bias, bias_init_with_prob(.01))
+
+ # per level
+ def _forward_single(self, x, scale):
+ reg_final = self.bbox_conv(x).features
+ reg_distance = torch.exp(scale(reg_final[:, :6]))
+ reg_angle = reg_final[:, 6:]
+ bbox_pred = torch.cat((reg_distance, reg_angle), dim=1)
+ cls_pred = self.cls_conv(x).features
+
+ bbox_preds, cls_preds, points = [], [], []
+ for permutation in x.decomposition_permutations:
+ bbox_preds.append(bbox_pred[permutation])
+ cls_preds.append(cls_pred[permutation])
+ points.append(x.coordinates[permutation][:, 1:] * self.voxel_size)
+
+ return bbox_preds, cls_preds, points
+
+ def forward(self, x):
+ bbox_preds, cls_preds, points = [], [], []
+ for i in range(len(x)):
+ bbox_pred, cls_pred, point = self._forward_single(
+ x[i], self.__getattr__(f'scale_{i}'))
+ bbox_preds.append(bbox_pred)
+ cls_preds.append(cls_pred)
+ points.append(point)
+ return bbox_preds, cls_preds, points
+
+ @staticmethod
+ def _bbox_to_loss(bbox):
+ """Transform box to the axis-aligned or rotated iou loss format.
+ Args:
+ bbox (Tensor): 3D box of shape (N, 6) or (N, 7).
+ Returns:
+ Tensor: Transformed 3D box of shape (N, 6) or (N, 7).
+ """
+ # rotated iou loss accepts (x, y, z, w, h, l, heading)
+ if bbox.shape[-1] != 6:
+ return bbox
+
+ # axis-aligned case: x, y, z, w, h, l -> x1, y1, z1, x2, y2, z2
+ return torch.stack(
+ (bbox[..., 0] - bbox[..., 3] / 2, bbox[..., 1] - bbox[..., 4] / 2,
+ bbox[..., 2] - bbox[..., 5] / 2, bbox[..., 0] + bbox[..., 3] / 2,
+ bbox[..., 1] + bbox[..., 4] / 2, bbox[..., 2] + bbox[..., 5] / 2),
+ dim=-1)
+
+ @staticmethod
+ def _bbox_pred_to_bbox(points, bbox_pred):
+ """Transform predicted bbox parameters to bbox.
+ Args:
+ points (Tensor): Final locations of shape (N, 3)
+ bbox_pred (Tensor): Predicted bbox parameters of shape (N, 6)
+ or (N, 8).
+ Returns:
+ Tensor: Transformed 3D box of shape (N, 6) or (N, 7).
+ """
+ if bbox_pred.shape[0] == 0:
+ return bbox_pred
+
+ x_center = points[:, 0] + (bbox_pred[:, 1] - bbox_pred[:, 0]) / 2
+ y_center = points[:, 1] + (bbox_pred[:, 3] - bbox_pred[:, 2]) / 2
+ z_center = points[:, 2] + (bbox_pred[:, 5] - bbox_pred[:, 4]) / 2
+
+ # dx_min, dx_max, dy_min, dy_max, dz_min, dz_max -> x, y, z, w, l, h
+ base_bbox = torch.stack([
+ x_center,
+ y_center,
+ z_center,
+ bbox_pred[:, 0] + bbox_pred[:, 1],
+ bbox_pred[:, 2] + bbox_pred[:, 3],
+ bbox_pred[:, 4] + bbox_pred[:, 5],
+ ], -1)
+
+ # axis-aligned case
+ if bbox_pred.shape[1] == 6:
+ return base_bbox
+
+ # rotated case: ..., sin(2a)ln(q), cos(2a)ln(q)
+ scale = bbox_pred[:, 0] + bbox_pred[:, 1] + \
+ bbox_pred[:, 2] + bbox_pred[:, 3]
+ q = torch.exp(
+ torch.sqrt(
+ torch.pow(bbox_pred[:, 6], 2) + torch.pow(bbox_pred[:, 7], 2)))
+ alpha = 0.5 * torch.atan2(bbox_pred[:, 6], bbox_pred[:, 7])
+ return torch.stack(
+ (x_center, y_center, z_center, scale / (1 + q), scale /
+ (1 + q) * q, bbox_pred[:, 5] + bbox_pred[:, 4], alpha),
+ dim=-1)
+
+ # per scene
+ def _loss_single(self,
+ bbox_preds,
+ cls_preds,
+ points,
+ gt_bboxes,
+ gt_labels,
+ img_meta):
+ assigned_ids = self.assigner.assign(points, gt_bboxes)
+ bbox_preds = torch.cat(bbox_preds)
+ cls_preds = torch.cat(cls_preds)
+ points = torch.cat(points)
+
+ # cls loss
+ n_classes = cls_preds.shape[1]
+ pos_mask = assigned_ids >= 0
+ cls_targets = torch.where(pos_mask, gt_labels[assigned_ids], n_classes)
+ avg_factor = max(pos_mask.sum(), 1)
+ cls_loss = self.cls_loss(cls_preds, cls_targets, avg_factor=avg_factor)
+
+ # bbox loss
+ pos_bbox_preds = bbox_preds[pos_mask]
+ if pos_mask.sum() > 0:
+ pos_points = points[pos_mask]
+ pos_bbox_preds = bbox_preds[pos_mask]
+ bbox_targets = torch.cat((gt_bboxes.gravity_center, gt_bboxes.tensor[:, 3:]), dim=1)
+ pos_bbox_targets = bbox_targets.to(points.device)[assigned_ids][pos_mask]
+ pos_bbox_targets = torch.cat((
+ pos_bbox_targets[:, :3],
+ pos_bbox_targets[:, 3:6] + self.padding,
+ pos_bbox_targets[:, 6:]), dim=1)
+ if pos_bbox_preds.shape[1] == 6:
+ pos_bbox_targets = pos_bbox_targets[:, :6]
+ bbox_loss = self.bbox_loss(
+ self._bbox_to_loss(
+ self._bbox_pred_to_bbox(pos_points, pos_bbox_preds)),
+ self._bbox_to_loss(pos_bbox_targets))
+ else:
+ bbox_loss = pos_bbox_preds.sum()
+ return bbox_loss, cls_loss
+
+ def _loss(self, bbox_preds, cls_preds, points,
+ gt_bboxes, gt_labels, img_metas):
+ bbox_losses, cls_losses = [], []
+ for i in range(len(img_metas)):
+ bbox_loss, cls_loss = self._loss_single(
+ bbox_preds=[x[i] for x in bbox_preds],
+ cls_preds=[x[i] for x in cls_preds],
+ points=[x[i] for x in points],
+ img_meta=img_metas[i],
+ gt_bboxes=gt_bboxes[i],
+ gt_labels=gt_labels[i])
+ bbox_losses.append(bbox_loss)
+ cls_losses.append(cls_loss)
+ return dict(
+ bbox_loss=torch.mean(torch.stack(bbox_losses)),
+ cls_loss=torch.mean(torch.stack(cls_losses)))
+
+ def forward_train(self, x, gt_bboxes, gt_labels, img_metas):
+ bbox_preds, cls_preds, points = self(x)
+ return self._loss(bbox_preds, cls_preds, points,
+ gt_bboxes, gt_labels, img_metas)
+
+ def _nms(self, bboxes, scores, img_meta):
+ """Multi-class nms for a single scene.
+ Args:
+ bboxes (Tensor): Predicted boxes of shape (N_boxes, 6) or
+ (N_boxes, 7).
+ scores (Tensor): Predicted scores of shape (N_boxes, N_classes).
+ img_meta (dict): Scene meta data.
+ Returns:
+ Tensor: Predicted bboxes.
+ Tensor: Predicted scores.
+ Tensor: Predicted labels.
+ """
+ n_classes = scores.shape[1]
+ yaw_flag = bboxes.shape[1] == 7
+ nms_bboxes, nms_scores, nms_labels = [], [], []
+ for i in range(n_classes):
+ ids = scores[:, i] > self.test_cfg.score_thr
+ if not ids.any():
+ continue
+
+ class_scores = scores[ids, i]
+ class_bboxes = bboxes[ids]
+ if yaw_flag:
+ nms_function = nms3d
+ else:
+ class_bboxes = torch.cat(
+ (class_bboxes, torch.zeros_like(class_bboxes[:, :1])),
+ dim=1)
+ nms_function = nms3d_normal
+
+ nms_ids = nms_function(class_bboxes, class_scores,
+ self.test_cfg.iou_thr)
+ nms_bboxes.append(class_bboxes[nms_ids])
+ nms_scores.append(class_scores[nms_ids])
+ nms_labels.append(
+ bboxes.new_full(
+ class_scores[nms_ids].shape, i, dtype=torch.long))
+
+ if len(nms_bboxes):
+ nms_bboxes = torch.cat(nms_bboxes, dim=0)
+ nms_scores = torch.cat(nms_scores, dim=0)
+ nms_labels = torch.cat(nms_labels, dim=0)
+ else:
+ nms_bboxes = bboxes.new_zeros((0, bboxes.shape[1]))
+ nms_scores = bboxes.new_zeros((0, ))
+ nms_labels = bboxes.new_zeros((0, ))
+
+ if yaw_flag:
+ box_dim = 7
+ with_yaw = True
+ else:
+ box_dim = 6
+ with_yaw = False
+ nms_bboxes = nms_bboxes[:, :6]
+ nms_bboxes = img_meta['box_type_3d'](
+ nms_bboxes,
+ box_dim=box_dim,
+ with_yaw=with_yaw,
+ origin=(.5, .5, .5))
+
+ return nms_bboxes, nms_scores, nms_labels
+
+ def _get_bboxes_single(self, bbox_preds, cls_preds, points, img_meta):
+ scores = torch.cat(cls_preds).sigmoid()
+ bbox_preds = torch.cat(bbox_preds)
+ points = torch.cat(points)
+ max_scores, _ = scores.max(dim=1)
+
+ if len(scores) > self.test_cfg.nms_pre > 0:
+ _, ids = max_scores.topk(self.test_cfg.nms_pre)
+ bbox_preds = bbox_preds[ids]
+ scores = scores[ids]
+ points = points[ids]
+
+ boxes = self._bbox_pred_to_bbox(points, bbox_preds)
+ boxes = torch.cat((
+ boxes[:, :3],
+ boxes[:, 3:6] - self.padding,
+ boxes[:, 6:]), dim=1)
+ boxes, scores, labels = self._nms(boxes, scores, img_meta)
+ return boxes, scores, labels
+
+ def _get_bboxes(self, bbox_preds, cls_preds, points, img_metas):
+ results = []
+ for i in range(len(img_metas)):
+ result = self._get_bboxes_single(
+ bbox_preds=[x[i] for x in bbox_preds],
+ cls_preds=[x[i] for x in cls_preds],
+ points=[x[i] for x in points],
+ img_meta=img_metas[i])
+ results.append(result)
+ return results
+
+ def forward_test(self, x, img_metas):
+ bbox_preds, cls_preds, points = self(x)
+ return self._get_bboxes(bbox_preds, cls_preds, points, img_metas)
+
+
+@BBOX_ASSIGNERS.register_module()
+class NgfcAssigner:
+ def __init__(self, min_pts_threshold, top_pts_threshold, padding):
+ # min_pts_threshold: per level
+ # top_pts_threshold: per box
+ self.min_pts_threshold = min_pts_threshold
+ self.top_pts_threshold = top_pts_threshold
+ self.padding = padding
+
+ @torch.no_grad()
+ def assign(self, points, gt_bboxes):
+ # -> object id or -1 for each point
+ float_max = points[0].new_tensor(1e8)
+ n_levels = len(points)
+ levels = torch.cat([points[i].new_tensor(i, dtype=torch.long).expand(len(points[i]))
+ for i in range(len(points))])
+ points = torch.cat(points)
+ n_points = len(points)
+ n_boxes = len(gt_bboxes)
+ volumes = gt_bboxes.volume.to(points.device).unsqueeze(0).expand(n_points, n_boxes)
+
+ # condition 1: point inside enlarged box
+ boxes = torch.cat((gt_bboxes.gravity_center, gt_bboxes.tensor[:, 3:]), dim=1)
+ boxes = boxes.to(points.device).expand(n_points, n_boxes, 7)
+ boxes = torch.cat((boxes[..., :3], boxes[..., 3:6] + self.padding, boxes[..., 6:]), dim=-1)
+ points = points.unsqueeze(1).expand(n_points, n_boxes, 3)
+ face_distances = get_face_distances(points, boxes)
+ inside_box_condition = face_distances.min(dim=-1).values > 0
+ # print(gt_bboxes.tensor)
+ # for i in range(n_levels):
+ # print(i, inside_box_condition[levels == i].sum(dim=0))
+
+ # condition 2: positive points per level >= limit
+ # calculate positive points per level
+ n_pos_points_per_level = []
+ for i in range(n_levels):
+ n_pos_points_per_level.append(torch.sum(inside_box_condition[levels == i], dim=0))
+ # find best level
+ n_pos_points_per_scale = torch.stack(n_pos_points_per_level, dim=0)
+ lower_limit_mask = n_pos_points_per_scale < self.min_pts_threshold
+ lower_index = torch.argmax(lower_limit_mask.int(), dim=0) - 1
+ lower_index = torch.where(lower_index < 0, 0, lower_index)
+ all_upper_limit_mask = torch.all(torch.logical_not(lower_limit_mask), dim=0)
+ best_level = torch.where(all_upper_limit_mask, n_levels - 1, lower_index)
+ # keep only points with best level
+ best_level = torch.unsqueeze(best_level, 0).expand(n_points, n_boxes)
+ levels = torch.unsqueeze(levels, 1).expand(n_points, n_boxes)
+ level_condition = best_level == levels
+
+ # condition 3: keep topk location per box by center distance
+ center_distances = torch.sum(torch.pow(boxes[..., :3] - points, 2), dim=-1)
+ center_distances = torch.where(inside_box_condition, center_distances, float_max)
+ center_distances = torch.where(level_condition, center_distances, float_max)
+ topk_distances = torch.topk(center_distances,
+ min(self.top_pts_threshold + 1, len(center_distances)),
+ largest=False, dim=0).values[-1]
+ topk_condition = center_distances < topk_distances.unsqueeze(0)
+
+ # condition 4: min volume box per point
+ volumes = torch.where(inside_box_condition, volumes, torch.ones_like(volumes) * float_max)
+ volumes = torch.where(level_condition, volumes, torch.ones_like(volumes) * float_max)
+ volumes = torch.where(topk_condition, volumes, torch.ones_like(volumes) * float_max)
+ min_volumes, min_ids = volumes.min(dim=1)
+ min_inds = torch.where(min_volumes < float_max, min_ids, -1)
+ return min_inds
+
+
+def get_face_distances(points, boxes):
+ # points: of shape (..., 3)
+ # boxes: of shape (..., 7)
+ # -> of shape (..., 6): dx_min, dx_max, dy_min, dy_max, dz_min, dz_max
+ shift = torch.stack((
+ points[..., 0] - boxes[..., 0],
+ points[..., 1] - boxes[..., 1],
+ points[..., 2] - boxes[..., 2]), dim=-1).permute(1, 0, 2)
+ shift = rotation_3d_in_axis(shift, -boxes[0, :, 6], axis=2).permute(1, 0, 2)
+ centers = boxes[..., :3] + shift
+ dx_min = centers[..., 0] - boxes[..., 0] + boxes[..., 3] / 2
+ dx_max = boxes[..., 0] + boxes[..., 3] / 2 - centers[..., 0]
+ dy_min = centers[..., 1] - boxes[..., 1] + boxes[..., 4] / 2
+ dy_max = boxes[..., 1] + boxes[..., 4] / 2 - centers[..., 1]
+ dz_min = centers[..., 2] - boxes[..., 2] + boxes[..., 5] / 2
+ dz_max = boxes[..., 2] + boxes[..., 5] / 2 - centers[..., 2]
+ return torch.stack((dx_min, dx_max, dy_min, dy_max, dz_min, dz_max), dim=-1)
diff --git a/mmdet3d/models/dense_heads/ngfc_head_v2.py b/mmdet3d/models/dense_heads/ngfc_head_v2.py
new file mode 100644
index 0000000..02ee0a8
--- /dev/null
+++ b/mmdet3d/models/dense_heads/ngfc_head_v2.py
@@ -0,0 +1,390 @@
+try:
+ import MinkowskiEngine as ME
+except ImportError:
+ import warnings
+ warnings.warn(
+ 'Please follow `getting_started.md` to install MinkowskiEngine.`')
+
+import torch
+from torch import nn
+
+from mmcv.runner import BaseModule
+from mmcv.cnn import Scale, bias_init_with_prob
+from mmcv.ops import nms3d, nms3d_normal
+from mmdet.core.bbox.builder import BBOX_ASSIGNERS, build_assigner
+from mmdet3d.models.builder import HEADS, build_loss
+from mmdet3d.core.bbox.structures import rotation_3d_in_axis
+
+
+@HEADS.register_module()
+class NgfcV2Head(BaseModule):
+ def __init__(self,
+ n_classes,
+ in_channels,
+ n_levels,
+ n_reg_outs,
+ padding,
+ voxel_size,
+ assigner,
+ bbox_loss=dict(type='AxisAlignedIoULoss'),
+ cls_loss=dict(type='FocalLoss'),
+ train_cfg=None,
+ test_cfg=None):
+ super(NgfcV2Head, self).__init__()
+ self.padding = padding
+ self.voxel_size = voxel_size
+ self.assigner = build_assigner(assigner)
+ self.bbox_loss = build_loss(bbox_loss)
+ self.cls_loss = build_loss(cls_loss)
+ self.train_cfg = train_cfg
+ self.test_cfg = test_cfg
+ self._init_layers(n_classes, in_channels, n_levels, n_reg_outs)
+
+ def _init_layers(self, n_classes, in_channels, n_levels, n_reg_outs):
+ for i in range(n_levels):
+ self.__setattr__(f'scale_{i}', Scale(1.))
+ self.bbox_conv = ME.MinkowskiConvolution(
+ in_channels, n_reg_outs, kernel_size=1, bias=True, dimension=3)
+ self.cls_conv = ME.MinkowskiConvolution(
+ in_channels, n_classes, kernel_size=1, bias=True, dimension=3)
+
+ def init_weights(self):
+ nn.init.normal_(self.bbox_conv.kernel, std=.01)
+ nn.init.normal_(self.cls_conv.kernel, std=.01)
+ nn.init.constant_(self.cls_conv.bias, bias_init_with_prob(.01))
+
+ # per level
+ def _forward_single(self, x, scale):
+ reg_final = self.bbox_conv(x).features
+ reg_distance = torch.exp(scale(reg_final[:, :6]))
+ reg_angle = reg_final[:, 6:]
+ bbox_pred = torch.cat((reg_distance, reg_angle), dim=1)
+ cls_pred = self.cls_conv(x).features
+
+ bbox_preds, cls_preds, points = [], [], []
+ for permutation in x.decomposition_permutations:
+ bbox_preds.append(bbox_pred[permutation])
+ cls_preds.append(cls_pred[permutation])
+ points.append(x.coordinates[permutation][:, 1:] * self.voxel_size)
+
+ return bbox_preds, cls_preds, points
+
+ def forward(self, x):
+ bbox_preds, cls_preds, points = [], [], []
+ for i in range(len(x)):
+ bbox_pred, cls_pred, point = self._forward_single(
+ x[i], self.__getattr__(f'scale_{i}'))
+ bbox_preds.append(bbox_pred)
+ cls_preds.append(cls_pred)
+ points.append(point)
+ return bbox_preds, cls_preds, points
+
+ @staticmethod
+ def _bbox_to_loss(bbox):
+ """Transform box to the axis-aligned or rotated iou loss format.
+ Args:
+ bbox (Tensor): 3D box of shape (N, 6) or (N, 7).
+ Returns:
+ Tensor: Transformed 3D box of shape (N, 6) or (N, 7).
+ """
+ # rotated iou loss accepts (x, y, z, w, h, l, heading)
+ if bbox.shape[-1] != 6:
+ return bbox
+
+ # axis-aligned case: x, y, z, w, h, l -> x1, y1, z1, x2, y2, z2
+ return torch.stack(
+ (bbox[..., 0] - bbox[..., 3] / 2, bbox[..., 1] - bbox[..., 4] / 2,
+ bbox[..., 2] - bbox[..., 5] / 2, bbox[..., 0] + bbox[..., 3] / 2,
+ bbox[..., 1] + bbox[..., 4] / 2, bbox[..., 2] + bbox[..., 5] / 2),
+ dim=-1)
+
+ @staticmethod
+ def _bbox_pred_to_bbox(points, bbox_pred):
+ """Transform predicted bbox parameters to bbox.
+ Args:
+ points (Tensor): Final locations of shape (N, 3)
+ bbox_pred (Tensor): Predicted bbox parameters of shape (N, 6)
+ or (N, 8).
+ Returns:
+ Tensor: Transformed 3D box of shape (N, 6) or (N, 7).
+ """
+ if bbox_pred.shape[0] == 0:
+ return bbox_pred
+
+ x_center = points[:, 0] + (bbox_pred[:, 1] - bbox_pred[:, 0]) / 2
+ y_center = points[:, 1] + (bbox_pred[:, 3] - bbox_pred[:, 2]) / 2
+ z_center = points[:, 2] + (bbox_pred[:, 5] - bbox_pred[:, 4]) / 2
+
+ # dx_min, dx_max, dy_min, dy_max, dz_min, dz_max -> x, y, z, w, l, h
+ base_bbox = torch.stack([
+ x_center,
+ y_center,
+ z_center,
+ bbox_pred[:, 0] + bbox_pred[:, 1],
+ bbox_pred[:, 2] + bbox_pred[:, 3],
+ bbox_pred[:, 4] + bbox_pred[:, 5],
+ ], -1)
+
+ # axis-aligned case
+ if bbox_pred.shape[1] == 6:
+ return base_bbox
+
+ # rotated case: ..., sin(2a)ln(q), cos(2a)ln(q)
+ scale = bbox_pred[:, 0] + bbox_pred[:, 1] + \
+ bbox_pred[:, 2] + bbox_pred[:, 3]
+ q = torch.exp(
+ torch.sqrt(
+ torch.pow(bbox_pred[:, 6], 2) + torch.pow(bbox_pred[:, 7], 2)))
+ alpha = 0.5 * torch.atan2(bbox_pred[:, 6], bbox_pred[:, 7])
+ return torch.stack(
+ (x_center, y_center, z_center, scale / (1 + q), scale /
+ (1 + q) * q, bbox_pred[:, 5] + bbox_pred[:, 4], alpha),
+ dim=-1)
+
+ # per scene
+ def _loss_single(self,
+ bbox_preds,
+ cls_preds,
+ points,
+ gt_bboxes,
+ gt_labels,
+ img_meta):
+ assigned_ids = self.assigner.assign(points, gt_bboxes, img_meta)
+ bbox_preds = torch.cat(bbox_preds)
+ cls_preds = torch.cat(cls_preds)
+ points = torch.cat(points)
+
+ # cls loss
+ n_classes = cls_preds.shape[1]
+ pos_mask = assigned_ids >= 0
+ cls_targets = torch.where(pos_mask, gt_labels[assigned_ids], n_classes)
+ avg_factor = max(pos_mask.sum(), 1)
+ cls_loss = self.cls_loss(cls_preds, cls_targets, avg_factor=avg_factor)
+
+ # bbox loss
+ pos_bbox_preds = bbox_preds[pos_mask]
+ if pos_mask.sum() > 0:
+ pos_points = points[pos_mask]
+ pos_bbox_preds = bbox_preds[pos_mask]
+ bbox_targets = torch.cat((gt_bboxes.gravity_center, gt_bboxes.tensor[:, 3:]), dim=1)
+ pos_bbox_targets = bbox_targets.to(points.device)[assigned_ids][pos_mask]
+ pos_bbox_targets = torch.cat((
+ pos_bbox_targets[:, :3],
+ pos_bbox_targets[:, 3:6] + self.padding,
+ pos_bbox_targets[:, 6:]), dim=1)
+ if pos_bbox_preds.shape[1] == 6:
+ pos_bbox_targets = pos_bbox_targets[:, :6]
+ bbox_loss = self.bbox_loss(
+ self._bbox_to_loss(
+ self._bbox_pred_to_bbox(pos_points, pos_bbox_preds)),
+ self._bbox_to_loss(pos_bbox_targets))
+ else:
+ bbox_loss = pos_bbox_preds.sum()
+ return bbox_loss, cls_loss
+
+ def _loss(self, bbox_preds, cls_preds, points,
+ gt_bboxes, gt_labels, img_metas):
+ bbox_losses, cls_losses = [], []
+ for i in range(len(img_metas)):
+ bbox_loss, cls_loss = self._loss_single(
+ bbox_preds=[x[i] for x in bbox_preds],
+ cls_preds=[x[i] for x in cls_preds],
+ points=[x[i] for x in points],
+ img_meta=img_metas[i],
+ gt_bboxes=gt_bboxes[i],
+ gt_labels=gt_labels[i])
+ bbox_losses.append(bbox_loss)
+ cls_losses.append(cls_loss)
+ return dict(
+ bbox_loss=torch.mean(torch.stack(bbox_losses)),
+ cls_loss=torch.mean(torch.stack(cls_losses)))
+
+ def forward_train(self, x, gt_bboxes, gt_labels, img_metas):
+ bbox_preds, cls_preds, points = self(x)
+ return self._loss(bbox_preds, cls_preds, points,
+ gt_bboxes, gt_labels, img_metas)
+
+ def _nms(self, bboxes, scores, img_meta):
+ """Multi-class nms for a single scene.
+ Args:
+ bboxes (Tensor): Predicted boxes of shape (N_boxes, 6) or
+ (N_boxes, 7).
+ scores (Tensor): Predicted scores of shape (N_boxes, N_classes).
+ img_meta (dict): Scene meta data.
+ Returns:
+ Tensor: Predicted bboxes.
+ Tensor: Predicted scores.
+ Tensor: Predicted labels.
+ """
+ n_classes = scores.shape[1]
+ yaw_flag = bboxes.shape[1] == 7
+ nms_bboxes, nms_scores, nms_labels = [], [], []
+ for i in range(n_classes):
+ ids = scores[:, i] > self.test_cfg.score_thr
+ if not ids.any():
+ continue
+
+ class_scores = scores[ids, i]
+ class_bboxes = bboxes[ids]
+ if yaw_flag:
+ nms_function = nms3d
+ else:
+ class_bboxes = torch.cat(
+ (class_bboxes, torch.zeros_like(class_bboxes[:, :1])),
+ dim=1)
+ nms_function = nms3d_normal
+
+ nms_ids = nms_function(class_bboxes, class_scores,
+ self.test_cfg.iou_thr)
+ nms_bboxes.append(class_bboxes[nms_ids])
+ nms_scores.append(class_scores[nms_ids])
+ nms_labels.append(
+ bboxes.new_full(
+ class_scores[nms_ids].shape, i, dtype=torch.long))
+
+ if len(nms_bboxes):
+ nms_bboxes = torch.cat(nms_bboxes, dim=0)
+ nms_scores = torch.cat(nms_scores, dim=0)
+ nms_labels = torch.cat(nms_labels, dim=0)
+ else:
+ nms_bboxes = bboxes.new_zeros((0, bboxes.shape[1]))
+ nms_scores = bboxes.new_zeros((0, ))
+ nms_labels = bboxes.new_zeros((0, ))
+
+ if yaw_flag:
+ box_dim = 7
+ with_yaw = True
+ else:
+ box_dim = 6
+ with_yaw = False
+ nms_bboxes = nms_bboxes[:, :6]
+ nms_bboxes = img_meta['box_type_3d'](
+ nms_bboxes,
+ box_dim=box_dim,
+ with_yaw=with_yaw,
+ origin=(.5, .5, .5))
+
+ return nms_bboxes, nms_scores, nms_labels
+
+ def _get_bboxes_single(self, bbox_preds, cls_preds, points, img_meta):
+ scores = torch.cat(cls_preds).sigmoid()
+ bbox_preds = torch.cat(bbox_preds)
+ points = torch.cat(points)
+ max_scores, _ = scores.max(dim=1)
+
+ if len(scores) > self.test_cfg.nms_pre > 0:
+ _, ids = max_scores.topk(self.test_cfg.nms_pre)
+ bbox_preds = bbox_preds[ids]
+ scores = scores[ids]
+ points = points[ids]
+
+ boxes = self._bbox_pred_to_bbox(points, bbox_preds)
+ boxes = torch.cat((
+ boxes[:, :3],
+ boxes[:, 3:6] - self.padding,
+ boxes[:, 6:]), dim=1)
+ boxes, scores, labels = self._nms(boxes, scores, img_meta)
+ return boxes, scores, labels
+
+ def _get_bboxes(self, bbox_preds, cls_preds, points, img_metas):
+ results = []
+ for i in range(len(img_metas)):
+ result = self._get_bboxes_single(
+ bbox_preds=[x[i] for x in bbox_preds],
+ cls_preds=[x[i] for x in cls_preds],
+ points=[x[i] for x in points],
+ img_meta=img_metas[i])
+ results.append(result)
+ return results
+
+ def forward_test(self, x, img_metas):
+ bbox_preds, cls_preds, points = self(x)
+ return self._get_bboxes(bbox_preds, cls_preds, points, img_metas)
+
+
+@BBOX_ASSIGNERS.register_module()
+class NgfcV2Assigner:
+ def __init__(self, min_pts_threshold, top_pts_threshold, padding):
+ # min_pts_threshold: per level
+ # top_pts_threshold: per box
+ self.min_pts_threshold = min_pts_threshold
+ self.top_pts_threshold = top_pts_threshold
+ self.padding = padding
+
+ @torch.no_grad()
+ def assign(self, points, gt_bboxes, img_meta):
+ # -> object id or -1 for each point
+ float_max = points[0].new_tensor(1e8)
+ n_levels = len(points)
+ levels = torch.cat([points[i].new_tensor(i, dtype=torch.long).expand(len(points[i]))
+ for i in range(len(points))])
+ points = torch.cat(points)
+ n_points = len(points)
+ n_boxes = len(gt_bboxes)
+ volumes = gt_bboxes.volume.to(points.device).unsqueeze(0).expand(n_points, n_boxes)
+
+ # condition 1: point inside enlarged box
+ boxes = torch.cat((gt_bboxes.gravity_center, gt_bboxes.tensor[:, 3:]), dim=1)
+ boxes = boxes.to(points.device).expand(n_points, n_boxes, 7)
+ boxes = torch.cat((boxes[..., :3], boxes[..., 3:6] + self.padding, boxes[..., 6:]), dim=-1)
+ points = points.unsqueeze(1).expand(n_points, n_boxes, 3)
+ face_distances = get_face_distances(points, boxes)
+ inside_box_condition = face_distances.min(dim=-1).values > 0
+ # print(gt_bboxes.tensor)
+ # for i in range(n_levels):
+ # print(i, inside_box_condition[levels == i].sum(dim=0))
+
+ # condition 2: positive points per level >= limit
+ # calculate positive points per level
+ n_pos_points_per_level = []
+ for i in range(n_levels):
+ n_pos_points_per_level.append(torch.sum(inside_box_condition[levels == i], dim=0))
+ # find best level
+ n_pos_points_per_scale = torch.stack(n_pos_points_per_level, dim=0)
+ lower_limit_mask = n_pos_points_per_scale < self.min_pts_threshold
+ lower_index = torch.argmax(lower_limit_mask.int(), dim=0) - 1
+ lower_index = torch.where(lower_index < 0, 0, lower_index)
+ all_upper_limit_mask = torch.all(torch.logical_not(lower_limit_mask), dim=0)
+ best_level = torch.where(all_upper_limit_mask, n_levels - 1, lower_index)
+ # keep only points with best level
+ best_level = torch.unsqueeze(best_level, 0).expand(n_points, n_boxes)
+ levels = torch.unsqueeze(levels, 1).expand(n_points, n_boxes)
+ level_condition = best_level == levels
+
+ # condition 3: keep topk location per box by center distance
+ center = boxes[..., :3]
+ center_distances = torch.sum(torch.pow(center - points, 2), dim=-1)
+ center_distances = torch.where(inside_box_condition, center_distances, float_max)
+ center_distances = torch.where(level_condition, center_distances, float_max)
+ topk_distances = torch.topk(center_distances,
+ min(self.top_pts_threshold + 1, len(center_distances)),
+ largest=False, dim=0).values[-1]
+ topk_condition = center_distances < topk_distances.unsqueeze(0)
+
+ # condition 4: min volume box per point
+ volumes = torch.where(inside_box_condition, volumes, torch.ones_like(volumes) * float_max)
+ volumes = torch.where(level_condition, volumes, torch.ones_like(volumes) * float_max)
+ volumes = torch.where(topk_condition, volumes, torch.ones_like(volumes) * float_max)
+ min_volumes, min_ids = volumes.min(dim=1)
+ min_inds = torch.where(min_volumes < float_max, min_ids, -1)
+ # print(gt_bboxes.tensor.shape, torch.unique(min_inds, return_counts=True))
+ return min_inds
+
+
+def get_face_distances(points, boxes):
+ # points: of shape (..., 3)
+ # boxes: of shape (..., 7)
+ # -> of shape (..., 6): dx_min, dx_max, dy_min, dy_max, dz_min, dz_max
+ shift = torch.stack((
+ points[..., 0] - boxes[..., 0],
+ points[..., 1] - boxes[..., 1],
+ points[..., 2] - boxes[..., 2]), dim=-1).permute(1, 0, 2)
+ shift = rotation_3d_in_axis(shift, -boxes[0, :, 6], axis=2).permute(1, 0, 2)
+ centers = boxes[..., :3] + shift
+ dx_min = centers[..., 0] - boxes[..., 0] + boxes[..., 3] / 2
+ dx_max = boxes[..., 0] + boxes[..., 3] / 2 - centers[..., 0]
+ dy_min = centers[..., 1] - boxes[..., 1] + boxes[..., 4] / 2
+ dy_max = boxes[..., 1] + boxes[..., 4] / 2 - centers[..., 1]
+ dz_min = centers[..., 2] - boxes[..., 2] + boxes[..., 5] / 2
+ dz_max = boxes[..., 2] + boxes[..., 5] / 2 - centers[..., 2]
+ return torch.stack((dx_min, dx_max, dy_min, dy_max, dz_min, dz_max), dim=-1)
diff --git a/mmdet3d/models/dense_heads/parta2_rpn_head.py b/mmdet3d/models/dense_heads/parta2_rpn_head.py
new file mode 100644
index 0000000..a57e1a1
--- /dev/null
+++ b/mmdet3d/models/dense_heads/parta2_rpn_head.py
@@ -0,0 +1,310 @@
+# Copyright (c) OpenMMLab. All rights reserved.
+import numpy as np
+import torch
+from mmcv.runner import force_fp32
+
+from mmdet3d.core import limit_period, xywhr2xyxyr
+from mmdet3d.core.post_processing import nms_bev, nms_normal_bev
+from ..builder import HEADS
+from .anchor3d_head import Anchor3DHead
+
+
+@HEADS.register_module()
+class PartA2RPNHead(Anchor3DHead):
+ """RPN head for PartA2.
+
+ Note:
+ The main difference between the PartA2 RPN head and the Anchor3DHead
+ lies in their output during inference. PartA2 RPN head further returns
+ the original classification score for the second stage since the bbox
+ head in RoI head does not do classification task.
+
+ Different from RPN heads in 2D detectors, this RPN head does
+ multi-class classification task and uses FocalLoss like the SECOND and
+ PointPillars do. But this head uses class agnostic nms rather than
+ multi-class nms.
+
+ Args:
+ num_classes (int): Number of classes.
+ in_channels (int): Number of channels in the input feature map.
+ train_cfg (dict): Train configs.
+ test_cfg (dict): Test configs.
+ feat_channels (int): Number of channels of the feature map.
+ use_direction_classifier (bool): Whether to add a direction classifier.
+ anchor_generator(dict): Config dict of anchor generator.
+ assigner_per_size (bool): Whether to do assignment for each separate
+ anchor size.
+ assign_per_class (bool): Whether to do assignment for each class.
+ diff_rad_by_sin (bool): Whether to change the difference into sin
+ difference for box regression loss.
+ dir_offset (float | int): The offset of BEV rotation angles
+ (TODO: may be moved into box coder)
+ dir_limit_offset (float | int): The limited range of BEV
+ rotation angles. (TODO: may be moved into box coder)
+ bbox_coder (dict): Config dict of box coders.
+ loss_cls (dict): Config of classification loss.
+ loss_bbox (dict): Config of localization loss.
+ loss_dir (dict): Config of direction classifier loss.
+ """
+
+ def __init__(self,
+ num_classes,
+ in_channels,
+ train_cfg,
+ test_cfg,
+ feat_channels=256,
+ use_direction_classifier=True,
+ anchor_generator=dict(
+ type='Anchor3DRangeGenerator',
+ range=[0, -39.68, -1.78, 69.12, 39.68, -1.78],
+ strides=[2],
+ sizes=[[3.9, 1.6, 1.56]],
+ rotations=[0, 1.57],
+ custom_values=[],
+ reshape_out=False),
+ assigner_per_size=False,
+ assign_per_class=False,
+ diff_rad_by_sin=True,
+ dir_offset=-np.pi / 2,
+ dir_limit_offset=0,
+ bbox_coder=dict(type='DeltaXYZWLHRBBoxCoder'),
+ loss_cls=dict(
+ type='CrossEntropyLoss',
+ use_sigmoid=True,
+ loss_weight=1.0),
+ loss_bbox=dict(
+ type='SmoothL1Loss', beta=1.0 / 9.0, loss_weight=2.0),
+ loss_dir=dict(type='CrossEntropyLoss', loss_weight=0.2),
+ init_cfg=None):
+ super().__init__(num_classes, in_channels, train_cfg, test_cfg,
+ feat_channels, use_direction_classifier,
+ anchor_generator, assigner_per_size, assign_per_class,
+ diff_rad_by_sin, dir_offset, dir_limit_offset,
+ bbox_coder, loss_cls, loss_bbox, loss_dir, init_cfg)
+
+ @force_fp32(apply_to=('cls_scores', 'bbox_preds', 'dir_cls_preds'))
+ def loss(self,
+ cls_scores,
+ bbox_preds,
+ dir_cls_preds,
+ gt_bboxes,
+ gt_labels,
+ input_metas,
+ gt_bboxes_ignore=None):
+ """Calculate losses.
+
+ Args:
+ cls_scores (list[torch.Tensor]): Multi-level class scores.
+ bbox_preds (list[torch.Tensor]): Multi-level bbox predictions.
+ dir_cls_preds (list[torch.Tensor]): Multi-level direction
+ class predictions.
+ gt_bboxes (list[:obj:`BaseInstance3DBoxes`]): Ground truth boxes
+ of each sample.
+ gt_labels (list[torch.Tensor]): Labels of each sample.
+ input_metas (list[dict]): Point cloud and image's meta info.
+ gt_bboxes_ignore (list[torch.Tensor]): Specify
+ which bounding.
+
+ Returns:
+ dict[str, list[torch.Tensor]]: Classification, bbox, and
+ direction losses of each level.
+
+ - loss_rpn_cls (list[torch.Tensor]): Classification losses.
+ - loss_rpn_bbox (list[torch.Tensor]): Box regression losses.
+ - loss_rpn_dir (list[torch.Tensor]): Direction classification
+ losses.
+ """
+ loss_dict = super().loss(cls_scores, bbox_preds, dir_cls_preds,
+ gt_bboxes, gt_labels, input_metas,
+ gt_bboxes_ignore)
+ # change the loss key names to avoid conflict
+ return dict(
+ loss_rpn_cls=loss_dict['loss_cls'],
+ loss_rpn_bbox=loss_dict['loss_bbox'],
+ loss_rpn_dir=loss_dict['loss_dir'])
+
+ def get_bboxes_single(self,
+ cls_scores,
+ bbox_preds,
+ dir_cls_preds,
+ mlvl_anchors,
+ input_meta,
+ cfg,
+ rescale=False):
+ """Get bboxes of single branch.
+
+ Args:
+ cls_scores (torch.Tensor): Class score in single batch.
+ bbox_preds (torch.Tensor): Bbox prediction in single batch.
+ dir_cls_preds (torch.Tensor): Predictions of direction class
+ in single batch.
+ mlvl_anchors (List[torch.Tensor]): Multi-level anchors
+ in single batch.
+ input_meta (list[dict]): Contain pcd and img's meta info.
+ cfg (:obj:`ConfigDict`): Training or testing config.
+ rescale (list[torch.Tensor]): whether th rescale bbox.
+
+ Returns:
+ dict: Predictions of single batch containing the following keys:
+
+ - boxes_3d (:obj:`BaseInstance3DBoxes`): Predicted 3d bboxes.
+ - scores_3d (torch.Tensor): Score of each bbox.
+ - labels_3d (torch.Tensor): Label of each bbox.
+ - cls_preds (torch.Tensor): Class score of each bbox.
+ """
+ assert len(cls_scores) == len(bbox_preds) == len(mlvl_anchors)
+ mlvl_bboxes = []
+ mlvl_max_scores = []
+ mlvl_label_pred = []
+ mlvl_dir_scores = []
+ mlvl_cls_score = []
+ for cls_score, bbox_pred, dir_cls_pred, anchors in zip(
+ cls_scores, bbox_preds, dir_cls_preds, mlvl_anchors):
+ assert cls_score.size()[-2:] == bbox_pred.size()[-2:]
+ assert cls_score.size()[-2:] == dir_cls_pred.size()[-2:]
+ dir_cls_pred = dir_cls_pred.permute(1, 2, 0).reshape(-1, 2)
+ dir_cls_score = torch.max(dir_cls_pred, dim=-1)[1]
+
+ cls_score = cls_score.permute(1, 2,
+ 0).reshape(-1, self.num_classes)
+
+ if self.use_sigmoid_cls:
+ scores = cls_score.sigmoid()
+ else:
+ scores = cls_score.softmax(-1)
+ bbox_pred = bbox_pred.permute(1, 2,
+ 0).reshape(-1, self.box_code_size)
+
+ nms_pre = cfg.get('nms_pre', -1)
+ if self.use_sigmoid_cls:
+ max_scores, pred_labels = scores.max(dim=1)
+ else:
+ max_scores, pred_labels = scores[:, :-1].max(dim=1)
+ # get topk
+ if nms_pre > 0 and scores.shape[0] > nms_pre:
+ topk_scores, topk_inds = max_scores.topk(nms_pre)
+ anchors = anchors[topk_inds, :]
+ bbox_pred = bbox_pred[topk_inds, :]
+ max_scores = topk_scores
+ cls_score = scores[topk_inds, :]
+ dir_cls_score = dir_cls_score[topk_inds]
+ pred_labels = pred_labels[topk_inds]
+
+ bboxes = self.bbox_coder.decode(anchors, bbox_pred)
+ mlvl_bboxes.append(bboxes)
+ mlvl_max_scores.append(max_scores)
+ mlvl_cls_score.append(cls_score)
+ mlvl_label_pred.append(pred_labels)
+ mlvl_dir_scores.append(dir_cls_score)
+
+ mlvl_bboxes = torch.cat(mlvl_bboxes)
+ mlvl_bboxes_for_nms = xywhr2xyxyr(input_meta['box_type_3d'](
+ mlvl_bboxes, box_dim=self.box_code_size).bev)
+ mlvl_max_scores = torch.cat(mlvl_max_scores)
+ mlvl_label_pred = torch.cat(mlvl_label_pred)
+ mlvl_dir_scores = torch.cat(mlvl_dir_scores)
+ # shape [k, num_class] before sigmoid
+ # PartA2 need to keep raw classification score
+ # because the bbox head in the second stage does not have
+ # classification branch,
+ # roi head need this score as classification score
+ mlvl_cls_score = torch.cat(mlvl_cls_score)
+
+ score_thr = cfg.get('score_thr', 0)
+ result = self.class_agnostic_nms(mlvl_bboxes, mlvl_bboxes_for_nms,
+ mlvl_max_scores, mlvl_label_pred,
+ mlvl_cls_score, mlvl_dir_scores,
+ score_thr, cfg.nms_post, cfg,
+ input_meta)
+
+ return result
+
+ def class_agnostic_nms(self, mlvl_bboxes, mlvl_bboxes_for_nms,
+ mlvl_max_scores, mlvl_label_pred, mlvl_cls_score,
+ mlvl_dir_scores, score_thr, max_num, cfg,
+ input_meta):
+ """Class agnostic nms for single batch.
+
+ Args:
+ mlvl_bboxes (torch.Tensor): Bboxes from Multi-level.
+ mlvl_bboxes_for_nms (torch.Tensor): Bboxes for nms
+ (bev or minmax boxes) from Multi-level.
+ mlvl_max_scores (torch.Tensor): Max scores of Multi-level bbox.
+ mlvl_label_pred (torch.Tensor): Class predictions
+ of Multi-level bbox.
+ mlvl_cls_score (torch.Tensor): Class scores of
+ Multi-level bbox.
+ mlvl_dir_scores (torch.Tensor): Direction scores of
+ Multi-level bbox.
+ score_thr (int): Score threshold.
+ max_num (int): Max number of bboxes after nms.
+ cfg (:obj:`ConfigDict`): Training or testing config.
+ input_meta (dict): Contain pcd and img's meta info.
+
+ Returns:
+ dict: Predictions of single batch. Contain the keys:
+
+ - boxes_3d (:obj:`BaseInstance3DBoxes`): Predicted 3d bboxes.
+ - scores_3d (torch.Tensor): Score of each bbox.
+ - labels_3d (torch.Tensor): Label of each bbox.
+ - cls_preds (torch.Tensor): Class score of each bbox.
+ """
+ bboxes = []
+ scores = []
+ labels = []
+ dir_scores = []
+ cls_scores = []
+ score_thr_inds = mlvl_max_scores > score_thr
+ _scores = mlvl_max_scores[score_thr_inds]
+ _bboxes_for_nms = mlvl_bboxes_for_nms[score_thr_inds, :]
+ if cfg.use_rotate_nms:
+ nms_func = nms_bev
+ else:
+ nms_func = nms_normal_bev
+ selected = nms_func(_bboxes_for_nms, _scores, cfg.nms_thr)
+
+ _mlvl_bboxes = mlvl_bboxes[score_thr_inds, :]
+ _mlvl_dir_scores = mlvl_dir_scores[score_thr_inds]
+ _mlvl_label_pred = mlvl_label_pred[score_thr_inds]
+ _mlvl_cls_score = mlvl_cls_score[score_thr_inds]
+
+ if len(selected) > 0:
+ bboxes.append(_mlvl_bboxes[selected])
+ scores.append(_scores[selected])
+ labels.append(_mlvl_label_pred[selected])
+ cls_scores.append(_mlvl_cls_score[selected])
+ dir_scores.append(_mlvl_dir_scores[selected])
+ dir_rot = limit_period(bboxes[-1][..., 6] - self.dir_offset,
+ self.dir_limit_offset, np.pi)
+ bboxes[-1][..., 6] = (
+ dir_rot + self.dir_offset +
+ np.pi * dir_scores[-1].to(bboxes[-1].dtype))
+
+ if bboxes:
+ bboxes = torch.cat(bboxes, dim=0)
+ scores = torch.cat(scores, dim=0)
+ cls_scores = torch.cat(cls_scores, dim=0)
+ labels = torch.cat(labels, dim=0)
+ if bboxes.shape[0] > max_num:
+ _, inds = scores.sort(descending=True)
+ inds = inds[:max_num]
+ bboxes = bboxes[inds, :]
+ labels = labels[inds]
+ scores = scores[inds]
+ cls_scores = cls_scores[inds]
+ bboxes = input_meta['box_type_3d'](
+ bboxes, box_dim=self.box_code_size)
+ return dict(
+ boxes_3d=bboxes,
+ scores_3d=scores,
+ labels_3d=labels,
+ cls_preds=cls_scores # raw scores [max_num, cls_num]
+ )
+ else:
+ return dict(
+ boxes_3d=input_meta['box_type_3d'](
+ mlvl_bboxes.new_zeros([0, self.box_code_size]),
+ box_dim=self.box_code_size),
+ scores_3d=mlvl_bboxes.new_zeros([0]),
+ labels_3d=mlvl_bboxes.new_zeros([0]),
+ cls_preds=mlvl_bboxes.new_zeros([0, mlvl_cls_score.shape[-1]]))
diff --git a/mmdet3d/models/dense_heads/pgd_head.py b/mmdet3d/models/dense_heads/pgd_head.py
new file mode 100644
index 0000000..d9bfadb
--- /dev/null
+++ b/mmdet3d/models/dense_heads/pgd_head.py
@@ -0,0 +1,1229 @@
+# Copyright (c) OpenMMLab. All rights reserved.
+import numpy as np
+import torch
+from mmcv.cnn import Scale, bias_init_with_prob, normal_init
+from mmcv.runner import force_fp32
+from torch import nn as nn
+from torch.nn import functional as F
+
+from mmdet3d.core import box3d_multiclass_nms, xywhr2xyxyr
+from mmdet3d.core.bbox import points_cam2img, points_img2cam
+from mmdet.core import distance2bbox, multi_apply
+from ..builder import HEADS, build_loss
+from .fcos_mono3d_head import FCOSMono3DHead
+
+
+@HEADS.register_module()
+class PGDHead(FCOSMono3DHead):
+ r"""Anchor-free head used in `PGD `_.
+
+ Args:
+ use_depth_classifer (bool, optional): Whether to use depth classifier.
+ Defaults to True.
+ use_only_reg_proj (bool, optional): Whether to use only direct
+ regressed depth in the re-projection (to make the network easier
+ to learn). Defaults to False.
+ weight_dim (int, optional): Dimension of the location-aware weight
+ map. Defaults to -1.
+ weight_branch (tuple[tuple[int]], optional): Feature map channels of
+ the convolutional branch for weight map. Defaults to ((256, ), ).
+ depth_branch (tuple[int], optional): Feature map channels of the
+ branch for probabilistic depth estimation. Defaults to (64, ),
+ depth_range (tuple[float], optional): Range of depth estimation.
+ Defaults to (0, 70),
+ depth_unit (int, optional): Unit of depth range division. Defaults to
+ 10.
+ division (str, optional): Depth division method. Options include
+ 'uniform', 'linear', 'log', 'loguniform'. Defaults to 'uniform'.
+ depth_bins (int, optional): Discrete bins of depth division. Defaults
+ to 8.
+ loss_depth (dict, optional): Depth loss. Defaults to dict(
+ type='SmoothL1Loss', beta=1.0 / 9.0, loss_weight=1.0).
+ loss_bbox2d (dict, optional): Loss for 2D box estimation. Defaults to
+ dict(type='SmoothL1Loss', beta=1.0 / 9.0, loss_weight=1.0).
+ loss_consistency (dict, optional): Consistency loss. Defaults to
+ dict(type='GIoULoss', loss_weight=1.0),
+ pred_velo (bool, optional): Whether to predict velocity. Defaults to
+ False.
+ pred_bbox2d (bool, optional): Whether to predict 2D bounding boxes.
+ Defaults to True.
+ pred_keypoints (bool, optional): Whether to predict keypoints.
+ Defaults to False,
+ bbox_coder (dict, optional): Bounding box coder. Defaults to
+ dict(type='PGDBBoxCoder', base_depths=((28.01, 16.32), ),
+ base_dims=((0.8, 1.73, 0.6), (1.76, 1.73, 0.6), (3.9, 1.56, 1.6)),
+ code_size=7).
+ """
+
+ def __init__(self,
+ use_depth_classifier=True,
+ use_onlyreg_proj=False,
+ weight_dim=-1,
+ weight_branch=((256, ), ),
+ depth_branch=(64, ),
+ depth_range=(0, 70),
+ depth_unit=10,
+ division='uniform',
+ depth_bins=8,
+ loss_depth=dict(
+ type='SmoothL1Loss', beta=1.0 / 9.0, loss_weight=1.0),
+ loss_bbox2d=dict(
+ type='SmoothL1Loss', beta=1.0 / 9.0, loss_weight=1.0),
+ loss_consistency=dict(type='GIoULoss', loss_weight=1.0),
+ pred_bbox2d=True,
+ pred_keypoints=False,
+ bbox_coder=dict(
+ type='PGDBBoxCoder',
+ base_depths=((28.01, 16.32), ),
+ base_dims=((0.8, 1.73, 0.6), (1.76, 1.73, 0.6),
+ (3.9, 1.56, 1.6)),
+ code_size=7),
+ **kwargs):
+ self.use_depth_classifier = use_depth_classifier
+ self.use_onlyreg_proj = use_onlyreg_proj
+ self.depth_branch = depth_branch
+ self.pred_keypoints = pred_keypoints
+ self.weight_dim = weight_dim
+ self.weight_branch = weight_branch
+ self.weight_out_channels = []
+ for weight_branch_channels in weight_branch:
+ if len(weight_branch_channels) > 0:
+ self.weight_out_channels.append(weight_branch_channels[-1])
+ else:
+ self.weight_out_channels.append(-1)
+ self.depth_range = depth_range
+ self.depth_unit = depth_unit
+ self.division = division
+ if self.division == 'uniform':
+ self.num_depth_cls = int(
+ (depth_range[1] - depth_range[0]) / depth_unit) + 1
+ if self.num_depth_cls != depth_bins:
+ print('Warning: The number of bins computed from ' +
+ 'depth_unit is different from given parameter! ' +
+ 'Depth_unit will be considered with priority in ' +
+ 'Uniform Division.')
+ else:
+ self.num_depth_cls = depth_bins
+ super().__init__(
+ pred_bbox2d=pred_bbox2d, bbox_coder=bbox_coder, **kwargs)
+ self.loss_depth = build_loss(loss_depth)
+ if self.pred_bbox2d:
+ self.loss_bbox2d = build_loss(loss_bbox2d)
+ self.loss_consistency = build_loss(loss_consistency)
+ if self.pred_keypoints:
+ self.kpts_start = 9 if self.pred_velo else 7
+
+ def _init_layers(self):
+ """Initialize layers of the head."""
+ super()._init_layers()
+ if self.pred_bbox2d:
+ self.scale_dim += 1
+ if self.pred_keypoints:
+ self.scale_dim += 1
+ self.scales = nn.ModuleList([
+ nn.ModuleList([Scale(1.0) for _ in range(self.scale_dim)])
+ for _ in self.strides
+ ])
+
+ def _init_predictor(self):
+ """Initialize predictor layers of the head."""
+ super()._init_predictor()
+
+ if self.use_depth_classifier:
+ self.conv_depth_cls_prev = self._init_branch(
+ conv_channels=self.depth_branch,
+ conv_strides=(1, ) * len(self.depth_branch))
+ self.conv_depth_cls = nn.Conv2d(self.depth_branch[-1],
+ self.num_depth_cls, 1)
+ # Data-agnostic single param lambda for local depth fusion
+ self.fuse_lambda = nn.Parameter(torch.tensor(10e-5))
+
+ if self.weight_dim != -1:
+ self.conv_weight_prevs = nn.ModuleList()
+ self.conv_weights = nn.ModuleList()
+ for i in range(self.weight_dim):
+ weight_branch_channels = self.weight_branch[i]
+ weight_out_channel = self.weight_out_channels[i]
+ if len(weight_branch_channels) > 0:
+ self.conv_weight_prevs.append(
+ self._init_branch(
+ conv_channels=weight_branch_channels,
+ conv_strides=(1, ) * len(weight_branch_channels)))
+ self.conv_weights.append(
+ nn.Conv2d(weight_out_channel, 1, 1))
+ else:
+ self.conv_weight_prevs.append(None)
+ self.conv_weights.append(
+ nn.Conv2d(self.feat_channels, 1, 1))
+
+ def init_weights(self):
+ """Initialize weights of the head.
+
+ We currently still use the customized defined init_weights because the
+ default init of DCN triggered by the init_cfg will init
+ conv_offset.weight, which mistakenly affects the training stability.
+ """
+ super().init_weights()
+
+ bias_cls = bias_init_with_prob(0.01)
+ if self.use_depth_classifier:
+ for m in self.conv_depth_cls_prev:
+ if isinstance(m.conv, nn.Conv2d):
+ normal_init(m.conv, std=0.01)
+ normal_init(self.conv_depth_cls, std=0.01, bias=bias_cls)
+
+ if self.weight_dim != -1:
+ for conv_weight_prev in self.conv_weight_prevs:
+ if conv_weight_prev is None:
+ continue
+ for m in conv_weight_prev:
+ if isinstance(m.conv, nn.Conv2d):
+ normal_init(m.conv, std=0.01)
+ for conv_weight in self.conv_weights:
+ normal_init(conv_weight, std=0.01)
+
+ def forward(self, feats):
+ """Forward features from the upstream network.
+
+ Args:
+ feats (tuple[Tensor]): Features from the upstream network, each is
+ a 4D-tensor.
+
+ Returns:
+ tuple:
+ cls_scores (list[Tensor]): Box scores for each scale level,
+ each is a 4D-tensor, the channel number is
+ num_points * num_classes.
+ bbox_preds (list[Tensor]): Box energies / deltas for each scale
+ level, each is a 4D-tensor, the channel number is
+ num_points * bbox_code_size.
+ dir_cls_preds (list[Tensor]): Box scores for direction class
+ predictions on each scale level, each is a 4D-tensor,
+ the channel number is num_points * 2. (bin = 2).
+ weight (list[Tensor]): Location-aware weight maps on each
+ scale level, each is a 4D-tensor, the channel number is
+ num_points * 1.
+ depth_cls_preds (list[Tensor]): Box scores for depth class
+ predictions on each scale level, each is a 4D-tensor,
+ the channel number is num_points * self.num_depth_cls.
+ attr_preds (list[Tensor]): Attribute scores for each scale
+ level, each is a 4D-tensor, the channel number is
+ num_points * num_attrs.
+ centernesses (list[Tensor]): Centerness for each scale level,
+ each is a 4D-tensor, the channel number is num_points * 1.
+ """
+ return multi_apply(self.forward_single, feats, self.scales,
+ self.strides)
+
+ def forward_single(self, x, scale, stride):
+ """Forward features of a single scale level.
+
+ Args:
+ x (Tensor): FPN feature maps of the specified stride.
+ scale (:obj: `mmcv.cnn.Scale`): Learnable scale module to resize
+ the bbox prediction.
+ stride (int): The corresponding stride for feature maps, only
+ used to normalize the bbox prediction when self.norm_on_bbox
+ is True.
+
+ Returns:
+ tuple: scores for each class, bbox and direction class
+ predictions, depth class predictions, location-aware weights,
+ attribute and centerness predictions of input feature maps.
+ """
+ cls_score, bbox_pred, dir_cls_pred, attr_pred, centerness, cls_feat, \
+ reg_feat = super().forward_single(x, scale, stride)
+
+ max_regress_range = stride * self.regress_ranges[0][1] / \
+ self.strides[0]
+ bbox_pred = self.bbox_coder.decode_2d(bbox_pred, scale, stride,
+ max_regress_range, self.training,
+ self.pred_keypoints,
+ self.pred_bbox2d)
+
+ depth_cls_pred = None
+ if self.use_depth_classifier:
+ clone_reg_feat = reg_feat.clone()
+ for conv_depth_cls_prev_layer in self.conv_depth_cls_prev:
+ clone_reg_feat = conv_depth_cls_prev_layer(clone_reg_feat)
+ depth_cls_pred = self.conv_depth_cls(clone_reg_feat)
+
+ weight = None
+ if self.weight_dim != -1:
+ weight = []
+ for i in range(self.weight_dim):
+ clone_reg_feat = reg_feat.clone()
+ if len(self.weight_branch[i]) > 0:
+ for conv_weight_prev_layer in self.conv_weight_prevs[i]:
+ clone_reg_feat = conv_weight_prev_layer(clone_reg_feat)
+ weight.append(self.conv_weights[i](clone_reg_feat))
+ weight = torch.cat(weight, dim=1)
+
+ return cls_score, bbox_pred, dir_cls_pred, depth_cls_pred, weight, \
+ attr_pred, centerness
+
+ def get_proj_bbox2d(self,
+ bbox_preds,
+ pos_dir_cls_preds,
+ labels_3d,
+ bbox_targets_3d,
+ pos_points,
+ pos_inds,
+ img_metas,
+ pos_depth_cls_preds=None,
+ pos_weights=None,
+ pos_cls_scores=None,
+ with_kpts=False):
+ """Decode box predictions and get projected 2D attributes.
+
+ Args:
+ bbox_preds (list[Tensor]): Box predictions for each scale
+ level, each is a 4D-tensor, the channel number is
+ num_points * bbox_code_size.
+ pos_dir_cls_preds (Tensor): Box scores for direction class
+ predictions of positive boxes on all the scale levels in shape
+ (num_pos_points, 2).
+ labels_3d (list[Tensor]): 3D box category labels for each scale
+ level, each is a 4D-tensor.
+ bbox_targets_3d (list[Tensor]): 3D box targets for each scale
+ level, each is a 4D-tensor, the channel number is
+ num_points * bbox_code_size.
+ pos_points (Tensor): Foreground points.
+ pos_inds (Tensor): Index of foreground points from flattened
+ tensors.
+ img_metas (list[dict]): Meta information of each image, e.g.,
+ image size, scaling factor, etc.
+ pos_depth_cls_preds (Tensor, optional): Probabilistic depth map of
+ positive boxes on all the scale levels in shape
+ (num_pos_points, self.num_depth_cls). Defaults to None.
+ pos_weights (Tensor, optional): Location-aware weights of positive
+ boxes in shape (num_pos_points, self.weight_dim). Defaults to
+ None.
+ pos_cls_scores (Tensor, optional): Classification scores of
+ positive boxes in shape (num_pos_points, self.num_classes).
+ Defaults to None.
+ with_kpts (bool, optional): Whether to output keypoints targets.
+ Defaults to False.
+
+ Returns:
+ tuple[Tensor]: Exterior 2D boxes from projected 3D boxes,
+ predicted 2D boxes and keypoint targets (if necessary).
+ """
+ views = [np.array(img_meta['cam2img']) for img_meta in img_metas]
+ num_imgs = len(img_metas)
+ img_idx = []
+ for label in labels_3d:
+ for idx in range(num_imgs):
+ img_idx.append(
+ labels_3d[0].new_ones(int(len(label) / num_imgs)) * idx)
+ img_idx = torch.cat(img_idx)
+ pos_img_idx = img_idx[pos_inds]
+
+ flatten_strided_bbox_preds = []
+ flatten_strided_bbox2d_preds = []
+ flatten_bbox_targets_3d = []
+ flatten_strides = []
+
+ for stride_idx, bbox_pred in enumerate(bbox_preds):
+ flatten_bbox_pred = bbox_pred.permute(0, 2, 3, 1).reshape(
+ -1, sum(self.group_reg_dims))
+ flatten_bbox_pred[:, :2] *= self.strides[stride_idx]
+ flatten_bbox_pred[:, -4:] *= self.strides[stride_idx]
+ flatten_strided_bbox_preds.append(
+ flatten_bbox_pred[:, :self.bbox_coder.bbox_code_size])
+ flatten_strided_bbox2d_preds.append(flatten_bbox_pred[:, -4:])
+
+ bbox_target_3d = bbox_targets_3d[stride_idx].clone()
+ bbox_target_3d[:, :2] *= self.strides[stride_idx]
+ bbox_target_3d[:, -4:] *= self.strides[stride_idx]
+ flatten_bbox_targets_3d.append(bbox_target_3d)
+
+ flatten_stride = flatten_bbox_pred.new_ones(
+ *flatten_bbox_pred.shape[:-1], 1) * self.strides[stride_idx]
+ flatten_strides.append(flatten_stride)
+
+ flatten_strided_bbox_preds = torch.cat(flatten_strided_bbox_preds)
+ flatten_strided_bbox2d_preds = torch.cat(flatten_strided_bbox2d_preds)
+ flatten_bbox_targets_3d = torch.cat(flatten_bbox_targets_3d)
+ flatten_strides = torch.cat(flatten_strides)
+ pos_strided_bbox_preds = flatten_strided_bbox_preds[pos_inds]
+ pos_strided_bbox2d_preds = flatten_strided_bbox2d_preds[pos_inds]
+ pos_bbox_targets_3d = flatten_bbox_targets_3d[pos_inds]
+ pos_strides = flatten_strides[pos_inds]
+
+ pos_decoded_bbox2d_preds = distance2bbox(pos_points,
+ pos_strided_bbox2d_preds)
+
+ pos_strided_bbox_preds[:, :2] = \
+ pos_points - pos_strided_bbox_preds[:, :2]
+ pos_bbox_targets_3d[:, :2] = \
+ pos_points - pos_bbox_targets_3d[:, :2]
+
+ if self.use_depth_classifier and (not self.use_onlyreg_proj):
+ pos_prob_depth_preds = self.bbox_coder.decode_prob_depth(
+ pos_depth_cls_preds, self.depth_range, self.depth_unit,
+ self.division, self.num_depth_cls)
+ sig_alpha = torch.sigmoid(self.fuse_lambda)
+ pos_strided_bbox_preds[:, 2] = \
+ sig_alpha * pos_strided_bbox_preds.clone()[:, 2] + \
+ (1 - sig_alpha) * pos_prob_depth_preds
+
+ box_corners_in_image = pos_strided_bbox_preds.new_zeros(
+ (*pos_strided_bbox_preds.shape[:-1], 8, 2))
+ box_corners_in_image_gt = pos_strided_bbox_preds.new_zeros(
+ (*pos_strided_bbox_preds.shape[:-1], 8, 2))
+
+ for idx in range(num_imgs):
+ mask = (pos_img_idx == idx)
+ if pos_strided_bbox_preds[mask].shape[0] == 0:
+ continue
+ cam2img = torch.eye(
+ 4,
+ dtype=pos_strided_bbox_preds.dtype,
+ device=pos_strided_bbox_preds.device)
+ view_shape = views[idx].shape
+ cam2img[:view_shape[0], :view_shape[1]] = \
+ pos_strided_bbox_preds.new_tensor(views[idx])
+
+ centers2d_preds = pos_strided_bbox_preds.clone()[mask, :2]
+ centers2d_targets = pos_bbox_targets_3d.clone()[mask, :2]
+ centers3d_targets = points_img2cam(pos_bbox_targets_3d[mask, :3],
+ views[idx])
+
+ # use predicted depth to re-project the 2.5D centers
+ pos_strided_bbox_preds[mask, :3] = points_img2cam(
+ pos_strided_bbox_preds[mask, :3], views[idx])
+ pos_bbox_targets_3d[mask, :3] = centers3d_targets
+
+ # depth fixed when computing re-project 3D bboxes
+ pos_strided_bbox_preds[mask, 2] = \
+ pos_bbox_targets_3d.clone()[mask, 2]
+
+ # decode yaws
+ if self.use_direction_classifier:
+ pos_dir_cls_scores = torch.max(
+ pos_dir_cls_preds[mask], dim=-1)[1]
+ pos_strided_bbox_preds[mask] = self.bbox_coder.decode_yaw(
+ pos_strided_bbox_preds[mask], centers2d_preds,
+ pos_dir_cls_scores, self.dir_offset, cam2img)
+ pos_bbox_targets_3d[mask, 6] = torch.atan2(
+ centers2d_targets[:, 0] - cam2img[0, 2],
+ cam2img[0, 0]) + pos_bbox_targets_3d[mask, 6]
+
+ corners = img_metas[0]['box_type_3d'](
+ pos_strided_bbox_preds[mask],
+ box_dim=self.bbox_coder.bbox_code_size,
+ origin=(0.5, 0.5, 0.5)).corners
+ box_corners_in_image[mask] = points_cam2img(corners, cam2img)
+
+ corners_gt = img_metas[0]['box_type_3d'](
+ pos_bbox_targets_3d[mask, :self.bbox_code_size],
+ box_dim=self.bbox_coder.bbox_code_size,
+ origin=(0.5, 0.5, 0.5)).corners
+ box_corners_in_image_gt[mask] = points_cam2img(corners_gt, cam2img)
+
+ minxy = torch.min(box_corners_in_image, dim=1)[0]
+ maxxy = torch.max(box_corners_in_image, dim=1)[0]
+ proj_bbox2d_preds = torch.cat([minxy, maxxy], dim=1)
+
+ outputs = (proj_bbox2d_preds, pos_decoded_bbox2d_preds)
+
+ if with_kpts:
+ norm_strides = pos_strides * self.regress_ranges[0][1] / \
+ self.strides[0]
+ kpts_targets = box_corners_in_image_gt - pos_points[..., None, :]
+ kpts_targets = kpts_targets.view(
+ (*pos_strided_bbox_preds.shape[:-1], 16))
+ kpts_targets /= norm_strides
+
+ outputs += (kpts_targets, )
+
+ return outputs
+
+ def get_pos_predictions(self, bbox_preds, dir_cls_preds, depth_cls_preds,
+ weights, attr_preds, centernesses, pos_inds,
+ img_metas):
+ """Flatten predictions and get positive ones.
+
+ Args:
+ bbox_preds (list[Tensor]): Box energies / deltas for each scale
+ level, each is a 4D-tensor, the channel number is
+ num_points * bbox_code_size.
+ dir_cls_preds (list[Tensor]): Box scores for direction class
+ predictions on each scale level, each is a 4D-tensor,
+ the channel number is num_points * 2. (bin = 2)
+ depth_cls_preds (list[Tensor]): Box scores for direction class
+ predictions on each scale level, each is a 4D-tensor,
+ the channel number is num_points * self.num_depth_cls.
+ attr_preds (list[Tensor]): Attribute scores for each scale level,
+ each is a 4D-tensor, the channel number is
+ num_points * num_attrs.
+ centernesses (list[Tensor]): Centerness for each scale level, each
+ is a 4D-tensor, the channel number is num_points * 1.
+ pos_inds (Tensor): Index of foreground points from flattened
+ tensors.
+ img_metas (list[dict]): Meta information of each image, e.g.,
+ image size, scaling factor, etc.
+
+ Returns:
+ tuple[Tensor]: Box predictions, direction classes, probabilistic
+ depth maps, location-aware weight maps, attributes and
+ centerness predictions.
+ """
+ flatten_bbox_preds = [
+ bbox_pred.permute(0, 2, 3, 1).reshape(-1, sum(self.group_reg_dims))
+ for bbox_pred in bbox_preds
+ ]
+ flatten_dir_cls_preds = [
+ dir_cls_pred.permute(0, 2, 3, 1).reshape(-1, 2)
+ for dir_cls_pred in dir_cls_preds
+ ]
+ flatten_centerness = [
+ centerness.permute(0, 2, 3, 1).reshape(-1)
+ for centerness in centernesses
+ ]
+ flatten_bbox_preds = torch.cat(flatten_bbox_preds)
+ flatten_dir_cls_preds = torch.cat(flatten_dir_cls_preds)
+ flatten_centerness = torch.cat(flatten_centerness)
+ pos_bbox_preds = flatten_bbox_preds[pos_inds]
+ pos_dir_cls_preds = flatten_dir_cls_preds[pos_inds]
+ pos_centerness = flatten_centerness[pos_inds]
+
+ pos_depth_cls_preds = None
+ if self.use_depth_classifier:
+ flatten_depth_cls_preds = [
+ depth_cls_pred.permute(0, 2, 3,
+ 1).reshape(-1, self.num_depth_cls)
+ for depth_cls_pred in depth_cls_preds
+ ]
+ flatten_depth_cls_preds = torch.cat(flatten_depth_cls_preds)
+ pos_depth_cls_preds = flatten_depth_cls_preds[pos_inds]
+
+ pos_weights = None
+ if self.weight_dim != -1:
+ flatten_weights = [
+ weight.permute(0, 2, 3, 1).reshape(-1, self.weight_dim)
+ for weight in weights
+ ]
+ flatten_weights = torch.cat(flatten_weights)
+ pos_weights = flatten_weights[pos_inds]
+
+ pos_attr_preds = None
+ if self.pred_attrs:
+ flatten_attr_preds = [
+ attr_pred.permute(0, 2, 3, 1).reshape(-1, self.num_attrs)
+ for attr_pred in attr_preds
+ ]
+ flatten_attr_preds = torch.cat(flatten_attr_preds)
+ pos_attr_preds = flatten_attr_preds[pos_inds]
+
+ return pos_bbox_preds, pos_dir_cls_preds, pos_depth_cls_preds, \
+ pos_weights, pos_attr_preds, pos_centerness
+
+ @force_fp32(
+ apply_to=('cls_scores', 'bbox_preds', 'dir_cls_preds',
+ 'depth_cls_preds', 'weights', 'attr_preds', 'centernesses'))
+ def loss(self,
+ cls_scores,
+ bbox_preds,
+ dir_cls_preds,
+ depth_cls_preds,
+ weights,
+ attr_preds,
+ centernesses,
+ gt_bboxes,
+ gt_labels,
+ gt_bboxes_3d,
+ gt_labels_3d,
+ centers2d,
+ depths,
+ attr_labels,
+ img_metas,
+ gt_bboxes_ignore=None):
+ """Compute loss of the head.
+
+ Args:
+ cls_scores (list[Tensor]): Box scores for each scale level,
+ each is a 4D-tensor, the channel number is
+ num_points * num_classes.
+ bbox_preds (list[Tensor]): Box energies / deltas for each scale
+ level, each is a 4D-tensor, the channel number is
+ num_points * bbox_code_size.
+ dir_cls_preds (list[Tensor]): Box scores for direction class
+ predictions on each scale level, each is a 4D-tensor,
+ the channel number is num_points * 2. (bin = 2)
+ depth_cls_preds (list[Tensor]): Box scores for direction class
+ predictions on each scale level, each is a 4D-tensor,
+ the channel number is num_points * self.num_depth_cls.
+ weights (list[Tensor]): Location-aware weights for each scale
+ level, each is a 4D-tensor, the channel number is
+ num_points * self.weight_dim.
+ attr_preds (list[Tensor]): Attribute scores for each scale level,
+ each is a 4D-tensor, the channel number is
+ num_points * num_attrs.
+ centernesses (list[Tensor]): Centerness for each scale level, each
+ is a 4D-tensor, the channel number is num_points * 1.
+ gt_bboxes (list[Tensor]): Ground truth bboxes for each image with
+ shape (num_gts, 4) in [tl_x, tl_y, br_x, br_y] format.
+ gt_labels (list[Tensor]): class indices corresponding to each box
+ gt_bboxes_3d (list[Tensor]): 3D boxes ground truth with shape of
+ (num_gts, code_size).
+ gt_labels_3d (list[Tensor]): same as gt_labels
+ centers2d (list[Tensor]): 2D centers on the image with shape of
+ (num_gts, 2).
+ depths (list[Tensor]): Depth ground truth with shape of
+ (num_gts, ).
+ attr_labels (list[Tensor]): Attributes indices of each box.
+ img_metas (list[dict]): Meta information of each image, e.g.,
+ image size, scaling factor, etc.
+ gt_bboxes_ignore (list[Tensor]): specify which bounding boxes can
+ be ignored when computing the loss. Defaults to None.
+
+ Returns:
+ dict[str, Tensor]: A dictionary of loss components.
+ """
+ assert len(cls_scores) == len(bbox_preds) == len(dir_cls_preds) == \
+ len(depth_cls_preds) == len(weights) == len(centernesses) == \
+ len(attr_preds), 'The length of cls_scores, bbox_preds, ' \
+ 'dir_cls_preds, depth_cls_preds, weights, centernesses, and' \
+ f'attr_preds: {len(cls_scores)}, {len(bbox_preds)}, ' \
+ f'{len(dir_cls_preds)}, {len(depth_cls_preds)}, {len(weights)}' \
+ f'{len(centernesses)}, {len(attr_preds)} are inconsistent.'
+ featmap_sizes = [featmap.size()[-2:] for featmap in cls_scores]
+ all_level_points = self.get_points(featmap_sizes, bbox_preds[0].dtype,
+ bbox_preds[0].device)
+ labels_3d, bbox_targets_3d, centerness_targets, attr_targets = \
+ self.get_targets(
+ all_level_points, gt_bboxes, gt_labels, gt_bboxes_3d,
+ gt_labels_3d, centers2d, depths, attr_labels)
+
+ num_imgs = cls_scores[0].size(0)
+ # flatten cls_scores and targets
+ flatten_cls_scores = [
+ cls_score.permute(0, 2, 3, 1).reshape(-1, self.cls_out_channels)
+ for cls_score in cls_scores
+ ]
+ flatten_cls_scores = torch.cat(flatten_cls_scores)
+ flatten_labels_3d = torch.cat(labels_3d)
+ flatten_bbox_targets_3d = torch.cat(bbox_targets_3d)
+ flatten_centerness_targets = torch.cat(centerness_targets)
+ flatten_points = torch.cat(
+ [points.repeat(num_imgs, 1) for points in all_level_points])
+ if self.pred_attrs:
+ flatten_attr_targets = torch.cat(attr_targets)
+
+ # FG cat_id: [0, num_classes -1], BG cat_id: num_classes
+ bg_class_ind = self.num_classes
+ pos_inds = ((flatten_labels_3d >= 0)
+ & (flatten_labels_3d < bg_class_ind)).nonzero().reshape(-1)
+ num_pos = len(pos_inds)
+
+ loss_dict = dict()
+
+ loss_dict['loss_cls'] = self.loss_cls(
+ flatten_cls_scores,
+ flatten_labels_3d,
+ avg_factor=num_pos + num_imgs) # avoid num_pos is 0
+
+ pos_bbox_preds, pos_dir_cls_preds, pos_depth_cls_preds, pos_weights, \
+ pos_attr_preds, pos_centerness = self.get_pos_predictions(
+ bbox_preds, dir_cls_preds, depth_cls_preds, weights,
+ attr_preds, centernesses, pos_inds, img_metas)
+
+ if num_pos > 0:
+ pos_bbox_targets_3d = flatten_bbox_targets_3d[pos_inds]
+ pos_centerness_targets = flatten_centerness_targets[pos_inds]
+ pos_points = flatten_points[pos_inds]
+ if self.pred_attrs:
+ pos_attr_targets = flatten_attr_targets[pos_inds]
+ if self.use_direction_classifier:
+ pos_dir_cls_targets = self.get_direction_target(
+ pos_bbox_targets_3d, self.dir_offset, one_hot=False)
+
+ bbox_weights = pos_centerness_targets.new_ones(
+ len(pos_centerness_targets), sum(self.group_reg_dims))
+ equal_weights = pos_centerness_targets.new_ones(
+ pos_centerness_targets.shape)
+ code_weight = self.train_cfg.get('code_weight', None)
+ if code_weight:
+ assert len(code_weight) == sum(self.group_reg_dims)
+ bbox_weights = bbox_weights * bbox_weights.new_tensor(
+ code_weight)
+
+ if self.diff_rad_by_sin:
+ pos_bbox_preds, pos_bbox_targets_3d = self.add_sin_difference(
+ pos_bbox_preds, pos_bbox_targets_3d)
+
+ loss_dict['loss_offset'] = self.loss_bbox(
+ pos_bbox_preds[:, :2],
+ pos_bbox_targets_3d[:, :2],
+ weight=bbox_weights[:, :2],
+ avg_factor=equal_weights.sum())
+ loss_dict['loss_size'] = self.loss_bbox(
+ pos_bbox_preds[:, 3:6],
+ pos_bbox_targets_3d[:, 3:6],
+ weight=bbox_weights[:, 3:6],
+ avg_factor=equal_weights.sum())
+ loss_dict['loss_rotsin'] = self.loss_bbox(
+ pos_bbox_preds[:, 6],
+ pos_bbox_targets_3d[:, 6],
+ weight=bbox_weights[:, 6],
+ avg_factor=equal_weights.sum())
+ if self.pred_velo:
+ loss_dict['loss_velo'] = self.loss_bbox(
+ pos_bbox_preds[:, 7:9],
+ pos_bbox_targets_3d[:, 7:9],
+ weight=bbox_weights[:, 7:9],
+ avg_factor=equal_weights.sum())
+
+ proj_bbox2d_inputs = (bbox_preds, pos_dir_cls_preds, labels_3d,
+ bbox_targets_3d, pos_points, pos_inds,
+ img_metas)
+
+ # direction classification loss
+ # TODO: add more check for use_direction_classifier
+ if self.use_direction_classifier:
+ loss_dict['loss_dir'] = self.loss_dir(
+ pos_dir_cls_preds,
+ pos_dir_cls_targets,
+ equal_weights,
+ avg_factor=equal_weights.sum())
+
+ # init depth loss with the one computed from direct regression
+ loss_dict['loss_depth'] = self.loss_bbox(
+ pos_bbox_preds[:, 2],
+ pos_bbox_targets_3d[:, 2],
+ weight=bbox_weights[:, 2],
+ avg_factor=equal_weights.sum())
+ # depth classification loss
+ if self.use_depth_classifier:
+ pos_prob_depth_preds = self.bbox_coder.decode_prob_depth(
+ pos_depth_cls_preds, self.depth_range, self.depth_unit,
+ self.division, self.num_depth_cls)
+ sig_alpha = torch.sigmoid(self.fuse_lambda)
+ if self.weight_dim != -1:
+ loss_fuse_depth = self.loss_depth(
+ sig_alpha * pos_bbox_preds[:, 2] +
+ (1 - sig_alpha) * pos_prob_depth_preds,
+ pos_bbox_targets_3d[:, 2],
+ sigma=pos_weights[:, 0],
+ weight=bbox_weights[:, 2],
+ avg_factor=equal_weights.sum())
+ else:
+ loss_fuse_depth = self.loss_depth(
+ sig_alpha * pos_bbox_preds[:, 2] +
+ (1 - sig_alpha) * pos_prob_depth_preds,
+ pos_bbox_targets_3d[:, 2],
+ weight=bbox_weights[:, 2],
+ avg_factor=equal_weights.sum())
+ loss_dict['loss_depth'] = loss_fuse_depth
+
+ proj_bbox2d_inputs += (pos_depth_cls_preds, )
+
+ if self.pred_keypoints:
+ # use smoothL1 to compute consistency loss for keypoints
+ # normalize the offsets with strides
+ proj_bbox2d_preds, pos_decoded_bbox2d_preds, kpts_targets = \
+ self.get_proj_bbox2d(*proj_bbox2d_inputs, with_kpts=True)
+ loss_dict['loss_kpts'] = self.loss_bbox(
+ pos_bbox_preds[:, self.kpts_start:self.kpts_start + 16],
+ kpts_targets,
+ weight=bbox_weights[:,
+ self.kpts_start:self.kpts_start + 16],
+ avg_factor=equal_weights.sum())
+
+ if self.pred_bbox2d:
+ loss_dict['loss_bbox2d'] = self.loss_bbox2d(
+ pos_bbox_preds[:, -4:],
+ pos_bbox_targets_3d[:, -4:],
+ weight=bbox_weights[:, -4:],
+ avg_factor=equal_weights.sum())
+ if not self.pred_keypoints:
+ proj_bbox2d_preds, pos_decoded_bbox2d_preds = \
+ self.get_proj_bbox2d(*proj_bbox2d_inputs)
+ loss_dict['loss_consistency'] = self.loss_consistency(
+ proj_bbox2d_preds,
+ pos_decoded_bbox2d_preds,
+ weight=bbox_weights[:, -4:],
+ avg_factor=equal_weights.sum())
+
+ loss_dict['loss_centerness'] = self.loss_centerness(
+ pos_centerness, pos_centerness_targets)
+
+ # attribute classification loss
+ if self.pred_attrs:
+ loss_dict['loss_attr'] = self.loss_attr(
+ pos_attr_preds,
+ pos_attr_targets,
+ pos_centerness_targets,
+ avg_factor=pos_centerness_targets.sum())
+
+ else:
+ # need absolute due to possible negative delta x/y
+ loss_dict['loss_offset'] = pos_bbox_preds[:, :2].sum()
+ loss_dict['loss_size'] = pos_bbox_preds[:, 3:6].sum()
+ loss_dict['loss_rotsin'] = pos_bbox_preds[:, 6].sum()
+ loss_dict['loss_depth'] = pos_bbox_preds[:, 2].sum()
+ if self.pred_velo:
+ loss_dict['loss_velo'] = pos_bbox_preds[:, 7:9].sum()
+ if self.pred_keypoints:
+ loss_dict['loss_kpts'] = pos_bbox_preds[:,
+ self.kpts_start:self.
+ kpts_start + 16].sum()
+ if self.pred_bbox2d:
+ loss_dict['loss_bbox2d'] = pos_bbox_preds[:, -4:].sum()
+ loss_dict['loss_consistency'] = pos_bbox_preds[:, -4:].sum()
+ loss_dict['loss_centerness'] = pos_centerness.sum()
+ if self.use_direction_classifier:
+ loss_dict['loss_dir'] = pos_dir_cls_preds.sum()
+ if self.use_depth_classifier:
+ sig_alpha = torch.sigmoid(self.fuse_lambda)
+ loss_fuse_depth = \
+ sig_alpha * pos_bbox_preds[:, 2].sum() + \
+ (1 - sig_alpha) * pos_depth_cls_preds.sum()
+ if self.weight_dim != -1:
+ loss_fuse_depth *= torch.exp(-pos_weights[:, 0].sum())
+ loss_dict['loss_depth'] = loss_fuse_depth
+ if self.pred_attrs:
+ loss_dict['loss_attr'] = pos_attr_preds.sum()
+
+ return loss_dict
+
+ @force_fp32(
+ apply_to=('cls_scores', 'bbox_preds', 'dir_cls_preds',
+ 'depth_cls_preds', 'weights', 'attr_preds', 'centernesses'))
+ def get_bboxes(self,
+ cls_scores,
+ bbox_preds,
+ dir_cls_preds,
+ depth_cls_preds,
+ weights,
+ attr_preds,
+ centernesses,
+ img_metas,
+ cfg=None,
+ rescale=None):
+ """Transform network output for a batch into bbox predictions.
+
+ Args:
+ cls_scores (list[Tensor]): Box scores for each scale level
+ Has shape (N, num_points * num_classes, H, W)
+ bbox_preds (list[Tensor]): Box energies / deltas for each scale
+ level with shape (N, num_points * 4, H, W)
+ dir_cls_preds (list[Tensor]): Box scores for direction class
+ predictions on each scale level, each is a 4D-tensor,
+ the channel number is num_points * 2. (bin = 2)
+ depth_cls_preds (list[Tensor]): Box scores for direction class
+ predictions on each scale level, each is a 4D-tensor,
+ the channel number is num_points * self.num_depth_cls.
+ weights (list[Tensor]): Location-aware weights for each scale
+ level, each is a 4D-tensor, the channel number is
+ num_points * self.weight_dim.
+ attr_preds (list[Tensor]): Attribute scores for each scale level
+ Has shape (N, num_points * num_attrs, H, W)
+ centernesses (list[Tensor]): Centerness for each scale level with
+ shape (N, num_points * 1, H, W)
+ img_metas (list[dict]): Meta information of each image, e.g.,
+ image size, scaling factor, etc.
+ cfg (mmcv.Config, optional): Test / postprocessing configuration,
+ if None, test_cfg would be used. Defaults to None.
+ rescale (bool, optional): If True, return boxes in original image
+ space. Defaults to None.
+
+ Returns:
+ list[tuple[Tensor]]: Each item in result_list is a tuple, which
+ consists of predicted 3D boxes, scores, labels, attributes and
+ 2D boxes (if necessary).
+ """
+ assert len(cls_scores) == len(bbox_preds) == len(dir_cls_preds) == \
+ len(depth_cls_preds) == len(weights) == len(centernesses) == \
+ len(attr_preds), 'The length of cls_scores, bbox_preds, ' \
+ 'dir_cls_preds, depth_cls_preds, weights, centernesses, and' \
+ f'attr_preds: {len(cls_scores)}, {len(bbox_preds)}, ' \
+ f'{len(dir_cls_preds)}, {len(depth_cls_preds)}, {len(weights)}' \
+ f'{len(centernesses)}, {len(attr_preds)} are inconsistent.'
+ num_levels = len(cls_scores)
+
+ featmap_sizes = [featmap.size()[-2:] for featmap in cls_scores]
+ mlvl_points = self.get_points(featmap_sizes, bbox_preds[0].dtype,
+ bbox_preds[0].device)
+ result_list = []
+ for img_id in range(len(img_metas)):
+ cls_score_list = [
+ cls_scores[i][img_id].detach() for i in range(num_levels)
+ ]
+ bbox_pred_list = [
+ bbox_preds[i][img_id].detach() for i in range(num_levels)
+ ]
+ if self.use_direction_classifier:
+ dir_cls_pred_list = [
+ dir_cls_preds[i][img_id].detach()
+ for i in range(num_levels)
+ ]
+ else:
+ dir_cls_pred_list = [
+ cls_scores[i][img_id].new_full(
+ [2, *cls_scores[i][img_id].shape[1:]], 0).detach()
+ for i in range(num_levels)
+ ]
+ if self.use_depth_classifier:
+ depth_cls_pred_list = [
+ depth_cls_preds[i][img_id].detach()
+ for i in range(num_levels)
+ ]
+ else:
+ depth_cls_pred_list = [
+ cls_scores[i][img_id].new_full(
+ [self.num_depth_cls, *cls_scores[i][img_id].shape[1:]],
+ 0).detach() for i in range(num_levels)
+ ]
+ if self.weight_dim != -1:
+ weight_list = [
+ weights[i][img_id].detach() for i in range(num_levels)
+ ]
+ else:
+ weight_list = [
+ cls_scores[i][img_id].new_full(
+ [1, *cls_scores[i][img_id].shape[1:]], 0).detach()
+ for i in range(num_levels)
+ ]
+ if self.pred_attrs:
+ attr_pred_list = [
+ attr_preds[i][img_id].detach() for i in range(num_levels)
+ ]
+ else:
+ attr_pred_list = [
+ cls_scores[i][img_id].new_full(
+ [self.num_attrs, *cls_scores[i][img_id].shape[1:]],
+ self.attr_background_label).detach()
+ for i in range(num_levels)
+ ]
+ centerness_pred_list = [
+ centernesses[i][img_id].detach() for i in range(num_levels)
+ ]
+ input_meta = img_metas[img_id]
+ det_bboxes = self._get_bboxes_single(
+ cls_score_list, bbox_pred_list, dir_cls_pred_list,
+ depth_cls_pred_list, weight_list, attr_pred_list,
+ centerness_pred_list, mlvl_points, input_meta, cfg, rescale)
+ result_list.append(det_bboxes)
+ return result_list
+
+ def _get_bboxes_single(self,
+ cls_scores,
+ bbox_preds,
+ dir_cls_preds,
+ depth_cls_preds,
+ weights,
+ attr_preds,
+ centernesses,
+ mlvl_points,
+ input_meta,
+ cfg,
+ rescale=False):
+ """Transform outputs for a single batch item into bbox predictions.
+
+ Args:
+ cls_scores (list[Tensor]): Box scores for a single scale level
+ Has shape (num_points * num_classes, H, W).
+ bbox_preds (list[Tensor]): Box energies / deltas for a single scale
+ level with shape (num_points * bbox_code_size, H, W).
+ dir_cls_preds (list[Tensor]): Box scores for direction class
+ predictions on a single scale level with shape
+ (num_points * 2, H, W)
+ depth_cls_preds (list[Tensor]): Box scores for probabilistic depth
+ predictions on a single scale level with shape
+ (num_points * self.num_depth_cls, H, W)
+ weights (list[Tensor]): Location-aware weight maps on a single
+ scale level with shape (num_points * self.weight_dim, H, W).
+ attr_preds (list[Tensor]): Attribute scores for each scale level
+ Has shape (N, num_points * num_attrs, H, W)
+ centernesses (list[Tensor]): Centerness for a single scale level
+ with shape (num_points, H, W).
+ mlvl_points (list[Tensor]): Box reference for a single scale level
+ with shape (num_total_points, 2).
+ input_meta (dict): Metadata of input image.
+ cfg (mmcv.Config): Test / postprocessing configuration,
+ if None, test_cfg would be used.
+ rescale (bool, optional): If True, return boxes in original image
+ space. Defaults to False.
+
+ Returns:
+ tuples[Tensor]: Predicted 3D boxes, scores, labels, attributes and
+ 2D boxes (if necessary).
+ """
+ view = np.array(input_meta['cam2img'])
+ scale_factor = input_meta['scale_factor']
+ cfg = self.test_cfg if cfg is None else cfg
+ assert len(cls_scores) == len(bbox_preds) == len(mlvl_points)
+ mlvl_centers2d = []
+ mlvl_bboxes = []
+ mlvl_scores = []
+ mlvl_dir_scores = []
+ mlvl_attr_scores = []
+ mlvl_centerness = []
+ mlvl_depth_cls_scores = []
+ mlvl_depth_uncertainty = []
+ mlvl_bboxes2d = None
+ if self.pred_bbox2d:
+ mlvl_bboxes2d = []
+
+ for cls_score, bbox_pred, dir_cls_pred, depth_cls_pred, weight, \
+ attr_pred, centerness, points in zip(
+ cls_scores, bbox_preds, dir_cls_preds, depth_cls_preds,
+ weights, attr_preds, centernesses, mlvl_points):
+ assert cls_score.size()[-2:] == bbox_pred.size()[-2:]
+ scores = cls_score.permute(1, 2, 0).reshape(
+ -1, self.cls_out_channels).sigmoid()
+ dir_cls_pred = dir_cls_pred.permute(1, 2, 0).reshape(-1, 2)
+ dir_cls_score = torch.max(dir_cls_pred, dim=-1)[1]
+ depth_cls_pred = depth_cls_pred.permute(1, 2, 0).reshape(
+ -1, self.num_depth_cls)
+ depth_cls_score = F.softmax(
+ depth_cls_pred, dim=-1).topk(
+ k=2, dim=-1)[0].mean(dim=-1)
+ if self.weight_dim != -1:
+ weight = weight.permute(1, 2, 0).reshape(-1, self.weight_dim)
+ else:
+ weight = weight.permute(1, 2, 0).reshape(-1, 1)
+ depth_uncertainty = torch.exp(-weight[:, -1])
+ attr_pred = attr_pred.permute(1, 2, 0).reshape(-1, self.num_attrs)
+ attr_score = torch.max(attr_pred, dim=-1)[1]
+ centerness = centerness.permute(1, 2, 0).reshape(-1).sigmoid()
+
+ bbox_pred = bbox_pred.permute(1, 2,
+ 0).reshape(-1,
+ sum(self.group_reg_dims))
+ bbox_pred3d = bbox_pred[:, :self.bbox_coder.bbox_code_size]
+ if self.pred_bbox2d:
+ bbox_pred2d = bbox_pred[:, -4:]
+ nms_pre = cfg.get('nms_pre', -1)
+ if nms_pre > 0 and scores.shape[0] > nms_pre:
+ merged_scores = scores * centerness[:, None]
+ if self.use_depth_classifier:
+ merged_scores *= depth_cls_score[:, None]
+ if self.weight_dim != -1:
+ merged_scores *= depth_uncertainty[:, None]
+ max_scores, _ = merged_scores.max(dim=1)
+ _, topk_inds = max_scores.topk(nms_pre)
+ points = points[topk_inds, :]
+ bbox_pred3d = bbox_pred3d[topk_inds, :]
+ scores = scores[topk_inds, :]
+ dir_cls_pred = dir_cls_pred[topk_inds, :]
+ depth_cls_pred = depth_cls_pred[topk_inds, :]
+ centerness = centerness[topk_inds]
+ dir_cls_score = dir_cls_score[topk_inds]
+ depth_cls_score = depth_cls_score[topk_inds]
+ depth_uncertainty = depth_uncertainty[topk_inds]
+ attr_score = attr_score[topk_inds]
+ if self.pred_bbox2d:
+ bbox_pred2d = bbox_pred2d[topk_inds, :]
+ # change the offset to actual center predictions
+ bbox_pred3d[:, :2] = points - bbox_pred3d[:, :2]
+ if rescale:
+ bbox_pred3d[:, :2] /= bbox_pred3d[:, :2].new_tensor(
+ scale_factor)
+ if self.pred_bbox2d:
+ bbox_pred2d /= bbox_pred2d.new_tensor(scale_factor)
+ if self.use_depth_classifier:
+ prob_depth_pred = self.bbox_coder.decode_prob_depth(
+ depth_cls_pred, self.depth_range, self.depth_unit,
+ self.division, self.num_depth_cls)
+ sig_alpha = torch.sigmoid(self.fuse_lambda)
+ bbox_pred3d[:, 2] = sig_alpha * bbox_pred3d[:, 2] + \
+ (1 - sig_alpha) * prob_depth_pred
+ pred_center2d = bbox_pred3d[:, :3].clone()
+ bbox_pred3d[:, :3] = points_img2cam(bbox_pred3d[:, :3], view)
+ mlvl_centers2d.append(pred_center2d)
+ mlvl_bboxes.append(bbox_pred3d)
+ mlvl_scores.append(scores)
+ mlvl_dir_scores.append(dir_cls_score)
+ mlvl_depth_cls_scores.append(depth_cls_score)
+ mlvl_attr_scores.append(attr_score)
+ mlvl_centerness.append(centerness)
+ mlvl_depth_uncertainty.append(depth_uncertainty)
+ if self.pred_bbox2d:
+ bbox_pred2d = distance2bbox(
+ points, bbox_pred2d, max_shape=input_meta['img_shape'])
+ mlvl_bboxes2d.append(bbox_pred2d)
+
+ mlvl_centers2d = torch.cat(mlvl_centers2d)
+ mlvl_bboxes = torch.cat(mlvl_bboxes)
+ mlvl_dir_scores = torch.cat(mlvl_dir_scores)
+ if self.pred_bbox2d:
+ mlvl_bboxes2d = torch.cat(mlvl_bboxes2d)
+
+ # change local yaw to global yaw for 3D nms
+ cam2img = torch.eye(
+ 4, dtype=mlvl_centers2d.dtype, device=mlvl_centers2d.device)
+ cam2img[:view.shape[0], :view.shape[1]] = \
+ mlvl_centers2d.new_tensor(view)
+ mlvl_bboxes = self.bbox_coder.decode_yaw(mlvl_bboxes, mlvl_centers2d,
+ mlvl_dir_scores,
+ self.dir_offset, cam2img)
+
+ mlvl_bboxes_for_nms = xywhr2xyxyr(input_meta['box_type_3d'](
+ mlvl_bboxes,
+ box_dim=self.bbox_coder.bbox_code_size,
+ origin=(0.5, 0.5, 0.5)).bev)
+
+ mlvl_scores = torch.cat(mlvl_scores)
+ padding = mlvl_scores.new_zeros(mlvl_scores.shape[0], 1)
+ # remind that we set FG labels to [0, num_class-1] since mmdet v2.0
+ # BG cat_id: num_class
+ mlvl_scores = torch.cat([mlvl_scores, padding], dim=1)
+ mlvl_attr_scores = torch.cat(mlvl_attr_scores)
+ mlvl_centerness = torch.cat(mlvl_centerness)
+ # no scale_factors in box3d_multiclass_nms
+ # Then we multiply it from outside
+ mlvl_nms_scores = mlvl_scores * mlvl_centerness[:, None]
+ if self.use_depth_classifier: # multiply the depth confidence
+ mlvl_depth_cls_scores = torch.cat(mlvl_depth_cls_scores)
+ mlvl_nms_scores *= mlvl_depth_cls_scores[:, None]
+ if self.weight_dim != -1:
+ mlvl_depth_uncertainty = torch.cat(mlvl_depth_uncertainty)
+ mlvl_nms_scores *= mlvl_depth_uncertainty[:, None]
+ results = box3d_multiclass_nms(mlvl_bboxes, mlvl_bboxes_for_nms,
+ mlvl_nms_scores, cfg.score_thr,
+ cfg.max_per_img, cfg, mlvl_dir_scores,
+ mlvl_attr_scores, mlvl_bboxes2d)
+ bboxes, scores, labels, dir_scores, attrs = results[0:5]
+ attrs = attrs.to(labels.dtype) # change data type to int
+ bboxes = input_meta['box_type_3d'](
+ bboxes,
+ box_dim=self.bbox_coder.bbox_code_size,
+ origin=(0.5, 0.5, 0.5))
+ # Note that the predictions use origin (0.5, 0.5, 0.5)
+ # Due to the ground truth centers2d are the gravity center of objects
+ # v0.10.0 fix inplace operation to the input tensor of cam_box3d
+ # So here we also need to add origin=(0.5, 0.5, 0.5)
+ if not self.pred_attrs:
+ attrs = None
+
+ outputs = (bboxes, scores, labels, attrs)
+ if self.pred_bbox2d:
+ bboxes2d = results[-1]
+ bboxes2d = torch.cat([bboxes2d, scores[:, None]], dim=1)
+ outputs = outputs + (bboxes2d, )
+
+ return outputs
+
+ def get_targets(self, points, gt_bboxes_list, gt_labels_list,
+ gt_bboxes_3d_list, gt_labels_3d_list, centers2d_list,
+ depths_list, attr_labels_list):
+ """Compute regression, classification and centerss targets for points
+ in multiple images.
+
+ Args:
+ points (list[Tensor]): Points of each fpn level, each has shape
+ (num_points, 2).
+ gt_bboxes_list (list[Tensor]): Ground truth bboxes of each image,
+ each has shape (num_gt, 4).
+ gt_labels_list (list[Tensor]): Ground truth labels of each box,
+ each has shape (num_gt,).
+ gt_bboxes_3d_list (list[Tensor]): 3D Ground truth bboxes of each
+ image, each has shape (num_gt, bbox_code_size).
+ gt_labels_3d_list (list[Tensor]): 3D Ground truth labels of each
+ box, each has shape (num_gt,).
+ centers2d_list (list[Tensor]): Projected 3D centers onto 2D image,
+ each has shape (num_gt, 2).
+ depths_list (list[Tensor]): Depth of projected 3D centers onto 2D
+ image, each has shape (num_gt, 1).
+ attr_labels_list (list[Tensor]): Attribute labels of each box,
+ each has shape (num_gt,).
+
+ Returns:
+ tuple:
+ concat_lvl_labels (list[Tensor]): Labels of each level. \
+ concat_lvl_bbox_targets (list[Tensor]): BBox targets of each \
+ level.
+ """
+ assert len(points) == len(self.regress_ranges)
+ num_levels = len(points)
+ # expand regress ranges to align with points
+ expanded_regress_ranges = [
+ points[i].new_tensor(self.regress_ranges[i])[None].expand_as(
+ points[i]) for i in range(num_levels)
+ ]
+ # concat all levels points and regress ranges
+ concat_regress_ranges = torch.cat(expanded_regress_ranges, dim=0)
+ concat_points = torch.cat(points, dim=0)
+
+ # the number of points per img, per lvl
+ num_points = [center.size(0) for center in points]
+
+ if attr_labels_list is None:
+ attr_labels_list = [
+ gt_labels.new_full(gt_labels.shape, self.attr_background_label)
+ for gt_labels in gt_labels_list
+ ]
+
+ # get labels and bbox_targets of each image
+ _, bbox_targets_list, labels_3d_list, bbox_targets_3d_list, \
+ centerness_targets_list, attr_targets_list = multi_apply(
+ self._get_target_single,
+ gt_bboxes_list,
+ gt_labels_list,
+ gt_bboxes_3d_list,
+ gt_labels_3d_list,
+ centers2d_list,
+ depths_list,
+ attr_labels_list,
+ points=concat_points,
+ regress_ranges=concat_regress_ranges,
+ num_points_per_lvl=num_points)
+
+ # split to per img, per level
+ bbox_targets_list = [
+ bbox_targets.split(num_points, 0)
+ for bbox_targets in bbox_targets_list
+ ]
+ labels_3d_list = [
+ labels_3d.split(num_points, 0) for labels_3d in labels_3d_list
+ ]
+ bbox_targets_3d_list = [
+ bbox_targets_3d.split(num_points, 0)
+ for bbox_targets_3d in bbox_targets_3d_list
+ ]
+ centerness_targets_list = [
+ centerness_targets.split(num_points, 0)
+ for centerness_targets in centerness_targets_list
+ ]
+ attr_targets_list = [
+ attr_targets.split(num_points, 0)
+ for attr_targets in attr_targets_list
+ ]
+
+ # concat per level image
+ concat_lvl_labels_3d = []
+ concat_lvl_bbox_targets_3d = []
+ concat_lvl_centerness_targets = []
+ concat_lvl_attr_targets = []
+ for i in range(num_levels):
+ concat_lvl_labels_3d.append(
+ torch.cat([labels[i] for labels in labels_3d_list]))
+ concat_lvl_centerness_targets.append(
+ torch.cat([
+ centerness_targets[i]
+ for centerness_targets in centerness_targets_list
+ ]))
+ bbox_targets_3d = torch.cat([
+ bbox_targets_3d[i] for bbox_targets_3d in bbox_targets_3d_list
+ ])
+ if self.pred_bbox2d:
+ bbox_targets = torch.cat(
+ [bbox_targets[i] for bbox_targets in bbox_targets_list])
+ bbox_targets_3d = torch.cat([bbox_targets_3d, bbox_targets],
+ dim=1)
+ concat_lvl_attr_targets.append(
+ torch.cat(
+ [attr_targets[i] for attr_targets in attr_targets_list]))
+ if self.norm_on_bbox:
+ bbox_targets_3d[:, :2] = \
+ bbox_targets_3d[:, :2] / self.strides[i]
+ if self.pred_bbox2d:
+ bbox_targets_3d[:, -4:] = \
+ bbox_targets_3d[:, -4:] / self.strides[i]
+ concat_lvl_bbox_targets_3d.append(bbox_targets_3d)
+ return concat_lvl_labels_3d, concat_lvl_bbox_targets_3d, \
+ concat_lvl_centerness_targets, concat_lvl_attr_targets
diff --git a/mmdet3d/models/dense_heads/point_rpn_head.py b/mmdet3d/models/dense_heads/point_rpn_head.py
new file mode 100644
index 0000000..546cf16
--- /dev/null
+++ b/mmdet3d/models/dense_heads/point_rpn_head.py
@@ -0,0 +1,381 @@
+# Copyright (c) OpenMMLab. All rights reserved.
+import torch
+from mmcv.runner import BaseModule, force_fp32
+from torch import nn as nn
+
+from mmdet3d.core import xywhr2xyxyr
+from mmdet3d.core.bbox.structures import (DepthInstance3DBoxes,
+ LiDARInstance3DBoxes)
+from mmdet3d.core.post_processing import nms_bev, nms_normal_bev
+from mmdet.core import build_bbox_coder, multi_apply
+from ..builder import HEADS, build_loss
+
+
+@HEADS.register_module()
+class PointRPNHead(BaseModule):
+ """RPN module for PointRCNN.
+
+ Args:
+ num_classes (int): Number of classes.
+ train_cfg (dict): Train configs.
+ test_cfg (dict): Test configs.
+ pred_layer_cfg (dict, optional): Config of classification and
+ regression prediction layers. Defaults to None.
+ enlarge_width (float, optional): Enlarge bbox for each side to ignore
+ close points. Defaults to 0.1.
+ cls_loss (dict, optional): Config of direction classification loss.
+ Defaults to None.
+ bbox_loss (dict, optional): Config of localization loss.
+ Defaults to None.
+ bbox_coder (dict, optional): Config dict of box coders.
+ Defaults to None.
+ init_cfg (dict, optional): Config of initialization. Defaults to None.
+ """
+
+ def __init__(self,
+ num_classes,
+ train_cfg,
+ test_cfg,
+ pred_layer_cfg=None,
+ enlarge_width=0.1,
+ cls_loss=None,
+ bbox_loss=None,
+ bbox_coder=None,
+ init_cfg=None):
+ super().__init__(init_cfg=init_cfg)
+ self.num_classes = num_classes
+ self.train_cfg = train_cfg
+ self.test_cfg = test_cfg
+ self.enlarge_width = enlarge_width
+
+ # build loss function
+ self.bbox_loss = build_loss(bbox_loss)
+ self.cls_loss = build_loss(cls_loss)
+
+ # build box coder
+ self.bbox_coder = build_bbox_coder(bbox_coder)
+
+ # build pred conv
+ self.cls_layers = self._make_fc_layers(
+ fc_cfg=pred_layer_cfg.cls_linear_channels,
+ input_channels=pred_layer_cfg.in_channels,
+ output_channels=self._get_cls_out_channels())
+
+ self.reg_layers = self._make_fc_layers(
+ fc_cfg=pred_layer_cfg.reg_linear_channels,
+ input_channels=pred_layer_cfg.in_channels,
+ output_channels=self._get_reg_out_channels())
+
+ def _make_fc_layers(self, fc_cfg, input_channels, output_channels):
+ """Make fully connect layers.
+
+ Args:
+ fc_cfg (dict): Config of fully connect.
+ input_channels (int): Input channels for fc_layers.
+ output_channels (int): Input channels for fc_layers.
+
+ Returns:
+ nn.Sequential: Fully connect layers.
+ """
+ fc_layers = []
+ c_in = input_channels
+ for k in range(0, fc_cfg.__len__()):
+ fc_layers.extend([
+ nn.Linear(c_in, fc_cfg[k], bias=False),
+ nn.BatchNorm1d(fc_cfg[k]),
+ nn.ReLU(),
+ ])
+ c_in = fc_cfg[k]
+ fc_layers.append(nn.Linear(c_in, output_channels, bias=True))
+ return nn.Sequential(*fc_layers)
+
+ def _get_cls_out_channels(self):
+ """Return the channel number of classification outputs."""
+ # Class numbers (k) + objectness (1)
+ return self.num_classes
+
+ def _get_reg_out_channels(self):
+ """Return the channel number of regression outputs."""
+ # Bbox classification and regression
+ # (center residual (3), size regression (3)
+ # torch.cos(yaw) (1), torch.sin(yaw) (1)
+ return self.bbox_coder.code_size
+
+ def forward(self, feat_dict):
+ """Forward pass.
+
+ Args:
+ feat_dict (dict): Feature dict from backbone.
+
+ Returns:
+ tuple[list[torch.Tensor]]: Predicted boxes and classification
+ scores.
+ """
+ point_features = feat_dict['fp_features']
+ point_features = point_features.permute(0, 2, 1).contiguous()
+ batch_size = point_features.shape[0]
+ feat_cls = point_features.view(-1, point_features.shape[-1])
+ feat_reg = point_features.view(-1, point_features.shape[-1])
+
+ point_cls_preds = self.cls_layers(feat_cls).reshape(
+ batch_size, -1, self._get_cls_out_channels())
+ point_box_preds = self.reg_layers(feat_reg).reshape(
+ batch_size, -1, self._get_reg_out_channels())
+ return point_box_preds, point_cls_preds
+
+ @force_fp32(apply_to=('bbox_preds'))
+ def loss(self,
+ bbox_preds,
+ cls_preds,
+ points,
+ gt_bboxes_3d,
+ gt_labels_3d,
+ img_metas=None):
+ """Compute loss.
+
+ Args:
+ bbox_preds (dict): Predictions from forward of PointRCNN RPN_Head.
+ cls_preds (dict): Classification from forward of PointRCNN
+ RPN_Head.
+ points (list[torch.Tensor]): Input points.
+ gt_bboxes_3d (list[:obj:`BaseInstance3DBoxes`]): Ground truth
+ bboxes of each sample.
+ gt_labels_3d (list[torch.Tensor]): Labels of each sample.
+ img_metas (list[dict], Optional): Contain pcd and img's meta info.
+ Defaults to None.
+
+ Returns:
+ dict: Losses of PointRCNN RPN module.
+ """
+ targets = self.get_targets(points, gt_bboxes_3d, gt_labels_3d)
+ (bbox_targets, mask_targets, positive_mask, negative_mask,
+ box_loss_weights, point_targets) = targets
+
+ # bbox loss
+ bbox_loss = self.bbox_loss(bbox_preds, bbox_targets,
+ box_loss_weights.unsqueeze(-1))
+ # calculate semantic loss
+ semantic_points = cls_preds.reshape(-1, self.num_classes)
+ semantic_targets = mask_targets
+ semantic_targets[negative_mask] = self.num_classes
+ semantic_points_label = semantic_targets
+ # for ignore, but now we do not have ignored label
+ semantic_loss_weight = negative_mask.float() + positive_mask.float()
+ semantic_loss = self.cls_loss(semantic_points,
+ semantic_points_label.reshape(-1),
+ semantic_loss_weight.reshape(-1))
+ semantic_loss /= positive_mask.float().sum()
+ losses = dict(bbox_loss=bbox_loss, semantic_loss=semantic_loss)
+
+ return losses
+
+ def get_targets(self, points, gt_bboxes_3d, gt_labels_3d):
+ """Generate targets of PointRCNN RPN head.
+
+ Args:
+ points (list[torch.Tensor]): Points of each batch.
+ gt_bboxes_3d (list[:obj:`BaseInstance3DBoxes`]): Ground truth
+ bboxes of each batch.
+ gt_labels_3d (list[torch.Tensor]): Labels of each batch.
+
+ Returns:
+ tuple[torch.Tensor]: Targets of PointRCNN RPN head.
+ """
+ # find empty example
+ for index in range(len(gt_labels_3d)):
+ if len(gt_labels_3d[index]) == 0:
+ fake_box = gt_bboxes_3d[index].tensor.new_zeros(
+ 1, gt_bboxes_3d[index].tensor.shape[-1])
+ gt_bboxes_3d[index] = gt_bboxes_3d[index].new_box(fake_box)
+ gt_labels_3d[index] = gt_labels_3d[index].new_zeros(1)
+
+ (bbox_targets, mask_targets, positive_mask, negative_mask,
+ point_targets) = multi_apply(self.get_targets_single, points,
+ gt_bboxes_3d, gt_labels_3d)
+
+ bbox_targets = torch.stack(bbox_targets)
+ mask_targets = torch.stack(mask_targets)
+ positive_mask = torch.stack(positive_mask)
+ negative_mask = torch.stack(negative_mask)
+ box_loss_weights = positive_mask / (positive_mask.sum() + 1e-6)
+
+ return (bbox_targets, mask_targets, positive_mask, negative_mask,
+ box_loss_weights, point_targets)
+
+ def get_targets_single(self, points, gt_bboxes_3d, gt_labels_3d):
+ """Generate targets of PointRCNN RPN head for single batch.
+
+ Args:
+ points (torch.Tensor): Points of each batch.
+ gt_bboxes_3d (:obj:`BaseInstance3DBoxes`): Ground truth
+ boxes of each batch.
+ gt_labels_3d (torch.Tensor): Labels of each batch.
+
+ Returns:
+ tuple[torch.Tensor]: Targets of ssd3d head.
+ """
+ gt_bboxes_3d = gt_bboxes_3d.to(points.device)
+
+ valid_gt = gt_labels_3d != -1
+ gt_bboxes_3d = gt_bboxes_3d[valid_gt]
+ gt_labels_3d = gt_labels_3d[valid_gt]
+
+ # transform the bbox coordinate to the point cloud coordinate
+ gt_bboxes_3d_tensor = gt_bboxes_3d.tensor.clone()
+ gt_bboxes_3d_tensor[..., 2] += gt_bboxes_3d_tensor[..., 5] / 2
+
+ points_mask, assignment = self._assign_targets_by_points_inside(
+ gt_bboxes_3d, points)
+ gt_bboxes_3d_tensor = gt_bboxes_3d_tensor[assignment]
+ mask_targets = gt_labels_3d[assignment]
+
+ bbox_targets = self.bbox_coder.encode(gt_bboxes_3d_tensor,
+ points[..., 0:3], mask_targets)
+
+ positive_mask = (points_mask.max(1)[0] > 0)
+ # add ignore_mask
+ extend_gt_bboxes_3d = gt_bboxes_3d.enlarged_box(self.enlarge_width)
+ points_mask, _ = self._assign_targets_by_points_inside(
+ extend_gt_bboxes_3d, points)
+ negative_mask = (points_mask.max(1)[0] == 0)
+
+ point_targets = points[..., 0:3]
+ return (bbox_targets, mask_targets, positive_mask, negative_mask,
+ point_targets)
+
+ def get_bboxes(self,
+ points,
+ bbox_preds,
+ cls_preds,
+ input_metas,
+ rescale=False):
+ """Generate bboxes from RPN head predictions.
+
+ Args:
+ points (torch.Tensor): Input points.
+ bbox_preds (dict): Regression predictions from PointRCNN head.
+ cls_preds (dict): Class scores predictions from PointRCNN head.
+ input_metas (list[dict]): Point cloud and image's meta info.
+ rescale (bool, optional): Whether to rescale bboxes.
+ Defaults to False.
+
+ Returns:
+ list[tuple[torch.Tensor]]: Bounding boxes, scores and labels.
+ """
+ sem_scores = cls_preds.sigmoid()
+ obj_scores = sem_scores.max(-1)[0]
+ object_class = sem_scores.argmax(dim=-1)
+
+ batch_size = sem_scores.shape[0]
+ results = list()
+ for b in range(batch_size):
+ bbox3d = self.bbox_coder.decode(bbox_preds[b], points[b, ..., :3],
+ object_class[b])
+ bbox_selected, score_selected, labels, cls_preds_selected = \
+ self.class_agnostic_nms(obj_scores[b], sem_scores[b], bbox3d,
+ points[b, ..., :3], input_metas[b])
+ bbox = input_metas[b]['box_type_3d'](
+ bbox_selected.clone(),
+ box_dim=bbox_selected.shape[-1],
+ with_yaw=True)
+ results.append((bbox, score_selected, labels, cls_preds_selected))
+ return results
+
+ def class_agnostic_nms(self, obj_scores, sem_scores, bbox, points,
+ input_meta):
+ """Class agnostic nms.
+
+ Args:
+ obj_scores (torch.Tensor): Objectness score of bounding boxes.
+ sem_scores (torch.Tensor): Semantic class score of bounding boxes.
+ bbox (torch.Tensor): Predicted bounding boxes.
+
+ Returns:
+ tuple[torch.Tensor]: Bounding boxes, scores and labels.
+ """
+ nms_cfg = self.test_cfg.nms_cfg if not self.training \
+ else self.train_cfg.nms_cfg
+ if nms_cfg.use_rotate_nms:
+ nms_func = nms_bev
+ else:
+ nms_func = nms_normal_bev
+
+ num_bbox = bbox.shape[0]
+ bbox = input_meta['box_type_3d'](
+ bbox.clone(),
+ box_dim=bbox.shape[-1],
+ with_yaw=True,
+ origin=(0.5, 0.5, 0.5))
+
+ if isinstance(bbox, LiDARInstance3DBoxes):
+ box_idx = bbox.points_in_boxes(points)
+ box_indices = box_idx.new_zeros([num_bbox + 1])
+ box_idx[box_idx == -1] = num_bbox
+ box_indices.scatter_add_(0, box_idx.long(),
+ box_idx.new_ones(box_idx.shape))
+ box_indices = box_indices[:-1]
+ nonempty_box_mask = box_indices >= 0
+ elif isinstance(bbox, DepthInstance3DBoxes):
+ box_indices = bbox.points_in_boxes(points)
+ nonempty_box_mask = box_indices.T.sum(1) >= 0
+ else:
+ raise NotImplementedError('Unsupported bbox type!')
+
+ bbox = bbox[nonempty_box_mask]
+
+ if self.test_cfg.score_thr is not None:
+ score_thr = self.test_cfg.score_thr
+ keep = (obj_scores >= score_thr)
+ obj_scores = obj_scores[keep]
+ sem_scores = sem_scores[keep]
+ bbox = bbox.tensor[keep]
+
+ if obj_scores.shape[0] > 0:
+ topk = min(nms_cfg.nms_pre, obj_scores.shape[0])
+ obj_scores_nms, indices = torch.topk(obj_scores, k=topk)
+ bbox_for_nms = xywhr2xyxyr(bbox[indices].bev)
+ sem_scores_nms = sem_scores[indices]
+
+ keep = nms_func(bbox_for_nms, obj_scores_nms, nms_cfg.iou_thr)
+ keep = keep[:nms_cfg.nms_post]
+
+ bbox_selected = bbox.tensor[indices][keep]
+ score_selected = obj_scores_nms[keep]
+ cls_preds = sem_scores_nms[keep]
+ labels = torch.argmax(cls_preds, -1)
+ else:
+ bbox_selected = bbox.tensor
+ score_selected = obj_scores.new_zeros([0])
+ labels = obj_scores.new_zeros([0])
+ cls_preds = obj_scores.new_zeros([0, sem_scores.shape[-1]])
+
+ return bbox_selected, score_selected, labels, cls_preds
+
+ def _assign_targets_by_points_inside(self, bboxes_3d, points):
+ """Compute assignment by checking whether point is inside bbox.
+
+ Args:
+ bboxes_3d (:obj:`BaseInstance3DBoxes`): Instance of bounding boxes.
+ points (torch.Tensor): Points of a batch.
+
+ Returns:
+ tuple[torch.Tensor]: Flags indicating whether each point is
+ inside bbox and the index of box where each point are in.
+ """
+ # TODO: align points_in_boxes function in each box_structures
+ num_bbox = bboxes_3d.tensor.shape[0]
+ if isinstance(bboxes_3d, LiDARInstance3DBoxes):
+ assignment = bboxes_3d.points_in_boxes(points[:, 0:3]).long()
+ points_mask = assignment.new_zeros(
+ [assignment.shape[0], num_bbox + 1])
+ assignment[assignment == -1] = num_bbox
+ points_mask.scatter_(1, assignment.unsqueeze(1), 1)
+ points_mask = points_mask[:, :-1]
+ assignment[assignment == num_bbox] = num_bbox - 1
+ elif isinstance(bboxes_3d, DepthInstance3DBoxes):
+ points_mask = bboxes_3d.points_in_boxes(points)
+ assignment = points_mask.argmax(dim=-1)
+ else:
+ raise NotImplementedError('Unsupported bbox type!')
+
+ return points_mask, assignment
diff --git a/mmdet3d/models/dense_heads/shape_aware_head.py b/mmdet3d/models/dense_heads/shape_aware_head.py
new file mode 100644
index 0000000..6c55571
--- /dev/null
+++ b/mmdet3d/models/dense_heads/shape_aware_head.py
@@ -0,0 +1,515 @@
+# Copyright (c) OpenMMLab. All rights reserved.
+import warnings
+
+import numpy as np
+import torch
+from mmcv.cnn import ConvModule
+from mmcv.runner import BaseModule
+from torch import nn as nn
+
+from mmdet3d.core import box3d_multiclass_nms, limit_period, xywhr2xyxyr
+from mmdet.core import multi_apply
+from ..builder import HEADS, build_head
+from .anchor3d_head import Anchor3DHead
+
+
+@HEADS.register_module()
+class BaseShapeHead(BaseModule):
+ """Base Shape-aware Head in Shape Signature Network.
+
+ Note:
+ This base shape-aware grouping head uses default settings for small
+ objects. For large and huge objects, it is recommended to use
+ heavier heads, like (64, 64, 64) and (128, 128, 64, 64, 64) in
+ shared conv channels, (2, 1, 1) and (2, 1, 2, 1, 1) in shared
+ conv strides. For tiny objects, we can use smaller heads, like
+ (32, 32) channels and (1, 1) strides.
+
+ Args:
+ num_cls (int): Number of classes.
+ num_base_anchors (int): Number of anchors per location.
+ box_code_size (int): The dimension of boxes to be encoded.
+ in_channels (int): Input channels for convolutional layers.
+ shared_conv_channels (tuple, optional): Channels for shared
+ convolutional layers. Default: (64, 64).
+ shared_conv_strides (tuple, optional): Strides for shared
+ convolutional layers. Default: (1, 1).
+ use_direction_classifier (bool, optional): Whether to use direction
+ classifier. Default: True.
+ conv_cfg (dict, optional): Config of conv layer.
+ Default: dict(type='Conv2d')
+ norm_cfg (dict, optional): Config of norm layer.
+ Default: dict(type='BN2d').
+ bias (bool | str, optional): Type of bias. Default: False.
+ """
+
+ def __init__(self,
+ num_cls,
+ num_base_anchors,
+ box_code_size,
+ in_channels,
+ shared_conv_channels=(64, 64),
+ shared_conv_strides=(1, 1),
+ use_direction_classifier=True,
+ conv_cfg=dict(type='Conv2d'),
+ norm_cfg=dict(type='BN2d'),
+ bias=False,
+ init_cfg=None):
+ super().__init__(init_cfg=init_cfg)
+ self.num_cls = num_cls
+ self.num_base_anchors = num_base_anchors
+ self.use_direction_classifier = use_direction_classifier
+ self.box_code_size = box_code_size
+
+ assert len(shared_conv_channels) == len(shared_conv_strides), \
+ 'Lengths of channels and strides list should be equal.'
+
+ self.shared_conv_channels = [in_channels] + list(shared_conv_channels)
+ self.shared_conv_strides = list(shared_conv_strides)
+
+ shared_conv = []
+ for i in range(len(self.shared_conv_strides)):
+ shared_conv.append(
+ ConvModule(
+ self.shared_conv_channels[i],
+ self.shared_conv_channels[i + 1],
+ kernel_size=3,
+ stride=self.shared_conv_strides[i],
+ padding=1,
+ conv_cfg=conv_cfg,
+ bias=bias,
+ norm_cfg=norm_cfg))
+
+ self.shared_conv = nn.Sequential(*shared_conv)
+
+ out_channels = self.shared_conv_channels[-1]
+ self.conv_cls = nn.Conv2d(out_channels, num_base_anchors * num_cls, 1)
+ self.conv_reg = nn.Conv2d(out_channels,
+ num_base_anchors * box_code_size, 1)
+
+ if use_direction_classifier:
+ self.conv_dir_cls = nn.Conv2d(out_channels, num_base_anchors * 2,
+ 1)
+ if init_cfg is None:
+ if use_direction_classifier:
+ self.init_cfg = dict(
+ type='Kaiming',
+ layer='Conv2d',
+ override=[
+ dict(type='Normal', name='conv_reg', std=0.01),
+ dict(
+ type='Normal',
+ name='conv_cls',
+ std=0.01,
+ bias_prob=0.01),
+ dict(
+ type='Normal',
+ name='conv_dir_cls',
+ std=0.01,
+ bias_prob=0.01)
+ ])
+ else:
+ self.init_cfg = dict(
+ type='Kaiming',
+ layer='Conv2d',
+ override=[
+ dict(type='Normal', name='conv_reg', std=0.01),
+ dict(
+ type='Normal',
+ name='conv_cls',
+ std=0.01,
+ bias_prob=0.01)
+ ])
+
+ def forward(self, x):
+ """Forward function for SmallHead.
+
+ Args:
+ x (torch.Tensor): Input feature map with the shape of
+ [B, C, H, W].
+
+ Returns:
+ dict[torch.Tensor]: Contain score of each class, bbox
+ regression and direction classification predictions.
+ Note that all the returned tensors are reshaped as
+ [bs*num_base_anchors*H*W, num_cls/box_code_size/dir_bins].
+ It is more convenient to concat anchors for different
+ classes even though they have different feature map sizes.
+ """
+ x = self.shared_conv(x)
+ cls_score = self.conv_cls(x)
+ bbox_pred = self.conv_reg(x)
+ featmap_size = bbox_pred.shape[-2:]
+ H, W = featmap_size
+ B = bbox_pred.shape[0]
+ cls_score = cls_score.view(-1, self.num_base_anchors, self.num_cls, H,
+ W).permute(0, 1, 3, 4,
+ 2).reshape(B, -1, self.num_cls)
+ bbox_pred = bbox_pred.view(-1, self.num_base_anchors,
+ self.box_code_size, H, W).permute(
+ 0, 1, 3, 4,
+ 2).reshape(B, -1, self.box_code_size)
+
+ dir_cls_preds = None
+ if self.use_direction_classifier:
+ dir_cls_preds = self.conv_dir_cls(x)
+ dir_cls_preds = dir_cls_preds.view(-1, self.num_base_anchors, 2, H,
+ W).permute(0, 1, 3, 4,
+ 2).reshape(B, -1, 2)
+ ret = dict(
+ cls_score=cls_score,
+ bbox_pred=bbox_pred,
+ dir_cls_preds=dir_cls_preds,
+ featmap_size=featmap_size)
+ return ret
+
+
+@HEADS.register_module()
+class ShapeAwareHead(Anchor3DHead):
+ """Shape-aware grouping head for SSN.
+
+ Args:
+ tasks (dict): Shape-aware groups of multi-class objects.
+ assign_per_class (bool, optional): Whether to do assignment for each
+ class. Default: True.
+ kwargs (dict): Other arguments are the same as those in
+ :class:`Anchor3DHead`.
+ """
+
+ def __init__(self, tasks, assign_per_class=True, init_cfg=None, **kwargs):
+ self.tasks = tasks
+ self.featmap_sizes = []
+ super().__init__(
+ assign_per_class=assign_per_class, init_cfg=init_cfg, **kwargs)
+
+ def init_weights(self):
+ if not self._is_init:
+ for m in self.heads:
+ if hasattr(m, 'init_weights'):
+ m.init_weights()
+ self._is_init = True
+ else:
+ warnings.warn(f'init_weights of {self.__class__.__name__} has '
+ f'been called more than once.')
+
+ def _init_layers(self):
+ """Initialize neural network layers of the head."""
+ self.heads = nn.ModuleList()
+ cls_ptr = 0
+ for task in self.tasks:
+ sizes = self.anchor_generator.sizes[cls_ptr:cls_ptr +
+ task['num_class']]
+ num_size = torch.tensor(sizes).reshape(-1, 3).size(0)
+ num_rot = len(self.anchor_generator.rotations)
+ num_base_anchors = num_rot * num_size
+ branch = dict(
+ type='BaseShapeHead',
+ num_cls=self.num_classes,
+ num_base_anchors=num_base_anchors,
+ box_code_size=self.box_code_size,
+ in_channels=self.in_channels,
+ shared_conv_channels=task['shared_conv_channels'],
+ shared_conv_strides=task['shared_conv_strides'])
+ self.heads.append(build_head(branch))
+ cls_ptr += task['num_class']
+
+ def forward_single(self, x):
+ """Forward function on a single-scale feature map.
+
+ Args:
+ x (torch.Tensor): Input features.
+ Returns:
+ tuple[torch.Tensor]: Contain score of each class, bbox
+ regression and direction classification predictions.
+ """
+ results = []
+
+ for head in self.heads:
+ results.append(head(x))
+
+ cls_score = torch.cat([result['cls_score'] for result in results],
+ dim=1)
+ bbox_pred = torch.cat([result['bbox_pred'] for result in results],
+ dim=1)
+ dir_cls_preds = None
+ if self.use_direction_classifier:
+ dir_cls_preds = torch.cat(
+ [result['dir_cls_preds'] for result in results], dim=1)
+
+ self.featmap_sizes = []
+ for i, task in enumerate(self.tasks):
+ for _ in range(task['num_class']):
+ self.featmap_sizes.append(results[i]['featmap_size'])
+ assert len(self.featmap_sizes) == len(self.anchor_generator.ranges), \
+ 'Length of feature map sizes must be equal to length of ' + \
+ 'different ranges of anchor generator.'
+
+ return cls_score, bbox_pred, dir_cls_preds
+
+ def loss_single(self, cls_score, bbox_pred, dir_cls_preds, labels,
+ label_weights, bbox_targets, bbox_weights, dir_targets,
+ dir_weights, num_total_samples):
+ """Calculate loss of Single-level results.
+
+ Args:
+ cls_score (torch.Tensor): Class score in single-level.
+ bbox_pred (torch.Tensor): Bbox prediction in single-level.
+ dir_cls_preds (torch.Tensor): Predictions of direction class
+ in single-level.
+ labels (torch.Tensor): Labels of class.
+ label_weights (torch.Tensor): Weights of class loss.
+ bbox_targets (torch.Tensor): Targets of bbox predictions.
+ bbox_weights (torch.Tensor): Weights of bbox loss.
+ dir_targets (torch.Tensor): Targets of direction predictions.
+ dir_weights (torch.Tensor): Weights of direction loss.
+ num_total_samples (int): The number of valid samples.
+
+ Returns:
+ tuple[torch.Tensor]: Losses of class, bbox
+ and direction, respectively.
+ """
+ # classification loss
+ if num_total_samples is None:
+ num_total_samples = int(cls_score.shape[0])
+ labels = labels.reshape(-1)
+ label_weights = label_weights.reshape(-1)
+ cls_score = cls_score.reshape(-1, self.num_classes)
+ loss_cls = self.loss_cls(
+ cls_score, labels, label_weights, avg_factor=num_total_samples)
+
+ # regression loss
+ bbox_targets = bbox_targets.reshape(-1, self.box_code_size)
+ bbox_weights = bbox_weights.reshape(-1, self.box_code_size)
+ code_weight = self.train_cfg.get('code_weight', None)
+
+ if code_weight:
+ bbox_weights = bbox_weights * bbox_weights.new_tensor(code_weight)
+ bbox_pred = bbox_pred.reshape(-1, self.box_code_size)
+ if self.diff_rad_by_sin:
+ bbox_pred, bbox_targets = self.add_sin_difference(
+ bbox_pred, bbox_targets)
+ loss_bbox = self.loss_bbox(
+ bbox_pred,
+ bbox_targets,
+ bbox_weights,
+ avg_factor=num_total_samples)
+
+ # direction classification loss
+ loss_dir = None
+ if self.use_direction_classifier:
+ dir_cls_preds = dir_cls_preds.reshape(-1, 2)
+ dir_targets = dir_targets.reshape(-1)
+ dir_weights = dir_weights.reshape(-1)
+ loss_dir = self.loss_dir(
+ dir_cls_preds,
+ dir_targets,
+ dir_weights,
+ avg_factor=num_total_samples)
+
+ return loss_cls, loss_bbox, loss_dir
+
+ def loss(self,
+ cls_scores,
+ bbox_preds,
+ dir_cls_preds,
+ gt_bboxes,
+ gt_labels,
+ input_metas,
+ gt_bboxes_ignore=None):
+ """Calculate losses.
+
+ Args:
+ cls_scores (list[torch.Tensor]): Multi-level class scores.
+ bbox_preds (list[torch.Tensor]): Multi-level bbox predictions.
+ dir_cls_preds (list[torch.Tensor]): Multi-level direction
+ class predictions.
+ gt_bboxes (list[:obj:`BaseInstance3DBoxes`]): Gt bboxes
+ of each sample.
+ gt_labels (list[torch.Tensor]): Gt labels of each sample.
+ input_metas (list[dict]): Contain pcd and img's meta info.
+ gt_bboxes_ignore (list[torch.Tensor]): Specify
+ which bounding.
+
+ Returns:
+ dict[str, list[torch.Tensor]]: Classification, bbox, and
+ direction losses of each level.
+
+ - loss_cls (list[torch.Tensor]): Classification losses.
+ - loss_bbox (list[torch.Tensor]): Box regression losses.
+ - loss_dir (list[torch.Tensor]): Direction classification
+ losses.
+ """
+ device = cls_scores[0].device
+ anchor_list = self.get_anchors(
+ self.featmap_sizes, input_metas, device=device)
+ cls_reg_targets = self.anchor_target_3d(
+ anchor_list,
+ gt_bboxes,
+ input_metas,
+ gt_bboxes_ignore_list=gt_bboxes_ignore,
+ gt_labels_list=gt_labels,
+ num_classes=self.num_classes,
+ sampling=self.sampling)
+
+ if cls_reg_targets is None:
+ return None
+ (labels_list, label_weights_list, bbox_targets_list, bbox_weights_list,
+ dir_targets_list, dir_weights_list, num_total_pos,
+ num_total_neg) = cls_reg_targets
+ num_total_samples = (
+ num_total_pos + num_total_neg if self.sampling else num_total_pos)
+
+ # num_total_samples = None
+ losses_cls, losses_bbox, losses_dir = multi_apply(
+ self.loss_single,
+ cls_scores,
+ bbox_preds,
+ dir_cls_preds,
+ labels_list,
+ label_weights_list,
+ bbox_targets_list,
+ bbox_weights_list,
+ dir_targets_list,
+ dir_weights_list,
+ num_total_samples=num_total_samples)
+ return dict(
+ loss_cls=losses_cls, loss_bbox=losses_bbox, loss_dir=losses_dir)
+
+ def get_bboxes(self,
+ cls_scores,
+ bbox_preds,
+ dir_cls_preds,
+ input_metas,
+ cfg=None,
+ rescale=False):
+ """Get bboxes of anchor head.
+
+ Args:
+ cls_scores (list[torch.Tensor]): Multi-level class scores.
+ bbox_preds (list[torch.Tensor]): Multi-level bbox predictions.
+ dir_cls_preds (list[torch.Tensor]): Multi-level direction
+ class predictions.
+ input_metas (list[dict]): Contain pcd and img's meta info.
+ cfg (:obj:`ConfigDict`, optional): Training or testing config.
+ Default: None.
+ rescale (list[torch.Tensor], optional): Whether to rescale bbox.
+ Default: False.
+
+ Returns:
+ list[tuple]: Prediction resultes of batches.
+ """
+ assert len(cls_scores) == len(bbox_preds)
+ assert len(cls_scores) == len(dir_cls_preds)
+ num_levels = len(cls_scores)
+ assert num_levels == 1, 'Only support single level inference.'
+ device = cls_scores[0].device
+ mlvl_anchors = self.anchor_generator.grid_anchors(
+ self.featmap_sizes, device=device)
+ # `anchor` is a list of anchors for different classes
+ mlvl_anchors = [torch.cat(anchor, dim=0) for anchor in mlvl_anchors]
+
+ result_list = []
+ for img_id in range(len(input_metas)):
+ cls_score_list = [
+ cls_scores[i][img_id].detach() for i in range(num_levels)
+ ]
+ bbox_pred_list = [
+ bbox_preds[i][img_id].detach() for i in range(num_levels)
+ ]
+ dir_cls_pred_list = [
+ dir_cls_preds[i][img_id].detach() for i in range(num_levels)
+ ]
+
+ input_meta = input_metas[img_id]
+ proposals = self.get_bboxes_single(cls_score_list, bbox_pred_list,
+ dir_cls_pred_list, mlvl_anchors,
+ input_meta, cfg, rescale)
+ result_list.append(proposals)
+ return result_list
+
+ def get_bboxes_single(self,
+ cls_scores,
+ bbox_preds,
+ dir_cls_preds,
+ mlvl_anchors,
+ input_meta,
+ cfg=None,
+ rescale=False):
+ """Get bboxes of single branch.
+
+ Args:
+ cls_scores (torch.Tensor): Class score in single batch.
+ bbox_preds (torch.Tensor): Bbox prediction in single batch.
+ dir_cls_preds (torch.Tensor): Predictions of direction class
+ in single batch.
+ mlvl_anchors (List[torch.Tensor]): Multi-level anchors
+ in single batch.
+ input_meta (list[dict]): Contain pcd and img's meta info.
+ cfg (:obj:`ConfigDict`): Training or testing config.
+ rescale (list[torch.Tensor], optional): whether to rescale bbox.
+ Default: False.
+
+ Returns:
+ tuple: Contain predictions of single batch.
+
+ - bboxes (:obj:`BaseInstance3DBoxes`): Predicted 3d bboxes.
+ - scores (torch.Tensor): Class score of each bbox.
+ - labels (torch.Tensor): Label of each bbox.
+ """
+ cfg = self.test_cfg if cfg is None else cfg
+ assert len(cls_scores) == len(bbox_preds) == len(mlvl_anchors)
+ mlvl_bboxes = []
+ mlvl_scores = []
+ mlvl_dir_scores = []
+ for cls_score, bbox_pred, dir_cls_pred, anchors in zip(
+ cls_scores, bbox_preds, dir_cls_preds, mlvl_anchors):
+ assert cls_score.size()[-2] == bbox_pred.size()[-2]
+ assert cls_score.size()[-2] == dir_cls_pred.size()[-2]
+ dir_cls_score = torch.max(dir_cls_pred, dim=-1)[1]
+
+ if self.use_sigmoid_cls:
+ scores = cls_score.sigmoid()
+ else:
+ scores = cls_score.softmax(-1)
+
+ nms_pre = cfg.get('nms_pre', -1)
+ if nms_pre > 0 and scores.shape[0] > nms_pre:
+ if self.use_sigmoid_cls:
+ max_scores, _ = scores.max(dim=1)
+ else:
+ max_scores, _ = scores[:, :-1].max(dim=1)
+ _, topk_inds = max_scores.topk(nms_pre)
+ anchors = anchors[topk_inds, :]
+ bbox_pred = bbox_pred[topk_inds, :]
+ scores = scores[topk_inds, :]
+ dir_cls_score = dir_cls_score[topk_inds]
+
+ bboxes = self.bbox_coder.decode(anchors, bbox_pred)
+ mlvl_bboxes.append(bboxes)
+ mlvl_scores.append(scores)
+ mlvl_dir_scores.append(dir_cls_score)
+
+ mlvl_bboxes = torch.cat(mlvl_bboxes)
+ mlvl_bboxes_for_nms = xywhr2xyxyr(input_meta['box_type_3d'](
+ mlvl_bboxes, box_dim=self.box_code_size).bev)
+ mlvl_scores = torch.cat(mlvl_scores)
+ mlvl_dir_scores = torch.cat(mlvl_dir_scores)
+
+ if self.use_sigmoid_cls:
+ # Add a dummy background class to the front when using sigmoid
+ padding = mlvl_scores.new_zeros(mlvl_scores.shape[0], 1)
+ mlvl_scores = torch.cat([mlvl_scores, padding], dim=1)
+
+ score_thr = cfg.get('score_thr', 0)
+ results = box3d_multiclass_nms(mlvl_bboxes, mlvl_bboxes_for_nms,
+ mlvl_scores, score_thr, cfg.max_num,
+ cfg, mlvl_dir_scores)
+ bboxes, scores, labels, dir_scores = results
+ if bboxes.shape[0] > 0:
+ dir_rot = limit_period(bboxes[..., 6] - self.dir_offset,
+ self.dir_limit_offset, np.pi)
+ bboxes[..., 6] = (
+ dir_rot + self.dir_offset +
+ np.pi * dir_scores.to(bboxes.dtype))
+ bboxes = input_meta['box_type_3d'](bboxes, box_dim=self.box_code_size)
+ return bboxes, scores, labels
diff --git a/mmdet3d/models/dense_heads/smoke_mono3d_head.py b/mmdet3d/models/dense_heads/smoke_mono3d_head.py
new file mode 100644
index 0000000..3459e09
--- /dev/null
+++ b/mmdet3d/models/dense_heads/smoke_mono3d_head.py
@@ -0,0 +1,516 @@
+# Copyright (c) OpenMMLab. All rights reserved.
+import torch
+from torch.nn import functional as F
+
+from mmdet.core import multi_apply
+from mmdet.core.bbox.builder import build_bbox_coder
+from mmdet.models.utils import gaussian_radius, gen_gaussian_target
+from mmdet.models.utils.gaussian_target import (get_local_maximum,
+ get_topk_from_heatmap,
+ transpose_and_gather_feat)
+from ..builder import HEADS
+from .anchor_free_mono3d_head import AnchorFreeMono3DHead
+
+
+@HEADS.register_module()
+class SMOKEMono3DHead(AnchorFreeMono3DHead):
+ r"""Anchor-free head used in `SMOKE `_
+
+ .. code-block:: none
+
+ /-----> 3*3 conv -----> 1*1 conv -----> cls
+ feature
+ \-----> 3*3 conv -----> 1*1 conv -----> reg
+
+ Args:
+ num_classes (int): Number of categories excluding the background
+ category.
+ in_channels (int): Number of channels in the input feature map.
+ dim_channel (list[int]): indices of dimension offset preds in
+ regression heatmap channels.
+ ori_channel (list[int]): indices of orientation offset pred in
+ regression heatmap channels.
+ bbox_coder (:obj:`CameraInstance3DBoxes`): Bbox coder
+ for encoding and decoding boxes.
+ loss_cls (dict, optional): Config of classification loss.
+ Default: loss_cls=dict(type='GaussionFocalLoss', loss_weight=1.0).
+ loss_bbox (dict, optional): Config of localization loss.
+ Default: loss_bbox=dict(type='L1Loss', loss_weight=10.0).
+ loss_dir (dict, optional): Config of direction classification loss.
+ In SMOKE, Default: None.
+ loss_attr (dict, optional): Config of attribute classification loss.
+ In SMOKE, Default: None.
+ loss_centerness (dict): Config of centerness loss.
+ norm_cfg (dict): Dictionary to construct and config norm layer.
+ Default: norm_cfg=dict(type='GN', num_groups=32, requires_grad=True).
+ init_cfg (dict): Initialization config dict. Default: None.
+ """ # noqa: E501
+
+ def __init__(self,
+ num_classes,
+ in_channels,
+ dim_channel,
+ ori_channel,
+ bbox_coder,
+ loss_cls=dict(type='GaussionFocalLoss', loss_weight=1.0),
+ loss_bbox=dict(type='L1Loss', loss_weight=0.1),
+ loss_dir=None,
+ loss_attr=None,
+ norm_cfg=dict(type='GN', num_groups=32, requires_grad=True),
+ init_cfg=None,
+ **kwargs):
+ super().__init__(
+ num_classes,
+ in_channels,
+ loss_cls=loss_cls,
+ loss_bbox=loss_bbox,
+ loss_dir=loss_dir,
+ loss_attr=loss_attr,
+ norm_cfg=norm_cfg,
+ init_cfg=init_cfg,
+ **kwargs)
+ self.dim_channel = dim_channel
+ self.ori_channel = ori_channel
+ self.bbox_coder = build_bbox_coder(bbox_coder)
+
+ def forward(self, feats):
+ """Forward features from the upstream network.
+
+ Args:
+ feats (tuple[Tensor]): Features from the upstream network, each is
+ a 4D-tensor.
+
+ Returns:
+ tuple:
+ cls_scores (list[Tensor]): Box scores for each scale level,
+ each is a 4D-tensor, the channel number is
+ num_points * num_classes.
+ bbox_preds (list[Tensor]): Box energies / deltas for each scale
+ level, each is a 4D-tensor, the channel number is
+ num_points * bbox_code_size.
+ """
+ return multi_apply(self.forward_single, feats)
+
+ def forward_single(self, x):
+ """Forward features of a single scale level.
+
+ Args:
+ x (Tensor): Input feature map.
+
+ Returns:
+ tuple: Scores for each class, bbox of input feature maps.
+ """
+ cls_score, bbox_pred, dir_cls_pred, attr_pred, cls_feat, reg_feat = \
+ super().forward_single(x)
+ cls_score = cls_score.sigmoid() # turn to 0-1
+ cls_score = cls_score.clamp(min=1e-4, max=1 - 1e-4)
+ # (N, C, H, W)
+ offset_dims = bbox_pred[:, self.dim_channel, ...]
+ bbox_pred[:, self.dim_channel, ...] = offset_dims.sigmoid() - 0.5
+ # (N, C, H, W)
+ vector_ori = bbox_pred[:, self.ori_channel, ...]
+ bbox_pred[:, self.ori_channel, ...] = F.normalize(vector_ori)
+ return cls_score, bbox_pred
+
+ def get_bboxes(self, cls_scores, bbox_preds, img_metas, rescale=None):
+ """Generate bboxes from bbox head predictions.
+
+ Args:
+ cls_scores (list[Tensor]): Box scores for each scale level.
+ bbox_preds (list[Tensor]): Box regression for each scale.
+ img_metas (list[dict]): Meta information of each image, e.g.,
+ image size, scaling factor, etc.
+ rescale (bool): If True, return boxes in original image space.
+
+ Returns:
+ list[tuple[:obj:`CameraInstance3DBoxes`, Tensor, Tensor, None]]:
+ Each item in result_list is 4-tuple.
+ """
+ assert len(cls_scores) == len(bbox_preds) == 1
+ cam2imgs = torch.stack([
+ cls_scores[0].new_tensor(img_meta['cam2img'])
+ for img_meta in img_metas
+ ])
+ trans_mats = torch.stack([
+ cls_scores[0].new_tensor(img_meta['trans_mat'])
+ for img_meta in img_metas
+ ])
+ batch_bboxes, batch_scores, batch_topk_labels = self.decode_heatmap(
+ cls_scores[0],
+ bbox_preds[0],
+ img_metas,
+ cam2imgs=cam2imgs,
+ trans_mats=trans_mats,
+ topk=100,
+ kernel=3)
+
+ result_list = []
+ for img_id in range(len(img_metas)):
+
+ bboxes = batch_bboxes[img_id]
+ scores = batch_scores[img_id]
+ labels = batch_topk_labels[img_id]
+
+ keep_idx = scores > 0.25
+ bboxes = bboxes[keep_idx]
+ scores = scores[keep_idx]
+ labels = labels[keep_idx]
+
+ bboxes = img_metas[img_id]['box_type_3d'](
+ bboxes, box_dim=self.bbox_code_size, origin=(0.5, 0.5, 0.5))
+ attrs = None
+ result_list.append((bboxes, scores, labels, attrs))
+
+ return result_list
+
+ def decode_heatmap(self,
+ cls_score,
+ reg_pred,
+ img_metas,
+ cam2imgs,
+ trans_mats,
+ topk=100,
+ kernel=3):
+ """Transform outputs into detections raw bbox predictions.
+
+ Args:
+ class_score (Tensor): Center predict heatmap,
+ shape (B, num_classes, H, W).
+ reg_pred (Tensor): Box regression map.
+ shape (B, channel, H , W).
+ img_metas (List[dict]): Meta information of each image, e.g.,
+ image size, scaling factor, etc.
+ cam2imgs (Tensor): Camera intrinsic matrixs.
+ shape (B, 4, 4)
+ trans_mats (Tensor): Transformation matrix from original image
+ to feature map.
+ shape: (batch, 3, 3)
+ topk (int): Get top k center keypoints from heatmap. Default 100.
+ kernel (int): Max pooling kernel for extract local maximum pixels.
+ Default 3.
+
+ Returns:
+ tuple[torch.Tensor]: Decoded output of SMOKEHead, containing
+ the following Tensors:
+ - batch_bboxes (Tensor): Coords of each 3D box.
+ shape (B, k, 7)
+ - batch_scores (Tensor): Scores of each 3D box.
+ shape (B, k)
+ - batch_topk_labels (Tensor): Categories of each 3D box.
+ shape (B, k)
+ """
+ img_h, img_w = img_metas[0]['pad_shape'][:2]
+ bs, _, feat_h, feat_w = cls_score.shape
+
+ center_heatmap_pred = get_local_maximum(cls_score, kernel=kernel)
+
+ *batch_dets, topk_ys, topk_xs = get_topk_from_heatmap(
+ center_heatmap_pred, k=topk)
+ batch_scores, batch_index, batch_topk_labels = batch_dets
+
+ regression = transpose_and_gather_feat(reg_pred, batch_index)
+ regression = regression.view(-1, 8)
+
+ points = torch.cat([topk_xs.view(-1, 1),
+ topk_ys.view(-1, 1).float()],
+ dim=1)
+ locations, dimensions, orientations = self.bbox_coder.decode(
+ regression, points, batch_topk_labels, cam2imgs, trans_mats)
+
+ batch_bboxes = torch.cat((locations, dimensions, orientations), dim=1)
+ batch_bboxes = batch_bboxes.view(bs, -1, self.bbox_code_size)
+ return batch_bboxes, batch_scores, batch_topk_labels
+
+ def get_predictions(self, labels3d, centers2d, gt_locations, gt_dimensions,
+ gt_orientations, indices, img_metas, pred_reg):
+ """Prepare predictions for computing loss.
+
+ Args:
+ labels3d (Tensor): Labels of each 3D box.
+ shape (B, max_objs, )
+ centers2d (Tensor): Coords of each projected 3D box
+ center on image. shape (B * max_objs, 2)
+ gt_locations (Tensor): Coords of each 3D box's location.
+ shape (B * max_objs, 3)
+ gt_dimensions (Tensor): Dimensions of each 3D box.
+ shape (N, 3)
+ gt_orientations (Tensor): Orientation(yaw) of each 3D box.
+ shape (N, 1)
+ indices (Tensor): Indices of the existence of the 3D box.
+ shape (B * max_objs, )
+ img_metas (list[dict]): Meta information of each image,
+ e.g., image size, scaling factor, etc.
+ pre_reg (Tensor): Box regression map.
+ shape (B, channel, H , W).
+
+ Returns:
+ dict: the dict has components below:
+ - bbox3d_yaws (:obj:`CameraInstance3DBoxes`):
+ bbox calculated using pred orientations.
+ - bbox3d_dims (:obj:`CameraInstance3DBoxes`):
+ bbox calculated using pred dimensions.
+ - bbox3d_locs (:obj:`CameraInstance3DBoxes`):
+ bbox calculated using pred locations.
+ """
+ batch, channel = pred_reg.shape[0], pred_reg.shape[1]
+ w = pred_reg.shape[3]
+ cam2imgs = torch.stack([
+ gt_locations.new_tensor(img_meta['cam2img'])
+ for img_meta in img_metas
+ ])
+ trans_mats = torch.stack([
+ gt_locations.new_tensor(img_meta['trans_mat'])
+ for img_meta in img_metas
+ ])
+ centers2d_inds = centers2d[:, 1] * w + centers2d[:, 0]
+ centers2d_inds = centers2d_inds.view(batch, -1)
+ pred_regression = transpose_and_gather_feat(pred_reg, centers2d_inds)
+ pred_regression_pois = pred_regression.view(-1, channel)
+ locations, dimensions, orientations = self.bbox_coder.decode(
+ pred_regression_pois, centers2d, labels3d, cam2imgs, trans_mats,
+ gt_locations)
+
+ locations, dimensions, orientations = locations[indices], dimensions[
+ indices], orientations[indices]
+
+ locations[:, 1] += dimensions[:, 1] / 2
+
+ gt_locations = gt_locations[indices]
+
+ assert len(locations) == len(gt_locations)
+ assert len(dimensions) == len(gt_dimensions)
+ assert len(orientations) == len(gt_orientations)
+ bbox3d_yaws = self.bbox_coder.encode(gt_locations, gt_dimensions,
+ orientations, img_metas)
+ bbox3d_dims = self.bbox_coder.encode(gt_locations, dimensions,
+ gt_orientations, img_metas)
+ bbox3d_locs = self.bbox_coder.encode(locations, gt_dimensions,
+ gt_orientations, img_metas)
+
+ pred_bboxes = dict(ori=bbox3d_yaws, dim=bbox3d_dims, loc=bbox3d_locs)
+
+ return pred_bboxes
+
+ def get_targets(self, gt_bboxes, gt_labels, gt_bboxes_3d, gt_labels_3d,
+ centers2d, feat_shape, img_shape, img_metas):
+ """Get training targets for batch images.
+
+ Args:
+ gt_bboxes (list[Tensor]): Ground truth bboxes of each image,
+ shape (num_gt, 4).
+ gt_labels (list[Tensor]): Ground truth labels of each box,
+ shape (num_gt,).
+ gt_bboxes_3d (list[:obj:`CameraInstance3DBoxes`]): 3D Ground
+ truth bboxes of each image,
+ shape (num_gt, bbox_code_size).
+ gt_labels_3d (list[Tensor]): 3D Ground truth labels of each
+ box, shape (num_gt,).
+ centers2d (list[Tensor]): Projected 3D centers onto 2D image,
+ shape (num_gt, 2).
+ feat_shape (tuple[int]): Feature map shape with value,
+ shape (B, _, H, W).
+ img_shape (tuple[int]): Image shape in [h, w] format.
+ img_metas (list[dict]): Meta information of each image, e.g.,
+ image size, scaling factor, etc.
+
+ Returns:
+ tuple[Tensor, dict]: The Tensor value is the targets of
+ center heatmap, the dict has components below:
+ - gt_centers2d (Tensor): Coords of each projected 3D box
+ center on image. shape (B * max_objs, 2)
+ - gt_labels3d (Tensor): Labels of each 3D box.
+ shape (B, max_objs, )
+ - indices (Tensor): Indices of the existence of the 3D box.
+ shape (B * max_objs, )
+ - affine_indices (Tensor): Indices of the affine of the 3D box.
+ shape (N, )
+ - gt_locs (Tensor): Coords of each 3D box's location.
+ shape (N, 3)
+ - gt_dims (Tensor): Dimensions of each 3D box.
+ shape (N, 3)
+ - gt_yaws (Tensor): Orientation(yaw) of each 3D box.
+ shape (N, 1)
+ - gt_cors (Tensor): Coords of the corners of each 3D box.
+ shape (N, 8, 3)
+ """
+
+ reg_mask = torch.stack([
+ gt_bboxes[0].new_tensor(
+ not img_meta['affine_aug'], dtype=torch.bool)
+ for img_meta in img_metas
+ ])
+
+ img_h, img_w = img_shape[:2]
+ bs, _, feat_h, feat_w = feat_shape
+
+ width_ratio = float(feat_w / img_w) # 1/4
+ height_ratio = float(feat_h / img_h) # 1/4
+
+ assert width_ratio == height_ratio
+
+ center_heatmap_target = gt_bboxes[-1].new_zeros(
+ [bs, self.num_classes, feat_h, feat_w])
+
+ gt_centers2d = centers2d.copy()
+
+ for batch_id in range(bs):
+ gt_bbox = gt_bboxes[batch_id]
+ gt_label = gt_labels[batch_id]
+ # project centers2d from input image to feat map
+ gt_center2d = gt_centers2d[batch_id] * width_ratio
+
+ for j, center in enumerate(gt_center2d):
+ center_x_int, center_y_int = center.int()
+ scale_box_h = (gt_bbox[j][3] - gt_bbox[j][1]) * height_ratio
+ scale_box_w = (gt_bbox[j][2] - gt_bbox[j][0]) * width_ratio
+ radius = gaussian_radius([scale_box_h, scale_box_w],
+ min_overlap=0.7)
+ radius = max(0, int(radius))
+ ind = gt_label[j]
+ gen_gaussian_target(center_heatmap_target[batch_id, ind],
+ [center_x_int, center_y_int], radius)
+
+ avg_factor = max(1, center_heatmap_target.eq(1).sum())
+ num_ctrs = [center2d.shape[0] for center2d in centers2d]
+ max_objs = max(num_ctrs)
+
+ reg_inds = torch.cat(
+ [reg_mask[i].repeat(num_ctrs[i]) for i in range(bs)])
+
+ inds = torch.zeros((bs, max_objs),
+ dtype=torch.bool).to(centers2d[0].device)
+
+ # put gt 3d bboxes to gpu
+ gt_bboxes_3d = [
+ gt_bbox_3d.to(centers2d[0].device) for gt_bbox_3d in gt_bboxes_3d
+ ]
+
+ batch_centers2d = centers2d[0].new_zeros((bs, max_objs, 2))
+ batch_labels_3d = gt_labels_3d[0].new_zeros((bs, max_objs))
+ batch_gt_locations = \
+ gt_bboxes_3d[0].tensor.new_zeros((bs, max_objs, 3))
+ for i in range(bs):
+ inds[i, :num_ctrs[i]] = 1
+ batch_centers2d[i, :num_ctrs[i]] = centers2d[i]
+ batch_labels_3d[i, :num_ctrs[i]] = gt_labels_3d[i]
+ batch_gt_locations[i, :num_ctrs[i]] = \
+ gt_bboxes_3d[i].tensor[:, :3]
+
+ inds = inds.flatten()
+ batch_centers2d = batch_centers2d.view(-1, 2) * width_ratio
+ batch_gt_locations = batch_gt_locations.view(-1, 3)
+
+ # filter the empty image, without gt_bboxes_3d
+ gt_bboxes_3d = [
+ gt_bbox_3d for gt_bbox_3d in gt_bboxes_3d
+ if gt_bbox_3d.tensor.shape[0] > 0
+ ]
+
+ gt_dimensions = torch.cat(
+ [gt_bbox_3d.tensor[:, 3:6] for gt_bbox_3d in gt_bboxes_3d])
+ gt_orientations = torch.cat([
+ gt_bbox_3d.tensor[:, 6].unsqueeze(-1)
+ for gt_bbox_3d in gt_bboxes_3d
+ ])
+ gt_corners = torch.cat(
+ [gt_bbox_3d.corners for gt_bbox_3d in gt_bboxes_3d])
+
+ target_labels = dict(
+ gt_centers2d=batch_centers2d.long(),
+ gt_labels3d=batch_labels_3d,
+ indices=inds,
+ reg_indices=reg_inds,
+ gt_locs=batch_gt_locations,
+ gt_dims=gt_dimensions,
+ gt_yaws=gt_orientations,
+ gt_cors=gt_corners)
+
+ return center_heatmap_target, avg_factor, target_labels
+
+ def loss(self,
+ cls_scores,
+ bbox_preds,
+ gt_bboxes,
+ gt_labels,
+ gt_bboxes_3d,
+ gt_labels_3d,
+ centers2d,
+ depths,
+ attr_labels,
+ img_metas,
+ gt_bboxes_ignore=None):
+ """Compute loss of the head.
+
+ Args:
+ cls_scores (list[Tensor]): Box scores for each scale level.
+ shape (num_gt, 4).
+ bbox_preds (list[Tensor]): Box dims is a 4D-tensor, the channel
+ number is bbox_code_size.
+ shape (B, 7, H, W).
+ gt_bboxes (list[Tensor]): Ground truth bboxes for each image.
+ shape (num_gts, 4) in [tl_x, tl_y, br_x, br_y] format.
+ gt_labels (list[Tensor]): Class indices corresponding to each box.
+ shape (num_gts, ).
+ gt_bboxes_3d (list[:obj:`CameraInstance3DBoxes`]): 3D boxes ground
+ truth. it is the flipped gt_bboxes
+ gt_labels_3d (list[Tensor]): Same as gt_labels.
+ centers2d (list[Tensor]): 2D centers on the image.
+ shape (num_gts, 2).
+ depths (list[Tensor]): Depth ground truth.
+ shape (num_gts, ).
+ attr_labels (list[Tensor]): Attributes indices of each box.
+ In kitti it's None.
+ img_metas (list[dict]): Meta information of each image, e.g.,
+ image size, scaling factor, etc.
+ gt_bboxes_ignore (None | list[Tensor]): Specify which bounding
+ boxes can be ignored when computing the loss.
+ Default: None.
+
+ Returns:
+ dict[str, Tensor]: A dictionary of loss components.
+ """
+ assert len(cls_scores) == len(bbox_preds) == 1
+ assert attr_labels is None
+ assert gt_bboxes_ignore is None
+ center2d_heatmap = cls_scores[0]
+ pred_reg = bbox_preds[0]
+
+ center2d_heatmap_target, avg_factor, target_labels = \
+ self.get_targets(gt_bboxes, gt_labels, gt_bboxes_3d,
+ gt_labels_3d, centers2d,
+ center2d_heatmap.shape,
+ img_metas[0]['pad_shape'],
+ img_metas)
+
+ pred_bboxes = self.get_predictions(
+ labels3d=target_labels['gt_labels3d'],
+ centers2d=target_labels['gt_centers2d'],
+ gt_locations=target_labels['gt_locs'],
+ gt_dimensions=target_labels['gt_dims'],
+ gt_orientations=target_labels['gt_yaws'],
+ indices=target_labels['indices'],
+ img_metas=img_metas,
+ pred_reg=pred_reg)
+
+ loss_cls = self.loss_cls(
+ center2d_heatmap, center2d_heatmap_target, avg_factor=avg_factor)
+
+ reg_inds = target_labels['reg_indices']
+
+ loss_bbox_oris = self.loss_bbox(
+ pred_bboxes['ori'].corners[reg_inds, ...],
+ target_labels['gt_cors'][reg_inds, ...])
+
+ loss_bbox_dims = self.loss_bbox(
+ pred_bboxes['dim'].corners[reg_inds, ...],
+ target_labels['gt_cors'][reg_inds, ...])
+
+ loss_bbox_locs = self.loss_bbox(
+ pred_bboxes['loc'].corners[reg_inds, ...],
+ target_labels['gt_cors'][reg_inds, ...])
+
+ loss_bbox = loss_bbox_dims + loss_bbox_locs + loss_bbox_oris
+
+ loss_dict = dict(loss_cls=loss_cls, loss_bbox=loss_bbox)
+
+ return loss_dict
diff --git a/mmdet3d/models/dense_heads/ssd_3d_head.py b/mmdet3d/models/dense_heads/ssd_3d_head.py
new file mode 100644
index 0000000..c20c4b1
--- /dev/null
+++ b/mmdet3d/models/dense_heads/ssd_3d_head.py
@@ -0,0 +1,557 @@
+# Copyright (c) OpenMMLab. All rights reserved.
+import torch
+from mmcv.ops.nms import batched_nms
+from mmcv.runner import force_fp32
+from torch.nn import functional as F
+
+from mmdet3d.core.bbox.structures import (DepthInstance3DBoxes,
+ LiDARInstance3DBoxes,
+ rotation_3d_in_axis)
+from mmdet.core import multi_apply
+from ..builder import HEADS, build_loss
+from .vote_head import VoteHead
+
+
+@HEADS.register_module()
+class SSD3DHead(VoteHead):
+ r"""Bbox head of `3DSSD `_.
+
+ Args:
+ num_classes (int): The number of class.
+ bbox_coder (:obj:`BaseBBoxCoder`): Bbox coder for encoding and
+ decoding boxes.
+ in_channels (int): The number of input feature channel.
+ train_cfg (dict): Config for training.
+ test_cfg (dict): Config for testing.
+ vote_module_cfg (dict): Config of VoteModule for point-wise votes.
+ vote_aggregation_cfg (dict): Config of vote aggregation layer.
+ pred_layer_cfg (dict): Config of classfication and regression
+ prediction layers.
+ conv_cfg (dict): Config of convolution in prediction layer.
+ norm_cfg (dict): Config of BN in prediction layer.
+ act_cfg (dict): Config of activation in prediction layer.
+ objectness_loss (dict): Config of objectness loss.
+ center_loss (dict): Config of center loss.
+ dir_class_loss (dict): Config of direction classification loss.
+ dir_res_loss (dict): Config of direction residual regression loss.
+ size_res_loss (dict): Config of size residual regression loss.
+ corner_loss (dict): Config of bbox corners regression loss.
+ vote_loss (dict): Config of candidate points regression loss.
+ """
+
+ def __init__(self,
+ num_classes,
+ bbox_coder,
+ in_channels=256,
+ train_cfg=None,
+ test_cfg=None,
+ vote_module_cfg=None,
+ vote_aggregation_cfg=None,
+ pred_layer_cfg=None,
+ conv_cfg=dict(type='Conv1d'),
+ norm_cfg=dict(type='BN1d'),
+ act_cfg=dict(type='ReLU'),
+ objectness_loss=None,
+ center_loss=None,
+ dir_class_loss=None,
+ dir_res_loss=None,
+ size_res_loss=None,
+ corner_loss=None,
+ vote_loss=None,
+ init_cfg=None):
+ super(SSD3DHead, self).__init__(
+ num_classes,
+ bbox_coder,
+ train_cfg=train_cfg,
+ test_cfg=test_cfg,
+ vote_module_cfg=vote_module_cfg,
+ vote_aggregation_cfg=vote_aggregation_cfg,
+ pred_layer_cfg=pred_layer_cfg,
+ conv_cfg=conv_cfg,
+ norm_cfg=norm_cfg,
+ objectness_loss=objectness_loss,
+ center_loss=center_loss,
+ dir_class_loss=dir_class_loss,
+ dir_res_loss=dir_res_loss,
+ size_class_loss=None,
+ size_res_loss=size_res_loss,
+ semantic_loss=None,
+ init_cfg=init_cfg)
+
+ self.corner_loss = build_loss(corner_loss)
+ self.vote_loss = build_loss(vote_loss)
+ self.num_candidates = vote_module_cfg['num_points']
+
+ def _get_cls_out_channels(self):
+ """Return the channel number of classification outputs."""
+ # Class numbers (k) + objectness (1)
+ return self.num_classes
+
+ def _get_reg_out_channels(self):
+ """Return the channel number of regression outputs."""
+ # Bbox classification and regression
+ # (center residual (3), size regression (3)
+ # heading class+residual (num_dir_bins*2)),
+ return 3 + 3 + self.num_dir_bins * 2
+
+ def _extract_input(self, feat_dict):
+ """Extract inputs from features dictionary.
+
+ Args:
+ feat_dict (dict): Feature dict from backbone.
+
+ Returns:
+ torch.Tensor: Coordinates of input points.
+ torch.Tensor: Features of input points.
+ torch.Tensor: Indices of input points.
+ """
+ seed_points = feat_dict['sa_xyz'][-1]
+ seed_features = feat_dict['sa_features'][-1]
+ seed_indices = feat_dict['sa_indices'][-1]
+
+ return seed_points, seed_features, seed_indices
+
+ @force_fp32(apply_to=('bbox_preds', ))
+ def loss(self,
+ bbox_preds,
+ points,
+ gt_bboxes_3d,
+ gt_labels_3d,
+ pts_semantic_mask=None,
+ pts_instance_mask=None,
+ img_metas=None,
+ gt_bboxes_ignore=None):
+ """Compute loss.
+
+ Args:
+ bbox_preds (dict): Predictions from forward of SSD3DHead.
+ points (list[torch.Tensor]): Input points.
+ gt_bboxes_3d (list[:obj:`BaseInstance3DBoxes`]): Ground truth
+ bboxes of each sample.
+ gt_labels_3d (list[torch.Tensor]): Labels of each sample.
+ pts_semantic_mask (list[torch.Tensor]): Point-wise
+ semantic mask.
+ pts_instance_mask (list[torch.Tensor]): Point-wise
+ instance mask.
+ img_metas (list[dict]): Contain pcd and img's meta info.
+ gt_bboxes_ignore (list[torch.Tensor]): Specify
+ which bounding.
+
+ Returns:
+ dict: Losses of 3DSSD.
+ """
+ targets = self.get_targets(points, gt_bboxes_3d, gt_labels_3d,
+ pts_semantic_mask, pts_instance_mask,
+ bbox_preds)
+ (vote_targets, center_targets, size_res_targets, dir_class_targets,
+ dir_res_targets, mask_targets, centerness_targets, corner3d_targets,
+ vote_mask, positive_mask, negative_mask, centerness_weights,
+ box_loss_weights, heading_res_loss_weight) = targets
+
+ # calculate centerness loss
+ centerness_loss = self.objectness_loss(
+ bbox_preds['obj_scores'].transpose(2, 1),
+ centerness_targets,
+ weight=centerness_weights)
+
+ # calculate center loss
+ center_loss = self.center_loss(
+ bbox_preds['center_offset'],
+ center_targets,
+ weight=box_loss_weights.unsqueeze(-1))
+
+ # calculate direction class loss
+ dir_class_loss = self.dir_class_loss(
+ bbox_preds['dir_class'].transpose(1, 2),
+ dir_class_targets,
+ weight=box_loss_weights)
+
+ # calculate direction residual loss
+ dir_res_loss = self.dir_res_loss(
+ bbox_preds['dir_res_norm'],
+ dir_res_targets.unsqueeze(-1).repeat(1, 1, self.num_dir_bins),
+ weight=heading_res_loss_weight)
+
+ # calculate size residual loss
+ size_loss = self.size_res_loss(
+ bbox_preds['size'],
+ size_res_targets,
+ weight=box_loss_weights.unsqueeze(-1))
+
+ # calculate corner loss
+ one_hot_dir_class_targets = dir_class_targets.new_zeros(
+ bbox_preds['dir_class'].shape)
+ one_hot_dir_class_targets.scatter_(2, dir_class_targets.unsqueeze(-1),
+ 1)
+ pred_bbox3d = self.bbox_coder.decode(
+ dict(
+ center=bbox_preds['center'],
+ dir_res=bbox_preds['dir_res'],
+ dir_class=one_hot_dir_class_targets,
+ size=bbox_preds['size']))
+ pred_bbox3d = pred_bbox3d.reshape(-1, pred_bbox3d.shape[-1])
+ pred_bbox3d = img_metas[0]['box_type_3d'](
+ pred_bbox3d.clone(),
+ box_dim=pred_bbox3d.shape[-1],
+ with_yaw=self.bbox_coder.with_rot,
+ origin=(0.5, 0.5, 0.5))
+ pred_corners3d = pred_bbox3d.corners.reshape(-1, 8, 3)
+ corner_loss = self.corner_loss(
+ pred_corners3d,
+ corner3d_targets.reshape(-1, 8, 3),
+ weight=box_loss_weights.view(-1, 1, 1))
+
+ # calculate vote loss
+ vote_loss = self.vote_loss(
+ bbox_preds['vote_offset'].transpose(1, 2),
+ vote_targets,
+ weight=vote_mask.unsqueeze(-1))
+
+ losses = dict(
+ centerness_loss=centerness_loss,
+ center_loss=center_loss,
+ dir_class_loss=dir_class_loss,
+ dir_res_loss=dir_res_loss,
+ size_res_loss=size_loss,
+ corner_loss=corner_loss,
+ vote_loss=vote_loss)
+
+ return losses
+
+ def get_targets(self,
+ points,
+ gt_bboxes_3d,
+ gt_labels_3d,
+ pts_semantic_mask=None,
+ pts_instance_mask=None,
+ bbox_preds=None):
+ """Generate targets of ssd3d head.
+
+ Args:
+ points (list[torch.Tensor]): Points of each batch.
+ gt_bboxes_3d (list[:obj:`BaseInstance3DBoxes`]): Ground truth
+ bboxes of each batch.
+ gt_labels_3d (list[torch.Tensor]): Labels of each batch.
+ pts_semantic_mask (list[torch.Tensor]): Point-wise semantic
+ label of each batch.
+ pts_instance_mask (list[torch.Tensor]): Point-wise instance
+ label of each batch.
+ bbox_preds (torch.Tensor): Bounding box predictions of ssd3d head.
+
+ Returns:
+ tuple[torch.Tensor]: Targets of ssd3d head.
+ """
+ # find empty example
+ for index in range(len(gt_labels_3d)):
+ if len(gt_labels_3d[index]) == 0:
+ fake_box = gt_bboxes_3d[index].tensor.new_zeros(
+ 1, gt_bboxes_3d[index].tensor.shape[-1])
+ gt_bboxes_3d[index] = gt_bboxes_3d[index].new_box(fake_box)
+ gt_labels_3d[index] = gt_labels_3d[index].new_zeros(1)
+
+ if pts_semantic_mask is None:
+ pts_semantic_mask = [None for i in range(len(gt_labels_3d))]
+ pts_instance_mask = [None for i in range(len(gt_labels_3d))]
+
+ aggregated_points = [
+ bbox_preds['aggregated_points'][i]
+ for i in range(len(gt_labels_3d))
+ ]
+
+ seed_points = [
+ bbox_preds['seed_points'][i, :self.num_candidates].detach()
+ for i in range(len(gt_labels_3d))
+ ]
+
+ (vote_targets, center_targets, size_res_targets, dir_class_targets,
+ dir_res_targets, mask_targets, centerness_targets, corner3d_targets,
+ vote_mask, positive_mask, negative_mask) = multi_apply(
+ self.get_targets_single, points, gt_bboxes_3d, gt_labels_3d,
+ pts_semantic_mask, pts_instance_mask, aggregated_points,
+ seed_points)
+
+ center_targets = torch.stack(center_targets)
+ positive_mask = torch.stack(positive_mask)
+ negative_mask = torch.stack(negative_mask)
+ dir_class_targets = torch.stack(dir_class_targets)
+ dir_res_targets = torch.stack(dir_res_targets)
+ size_res_targets = torch.stack(size_res_targets)
+ mask_targets = torch.stack(mask_targets)
+ centerness_targets = torch.stack(centerness_targets).detach()
+ corner3d_targets = torch.stack(corner3d_targets)
+ vote_targets = torch.stack(vote_targets)
+ vote_mask = torch.stack(vote_mask)
+
+ center_targets -= bbox_preds['aggregated_points']
+
+ centerness_weights = (positive_mask +
+ negative_mask).unsqueeze(-1).repeat(
+ 1, 1, self.num_classes).float()
+ centerness_weights = centerness_weights / \
+ (centerness_weights.sum() + 1e-6)
+ vote_mask = vote_mask / (vote_mask.sum() + 1e-6)
+
+ box_loss_weights = positive_mask / (positive_mask.sum() + 1e-6)
+
+ batch_size, proposal_num = dir_class_targets.shape[:2]
+ heading_label_one_hot = dir_class_targets.new_zeros(
+ (batch_size, proposal_num, self.num_dir_bins))
+ heading_label_one_hot.scatter_(2, dir_class_targets.unsqueeze(-1), 1)
+ heading_res_loss_weight = heading_label_one_hot * \
+ box_loss_weights.unsqueeze(-1)
+
+ return (vote_targets, center_targets, size_res_targets,
+ dir_class_targets, dir_res_targets, mask_targets,
+ centerness_targets, corner3d_targets, vote_mask, positive_mask,
+ negative_mask, centerness_weights, box_loss_weights,
+ heading_res_loss_weight)
+
+ def get_targets_single(self,
+ points,
+ gt_bboxes_3d,
+ gt_labels_3d,
+ pts_semantic_mask=None,
+ pts_instance_mask=None,
+ aggregated_points=None,
+ seed_points=None):
+ """Generate targets of ssd3d head for single batch.
+
+ Args:
+ points (torch.Tensor): Points of each batch.
+ gt_bboxes_3d (:obj:`BaseInstance3DBoxes`): Ground truth
+ boxes of each batch.
+ gt_labels_3d (torch.Tensor): Labels of each batch.
+ pts_semantic_mask (torch.Tensor): Point-wise semantic
+ label of each batch.
+ pts_instance_mask (torch.Tensor): Point-wise instance
+ label of each batch.
+ aggregated_points (torch.Tensor): Aggregated points from
+ candidate points layer.
+ seed_points (torch.Tensor): Seed points of candidate points.
+
+ Returns:
+ tuple[torch.Tensor]: Targets of ssd3d head.
+ """
+ assert self.bbox_coder.with_rot or pts_semantic_mask is not None
+ gt_bboxes_3d = gt_bboxes_3d.to(points.device)
+ valid_gt = gt_labels_3d != -1
+ gt_bboxes_3d = gt_bboxes_3d[valid_gt]
+ gt_labels_3d = gt_labels_3d[valid_gt]
+
+ # Generate fake GT for empty scene
+ if valid_gt.sum() == 0:
+ vote_targets = points.new_zeros(self.num_candidates, 3)
+ center_targets = points.new_zeros(self.num_candidates, 3)
+ size_res_targets = points.new_zeros(self.num_candidates, 3)
+ dir_class_targets = points.new_zeros(
+ self.num_candidates, dtype=torch.int64)
+ dir_res_targets = points.new_zeros(self.num_candidates)
+ mask_targets = points.new_zeros(
+ self.num_candidates, dtype=torch.int64)
+ centerness_targets = points.new_zeros(self.num_candidates,
+ self.num_classes)
+ corner3d_targets = points.new_zeros(self.num_candidates, 8, 3)
+ vote_mask = points.new_zeros(self.num_candidates, dtype=torch.bool)
+ positive_mask = points.new_zeros(
+ self.num_candidates, dtype=torch.bool)
+ negative_mask = points.new_ones(
+ self.num_candidates, dtype=torch.bool)
+ return (vote_targets, center_targets, size_res_targets,
+ dir_class_targets, dir_res_targets, mask_targets,
+ centerness_targets, corner3d_targets, vote_mask,
+ positive_mask, negative_mask)
+
+ gt_corner3d = gt_bboxes_3d.corners
+
+ (center_targets, size_targets, dir_class_targets,
+ dir_res_targets) = self.bbox_coder.encode(gt_bboxes_3d, gt_labels_3d)
+
+ points_mask, assignment = self._assign_targets_by_points_inside(
+ gt_bboxes_3d, aggregated_points)
+
+ center_targets = center_targets[assignment]
+ size_res_targets = size_targets[assignment]
+ mask_targets = gt_labels_3d[assignment]
+ dir_class_targets = dir_class_targets[assignment]
+ dir_res_targets = dir_res_targets[assignment]
+ corner3d_targets = gt_corner3d[assignment]
+
+ top_center_targets = center_targets.clone()
+ top_center_targets[:, 2] += size_res_targets[:, 2]
+ dist = torch.norm(aggregated_points - top_center_targets, dim=1)
+ dist_mask = dist < self.train_cfg.pos_distance_thr
+ positive_mask = (points_mask.max(1)[0] > 0) * dist_mask
+ negative_mask = (points_mask.max(1)[0] == 0)
+
+ # Centerness loss targets
+ canonical_xyz = aggregated_points - center_targets
+ if self.bbox_coder.with_rot:
+ # TODO: Align points rotation implementation of
+ # LiDARInstance3DBoxes and DepthInstance3DBoxes
+ canonical_xyz = rotation_3d_in_axis(
+ canonical_xyz.unsqueeze(0).transpose(0, 1),
+ -gt_bboxes_3d.yaw[assignment],
+ axis=2).squeeze(1)
+ distance_front = torch.clamp(
+ size_res_targets[:, 0] - canonical_xyz[:, 0], min=0)
+ distance_back = torch.clamp(
+ size_res_targets[:, 0] + canonical_xyz[:, 0], min=0)
+ distance_left = torch.clamp(
+ size_res_targets[:, 1] - canonical_xyz[:, 1], min=0)
+ distance_right = torch.clamp(
+ size_res_targets[:, 1] + canonical_xyz[:, 1], min=0)
+ distance_top = torch.clamp(
+ size_res_targets[:, 2] - canonical_xyz[:, 2], min=0)
+ distance_bottom = torch.clamp(
+ size_res_targets[:, 2] + canonical_xyz[:, 2], min=0)
+
+ centerness_l = torch.min(distance_front, distance_back) / torch.max(
+ distance_front, distance_back)
+ centerness_w = torch.min(distance_left, distance_right) / torch.max(
+ distance_left, distance_right)
+ centerness_h = torch.min(distance_bottom, distance_top) / torch.max(
+ distance_bottom, distance_top)
+ centerness_targets = torch.clamp(
+ centerness_l * centerness_w * centerness_h, min=0)
+ centerness_targets = centerness_targets.pow(1 / 3.0)
+ centerness_targets = torch.clamp(centerness_targets, min=0, max=1)
+
+ proposal_num = centerness_targets.shape[0]
+ one_hot_centerness_targets = centerness_targets.new_zeros(
+ (proposal_num, self.num_classes))
+ one_hot_centerness_targets.scatter_(1, mask_targets.unsqueeze(-1), 1)
+ centerness_targets = centerness_targets.unsqueeze(
+ 1) * one_hot_centerness_targets
+
+ # Vote loss targets
+ enlarged_gt_bboxes_3d = gt_bboxes_3d.enlarged_box(
+ self.train_cfg.expand_dims_length)
+ enlarged_gt_bboxes_3d.tensor[:, 2] -= self.train_cfg.expand_dims_length
+ vote_mask, vote_assignment = self._assign_targets_by_points_inside(
+ enlarged_gt_bboxes_3d, seed_points)
+
+ vote_targets = gt_bboxes_3d.gravity_center
+ vote_targets = vote_targets[vote_assignment] - seed_points
+ vote_mask = vote_mask.max(1)[0] > 0
+
+ return (vote_targets, center_targets, size_res_targets,
+ dir_class_targets, dir_res_targets, mask_targets,
+ centerness_targets, corner3d_targets, vote_mask, positive_mask,
+ negative_mask)
+
+ def get_bboxes(self, points, bbox_preds, input_metas, rescale=False):
+ """Generate bboxes from 3DSSD head predictions.
+
+ Args:
+ points (torch.Tensor): Input points.
+ bbox_preds (dict): Predictions from sdd3d head.
+ input_metas (list[dict]): Point cloud and image's meta info.
+ rescale (bool): Whether to rescale bboxes.
+
+ Returns:
+ list[tuple[torch.Tensor]]: Bounding boxes, scores and labels.
+ """
+ # decode boxes
+ sem_scores = F.sigmoid(bbox_preds['obj_scores']).transpose(1, 2)
+ obj_scores = sem_scores.max(-1)[0]
+ bbox3d = self.bbox_coder.decode(bbox_preds)
+
+ batch_size = bbox3d.shape[0]
+ results = list()
+
+ for b in range(batch_size):
+ bbox_selected, score_selected, labels = self.multiclass_nms_single(
+ obj_scores[b], sem_scores[b], bbox3d[b], points[b, ..., :3],
+ input_metas[b])
+
+ bbox = input_metas[b]['box_type_3d'](
+ bbox_selected.clone(),
+ box_dim=bbox_selected.shape[-1],
+ with_yaw=self.bbox_coder.with_rot)
+ results.append((bbox, score_selected, labels))
+
+ return results
+
+ def multiclass_nms_single(self, obj_scores, sem_scores, bbox, points,
+ input_meta):
+ """Multi-class nms in single batch.
+
+ Args:
+ obj_scores (torch.Tensor): Objectness score of bounding boxes.
+ sem_scores (torch.Tensor): Semantic class score of bounding boxes.
+ bbox (torch.Tensor): Predicted bounding boxes.
+ points (torch.Tensor): Input points.
+ input_meta (dict): Point cloud and image's meta info.
+
+ Returns:
+ tuple[torch.Tensor]: Bounding boxes, scores and labels.
+ """
+ bbox = input_meta['box_type_3d'](
+ bbox.clone(),
+ box_dim=bbox.shape[-1],
+ with_yaw=self.bbox_coder.with_rot,
+ origin=(0.5, 0.5, 0.5))
+
+ if isinstance(bbox, (LiDARInstance3DBoxes, DepthInstance3DBoxes)):
+ box_indices = bbox.points_in_boxes_all(points)
+ nonempty_box_mask = box_indices.T.sum(1) >= 0
+ else:
+ raise NotImplementedError('Unsupported bbox type!')
+
+ corner3d = bbox.corners
+ minmax_box3d = corner3d.new(torch.Size((corner3d.shape[0], 6)))
+ minmax_box3d[:, :3] = torch.min(corner3d, dim=1)[0]
+ minmax_box3d[:, 3:] = torch.max(corner3d, dim=1)[0]
+
+ bbox_classes = torch.argmax(sem_scores, -1)
+ nms_keep = batched_nms(
+ minmax_box3d[nonempty_box_mask][:, [0, 1, 3, 4]],
+ obj_scores[nonempty_box_mask], bbox_classes[nonempty_box_mask],
+ self.test_cfg.nms_cfg)[1]
+
+ if nms_keep.shape[0] > self.test_cfg.max_output_num:
+ nms_keep = nms_keep[:self.test_cfg.max_output_num]
+
+ # filter empty boxes and boxes with low score
+ scores_mask = (obj_scores >= self.test_cfg.score_thr)
+ nonempty_box_inds = torch.nonzero(
+ nonempty_box_mask, as_tuple=False).flatten()
+ nonempty_mask = torch.zeros_like(bbox_classes).scatter(
+ 0, nonempty_box_inds[nms_keep], 1)
+ selected = (nonempty_mask.bool() & scores_mask.bool())
+
+ if self.test_cfg.per_class_proposal:
+ bbox_selected, score_selected, labels = [], [], []
+ for k in range(sem_scores.shape[-1]):
+ bbox_selected.append(bbox[selected].tensor)
+ score_selected.append(obj_scores[selected])
+ labels.append(
+ torch.zeros_like(bbox_classes[selected]).fill_(k))
+ bbox_selected = torch.cat(bbox_selected, 0)
+ score_selected = torch.cat(score_selected, 0)
+ labels = torch.cat(labels, 0)
+ else:
+ bbox_selected = bbox[selected].tensor
+ score_selected = obj_scores[selected]
+ labels = bbox_classes[selected]
+
+ return bbox_selected, score_selected, labels
+
+ def _assign_targets_by_points_inside(self, bboxes_3d, points):
+ """Compute assignment by checking whether point is inside bbox.
+
+ Args:
+ bboxes_3d (BaseInstance3DBoxes): Instance of bounding boxes.
+ points (torch.Tensor): Points of a batch.
+
+ Returns:
+ tuple[torch.Tensor]: Flags indicating whether each point is
+ inside bbox and the index of box where each point are in.
+ """
+ if isinstance(bboxes_3d, (LiDARInstance3DBoxes, DepthInstance3DBoxes)):
+ points_mask = bboxes_3d.points_in_boxes_all(points)
+ assignment = points_mask.argmax(dim=-1)
+ else:
+ raise NotImplementedError('Unsupported bbox type!')
+
+ return points_mask, assignment
diff --git a/mmdet3d/models/dense_heads/train_mixins.py b/mmdet3d/models/dense_heads/train_mixins.py
new file mode 100644
index 0000000..90c9cbb
--- /dev/null
+++ b/mmdet3d/models/dense_heads/train_mixins.py
@@ -0,0 +1,349 @@
+# Copyright (c) OpenMMLab. All rights reserved.
+import numpy as np
+import torch
+
+from mmdet3d.core import limit_period
+from mmdet.core import images_to_levels, multi_apply
+
+
+class AnchorTrainMixin(object):
+ """Mixin class for target assigning of dense heads."""
+
+ def anchor_target_3d(self,
+ anchor_list,
+ gt_bboxes_list,
+ input_metas,
+ gt_bboxes_ignore_list=None,
+ gt_labels_list=None,
+ label_channels=1,
+ num_classes=1,
+ sampling=True):
+ """Compute regression and classification targets for anchors.
+
+ Args:
+ anchor_list (list[list]): Multi level anchors of each image.
+ gt_bboxes_list (list[:obj:`BaseInstance3DBoxes`]): Ground truth
+ bboxes of each image.
+ input_metas (list[dict]): Meta info of each image.
+ gt_bboxes_ignore_list (list): Ignore list of gt bboxes.
+ gt_labels_list (list[torch.Tensor]): Gt labels of batches.
+ label_channels (int): The channel of labels.
+ num_classes (int): The number of classes.
+ sampling (bool): Whether to sample anchors.
+
+ Returns:
+ tuple (list, list, list, list, list, list, int, int):
+ Anchor targets, including labels, label weights,
+ bbox targets, bbox weights, direction targets,
+ direction weights, number of positive anchors and
+ number of negative anchors.
+ """
+ num_imgs = len(input_metas)
+ assert len(anchor_list) == num_imgs
+
+ if isinstance(anchor_list[0][0], list):
+ # sizes of anchors are different
+ # anchor number of a single level
+ num_level_anchors = [
+ sum([anchor.size(0) for anchor in anchors])
+ for anchors in anchor_list[0]
+ ]
+ for i in range(num_imgs):
+ anchor_list[i] = anchor_list[i][0]
+ else:
+ # anchor number of multi levels
+ num_level_anchors = [
+ anchors.view(-1, self.box_code_size).size(0)
+ for anchors in anchor_list[0]
+ ]
+ # concat all level anchors and flags to a single tensor
+ for i in range(num_imgs):
+ anchor_list[i] = torch.cat(anchor_list[i])
+
+ # compute targets for each image
+ if gt_bboxes_ignore_list is None:
+ gt_bboxes_ignore_list = [None for _ in range(num_imgs)]
+ if gt_labels_list is None:
+ gt_labels_list = [None for _ in range(num_imgs)]
+
+ (all_labels, all_label_weights, all_bbox_targets, all_bbox_weights,
+ all_dir_targets, all_dir_weights, pos_inds_list,
+ neg_inds_list) = multi_apply(
+ self.anchor_target_3d_single,
+ anchor_list,
+ gt_bboxes_list,
+ gt_bboxes_ignore_list,
+ gt_labels_list,
+ input_metas,
+ label_channels=label_channels,
+ num_classes=num_classes,
+ sampling=sampling)
+
+ # no valid anchors
+ if any([labels is None for labels in all_labels]):
+ return None
+ # sampled anchors of all images
+ num_total_pos = sum([max(inds.numel(), 1) for inds in pos_inds_list])
+ num_total_neg = sum([max(inds.numel(), 1) for inds in neg_inds_list])
+ # split targets to a list w.r.t. multiple levels
+ labels_list = images_to_levels(all_labels, num_level_anchors)
+ label_weights_list = images_to_levels(all_label_weights,
+ num_level_anchors)
+ bbox_targets_list = images_to_levels(all_bbox_targets,
+ num_level_anchors)
+ bbox_weights_list = images_to_levels(all_bbox_weights,
+ num_level_anchors)
+ dir_targets_list = images_to_levels(all_dir_targets, num_level_anchors)
+ dir_weights_list = images_to_levels(all_dir_weights, num_level_anchors)
+ return (labels_list, label_weights_list, bbox_targets_list,
+ bbox_weights_list, dir_targets_list, dir_weights_list,
+ num_total_pos, num_total_neg)
+
+ def anchor_target_3d_single(self,
+ anchors,
+ gt_bboxes,
+ gt_bboxes_ignore,
+ gt_labels,
+ input_meta,
+ label_channels=1,
+ num_classes=1,
+ sampling=True):
+ """Compute targets of anchors in single batch.
+
+ Args:
+ anchors (torch.Tensor): Concatenated multi-level anchor.
+ gt_bboxes (:obj:`BaseInstance3DBoxes`): Gt bboxes.
+ gt_bboxes_ignore (torch.Tensor): Ignored gt bboxes.
+ gt_labels (torch.Tensor): Gt class labels.
+ input_meta (dict): Meta info of each image.
+ label_channels (int): The channel of labels.
+ num_classes (int): The number of classes.
+ sampling (bool): Whether to sample anchors.
+
+ Returns:
+ tuple[torch.Tensor]: Anchor targets.
+ """
+ if isinstance(self.bbox_assigner,
+ list) and (not isinstance(anchors, list)):
+ feat_size = anchors.size(0) * anchors.size(1) * anchors.size(2)
+ rot_angles = anchors.size(-2)
+ assert len(self.bbox_assigner) == anchors.size(-3)
+ (total_labels, total_label_weights, total_bbox_targets,
+ total_bbox_weights, total_dir_targets, total_dir_weights,
+ total_pos_inds, total_neg_inds) = [], [], [], [], [], [], [], []
+ current_anchor_num = 0
+ for i, assigner in enumerate(self.bbox_assigner):
+ current_anchors = anchors[..., i, :, :].reshape(
+ -1, self.box_code_size)
+ current_anchor_num += current_anchors.size(0)
+ if self.assign_per_class:
+ gt_per_cls = (gt_labels == i)
+ anchor_targets = self.anchor_target_single_assigner(
+ assigner, current_anchors, gt_bboxes[gt_per_cls, :],
+ gt_bboxes_ignore, gt_labels[gt_per_cls], input_meta,
+ num_classes, sampling)
+ else:
+ anchor_targets = self.anchor_target_single_assigner(
+ assigner, current_anchors, gt_bboxes, gt_bboxes_ignore,
+ gt_labels, input_meta, num_classes, sampling)
+
+ (labels, label_weights, bbox_targets, bbox_weights,
+ dir_targets, dir_weights, pos_inds, neg_inds) = anchor_targets
+ total_labels.append(labels.reshape(feat_size, 1, rot_angles))
+ total_label_weights.append(
+ label_weights.reshape(feat_size, 1, rot_angles))
+ total_bbox_targets.append(
+ bbox_targets.reshape(feat_size, 1, rot_angles,
+ anchors.size(-1)))
+ total_bbox_weights.append(
+ bbox_weights.reshape(feat_size, 1, rot_angles,
+ anchors.size(-1)))
+ total_dir_targets.append(
+ dir_targets.reshape(feat_size, 1, rot_angles))
+ total_dir_weights.append(
+ dir_weights.reshape(feat_size, 1, rot_angles))
+ total_pos_inds.append(pos_inds)
+ total_neg_inds.append(neg_inds)
+
+ total_labels = torch.cat(total_labels, dim=-2).reshape(-1)
+ total_label_weights = torch.cat(
+ total_label_weights, dim=-2).reshape(-1)
+ total_bbox_targets = torch.cat(
+ total_bbox_targets, dim=-3).reshape(-1, anchors.size(-1))
+ total_bbox_weights = torch.cat(
+ total_bbox_weights, dim=-3).reshape(-1, anchors.size(-1))
+ total_dir_targets = torch.cat(
+ total_dir_targets, dim=-2).reshape(-1)
+ total_dir_weights = torch.cat(
+ total_dir_weights, dim=-2).reshape(-1)
+ total_pos_inds = torch.cat(total_pos_inds, dim=0).reshape(-1)
+ total_neg_inds = torch.cat(total_neg_inds, dim=0).reshape(-1)
+ return (total_labels, total_label_weights, total_bbox_targets,
+ total_bbox_weights, total_dir_targets, total_dir_weights,
+ total_pos_inds, total_neg_inds)
+ elif isinstance(self.bbox_assigner, list) and isinstance(
+ anchors, list):
+ # class-aware anchors with different feature map sizes
+ assert len(self.bbox_assigner) == len(anchors), \
+ 'The number of bbox assigners and anchors should be the same.'
+ (total_labels, total_label_weights, total_bbox_targets,
+ total_bbox_weights, total_dir_targets, total_dir_weights,
+ total_pos_inds, total_neg_inds) = [], [], [], [], [], [], [], []
+ current_anchor_num = 0
+ for i, assigner in enumerate(self.bbox_assigner):
+ current_anchors = anchors[i]
+ current_anchor_num += current_anchors.size(0)
+ if self.assign_per_class:
+ gt_per_cls = (gt_labels == i)
+ anchor_targets = self.anchor_target_single_assigner(
+ assigner, current_anchors, gt_bboxes[gt_per_cls, :],
+ gt_bboxes_ignore, gt_labels[gt_per_cls], input_meta,
+ num_classes, sampling)
+ else:
+ anchor_targets = self.anchor_target_single_assigner(
+ assigner, current_anchors, gt_bboxes, gt_bboxes_ignore,
+ gt_labels, input_meta, num_classes, sampling)
+
+ (labels, label_weights, bbox_targets, bbox_weights,
+ dir_targets, dir_weights, pos_inds, neg_inds) = anchor_targets
+ total_labels.append(labels)
+ total_label_weights.append(label_weights)
+ total_bbox_targets.append(
+ bbox_targets.reshape(-1, anchors[i].size(-1)))
+ total_bbox_weights.append(
+ bbox_weights.reshape(-1, anchors[i].size(-1)))
+ total_dir_targets.append(dir_targets)
+ total_dir_weights.append(dir_weights)
+ total_pos_inds.append(pos_inds)
+ total_neg_inds.append(neg_inds)
+
+ total_labels = torch.cat(total_labels, dim=0)
+ total_label_weights = torch.cat(total_label_weights, dim=0)
+ total_bbox_targets = torch.cat(total_bbox_targets, dim=0)
+ total_bbox_weights = torch.cat(total_bbox_weights, dim=0)
+ total_dir_targets = torch.cat(total_dir_targets, dim=0)
+ total_dir_weights = torch.cat(total_dir_weights, dim=0)
+ total_pos_inds = torch.cat(total_pos_inds, dim=0)
+ total_neg_inds = torch.cat(total_neg_inds, dim=0)
+ return (total_labels, total_label_weights, total_bbox_targets,
+ total_bbox_weights, total_dir_targets, total_dir_weights,
+ total_pos_inds, total_neg_inds)
+ else:
+ return self.anchor_target_single_assigner(self.bbox_assigner,
+ anchors, gt_bboxes,
+ gt_bboxes_ignore,
+ gt_labels, input_meta,
+ num_classes, sampling)
+
+ def anchor_target_single_assigner(self,
+ bbox_assigner,
+ anchors,
+ gt_bboxes,
+ gt_bboxes_ignore,
+ gt_labels,
+ input_meta,
+ num_classes=1,
+ sampling=True):
+ """Assign anchors and encode positive anchors.
+
+ Args:
+ bbox_assigner (BaseAssigner): assign positive and negative boxes.
+ anchors (torch.Tensor): Concatenated multi-level anchor.
+ gt_bboxes (:obj:`BaseInstance3DBoxes`): Gt bboxes.
+ gt_bboxes_ignore (torch.Tensor): Ignored gt bboxes.
+ gt_labels (torch.Tensor): Gt class labels.
+ input_meta (dict): Meta info of each image.
+ num_classes (int): The number of classes.
+ sampling (bool): Whether to sample anchors.
+
+ Returns:
+ tuple[torch.Tensor]: Anchor targets.
+ """
+ anchors = anchors.reshape(-1, anchors.size(-1))
+ num_valid_anchors = anchors.shape[0]
+ bbox_targets = torch.zeros_like(anchors)
+ bbox_weights = torch.zeros_like(anchors)
+ dir_targets = anchors.new_zeros((anchors.shape[0]), dtype=torch.long)
+ dir_weights = anchors.new_zeros((anchors.shape[0]), dtype=torch.float)
+ labels = anchors.new_zeros(num_valid_anchors, dtype=torch.long)
+ label_weights = anchors.new_zeros(num_valid_anchors, dtype=torch.float)
+ if len(gt_bboxes) > 0:
+ if not isinstance(gt_bboxes, torch.Tensor):
+ gt_bboxes = gt_bboxes.tensor.to(anchors.device)
+ assign_result = bbox_assigner.assign(anchors, gt_bboxes,
+ gt_bboxes_ignore, gt_labels)
+ sampling_result = self.bbox_sampler.sample(assign_result, anchors,
+ gt_bboxes)
+ pos_inds = sampling_result.pos_inds
+ neg_inds = sampling_result.neg_inds
+ else:
+ pos_inds = torch.nonzero(
+ anchors.new_zeros((anchors.shape[0], ), dtype=torch.bool) > 0,
+ as_tuple=False).squeeze(-1).unique()
+ neg_inds = torch.nonzero(
+ anchors.new_zeros((anchors.shape[0], ), dtype=torch.bool) == 0,
+ as_tuple=False).squeeze(-1).unique()
+
+ if gt_labels is not None:
+ labels += num_classes
+ if len(pos_inds) > 0:
+ pos_bbox_targets = self.bbox_coder.encode(
+ sampling_result.pos_bboxes, sampling_result.pos_gt_bboxes)
+ pos_dir_targets = get_direction_target(
+ sampling_result.pos_bboxes,
+ pos_bbox_targets,
+ self.dir_offset,
+ self.dir_limit_offset,
+ one_hot=False)
+ bbox_targets[pos_inds, :] = pos_bbox_targets
+ bbox_weights[pos_inds, :] = 1.0
+ dir_targets[pos_inds] = pos_dir_targets
+ dir_weights[pos_inds] = 1.0
+
+ if gt_labels is None:
+ labels[pos_inds] = 1
+ else:
+ labels[pos_inds] = gt_labels[
+ sampling_result.pos_assigned_gt_inds]
+ if self.train_cfg.pos_weight <= 0:
+ label_weights[pos_inds] = 1.0
+ else:
+ label_weights[pos_inds] = self.train_cfg.pos_weight
+
+ if len(neg_inds) > 0:
+ label_weights[neg_inds] = 1.0
+ return (labels, label_weights, bbox_targets, bbox_weights, dir_targets,
+ dir_weights, pos_inds, neg_inds)
+
+
+def get_direction_target(anchors,
+ reg_targets,
+ dir_offset=0,
+ dir_limit_offset=0,
+ num_bins=2,
+ one_hot=True):
+ """Encode direction to 0 ~ num_bins-1.
+
+ Args:
+ anchors (torch.Tensor): Concatenated multi-level anchor.
+ reg_targets (torch.Tensor): Bbox regression targets.
+ dir_offset (int): Direction offset.
+ num_bins (int): Number of bins to divide 2*PI.
+ one_hot (bool): Whether to encode as one hot.
+
+ Returns:
+ torch.Tensor: Encoded direction targets.
+ """
+ rot_gt = reg_targets[..., 6] + anchors[..., 6]
+ offset_rot = limit_period(rot_gt - dir_offset, dir_limit_offset, 2 * np.pi)
+ dir_cls_targets = torch.floor(offset_rot / (2 * np.pi / num_bins)).long()
+ dir_cls_targets = torch.clamp(dir_cls_targets, min=0, max=num_bins - 1)
+ if one_hot:
+ dir_targets = torch.zeros(
+ *list(dir_cls_targets.shape),
+ num_bins,
+ dtype=anchors.dtype,
+ device=dir_cls_targets.device)
+ dir_targets.scatter_(dir_cls_targets.unsqueeze(dim=-1).long(), 1.0)
+ dir_cls_targets = dir_targets
+ return dir_cls_targets
diff --git a/mmdet3d/models/dense_heads/vote_head.py b/mmdet3d/models/dense_heads/vote_head.py
new file mode 100644
index 0000000..53b1154
--- /dev/null
+++ b/mmdet3d/models/dense_heads/vote_head.py
@@ -0,0 +1,663 @@
+# Copyright (c) OpenMMLab. All rights reserved.
+import numpy as np
+import torch
+from mmcv.ops import furthest_point_sample
+from mmcv.runner import BaseModule, force_fp32
+from torch.nn import functional as F
+
+from mmdet3d.core.post_processing import aligned_3d_nms
+from mmdet3d.models.losses import chamfer_distance
+from mmdet3d.models.model_utils import VoteModule
+from mmdet3d.ops import build_sa_module
+from mmdet.core import build_bbox_coder, multi_apply
+from ..builder import HEADS, build_loss
+from .base_conv_bbox_head import BaseConvBboxHead
+
+
+@HEADS.register_module()
+class VoteHead(BaseModule):
+ r"""Bbox head of `Votenet `_.
+
+ Args:
+ num_classes (int): The number of class.
+ bbox_coder (:obj:`BaseBBoxCoder`): Bbox coder for encoding and
+ decoding boxes.
+ train_cfg (dict): Config for training.
+ test_cfg (dict): Config for testing.
+ vote_module_cfg (dict): Config of VoteModule for point-wise votes.
+ vote_aggregation_cfg (dict): Config of vote aggregation layer.
+ pred_layer_cfg (dict): Config of classfication and regression
+ prediction layers.
+ conv_cfg (dict): Config of convolution in prediction layer.
+ norm_cfg (dict): Config of BN in prediction layer.
+ objectness_loss (dict): Config of objectness loss.
+ center_loss (dict): Config of center loss.
+ dir_class_loss (dict): Config of direction classification loss.
+ dir_res_loss (dict): Config of direction residual regression loss.
+ size_class_loss (dict): Config of size classification loss.
+ size_res_loss (dict): Config of size residual regression loss.
+ semantic_loss (dict): Config of point-wise semantic segmentation loss.
+ """
+
+ def __init__(self,
+ num_classes,
+ bbox_coder,
+ train_cfg=None,
+ test_cfg=None,
+ vote_module_cfg=None,
+ vote_aggregation_cfg=None,
+ pred_layer_cfg=None,
+ conv_cfg=dict(type='Conv1d'),
+ norm_cfg=dict(type='BN1d'),
+ objectness_loss=None,
+ center_loss=None,
+ dir_class_loss=None,
+ dir_res_loss=None,
+ size_class_loss=None,
+ size_res_loss=None,
+ semantic_loss=None,
+ iou_loss=None,
+ init_cfg=None):
+ super(VoteHead, self).__init__(init_cfg=init_cfg)
+ self.num_classes = num_classes
+ self.train_cfg = train_cfg
+ self.test_cfg = test_cfg
+ self.gt_per_seed = vote_module_cfg['gt_per_seed']
+ self.num_proposal = vote_aggregation_cfg['num_point']
+
+ self.objectness_loss = build_loss(objectness_loss)
+ self.center_loss = build_loss(center_loss)
+ self.dir_res_loss = build_loss(dir_res_loss)
+ self.dir_class_loss = build_loss(dir_class_loss)
+ self.size_res_loss = build_loss(size_res_loss)
+ if size_class_loss is not None:
+ self.size_class_loss = build_loss(size_class_loss)
+ if semantic_loss is not None:
+ self.semantic_loss = build_loss(semantic_loss)
+ if iou_loss is not None:
+ self.iou_loss = build_loss(iou_loss)
+ else:
+ self.iou_loss = None
+
+ self.bbox_coder = build_bbox_coder(bbox_coder)
+ self.num_sizes = self.bbox_coder.num_sizes
+ self.num_dir_bins = self.bbox_coder.num_dir_bins
+
+ self.vote_module = VoteModule(**vote_module_cfg)
+ self.vote_aggregation = build_sa_module(vote_aggregation_cfg)
+ self.fp16_enabled = False
+
+ # Bbox classification and regression
+ self.conv_pred = BaseConvBboxHead(
+ **pred_layer_cfg,
+ num_cls_out_channels=self._get_cls_out_channels(),
+ num_reg_out_channels=self._get_reg_out_channels())
+
+ def _get_cls_out_channels(self):
+ """Return the channel number of classification outputs."""
+ # Class numbers (k) + objectness (2)
+ return self.num_classes + 2
+
+ def _get_reg_out_channels(self):
+ """Return the channel number of regression outputs."""
+ # Objectness scores (2), center residual (3),
+ # heading class+residual (num_dir_bins*2),
+ # size class+residual(num_sizes*4)
+ return 3 + self.num_dir_bins * 2 + self.num_sizes * 4
+
+ def _extract_input(self, feat_dict):
+ """Extract inputs from features dictionary.
+
+ Args:
+ feat_dict (dict): Feature dict from backbone.
+
+ Returns:
+ torch.Tensor: Coordinates of input points.
+ torch.Tensor: Features of input points.
+ torch.Tensor: Indices of input points.
+ """
+
+ # for imvotenet
+ if 'seed_points' in feat_dict and \
+ 'seed_features' in feat_dict and \
+ 'seed_indices' in feat_dict:
+ seed_points = feat_dict['seed_points']
+ seed_features = feat_dict['seed_features']
+ seed_indices = feat_dict['seed_indices']
+ # for votenet
+ else:
+ seed_points = feat_dict['fp_xyz'][-1]
+ seed_features = feat_dict['fp_features'][-1]
+ seed_indices = feat_dict['fp_indices'][-1]
+
+ return seed_points, seed_features, seed_indices
+
+ def forward(self, feat_dict, sample_mod):
+ """Forward pass.
+
+ Note:
+ The forward of VoteHead is divided into 4 steps:
+
+ 1. Generate vote_points from seed_points.
+ 2. Aggregate vote_points.
+ 3. Predict bbox and score.
+ 4. Decode predictions.
+
+ Args:
+ feat_dict (dict): Feature dict from backbone.
+ sample_mod (str): Sample mode for vote aggregation layer.
+ valid modes are "vote", "seed", "random" and "spec".
+
+ Returns:
+ dict: Predictions of vote head.
+ """
+ assert sample_mod in ['vote', 'seed', 'random', 'spec']
+
+ seed_points, seed_features, seed_indices = self._extract_input(
+ feat_dict)
+
+ # 1. generate vote_points from seed_points
+ vote_points, vote_features, vote_offset = self.vote_module(
+ seed_points, seed_features)
+ results = dict(
+ seed_points=seed_points,
+ seed_indices=seed_indices,
+ vote_points=vote_points,
+ vote_features=vote_features,
+ vote_offset=vote_offset)
+
+ # 2. aggregate vote_points
+ if sample_mod == 'vote':
+ # use fps in vote_aggregation
+ aggregation_inputs = dict(
+ points_xyz=vote_points, features=vote_features)
+ elif sample_mod == 'seed':
+ # FPS on seed and choose the votes corresponding to the seeds
+ sample_indices = furthest_point_sample(seed_points,
+ self.num_proposal)
+ aggregation_inputs = dict(
+ points_xyz=vote_points,
+ features=vote_features,
+ indices=sample_indices)
+ elif sample_mod == 'random':
+ # Random sampling from the votes
+ batch_size, num_seed = seed_points.shape[:2]
+ sample_indices = seed_points.new_tensor(
+ torch.randint(0, num_seed, (batch_size, self.num_proposal)),
+ dtype=torch.int32)
+ aggregation_inputs = dict(
+ points_xyz=vote_points,
+ features=vote_features,
+ indices=sample_indices)
+ elif sample_mod == 'spec':
+ # Specify the new center in vote_aggregation
+ aggregation_inputs = dict(
+ points_xyz=seed_points,
+ features=seed_features,
+ target_xyz=vote_points)
+ else:
+ raise NotImplementedError(
+ f'Sample mode {sample_mod} is not supported!')
+
+ vote_aggregation_ret = self.vote_aggregation(**aggregation_inputs)
+ aggregated_points, features, aggregated_indices = vote_aggregation_ret
+
+ results['aggregated_points'] = aggregated_points
+ results['aggregated_features'] = features
+ results['aggregated_indices'] = aggregated_indices
+
+ # 3. predict bbox and score
+ cls_predictions, reg_predictions = self.conv_pred(features)
+
+ # 4. decode predictions
+ decode_res = self.bbox_coder.split_pred(cls_predictions,
+ reg_predictions,
+ aggregated_points)
+
+ results.update(decode_res)
+
+ return results
+
+ @force_fp32(apply_to=('bbox_preds', ))
+ def loss(self,
+ bbox_preds,
+ points,
+ gt_bboxes_3d,
+ gt_labels_3d,
+ pts_semantic_mask=None,
+ pts_instance_mask=None,
+ img_metas=None,
+ gt_bboxes_ignore=None,
+ ret_target=False):
+ """Compute loss.
+
+ Args:
+ bbox_preds (dict): Predictions from forward of vote head.
+ points (list[torch.Tensor]): Input points.
+ gt_bboxes_3d (list[:obj:`BaseInstance3DBoxes`]): Ground truth
+ bboxes of each sample.
+ gt_labels_3d (list[torch.Tensor]): Labels of each sample.
+ pts_semantic_mask (list[torch.Tensor]): Point-wise
+ semantic mask.
+ pts_instance_mask (list[torch.Tensor]): Point-wise
+ instance mask.
+ img_metas (list[dict]): Contain pcd and img's meta info.
+ gt_bboxes_ignore (list[torch.Tensor]): Specify
+ which bounding.
+ ret_target (Bool): Return targets or not.
+
+ Returns:
+ dict: Losses of Votenet.
+ """
+ targets = self.get_targets(points, gt_bboxes_3d, gt_labels_3d,
+ pts_semantic_mask, pts_instance_mask,
+ bbox_preds)
+ (vote_targets, vote_target_masks, size_class_targets, size_res_targets,
+ dir_class_targets, dir_res_targets, center_targets,
+ assigned_center_targets, mask_targets, valid_gt_masks,
+ objectness_targets, objectness_weights, box_loss_weights,
+ valid_gt_weights) = targets
+
+ # calculate vote loss
+ vote_loss = self.vote_module.get_loss(bbox_preds['seed_points'],
+ bbox_preds['vote_points'],
+ bbox_preds['seed_indices'],
+ vote_target_masks, vote_targets)
+
+ # calculate objectness loss
+ objectness_loss = self.objectness_loss(
+ bbox_preds['obj_scores'].transpose(2, 1),
+ objectness_targets,
+ weight=objectness_weights)
+
+ # calculate center loss
+ source2target_loss, target2source_loss = self.center_loss(
+ bbox_preds['center'],
+ center_targets,
+ src_weight=box_loss_weights,
+ dst_weight=valid_gt_weights)
+ center_loss = source2target_loss + target2source_loss
+
+ # calculate direction class loss
+ dir_class_loss = self.dir_class_loss(
+ bbox_preds['dir_class'].transpose(2, 1),
+ dir_class_targets,
+ weight=box_loss_weights)
+
+ # calculate direction residual loss
+ batch_size, proposal_num = size_class_targets.shape[:2]
+ heading_label_one_hot = vote_targets.new_zeros(
+ (batch_size, proposal_num, self.num_dir_bins))
+ heading_label_one_hot.scatter_(2, dir_class_targets.unsqueeze(-1), 1)
+ dir_res_norm = torch.sum(
+ bbox_preds['dir_res_norm'] * heading_label_one_hot, -1)
+ dir_res_loss = self.dir_res_loss(
+ dir_res_norm, dir_res_targets, weight=box_loss_weights)
+
+ # calculate size class loss
+ size_class_loss = self.size_class_loss(
+ bbox_preds['size_class'].transpose(2, 1),
+ size_class_targets,
+ weight=box_loss_weights)
+
+ # calculate size residual loss
+ one_hot_size_targets = vote_targets.new_zeros(
+ (batch_size, proposal_num, self.num_sizes))
+ one_hot_size_targets.scatter_(2, size_class_targets.unsqueeze(-1), 1)
+ one_hot_size_targets_expand = one_hot_size_targets.unsqueeze(
+ -1).repeat(1, 1, 1, 3).contiguous()
+ size_residual_norm = torch.sum(
+ bbox_preds['size_res_norm'] * one_hot_size_targets_expand, 2)
+ box_loss_weights_expand = box_loss_weights.unsqueeze(-1).repeat(
+ 1, 1, 3)
+ size_res_loss = self.size_res_loss(
+ size_residual_norm,
+ size_res_targets,
+ weight=box_loss_weights_expand)
+
+ # calculate semantic loss
+ semantic_loss = self.semantic_loss(
+ bbox_preds['sem_scores'].transpose(2, 1),
+ mask_targets,
+ weight=box_loss_weights)
+
+ losses = dict(
+ vote_loss=vote_loss,
+ objectness_loss=objectness_loss,
+ semantic_loss=semantic_loss,
+ center_loss=center_loss,
+ dir_class_loss=dir_class_loss,
+ dir_res_loss=dir_res_loss,
+ size_class_loss=size_class_loss,
+ size_res_loss=size_res_loss)
+
+ if self.iou_loss:
+ corners_pred = self.bbox_coder.decode_corners(
+ bbox_preds['center'], size_residual_norm,
+ one_hot_size_targets_expand)
+ corners_target = self.bbox_coder.decode_corners(
+ assigned_center_targets, size_res_targets,
+ one_hot_size_targets_expand)
+ iou_loss = self.iou_loss(
+ corners_pred, corners_target, weight=box_loss_weights)
+ losses['iou_loss'] = iou_loss
+
+ if ret_target:
+ losses['targets'] = targets
+
+ return losses
+
+ def get_targets(self,
+ points,
+ gt_bboxes_3d,
+ gt_labels_3d,
+ pts_semantic_mask=None,
+ pts_instance_mask=None,
+ bbox_preds=None):
+ """Generate targets of vote head.
+
+ Args:
+ points (list[torch.Tensor]): Points of each batch.
+ gt_bboxes_3d (list[:obj:`BaseInstance3DBoxes`]): Ground truth
+ bboxes of each batch.
+ gt_labels_3d (list[torch.Tensor]): Labels of each batch.
+ pts_semantic_mask (list[torch.Tensor]): Point-wise semantic
+ label of each batch.
+ pts_instance_mask (list[torch.Tensor]): Point-wise instance
+ label of each batch.
+ bbox_preds (torch.Tensor): Bounding box predictions of vote head.
+
+ Returns:
+ tuple[torch.Tensor]: Targets of vote head.
+ """
+ # find empty example
+ valid_gt_masks = list()
+ gt_num = list()
+ for index in range(len(gt_labels_3d)):
+ if len(gt_labels_3d[index]) == 0:
+ fake_box = gt_bboxes_3d[index].tensor.new_zeros(
+ 1, gt_bboxes_3d[index].tensor.shape[-1])
+ gt_bboxes_3d[index] = gt_bboxes_3d[index].new_box(fake_box)
+ gt_labels_3d[index] = gt_labels_3d[index].new_zeros(1)
+ valid_gt_masks.append(gt_labels_3d[index].new_zeros(1))
+ gt_num.append(1)
+ else:
+ valid_gt_masks.append(gt_labels_3d[index].new_ones(
+ gt_labels_3d[index].shape))
+ gt_num.append(gt_labels_3d[index].shape[0])
+ max_gt_num = max(gt_num)
+
+ if pts_semantic_mask is None:
+ pts_semantic_mask = [None for i in range(len(gt_labels_3d))]
+ pts_instance_mask = [None for i in range(len(gt_labels_3d))]
+
+ aggregated_points = [
+ bbox_preds['aggregated_points'][i]
+ for i in range(len(gt_labels_3d))
+ ]
+
+ (vote_targets, vote_target_masks, size_class_targets, size_res_targets,
+ dir_class_targets, dir_res_targets, center_targets,
+ assigned_center_targets, mask_targets, objectness_targets,
+ objectness_masks) = multi_apply(self.get_targets_single, points,
+ gt_bboxes_3d, gt_labels_3d,
+ pts_semantic_mask, pts_instance_mask,
+ aggregated_points)
+
+ # pad targets as original code of votenet.
+ for index in range(len(gt_labels_3d)):
+ pad_num = max_gt_num - gt_labels_3d[index].shape[0]
+ center_targets[index] = F.pad(center_targets[index],
+ (0, 0, 0, pad_num))
+ valid_gt_masks[index] = F.pad(valid_gt_masks[index], (0, pad_num))
+
+ vote_targets = torch.stack(vote_targets)
+ vote_target_masks = torch.stack(vote_target_masks)
+ center_targets = torch.stack(center_targets)
+ valid_gt_masks = torch.stack(valid_gt_masks)
+
+ assigned_center_targets = torch.stack(assigned_center_targets)
+ objectness_targets = torch.stack(objectness_targets)
+ objectness_weights = torch.stack(objectness_masks)
+ objectness_weights /= (torch.sum(objectness_weights) + 1e-6)
+ box_loss_weights = objectness_targets.float() / (
+ torch.sum(objectness_targets).float() + 1e-6)
+ valid_gt_weights = valid_gt_masks.float() / (
+ torch.sum(valid_gt_masks.float()) + 1e-6)
+ dir_class_targets = torch.stack(dir_class_targets)
+ dir_res_targets = torch.stack(dir_res_targets)
+ size_class_targets = torch.stack(size_class_targets)
+ size_res_targets = torch.stack(size_res_targets)
+ mask_targets = torch.stack(mask_targets)
+
+ return (vote_targets, vote_target_masks, size_class_targets,
+ size_res_targets, dir_class_targets, dir_res_targets,
+ center_targets, assigned_center_targets, mask_targets,
+ valid_gt_masks, objectness_targets, objectness_weights,
+ box_loss_weights, valid_gt_weights)
+
+ def get_targets_single(self,
+ points,
+ gt_bboxes_3d,
+ gt_labels_3d,
+ pts_semantic_mask=None,
+ pts_instance_mask=None,
+ aggregated_points=None):
+ """Generate targets of vote head for single batch.
+
+ Args:
+ points (torch.Tensor): Points of each batch.
+ gt_bboxes_3d (:obj:`BaseInstance3DBoxes`): Ground truth
+ boxes of each batch.
+ gt_labels_3d (torch.Tensor): Labels of each batch.
+ pts_semantic_mask (torch.Tensor): Point-wise semantic
+ label of each batch.
+ pts_instance_mask (torch.Tensor): Point-wise instance
+ label of each batch.
+ aggregated_points (torch.Tensor): Aggregated points from
+ vote aggregation layer.
+
+ Returns:
+ tuple[torch.Tensor]: Targets of vote head.
+ """
+ assert self.bbox_coder.with_rot or pts_semantic_mask is not None
+
+ gt_bboxes_3d = gt_bboxes_3d.to(points.device)
+
+ # generate votes target
+ num_points = points.shape[0]
+ if self.bbox_coder.with_rot:
+ vote_targets = points.new_zeros([num_points, 3 * self.gt_per_seed])
+ vote_target_masks = points.new_zeros([num_points],
+ dtype=torch.long)
+ vote_target_idx = points.new_zeros([num_points], dtype=torch.long)
+ box_indices_all = gt_bboxes_3d.points_in_boxes_all(points)
+ for i in range(gt_labels_3d.shape[0]):
+ box_indices = box_indices_all[:, i]
+ indices = torch.nonzero(
+ box_indices, as_tuple=False).squeeze(-1)
+ selected_points = points[indices]
+ vote_target_masks[indices] = 1
+ vote_targets_tmp = vote_targets[indices]
+ votes = gt_bboxes_3d.gravity_center[i].unsqueeze(
+ 0) - selected_points[:, :3]
+
+ for j in range(self.gt_per_seed):
+ column_indices = torch.nonzero(
+ vote_target_idx[indices] == j,
+ as_tuple=False).squeeze(-1)
+ vote_targets_tmp[column_indices,
+ int(j * 3):int(j * 3 +
+ 3)] = votes[column_indices]
+ if j == 0:
+ vote_targets_tmp[column_indices] = votes[
+ column_indices].repeat(1, self.gt_per_seed)
+
+ vote_targets[indices] = vote_targets_tmp
+ vote_target_idx[indices] = torch.clamp(
+ vote_target_idx[indices] + 1, max=2)
+ elif pts_semantic_mask is not None:
+ vote_targets = points.new_zeros([num_points, 3])
+ vote_target_masks = points.new_zeros([num_points],
+ dtype=torch.long)
+
+ for i in torch.unique(pts_instance_mask):
+ indices = torch.nonzero(
+ pts_instance_mask == i, as_tuple=False).squeeze(-1)
+ if pts_semantic_mask[indices[0]] < self.num_classes:
+ selected_points = points[indices, :3]
+ center = 0.5 * (
+ selected_points.min(0)[0] + selected_points.max(0)[0])
+ vote_targets[indices, :] = center - selected_points
+ vote_target_masks[indices] = 1
+ vote_targets = vote_targets.repeat((1, self.gt_per_seed))
+ else:
+ raise NotImplementedError
+
+ (center_targets, size_class_targets, size_res_targets,
+ dir_class_targets,
+ dir_res_targets) = self.bbox_coder.encode(gt_bboxes_3d, gt_labels_3d)
+
+ proposal_num = aggregated_points.shape[0]
+ distance1, _, assignment, _ = chamfer_distance(
+ aggregated_points.unsqueeze(0),
+ center_targets.unsqueeze(0),
+ reduction='none')
+ assignment = assignment.squeeze(0)
+ euclidean_distance1 = torch.sqrt(distance1.squeeze(0) + 1e-6)
+
+ objectness_targets = points.new_zeros((proposal_num), dtype=torch.long)
+ objectness_targets[
+ euclidean_distance1 < self.train_cfg['pos_distance_thr']] = 1
+
+ objectness_masks = points.new_zeros((proposal_num))
+ objectness_masks[
+ euclidean_distance1 < self.train_cfg['pos_distance_thr']] = 1.0
+ objectness_masks[
+ euclidean_distance1 > self.train_cfg['neg_distance_thr']] = 1.0
+
+ dir_class_targets = dir_class_targets[assignment]
+ dir_res_targets = dir_res_targets[assignment]
+ dir_res_targets /= (np.pi / self.num_dir_bins)
+ size_class_targets = size_class_targets[assignment]
+ size_res_targets = size_res_targets[assignment]
+
+ one_hot_size_targets = gt_bboxes_3d.tensor.new_zeros(
+ (proposal_num, self.num_sizes))
+ one_hot_size_targets.scatter_(1, size_class_targets.unsqueeze(-1), 1)
+ one_hot_size_targets = one_hot_size_targets.unsqueeze(-1).repeat(
+ 1, 1, 3)
+ mean_sizes = size_res_targets.new_tensor(
+ self.bbox_coder.mean_sizes).unsqueeze(0)
+ pos_mean_sizes = torch.sum(one_hot_size_targets * mean_sizes, 1)
+ size_res_targets /= pos_mean_sizes
+
+ mask_targets = gt_labels_3d[assignment]
+ assigned_center_targets = center_targets[assignment]
+
+ return (vote_targets, vote_target_masks, size_class_targets,
+ size_res_targets, dir_class_targets,
+ dir_res_targets, center_targets, assigned_center_targets,
+ mask_targets.long(), objectness_targets, objectness_masks)
+
+ def get_bboxes(self,
+ points,
+ bbox_preds,
+ input_metas,
+ rescale=False,
+ use_nms=True):
+ """Generate bboxes from vote head predictions.
+
+ Args:
+ points (torch.Tensor): Input points.
+ bbox_preds (dict): Predictions from vote head.
+ input_metas (list[dict]): Point cloud and image's meta info.
+ rescale (bool): Whether to rescale bboxes.
+ use_nms (bool): Whether to apply NMS, skip nms postprocessing
+ while using vote head in rpn stage.
+
+ Returns:
+ list[tuple[torch.Tensor]]: Bounding boxes, scores and labels.
+ """
+ # decode boxes
+ obj_scores = F.softmax(bbox_preds['obj_scores'], dim=-1)[..., -1]
+ sem_scores = F.softmax(bbox_preds['sem_scores'], dim=-1)
+ bbox3d = self.bbox_coder.decode(bbox_preds)
+
+ if use_nms:
+ batch_size = bbox3d.shape[0]
+ results = list()
+ for b in range(batch_size):
+ bbox_selected, score_selected, labels = \
+ self.multiclass_nms_single(obj_scores[b], sem_scores[b],
+ bbox3d[b], points[b, ..., :3],
+ input_metas[b])
+ bbox = input_metas[b]['box_type_3d'](
+ bbox_selected,
+ box_dim=bbox_selected.shape[-1],
+ with_yaw=self.bbox_coder.with_rot)
+ results.append((bbox, score_selected, labels))
+
+ return results
+ else:
+ return bbox3d
+
+ def multiclass_nms_single(self, obj_scores, sem_scores, bbox, points,
+ input_meta):
+ """Multi-class nms in single batch.
+
+ Args:
+ obj_scores (torch.Tensor): Objectness score of bounding boxes.
+ sem_scores (torch.Tensor): semantic class score of bounding boxes.
+ bbox (torch.Tensor): Predicted bounding boxes.
+ points (torch.Tensor): Input points.
+ input_meta (dict): Point cloud and image's meta info.
+
+ Returns:
+ tuple[torch.Tensor]: Bounding boxes, scores and labels.
+ """
+ bbox = input_meta['box_type_3d'](
+ bbox,
+ box_dim=bbox.shape[-1],
+ with_yaw=self.bbox_coder.with_rot,
+ origin=(0.5, 0.5, 0.5))
+ box_indices = bbox.points_in_boxes_all(points)
+
+ corner3d = bbox.corners
+ minmax_box3d = corner3d.new(torch.Size((corner3d.shape[0], 6)))
+ minmax_box3d[:, :3] = torch.min(corner3d, dim=1)[0]
+ minmax_box3d[:, 3:] = torch.max(corner3d, dim=1)[0]
+
+ nonempty_box_mask = box_indices.T.sum(1) > 5
+
+ bbox_classes = torch.argmax(sem_scores, -1)
+ nms_selected = aligned_3d_nms(minmax_box3d[nonempty_box_mask],
+ obj_scores[nonempty_box_mask],
+ bbox_classes[nonempty_box_mask],
+ self.test_cfg.nms_thr)
+
+ # filter empty boxes and boxes with low score
+ scores_mask = (obj_scores > self.test_cfg.score_thr)
+ nonempty_box_inds = torch.nonzero(
+ nonempty_box_mask, as_tuple=False).flatten()
+ nonempty_mask = torch.zeros_like(bbox_classes).scatter(
+ 0, nonempty_box_inds[nms_selected], 1)
+ selected = (nonempty_mask.bool() & scores_mask.bool())
+
+ if self.test_cfg.per_class_proposal:
+ bbox_selected, score_selected, labels = [], [], []
+ for k in range(sem_scores.shape[-1]):
+ bbox_selected.append(bbox[selected].tensor)
+ score_selected.append(obj_scores[selected] *
+ sem_scores[selected][:, k])
+ labels.append(
+ torch.zeros_like(bbox_classes[selected]).fill_(k))
+ bbox_selected = torch.cat(bbox_selected, 0)
+ score_selected = torch.cat(score_selected, 0)
+ labels = torch.cat(labels, 0)
+ else:
+ bbox_selected = bbox[selected].tensor
+ score_selected = obj_scores[selected]
+ labels = bbox_classes[selected]
+
+ return bbox_selected, score_selected, labels
diff --git a/mmdet3d/models/detectors/__init__.py b/mmdet3d/models/detectors/__init__.py
new file mode 100644
index 0000000..aca20fa
--- /dev/null
+++ b/mmdet3d/models/detectors/__init__.py
@@ -0,0 +1,31 @@
+# Copyright (c) OpenMMLab. All rights reserved.
+from .base import Base3DDetector
+from .centerpoint import CenterPoint
+from .dynamic_voxelnet import DynamicVoxelNet
+from .fcos_mono3d import FCOSMono3D
+from .groupfree3dnet import GroupFree3DNet
+from .h3dnet import H3DNet
+from .imvotenet import ImVoteNet
+from .imvoxelnet import ImVoxelNet
+from .mink_single_stage import MinkSingleStage3DDetector
+from .mvx_faster_rcnn import DynamicMVXFasterRCNN, MVXFasterRCNN
+from .mvx_two_stage import MVXTwoStageDetector
+from .ngfc import Ngfc3DDetector
+from .ngfc_v2 import NgfcV23DDetector
+from .td3d_instance_segmentor import TD3DInstanceSegmentor
+from .parta2 import PartA2
+from .point_rcnn import PointRCNN
+from .sassd import SASSD
+from .single_stage_mono3d import SingleStageMono3DDetector
+from .smoke_mono3d import SMOKEMono3D
+from .ssd3dnet import SSD3DNet
+from .votenet import VoteNet
+from .voxelnet import VoxelNet
+
+__all__ = [
+ 'Base3DDetector', 'VoxelNet', 'DynamicVoxelNet', 'MVXTwoStageDetector',
+ 'DynamicMVXFasterRCNN', 'MVXFasterRCNN', 'PartA2', 'VoteNet', 'H3DNet',
+ 'CenterPoint', 'SSD3DNet', 'ImVoteNet', 'SingleStageMono3DDetector',
+ 'FCOSMono3D', 'ImVoxelNet', 'GroupFree3DNet', 'PointRCNN', 'SMOKEMono3D',
+ 'MinkSingleStage3DDetector', 'SASSD', 'Ngfc3DDetector'
+]
diff --git a/mmdet3d/models/detectors/base.py b/mmdet3d/models/detectors/base.py
new file mode 100644
index 0000000..4985c1d
--- /dev/null
+++ b/mmdet3d/models/detectors/base.py
@@ -0,0 +1,127 @@
+# Copyright (c) OpenMMLab. All rights reserved.
+from os import path as osp
+
+import mmcv
+import torch
+from mmcv.parallel import DataContainer as DC
+from mmcv.runner import auto_fp16
+
+from mmdet3d.core import Box3DMode, Coord3DMode, show_result
+from mmdet.models.detectors import BaseDetector
+
+
+class Base3DDetector(BaseDetector):
+ """Base class for detectors."""
+
+ def forward_test(self, points, img_metas, img=None, **kwargs):
+ """
+ Args:
+ points (list[torch.Tensor]): the outer list indicates test-time
+ augmentations and inner torch.Tensor should have a shape NxC,
+ which contains all points in the batch.
+ img_metas (list[list[dict]]): the outer list indicates test-time
+ augs (multiscale, flip, etc.) and the inner list indicates
+ images in a batch
+ img (list[torch.Tensor], optional): the outer
+ list indicates test-time augmentations and inner
+ torch.Tensor should have a shape NxCxHxW, which contains
+ all images in the batch. Defaults to None.
+ """
+ for var, name in [(points, 'points'), (img_metas, 'img_metas')]:
+ if not isinstance(var, list):
+ raise TypeError('{} must be a list, but got {}'.format(
+ name, type(var)))
+
+ num_augs = len(points)
+ if num_augs != len(img_metas):
+ raise ValueError(
+ 'num of augmentations ({}) != num of image meta ({})'.format(
+ len(points), len(img_metas)))
+
+ if num_augs == 1:
+ img = [img] if img is None else img
+ return self.simple_test(points[0], img_metas[0], img[0], **kwargs)
+ else:
+ return self.aug_test(points, img_metas, img, **kwargs)
+
+ @auto_fp16(apply_to=('img', 'points'))
+ def forward(self, return_loss=True, **kwargs):
+ """Calls either forward_train or forward_test depending on whether
+ return_loss=True.
+
+ Note this setting will change the expected inputs. When
+ `return_loss=True`, img and img_metas are single-nested (i.e.
+ torch.Tensor and list[dict]), and when `resturn_loss=False`, img and
+ img_metas should be double nested (i.e. list[torch.Tensor],
+ list[list[dict]]), with the outer list indicating test time
+ augmentations.
+ """
+ if return_loss:
+ return self.forward_train(**kwargs)
+ else:
+ return self.forward_test(**kwargs)
+
+ def show_results(self, data, result, out_dir, show=False, score_thr=None):
+ """Results visualization.
+
+ Args:
+ data (list[dict]): Input points and the information of the sample.
+ result (list[dict]): Prediction results.
+ out_dir (str): Output directory of visualization result.
+ show (bool, optional): Determines whether you are
+ going to show result by open3d.
+ Defaults to False.
+ score_thr (float, optional): Score threshold of bounding boxes.
+ Default to None.
+ """
+ for batch_id in range(len(result)):
+ if isinstance(data['points'][0], DC):
+ points = data['points'][0]._data[0][batch_id].numpy()
+ elif mmcv.is_list_of(data['points'][0], torch.Tensor):
+ points = data['points'][0][batch_id]
+ else:
+ ValueError(f"Unsupported data type {type(data['points'][0])} "
+ f'for visualization!')
+ if isinstance(data['img_metas'][0], DC):
+ pts_filename = data['img_metas'][0]._data[0][batch_id][
+ 'pts_filename']
+ box_mode_3d = data['img_metas'][0]._data[0][batch_id][
+ 'box_mode_3d']
+ elif mmcv.is_list_of(data['img_metas'][0], dict):
+ pts_filename = data['img_metas'][0][batch_id]['pts_filename']
+ box_mode_3d = data['img_metas'][0][batch_id]['box_mode_3d']
+ else:
+ ValueError(
+ f"Unsupported data type {type(data['img_metas'][0])} "
+ f'for visualization!')
+ file_name = osp.split(pts_filename)[-1].split('.')[0]
+
+ assert out_dir is not None, 'Expect out_dir, got none.'
+
+ pred_bboxes = result[batch_id]['boxes_3d']
+ pred_labels = result[batch_id]['labels_3d']
+
+ if score_thr is not None:
+ mask = result[batch_id]['scores_3d'] > score_thr
+ pred_bboxes = pred_bboxes[mask]
+ pred_labels = pred_labels[mask]
+
+ # for now we convert points and bbox into depth mode
+ if (box_mode_3d == Box3DMode.CAM) or (box_mode_3d
+ == Box3DMode.LIDAR):
+ points = Coord3DMode.convert_point(points, Coord3DMode.LIDAR,
+ Coord3DMode.DEPTH)
+ pred_bboxes = Box3DMode.convert(pred_bboxes, box_mode_3d,
+ Box3DMode.DEPTH)
+ elif box_mode_3d != Box3DMode.DEPTH:
+ ValueError(
+ f'Unsupported box_mode_3d {box_mode_3d} for conversion!')
+ pred_bboxes = pred_bboxes.tensor.cpu().numpy()
+ show_result(
+ points,
+ None,
+ pred_bboxes,
+ out_dir,
+ file_name,
+ show=show,
+ pred_labels=pred_labels)
diff --git a/mmdet3d/models/detectors/centerpoint.py b/mmdet3d/models/detectors/centerpoint.py
new file mode 100644
index 0000000..290af5b
--- /dev/null
+++ b/mmdet3d/models/detectors/centerpoint.py
@@ -0,0 +1,196 @@
+# Copyright (c) OpenMMLab. All rights reserved.
+import torch
+
+from mmdet3d.core import bbox3d2result, merge_aug_bboxes_3d
+from ..builder import DETECTORS
+from .mvx_two_stage import MVXTwoStageDetector
+
+
+@DETECTORS.register_module()
+class CenterPoint(MVXTwoStageDetector):
+ """Base class of Multi-modality VoxelNet."""
+
+ def __init__(self,
+ pts_voxel_layer=None,
+ pts_voxel_encoder=None,
+ pts_middle_encoder=None,
+ pts_fusion_layer=None,
+ img_backbone=None,
+ pts_backbone=None,
+ img_neck=None,
+ pts_neck=None,
+ pts_bbox_head=None,
+ img_roi_head=None,
+ img_rpn_head=None,
+ train_cfg=None,
+ test_cfg=None,
+ pretrained=None,
+ init_cfg=None):
+ super(CenterPoint,
+ self).__init__(pts_voxel_layer, pts_voxel_encoder,
+ pts_middle_encoder, pts_fusion_layer,
+ img_backbone, pts_backbone, img_neck, pts_neck,
+ pts_bbox_head, img_roi_head, img_rpn_head,
+ train_cfg, test_cfg, pretrained, init_cfg)
+
+ def extract_pts_feat(self, pts, img_feats, img_metas):
+ """Extract features of points."""
+ if not self.with_pts_bbox:
+ return None
+ voxels, num_points, coors = self.voxelize(pts)
+
+ voxel_features = self.pts_voxel_encoder(voxels, num_points, coors)
+ batch_size = coors[-1, 0] + 1
+ x = self.pts_middle_encoder(voxel_features, coors, batch_size)
+ x = self.pts_backbone(x)
+ if self.with_pts_neck:
+ x = self.pts_neck(x)
+ return x
+
+ def forward_pts_train(self,
+ pts_feats,
+ gt_bboxes_3d,
+ gt_labels_3d,
+ img_metas,
+ gt_bboxes_ignore=None):
+ """Forward function for point cloud branch.
+
+ Args:
+ pts_feats (list[torch.Tensor]): Features of point cloud branch
+ gt_bboxes_3d (list[:obj:`BaseInstance3DBoxes`]): Ground truth
+ boxes for each sample.
+ gt_labels_3d (list[torch.Tensor]): Ground truth labels for
+ boxes of each sampole
+ img_metas (list[dict]): Meta information of samples.
+ gt_bboxes_ignore (list[torch.Tensor], optional): Ground truth
+ boxes to be ignored. Defaults to None.
+
+ Returns:
+ dict: Losses of each branch.
+ """
+ outs = self.pts_bbox_head(pts_feats)
+ loss_inputs = [gt_bboxes_3d, gt_labels_3d, outs]
+ losses = self.pts_bbox_head.loss(*loss_inputs)
+ return losses
+
+ def simple_test_pts(self, x, img_metas, rescale=False):
+ """Test function of point cloud branch."""
+ outs = self.pts_bbox_head(x)
+ bbox_list = self.pts_bbox_head.get_bboxes(
+ outs, img_metas, rescale=rescale)
+ bbox_results = [
+ bbox3d2result(bboxes, scores, labels)
+ for bboxes, scores, labels in bbox_list
+ ]
+ return bbox_results
+
+ def aug_test_pts(self, feats, img_metas, rescale=False):
+ """Test function of point cloud branch with augmentaiton.
+
+ The function implementation process is as follows:
+
+ - step 1: map features back for double-flip augmentation.
+ - step 2: merge all features and generate boxes.
+ - step 3: map boxes back for scale augmentation.
+ - step 4: merge results.
+
+ Args:
+ feats (list[torch.Tensor]): Feature of point cloud.
+ img_metas (list[dict]): Meta information of samples.
+ rescale (bool, optional): Whether to rescale bboxes.
+ Default: False.
+
+ Returns:
+ dict: Returned bboxes consists of the following keys:
+
+ - boxes_3d (:obj:`LiDARInstance3DBoxes`): Predicted bboxes.
+ - scores_3d (torch.Tensor): Scores of predicted boxes.
+ - labels_3d (torch.Tensor): Labels of predicted boxes.
+ """
+ # only support aug_test for one sample
+ outs_list = []
+ for x, img_meta in zip(feats, img_metas):
+ outs = self.pts_bbox_head(x)
+ # merge augmented outputs before decoding bboxes
+ for task_id, out in enumerate(outs):
+ for key in out[0].keys():
+ if img_meta[0]['pcd_horizontal_flip']:
+ outs[task_id][0][key] = torch.flip(
+ outs[task_id][0][key], dims=[2])
+ if key == 'reg':
+ outs[task_id][0][key][:, 1, ...] = 1 - outs[
+ task_id][0][key][:, 1, ...]
+ elif key == 'rot':
+ outs[task_id][0][
+ key][:, 0,
+ ...] = -outs[task_id][0][key][:, 0, ...]
+ elif key == 'vel':
+ outs[task_id][0][
+ key][:, 1,
+ ...] = -outs[task_id][0][key][:, 1, ...]
+ if img_meta[0]['pcd_vertical_flip']:
+ outs[task_id][0][key] = torch.flip(
+ outs[task_id][0][key], dims=[3])
+ if key == 'reg':
+ outs[task_id][0][key][:, 0, ...] = 1 - outs[
+ task_id][0][key][:, 0, ...]
+ elif key == 'rot':
+ outs[task_id][0][
+ key][:, 1,
+ ...] = -outs[task_id][0][key][:, 1, ...]
+ elif key == 'vel':
+ outs[task_id][0][
+ key][:, 0,
+ ...] = -outs[task_id][0][key][:, 0, ...]
+
+ outs_list.append(outs)
+
+ preds_dicts = dict()
+ scale_img_metas = []
+
+ # concat outputs sharing the same pcd_scale_factor
+ for i, (img_meta, outs) in enumerate(zip(img_metas, outs_list)):
+ pcd_scale_factor = img_meta[0]['pcd_scale_factor']
+ if pcd_scale_factor not in preds_dicts.keys():
+ preds_dicts[pcd_scale_factor] = outs
+ scale_img_metas.append(img_meta)
+ else:
+ for task_id, out in enumerate(outs):
+ for key in out[0].keys():
+ preds_dicts[pcd_scale_factor][task_id][0][key] += out[
+ 0][key]
+
+ aug_bboxes = []
+
+ for pcd_scale_factor, preds_dict in preds_dicts.items():
+ for task_id, pred_dict in enumerate(preds_dict):
+ # merge outputs with different flips before decoding bboxes
+ for key in pred_dict[0].keys():
+ preds_dict[task_id][0][key] /= len(outs_list) / len(
+ preds_dicts.keys())
+ bbox_list = self.pts_bbox_head.get_bboxes(
+ preds_dict, img_metas[0], rescale=rescale)
+ bbox_list = [
+ dict(boxes_3d=bboxes, scores_3d=scores, labels_3d=labels)
+ for bboxes, scores, labels in bbox_list
+ ]
+ aug_bboxes.append(bbox_list[0])
+
+ if len(preds_dicts.keys()) > 1:
+ # merge outputs with different scales after decoding bboxes
+ merged_bboxes = merge_aug_bboxes_3d(aug_bboxes, scale_img_metas,
+ self.pts_bbox_head.test_cfg)
+ return merged_bboxes
+ else:
+ for key in bbox_list[0].keys():
+ bbox_list[0][key] = bbox_list[0][key].to('cpu')
+ return bbox_list[0]
+
+ def aug_test(self, points, img_metas, imgs=None, rescale=False):
+ """Test function with augmentaiton."""
+ img_feats, pts_feats = self.extract_feats(points, img_metas, imgs)
+ bbox_list = dict()
+ if pts_feats and self.with_pts_bbox:
+ pts_bbox = self.aug_test_pts(pts_feats, img_metas, rescale)
+ bbox_list.update(pts_bbox=pts_bbox)
+ return [bbox_list]
diff --git a/mmdet3d/models/detectors/dynamic_voxelnet.py b/mmdet3d/models/detectors/dynamic_voxelnet.py
new file mode 100644
index 0000000..c4226ec
--- /dev/null
+++ b/mmdet3d/models/detectors/dynamic_voxelnet.py
@@ -0,0 +1,71 @@
+# Copyright (c) OpenMMLab. All rights reserved.
+import torch
+from mmcv.runner import force_fp32
+from torch.nn import functional as F
+
+from ..builder import DETECTORS
+from .voxelnet import VoxelNet
+
+
+@DETECTORS.register_module()
+class DynamicVoxelNet(VoxelNet):
+ r"""VoxelNet using `dynamic voxelization `_.
+ """
+
+ def __init__(self,
+ voxel_layer,
+ voxel_encoder,
+ middle_encoder,
+ backbone,
+ neck=None,
+ bbox_head=None,
+ train_cfg=None,
+ test_cfg=None,
+ pretrained=None,
+ init_cfg=None):
+ super(DynamicVoxelNet, self).__init__(
+ voxel_layer=voxel_layer,
+ voxel_encoder=voxel_encoder,
+ middle_encoder=middle_encoder,
+ backbone=backbone,
+ neck=neck,
+ bbox_head=bbox_head,
+ train_cfg=train_cfg,
+ test_cfg=test_cfg,
+ pretrained=pretrained,
+ init_cfg=init_cfg)
+
+ def extract_feat(self, points, img_metas):
+ """Extract features from points."""
+ voxels, coors = self.voxelize(points)
+ voxel_features, feature_coors = self.voxel_encoder(voxels, coors)
+ batch_size = coors[-1, 0].item() + 1
+ x = self.middle_encoder(voxel_features, feature_coors, batch_size)
+ x = self.backbone(x)
+ if self.with_neck:
+ x = self.neck(x)
+ return x
+
+ @torch.no_grad()
+ @force_fp32()
+ def voxelize(self, points):
+ """Apply dynamic voxelization to points.
+
+ Args:
+ points (list[torch.Tensor]): Points of each sample.
+
+ Returns:
+ tuple[torch.Tensor]: Concatenated points and coordinates.
+ """
+ coors = []
+ # dynamic voxelization only provide a coors mapping
+ for res in points:
+ res_coors = self.voxel_layer(res)
+ coors.append(res_coors)
+ points = torch.cat(points, dim=0)
+ coors_batch = []
+ for i, coor in enumerate(coors):
+ coor_pad = F.pad(coor, (1, 0), mode='constant', value=i)
+ coors_batch.append(coor_pad)
+ coors_batch = torch.cat(coors_batch, dim=0)
+ return points, coors_batch
diff --git a/mmdet3d/models/detectors/fcos_mono3d.py b/mmdet3d/models/detectors/fcos_mono3d.py
new file mode 100644
index 0000000..5baed7b
--- /dev/null
+++ b/mmdet3d/models/detectors/fcos_mono3d.py
@@ -0,0 +1,22 @@
+# Copyright (c) OpenMMLab. All rights reserved.
+from ..builder import DETECTORS
+from .single_stage_mono3d import SingleStageMono3DDetector
+
+
+@DETECTORS.register_module()
+class FCOSMono3D(SingleStageMono3DDetector):
+ r"""`FCOS3D `_ for monocular 3D object detection.
+
+ Currently please refer to our entry on the
+ `leaderboard `_.
+ """ # noqa: E501
+
+ def __init__(self,
+ backbone,
+ neck,
+ bbox_head,
+ train_cfg=None,
+ test_cfg=None,
+ pretrained=None):
+ super(FCOSMono3D, self).__init__(backbone, neck, bbox_head, train_cfg,
+ test_cfg, pretrained)
diff --git a/mmdet3d/models/detectors/groupfree3dnet.py b/mmdet3d/models/detectors/groupfree3dnet.py
new file mode 100644
index 0000000..71bd002
--- /dev/null
+++ b/mmdet3d/models/detectors/groupfree3dnet.py
@@ -0,0 +1,105 @@
+# Copyright (c) OpenMMLab. All rights reserved.
+import torch
+
+from mmdet3d.core import bbox3d2result, merge_aug_bboxes_3d
+from ..builder import DETECTORS
+from .single_stage import SingleStage3DDetector
+
+
+@DETECTORS.register_module()
+class GroupFree3DNet(SingleStage3DDetector):
+ """`Group-Free 3D `_."""
+
+ def __init__(self,
+ backbone,
+ bbox_head=None,
+ train_cfg=None,
+ test_cfg=None,
+ pretrained=None):
+ super(GroupFree3DNet, self).__init__(
+ backbone=backbone,
+ bbox_head=bbox_head,
+ train_cfg=train_cfg,
+ test_cfg=test_cfg,
+ pretrained=pretrained)
+
+ def forward_train(self,
+ points,
+ img_metas,
+ gt_bboxes_3d,
+ gt_labels_3d,
+ pts_semantic_mask=None,
+ pts_instance_mask=None,
+ gt_bboxes_ignore=None):
+ """Forward of training.
+
+ Args:
+ points (list[torch.Tensor]): Points of each batch.
+ img_metas (list): Image metas.
+ gt_bboxes_3d (:obj:`BaseInstance3DBoxes`): gt bboxes of each batch.
+ gt_labels_3d (list[torch.Tensor]): gt class labels of each batch.
+ pts_semantic_mask (list[torch.Tensor]): point-wise semantic
+ label of each batch.
+ pts_instance_mask (list[torch.Tensor]): point-wise instance
+ label of each batch.
+ gt_bboxes_ignore (list[torch.Tensor]): Specify
+ which bounding.
+
+ Returns:
+ dict[str: torch.Tensor]: Losses.
+ """
+ # TODO: refactor votenet series to reduce redundant codes.
+ points_cat = torch.stack(points)
+
+ x = self.extract_feat(points_cat)
+ bbox_preds = self.bbox_head(x, self.train_cfg.sample_mod)
+ loss_inputs = (points, gt_bboxes_3d, gt_labels_3d, pts_semantic_mask,
+ pts_instance_mask, img_metas)
+ losses = self.bbox_head.loss(
+ bbox_preds, *loss_inputs, gt_bboxes_ignore=gt_bboxes_ignore)
+ return losses
+
+ def simple_test(self, points, img_metas, imgs=None, rescale=False):
+ """Forward of testing.
+
+ Args:
+ points (list[torch.Tensor]): Points of each sample.
+ img_metas (list): Image metas.
+ rescale (bool): Whether to rescale results.
+ Returns:
+ list: Predicted 3d boxes.
+ """
+ points_cat = torch.stack(points)
+
+ x = self.extract_feat(points_cat)
+ bbox_preds = self.bbox_head(x, self.test_cfg.sample_mod)
+ bbox_list = self.bbox_head.get_bboxes(
+ points_cat, bbox_preds, img_metas, rescale=rescale)
+ bbox_results = [
+ bbox3d2result(bboxes, scores, labels)
+ for bboxes, scores, labels in bbox_list
+ ]
+ return bbox_results
+
+ def aug_test(self, points, img_metas, imgs=None, rescale=False):
+ """Test with augmentation."""
+ points_cat = [torch.stack(pts) for pts in points]
+ feats = self.extract_feats(points_cat, img_metas)
+
+ # only support aug_test for one sample
+ aug_bboxes = []
+ for x, pts_cat, img_meta in zip(feats, points_cat, img_metas):
+ bbox_preds = self.bbox_head(x, self.test_cfg.sample_mod)
+ bbox_list = self.bbox_head.get_bboxes(
+ pts_cat, bbox_preds, img_meta, rescale=rescale)
+ bbox_list = [
+ dict(boxes_3d=bboxes, scores_3d=scores, labels_3d=labels)
+ for bboxes, scores, labels in bbox_list
+ ]
+ aug_bboxes.append(bbox_list[0])
+
+ # after merging, bboxes will be rescaled to the original image size
+ merged_bboxes = merge_aug_bboxes_3d(aug_bboxes, img_metas,
+ self.bbox_head.test_cfg)
+
+ return [merged_bboxes]
diff --git a/mmdet3d/models/detectors/h3dnet.py b/mmdet3d/models/detectors/h3dnet.py
new file mode 100644
index 0000000..033a9a1
--- /dev/null
+++ b/mmdet3d/models/detectors/h3dnet.py
@@ -0,0 +1,176 @@
+# Copyright (c) OpenMMLab. All rights reserved.
+import torch
+
+from mmdet3d.core import merge_aug_bboxes_3d
+from ..builder import DETECTORS
+from .two_stage import TwoStage3DDetector
+
+
+@DETECTORS.register_module()
+class H3DNet(TwoStage3DDetector):
+ r"""H3DNet model.
+
+ Please refer to the `paper `_
+ """
+
+ def __init__(self,
+ backbone,
+ neck=None,
+ rpn_head=None,
+ roi_head=None,
+ train_cfg=None,
+ test_cfg=None,
+ pretrained=None,
+ init_cfg=None):
+ super(H3DNet, self).__init__(
+ backbone=backbone,
+ neck=neck,
+ rpn_head=rpn_head,
+ roi_head=roi_head,
+ train_cfg=train_cfg,
+ test_cfg=test_cfg,
+ pretrained=pretrained,
+ init_cfg=init_cfg)
+
+ def forward_train(self,
+ points,
+ img_metas,
+ gt_bboxes_3d,
+ gt_labels_3d,
+ pts_semantic_mask=None,
+ pts_instance_mask=None,
+ gt_bboxes_ignore=None):
+ """Forward of training.
+
+ Args:
+ points (list[torch.Tensor]): Points of each batch.
+ img_metas (list): Image metas.
+ gt_bboxes_3d (:obj:`BaseInstance3DBoxes`): gt bboxes of each batch.
+ gt_labels_3d (list[torch.Tensor]): gt class labels of each batch.
+ pts_semantic_mask (list[torch.Tensor]): point-wise semantic
+ label of each batch.
+ pts_instance_mask (list[torch.Tensor]): point-wise instance
+ label of each batch.
+ gt_bboxes_ignore (list[torch.Tensor]): Specify
+ which bounding.
+
+ Returns:
+ dict: Losses.
+ """
+ points_cat = torch.stack(points)
+
+ feats_dict = self.extract_feat(points_cat)
+ feats_dict['fp_xyz'] = [feats_dict['fp_xyz_net0'][-1]]
+ feats_dict['fp_features'] = [feats_dict['hd_feature']]
+ feats_dict['fp_indices'] = [feats_dict['fp_indices_net0'][-1]]
+
+ losses = dict()
+ if self.with_rpn:
+ rpn_outs = self.rpn_head(feats_dict, self.train_cfg.rpn.sample_mod)
+ feats_dict.update(rpn_outs)
+
+ rpn_loss_inputs = (points, gt_bboxes_3d, gt_labels_3d,
+ pts_semantic_mask, pts_instance_mask, img_metas)
+ rpn_losses = self.rpn_head.loss(
+ rpn_outs,
+ *rpn_loss_inputs,
+ gt_bboxes_ignore=gt_bboxes_ignore,
+ ret_target=True)
+ feats_dict['targets'] = rpn_losses.pop('targets')
+ losses.update(rpn_losses)
+
+ # Generate rpn proposals
+ proposal_cfg = self.train_cfg.get('rpn_proposal',
+ self.test_cfg.rpn)
+ proposal_inputs = (points, rpn_outs, img_metas)
+ proposal_list = self.rpn_head.get_bboxes(
+ *proposal_inputs, use_nms=proposal_cfg.use_nms)
+ feats_dict['proposal_list'] = proposal_list
+ else:
+ raise NotImplementedError
+
+ roi_losses = self.roi_head.forward_train(feats_dict, img_metas, points,
+ gt_bboxes_3d, gt_labels_3d,
+ pts_semantic_mask,
+ pts_instance_mask,
+ gt_bboxes_ignore)
+ losses.update(roi_losses)
+
+ return losses
+
+ def simple_test(self, points, img_metas, imgs=None, rescale=False):
+ """Forward of testing.
+
+ Args:
+ points (list[torch.Tensor]): Points of each sample.
+ img_metas (list): Image metas.
+ rescale (bool): Whether to rescale results.
+
+ Returns:
+ list: Predicted 3d boxes.
+ """
+ points_cat = torch.stack(points)
+
+ feats_dict = self.extract_feat(points_cat)
+ feats_dict['fp_xyz'] = [feats_dict['fp_xyz_net0'][-1]]
+ feats_dict['fp_features'] = [feats_dict['hd_feature']]
+ feats_dict['fp_indices'] = [feats_dict['fp_indices_net0'][-1]]
+
+ if self.with_rpn:
+ proposal_cfg = self.test_cfg.rpn
+ rpn_outs = self.rpn_head(feats_dict, proposal_cfg.sample_mod)
+ feats_dict.update(rpn_outs)
+ # Generate rpn proposals
+ proposal_list = self.rpn_head.get_bboxes(
+ points, rpn_outs, img_metas, use_nms=proposal_cfg.use_nms)
+ feats_dict['proposal_list'] = proposal_list
+ else:
+ raise NotImplementedError
+
+ return self.roi_head.simple_test(
+ feats_dict, img_metas, points_cat, rescale=rescale)
+
+ def aug_test(self, points, img_metas, imgs=None, rescale=False):
+ """Test with augmentation."""
+ points_cat = [torch.stack(pts) for pts in points]
+ feats_dict = self.extract_feats(points_cat, img_metas)
+ for feat_dict in feats_dict:
+ feat_dict['fp_xyz'] = [feat_dict['fp_xyz_net0'][-1]]
+ feat_dict['fp_features'] = [feat_dict['hd_feature']]
+ feat_dict['fp_indices'] = [feat_dict['fp_indices_net0'][-1]]
+
+ # only support aug_test for one sample
+ aug_bboxes = []
+ for feat_dict, pts_cat, img_meta in zip(feats_dict, points_cat,
+ img_metas):
+ if self.with_rpn:
+ proposal_cfg = self.test_cfg.rpn
+ rpn_outs = self.rpn_head(feat_dict, proposal_cfg.sample_mod)
+ feat_dict.update(rpn_outs)
+ # Generate rpn proposals
+ proposal_list = self.rpn_head.get_bboxes(
+ points, rpn_outs, img_metas, use_nms=proposal_cfg.use_nms)
+ feat_dict['proposal_list'] = proposal_list
+ else:
+ raise NotImplementedError
+
+ bbox_results = self.roi_head.simple_test(
+ feat_dict,
+ self.test_cfg.rcnn.sample_mod,
+ img_meta,
+ pts_cat,
+ rescale=rescale)
+ aug_bboxes.append(bbox_results)
+
+ # after merging, bboxes will be rescaled to the original image size
+ merged_bboxes = merge_aug_bboxes_3d(aug_bboxes, img_metas,
+ self.bbox_head.test_cfg)
+
+ return [merged_bboxes]
+
+ def extract_feats(self, points, img_metas):
+ """Extract features of multiple samples."""
+ return [
+ self.extract_feat(pts, img_meta)
+ for pts, img_meta in zip(points, img_metas)
+ ]
diff --git a/mmdet3d/models/detectors/imvotenet.py b/mmdet3d/models/detectors/imvotenet.py
new file mode 100644
index 0000000..9f48b81
--- /dev/null
+++ b/mmdet3d/models/detectors/imvotenet.py
@@ -0,0 +1,819 @@
+# Copyright (c) OpenMMLab. All rights reserved.
+import warnings
+
+import numpy as np
+import torch
+
+from mmdet3d.core import bbox3d2result, merge_aug_bboxes_3d
+from mmdet3d.models.utils import MLP
+from .. import builder
+from ..builder import DETECTORS
+from .base import Base3DDetector
+
+
+def sample_valid_seeds(mask, num_sampled_seed=1024):
+ r"""Randomly sample seeds from all imvotes.
+
+ Modified from ``_
+
+ Args:
+ mask (torch.Tensor): Bool tensor in shape (
+ seed_num*max_imvote_per_pixel), indicates
+ whether this imvote corresponds to a 2D bbox.
+ num_sampled_seed (int): How many to sample from all imvotes.
+
+ Returns:
+ torch.Tensor: Indices with shape (num_sampled_seed).
+ """ # noqa: E501
+ device = mask.device
+ batch_size = mask.shape[0]
+ sample_inds = mask.new_zeros((batch_size, num_sampled_seed),
+ dtype=torch.int64)
+ for bidx in range(batch_size):
+ # return index of non zero elements
+ valid_inds = torch.nonzero(mask[bidx, :]).squeeze(-1)
+ if len(valid_inds) < num_sampled_seed:
+ # compute set t1 - t2
+ t1 = torch.arange(num_sampled_seed, device=device)
+ t2 = valid_inds % num_sampled_seed
+ combined = torch.cat((t1, t2))
+ uniques, counts = combined.unique(return_counts=True)
+ difference = uniques[counts == 1]
+
+ rand_inds = torch.randperm(
+ len(difference),
+ device=device)[:num_sampled_seed - len(valid_inds)]
+ cur_sample_inds = difference[rand_inds]
+ cur_sample_inds = torch.cat((valid_inds, cur_sample_inds))
+ else:
+ rand_inds = torch.randperm(
+ len(valid_inds), device=device)[:num_sampled_seed]
+ cur_sample_inds = valid_inds[rand_inds]
+ sample_inds[bidx, :] = cur_sample_inds
+ return sample_inds
+
+
+@DETECTORS.register_module()
+class ImVoteNet(Base3DDetector):
+ r"""`ImVoteNet `_ for 3D detection."""
+
+ def __init__(self,
+ pts_backbone=None,
+ pts_bbox_heads=None,
+ pts_neck=None,
+ img_backbone=None,
+ img_neck=None,
+ img_roi_head=None,
+ img_rpn_head=None,
+ img_mlp=None,
+ freeze_img_branch=False,
+ fusion_layer=None,
+ num_sampled_seed=None,
+ train_cfg=None,
+ test_cfg=None,
+ pretrained=None,
+ init_cfg=None):
+
+ super(ImVoteNet, self).__init__(init_cfg=init_cfg)
+
+ # point branch
+ if pts_backbone is not None:
+ self.pts_backbone = builder.build_backbone(pts_backbone)
+ if pts_neck is not None:
+ self.pts_neck = builder.build_neck(pts_neck)
+ if pts_bbox_heads is not None:
+ pts_bbox_head_common = pts_bbox_heads.common
+ pts_bbox_head_common.update(
+ train_cfg=train_cfg.pts if train_cfg is not None else None)
+ pts_bbox_head_common.update(test_cfg=test_cfg.pts)
+ pts_bbox_head_joint = pts_bbox_head_common.copy()
+ pts_bbox_head_joint.update(pts_bbox_heads.joint)
+ pts_bbox_head_pts = pts_bbox_head_common.copy()
+ pts_bbox_head_pts.update(pts_bbox_heads.pts)
+ pts_bbox_head_img = pts_bbox_head_common.copy()
+ pts_bbox_head_img.update(pts_bbox_heads.img)
+
+ self.pts_bbox_head_joint = builder.build_head(pts_bbox_head_joint)
+ self.pts_bbox_head_pts = builder.build_head(pts_bbox_head_pts)
+ self.pts_bbox_head_img = builder.build_head(pts_bbox_head_img)
+ self.pts_bbox_heads = [
+ self.pts_bbox_head_joint, self.pts_bbox_head_pts,
+ self.pts_bbox_head_img
+ ]
+ self.loss_weights = pts_bbox_heads.loss_weights
+
+ # image branch
+ if img_backbone:
+ self.img_backbone = builder.build_backbone(img_backbone)
+ if img_neck is not None:
+ self.img_neck = builder.build_neck(img_neck)
+ if img_rpn_head is not None:
+ rpn_train_cfg = train_cfg.img_rpn if train_cfg \
+ is not None else None
+ img_rpn_head_ = img_rpn_head.copy()
+ img_rpn_head_.update(
+ train_cfg=rpn_train_cfg, test_cfg=test_cfg.img_rpn)
+ self.img_rpn_head = builder.build_head(img_rpn_head_)
+ if img_roi_head is not None:
+ rcnn_train_cfg = train_cfg.img_rcnn if train_cfg \
+ is not None else None
+ img_roi_head.update(
+ train_cfg=rcnn_train_cfg, test_cfg=test_cfg.img_rcnn)
+ self.img_roi_head = builder.build_head(img_roi_head)
+
+ # fusion
+ if fusion_layer is not None:
+ self.fusion_layer = builder.build_fusion_layer(fusion_layer)
+ self.max_imvote_per_pixel = fusion_layer.max_imvote_per_pixel
+
+ self.freeze_img_branch = freeze_img_branch
+ if freeze_img_branch:
+ self.freeze_img_branch_params()
+
+ if img_mlp is not None:
+ self.img_mlp = MLP(**img_mlp)
+
+ self.num_sampled_seed = num_sampled_seed
+
+ self.train_cfg = train_cfg
+ self.test_cfg = test_cfg
+
+ if pretrained is None:
+ img_pretrained = None
+ pts_pretrained = None
+ elif isinstance(pretrained, dict):
+ img_pretrained = pretrained.get('img', None)
+ pts_pretrained = pretrained.get('pts', None)
+ else:
+ raise ValueError(
+ f'pretrained should be a dict, got {type(pretrained)}')
+
+ if self.with_img_backbone:
+ if img_pretrained is not None:
+ warnings.warn('DeprecationWarning: pretrained is a deprecated '
+ 'key, please consider using init_cfg.')
+ self.img_backbone.init_cfg = dict(
+ type='Pretrained', checkpoint=img_pretrained)
+ if self.with_img_roi_head:
+ if img_pretrained is not None:
+ warnings.warn('DeprecationWarning: pretrained is a deprecated '
+ 'key, please consider using init_cfg.')
+ self.img_roi_head.init_cfg = dict(
+ type='Pretrained', checkpoint=img_pretrained)
+
+ if self.with_pts_backbone:
+ if img_pretrained is not None:
+ warnings.warn('DeprecationWarning: pretrained is a deprecated '
+ 'key, please consider using init_cfg.')
+ self.pts_backbone.init_cfg = dict(
+ type='Pretrained', checkpoint=pts_pretrained)
+
+ def freeze_img_branch_params(self):
+ """Freeze all image branch parameters."""
+ if self.with_img_bbox_head:
+ for param in self.img_bbox_head.parameters():
+ param.requires_grad = False
+ if self.with_img_backbone:
+ for param in self.img_backbone.parameters():
+ param.requires_grad = False
+ if self.with_img_neck:
+ for param in self.img_neck.parameters():
+ param.requires_grad = False
+ if self.with_img_rpn:
+ for param in self.img_rpn_head.parameters():
+ param.requires_grad = False
+ if self.with_img_roi_head:
+ for param in self.img_roi_head.parameters():
+ param.requires_grad = False
+
+ def _load_from_state_dict(self, state_dict, prefix, local_metadata, strict,
+ missing_keys, unexpected_keys, error_msgs):
+ """Overload in order to load img network ckpts into img branch."""
+ module_names = ['backbone', 'neck', 'roi_head', 'rpn_head']
+ for key in list(state_dict):
+ for module_name in module_names:
+ if key.startswith(module_name) and ('img_' +
+ key) not in state_dict:
+ state_dict['img_' + key] = state_dict.pop(key)
+
+ super()._load_from_state_dict(state_dict, prefix, local_metadata,
+ strict, missing_keys, unexpected_keys,
+ error_msgs)
+
+ def train(self, mode=True):
+ """Overload in order to keep image branch modules in eval mode."""
+ super(ImVoteNet, self).train(mode)
+ if self.freeze_img_branch:
+ if self.with_img_bbox_head:
+ self.img_bbox_head.eval()
+ if self.with_img_backbone:
+ self.img_backbone.eval()
+ if self.with_img_neck:
+ self.img_neck.eval()
+ if self.with_img_rpn:
+ self.img_rpn_head.eval()
+ if self.with_img_roi_head:
+ self.img_roi_head.eval()
+
+ @property
+ def with_img_bbox(self):
+ """bool: Whether the detector has a 2D image box head."""
+ return ((hasattr(self, 'img_roi_head') and self.img_roi_head.with_bbox)
+ or (hasattr(self, 'img_bbox_head')
+ and self.img_bbox_head is not None))
+
+ @property
+ def with_img_bbox_head(self):
+ """bool: Whether the detector has a 2D image box head (not roi)."""
+ return hasattr(self,
+ 'img_bbox_head') and self.img_bbox_head is not None
+
+ @property
+ def with_img_backbone(self):
+ """bool: Whether the detector has a 2D image backbone."""
+ return hasattr(self, 'img_backbone') and self.img_backbone is not None
+
+ @property
+ def with_img_neck(self):
+ """bool: Whether the detector has a neck in image branch."""
+ return hasattr(self, 'img_neck') and self.img_neck is not None
+
+ @property
+ def with_img_rpn(self):
+ """bool: Whether the detector has a 2D RPN in image detector branch."""
+ return hasattr(self, 'img_rpn_head') and self.img_rpn_head is not None
+
+ @property
+ def with_img_roi_head(self):
+ """bool: Whether the detector has a RoI Head in image branch."""
+ return hasattr(self, 'img_roi_head') and self.img_roi_head is not None
+
+ @property
+ def with_pts_bbox(self):
+ """bool: Whether the detector has a 3D box head."""
+ return hasattr(self,
+ 'pts_bbox_head') and self.pts_bbox_head is not None
+
+ @property
+ def with_pts_backbone(self):
+ """bool: Whether the detector has a 3D backbone."""
+ return hasattr(self, 'pts_backbone') and self.pts_backbone is not None
+
+ @property
+ def with_pts_neck(self):
+ """bool: Whether the detector has a neck in 3D detector branch."""
+ return hasattr(self, 'pts_neck') and self.pts_neck is not None
+
+ def extract_feat(self, imgs):
+ """Just to inherit from abstract method."""
+ pass
+
+ def extract_img_feat(self, img):
+ """Directly extract features from the img backbone+neck."""
+ x = self.img_backbone(img)
+ if self.with_img_neck:
+ x = self.img_neck(x)
+ return x
+
+ def extract_img_feats(self, imgs):
+ """Extract features from multiple images.
+
+ Args:
+ imgs (list[torch.Tensor]): A list of images. The images are
+ augmented from the same image but in different ways.
+
+ Returns:
+ list[torch.Tensor]: Features of different images
+ """
+
+ assert isinstance(imgs, list)
+ return [self.extract_img_feat(img) for img in imgs]
+
+ def extract_pts_feat(self, pts):
+ """Extract features of points."""
+ x = self.pts_backbone(pts)
+ if self.with_pts_neck:
+ x = self.pts_neck(x)
+
+ seed_points = x['fp_xyz'][-1]
+ seed_features = x['fp_features'][-1]
+ seed_indices = x['fp_indices'][-1]
+
+ return (seed_points, seed_features, seed_indices)
+
+ def extract_pts_feats(self, pts):
+ """Extract features of points from multiple samples."""
+ assert isinstance(pts, list)
+ return [self.extract_pts_feat(pt) for pt in pts]
+
+ @torch.no_grad()
+ def extract_bboxes_2d(self,
+ img,
+ img_metas,
+ train=True,
+ bboxes_2d=None,
+ **kwargs):
+ """Extract bounding boxes from 2d detector.
+
+ Args:
+ img (torch.Tensor): of shape (N, C, H, W) encoding input images.
+ Typically these should be mean centered and std scaled.
+ img_metas (list[dict]): Image meta info.
+ train (bool): train-time or not.
+ bboxes_2d (list[torch.Tensor]): provided 2d bboxes,
+ not supported yet.
+
+ Return:
+ list[torch.Tensor]: a list of processed 2d bounding boxes.
+ """
+ if bboxes_2d is None:
+ x = self.extract_img_feat(img)
+ proposal_list = self.img_rpn_head.simple_test_rpn(x, img_metas)
+ rets = self.img_roi_head.simple_test(
+ x, proposal_list, img_metas, rescale=False)
+
+ rets_processed = []
+ for ret in rets:
+ tmp = np.concatenate(ret, axis=0)
+ sem_class = img.new_zeros((len(tmp)))
+ start = 0
+ for i, bboxes in enumerate(ret):
+ sem_class[start:start + len(bboxes)] = i
+ start += len(bboxes)
+ ret = img.new_tensor(tmp)
+
+ # append class index
+ ret = torch.cat([ret, sem_class[:, None]], dim=-1)
+ inds = torch.argsort(ret[:, 4], descending=True)
+ ret = ret.index_select(0, inds)
+
+ # drop half bboxes during training for better generalization
+ if train:
+ rand_drop = torch.randperm(len(ret))[:(len(ret) + 1) // 2]
+ rand_drop = torch.sort(rand_drop)[0]
+ ret = ret[rand_drop]
+
+ rets_processed.append(ret.float())
+ return rets_processed
+ else:
+ rets_processed = []
+ for ret in bboxes_2d:
+ if len(ret) > 0 and train:
+ rand_drop = torch.randperm(len(ret))[:(len(ret) + 1) // 2]
+ rand_drop = torch.sort(rand_drop)[0]
+ ret = ret[rand_drop]
+ rets_processed.append(ret.float())
+ return rets_processed
+
+ def forward_train(self,
+ points=None,
+ img=None,
+ img_metas=None,
+ gt_bboxes=None,
+ gt_labels=None,
+ gt_bboxes_ignore=None,
+ gt_masks=None,
+ proposals=None,
+ bboxes_2d=None,
+ gt_bboxes_3d=None,
+ gt_labels_3d=None,
+ pts_semantic_mask=None,
+ pts_instance_mask=None,
+ **kwargs):
+ """Forwarding of train for image branch pretrain or stage 2 train.
+
+ Args:
+ points (list[torch.Tensor]): Points of each batch.
+ img (torch.Tensor): of shape (N, C, H, W) encoding input images.
+ Typically these should be mean centered and std scaled.
+ img_metas (list[dict]): list of image and point cloud meta info
+ dict. For example, keys include 'ori_shape', 'img_norm_cfg',
+ and 'transformation_3d_flow'. For details on the values of
+ the keys see `mmdet/datasets/pipelines/formatting.py:Collect`.
+ gt_bboxes (list[torch.Tensor]): Ground truth bboxes for each image
+ with shape (num_gts, 4) in [tl_x, tl_y, br_x, br_y] format.
+ gt_labels (list[torch.Tensor]): class indices for each
+ 2d bounding box.
+ gt_bboxes_ignore (list[torch.Tensor]): specify which
+ 2d bounding boxes can be ignored when computing the loss.
+ gt_masks (torch.Tensor): true segmentation masks for each
+ 2d bbox, used if the architecture supports a segmentation task.
+ proposals: override rpn proposals (2d) with custom proposals.
+ Use when `with_rpn` is False.
+ bboxes_2d (list[torch.Tensor]): provided 2d bboxes,
+ not supported yet.
+ gt_bboxes_3d (:obj:`BaseInstance3DBoxes`): 3d gt bboxes.
+ gt_labels_3d (list[torch.Tensor]): gt class labels for 3d bboxes.
+ pts_semantic_mask (list[torch.Tensor]): point-wise semantic
+ label of each batch.
+ pts_instance_mask (list[torch.Tensor]): point-wise instance
+ label of each batch.
+
+ Returns:
+ dict[str, torch.Tensor]: a dictionary of loss components.
+ """
+ if points is None:
+ x = self.extract_img_feat(img)
+ losses = dict()
+
+ # RPN forward and loss
+ if self.with_img_rpn:
+ proposal_cfg = self.train_cfg.get('img_rpn_proposal',
+ self.test_cfg.img_rpn)
+ rpn_losses, proposal_list = self.img_rpn_head.forward_train(
+ x,
+ img_metas,
+ gt_bboxes,
+ gt_labels=None,
+ gt_bboxes_ignore=gt_bboxes_ignore,
+ proposal_cfg=proposal_cfg)
+ losses.update(rpn_losses)
+ else:
+ proposal_list = proposals
+
+ roi_losses = self.img_roi_head.forward_train(
+ x, img_metas, proposal_list, gt_bboxes, gt_labels,
+ gt_bboxes_ignore, gt_masks, **kwargs)
+ losses.update(roi_losses)
+ return losses
+ else:
+ bboxes_2d = self.extract_bboxes_2d(
+ img, img_metas, bboxes_2d=bboxes_2d, **kwargs)
+
+ points = torch.stack(points)
+ seeds_3d, seed_3d_features, seed_indices = \
+ self.extract_pts_feat(points)
+
+ img_features, masks = self.fusion_layer(img, bboxes_2d, seeds_3d,
+ img_metas)
+
+ inds = sample_valid_seeds(masks, self.num_sampled_seed)
+ batch_size, img_feat_size = img_features.shape[:2]
+ pts_feat_size = seed_3d_features.shape[1]
+ inds_img = inds.view(batch_size, 1,
+ -1).expand(-1, img_feat_size, -1)
+ img_features = img_features.gather(-1, inds_img)
+ inds = inds % inds.shape[1]
+ inds_seed_xyz = inds.view(batch_size, -1, 1).expand(-1, -1, 3)
+ seeds_3d = seeds_3d.gather(1, inds_seed_xyz)
+ inds_seed_feats = inds.view(batch_size, 1,
+ -1).expand(-1, pts_feat_size, -1)
+ seed_3d_features = seed_3d_features.gather(-1, inds_seed_feats)
+ seed_indices = seed_indices.gather(1, inds)
+
+ img_features = self.img_mlp(img_features)
+ fused_features = torch.cat([seed_3d_features, img_features], dim=1)
+
+ feat_dict_joint = dict(
+ seed_points=seeds_3d,
+ seed_features=fused_features,
+ seed_indices=seed_indices)
+ feat_dict_pts = dict(
+ seed_points=seeds_3d,
+ seed_features=seed_3d_features,
+ seed_indices=seed_indices)
+ feat_dict_img = dict(
+ seed_points=seeds_3d,
+ seed_features=img_features,
+ seed_indices=seed_indices)
+
+ loss_inputs = (points, gt_bboxes_3d, gt_labels_3d,
+ pts_semantic_mask, pts_instance_mask, img_metas)
+ bbox_preds_joints = self.pts_bbox_head_joint(
+ feat_dict_joint, self.train_cfg.pts.sample_mod)
+ bbox_preds_pts = self.pts_bbox_head_pts(
+ feat_dict_pts, self.train_cfg.pts.sample_mod)
+ bbox_preds_img = self.pts_bbox_head_img(
+ feat_dict_img, self.train_cfg.pts.sample_mod)
+ losses_towers = []
+ losses_joint = self.pts_bbox_head_joint.loss(
+ bbox_preds_joints,
+ *loss_inputs,
+ gt_bboxes_ignore=gt_bboxes_ignore)
+ losses_pts = self.pts_bbox_head_pts.loss(
+ bbox_preds_pts,
+ *loss_inputs,
+ gt_bboxes_ignore=gt_bboxes_ignore)
+ losses_img = self.pts_bbox_head_img.loss(
+ bbox_preds_img,
+ *loss_inputs,
+ gt_bboxes_ignore=gt_bboxes_ignore)
+ losses_towers.append(losses_joint)
+ losses_towers.append(losses_pts)
+ losses_towers.append(losses_img)
+ combined_losses = dict()
+ for loss_term in losses_joint:
+ if 'loss' in loss_term:
+ combined_losses[loss_term] = 0
+ for i in range(len(losses_towers)):
+ combined_losses[loss_term] += \
+ losses_towers[i][loss_term] * \
+ self.loss_weights[i]
+ else:
+ # only save the metric of the joint head
+ # if it is not a loss
+ combined_losses[loss_term] = \
+ losses_towers[0][loss_term]
+
+ return combined_losses
+
+ def forward_test(self,
+ points=None,
+ img_metas=None,
+ img=None,
+ bboxes_2d=None,
+ **kwargs):
+ """Forwarding of test for image branch pretrain or stage 2 train.
+
+ Args:
+ points (list[list[torch.Tensor]], optional): the outer
+ list indicates test-time augmentations and the inner
+ list contains all points in the batch, where each Tensor
+ should have a shape NxC. Defaults to None.
+ img_metas (list[list[dict]], optional): the outer list
+ indicates test-time augs (multiscale, flip, etc.)
+ and the inner list indicates images in a batch.
+ Defaults to None.
+ img (list[list[torch.Tensor]], optional): the outer
+ list indicates test-time augmentations and inner Tensor
+ should have a shape NxCxHxW, which contains all images
+ in the batch. Defaults to None. Defaults to None.
+ bboxes_2d (list[list[torch.Tensor]], optional):
+ Provided 2d bboxes, not supported yet. Defaults to None.
+
+ Returns:
+ list[list[torch.Tensor]]|list[dict]: Predicted 2d or 3d boxes.
+ """
+ if points is None:
+ for var, name in [(img, 'img'), (img_metas, 'img_metas')]:
+ if not isinstance(var, list):
+ raise TypeError(
+ f'{name} must be a list, but got {type(var)}')
+
+ num_augs = len(img)
+ if num_augs != len(img_metas):
+ raise ValueError(f'num of augmentations ({len(img)}) '
+ f'!= num of image meta ({len(img_metas)})')
+
+ if num_augs == 1:
+ # proposals (List[List[Tensor]]): the outer list indicates
+ # test-time augs (multiscale, flip, etc.) and the inner list
+ # indicates images in a batch.
+ # The Tensor should have a shape Px4, where P is the number of
+ # proposals.
+ if 'proposals' in kwargs:
+ kwargs['proposals'] = kwargs['proposals'][0]
+ return self.simple_test_img_only(
+ img=img[0], img_metas=img_metas[0], **kwargs)
+ else:
+ assert img[0].size(0) == 1, 'aug test does not support ' \
+ 'inference with batch size ' \
+ f'{img[0].size(0)}'
+ # TODO: support test augmentation for predefined proposals
+ assert 'proposals' not in kwargs
+ return self.aug_test_img_only(
+ img=img, img_metas=img_metas, **kwargs)
+
+ else:
+ for var, name in [(points, 'points'), (img_metas, 'img_metas')]:
+ if not isinstance(var, list):
+ raise TypeError('{} must be a list, but got {}'.format(
+ name, type(var)))
+
+ num_augs = len(points)
+ if num_augs != len(img_metas):
+ raise ValueError(
+ 'num of augmentations ({}) != num of image meta ({})'.
+ format(len(points), len(img_metas)))
+
+ if num_augs == 1:
+ return self.simple_test(
+ points[0],
+ img_metas[0],
+ img[0],
+ bboxes_2d=bboxes_2d[0] if bboxes_2d is not None else None,
+ **kwargs)
+ else:
+ return self.aug_test(points, img_metas, img, bboxes_2d,
+ **kwargs)
+
+ def simple_test_img_only(self,
+ img,
+ img_metas,
+ proposals=None,
+ rescale=False):
+ r"""Test without augmentation, image network pretrain. May refer to
+ ``_.
+
+ Args:
+ img (torch.Tensor): Should have a shape NxCxHxW, which contains
+ all images in the batch.
+ img_metas (list[dict]):
+ proposals (list[Tensor], optional): override rpn proposals
+ with custom proposals. Defaults to None.
+ rescale (bool, optional): Whether or not rescale bboxes to the
+ original shape of input image. Defaults to False.
+
+ Returns:
+ list[list[torch.Tensor]]: Predicted 2d boxes.
+ """ # noqa: E501
+ assert self.with_img_bbox, 'Img bbox head must be implemented.'
+ assert self.with_img_backbone, 'Img backbone must be implemented.'
+ assert self.with_img_rpn, 'Img rpn must be implemented.'
+ assert self.with_img_roi_head, 'Img roi head must be implemented.'
+
+ x = self.extract_img_feat(img)
+
+ if proposals is None:
+ proposal_list = self.img_rpn_head.simple_test_rpn(x, img_metas)
+ else:
+ proposal_list = proposals
+
+ ret = self.img_roi_head.simple_test(
+ x, proposal_list, img_metas, rescale=rescale)
+
+ return ret
+
+ def simple_test(self,
+ points=None,
+ img_metas=None,
+ img=None,
+ bboxes_2d=None,
+ rescale=False,
+ **kwargs):
+ """Test without augmentation, stage 2.
+
+ Args:
+ points (list[torch.Tensor], optional): Elements in the list
+ should have a shape NxC, the list indicates all point-clouds
+ in the batch. Defaults to None.
+ img_metas (list[dict], optional): List indicates
+ images in a batch. Defaults to None.
+ img (torch.Tensor, optional): Should have a shape NxCxHxW,
+ which contains all images in the batch. Defaults to None.
+ bboxes_2d (list[torch.Tensor], optional):
+ Provided 2d bboxes, not supported yet. Defaults to None.
+ rescale (bool, optional): Whether or not rescale bboxes.
+ Defaults to False.
+
+ Returns:
+ list[dict]: Predicted 3d boxes.
+ """
+ bboxes_2d = self.extract_bboxes_2d(
+ img, img_metas, train=False, bboxes_2d=bboxes_2d, **kwargs)
+
+ points = torch.stack(points)
+ seeds_3d, seed_3d_features, seed_indices = \
+ self.extract_pts_feat(points)
+
+ img_features, masks = self.fusion_layer(img, bboxes_2d, seeds_3d,
+ img_metas)
+
+ inds = sample_valid_seeds(masks, self.num_sampled_seed)
+ batch_size, img_feat_size = img_features.shape[:2]
+ pts_feat_size = seed_3d_features.shape[1]
+ inds_img = inds.view(batch_size, 1, -1).expand(-1, img_feat_size, -1)
+ img_features = img_features.gather(-1, inds_img)
+ inds = inds % inds.shape[1]
+ inds_seed_xyz = inds.view(batch_size, -1, 1).expand(-1, -1, 3)
+ seeds_3d = seeds_3d.gather(1, inds_seed_xyz)
+ inds_seed_feats = inds.view(batch_size, 1,
+ -1).expand(-1, pts_feat_size, -1)
+ seed_3d_features = seed_3d_features.gather(-1, inds_seed_feats)
+ seed_indices = seed_indices.gather(1, inds)
+
+ img_features = self.img_mlp(img_features)
+
+ fused_features = torch.cat([seed_3d_features, img_features], dim=1)
+
+ feat_dict = dict(
+ seed_points=seeds_3d,
+ seed_features=fused_features,
+ seed_indices=seed_indices)
+ bbox_preds = self.pts_bbox_head_joint(feat_dict,
+ self.test_cfg.pts.sample_mod)
+ bbox_list = self.pts_bbox_head_joint.get_bboxes(
+ points, bbox_preds, img_metas, rescale=rescale)
+ bbox_results = [
+ bbox3d2result(bboxes, scores, labels)
+ for bboxes, scores, labels in bbox_list
+ ]
+ return bbox_results
+
+ def aug_test_img_only(self, img, img_metas, rescale=False):
+ r"""Test function with augmentation, image network pretrain. May refer
+ to ``_.
+
+ Args:
+ img (list[list[torch.Tensor]], optional): the outer
+ list indicates test-time augmentations and inner Tensor
+ should have a shape NxCxHxW, which contains all images
+ in the batch. Defaults to None. Defaults to None.
+ img_metas (list[list[dict]], optional): the outer list
+ indicates test-time augs (multiscale, flip, etc.)
+ and the inner list indicates images in a batch.
+ Defaults to None.
+ rescale (bool, optional): Whether or not rescale bboxes to the
+ original shape of input image. If rescale is False, then
+ returned bboxes and masks will fit the scale of imgs[0].
+ Defaults to None.
+
+ Returns:
+ list[list[torch.Tensor]]: Predicted 2d boxes.
+ """ # noqa: E501
+ assert self.with_img_bbox, 'Img bbox head must be implemented.'
+ assert self.with_img_backbone, 'Img backbone must be implemented.'
+ assert self.with_img_rpn, 'Img rpn must be implemented.'
+ assert self.with_img_roi_head, 'Img roi head must be implemented.'
+
+ x = self.extract_img_feats(img)
+ proposal_list = self.img_rpn_head.aug_test_rpn(x, img_metas)
+
+ return self.img_roi_head.aug_test(
+ x, proposal_list, img_metas, rescale=rescale)
+
+ def aug_test(self,
+ points=None,
+ img_metas=None,
+ imgs=None,
+ bboxes_2d=None,
+ rescale=False,
+ **kwargs):
+ """Test function with augmentation, stage 2.
+
+ Args:
+ points (list[list[torch.Tensor]], optional): the outer
+ list indicates test-time augmentations and the inner
+ list contains all points in the batch, where each Tensor
+ should have a shape NxC. Defaults to None.
+ img_metas (list[list[dict]], optional): the outer list
+ indicates test-time augs (multiscale, flip, etc.)
+ and the inner list indicates images in a batch.
+ Defaults to None.
+ imgs (list[list[torch.Tensor]], optional): the outer
+ list indicates test-time augmentations and inner Tensor
+ should have a shape NxCxHxW, which contains all images
+ in the batch. Defaults to None. Defaults to None.
+ bboxes_2d (list[list[torch.Tensor]], optional):
+ Provided 2d bboxes, not supported yet. Defaults to None.
+ rescale (bool, optional): Whether or not rescale bboxes.
+ Defaults to False.
+
+ Returns:
+ list[dict]: Predicted 3d boxes.
+ """
+ points_cat = [torch.stack(pts) for pts in points]
+ feats = self.extract_pts_feats(points_cat, img_metas)
+
+ # only support aug_test for one sample
+ aug_bboxes = []
+ for x, pts_cat, img_meta, bbox_2d, img in zip(feats, points_cat,
+ img_metas, bboxes_2d,
+ imgs):
+
+ bbox_2d = self.extract_bboxes_2d(
+ img, img_metas, train=False, bboxes_2d=bbox_2d, **kwargs)
+
+ seeds_3d, seed_3d_features, seed_indices = x
+
+ img_features, masks = self.fusion_layer(img, bbox_2d, seeds_3d,
+ img_metas)
+
+ inds = sample_valid_seeds(masks, self.num_sampled_seed)
+ batch_size, img_feat_size = img_features.shape[:2]
+ pts_feat_size = seed_3d_features.shape[1]
+ inds_img = inds.view(batch_size, 1,
+ -1).expand(-1, img_feat_size, -1)
+ img_features = img_features.gather(-1, inds_img)
+ inds = inds % inds.shape[1]
+ inds_seed_xyz = inds.view(batch_size, -1, 1).expand(-1, -1, 3)
+ seeds_3d = seeds_3d.gather(1, inds_seed_xyz)
+ inds_seed_feats = inds.view(batch_size, 1,
+ -1).expand(-1, pts_feat_size, -1)
+ seed_3d_features = seed_3d_features.gather(-1, inds_seed_feats)
+ seed_indices = seed_indices.gather(1, inds)
+
+ img_features = self.img_mlp(img_features)
+
+ fused_features = torch.cat([seed_3d_features, img_features], dim=1)
+
+ feat_dict = dict(
+ seed_points=seeds_3d,
+ seed_features=fused_features,
+ seed_indices=seed_indices)
+ bbox_preds = self.pts_bbox_head_joint(feat_dict,
+ self.test_cfg.pts.sample_mod)
+ bbox_list = self.pts_bbox_head_joint.get_bboxes(
+ pts_cat, bbox_preds, img_metas, rescale=rescale)
+
+ bbox_list = [
+ dict(boxes_3d=bboxes, scores_3d=scores, labels_3d=labels)
+ for bboxes, scores, labels in bbox_list
+ ]
+ aug_bboxes.append(bbox_list[0])
+
+ # after merging, bboxes will be rescaled to the original image size
+ merged_bboxes = merge_aug_bboxes_3d(aug_bboxes, img_metas,
+ self.bbox_head.test_cfg)
+
+ return [merged_bboxes]
diff --git a/mmdet3d/models/detectors/imvoxelnet.py b/mmdet3d/models/detectors/imvoxelnet.py
new file mode 100644
index 0000000..ca65b33
--- /dev/null
+++ b/mmdet3d/models/detectors/imvoxelnet.py
@@ -0,0 +1,138 @@
+# Copyright (c) OpenMMLab. All rights reserved.
+import torch
+
+from mmdet3d.core import bbox3d2result, build_prior_generator
+from mmdet3d.models.fusion_layers.point_fusion import point_sample
+from mmdet.models.detectors import BaseDetector
+from ..builder import DETECTORS, build_backbone, build_head, build_neck
+
+
+@DETECTORS.register_module()
+class ImVoxelNet(BaseDetector):
+ r"""`ImVoxelNet `_."""
+
+ def __init__(self,
+ backbone,
+ neck,
+ neck_3d,
+ bbox_head,
+ n_voxels,
+ anchor_generator,
+ train_cfg=None,
+ test_cfg=None,
+ pretrained=None,
+ init_cfg=None):
+ super().__init__(init_cfg=init_cfg)
+ self.backbone = build_backbone(backbone)
+ self.neck = build_neck(neck)
+ self.neck_3d = build_neck(neck_3d)
+ bbox_head.update(train_cfg=train_cfg)
+ bbox_head.update(test_cfg=test_cfg)
+ self.bbox_head = build_head(bbox_head)
+ self.n_voxels = n_voxels
+ self.anchor_generator = build_prior_generator(anchor_generator)
+ self.train_cfg = train_cfg
+ self.test_cfg = test_cfg
+
+ def extract_feat(self, img, img_metas):
+ """Extract 3d features from the backbone -> fpn -> 3d projection.
+
+ Args:
+ img (torch.Tensor): Input images of shape (N, C_in, H, W).
+ img_metas (list): Image metas.
+
+ Returns:
+ torch.Tensor: of shape (N, C_out, N_x, N_y, N_z)
+ """
+ x = self.backbone(img)
+ x = self.neck(x)[0]
+ points = self.anchor_generator.grid_anchors(
+ [self.n_voxels[::-1]], device=img.device)[0][:, :3]
+ volumes = []
+ for feature, img_meta in zip(x, img_metas):
+ img_scale_factor = (
+ points.new_tensor(img_meta['scale_factor'][:2])
+ if 'scale_factor' in img_meta.keys() else 1)
+ img_flip = img_meta['flip'] if 'flip' in img_meta.keys() else False
+ img_crop_offset = (
+ points.new_tensor(img_meta['img_crop_offset'])
+ if 'img_crop_offset' in img_meta.keys() else 0)
+ volume = point_sample(
+ img_meta,
+ img_features=feature[None, ...],
+ points=points,
+ proj_mat=points.new_tensor(img_meta['lidar2img']),
+ coord_type='LIDAR',
+ img_scale_factor=img_scale_factor,
+ img_crop_offset=img_crop_offset,
+ img_flip=img_flip,
+ img_pad_shape=img.shape[-2:],
+ img_shape=img_meta['img_shape'][:2],
+ aligned=False)
+ volumes.append(
+ volume.reshape(self.n_voxels[::-1] + [-1]).permute(3, 2, 1, 0))
+ x = torch.stack(volumes)
+ x = self.neck_3d(x)
+ return x
+
+ def forward_train(self, img, img_metas, gt_bboxes_3d, gt_labels_3d,
+ **kwargs):
+ """Forward of training.
+
+ Args:
+ img (torch.Tensor): Input images of shape (N, C_in, H, W).
+ img_metas (list): Image metas.
+ gt_bboxes_3d (:obj:`BaseInstance3DBoxes`): gt bboxes of each batch.
+ gt_labels_3d (list[torch.Tensor]): gt class labels of each batch.
+
+ Returns:
+ dict[str, torch.Tensor]: A dictionary of loss components.
+ """
+ x = self.extract_feat(img, img_metas)
+ x = self.bbox_head(x)
+ losses = self.bbox_head.loss(*x, gt_bboxes_3d, gt_labels_3d, img_metas)
+ return losses
+
+ def forward_test(self, img, img_metas, **kwargs):
+ """Forward of testing.
+
+ Args:
+ img (torch.Tensor): Input images of shape (N, C_in, H, W).
+ img_metas (list): Image metas.
+
+ Returns:
+ list[dict]: Predicted 3d boxes.
+ """
+ # not supporting aug_test for now
+ return self.simple_test(img, img_metas)
+
+ def simple_test(self, img, img_metas):
+ """Test without augmentations.
+
+ Args:
+ img (torch.Tensor): Input images of shape (N, C_in, H, W).
+ img_metas (list): Image metas.
+
+ Returns:
+ list[dict]: Predicted 3d boxes.
+ """
+ x = self.extract_feat(img, img_metas)
+ x = self.bbox_head(x)
+ bbox_list = self.bbox_head.get_bboxes(*x, img_metas)
+ bbox_results = [
+ bbox3d2result(det_bboxes, det_scores, det_labels)
+ for det_bboxes, det_scores, det_labels in bbox_list
+ ]
+ return bbox_results
+
+ def aug_test(self, imgs, img_metas, **kwargs):
+ """Test with augmentations.
+
+ Args:
+ imgs (list[torch.Tensor]): Input images of shape (N, C_in, H, W).
+ img_metas (list): Image metas.
+
+ Returns:
+ list[dict]: Predicted 3d boxes.
+ """
+ raise NotImplementedError
diff --git a/mmdet3d/models/detectors/mink_single_stage.py b/mmdet3d/models/detectors/mink_single_stage.py
new file mode 100644
index 0000000..ab5849a
--- /dev/null
+++ b/mmdet3d/models/detectors/mink_single_stage.py
@@ -0,0 +1,113 @@
+# Copyright (c) OpenMMLab. All rights reserved.
+# Adapted from https://github.com/SamsungLabs/fcaf3d/blob/master/mmdet3d/models/detectors/single_stage_sparse.py # noqa
+try:
+ import MinkowskiEngine as ME
+except ImportError:
+ import warnings
+ warnings.warn(
+ 'Please follow `getting_started.md` to install MinkowskiEngine.`')
+
+from mmdet3d.core import bbox3d2result
+from mmdet3d.models import DETECTORS, build_backbone, build_head
+from .base import Base3DDetector
+
+
+@DETECTORS.register_module()
+class MinkSingleStage3DDetector(Base3DDetector):
+ r"""Single stage detector based on MinkowskiEngine `GSDN
+ `_.
+
+ Args:
+ backbone (dict): Config of the backbone.
+ head (dict): Config of the head.
+ voxel_size (float): Voxel size in meters.
+ train_cfg (dict, optional): Config for train stage. Defaults to None.
+ test_cfg (dict, optional): Config for test stage. Defaults to None.
+ init_cfg (dict, optional): Config for weight initialization.
+ Defaults to None.
+ pretrained (str, optional): Deprecated initialization parameter.
+ Defaults to None.
+ """
+
+ def __init__(self,
+ backbone,
+ head,
+ voxel_size,
+ train_cfg=None,
+ test_cfg=None,
+ init_cfg=None,
+ pretrained=None):
+ super(MinkSingleStage3DDetector, self).__init__(init_cfg)
+ self.backbone = build_backbone(backbone)
+ head.update(train_cfg=train_cfg)
+ head.update(test_cfg=test_cfg)
+ self.head = build_head(head)
+ self.voxel_size = voxel_size
+ self.init_weights()
+
+ def extract_feat(self, *args):
+ """Just implement @abstractmethod of BaseModule."""
+
+ def extract_feats(self, points):
+ """Extract features from points.
+
+ Args:
+ points (list[Tensor]): Raw point clouds.
+
+ Returns:
+ SparseTensor: Voxelized point clouds.
+ """
+ coordinates, features = ME.utils.batch_sparse_collate(
+ [(p[:, :3] / self.voxel_size, p[:, 3:]) for p in points],
+ device=points[0].device)
+ x = ME.SparseTensor(coordinates=coordinates, features=features)
+ x = self.backbone(x)
+ return x
+
+ def forward_train(self, points, gt_bboxes_3d, gt_labels_3d, img_metas):
+ """Forward of training.
+
+ Args:
+ points (list[Tensor]): Raw point clouds.
+ gt_bboxes (list[BaseInstance3DBoxes]): Ground truth
+ bboxes of each sample.
+ gt_labels(list[torch.Tensor]): Labels of each sample.
+ img_metas (list[dict]): Contains scene meta infos.
+
+ Returns:
+ dict: Centerness, bbox and classification loss values.
+ """
+ x = self.extract_feats(points)
+ losses = self.head.forward_train(x, gt_bboxes_3d, gt_labels_3d,
+ img_metas)
+ return losses
+
+ def simple_test(self, points, img_metas, *args, **kwargs):
+ """Test without augmentations.
+
+ Args:
+ points (list[torch.Tensor]): Points of each sample.
+ img_metas (list[dict]): Contains scene meta infos.
+
+ Returns:
+ list[dict]: Predicted 3d boxes.
+ """
+ x = self.extract_feats(points)
+ bbox_list = self.head.forward_test(x, img_metas)
+ bbox_results = [
+ bbox3d2result(bboxes, scores, labels)
+ for bboxes, scores, labels in bbox_list
+ ]
+ return bbox_results
+
+ def aug_test(self, points, img_metas, **kwargs):
+ """Test with augmentations.
+
+ Args:
+ points (list[list[torch.Tensor]]): Points of each sample.
+ img_metas (list[dict]): Contains scene meta infos.
+
+ Returns:
+ list[dict]: Predicted 3d boxes.
+ """
+ raise NotImplementedError
diff --git a/mmdet3d/models/detectors/mvx_faster_rcnn.py b/mmdet3d/models/detectors/mvx_faster_rcnn.py
new file mode 100644
index 0000000..07efad6
--- /dev/null
+++ b/mmdet3d/models/detectors/mvx_faster_rcnn.py
@@ -0,0 +1,61 @@
+# Copyright (c) OpenMMLab. All rights reserved.
+import torch
+from mmcv.runner import force_fp32
+from torch.nn import functional as F
+
+from ..builder import DETECTORS
+from .mvx_two_stage import MVXTwoStageDetector
+
+
+@DETECTORS.register_module()
+class MVXFasterRCNN(MVXTwoStageDetector):
+ """Multi-modality VoxelNet using Faster R-CNN."""
+
+ def __init__(self, **kwargs):
+ super(MVXFasterRCNN, self).__init__(**kwargs)
+
+
+@DETECTORS.register_module()
+class DynamicMVXFasterRCNN(MVXTwoStageDetector):
+ """Multi-modality VoxelNet using Faster R-CNN and dynamic voxelization."""
+
+ def __init__(self, **kwargs):
+ super(DynamicMVXFasterRCNN, self).__init__(**kwargs)
+
+ @torch.no_grad()
+ @force_fp32()
+ def voxelize(self, points):
+ """Apply dynamic voxelization to points.
+
+ Args:
+ points (list[torch.Tensor]): Points of each sample.
+
+ Returns:
+ tuple[torch.Tensor]: Concatenated points and coordinates.
+ """
+ coors = []
+ # dynamic voxelization only provide a coors mapping
+ for res in points:
+ res_coors = self.pts_voxel_layer(res)
+ coors.append(res_coors)
+ points = torch.cat(points, dim=0)
+ coors_batch = []
+ for i, coor in enumerate(coors):
+ coor_pad = F.pad(coor, (1, 0), mode='constant', value=i)
+ coors_batch.append(coor_pad)
+ coors_batch = torch.cat(coors_batch, dim=0)
+ return points, coors_batch
+
+ def extract_pts_feat(self, points, img_feats, img_metas):
+ """Extract point features."""
+ if not self.with_pts_bbox:
+ return None
+ voxels, coors = self.voxelize(points)
+ voxel_features, feature_coors = self.pts_voxel_encoder(
+ voxels, coors, points, img_feats, img_metas)
+ batch_size = coors[-1, 0] + 1
+ x = self.pts_middle_encoder(voxel_features, feature_coors, batch_size)
+ x = self.pts_backbone(x)
+ if self.with_pts_neck:
+ x = self.pts_neck(x)
+ return x
diff --git a/mmdet3d/models/detectors/mvx_two_stage.py b/mmdet3d/models/detectors/mvx_two_stage.py
new file mode 100644
index 0000000..1eba10d
--- /dev/null
+++ b/mmdet3d/models/detectors/mvx_two_stage.py
@@ -0,0 +1,503 @@
+# Copyright (c) OpenMMLab. All rights reserved.
+import warnings
+from os import path as osp
+
+import mmcv
+import torch
+from mmcv.ops import Voxelization
+from mmcv.parallel import DataContainer as DC
+from mmcv.runner import force_fp32
+from torch.nn import functional as F
+
+from mmdet3d.core import (Box3DMode, Coord3DMode, bbox3d2result,
+ merge_aug_bboxes_3d, show_result)
+from mmdet.core import multi_apply
+from .. import builder
+from ..builder import DETECTORS
+from .base import Base3DDetector
+
+
+@DETECTORS.register_module()
+class MVXTwoStageDetector(Base3DDetector):
+ """Base class of Multi-modality VoxelNet."""
+
+ def __init__(self,
+ pts_voxel_layer=None,
+ pts_voxel_encoder=None,
+ pts_middle_encoder=None,
+ pts_fusion_layer=None,
+ img_backbone=None,
+ pts_backbone=None,
+ img_neck=None,
+ pts_neck=None,
+ pts_bbox_head=None,
+ img_roi_head=None,
+ img_rpn_head=None,
+ train_cfg=None,
+ test_cfg=None,
+ pretrained=None,
+ init_cfg=None):
+ super(MVXTwoStageDetector, self).__init__(init_cfg=init_cfg)
+
+ if pts_voxel_layer:
+ self.pts_voxel_layer = Voxelization(**pts_voxel_layer)
+ if pts_voxel_encoder:
+ self.pts_voxel_encoder = builder.build_voxel_encoder(
+ pts_voxel_encoder)
+ if pts_middle_encoder:
+ self.pts_middle_encoder = builder.build_middle_encoder(
+ pts_middle_encoder)
+ if pts_backbone:
+ self.pts_backbone = builder.build_backbone(pts_backbone)
+ if pts_fusion_layer:
+ self.pts_fusion_layer = builder.build_fusion_layer(
+ pts_fusion_layer)
+ if pts_neck is not None:
+ self.pts_neck = builder.build_neck(pts_neck)
+ if pts_bbox_head:
+ pts_train_cfg = train_cfg.pts if train_cfg else None
+ pts_bbox_head.update(train_cfg=pts_train_cfg)
+ pts_test_cfg = test_cfg.pts if test_cfg else None
+ pts_bbox_head.update(test_cfg=pts_test_cfg)
+ self.pts_bbox_head = builder.build_head(pts_bbox_head)
+
+ if img_backbone:
+ self.img_backbone = builder.build_backbone(img_backbone)
+ if img_neck is not None:
+ self.img_neck = builder.build_neck(img_neck)
+ if img_rpn_head is not None:
+ self.img_rpn_head = builder.build_head(img_rpn_head)
+ if img_roi_head is not None:
+ self.img_roi_head = builder.build_head(img_roi_head)
+
+ self.train_cfg = train_cfg
+ self.test_cfg = test_cfg
+
+ if pretrained is None:
+ img_pretrained = None
+ pts_pretrained = None
+ elif isinstance(pretrained, dict):
+ img_pretrained = pretrained.get('img', None)
+ pts_pretrained = pretrained.get('pts', None)
+ else:
+ raise ValueError(
+ f'pretrained should be a dict, got {type(pretrained)}')
+
+ if self.with_img_backbone:
+ if img_pretrained is not None:
+ warnings.warn('DeprecationWarning: pretrained is a deprecated '
+ 'key, please consider using init_cfg.')
+ self.img_backbone.init_cfg = dict(
+ type='Pretrained', checkpoint=img_pretrained)
+ if self.with_img_roi_head:
+ if img_pretrained is not None:
+ warnings.warn('DeprecationWarning: pretrained is a deprecated '
+ 'key, please consider using init_cfg.')
+ self.img_roi_head.init_cfg = dict(
+ type='Pretrained', checkpoint=img_pretrained)
+ if self.with_pts_backbone:
+ if pts_pretrained is not None:
+ warnings.warn('DeprecationWarning: pretrained is a deprecated '
+ 'key, please consider using init_cfg')
+ self.pts_backbone.init_cfg = dict(
+ type='Pretrained', checkpoint=pts_pretrained)
+
+ @property
+ def with_img_shared_head(self):
+ """bool: Whether the detector has a shared head in image branch."""
+ return hasattr(self,
+ 'img_shared_head') and self.img_shared_head is not None
+
+ @property
+ def with_pts_bbox(self):
+ """bool: Whether the detector has a 3D box head."""
+ return hasattr(self,
+ 'pts_bbox_head') and self.pts_bbox_head is not None
+
+ @property
+ def with_img_bbox(self):
+ """bool: Whether the detector has a 2D image box head."""
+ return hasattr(self,
+ 'img_bbox_head') and self.img_bbox_head is not None
+
+ @property
+ def with_img_backbone(self):
+ """bool: Whether the detector has a 2D image backbone."""
+ return hasattr(self, 'img_backbone') and self.img_backbone is not None
+
+ @property
+ def with_pts_backbone(self):
+ """bool: Whether the detector has a 3D backbone."""
+ return hasattr(self, 'pts_backbone') and self.pts_backbone is not None
+
+ @property
+ def with_fusion(self):
+ """bool: Whether the detector has a fusion layer."""
+ return hasattr(self,
+ 'pts_fusion_layer') and self.fusion_layer is not None
+
+ @property
+ def with_img_neck(self):
+ """bool: Whether the detector has a neck in image branch."""
+ return hasattr(self, 'img_neck') and self.img_neck is not None
+
+ @property
+ def with_pts_neck(self):
+ """bool: Whether the detector has a neck in 3D detector branch."""
+ return hasattr(self, 'pts_neck') and self.pts_neck is not None
+
+ @property
+ def with_img_rpn(self):
+ """bool: Whether the detector has a 2D RPN in image detector branch."""
+ return hasattr(self, 'img_rpn_head') and self.img_rpn_head is not None
+
+ @property
+ def with_img_roi_head(self):
+ """bool: Whether the detector has a RoI Head in image branch."""
+ return hasattr(self, 'img_roi_head') and self.img_roi_head is not None
+
+ @property
+ def with_voxel_encoder(self):
+ """bool: Whether the detector has a voxel encoder."""
+ return hasattr(self,
+ 'voxel_encoder') and self.voxel_encoder is not None
+
+ @property
+ def with_middle_encoder(self):
+ """bool: Whether the detector has a middle encoder."""
+ return hasattr(self,
+ 'middle_encoder') and self.middle_encoder is not None
+
+ def extract_img_feat(self, img, img_metas):
+ """Extract features of images."""
+ if self.with_img_backbone and img is not None:
+ input_shape = img.shape[-2:]
+ # update real input shape of each single img
+ for img_meta in img_metas:
+ img_meta.update(input_shape=input_shape)
+
+ if img.dim() == 5 and img.size(0) == 1:
+ img.squeeze_()
+ elif img.dim() == 5 and img.size(0) > 1:
+ B, N, C, H, W = img.size()
+ img = img.view(B * N, C, H, W)
+ img_feats = self.img_backbone(img)
+ else:
+ return None
+ if self.with_img_neck:
+ img_feats = self.img_neck(img_feats)
+ return img_feats
+
+ def extract_pts_feat(self, pts, img_feats, img_metas):
+ """Extract features of points."""
+ if not self.with_pts_bbox:
+ return None
+ voxels, num_points, coors = self.voxelize(pts)
+ voxel_features = self.pts_voxel_encoder(voxels, num_points, coors,
+ img_feats, img_metas)
+ batch_size = coors[-1, 0] + 1
+ x = self.pts_middle_encoder(voxel_features, coors, batch_size)
+ x = self.pts_backbone(x)
+ if self.with_pts_neck:
+ x = self.pts_neck(x)
+ return x
+
+ def extract_feat(self, points, img, img_metas):
+ """Extract features from images and points."""
+ img_feats = self.extract_img_feat(img, img_metas)
+ pts_feats = self.extract_pts_feat(points, img_feats, img_metas)
+ return (img_feats, pts_feats)
+
+ @torch.no_grad()
+ @force_fp32()
+ def voxelize(self, points):
+ """Apply dynamic voxelization to points.
+
+ Args:
+ points (list[torch.Tensor]): Points of each sample.
+
+ Returns:
+ tuple[torch.Tensor]: Concatenated points, number of points
+ per voxel, and coordinates.
+ """
+ voxels, coors, num_points = [], [], []
+ for res in points:
+ res_voxels, res_coors, res_num_points = self.pts_voxel_layer(res)
+ voxels.append(res_voxels)
+ coors.append(res_coors)
+ num_points.append(res_num_points)
+ voxels = torch.cat(voxels, dim=0)
+ num_points = torch.cat(num_points, dim=0)
+ coors_batch = []
+ for i, coor in enumerate(coors):
+ coor_pad = F.pad(coor, (1, 0), mode='constant', value=i)
+ coors_batch.append(coor_pad)
+ coors_batch = torch.cat(coors_batch, dim=0)
+ return voxels, num_points, coors_batch
+
+ def forward_train(self,
+ points=None,
+ img_metas=None,
+ gt_bboxes_3d=None,
+ gt_labels_3d=None,
+ gt_labels=None,
+ gt_bboxes=None,
+ img=None,
+ proposals=None,
+ gt_bboxes_ignore=None):
+ """Forward training function.
+
+ Args:
+ points (list[torch.Tensor], optional): Points of each sample.
+ Defaults to None.
+ img_metas (list[dict], optional): Meta information of each sample.
+ Defaults to None.
+ gt_bboxes_3d (list[:obj:`BaseInstance3DBoxes`], optional):
+ Ground truth 3D boxes. Defaults to None.
+ gt_labels_3d (list[torch.Tensor], optional): Ground truth labels
+ of 3D boxes. Defaults to None.
+ gt_labels (list[torch.Tensor], optional): Ground truth labels
+ of 2D boxes in images. Defaults to None.
+ gt_bboxes (list[torch.Tensor], optional): Ground truth 2D boxes in
+ images. Defaults to None.
+ img (torch.Tensor, optional): Images of each sample with shape
+ (N, C, H, W). Defaults to None.
+ proposals ([list[torch.Tensor], optional): Predicted proposals
+ used for training Fast RCNN. Defaults to None.
+ gt_bboxes_ignore (list[torch.Tensor], optional): Ground truth
+ 2D boxes in images to be ignored. Defaults to None.
+
+ Returns:
+ dict: Losses of different branches.
+ """
+ img_feats, pts_feats = self.extract_feat(
+ points, img=img, img_metas=img_metas)
+ losses = dict()
+ if pts_feats:
+ losses_pts = self.forward_pts_train(pts_feats, gt_bboxes_3d,
+ gt_labels_3d, img_metas,
+ gt_bboxes_ignore)
+ losses.update(losses_pts)
+ if img_feats:
+ losses_img = self.forward_img_train(
+ img_feats,
+ img_metas=img_metas,
+ gt_bboxes=gt_bboxes,
+ gt_labels=gt_labels,
+ gt_bboxes_ignore=gt_bboxes_ignore,
+ proposals=proposals)
+ losses.update(losses_img)
+ return losses
+
+ def forward_pts_train(self,
+ pts_feats,
+ gt_bboxes_3d,
+ gt_labels_3d,
+ img_metas,
+ gt_bboxes_ignore=None):
+ """Forward function for point cloud branch.
+
+ Args:
+ pts_feats (list[torch.Tensor]): Features of point cloud branch
+ gt_bboxes_3d (list[:obj:`BaseInstance3DBoxes`]): Ground truth
+ boxes for each sample.
+ gt_labels_3d (list[torch.Tensor]): Ground truth labels for
+ boxes of each sampole
+ img_metas (list[dict]): Meta information of samples.
+ gt_bboxes_ignore (list[torch.Tensor], optional): Ground truth
+ boxes to be ignored. Defaults to None.
+
+ Returns:
+ dict: Losses of each branch.
+ """
+ outs = self.pts_bbox_head(pts_feats)
+ loss_inputs = outs + (gt_bboxes_3d, gt_labels_3d, img_metas)
+ losses = self.pts_bbox_head.loss(
+ *loss_inputs, gt_bboxes_ignore=gt_bboxes_ignore)
+ return losses
+
+ def forward_img_train(self,
+ x,
+ img_metas,
+ gt_bboxes,
+ gt_labels,
+ gt_bboxes_ignore=None,
+ proposals=None,
+ **kwargs):
+ """Forward function for image branch.
+
+ This function works similar to the forward function of Faster R-CNN.
+
+ Args:
+ x (list[torch.Tensor]): Image features of shape (B, C, H, W)
+ of multiple levels.
+ img_metas (list[dict]): Meta information of images.
+ gt_bboxes (list[torch.Tensor]): Ground truth boxes of each image
+ sample.
+ gt_labels (list[torch.Tensor]): Ground truth labels of boxes.
+ gt_bboxes_ignore (list[torch.Tensor], optional): Ground truth
+ boxes to be ignored. Defaults to None.
+ proposals (list[torch.Tensor], optional): Proposals of each sample.
+ Defaults to None.
+
+ Returns:
+ dict: Losses of each branch.
+ """
+ losses = dict()
+ # RPN forward and loss
+ if self.with_img_rpn:
+ rpn_outs = self.img_rpn_head(x)
+ rpn_loss_inputs = rpn_outs + (gt_bboxes, img_metas,
+ self.train_cfg.img_rpn)
+ rpn_losses = self.img_rpn_head.loss(
+ *rpn_loss_inputs, gt_bboxes_ignore=gt_bboxes_ignore)
+ losses.update(rpn_losses)
+
+ proposal_cfg = self.train_cfg.get('img_rpn_proposal',
+ self.test_cfg.img_rpn)
+ proposal_inputs = rpn_outs + (img_metas, proposal_cfg)
+ proposal_list = self.img_rpn_head.get_bboxes(*proposal_inputs)
+ else:
+ proposal_list = proposals
+
+ # bbox head forward and loss
+ if self.with_img_bbox:
+ # bbox head forward and loss
+ img_roi_losses = self.img_roi_head.forward_train(
+ x, img_metas, proposal_list, gt_bboxes, gt_labels,
+ gt_bboxes_ignore, **kwargs)
+ losses.update(img_roi_losses)
+
+ return losses
+
+ def simple_test_img(self, x, img_metas, proposals=None, rescale=False):
+ """Test without augmentation."""
+ if proposals is None:
+ proposal_list = self.simple_test_rpn(x, img_metas,
+ self.test_cfg.img_rpn)
+ else:
+ proposal_list = proposals
+
+ return self.img_roi_head.simple_test(
+ x, proposal_list, img_metas, rescale=rescale)
+
+ def simple_test_rpn(self, x, img_metas, rpn_test_cfg):
+ """RPN test function."""
+ rpn_outs = self.img_rpn_head(x)
+ proposal_inputs = rpn_outs + (img_metas, rpn_test_cfg)
+ proposal_list = self.img_rpn_head.get_bboxes(*proposal_inputs)
+ return proposal_list
+
+ def simple_test_pts(self, x, img_metas, rescale=False):
+ """Test function of point cloud branch."""
+ outs = self.pts_bbox_head(x)
+ bbox_list = self.pts_bbox_head.get_bboxes(
+ *outs, img_metas, rescale=rescale)
+ bbox_results = [
+ bbox3d2result(bboxes, scores, labels)
+ for bboxes, scores, labels in bbox_list
+ ]
+ return bbox_results
+
+ def simple_test(self, points, img_metas, img=None, rescale=False):
+ """Test function without augmentaiton."""
+ img_feats, pts_feats = self.extract_feat(
+ points, img=img, img_metas=img_metas)
+
+ bbox_list = [dict() for i in range(len(img_metas))]
+ if pts_feats and self.with_pts_bbox:
+ bbox_pts = self.simple_test_pts(
+ pts_feats, img_metas, rescale=rescale)
+ for result_dict, pts_bbox in zip(bbox_list, bbox_pts):
+ result_dict['pts_bbox'] = pts_bbox
+ if img_feats and self.with_img_bbox:
+ bbox_img = self.simple_test_img(
+ img_feats, img_metas, rescale=rescale)
+ for result_dict, img_bbox in zip(bbox_list, bbox_img):
+ result_dict['img_bbox'] = img_bbox
+ return bbox_list
+
+ def aug_test(self, points, img_metas, imgs=None, rescale=False):
+ """Test function with augmentaiton."""
+ img_feats, pts_feats = self.extract_feats(points, img_metas, imgs)
+
+ bbox_list = dict()
+ if pts_feats and self.with_pts_bbox:
+ bbox_pts = self.aug_test_pts(pts_feats, img_metas, rescale)
+ bbox_list.update(pts_bbox=bbox_pts)
+ return [bbox_list]
+
+ def extract_feats(self, points, img_metas, imgs=None):
+ """Extract point and image features of multiple samples."""
+ if imgs is None:
+ imgs = [None] * len(img_metas)
+ img_feats, pts_feats = multi_apply(self.extract_feat, points, imgs,
+ img_metas)
+ return img_feats, pts_feats
+
+ def aug_test_pts(self, feats, img_metas, rescale=False):
+ """Test function of point cloud branch with augmentaiton."""
+ # only support aug_test for one sample
+ aug_bboxes = []
+ for x, img_meta in zip(feats, img_metas):
+ outs = self.pts_bbox_head(x)
+ bbox_list = self.pts_bbox_head.get_bboxes(
+ *outs, img_meta, rescale=rescale)
+ bbox_list = [
+ dict(boxes_3d=bboxes, scores_3d=scores, labels_3d=labels)
+ for bboxes, scores, labels in bbox_list
+ ]
+ aug_bboxes.append(bbox_list[0])
+
+ # after merging, bboxes will be rescaled to the original image size
+ merged_bboxes = merge_aug_bboxes_3d(aug_bboxes, img_metas,
+ self.pts_bbox_head.test_cfg)
+ return merged_bboxes
+
+ def show_results(self, data, result, out_dir):
+ """Results visualization.
+
+ Args:
+ data (dict): Input points and the information of the sample.
+ result (dict): Prediction results.
+ out_dir (str): Output directory of visualization result.
+ """
+ for batch_id in range(len(result)):
+ if isinstance(data['points'][0], DC):
+ points = data['points'][0]._data[0][batch_id].numpy()
+ elif mmcv.is_list_of(data['points'][0], torch.Tensor):
+ points = data['points'][0][batch_id]
+ else:
+ ValueError(f"Unsupported data type {type(data['points'][0])} "
+ f'for visualization!')
+ if isinstance(data['img_metas'][0], DC):
+ pts_filename = data['img_metas'][0]._data[0][batch_id][
+ 'pts_filename']
+ box_mode_3d = data['img_metas'][0]._data[0][batch_id][
+ 'box_mode_3d']
+ elif mmcv.is_list_of(data['img_metas'][0], dict):
+ pts_filename = data['img_metas'][0][batch_id]['pts_filename']
+ box_mode_3d = data['img_metas'][0][batch_id]['box_mode_3d']
+ else:
+ ValueError(
+ f"Unsupported data type {type(data['img_metas'][0])} "
+ f'for visualization!')
+ file_name = osp.split(pts_filename)[-1].split('.')[0]
+
+ assert out_dir is not None, 'Expect out_dir, got none.'
+ inds = result[batch_id]['pts_bbox']['scores_3d'] > 0.1
+ pred_bboxes = result[batch_id]['pts_bbox']['boxes_3d'][inds]
+
+ # for now we convert points and bbox into depth mode
+ if (box_mode_3d == Box3DMode.CAM) or (box_mode_3d
+ == Box3DMode.LIDAR):
+ points = Coord3DMode.convert_point(points, Coord3DMode.LIDAR,
+ Coord3DMode.DEPTH)
+ pred_bboxes = Box3DMode.convert(pred_bboxes, box_mode_3d,
+ Box3DMode.DEPTH)
+ elif box_mode_3d != Box3DMode.DEPTH:
+ ValueError(
+ f'Unsupported box_mode_3d {box_mode_3d} for conversion!')
+
+ pred_bboxes = pred_bboxes.tensor.cpu().numpy()
+ show_result(points, None, pred_bboxes, out_dir, file_name)
diff --git a/mmdet3d/models/detectors/ngfc.py b/mmdet3d/models/detectors/ngfc.py
new file mode 100644
index 0000000..7bf87a9
--- /dev/null
+++ b/mmdet3d/models/detectors/ngfc.py
@@ -0,0 +1,134 @@
+# Copyright (c) OpenMMLab. All rights reserved.
+# Adapted from https://github.com/SamsungLabs/fcaf3d/blob/master/mmdet3d/models/detectors/single_stage_sparse.py # noqa
+try:
+ import MinkowskiEngine as ME
+except ImportError:
+ import warnings
+ warnings.warn(
+ 'Please follow `getting_started.md` to install MinkowskiEngine.`')
+
+from mmdet3d.core import bbox3d2result
+from mmdet3d.models import DETECTORS, build_backbone, build_neck, build_head
+from .base import Base3DDetector
+
+
+@DETECTORS.register_module()
+class Ngfc3DDetector(Base3DDetector):
+ r"""Single stage detector based on MinkowskiEngine `GSDN
+ `_.
+
+ Args:
+ backbone (dict): Config of the backbone.
+ neck (dict): Config of the neck.
+ offset_head (dict): Config of the offset head.
+ tiny_backbone (dict): Config of the tine backbone.
+ tiny_neck (dict): Config of the tine neck.
+ head (dict): Config of the head.
+ voxel_size (float): Voxel size in meters.
+ train_cfg (dict, optional): Config for train stage. Defaults to None.
+ test_cfg (dict, optional): Config for test stage. Defaults to None.
+ init_cfg (dict, optional): Config for weight initialization.
+ Defaults to None.
+ pretrained (str, optional): Deprecated initialization parameter.
+ Defaults to None.
+ """
+
+ def __init__(self,
+ backbone,
+ neck,
+ offset_head,
+ tiny_backbone,
+ tiny_neck,
+ head,
+ voxel_size,
+ train_cfg=None,
+ test_cfg=None,
+ init_cfg=None,
+ pretrained=None):
+ super(Ngfc3DDetector, self).__init__(init_cfg)
+ self.backbone = build_backbone(backbone)
+ self.neck = build_neck(neck)
+ self.offset_head = build_head(offset_head)
+ self.tiny_backbone = build_backbone(tiny_backbone)
+ self.tiny_neck = build_neck(tiny_neck)
+ head.update(train_cfg=train_cfg)
+ head.update(test_cfg=test_cfg)
+ self.head = build_head(head)
+ self.voxel_size = voxel_size
+ self.init_weights()
+
+ def extract_feat(self, *args):
+ """Just implement @abstractmethod of BaseModule."""
+
+ def extract_feats(self, points):
+ """Extract features from points.
+
+ Args:
+ points (list[Tensor]): Raw point clouds.
+
+ Returns:
+ SparseTensor: Voxelized point clouds.
+ """
+ coordinates, features = ME.utils.batch_sparse_collate(
+ [(p[:, :3] / self.voxel_size, p[:, 3:]) for p in points],
+ device=points[0].device)
+ x = ME.SparseTensor(coordinates=coordinates, features=features)
+ x = self.backbone(x)
+ x = self.neck(x)
+ return x
+
+ def forward_train(self, points, gt_bboxes_3d, gt_labels_3d, img_metas):
+ """Forward of training.
+
+ Args:
+ points (list[Tensor]): Raw point clouds.
+ gt_bboxes (list[BaseInstance3DBoxes]): Ground truth
+ bboxes of each sample.
+ gt_labels(list[torch.Tensor]): Labels of each sample.
+ img_metas (list[dict]): Contains scene meta infos.
+
+ Returns:
+ dict: Centerness, bbox and classification loss values.
+ """
+ x = self.extract_feats(points)
+ x, offset_losses = self.offset_head.forward_train(
+ x, gt_bboxes_3d, gt_labels_3d, img_metas)
+ x = self.tiny_backbone(x)
+ x = self.tiny_neck(x)
+ losses = self.head.forward_train(x, gt_bboxes_3d, gt_labels_3d,
+ img_metas)
+ losses.update(offset_losses)
+ return losses
+
+ def simple_test(self, points, img_metas, *args, **kwargs):
+ """Test without augmentations.
+
+ Args:
+ points (list[torch.Tensor]): Points of each sample.
+ img_metas (list[dict]): Contains scene meta infos.
+
+ Returns:
+ list[dict]: Predicted 3d boxes.
+ """
+ x = self.extract_feats(points)
+ x = self.offset_head.forward_test(x, img_metas)
+ x = self.tiny_backbone(x)
+ x = self.tiny_neck(x)
+ bbox_list = self.head.forward_test(x, img_metas)
+ bbox_results = [
+ bbox3d2result(bboxes, scores, labels)
+ for bboxes, scores, labels in bbox_list
+ ]
+ return bbox_results
+
+ def aug_test(self, points, img_metas, **kwargs):
+ """Test with augmentations.
+
+ Args:
+ points (list[list[torch.Tensor]]): Points of each sample.
+ img_metas (list[dict]): Contains scene meta infos.
+
+ Returns:
+ list[dict]: Predicted 3d boxes.
+ """
+ raise NotImplementedError
diff --git a/mmdet3d/models/detectors/ngfc_v2.py b/mmdet3d/models/detectors/ngfc_v2.py
new file mode 100644
index 0000000..b3a20c0
--- /dev/null
+++ b/mmdet3d/models/detectors/ngfc_v2.py
@@ -0,0 +1,120 @@
+# Copyright (c) OpenMMLab. All rights reserved.
+# Adapted from https://github.com/SamsungLabs/fcaf3d/blob/master/mmdet3d/models/detectors/single_stage_sparse.py # noqa
+try:
+ import MinkowskiEngine as ME
+except ImportError:
+ import warnings
+ warnings.warn(
+ 'Please follow `getting_started.md` to install MinkowskiEngine.`')
+
+from mmdet3d.core import bbox3d2result
+from mmdet3d.models import DETECTORS, build_backbone, build_neck, build_head
+from .base import Base3DDetector
+
+
+@DETECTORS.register_module()
+class NgfcV23DDetector(Base3DDetector):
+ r"""Single stage detector based on MinkowskiEngine `GSDN
+ `_.
+
+ Args:
+ backbone (dict): Config of the backbone.
+ neck (dict): Config of the neck.
+ offset_head (dict): Config of the offset head.
+ tiny_backbone (dict): Config of the tine backbone.
+ tiny_neck (dict): Config of the tine neck.
+ head (dict): Config of the head.
+ voxel_size (float): Voxel size in meters.
+ train_cfg (dict, optional): Config for train stage. Defaults to None.
+ test_cfg (dict, optional): Config for test stage. Defaults to None.
+ init_cfg (dict, optional): Config for weight initialization.
+ Defaults to None.
+ pretrained (str, optional): Deprecated initialization parameter.
+ Defaults to None.
+ """
+
+ def __init__(self,
+ backbone,
+ neck,
+ head,
+ voxel_size,
+ train_cfg=None,
+ test_cfg=None,
+ init_cfg=None,
+ pretrained=None):
+ super(NgfcV23DDetector, self).__init__(init_cfg)
+ self.backbone = build_backbone(backbone)
+ self.neck = build_neck(neck)
+ head.update(train_cfg=train_cfg)
+ head.update(test_cfg=test_cfg)
+ self.head = build_head(head)
+ self.voxel_size = voxel_size
+ self.init_weights()
+
+ def extract_feat(self, *args):
+ """Just implement @abstractmethod of BaseModule."""
+
+ def extract_feats(self, points):
+ """Extract features from points.
+
+ Args:
+ points (list[Tensor]): Raw point clouds.
+
+ Returns:
+ SparseTensor: Voxelized point clouds.
+ """
+ coordinates, features = ME.utils.batch_sparse_collate(
+ [(p[:, :3] / self.voxel_size, p[:, 3:]) for p in points],
+ device=points[0].device)
+ x = ME.SparseTensor(coordinates=coordinates, features=features)
+ x = self.backbone(x)
+ x = self.neck(x)
+ return x
+
+ def forward_train(self, points, gt_bboxes_3d, gt_labels_3d, img_metas):
+ """Forward of training.
+
+ Args:
+ points (list[Tensor]): Raw point clouds.
+ gt_bboxes (list[BaseInstance3DBoxes]): Ground truth
+ bboxes of each sample.
+ gt_labels(list[torch.Tensor]): Labels of each sample.
+ img_metas (list[dict]): Contains scene meta infos.
+
+ Returns:
+ dict: Centerness, bbox and classification loss values.
+ """
+ x = self.extract_feats(points)
+ losses = self.head.forward_train(x, gt_bboxes_3d, gt_labels_3d,
+ img_metas)
+ return losses
+
+ def simple_test(self, points, img_metas, *args, **kwargs):
+ """Test without augmentations.
+
+ Args:
+ points (list[torch.Tensor]): Points of each sample.
+ img_metas (list[dict]): Contains scene meta infos.
+
+ Returns:
+ list[dict]: Predicted 3d boxes.
+ """
+ x = self.extract_feats(points)
+ bbox_list = self.head.forward_test(x, img_metas)
+ bbox_results = [
+ bbox3d2result(bboxes, scores, labels)
+ for bboxes, scores, labels in bbox_list
+ ]
+ return bbox_results
+
+ def aug_test(self, points, img_metas, **kwargs):
+ """Test with augmentations.
+
+ Args:
+ points (list[list[torch.Tensor]]): Points of each sample.
+ img_metas (list[dict]): Contains scene meta infos.
+
+ Returns:
+ list[dict]: Predicted 3d boxes.
+ """
+ raise NotImplementedError
diff --git a/mmdet3d/models/detectors/parta2.py b/mmdet3d/models/detectors/parta2.py
new file mode 100644
index 0000000..459a915
--- /dev/null
+++ b/mmdet3d/models/detectors/parta2.py
@@ -0,0 +1,151 @@
+# Copyright (c) OpenMMLab. All rights reserved.
+import torch
+from mmcv.ops import Voxelization
+from torch.nn import functional as F
+
+from .. import builder
+from ..builder import DETECTORS
+from .two_stage import TwoStage3DDetector
+
+
+@DETECTORS.register_module()
+class PartA2(TwoStage3DDetector):
+ r"""Part-A2 detector.
+
+ Please refer to the `paper `_
+ """
+
+ def __init__(self,
+ voxel_layer,
+ voxel_encoder,
+ middle_encoder,
+ backbone,
+ neck=None,
+ rpn_head=None,
+ roi_head=None,
+ train_cfg=None,
+ test_cfg=None,
+ pretrained=None,
+ init_cfg=None):
+ super(PartA2, self).__init__(
+ backbone=backbone,
+ neck=neck,
+ rpn_head=rpn_head,
+ roi_head=roi_head,
+ train_cfg=train_cfg,
+ test_cfg=test_cfg,
+ pretrained=pretrained,
+ init_cfg=init_cfg)
+ self.voxel_layer = Voxelization(**voxel_layer)
+ self.voxel_encoder = builder.build_voxel_encoder(voxel_encoder)
+ self.middle_encoder = builder.build_middle_encoder(middle_encoder)
+
+ def extract_feat(self, points, img_metas):
+ """Extract features from points."""
+ voxel_dict = self.voxelize(points)
+ voxel_features = self.voxel_encoder(voxel_dict['voxels'],
+ voxel_dict['num_points'],
+ voxel_dict['coors'])
+ batch_size = voxel_dict['coors'][-1, 0].item() + 1
+ feats_dict = self.middle_encoder(voxel_features, voxel_dict['coors'],
+ batch_size)
+ x = self.backbone(feats_dict['spatial_features'])
+ if self.with_neck:
+ neck_feats = self.neck(x)
+ feats_dict.update({'neck_feats': neck_feats})
+ return feats_dict, voxel_dict
+
+ @torch.no_grad()
+ def voxelize(self, points):
+ """Apply hard voxelization to points."""
+ voxels, coors, num_points, voxel_centers = [], [], [], []
+ for res in points:
+ res_voxels, res_coors, res_num_points = self.voxel_layer(res)
+ res_voxel_centers = (
+ res_coors[:, [2, 1, 0]] + 0.5) * res_voxels.new_tensor(
+ self.voxel_layer.voxel_size) + res_voxels.new_tensor(
+ self.voxel_layer.point_cloud_range[0:3])
+ voxels.append(res_voxels)
+ coors.append(res_coors)
+ num_points.append(res_num_points)
+ voxel_centers.append(res_voxel_centers)
+
+ voxels = torch.cat(voxels, dim=0)
+ num_points = torch.cat(num_points, dim=0)
+ voxel_centers = torch.cat(voxel_centers, dim=0)
+ coors_batch = []
+ for i, coor in enumerate(coors):
+ coor_pad = F.pad(coor, (1, 0), mode='constant', value=i)
+ coors_batch.append(coor_pad)
+ coors_batch = torch.cat(coors_batch, dim=0)
+
+ voxel_dict = dict(
+ voxels=voxels,
+ num_points=num_points,
+ coors=coors_batch,
+ voxel_centers=voxel_centers)
+ return voxel_dict
+
+ def forward_train(self,
+ points,
+ img_metas,
+ gt_bboxes_3d,
+ gt_labels_3d,
+ gt_bboxes_ignore=None,
+ proposals=None):
+ """Training forward function.
+
+ Args:
+ points (list[torch.Tensor]): Point cloud of each sample.
+ img_metas (list[dict]): Meta information of each sample
+ gt_bboxes_3d (list[:obj:`BaseInstance3DBoxes`]): Ground truth
+ boxes for each sample.
+ gt_labels_3d (list[torch.Tensor]): Ground truth labels for
+ boxes of each sampole
+ gt_bboxes_ignore (list[torch.Tensor], optional): Ground truth
+ boxes to be ignored. Defaults to None.
+
+ Returns:
+ dict: Losses of each branch.
+ """
+ feats_dict, voxels_dict = self.extract_feat(points, img_metas)
+
+ losses = dict()
+
+ if self.with_rpn:
+ rpn_outs = self.rpn_head(feats_dict['neck_feats'])
+ rpn_loss_inputs = rpn_outs + (gt_bboxes_3d, gt_labels_3d,
+ img_metas)
+ rpn_losses = self.rpn_head.loss(
+ *rpn_loss_inputs, gt_bboxes_ignore=gt_bboxes_ignore)
+ losses.update(rpn_losses)
+
+ proposal_cfg = self.train_cfg.get('rpn_proposal',
+ self.test_cfg.rpn)
+ proposal_inputs = rpn_outs + (img_metas, proposal_cfg)
+ proposal_list = self.rpn_head.get_bboxes(*proposal_inputs)
+ else:
+ proposal_list = proposals
+
+ roi_losses = self.roi_head.forward_train(feats_dict, voxels_dict,
+ img_metas, proposal_list,
+ gt_bboxes_3d, gt_labels_3d)
+
+ losses.update(roi_losses)
+
+ return losses
+
+ def simple_test(self, points, img_metas, proposals=None, rescale=False):
+ """Test function without augmentaiton."""
+ feats_dict, voxels_dict = self.extract_feat(points, img_metas)
+
+ if self.with_rpn:
+ rpn_outs = self.rpn_head(feats_dict['neck_feats'])
+ proposal_cfg = self.test_cfg.rpn
+ bbox_inputs = rpn_outs + (img_metas, proposal_cfg)
+ proposal_list = self.rpn_head.get_bboxes(*bbox_inputs)
+ else:
+ proposal_list = proposals
+
+ return self.roi_head.simple_test(feats_dict, voxels_dict, img_metas,
+ proposal_list)
diff --git a/mmdet3d/models/detectors/point_rcnn.py b/mmdet3d/models/detectors/point_rcnn.py
new file mode 100644
index 0000000..31c8693
--- /dev/null
+++ b/mmdet3d/models/detectors/point_rcnn.py
@@ -0,0 +1,148 @@
+# Copyright (c) OpenMMLab. All rights reserved.
+import torch
+
+from ..builder import DETECTORS
+from .two_stage import TwoStage3DDetector
+
+
+@DETECTORS.register_module()
+class PointRCNN(TwoStage3DDetector):
+ r"""PointRCNN detector.
+
+ Please refer to the `PointRCNN `_
+
+ Args:
+ backbone (dict): Config dict of detector's backbone.
+ neck (dict, optional): Config dict of neck. Defaults to None.
+ rpn_head (dict, optional): Config of RPN head. Defaults to None.
+ roi_head (dict, optional): Config of ROI head. Defaults to None.
+ train_cfg (dict, optional): Train configs. Defaults to None.
+ test_cfg (dict, optional): Test configs. Defaults to None.
+ pretrained (str, optional): Model pretrained path. Defaults to None.
+ init_cfg (dict, optional): Config of initialization. Defaults to None.
+ """
+
+ def __init__(self,
+ backbone,
+ neck=None,
+ rpn_head=None,
+ roi_head=None,
+ train_cfg=None,
+ test_cfg=None,
+ pretrained=None,
+ init_cfg=None):
+ super(PointRCNN, self).__init__(
+ backbone=backbone,
+ neck=neck,
+ rpn_head=rpn_head,
+ roi_head=roi_head,
+ train_cfg=train_cfg,
+ test_cfg=test_cfg,
+ pretrained=pretrained,
+ init_cfg=init_cfg)
+
+ def extract_feat(self, points):
+ """Directly extract features from the backbone+neck.
+
+ Args:
+ points (torch.Tensor): Input points.
+
+ Returns:
+ dict: Features from the backbone+neck
+ """
+ x = self.backbone(points)
+
+ if self.with_neck:
+ x = self.neck(x)
+ return x
+
+ def forward_train(self, points, img_metas, gt_bboxes_3d, gt_labels_3d):
+ """Forward of training.
+
+ Args:
+ points (list[torch.Tensor]): Points of each batch.
+ img_metas (list[dict]): Meta information of each sample.
+ gt_bboxes_3d (:obj:`BaseInstance3DBoxes`): gt bboxes of each batch.
+ gt_labels_3d (list[torch.Tensor]): gt class labels of each batch.
+
+ Returns:
+ dict: Losses.
+ """
+ losses = dict()
+ points_cat = torch.stack(points)
+ x = self.extract_feat(points_cat)
+
+ # features for rcnn
+ backbone_feats = x['fp_features'].clone()
+ backbone_xyz = x['fp_xyz'].clone()
+ rcnn_feats = {'features': backbone_feats, 'points': backbone_xyz}
+
+ bbox_preds, cls_preds = self.rpn_head(x)
+
+ rpn_loss = self.rpn_head.loss(
+ bbox_preds=bbox_preds,
+ cls_preds=cls_preds,
+ points=points,
+ gt_bboxes_3d=gt_bboxes_3d,
+ gt_labels_3d=gt_labels_3d,
+ img_metas=img_metas)
+ losses.update(rpn_loss)
+
+ bbox_list = self.rpn_head.get_bboxes(points_cat, bbox_preds, cls_preds,
+ img_metas)
+ proposal_list = [
+ dict(
+ boxes_3d=bboxes,
+ scores_3d=scores,
+ labels_3d=labels,
+ cls_preds=preds_cls)
+ for bboxes, scores, labels, preds_cls in bbox_list
+ ]
+ rcnn_feats.update({'points_cls_preds': cls_preds})
+
+ roi_losses = self.roi_head.forward_train(rcnn_feats, img_metas,
+ proposal_list, gt_bboxes_3d,
+ gt_labels_3d)
+ losses.update(roi_losses)
+
+ return losses
+
+ def simple_test(self, points, img_metas, imgs=None, rescale=False):
+ """Forward of testing.
+
+ Args:
+ points (list[torch.Tensor]): Points of each sample.
+ img_metas (list[dict]): Image metas.
+ imgs (list[torch.Tensor], optional): Images of each sample.
+ Defaults to None.
+ rescale (bool, optional): Whether to rescale results.
+ Defaults to False.
+
+ Returns:
+ list: Predicted 3d boxes.
+ """
+ points_cat = torch.stack(points)
+
+ x = self.extract_feat(points_cat)
+ # features for rcnn
+ backbone_feats = x['fp_features'].clone()
+ backbone_xyz = x['fp_xyz'].clone()
+ rcnn_feats = {'features': backbone_feats, 'points': backbone_xyz}
+ bbox_preds, cls_preds = self.rpn_head(x)
+ rcnn_feats.update({'points_cls_preds': cls_preds})
+
+ bbox_list = self.rpn_head.get_bboxes(
+ points_cat, bbox_preds, cls_preds, img_metas, rescale=rescale)
+
+ proposal_list = [
+ dict(
+ boxes_3d=bboxes,
+ scores_3d=scores,
+ labels_3d=labels,
+ cls_preds=preds_cls)
+ for bboxes, scores, labels, preds_cls in bbox_list
+ ]
+ bbox_results = self.roi_head.simple_test(rcnn_feats, img_metas,
+ proposal_list)
+
+ return bbox_results
diff --git a/mmdet3d/models/detectors/sassd.py b/mmdet3d/models/detectors/sassd.py
new file mode 100644
index 0000000..2151c4e
--- /dev/null
+++ b/mmdet3d/models/detectors/sassd.py
@@ -0,0 +1,136 @@
+# Copyright (c) OpenMMLab. All rights reserved.
+import torch
+from mmcv.ops import Voxelization
+from mmcv.runner import force_fp32
+from torch.nn import functional as F
+
+from mmdet3d.core import bbox3d2result, merge_aug_bboxes_3d
+from mmdet.models.builder import DETECTORS
+from .. import builder
+from .single_stage import SingleStage3DDetector
+
+
+@DETECTORS.register_module()
+class SASSD(SingleStage3DDetector):
+ r"""`SASSD ` _ for 3D detection."""
+
+ def __init__(self,
+ voxel_layer,
+ voxel_encoder,
+ middle_encoder,
+ backbone,
+ neck=None,
+ bbox_head=None,
+ train_cfg=None,
+ test_cfg=None,
+ init_cfg=None,
+ pretrained=None):
+ super(SASSD, self).__init__(
+ backbone=backbone,
+ neck=neck,
+ bbox_head=bbox_head,
+ train_cfg=train_cfg,
+ test_cfg=test_cfg,
+ init_cfg=init_cfg,
+ pretrained=pretrained)
+
+ self.voxel_layer = Voxelization(**voxel_layer)
+ self.voxel_encoder = builder.build_voxel_encoder(voxel_encoder)
+ self.middle_encoder = builder.build_middle_encoder(middle_encoder)
+
+ def extract_feat(self, points, img_metas=None, test_mode=False):
+ """Extract features from points."""
+ voxels, num_points, coors = self.voxelize(points)
+ voxel_features = self.voxel_encoder(voxels, num_points, coors)
+ batch_size = coors[-1, 0].item() + 1
+ x, point_misc = self.middle_encoder(voxel_features, coors, batch_size,
+ test_mode)
+ x = self.backbone(x)
+ if self.with_neck:
+ x = self.neck(x)
+ return x, point_misc
+
+ @torch.no_grad()
+ @force_fp32()
+ def voxelize(self, points):
+ """Apply hard voxelization to points."""
+ voxels, coors, num_points = [], [], []
+ for res in points:
+ res_voxels, res_coors, res_num_points = self.voxel_layer(res)
+ voxels.append(res_voxels)
+ coors.append(res_coors)
+ num_points.append(res_num_points)
+ voxels = torch.cat(voxels, dim=0)
+ num_points = torch.cat(num_points, dim=0)
+ coors_batch = []
+ for i, coor in enumerate(coors):
+ coor_pad = F.pad(coor, (1, 0), mode='constant', value=i)
+ coors_batch.append(coor_pad)
+ coors_batch = torch.cat(coors_batch, dim=0)
+ return voxels, num_points, coors_batch
+
+ def forward_train(self,
+ points,
+ img_metas,
+ gt_bboxes_3d,
+ gt_labels_3d,
+ gt_bboxes_ignore=None):
+ """Training forward function.
+
+ Args:
+ points (list[torch.Tensor]): Point cloud of each sample.
+ img_metas (list[dict]): Meta information of each sample
+ gt_bboxes_3d (list[:obj:`BaseInstance3DBoxes`]): Ground truth
+ boxes for each sample.
+ gt_labels_3d (list[torch.Tensor]): Ground truth labels for
+ boxes of each sampole
+ gt_bboxes_ignore (list[torch.Tensor], optional): Ground truth
+ boxes to be ignored. Defaults to None.
+
+ Returns:
+ dict: Losses of each branch.
+ """
+
+ x, point_misc = self.extract_feat(points, img_metas, test_mode=False)
+ aux_loss = self.middle_encoder.aux_loss(*point_misc, gt_bboxes_3d)
+
+ outs = self.bbox_head(x)
+ loss_inputs = outs + (gt_bboxes_3d, gt_labels_3d, img_metas)
+ losses = self.bbox_head.loss(
+ *loss_inputs, gt_bboxes_ignore=gt_bboxes_ignore)
+ losses.update(aux_loss)
+ return losses
+
+ def simple_test(self, points, img_metas, imgs=None, rescale=False):
+ """Test function without augmentaiton."""
+ x, _ = self.extract_feat(points, img_metas, test_mode=True)
+ outs = self.bbox_head(x)
+ bbox_list = self.bbox_head.get_bboxes(
+ *outs, img_metas, rescale=rescale)
+ bbox_results = [
+ bbox3d2result(bboxes, scores, labels)
+ for bboxes, scores, labels in bbox_list
+ ]
+ return bbox_results
+
+ def aug_test(self, points, img_metas, imgs=None, rescale=False):
+ """Test function with augmentaiton."""
+ feats = self.extract_feats(points, img_metas, test_mode=True)
+
+ # only support aug_test for one sample
+ aug_bboxes = []
+ for x, img_meta in zip(feats, img_metas):
+ outs = self.bbox_head(x)
+ bbox_list = self.bbox_head.get_bboxes(
+ *outs, img_meta, rescale=rescale)
+ bbox_list = [
+ dict(boxes_3d=bboxes, scores_3d=scores, labels_3d=labels)
+ for bboxes, scores, labels in bbox_list
+ ]
+ aug_bboxes.append(bbox_list[0])
+
+ # after merging, bboxes will be rescaled to the original image size
+ merged_bboxes = merge_aug_bboxes_3d(aug_bboxes, img_metas,
+ self.bbox_head.test_cfg)
+
+ return [merged_bboxes]
diff --git a/mmdet3d/models/detectors/single_stage.py b/mmdet3d/models/detectors/single_stage.py
new file mode 100644
index 0000000..11f8479
--- /dev/null
+++ b/mmdet3d/models/detectors/single_stage.py
@@ -0,0 +1,71 @@
+# Copyright (c) OpenMMLab. All rights reserved.
+from ..builder import DETECTORS, build_backbone, build_head, build_neck
+from .base import Base3DDetector
+
+
+@DETECTORS.register_module()
+class SingleStage3DDetector(Base3DDetector):
+ """SingleStage3DDetector.
+
+ This class serves as a base class for single-stage 3D detectors.
+
+ Args:
+ backbone (dict): Config dict of detector's backbone.
+ neck (dict, optional): Config dict of neck. Defaults to None.
+ bbox_head (dict, optional): Config dict of box head. Defaults to None.
+ train_cfg (dict, optional): Config dict of training hyper-parameters.
+ Defaults to None.
+ test_cfg (dict, optional): Config dict of test hyper-parameters.
+ Defaults to None.
+ pretrained (str, optional): Path of pretrained models.
+ Defaults to None.
+ """
+
+ def __init__(self,
+ backbone,
+ neck=None,
+ bbox_head=None,
+ train_cfg=None,
+ test_cfg=None,
+ init_cfg=None,
+ pretrained=None):
+ super(SingleStage3DDetector, self).__init__(init_cfg)
+ self.backbone = build_backbone(backbone)
+ if neck is not None:
+ self.neck = build_neck(neck)
+ bbox_head.update(train_cfg=train_cfg)
+ bbox_head.update(test_cfg=test_cfg)
+ self.bbox_head = build_head(bbox_head)
+ self.train_cfg = train_cfg
+ self.test_cfg = test_cfg
+
+ def forward_dummy(self, points):
+ """Used for computing network flops.
+
+ See `mmdetection/tools/analysis_tools/get_flops.py`
+ """
+ x = self.extract_feat(points)
+ try:
+ sample_mod = self.train_cfg.sample_mod
+ outs = self.bbox_head(x, sample_mod)
+ except AttributeError:
+ outs = self.bbox_head(x)
+ return outs
+
+ def extract_feat(self, points, img_metas=None):
+ """Directly extract features from the backbone+neck.
+
+ Args:
+ points (torch.Tensor): Input points.
+ """
+ x = self.backbone(points)
+ if self.with_neck:
+ x = self.neck(x)
+ return x
+
+ def extract_feats(self, points, img_metas):
+ """Extract features of multiple samples."""
+ return [
+ self.extract_feat(pts, img_meta)
+ for pts, img_meta in zip(points, img_metas)
+ ]
diff --git a/mmdet3d/models/detectors/single_stage_mono3d.py b/mmdet3d/models/detectors/single_stage_mono3d.py
new file mode 100644
index 0000000..464fab0
--- /dev/null
+++ b/mmdet3d/models/detectors/single_stage_mono3d.py
@@ -0,0 +1,250 @@
+# Copyright (c) OpenMMLab. All rights reserved.
+import warnings
+from os import path as osp
+
+import mmcv
+import numpy as np
+import torch
+from mmcv.parallel import DataContainer as DC
+
+from mmdet3d.core import (CameraInstance3DBoxes, bbox3d2result,
+ show_multi_modality_result)
+from mmdet.models.detectors import SingleStageDetector
+from ..builder import DETECTORS, build_backbone, build_head, build_neck
+
+
+@DETECTORS.register_module()
+class SingleStageMono3DDetector(SingleStageDetector):
+ """Base class for monocular 3D single-stage detectors.
+
+ Single-stage detectors directly and densely predict bounding boxes on the
+ output features of the backbone+neck.
+ """
+
+ def __init__(self,
+ backbone,
+ neck=None,
+ bbox_head=None,
+ train_cfg=None,
+ test_cfg=None,
+ pretrained=None,
+ init_cfg=None):
+ super(SingleStageDetector, self).__init__(init_cfg)
+ if pretrained:
+ warnings.warn('DeprecationWarning: pretrained is deprecated, '
+ 'please use "init_cfg" instead')
+ backbone.pretrained = pretrained
+ self.backbone = build_backbone(backbone)
+ if neck is not None:
+ self.neck = build_neck(neck)
+ bbox_head.update(train_cfg=train_cfg)
+ bbox_head.update(test_cfg=test_cfg)
+ self.bbox_head = build_head(bbox_head)
+ self.train_cfg = train_cfg
+ self.test_cfg = test_cfg
+
+ def extract_feats(self, imgs):
+ """Directly extract features from the backbone+neck."""
+ assert isinstance(imgs, list)
+ return [self.extract_feat(img) for img in imgs]
+
+ def forward_train(self,
+ img,
+ img_metas,
+ gt_bboxes,
+ gt_labels,
+ gt_bboxes_3d,
+ gt_labels_3d,
+ centers2d,
+ depths,
+ attr_labels=None,
+ gt_bboxes_ignore=None):
+ """
+ Args:
+ img (Tensor): Input images of shape (N, C, H, W).
+ Typically these should be mean centered and std scaled.
+ img_metas (list[dict]): A List of image info dict where each dict
+ has: 'img_shape', 'scale_factor', 'flip', and may also contain
+ 'filename', 'ori_shape', 'pad_shape', and 'img_norm_cfg'.
+ For details on the values of these keys see
+ :class:`mmdet.datasets.pipelines.Collect`.
+ gt_bboxes (list[Tensor]): Each item are the truth boxes for each
+ image in [tl_x, tl_y, br_x, br_y] format.
+ gt_labels (list[Tensor]): Class indices corresponding to each box
+ gt_bboxes_3d (list[Tensor]): Each item are the 3D truth boxes for
+ each image in [x, y, z, x_size, y_size, z_size, yaw, vx, vy]
+ format.
+ gt_labels_3d (list[Tensor]): 3D class indices corresponding to
+ each box.
+ centers2d (list[Tensor]): Projected 3D centers onto 2D images.
+ depths (list[Tensor]): Depth of projected centers on 2D images.
+ attr_labels (list[Tensor], optional): Attribute indices
+ corresponding to each box
+ gt_bboxes_ignore (list[Tensor]): Specify which bounding
+ boxes can be ignored when computing the loss.
+
+ Returns:
+ dict[str, Tensor]: A dictionary of loss components.
+ """
+ x = self.extract_feat(img)
+ losses = self.bbox_head.forward_train(x, img_metas, gt_bboxes,
+ gt_labels, gt_bboxes_3d,
+ gt_labels_3d, centers2d, depths,
+ attr_labels, gt_bboxes_ignore)
+ return losses
+
+ def simple_test(self, img, img_metas, rescale=False):
+ """Test function without test time augmentation.
+
+ Args:
+ imgs (list[torch.Tensor]): List of multiple images
+ img_metas (list[dict]): List of image information.
+ rescale (bool, optional): Whether to rescale the results.
+ Defaults to False.
+
+ Returns:
+ list[list[np.ndarray]]: BBox results of each image and classes.
+ The outer list corresponds to each image. The inner list
+ corresponds to each class.
+ """
+ x = self.extract_feat(img)
+ outs = self.bbox_head(x)
+ bbox_outputs = self.bbox_head.get_bboxes(
+ *outs, img_metas, rescale=rescale)
+
+ if self.bbox_head.pred_bbox2d:
+ from mmdet.core import bbox2result
+ bbox2d_img = [
+ bbox2result(bboxes2d, labels, self.bbox_head.num_classes)
+ for bboxes, scores, labels, attrs, bboxes2d in bbox_outputs
+ ]
+ bbox_outputs = [bbox_outputs[0][:-1]]
+
+ bbox_img = [
+ bbox3d2result(bboxes, scores, labels, attrs)
+ for bboxes, scores, labels, attrs in bbox_outputs
+ ]
+
+ bbox_list = [dict() for i in range(len(img_metas))]
+ for result_dict, img_bbox in zip(bbox_list, bbox_img):
+ result_dict['img_bbox'] = img_bbox
+ if self.bbox_head.pred_bbox2d:
+ for result_dict, img_bbox2d in zip(bbox_list, bbox2d_img):
+ result_dict['img_bbox2d'] = img_bbox2d
+ return bbox_list
+
+ def aug_test(self, imgs, img_metas, rescale=False):
+ """Test function with test time augmentation."""
+ feats = self.extract_feats(imgs)
+
+ # only support aug_test for one sample
+ outs_list = [self.bbox_head(x) for x in feats]
+ for i, img_meta in enumerate(img_metas):
+ if img_meta[0]['pcd_horizontal_flip']:
+ for j in range(len(outs_list[i])): # for each prediction
+ if outs_list[i][j][0] is None:
+ continue
+ for k in range(len(outs_list[i][j])):
+ # every stride of featmap
+ outs_list[i][j][k] = torch.flip(
+ outs_list[i][j][k], dims=[3])
+ reg = outs_list[i][1]
+ for reg_feat in reg:
+ # offset_x
+ reg_feat[:, 0, :, :] = 1 - reg_feat[:, 0, :, :]
+ # velo_x
+ if self.bbox_head.pred_velo:
+ reg_feat[:, 7, :, :] = -reg_feat[:, 7, :, :]
+ # rotation
+ reg_feat[:, 6, :, :] = -reg_feat[:, 6, :, :] + np.pi
+
+ merged_outs = []
+ for i in range(len(outs_list[0])): # for each prediction
+ merged_feats = []
+ for j in range(len(outs_list[0][i])):
+ if outs_list[0][i][0] is None:
+ merged_feats.append(None)
+ continue
+ # for each stride of featmap
+ avg_feats = torch.mean(
+ torch.cat([x[i][j] for x in outs_list]),
+ dim=0,
+ keepdim=True)
+ if i == 1: # regression predictions
+ # rot/velo/2d det keeps the original
+ avg_feats[:, 6:, :, :] = \
+ outs_list[0][i][j][:, 6:, :, :]
+ if i == 2:
+ # dir_cls keeps the original
+ avg_feats = outs_list[0][i][j]
+ merged_feats.append(avg_feats)
+ merged_outs.append(merged_feats)
+ merged_outs = tuple(merged_outs)
+
+ bbox_outputs = self.bbox_head.get_bboxes(
+ *merged_outs, img_metas[0], rescale=rescale)
+ if self.bbox_head.pred_bbox2d:
+ from mmdet.core import bbox2result
+ bbox2d_img = [
+ bbox2result(bboxes2d, labels, self.bbox_head.num_classes)
+ for bboxes, scores, labels, attrs, bboxes2d in bbox_outputs
+ ]
+ bbox_outputs = [bbox_outputs[0][:-1]]
+
+ bbox_img = [
+ bbox3d2result(bboxes, scores, labels, attrs)
+ for bboxes, scores, labels, attrs in bbox_outputs
+ ]
+
+ bbox_list = dict()
+ bbox_list.update(img_bbox=bbox_img[0])
+ if self.bbox_head.pred_bbox2d:
+ bbox_list.update(img_bbox2d=bbox2d_img[0])
+
+ return [bbox_list]
+
+ def show_results(self, data, result, out_dir, show=False, score_thr=None):
+ """Results visualization.
+
+ Args:
+ data (list[dict]): Input images and the information of the sample.
+ result (list[dict]): Prediction results.
+ out_dir (str): Output directory of visualization result.
+ show (bool, optional): Determines whether you are
+ going to show result by open3d.
+ Defaults to False.
+ TODO: implement score_thr of single_stage_mono3d.
+ score_thr (float, optional): Score threshold of bounding boxes.
+ Default to None.
+ Not implemented yet, but it is here for unification.
+ """
+ for batch_id in range(len(result)):
+ if isinstance(data['img_metas'][0], DC):
+ img_filename = data['img_metas'][0]._data[0][batch_id][
+ 'filename']
+ cam2img = data['img_metas'][0]._data[0][batch_id]['cam2img']
+ elif mmcv.is_list_of(data['img_metas'][0], dict):
+ img_filename = data['img_metas'][0][batch_id]['filename']
+ cam2img = data['img_metas'][0][batch_id]['cam2img']
+ else:
+ ValueError(
+ f"Unsupported data type {type(data['img_metas'][0])} "
+ f'for visualization!')
+ img = mmcv.imread(img_filename)
+ file_name = osp.split(img_filename)[-1].split('.')[0]
+
+ assert out_dir is not None, 'Expect out_dir, got none.'
+
+ pred_bboxes = result[batch_id]['img_bbox']['boxes_3d']
+ assert isinstance(pred_bboxes, CameraInstance3DBoxes), \
+ f'unsupported predicted bbox type {type(pred_bboxes)}'
+
+ show_multi_modality_result(
+ img,
+ None,
+ pred_bboxes,
+ cam2img,
+ out_dir,
+ file_name,
+ 'camera',
+ show=show)
diff --git a/mmdet3d/models/detectors/smoke_mono3d.py b/mmdet3d/models/detectors/smoke_mono3d.py
new file mode 100644
index 0000000..241187f
--- /dev/null
+++ b/mmdet3d/models/detectors/smoke_mono3d.py
@@ -0,0 +1,21 @@
+# Copyright (c) OpenMMLab. All rights reserved.
+from ..builder import DETECTORS
+from .single_stage_mono3d import SingleStageMono3DDetector
+
+
+@DETECTORS.register_module()
+class SMOKEMono3D(SingleStageMono3DDetector):
+ r"""SMOKE `_ for monocular 3D object
+ detection.
+
+ """
+
+ def __init__(self,
+ backbone,
+ neck,
+ bbox_head,
+ train_cfg=None,
+ test_cfg=None,
+ pretrained=None):
+ super(SMOKEMono3D, self).__init__(backbone, neck, bbox_head, train_cfg,
+ test_cfg, pretrained)
diff --git a/mmdet3d/models/detectors/ssd3dnet.py b/mmdet3d/models/detectors/ssd3dnet.py
new file mode 100644
index 0000000..fd5e310
--- /dev/null
+++ b/mmdet3d/models/detectors/ssd3dnet.py
@@ -0,0 +1,26 @@
+# Copyright (c) OpenMMLab. All rights reserved.
+from ..builder import DETECTORS
+from .votenet import VoteNet
+
+
+@DETECTORS.register_module()
+class SSD3DNet(VoteNet):
+ """3DSSDNet model.
+
+ https://arxiv.org/abs/2002.10187.pdf
+ """
+
+ def __init__(self,
+ backbone,
+ bbox_head=None,
+ train_cfg=None,
+ test_cfg=None,
+ init_cfg=None,
+ pretrained=None):
+ super(SSD3DNet, self).__init__(
+ backbone=backbone,
+ bbox_head=bbox_head,
+ train_cfg=train_cfg,
+ test_cfg=test_cfg,
+ init_cfg=init_cfg,
+ pretrained=pretrained)
diff --git a/mmdet3d/models/detectors/td3d_instance_segmentor.py b/mmdet3d/models/detectors/td3d_instance_segmentor.py
new file mode 100644
index 0000000..02a4eb1
--- /dev/null
+++ b/mmdet3d/models/detectors/td3d_instance_segmentor.py
@@ -0,0 +1,141 @@
+try:
+ import MinkowskiEngine as ME
+except ImportError:
+ import warnings
+ warnings.warn(
+ 'Please follow `getting_started.md` to install MinkowskiEngine.`')
+
+from mmdet3d.models import DETECTORS, build_backbone, build_neck, build_head
+from .base import Base3DDetector
+import torch
+
+@DETECTORS.register_module()
+class TD3DInstanceSegmentor(Base3DDetector):
+ r"""Two-stage instance segmentor based on MinkowskiEngine.
+ The first stage is bbox detector. The second stage is two-class pointwise segmentor (foreground/background).
+
+ Args:
+ backbone (dict): Config of the backbone.
+ neck (dict): Config of the neck.
+ head (dict): Config of the head.
+ voxel_size (float): Voxel size in meters.
+ train_cfg (dict, optional): Config for train stage. Defaults to None.
+ test_cfg (dict, optional): Config for test stage. Defaults to None.
+ init_cfg (dict, optional): Config for weight initialization.
+ Defaults to None.
+ pretrained (str, optional): Deprecated initialization parameter.
+ Defaults to None.
+ """
+ def __init__(self,
+ backbone,
+ neck,
+ head,
+ voxel_size,
+ train_cfg=None,
+ test_cfg=None,
+ init_cfg=None,
+ pretrained=None):
+ super(TD3DInstanceSegmentor, self).__init__(init_cfg)
+ self.backbone = build_backbone(backbone)
+ self.neck = build_neck(neck)
+ head.update(train_cfg=train_cfg)
+ head.update(test_cfg=test_cfg)
+ self.head = build_head(head)
+ self.voxel_size = voxel_size
+ self.init_weights()
+
+ def extract_feat(self, points):
+ """Extract features from points.
+
+ Args:
+ points (list[Tensor]): Raw point clouds.
+
+ Returns:
+ SparseTensor: Voxelized point clouds.
+ """
+ x = self.backbone(points)
+ x = self.neck(x)
+ return x
+
+ def collate(self, points, quantization_mode):
+ coordinates, features = ME.utils.batch_sparse_collate(
+ [(p[:, :3] / self.voxel_size, p[:, 3:]) for p in points],
+ dtype=points[0].dtype,
+ device=points[0].device)
+ return ME.TensorField(
+ features=features,
+ coordinates=coordinates,
+ quantization_mode=quantization_mode,
+ minkowski_algorithm=ME.MinkowskiAlgorithm.SPEED_OPTIMIZED,
+ device=points[0].device,
+ )
+
+ def forward_train(self, points, gt_bboxes_3d, gt_labels_3d,
+ pts_semantic_mask, pts_instance_mask, img_metas):
+ """Forward of training.
+
+ Args:
+ points (list[Tensor]): Raw point clouds.
+ gt_bboxes_3d (list[BaseInstance3DBoxes]): Ground truth
+ bboxes of each sample.
+ gt_labels_3d (list[torch.Tensor]): Labels of each sample.
+ pts_semantic_mask (list[torch.Tensor]): Per point semantic labels
+ of each sample.
+ pts_instance_mask (list[torch.Tensor]): Per point instance labels
+ of each sample.
+ img_metas (list[dict]): Contains scene meta infos.
+
+ Returns:
+ dict: Loss values.
+ """
+
+ # points = [torch.cat([p, torch.unsqueeze(m, 1)], dim=1) for p, m in zip(points, pts_instance_mask)]
+ points = [torch.cat([p, torch.unsqueeze(inst, 1), torch.unsqueeze(sem, 1)], dim=1) for p, inst, sem in zip(points, pts_instance_mask, pts_semantic_mask)]
+ field = self.collate(points, ME.SparseTensorQuantizationMode.RANDOM_SUBSAMPLE)
+ x = field.sparse()
+ targets = x.features[:, 3:].round().long()
+ x = ME.SparseTensor(
+ x.features[:, :3],
+ coordinate_map_key=x.coordinate_map_key,
+ coordinate_manager=x.coordinate_manager,
+ )
+ x = self.extract_feat(x)
+
+ losses = self.head.forward_train(x, targets, field, gt_bboxes_3d, gt_labels_3d,
+ pts_semantic_mask, pts_instance_mask, img_metas)
+ return losses
+
+ def simple_test(self, points, img_metas, *args, **kwargs):
+ """Test without augmentations.
+
+ Args:
+ points (list[torch.Tensor]): Points of each sample.
+ img_metas (list[dict]): Contains scene meta infos.
+
+ Returns:
+ list[dict]: Predicted 3d instances.
+ """
+
+ field = self.collate(points, ME.SparseTensorQuantizationMode.UNWEIGHTED_AVERAGE)
+ x = self.extract_feat(field.sparse())
+
+ instances = self.head.forward_test(x, field, img_metas)
+ results = []
+ for mask, label, score in instances:
+ results.append(dict(
+ instance_mask=mask.cpu(),
+ instance_label=label.cpu(),
+ instance_score=score.cpu()))
+ return results
+
+ def aug_test(self, points, img_metas, **kwargs):
+ """Test with augmentations.
+
+ Args:
+ points (list[list[torch.Tensor]]): Points of each sample.
+ img_metas (list[dict]): Contains scene meta infos.
+
+ Returns:
+ list[dict]: Predicted 3d boxes.
+ """
+ raise NotImplementedError
diff --git a/mmdet3d/models/detectors/two_stage.py b/mmdet3d/models/detectors/two_stage.py
new file mode 100644
index 0000000..707f706
--- /dev/null
+++ b/mmdet3d/models/detectors/two_stage.py
@@ -0,0 +1,51 @@
+# Copyright (c) OpenMMLab. All rights reserved.
+import warnings
+
+from mmdet.models import TwoStageDetector
+from ..builder import DETECTORS, build_backbone, build_head, build_neck
+from .base import Base3DDetector
+
+
+@DETECTORS.register_module()
+class TwoStage3DDetector(Base3DDetector, TwoStageDetector):
+ """Base class of two-stage 3D detector.
+
+ It inherits original ``:class:TwoStageDetector`` and
+ ``:class:Base3DDetector``. This class could serve as a base class for all
+ two-stage 3D detectors.
+ """
+
+ def __init__(self,
+ backbone,
+ neck=None,
+ rpn_head=None,
+ roi_head=None,
+ train_cfg=None,
+ test_cfg=None,
+ pretrained=None,
+ init_cfg=None):
+ super(TwoStageDetector, self).__init__(init_cfg)
+ if pretrained:
+ warnings.warn('DeprecationWarning: pretrained is deprecated, '
+ 'please use "init_cfg" instead')
+ backbone.pretrained = pretrained
+ self.backbone = build_backbone(backbone)
+ self.train_cfg = train_cfg
+ self.test_cfg = test_cfg
+ if neck is not None:
+ self.neck = build_neck(neck)
+
+ if rpn_head is not None:
+ rpn_train_cfg = train_cfg.rpn if train_cfg is not None else None
+ rpn_head_ = rpn_head.copy()
+ rpn_head_.update(train_cfg=rpn_train_cfg, test_cfg=test_cfg.rpn)
+ self.rpn_head = build_head(rpn_head_)
+
+ if roi_head is not None:
+ # update train and test cfg here for now
+ # TODO: refactor assigner & sampler
+ rcnn_train_cfg = train_cfg.rcnn if train_cfg is not None else None
+ roi_head.update(train_cfg=rcnn_train_cfg)
+ roi_head.update(test_cfg=test_cfg.rcnn)
+ roi_head.pretrained = pretrained
+ self.roi_head = build_head(roi_head)
diff --git a/mmdet3d/models/detectors/votenet.py b/mmdet3d/models/detectors/votenet.py
new file mode 100644
index 0000000..41e4144
--- /dev/null
+++ b/mmdet3d/models/detectors/votenet.py
@@ -0,0 +1,107 @@
+# Copyright (c) OpenMMLab. All rights reserved.
+import torch
+
+from mmdet3d.core import bbox3d2result, merge_aug_bboxes_3d
+from ..builder import DETECTORS
+from .single_stage import SingleStage3DDetector
+
+
+@DETECTORS.register_module()
+class VoteNet(SingleStage3DDetector):
+ r"""`VoteNet `_ for 3D detection."""
+
+ def __init__(self,
+ backbone,
+ bbox_head=None,
+ train_cfg=None,
+ test_cfg=None,
+ init_cfg=None,
+ pretrained=None):
+ super(VoteNet, self).__init__(
+ backbone=backbone,
+ bbox_head=bbox_head,
+ train_cfg=train_cfg,
+ test_cfg=test_cfg,
+ init_cfg=None,
+ pretrained=pretrained)
+
+ def forward_train(self,
+ points,
+ img_metas,
+ gt_bboxes_3d,
+ gt_labels_3d,
+ pts_semantic_mask=None,
+ pts_instance_mask=None,
+ gt_bboxes_ignore=None):
+ """Forward of training.
+
+ Args:
+ points (list[torch.Tensor]): Points of each batch.
+ img_metas (list): Image metas.
+ gt_bboxes_3d (:obj:`BaseInstance3DBoxes`): gt bboxes of each batch.
+ gt_labels_3d (list[torch.Tensor]): gt class labels of each batch.
+ pts_semantic_mask (list[torch.Tensor]): point-wise semantic
+ label of each batch.
+ pts_instance_mask (list[torch.Tensor]): point-wise instance
+ label of each batch.
+ gt_bboxes_ignore (list[torch.Tensor]): Specify
+ which bounding.
+
+ Returns:
+ dict: Losses.
+ """
+ points_cat = torch.stack(points)
+
+ x = self.extract_feat(points_cat)
+ bbox_preds = self.bbox_head(x, self.train_cfg.sample_mod)
+ loss_inputs = (points, gt_bboxes_3d, gt_labels_3d, pts_semantic_mask,
+ pts_instance_mask, img_metas)
+ losses = self.bbox_head.loss(
+ bbox_preds, *loss_inputs, gt_bboxes_ignore=gt_bboxes_ignore)
+ return losses
+
+ def simple_test(self, points, img_metas, imgs=None, rescale=False):
+ """Forward of testing.
+
+ Args:
+ points (list[torch.Tensor]): Points of each sample.
+ img_metas (list): Image metas.
+ rescale (bool): Whether to rescale results.
+
+ Returns:
+ list: Predicted 3d boxes.
+ """
+ points_cat = torch.stack(points)
+
+ x = self.extract_feat(points_cat)
+ bbox_preds = self.bbox_head(x, self.test_cfg.sample_mod)
+ bbox_list = self.bbox_head.get_bboxes(
+ points_cat, bbox_preds, img_metas, rescale=rescale)
+ bbox_results = [
+ bbox3d2result(bboxes, scores, labels)
+ for bboxes, scores, labels in bbox_list
+ ]
+ return bbox_results
+
+ def aug_test(self, points, img_metas, imgs=None, rescale=False):
+ """Test with augmentation."""
+ points_cat = [torch.stack(pts) for pts in points]
+ feats = self.extract_feats(points_cat, img_metas)
+
+ # only support aug_test for one sample
+ aug_bboxes = []
+ for x, pts_cat, img_meta in zip(feats, points_cat, img_metas):
+ bbox_preds = self.bbox_head(x, self.test_cfg.sample_mod)
+ bbox_list = self.bbox_head.get_bboxes(
+ pts_cat, bbox_preds, img_meta, rescale=rescale)
+ bbox_list = [
+ dict(boxes_3d=bboxes, scores_3d=scores, labels_3d=labels)
+ for bboxes, scores, labels in bbox_list
+ ]
+ aug_bboxes.append(bbox_list[0])
+
+ # after merging, bboxes will be rescaled to the original image size
+ merged_bboxes = merge_aug_bboxes_3d(aug_bboxes, img_metas,
+ self.bbox_head.test_cfg)
+
+ return [merged_bboxes]
diff --git a/mmdet3d/models/detectors/voxelnet.py b/mmdet3d/models/detectors/voxelnet.py
new file mode 100644
index 0000000..9276b7d
--- /dev/null
+++ b/mmdet3d/models/detectors/voxelnet.py
@@ -0,0 +1,130 @@
+# Copyright (c) OpenMMLab. All rights reserved.
+import torch
+from mmcv.ops import Voxelization
+from mmcv.runner import force_fp32
+from torch.nn import functional as F
+
+from mmdet3d.core import bbox3d2result, merge_aug_bboxes_3d
+from .. import builder
+from ..builder import DETECTORS
+from .single_stage import SingleStage3DDetector
+
+
+@DETECTORS.register_module()
+class VoxelNet(SingleStage3DDetector):
+ r"""`VoxelNet `_ for 3D detection."""
+
+ def __init__(self,
+ voxel_layer,
+ voxel_encoder,
+ middle_encoder,
+ backbone,
+ neck=None,
+ bbox_head=None,
+ train_cfg=None,
+ test_cfg=None,
+ init_cfg=None,
+ pretrained=None):
+ super(VoxelNet, self).__init__(
+ backbone=backbone,
+ neck=neck,
+ bbox_head=bbox_head,
+ train_cfg=train_cfg,
+ test_cfg=test_cfg,
+ init_cfg=init_cfg,
+ pretrained=pretrained)
+ self.voxel_layer = Voxelization(**voxel_layer)
+ self.voxel_encoder = builder.build_voxel_encoder(voxel_encoder)
+ self.middle_encoder = builder.build_middle_encoder(middle_encoder)
+
+ def extract_feat(self, points, img_metas=None):
+ """Extract features from points."""
+ voxels, num_points, coors = self.voxelize(points)
+ voxel_features = self.voxel_encoder(voxels, num_points, coors)
+ batch_size = coors[-1, 0].item() + 1
+ x = self.middle_encoder(voxel_features, coors, batch_size)
+ x = self.backbone(x)
+ if self.with_neck:
+ x = self.neck(x)
+ return x
+
+ @torch.no_grad()
+ @force_fp32()
+ def voxelize(self, points):
+ """Apply hard voxelization to points."""
+ voxels, coors, num_points = [], [], []
+ for res in points:
+ res_voxels, res_coors, res_num_points = self.voxel_layer(res)
+ voxels.append(res_voxels)
+ coors.append(res_coors)
+ num_points.append(res_num_points)
+ voxels = torch.cat(voxels, dim=0)
+ num_points = torch.cat(num_points, dim=0)
+ coors_batch = []
+ for i, coor in enumerate(coors):
+ coor_pad = F.pad(coor, (1, 0), mode='constant', value=i)
+ coors_batch.append(coor_pad)
+ coors_batch = torch.cat(coors_batch, dim=0)
+ return voxels, num_points, coors_batch
+
+ def forward_train(self,
+ points,
+ img_metas,
+ gt_bboxes_3d,
+ gt_labels_3d,
+ gt_bboxes_ignore=None):
+ """Training forward function.
+
+ Args:
+ points (list[torch.Tensor]): Point cloud of each sample.
+ img_metas (list[dict]): Meta information of each sample
+ gt_bboxes_3d (list[:obj:`BaseInstance3DBoxes`]): Ground truth
+ boxes for each sample.
+ gt_labels_3d (list[torch.Tensor]): Ground truth labels for
+ boxes of each sampole
+ gt_bboxes_ignore (list[torch.Tensor], optional): Ground truth
+ boxes to be ignored. Defaults to None.
+
+ Returns:
+ dict: Losses of each branch.
+ """
+ x = self.extract_feat(points, img_metas)
+ outs = self.bbox_head(x)
+ loss_inputs = outs + (gt_bboxes_3d, gt_labels_3d, img_metas)
+ losses = self.bbox_head.loss(
+ *loss_inputs, gt_bboxes_ignore=gt_bboxes_ignore)
+ return losses
+
+ def simple_test(self, points, img_metas, imgs=None, rescale=False):
+ """Test function without augmentaiton."""
+ x = self.extract_feat(points, img_metas)
+ outs = self.bbox_head(x)
+ bbox_list = self.bbox_head.get_bboxes(
+ *outs, img_metas, rescale=rescale)
+ bbox_results = [
+ bbox3d2result(bboxes, scores, labels)
+ for bboxes, scores, labels in bbox_list
+ ]
+ return bbox_results
+
+ def aug_test(self, points, img_metas, imgs=None, rescale=False):
+ """Test function with augmentaiton."""
+ feats = self.extract_feats(points, img_metas)
+
+ # only support aug_test for one sample
+ aug_bboxes = []
+ for x, img_meta in zip(feats, img_metas):
+ outs = self.bbox_head(x)
+ bbox_list = self.bbox_head.get_bboxes(
+ *outs, img_meta, rescale=rescale)
+ bbox_list = [
+ dict(boxes_3d=bboxes, scores_3d=scores, labels_3d=labels)
+ for bboxes, scores, labels in bbox_list
+ ]
+ aug_bboxes.append(bbox_list[0])
+
+ # after merging, bboxes will be rescaled to the original image size
+ merged_bboxes = merge_aug_bboxes_3d(aug_bboxes, img_metas,
+ self.bbox_head.test_cfg)
+
+ return [merged_bboxes]
diff --git a/mmdet3d/models/fusion_layers/__init__.py b/mmdet3d/models/fusion_layers/__init__.py
new file mode 100644
index 0000000..6df4741
--- /dev/null
+++ b/mmdet3d/models/fusion_layers/__init__.py
@@ -0,0 +1,10 @@
+# Copyright (c) OpenMMLab. All rights reserved.
+from .coord_transform import (apply_3d_transformation, bbox_2d_transform,
+ coord_2d_transform)
+from .point_fusion import PointFusion
+from .vote_fusion import VoteFusion
+
+__all__ = [
+ 'PointFusion', 'VoteFusion', 'apply_3d_transformation',
+ 'bbox_2d_transform', 'coord_2d_transform'
+]
diff --git a/mmdet3d/models/fusion_layers/coord_transform.py b/mmdet3d/models/fusion_layers/coord_transform.py
new file mode 100644
index 0000000..7cdcac9
--- /dev/null
+++ b/mmdet3d/models/fusion_layers/coord_transform.py
@@ -0,0 +1,216 @@
+# Copyright (c) OpenMMLab. All rights reserved.
+from functools import partial
+
+import torch
+
+from mmdet3d.core.points import get_points_type
+
+
+def apply_3d_transformation(pcd, coord_type, img_meta, reverse=False):
+ """Apply transformation to input point cloud.
+
+ Args:
+ pcd (torch.Tensor): The point cloud to be transformed.
+ coord_type (str): 'DEPTH' or 'CAMERA' or 'LIDAR'.
+ img_meta(dict): Meta info regarding data transformation.
+ reverse (bool): Reversed transformation or not.
+
+ Note:
+ The elements in img_meta['transformation_3d_flow']:
+ "T" stands for translation;
+ "S" stands for scale;
+ "R" stands for rotation;
+ "HF" stands for horizontal flip;
+ "VF" stands for vertical flip.
+
+ Returns:
+ torch.Tensor: The transformed point cloud.
+ """
+
+ dtype = pcd.dtype
+ device = pcd.device
+
+ pcd_rotate_mat = (
+ torch.tensor(img_meta['pcd_rotation'], dtype=dtype, device=device)
+ if 'pcd_rotation' in img_meta else torch.eye(
+ 3, dtype=dtype, device=device))
+
+ pcd_scale_factor = (
+ img_meta['pcd_scale_factor'] if 'pcd_scale_factor' in img_meta else 1.)
+
+ pcd_trans_factor = (
+ torch.tensor(img_meta['pcd_trans'], dtype=dtype, device=device)
+ if 'pcd_trans' in img_meta else torch.zeros(
+ (3), dtype=dtype, device=device))
+
+ pcd_horizontal_flip = img_meta[
+ 'pcd_horizontal_flip'] if 'pcd_horizontal_flip' in \
+ img_meta else False
+
+ pcd_vertical_flip = img_meta[
+ 'pcd_vertical_flip'] if 'pcd_vertical_flip' in \
+ img_meta else False
+
+ flow = img_meta['transformation_3d_flow'] \
+ if 'transformation_3d_flow' in img_meta else []
+
+ pcd = pcd.clone() # prevent inplace modification
+ pcd = get_points_type(coord_type)(pcd)
+
+ horizontal_flip_func = partial(pcd.flip, bev_direction='horizontal') \
+ if pcd_horizontal_flip else lambda: None
+ vertical_flip_func = partial(pcd.flip, bev_direction='vertical') \
+ if pcd_vertical_flip else lambda: None
+ if reverse:
+ scale_func = partial(pcd.scale, scale_factor=1.0 / pcd_scale_factor)
+ translate_func = partial(pcd.translate, trans_vector=-pcd_trans_factor)
+ # pcd_rotate_mat @ pcd_rotate_mat.inverse() is not
+ # exactly an identity matrix
+ # use angle to create the inverse rot matrix neither.
+ rotate_func = partial(pcd.rotate, rotation=pcd_rotate_mat.inverse())
+
+ # reverse the pipeline
+ flow = flow[::-1]
+ else:
+ scale_func = partial(pcd.scale, scale_factor=pcd_scale_factor)
+ translate_func = partial(pcd.translate, trans_vector=pcd_trans_factor)
+ rotate_func = partial(pcd.rotate, rotation=pcd_rotate_mat)
+
+ flow_mapping = {
+ 'T': translate_func,
+ 'S': scale_func,
+ 'R': rotate_func,
+ 'HF': horizontal_flip_func,
+ 'VF': vertical_flip_func
+ }
+ for op in flow:
+ assert op in flow_mapping, f'This 3D data '\
+ f'transformation op ({op}) is not supported'
+ func = flow_mapping[op]
+ func()
+
+ return pcd.coord
+
+
+def extract_2d_info(img_meta, tensor):
+ """Extract image augmentation information from img_meta.
+
+ Args:
+ img_meta(dict): Meta info regarding data transformation.
+ tensor(torch.Tensor): Input tensor used to create new ones.
+
+ Returns:
+ (int, int, int, int, torch.Tensor, bool, torch.Tensor):
+ The extracted information.
+ """
+ img_shape = img_meta['img_shape']
+ ori_shape = img_meta['ori_shape']
+ img_h, img_w, _ = img_shape
+ ori_h, ori_w, _ = ori_shape
+
+ img_scale_factor = (
+ tensor.new_tensor(img_meta['scale_factor'][:2])
+ if 'scale_factor' in img_meta else tensor.new_tensor([1.0, 1.0]))
+ img_flip = img_meta['flip'] if 'flip' in img_meta else False
+ img_crop_offset = (
+ tensor.new_tensor(img_meta['img_crop_offset'])
+ if 'img_crop_offset' in img_meta else tensor.new_tensor([0.0, 0.0]))
+
+ return (img_h, img_w, ori_h, ori_w, img_scale_factor, img_flip,
+ img_crop_offset)
+
+
+def bbox_2d_transform(img_meta, bbox_2d, ori2new):
+ """Transform 2d bbox according to img_meta.
+
+ Args:
+ img_meta(dict): Meta info regarding data transformation.
+ bbox_2d (torch.Tensor): Shape (..., >4)
+ The input 2d bboxes to transform.
+ ori2new (bool): Origin img coord system to new or not.
+
+ Returns:
+ torch.Tensor: The transformed 2d bboxes.
+ """
+
+ img_h, img_w, ori_h, ori_w, img_scale_factor, img_flip, \
+ img_crop_offset = extract_2d_info(img_meta, bbox_2d)
+
+ bbox_2d_new = bbox_2d.clone()
+
+ if ori2new:
+ bbox_2d_new[:, 0] = bbox_2d_new[:, 0] * img_scale_factor[0]
+ bbox_2d_new[:, 2] = bbox_2d_new[:, 2] * img_scale_factor[0]
+ bbox_2d_new[:, 1] = bbox_2d_new[:, 1] * img_scale_factor[1]
+ bbox_2d_new[:, 3] = bbox_2d_new[:, 3] * img_scale_factor[1]
+
+ bbox_2d_new[:, 0] = bbox_2d_new[:, 0] + img_crop_offset[0]
+ bbox_2d_new[:, 2] = bbox_2d_new[:, 2] + img_crop_offset[0]
+ bbox_2d_new[:, 1] = bbox_2d_new[:, 1] + img_crop_offset[1]
+ bbox_2d_new[:, 3] = bbox_2d_new[:, 3] + img_crop_offset[1]
+
+ if img_flip:
+ bbox_2d_r = img_w - bbox_2d_new[:, 0]
+ bbox_2d_l = img_w - bbox_2d_new[:, 2]
+ bbox_2d_new[:, 0] = bbox_2d_l
+ bbox_2d_new[:, 2] = bbox_2d_r
+ else:
+ if img_flip:
+ bbox_2d_r = img_w - bbox_2d_new[:, 0]
+ bbox_2d_l = img_w - bbox_2d_new[:, 2]
+ bbox_2d_new[:, 0] = bbox_2d_l
+ bbox_2d_new[:, 2] = bbox_2d_r
+
+ bbox_2d_new[:, 0] = bbox_2d_new[:, 0] - img_crop_offset[0]
+ bbox_2d_new[:, 2] = bbox_2d_new[:, 2] - img_crop_offset[0]
+ bbox_2d_new[:, 1] = bbox_2d_new[:, 1] - img_crop_offset[1]
+ bbox_2d_new[:, 3] = bbox_2d_new[:, 3] - img_crop_offset[1]
+
+ bbox_2d_new[:, 0] = bbox_2d_new[:, 0] / img_scale_factor[0]
+ bbox_2d_new[:, 2] = bbox_2d_new[:, 2] / img_scale_factor[0]
+ bbox_2d_new[:, 1] = bbox_2d_new[:, 1] / img_scale_factor[1]
+ bbox_2d_new[:, 3] = bbox_2d_new[:, 3] / img_scale_factor[1]
+
+ return bbox_2d_new
+
+
+def coord_2d_transform(img_meta, coord_2d, ori2new):
+ """Transform 2d pixel coordinates according to img_meta.
+
+ Args:
+ img_meta(dict): Meta info regarding data transformation.
+ coord_2d (torch.Tensor): Shape (..., 2)
+ The input 2d coords to transform.
+ ori2new (bool): Origin img coord system to new or not.
+
+ Returns:
+ torch.Tensor: The transformed 2d coordinates.
+ """
+
+ img_h, img_w, ori_h, ori_w, img_scale_factor, img_flip, \
+ img_crop_offset = extract_2d_info(img_meta, coord_2d)
+
+ coord_2d_new = coord_2d.clone()
+
+ if ori2new:
+ # TODO here we assume this order of transformation
+ coord_2d_new[..., 0] = coord_2d_new[..., 0] * img_scale_factor[0]
+ coord_2d_new[..., 1] = coord_2d_new[..., 1] * img_scale_factor[1]
+
+ coord_2d_new[..., 0] += img_crop_offset[0]
+ coord_2d_new[..., 1] += img_crop_offset[1]
+
+ # flip uv coordinates and bbox
+ if img_flip:
+ coord_2d_new[..., 0] = img_w - coord_2d_new[..., 0]
+ else:
+ if img_flip:
+ coord_2d_new[..., 0] = img_w - coord_2d_new[..., 0]
+
+ coord_2d_new[..., 0] -= img_crop_offset[0]
+ coord_2d_new[..., 1] -= img_crop_offset[1]
+
+ coord_2d_new[..., 0] = coord_2d_new[..., 0] / img_scale_factor[0]
+ coord_2d_new[..., 1] = coord_2d_new[..., 1] / img_scale_factor[1]
+
+ return coord_2d_new
diff --git a/mmdet3d/models/fusion_layers/point_fusion.py b/mmdet3d/models/fusion_layers/point_fusion.py
new file mode 100644
index 0000000..97b4177
--- /dev/null
+++ b/mmdet3d/models/fusion_layers/point_fusion.py
@@ -0,0 +1,306 @@
+# Copyright (c) OpenMMLab. All rights reserved.
+import torch
+from mmcv.cnn import ConvModule
+from mmcv.runner import BaseModule
+from torch import nn as nn
+from torch.nn import functional as F
+
+from mmdet3d.core.bbox.structures import (get_proj_mat_by_coord_type,
+ points_cam2img)
+from ..builder import FUSION_LAYERS
+from . import apply_3d_transformation
+
+
+def point_sample(img_meta,
+ img_features,
+ points,
+ proj_mat,
+ coord_type,
+ img_scale_factor,
+ img_crop_offset,
+ img_flip,
+ img_pad_shape,
+ img_shape,
+ aligned=True,
+ padding_mode='zeros',
+ align_corners=True):
+ """Obtain image features using points.
+
+ Args:
+ img_meta (dict): Meta info.
+ img_features (torch.Tensor): 1 x C x H x W image features.
+ points (torch.Tensor): Nx3 point cloud in LiDAR coordinates.
+ proj_mat (torch.Tensor): 4x4 transformation matrix.
+ coord_type (str): 'DEPTH' or 'CAMERA' or 'LIDAR'.
+ img_scale_factor (torch.Tensor): Scale factor with shape of
+ (w_scale, h_scale).
+ img_crop_offset (torch.Tensor): Crop offset used to crop
+ image during data augmentation with shape of (w_offset, h_offset).
+ img_flip (bool): Whether the image is flipped.
+ img_pad_shape (tuple[int]): int tuple indicates the h & w after
+ padding, this is necessary to obtain features in feature map.
+ img_shape (tuple[int]): int tuple indicates the h & w before padding
+ after scaling, this is necessary for flipping coordinates.
+ aligned (bool, optional): Whether use bilinear interpolation when
+ sampling image features for each point. Defaults to True.
+ padding_mode (str, optional): Padding mode when padding values for
+ features of out-of-image points. Defaults to 'zeros'.
+ align_corners (bool, optional): Whether to align corners when
+ sampling image features for each point. Defaults to True.
+
+ Returns:
+ torch.Tensor: NxC image features sampled by point coordinates.
+ """
+
+ # apply transformation based on info in img_meta
+ points = apply_3d_transformation(
+ points, coord_type, img_meta, reverse=True)
+
+ # project points to camera coordinate
+ pts_2d = points_cam2img(points, proj_mat)
+
+ # img transformation: scale -> crop -> flip
+ # the image is resized by img_scale_factor
+ img_coors = pts_2d[:, 0:2] * img_scale_factor # Nx2
+ img_coors -= img_crop_offset
+
+ # grid sample, the valid grid range should be in [-1,1]
+ coor_x, coor_y = torch.split(img_coors, 1, dim=1) # each is Nx1
+
+ if img_flip:
+ # by default we take it as horizontal flip
+ # use img_shape before padding for flip
+ orig_h, orig_w = img_shape
+ coor_x = orig_w - coor_x
+
+ h, w = img_pad_shape
+ coor_y = coor_y / h * 2 - 1
+ coor_x = coor_x / w * 2 - 1
+ grid = torch.cat([coor_x, coor_y],
+ dim=1).unsqueeze(0).unsqueeze(0) # Nx2 -> 1x1xNx2
+
+ # align_corner=True provides higher performance
+ mode = 'bilinear' if aligned else 'nearest'
+ point_features = F.grid_sample(
+ img_features,
+ grid,
+ mode=mode,
+ padding_mode=padding_mode,
+ align_corners=align_corners) # 1xCx1xN feats
+
+ return point_features.squeeze().t()
+
+
+@FUSION_LAYERS.register_module()
+class PointFusion(BaseModule):
+ """Fuse image features from multi-scale features.
+
+ Args:
+ img_channels (list[int] | int): Channels of image features.
+ It could be a list if the input is multi-scale image features.
+ pts_channels (int): Channels of point features
+ mid_channels (int): Channels of middle layers
+ out_channels (int): Channels of output fused features
+ img_levels (int, optional): Number of image levels. Defaults to 3.
+ coord_type (str): 'DEPTH' or 'CAMERA' or 'LIDAR'.
+ Defaults to 'LIDAR'.
+ conv_cfg (dict, optional): Dict config of conv layers of middle
+ layers. Defaults to None.
+ norm_cfg (dict, optional): Dict config of norm layers of middle
+ layers. Defaults to None.
+ act_cfg (dict, optional): Dict config of activatation layers.
+ Defaults to None.
+ activate_out (bool, optional): Whether to apply relu activation
+ to output features. Defaults to True.
+ fuse_out (bool, optional): Whether apply conv layer to the fused
+ features. Defaults to False.
+ dropout_ratio (int, float, optional): Dropout ratio of image
+ features to prevent overfitting. Defaults to 0.
+ aligned (bool, optional): Whether apply aligned feature fusion.
+ Defaults to True.
+ align_corners (bool, optional): Whether to align corner when
+ sampling features according to points. Defaults to True.
+ padding_mode (str, optional): Mode used to pad the features of
+ points that do not have corresponding image features.
+ Defaults to 'zeros'.
+ lateral_conv (bool, optional): Whether to apply lateral convs
+ to image features. Defaults to True.
+ """
+
+ def __init__(self,
+ img_channels,
+ pts_channels,
+ mid_channels,
+ out_channels,
+ img_levels=3,
+ coord_type='LIDAR',
+ conv_cfg=None,
+ norm_cfg=None,
+ act_cfg=None,
+ init_cfg=None,
+ activate_out=True,
+ fuse_out=False,
+ dropout_ratio=0,
+ aligned=True,
+ align_corners=True,
+ padding_mode='zeros',
+ lateral_conv=True):
+ super(PointFusion, self).__init__(init_cfg=init_cfg)
+ if isinstance(img_levels, int):
+ img_levels = [img_levels]
+ if isinstance(img_channels, int):
+ img_channels = [img_channels] * len(img_levels)
+ assert isinstance(img_levels, list)
+ assert isinstance(img_channels, list)
+ assert len(img_channels) == len(img_levels)
+
+ self.img_levels = img_levels
+ self.coord_type = coord_type
+ self.act_cfg = act_cfg
+ self.activate_out = activate_out
+ self.fuse_out = fuse_out
+ self.dropout_ratio = dropout_ratio
+ self.img_channels = img_channels
+ self.aligned = aligned
+ self.align_corners = align_corners
+ self.padding_mode = padding_mode
+
+ self.lateral_convs = None
+ if lateral_conv:
+ self.lateral_convs = nn.ModuleList()
+ for i in range(len(img_channels)):
+ l_conv = ConvModule(
+ img_channels[i],
+ mid_channels,
+ 3,
+ padding=1,
+ conv_cfg=conv_cfg,
+ norm_cfg=norm_cfg,
+ act_cfg=self.act_cfg,
+ inplace=False)
+ self.lateral_convs.append(l_conv)
+ self.img_transform = nn.Sequential(
+ nn.Linear(mid_channels * len(img_channels), out_channels),
+ nn.BatchNorm1d(out_channels, eps=1e-3, momentum=0.01),
+ )
+ else:
+ self.img_transform = nn.Sequential(
+ nn.Linear(sum(img_channels), out_channels),
+ nn.BatchNorm1d(out_channels, eps=1e-3, momentum=0.01),
+ )
+ self.pts_transform = nn.Sequential(
+ nn.Linear(pts_channels, out_channels),
+ nn.BatchNorm1d(out_channels, eps=1e-3, momentum=0.01),
+ )
+
+ if self.fuse_out:
+ self.fuse_conv = nn.Sequential(
+ nn.Linear(mid_channels, out_channels),
+ # For pts the BN is initialized differently by default
+ # TODO: check whether this is necessary
+ nn.BatchNorm1d(out_channels, eps=1e-3, momentum=0.01),
+ nn.ReLU(inplace=False))
+
+ if init_cfg is None:
+ self.init_cfg = [
+ dict(type='Xavier', layer='Conv2d', distribution='uniform'),
+ dict(type='Xavier', layer='Linear', distribution='uniform')
+ ]
+
+ def forward(self, img_feats, pts, pts_feats, img_metas):
+ """Forward function.
+
+ Args:
+ img_feats (list[torch.Tensor]): Image features.
+ pts: [list[torch.Tensor]]: A batch of points with shape N x 3.
+ pts_feats (torch.Tensor): A tensor consist of point features of the
+ total batch.
+ img_metas (list[dict]): Meta information of images.
+
+ Returns:
+ torch.Tensor: Fused features of each point.
+ """
+ img_pts = self.obtain_mlvl_feats(img_feats, pts, img_metas)
+ img_pre_fuse = self.img_transform(img_pts)
+ if self.training and self.dropout_ratio > 0:
+ img_pre_fuse = F.dropout(img_pre_fuse, self.dropout_ratio)
+ pts_pre_fuse = self.pts_transform(pts_feats)
+
+ fuse_out = img_pre_fuse + pts_pre_fuse
+ if self.activate_out:
+ fuse_out = F.relu(fuse_out)
+ if self.fuse_out:
+ fuse_out = self.fuse_conv(fuse_out)
+
+ return fuse_out
+
+ def obtain_mlvl_feats(self, img_feats, pts, img_metas):
+ """Obtain multi-level features for each point.
+
+ Args:
+ img_feats (list(torch.Tensor)): Multi-scale image features produced
+ by image backbone in shape (N, C, H, W).
+ pts (list[torch.Tensor]): Points of each sample.
+ img_metas (list[dict]): Meta information for each sample.
+
+ Returns:
+ torch.Tensor: Corresponding image features of each point.
+ """
+ if self.lateral_convs is not None:
+ img_ins = [
+ lateral_conv(img_feats[i])
+ for i, lateral_conv in zip(self.img_levels, self.lateral_convs)
+ ]
+ else:
+ img_ins = img_feats
+ img_feats_per_point = []
+ # Sample multi-level features
+ for i in range(len(img_metas)):
+ mlvl_img_feats = []
+ for level in range(len(self.img_levels)):
+ mlvl_img_feats.append(
+ self.sample_single(img_ins[level][i:i + 1], pts[i][:, :3],
+ img_metas[i]))
+ mlvl_img_feats = torch.cat(mlvl_img_feats, dim=-1)
+ img_feats_per_point.append(mlvl_img_feats)
+
+ img_pts = torch.cat(img_feats_per_point, dim=0)
+ return img_pts
+
+ def sample_single(self, img_feats, pts, img_meta):
+ """Sample features from single level image feature map.
+
+ Args:
+ img_feats (torch.Tensor): Image feature map in shape
+ (1, C, H, W).
+ pts (torch.Tensor): Points of a single sample.
+ img_meta (dict): Meta information of the single sample.
+
+ Returns:
+ torch.Tensor: Single level image features of each point.
+ """
+ # TODO: image transformation also extracted
+ img_scale_factor = (
+ pts.new_tensor(img_meta['scale_factor'][:2])
+ if 'scale_factor' in img_meta.keys() else 1)
+ img_flip = img_meta['flip'] if 'flip' in img_meta.keys() else False
+ img_crop_offset = (
+ pts.new_tensor(img_meta['img_crop_offset'])
+ if 'img_crop_offset' in img_meta.keys() else 0)
+ proj_mat = get_proj_mat_by_coord_type(img_meta, self.coord_type)
+ img_pts = point_sample(
+ img_meta=img_meta,
+ img_features=img_feats,
+ points=pts,
+ proj_mat=pts.new_tensor(proj_mat),
+ coord_type=self.coord_type,
+ img_scale_factor=img_scale_factor,
+ img_crop_offset=img_crop_offset,
+ img_flip=img_flip,
+ img_pad_shape=img_meta['input_shape'][:2],
+ img_shape=img_meta['img_shape'][:2],
+ aligned=self.aligned,
+ padding_mode=self.padding_mode,
+ align_corners=self.align_corners,
+ )
+ return img_pts
diff --git a/mmdet3d/models/fusion_layers/vote_fusion.py b/mmdet3d/models/fusion_layers/vote_fusion.py
new file mode 100644
index 0000000..3633e4d
--- /dev/null
+++ b/mmdet3d/models/fusion_layers/vote_fusion.py
@@ -0,0 +1,200 @@
+# Copyright (c) OpenMMLab. All rights reserved.
+import torch
+from torch import nn as nn
+
+from mmdet3d.core.bbox import points_cam2img
+from ..builder import FUSION_LAYERS
+from . import apply_3d_transformation, bbox_2d_transform, coord_2d_transform
+
+EPS = 1e-6
+
+
+@FUSION_LAYERS.register_module()
+class VoteFusion(nn.Module):
+ """Fuse 2d features from 3d seeds.
+
+ Args:
+ num_classes (int): number of classes.
+ max_imvote_per_pixel (int): max number of imvotes.
+ """
+
+ def __init__(self, num_classes=10, max_imvote_per_pixel=3):
+ super(VoteFusion, self).__init__()
+ self.num_classes = num_classes
+ self.max_imvote_per_pixel = max_imvote_per_pixel
+
+ def forward(self, imgs, bboxes_2d_rescaled, seeds_3d_depth, img_metas):
+ """Forward function.
+
+ Args:
+ imgs (list[torch.Tensor]): Image features.
+ bboxes_2d_rescaled (list[torch.Tensor]): 2D bboxes.
+ seeds_3d_depth (torch.Tensor): 3D seeds.
+ img_metas (list[dict]): Meta information of images.
+
+ Returns:
+ torch.Tensor: Concatenated cues of each point.
+ torch.Tensor: Validity mask of each feature.
+ """
+ img_features = []
+ masks = []
+ for i, data in enumerate(
+ zip(imgs, bboxes_2d_rescaled, seeds_3d_depth, img_metas)):
+ img, bbox_2d_rescaled, seed_3d_depth, img_meta = data
+ bbox_num = bbox_2d_rescaled.shape[0]
+ seed_num = seed_3d_depth.shape[0]
+
+ img_shape = img_meta['img_shape']
+ img_h, img_w, _ = img_shape
+
+ # first reverse the data transformations
+ xyz_depth = apply_3d_transformation(
+ seed_3d_depth, 'DEPTH', img_meta, reverse=True)
+
+ # project points from depth to image
+ depth2img = xyz_depth.new_tensor(img_meta['depth2img'])
+ uvz_origin = points_cam2img(xyz_depth, depth2img, True)
+ z_cam = uvz_origin[..., 2]
+ uv_origin = (uvz_origin[..., :2] - 1).round()
+
+ # rescale 2d coordinates and bboxes
+ uv_rescaled = coord_2d_transform(img_meta, uv_origin, True)
+ bbox_2d_origin = bbox_2d_transform(img_meta, bbox_2d_rescaled,
+ False)
+
+ if bbox_num == 0:
+ imvote_num = seed_num * self.max_imvote_per_pixel
+
+ # use zero features
+ two_cues = torch.zeros((15, imvote_num),
+ device=seed_3d_depth.device)
+ mask_zero = torch.zeros(
+ imvote_num - seed_num, device=seed_3d_depth.device).bool()
+ mask_one = torch.ones(
+ seed_num, device=seed_3d_depth.device).bool()
+ mask = torch.cat([mask_one, mask_zero], dim=0)
+ else:
+ # expand bboxes and seeds
+ bbox_expanded = bbox_2d_origin.view(1, bbox_num, -1).expand(
+ seed_num, -1, -1)
+ seed_2d_expanded = uv_origin.view(seed_num, 1,
+ -1).expand(-1, bbox_num, -1)
+ seed_2d_expanded_x, seed_2d_expanded_y = \
+ seed_2d_expanded.split(1, dim=-1)
+
+ bbox_expanded_l, bbox_expanded_t, bbox_expanded_r, \
+ bbox_expanded_b, bbox_expanded_conf, bbox_expanded_cls = \
+ bbox_expanded.split(1, dim=-1)
+ bbox_expanded_midx = (bbox_expanded_l + bbox_expanded_r) / 2
+ bbox_expanded_midy = (bbox_expanded_t + bbox_expanded_b) / 2
+
+ seed_2d_in_bbox_x = (seed_2d_expanded_x > bbox_expanded_l) * \
+ (seed_2d_expanded_x < bbox_expanded_r)
+ seed_2d_in_bbox_y = (seed_2d_expanded_y > bbox_expanded_t) * \
+ (seed_2d_expanded_y < bbox_expanded_b)
+ seed_2d_in_bbox = seed_2d_in_bbox_x * seed_2d_in_bbox_y
+
+ # semantic cues, dim=class_num
+ sem_cue = torch.zeros_like(bbox_expanded_conf).expand(
+ -1, -1, self.num_classes)
+ sem_cue = sem_cue.scatter(-1, bbox_expanded_cls.long(),
+ bbox_expanded_conf)
+
+ # bbox center - uv
+ delta_u = bbox_expanded_midx - seed_2d_expanded_x
+ delta_v = bbox_expanded_midy - seed_2d_expanded_y
+
+ seed_3d_expanded = seed_3d_depth.view(seed_num, 1, -1).expand(
+ -1, bbox_num, -1)
+
+ z_cam = z_cam.view(seed_num, 1, 1).expand(-1, bbox_num, -1)
+ imvote = torch.cat(
+ [delta_u, delta_v,
+ torch.zeros_like(delta_v)], dim=-1).view(-1, 3)
+ imvote = imvote * z_cam.reshape(-1, 1)
+ imvote = imvote @ torch.inverse(depth2img.t())
+
+ # apply transformation to lifted imvotes
+ imvote = apply_3d_transformation(
+ imvote, 'DEPTH', img_meta, reverse=False)
+
+ seed_3d_expanded = seed_3d_expanded.reshape(imvote.shape)
+
+ # ray angle
+ ray_angle = seed_3d_expanded + imvote
+ ray_angle /= torch.sqrt(torch.sum(ray_angle**2, -1) +
+ EPS).unsqueeze(-1)
+
+ # imvote lifted to 3d
+ xz = ray_angle[:, [0, 2]] / (ray_angle[:, [1]] + EPS) \
+ * seed_3d_expanded[:, [1]] - seed_3d_expanded[:, [0, 2]]
+
+ # geometric cues, dim=5
+ geo_cue = torch.cat([xz, ray_angle],
+ dim=-1).view(seed_num, -1, 5)
+
+ two_cues = torch.cat([geo_cue, sem_cue], dim=-1)
+ # mask to 0 if seed not in bbox
+ two_cues = two_cues * seed_2d_in_bbox.float()
+
+ feature_size = two_cues.shape[-1]
+ # if bbox number is too small, append zeros
+ if bbox_num < self.max_imvote_per_pixel:
+ append_num = self.max_imvote_per_pixel - bbox_num
+ append_zeros = torch.zeros(
+ (seed_num, append_num, 1),
+ device=seed_2d_in_bbox.device).bool()
+ seed_2d_in_bbox = torch.cat(
+ [seed_2d_in_bbox, append_zeros], dim=1)
+ append_zeros = torch.zeros(
+ (seed_num, append_num, feature_size),
+ device=two_cues.device)
+ two_cues = torch.cat([two_cues, append_zeros], dim=1)
+ append_zeros = torch.zeros((seed_num, append_num, 1),
+ device=two_cues.device)
+ bbox_expanded_conf = torch.cat(
+ [bbox_expanded_conf, append_zeros], dim=1)
+
+ # sort the valid seed-bbox pair according to confidence
+ pair_score = seed_2d_in_bbox.float() + bbox_expanded_conf
+ # and find the largests
+ mask, indices = pair_score.topk(
+ self.max_imvote_per_pixel,
+ dim=1,
+ largest=True,
+ sorted=True)
+
+ indices_img = indices.expand(-1, -1, feature_size)
+ two_cues = two_cues.gather(dim=1, index=indices_img)
+ two_cues = two_cues.transpose(1, 0)
+ two_cues = two_cues.reshape(-1, feature_size).transpose(
+ 1, 0).contiguous()
+
+ # since conf is ~ (0, 1), floor gives us validity
+ mask = mask.floor().int()
+ mask = mask.transpose(1, 0).reshape(-1).bool()
+
+ # clear the padding
+ img = img[:, :img_shape[0], :img_shape[1]]
+ img_flatten = img.reshape(3, -1).float()
+ img_flatten /= 255.
+
+ # take the normalized pixel value as texture cue
+ uv_rescaled[:, 0] = torch.clamp(uv_rescaled[:, 0].round(), 0,
+ img_shape[1] - 1)
+ uv_rescaled[:, 1] = torch.clamp(uv_rescaled[:, 1].round(), 0,
+ img_shape[0] - 1)
+ uv_flatten = uv_rescaled[:, 1].round() * \
+ img_shape[1] + uv_rescaled[:, 0].round()
+ uv_expanded = uv_flatten.unsqueeze(0).expand(3, -1).long()
+ txt_cue = torch.gather(img_flatten, dim=-1, index=uv_expanded)
+ txt_cue = txt_cue.unsqueeze(1).expand(-1,
+ self.max_imvote_per_pixel,
+ -1).reshape(3, -1)
+
+ # append texture cue
+ img_feature = torch.cat([two_cues, txt_cue], dim=0)
+ img_features.append(img_feature)
+ masks.append(mask)
+
+ return torch.stack(img_features, 0), torch.stack(masks, 0)
diff --git a/mmdet3d/models/losses/__init__.py b/mmdet3d/models/losses/__init__.py
new file mode 100644
index 0000000..c3c0943
--- /dev/null
+++ b/mmdet3d/models/losses/__init__.py
@@ -0,0 +1,15 @@
+# Copyright (c) OpenMMLab. All rights reserved.
+from mmdet.models.losses import FocalLoss, SmoothL1Loss, binary_cross_entropy
+from .axis_aligned_iou_loss import AxisAlignedIoULoss, axis_aligned_iou_loss
+from .chamfer_distance import ChamferDistance, chamfer_distance
+from .multibin_loss import MultiBinLoss
+from .paconv_regularization_loss import PAConvRegularizationLoss
+from .rotated_iou_loss import RotatedIoU3DLoss
+from .uncertain_smooth_l1_loss import UncertainL1Loss, UncertainSmoothL1Loss
+
+__all__ = [
+ 'FocalLoss', 'SmoothL1Loss', 'binary_cross_entropy', 'ChamferDistance',
+ 'chamfer_distance', 'axis_aligned_iou_loss', 'AxisAlignedIoULoss',
+ 'PAConvRegularizationLoss', 'UncertainL1Loss', 'UncertainSmoothL1Loss',
+ 'MultiBinLoss', 'RotatedIoU3DLoss'
+]
diff --git a/mmdet3d/models/losses/axis_aligned_iou_loss.py b/mmdet3d/models/losses/axis_aligned_iou_loss.py
new file mode 100644
index 0000000..b446dce
--- /dev/null
+++ b/mmdet3d/models/losses/axis_aligned_iou_loss.py
@@ -0,0 +1,117 @@
+# Copyright (c) OpenMMLab. All rights reserved.
+import torch
+from torch import nn as nn
+
+from mmdet.models.losses.utils import weighted_loss
+from ...core.bbox import AxisAlignedBboxOverlaps3D
+from ..builder import LOSSES
+
+
+@weighted_loss
+def axis_aligned_iou_loss(pred, target):
+ """Calculate the IoU loss (1-IoU) of two sets of axis aligned bounding
+ boxes. Note that predictions and targets are one-to-one corresponded.
+ Args:
+ pred (torch.Tensor): Bbox predictions with shape [..., 6]
+ (x1, y1, z1, x2, y2, z2).
+ target (torch.Tensor): Bbox targets (gt) with shape [..., 6]
+ (x1, y1, z1, x2, y2, z2).
+ Returns:
+ torch.Tensor: IoU loss between predictions and targets.
+ """
+ axis_aligned_iou = AxisAlignedBboxOverlaps3D()(
+ pred, target, is_aligned=True)
+ iou_loss = 1 - axis_aligned_iou
+ return iou_loss
+
+
+@weighted_loss
+def axis_aligned_diou_loss(pred, target):
+ """Calculate the DIoU loss (1-DIoU) of two sets of axis aligned bounding
+ boxes. Note that predictions and targets are one-to-one corresponded.
+ Args:
+ pred (torch.Tensor): Bbox predictions with shape [..., 6]
+ (x1, y1, z1, x2, y2, z2).
+ target (torch.Tensor): Bbox targets (gt) with shape [..., 6]
+ (x1, y1, z1, x2, y2, z2).
+ Returns:
+ torch.Tensor: IoU loss between predictions and targets.
+ """
+ axis_aligned_iou = AxisAlignedBboxOverlaps3D()(
+ pred, target, is_aligned=True)
+ iou_loss = 1 - axis_aligned_iou
+
+ xp1, yp1, zp1, xp2, yp2, zp2 = pred.split(1, dim=-1)
+ xt1, yt1, zt1, xt2, yt2, zt2 = target.split(1, dim=-1)
+
+ xpc = (xp1 + xp2) / 2
+ ypc = (yp1 + yp2) / 2
+ zpc = (zp1 + zp2) / 2
+ xtc = (xt1 + xt2) / 2
+ ytc = (yt1 + yt2) / 2
+ ztc = (zt1 + zt2) / 2
+ r2 = (xpc - xtc) ** 2 + (ypc - ytc) ** 2 + (zpc - ztc) ** 2
+
+ x_min = torch.minimum(xp1, xt1)
+ x_max = torch.maximum(xp2, xt2)
+ y_min = torch.minimum(yp1, yt1)
+ y_max = torch.maximum(yp2, yt2)
+ z_min = torch.minimum(zp1, zt1)
+ z_max = torch.maximum(zp2, zt2)
+ c2 = (x_min - x_max) ** 2 + (y_min - y_max) ** 2 + (z_min - z_max) ** 2
+
+ diou_loss = iou_loss + (r2 / c2)[:, 0]
+ return diou_loss
+
+
+@LOSSES.register_module()
+class AxisAlignedIoULoss(nn.Module):
+ """Calculate the IoU loss (1-IoU) of axis aligned bounding boxes.
+ Args:
+ reduction (str): Method to reduce losses.
+ The valid reduction method are none, sum or mean.
+ loss_weight (float, optional): Weight of loss. Defaults to 1.0.
+ """
+
+ def __init__(self, mode='iou', reduction='mean', loss_weight=1.0):
+ super(AxisAlignedIoULoss, self).__init__()
+ self.loss = axis_aligned_iou_loss if mode == 'iou' else axis_aligned_diou_loss
+ assert reduction in ['none', 'sum', 'mean']
+ self.reduction = reduction
+ self.loss_weight = loss_weight
+
+ def forward(self,
+ pred,
+ target,
+ weight=None,
+ avg_factor=None,
+ reduction_override=None,
+ **kwargs):
+ """Forward function of loss calculation.
+ Args:
+ pred (torch.Tensor): Bbox predictions with shape [..., 6]
+ (x1, y1, z1, x2, y2, z2).
+ target (torch.Tensor): Bbox targets (gt) with shape [..., 6]
+ (x1, y1, z1, x2, y2, z2).
+ weight (torch.Tensor | float, optional): Weight of loss.
+ Defaults to None.
+ avg_factor (int, optional): Average factor that is used to average
+ the loss. Defaults to None.
+ reduction_override (str, optional): Method to reduce losses.
+ The valid reduction method are 'none', 'sum' or 'mean'.
+ Defaults to None.
+ Returns:
+ torch.Tensor: IoU loss between predictions and targets.
+ """
+ assert reduction_override in (None, 'none', 'mean', 'sum')
+ reduction = (
+ reduction_override if reduction_override else self.reduction)
+ if (weight is not None) and (not torch.any(weight > 0)) and (
+ reduction != 'none'):
+ return (pred * weight).sum()
+ return self.loss(
+ pred,
+ target,
+ weight=weight,
+ avg_factor=avg_factor,
+ reduction=reduction) * self.loss_weight
\ No newline at end of file
diff --git a/mmdet3d/models/losses/chamfer_distance.py b/mmdet3d/models/losses/chamfer_distance.py
new file mode 100644
index 0000000..8ad109d
--- /dev/null
+++ b/mmdet3d/models/losses/chamfer_distance.py
@@ -0,0 +1,147 @@
+# Copyright (c) OpenMMLab. All rights reserved.
+import torch
+from torch import nn as nn
+from torch.nn.functional import l1_loss, mse_loss, smooth_l1_loss
+
+from ..builder import LOSSES
+
+
+def chamfer_distance(src,
+ dst,
+ src_weight=1.0,
+ dst_weight=1.0,
+ criterion_mode='l2',
+ reduction='mean'):
+ """Calculate Chamfer Distance of two sets.
+
+ Args:
+ src (torch.Tensor): Source set with shape [B, N, C] to
+ calculate Chamfer Distance.
+ dst (torch.Tensor): Destination set with shape [B, M, C] to
+ calculate Chamfer Distance.
+ src_weight (torch.Tensor or float): Weight of source loss.
+ dst_weight (torch.Tensor or float): Weight of destination loss.
+ criterion_mode (str): Criterion mode to calculate distance.
+ The valid modes are smooth_l1, l1 or l2.
+ reduction (str): Method to reduce losses.
+ The valid reduction method are 'none', 'sum' or 'mean'.
+
+ Returns:
+ tuple: Source and Destination loss with the corresponding indices.
+
+ - loss_src (torch.Tensor): The min distance
+ from source to destination.
+ - loss_dst (torch.Tensor): The min distance
+ from destination to source.
+ - indices1 (torch.Tensor): Index the min distance point
+ for each point in source to destination.
+ - indices2 (torch.Tensor): Index the min distance point
+ for each point in destination to source.
+ """
+
+ if criterion_mode == 'smooth_l1':
+ criterion = smooth_l1_loss
+ elif criterion_mode == 'l1':
+ criterion = l1_loss
+ elif criterion_mode == 'l2':
+ criterion = mse_loss
+ else:
+ raise NotImplementedError
+
+ src_expand = src.unsqueeze(2).repeat(1, 1, dst.shape[1], 1)
+ dst_expand = dst.unsqueeze(1).repeat(1, src.shape[1], 1, 1)
+
+ distance = criterion(src_expand, dst_expand, reduction='none').sum(-1)
+ src2dst_distance, indices1 = torch.min(distance, dim=2) # (B,N)
+ dst2src_distance, indices2 = torch.min(distance, dim=1) # (B,M)
+
+ loss_src = (src2dst_distance * src_weight)
+ loss_dst = (dst2src_distance * dst_weight)
+
+ if reduction == 'sum':
+ loss_src = torch.sum(loss_src)
+ loss_dst = torch.sum(loss_dst)
+ elif reduction == 'mean':
+ loss_src = torch.mean(loss_src)
+ loss_dst = torch.mean(loss_dst)
+ elif reduction == 'none':
+ pass
+ else:
+ raise NotImplementedError
+
+ return loss_src, loss_dst, indices1, indices2
+
+
+@LOSSES.register_module()
+class ChamferDistance(nn.Module):
+ """Calculate Chamfer Distance of two sets.
+
+ Args:
+ mode (str): Criterion mode to calculate distance.
+ The valid modes are smooth_l1, l1 or l2.
+ reduction (str): Method to reduce losses.
+ The valid reduction method are none, sum or mean.
+ loss_src_weight (float): Weight of loss_source.
+ loss_dst_weight (float): Weight of loss_target.
+ """
+
+ def __init__(self,
+ mode='l2',
+ reduction='mean',
+ loss_src_weight=1.0,
+ loss_dst_weight=1.0):
+ super(ChamferDistance, self).__init__()
+
+ assert mode in ['smooth_l1', 'l1', 'l2']
+ assert reduction in ['none', 'sum', 'mean']
+ self.mode = mode
+ self.reduction = reduction
+ self.loss_src_weight = loss_src_weight
+ self.loss_dst_weight = loss_dst_weight
+
+ def forward(self,
+ source,
+ target,
+ src_weight=1.0,
+ dst_weight=1.0,
+ reduction_override=None,
+ return_indices=False,
+ **kwargs):
+ """Forward function of loss calculation.
+
+ Args:
+ source (torch.Tensor): Source set with shape [B, N, C] to
+ calculate Chamfer Distance.
+ target (torch.Tensor): Destination set with shape [B, M, C] to
+ calculate Chamfer Distance.
+ src_weight (torch.Tensor | float, optional):
+ Weight of source loss. Defaults to 1.0.
+ dst_weight (torch.Tensor | float, optional):
+ Weight of destination loss. Defaults to 1.0.
+ reduction_override (str, optional): Method to reduce losses.
+ The valid reduction method are 'none', 'sum' or 'mean'.
+ Defaults to None.
+ return_indices (bool, optional): Whether to return indices.
+ Defaults to False.
+
+ Returns:
+ tuple[torch.Tensor]: If ``return_indices=True``, return losses of
+ source and target with their corresponding indices in the
+ order of ``(loss_source, loss_target, indices1, indices2)``.
+ If ``return_indices=False``, return
+ ``(loss_source, loss_target)``.
+ """
+ assert reduction_override in (None, 'none', 'mean', 'sum')
+ reduction = (
+ reduction_override if reduction_override else self.reduction)
+
+ loss_source, loss_target, indices1, indices2 = chamfer_distance(
+ source, target, src_weight, dst_weight, self.mode, reduction)
+
+ loss_source *= self.loss_src_weight
+ loss_target *= self.loss_dst_weight
+
+ if return_indices:
+ return loss_source, loss_target, indices1, indices2
+ else:
+ return loss_source, loss_target
diff --git a/mmdet3d/models/losses/multibin_loss.py b/mmdet3d/models/losses/multibin_loss.py
new file mode 100644
index 0000000..461a19c
--- /dev/null
+++ b/mmdet3d/models/losses/multibin_loss.py
@@ -0,0 +1,93 @@
+# Copyright (c) OpenMMLab. All rights reserved.
+import torch
+from torch import nn as nn
+from torch.nn import functional as F
+
+from mmdet.models.losses.utils import weighted_loss
+from ..builder import LOSSES
+
+
+@weighted_loss
+def multibin_loss(pred_orientations, gt_orientations, num_dir_bins=4):
+ """Multi-Bin Loss.
+
+ Args:
+ pred_orientations(torch.Tensor): Predicted local vector
+ orientation in [axis_cls, head_cls, sin, cos] format.
+ shape (N, num_dir_bins * 4)
+ gt_orientations(torch.Tensor): Corresponding gt bboxes,
+ shape (N, num_dir_bins * 2).
+ num_dir_bins(int, optional): Number of bins to encode
+ direction angle.
+ Defaults: 4.
+
+ Return:
+ torch.Tensor: Loss tensor.
+ """
+ cls_losses = 0
+ reg_losses = 0
+ reg_cnt = 0
+ for i in range(num_dir_bins):
+ # bin cls loss
+ cls_ce_loss = F.cross_entropy(
+ pred_orientations[:, (i * 2):(i * 2 + 2)],
+ gt_orientations[:, i].long(),
+ reduction='mean')
+ # regression loss
+ valid_mask_i = (gt_orientations[:, i] == 1)
+ cls_losses += cls_ce_loss
+ if valid_mask_i.sum() > 0:
+ start = num_dir_bins * 2 + i * 2
+ end = start + 2
+ pred_offset = F.normalize(pred_orientations[valid_mask_i,
+ start:end])
+ gt_offset_sin = torch.sin(gt_orientations[valid_mask_i,
+ num_dir_bins + i])
+ gt_offset_cos = torch.cos(gt_orientations[valid_mask_i,
+ num_dir_bins + i])
+ reg_loss = \
+ F.l1_loss(pred_offset[:, 0], gt_offset_sin,
+ reduction='none') + \
+ F.l1_loss(pred_offset[:, 1], gt_offset_cos,
+ reduction='none')
+
+ reg_losses += reg_loss.sum()
+ reg_cnt += valid_mask_i.sum()
+
+ return cls_losses / num_dir_bins + reg_losses / reg_cnt
+
+
+@LOSSES.register_module()
+class MultiBinLoss(nn.Module):
+ """Multi-Bin Loss for orientation.
+
+ Args:
+ reduction (str, optional): The method to reduce the loss.
+ Options are 'none', 'mean' and 'sum'. Defaults to 'none'.
+ loss_weight (float, optional): The weight of loss. Defaults
+ to 1.0.
+ """
+
+ def __init__(self, reduction='none', loss_weight=1.0):
+ super(MultiBinLoss, self).__init__()
+ assert reduction in ['none', 'sum', 'mean']
+ self.reduction = reduction
+ self.loss_weight = loss_weight
+
+ def forward(self, pred, target, num_dir_bins, reduction_override=None):
+ """Forward function.
+
+ Args:
+ pred (torch.Tensor): The prediction.
+ target (torch.Tensor): The learning target of the prediction.
+ num_dir_bins (int): Number of bins to encode direction angle.
+ reduction_override (str, optional): The reduction method used to
+ override the original reduction method of the loss.
+ Defaults to None.
+ """
+ assert reduction_override in (None, 'none', 'mean', 'sum')
+ reduction = (
+ reduction_override if reduction_override else self.reduction)
+ loss = self.loss_weight * multibin_loss(
+ pred, target, num_dir_bins=num_dir_bins, reduction=reduction)
+ return loss
diff --git a/mmdet3d/models/losses/paconv_regularization_loss.py b/mmdet3d/models/losses/paconv_regularization_loss.py
new file mode 100644
index 0000000..2001790
--- /dev/null
+++ b/mmdet3d/models/losses/paconv_regularization_loss.py
@@ -0,0 +1,108 @@
+# Copyright (c) OpenMMLab. All rights reserved.
+import torch
+from torch import nn as nn
+
+from mmdet3d.ops import PAConv, PAConvCUDA
+from mmdet.models.losses.utils import weight_reduce_loss
+from ..builder import LOSSES
+
+
+def weight_correlation(conv):
+ """Calculate correlations between kernel weights in Conv's weight bank as
+ regularization loss. The cosine similarity is used as metrics.
+
+ Args:
+ conv (nn.Module): A Conv modules to be regularized.
+ Currently we only support `PAConv` and `PAConvCUDA`.
+
+ Returns:
+ torch.Tensor: Correlations between each kernel weights in weight bank.
+ """
+ assert isinstance(conv, (PAConv, PAConvCUDA)), \
+ f'unsupported module type {type(conv)}'
+ kernels = conv.weight_bank # [C_in, num_kernels * C_out]
+ in_channels = conv.in_channels
+ out_channels = conv.out_channels
+ num_kernels = conv.num_kernels
+
+ # [num_kernels, Cin * Cout]
+ flatten_kernels = kernels.view(in_channels, num_kernels, out_channels).\
+ permute(1, 0, 2).reshape(num_kernels, -1)
+ # [num_kernels, num_kernels]
+ inner_product = torch.matmul(flatten_kernels, flatten_kernels.T)
+ # [num_kernels, 1]
+ kernel_norms = torch.sum(flatten_kernels**2, dim=-1, keepdim=True)**0.5
+ # [num_kernels, num_kernels]
+ kernel_norms = torch.matmul(kernel_norms, kernel_norms.T)
+ cosine_sims = inner_product / kernel_norms
+ # take upper triangular part excluding diagonal since we only compute
+ # correlation between different kernels once
+ # the square is to ensure positive loss, refer to:
+ # https://github.com/CVMI-Lab/PAConv/blob/main/scene_seg/tool/train.py#L208
+ corr = torch.sum(torch.triu(cosine_sims, diagonal=1)**2)
+
+ return corr
+
+
+def paconv_regularization_loss(modules, reduction):
+ """Computes correlation loss of PAConv weight kernels as regularization.
+
+ Args:
+ modules (List[nn.Module] | :obj:`generator`):
+ A list or a python generator of torch.nn.Modules.
+ reduction (str): Method to reduce losses among PAConv modules.
+ The valid reduction method are none, sum or mean.
+
+ Returns:
+ torch.Tensor: Correlation loss of kernel weights.
+ """
+ corr_loss = []
+ for module in modules:
+ if isinstance(module, (PAConv, PAConvCUDA)):
+ corr_loss.append(weight_correlation(module))
+ corr_loss = torch.stack(corr_loss)
+
+ # perform reduction
+ corr_loss = weight_reduce_loss(corr_loss, reduction=reduction)
+
+ return corr_loss
+
+
+@LOSSES.register_module()
+class PAConvRegularizationLoss(nn.Module):
+ """Calculate correlation loss of kernel weights in PAConv's weight bank.
+
+ This is used as a regularization term in PAConv model training.
+
+ Args:
+ reduction (str): Method to reduce losses. The reduction is performed
+ among all PAConv modules instead of prediction tensors.
+ The valid reduction method are none, sum or mean.
+ loss_weight (float, optional): Weight of loss. Defaults to 1.0.
+ """
+
+ def __init__(self, reduction='mean', loss_weight=1.0):
+ super(PAConvRegularizationLoss, self).__init__()
+ assert reduction in ['none', 'sum', 'mean']
+ self.reduction = reduction
+ self.loss_weight = loss_weight
+
+ def forward(self, modules, reduction_override=None, **kwargs):
+ """Forward function of loss calculation.
+
+ Args:
+ modules (List[nn.Module] | :obj:`generator`):
+ A list or a python generator of torch.nn.Modules.
+ reduction_override (str, optional): Method to reduce losses.
+ The valid reduction method are 'none', 'sum' or 'mean'.
+ Defaults to None.
+
+ Returns:
+ torch.Tensor: Correlation loss of kernel weights.
+ """
+ assert reduction_override in (None, 'none', 'mean', 'sum')
+ reduction = (
+ reduction_override if reduction_override else self.reduction)
+
+ return self.loss_weight * paconv_regularization_loss(
+ modules, reduction=reduction)
diff --git a/mmdet3d/models/losses/rotated_iou_loss.py b/mmdet3d/models/losses/rotated_iou_loss.py
new file mode 100644
index 0000000..47c9139
--- /dev/null
+++ b/mmdet3d/models/losses/rotated_iou_loss.py
@@ -0,0 +1,84 @@
+# Copyright (c) OpenMMLab. All rights reserved.
+import torch
+from mmcv.ops import diff_iou_rotated_3d
+from torch import nn as nn
+
+from mmdet.models.losses.utils import weighted_loss
+from ..builder import LOSSES
+
+
+@weighted_loss
+def rotated_iou_3d_loss(pred, target):
+ """Calculate the IoU loss (1-IoU) of two sets of rotated bounding boxes.
+ Note that predictions and targets are one-to-one corresponded.
+
+ Args:
+ pred (torch.Tensor): Bbox predictions with shape [N, 7]
+ (x, y, z, w, l, h, alpha).
+ target (torch.Tensor): Bbox targets (gt) with shape [N, 7]
+ (x, y, z, w, l, h, alpha).
+
+ Returns:
+ torch.Tensor: IoU loss between predictions and targets.
+ """
+ iou_loss = 1 - diff_iou_rotated_3d(pred.unsqueeze(0),
+ target.unsqueeze(0))[0]
+ return iou_loss
+
+
+@LOSSES.register_module()
+class RotatedIoU3DLoss(nn.Module):
+ """Calculate the IoU loss (1-IoU) of rotated bounding boxes.
+
+ Args:
+ reduction (str): Method to reduce losses.
+ The valid reduction method are none, sum or mean.
+ loss_weight (float, optional): Weight of loss. Defaults to 1.0.
+ """
+
+ def __init__(self, reduction='mean', loss_weight=1.0):
+ super().__init__()
+ self.reduction = reduction
+ self.loss_weight = loss_weight
+
+ def forward(self,
+ pred,
+ target,
+ weight=None,
+ avg_factor=None,
+ reduction_override=None,
+ **kwargs):
+ """Forward function of loss calculation.
+
+ Args:
+ pred (torch.Tensor): Bbox predictions with shape [..., 7]
+ (x, y, z, w, l, h, alpha).
+ target (torch.Tensor): Bbox targets (gt) with shape [..., 7]
+ (x, y, z, w, l, h, alpha).
+ weight (torch.Tensor | float, optional): Weight of loss.
+ Defaults to None.
+ avg_factor (int, optional): Average factor that is used to average
+ the loss. Defaults to None.
+ reduction_override (str, optional): Method to reduce losses.
+ The valid reduction method are 'none', 'sum' or 'mean'.
+ Defaults to None.
+
+ Returns:
+ torch.Tensor: IoU loss between predictions and targets.
+ """
+ if weight is not None and not torch.any(weight > 0):
+ return pred.sum() * weight.sum() # 0
+ assert reduction_override in (None, 'none', 'mean', 'sum')
+ reduction = (
+ reduction_override if reduction_override else self.reduction)
+ if weight is not None and weight.dim() > 1:
+ weight = weight.mean(-1)
+ loss = self.loss_weight * rotated_iou_3d_loss(
+ pred,
+ target,
+ weight,
+ reduction=reduction,
+ avg_factor=avg_factor,
+ **kwargs)
+
+ return loss
diff --git a/mmdet3d/models/losses/uncertain_smooth_l1_loss.py b/mmdet3d/models/losses/uncertain_smooth_l1_loss.py
new file mode 100644
index 0000000..e80c08f
--- /dev/null
+++ b/mmdet3d/models/losses/uncertain_smooth_l1_loss.py
@@ -0,0 +1,176 @@
+# Copyright (c) OpenMMLab. All rights reserved.
+import torch
+from torch import nn as nn
+
+from mmdet.models.losses.utils import weighted_loss
+from ..builder import LOSSES
+
+
+@weighted_loss
+def uncertain_smooth_l1_loss(pred, target, sigma, alpha=1.0, beta=1.0):
+ """Smooth L1 loss with uncertainty.
+
+ Args:
+ pred (torch.Tensor): The prediction.
+ target (torch.Tensor): The learning target of the prediction.
+ sigma (torch.Tensor): The sigma for uncertainty.
+ alpha (float, optional): The coefficient of log(sigma).
+ Defaults to 1.0.
+ beta (float, optional): The threshold in the piecewise function.
+ Defaults to 1.0.
+
+ Returns:
+ torch.Tensor: Calculated loss
+ """
+ assert beta > 0
+ assert target.numel() > 0
+ assert pred.size() == target.size() == sigma.size(), 'The size of pred ' \
+ f'{pred.size()}, target {target.size()}, and sigma {sigma.size()} ' \
+ 'are inconsistent.'
+ diff = torch.abs(pred - target)
+ loss = torch.where(diff < beta, 0.5 * diff * diff / beta,
+ diff - 0.5 * beta)
+ loss = torch.exp(-sigma) * loss + alpha * sigma
+
+ return loss
+
+
+@weighted_loss
+def uncertain_l1_loss(pred, target, sigma, alpha=1.0):
+ """L1 loss with uncertainty.
+
+ Args:
+ pred (torch.Tensor): The prediction.
+ target (torch.Tensor): The learning target of the prediction.
+ sigma (torch.Tensor): The sigma for uncertainty.
+ alpha (float, optional): The coefficient of log(sigma).
+ Defaults to 1.0.
+
+ Returns:
+ torch.Tensor: Calculated loss
+ """
+ assert target.numel() > 0
+ assert pred.size() == target.size() == sigma.size(), 'The size of pred ' \
+ f'{pred.size()}, target {target.size()}, and sigma {sigma.size()} ' \
+ 'are inconsistent.'
+ loss = torch.abs(pred - target)
+ loss = torch.exp(-sigma) * loss + alpha * sigma
+ return loss
+
+
+@LOSSES.register_module()
+class UncertainSmoothL1Loss(nn.Module):
+ r"""Smooth L1 loss with uncertainty.
+
+ Please refer to `PGD `_ and
+ `Multi-Task Learning Using Uncertainty to Weigh Losses for Scene Geometry
+ and Semantics `_ for more details.
+
+ Args:
+ alpha (float, optional): The coefficient of log(sigma).
+ Defaults to 1.0.
+ beta (float, optional): The threshold in the piecewise function.
+ Defaults to 1.0.
+ reduction (str, optional): The method to reduce the loss.
+ Options are 'none', 'mean' and 'sum'. Defaults to 'mean'.
+ loss_weight (float, optional): The weight of loss. Defaults to 1.0
+ """
+
+ def __init__(self, alpha=1.0, beta=1.0, reduction='mean', loss_weight=1.0):
+ super(UncertainSmoothL1Loss, self).__init__()
+ assert reduction in ['none', 'sum', 'mean']
+ self.alpha = alpha
+ self.beta = beta
+ self.reduction = reduction
+ self.loss_weight = loss_weight
+
+ def forward(self,
+ pred,
+ target,
+ sigma,
+ weight=None,
+ avg_factor=None,
+ reduction_override=None,
+ **kwargs):
+ """Forward function.
+
+ Args:
+ pred (torch.Tensor): The prediction.
+ target (torch.Tensor): The learning target of the prediction.
+ sigma (torch.Tensor): The sigma for uncertainty.
+ weight (torch.Tensor, optional): The weight of loss for each
+ prediction. Defaults to None.
+ avg_factor (int, optional): Average factor that is used to average
+ the loss. Defaults to None.
+ reduction_override (str, optional): The reduction method used to
+ override the original reduction method of the loss.
+ Defaults to None.
+ """
+ assert reduction_override in (None, 'none', 'mean', 'sum')
+ reduction = (
+ reduction_override if reduction_override else self.reduction)
+ loss_bbox = self.loss_weight * uncertain_smooth_l1_loss(
+ pred,
+ target,
+ weight,
+ sigma=sigma,
+ alpha=self.alpha,
+ beta=self.beta,
+ reduction=reduction,
+ avg_factor=avg_factor,
+ **kwargs)
+ return loss_bbox
+
+
+@LOSSES.register_module()
+class UncertainL1Loss(nn.Module):
+ """L1 loss with uncertainty.
+
+ Args:
+ alpha (float, optional): The coefficient of log(sigma).
+ Defaults to 1.0.
+ reduction (str, optional): The method to reduce the loss.
+ Options are 'none', 'mean' and 'sum'. Defaults to 'mean'.
+ loss_weight (float, optional): The weight of loss. Defaults to 1.0.
+ """
+
+ def __init__(self, alpha=1.0, reduction='mean', loss_weight=1.0):
+ super(UncertainL1Loss, self).__init__()
+ assert reduction in ['none', 'sum', 'mean']
+ self.alpha = alpha
+ self.reduction = reduction
+ self.loss_weight = loss_weight
+
+ def forward(self,
+ pred,
+ target,
+ sigma,
+ weight=None,
+ avg_factor=None,
+ reduction_override=None):
+ """Forward function.
+
+ Args:
+ pred (torch.Tensor): The prediction.
+ target (torch.Tensor): The learning target of the prediction.
+ sigma (torch.Tensor): The sigma for uncertainty.
+ weight (torch.Tensor, optional): The weight of loss for each
+ prediction. Defaults to None.
+ avg_factor (int, optional): Average factor that is used to average
+ the loss. Defaults to None.
+ reduction_override (str, optional): The reduction method used to
+ override the original reduction method of the loss.
+ Defaults to None.
+ """
+ assert reduction_override in (None, 'none', 'mean', 'sum')
+ reduction = (
+ reduction_override if reduction_override else self.reduction)
+ loss_bbox = self.loss_weight * uncertain_l1_loss(
+ pred,
+ target,
+ weight,
+ sigma=sigma,
+ alpha=self.alpha,
+ reduction=reduction,
+ avg_factor=avg_factor)
+ return loss_bbox
diff --git a/mmdet3d/models/middle_encoders/__init__.py b/mmdet3d/models/middle_encoders/__init__.py
new file mode 100644
index 0000000..d7b4435
--- /dev/null
+++ b/mmdet3d/models/middle_encoders/__init__.py
@@ -0,0 +1,8 @@
+# Copyright (c) OpenMMLab. All rights reserved.
+from .pillar_scatter import PointPillarsScatter
+from .sparse_encoder import SparseEncoder, SparseEncoderSASSD
+from .sparse_unet import SparseUNet
+
+__all__ = [
+ 'PointPillarsScatter', 'SparseEncoder', 'SparseEncoderSASSD', 'SparseUNet'
+]
diff --git a/mmdet3d/models/middle_encoders/pillar_scatter.py b/mmdet3d/models/middle_encoders/pillar_scatter.py
new file mode 100644
index 0000000..725ce29
--- /dev/null
+++ b/mmdet3d/models/middle_encoders/pillar_scatter.py
@@ -0,0 +1,102 @@
+# Copyright (c) OpenMMLab. All rights reserved.
+import torch
+from mmcv.runner import auto_fp16
+from torch import nn
+
+from ..builder import MIDDLE_ENCODERS
+
+
+@MIDDLE_ENCODERS.register_module()
+class PointPillarsScatter(nn.Module):
+ """Point Pillar's Scatter.
+
+ Converts learned features from dense tensor to sparse pseudo image.
+
+ Args:
+ in_channels (int): Channels of input features.
+ output_shape (list[int]): Required output shape of features.
+ """
+
+ def __init__(self, in_channels, output_shape):
+ super().__init__()
+ self.output_shape = output_shape
+ self.ny = output_shape[0]
+ self.nx = output_shape[1]
+ self.in_channels = in_channels
+ self.fp16_enabled = False
+
+ @auto_fp16(apply_to=('voxel_features', ))
+ def forward(self, voxel_features, coors, batch_size=None):
+ """Foraward function to scatter features."""
+ # TODO: rewrite the function in a batch manner
+ # no need to deal with different batch cases
+ if batch_size is not None:
+ return self.forward_batch(voxel_features, coors, batch_size)
+ else:
+ return self.forward_single(voxel_features, coors)
+
+ def forward_single(self, voxel_features, coors):
+ """Scatter features of single sample.
+
+ Args:
+ voxel_features (torch.Tensor): Voxel features in shape (N, M, C).
+ coors (torch.Tensor): Coordinates of each voxel.
+ The first column indicates the sample ID.
+ """
+ # Create the canvas for this sample
+ canvas = torch.zeros(
+ self.in_channels,
+ self.nx * self.ny,
+ dtype=voxel_features.dtype,
+ device=voxel_features.device)
+
+ indices = coors[:, 2] * self.nx + coors[:, 3]
+ indices = indices.long()
+ voxels = voxel_features.t()
+ # Now scatter the blob back to the canvas.
+ canvas[:, indices] = voxels
+ # Undo the column stacking to final 4-dim tensor
+ canvas = canvas.view(1, self.in_channels, self.ny, self.nx)
+ return canvas
+
+ def forward_batch(self, voxel_features, coors, batch_size):
+ """Scatter features of single sample.
+
+ Args:
+ voxel_features (torch.Tensor): Voxel features in shape (N, M, C).
+ coors (torch.Tensor): Coordinates of each voxel in shape (N, 4).
+ The first column indicates the sample ID.
+ batch_size (int): Number of samples in the current batch.
+ """
+ # batch_canvas will be the final output.
+ batch_canvas = []
+ for batch_itt in range(batch_size):
+ # Create the canvas for this sample
+ canvas = torch.zeros(
+ self.in_channels,
+ self.nx * self.ny,
+ dtype=voxel_features.dtype,
+ device=voxel_features.device)
+
+ # Only include non-empty pillars
+ batch_mask = coors[:, 0] == batch_itt
+ this_coors = coors[batch_mask, :]
+ indices = this_coors[:, 2] * self.nx + this_coors[:, 3]
+ indices = indices.type(torch.long)
+ voxels = voxel_features[batch_mask, :]
+ voxels = voxels.t()
+
+ # Now scatter the blob back to the canvas.
+ canvas[:, indices] = voxels
+
+ # Append to a list for later stacking.
+ batch_canvas.append(canvas)
+
+ # Stack to 3-dim tensor (batch-size, in_channels, nrows*ncols)
+ batch_canvas = torch.stack(batch_canvas, 0)
+
+ # Undo the column stacking to final 4-dim tensor
+ batch_canvas = batch_canvas.view(batch_size, self.in_channels, self.ny,
+ self.nx)
+
+ return batch_canvas
diff --git a/mmdet3d/models/middle_encoders/sparse_encoder.py b/mmdet3d/models/middle_encoders/sparse_encoder.py
new file mode 100644
index 0000000..83a7a30
--- /dev/null
+++ b/mmdet3d/models/middle_encoders/sparse_encoder.py
@@ -0,0 +1,491 @@
+# Copyright (c) OpenMMLab. All rights reserved.
+import torch
+from mmcv.ops import points_in_boxes_all, three_interpolate, three_nn
+from mmcv.runner import auto_fp16
+from torch import nn as nn
+
+from mmdet3d.ops import SparseBasicBlock, make_sparse_convmodule
+from mmdet3d.ops.spconv import IS_SPCONV2_AVAILABLE
+from mmdet.models.losses import sigmoid_focal_loss, smooth_l1_loss
+from ..builder import MIDDLE_ENCODERS
+
+if IS_SPCONV2_AVAILABLE:
+ from spconv.pytorch import SparseConvTensor, SparseSequential
+else:
+ from mmcv.ops import SparseConvTensor, SparseSequential
+
+
+@MIDDLE_ENCODERS.register_module()
+class SparseEncoder(nn.Module):
+ r"""Sparse encoder for SECOND and Part-A2.
+
+ Args:
+ in_channels (int): The number of input channels.
+ sparse_shape (list[int]): The sparse shape of input tensor.
+ order (list[str], optional): Order of conv module.
+ Defaults to ('conv', 'norm', 'act').
+ norm_cfg (dict, optional): Config of normalization layer. Defaults to
+ dict(type='BN1d', eps=1e-3, momentum=0.01).
+ base_channels (int, optional): Out channels for conv_input layer.
+ Defaults to 16.
+ output_channels (int, optional): Out channels for conv_out layer.
+ Defaults to 128.
+ encoder_channels (tuple[tuple[int]], optional):
+ Convolutional channels of each encode block.
+ Defaults to ((16, ), (32, 32, 32), (64, 64, 64), (64, 64, 64)).
+ encoder_paddings (tuple[tuple[int]], optional):
+ Paddings of each encode block.
+ Defaults to ((1, ), (1, 1, 1), (1, 1, 1), ((0, 1, 1), 1, 1)).
+ block_type (str, optional): Type of the block to use.
+ Defaults to 'conv_module'.
+ """
+
+ def __init__(self,
+ in_channels,
+ sparse_shape,
+ order=('conv', 'norm', 'act'),
+ norm_cfg=dict(type='BN1d', eps=1e-3, momentum=0.01),
+ base_channels=16,
+ output_channels=128,
+ encoder_channels=((16, ), (32, 32, 32), (64, 64, 64), (64, 64,
+ 64)),
+ encoder_paddings=((1, ), (1, 1, 1), (1, 1, 1), ((0, 1, 1), 1,
+ 1)),
+ block_type='conv_module'):
+ super().__init__()
+ assert block_type in ['conv_module', 'basicblock']
+ self.sparse_shape = sparse_shape
+ self.in_channels = in_channels
+ self.order = order
+ self.base_channels = base_channels
+ self.output_channels = output_channels
+ self.encoder_channels = encoder_channels
+ self.encoder_paddings = encoder_paddings
+ self.stage_num = len(self.encoder_channels)
+ self.fp16_enabled = False
+ # Spconv init all weight on its own
+
+ assert isinstance(order, tuple) and len(order) == 3
+ assert set(order) == {'conv', 'norm', 'act'}
+
+ if self.order[0] != 'conv': # pre activate
+ self.conv_input = make_sparse_convmodule(
+ in_channels,
+ self.base_channels,
+ 3,
+ norm_cfg=norm_cfg,
+ padding=1,
+ indice_key='subm1',
+ conv_type='SubMConv3d',
+ order=('conv', ))
+ else: # post activate
+ self.conv_input = make_sparse_convmodule(
+ in_channels,
+ self.base_channels,
+ 3,
+ norm_cfg=norm_cfg,
+ padding=1,
+ indice_key='subm1',
+ conv_type='SubMConv3d')
+
+ encoder_out_channels = self.make_encoder_layers(
+ make_sparse_convmodule,
+ norm_cfg,
+ self.base_channels,
+ block_type=block_type)
+
+ self.conv_out = make_sparse_convmodule(
+ encoder_out_channels,
+ self.output_channels,
+ kernel_size=(3, 1, 1),
+ stride=(2, 1, 1),
+ norm_cfg=norm_cfg,
+ padding=0,
+ indice_key='spconv_down2',
+ conv_type='SparseConv3d')
+
+ @auto_fp16(apply_to=('voxel_features', ))
+ def forward(self, voxel_features, coors, batch_size):
+ """Forward of SparseEncoder.
+
+ Args:
+ voxel_features (torch.Tensor): Voxel features in shape (N, C).
+ coors (torch.Tensor): Coordinates in shape (N, 4),
+ the columns in the order of (batch_idx, z_idx, y_idx, x_idx).
+ batch_size (int): Batch size.
+
+ Returns:
+ dict: Backbone features.
+ """
+ coors = coors.int()
+ input_sp_tensor = SparseConvTensor(voxel_features, coors,
+ self.sparse_shape, batch_size)
+ x = self.conv_input(input_sp_tensor)
+
+ encode_features = []
+ for encoder_layer in self.encoder_layers:
+ x = encoder_layer(x)
+ encode_features.append(x)
+
+ # for detection head
+ # [200, 176, 5] -> [200, 176, 2]
+ out = self.conv_out(encode_features[-1])
+ spatial_features = out.dense()
+
+ N, C, D, H, W = spatial_features.shape
+ spatial_features = spatial_features.view(N, C * D, H, W)
+
+ return spatial_features
+
+ def make_encoder_layers(self,
+ make_block,
+ norm_cfg,
+ in_channels,
+ block_type='conv_module',
+ conv_cfg=dict(type='SubMConv3d')):
+ """make encoder layers using sparse convs.
+
+ Args:
+ make_block (method): A bounded function to build blocks.
+ norm_cfg (dict[str]): Config of normalization layer.
+ in_channels (int): The number of encoder input channels.
+ block_type (str, optional): Type of the block to use.
+ Defaults to 'conv_module'.
+ conv_cfg (dict, optional): Config of conv layer. Defaults to
+ dict(type='SubMConv3d').
+
+ Returns:
+ int: The number of encoder output channels.
+ """
+ assert block_type in ['conv_module', 'basicblock']
+ self.encoder_layers = SparseSequential()
+
+ for i, blocks in enumerate(self.encoder_channels):
+ blocks_list = []
+ for j, out_channels in enumerate(tuple(blocks)):
+ padding = tuple(self.encoder_paddings[i])[j]
+ # each stage started with a spconv layer
+ # except the first stage
+ if i != 0 and j == 0 and block_type == 'conv_module':
+ blocks_list.append(
+ make_block(
+ in_channels,
+ out_channels,
+ 3,
+ norm_cfg=norm_cfg,
+ stride=2,
+ padding=padding,
+ indice_key=f'spconv{i + 1}',
+ conv_type='SparseConv3d'))
+ elif block_type == 'basicblock':
+ if j == len(blocks) - 1 and i != len(
+ self.encoder_channels) - 1:
+ blocks_list.append(
+ make_block(
+ in_channels,
+ out_channels,
+ 3,
+ norm_cfg=norm_cfg,
+ stride=2,
+ padding=padding,
+ indice_key=f'spconv{i + 1}',
+ conv_type='SparseConv3d'))
+ else:
+ blocks_list.append(
+ SparseBasicBlock(
+ out_channels,
+ out_channels,
+ norm_cfg=norm_cfg,
+ conv_cfg=conv_cfg))
+ else:
+ blocks_list.append(
+ make_block(
+ in_channels,
+ out_channels,
+ 3,
+ norm_cfg=norm_cfg,
+ padding=padding,
+ indice_key=f'subm{i + 1}',
+ conv_type='SubMConv3d'))
+ in_channels = out_channels
+ stage_name = f'encoder_layer{i + 1}'
+ stage_layers = SparseSequential(*blocks_list)
+ self.encoder_layers.add_module(stage_name, stage_layers)
+ return out_channels
+
+
+@MIDDLE_ENCODERS.register_module()
+class SparseEncoderSASSD(SparseEncoder):
+ r"""Sparse encoder for `SASSD `_
+
+ Args:
+ in_channels (int): The number of input channels.
+ sparse_shape (list[int]): The sparse shape of input tensor.
+ order (list[str], optional): Order of conv module.
+ Defaults to ('conv', 'norm', 'act').
+ norm_cfg (dict, optional): Config of normalization layer. Defaults to
+ dict(type='BN1d', eps=1e-3, momentum=0.01).
+ base_channels (int, optional): Out channels for conv_input layer.
+ Defaults to 16.
+ output_channels (int, optional): Out channels for conv_out layer.
+ Defaults to 128.
+ encoder_channels (tuple[tuple[int]], optional):
+ Convolutional channels of each encode block.
+ Defaults to ((16, ), (32, 32, 32), (64, 64, 64), (64, 64, 64)).
+ encoder_paddings (tuple[tuple[int]], optional):
+ Paddings of each encode block.
+ Defaults to ((1, ), (1, 1, 1), (1, 1, 1), ((0, 1, 1), 1, 1)).
+ block_type (str, optional): Type of the block to use.
+ Defaults to 'conv_module'.
+ """
+
+ def __init__(self,
+ in_channels,
+ sparse_shape,
+ order=('conv', 'norm', 'act'),
+ norm_cfg=dict(type='BN1d', eps=1e-3, momentum=0.01),
+ base_channels=16,
+ output_channels=128,
+ encoder_channels=((16, ), (32, 32, 32), (64, 64, 64), (64, 64,
+ 64)),
+ encoder_paddings=((1, ), (1, 1, 1), (1, 1, 1), ((0, 1, 1), 1,
+ 1)),
+ block_type='conv_module'):
+ super(SparseEncoderSASSD, self).__init__(
+ in_channels=in_channels,
+ sparse_shape=sparse_shape,
+ order=order,
+ norm_cfg=norm_cfg,
+ base_channels=base_channels,
+ output_channels=output_channels,
+ encoder_channels=encoder_channels,
+ encoder_paddings=encoder_paddings,
+ block_type=block_type)
+
+ self.point_fc = nn.Linear(112, 64, bias=False)
+ self.point_cls = nn.Linear(64, 1, bias=False)
+ self.point_reg = nn.Linear(64, 3, bias=False)
+
+ @auto_fp16(apply_to=('voxel_features', ))
+ def forward(self, voxel_features, coors, batch_size, test_mode=False):
+ """Forward of SparseEncoder.
+
+ Args:
+ voxel_features (torch.Tensor): Voxel features in shape (N, C).
+ coors (torch.Tensor): Coordinates in shape (N, 4),
+ the columns in the order of (batch_idx, z_idx, y_idx, x_idx).
+ batch_size (int): Batch size.
+ test_mode (bool, optional): Whether in test mode.
+ Defaults to False.
+
+ Returns:
+ dict: Backbone features.
+ tuple[torch.Tensor]: Mean feature value of the points,
+ Classificaion result of the points,
+ Regression offsets of the points.
+ """
+ coors = coors.int()
+ input_sp_tensor = SparseConvTensor(voxel_features, coors,
+ self.sparse_shape, batch_size)
+ x = self.conv_input(input_sp_tensor)
+
+ encode_features = []
+ for encoder_layer in self.encoder_layers:
+ x = encoder_layer(x)
+ encode_features.append(x)
+
+ # for detection head
+ # [200, 176, 5] -> [200, 176, 2]
+ out = self.conv_out(encode_features[-1])
+ spatial_features = out.dense()
+
+ N, C, D, H, W = spatial_features.shape
+ spatial_features = spatial_features.view(N, C * D, H, W)
+
+ if test_mode:
+ return spatial_features, None
+
+ points_mean = torch.zeros_like(voxel_features)
+ points_mean[:, 0] = coors[:, 0]
+ points_mean[:, 1:] = voxel_features[:, :3]
+
+ # auxiliary network
+ p0 = self.make_auxiliary_points(
+ encode_features[0],
+ points_mean,
+ offset=(0, -40., -3.),
+ voxel_size=(.1, .1, .2))
+
+ p1 = self.make_auxiliary_points(
+ encode_features[1],
+ points_mean,
+ offset=(0, -40., -3.),
+ voxel_size=(.2, .2, .4))
+
+ p2 = self.make_auxiliary_points(
+ encode_features[2],
+ points_mean,
+ offset=(0, -40., -3.),
+ voxel_size=(.4, .4, .8))
+
+ pointwise = torch.cat([p0, p1, p2], dim=-1)
+ pointwise = self.point_fc(pointwise)
+ point_cls = self.point_cls(pointwise)
+ point_reg = self.point_reg(pointwise)
+ point_misc = (points_mean, point_cls, point_reg)
+
+ return spatial_features, point_misc
+
+ def get_auxiliary_targets(self, nxyz, gt_boxes3d, enlarge=1.0):
+ """Get auxiliary target.
+
+ Args:
+ nxyz (torch.Tensor): Mean features of the points.
+ gt_boxes3d (torch.Tensor): Coordinates in shape (N, 4),
+ the columns in the order of (batch_idx, z_idx, y_idx, x_idx).
+ enlarge (int, optional): Enlaged scale. Defaults to 1.0.
+
+ Returns:
+ tuple[torch.Tensor]: Label of the points and
+ center offsets of the points.
+ """
+ center_offsets = list()
+ pts_labels = list()
+ for i in range(len(gt_boxes3d)):
+ boxes3d = gt_boxes3d[i].tensor.cpu()
+ idx = torch.nonzero(nxyz[:, 0] == i).view(-1)
+ new_xyz = nxyz[idx, 1:].cpu()
+
+ boxes3d[:, 3:6] *= enlarge
+
+ pts_in_flag, center_offset = self.calculate_pts_offsets(
+ new_xyz, boxes3d)
+ pts_label = pts_in_flag.max(0)[0].byte()
+ pts_labels.append(pts_label)
+ center_offsets.append(center_offset)
+
+ center_offsets = torch.cat(center_offsets).cuda()
+ pts_labels = torch.cat(pts_labels).to(center_offsets.device)
+
+ return pts_labels, center_offsets
+
+ def calculate_pts_offsets(self, points, boxes):
+ """Find all boxes in which each point is, as well as the offsets from
+ the box centers.
+
+ Args:
+ points (torch.Tensor): [M, 3], [x, y, z] in LiDAR/DEPTH coordinate
+ boxes (torch.Tensor): [T, 7],
+ num_valid_boxes <= T, [x, y, z, x_size, y_size, z_size, rz],
+ (x, y, z) is the bottom center.
+
+ Returns:
+ tuple[torch.Tensor]: Point indices of boxes with the shape of
+ (T, M). Default background = 0.
+ And offsets from the box centers of points,
+ if it belows to the box, with the shape of (M, 3).
+ Default background = 0.
+ """
+ boxes_num = len(boxes)
+ pts_num = len(points)
+ points = points.cuda()
+ boxes = boxes.to(points.device)
+
+ box_idxs_of_pts = points_in_boxes_all(points[None, ...], boxes[None,
+ ...])
+
+ pts_indices = box_idxs_of_pts.squeeze(0).transpose(0, 1)
+
+ center_offsets = torch.zeros_like(points).to(points.device)
+
+ for i in range(boxes_num):
+ for j in range(pts_num):
+ if pts_indices[i][j] == 1:
+ center_offsets[j][0] = points[j][0] - boxes[i][0]
+ center_offsets[j][1] = points[j][1] - boxes[i][1]
+ center_offsets[j][2] = (
+ points[j][2] - (boxes[i][2] + boxes[i][2] / 2.0))
+ return pts_indices.cpu(), center_offsets.cpu()
+
+ def aux_loss(self, points, point_cls, point_reg, gt_bboxes):
+ """Calculate auxiliary loss.
+
+ Args:
+ points (torch.Tensor): Mean feature value of the points.
+ point_cls (torch.Tensor): Classificaion result of the points.
+ point_reg (torch.Tensor): Regression offsets of the points.
+ gt_bboxes (list[:obj:`BaseInstance3DBoxes`]): Ground truth
+ boxes for each sample.
+
+ Returns:
+ dict: Backbone features.
+ """
+ num_boxes = len(gt_bboxes)
+
+ pts_labels, center_targets = self.get_auxiliary_targets(
+ points, gt_bboxes)
+
+ rpn_cls_target = pts_labels.long()
+ pos = (pts_labels > 0).float()
+ neg = (pts_labels == 0).float()
+
+ pos_normalizer = pos.sum().clamp(min=1.0)
+
+ cls_weights = pos + neg
+ reg_weights = pos
+ reg_weights = reg_weights / pos_normalizer
+
+ aux_loss_cls = sigmoid_focal_loss(
+ point_cls,
+ rpn_cls_target,
+ weight=cls_weights,
+ avg_factor=pos_normalizer)
+
+ aux_loss_cls /= num_boxes
+
+ weight = reg_weights[..., None]
+ aux_loss_reg = smooth_l1_loss(point_reg, center_targets, beta=1 / 9.)
+ aux_loss_reg = torch.sum(aux_loss_reg * weight)[None]
+ aux_loss_reg /= num_boxes
+
+ aux_loss_cls, aux_loss_reg = [aux_loss_cls], [aux_loss_reg]
+
+ return dict(aux_loss_cls=aux_loss_cls, aux_loss_reg=aux_loss_reg)
+
+ def make_auxiliary_points(self,
+ source_tensor,
+ target,
+ offset=(0., -40., -3.),
+ voxel_size=(.05, .05, .1)):
+ """Make auxiliary points for loss computation.
+
+ Args:
+ source_tensor (torch.Tensor): (M, C) features to be propigated.
+ target (torch.Tensor): (N, 4) bxyz positions of the
+ target features.
+ offset (tuple[float], optional): Voxelization offset.
+ Defaults to (0., -40., -3.)
+ voxel_size (tuple[float], optional): Voxelization size.
+ Defaults to (.05, .05, .1)
+
+ Returns:
+ torch.Tensor: (N, C) tensor of the features of the target features.
+ """
+ # Tansfer tensor to points
+ source = source_tensor.indices.float()
+ offset = torch.Tensor(offset).to(source.device)
+ voxel_size = torch.Tensor(voxel_size).to(source.device)
+ source[:, 1:] = (
+ source[:, [3, 2, 1]] * voxel_size + offset + .5 * voxel_size)
+
+ source_feats = source_tensor.features[None, ...].transpose(1, 2)
+
+ # Interplate auxiliary points
+ dist, idx = three_nn(target[None, ...], source[None, ...])
+ dist_recip = 1.0 / (dist + 1e-8)
+ norm = torch.sum(dist_recip, dim=2, keepdim=True)
+ weight = dist_recip / norm
+ new_features = three_interpolate(source_feats.contiguous(), idx,
+ weight)
+
+ return new_features.squeeze(0).transpose(0, 1)
diff --git a/mmdet3d/models/middle_encoders/sparse_unet.py b/mmdet3d/models/middle_encoders/sparse_unet.py
new file mode 100644
index 0000000..005e34e
--- /dev/null
+++ b/mmdet3d/models/middle_encoders/sparse_unet.py
@@ -0,0 +1,300 @@
+# Copyright (c) OpenMMLab. All rights reserved.
+import torch
+
+from mmdet3d.ops.spconv import IS_SPCONV2_AVAILABLE
+
+if IS_SPCONV2_AVAILABLE:
+ from spconv.pytorch import SparseConvTensor, SparseSequential
+else:
+ from mmcv.ops import SparseConvTensor, SparseSequential
+
+from mmcv.runner import BaseModule, auto_fp16
+
+from mmdet3d.ops import SparseBasicBlock, make_sparse_convmodule
+from mmdet3d.ops.sparse_block import replace_feature
+from ..builder import MIDDLE_ENCODERS
+
+
+@MIDDLE_ENCODERS.register_module()
+class SparseUNet(BaseModule):
+ r"""SparseUNet for PartA^2.
+
+ See the `paper `_ for more details.
+
+ Args:
+ in_channels (int): The number of input channels.
+ sparse_shape (list[int]): The sparse shape of input tensor.
+ norm_cfg (dict): Config of normalization layer.
+ base_channels (int): Out channels for conv_input layer.
+ output_channels (int): Out channels for conv_out layer.
+ encoder_channels (tuple[tuple[int]]):
+ Convolutional channels of each encode block.
+ encoder_paddings (tuple[tuple[int]]): Paddings of each encode block.
+ decoder_channels (tuple[tuple[int]]):
+ Convolutional channels of each decode block.
+ decoder_paddings (tuple[tuple[int]]): Paddings of each decode block.
+ """
+
+ def __init__(self,
+ in_channels,
+ sparse_shape,
+ order=('conv', 'norm', 'act'),
+ norm_cfg=dict(type='BN1d', eps=1e-3, momentum=0.01),
+ base_channels=16,
+ output_channels=128,
+ encoder_channels=((16, ), (32, 32, 32), (64, 64, 64), (64, 64,
+ 64)),
+ encoder_paddings=((1, ), (1, 1, 1), (1, 1, 1), ((0, 1, 1), 1,
+ 1)),
+ decoder_channels=((64, 64, 64), (64, 64, 32), (32, 32, 16),
+ (16, 16, 16)),
+ decoder_paddings=((1, 0), (1, 0), (0, 0), (0, 1)),
+ init_cfg=None):
+ super().__init__(init_cfg=init_cfg)
+ self.sparse_shape = sparse_shape
+ self.in_channels = in_channels
+ self.order = order
+ self.base_channels = base_channels
+ self.output_channels = output_channels
+ self.encoder_channels = encoder_channels
+ self.encoder_paddings = encoder_paddings
+ self.decoder_channels = decoder_channels
+ self.decoder_paddings = decoder_paddings
+ self.stage_num = len(self.encoder_channels)
+ self.fp16_enabled = False
+ # Spconv init all weight on its own
+
+ assert isinstance(order, tuple) and len(order) == 3
+ assert set(order) == {'conv', 'norm', 'act'}
+
+ if self.order[0] != 'conv': # pre activate
+ self.conv_input = make_sparse_convmodule(
+ in_channels,
+ self.base_channels,
+ 3,
+ norm_cfg=norm_cfg,
+ padding=1,
+ indice_key='subm1',
+ conv_type='SubMConv3d',
+ order=('conv', ))
+ else: # post activate
+ self.conv_input = make_sparse_convmodule(
+ in_channels,
+ self.base_channels,
+ 3,
+ norm_cfg=norm_cfg,
+ padding=1,
+ indice_key='subm1',
+ conv_type='SubMConv3d')
+
+ encoder_out_channels = self.make_encoder_layers(
+ make_sparse_convmodule, norm_cfg, self.base_channels)
+ self.make_decoder_layers(make_sparse_convmodule, norm_cfg,
+ encoder_out_channels)
+
+ self.conv_out = make_sparse_convmodule(
+ encoder_out_channels,
+ self.output_channels,
+ kernel_size=(3, 1, 1),
+ stride=(2, 1, 1),
+ norm_cfg=norm_cfg,
+ padding=0,
+ indice_key='spconv_down2',
+ conv_type='SparseConv3d')
+
+ @auto_fp16(apply_to=('voxel_features', ))
+ def forward(self, voxel_features, coors, batch_size):
+ """Forward of SparseUNet.
+
+ Args:
+ voxel_features (torch.float32): Voxel features in shape [N, C].
+ coors (torch.int32): Coordinates in shape [N, 4],
+ the columns in the order of (batch_idx, z_idx, y_idx, x_idx).
+ batch_size (int): Batch size.
+
+ Returns:
+ dict[str, torch.Tensor]: Backbone features.
+ """
+ coors = coors.int()
+ input_sp_tensor = SparseConvTensor(voxel_features, coors,
+ self.sparse_shape, batch_size)
+ x = self.conv_input(input_sp_tensor)
+
+ encode_features = []
+ for encoder_layer in self.encoder_layers:
+ x = encoder_layer(x)
+ encode_features.append(x)
+
+ # for detection head
+ # [200, 176, 5] -> [200, 176, 2]
+ out = self.conv_out(encode_features[-1])
+ spatial_features = out.dense()
+
+ N, C, D, H, W = spatial_features.shape
+ spatial_features = spatial_features.view(N, C * D, H, W)
+
+ # for segmentation head, with output shape:
+ # [400, 352, 11] <- [200, 176, 5]
+ # [800, 704, 21] <- [400, 352, 11]
+ # [1600, 1408, 41] <- [800, 704, 21]
+ # [1600, 1408, 41] <- [1600, 1408, 41]
+ decode_features = []
+ x = encode_features[-1]
+ for i in range(self.stage_num, 0, -1):
+ x = self.decoder_layer_forward(encode_features[i - 1], x,
+ getattr(self, f'lateral_layer{i}'),
+ getattr(self, f'merge_layer{i}'),
+ getattr(self, f'upsample_layer{i}'))
+ decode_features.append(x)
+
+ seg_features = decode_features[-1].features
+
+ ret = dict(
+ spatial_features=spatial_features, seg_features=seg_features)
+
+ return ret
+
+ def decoder_layer_forward(self, x_lateral, x_bottom, lateral_layer,
+ merge_layer, upsample_layer):
+ """Forward of upsample and residual block.
+
+ Args:
+ x_lateral (:obj:`SparseConvTensor`): Lateral tensor.
+ x_bottom (:obj:`SparseConvTensor`): Feature from bottom layer.
+ lateral_layer (SparseBasicBlock): Convolution for lateral tensor.
+ merge_layer (SparseSequential): Convolution for merging features.
+ upsample_layer (SparseSequential): Convolution for upsampling.
+
+ Returns:
+ :obj:`SparseConvTensor`: Upsampled feature.
+ """
+ x = lateral_layer(x_lateral)
+ x = replace_feature(x, torch.cat((x_bottom.features, x.features),
+ dim=1))
+ x_merge = merge_layer(x)
+ x = self.reduce_channel(x, x_merge.features.shape[1])
+ x = replace_feature(x, x_merge.features + x.features)
+ x = upsample_layer(x)
+ return x
+
+ @staticmethod
+ def reduce_channel(x, out_channels):
+ """reduce channel for element-wise addition.
+
+ Args:
+ x (:obj:`SparseConvTensor`): Sparse tensor, ``x.features``
+ are in shape (N, C1).
+ out_channels (int): The number of channel after reduction.
+
+ Returns:
+ :obj:`SparseConvTensor`: Channel reduced feature.
+ """
+ features = x.features
+ n, in_channels = features.shape
+ assert (in_channels % out_channels
+ == 0) and (in_channels >= out_channels)
+ x = replace_feature(x, features.view(n, out_channels, -1).sum(dim=2))
+ return x
+
+ def make_encoder_layers(self, make_block, norm_cfg, in_channels):
+ """make encoder layers using sparse convs.
+
+ Args:
+ make_block (method): A bounded function to build blocks.
+ norm_cfg (dict[str]): Config of normalization layer.
+ in_channels (int): The number of encoder input channels.
+
+ Returns:
+ int: The number of encoder output channels.
+ """
+ self.encoder_layers = SparseSequential()
+
+ for i, blocks in enumerate(self.encoder_channels):
+ blocks_list = []
+ for j, out_channels in enumerate(tuple(blocks)):
+ padding = tuple(self.encoder_paddings[i])[j]
+ # each stage started with a spconv layer
+ # except the first stage
+ if i != 0 and j == 0:
+ blocks_list.append(
+ make_block(
+ in_channels,
+ out_channels,
+ 3,
+ norm_cfg=norm_cfg,
+ stride=2,
+ padding=padding,
+ indice_key=f'spconv{i + 1}',
+ conv_type='SparseConv3d'))
+ else:
+ blocks_list.append(
+ make_block(
+ in_channels,
+ out_channels,
+ 3,
+ norm_cfg=norm_cfg,
+ padding=padding,
+ indice_key=f'subm{i + 1}',
+ conv_type='SubMConv3d'))
+ in_channels = out_channels
+ stage_name = f'encoder_layer{i + 1}'
+ stage_layers = SparseSequential(*blocks_list)
+ self.encoder_layers.add_module(stage_name, stage_layers)
+ return out_channels
+
+ def make_decoder_layers(self, make_block, norm_cfg, in_channels):
+ """make decoder layers using sparse convs.
+
+ Args:
+ make_block (method): A bounded function to build blocks.
+ norm_cfg (dict[str]): Config of normalization layer.
+ in_channels (int): The number of encoder input channels.
+
+ Returns:
+ int: The number of encoder output channels.
+ """
+ block_num = len(self.decoder_channels)
+ for i, block_channels in enumerate(self.decoder_channels):
+ paddings = self.decoder_paddings[i]
+ setattr(
+ self, f'lateral_layer{block_num - i}',
+ SparseBasicBlock(
+ in_channels,
+ block_channels[0],
+ conv_cfg=dict(
+ type='SubMConv3d', indice_key=f'subm{block_num - i}'),
+ norm_cfg=norm_cfg))
+ setattr(
+ self, f'merge_layer{block_num - i}',
+ make_block(
+ in_channels * 2,
+ block_channels[1],
+ 3,
+ norm_cfg=norm_cfg,
+ padding=paddings[0],
+ indice_key=f'subm{block_num - i}',
+ conv_type='SubMConv3d'))
+ if block_num - i != 1:
+ setattr(
+ self, f'upsample_layer{block_num - i}',
+ make_block(
+ in_channels,
+ block_channels[2],
+ 3,
+ norm_cfg=norm_cfg,
+ indice_key=f'spconv{block_num - i}',
+ conv_type='SparseInverseConv3d'))
+ else:
+ # use submanifold conv instead of inverse conv
+ # in the last block
+ setattr(
+ self, f'upsample_layer{block_num - i}',
+ make_block(
+ in_channels,
+ block_channels[2],
+ 3,
+ norm_cfg=norm_cfg,
+ padding=paddings[1],
+ indice_key='subm1',
+ conv_type='SubMConv3d'))
+ in_channels = block_channels[2]
diff --git a/mmdet3d/models/model_utils/__init__.py b/mmdet3d/models/model_utils/__init__.py
new file mode 100644
index 0000000..34df79a
--- /dev/null
+++ b/mmdet3d/models/model_utils/__init__.py
@@ -0,0 +1,6 @@
+# Copyright (c) OpenMMLab. All rights reserved.
+from .edge_fusion_module import EdgeFusionModule
+from .transformer import GroupFree3DMHA
+from .vote_module import VoteModule
+
+__all__ = ['VoteModule', 'GroupFree3DMHA', 'EdgeFusionModule']
diff --git a/mmdet3d/models/model_utils/edge_fusion_module.py b/mmdet3d/models/model_utils/edge_fusion_module.py
new file mode 100644
index 0000000..2d9e09e
--- /dev/null
+++ b/mmdet3d/models/model_utils/edge_fusion_module.py
@@ -0,0 +1,78 @@
+# Copyright (c) OpenMMLab. All rights reserved.
+from mmcv.cnn import ConvModule
+from mmcv.runner import BaseModule
+from torch import nn as nn
+from torch.nn import functional as F
+
+
+class EdgeFusionModule(BaseModule):
+ """Edge Fusion Module for feature map.
+
+ Args:
+ out_channels (int): The number of output channels.
+ feat_channels (int): The number of channels in feature map
+ during edge feature fusion.
+ kernel_size (int, optional): Kernel size of convolution.
+ Default: 3.
+ act_cfg (dict, optional): Config of activation.
+ Default: dict(type='ReLU').
+ norm_cfg (dict, optional): Config of normalization.
+ Default: dict(type='BN1d')).
+ """
+
+ def __init__(self,
+ out_channels,
+ feat_channels,
+ kernel_size=3,
+ act_cfg=dict(type='ReLU'),
+ norm_cfg=dict(type='BN1d')):
+ super().__init__()
+ self.edge_convs = nn.Sequential(
+ ConvModule(
+ feat_channels,
+ feat_channels,
+ kernel_size=kernel_size,
+ padding=kernel_size // 2,
+ conv_cfg=dict(type='Conv1d'),
+ norm_cfg=norm_cfg,
+ act_cfg=act_cfg),
+ nn.Conv1d(feat_channels, out_channels, kernel_size=1))
+ self.feat_channels = feat_channels
+
+ def forward(self, features, fused_features, edge_indices, edge_lens,
+ output_h, output_w):
+ """Forward pass.
+
+ Args:
+ features (torch.Tensor): Different representative features
+ for fusion.
+ fused_features (torch.Tensor): Different representative
+ features to be fused.
+ edge_indices (torch.Tensor): Batch image edge indices.
+ edge_lens (list[int]): List of edge length of each image.
+ output_h (int): Height of output feature map.
+ output_w (int): Width of output feature map.
+
+ Returns:
+ torch.Tensor: Fused feature maps.
+ """
+ batch_size = features.shape[0]
+ # normalize
+ grid_edge_indices = edge_indices.view(batch_size, -1, 1, 2).float()
+ grid_edge_indices[..., 0] = \
+ grid_edge_indices[..., 0] / (output_w - 1) * 2 - 1
+ grid_edge_indices[..., 1] = \
+ grid_edge_indices[..., 1] / (output_h - 1) * 2 - 1
+
+ # apply edge fusion
+ edge_features = F.grid_sample(
+ features, grid_edge_indices, align_corners=True).squeeze(-1)
+ edge_output = self.edge_convs(edge_features)
+
+ for k in range(batch_size):
+ edge_indice_k = edge_indices[k, :edge_lens[k]]
+ fused_features[k, :, edge_indice_k[:, 1],
+ edge_indice_k[:, 0]] += edge_output[
+ k, :, :edge_lens[k]]
+
+ return fused_features
diff --git a/mmdet3d/models/model_utils/transformer.py b/mmdet3d/models/model_utils/transformer.py
new file mode 100644
index 0000000..4f9a833
--- /dev/null
+++ b/mmdet3d/models/model_utils/transformer.py
@@ -0,0 +1,139 @@
+# Copyright (c) OpenMMLab. All rights reserved.
+from mmcv.cnn.bricks.registry import ATTENTION
+from mmcv.cnn.bricks.transformer import POSITIONAL_ENCODING, MultiheadAttention
+from torch import nn as nn
+
+
+@ATTENTION.register_module()
+class GroupFree3DMHA(MultiheadAttention):
+ """A warpper for torch.nn.MultiheadAttention for GroupFree3D.
+
+ This module implements MultiheadAttention with identity connection,
+ and positional encoding used in DETR is also passed as input.
+
+ Args:
+ embed_dims (int): The embedding dimension.
+ num_heads (int): Parallel attention heads. Same as
+ `nn.MultiheadAttention`.
+ attn_drop (float, optional): A Dropout layer on attn_output_weights.
+ Defaults to 0.0.
+ proj_drop (float, optional): A Dropout layer. Defaults to 0.0.
+ dropout_layer (obj:`ConfigDict`, optional): The dropout_layer used
+ when adding the shortcut.
+ init_cfg (obj:`mmcv.ConfigDict`, optional): The Config for
+ initialization. Default: None.
+ batch_first (bool, optional): Key, Query and Value are shape of
+ (batch, n, embed_dim)
+ or (n, batch, embed_dim). Defaults to False.
+ """
+
+ def __init__(self,
+ embed_dims,
+ num_heads,
+ attn_drop=0.,
+ proj_drop=0.,
+ dropout_layer=dict(type='DropOut', drop_prob=0.),
+ init_cfg=None,
+ batch_first=False,
+ **kwargs):
+ super().__init__(embed_dims, num_heads, attn_drop, proj_drop,
+ dropout_layer, init_cfg, batch_first, **kwargs)
+
+ def forward(self,
+ query,
+ key,
+ value,
+ identity,
+ query_pos=None,
+ key_pos=None,
+ attn_mask=None,
+ key_padding_mask=None,
+ **kwargs):
+ """Forward function for `GroupFree3DMHA`.
+
+ **kwargs allow passing a more general data flow when combining
+ with other operations in `transformerlayer`.
+
+ Args:
+ query (Tensor): The input query with shape [num_queries, bs,
+ embed_dims]. Same in `nn.MultiheadAttention.forward`.
+ key (Tensor): The key tensor with shape [num_keys, bs,
+ embed_dims]. Same in `nn.MultiheadAttention.forward`.
+ If None, the ``query`` will be used.
+ value (Tensor): The value tensor with same shape as `key`.
+ Same in `nn.MultiheadAttention.forward`.
+ If None, the `key` will be used.
+ identity (Tensor): This tensor, with the same shape as x,
+ will be used for the identity link. If None, `x` will be used.
+ query_pos (Tensor, optional): The positional encoding for query,
+ with the same shape as `x`. Defaults to None.
+ If not None, it will be added to `x` before forward function.
+ key_pos (Tensor, optional): The positional encoding for `key`,
+ with the same shape as `key`. Defaults to None. If not None,
+ it will be added to `key` before forward function. If None,
+ and `query_pos` has the same shape as `key`, then `query_pos`
+ will be used for `key_pos`. Defaults to None.
+ attn_mask (Tensor, optional): ByteTensor mask with shape
+ [num_queries, num_keys].
+ Same in `nn.MultiheadAttention.forward`. Defaults to None.
+ key_padding_mask (Tensor, optional): ByteTensor with shape
+ [bs, num_keys]. Same in `nn.MultiheadAttention.forward`.
+ Defaults to None.
+
+ Returns:
+ Tensor: forwarded results with shape [num_queries, bs, embed_dims].
+ """
+
+ if hasattr(self, 'operation_name'):
+ if self.operation_name == 'self_attn':
+ value = value + query_pos
+ elif self.operation_name == 'cross_attn':
+ value = value + key_pos
+ else:
+ raise NotImplementedError(
+ f'{self.__class__.name} '
+ f"can't be used as {self.operation_name}")
+ else:
+ value = value + query_pos
+
+ return super(GroupFree3DMHA, self).forward(
+ query=query,
+ key=key,
+ value=value,
+ identity=identity,
+ query_pos=query_pos,
+ key_pos=key_pos,
+ attn_mask=attn_mask,
+ key_padding_mask=key_padding_mask,
+ **kwargs)
+
+
+@POSITIONAL_ENCODING.register_module()
+class ConvBNPositionalEncoding(nn.Module):
+ """Absolute position embedding with Conv learning.
+
+ Args:
+ input_channel (int): input features dim.
+ num_pos_feats (int, optional): output position features dim.
+ Defaults to 288 to be consistent with seed features dim.
+ """
+
+ def __init__(self, input_channel, num_pos_feats=288):
+ super().__init__()
+ self.position_embedding_head = nn.Sequential(
+ nn.Conv1d(input_channel, num_pos_feats, kernel_size=1),
+ nn.BatchNorm1d(num_pos_feats), nn.ReLU(inplace=True),
+ nn.Conv1d(num_pos_feats, num_pos_feats, kernel_size=1))
+
+ def forward(self, xyz):
+ """Forward pass.
+
+ Args:
+ xyz (Tensor): (B, N, 3) the coordinates to embed.
+
+ Returns:
+ Tensor: (B, num_pos_feats, N) the embedded position features.
+ """
+ xyz = xyz.permute(0, 2, 1)
+ position_embedding = self.position_embedding_head(xyz)
+ return position_embedding
diff --git a/mmdet3d/models/model_utils/vote_module.py b/mmdet3d/models/model_utils/vote_module.py
new file mode 100644
index 0000000..5cc52ad
--- /dev/null
+++ b/mmdet3d/models/model_utils/vote_module.py
@@ -0,0 +1,184 @@
+# Copyright (c) OpenMMLab. All rights reserved.
+import torch
+from mmcv import is_tuple_of
+from mmcv.cnn import ConvModule
+from torch import nn as nn
+
+from mmdet3d.models.builder import build_loss
+
+
+class VoteModule(nn.Module):
+ """Vote module.
+
+ Generate votes from seed point features.
+
+ Args:
+ in_channels (int): Number of channels of seed point features.
+ vote_per_seed (int, optional): Number of votes generated from
+ each seed point. Default: 1.
+ gt_per_seed (int, optional): Number of ground truth votes generated
+ from each seed point. Default: 3.
+ num_points (int, optional): Number of points to be used for voting.
+ Default: 1.
+ conv_channels (tuple[int], optional): Out channels of vote
+ generating convolution. Default: (16, 16).
+ conv_cfg (dict, optional): Config of convolution.
+ Default: dict(type='Conv1d').
+ norm_cfg (dict, optional): Config of normalization.
+ Default: dict(type='BN1d').
+ norm_feats (bool, optional): Whether to normalize features.
+ Default: True.
+ with_res_feat (bool, optional): Whether to predict residual features.
+ Default: True.
+ vote_xyz_range (list[float], optional):
+ The range of points translation. Default: None.
+ vote_loss (dict, optional): Config of vote loss. Default: None.
+ """
+
+ def __init__(self,
+ in_channels,
+ vote_per_seed=1,
+ gt_per_seed=3,
+ num_points=-1,
+ conv_channels=(16, 16),
+ conv_cfg=dict(type='Conv1d'),
+ norm_cfg=dict(type='BN1d'),
+ act_cfg=dict(type='ReLU'),
+ norm_feats=True,
+ with_res_feat=True,
+ vote_xyz_range=None,
+ vote_loss=None):
+ super().__init__()
+ self.in_channels = in_channels
+ self.vote_per_seed = vote_per_seed
+ self.gt_per_seed = gt_per_seed
+ self.num_points = num_points
+ self.norm_feats = norm_feats
+ self.with_res_feat = with_res_feat
+
+ assert vote_xyz_range is None or is_tuple_of(vote_xyz_range, float)
+ self.vote_xyz_range = vote_xyz_range
+
+ if vote_loss is not None:
+ self.vote_loss = build_loss(vote_loss)
+
+ prev_channels = in_channels
+ vote_conv_list = list()
+ for k in range(len(conv_channels)):
+ vote_conv_list.append(
+ ConvModule(
+ prev_channels,
+ conv_channels[k],
+ 1,
+ padding=0,
+ conv_cfg=conv_cfg,
+ norm_cfg=norm_cfg,
+ act_cfg=act_cfg,
+ bias=True,
+ inplace=True))
+ prev_channels = conv_channels[k]
+ self.vote_conv = nn.Sequential(*vote_conv_list)
+
+ # conv_out predicts coordinate and residual features
+ if with_res_feat:
+ out_channel = (3 + in_channels) * self.vote_per_seed
+ else:
+ out_channel = 3 * self.vote_per_seed
+ self.conv_out = nn.Conv1d(prev_channels, out_channel, 1)
+
+ def forward(self, seed_points, seed_feats):
+ """forward.
+
+ Args:
+ seed_points (torch.Tensor): Coordinate of the seed
+ points in shape (B, N, 3).
+ seed_feats (torch.Tensor): Features of the seed points in shape
+ (B, C, N).
+
+ Returns:
+ tuple[torch.Tensor]:
+
+ - vote_points: Voted xyz based on the seed points
+ with shape (B, M, 3), ``M=num_seed*vote_per_seed``.
+ - vote_features: Voted features based on the seed points with
+ shape (B, C, M) where ``M=num_seed*vote_per_seed``,
+ ``C=vote_feature_dim``.
+ """
+ if self.num_points != -1:
+ assert self.num_points < seed_points.shape[1], \
+ f'Number of vote points ({self.num_points}) should be '\
+ f'smaller than seed points size ({seed_points.shape[1]})'
+ seed_points = seed_points[:, :self.num_points]
+ seed_feats = seed_feats[..., :self.num_points]
+
+ batch_size, feat_channels, num_seed = seed_feats.shape
+ num_vote = num_seed * self.vote_per_seed
+ x = self.vote_conv(seed_feats)
+ # (batch_size, (3+out_dim)*vote_per_seed, num_seed)
+ votes = self.conv_out(x)
+
+ votes = votes.transpose(2, 1).view(batch_size, num_seed,
+ self.vote_per_seed, -1)
+
+ offset = votes[:, :, :, 0:3]
+ if self.vote_xyz_range is not None:
+ limited_offset_list = []
+ for axis in range(len(self.vote_xyz_range)):
+ limited_offset_list.append(offset[..., axis].clamp(
+ min=-self.vote_xyz_range[axis],
+ max=self.vote_xyz_range[axis]))
+ limited_offset = torch.stack(limited_offset_list, -1)
+ vote_points = (seed_points.unsqueeze(2) +
+ limited_offset).contiguous()
+ else:
+ vote_points = (seed_points.unsqueeze(2) + offset).contiguous()
+ vote_points = vote_points.view(batch_size, num_vote, 3)
+ offset = offset.reshape(batch_size, num_vote, 3).transpose(2, 1)
+
+ if self.with_res_feat:
+ res_feats = votes[:, :, :, 3:]
+ vote_feats = (seed_feats.transpose(2, 1).unsqueeze(2) +
+ res_feats).contiguous()
+ vote_feats = vote_feats.view(batch_size,
+ num_vote, feat_channels).transpose(
+ 2, 1).contiguous()
+
+ if self.norm_feats:
+ features_norm = torch.norm(vote_feats, p=2, dim=1)
+ vote_feats = vote_feats.div(features_norm.unsqueeze(1))
+ else:
+ vote_feats = seed_feats
+ return vote_points, vote_feats, offset
+
+ def get_loss(self, seed_points, vote_points, seed_indices,
+ vote_targets_mask, vote_targets):
+ """Calculate loss of voting module.
+
+ Args:
+ seed_points (torch.Tensor): Coordinate of the seed points.
+ vote_points (torch.Tensor): Coordinate of the vote points.
+ seed_indices (torch.Tensor): Indices of seed points in raw points.
+ vote_targets_mask (torch.Tensor): Mask of valid vote targets.
+ vote_targets (torch.Tensor): Targets of votes.
+
+ Returns:
+ torch.Tensor: Weighted vote loss.
+ """
+ batch_size, num_seed = seed_points.shape[:2]
+
+ seed_gt_votes_mask = torch.gather(vote_targets_mask, 1,
+ seed_indices).float()
+
+ seed_indices_expand = seed_indices.unsqueeze(-1).repeat(
+ 1, 1, 3 * self.gt_per_seed)
+ seed_gt_votes = torch.gather(vote_targets, 1, seed_indices_expand)
+ seed_gt_votes += seed_points.repeat(1, 1, self.gt_per_seed)
+
+ weight = seed_gt_votes_mask / (torch.sum(seed_gt_votes_mask) + 1e-6)
+ distance = self.vote_loss(
+ vote_points.view(batch_size * num_seed, -1, 3),
+ seed_gt_votes.view(batch_size * num_seed, -1, 3),
+ dst_weight=weight.view(batch_size * num_seed, 1))[1]
+ vote_loss = torch.sum(torch.min(distance, dim=1)[0])
+
+ return vote_loss
diff --git a/mmdet3d/models/necks/__init__.py b/mmdet3d/models/necks/__init__.py
new file mode 100644
index 0000000..d1472a6
--- /dev/null
+++ b/mmdet3d/models/necks/__init__.py
@@ -0,0 +1,12 @@
+# Copyright (c) OpenMMLab. All rights reserved.
+from mmdet.models.necks.fpn import FPN
+from .dla_neck import DLANeck
+from .imvoxel_neck import OutdoorImVoxelNeck
+from .ngfc_neck import NgfcNeck, NgfcTinyNeck, NgfcTinySegmentationNeck
+from .pointnet2_fp_neck import PointNetFPNeck
+from .second_fpn import SECONDFPN
+
+__all__ = [
+ 'FPN', 'SECONDFPN', 'OutdoorImVoxelNeck', 'PointNetFPNeck', 'DLANeck',
+ 'NgfcNeck', 'NgfcTinyNeck', 'NgfcTinySegmentationNeck'
+]
diff --git a/mmdet3d/models/necks/dla_neck.py b/mmdet3d/models/necks/dla_neck.py
new file mode 100644
index 0000000..c32e8bb
--- /dev/null
+++ b/mmdet3d/models/necks/dla_neck.py
@@ -0,0 +1,233 @@
+# Copyright (c) OpenMMLab. All rights reserved.
+import math
+
+import numpy as np
+from mmcv.cnn import ConvModule, build_conv_layer
+from mmcv.runner import BaseModule
+from torch import nn as nn
+
+from ..builder import NECKS
+
+
+def fill_up_weights(up):
+ """Simulated bilinear upsampling kernel.
+
+ Args:
+ up (nn.Module): ConvTranspose2d module.
+ """
+ w = up.weight.data
+ f = math.ceil(w.size(2) / 2)
+ c = (2 * f - 1 - f % 2) / (2. * f)
+ for i in range(w.size(2)):
+ for j in range(w.size(3)):
+ w[0, 0, i, j] = \
+ (1 - math.fabs(i / f - c)) * (1 - math.fabs(j / f - c))
+ for c in range(1, w.size(0)):
+ w[c, 0, :, :] = w[0, 0, :, :]
+
+
+class IDAUpsample(BaseModule):
+ """Iterative Deep Aggregation (IDA) Upsampling module to upsample features
+ of different scales to a similar scale.
+
+ Args:
+ out_channels (int): Number of output channels for DeformConv.
+ in_channels (List[int]): List of input channels of multi-scale
+ feature maps.
+ kernel_sizes (List[int]): List of size of the convolving
+ kernel of different scales.
+ norm_cfg (dict, optional): Config dict for normalization layer.
+ Default: None.
+ use_dcn (bool, optional): If True, use DCNv2. Default: True.
+ """
+
+ def __init__(
+ self,
+ out_channels,
+ in_channels,
+ kernel_sizes,
+ norm_cfg=None,
+ use_dcn=True,
+ init_cfg=None,
+ ):
+ super(IDAUpsample, self).__init__(init_cfg)
+ self.use_dcn = use_dcn
+ self.projs = nn.ModuleList()
+ self.ups = nn.ModuleList()
+ self.nodes = nn.ModuleList()
+
+ for i in range(1, len(in_channels)):
+ in_channel = in_channels[i]
+ up_kernel_size = int(kernel_sizes[i])
+ proj = ConvModule(
+ in_channel,
+ out_channels,
+ 3,
+ padding=1,
+ bias=True,
+ conv_cfg=dict(type='DCNv2') if self.use_dcn else None,
+ norm_cfg=norm_cfg)
+ node = ConvModule(
+ out_channels,
+ out_channels,
+ 3,
+ padding=1,
+ bias=True,
+ conv_cfg=dict(type='DCNv2') if self.use_dcn else None,
+ norm_cfg=norm_cfg)
+ up = build_conv_layer(
+ dict(type='deconv'),
+ out_channels,
+ out_channels,
+ up_kernel_size * 2,
+ stride=up_kernel_size,
+ padding=up_kernel_size // 2,
+ output_padding=0,
+ groups=out_channels,
+ bias=False)
+
+ self.projs.append(proj)
+ self.ups.append(up)
+ self.nodes.append(node)
+
+ def forward(self, mlvl_features, start_level, end_level):
+ """Forward function.
+
+ Args:
+ mlvl_features (list[torch.Tensor]): Features from multiple layers.
+ start_level (int): Start layer for feature upsampling.
+ end_level (int): End layer for feature upsampling.
+ """
+ for i in range(start_level, end_level - 1):
+ upsample = self.ups[i - start_level]
+ project = self.projs[i - start_level]
+ mlvl_features[i + 1] = upsample(project(mlvl_features[i + 1]))
+ node = self.nodes[i - start_level]
+ mlvl_features[i + 1] = node(mlvl_features[i + 1] +
+ mlvl_features[i])
+
+
+class DLAUpsample(BaseModule):
+ """Deep Layer Aggregation (DLA) Upsampling module for different scales
+ feature extraction, upsampling and fusion, It consists of groups of
+ IDAupsample modules.
+
+ Args:
+ start_level (int): The start layer.
+ channels (List[int]): List of input channels of multi-scale
+ feature maps.
+ scales(List[int]): List of scale of different layers' feature.
+ in_channels (NoneType, optional): List of input channels of
+ different scales. Default: None.
+ norm_cfg (dict, optional): Config dict for normalization layer.
+ Default: None.
+ use_dcn (bool, optional): Whether to use dcn in IDAup module.
+ Default: True.
+ """
+
+ def __init__(self,
+ start_level,
+ channels,
+ scales,
+ in_channels=None,
+ norm_cfg=None,
+ use_dcn=True,
+ init_cfg=None):
+ super(DLAUpsample, self).__init__(init_cfg)
+ self.start_level = start_level
+ if in_channels is None:
+ in_channels = channels
+ self.channels = channels
+ channels = list(channels)
+ scales = np.array(scales, dtype=int)
+ for i in range(len(channels) - 1):
+ j = -i - 2
+ setattr(
+ self, 'ida_{}'.format(i),
+ IDAUpsample(channels[j], in_channels[j:],
+ scales[j:] // scales[j], norm_cfg, use_dcn))
+ scales[j + 1:] = scales[j]
+ in_channels[j + 1:] = [channels[j] for _ in channels[j + 1:]]
+
+ def forward(self, mlvl_features):
+ """Forward function.
+
+ Args:
+ mlvl_features(list[torch.Tensor]): Features from multi-scale
+ layers.
+
+ Returns:
+ tuple[torch.Tensor]: Up-sampled features of different layers.
+ """
+ outs = [mlvl_features[-1]]
+ for i in range(len(mlvl_features) - self.start_level - 1):
+ ida = getattr(self, 'ida_{}'.format(i))
+ ida(mlvl_features, len(mlvl_features) - i - 2, len(mlvl_features))
+ outs.insert(0, mlvl_features[-1])
+ return outs
+
+
+@NECKS.register_module()
+class DLANeck(BaseModule):
+ """DLA Neck.
+
+ Args:
+ in_channels (list[int], optional): List of input channels
+ of multi-scale feature map.
+ start_level (int, optional): The scale level where upsampling
+ starts. Default: 2.
+ end_level (int, optional): The scale level where upsampling
+ ends. Default: 5.
+ norm_cfg (dict, optional): Config dict for normalization
+ layer. Default: None.
+ use_dcn (bool, optional): Whether to use dcn in IDAup module.
+ Default: True.
+ """
+
+ def __init__(self,
+ in_channels=[16, 32, 64, 128, 256, 512],
+ start_level=2,
+ end_level=5,
+ norm_cfg=None,
+ use_dcn=True,
+ init_cfg=None):
+ super(DLANeck, self).__init__(init_cfg)
+ self.start_level = start_level
+ self.end_level = end_level
+ scales = [2**i for i in range(len(in_channels[self.start_level:]))]
+ self.dla_up = DLAUpsample(
+ start_level=self.start_level,
+ channels=in_channels[self.start_level:],
+ scales=scales,
+ norm_cfg=norm_cfg,
+ use_dcn=use_dcn)
+ self.ida_up = IDAUpsample(
+ in_channels[self.start_level],
+ in_channels[self.start_level:self.end_level],
+ [2**i for i in range(self.end_level - self.start_level)], norm_cfg,
+ use_dcn)
+
+ def forward(self, x):
+ mlvl_features = [x[i] for i in range(len(x))]
+ mlvl_features = self.dla_up(mlvl_features)
+ outs = []
+ for i in range(self.end_level - self.start_level):
+ outs.append(mlvl_features[i].clone())
+ self.ida_up(outs, 0, len(outs))
+ return [outs[-1]]
+
+ def init_weights(self):
+ for m in self.modules():
+ if isinstance(m, nn.ConvTranspose2d):
+ # In order to be consistent with the source code,
+ # reset the ConvTranspose2d initialization parameters
+ m.reset_parameters()
+ # Simulated bilinear upsampling kernel
+ fill_up_weights(m)
+ elif isinstance(m, (nn.BatchNorm2d, nn.GroupNorm)):
+ nn.init.constant_(m.weight, 1)
+ nn.init.constant_(m.bias, 0)
+ elif isinstance(m, nn.Conv2d):
+ # In order to be consistent with the source code,
+ # reset the Conv2d initialization parameters
+ m.reset_parameters()
diff --git a/mmdet3d/models/necks/imvoxel_neck.py b/mmdet3d/models/necks/imvoxel_neck.py
new file mode 100644
index 0000000..8881491
--- /dev/null
+++ b/mmdet3d/models/necks/imvoxel_neck.py
@@ -0,0 +1,110 @@
+# Copyright (c) OpenMMLab. All rights reserved.
+from mmcv.cnn import ConvModule
+from torch import nn
+
+from ..builder import NECKS
+
+
+@NECKS.register_module()
+class OutdoorImVoxelNeck(nn.Module):
+ """Neck for ImVoxelNet outdoor scenario.
+
+ Args:
+ in_channels (int): Input channels of multi-scale feature map.
+ out_channels (int): Output channels of multi-scale feature map.
+ """
+
+ def __init__(self, in_channels, out_channels):
+ super().__init__()
+ self.model = nn.Sequential(
+ ResModule(in_channels),
+ ConvModule(
+ in_channels=in_channels,
+ out_channels=in_channels * 2,
+ kernel_size=3,
+ stride=(1, 1, 2),
+ padding=1,
+ conv_cfg=dict(type='Conv3d'),
+ norm_cfg=dict(type='BN3d'),
+ act_cfg=dict(type='ReLU', inplace=True)),
+ ResModule(in_channels * 2),
+ ConvModule(
+ in_channels=in_channels * 2,
+ out_channels=in_channels * 4,
+ kernel_size=3,
+ stride=(1, 1, 2),
+ padding=1,
+ conv_cfg=dict(type='Conv3d'),
+ norm_cfg=dict(type='BN3d'),
+ act_cfg=dict(type='ReLU', inplace=True)),
+ ResModule(in_channels * 4),
+ ConvModule(
+ in_channels=in_channels * 4,
+ out_channels=out_channels,
+ kernel_size=3,
+ padding=(1, 1, 0),
+ conv_cfg=dict(type='Conv3d'),
+ norm_cfg=dict(type='BN3d'),
+ act_cfg=dict(type='ReLU', inplace=True)))
+
+ def forward(self, x):
+ """Forward function.
+
+ Args:
+ x (torch.Tensor): of shape (N, C_in, N_x, N_y, N_z).
+
+ Returns:
+ list[torch.Tensor]: of shape (N, C_out, N_y, N_x).
+ """
+ x = self.model.forward(x)
+ assert x.shape[-1] == 1
+ # Anchor3DHead axis order is (y, x).
+ return [x[..., 0].transpose(-1, -2)]
+
+ def init_weights(self):
+ """Initialize weights of neck."""
+ pass
+
+
+class ResModule(nn.Module):
+ """3d residual block for ImVoxelNeck.
+
+ Args:
+ n_channels (int): Input channels of a feature map.
+ """
+
+ def __init__(self, n_channels):
+ super().__init__()
+ self.conv0 = ConvModule(
+ in_channels=n_channels,
+ out_channels=n_channels,
+ kernel_size=3,
+ padding=1,
+ conv_cfg=dict(type='Conv3d'),
+ norm_cfg=dict(type='BN3d'),
+ act_cfg=dict(type='ReLU', inplace=True))
+ self.conv1 = ConvModule(
+ in_channels=n_channels,
+ out_channels=n_channels,
+ kernel_size=3,
+ padding=1,
+ conv_cfg=dict(type='Conv3d'),
+ norm_cfg=dict(type='BN3d'),
+ act_cfg=None)
+ self.activation = nn.ReLU(inplace=True)
+
+ def forward(self, x):
+ """Forward function.
+
+ Args:
+ x (torch.Tensor): of shape (N, C, N_x, N_y, N_z).
+
+ Returns:
+ torch.Tensor: 5d feature map.
+ """
+ identity = x
+ x = self.conv0(x)
+ x = self.conv1(x)
+ x = identity + x
+ x = self.activation(x)
+ return x
diff --git a/mmdet3d/models/necks/ngfc_neck.py b/mmdet3d/models/necks/ngfc_neck.py
new file mode 100644
index 0000000..5b2e63c
--- /dev/null
+++ b/mmdet3d/models/necks/ngfc_neck.py
@@ -0,0 +1,268 @@
+try:
+ import MinkowskiEngine as ME
+ from MinkowskiEngine.modules.resnet_block import BasicBlock
+except ImportError:
+ import warnings
+ warnings.warn(
+ 'Please follow `getting_started.md` to install MinkowskiEngine.`')
+
+from torch import nn
+
+from mmcv.runner import BaseModule
+from mmdet3d.models.builder import NECKS
+
+
+@NECKS.register_module()
+class NgfcNeck(BaseModule):
+ def __init__(self, in_channels):
+ super(NgfcNeck, self).__init__()
+ self._init_layers(in_channels)
+
+ def _init_layers(self, in_channels):
+ for i in range(len(in_channels)):
+ if i > 0:
+ self.__setattr__(f'up_block_{i}', make_up_block(in_channels[i], in_channels[i - 1]))
+ if i < len(in_channels) - 1:
+ self.__setattr__(f'lateral_block_{i}',
+ make_block(in_channels[i], in_channels[i]))
+
+ def init_weights(self):
+ for m in self.modules():
+ if isinstance(m, ME.MinkowskiConvolution):
+ ME.utils.kaiming_normal_(
+ m.kernel, mode='fan_out', nonlinearity='relu')
+
+ if isinstance(m, ME.MinkowskiBatchNorm):
+ nn.init.constant_(m.bn.weight, 1)
+ nn.init.constant_(m.bn.bias, 0)
+
+ def forward(self, x):
+ inputs = x
+ x = inputs[-1]
+ for i in range(len(inputs) - 1, -1, -1):
+ if i < len(inputs) - 1:
+ x = self.__getattr__(f'up_block_{i + 1}')(x)
+ # print('NgfcNeck', i, x.features.shape, inputs[i].features.shape)
+ x = inputs[i] + x
+ x = self.__getattr__(f'lateral_block_{i}')(x)
+ return x
+
+
+@NECKS.register_module()
+class NgfcTinyNeck(BaseModule):
+ def __init__(self, in_channels, out_channels):
+ super(NgfcTinyNeck, self).__init__()
+ self._init_layers(in_channels, out_channels)
+
+ def _init_layers(self, in_channels, out_channels):
+ for i in range(len(in_channels)):
+ if i > 0:
+ self.__setattr__(
+ f'up_block_{i}',
+ make_up_block(in_channels[i], in_channels[i - 1]))
+ if i < len(in_channels) - 1:
+ self.__setattr__(
+ f'lateral_block_{i}',
+ make_block(in_channels[i], in_channels[i]))
+ self.__setattr__(
+ f'out_block_{i}',
+ make_block(in_channels[i], out_channels))
+
+ def init_weights(self):
+ for m in self.modules():
+ if isinstance(m, ME.MinkowskiConvolution):
+ ME.utils.kaiming_normal_(
+ m.kernel, mode='fan_out', nonlinearity='relu')
+
+ if isinstance(m, ME.MinkowskiBatchNorm):
+ nn.init.constant_(m.bn.weight, 1)
+ nn.init.constant_(m.bn.bias, 0)
+
+ def forward(self, x):
+ outs = []
+ inputs = x
+ x = inputs[-1]
+ for i in range(len(inputs) - 1, -1, -1):
+ if i < len(inputs) - 1:
+ x = self.__getattr__(f'up_block_{i + 1}')(x)
+ # print('NgfcTinyNeck', i, x.features.shape, inputs[i].features.shape)
+ x = inputs[i] + x
+ x = self.__getattr__(f'lateral_block_{i}')(x)
+ out = self.__getattr__(f'out_block_{i}')(x)
+ outs.append(out)
+ return outs[::-1]
+
+
+@NECKS.register_module()
+class NgfcTinySegmentationNeck(BaseModule):
+ def __init__(self, in_channels, out_channels):
+ super(NgfcTinySegmentationNeck, self).__init__()
+ self._init_layers(in_channels, out_channels)
+
+ self.upsample_st_4 = nn.Sequential(
+ ME.MinkowskiConvolutionTranspose(
+ 128,
+ 64,
+ kernel_size=3,
+ stride=4,
+ dimension=3),
+ ME.MinkowskiBatchNorm(64),
+ ME.MinkowskiReLU(inplace=True))
+
+ self.conv_32_ch = nn.Sequential(
+ ME.MinkowskiConvolution(
+ 64,
+ 32,
+ kernel_size=3,
+ stride=1,
+ dimension=3),
+ ME.MinkowskiBatchNorm(32),
+ ME.MinkowskiReLU(inplace=True))
+
+ def _init_layers(self, in_channels, out_channels):
+ for i in range(len(in_channels)):
+ if i > 0:
+ self.__setattr__(
+ f'up_block_{i}',
+ make_up_block(in_channels[i], in_channels[i - 1]))
+ if i < len(in_channels) - 1:
+ self.__setattr__(
+ f'lateral_block_{i}',
+ make_block(in_channels[i], in_channels[i]))
+ self.__setattr__(
+ f'out_block_{i}',
+ make_block(in_channels[i], out_channels))
+
+ def init_weights(self):
+ for m in self.modules():
+ if isinstance(m, ME.MinkowskiConvolution):
+ ME.utils.kaiming_normal_(
+ m.kernel, mode='fan_out', nonlinearity='relu')
+
+ if isinstance(m, ME.MinkowskiBatchNorm):
+ nn.init.constant_(m.bn.weight, 1)
+ nn.init.constant_(m.bn.bias, 0)
+
+ def forward(self, x):
+ feats_st_2 = x[0]
+ outs = []
+ inputs = x[1:]
+ x = inputs[-1]
+ for i in range(len(inputs) - 1, -1, -1):
+ if i < len(inputs) - 1:
+ x = self.__getattr__(f'up_block_{i + 1}')(x)
+ x = inputs[i] + x
+ x = self.__getattr__(f'lateral_block_{i}')(x)
+ out = self.__getattr__(f'out_block_{i}')(x)
+ outs.append(out)
+
+ outs = outs[::-1]
+
+ seg_feats = self.conv_32_ch(self.upsample_st_4(outs[0]) + feats_st_2)
+ return [seg_feats] + outs
+
+
+class BiFPNLayer(BaseModule):
+ def __init__(self, n_channels, n_levels):
+ super(BiFPNLayer, self).__init__()
+ self._init_layers(n_channels, n_levels)
+
+ def _init_layers(self, n_channels, n_levels):
+ for i in range(n_levels):
+ if i > 0:
+ self.__setattr__(
+ f'up_block_{i}',
+ make_up_block(n_channels, n_channels))
+ self.__setattr__(
+ f'down_block_{i}',
+ make_up_block(n_channels, n_channels))
+
+ def init_weights(self):
+ for m in self.modules():
+ if isinstance(m, ME.MinkowskiConvolution):
+ ME.utils.kaiming_normal_(
+ m.kernel, mode='fan_out', nonlinearity='relu')
+
+ if isinstance(m, ME.MinkowskiBatchNorm):
+ nn.init.constant_(m.bn.weight, 1)
+ nn.init.constant_(m.bn.bias, 0)
+
+ def forward(self, x):
+ x1s = []
+ inputs = x
+ x = inputs[-1]
+ for i in range(len(inputs) - 1, -1, -1):
+ if i < len(inputs) - 1:
+ x = self.__getattr__(f'up_block_{i + 1}')(x)
+ x = inputs[i] + x
+ x1s.append(x)
+ x1s = x1s[::-1]
+ x2s = [x]
+ for i in range(1, len(inputs)):
+ x = self.__getattr__(f'down_block_{i}')(x)
+ x = x + inputs[i]
+ if i < len(inputs) - 1:
+ x = x + x1s[i]
+ x2s.append(x)
+ return x2s
+
+
+@NECKS.register_module()
+class BiFPNNeck(BaseModule):
+ def __init__(self, in_channels, out_channels, n_blocks):
+ super(BiFPNNeck, self).__init__()
+ self.n_levels = len(in_channels)
+ self.n_blocks = n_blocks
+ self._init_layers(in_channels, out_channels, n_blocks)
+
+ def _init_layers(self, in_channels, out_channels):
+ for i in range(len(in_channels)):
+ self.__setattr__(
+ f'in_block_{i}',
+ make_block(in_channels[i], out_channels, 1))
+ for i in range(self.n_blocks):
+ self.__setattr__(
+ f'block_{i}',
+ BiFPNLayer(out_channels, self.n_levels))
+
+
+ def init_weights(self):
+ for m in self.modules():
+ if isinstance(m, ME.MinkowskiConvolution):
+ ME.utils.kaiming_normal_(
+ m.kernel, mode='fan_out', nonlinearity='relu')
+
+ if isinstance(m, ME.MinkowskiBatchNorm):
+ nn.init.constant_(m.bn.weight, 1)
+ nn.init.constant_(m.bn.bias, 0)
+
+ def forward(self, x):
+ pass # todo: !!!!!!!!!!!!!!!!!!!!!!!!!!!!!
+
+
+def make_block(in_channels, out_channels, kernel_size=3):
+ return nn.Sequential(
+ ME.MinkowskiConvolution(in_channels, out_channels,
+ kernel_size=kernel_size, dimension=3),
+ ME.MinkowskiBatchNorm(out_channels),
+ ME.MinkowskiReLU(inplace=True))
+
+
+def make_down_block(in_channels, out_channels):
+ return nn.Sequential(
+ ME.MinkowskiConvolution(in_channels, out_channels, kernel_size=3,
+ stride=2, dimension=3),
+ ME.MinkowskiBatchNorm(out_channels),
+ ME.MinkowskiReLU(inplace=True))
+
+
+def make_up_block(in_channels, out_channels):
+ return nn.Sequential(
+ ME.MinkowskiConvolutionTranspose(
+ in_channels,
+ out_channels,
+ kernel_size=3,
+ stride=2,
+ dimension=3),
+ ME.MinkowskiBatchNorm(out_channels),
+ ME.MinkowskiReLU(inplace=True))
diff --git a/mmdet3d/models/necks/pointnet2_fp_neck.py b/mmdet3d/models/necks/pointnet2_fp_neck.py
new file mode 100644
index 0000000..62db0c1
--- /dev/null
+++ b/mmdet3d/models/necks/pointnet2_fp_neck.py
@@ -0,0 +1,89 @@
+# Copyright (c) OpenMMLab. All rights reserved.
+from mmcv.runner import BaseModule
+from torch import nn as nn
+
+from mmdet3d.ops import PointFPModule
+from ..builder import NECKS
+
+
+@NECKS.register_module()
+class PointNetFPNeck(BaseModule):
+ r"""PointNet FP Module used in PointRCNN.
+
+ Refer to the `official code `_.
+
+ .. code-block:: none
+
+ sa_n ----------------------------------------
+ |
+ ... --------------------------------- |
+ | |
+ sa_1 ------------- | |
+ | | |
+ sa_0 -> fp_0 -> fp_module ->fp_1 -> ... -> fp_module -> fp_n
+
+ sa_n including sa_xyz (torch.Tensor) and sa_features (torch.Tensor)
+ fp_n including fp_xyz (torch.Tensor) and fp_features (torch.Tensor)
+
+ Args:
+ fp_channels (tuple[tuple[int]]): Tuple of mlp channels in FP modules.
+ init_cfg (dict or list[dict], optional): Initialization config dict.
+ Default: None
+ """
+
+ def __init__(self, fp_channels, init_cfg=None):
+ super(PointNetFPNeck, self).__init__(init_cfg=init_cfg)
+
+ self.num_fp = len(fp_channels)
+ self.FP_modules = nn.ModuleList()
+ for cur_fp_mlps in fp_channels:
+ self.FP_modules.append(PointFPModule(mlp_channels=cur_fp_mlps))
+
+ def _extract_input(self, feat_dict):
+ """Extract inputs from features dictionary.
+
+ Args:
+ feat_dict (dict): Feature dict from backbone, which may contain
+ the following keys and values:
+
+ - sa_xyz (list[torch.Tensor]): Points of each sa module
+ in shape (N, 3).
+ - sa_features (list[torch.Tensor]): Output features of
+ each sa module in shape (N, M).
+
+ Returns:
+ list[torch.Tensor]: Coordinates of multiple levels of points.
+ list[torch.Tensor]: Features of multiple levels of points.
+ """
+ sa_xyz = feat_dict['sa_xyz']
+ sa_features = feat_dict['sa_features']
+ assert len(sa_xyz) == len(sa_features)
+
+ return sa_xyz, sa_features
+
+ def forward(self, feat_dict):
+ """Forward pass.
+
+ Args:
+ feat_dict (dict): Feature dict from backbone.
+
+ Returns:
+ dict[str, torch.Tensor]: Outputs of the Neck.
+
+ - fp_xyz (torch.Tensor): The coordinates of fp features.
+ - fp_features (torch.Tensor): The features from the last
+ feature propagation layers.
+ """
+ sa_xyz, sa_features = self._extract_input(feat_dict)
+
+ fp_feature = sa_features[-1]
+ fp_xyz = sa_xyz[-1]
+
+ for i in range(self.num_fp):
+ # consume the points in a bottom-up manner
+ fp_feature = self.FP_modules[i](sa_xyz[-(i + 2)], sa_xyz[-(i + 1)],
+ sa_features[-(i + 2)], fp_feature)
+ fp_xyz = sa_xyz[-(i + 2)]
+
+ ret = dict(fp_xyz=fp_xyz, fp_features=fp_feature)
+ return ret
diff --git a/mmdet3d/models/necks/second_fpn.py b/mmdet3d/models/necks/second_fpn.py
new file mode 100644
index 0000000..ef1b3de
--- /dev/null
+++ b/mmdet3d/models/necks/second_fpn.py
@@ -0,0 +1,91 @@
+# Copyright (c) OpenMMLab. All rights reserved.
+import numpy as np
+import torch
+from mmcv.cnn import build_conv_layer, build_norm_layer, build_upsample_layer
+from mmcv.runner import BaseModule, auto_fp16
+from torch import nn as nn
+
+from ..builder import NECKS
+
+
+@NECKS.register_module()
+class SECONDFPN(BaseModule):
+ """FPN used in SECOND/PointPillars/PartA2/MVXNet.
+
+ Args:
+ in_channels (list[int]): Input channels of multi-scale feature maps.
+ out_channels (list[int]): Output channels of feature maps.
+ upsample_strides (list[int]): Strides used to upsample the
+ feature maps.
+ norm_cfg (dict): Config dict of normalization layers.
+ upsample_cfg (dict): Config dict of upsample layers.
+ conv_cfg (dict): Config dict of conv layers.
+ use_conv_for_no_stride (bool): Whether to use conv when stride is 1.
+ """
+
+ def __init__(self,
+ in_channels=[128, 128, 256],
+ out_channels=[256, 256, 256],
+ upsample_strides=[1, 2, 4],
+ norm_cfg=dict(type='BN', eps=1e-3, momentum=0.01),
+ upsample_cfg=dict(type='deconv', bias=False),
+ conv_cfg=dict(type='Conv2d', bias=False),
+ use_conv_for_no_stride=False,
+ init_cfg=None):
+ # if for GroupNorm,
+ # cfg is dict(type='GN', num_groups=num_groups, eps=1e-3, affine=True)
+ super(SECONDFPN, self).__init__(init_cfg=init_cfg)
+ assert len(out_channels) == len(upsample_strides) == len(in_channels)
+ self.in_channels = in_channels
+ self.out_channels = out_channels
+ self.fp16_enabled = False
+
+ deblocks = []
+ for i, out_channel in enumerate(out_channels):
+ stride = upsample_strides[i]
+ if stride > 1 or (stride == 1 and not use_conv_for_no_stride):
+ upsample_layer = build_upsample_layer(
+ upsample_cfg,
+ in_channels=in_channels[i],
+ out_channels=out_channel,
+ kernel_size=upsample_strides[i],
+ stride=upsample_strides[i])
+ else:
+ stride = np.round(1 / stride).astype(np.int64)
+ upsample_layer = build_conv_layer(
+ conv_cfg,
+ in_channels=in_channels[i],
+ out_channels=out_channel,
+ kernel_size=stride,
+ stride=stride)
+
+ deblock = nn.Sequential(upsample_layer,
+ build_norm_layer(norm_cfg, out_channel)[1],
+ nn.ReLU(inplace=True))
+ deblocks.append(deblock)
+ self.deblocks = nn.ModuleList(deblocks)
+
+ if init_cfg is None:
+ self.init_cfg = [
+ dict(type='Kaiming', layer='ConvTranspose2d'),
+ dict(type='Constant', layer='NaiveSyncBatchNorm2d', val=1.0)
+ ]
+
+ @auto_fp16()
+ def forward(self, x):
+ """Forward function.
+
+ Args:
+ x (torch.Tensor): 4D Tensor in (N, C, H, W) shape.
+
+ Returns:
+ list[torch.Tensor]: Multi-level feature maps.
+ """
+ assert len(x) == len(self.in_channels)
+ ups = [deblock(x[i]) for i, deblock in enumerate(self.deblocks)]
+
+ if len(ups) > 1:
+ out = torch.cat(ups, dim=1)
+ else:
+ out = ups[0]
+ return [out]
diff --git a/mmdet3d/models/roi_heads/__init__.py b/mmdet3d/models/roi_heads/__init__.py
new file mode 100644
index 0000000..e607570
--- /dev/null
+++ b/mmdet3d/models/roi_heads/__init__.py
@@ -0,0 +1,14 @@
+# Copyright (c) OpenMMLab. All rights reserved.
+from .base_3droi_head import Base3DRoIHead
+from .bbox_heads import PartA2BboxHead
+from .h3d_roi_head import H3DRoIHead
+from .mask_heads import PointwiseSemanticHead, PrimitiveHead
+from .part_aggregation_roi_head import PartAggregationROIHead
+from .point_rcnn_roi_head import PointRCNNRoIHead
+from .roi_extractors import Single3DRoIAwareExtractor, SingleRoIExtractor
+
+__all__ = [
+ 'Base3DRoIHead', 'PartAggregationROIHead', 'PointwiseSemanticHead',
+ 'Single3DRoIAwareExtractor', 'PartA2BboxHead', 'SingleRoIExtractor',
+ 'H3DRoIHead', 'PrimitiveHead', 'PointRCNNRoIHead'
+]
diff --git a/mmdet3d/models/roi_heads/base_3droi_head.py b/mmdet3d/models/roi_heads/base_3droi_head.py
new file mode 100644
index 0000000..e1816ff
--- /dev/null
+++ b/mmdet3d/models/roi_heads/base_3droi_head.py
@@ -0,0 +1,98 @@
+# Copyright (c) OpenMMLab. All rights reserved.
+from abc import ABCMeta, abstractmethod
+
+from mmcv.runner import BaseModule
+
+
+class Base3DRoIHead(BaseModule, metaclass=ABCMeta):
+ """Base class for 3d RoIHeads."""
+
+ def __init__(self,
+ bbox_head=None,
+ mask_roi_extractor=None,
+ mask_head=None,
+ train_cfg=None,
+ test_cfg=None,
+ pretrained=None,
+ init_cfg=None):
+ super(Base3DRoIHead, self).__init__(init_cfg=init_cfg)
+ self.train_cfg = train_cfg
+ self.test_cfg = test_cfg
+
+ if bbox_head is not None:
+ self.init_bbox_head(bbox_head)
+
+ if mask_head is not None:
+ self.init_mask_head(mask_roi_extractor, mask_head)
+
+ self.init_assigner_sampler()
+
+ @property
+ def with_bbox(self):
+ """bool: whether the RoIHead has box head"""
+ return hasattr(self, 'bbox_head') and self.bbox_head is not None
+
+ @property
+ def with_mask(self):
+ """bool: whether the RoIHead has mask head"""
+ return hasattr(self, 'mask_head') and self.mask_head is not None
+
+ @abstractmethod
+ def init_bbox_head(self):
+ """Initialize the box head."""
+ pass
+
+ @abstractmethod
+ def init_mask_head(self):
+ """Initialize maek head."""
+ pass
+
+ @abstractmethod
+ def init_assigner_sampler(self):
+ """Initialize assigner and sampler."""
+ pass
+
+ @abstractmethod
+ def forward_train(self,
+ x,
+ img_metas,
+ proposal_list,
+ gt_bboxes,
+ gt_labels,
+ gt_bboxes_ignore=None,
+ **kwargs):
+ """Forward function during training.
+
+ Args:
+ x (dict): Contains features from the first stage.
+ img_metas (list[dict]): Meta info of each image.
+ proposal_list (list[dict]): Proposal information from rpn.
+ gt_bboxes (list[:obj:`BaseInstance3DBoxes`]):
+ GT bboxes of each sample. The bboxes are encapsulated
+ by 3D box structures.
+ gt_labels (list[torch.LongTensor]): GT labels of each sample.
+ gt_bboxes_ignore (list[torch.Tensor], optional):
+ Ground truth boxes to be ignored.
+
+ Returns:
+ dict[str, torch.Tensor]: Losses from each head.
+ """
+ pass
+
+ def simple_test(self,
+ x,
+ proposal_list,
+ img_metas,
+ proposals=None,
+ rescale=False,
+ **kwargs):
+ """Test without augmentation."""
+ pass
+
+ def aug_test(self, x, proposal_list, img_metas, rescale=False, **kwargs):
+ """Test with augmentations.
+
+ If rescale is False, then returned bboxes and masks will fit the scale
+ of imgs[0].
+ """
+ pass
diff --git a/mmdet3d/models/roi_heads/bbox_heads/__init__.py b/mmdet3d/models/roi_heads/bbox_heads/__init__.py
new file mode 100644
index 0000000..fd7a6b0
--- /dev/null
+++ b/mmdet3d/models/roi_heads/bbox_heads/__init__.py
@@ -0,0 +1,14 @@
+# Copyright (c) OpenMMLab. All rights reserved.
+from mmdet.models.roi_heads.bbox_heads import (BBoxHead, ConvFCBBoxHead,
+ DoubleConvFCBBoxHead,
+ Shared2FCBBoxHead,
+ Shared4Conv1FCBBoxHead)
+from .h3d_bbox_head import H3DBboxHead
+from .parta2_bbox_head import PartA2BboxHead
+from .point_rcnn_bbox_head import PointRCNNBboxHead
+
+__all__ = [
+ 'BBoxHead', 'ConvFCBBoxHead', 'Shared2FCBBoxHead',
+ 'Shared4Conv1FCBBoxHead', 'DoubleConvFCBBoxHead', 'PartA2BboxHead',
+ 'H3DBboxHead', 'PointRCNNBboxHead'
+]
diff --git a/mmdet3d/models/roi_heads/bbox_heads/h3d_bbox_head.py b/mmdet3d/models/roi_heads/bbox_heads/h3d_bbox_head.py
new file mode 100644
index 0000000..a8bd11a
--- /dev/null
+++ b/mmdet3d/models/roi_heads/bbox_heads/h3d_bbox_head.py
@@ -0,0 +1,925 @@
+# Copyright (c) OpenMMLab. All rights reserved.
+import torch
+from mmcv.cnn import ConvModule
+from mmcv.runner import BaseModule
+from torch import nn as nn
+from torch.nn import functional as F
+
+from mmdet3d.core.bbox import DepthInstance3DBoxes
+from mmdet3d.core.post_processing import aligned_3d_nms
+from mmdet3d.models.builder import HEADS, build_loss
+from mmdet3d.models.losses import chamfer_distance
+from mmdet3d.ops import build_sa_module
+from mmdet.core import build_bbox_coder, multi_apply
+
+
+@HEADS.register_module()
+class H3DBboxHead(BaseModule):
+ r"""Bbox head of `H3DNet `_.
+
+ Args:
+ num_classes (int): The number of classes.
+ surface_matching_cfg (dict): Config for surface primitive matching.
+ line_matching_cfg (dict): Config for line primitive matching.
+ bbox_coder (:obj:`BaseBBoxCoder`): Bbox coder for encoding and
+ decoding boxes.
+ train_cfg (dict): Config for training.
+ test_cfg (dict): Config for testing.
+ gt_per_seed (int): Number of ground truth votes generated
+ from each seed point.
+ num_proposal (int): Number of proposal votes generated.
+ feat_channels (tuple[int]): Convolution channels of
+ prediction layer.
+ primitive_feat_refine_streams (int): The number of mlps to
+ refine primitive feature.
+ primitive_refine_channels (tuple[int]): Convolution channels of
+ prediction layer.
+ upper_thresh (float): Threshold for line matching.
+ surface_thresh (float): Threshold for surface matching.
+ line_thresh (float): Threshold for line matching.
+ conv_cfg (dict): Config of convolution in prediction layer.
+ norm_cfg (dict): Config of BN in prediction layer.
+ objectness_loss (dict): Config of objectness loss.
+ center_loss (dict): Config of center loss.
+ dir_class_loss (dict): Config of direction classification loss.
+ dir_res_loss (dict): Config of direction residual regression loss.
+ size_class_loss (dict): Config of size classification loss.
+ size_res_loss (dict): Config of size residual regression loss.
+ semantic_loss (dict): Config of point-wise semantic segmentation loss.
+ cues_objectness_loss (dict): Config of cues objectness loss.
+ cues_semantic_loss (dict): Config of cues semantic loss.
+ proposal_objectness_loss (dict): Config of proposal objectness
+ loss.
+ primitive_center_loss (dict): Config of primitive center regression
+ loss.
+ """
+
+ def __init__(self,
+ num_classes,
+ suface_matching_cfg,
+ line_matching_cfg,
+ bbox_coder,
+ train_cfg=None,
+ test_cfg=None,
+ gt_per_seed=1,
+ num_proposal=256,
+ feat_channels=(128, 128),
+ primitive_feat_refine_streams=2,
+ primitive_refine_channels=[128, 128, 128],
+ upper_thresh=100.0,
+ surface_thresh=0.5,
+ line_thresh=0.5,
+ conv_cfg=dict(type='Conv1d'),
+ norm_cfg=dict(type='BN1d'),
+ objectness_loss=None,
+ center_loss=None,
+ dir_class_loss=None,
+ dir_res_loss=None,
+ size_class_loss=None,
+ size_res_loss=None,
+ semantic_loss=None,
+ cues_objectness_loss=None,
+ cues_semantic_loss=None,
+ proposal_objectness_loss=None,
+ primitive_center_loss=None,
+ init_cfg=None):
+ super(H3DBboxHead, self).__init__(init_cfg=init_cfg)
+ self.num_classes = num_classes
+ self.train_cfg = train_cfg
+ self.test_cfg = test_cfg
+ self.gt_per_seed = gt_per_seed
+ self.num_proposal = num_proposal
+ self.with_angle = bbox_coder['with_rot']
+ self.upper_thresh = upper_thresh
+ self.surface_thresh = surface_thresh
+ self.line_thresh = line_thresh
+
+ self.objectness_loss = build_loss(objectness_loss)
+ self.center_loss = build_loss(center_loss)
+ self.dir_class_loss = build_loss(dir_class_loss)
+ self.dir_res_loss = build_loss(dir_res_loss)
+ self.size_class_loss = build_loss(size_class_loss)
+ self.size_res_loss = build_loss(size_res_loss)
+ self.semantic_loss = build_loss(semantic_loss)
+
+ self.bbox_coder = build_bbox_coder(bbox_coder)
+ self.num_sizes = self.bbox_coder.num_sizes
+ self.num_dir_bins = self.bbox_coder.num_dir_bins
+
+ self.cues_objectness_loss = build_loss(cues_objectness_loss)
+ self.cues_semantic_loss = build_loss(cues_semantic_loss)
+ self.proposal_objectness_loss = build_loss(proposal_objectness_loss)
+ self.primitive_center_loss = build_loss(primitive_center_loss)
+
+ assert suface_matching_cfg['mlp_channels'][-1] == \
+ line_matching_cfg['mlp_channels'][-1]
+
+ # surface center matching
+ self.surface_center_matcher = build_sa_module(suface_matching_cfg)
+ # line center matching
+ self.line_center_matcher = build_sa_module(line_matching_cfg)
+
+ # Compute the matching scores
+ matching_feat_dims = suface_matching_cfg['mlp_channels'][-1]
+ self.matching_conv = ConvModule(
+ matching_feat_dims,
+ matching_feat_dims,
+ 1,
+ padding=0,
+ conv_cfg=conv_cfg,
+ norm_cfg=norm_cfg,
+ bias=True,
+ inplace=True)
+ self.matching_pred = nn.Conv1d(matching_feat_dims, 2, 1)
+
+ # Compute the semantic matching scores
+ self.semantic_matching_conv = ConvModule(
+ matching_feat_dims,
+ matching_feat_dims,
+ 1,
+ padding=0,
+ conv_cfg=conv_cfg,
+ norm_cfg=norm_cfg,
+ bias=True,
+ inplace=True)
+ self.semantic_matching_pred = nn.Conv1d(matching_feat_dims, 2, 1)
+
+ # Surface feature aggregation
+ self.surface_feats_aggregation = list()
+ for k in range(primitive_feat_refine_streams):
+ self.surface_feats_aggregation.append(
+ ConvModule(
+ matching_feat_dims,
+ matching_feat_dims,
+ 1,
+ padding=0,
+ conv_cfg=conv_cfg,
+ norm_cfg=norm_cfg,
+ bias=True,
+ inplace=True))
+ self.surface_feats_aggregation = nn.Sequential(
+ *self.surface_feats_aggregation)
+
+ # Line feature aggregation
+ self.line_feats_aggregation = list()
+ for k in range(primitive_feat_refine_streams):
+ self.line_feats_aggregation.append(
+ ConvModule(
+ matching_feat_dims,
+ matching_feat_dims,
+ 1,
+ padding=0,
+ conv_cfg=conv_cfg,
+ norm_cfg=norm_cfg,
+ bias=True,
+ inplace=True))
+ self.line_feats_aggregation = nn.Sequential(
+ *self.line_feats_aggregation)
+
+ # surface center(6) + line center(12)
+ prev_channel = 18 * matching_feat_dims
+ self.bbox_pred = nn.ModuleList()
+ for k in range(len(primitive_refine_channels)):
+ self.bbox_pred.append(
+ ConvModule(
+ prev_channel,
+ primitive_refine_channels[k],
+ 1,
+ padding=0,
+ conv_cfg=conv_cfg,
+ norm_cfg=norm_cfg,
+ bias=True,
+ inplace=False))
+ prev_channel = primitive_refine_channels[k]
+
+ # Final object detection
+ # Objectness scores (2), center residual (3),
+ # heading class+residual (num_heading_bin*2), size class +
+ # residual(num_size_cluster*4)
+ conv_out_channel = (2 + 3 + bbox_coder['num_dir_bins'] * 2 +
+ bbox_coder['num_sizes'] * 4 + self.num_classes)
+ self.bbox_pred.append(nn.Conv1d(prev_channel, conv_out_channel, 1))
+
+ def forward(self, feats_dict, sample_mod):
+ """Forward pass.
+
+ Args:
+ feats_dict (dict): Feature dict from backbone.
+ sample_mod (str): Sample mode for vote aggregation layer.
+ valid modes are "vote", "seed" and "random".
+
+ Returns:
+ dict: Predictions of vote head.
+ """
+ ret_dict = {}
+ aggregated_points = feats_dict['aggregated_points']
+ original_feature = feats_dict['aggregated_features']
+ batch_size = original_feature.shape[0]
+ object_proposal = original_feature.shape[2]
+
+ # Extract surface center, features and semantic predictions
+ z_center = feats_dict['pred_z_center']
+ xy_center = feats_dict['pred_xy_center']
+ z_semantic = feats_dict['sem_cls_scores_z']
+ xy_semantic = feats_dict['sem_cls_scores_xy']
+ z_feature = feats_dict['aggregated_features_z']
+ xy_feature = feats_dict['aggregated_features_xy']
+ # Extract line points and features
+ line_center = feats_dict['pred_line_center']
+ line_feature = feats_dict['aggregated_features_line']
+
+ surface_center_pred = torch.cat((z_center, xy_center), dim=1)
+ ret_dict['surface_center_pred'] = surface_center_pred
+ ret_dict['surface_sem_pred'] = torch.cat((z_semantic, xy_semantic),
+ dim=1)
+
+ # Extract the surface and line centers of rpn proposals
+ rpn_proposals = feats_dict['proposal_list']
+ rpn_proposals_bbox = DepthInstance3DBoxes(
+ rpn_proposals.reshape(-1, 7).clone(),
+ box_dim=rpn_proposals.shape[-1],
+ with_yaw=self.with_angle,
+ origin=(0.5, 0.5, 0.5))
+
+ obj_surface_center, obj_line_center = \
+ rpn_proposals_bbox.get_surface_line_center()
+ obj_surface_center = obj_surface_center.reshape(
+ batch_size, -1, 6, 3).transpose(1, 2).reshape(batch_size, -1, 3)
+ obj_line_center = obj_line_center.reshape(batch_size, -1, 12,
+ 3).transpose(1, 2).reshape(
+ batch_size, -1, 3)
+ ret_dict['surface_center_object'] = obj_surface_center
+ ret_dict['line_center_object'] = obj_line_center
+
+ # aggregate primitive z and xy features to rpn proposals
+ surface_center_feature_pred = torch.cat((z_feature, xy_feature), dim=2)
+ surface_center_feature_pred = torch.cat(
+ (surface_center_feature_pred.new_zeros(
+ (batch_size, 6, surface_center_feature_pred.shape[2])),
+ surface_center_feature_pred),
+ dim=1)
+
+ surface_xyz, surface_features, _ = self.surface_center_matcher(
+ surface_center_pred,
+ surface_center_feature_pred,
+ target_xyz=obj_surface_center)
+
+ # aggregate primitive line features to rpn proposals
+ line_feature = torch.cat((line_feature.new_zeros(
+ (batch_size, 12, line_feature.shape[2])), line_feature),
+ dim=1)
+ line_xyz, line_features, _ = self.line_center_matcher(
+ line_center, line_feature, target_xyz=obj_line_center)
+
+ # combine the surface and line features
+ combine_features = torch.cat((surface_features, line_features), dim=2)
+
+ matching_features = self.matching_conv(combine_features)
+ matching_score = self.matching_pred(matching_features)
+ ret_dict['matching_score'] = matching_score.transpose(2, 1)
+
+ semantic_matching_features = self.semantic_matching_conv(
+ combine_features)
+ semantic_matching_score = self.semantic_matching_pred(
+ semantic_matching_features)
+ ret_dict['semantic_matching_score'] = \
+ semantic_matching_score.transpose(2, 1)
+
+ surface_features = self.surface_feats_aggregation(surface_features)
+ line_features = self.line_feats_aggregation(line_features)
+
+ # Combine all surface and line features
+ surface_features = surface_features.view(batch_size, -1,
+ object_proposal)
+ line_features = line_features.view(batch_size, -1, object_proposal)
+
+ combine_feature = torch.cat((surface_features, line_features), dim=1)
+
+ # Final bbox predictions
+ bbox_predictions = self.bbox_pred[0](combine_feature)
+ bbox_predictions += original_feature
+ for conv_module in self.bbox_pred[1:]:
+ bbox_predictions = conv_module(bbox_predictions)
+
+ refine_decode_res = self.bbox_coder.split_pred(
+ bbox_predictions[:, :self.num_classes + 2],
+ bbox_predictions[:, self.num_classes + 2:], aggregated_points)
+ for key in refine_decode_res.keys():
+ ret_dict[key + '_optimized'] = refine_decode_res[key]
+ return ret_dict
+
+ def loss(self,
+ bbox_preds,
+ points,
+ gt_bboxes_3d,
+ gt_labels_3d,
+ pts_semantic_mask=None,
+ pts_instance_mask=None,
+ img_metas=None,
+ rpn_targets=None,
+ gt_bboxes_ignore=None):
+ """Compute loss.
+
+ Args:
+ bbox_preds (dict): Predictions from forward of h3d bbox head.
+ points (list[torch.Tensor]): Input points.
+ gt_bboxes_3d (list[:obj:`BaseInstance3DBoxes`]): Ground truth
+ bboxes of each sample.
+ gt_labels_3d (list[torch.Tensor]): Labels of each sample.
+ pts_semantic_mask (list[torch.Tensor]): Point-wise
+ semantic mask.
+ pts_instance_mask (list[torch.Tensor]): Point-wise
+ instance mask.
+ img_metas (list[dict]): Contain pcd and img's meta info.
+ rpn_targets (Tuple) : Targets generated by rpn head.
+ gt_bboxes_ignore (list[torch.Tensor]): Specify
+ which bounding.
+
+ Returns:
+ dict: Losses of H3dnet.
+ """
+ (vote_targets, vote_target_masks, size_class_targets, size_res_targets,
+ dir_class_targets, dir_res_targets, center_targets, _, mask_targets,
+ valid_gt_masks, objectness_targets, objectness_weights,
+ box_loss_weights, valid_gt_weights) = rpn_targets
+
+ losses = {}
+
+ # calculate refined proposal loss
+ refined_proposal_loss = self.get_proposal_stage_loss(
+ bbox_preds,
+ size_class_targets,
+ size_res_targets,
+ dir_class_targets,
+ dir_res_targets,
+ center_targets,
+ mask_targets,
+ objectness_targets,
+ objectness_weights,
+ box_loss_weights,
+ valid_gt_weights,
+ suffix='_optimized')
+ for key in refined_proposal_loss.keys():
+ losses[key + '_optimized'] = refined_proposal_loss[key]
+
+ bbox3d_optimized = self.bbox_coder.decode(
+ bbox_preds, suffix='_optimized')
+
+ targets = self.get_targets(points, gt_bboxes_3d, gt_labels_3d,
+ pts_semantic_mask, pts_instance_mask,
+ bbox_preds)
+
+ (cues_objectness_label, cues_sem_label, proposal_objectness_label,
+ cues_mask, cues_match_mask, proposal_objectness_mask,
+ cues_matching_label, obj_surface_line_center) = targets
+
+ # match scores for each geometric primitive
+ objectness_scores = bbox_preds['matching_score']
+ # match scores for the semantics of primitives
+ objectness_scores_sem = bbox_preds['semantic_matching_score']
+
+ primitive_objectness_loss = self.cues_objectness_loss(
+ objectness_scores.transpose(2, 1),
+ cues_objectness_label,
+ weight=cues_mask,
+ avg_factor=cues_mask.sum() + 1e-6)
+
+ primitive_sem_loss = self.cues_semantic_loss(
+ objectness_scores_sem.transpose(2, 1),
+ cues_sem_label,
+ weight=cues_mask,
+ avg_factor=cues_mask.sum() + 1e-6)
+
+ objectness_scores = bbox_preds['obj_scores_optimized']
+ objectness_loss_refine = self.proposal_objectness_loss(
+ objectness_scores.transpose(2, 1), proposal_objectness_label)
+ primitive_matching_loss = (objectness_loss_refine *
+ cues_match_mask).sum() / (
+ cues_match_mask.sum() + 1e-6) * 0.5
+ primitive_sem_matching_loss = (
+ objectness_loss_refine * proposal_objectness_mask).sum() / (
+ proposal_objectness_mask.sum() + 1e-6) * 0.5
+
+ # Get the object surface center here
+ batch_size, object_proposal = bbox3d_optimized.shape[:2]
+ refined_bbox = DepthInstance3DBoxes(
+ bbox3d_optimized.reshape(-1, 7).clone(),
+ box_dim=bbox3d_optimized.shape[-1],
+ with_yaw=self.with_angle,
+ origin=(0.5, 0.5, 0.5))
+
+ pred_obj_surface_center, pred_obj_line_center = \
+ refined_bbox.get_surface_line_center()
+ pred_obj_surface_center = pred_obj_surface_center.reshape(
+ batch_size, -1, 6, 3).transpose(1, 2).reshape(batch_size, -1, 3)
+ pred_obj_line_center = pred_obj_line_center.reshape(
+ batch_size, -1, 12, 3).transpose(1, 2).reshape(batch_size, -1, 3)
+ pred_surface_line_center = torch.cat(
+ (pred_obj_surface_center, pred_obj_line_center), 1)
+
+ square_dist = self.primitive_center_loss(pred_surface_line_center,
+ obj_surface_line_center)
+
+ match_dist = torch.sqrt(square_dist.sum(dim=-1) + 1e-6)
+ primitive_centroid_reg_loss = torch.sum(
+ match_dist * cues_matching_label) / (
+ cues_matching_label.sum() + 1e-6)
+
+ refined_loss = dict(
+ primitive_objectness_loss=primitive_objectness_loss,
+ primitive_sem_loss=primitive_sem_loss,
+ primitive_matching_loss=primitive_matching_loss,
+ primitive_sem_matching_loss=primitive_sem_matching_loss,
+ primitive_centroid_reg_loss=primitive_centroid_reg_loss)
+
+ losses.update(refined_loss)
+
+ return losses
+
+ def get_bboxes(self,
+ points,
+ bbox_preds,
+ input_metas,
+ rescale=False,
+ suffix=''):
+ """Generate bboxes from vote head predictions.
+
+ Args:
+ points (torch.Tensor): Input points.
+ bbox_preds (dict): Predictions from vote head.
+ input_metas (list[dict]): Point cloud and image's meta info.
+ rescale (bool): Whether to rescale bboxes.
+
+ Returns:
+ list[tuple[torch.Tensor]]: Bounding boxes, scores and labels.
+ """
+ # decode boxes
+ obj_scores = F.softmax(
+ bbox_preds['obj_scores' + suffix], dim=-1)[..., -1]
+
+ sem_scores = F.softmax(bbox_preds['sem_scores'], dim=-1)
+
+ prediction_collection = {}
+ prediction_collection['center'] = bbox_preds['center' + suffix]
+ prediction_collection['dir_class'] = bbox_preds['dir_class']
+ prediction_collection['dir_res'] = bbox_preds['dir_res' + suffix]
+ prediction_collection['size_class'] = bbox_preds['size_class']
+ prediction_collection['size_res'] = bbox_preds['size_res' + suffix]
+
+ bbox3d = self.bbox_coder.decode(prediction_collection)
+
+ batch_size = bbox3d.shape[0]
+ results = list()
+ for b in range(batch_size):
+ bbox_selected, score_selected, labels = self.multiclass_nms_single(
+ obj_scores[b], sem_scores[b], bbox3d[b], points[b, ..., :3],
+ input_metas[b])
+ bbox = input_metas[b]['box_type_3d'](
+ bbox_selected,
+ box_dim=bbox_selected.shape[-1],
+ with_yaw=self.bbox_coder.with_rot)
+ results.append((bbox, score_selected, labels))
+
+ return results
+
+ def multiclass_nms_single(self, obj_scores, sem_scores, bbox, points,
+ input_meta):
+ """Multi-class nms in single batch.
+
+ Args:
+ obj_scores (torch.Tensor): Objectness score of bounding boxes.
+ sem_scores (torch.Tensor): semantic class score of bounding boxes.
+ bbox (torch.Tensor): Predicted bounding boxes.
+ points (torch.Tensor): Input points.
+ input_meta (dict): Point cloud and image's meta info.
+
+ Returns:
+ tuple[torch.Tensor]: Bounding boxes, scores and labels.
+ """
+ bbox = input_meta['box_type_3d'](
+ bbox,
+ box_dim=bbox.shape[-1],
+ with_yaw=self.bbox_coder.with_rot,
+ origin=(0.5, 0.5, 0.5))
+ box_indices = bbox.points_in_boxes_all(points)
+
+ corner3d = bbox.corners
+ minmax_box3d = corner3d.new(torch.Size((corner3d.shape[0], 6)))
+ minmax_box3d[:, :3] = torch.min(corner3d, dim=1)[0]
+ minmax_box3d[:, 3:] = torch.max(corner3d, dim=1)[0]
+
+ nonempty_box_mask = box_indices.T.sum(1) > 5
+
+ bbox_classes = torch.argmax(sem_scores, -1)
+ nms_selected = aligned_3d_nms(minmax_box3d[nonempty_box_mask],
+ obj_scores[nonempty_box_mask],
+ bbox_classes[nonempty_box_mask],
+ self.test_cfg.nms_thr)
+
+ # filter empty boxes and boxes with low score
+ scores_mask = (obj_scores > self.test_cfg.score_thr)
+ nonempty_box_inds = torch.nonzero(
+ nonempty_box_mask, as_tuple=False).flatten()
+ nonempty_mask = torch.zeros_like(bbox_classes).scatter(
+ 0, nonempty_box_inds[nms_selected], 1)
+ selected = (nonempty_mask.bool() & scores_mask.bool())
+
+ if self.test_cfg.per_class_proposal:
+ bbox_selected, score_selected, labels = [], [], []
+ for k in range(sem_scores.shape[-1]):
+ bbox_selected.append(bbox[selected].tensor)
+ score_selected.append(obj_scores[selected] *
+ sem_scores[selected][:, k])
+ labels.append(
+ torch.zeros_like(bbox_classes[selected]).fill_(k))
+ bbox_selected = torch.cat(bbox_selected, 0)
+ score_selected = torch.cat(score_selected, 0)
+ labels = torch.cat(labels, 0)
+ else:
+ bbox_selected = bbox[selected].tensor
+ score_selected = obj_scores[selected]
+ labels = bbox_classes[selected]
+
+ return bbox_selected, score_selected, labels
+
+ def get_proposal_stage_loss(self,
+ bbox_preds,
+ size_class_targets,
+ size_res_targets,
+ dir_class_targets,
+ dir_res_targets,
+ center_targets,
+ mask_targets,
+ objectness_targets,
+ objectness_weights,
+ box_loss_weights,
+ valid_gt_weights,
+ suffix=''):
+ """Compute loss for the aggregation module.
+
+ Args:
+ bbox_preds (dict): Predictions from forward of vote head.
+ size_class_targets (torch.Tensor): Ground truth
+ size class of each prediction bounding box.
+ size_res_targets (torch.Tensor): Ground truth
+ size residual of each prediction bounding box.
+ dir_class_targets (torch.Tensor): Ground truth
+ direction class of each prediction bounding box.
+ dir_res_targets (torch.Tensor): Ground truth
+ direction residual of each prediction bounding box.
+ center_targets (torch.Tensor): Ground truth center
+ of each prediction bounding box.
+ mask_targets (torch.Tensor): Validation of each
+ prediction bounding box.
+ objectness_targets (torch.Tensor): Ground truth
+ objectness label of each prediction bounding box.
+ objectness_weights (torch.Tensor): Weights of objectness
+ loss for each prediction bounding box.
+ box_loss_weights (torch.Tensor): Weights of regression
+ loss for each prediction bounding box.
+ valid_gt_weights (torch.Tensor): Validation of each
+ ground truth bounding box.
+
+ Returns:
+ dict: Losses of aggregation module.
+ """
+ # calculate objectness loss
+ objectness_loss = self.objectness_loss(
+ bbox_preds['obj_scores' + suffix].transpose(2, 1),
+ objectness_targets,
+ weight=objectness_weights)
+
+ # calculate center loss
+ source2target_loss, target2source_loss = self.center_loss(
+ bbox_preds['center' + suffix],
+ center_targets,
+ src_weight=box_loss_weights,
+ dst_weight=valid_gt_weights)
+ center_loss = source2target_loss + target2source_loss
+
+ # calculate direction class loss
+ dir_class_loss = self.dir_class_loss(
+ bbox_preds['dir_class' + suffix].transpose(2, 1),
+ dir_class_targets,
+ weight=box_loss_weights)
+
+ # calculate direction residual loss
+ batch_size, proposal_num = size_class_targets.shape[:2]
+ heading_label_one_hot = dir_class_targets.new_zeros(
+ (batch_size, proposal_num, self.num_dir_bins))
+ heading_label_one_hot.scatter_(2, dir_class_targets.unsqueeze(-1), 1)
+ dir_res_norm = (bbox_preds['dir_res_norm' + suffix] *
+ heading_label_one_hot).sum(dim=-1)
+ dir_res_loss = self.dir_res_loss(
+ dir_res_norm, dir_res_targets, weight=box_loss_weights)
+
+ # calculate size class loss
+ size_class_loss = self.size_class_loss(
+ bbox_preds['size_class' + suffix].transpose(2, 1),
+ size_class_targets,
+ weight=box_loss_weights)
+
+ # calculate size residual loss
+ one_hot_size_targets = box_loss_weights.new_zeros(
+ (batch_size, proposal_num, self.num_sizes))
+ one_hot_size_targets.scatter_(2, size_class_targets.unsqueeze(-1), 1)
+ one_hot_size_targets_expand = one_hot_size_targets.unsqueeze(
+ -1).repeat(1, 1, 1, 3)
+ size_residual_norm = (bbox_preds['size_res_norm' + suffix] *
+ one_hot_size_targets_expand).sum(dim=2)
+ box_loss_weights_expand = box_loss_weights.unsqueeze(-1).repeat(
+ 1, 1, 3)
+ size_res_loss = self.size_res_loss(
+ size_residual_norm,
+ size_res_targets,
+ weight=box_loss_weights_expand)
+
+ # calculate semantic loss
+ semantic_loss = self.semantic_loss(
+ bbox_preds['sem_scores' + suffix].transpose(2, 1),
+ mask_targets,
+ weight=box_loss_weights)
+
+ losses = dict(
+ objectness_loss=objectness_loss,
+ semantic_loss=semantic_loss,
+ center_loss=center_loss,
+ dir_class_loss=dir_class_loss,
+ dir_res_loss=dir_res_loss,
+ size_class_loss=size_class_loss,
+ size_res_loss=size_res_loss)
+
+ return losses
+
+ def get_targets(self,
+ points,
+ gt_bboxes_3d,
+ gt_labels_3d,
+ pts_semantic_mask=None,
+ pts_instance_mask=None,
+ bbox_preds=None):
+ """Generate targets of proposal module.
+
+ Args:
+ points (list[torch.Tensor]): Points of each batch.
+ gt_bboxes_3d (list[:obj:`BaseInstance3DBoxes`]): Ground truth
+ bboxes of each batch.
+ gt_labels_3d (list[torch.Tensor]): Labels of each batch.
+ pts_semantic_mask (list[torch.Tensor]): Point-wise semantic
+ label of each batch.
+ pts_instance_mask (list[torch.Tensor]): Point-wise instance
+ label of each batch.
+ bbox_preds (torch.Tensor): Bounding box predictions of vote head.
+
+ Returns:
+ tuple[torch.Tensor]: Targets of proposal module.
+ """
+ # find empty example
+ valid_gt_masks = list()
+ gt_num = list()
+ for index in range(len(gt_labels_3d)):
+ if len(gt_labels_3d[index]) == 0:
+ fake_box = gt_bboxes_3d[index].tensor.new_zeros(
+ 1, gt_bboxes_3d[index].tensor.shape[-1])
+ gt_bboxes_3d[index] = gt_bboxes_3d[index].new_box(fake_box)
+ gt_labels_3d[index] = gt_labels_3d[index].new_zeros(1)
+ valid_gt_masks.append(gt_labels_3d[index].new_zeros(1))
+ gt_num.append(1)
+ else:
+ valid_gt_masks.append(gt_labels_3d[index].new_ones(
+ gt_labels_3d[index].shape))
+ gt_num.append(gt_labels_3d[index].shape[0])
+
+ if pts_semantic_mask is None:
+ pts_semantic_mask = [None for i in range(len(gt_labels_3d))]
+ pts_instance_mask = [None for i in range(len(gt_labels_3d))]
+
+ aggregated_points = [
+ bbox_preds['aggregated_points'][i]
+ for i in range(len(gt_labels_3d))
+ ]
+
+ surface_center_pred = [
+ bbox_preds['surface_center_pred'][i]
+ for i in range(len(gt_labels_3d))
+ ]
+
+ line_center_pred = [
+ bbox_preds['pred_line_center'][i]
+ for i in range(len(gt_labels_3d))
+ ]
+
+ surface_center_object = [
+ bbox_preds['surface_center_object'][i]
+ for i in range(len(gt_labels_3d))
+ ]
+
+ line_center_object = [
+ bbox_preds['line_center_object'][i]
+ for i in range(len(gt_labels_3d))
+ ]
+
+ surface_sem_pred = [
+ bbox_preds['surface_sem_pred'][i]
+ for i in range(len(gt_labels_3d))
+ ]
+
+ line_sem_pred = [
+ bbox_preds['sem_cls_scores_line'][i]
+ for i in range(len(gt_labels_3d))
+ ]
+
+ (cues_objectness_label, cues_sem_label, proposal_objectness_label,
+ cues_mask, cues_match_mask, proposal_objectness_mask,
+ cues_matching_label, obj_surface_line_center) = multi_apply(
+ self.get_targets_single, points, gt_bboxes_3d, gt_labels_3d,
+ pts_semantic_mask, pts_instance_mask, aggregated_points,
+ surface_center_pred, line_center_pred, surface_center_object,
+ line_center_object, surface_sem_pred, line_sem_pred)
+
+ cues_objectness_label = torch.stack(cues_objectness_label)
+ cues_sem_label = torch.stack(cues_sem_label)
+ proposal_objectness_label = torch.stack(proposal_objectness_label)
+ cues_mask = torch.stack(cues_mask)
+ cues_match_mask = torch.stack(cues_match_mask)
+ proposal_objectness_mask = torch.stack(proposal_objectness_mask)
+ cues_matching_label = torch.stack(cues_matching_label)
+ obj_surface_line_center = torch.stack(obj_surface_line_center)
+
+ return (cues_objectness_label, cues_sem_label,
+ proposal_objectness_label, cues_mask, cues_match_mask,
+ proposal_objectness_mask, cues_matching_label,
+ obj_surface_line_center)
+
+ def get_targets_single(self,
+ points,
+ gt_bboxes_3d,
+ gt_labels_3d,
+ pts_semantic_mask=None,
+ pts_instance_mask=None,
+ aggregated_points=None,
+ pred_surface_center=None,
+ pred_line_center=None,
+ pred_obj_surface_center=None,
+ pred_obj_line_center=None,
+ pred_surface_sem=None,
+ pred_line_sem=None):
+ """Generate targets for primitive cues for single batch.
+
+ Args:
+ points (torch.Tensor): Points of each batch.
+ gt_bboxes_3d (:obj:`BaseInstance3DBoxes`): Ground truth
+ boxes of each batch.
+ gt_labels_3d (torch.Tensor): Labels of each batch.
+ pts_semantic_mask (torch.Tensor): Point-wise semantic
+ label of each batch.
+ pts_instance_mask (torch.Tensor): Point-wise instance
+ label of each batch.
+ aggregated_points (torch.Tensor): Aggregated points from
+ vote aggregation layer.
+ pred_surface_center (torch.Tensor): Prediction of surface center.
+ pred_line_center (torch.Tensor): Prediction of line center.
+ pred_obj_surface_center (torch.Tensor): Objectness prediction
+ of surface center.
+ pred_obj_line_center (torch.Tensor): Objectness prediction of
+ line center.
+ pred_surface_sem (torch.Tensor): Semantic prediction of
+ surface center.
+ pred_line_sem (torch.Tensor): Semantic prediction of line center.
+ Returns:
+ tuple[torch.Tensor]: Targets for primitive cues.
+ """
+ device = points.device
+ gt_bboxes_3d = gt_bboxes_3d.to(device)
+ num_proposals = aggregated_points.shape[0]
+ gt_center = gt_bboxes_3d.gravity_center
+
+ dist1, dist2, ind1, _ = chamfer_distance(
+ aggregated_points.unsqueeze(0),
+ gt_center.unsqueeze(0),
+ reduction='none')
+ # Set assignment
+ object_assignment = ind1.squeeze(0)
+
+ # Generate objectness label and mask
+ # objectness_label: 1 if pred object center is within
+ # self.train_cfg['near_threshold'] of any GT object
+ # objectness_mask: 0 if pred object center is in gray
+ # zone (DONOTCARE), 1 otherwise
+ euclidean_dist1 = torch.sqrt(dist1.squeeze(0) + 1e-6)
+ proposal_objectness_label = euclidean_dist1.new_zeros(
+ num_proposals, dtype=torch.long)
+ proposal_objectness_mask = euclidean_dist1.new_zeros(num_proposals)
+
+ gt_sem = gt_labels_3d[object_assignment]
+
+ obj_surface_center, obj_line_center = \
+ gt_bboxes_3d.get_surface_line_center()
+ obj_surface_center = obj_surface_center.reshape(-1, 6,
+ 3).transpose(0, 1)
+ obj_line_center = obj_line_center.reshape(-1, 12, 3).transpose(0, 1)
+ obj_surface_center = obj_surface_center[:, object_assignment].reshape(
+ 1, -1, 3)
+ obj_line_center = obj_line_center[:,
+ object_assignment].reshape(1, -1, 3)
+
+ surface_sem = torch.argmax(pred_surface_sem, dim=1).float()
+ line_sem = torch.argmax(pred_line_sem, dim=1).float()
+
+ dist_surface, _, surface_ind, _ = chamfer_distance(
+ obj_surface_center,
+ pred_surface_center.unsqueeze(0),
+ reduction='none')
+ dist_line, _, line_ind, _ = chamfer_distance(
+ obj_line_center, pred_line_center.unsqueeze(0), reduction='none')
+
+ surface_sel = pred_surface_center[surface_ind.squeeze(0)]
+ line_sel = pred_line_center[line_ind.squeeze(0)]
+ surface_sel_sem = surface_sem[surface_ind.squeeze(0)]
+ line_sel_sem = line_sem[line_ind.squeeze(0)]
+
+ surface_sel_sem_gt = gt_sem.repeat(6).float()
+ line_sel_sem_gt = gt_sem.repeat(12).float()
+
+ euclidean_dist_surface = torch.sqrt(dist_surface.squeeze(0) + 1e-6)
+ euclidean_dist_line = torch.sqrt(dist_line.squeeze(0) + 1e-6)
+ objectness_label_surface = euclidean_dist_line.new_zeros(
+ num_proposals * 6, dtype=torch.long)
+ objectness_mask_surface = euclidean_dist_line.new_zeros(num_proposals *
+ 6)
+ objectness_label_line = euclidean_dist_line.new_zeros(
+ num_proposals * 12, dtype=torch.long)
+ objectness_mask_line = euclidean_dist_line.new_zeros(num_proposals *
+ 12)
+ objectness_label_surface_sem = euclidean_dist_line.new_zeros(
+ num_proposals * 6, dtype=torch.long)
+ objectness_label_line_sem = euclidean_dist_line.new_zeros(
+ num_proposals * 12, dtype=torch.long)
+
+ euclidean_dist_obj_surface = torch.sqrt((
+ (pred_obj_surface_center - surface_sel)**2).sum(dim=-1) + 1e-6)
+ euclidean_dist_obj_line = torch.sqrt(
+ torch.sum((pred_obj_line_center - line_sel)**2, dim=-1) + 1e-6)
+
+ # Objectness score just with centers
+ proposal_objectness_label[
+ euclidean_dist1 < self.train_cfg['near_threshold']] = 1
+ proposal_objectness_mask[
+ euclidean_dist1 < self.train_cfg['near_threshold']] = 1
+ proposal_objectness_mask[
+ euclidean_dist1 > self.train_cfg['far_threshold']] = 1
+
+ objectness_label_surface[
+ (euclidean_dist_obj_surface <
+ self.train_cfg['label_surface_threshold']) *
+ (euclidean_dist_surface <
+ self.train_cfg['mask_surface_threshold'])] = 1
+ objectness_label_surface_sem[
+ (euclidean_dist_obj_surface <
+ self.train_cfg['label_surface_threshold']) *
+ (euclidean_dist_surface < self.train_cfg['mask_surface_threshold'])
+ * (surface_sel_sem == surface_sel_sem_gt)] = 1
+
+ objectness_label_line[
+ (euclidean_dist_obj_line < self.train_cfg['label_line_threshold'])
+ *
+ (euclidean_dist_line < self.train_cfg['mask_line_threshold'])] = 1
+ objectness_label_line_sem[
+ (euclidean_dist_obj_line < self.train_cfg['label_line_threshold'])
+ * (euclidean_dist_line < self.train_cfg['mask_line_threshold']) *
+ (line_sel_sem == line_sel_sem_gt)] = 1
+
+ objectness_label_surface_obj = proposal_objectness_label.repeat(6)
+ objectness_mask_surface_obj = proposal_objectness_mask.repeat(6)
+ objectness_label_line_obj = proposal_objectness_label.repeat(12)
+ objectness_mask_line_obj = proposal_objectness_mask.repeat(12)
+
+ objectness_mask_surface = objectness_mask_surface_obj
+ objectness_mask_line = objectness_mask_line_obj
+
+ cues_objectness_label = torch.cat(
+ (objectness_label_surface, objectness_label_line), 0)
+ cues_sem_label = torch.cat(
+ (objectness_label_surface_sem, objectness_label_line_sem), 0)
+ cues_mask = torch.cat((objectness_mask_surface, objectness_mask_line),
+ 0)
+
+ objectness_label_surface *= objectness_label_surface_obj
+ objectness_label_line *= objectness_label_line_obj
+ cues_matching_label = torch.cat(
+ (objectness_label_surface, objectness_label_line), 0)
+
+ objectness_label_surface_sem *= objectness_label_surface_obj
+ objectness_label_line_sem *= objectness_label_line_obj
+
+ cues_match_mask = (torch.sum(
+ cues_objectness_label.view(18, num_proposals), dim=0) >=
+ 1).float()
+
+ obj_surface_line_center = torch.cat(
+ (obj_surface_center, obj_line_center), 1).squeeze(0)
+
+ return (cues_objectness_label, cues_sem_label,
+ proposal_objectness_label, cues_mask, cues_match_mask,
+ proposal_objectness_mask, cues_matching_label,
+ obj_surface_line_center)
diff --git a/mmdet3d/models/roi_heads/bbox_heads/parta2_bbox_head.py b/mmdet3d/models/roi_heads/bbox_heads/parta2_bbox_head.py
new file mode 100644
index 0000000..6f5ea72
--- /dev/null
+++ b/mmdet3d/models/roi_heads/bbox_heads/parta2_bbox_head.py
@@ -0,0 +1,629 @@
+# Copyright (c) OpenMMLab. All rights reserved.
+import numpy as np
+import torch
+from mmcv.cnn import ConvModule, normal_init
+
+from mmdet3d.ops.spconv import IS_SPCONV2_AVAILABLE
+
+if IS_SPCONV2_AVAILABLE:
+ from spconv.pytorch import (SparseConvTensor, SparseMaxPool3d,
+ SparseSequential)
+else:
+ from mmcv.ops import SparseConvTensor, SparseMaxPool3d, SparseSequential
+
+from mmcv.runner import BaseModule
+from torch import nn as nn
+
+from mmdet3d.core.bbox.structures import (LiDARInstance3DBoxes,
+ rotation_3d_in_axis, xywhr2xyxyr)
+from mmdet3d.core.post_processing import nms_bev, nms_normal_bev
+from mmdet3d.models.builder import HEADS, build_loss
+from mmdet3d.ops import make_sparse_convmodule
+from mmdet.core import build_bbox_coder, multi_apply
+
+
+@HEADS.register_module()
+class PartA2BboxHead(BaseModule):
+ """PartA2 RoI head.
+
+ Args:
+ num_classes (int): The number of classes to prediction.
+ seg_in_channels (int): Input channels of segmentation
+ convolution layer.
+ part_in_channels (int): Input channels of part convolution layer.
+ seg_conv_channels (list(int)): Out channels of each
+ segmentation convolution layer.
+ part_conv_channels (list(int)): Out channels of each
+ part convolution layer.
+ merge_conv_channels (list(int)): Out channels of each
+ feature merged convolution layer.
+ down_conv_channels (list(int)): Out channels of each
+ downsampled convolution layer.
+ shared_fc_channels (list(int)): Out channels of each shared fc layer.
+ cls_channels (list(int)): Out channels of each classification layer.
+ reg_channels (list(int)): Out channels of each regression layer.
+ dropout_ratio (float): Dropout ratio of classification and
+ regression layers.
+ roi_feat_size (int): The size of pooled roi features.
+ with_corner_loss (bool): Whether to use corner loss or not.
+ bbox_coder (:obj:`BaseBBoxCoder`): Bbox coder for box head.
+ conv_cfg (dict): Config dict of convolutional layers
+ norm_cfg (dict): Config dict of normalization layers
+ loss_bbox (dict): Config dict of box regression loss.
+ loss_cls (dict): Config dict of classifacation loss.
+ """
+
+ def __init__(self,
+ num_classes,
+ seg_in_channels,
+ part_in_channels,
+ seg_conv_channels=None,
+ part_conv_channels=None,
+ merge_conv_channels=None,
+ down_conv_channels=None,
+ shared_fc_channels=None,
+ cls_channels=None,
+ reg_channels=None,
+ dropout_ratio=0.1,
+ roi_feat_size=14,
+ with_corner_loss=True,
+ bbox_coder=dict(type='DeltaXYZWLHRBBoxCoder'),
+ conv_cfg=dict(type='Conv1d'),
+ norm_cfg=dict(type='BN1d', eps=1e-3, momentum=0.01),
+ loss_bbox=dict(
+ type='SmoothL1Loss', beta=1.0 / 9.0, loss_weight=2.0),
+ loss_cls=dict(
+ type='CrossEntropyLoss',
+ use_sigmoid=True,
+ reduction='none',
+ loss_weight=1.0),
+ init_cfg=None):
+ super(PartA2BboxHead, self).__init__(init_cfg=init_cfg)
+ self.num_classes = num_classes
+ self.with_corner_loss = with_corner_loss
+ self.bbox_coder = build_bbox_coder(bbox_coder)
+ self.loss_bbox = build_loss(loss_bbox)
+ self.loss_cls = build_loss(loss_cls)
+ self.use_sigmoid_cls = loss_cls.get('use_sigmoid', False)
+
+ assert down_conv_channels[-1] == shared_fc_channels[0]
+
+ # init layers
+ part_channel_last = part_in_channels
+ part_conv = []
+ for i, channel in enumerate(part_conv_channels):
+ part_conv.append(
+ make_sparse_convmodule(
+ part_channel_last,
+ channel,
+ 3,
+ padding=1,
+ norm_cfg=norm_cfg,
+ indice_key=f'rcnn_part{i}',
+ conv_type='SubMConv3d'))
+ part_channel_last = channel
+ self.part_conv = SparseSequential(*part_conv)
+
+ seg_channel_last = seg_in_channels
+ seg_conv = []
+ for i, channel in enumerate(seg_conv_channels):
+ seg_conv.append(
+ make_sparse_convmodule(
+ seg_channel_last,
+ channel,
+ 3,
+ padding=1,
+ norm_cfg=norm_cfg,
+ indice_key=f'rcnn_seg{i}',
+ conv_type='SubMConv3d'))
+ seg_channel_last = channel
+ self.seg_conv = SparseSequential(*seg_conv)
+
+ self.conv_down = SparseSequential()
+
+ merge_conv_channel_last = part_channel_last + seg_channel_last
+ merge_conv = []
+ for i, channel in enumerate(merge_conv_channels):
+ merge_conv.append(
+ make_sparse_convmodule(
+ merge_conv_channel_last,
+ channel,
+ 3,
+ padding=1,
+ norm_cfg=norm_cfg,
+ indice_key='rcnn_down0'))
+ merge_conv_channel_last = channel
+
+ down_conv_channel_last = merge_conv_channel_last
+ conv_down = []
+ for i, channel in enumerate(down_conv_channels):
+ conv_down.append(
+ make_sparse_convmodule(
+ down_conv_channel_last,
+ channel,
+ 3,
+ padding=1,
+ norm_cfg=norm_cfg,
+ indice_key='rcnn_down1'))
+ down_conv_channel_last = channel
+
+ self.conv_down.add_module('merge_conv', SparseSequential(*merge_conv))
+ self.conv_down.add_module('max_pool3d',
+ SparseMaxPool3d(kernel_size=2, stride=2))
+ self.conv_down.add_module('down_conv', SparseSequential(*conv_down))
+
+ shared_fc_list = []
+ pool_size = roi_feat_size // 2
+ pre_channel = shared_fc_channels[0] * pool_size**3
+ for k in range(1, len(shared_fc_channels)):
+ shared_fc_list.append(
+ ConvModule(
+ pre_channel,
+ shared_fc_channels[k],
+ 1,
+ padding=0,
+ conv_cfg=conv_cfg,
+ norm_cfg=norm_cfg,
+ inplace=True))
+ pre_channel = shared_fc_channels[k]
+
+ if k != len(shared_fc_channels) - 1 and dropout_ratio > 0:
+ shared_fc_list.append(nn.Dropout(dropout_ratio))
+
+ self.shared_fc = nn.Sequential(*shared_fc_list)
+
+ # Classification layer
+ channel_in = shared_fc_channels[-1]
+ cls_channel = 1
+ cls_layers = []
+ pre_channel = channel_in
+ for k in range(0, len(cls_channels)):
+ cls_layers.append(
+ ConvModule(
+ pre_channel,
+ cls_channels[k],
+ 1,
+ padding=0,
+ conv_cfg=conv_cfg,
+ norm_cfg=norm_cfg,
+ inplace=True))
+ pre_channel = cls_channels[k]
+ cls_layers.append(
+ ConvModule(
+ pre_channel,
+ cls_channel,
+ 1,
+ padding=0,
+ conv_cfg=conv_cfg,
+ act_cfg=None))
+ if dropout_ratio >= 0:
+ cls_layers.insert(1, nn.Dropout(dropout_ratio))
+
+ self.conv_cls = nn.Sequential(*cls_layers)
+
+ # Regression layer
+ reg_layers = []
+ pre_channel = channel_in
+ for k in range(0, len(reg_channels)):
+ reg_layers.append(
+ ConvModule(
+ pre_channel,
+ reg_channels[k],
+ 1,
+ padding=0,
+ conv_cfg=conv_cfg,
+ norm_cfg=norm_cfg,
+ inplace=True))
+ pre_channel = reg_channels[k]
+ reg_layers.append(
+ ConvModule(
+ pre_channel,
+ self.bbox_coder.code_size,
+ 1,
+ padding=0,
+ conv_cfg=conv_cfg,
+ act_cfg=None))
+ if dropout_ratio >= 0:
+ reg_layers.insert(1, nn.Dropout(dropout_ratio))
+
+ self.conv_reg = nn.Sequential(*reg_layers)
+
+ if init_cfg is None:
+ self.init_cfg = dict(
+ type='Xavier',
+ layer=['Conv2d', 'Conv1d'],
+ distribution='uniform')
+
+ def init_weights(self):
+ super().init_weights()
+ normal_init(self.conv_reg[-1].conv, mean=0, std=0.001)
+
+ def forward(self, seg_feats, part_feats):
+ """Forward pass.
+
+ Args:
+ seg_feats (torch.Tensor): Point-wise semantic features.
+ part_feats (torch.Tensor): Point-wise part prediction features.
+
+ Returns:
+ tuple[torch.Tensor]: Score of class and bbox predictions.
+ """
+ # (B * N, out_x, out_y, out_z, 4)
+ rcnn_batch_size = part_feats.shape[0]
+
+ # transform to sparse tensors
+ sparse_shape = part_feats.shape[1:4]
+ # (non_empty_num, 4) ==> [bs_idx, x_idx, y_idx, z_idx]
+ sparse_idx = part_feats.sum(dim=-1).nonzero(as_tuple=False)
+
+ part_features = part_feats[sparse_idx[:, 0], sparse_idx[:, 1],
+ sparse_idx[:, 2], sparse_idx[:, 3]]
+ seg_features = seg_feats[sparse_idx[:, 0], sparse_idx[:, 1],
+ sparse_idx[:, 2], sparse_idx[:, 3]]
+ coords = sparse_idx.int().contiguous()
+ part_features = SparseConvTensor(part_features, coords, sparse_shape,
+ rcnn_batch_size)
+ seg_features = SparseConvTensor(seg_features, coords, sparse_shape,
+ rcnn_batch_size)
+
+ # forward rcnn network
+ x_part = self.part_conv(part_features)
+ x_rpn = self.seg_conv(seg_features)
+
+ merged_feature = torch.cat((x_rpn.features, x_part.features),
+ dim=1) # (N, C)
+ shared_feature = SparseConvTensor(merged_feature, coords, sparse_shape,
+ rcnn_batch_size)
+
+ x = self.conv_down(shared_feature)
+
+ shared_feature = x.dense().view(rcnn_batch_size, -1, 1)
+
+ shared_feature = self.shared_fc(shared_feature)
+
+ cls_score = self.conv_cls(shared_feature).transpose(
+ 1, 2).contiguous().squeeze(dim=1) # (B, 1)
+ bbox_pred = self.conv_reg(shared_feature).transpose(
+ 1, 2).contiguous().squeeze(dim=1) # (B, C)
+
+ return cls_score, bbox_pred
+
+ def loss(self, cls_score, bbox_pred, rois, labels, bbox_targets,
+ pos_gt_bboxes, reg_mask, label_weights, bbox_weights):
+ """Computing losses.
+
+ Args:
+ cls_score (torch.Tensor): Scores of each roi.
+ bbox_pred (torch.Tensor): Predictions of bboxes.
+ rois (torch.Tensor): Roi bboxes.
+ labels (torch.Tensor): Labels of class.
+ bbox_targets (torch.Tensor): Target of positive bboxes.
+ pos_gt_bboxes (torch.Tensor): Ground truths of positive bboxes.
+ reg_mask (torch.Tensor): Mask for positive bboxes.
+ label_weights (torch.Tensor): Weights of class loss.
+ bbox_weights (torch.Tensor): Weights of bbox loss.
+
+ Returns:
+ dict: Computed losses.
+
+ - loss_cls (torch.Tensor): Loss of classes.
+ - loss_bbox (torch.Tensor): Loss of bboxes.
+ - loss_corner (torch.Tensor): Loss of corners.
+ """
+ losses = dict()
+ rcnn_batch_size = cls_score.shape[0]
+
+ # calculate class loss
+ cls_flat = cls_score.view(-1)
+ loss_cls = self.loss_cls(cls_flat, labels, label_weights)
+ losses['loss_cls'] = loss_cls
+
+ # calculate regression loss
+ code_size = self.bbox_coder.code_size
+ pos_inds = (reg_mask > 0)
+ if pos_inds.any() == 0:
+ # fake a part loss
+ losses['loss_bbox'] = loss_cls.new_tensor(0)
+ if self.with_corner_loss:
+ losses['loss_corner'] = loss_cls.new_tensor(0)
+ else:
+ pos_bbox_pred = bbox_pred.view(rcnn_batch_size, -1)[pos_inds]
+ bbox_weights_flat = bbox_weights[pos_inds].view(-1, 1).repeat(
+ 1, pos_bbox_pred.shape[-1])
+ loss_bbox = self.loss_bbox(
+ pos_bbox_pred.unsqueeze(dim=0), bbox_targets.unsqueeze(dim=0),
+ bbox_weights_flat.unsqueeze(dim=0))
+ losses['loss_bbox'] = loss_bbox
+
+ if self.with_corner_loss:
+ pos_roi_boxes3d = rois[..., 1:].view(-1, code_size)[pos_inds]
+ pos_roi_boxes3d = pos_roi_boxes3d.view(-1, code_size)
+ batch_anchors = pos_roi_boxes3d.clone().detach()
+ pos_rois_rotation = pos_roi_boxes3d[..., 6].view(-1)
+ roi_xyz = pos_roi_boxes3d[..., 0:3].view(-1, 3)
+ batch_anchors[..., 0:3] = 0
+ # decode boxes
+ pred_boxes3d = self.bbox_coder.decode(
+ batch_anchors,
+ pos_bbox_pred.view(-1, code_size)).view(-1, code_size)
+
+ pred_boxes3d[..., 0:3] = rotation_3d_in_axis(
+ pred_boxes3d[..., 0:3].unsqueeze(1),
+ pos_rois_rotation,
+ axis=2).squeeze(1)
+
+ pred_boxes3d[:, 0:3] += roi_xyz
+
+ # calculate corner loss
+ loss_corner = self.get_corner_loss_lidar(
+ pred_boxes3d, pos_gt_bboxes)
+ losses['loss_corner'] = loss_corner
+
+ return losses
+
+ def get_targets(self, sampling_results, rcnn_train_cfg, concat=True):
+ """Generate targets.
+
+ Args:
+ sampling_results (list[:obj:`SamplingResult`]):
+ Sampled results from rois.
+ rcnn_train_cfg (:obj:`ConfigDict`): Training config of rcnn.
+ concat (bool): Whether to concatenate targets between batches.
+
+ Returns:
+ tuple[torch.Tensor]: Targets of boxes and class prediction.
+ """
+ pos_bboxes_list = [res.pos_bboxes for res in sampling_results]
+ pos_gt_bboxes_list = [res.pos_gt_bboxes for res in sampling_results]
+ iou_list = [res.iou for res in sampling_results]
+ targets = multi_apply(
+ self._get_target_single,
+ pos_bboxes_list,
+ pos_gt_bboxes_list,
+ iou_list,
+ cfg=rcnn_train_cfg)
+
+ (label, bbox_targets, pos_gt_bboxes, reg_mask, label_weights,
+ bbox_weights) = targets
+
+ if concat:
+ label = torch.cat(label, 0)
+ bbox_targets = torch.cat(bbox_targets, 0)
+ pos_gt_bboxes = torch.cat(pos_gt_bboxes, 0)
+ reg_mask = torch.cat(reg_mask, 0)
+
+ label_weights = torch.cat(label_weights, 0)
+ label_weights /= torch.clamp(label_weights.sum(), min=1.0)
+
+ bbox_weights = torch.cat(bbox_weights, 0)
+ bbox_weights /= torch.clamp(bbox_weights.sum(), min=1.0)
+
+ return (label, bbox_targets, pos_gt_bboxes, reg_mask, label_weights,
+ bbox_weights)
+
+ def _get_target_single(self, pos_bboxes, pos_gt_bboxes, ious, cfg):
+ """Generate training targets for a single sample.
+
+ Args:
+ pos_bboxes (torch.Tensor): Positive boxes with shape
+ (N, 7).
+ pos_gt_bboxes (torch.Tensor): Ground truth boxes with shape
+ (M, 7).
+ ious (torch.Tensor): IoU between `pos_bboxes` and `pos_gt_bboxes`
+ in shape (N, M).
+ cfg (dict): Training configs.
+
+ Returns:
+ tuple[torch.Tensor]: Target for positive boxes.
+ (label, bbox_targets, pos_gt_bboxes, reg_mask, label_weights,
+ bbox_weights)
+ """
+ cls_pos_mask = ious > cfg.cls_pos_thr
+ cls_neg_mask = ious < cfg.cls_neg_thr
+ interval_mask = (cls_pos_mask == 0) & (cls_neg_mask == 0)
+
+ # iou regression target
+ label = (cls_pos_mask > 0).float()
+ label[interval_mask] = ious[interval_mask] * 2 - 0.5
+ # label weights
+ label_weights = (label >= 0).float()
+
+ # box regression target
+ reg_mask = pos_bboxes.new_zeros(ious.size(0)).long()
+ reg_mask[0:pos_gt_bboxes.size(0)] = 1
+ bbox_weights = (reg_mask > 0).float()
+ if reg_mask.bool().any():
+ pos_gt_bboxes_ct = pos_gt_bboxes.clone().detach()
+ roi_center = pos_bboxes[..., 0:3]
+ roi_ry = pos_bboxes[..., 6] % (2 * np.pi)
+
+ # canonical transformation
+ pos_gt_bboxes_ct[..., 0:3] -= roi_center
+ pos_gt_bboxes_ct[..., 6] -= roi_ry
+ pos_gt_bboxes_ct[..., 0:3] = rotation_3d_in_axis(
+ pos_gt_bboxes_ct[..., 0:3].unsqueeze(1), -roi_ry,
+ axis=2).squeeze(1)
+
+ # flip orientation if rois have opposite orientation
+ ry_label = pos_gt_bboxes_ct[..., 6] % (2 * np.pi) # 0 ~ 2pi
+ opposite_flag = (ry_label > np.pi * 0.5) & (ry_label < np.pi * 1.5)
+ ry_label[opposite_flag] = (ry_label[opposite_flag] + np.pi) % (
+ 2 * np.pi) # (0 ~ pi/2, 3pi/2 ~ 2pi)
+ flag = ry_label > np.pi
+ ry_label[flag] = ry_label[flag] - np.pi * 2 # (-pi/2, pi/2)
+ ry_label = torch.clamp(ry_label, min=-np.pi / 2, max=np.pi / 2)
+ pos_gt_bboxes_ct[..., 6] = ry_label
+
+ rois_anchor = pos_bboxes.clone().detach()
+ rois_anchor[:, 0:3] = 0
+ rois_anchor[:, 6] = 0
+ bbox_targets = self.bbox_coder.encode(rois_anchor,
+ pos_gt_bboxes_ct)
+ else:
+ # no fg bbox
+ bbox_targets = pos_gt_bboxes.new_empty((0, 7))
+
+ return (label, bbox_targets, pos_gt_bboxes, reg_mask, label_weights,
+ bbox_weights)
+
+ def get_corner_loss_lidar(self, pred_bbox3d, gt_bbox3d, delta=1.0):
+ """Calculate corner loss of given boxes.
+
+ Args:
+ pred_bbox3d (torch.FloatTensor): Predicted boxes in shape (N, 7).
+ gt_bbox3d (torch.FloatTensor): Ground truth boxes in shape (N, 7).
+ delta (float, optional): huber loss threshold. Defaults to 1.0
+
+ Returns:
+ torch.FloatTensor: Calculated corner loss in shape (N).
+ """
+ assert pred_bbox3d.shape[0] == gt_bbox3d.shape[0]
+
+ # This is a little bit hack here because we assume the box for
+ # Part-A2 is in LiDAR coordinates
+ gt_boxes_structure = LiDARInstance3DBoxes(gt_bbox3d)
+ pred_box_corners = LiDARInstance3DBoxes(pred_bbox3d).corners
+ gt_box_corners = gt_boxes_structure.corners
+
+ # This flip only changes the heading direction of GT boxes
+ gt_bbox3d_flip = gt_boxes_structure.clone()
+ gt_bbox3d_flip.tensor[:, 6] += np.pi
+ gt_box_corners_flip = gt_bbox3d_flip.corners
+
+ corner_dist = torch.min(
+ torch.norm(pred_box_corners - gt_box_corners, dim=2),
+ torch.norm(pred_box_corners - gt_box_corners_flip,
+ dim=2)) # (N, 8)
+ # huber loss
+ abs_error = corner_dist.abs()
+ quadratic = abs_error.clamp(max=delta)
+ linear = (abs_error - quadratic)
+ corner_loss = 0.5 * quadratic**2 + delta * linear
+
+ return corner_loss.mean(dim=1)
+
+ def get_bboxes(self,
+ rois,
+ cls_score,
+ bbox_pred,
+ class_labels,
+ class_pred,
+ img_metas,
+ cfg=None):
+ """Generate bboxes from bbox head predictions.
+
+ Args:
+ rois (torch.Tensor): Roi bounding boxes.
+ cls_score (torch.Tensor): Scores of bounding boxes.
+ bbox_pred (torch.Tensor): Bounding boxes predictions
+ class_labels (torch.Tensor): Label of classes
+ class_pred (torch.Tensor): Score for nms.
+ img_metas (list[dict]): Point cloud and image's meta info.
+ cfg (:obj:`ConfigDict`): Testing config.
+
+ Returns:
+ list[tuple]: Decoded bbox, scores and labels after nms.
+ """
+ roi_batch_id = rois[..., 0]
+ roi_boxes = rois[..., 1:] # boxes without batch id
+ batch_size = int(roi_batch_id.max().item() + 1)
+
+ # decode boxes
+ roi_ry = roi_boxes[..., 6].view(-1)
+ roi_xyz = roi_boxes[..., 0:3].view(-1, 3)
+ local_roi_boxes = roi_boxes.clone().detach()
+ local_roi_boxes[..., 0:3] = 0
+ rcnn_boxes3d = self.bbox_coder.decode(local_roi_boxes, bbox_pred)
+ rcnn_boxes3d[..., 0:3] = rotation_3d_in_axis(
+ rcnn_boxes3d[..., 0:3].unsqueeze(1), roi_ry, axis=2).squeeze(1)
+ rcnn_boxes3d[:, 0:3] += roi_xyz
+
+ # post processing
+ result_list = []
+ for batch_id in range(batch_size):
+ cur_class_labels = class_labels[batch_id]
+ cur_cls_score = cls_score[roi_batch_id == batch_id].view(-1)
+
+ cur_box_prob = class_pred[batch_id]
+ cur_rcnn_boxes3d = rcnn_boxes3d[roi_batch_id == batch_id]
+ keep = self.multi_class_nms(cur_box_prob, cur_rcnn_boxes3d,
+ cfg.score_thr, cfg.nms_thr,
+ img_metas[batch_id],
+ cfg.use_rotate_nms)
+ selected_bboxes = cur_rcnn_boxes3d[keep]
+ selected_label_preds = cur_class_labels[keep]
+ selected_scores = cur_cls_score[keep]
+
+ result_list.append(
+ (img_metas[batch_id]['box_type_3d'](selected_bboxes,
+ self.bbox_coder.code_size),
+ selected_scores, selected_label_preds))
+ return result_list
+
+ def multi_class_nms(self,
+ box_probs,
+ box_preds,
+ score_thr,
+ nms_thr,
+ input_meta,
+ use_rotate_nms=True):
+ """Multi-class NMS for box head.
+
+ Note:
+ This function has large overlap with the `box3d_multiclass_nms`
+ implemented in `mmdet3d.core.post_processing`. We are considering
+ merging these two functions in the future.
+
+ Args:
+ box_probs (torch.Tensor): Predicted boxes probabitilies in
+ shape (N,).
+ box_preds (torch.Tensor): Predicted boxes in shape (N, 7+C).
+ score_thr (float): Threshold of scores.
+ nms_thr (float): Threshold for NMS.
+ input_meta (dict): Meta information of the current sample.
+ use_rotate_nms (bool, optional): Whether to use rotated nms.
+ Defaults to True.
+
+ Returns:
+ torch.Tensor: Selected indices.
+ """
+ if use_rotate_nms:
+ nms_func = nms_bev
+ else:
+ nms_func = nms_normal_bev
+
+ assert box_probs.shape[
+ 1] == self.num_classes, f'box_probs shape: {str(box_probs.shape)}'
+ selected_list = []
+ selected_labels = []
+ boxes_for_nms = xywhr2xyxyr(input_meta['box_type_3d'](
+ box_preds, self.bbox_coder.code_size).bev)
+
+ score_thresh = score_thr if isinstance(
+ score_thr, list) else [score_thr for x in range(self.num_classes)]
+ nms_thresh = nms_thr if isinstance(
+ nms_thr, list) else [nms_thr for x in range(self.num_classes)]
+ for k in range(0, self.num_classes):
+ class_scores_keep = box_probs[:, k] >= score_thresh[k]
+
+ if class_scores_keep.int().sum() > 0:
+ original_idxs = class_scores_keep.nonzero(
+ as_tuple=False).view(-1)
+ cur_boxes_for_nms = boxes_for_nms[class_scores_keep]
+ cur_rank_scores = box_probs[class_scores_keep, k]
+
+ cur_selected = nms_func(cur_boxes_for_nms, cur_rank_scores,
+ nms_thresh[k])
+
+ if cur_selected.shape[0] == 0:
+ continue
+ selected_list.append(original_idxs[cur_selected])
+ selected_labels.append(
+ torch.full([cur_selected.shape[0]],
+ k + 1,
+ dtype=torch.int64,
+ device=box_preds.device))
+
+ keep = torch.cat(
+ selected_list, dim=0) if len(selected_list) > 0 else []
+ return keep
diff --git a/mmdet3d/models/roi_heads/bbox_heads/point_rcnn_bbox_head.py b/mmdet3d/models/roi_heads/bbox_heads/point_rcnn_bbox_head.py
new file mode 100644
index 0000000..df46921
--- /dev/null
+++ b/mmdet3d/models/roi_heads/bbox_heads/point_rcnn_bbox_head.py
@@ -0,0 +1,575 @@
+# Copyright (c) OpenMMLab. All rights reserved.
+import numpy as np
+import torch
+from mmcv.cnn import ConvModule, normal_init
+from mmcv.cnn.bricks import build_conv_layer
+from mmcv.runner import BaseModule
+from torch import nn as nn
+
+from mmdet3d.core.bbox.structures import (LiDARInstance3DBoxes,
+ rotation_3d_in_axis, xywhr2xyxyr)
+from mmdet3d.core.post_processing import nms_bev, nms_normal_bev
+from mmdet3d.models.builder import HEADS, build_loss
+from mmdet3d.ops import build_sa_module
+from mmdet.core import build_bbox_coder, multi_apply
+
+
+@HEADS.register_module()
+class PointRCNNBboxHead(BaseModule):
+ """PointRCNN RoI Bbox head.
+
+ Args:
+ num_classes (int): The number of classes to prediction.
+ in_channels (int): Input channels of point features.
+ mlp_channels (list[int]): the number of mlp channels
+ pred_layer_cfg (dict, optional): Config of classfication and
+ regression prediction layers. Defaults to None.
+ num_points (tuple, optional): The number of points which each SA
+ module samples. Defaults to (128, 32, -1).
+ radius (tuple, optional): Sampling radius of each SA module.
+ Defaults to (0.2, 0.4, 100).
+ num_samples (tuple, optional): The number of samples for ball query
+ in each SA module. Defaults to (64, 64, 64).
+ sa_channels (tuple, optional): Out channels of each mlp in SA module.
+ Defaults to ((128, 128, 128), (128, 128, 256), (256, 256, 512)).
+ bbox_coder (dict, optional): Config dict of box coders.
+ Defaults to dict(type='DeltaXYZWLHRBBoxCoder').
+ sa_cfg (dict, optional): Config of set abstraction module, which may
+ contain the following keys and values:
+
+ - pool_mod (str): Pool method ('max' or 'avg') for SA modules.
+ - use_xyz (bool): Whether to use xyz as a part of features.
+ - normalize_xyz (bool): Whether to normalize xyz with radii in
+ each SA module.
+ Defaults to dict(type='PointSAModule', pool_mod='max',
+ use_xyz=True).
+ conv_cfg (dict, optional): Config dict of convolutional layers.
+ Defaults to dict(type='Conv1d').
+ norm_cfg (dict, optional): Config dict of normalization layers.
+ Defaults to dict(type='BN1d').
+ act_cfg (dict, optional): Config dict of activation layers.
+ Defaults to dict(type='ReLU').
+ bias (str, optional): Type of bias. Defaults to 'auto'.
+ loss_bbox (dict, optional): Config of regression loss function.
+ Defaults to dict(type='SmoothL1Loss', beta=1.0 / 9.0,
+ reduction='sum', loss_weight=1.0).
+ loss_cls (dict, optional): Config of classification loss function.
+ Defaults to dict(type='CrossEntropyLoss', use_sigmoid=True,
+ reduction='sum', loss_weight=1.0).
+ with_corner_loss (bool, optional): Whether using corner loss.
+ Defaults to True.
+ init_cfg (dict, optional): Config of initialization. Defaults to None.
+ """
+
+ def __init__(
+ self,
+ num_classes,
+ in_channels,
+ mlp_channels,
+ pred_layer_cfg=None,
+ num_points=(128, 32, -1),
+ radius=(0.2, 0.4, 100),
+ num_samples=(64, 64, 64),
+ sa_channels=((128, 128, 128), (128, 128, 256), (256, 256, 512)),
+ bbox_coder=dict(type='DeltaXYZWLHRBBoxCoder'),
+ sa_cfg=dict(type='PointSAModule', pool_mod='max', use_xyz=True),
+ conv_cfg=dict(type='Conv1d'),
+ norm_cfg=dict(type='BN1d'),
+ act_cfg=dict(type='ReLU'),
+ bias='auto',
+ loss_bbox=dict(
+ type='SmoothL1Loss',
+ beta=1.0 / 9.0,
+ reduction='sum',
+ loss_weight=1.0),
+ loss_cls=dict(
+ type='CrossEntropyLoss',
+ use_sigmoid=True,
+ reduction='sum',
+ loss_weight=1.0),
+ with_corner_loss=True,
+ init_cfg=None):
+ super(PointRCNNBboxHead, self).__init__(init_cfg=init_cfg)
+ self.num_classes = num_classes
+ self.num_sa = len(sa_channels)
+ self.with_corner_loss = with_corner_loss
+ self.conv_cfg = conv_cfg
+ self.norm_cfg = norm_cfg
+ self.act_cfg = act_cfg
+ self.bias = bias
+
+ self.loss_bbox = build_loss(loss_bbox)
+ self.loss_cls = build_loss(loss_cls)
+ self.bbox_coder = build_bbox_coder(bbox_coder)
+ self.use_sigmoid_cls = loss_cls.get('use_sigmoid', False)
+
+ self.in_channels = in_channels
+ mlp_channels = [self.in_channels] + mlp_channels
+ shared_mlps = nn.Sequential()
+ for i in range(len(mlp_channels) - 1):
+ shared_mlps.add_module(
+ f'layer{i}',
+ ConvModule(
+ mlp_channels[i],
+ mlp_channels[i + 1],
+ kernel_size=(1, 1),
+ stride=(1, 1),
+ inplace=False,
+ conv_cfg=dict(type='Conv2d')))
+ self.xyz_up_layer = nn.Sequential(*shared_mlps)
+
+ c_out = mlp_channels[-1]
+ self.merge_down_layer = ConvModule(
+ c_out * 2,
+ c_out,
+ kernel_size=(1, 1),
+ stride=(1, 1),
+ inplace=False,
+ conv_cfg=dict(type='Conv2d'))
+
+ pre_channels = c_out
+
+ self.SA_modules = nn.ModuleList()
+ sa_in_channel = pre_channels
+
+ for sa_index in range(self.num_sa):
+ cur_sa_mlps = list(sa_channels[sa_index])
+ cur_sa_mlps = [sa_in_channel] + cur_sa_mlps
+ sa_out_channel = cur_sa_mlps[-1]
+
+ cur_num_points = num_points[sa_index]
+ if cur_num_points <= 0:
+ cur_num_points = None
+ self.SA_modules.append(
+ build_sa_module(
+ num_point=cur_num_points,
+ radius=radius[sa_index],
+ num_sample=num_samples[sa_index],
+ mlp_channels=cur_sa_mlps,
+ cfg=sa_cfg))
+ sa_in_channel = sa_out_channel
+ self.cls_convs = self._add_conv_branch(
+ pred_layer_cfg.in_channels, pred_layer_cfg.cls_conv_channels)
+ self.reg_convs = self._add_conv_branch(
+ pred_layer_cfg.in_channels, pred_layer_cfg.reg_conv_channels)
+
+ prev_channel = pred_layer_cfg.cls_conv_channels[-1]
+ self.conv_cls = build_conv_layer(
+ self.conv_cfg,
+ in_channels=prev_channel,
+ out_channels=self.num_classes,
+ kernel_size=1)
+ prev_channel = pred_layer_cfg.reg_conv_channels[-1]
+ self.conv_reg = build_conv_layer(
+ self.conv_cfg,
+ in_channels=prev_channel,
+ out_channels=self.bbox_coder.code_size * self.num_classes,
+ kernel_size=1)
+
+ if init_cfg is None:
+ self.init_cfg = dict(type='Xavier', layer=['Conv2d', 'Conv1d'])
+
+ def _add_conv_branch(self, in_channels, conv_channels):
+ """Add shared or separable branch.
+
+ Args:
+ in_channels (int): Input feature channel.
+ conv_channels (tuple): Middle feature channels.
+ """
+ conv_spec = [in_channels] + list(conv_channels)
+ # add branch specific conv layers
+ conv_layers = nn.Sequential()
+ for i in range(len(conv_spec) - 1):
+ conv_layers.add_module(
+ f'layer{i}',
+ ConvModule(
+ conv_spec[i],
+ conv_spec[i + 1],
+ kernel_size=1,
+ padding=0,
+ conv_cfg=self.conv_cfg,
+ norm_cfg=self.norm_cfg,
+ act_cfg=self.act_cfg,
+ bias=self.bias,
+ inplace=True))
+ return conv_layers
+
+ def init_weights(self):
+ """Initialize weights of the head."""
+ super().init_weights()
+ for m in self.modules():
+ if isinstance(m, nn.Conv2d) or isinstance(m, nn.Conv1d):
+ if m.bias is not None:
+ nn.init.constant_(m.bias, 0)
+ normal_init(self.conv_reg.weight, mean=0, std=0.001)
+
+ def forward(self, feats):
+ """Forward pass.
+
+ Args:
+ feats (torch.Torch): Features from RCNN modules.
+
+ Returns:
+ tuple[torch.Tensor]: Score of class and bbox predictions.
+ """
+ input_data = feats.clone().detach()
+ xyz_input = input_data[..., 0:self.in_channels].transpose(
+ 1, 2).unsqueeze(dim=3).contiguous().clone().detach()
+ xyz_features = self.xyz_up_layer(xyz_input)
+ rpn_features = input_data[..., self.in_channels:].transpose(
+ 1, 2).unsqueeze(dim=3)
+ merged_features = torch.cat((xyz_features, rpn_features), dim=1)
+ merged_features = self.merge_down_layer(merged_features)
+ l_xyz, l_features = [input_data[..., 0:3].contiguous()], \
+ [merged_features.squeeze(dim=3)]
+ for i in range(len(self.SA_modules)):
+ li_xyz, li_features, cur_indices = \
+ self.SA_modules[i](l_xyz[i], l_features[i])
+ l_xyz.append(li_xyz)
+ l_features.append(li_features)
+
+ shared_features = l_features[-1]
+ x_cls = shared_features
+ x_reg = shared_features
+ x_cls = self.cls_convs(x_cls)
+ rcnn_cls = self.conv_cls(x_cls)
+ x_reg = self.reg_convs(x_reg)
+ rcnn_reg = self.conv_reg(x_reg)
+ rcnn_cls = rcnn_cls.transpose(1, 2).contiguous().squeeze(dim=1)
+ rcnn_reg = rcnn_reg.transpose(1, 2).contiguous().squeeze(dim=1)
+ return rcnn_cls, rcnn_reg
+
+ def loss(self, cls_score, bbox_pred, rois, labels, bbox_targets,
+ pos_gt_bboxes, reg_mask, label_weights, bbox_weights):
+ """Computing losses.
+
+ Args:
+ cls_score (torch.Tensor): Scores of each RoI.
+ bbox_pred (torch.Tensor): Predictions of bboxes.
+ rois (torch.Tensor): RoI bboxes.
+ labels (torch.Tensor): Labels of class.
+ bbox_targets (torch.Tensor): Target of positive bboxes.
+ pos_gt_bboxes (torch.Tensor): Ground truths of positive bboxes.
+ reg_mask (torch.Tensor): Mask for positive bboxes.
+ label_weights (torch.Tensor): Weights of class loss.
+ bbox_weights (torch.Tensor): Weights of bbox loss.
+
+ Returns:
+ dict: Computed losses.
+
+ - loss_cls (torch.Tensor): Loss of classes.
+ - loss_bbox (torch.Tensor): Loss of bboxes.
+ - loss_corner (torch.Tensor): Loss of corners.
+ """
+ losses = dict()
+ rcnn_batch_size = cls_score.shape[0]
+ # calculate class loss
+ cls_flat = cls_score.view(-1)
+ loss_cls = self.loss_cls(cls_flat, labels, label_weights)
+ losses['loss_cls'] = loss_cls
+
+ # calculate regression loss
+ code_size = self.bbox_coder.code_size
+ pos_inds = (reg_mask > 0)
+
+ pos_bbox_pred = bbox_pred.view(rcnn_batch_size, -1)[pos_inds].clone()
+ bbox_weights_flat = bbox_weights[pos_inds].view(-1, 1).repeat(
+ 1, pos_bbox_pred.shape[-1])
+ loss_bbox = self.loss_bbox(
+ pos_bbox_pred.unsqueeze(dim=0),
+ bbox_targets.unsqueeze(dim=0).detach(),
+ bbox_weights_flat.unsqueeze(dim=0))
+ losses['loss_bbox'] = loss_bbox
+
+ if pos_inds.any() != 0 and self.with_corner_loss:
+ rois = rois.detach()
+ pos_roi_boxes3d = rois[..., 1:].view(-1, code_size)[pos_inds]
+ pos_roi_boxes3d = pos_roi_boxes3d.view(-1, code_size)
+ batch_anchors = pos_roi_boxes3d.clone().detach()
+ pos_rois_rotation = pos_roi_boxes3d[..., 6].view(-1)
+ roi_xyz = pos_roi_boxes3d[..., 0:3].view(-1, 3)
+ batch_anchors[..., 0:3] = 0
+ # decode boxes
+ pred_boxes3d = self.bbox_coder.decode(
+ batch_anchors,
+ pos_bbox_pred.view(-1, code_size)).view(-1, code_size)
+
+ pred_boxes3d[..., 0:3] = rotation_3d_in_axis(
+ pred_boxes3d[..., 0:3].unsqueeze(1), (pos_rois_rotation),
+ axis=2).squeeze(1)
+
+ pred_boxes3d[:, 0:3] += roi_xyz
+
+ # calculate corner loss
+ loss_corner = self.get_corner_loss_lidar(pred_boxes3d,
+ pos_gt_bboxes)
+
+ losses['loss_corner'] = loss_corner
+ else:
+ losses['loss_corner'] = loss_cls.new_tensor(0)
+
+ return losses
+
+ def get_corner_loss_lidar(self, pred_bbox3d, gt_bbox3d, delta=1.0):
+ """Calculate corner loss of given boxes.
+
+ Args:
+ pred_bbox3d (torch.FloatTensor): Predicted boxes in shape (N, 7).
+ gt_bbox3d (torch.FloatTensor): Ground truth boxes in shape (N, 7).
+ delta (float, optional): huber loss threshold. Defaults to 1.0
+
+ Returns:
+ torch.FloatTensor: Calculated corner loss in shape (N).
+ """
+ assert pred_bbox3d.shape[0] == gt_bbox3d.shape[0]
+
+ # This is a little bit hack here because we assume the box for
+ # PointRCNN is in LiDAR coordinates
+
+ gt_boxes_structure = LiDARInstance3DBoxes(gt_bbox3d)
+ pred_box_corners = LiDARInstance3DBoxes(pred_bbox3d).corners
+ gt_box_corners = gt_boxes_structure.corners
+
+ # This flip only changes the heading direction of GT boxes
+ gt_bbox3d_flip = gt_boxes_structure.clone()
+ gt_bbox3d_flip.tensor[:, 6] += np.pi
+ gt_box_corners_flip = gt_bbox3d_flip.corners
+
+ corner_dist = torch.min(
+ torch.norm(pred_box_corners - gt_box_corners, dim=2),
+ torch.norm(pred_box_corners - gt_box_corners_flip, dim=2))
+ # huber loss
+ abs_error = corner_dist.abs()
+ quadratic = abs_error.clamp(max=delta)
+ linear = (abs_error - quadratic)
+ corner_loss = 0.5 * quadratic**2 + delta * linear
+ return corner_loss.mean(dim=1)
+
+ def get_targets(self, sampling_results, rcnn_train_cfg, concat=True):
+ """Generate targets.
+
+ Args:
+ sampling_results (list[:obj:`SamplingResult`]):
+ Sampled results from rois.
+ rcnn_train_cfg (:obj:`ConfigDict`): Training config of rcnn.
+ concat (bool, optional): Whether to concatenate targets between
+ batches. Defaults to True.
+
+ Returns:
+ tuple[torch.Tensor]: Targets of boxes and class prediction.
+ """
+ pos_bboxes_list = [res.pos_bboxes for res in sampling_results]
+ pos_gt_bboxes_list = [res.pos_gt_bboxes for res in sampling_results]
+ iou_list = [res.iou for res in sampling_results]
+ targets = multi_apply(
+ self._get_target_single,
+ pos_bboxes_list,
+ pos_gt_bboxes_list,
+ iou_list,
+ cfg=rcnn_train_cfg)
+ (label, bbox_targets, pos_gt_bboxes, reg_mask, label_weights,
+ bbox_weights) = targets
+
+ if concat:
+ label = torch.cat(label, 0)
+ bbox_targets = torch.cat(bbox_targets, 0)
+ pos_gt_bboxes = torch.cat(pos_gt_bboxes, 0)
+ reg_mask = torch.cat(reg_mask, 0)
+
+ label_weights = torch.cat(label_weights, 0)
+ label_weights /= torch.clamp(label_weights.sum(), min=1.0)
+
+ bbox_weights = torch.cat(bbox_weights, 0)
+ bbox_weights /= torch.clamp(bbox_weights.sum(), min=1.0)
+
+ return (label, bbox_targets, pos_gt_bboxes, reg_mask, label_weights,
+ bbox_weights)
+
+ def _get_target_single(self, pos_bboxes, pos_gt_bboxes, ious, cfg):
+ """Generate training targets for a single sample.
+
+ Args:
+ pos_bboxes (torch.Tensor): Positive boxes with shape
+ (N, 7).
+ pos_gt_bboxes (torch.Tensor): Ground truth boxes with shape
+ (M, 7).
+ ious (torch.Tensor): IoU between `pos_bboxes` and `pos_gt_bboxes`
+ in shape (N, M).
+ cfg (dict): Training configs.
+
+ Returns:
+ tuple[torch.Tensor]: Target for positive boxes.
+ (label, bbox_targets, pos_gt_bboxes, reg_mask, label_weights,
+ bbox_weights)
+ """
+ cls_pos_mask = ious > cfg.cls_pos_thr
+ cls_neg_mask = ious < cfg.cls_neg_thr
+ interval_mask = (cls_pos_mask == 0) & (cls_neg_mask == 0)
+ # iou regression target
+ label = (cls_pos_mask > 0).float()
+ label[interval_mask] = (ious[interval_mask] - cfg.cls_neg_thr) / \
+ (cfg.cls_pos_thr - cfg.cls_neg_thr)
+ # label weights
+ label_weights = (label >= 0).float()
+ # box regression target
+ reg_mask = pos_bboxes.new_zeros(ious.size(0)).long()
+ reg_mask[0:pos_gt_bboxes.size(0)] = 1
+ bbox_weights = (reg_mask > 0).float()
+ if reg_mask.bool().any():
+ pos_gt_bboxes_ct = pos_gt_bboxes.clone().detach()
+ roi_center = pos_bboxes[..., 0:3]
+ roi_ry = pos_bboxes[..., 6] % (2 * np.pi)
+
+ # canonical transformation
+ pos_gt_bboxes_ct[..., 0:3] -= roi_center
+ pos_gt_bboxes_ct[..., 6] -= roi_ry
+ pos_gt_bboxes_ct[..., 0:3] = rotation_3d_in_axis(
+ pos_gt_bboxes_ct[..., 0:3].unsqueeze(1), -(roi_ry),
+ axis=2).squeeze(1)
+
+ # flip orientation if gt have opposite orientation
+ ry_label = pos_gt_bboxes_ct[..., 6] % (2 * np.pi) # 0 ~ 2pi
+ is_opposite = (ry_label > np.pi * 0.5) & (ry_label < np.pi * 1.5)
+ ry_label[is_opposite] = (ry_label[is_opposite] + np.pi) % (
+ 2 * np.pi) # (0 ~ pi/2, 3pi/2 ~ 2pi)
+ flag = ry_label > np.pi
+ ry_label[flag] = ry_label[flag] - np.pi * 2 # (-pi/2, pi/2)
+ ry_label = torch.clamp(ry_label, min=-np.pi / 2, max=np.pi / 2)
+ pos_gt_bboxes_ct[..., 6] = ry_label
+
+ rois_anchor = pos_bboxes.clone().detach()
+ rois_anchor[:, 0:3] = 0
+ rois_anchor[:, 6] = 0
+ bbox_targets = self.bbox_coder.encode(rois_anchor,
+ pos_gt_bboxes_ct)
+ else:
+ # no fg bbox
+ bbox_targets = pos_gt_bboxes.new_empty((0, 7))
+
+ return (label, bbox_targets, pos_gt_bboxes, reg_mask, label_weights,
+ bbox_weights)
+
+ def get_bboxes(self,
+ rois,
+ cls_score,
+ bbox_pred,
+ class_labels,
+ img_metas,
+ cfg=None):
+ """Generate bboxes from bbox head predictions.
+
+ Args:
+ rois (torch.Tensor): RoI bounding boxes.
+ cls_score (torch.Tensor): Scores of bounding boxes.
+ bbox_pred (torch.Tensor): Bounding boxes predictions
+ class_labels (torch.Tensor): Label of classes
+ img_metas (list[dict]): Point cloud and image's meta info.
+ cfg (:obj:`ConfigDict`, optional): Testing config.
+ Defaults to None.
+
+ Returns:
+ list[tuple]: Decoded bbox, scores and labels after nms.
+ """
+ roi_batch_id = rois[..., 0]
+ roi_boxes = rois[..., 1:] # boxes without batch id
+ batch_size = int(roi_batch_id.max().item() + 1)
+
+ # decode boxes
+ roi_ry = roi_boxes[..., 6].view(-1)
+ roi_xyz = roi_boxes[..., 0:3].view(-1, 3)
+ local_roi_boxes = roi_boxes.clone().detach()
+ local_roi_boxes[..., 0:3] = 0
+ rcnn_boxes3d = self.bbox_coder.decode(local_roi_boxes, bbox_pred)
+ rcnn_boxes3d[..., 0:3] = rotation_3d_in_axis(
+ rcnn_boxes3d[..., 0:3].unsqueeze(1), roi_ry, axis=2).squeeze(1)
+ rcnn_boxes3d[:, 0:3] += roi_xyz
+
+ # post processing
+ result_list = []
+ for batch_id in range(batch_size):
+ cur_class_labels = class_labels[batch_id]
+ cur_cls_score = cls_score[roi_batch_id == batch_id].view(-1)
+
+ cur_box_prob = cur_cls_score.unsqueeze(1)
+ cur_rcnn_boxes3d = rcnn_boxes3d[roi_batch_id == batch_id]
+ keep = self.multi_class_nms(cur_box_prob, cur_rcnn_boxes3d,
+ cfg.score_thr, cfg.nms_thr,
+ img_metas[batch_id],
+ cfg.use_rotate_nms)
+ selected_bboxes = cur_rcnn_boxes3d[keep]
+ selected_label_preds = cur_class_labels[keep]
+ selected_scores = cur_cls_score[keep]
+
+ result_list.append(
+ (img_metas[batch_id]['box_type_3d'](selected_bboxes,
+ self.bbox_coder.code_size),
+ selected_scores, selected_label_preds))
+ return result_list
+
+ def multi_class_nms(self,
+ box_probs,
+ box_preds,
+ score_thr,
+ nms_thr,
+ input_meta,
+ use_rotate_nms=True):
+ """Multi-class NMS for box head.
+
+ Note:
+ This function has large overlap with the `box3d_multiclass_nms`
+ implemented in `mmdet3d.core.post_processing`. We are considering
+ merging these two functions in the future.
+
+ Args:
+ box_probs (torch.Tensor): Predicted boxes probabilities in
+ shape (N,).
+ box_preds (torch.Tensor): Predicted boxes in shape (N, 7+C).
+ score_thr (float): Threshold of scores.
+ nms_thr (float): Threshold for NMS.
+ input_meta (dict): Meta information of the current sample.
+ use_rotate_nms (bool, optional): Whether to use rotated nms.
+ Defaults to True.
+
+ Returns:
+ torch.Tensor: Selected indices.
+ """
+ if use_rotate_nms:
+ nms_func = nms_bev
+ else:
+ nms_func = nms_normal_bev
+
+ assert box_probs.shape[
+ 1] == self.num_classes, f'box_probs shape: {str(box_probs.shape)}'
+ selected_list = []
+ selected_labels = []
+ boxes_for_nms = xywhr2xyxyr(input_meta['box_type_3d'](
+ box_preds, self.bbox_coder.code_size).bev)
+
+ score_thresh = score_thr if isinstance(
+ score_thr, list) else [score_thr for x in range(self.num_classes)]
+ nms_thresh = nms_thr if isinstance(
+ nms_thr, list) else [nms_thr for x in range(self.num_classes)]
+ for k in range(0, self.num_classes):
+ class_scores_keep = box_probs[:, k] >= score_thresh[k]
+
+ if class_scores_keep.int().sum() > 0:
+ original_idxs = class_scores_keep.nonzero(
+ as_tuple=False).view(-1)
+ cur_boxes_for_nms = boxes_for_nms[class_scores_keep]
+ cur_rank_scores = box_probs[class_scores_keep, k]
+
+ cur_selected = nms_func(cur_boxes_for_nms, cur_rank_scores,
+ nms_thresh[k])
+
+ if cur_selected.shape[0] == 0:
+ continue
+ selected_list.append(original_idxs[cur_selected])
+ selected_labels.append(
+ torch.full([cur_selected.shape[0]],
+ k + 1,
+ dtype=torch.int64,
+ device=box_preds.device))
+
+ keep = torch.cat(
+ selected_list, dim=0) if len(selected_list) > 0 else []
+ return keep
diff --git a/mmdet3d/models/roi_heads/h3d_roi_head.py b/mmdet3d/models/roi_heads/h3d_roi_head.py
new file mode 100644
index 0000000..b6b9597
--- /dev/null
+++ b/mmdet3d/models/roi_heads/h3d_roi_head.py
@@ -0,0 +1,159 @@
+# Copyright (c) OpenMMLab. All rights reserved.
+from mmdet3d.core.bbox import bbox3d2result
+from ..builder import HEADS, build_head
+from .base_3droi_head import Base3DRoIHead
+
+
+@HEADS.register_module()
+class H3DRoIHead(Base3DRoIHead):
+ """H3D roi head for H3DNet.
+
+ Args:
+ primitive_list (List): Configs of primitive heads.
+ bbox_head (ConfigDict): Config of bbox_head.
+ train_cfg (ConfigDict): Training config.
+ test_cfg (ConfigDict): Testing config.
+ """
+
+ def __init__(self,
+ primitive_list,
+ bbox_head=None,
+ train_cfg=None,
+ test_cfg=None,
+ pretrained=None,
+ init_cfg=None):
+ super(H3DRoIHead, self).__init__(
+ bbox_head=bbox_head,
+ train_cfg=train_cfg,
+ test_cfg=test_cfg,
+ pretrained=pretrained,
+ init_cfg=init_cfg)
+ # Primitive module
+ assert len(primitive_list) == 3
+ self.primitive_z = build_head(primitive_list[0])
+ self.primitive_xy = build_head(primitive_list[1])
+ self.primitive_line = build_head(primitive_list[2])
+
+ def init_mask_head(self):
+ """Initialize mask head, skip since ``H3DROIHead`` does not have
+ one."""
+ pass
+
+ def init_bbox_head(self, bbox_head):
+ """Initialize box head."""
+ bbox_head['train_cfg'] = self.train_cfg
+ bbox_head['test_cfg'] = self.test_cfg
+ self.bbox_head = build_head(bbox_head)
+
+ def init_assigner_sampler(self):
+ """Initialize assigner and sampler."""
+ pass
+
+ def forward_train(self,
+ feats_dict,
+ img_metas,
+ points,
+ gt_bboxes_3d,
+ gt_labels_3d,
+ pts_semantic_mask,
+ pts_instance_mask,
+ gt_bboxes_ignore=None):
+ """Training forward function of PartAggregationROIHead.
+
+ Args:
+ feats_dict (dict): Contains features from the first stage.
+ img_metas (list[dict]): Contain pcd and img's meta info.
+ points (list[torch.Tensor]): Input points.
+ gt_bboxes_3d (list[:obj:`BaseInstance3DBoxes`]): Ground truth
+ bboxes of each sample.
+ gt_labels_3d (list[torch.Tensor]): Labels of each sample.
+ pts_semantic_mask (list[torch.Tensor]): Point-wise
+ semantic mask.
+ pts_instance_mask (list[torch.Tensor]): Point-wise
+ instance mask.
+ gt_bboxes_ignore (list[torch.Tensor]): Specify
+ which bounding boxes to ignore.
+
+ Returns:
+ dict: losses from each head.
+ """
+ losses = dict()
+
+ sample_mod = self.train_cfg.sample_mod
+ assert sample_mod in ['vote', 'seed', 'random']
+ result_z = self.primitive_z(feats_dict, sample_mod)
+ feats_dict.update(result_z)
+
+ result_xy = self.primitive_xy(feats_dict, sample_mod)
+ feats_dict.update(result_xy)
+
+ result_line = self.primitive_line(feats_dict, sample_mod)
+ feats_dict.update(result_line)
+
+ primitive_loss_inputs = (feats_dict, points, gt_bboxes_3d,
+ gt_labels_3d, pts_semantic_mask,
+ pts_instance_mask, img_metas,
+ gt_bboxes_ignore)
+
+ loss_z = self.primitive_z.loss(*primitive_loss_inputs)
+ losses.update(loss_z)
+
+ loss_xy = self.primitive_xy.loss(*primitive_loss_inputs)
+ losses.update(loss_xy)
+
+ loss_line = self.primitive_line.loss(*primitive_loss_inputs)
+ losses.update(loss_line)
+
+ targets = feats_dict.pop('targets')
+
+ bbox_results = self.bbox_head(feats_dict, sample_mod)
+
+ feats_dict.update(bbox_results)
+ bbox_loss = self.bbox_head.loss(feats_dict, points, gt_bboxes_3d,
+ gt_labels_3d, pts_semantic_mask,
+ pts_instance_mask, img_metas, targets,
+ gt_bboxes_ignore)
+ losses.update(bbox_loss)
+
+ return losses
+
+ def simple_test(self, feats_dict, img_metas, points, rescale=False):
+ """Simple testing forward function of PartAggregationROIHead.
+
+ Note:
+ This function assumes that the batch size is 1
+
+ Args:
+ feats_dict (dict): Contains features from the first stage.
+ img_metas (list[dict]): Contain pcd and img's meta info.
+ points (torch.Tensor): Input points.
+ rescale (bool): Whether to rescale results.
+
+ Returns:
+ dict: Bbox results of one frame.
+ """
+ sample_mod = self.test_cfg.sample_mod
+ assert sample_mod in ['vote', 'seed', 'random']
+
+ result_z = self.primitive_z(feats_dict, sample_mod)
+ feats_dict.update(result_z)
+
+ result_xy = self.primitive_xy(feats_dict, sample_mod)
+ feats_dict.update(result_xy)
+
+ result_line = self.primitive_line(feats_dict, sample_mod)
+ feats_dict.update(result_line)
+
+ bbox_preds = self.bbox_head(feats_dict, sample_mod)
+ feats_dict.update(bbox_preds)
+ bbox_list = self.bbox_head.get_bboxes(
+ points,
+ feats_dict,
+ img_metas,
+ rescale=rescale,
+ suffix='_optimized')
+ bbox_results = [
+ bbox3d2result(bboxes, scores, labels)
+ for bboxes, scores, labels in bbox_list
+ ]
+ return bbox_results
diff --git a/mmdet3d/models/roi_heads/mask_heads/__init__.py b/mmdet3d/models/roi_heads/mask_heads/__init__.py
new file mode 100644
index 0000000..0aa1156
--- /dev/null
+++ b/mmdet3d/models/roi_heads/mask_heads/__init__.py
@@ -0,0 +1,5 @@
+# Copyright (c) OpenMMLab. All rights reserved.
+from .pointwise_semantic_head import PointwiseSemanticHead
+from .primitive_head import PrimitiveHead
+
+__all__ = ['PointwiseSemanticHead', 'PrimitiveHead']
diff --git a/mmdet3d/models/roi_heads/mask_heads/pointwise_semantic_head.py b/mmdet3d/models/roi_heads/mask_heads/pointwise_semantic_head.py
new file mode 100644
index 0000000..fc0bcf5
--- /dev/null
+++ b/mmdet3d/models/roi_heads/mask_heads/pointwise_semantic_head.py
@@ -0,0 +1,202 @@
+# Copyright (c) OpenMMLab. All rights reserved.
+import torch
+from mmcv.runner import BaseModule
+from torch import nn as nn
+from torch.nn import functional as F
+
+from mmdet3d.core.bbox.structures import rotation_3d_in_axis
+from mmdet3d.models.builder import HEADS, build_loss
+from mmdet.core import multi_apply
+
+
+@HEADS.register_module()
+class PointwiseSemanticHead(BaseModule):
+ """Semantic segmentation head for point-wise segmentation.
+
+ Predict point-wise segmentation and part regression results for PartA2.
+ See `paper `_ for more details.
+
+ Args:
+ in_channels (int): The number of input channel.
+ num_classes (int): The number of class.
+ extra_width (float): Boxes enlarge width.
+ loss_seg (dict): Config of segmentation loss.
+ loss_part (dict): Config of part prediction loss.
+ """
+
+ def __init__(self,
+ in_channels,
+ num_classes=3,
+ extra_width=0.2,
+ seg_score_thr=0.3,
+ init_cfg=None,
+ loss_seg=dict(
+ type='FocalLoss',
+ use_sigmoid=True,
+ reduction='sum',
+ gamma=2.0,
+ alpha=0.25,
+ loss_weight=1.0),
+ loss_part=dict(
+ type='CrossEntropyLoss',
+ use_sigmoid=True,
+ loss_weight=1.0)):
+ super(PointwiseSemanticHead, self).__init__(init_cfg=init_cfg)
+ self.extra_width = extra_width
+ self.num_classes = num_classes
+ self.seg_score_thr = seg_score_thr
+ self.seg_cls_layer = nn.Linear(in_channels, 1, bias=True)
+ self.seg_reg_layer = nn.Linear(in_channels, 3, bias=True)
+
+ self.loss_seg = build_loss(loss_seg)
+ self.loss_part = build_loss(loss_part)
+
+ def forward(self, x):
+ """Forward pass.
+
+ Args:
+ x (torch.Tensor): Features from the first stage.
+
+ Returns:
+ dict: Part features, segmentation and part predictions.
+
+ - seg_preds (torch.Tensor): Segment predictions.
+ - part_preds (torch.Tensor): Part predictions.
+ - part_feats (torch.Tensor): Feature predictions.
+ """
+ seg_preds = self.seg_cls_layer(x) # (N, 1)
+ part_preds = self.seg_reg_layer(x) # (N, 3)
+
+ seg_scores = torch.sigmoid(seg_preds).detach()
+ seg_mask = (seg_scores > self.seg_score_thr)
+
+ part_offsets = torch.sigmoid(part_preds).clone().detach()
+ part_offsets[seg_mask.view(-1) == 0] = 0
+ part_feats = torch.cat((part_offsets, seg_scores),
+ dim=-1) # shape (npoints, 4)
+ return dict(
+ seg_preds=seg_preds, part_preds=part_preds, part_feats=part_feats)
+
+ def get_targets_single(self, voxel_centers, gt_bboxes_3d, gt_labels_3d):
+ """generate segmentation and part prediction targets for a single
+ sample.
+
+ Args:
+ voxel_centers (torch.Tensor): The center of voxels in shape
+ (voxel_num, 3).
+ gt_bboxes_3d (:obj:`BaseInstance3DBoxes`): Ground truth boxes in
+ shape (box_num, 7).
+ gt_labels_3d (torch.Tensor): Class labels of ground truths in
+ shape (box_num).
+
+ Returns:
+ tuple[torch.Tensor]: Segmentation targets with shape [voxel_num]
+ part prediction targets with shape [voxel_num, 3]
+ """
+ gt_bboxes_3d = gt_bboxes_3d.to(voxel_centers.device)
+ enlarged_gt_boxes = gt_bboxes_3d.enlarged_box(self.extra_width)
+
+ part_targets = voxel_centers.new_zeros((voxel_centers.shape[0], 3),
+ dtype=torch.float32)
+ box_idx = gt_bboxes_3d.points_in_boxes_part(voxel_centers)
+ enlarge_box_idx = enlarged_gt_boxes.points_in_boxes_part(
+ voxel_centers).long()
+
+ gt_labels_pad = F.pad(
+ gt_labels_3d, (1, 0), mode='constant', value=self.num_classes)
+ seg_targets = gt_labels_pad[(box_idx.long() + 1)]
+ fg_pt_flag = box_idx > -1
+ ignore_flag = fg_pt_flag ^ (enlarge_box_idx > -1)
+ seg_targets[ignore_flag] = -1
+
+ for k in range(len(gt_bboxes_3d)):
+ k_box_flag = box_idx == k
+ # no point in current box (caused by velodyne reduce)
+ if not k_box_flag.any():
+ continue
+ fg_voxels = voxel_centers[k_box_flag]
+ transformed_voxels = fg_voxels - gt_bboxes_3d.bottom_center[k]
+ transformed_voxels = rotation_3d_in_axis(
+ transformed_voxels.unsqueeze(0),
+ -gt_bboxes_3d.yaw[k].view(1),
+ axis=2)
+ part_targets[k_box_flag] = transformed_voxels / gt_bboxes_3d.dims[
+ k] + voxel_centers.new_tensor([0.5, 0.5, 0])
+
+ part_targets = torch.clamp(part_targets, min=0)
+ return seg_targets, part_targets
+
+ def get_targets(self, voxels_dict, gt_bboxes_3d, gt_labels_3d):
+ """generate segmentation and part prediction targets.
+
+ Args:
+ voxel_centers (torch.Tensor): The center of voxels in shape
+ (voxel_num, 3).
+ gt_bboxes_3d (:obj:`BaseInstance3DBoxes`): Ground truth boxes in
+ shape (box_num, 7).
+ gt_labels_3d (torch.Tensor): Class labels of ground truths in
+ shape (box_num).
+
+ Returns:
+ dict: Prediction targets
+
+ - seg_targets (torch.Tensor): Segmentation targets
+ with shape [voxel_num].
+ - part_targets (torch.Tensor): Part prediction targets
+ with shape [voxel_num, 3].
+ """
+ batch_size = len(gt_labels_3d)
+ voxel_center_list = []
+ for idx in range(batch_size):
+ coords_idx = voxels_dict['coors'][:, 0] == idx
+ voxel_center_list.append(voxels_dict['voxel_centers'][coords_idx])
+
+ seg_targets, part_targets = multi_apply(self.get_targets_single,
+ voxel_center_list,
+ gt_bboxes_3d, gt_labels_3d)
+ seg_targets = torch.cat(seg_targets, dim=0)
+ part_targets = torch.cat(part_targets, dim=0)
+ return dict(seg_targets=seg_targets, part_targets=part_targets)
+
+ def loss(self, semantic_results, semantic_targets):
+ """Calculate point-wise segmentation and part prediction losses.
+
+ Args:
+ semantic_results (dict): Results from semantic head.
+
+ - seg_preds: Segmentation predictions.
+ - part_preds: Part predictions.
+
+ semantic_targets (dict): Targets of semantic results.
+
+ - seg_preds: Segmentation targets.
+ - part_preds: Part targets.
+
+ Returns:
+ dict: Loss of segmentation and part prediction.
+
+ - loss_seg (torch.Tensor): Segmentation prediction loss.
+ - loss_part (torch.Tensor): Part prediction loss.
+ """
+ seg_preds = semantic_results['seg_preds']
+ part_preds = semantic_results['part_preds']
+ seg_targets = semantic_targets['seg_targets']
+ part_targets = semantic_targets['part_targets']
+
+ pos_mask = (seg_targets > -1) & (seg_targets < self.num_classes)
+ binary_seg_target = pos_mask.long()
+ pos = pos_mask.float()
+ neg = (seg_targets == self.num_classes).float()
+ seg_weights = pos + neg
+ pos_normalizer = pos.sum()
+ seg_weights = seg_weights / torch.clamp(pos_normalizer, min=1.0)
+ loss_seg = self.loss_seg(seg_preds, binary_seg_target, seg_weights)
+
+ if pos_normalizer > 0:
+ loss_part = self.loss_part(part_preds[pos_mask],
+ part_targets[pos_mask])
+ else:
+ # fake a part loss
+ loss_part = loss_seg.new_tensor(0)
+
+ return dict(loss_seg=loss_seg, loss_part=loss_part)
diff --git a/mmdet3d/models/roi_heads/mask_heads/primitive_head.py b/mmdet3d/models/roi_heads/mask_heads/primitive_head.py
new file mode 100644
index 0000000..4c9c28b
--- /dev/null
+++ b/mmdet3d/models/roi_heads/mask_heads/primitive_head.py
@@ -0,0 +1,966 @@
+# Copyright (c) OpenMMLab. All rights reserved.
+import torch
+from mmcv.cnn import ConvModule
+from mmcv.ops import furthest_point_sample
+from mmcv.runner import BaseModule
+from torch import nn as nn
+from torch.nn import functional as F
+
+from mmdet3d.models.builder import HEADS, build_loss
+from mmdet3d.models.model_utils import VoteModule
+from mmdet3d.ops import build_sa_module
+from mmdet.core import multi_apply
+
+
+@HEADS.register_module()
+class PrimitiveHead(BaseModule):
+ r"""Primitive head of `H3DNet `_.
+
+ Args:
+ num_dims (int): The dimension of primitive semantic information.
+ num_classes (int): The number of class.
+ primitive_mode (str): The mode of primitive module,
+ available mode ['z', 'xy', 'line'].
+ bbox_coder (:obj:`BaseBBoxCoder`): Bbox coder for encoding and
+ decoding boxes.
+ train_cfg (dict): Config for training.
+ test_cfg (dict): Config for testing.
+ vote_module_cfg (dict): Config of VoteModule for point-wise votes.
+ vote_aggregation_cfg (dict): Config of vote aggregation layer.
+ feat_channels (tuple[int]): Convolution channels of
+ prediction layer.
+ upper_thresh (float): Threshold for line matching.
+ surface_thresh (float): Threshold for surface matching.
+ conv_cfg (dict): Config of convolution in prediction layer.
+ norm_cfg (dict): Config of BN in prediction layer.
+ objectness_loss (dict): Config of objectness loss.
+ center_loss (dict): Config of center loss.
+ semantic_loss (dict): Config of point-wise semantic segmentation loss.
+ """
+
+ def __init__(self,
+ num_dims,
+ num_classes,
+ primitive_mode,
+ train_cfg=None,
+ test_cfg=None,
+ vote_module_cfg=None,
+ vote_aggregation_cfg=None,
+ feat_channels=(128, 128),
+ upper_thresh=100.0,
+ surface_thresh=0.5,
+ conv_cfg=dict(type='Conv1d'),
+ norm_cfg=dict(type='BN1d'),
+ objectness_loss=None,
+ center_loss=None,
+ semantic_reg_loss=None,
+ semantic_cls_loss=None,
+ init_cfg=None):
+ super(PrimitiveHead, self).__init__(init_cfg=init_cfg)
+ assert primitive_mode in ['z', 'xy', 'line']
+ # The dimension of primitive semantic information.
+ self.num_dims = num_dims
+ self.num_classes = num_classes
+ self.primitive_mode = primitive_mode
+ self.train_cfg = train_cfg
+ self.test_cfg = test_cfg
+ self.gt_per_seed = vote_module_cfg['gt_per_seed']
+ self.num_proposal = vote_aggregation_cfg['num_point']
+ self.upper_thresh = upper_thresh
+ self.surface_thresh = surface_thresh
+
+ self.objectness_loss = build_loss(objectness_loss)
+ self.center_loss = build_loss(center_loss)
+ self.semantic_reg_loss = build_loss(semantic_reg_loss)
+ self.semantic_cls_loss = build_loss(semantic_cls_loss)
+
+ assert vote_aggregation_cfg['mlp_channels'][0] == vote_module_cfg[
+ 'in_channels']
+
+ # Primitive existence flag prediction
+ self.flag_conv = ConvModule(
+ vote_module_cfg['conv_channels'][-1],
+ vote_module_cfg['conv_channels'][-1] // 2,
+ 1,
+ padding=0,
+ conv_cfg=conv_cfg,
+ norm_cfg=norm_cfg,
+ bias=True,
+ inplace=True)
+ self.flag_pred = torch.nn.Conv1d(
+ vote_module_cfg['conv_channels'][-1] // 2, 2, 1)
+
+ self.vote_module = VoteModule(**vote_module_cfg)
+ self.vote_aggregation = build_sa_module(vote_aggregation_cfg)
+
+ prev_channel = vote_aggregation_cfg['mlp_channels'][-1]
+ conv_pred_list = list()
+ for k in range(len(feat_channels)):
+ conv_pred_list.append(
+ ConvModule(
+ prev_channel,
+ feat_channels[k],
+ 1,
+ padding=0,
+ conv_cfg=conv_cfg,
+ norm_cfg=norm_cfg,
+ bias=True,
+ inplace=True))
+ prev_channel = feat_channels[k]
+ self.conv_pred = nn.Sequential(*conv_pred_list)
+
+ conv_out_channel = 3 + num_dims + num_classes
+ self.conv_pred.add_module('conv_out',
+ nn.Conv1d(prev_channel, conv_out_channel, 1))
+
+ def forward(self, feats_dict, sample_mod):
+ """Forward pass.
+
+ Args:
+ feats_dict (dict): Feature dict from backbone.
+ sample_mod (str): Sample mode for vote aggregation layer.
+ valid modes are "vote", "seed" and "random".
+
+ Returns:
+ dict: Predictions of primitive head.
+ """
+ assert sample_mod in ['vote', 'seed', 'random']
+
+ seed_points = feats_dict['fp_xyz_net0'][-1]
+ seed_features = feats_dict['hd_feature']
+ results = {}
+
+ primitive_flag = self.flag_conv(seed_features)
+ primitive_flag = self.flag_pred(primitive_flag)
+
+ results['pred_flag_' + self.primitive_mode] = primitive_flag
+
+ # 1. generate vote_points from seed_points
+ vote_points, vote_features, _ = self.vote_module(
+ seed_points, seed_features)
+ results['vote_' + self.primitive_mode] = vote_points
+ results['vote_features_' + self.primitive_mode] = vote_features
+
+ # 2. aggregate vote_points
+ if sample_mod == 'vote':
+ # use fps in vote_aggregation
+ sample_indices = None
+ elif sample_mod == 'seed':
+ # FPS on seed and choose the votes corresponding to the seeds
+ sample_indices = furthest_point_sample(seed_points,
+ self.num_proposal)
+ elif sample_mod == 'random':
+ # Random sampling from the votes
+ batch_size, num_seed = seed_points.shape[:2]
+ sample_indices = torch.randint(
+ 0,
+ num_seed, (batch_size, self.num_proposal),
+ dtype=torch.int32,
+ device=seed_points.device)
+ else:
+ raise NotImplementedError('Unsupported sample mod!')
+
+ vote_aggregation_ret = self.vote_aggregation(vote_points,
+ vote_features,
+ sample_indices)
+ aggregated_points, features, aggregated_indices = vote_aggregation_ret
+ results['aggregated_points_' + self.primitive_mode] = aggregated_points
+ results['aggregated_features_' + self.primitive_mode] = features
+ results['aggregated_indices_' +
+ self.primitive_mode] = aggregated_indices
+
+ # 3. predict primitive offsets and semantic information
+ predictions = self.conv_pred(features)
+
+ # 4. decode predictions
+ decode_ret = self.primitive_decode_scores(predictions,
+ aggregated_points)
+ results.update(decode_ret)
+
+ center, pred_ind = self.get_primitive_center(
+ primitive_flag, decode_ret['center_' + self.primitive_mode])
+
+ results['pred_' + self.primitive_mode + '_ind'] = pred_ind
+ results['pred_' + self.primitive_mode + '_center'] = center
+ return results
+
+ def loss(self,
+ bbox_preds,
+ points,
+ gt_bboxes_3d,
+ gt_labels_3d,
+ pts_semantic_mask=None,
+ pts_instance_mask=None,
+ img_metas=None,
+ gt_bboxes_ignore=None):
+ """Compute loss.
+
+ Args:
+ bbox_preds (dict): Predictions from forward of primitive head.
+ points (list[torch.Tensor]): Input points.
+ gt_bboxes_3d (list[:obj:`BaseInstance3DBoxes`]): Ground truth
+ bboxes of each sample.
+ gt_labels_3d (list[torch.Tensor]): Labels of each sample.
+ pts_semantic_mask (list[torch.Tensor]): Point-wise
+ semantic mask.
+ pts_instance_mask (list[torch.Tensor]): Point-wise
+ instance mask.
+ img_metas (list[dict]): Contain pcd and img's meta info.
+ gt_bboxes_ignore (list[torch.Tensor]): Specify
+ which bounding.
+
+ Returns:
+ dict: Losses of Primitive Head.
+ """
+ targets = self.get_targets(points, gt_bboxes_3d, gt_labels_3d,
+ pts_semantic_mask, pts_instance_mask,
+ bbox_preds)
+
+ (point_mask, point_offset, gt_primitive_center, gt_primitive_semantic,
+ gt_sem_cls_label, gt_primitive_mask) = targets
+
+ losses = {}
+ # Compute the loss of primitive existence flag
+ pred_flag = bbox_preds['pred_flag_' + self.primitive_mode]
+ flag_loss = self.objectness_loss(pred_flag, gt_primitive_mask.long())
+ losses['flag_loss_' + self.primitive_mode] = flag_loss
+
+ # calculate vote loss
+ vote_loss = self.vote_module.get_loss(
+ bbox_preds['seed_points'],
+ bbox_preds['vote_' + self.primitive_mode],
+ bbox_preds['seed_indices'], point_mask, point_offset)
+ losses['vote_loss_' + self.primitive_mode] = vote_loss
+
+ num_proposal = bbox_preds['aggregated_points_' +
+ self.primitive_mode].shape[1]
+ primitive_center = bbox_preds['center_' + self.primitive_mode]
+ if self.primitive_mode != 'line':
+ primitive_semantic = bbox_preds['size_residuals_' +
+ self.primitive_mode].contiguous()
+ else:
+ primitive_semantic = None
+ semancitc_scores = bbox_preds['sem_cls_scores_' +
+ self.primitive_mode].transpose(2, 1)
+
+ gt_primitive_mask = gt_primitive_mask / \
+ (gt_primitive_mask.sum() + 1e-6)
+ center_loss, size_loss, sem_cls_loss = self.compute_primitive_loss(
+ primitive_center, primitive_semantic, semancitc_scores,
+ num_proposal, gt_primitive_center, gt_primitive_semantic,
+ gt_sem_cls_label, gt_primitive_mask)
+ losses['center_loss_' + self.primitive_mode] = center_loss
+ losses['size_loss_' + self.primitive_mode] = size_loss
+ losses['sem_loss_' + self.primitive_mode] = sem_cls_loss
+
+ return losses
+
+ def get_targets(self,
+ points,
+ gt_bboxes_3d,
+ gt_labels_3d,
+ pts_semantic_mask=None,
+ pts_instance_mask=None,
+ bbox_preds=None):
+ """Generate targets of primitive head.
+
+ Args:
+ points (list[torch.Tensor]): Points of each batch.
+ gt_bboxes_3d (list[:obj:`BaseInstance3DBoxes`]): Ground truth
+ bboxes of each batch.
+ gt_labels_3d (list[torch.Tensor]): Labels of each batch.
+ pts_semantic_mask (list[torch.Tensor]): Point-wise semantic
+ label of each batch.
+ pts_instance_mask (list[torch.Tensor]): Point-wise instance
+ label of each batch.
+ bbox_preds (dict): Predictions from forward of primitive head.
+
+ Returns:
+ tuple[torch.Tensor]: Targets of primitive head.
+ """
+ for index in range(len(gt_labels_3d)):
+ if len(gt_labels_3d[index]) == 0:
+ fake_box = gt_bboxes_3d[index].tensor.new_zeros(
+ 1, gt_bboxes_3d[index].tensor.shape[-1])
+ gt_bboxes_3d[index] = gt_bboxes_3d[index].new_box(fake_box)
+ gt_labels_3d[index] = gt_labels_3d[index].new_zeros(1)
+
+ if pts_semantic_mask is None:
+ pts_semantic_mask = [None for i in range(len(gt_labels_3d))]
+ pts_instance_mask = [None for i in range(len(gt_labels_3d))]
+
+ (point_mask, point_sem,
+ point_offset) = multi_apply(self.get_targets_single, points,
+ gt_bboxes_3d, gt_labels_3d,
+ pts_semantic_mask, pts_instance_mask)
+
+ point_mask = torch.stack(point_mask)
+ point_sem = torch.stack(point_sem)
+ point_offset = torch.stack(point_offset)
+
+ batch_size = point_mask.shape[0]
+ num_proposal = bbox_preds['aggregated_points_' +
+ self.primitive_mode].shape[1]
+ num_seed = bbox_preds['seed_points'].shape[1]
+ seed_inds = bbox_preds['seed_indices'].long()
+ seed_inds_expand = seed_inds.view(batch_size, num_seed,
+ 1).repeat(1, 1, 3)
+ seed_gt_votes = torch.gather(point_offset, 1, seed_inds_expand)
+ seed_gt_votes += bbox_preds['seed_points']
+ gt_primitive_center = seed_gt_votes.view(batch_size * num_proposal, 1,
+ 3)
+
+ seed_inds_expand_sem = seed_inds.view(batch_size, num_seed, 1).repeat(
+ 1, 1, 4 + self.num_dims)
+ seed_gt_sem = torch.gather(point_sem, 1, seed_inds_expand_sem)
+ gt_primitive_semantic = seed_gt_sem[:, :, 3:3 + self.num_dims].view(
+ batch_size * num_proposal, 1, self.num_dims).contiguous()
+
+ gt_sem_cls_label = seed_gt_sem[:, :, -1].long()
+
+ gt_votes_mask = torch.gather(point_mask, 1, seed_inds)
+
+ return (point_mask, point_offset, gt_primitive_center,
+ gt_primitive_semantic, gt_sem_cls_label, gt_votes_mask)
+
+ def get_targets_single(self,
+ points,
+ gt_bboxes_3d,
+ gt_labels_3d,
+ pts_semantic_mask=None,
+ pts_instance_mask=None):
+ """Generate targets of primitive head for single batch.
+
+ Args:
+ points (torch.Tensor): Points of each batch.
+ gt_bboxes_3d (:obj:`BaseInstance3DBoxes`): Ground truth
+ boxes of each batch.
+ gt_labels_3d (torch.Tensor): Labels of each batch.
+ pts_semantic_mask (torch.Tensor): Point-wise semantic
+ label of each batch.
+ pts_instance_mask (torch.Tensor): Point-wise instance
+ label of each batch.
+
+ Returns:
+ tuple[torch.Tensor]: Targets of primitive head.
+ """
+ gt_bboxes_3d = gt_bboxes_3d.to(points.device)
+ num_points = points.shape[0]
+
+ point_mask = points.new_zeros(num_points)
+ # Offset to the primitive center
+ point_offset = points.new_zeros([num_points, 3])
+ # Semantic information of primitive center
+ point_sem = points.new_zeros([num_points, 3 + self.num_dims + 1])
+
+ # Generate pts_semantic_mask and pts_instance_mask when they are None
+ if pts_semantic_mask is None or pts_instance_mask is None:
+ points2box_mask = gt_bboxes_3d.points_in_boxes_all(points)
+ assignment = points2box_mask.argmax(1)
+ background_mask = points2box_mask.max(1)[0] == 0
+
+ if pts_semantic_mask is None:
+ pts_semantic_mask = gt_labels_3d[assignment]
+ pts_semantic_mask[background_mask] = self.num_classes
+
+ if pts_instance_mask is None:
+ pts_instance_mask = assignment
+ pts_instance_mask[background_mask] = gt_labels_3d.shape[0]
+
+ instance_flag = torch.nonzero(
+ pts_semantic_mask != self.num_classes, as_tuple=False).squeeze(1)
+ instance_labels = pts_instance_mask[instance_flag].unique()
+
+ with_yaw = gt_bboxes_3d.with_yaw
+ for i, i_instance in enumerate(instance_labels):
+ indices = instance_flag[pts_instance_mask[instance_flag] ==
+ i_instance]
+ coords = points[indices, :3]
+ cur_cls_label = pts_semantic_mask[indices][0]
+
+ # Bbox Corners
+ cur_corners = gt_bboxes_3d.corners[i]
+
+ plane_lower_temp = points.new_tensor(
+ [0, 0, 1, -cur_corners[7, -1]])
+ upper_points = cur_corners[[1, 2, 5, 6]]
+ refined_distance = (upper_points * plane_lower_temp[:3]).sum(dim=1)
+
+ if self.check_horizon(upper_points) and \
+ plane_lower_temp[0] + plane_lower_temp[1] < \
+ self.train_cfg['lower_thresh']:
+ plane_lower = points.new_tensor(
+ [0, 0, 1, plane_lower_temp[-1]])
+ plane_upper = points.new_tensor(
+ [0, 0, 1, -torch.mean(refined_distance)])
+ else:
+ raise NotImplementedError('Only horizontal plane is support!')
+
+ if self.check_dist(plane_upper, upper_points) is False:
+ raise NotImplementedError(
+ 'Mean distance to plane should be lower than thresh!')
+
+ # Get the boundary points here
+ point2plane_dist, selected = self.match_point2plane(
+ plane_lower, coords)
+
+ # Get bottom four lines
+ if self.primitive_mode == 'line':
+ point2line_matching = self.match_point2line(
+ coords[selected], cur_corners, with_yaw, mode='bottom')
+
+ point_mask, point_offset, point_sem = \
+ self._assign_primitive_line_targets(point_mask,
+ point_offset,
+ point_sem,
+ coords[selected],
+ indices[selected],
+ cur_cls_label,
+ point2line_matching,
+ cur_corners,
+ [1, 1, 0, 0],
+ with_yaw,
+ mode='bottom')
+
+ # Set the surface labels here
+ if self.primitive_mode == 'z' and \
+ selected.sum() > self.train_cfg['num_point'] and \
+ point2plane_dist[selected].var() < \
+ self.train_cfg['var_thresh']:
+
+ point_mask, point_offset, point_sem = \
+ self._assign_primitive_surface_targets(point_mask,
+ point_offset,
+ point_sem,
+ coords[selected],
+ indices[selected],
+ cur_cls_label,
+ cur_corners,
+ with_yaw,
+ mode='bottom')
+
+ # Get the boundary points here
+ point2plane_dist, selected = self.match_point2plane(
+ plane_upper, coords)
+
+ # Get top four lines
+ if self.primitive_mode == 'line':
+ point2line_matching = self.match_point2line(
+ coords[selected], cur_corners, with_yaw, mode='top')
+
+ point_mask, point_offset, point_sem = \
+ self._assign_primitive_line_targets(point_mask,
+ point_offset,
+ point_sem,
+ coords[selected],
+ indices[selected],
+ cur_cls_label,
+ point2line_matching,
+ cur_corners,
+ [1, 1, 0, 0],
+ with_yaw,
+ mode='top')
+
+ if self.primitive_mode == 'z' and \
+ selected.sum() > self.train_cfg['num_point'] and \
+ point2plane_dist[selected].var() < \
+ self.train_cfg['var_thresh']:
+
+ point_mask, point_offset, point_sem = \
+ self._assign_primitive_surface_targets(point_mask,
+ point_offset,
+ point_sem,
+ coords[selected],
+ indices[selected],
+ cur_cls_label,
+ cur_corners,
+ with_yaw,
+ mode='top')
+
+ # Get left two lines
+ plane_left_temp = self._get_plane_fomulation(
+ cur_corners[2] - cur_corners[3],
+ cur_corners[3] - cur_corners[0], cur_corners[0])
+
+ right_points = cur_corners[[4, 5, 7, 6]]
+ plane_left_temp /= torch.norm(plane_left_temp[:3])
+ refined_distance = (right_points * plane_left_temp[:3]).sum(dim=1)
+
+ if plane_left_temp[2] < self.train_cfg['lower_thresh']:
+ plane_left = plane_left_temp
+ plane_right = points.new_tensor([
+ plane_left_temp[0], plane_left_temp[1], plane_left_temp[2],
+ -refined_distance.mean()
+ ])
+ else:
+ raise NotImplementedError(
+ 'Normal vector of the plane should be horizontal!')
+
+ # Get the boundary points here
+ point2plane_dist, selected = self.match_point2plane(
+ plane_left, coords)
+
+ # Get left four lines
+ if self.primitive_mode == 'line':
+ point2line_matching = self.match_point2line(
+ coords[selected], cur_corners, with_yaw, mode='left')
+ point_mask, point_offset, point_sem = \
+ self._assign_primitive_line_targets(
+ point_mask, point_offset, point_sem,
+ coords[selected], indices[selected], cur_cls_label,
+ point2line_matching[2:], cur_corners, [2, 2],
+ with_yaw, mode='left')
+
+ if self.primitive_mode == 'xy' and \
+ selected.sum() > self.train_cfg['num_point'] and \
+ point2plane_dist[selected].var() < \
+ self.train_cfg['var_thresh']:
+
+ point_mask, point_offset, point_sem = \
+ self._assign_primitive_surface_targets(
+ point_mask, point_offset, point_sem,
+ coords[selected], indices[selected], cur_cls_label,
+ cur_corners, with_yaw, mode='left')
+
+ # Get the boundary points here
+ point2plane_dist, selected = self.match_point2plane(
+ plane_right, coords)
+
+ # Get right four lines
+ if self.primitive_mode == 'line':
+ point2line_matching = self.match_point2line(
+ coords[selected], cur_corners, with_yaw, mode='right')
+
+ point_mask, point_offset, point_sem = \
+ self._assign_primitive_line_targets(
+ point_mask, point_offset, point_sem,
+ coords[selected], indices[selected], cur_cls_label,
+ point2line_matching[2:], cur_corners, [2, 2],
+ with_yaw, mode='right')
+
+ if self.primitive_mode == 'xy' and \
+ selected.sum() > self.train_cfg['num_point'] and \
+ point2plane_dist[selected].var() < \
+ self.train_cfg['var_thresh']:
+
+ point_mask, point_offset, point_sem = \
+ self._assign_primitive_surface_targets(
+ point_mask, point_offset, point_sem,
+ coords[selected], indices[selected], cur_cls_label,
+ cur_corners, with_yaw, mode='right')
+
+ plane_front_temp = self._get_plane_fomulation(
+ cur_corners[0] - cur_corners[4],
+ cur_corners[4] - cur_corners[5], cur_corners[5])
+
+ back_points = cur_corners[[3, 2, 7, 6]]
+ plane_front_temp /= torch.norm(plane_front_temp[:3])
+ refined_distance = (back_points * plane_front_temp[:3]).sum(dim=1)
+
+ if plane_front_temp[2] < self.train_cfg['lower_thresh']:
+ plane_front = plane_front_temp
+ plane_back = points.new_tensor([
+ plane_front_temp[0], plane_front_temp[1],
+ plane_front_temp[2], -torch.mean(refined_distance)
+ ])
+ else:
+ raise NotImplementedError(
+ 'Normal vector of the plane should be horizontal!')
+
+ # Get the boundary points here
+ point2plane_dist, selected = self.match_point2plane(
+ plane_front, coords)
+
+ if self.primitive_mode == 'xy' and \
+ selected.sum() > self.train_cfg['num_point'] and \
+ (point2plane_dist[selected]).var() < \
+ self.train_cfg['var_thresh']:
+
+ point_mask, point_offset, point_sem = \
+ self._assign_primitive_surface_targets(
+ point_mask, point_offset, point_sem,
+ coords[selected], indices[selected], cur_cls_label,
+ cur_corners, with_yaw, mode='front')
+
+ # Get the boundary points here
+ point2plane_dist, selected = self.match_point2plane(
+ plane_back, coords)
+
+ if self.primitive_mode == 'xy' and \
+ selected.sum() > self.train_cfg['num_point'] and \
+ point2plane_dist[selected].var() < \
+ self.train_cfg['var_thresh']:
+
+ point_mask, point_offset, point_sem = \
+ self._assign_primitive_surface_targets(
+ point_mask, point_offset, point_sem,
+ coords[selected], indices[selected], cur_cls_label,
+ cur_corners, with_yaw, mode='back')
+
+ return (point_mask, point_sem, point_offset)
+
+ def primitive_decode_scores(self, predictions, aggregated_points):
+ """Decode predicted parts to primitive head.
+
+ Args:
+ predictions (torch.Tensor): primitive pridictions of each batch.
+ aggregated_points (torch.Tensor): The aggregated points
+ of vote stage.
+
+ Returns:
+ Dict: Predictions of primitive head, including center,
+ semantic size and semantic scores.
+ """
+
+ ret_dict = {}
+ pred_transposed = predictions.transpose(2, 1)
+
+ center = aggregated_points + pred_transposed[:, :, 0:3]
+ ret_dict['center_' + self.primitive_mode] = center
+
+ if self.primitive_mode in ['z', 'xy']:
+ ret_dict['size_residuals_' + self.primitive_mode] = \
+ pred_transposed[:, :, 3:3 + self.num_dims]
+
+ ret_dict['sem_cls_scores_' + self.primitive_mode] = \
+ pred_transposed[:, :, 3 + self.num_dims:]
+
+ return ret_dict
+
+ def check_horizon(self, points):
+ """Check whether is a horizontal plane.
+
+ Args:
+ points (torch.Tensor): Points of input.
+
+ Returns:
+ Bool: Flag of result.
+ """
+ return (points[0][-1] == points[1][-1]) and \
+ (points[1][-1] == points[2][-1]) and \
+ (points[2][-1] == points[3][-1])
+
+ def check_dist(self, plane_equ, points):
+ """Whether the mean of points to plane distance is lower than thresh.
+
+ Args:
+ plane_equ (torch.Tensor): Plane to be checked.
+ points (torch.Tensor): Points to be checked.
+
+ Returns:
+ Tuple: Flag of result.
+ """
+ return (points[:, 2] +
+ plane_equ[-1]).sum() / 4.0 < self.train_cfg['lower_thresh']
+
+ def point2line_dist(self, points, pts_a, pts_b):
+ """Calculate the distance from point to line.
+
+ Args:
+ points (torch.Tensor): Points of input.
+ pts_a (torch.Tensor): Point on the specific line.
+ pts_b (torch.Tensor): Point on the specific line.
+
+ Returns:
+ torch.Tensor: Distance between each point to line.
+ """
+ line_a2b = pts_b - pts_a
+ line_a2pts = points - pts_a
+ length = (line_a2pts * line_a2b.view(1, 3)).sum(1) / \
+ line_a2b.norm()
+ dist = (line_a2pts.norm(dim=1)**2 - length**2).sqrt()
+
+ return dist
+
+ def match_point2line(self, points, corners, with_yaw, mode='bottom'):
+ """Match points to corresponding line.
+
+ Args:
+ points (torch.Tensor): Points of input.
+ corners (torch.Tensor): Eight corners of a bounding box.
+ with_yaw (Bool): Whether the boundind box is with rotation.
+ mode (str, optional): Specify which line should be matched,
+ available mode are ('bottom', 'top', 'left', 'right').
+ Defaults to 'bottom'.
+
+ Returns:
+ Tuple: Flag of matching correspondence.
+ """
+ if with_yaw:
+ corners_pair = {
+ 'bottom': [[0, 3], [4, 7], [0, 4], [3, 7]],
+ 'top': [[1, 2], [5, 6], [1, 5], [2, 6]],
+ 'left': [[0, 1], [3, 2], [0, 1], [3, 2]],
+ 'right': [[4, 5], [7, 6], [4, 5], [7, 6]]
+ }
+ selected_list = []
+ for pair_index in corners_pair[mode]:
+ selected = self.point2line_dist(
+ points, corners[pair_index[0]], corners[pair_index[1]]) \
+ < self.train_cfg['line_thresh']
+ selected_list.append(selected)
+ else:
+ xmin, ymin, _ = corners.min(0)[0]
+ xmax, ymax, _ = corners.max(0)[0]
+ sel1 = torch.abs(points[:, 0] -
+ xmin) < self.train_cfg['line_thresh']
+ sel2 = torch.abs(points[:, 0] -
+ xmax) < self.train_cfg['line_thresh']
+ sel3 = torch.abs(points[:, 1] -
+ ymin) < self.train_cfg['line_thresh']
+ sel4 = torch.abs(points[:, 1] -
+ ymax) < self.train_cfg['line_thresh']
+ selected_list = [sel1, sel2, sel3, sel4]
+ return selected_list
+
+ def match_point2plane(self, plane, points):
+ """Match points to plane.
+
+ Args:
+ plane (torch.Tensor): Equation of the plane.
+ points (torch.Tensor): Points of input.
+
+ Returns:
+ Tuple: Distance of each point to the plane and
+ flag of matching correspondence.
+ """
+ point2plane_dist = torch.abs((points * plane[:3]).sum(dim=1) +
+ plane[-1])
+ min_dist = point2plane_dist.min()
+ selected = torch.abs(point2plane_dist -
+ min_dist) < self.train_cfg['dist_thresh']
+ return point2plane_dist, selected
+
+ def compute_primitive_loss(self, primitive_center, primitive_semantic,
+ semantic_scores, num_proposal,
+ gt_primitive_center, gt_primitive_semantic,
+ gt_sem_cls_label, gt_primitive_mask):
+ """Compute loss of primitive module.
+
+ Args:
+ primitive_center (torch.Tensor): Pridictions of primitive center.
+ primitive_semantic (torch.Tensor): Pridictions of primitive
+ semantic.
+ semantic_scores (torch.Tensor): Pridictions of primitive
+ semantic scores.
+ num_proposal (int): The number of primitive proposal.
+ gt_primitive_center (torch.Tensor): Ground truth of
+ primitive center.
+ gt_votes_sem (torch.Tensor): Ground truth of primitive semantic.
+ gt_sem_cls_label (torch.Tensor): Ground truth of primitive
+ semantic class.
+ gt_primitive_mask (torch.Tensor): Ground truth of primitive mask.
+
+ Returns:
+ Tuple: Loss of primitive module.
+ """
+ batch_size = primitive_center.shape[0]
+ vote_xyz_reshape = primitive_center.view(batch_size * num_proposal, -1,
+ 3)
+
+ center_loss = self.center_loss(
+ vote_xyz_reshape,
+ gt_primitive_center,
+ dst_weight=gt_primitive_mask.view(batch_size * num_proposal, 1))[1]
+
+ if self.primitive_mode != 'line':
+ size_xyz_reshape = primitive_semantic.view(
+ batch_size * num_proposal, -1, self.num_dims).contiguous()
+ size_loss = self.semantic_reg_loss(
+ size_xyz_reshape,
+ gt_primitive_semantic,
+ dst_weight=gt_primitive_mask.view(batch_size * num_proposal,
+ 1))[1]
+ else:
+ size_loss = center_loss.new_tensor(0.0)
+
+ # Semantic cls loss
+ sem_cls_loss = self.semantic_cls_loss(
+ semantic_scores, gt_sem_cls_label, weight=gt_primitive_mask)
+
+ return center_loss, size_loss, sem_cls_loss
+
+ def get_primitive_center(self, pred_flag, center):
+ """Generate primitive center from predictions.
+
+ Args:
+ pred_flag (torch.Tensor): Scores of primitive center.
+ center (torch.Tensor): Pridictions of primitive center.
+
+ Returns:
+ Tuple: Primitive center and the prediction indices.
+ """
+ ind_normal = F.softmax(pred_flag, dim=1)
+ pred_indices = (ind_normal[:, 1, :] >
+ self.surface_thresh).detach().float()
+ selected = (ind_normal[:, 1, :] <=
+ self.surface_thresh).detach().float()
+ offset = torch.ones_like(center) * self.upper_thresh
+ center = center + offset * selected.unsqueeze(-1)
+ return center, pred_indices
+
+ def _assign_primitive_line_targets(self,
+ point_mask,
+ point_offset,
+ point_sem,
+ coords,
+ indices,
+ cls_label,
+ point2line_matching,
+ corners,
+ center_axises,
+ with_yaw,
+ mode='bottom'):
+ """Generate targets of line primitive.
+
+ Args:
+ point_mask (torch.Tensor): Tensor to store the ground
+ truth of mask.
+ point_offset (torch.Tensor): Tensor to store the ground
+ truth of offset.
+ point_sem (torch.Tensor): Tensor to store the ground
+ truth of semantic.
+ coords (torch.Tensor): The selected points.
+ indices (torch.Tensor): Indices of the selected points.
+ cls_label (int): Class label of the ground truth bounding box.
+ point2line_matching (torch.Tensor): Flag indicate that
+ matching line of each point.
+ corners (torch.Tensor): Corners of the ground truth bounding box.
+ center_axises (list[int]): Indicate in which axis the line center
+ should be refined.
+ with_yaw (Bool): Whether the boundind box is with rotation.
+ mode (str, optional): Specify which line should be matched,
+ available mode are ('bottom', 'top', 'left', 'right').
+ Defaults to 'bottom'.
+
+ Returns:
+ Tuple: Targets of the line primitive.
+ """
+ corners_pair = {
+ 'bottom': [[0, 3], [4, 7], [0, 4], [3, 7]],
+ 'top': [[1, 2], [5, 6], [1, 5], [2, 6]],
+ 'left': [[0, 1], [3, 2]],
+ 'right': [[4, 5], [7, 6]]
+ }
+ corners_pair = corners_pair[mode]
+ assert len(corners_pair) == len(point2line_matching) == len(
+ center_axises)
+ for line_select, center_axis, pair_index in zip(
+ point2line_matching, center_axises, corners_pair):
+ if line_select.sum() > self.train_cfg['num_point_line']:
+ point_mask[indices[line_select]] = 1.0
+
+ if with_yaw:
+ line_center = (corners[pair_index[0]] +
+ corners[pair_index[1]]) / 2
+ else:
+ line_center = coords[line_select].mean(dim=0)
+ line_center[center_axis] = corners[:, center_axis].mean()
+
+ point_offset[indices[line_select]] = \
+ line_center - coords[line_select]
+ point_sem[indices[line_select]] = \
+ point_sem.new_tensor([line_center[0], line_center[1],
+ line_center[2], cls_label])
+ return point_mask, point_offset, point_sem
+
+ def _assign_primitive_surface_targets(self,
+ point_mask,
+ point_offset,
+ point_sem,
+ coords,
+ indices,
+ cls_label,
+ corners,
+ with_yaw,
+ mode='bottom'):
+ """Generate targets for primitive z and primitive xy.
+
+ Args:
+ point_mask (torch.Tensor): Tensor to store the ground
+ truth of mask.
+ point_offset (torch.Tensor): Tensor to store the ground
+ truth of offset.
+ point_sem (torch.Tensor): Tensor to store the ground
+ truth of semantic.
+ coords (torch.Tensor): The selected points.
+ indices (torch.Tensor): Indices of the selected points.
+ cls_label (int): Class label of the ground truth bounding box.
+ corners (torch.Tensor): Corners of the ground truth bounding box.
+ with_yaw (Bool): Whether the boundind box is with rotation.
+ mode (str, optional): Specify which line should be matched,
+ available mode are ('bottom', 'top', 'left', 'right',
+ 'front', 'back').
+ Defaults to 'bottom'.
+
+ Returns:
+ Tuple: Targets of the center primitive.
+ """
+ point_mask[indices] = 1.0
+ corners_pair = {
+ 'bottom': [0, 7],
+ 'top': [1, 6],
+ 'left': [0, 1],
+ 'right': [4, 5],
+ 'front': [0, 1],
+ 'back': [3, 2]
+ }
+ pair_index = corners_pair[mode]
+ if self.primitive_mode == 'z':
+ if with_yaw:
+ center = (corners[pair_index[0]] +
+ corners[pair_index[1]]) / 2.0
+ center[2] = coords[:, 2].mean()
+ point_sem[indices] = point_sem.new_tensor([
+ center[0], center[1],
+ center[2], (corners[4] - corners[0]).norm(),
+ (corners[3] - corners[0]).norm(), cls_label
+ ])
+ else:
+ center = point_mask.new_tensor([
+ corners[:, 0].mean(), corners[:, 1].mean(),
+ coords[:, 2].mean()
+ ])
+ point_sem[indices] = point_sem.new_tensor([
+ center[0], center[1], center[2],
+ corners[:, 0].max() - corners[:, 0].min(),
+ corners[:, 1].max() - corners[:, 1].min(), cls_label
+ ])
+ elif self.primitive_mode == 'xy':
+ if with_yaw:
+ center = coords.mean(0)
+ center[2] = (corners[pair_index[0], 2] +
+ corners[pair_index[1], 2]) / 2.0
+ point_sem[indices] = point_sem.new_tensor([
+ center[0], center[1], center[2],
+ corners[pair_index[1], 2] - corners[pair_index[0], 2],
+ cls_label
+ ])
+ else:
+ center = point_mask.new_tensor([
+ coords[:, 0].mean(), coords[:, 1].mean(),
+ corners[:, 2].mean()
+ ])
+ point_sem[indices] = point_sem.new_tensor([
+ center[0], center[1], center[2],
+ corners[:, 2].max() - corners[:, 2].min(), cls_label
+ ])
+ point_offset[indices] = center - coords
+ return point_mask, point_offset, point_sem
+
+ def _get_plane_fomulation(self, vector1, vector2, point):
+ """Compute the equation of the plane.
+
+ Args:
+ vector1 (torch.Tensor): Parallel vector of the plane.
+ vector2 (torch.Tensor): Parallel vector of the plane.
+ point (torch.Tensor): Point on the plane.
+
+ Returns:
+ torch.Tensor: Equation of the plane.
+ """
+ surface_norm = torch.cross(vector1, vector2)
+ surface_dis = -torch.dot(surface_norm, point)
+ plane = point.new_tensor(
+ [surface_norm[0], surface_norm[1], surface_norm[2], surface_dis])
+ return plane
diff --git a/mmdet3d/models/roi_heads/part_aggregation_roi_head.py b/mmdet3d/models/roi_heads/part_aggregation_roi_head.py
new file mode 100644
index 0000000..a3e49ea
--- /dev/null
+++ b/mmdet3d/models/roi_heads/part_aggregation_roi_head.py
@@ -0,0 +1,325 @@
+# Copyright (c) OpenMMLab. All rights reserved.
+import warnings
+
+from torch.nn import functional as F
+
+from mmdet3d.core import AssignResult
+from mmdet3d.core.bbox import bbox3d2result, bbox3d2roi
+from mmdet.core import build_assigner, build_sampler
+from ..builder import HEADS, build_head, build_roi_extractor
+from .base_3droi_head import Base3DRoIHead
+
+
+@HEADS.register_module()
+class PartAggregationROIHead(Base3DRoIHead):
+ """Part aggregation roi head for PartA2.
+
+ Args:
+ semantic_head (ConfigDict): Config of semantic head.
+ num_classes (int): The number of classes.
+ seg_roi_extractor (ConfigDict): Config of seg_roi_extractor.
+ part_roi_extractor (ConfigDict): Config of part_roi_extractor.
+ bbox_head (ConfigDict): Config of bbox_head.
+ train_cfg (ConfigDict): Training config.
+ test_cfg (ConfigDict): Testing config.
+ """
+
+ def __init__(self,
+ semantic_head,
+ num_classes=3,
+ seg_roi_extractor=None,
+ part_roi_extractor=None,
+ bbox_head=None,
+ train_cfg=None,
+ test_cfg=None,
+ pretrained=None,
+ init_cfg=None):
+ super(PartAggregationROIHead, self).__init__(
+ bbox_head=bbox_head,
+ train_cfg=train_cfg,
+ test_cfg=test_cfg,
+ init_cfg=init_cfg)
+ self.num_classes = num_classes
+ assert semantic_head is not None
+ self.semantic_head = build_head(semantic_head)
+
+ if seg_roi_extractor is not None:
+ self.seg_roi_extractor = build_roi_extractor(seg_roi_extractor)
+ if part_roi_extractor is not None:
+ self.part_roi_extractor = build_roi_extractor(part_roi_extractor)
+
+ self.init_assigner_sampler()
+
+ assert not (init_cfg and pretrained), \
+ 'init_cfg and pretrained cannot be setting at the same time'
+ if isinstance(pretrained, str):
+ warnings.warn('DeprecationWarning: pretrained is a deprecated, '
+ 'please use "init_cfg" instead')
+ self.init_cfg = dict(type='Pretrained', checkpoint=pretrained)
+
+ def init_mask_head(self):
+ """Initialize mask head, skip since ``PartAggregationROIHead`` does not
+ have one."""
+ pass
+
+ def init_bbox_head(self, bbox_head):
+ """Initialize box head."""
+ self.bbox_head = build_head(bbox_head)
+
+ def init_assigner_sampler(self):
+ """Initialize assigner and sampler."""
+ self.bbox_assigner = None
+ self.bbox_sampler = None
+ if self.train_cfg:
+ if isinstance(self.train_cfg.assigner, dict):
+ self.bbox_assigner = build_assigner(self.train_cfg.assigner)
+ elif isinstance(self.train_cfg.assigner, list):
+ self.bbox_assigner = [
+ build_assigner(res) for res in self.train_cfg.assigner
+ ]
+ self.bbox_sampler = build_sampler(self.train_cfg.sampler)
+
+ @property
+ def with_semantic(self):
+ """bool: whether the head has semantic branch"""
+ return hasattr(self,
+ 'semantic_head') and self.semantic_head is not None
+
+ def forward_train(self, feats_dict, voxels_dict, img_metas, proposal_list,
+ gt_bboxes_3d, gt_labels_3d):
+ """Training forward function of PartAggregationROIHead.
+
+ Args:
+ feats_dict (dict): Contains features from the first stage.
+ voxels_dict (dict): Contains information of voxels.
+ img_metas (list[dict]): Meta info of each image.
+ proposal_list (list[dict]): Proposal information from rpn.
+ The dictionary should contain the following keys:
+
+ - boxes_3d (:obj:`BaseInstance3DBoxes`): Proposal bboxes
+ - labels_3d (torch.Tensor): Labels of proposals
+ - cls_preds (torch.Tensor): Original scores of proposals
+ gt_bboxes_3d (list[:obj:`BaseInstance3DBoxes`]):
+ GT bboxes of each sample. The bboxes are encapsulated
+ by 3D box structures.
+ gt_labels_3d (list[LongTensor]): GT labels of each sample.
+
+ Returns:
+ dict: losses from each head.
+
+ - loss_semantic (torch.Tensor): loss of semantic head
+ - loss_bbox (torch.Tensor): loss of bboxes
+ """
+ losses = dict()
+ if self.with_semantic:
+ semantic_results = self._semantic_forward_train(
+ feats_dict['seg_features'], voxels_dict, gt_bboxes_3d,
+ gt_labels_3d)
+ losses.update(semantic_results['loss_semantic'])
+
+ sample_results = self._assign_and_sample(proposal_list, gt_bboxes_3d,
+ gt_labels_3d)
+ if self.with_bbox:
+ bbox_results = self._bbox_forward_train(
+ feats_dict['seg_features'], semantic_results['part_feats'],
+ voxels_dict, sample_results)
+ losses.update(bbox_results['loss_bbox'])
+
+ return losses
+
+ def simple_test(self, feats_dict, voxels_dict, img_metas, proposal_list,
+ **kwargs):
+ """Simple testing forward function of PartAggregationROIHead.
+
+ Note:
+ This function assumes that the batch size is 1
+
+ Args:
+ feats_dict (dict): Contains features from the first stage.
+ voxels_dict (dict): Contains information of voxels.
+ img_metas (list[dict]): Meta info of each image.
+ proposal_list (list[dict]): Proposal information from rpn.
+
+ Returns:
+ dict: Bbox results of one frame.
+ """
+ assert self.with_bbox, 'Bbox head must be implemented.'
+ assert self.with_semantic
+
+ semantic_results = self.semantic_head(feats_dict['seg_features'])
+
+ rois = bbox3d2roi([res['boxes_3d'].tensor for res in proposal_list])
+ labels_3d = [res['labels_3d'] for res in proposal_list]
+ cls_preds = [res['cls_preds'] for res in proposal_list]
+ bbox_results = self._bbox_forward(feats_dict['seg_features'],
+ semantic_results['part_feats'],
+ voxels_dict, rois)
+
+ bbox_list = self.bbox_head.get_bboxes(
+ rois,
+ bbox_results['cls_score'],
+ bbox_results['bbox_pred'],
+ labels_3d,
+ cls_preds,
+ img_metas,
+ cfg=self.test_cfg)
+
+ bbox_results = [
+ bbox3d2result(bboxes, scores, labels)
+ for bboxes, scores, labels in bbox_list
+ ]
+ return bbox_results
+
+ def _bbox_forward_train(self, seg_feats, part_feats, voxels_dict,
+ sampling_results):
+ """Forward training function of roi_extractor and bbox_head.
+
+ Args:
+ seg_feats (torch.Tensor): Point-wise semantic features.
+ part_feats (torch.Tensor): Point-wise part prediction features.
+ voxels_dict (dict): Contains information of voxels.
+ sampling_results (:obj:`SamplingResult`): Sampled results used
+ for training.
+
+ Returns:
+ dict: Forward results including losses and predictions.
+ """
+ rois = bbox3d2roi([res.bboxes for res in sampling_results])
+ bbox_results = self._bbox_forward(seg_feats, part_feats, voxels_dict,
+ rois)
+
+ bbox_targets = self.bbox_head.get_targets(sampling_results,
+ self.train_cfg)
+ loss_bbox = self.bbox_head.loss(bbox_results['cls_score'],
+ bbox_results['bbox_pred'], rois,
+ *bbox_targets)
+
+ bbox_results.update(loss_bbox=loss_bbox)
+ return bbox_results
+
+ def _bbox_forward(self, seg_feats, part_feats, voxels_dict, rois):
+ """Forward function of roi_extractor and bbox_head used in both
+ training and testing.
+
+ Args:
+ seg_feats (torch.Tensor): Point-wise semantic features.
+ part_feats (torch.Tensor): Point-wise part prediction features.
+ voxels_dict (dict): Contains information of voxels.
+ rois (Tensor): Roi boxes.
+
+ Returns:
+ dict: Contains predictions of bbox_head and
+ features of roi_extractor.
+ """
+ pooled_seg_feats = self.seg_roi_extractor(seg_feats,
+ voxels_dict['voxel_centers'],
+ voxels_dict['coors'][..., 0],
+ rois)
+ pooled_part_feats = self.part_roi_extractor(
+ part_feats, voxels_dict['voxel_centers'],
+ voxels_dict['coors'][..., 0], rois)
+ cls_score, bbox_pred = self.bbox_head(pooled_seg_feats,
+ pooled_part_feats)
+
+ bbox_results = dict(
+ cls_score=cls_score,
+ bbox_pred=bbox_pred,
+ pooled_seg_feats=pooled_seg_feats,
+ pooled_part_feats=pooled_part_feats)
+ return bbox_results
+
+ def _assign_and_sample(self, proposal_list, gt_bboxes_3d, gt_labels_3d):
+ """Assign and sample proposals for training.
+
+ Args:
+ proposal_list (list[dict]): Proposals produced by RPN.
+ gt_bboxes_3d (list[:obj:`BaseInstance3DBoxes`]): Ground truth
+ boxes.
+ gt_labels_3d (list[torch.Tensor]): Ground truth labels
+
+ Returns:
+ list[:obj:`SamplingResult`]: Sampled results of each training
+ sample.
+ """
+ sampling_results = []
+ # bbox assign
+ for batch_idx in range(len(proposal_list)):
+ cur_proposal_list = proposal_list[batch_idx]
+ cur_boxes = cur_proposal_list['boxes_3d']
+ cur_labels_3d = cur_proposal_list['labels_3d']
+ cur_gt_bboxes = gt_bboxes_3d[batch_idx].to(cur_boxes.device)
+ cur_gt_labels = gt_labels_3d[batch_idx]
+
+ batch_num_gts = 0
+ # 0 is bg
+ batch_gt_indis = cur_gt_labels.new_full((len(cur_boxes), ), 0)
+ batch_max_overlaps = cur_boxes.tensor.new_zeros(len(cur_boxes))
+ # -1 is bg
+ batch_gt_labels = cur_gt_labels.new_full((len(cur_boxes), ), -1)
+
+ # each class may have its own assigner
+ if isinstance(self.bbox_assigner, list):
+ for i, assigner in enumerate(self.bbox_assigner):
+ gt_per_cls = (cur_gt_labels == i)
+ pred_per_cls = (cur_labels_3d == i)
+ cur_assign_res = assigner.assign(
+ cur_boxes.tensor[pred_per_cls],
+ cur_gt_bboxes.tensor[gt_per_cls],
+ gt_labels=cur_gt_labels[gt_per_cls])
+ # gather assign_results in different class into one result
+ batch_num_gts += cur_assign_res.num_gts
+ # gt inds (1-based)
+ gt_inds_arange_pad = gt_per_cls.nonzero(
+ as_tuple=False).view(-1) + 1
+ # pad 0 for indice unassigned
+ gt_inds_arange_pad = F.pad(
+ gt_inds_arange_pad, (1, 0), mode='constant', value=0)
+ # pad -1 for indice ignore
+ gt_inds_arange_pad = F.pad(
+ gt_inds_arange_pad, (1, 0), mode='constant', value=-1)
+ # convert to 0~gt_num+2 for indices
+ gt_inds_arange_pad += 1
+ # now 0 is bg, >1 is fg in batch_gt_indis
+ batch_gt_indis[pred_per_cls] = gt_inds_arange_pad[
+ cur_assign_res.gt_inds + 1] - 1
+ batch_max_overlaps[
+ pred_per_cls] = cur_assign_res.max_overlaps
+ batch_gt_labels[pred_per_cls] = cur_assign_res.labels
+
+ assign_result = AssignResult(batch_num_gts, batch_gt_indis,
+ batch_max_overlaps,
+ batch_gt_labels)
+ else: # for single class
+ assign_result = self.bbox_assigner.assign(
+ cur_boxes.tensor,
+ cur_gt_bboxes.tensor,
+ gt_labels=cur_gt_labels)
+ # sample boxes
+ sampling_result = self.bbox_sampler.sample(assign_result,
+ cur_boxes.tensor,
+ cur_gt_bboxes.tensor,
+ cur_gt_labels)
+ sampling_results.append(sampling_result)
+ return sampling_results
+
+ def _semantic_forward_train(self, x, voxels_dict, gt_bboxes_3d,
+ gt_labels_3d):
+ """Train semantic head.
+
+ Args:
+ x (torch.Tensor): Point-wise semantic features for segmentation
+ voxels_dict (dict): Contains information of voxels.
+ gt_bboxes_3d (list[:obj:`BaseInstance3DBoxes`]): Ground truth
+ boxes.
+ gt_labels_3d (list[torch.Tensor]): Ground truth labels
+
+ Returns:
+ dict: Segmentation results including losses
+ """
+ semantic_results = self.semantic_head(x)
+ semantic_targets = self.semantic_head.get_targets(
+ voxels_dict, gt_bboxes_3d, gt_labels_3d)
+ loss_semantic = self.semantic_head.loss(semantic_results,
+ semantic_targets)
+ semantic_results.update(loss_semantic=loss_semantic)
+ return semantic_results
diff --git a/mmdet3d/models/roi_heads/point_rcnn_roi_head.py b/mmdet3d/models/roi_heads/point_rcnn_roi_head.py
new file mode 100644
index 0000000..acf7c16
--- /dev/null
+++ b/mmdet3d/models/roi_heads/point_rcnn_roi_head.py
@@ -0,0 +1,286 @@
+# Copyright (c) OpenMMLab. All rights reserved.
+import torch
+from torch.nn import functional as F
+
+from mmdet3d.core import AssignResult
+from mmdet3d.core.bbox import bbox3d2result, bbox3d2roi
+from mmdet.core import build_assigner, build_sampler
+from ..builder import HEADS, build_head, build_roi_extractor
+from .base_3droi_head import Base3DRoIHead
+
+
+@HEADS.register_module()
+class PointRCNNRoIHead(Base3DRoIHead):
+ """RoI head for PointRCNN.
+
+ Args:
+ bbox_head (dict): Config of bbox_head.
+ point_roi_extractor (dict): Config of RoI extractor.
+ train_cfg (dict): Train configs.
+ test_cfg (dict): Test configs.
+ depth_normalizer (float, optional): Normalize depth feature.
+ Defaults to 70.0.
+ init_cfg (dict, optional): Config of initialization. Defaults to None.
+ """
+
+ def __init__(self,
+ bbox_head,
+ point_roi_extractor,
+ train_cfg,
+ test_cfg,
+ depth_normalizer=70.0,
+ pretrained=None,
+ init_cfg=None):
+ super(PointRCNNRoIHead, self).__init__(
+ bbox_head=bbox_head,
+ train_cfg=train_cfg,
+ test_cfg=test_cfg,
+ pretrained=pretrained,
+ init_cfg=init_cfg)
+ self.depth_normalizer = depth_normalizer
+
+ if point_roi_extractor is not None:
+ self.point_roi_extractor = build_roi_extractor(point_roi_extractor)
+
+ self.init_assigner_sampler()
+
+ def init_bbox_head(self, bbox_head):
+ """Initialize box head.
+
+ Args:
+ bbox_head (dict): Config dict of RoI Head.
+ """
+ self.bbox_head = build_head(bbox_head)
+
+ def init_mask_head(self):
+ """Initialize maek head."""
+ pass
+
+ def init_assigner_sampler(self):
+ """Initialize assigner and sampler."""
+ self.bbox_assigner = None
+ self.bbox_sampler = None
+ if self.train_cfg:
+ if isinstance(self.train_cfg.assigner, dict):
+ self.bbox_assigner = build_assigner(self.train_cfg.assigner)
+ elif isinstance(self.train_cfg.assigner, list):
+ self.bbox_assigner = [
+ build_assigner(res) for res in self.train_cfg.assigner
+ ]
+ self.bbox_sampler = build_sampler(self.train_cfg.sampler)
+
+ def forward_train(self, feats_dict, input_metas, proposal_list,
+ gt_bboxes_3d, gt_labels_3d):
+ """Training forward function of PointRCNNRoIHead.
+
+ Args:
+ feats_dict (dict): Contains features from the first stage.
+ imput_metas (list[dict]): Meta info of each input.
+ proposal_list (list[dict]): Proposal information from rpn.
+ The dictionary should contain the following keys:
+
+ - boxes_3d (:obj:`BaseInstance3DBoxes`): Proposal bboxes
+ - labels_3d (torch.Tensor): Labels of proposals
+ gt_bboxes_3d (list[:obj:`BaseInstance3DBoxes`]):
+ GT bboxes of each sample. The bboxes are encapsulated
+ by 3D box structures.
+ gt_labels_3d (list[LongTensor]): GT labels of each sample.
+
+ Returns:
+ dict: Losses from RoI RCNN head.
+ - loss_bbox (torch.Tensor): Loss of bboxes
+ """
+ features = feats_dict['features']
+ points = feats_dict['points']
+ point_cls_preds = feats_dict['points_cls_preds']
+ sem_scores = point_cls_preds.sigmoid()
+ point_scores = sem_scores.max(-1)[0]
+
+ sample_results = self._assign_and_sample(proposal_list, gt_bboxes_3d,
+ gt_labels_3d)
+
+ # concat the depth, semantic features and backbone features
+ features = features.transpose(1, 2).contiguous()
+ point_depths = points.norm(dim=2) / self.depth_normalizer - 0.5
+ features_list = [
+ point_scores.unsqueeze(2),
+ point_depths.unsqueeze(2), features
+ ]
+ features = torch.cat(features_list, dim=2)
+
+ bbox_results = self._bbox_forward_train(features, points,
+ sample_results)
+ losses = dict()
+ losses.update(bbox_results['loss_bbox'])
+
+ return losses
+
+ def simple_test(self, feats_dict, img_metas, proposal_list, **kwargs):
+ """Simple testing forward function of PointRCNNRoIHead.
+
+ Note:
+ This function assumes that the batch size is 1
+
+ Args:
+ feats_dict (dict): Contains features from the first stage.
+ img_metas (list[dict]): Meta info of each image.
+ proposal_list (list[dict]): Proposal information from rpn.
+
+ Returns:
+ dict: Bbox results of one frame.
+ """
+ rois = bbox3d2roi([res['boxes_3d'].tensor for res in proposal_list])
+ labels_3d = [res['labels_3d'] for res in proposal_list]
+
+ features = feats_dict['features']
+ points = feats_dict['points']
+ point_cls_preds = feats_dict['points_cls_preds']
+ sem_scores = point_cls_preds.sigmoid()
+ point_scores = sem_scores.max(-1)[0]
+
+ features = features.transpose(1, 2).contiguous()
+ point_depths = points.norm(dim=2) / self.depth_normalizer - 0.5
+ features_list = [
+ point_scores.unsqueeze(2),
+ point_depths.unsqueeze(2), features
+ ]
+
+ features = torch.cat(features_list, dim=2)
+ batch_size = features.shape[0]
+ bbox_results = self._bbox_forward(features, points, batch_size, rois)
+ object_score = bbox_results['cls_score'].sigmoid()
+ bbox_list = self.bbox_head.get_bboxes(
+ rois,
+ object_score,
+ bbox_results['bbox_pred'],
+ labels_3d,
+ img_metas,
+ cfg=self.test_cfg)
+
+ bbox_results = [
+ bbox3d2result(bboxes, scores, labels)
+ for bboxes, scores, labels in bbox_list
+ ]
+ return bbox_results
+
+ def _bbox_forward_train(self, features, points, sampling_results):
+ """Forward training function of roi_extractor and bbox_head.
+
+ Args:
+ features (torch.Tensor): Backbone features with depth and \
+ semantic features.
+ points (torch.Tensor): Pointcloud.
+ sampling_results (:obj:`SamplingResult`): Sampled results used
+ for training.
+
+ Returns:
+ dict: Forward results including losses and predictions.
+ """
+ rois = bbox3d2roi([res.bboxes for res in sampling_results])
+ batch_size = features.shape[0]
+ bbox_results = self._bbox_forward(features, points, batch_size, rois)
+ bbox_targets = self.bbox_head.get_targets(sampling_results,
+ self.train_cfg)
+
+ loss_bbox = self.bbox_head.loss(bbox_results['cls_score'],
+ bbox_results['bbox_pred'], rois,
+ *bbox_targets)
+
+ bbox_results.update(loss_bbox=loss_bbox)
+ return bbox_results
+
+ def _bbox_forward(self, features, points, batch_size, rois):
+ """Forward function of roi_extractor and bbox_head used in both
+ training and testing.
+
+ Args:
+ features (torch.Tensor): Backbone features with depth and
+ semantic features.
+ points (torch.Tensor): Pointcloud.
+ batch_size (int): Batch size.
+ rois (torch.Tensor): RoI boxes.
+
+ Returns:
+ dict: Contains predictions of bbox_head and
+ features of roi_extractor.
+ """
+ pooled_point_feats = self.point_roi_extractor(features, points,
+ batch_size, rois)
+
+ cls_score, bbox_pred = self.bbox_head(pooled_point_feats)
+ bbox_results = dict(cls_score=cls_score, bbox_pred=bbox_pred)
+ return bbox_results
+
+ def _assign_and_sample(self, proposal_list, gt_bboxes_3d, gt_labels_3d):
+ """Assign and sample proposals for training.
+
+ Args:
+ proposal_list (list[dict]): Proposals produced by RPN.
+ gt_bboxes_3d (list[:obj:`BaseInstance3DBoxes`]): Ground truth
+ boxes.
+ gt_labels_3d (list[torch.Tensor]): Ground truth labels
+
+ Returns:
+ list[:obj:`SamplingResult`]: Sampled results of each training
+ sample.
+ """
+ sampling_results = []
+ # bbox assign
+ for batch_idx in range(len(proposal_list)):
+ cur_proposal_list = proposal_list[batch_idx]
+ cur_boxes = cur_proposal_list['boxes_3d']
+ cur_labels_3d = cur_proposal_list['labels_3d']
+ cur_gt_bboxes = gt_bboxes_3d[batch_idx].to(cur_boxes.device)
+ cur_gt_labels = gt_labels_3d[batch_idx]
+ batch_num_gts = 0
+ # 0 is bg
+ batch_gt_indis = cur_gt_labels.new_full((len(cur_boxes), ), 0)
+ batch_max_overlaps = cur_boxes.tensor.new_zeros(len(cur_boxes))
+ # -1 is bg
+ batch_gt_labels = cur_gt_labels.new_full((len(cur_boxes), ), -1)
+
+ # each class may have its own assigner
+ if isinstance(self.bbox_assigner, list):
+ for i, assigner in enumerate(self.bbox_assigner):
+ gt_per_cls = (cur_gt_labels == i)
+ pred_per_cls = (cur_labels_3d == i)
+ cur_assign_res = assigner.assign(
+ cur_boxes.tensor[pred_per_cls],
+ cur_gt_bboxes.tensor[gt_per_cls],
+ gt_labels=cur_gt_labels[gt_per_cls])
+ # gather assign_results in different class into one result
+ batch_num_gts += cur_assign_res.num_gts
+ # gt inds (1-based)
+ gt_inds_arange_pad = gt_per_cls.nonzero(
+ as_tuple=False).view(-1) + 1
+ # pad 0 for indice unassigned
+ gt_inds_arange_pad = F.pad(
+ gt_inds_arange_pad, (1, 0), mode='constant', value=0)
+ # pad -1 for indice ignore
+ gt_inds_arange_pad = F.pad(
+ gt_inds_arange_pad, (1, 0), mode='constant', value=-1)
+ # convert to 0~gt_num+2 for indices
+ gt_inds_arange_pad += 1
+ # now 0 is bg, >1 is fg in batch_gt_indis
+ batch_gt_indis[pred_per_cls] = gt_inds_arange_pad[
+ cur_assign_res.gt_inds + 1] - 1
+ batch_max_overlaps[
+ pred_per_cls] = cur_assign_res.max_overlaps
+ batch_gt_labels[pred_per_cls] = cur_assign_res.labels
+
+ assign_result = AssignResult(batch_num_gts, batch_gt_indis,
+ batch_max_overlaps,
+ batch_gt_labels)
+ else: # for single class
+ assign_result = self.bbox_assigner.assign(
+ cur_boxes.tensor,
+ cur_gt_bboxes.tensor,
+ gt_labels=cur_gt_labels)
+
+ # sample boxes
+ sampling_result = self.bbox_sampler.sample(assign_result,
+ cur_boxes.tensor,
+ cur_gt_bboxes.tensor,
+ cur_gt_labels)
+ sampling_results.append(sampling_result)
+ return sampling_results
diff --git a/mmdet3d/models/roi_heads/roi_extractors/__init__.py b/mmdet3d/models/roi_heads/roi_extractors/__init__.py
new file mode 100644
index 0000000..70c2881
--- /dev/null
+++ b/mmdet3d/models/roi_heads/roi_extractors/__init__.py
@@ -0,0 +1,9 @@
+# Copyright (c) OpenMMLab. All rights reserved.
+from mmdet.models.roi_heads.roi_extractors import SingleRoIExtractor
+from .single_roiaware_extractor import Single3DRoIAwareExtractor
+from .single_roipoint_extractor import Single3DRoIPointExtractor
+
+__all__ = [
+ 'SingleRoIExtractor', 'Single3DRoIAwareExtractor',
+ 'Single3DRoIPointExtractor'
+]
diff --git a/mmdet3d/models/roi_heads/roi_extractors/single_roiaware_extractor.py b/mmdet3d/models/roi_heads/roi_extractors/single_roiaware_extractor.py
new file mode 100644
index 0000000..c27a004
--- /dev/null
+++ b/mmdet3d/models/roi_heads/roi_extractors/single_roiaware_extractor.py
@@ -0,0 +1,54 @@
+# Copyright (c) OpenMMLab. All rights reserved.
+import torch
+from mmcv import ops
+from mmcv.runner import BaseModule
+
+from mmdet3d.models.builder import ROI_EXTRACTORS
+
+
+@ROI_EXTRACTORS.register_module()
+class Single3DRoIAwareExtractor(BaseModule):
+ """Point-wise roi-aware Extractor.
+
+ Extract Point-wise roi features.
+
+ Args:
+ roi_layer (dict): The config of roi layer.
+ """
+
+ def __init__(self, roi_layer=None, init_cfg=None):
+ super(Single3DRoIAwareExtractor, self).__init__(init_cfg=init_cfg)
+ self.roi_layer = self.build_roi_layers(roi_layer)
+
+ def build_roi_layers(self, layer_cfg):
+ """Build roi layers using `layer_cfg`"""
+ cfg = layer_cfg.copy()
+ layer_type = cfg.pop('type')
+ assert hasattr(ops, layer_type)
+ layer_cls = getattr(ops, layer_type)
+ roi_layers = layer_cls(**cfg)
+ return roi_layers
+
+ def forward(self, feats, coordinate, batch_inds, rois):
+ """Extract point-wise roi features.
+
+ Args:
+ feats (torch.FloatTensor): Point-wise features with
+ shape (batch, npoints, channels) for pooling.
+ coordinate (torch.FloatTensor): Coordinate of each point.
+ batch_inds (torch.LongTensor): Indicate the batch of each point.
+ rois (torch.FloatTensor): Roi boxes with batch indices.
+
+ Returns:
+ torch.FloatTensor: Pooled features
+ """
+ pooled_roi_feats = []
+ for batch_idx in range(int(batch_inds.max()) + 1):
+ roi_inds = (rois[..., 0].int() == batch_idx)
+ coors_inds = (batch_inds.int() == batch_idx)
+ pooled_roi_feat = self.roi_layer(rois[..., 1:][roi_inds],
+ coordinate[coors_inds],
+ feats[coors_inds])
+ pooled_roi_feats.append(pooled_roi_feat)
+ pooled_roi_feats = torch.cat(pooled_roi_feats, 0)
+ return pooled_roi_feats
diff --git a/mmdet3d/models/roi_heads/roi_extractors/single_roipoint_extractor.py b/mmdet3d/models/roi_heads/roi_extractors/single_roipoint_extractor.py
new file mode 100644
index 0000000..4983a01
--- /dev/null
+++ b/mmdet3d/models/roi_heads/roi_extractors/single_roipoint_extractor.py
@@ -0,0 +1,64 @@
+# Copyright (c) OpenMMLab. All rights reserved.
+import torch
+from mmcv import ops
+from torch import nn as nn
+
+from mmdet3d.core.bbox.structures import rotation_3d_in_axis
+from mmdet3d.models.builder import ROI_EXTRACTORS
+
+
+@ROI_EXTRACTORS.register_module()
+class Single3DRoIPointExtractor(nn.Module):
+ """Point-wise roi-aware Extractor.
+
+ Extract Point-wise roi features.
+
+ Args:
+ roi_layer (dict): The config of roi layer.
+ """
+
+ def __init__(self, roi_layer=None):
+ super(Single3DRoIPointExtractor, self).__init__()
+ self.roi_layer = self.build_roi_layers(roi_layer)
+
+ def build_roi_layers(self, layer_cfg):
+ """Build roi layers using `layer_cfg`"""
+ cfg = layer_cfg.copy()
+ layer_type = cfg.pop('type')
+ assert hasattr(ops, layer_type)
+ layer_cls = getattr(ops, layer_type)
+ roi_layers = layer_cls(**cfg)
+ return roi_layers
+
+ def forward(self, feats, coordinate, batch_inds, rois):
+ """Extract point-wise roi features.
+
+ Args:
+ feats (torch.FloatTensor): Point-wise features with
+ shape (batch, npoints, channels) for pooling.
+ coordinate (torch.FloatTensor): Coordinate of each point.
+ batch_inds (torch.LongTensor): Indicate the batch of each point.
+ rois (torch.FloatTensor): Roi boxes with batch indices.
+
+ Returns:
+ torch.FloatTensor: Pooled features
+ """
+ rois = rois[..., 1:]
+ rois = rois.view(batch_inds, -1, rois.shape[-1])
+ with torch.no_grad():
+ pooled_roi_feat, pooled_empty_flag = self.roi_layer(
+ coordinate, feats, rois)
+
+ # canonical transformation
+ roi_center = rois[:, :, 0:3]
+ pooled_roi_feat[:, :, :, 0:3] -= roi_center.unsqueeze(dim=2)
+ pooled_roi_feat = pooled_roi_feat.view(-1,
+ pooled_roi_feat.shape[-2],
+ pooled_roi_feat.shape[-1])
+ pooled_roi_feat[:, :, 0:3] = rotation_3d_in_axis(
+ pooled_roi_feat[:, :, 0:3],
+ -(rois.view(-1, rois.shape[-1])[:, 6]),
+ axis=2)
+ pooled_roi_feat[pooled_empty_flag.view(-1) > 0] = 0
+
+ return pooled_roi_feat
diff --git a/mmdet3d/models/segmentors/__init__.py b/mmdet3d/models/segmentors/__init__.py
new file mode 100644
index 0000000..29fbc33
--- /dev/null
+++ b/mmdet3d/models/segmentors/__init__.py
@@ -0,0 +1,5 @@
+# Copyright (c) OpenMMLab. All rights reserved.
+from .base import Base3DSegmentor
+from .encoder_decoder import EncoderDecoder3D
+
+__all__ = ['Base3DSegmentor', 'EncoderDecoder3D']
diff --git a/mmdet3d/models/segmentors/base.py b/mmdet3d/models/segmentors/base.py
new file mode 100644
index 0000000..9913698
--- /dev/null
+++ b/mmdet3d/models/segmentors/base.py
@@ -0,0 +1,136 @@
+# Copyright (c) OpenMMLab. All rights reserved.
+from os import path as osp
+
+import mmcv
+import numpy as np
+import torch
+from mmcv.parallel import DataContainer as DC
+from mmcv.runner import auto_fp16
+
+from mmdet3d.core import show_seg_result
+from mmseg.models.segmentors import BaseSegmentor
+
+
+class Base3DSegmentor(BaseSegmentor):
+ """Base class for 3D segmentors.
+
+ The main difference with `BaseSegmentor` is that we modify the keys in
+ data_dict and use a 3D seg specific visualization function.
+ """
+
+ @property
+ def with_regularization_loss(self):
+ """bool: whether the segmentor has regularization loss for weight"""
+ return hasattr(self, 'loss_regularization') and \
+ self.loss_regularization is not None
+
+ def forward_test(self, points, img_metas, **kwargs):
+ """Calls either simple_test or aug_test depending on the length of
+ outer list of points. If len(points) == 1, call simple_test. Otherwise
+ call aug_test to aggregate the test results by e.g. voting.
+
+ Args:
+ points (list[list[torch.Tensor]]): the outer list indicates
+ test-time augmentations and inner torch.Tensor should have a
+ shape BXNxC, which contains all points in the batch.
+ img_metas (list[list[dict]]): the outer list indicates test-time
+ augs (multiscale, flip, etc.) and the inner list indicates
+ images in a batch.
+ """
+ for var, name in [(points, 'points'), (img_metas, 'img_metas')]:
+ if not isinstance(var, list):
+ raise TypeError(f'{name} must be a list, but got {type(var)}')
+
+ num_augs = len(points)
+ if num_augs != len(img_metas):
+ raise ValueError(f'num of augmentations ({len(points)}) != '
+ f'num of image meta ({len(img_metas)})')
+
+ if num_augs == 1:
+ return self.simple_test(points[0], img_metas[0], **kwargs)
+ else:
+ return self.aug_test(points, img_metas, **kwargs)
+
+ @auto_fp16(apply_to=('points'))
+ def forward(self, return_loss=True, **kwargs):
+ """Calls either forward_train or forward_test depending on whether
+ return_loss=True.
+
+ Note this setting will change the expected inputs. When
+ `return_loss=True`, point and img_metas are single-nested (i.e.
+ torch.Tensor and list[dict]), and when `resturn_loss=False`, point and
+ img_metas should be double nested (i.e. list[torch.Tensor],
+ list[list[dict]]), with the outer list indicating test time
+ augmentations.
+ """
+ if return_loss:
+ return self.forward_train(**kwargs)
+ else:
+ return self.forward_test(**kwargs)
+
+ def show_results(self,
+ data,
+ result,
+ palette=None,
+ out_dir=None,
+ ignore_index=None,
+ show=False,
+ score_thr=None):
+ """Results visualization.
+
+ Args:
+ data (list[dict]): Input points and the information of the sample.
+ result (list[dict]): Prediction results.
+ palette (list[list[int]]] | np.ndarray): The palette of
+ segmentation map. If None is given, random palette will be
+ generated. Default: None
+ out_dir (str): Output directory of visualization result.
+ ignore_index (int, optional): The label index to be ignored, e.g.
+ unannotated points. If None is given, set to len(self.CLASSES).
+ Defaults to None.
+ show (bool, optional): Determines whether you are
+ going to show result by open3d.
+ Defaults to False.
+ TODO: implement score_thr of Base3DSegmentor.
+ score_thr (float, optional): Score threshold of bounding boxes.
+ Default to None.
+ Not implemented yet, but it is here for unification.
+ """
+ assert out_dir is not None, 'Expect out_dir, got none.'
+ if palette is None:
+ if self.PALETTE is None:
+ palette = np.random.randint(
+ 0, 255, size=(len(self.CLASSES), 3))
+ else:
+ palette = self.PALETTE
+ palette = np.array(palette)
+ for batch_id in range(len(result)):
+ if isinstance(data['points'][0], DC):
+ points = data['points'][0]._data[0][batch_id].numpy()
+ elif mmcv.is_list_of(data['points'][0], torch.Tensor):
+ points = data['points'][0][batch_id]
+ else:
+ ValueError(f"Unsupported data type {type(data['points'][0])} "
+ f'for visualization!')
+ if isinstance(data['img_metas'][0], DC):
+ pts_filename = data['img_metas'][0]._data[0][batch_id][
+ 'pts_filename']
+ elif mmcv.is_list_of(data['img_metas'][0], dict):
+ pts_filename = data['img_metas'][0][batch_id]['pts_filename']
+ else:
+ ValueError(
+ f"Unsupported data type {type(data['img_metas'][0])} "
+ f'for visualization!')
+ file_name = osp.split(pts_filename)[-1].split('.')[0]
+
+ pred_sem_mask = result[batch_id]['semantic_mask'].cpu().numpy()
+
+ show_seg_result(
+ points,
+ None,
+ pred_sem_mask,
+ out_dir,
+ file_name,
+ palette,
+ ignore_index,
+ show=show)
diff --git a/mmdet3d/models/segmentors/encoder_decoder.py b/mmdet3d/models/segmentors/encoder_decoder.py
new file mode 100644
index 0000000..1a4fee9
--- /dev/null
+++ b/mmdet3d/models/segmentors/encoder_decoder.py
@@ -0,0 +1,454 @@
+# Copyright (c) OpenMMLab. All rights reserved.
+import numpy as np
+import torch
+from torch import nn as nn
+from torch.nn import functional as F
+
+from mmseg.core import add_prefix
+from ..builder import (SEGMENTORS, build_backbone, build_head, build_loss,
+ build_neck)
+from .base import Base3DSegmentor
+
+
+@SEGMENTORS.register_module()
+class EncoderDecoder3D(Base3DSegmentor):
+ """3D Encoder Decoder segmentors.
+
+ EncoderDecoder typically consists of backbone, decode_head, auxiliary_head.
+ Note that auxiliary_head is only used for deep supervision during training,
+ which could be thrown during inference.
+ """
+
+ def __init__(self,
+ backbone,
+ decode_head,
+ neck=None,
+ auxiliary_head=None,
+ loss_regularization=None,
+ train_cfg=None,
+ test_cfg=None,
+ pretrained=None,
+ init_cfg=None):
+ super(EncoderDecoder3D, self).__init__(init_cfg=init_cfg)
+ self.backbone = build_backbone(backbone)
+ if neck is not None:
+ self.neck = build_neck(neck)
+ self._init_decode_head(decode_head)
+ self._init_auxiliary_head(auxiliary_head)
+ self._init_loss_regularization(loss_regularization)
+
+ self.train_cfg = train_cfg
+ self.test_cfg = test_cfg
+ assert self.with_decode_head, \
+ '3D EncoderDecoder Segmentor should have a decode_head'
+
+ def _init_decode_head(self, decode_head):
+ """Initialize ``decode_head``"""
+ self.decode_head = build_head(decode_head)
+ self.num_classes = self.decode_head.num_classes
+
+ def _init_auxiliary_head(self, auxiliary_head):
+ """Initialize ``auxiliary_head``"""
+ if auxiliary_head is not None:
+ if isinstance(auxiliary_head, list):
+ self.auxiliary_head = nn.ModuleList()
+ for head_cfg in auxiliary_head:
+ self.auxiliary_head.append(build_head(head_cfg))
+ else:
+ self.auxiliary_head = build_head(auxiliary_head)
+
+ def _init_loss_regularization(self, loss_regularization):
+ """Initialize ``loss_regularization``"""
+ if loss_regularization is not None:
+ if isinstance(loss_regularization, list):
+ self.loss_regularization = nn.ModuleList()
+ for loss_cfg in loss_regularization:
+ self.loss_regularization.append(build_loss(loss_cfg))
+ else:
+ self.loss_regularization = build_loss(loss_regularization)
+
+ def extract_feat(self, points):
+ """Extract features from points."""
+ x = self.backbone(points)
+ if self.with_neck:
+ x = self.neck(x)
+ return x
+
+ def encode_decode(self, points, img_metas):
+ """Encode points with backbone and decode into a semantic segmentation
+ map of the same size as input.
+
+ Args:
+ points (torch.Tensor): Input points of shape [B, N, 3+C].
+ img_metas (list[dict]): Meta information of each sample.
+
+ Returns:
+ torch.Tensor: Segmentation logits of shape [B, num_classes, N].
+ """
+ x = self.extract_feat(points)
+ out = self._decode_head_forward_test(x, img_metas)
+ return out
+
+ def _decode_head_forward_train(self, x, img_metas, pts_semantic_mask):
+ """Run forward function and calculate loss for decode head in
+ training."""
+ losses = dict()
+ loss_decode = self.decode_head.forward_train(x, img_metas,
+ pts_semantic_mask,
+ self.train_cfg)
+
+ losses.update(add_prefix(loss_decode, 'decode'))
+ return losses
+
+ def _decode_head_forward_test(self, x, img_metas):
+ """Run forward function and calculate loss for decode head in
+ inference."""
+ seg_logits = self.decode_head.forward_test(x, img_metas, self.test_cfg)
+ return seg_logits
+
+ def _auxiliary_head_forward_train(self, x, img_metas, pts_semantic_mask):
+ """Run forward function and calculate loss for auxiliary head in
+ training."""
+ losses = dict()
+ if isinstance(self.auxiliary_head, nn.ModuleList):
+ for idx, aux_head in enumerate(self.auxiliary_head):
+ loss_aux = aux_head.forward_train(x, img_metas,
+ pts_semantic_mask,
+ self.train_cfg)
+ losses.update(add_prefix(loss_aux, f'aux_{idx}'))
+ else:
+ loss_aux = self.auxiliary_head.forward_train(
+ x, img_metas, pts_semantic_mask, self.train_cfg)
+ losses.update(add_prefix(loss_aux, 'aux'))
+
+ return losses
+
+ def _loss_regularization_forward_train(self):
+ """Calculate regularization loss for model weight in training."""
+ losses = dict()
+ if isinstance(self.loss_regularization, nn.ModuleList):
+ for idx, regularize_loss in enumerate(self.loss_regularization):
+ loss_regularize = dict(
+ loss_regularize=regularize_loss(self.modules()))
+ losses.update(add_prefix(loss_regularize, f'regularize_{idx}'))
+ else:
+ loss_regularize = dict(
+ loss_regularize=self.loss_regularization(self.modules()))
+ losses.update(add_prefix(loss_regularize, 'regularize'))
+
+ return losses
+
+ def forward_dummy(self, points):
+ """Dummy forward function."""
+ seg_logit = self.encode_decode(points, None)
+
+ return seg_logit
+
+ def forward_train(self, points, img_metas, pts_semantic_mask):
+ """Forward function for training.
+
+ Args:
+ points (list[torch.Tensor]): List of points of shape [N, C].
+ img_metas (list): Image metas.
+ pts_semantic_mask (list[torch.Tensor]): List of point-wise semantic
+ labels of shape [N].
+
+ Returns:
+ dict[str, Tensor]: Losses.
+ """
+ points_cat = torch.stack(points)
+ pts_semantic_mask_cat = torch.stack(pts_semantic_mask)
+
+ # extract features using backbone
+ x = self.extract_feat(points_cat)
+
+ losses = dict()
+
+ loss_decode = self._decode_head_forward_train(x, img_metas,
+ pts_semantic_mask_cat)
+ losses.update(loss_decode)
+
+ if self.with_auxiliary_head:
+ loss_aux = self._auxiliary_head_forward_train(
+ x, img_metas, pts_semantic_mask_cat)
+ losses.update(loss_aux)
+
+ if self.with_regularization_loss:
+ loss_regularize = self._loss_regularization_forward_train()
+ losses.update(loss_regularize)
+
+ return losses
+
+ @staticmethod
+ def _input_generation(coords,
+ patch_center,
+ coord_max,
+ feats,
+ use_normalized_coord=False):
+ """Generating model input.
+
+ Generate input by subtracting patch center and adding additional
+ features. Currently support colors and normalized xyz as features.
+
+ Args:
+ coords (torch.Tensor): Sampled 3D point coordinate of shape [S, 3].
+ patch_center (torch.Tensor): Center coordinate of the patch.
+ coord_max (torch.Tensor): Max coordinate of all 3D points.
+ feats (torch.Tensor): Features of sampled points of shape [S, C].
+ use_normalized_coord (bool, optional): Whether to use normalized
+ xyz as additional features. Defaults to False.
+
+ Returns:
+ torch.Tensor: The generated input data of shape [S, 3+C'].
+ """
+ # subtract patch center, the z dimension is not centered
+ centered_coords = coords.clone()
+ centered_coords[:, 0] -= patch_center[0]
+ centered_coords[:, 1] -= patch_center[1]
+
+ # normalized coordinates as extra features
+ if use_normalized_coord:
+ normalized_coord = coords / coord_max
+ feats = torch.cat([feats, normalized_coord], dim=1)
+
+ points = torch.cat([centered_coords, feats], dim=1)
+
+ return points
+
+ def _sliding_patch_generation(self,
+ points,
+ num_points,
+ block_size,
+ sample_rate=0.5,
+ use_normalized_coord=False,
+ eps=1e-3):
+ """Sampling points in a sliding window fashion.
+
+ First sample patches to cover all the input points.
+ Then sample points in each patch to batch points of a certain number.
+
+ Args:
+ points (torch.Tensor): Input points of shape [N, 3+C].
+ num_points (int): Number of points to be sampled in each patch.
+ block_size (float, optional): Size of a patch to sample.
+ sample_rate (float, optional): Stride used in sliding patch.
+ Defaults to 0.5.
+ use_normalized_coord (bool, optional): Whether to use normalized
+ xyz as additional features. Defaults to False.
+ eps (float, optional): A value added to patch boundary to guarantee
+ points coverage. Defaults to 1e-3.
+
+ Returns:
+ np.ndarray | np.ndarray:
+
+ - patch_points (torch.Tensor): Points of different patches of
+ shape [K, N, 3+C].
+ - patch_idxs (torch.Tensor): Index of each point in
+ `patch_points`, of shape [K, N].
+ """
+ device = points.device
+ # we assume the first three dims are points' 3D coordinates
+ # and the rest dims are their per-point features
+ coords = points[:, :3]
+ feats = points[:, 3:]
+
+ coord_max = coords.max(0)[0]
+ coord_min = coords.min(0)[0]
+ stride = block_size * sample_rate
+ num_grid_x = int(
+ torch.ceil((coord_max[0] - coord_min[0] - block_size) /
+ stride).item() + 1)
+ num_grid_y = int(
+ torch.ceil((coord_max[1] - coord_min[1] - block_size) /
+ stride).item() + 1)
+
+ patch_points, patch_idxs = [], []
+ for idx_y in range(num_grid_y):
+ s_y = coord_min[1] + idx_y * stride
+ e_y = torch.min(s_y + block_size, coord_max[1])
+ s_y = e_y - block_size
+ for idx_x in range(num_grid_x):
+ s_x = coord_min[0] + idx_x * stride
+ e_x = torch.min(s_x + block_size, coord_max[0])
+ s_x = e_x - block_size
+
+ # extract points within this patch
+ cur_min = torch.tensor([s_x, s_y, coord_min[2]]).to(device)
+ cur_max = torch.tensor([e_x, e_y, coord_max[2]]).to(device)
+ cur_choice = ((coords >= cur_min - eps) &
+ (coords <= cur_max + eps)).all(dim=1)
+
+ if not cur_choice.any(): # no points in this patch
+ continue
+
+ # sample points in this patch to multiple batches
+ cur_center = cur_min + block_size / 2.0
+ point_idxs = torch.nonzero(cur_choice, as_tuple=True)[0]
+ num_batch = int(np.ceil(point_idxs.shape[0] / num_points))
+ point_size = int(num_batch * num_points)
+ replace = point_size > 2 * point_idxs.shape[0]
+ num_repeat = point_size - point_idxs.shape[0]
+ if replace: # duplicate
+ point_idxs_repeat = point_idxs[torch.randint(
+ 0, point_idxs.shape[0],
+ size=(num_repeat, )).to(device)]
+ else:
+ point_idxs_repeat = point_idxs[torch.randperm(
+ point_idxs.shape[0])[:num_repeat]]
+
+ choices = torch.cat([point_idxs, point_idxs_repeat], dim=0)
+ choices = choices[torch.randperm(choices.shape[0])]
+
+ # construct model input
+ point_batches = self._input_generation(
+ coords[choices],
+ cur_center,
+ coord_max,
+ feats[choices],
+ use_normalized_coord=use_normalized_coord)
+
+ patch_points.append(point_batches)
+ patch_idxs.append(choices)
+
+ patch_points = torch.cat(patch_points, dim=0)
+ patch_idxs = torch.cat(patch_idxs, dim=0)
+
+ # make sure all points are sampled at least once
+ assert torch.unique(patch_idxs).shape[0] == points.shape[0], \
+ 'some points are not sampled in sliding inference'
+
+ return patch_points, patch_idxs
+
+ def slide_inference(self, point, img_meta, rescale):
+ """Inference by sliding-window with overlap.
+
+ Args:
+ point (torch.Tensor): Input points of shape [N, 3+C].
+ img_meta (dict): Meta information of input sample.
+ rescale (bool): Whether transform to original number of points.
+ Will be used for voxelization based segmentors.
+
+ Returns:
+ Tensor: The output segmentation map of shape [num_classes, N].
+ """
+ num_points = self.test_cfg.num_points
+ block_size = self.test_cfg.block_size
+ sample_rate = self.test_cfg.sample_rate
+ use_normalized_coord = self.test_cfg.use_normalized_coord
+ batch_size = self.test_cfg.batch_size * num_points
+
+ # patch_points is of shape [K*N, 3+C], patch_idxs is of shape [K*N]
+ patch_points, patch_idxs = self._sliding_patch_generation(
+ point, num_points, block_size, sample_rate, use_normalized_coord)
+ feats_dim = patch_points.shape[1]
+ seg_logits = [] # save patch predictions
+
+ for batch_idx in range(0, patch_points.shape[0], batch_size):
+ batch_points = patch_points[batch_idx:batch_idx + batch_size]
+ batch_points = batch_points.view(-1, num_points, feats_dim)
+ # batch_seg_logit is of shape [B, num_classes, N]
+ batch_seg_logit = self.encode_decode(batch_points, img_meta)
+ batch_seg_logit = batch_seg_logit.transpose(1, 2).contiguous()
+ seg_logits.append(batch_seg_logit.view(-1, self.num_classes))
+
+ # aggregate per-point logits by indexing sum and dividing count
+ seg_logits = torch.cat(seg_logits, dim=0) # [K*N, num_classes]
+ expand_patch_idxs = patch_idxs.unsqueeze(1).repeat(1, self.num_classes)
+ preds = point.new_zeros((point.shape[0], self.num_classes)).\
+ scatter_add_(dim=0, index=expand_patch_idxs, src=seg_logits)
+ count_mat = torch.bincount(patch_idxs)
+ preds = preds / count_mat[:, None]
+
+ # TODO: if rescale and voxelization segmentor
+
+ return preds.transpose(0, 1) # to [num_classes, K*N]
+
+ def whole_inference(self, points, img_metas, rescale):
+ """Inference with full scene (one forward pass without sliding)."""
+ seg_logit = self.encode_decode(points, img_metas)
+ # TODO: if rescale and voxelization segmentor
+ return seg_logit
+
+ def inference(self, points, img_metas, rescale):
+ """Inference with slide/whole style.
+
+ Args:
+ points (torch.Tensor): Input points of shape [B, N, 3+C].
+ img_metas (list[dict]): Meta information of each sample.
+ rescale (bool): Whether transform to original number of points.
+ Will be used for voxelization based segmentors.
+
+ Returns:
+ Tensor: The output segmentation map.
+ """
+ assert self.test_cfg.mode in ['slide', 'whole']
+ if self.test_cfg.mode == 'slide':
+ seg_logit = torch.stack([
+ self.slide_inference(point, img_meta, rescale)
+ for point, img_meta in zip(points, img_metas)
+ ], 0)
+ else:
+ seg_logit = self.whole_inference(points, img_metas, rescale)
+ output = F.softmax(seg_logit, dim=1)
+ return output
+
+ def simple_test(self, points, img_metas, rescale=True):
+ """Simple test with single scene.
+
+ Args:
+ points (list[torch.Tensor]): List of points of shape [N, 3+C].
+ img_metas (list[dict]): Meta information of each sample.
+ rescale (bool): Whether transform to original number of points.
+ Will be used for voxelization based segmentors.
+ Defaults to True.
+
+ Returns:
+ list[dict]: The output prediction result with following keys:
+
+ - semantic_mask (Tensor): Segmentation mask of shape [N].
+ """
+ # 3D segmentation requires per-point prediction, so it's impossible
+ # to use down-sampling to get a batch of scenes with same num_points
+ # therefore, we only support testing one scene every time
+ seg_pred = []
+ for point, img_meta in zip(points, img_metas):
+ seg_prob = self.inference(point.unsqueeze(0), [img_meta],
+ rescale)[0]
+ seg_map = seg_prob.argmax(0) # [N]
+ # to cpu tensor for consistency with det3d
+ seg_map = seg_map.cpu()
+ seg_pred.append(seg_map)
+ # warp in dict
+ seg_pred = [dict(semantic_mask=seg_map) for seg_map in seg_pred]
+ return seg_pred
+
+ def aug_test(self, points, img_metas, rescale=True):
+ """Test with augmentations.
+
+ Args:
+ points (list[torch.Tensor]): List of points of shape [B, N, 3+C].
+ img_metas (list[list[dict]]): Meta information of each sample.
+ Outer list are different samples while inner is different augs.
+ rescale (bool): Whether transform to original number of points.
+ Will be used for voxelization based segmentors.
+ Defaults to True.
+
+ Returns:
+ list[dict]: The output prediction result with following keys:
+
+ - semantic_mask (Tensor): Segmentation mask of shape [N].
+ """
+ # in aug_test, one scene going through different augmentations could
+ # have the same number of points and are stacked as a batch
+ # to save memory, we get augmented seg logit inplace
+ seg_pred = []
+ for point, img_meta in zip(points, img_metas):
+ seg_prob = self.inference(point, img_meta, rescale)
+ seg_prob = seg_prob.mean(0) # [num_classes, N]
+ seg_map = seg_prob.argmax(0) # [N]
+ # to cpu tensor for consistency with det3d
+ seg_map = seg_map.cpu()
+ seg_pred.append(seg_map)
+ # warp in dict
+ seg_pred = [dict(semantic_mask=seg_map) for seg_map in seg_pred]
+ return seg_pred
diff --git a/mmdet3d/models/utils/__init__.py b/mmdet3d/models/utils/__init__.py
new file mode 100644
index 0000000..92a0499
--- /dev/null
+++ b/mmdet3d/models/utils/__init__.py
@@ -0,0 +1,11 @@
+# Copyright (c) OpenMMLab. All rights reserved.
+from .clip_sigmoid import clip_sigmoid
+from .edge_indices import get_edge_indices
+from .gen_keypoints import get_keypoints
+from .handle_objs import filter_outside_objs, handle_proj_objs
+from .mlp import MLP
+
+__all__ = [
+ 'clip_sigmoid', 'MLP', 'get_edge_indices', 'filter_outside_objs',
+ 'handle_proj_objs', 'get_keypoints'
+]
diff --git a/mmdet3d/models/utils/clip_sigmoid.py b/mmdet3d/models/utils/clip_sigmoid.py
new file mode 100644
index 0000000..3afd4ed
--- /dev/null
+++ b/mmdet3d/models/utils/clip_sigmoid.py
@@ -0,0 +1,17 @@
+# Copyright (c) OpenMMLab. All rights reserved.
+import torch
+
+
+def clip_sigmoid(x, eps=1e-4):
+ """Sigmoid function for input feature.
+
+ Args:
+ x (torch.Tensor): Input feature map with the shape of [B, N, H, W].
+ eps (float, optional): Lower bound of the range to be clamped to.
+ Defaults to 1e-4.
+
+ Returns:
+ torch.Tensor: Feature map after sigmoid.
+ """
+ y = torch.clamp(x.sigmoid_(), min=eps, max=1 - eps)
+ return y
diff --git a/mmdet3d/models/utils/edge_indices.py b/mmdet3d/models/utils/edge_indices.py
new file mode 100644
index 0000000..5dcb71f
--- /dev/null
+++ b/mmdet3d/models/utils/edge_indices.py
@@ -0,0 +1,88 @@
+# Copyright (c) OpenMMLab. All rights reserved.
+import numpy as np
+import torch
+
+
+def get_edge_indices(img_metas,
+ downsample_ratio,
+ step=1,
+ pad_mode='default',
+ dtype=np.float32,
+ device='cpu'):
+ """Function to filter the objects label outside the image.
+ The edge_indices are generated using numpy on cpu rather
+ than on CUDA due to the latency issue. When batch size = 8,
+ this function with numpy array is ~8 times faster than that
+ with CUDA tensor (0.09s and 0.72s in 100 runs).
+
+ Args:
+ img_metas (list[dict]): Meta information of each image, e.g.,
+ image size, scaling factor, etc.
+ downsample_ratio (int): Downsample ratio of output feature,
+ step (int, optional): Step size used for generateing
+ edge indices. Default: 1.
+ pad_mode (str, optional): Padding mode during data pipeline.
+ Default: 'default'.
+ dtype (torch.dtype, optional): Dtype of edge indices tensor.
+ Default: np.float32.
+ device (str, optional): Device of edge indices tensor.
+ Default: 'cpu'.
+
+ Returns:
+ list[Tensor]: Edge indices for each image in batch data.
+ """
+ edge_indices_list = []
+ for i in range(len(img_metas)):
+ img_shape = img_metas[i]['img_shape']
+ pad_shape = img_metas[i]['pad_shape']
+ h, w = img_shape[:2]
+ pad_h, pad_w = pad_shape
+ edge_indices = []
+
+ if pad_mode == 'default':
+ x_min = 0
+ y_min = 0
+ x_max = (w - 1) // downsample_ratio
+ y_max = (h - 1) // downsample_ratio
+ elif pad_mode == 'center':
+ x_min = np.ceil((pad_w - w) / 2 * downsample_ratio)
+ y_min = np.ceil((pad_h - h) / 2 * downsample_ratio)
+ x_max = x_min + w // downsample_ratio
+ y_max = y_min + h // downsample_ratio
+ else:
+ raise NotImplementedError
+
+ # left
+ y = np.arange(y_min, y_max, step, dtype=dtype)
+ x = np.ones(len(y)) * x_min
+
+ edge_indices_edge = np.stack((x, y), axis=1)
+ edge_indices.append(edge_indices_edge)
+
+ # bottom
+ x = np.arange(x_min, x_max, step, dtype=dtype)
+ y = np.ones(len(x)) * y_max
+
+ edge_indices_edge = np.stack((x, y), axis=1)
+ edge_indices.append(edge_indices_edge)
+
+ # right
+ y = np.arange(y_max, y_min, -step, dtype=dtype)
+ x = np.ones(len(y)) * x_max
+
+ edge_indices_edge = np.stack((x, y), axis=1)
+ edge_indices.append(edge_indices_edge)
+
+ # top
+ x = np.arange(x_max, x_min, -step, dtype=dtype)
+ y = np.ones(len(x)) * y_min
+
+ edge_indices_edge = np.stack((x, y), axis=1)
+ edge_indices.append(edge_indices_edge)
+
+ edge_indices = \
+ np.concatenate([index for index in edge_indices], axis=0)
+ edge_indices = torch.from_numpy(edge_indices).to(device).long()
+ edge_indices_list.append(edge_indices)
+
+ return edge_indices_list
diff --git a/mmdet3d/models/utils/gen_keypoints.py b/mmdet3d/models/utils/gen_keypoints.py
new file mode 100644
index 0000000..8c7909b
--- /dev/null
+++ b/mmdet3d/models/utils/gen_keypoints.py
@@ -0,0 +1,80 @@
+# Copyright (c) OpenMMLab. All rights reserved.
+import torch
+
+from mmdet3d.core.bbox import points_cam2img
+
+
+def get_keypoints(gt_bboxes_3d_list,
+ centers2d_list,
+ img_metas,
+ use_local_coords=True):
+ """Function to filter the objects label outside the image.
+
+ Args:
+ gt_bboxes_list (list[Tensor]): Ground truth bboxes of each image,
+ shape (num_gt, 4).
+ centers2d_list (list[Tensor]): Projected 3D centers onto 2D image,
+ shape (num_gt, 2).
+ img_metas (list[dict]): Meta information of each image, e.g.,
+ image size, scaling factor, etc.
+ use_local_coords (bool, optional): Wheher to use local coordinates
+ for keypoints. Default: True.
+
+ Returns:
+ tuple[list[Tensor]]: It contains two elements, the first is the
+ keypoints for each projected 2D bbox in batch data. The second is
+ the visible mask of depth calculated by keypoints.
+ """
+
+ assert len(gt_bboxes_3d_list) == len(centers2d_list)
+ bs = len(gt_bboxes_3d_list)
+ keypoints2d_list = []
+ keypoints_depth_mask_list = []
+
+ for i in range(bs):
+ gt_bboxes_3d = gt_bboxes_3d_list[i]
+ centers2d = centers2d_list[i]
+ img_shape = img_metas[i]['img_shape']
+ cam2img = img_metas[i]['cam2img']
+ h, w = img_shape[:2]
+ # (N, 8, 3)
+ corners3d = gt_bboxes_3d.corners
+ top_centers3d = torch.mean(corners3d[:, [0, 1, 4, 5], :], dim=1)
+ bot_centers3d = torch.mean(corners3d[:, [2, 3, 6, 7], :], dim=1)
+ # (N, 2, 3)
+ top_bot_centers3d = torch.stack((top_centers3d, bot_centers3d), dim=1)
+ keypoints3d = torch.cat((corners3d, top_bot_centers3d), dim=1)
+ # (N, 10, 2)
+ keypoints2d = points_cam2img(keypoints3d, cam2img)
+
+ # keypoints mask: keypoints must be inside
+ # the image and in front of the camera
+ keypoints_x_visible = (keypoints2d[..., 0] >= 0) & (
+ keypoints2d[..., 0] <= w - 1)
+ keypoints_y_visible = (keypoints2d[..., 1] >= 0) & (
+ keypoints2d[..., 1] <= h - 1)
+ keypoints_z_visible = (keypoints3d[..., -1] > 0)
+
+ # (N, 1O)
+ keypoints_visible = keypoints_x_visible & \
+ keypoints_y_visible & keypoints_z_visible
+ # center, diag-02, diag-13
+ keypoints_depth_valid = torch.stack(
+ (keypoints_visible[:, [8, 9]].all(dim=1),
+ keypoints_visible[:, [0, 3, 5, 6]].all(dim=1),
+ keypoints_visible[:, [1, 2, 4, 7]].all(dim=1)),
+ dim=1)
+ keypoints_visible = keypoints_visible.float()
+
+ if use_local_coords:
+ keypoints2d = torch.cat((keypoints2d - centers2d.unsqueeze(1),
+ keypoints_visible.unsqueeze(-1)),
+ dim=2)
+ else:
+ keypoints2d = torch.cat(
+ (keypoints2d, keypoints_visible.unsqueeze(-1)), dim=2)
+
+ keypoints2d_list.append(keypoints2d)
+ keypoints_depth_mask_list.append(keypoints_depth_valid)
+
+ return (keypoints2d_list, keypoints_depth_mask_list)
diff --git a/mmdet3d/models/utils/handle_objs.py b/mmdet3d/models/utils/handle_objs.py
new file mode 100644
index 0000000..25fd793
--- /dev/null
+++ b/mmdet3d/models/utils/handle_objs.py
@@ -0,0 +1,135 @@
+# Copyright (c) OpenMMLab. All rights reserved.
+import torch
+
+
+def filter_outside_objs(gt_bboxes_list, gt_labels_list, gt_bboxes_3d_list,
+ gt_labels_3d_list, centers2d_list, img_metas):
+ """Function to filter the objects label outside the image.
+
+ Args:
+ gt_bboxes_list (list[Tensor]): Ground truth bboxes of each image,
+ each has shape (num_gt, 4).
+ gt_labels_list (list[Tensor]): Ground truth labels of each box,
+ each has shape (num_gt,).
+ gt_bboxes_3d_list (list[Tensor]): 3D Ground truth bboxes of each
+ image, each has shape (num_gt, bbox_code_size).
+ gt_labels_3d_list (list[Tensor]): 3D Ground truth labels of each
+ box, each has shape (num_gt,).
+ centers2d_list (list[Tensor]): Projected 3D centers onto 2D image,
+ each has shape (num_gt, 2).
+ img_metas (list[dict]): Meta information of each image, e.g.,
+ image size, scaling factor, etc.
+ """
+ bs = len(centers2d_list)
+
+ for i in range(bs):
+ centers2d = centers2d_list[i].clone()
+ img_shape = img_metas[i]['img_shape']
+ keep_inds = (centers2d[:, 0] > 0) & \
+ (centers2d[:, 0] < img_shape[1]) & \
+ (centers2d[:, 1] > 0) & \
+ (centers2d[:, 1] < img_shape[0])
+ centers2d_list[i] = centers2d[keep_inds]
+ gt_labels_list[i] = gt_labels_list[i][keep_inds]
+ gt_bboxes_list[i] = gt_bboxes_list[i][keep_inds]
+ gt_bboxes_3d_list[i].tensor = gt_bboxes_3d_list[i].tensor[keep_inds]
+ gt_labels_3d_list[i] = gt_labels_3d_list[i][keep_inds]
+
+
+def get_centers2d_target(centers2d, centers, img_shape):
+ """Function to get target centers2d.
+
+ Args:
+ centers2d (Tensor): Projected 3D centers onto 2D images.
+ centers (Tensor): Centers of 2d gt bboxes.
+ img_shape (tuple): Resized image shape.
+
+ Returns:
+ torch.Tensor: Projected 3D centers (centers2D) target.
+ """
+ N = centers2d.shape[0]
+ h, w = img_shape[:2]
+ valid_intersects = centers2d.new_zeros((N, 2))
+ a = (centers[:, 1] - centers2d[:, 1]) / (centers[:, 0] - centers2d[:, 0])
+ b = centers[:, 1] - a * centers[:, 0]
+ left_y = b
+ right_y = (w - 1) * a + b
+ top_x = -b / a
+ bottom_x = (h - 1 - b) / a
+
+ left_coors = torch.stack((left_y.new_zeros(N, ), left_y), dim=1)
+ right_coors = torch.stack((right_y.new_full((N, ), w - 1), right_y), dim=1)
+ top_coors = torch.stack((top_x, top_x.new_zeros(N, )), dim=1)
+ bottom_coors = torch.stack((bottom_x, bottom_x.new_full((N, ), h - 1)),
+ dim=1)
+
+ intersects = torch.stack(
+ [left_coors, right_coors, top_coors, bottom_coors], dim=1)
+ intersects_x = intersects[:, :, 0]
+ intersects_y = intersects[:, :, 1]
+ inds = (intersects_x >= 0) & (intersects_x <=
+ w - 1) & (intersects_y >= 0) & (
+ intersects_y <= h - 1)
+ valid_intersects = intersects[inds].reshape(N, 2, 2)
+ dist = torch.norm(valid_intersects - centers2d.unsqueeze(1), dim=2)
+ min_idx = torch.argmin(dist, dim=1)
+
+ min_idx = min_idx.unsqueeze(-1).unsqueeze(-1).expand(-1, -1, 2)
+ centers2d_target = valid_intersects.gather(dim=1, index=min_idx).squeeze(1)
+
+ return centers2d_target
+
+
+def handle_proj_objs(centers2d_list, gt_bboxes_list, img_metas):
+ """Function to handle projected object centers2d, generate target
+ centers2d.
+
+ Args:
+ gt_bboxes_list (list[Tensor]): Ground truth bboxes of each image,
+ shape (num_gt, 4).
+ centers2d_list (list[Tensor]): Projected 3D centers onto 2D image,
+ shape (num_gt, 2).
+ img_metas (list[dict]): Meta information of each image, e.g.,
+ image size, scaling factor, etc.
+
+ Returns:
+ tuple[list[Tensor]]: It contains three elements. The first is the
+ target centers2d after handling the truncated objects. The second
+ is the offsets between target centers2d and round int dtype
+ centers2d,and the last is the truncation mask for each object in
+ batch data.
+ """
+ bs = len(centers2d_list)
+ centers2d_target_list = []
+ trunc_mask_list = []
+ offsets2d_list = []
+ # for now, only pad mode that img is padded by right and
+ # bottom side is supported.
+ for i in range(bs):
+ centers2d = centers2d_list[i]
+ gt_bbox = gt_bboxes_list[i]
+ img_shape = img_metas[i]['img_shape']
+ centers2d_target = centers2d.clone()
+ inside_inds = (centers2d[:, 0] > 0) & \
+ (centers2d[:, 0] < img_shape[1]) & \
+ (centers2d[:, 1] > 0) & \
+ (centers2d[:, 1] < img_shape[0])
+ outside_inds = ~inside_inds
+
+ # if there are outside objects
+ if outside_inds.any():
+ centers = (gt_bbox[:, :2] + gt_bbox[:, 2:]) / 2
+ outside_centers2d = centers2d[outside_inds]
+ match_centers = centers[outside_inds]
+ target_outside_centers2d = get_centers2d_target(
+ outside_centers2d, match_centers, img_shape)
+ centers2d_target[outside_inds] = target_outside_centers2d
+
+ offsets2d = centers2d - centers2d_target.round().int()
+ trunc_mask = outside_inds
+
+ centers2d_target_list.append(centers2d_target)
+ trunc_mask_list.append(trunc_mask)
+ offsets2d_list.append(offsets2d)
+
+ return (centers2d_target_list, offsets2d_list, trunc_mask_list)
diff --git a/mmdet3d/models/utils/mlp.py b/mmdet3d/models/utils/mlp.py
new file mode 100644
index 0000000..0b499bb
--- /dev/null
+++ b/mmdet3d/models/utils/mlp.py
@@ -0,0 +1,51 @@
+# Copyright (c) OpenMMLab. All rights reserved.
+from mmcv.cnn import ConvModule
+from mmcv.runner import BaseModule
+from torch import nn as nn
+
+
+class MLP(BaseModule):
+ """A simple MLP module.
+
+ Pass features (B, C, N) through an MLP.
+
+ Args:
+ in_channels (int, optional): Number of channels of input features.
+ Default: 18.
+ conv_channels (tuple[int], optional): Out channels of the convolution.
+ Default: (256, 256).
+ conv_cfg (dict, optional): Config of convolution.
+ Default: dict(type='Conv1d').
+ norm_cfg (dict, optional): Config of normalization.
+ Default: dict(type='BN1d').
+ act_cfg (dict, optional): Config of activation.
+ Default: dict(type='ReLU').
+ """
+
+ def __init__(self,
+ in_channel=18,
+ conv_channels=(256, 256),
+ conv_cfg=dict(type='Conv1d'),
+ norm_cfg=dict(type='BN1d'),
+ act_cfg=dict(type='ReLU'),
+ init_cfg=None):
+ super().__init__(init_cfg=init_cfg)
+ self.mlp = nn.Sequential()
+ prev_channels = in_channel
+ for i, conv_channel in enumerate(conv_channels):
+ self.mlp.add_module(
+ f'layer{i}',
+ ConvModule(
+ prev_channels,
+ conv_channels[i],
+ 1,
+ padding=0,
+ conv_cfg=conv_cfg,
+ norm_cfg=norm_cfg,
+ act_cfg=act_cfg,
+ bias=True,
+ inplace=True))
+ prev_channels = conv_channels[i]
+
+ def forward(self, img_features):
+ return self.mlp(img_features)
diff --git a/mmdet3d/models/voxel_encoders/__init__.py b/mmdet3d/models/voxel_encoders/__init__.py
new file mode 100644
index 0000000..2926a83
--- /dev/null
+++ b/mmdet3d/models/voxel_encoders/__init__.py
@@ -0,0 +1,8 @@
+# Copyright (c) OpenMMLab. All rights reserved.
+from .pillar_encoder import DynamicPillarFeatureNet, PillarFeatureNet
+from .voxel_encoder import DynamicSimpleVFE, DynamicVFE, HardSimpleVFE, HardVFE
+
+__all__ = [
+ 'PillarFeatureNet', 'DynamicPillarFeatureNet', 'HardVFE', 'DynamicVFE',
+ 'HardSimpleVFE', 'DynamicSimpleVFE'
+]
diff --git a/mmdet3d/models/voxel_encoders/pillar_encoder.py b/mmdet3d/models/voxel_encoders/pillar_encoder.py
new file mode 100644
index 0000000..39bdc72
--- /dev/null
+++ b/mmdet3d/models/voxel_encoders/pillar_encoder.py
@@ -0,0 +1,323 @@
+# Copyright (c) OpenMMLab. All rights reserved.
+import torch
+from mmcv.cnn import build_norm_layer
+from mmcv.ops import DynamicScatter
+from mmcv.runner import force_fp32
+from torch import nn
+
+from ..builder import VOXEL_ENCODERS
+from .utils import PFNLayer, get_paddings_indicator
+
+
+@VOXEL_ENCODERS.register_module()
+class PillarFeatureNet(nn.Module):
+ """Pillar Feature Net.
+
+ The network prepares the pillar features and performs forward pass
+ through PFNLayers.
+
+ Args:
+ in_channels (int, optional): Number of input features,
+ either x, y, z or x, y, z, r. Defaults to 4.
+ feat_channels (tuple, optional): Number of features in each of the
+ N PFNLayers. Defaults to (64, ).
+ with_distance (bool, optional): Whether to include Euclidean distance
+ to points. Defaults to False.
+ with_cluster_center (bool, optional): [description]. Defaults to True.
+ with_voxel_center (bool, optional): [description]. Defaults to True.
+ voxel_size (tuple[float], optional): Size of voxels, only utilize x
+ and y size. Defaults to (0.2, 0.2, 4).
+ point_cloud_range (tuple[float], optional): Point cloud range, only
+ utilizes x and y min. Defaults to (0, -40, -3, 70.4, 40, 1).
+ norm_cfg ([type], optional): [description].
+ Defaults to dict(type='BN1d', eps=1e-3, momentum=0.01).
+ mode (str, optional): The mode to gather point features. Options are
+ 'max' or 'avg'. Defaults to 'max'.
+ legacy (bool, optional): Whether to use the new behavior or
+ the original behavior. Defaults to True.
+ """
+
+ def __init__(self,
+ in_channels=4,
+ feat_channels=(64, ),
+ with_distance=False,
+ with_cluster_center=True,
+ with_voxel_center=True,
+ voxel_size=(0.2, 0.2, 4),
+ point_cloud_range=(0, -40, -3, 70.4, 40, 1),
+ norm_cfg=dict(type='BN1d', eps=1e-3, momentum=0.01),
+ mode='max',
+ legacy=True):
+ super(PillarFeatureNet, self).__init__()
+ assert len(feat_channels) > 0
+ self.legacy = legacy
+ if with_cluster_center:
+ in_channels += 3
+ if with_voxel_center:
+ in_channels += 3
+ if with_distance:
+ in_channels += 1
+ self._with_distance = with_distance
+ self._with_cluster_center = with_cluster_center
+ self._with_voxel_center = with_voxel_center
+ self.fp16_enabled = False
+ # Create PillarFeatureNet layers
+ self.in_channels = in_channels
+ feat_channels = [in_channels] + list(feat_channels)
+ pfn_layers = []
+ for i in range(len(feat_channels) - 1):
+ in_filters = feat_channels[i]
+ out_filters = feat_channels[i + 1]
+ if i < len(feat_channels) - 2:
+ last_layer = False
+ else:
+ last_layer = True
+ pfn_layers.append(
+ PFNLayer(
+ in_filters,
+ out_filters,
+ norm_cfg=norm_cfg,
+ last_layer=last_layer,
+ mode=mode))
+ self.pfn_layers = nn.ModuleList(pfn_layers)
+
+ # Need pillar (voxel) size and x/y offset in order to calculate offset
+ self.vx = voxel_size[0]
+ self.vy = voxel_size[1]
+ self.vz = voxel_size[2]
+ self.x_offset = self.vx / 2 + point_cloud_range[0]
+ self.y_offset = self.vy / 2 + point_cloud_range[1]
+ self.z_offset = self.vz / 2 + point_cloud_range[2]
+ self.point_cloud_range = point_cloud_range
+
+ @force_fp32(out_fp16=True)
+ def forward(self, features, num_points, coors):
+ """Forward function.
+
+ Args:
+ features (torch.Tensor): Point features or raw points in shape
+ (N, M, C).
+ num_points (torch.Tensor): Number of points in each pillar.
+ coors (torch.Tensor): Coordinates of each voxel.
+
+ Returns:
+ torch.Tensor: Features of pillars.
+ """
+ features_ls = [features]
+ # Find distance of x, y, and z from cluster center
+ if self._with_cluster_center:
+ points_mean = features[:, :, :3].sum(
+ dim=1, keepdim=True) / num_points.type_as(features).view(
+ -1, 1, 1)
+ f_cluster = features[:, :, :3] - points_mean
+ features_ls.append(f_cluster)
+
+ # Find distance of x, y, and z from pillar center
+ dtype = features.dtype
+ if self._with_voxel_center:
+ if not self.legacy:
+ f_center = torch.zeros_like(features[:, :, :3])
+ f_center[:, :, 0] = features[:, :, 0] - (
+ coors[:, 3].to(dtype).unsqueeze(1) * self.vx +
+ self.x_offset)
+ f_center[:, :, 1] = features[:, :, 1] - (
+ coors[:, 2].to(dtype).unsqueeze(1) * self.vy +
+ self.y_offset)
+ f_center[:, :, 2] = features[:, :, 2] - (
+ coors[:, 1].to(dtype).unsqueeze(1) * self.vz +
+ self.z_offset)
+ else:
+ f_center = features[:, :, :3]
+ f_center[:, :, 0] = f_center[:, :, 0] - (
+ coors[:, 3].type_as(features).unsqueeze(1) * self.vx +
+ self.x_offset)
+ f_center[:, :, 1] = f_center[:, :, 1] - (
+ coors[:, 2].type_as(features).unsqueeze(1) * self.vy +
+ self.y_offset)
+ f_center[:, :, 2] = f_center[:, :, 2] - (
+ coors[:, 1].type_as(features).unsqueeze(1) * self.vz +
+ self.z_offset)
+ features_ls.append(f_center)
+
+ if self._with_distance:
+ points_dist = torch.norm(features[:, :, :3], 2, 2, keepdim=True)
+ features_ls.append(points_dist)
+
+ # Combine together feature decorations
+ features = torch.cat(features_ls, dim=-1)
+ # The feature decorations were calculated without regard to whether
+ # pillar was empty. Need to ensure that
+ # empty pillars remain set to zeros.
+ voxel_count = features.shape[1]
+ mask = get_paddings_indicator(num_points, voxel_count, axis=0)
+ mask = torch.unsqueeze(mask, -1).type_as(features)
+ features *= mask
+
+ for pfn in self.pfn_layers:
+ features = pfn(features, num_points)
+
+ return features.squeeze(1)
+
+
+@VOXEL_ENCODERS.register_module()
+class DynamicPillarFeatureNet(PillarFeatureNet):
+ """Pillar Feature Net using dynamic voxelization.
+
+ The network prepares the pillar features and performs forward pass
+ through PFNLayers. The main difference is that it is used for
+ dynamic voxels, which contains different number of points inside a voxel
+ without limits.
+
+ Args:
+ in_channels (int, optional): Number of input features,
+ either x, y, z or x, y, z, r. Defaults to 4.
+ feat_channels (tuple, optional): Number of features in each of the
+ N PFNLayers. Defaults to (64, ).
+ with_distance (bool, optional): Whether to include Euclidean distance
+ to points. Defaults to False.
+ with_cluster_center (bool, optional): [description]. Defaults to True.
+ with_voxel_center (bool, optional): [description]. Defaults to True.
+ voxel_size (tuple[float], optional): Size of voxels, only utilize x
+ and y size. Defaults to (0.2, 0.2, 4).
+ point_cloud_range (tuple[float], optional): Point cloud range, only
+ utilizes x and y min. Defaults to (0, -40, -3, 70.4, 40, 1).
+ norm_cfg ([type], optional): [description].
+ Defaults to dict(type='BN1d', eps=1e-3, momentum=0.01).
+ mode (str, optional): The mode to gather point features. Options are
+ 'max' or 'avg'. Defaults to 'max'.
+ legacy (bool, optional): Whether to use the new behavior or
+ the original behavior. Defaults to True.
+ """
+
+ def __init__(self,
+ in_channels=4,
+ feat_channels=(64, ),
+ with_distance=False,
+ with_cluster_center=True,
+ with_voxel_center=True,
+ voxel_size=(0.2, 0.2, 4),
+ point_cloud_range=(0, -40, -3, 70.4, 40, 1),
+ norm_cfg=dict(type='BN1d', eps=1e-3, momentum=0.01),
+ mode='max',
+ legacy=True):
+ super(DynamicPillarFeatureNet, self).__init__(
+ in_channels,
+ feat_channels,
+ with_distance,
+ with_cluster_center=with_cluster_center,
+ with_voxel_center=with_voxel_center,
+ voxel_size=voxel_size,
+ point_cloud_range=point_cloud_range,
+ norm_cfg=norm_cfg,
+ mode=mode,
+ legacy=legacy)
+ self.fp16_enabled = False
+ feat_channels = [self.in_channels] + list(feat_channels)
+ pfn_layers = []
+ # TODO: currently only support one PFNLayer
+
+ for i in range(len(feat_channels) - 1):
+ in_filters = feat_channels[i]
+ out_filters = feat_channels[i + 1]
+ if i > 0:
+ in_filters *= 2
+ norm_name, norm_layer = build_norm_layer(norm_cfg, out_filters)
+ pfn_layers.append(
+ nn.Sequential(
+ nn.Linear(in_filters, out_filters, bias=False), norm_layer,
+ nn.ReLU(inplace=True)))
+ self.num_pfn = len(pfn_layers)
+ self.pfn_layers = nn.ModuleList(pfn_layers)
+ self.pfn_scatter = DynamicScatter(voxel_size, point_cloud_range,
+ (mode != 'max'))
+ self.cluster_scatter = DynamicScatter(
+ voxel_size, point_cloud_range, average_points=True)
+
+ def map_voxel_center_to_point(self, pts_coors, voxel_mean, voxel_coors):
+ """Map the centers of voxels to its corresponding points.
+
+ Args:
+ pts_coors (torch.Tensor): The coordinates of each points, shape
+ (M, 3), where M is the number of points.
+ voxel_mean (torch.Tensor): The mean or aggregated features of a
+ voxel, shape (N, C), where N is the number of voxels.
+ voxel_coors (torch.Tensor): The coordinates of each voxel.
+
+ Returns:
+ torch.Tensor: Corresponding voxel centers of each points, shape
+ (M, C), where M is the number of points.
+ """
+ # Step 1: scatter voxel into canvas
+ # Calculate necessary things for canvas creation
+ canvas_y = int(
+ (self.point_cloud_range[4] - self.point_cloud_range[1]) / self.vy)
+ canvas_x = int(
+ (self.point_cloud_range[3] - self.point_cloud_range[0]) / self.vx)
+ canvas_channel = voxel_mean.size(1)
+ batch_size = pts_coors[-1, 0] + 1
+ canvas_len = canvas_y * canvas_x * batch_size
+ # Create the canvas for this sample
+ canvas = voxel_mean.new_zeros(canvas_channel, canvas_len)
+ # Only include non-empty pillars
+ indices = (
+ voxel_coors[:, 0] * canvas_y * canvas_x +
+ voxel_coors[:, 2] * canvas_x + voxel_coors[:, 3])
+ # Scatter the blob back to the canvas
+ canvas[:, indices.long()] = voxel_mean.t()
+
+ # Step 2: get voxel mean for each point
+ voxel_index = (
+ pts_coors[:, 0] * canvas_y * canvas_x +
+ pts_coors[:, 2] * canvas_x + pts_coors[:, 3])
+ center_per_point = canvas[:, voxel_index.long()].t()
+ return center_per_point
+
+ @force_fp32(out_fp16=True)
+ def forward(self, features, coors):
+ """Forward function.
+
+ Args:
+ features (torch.Tensor): Point features or raw points in shape
+ (N, M, C).
+ coors (torch.Tensor): Coordinates of each voxel
+
+ Returns:
+ torch.Tensor: Features of pillars.
+ """
+ features_ls = [features]
+ # Find distance of x, y, and z from cluster center
+ if self._with_cluster_center:
+ voxel_mean, mean_coors = self.cluster_scatter(features, coors)
+ points_mean = self.map_voxel_center_to_point(
+ coors, voxel_mean, mean_coors)
+ # TODO: maybe also do cluster for reflectivity
+ f_cluster = features[:, :3] - points_mean[:, :3]
+ features_ls.append(f_cluster)
+
+ # Find distance of x, y, and z from pillar center
+ if self._with_voxel_center:
+ f_center = features.new_zeros(size=(features.size(0), 3))
+ f_center[:, 0] = features[:, 0] - (
+ coors[:, 3].type_as(features) * self.vx + self.x_offset)
+ f_center[:, 1] = features[:, 1] - (
+ coors[:, 2].type_as(features) * self.vy + self.y_offset)
+ f_center[:, 2] = features[:, 2] - (
+ coors[:, 1].type_as(features) * self.vz + self.z_offset)
+ features_ls.append(f_center)
+
+ if self._with_distance:
+ points_dist = torch.norm(features[:, :3], 2, 1, keepdim=True)
+ features_ls.append(points_dist)
+
+ # Combine together feature decorations
+ features = torch.cat(features_ls, dim=-1)
+ for i, pfn in enumerate(self.pfn_layers):
+ point_feats = pfn(features)
+ voxel_feats, voxel_coors = self.pfn_scatter(point_feats, coors)
+ if i != len(self.pfn_layers) - 1:
+ # need to concat voxel feats if it is not the last pfn
+ feat_per_point = self.map_voxel_center_to_point(
+ coors, voxel_feats, voxel_coors)
+ features = torch.cat([point_feats, feat_per_point], dim=1)
+
+ return voxel_feats, voxel_coors
diff --git a/mmdet3d/models/voxel_encoders/utils.py b/mmdet3d/models/voxel_encoders/utils.py
new file mode 100644
index 0000000..8c54fc2
--- /dev/null
+++ b/mmdet3d/models/voxel_encoders/utils.py
@@ -0,0 +1,182 @@
+# Copyright (c) OpenMMLab. All rights reserved.
+import torch
+from mmcv.cnn import build_norm_layer
+from mmcv.runner import auto_fp16
+from torch import nn
+from torch.nn import functional as F
+
+
+def get_paddings_indicator(actual_num, max_num, axis=0):
+ """Create boolean mask by actually number of a padded tensor.
+
+ Args:
+ actual_num (torch.Tensor): Actual number of points in each voxel.
+ max_num (int): Max number of points in each voxel
+
+ Returns:
+ torch.Tensor: Mask indicates which points are valid inside a voxel.
+ """
+ actual_num = torch.unsqueeze(actual_num, axis + 1)
+ # tiled_actual_num: [N, M, 1]
+ max_num_shape = [1] * len(actual_num.shape)
+ max_num_shape[axis + 1] = -1
+ max_num = torch.arange(
+ max_num, dtype=torch.int, device=actual_num.device).view(max_num_shape)
+ # tiled_actual_num: [[3,3,3,3,3], [4,4,4,4,4], [2,2,2,2,2]]
+ # tiled_max_num: [[0,1,2,3,4], [0,1,2,3,4], [0,1,2,3,4]]
+ paddings_indicator = actual_num.int() > max_num
+ # paddings_indicator shape: [batch_size, max_num]
+ return paddings_indicator
+
+
+class VFELayer(nn.Module):
+ """Voxel Feature Encoder layer.
+
+ The voxel encoder is composed of a series of these layers.
+ This module do not support average pooling and only support to use
+ max pooling to gather features inside a VFE.
+
+ Args:
+ in_channels (int): Number of input channels.
+ out_channels (int): Number of output channels.
+ norm_cfg (dict): Config dict of normalization layers
+ max_out (bool): Whether aggregate the features of points inside
+ each voxel and only return voxel features.
+ cat_max (bool): Whether concatenate the aggregated features
+ and pointwise features.
+ """
+
+ def __init__(self,
+ in_channels,
+ out_channels,
+ norm_cfg=dict(type='BN1d', eps=1e-3, momentum=0.01),
+ max_out=True,
+ cat_max=True):
+ super(VFELayer, self).__init__()
+ self.fp16_enabled = False
+ self.cat_max = cat_max
+ self.max_out = max_out
+ # self.units = int(out_channels / 2)
+
+ self.norm = build_norm_layer(norm_cfg, out_channels)[1]
+ self.linear = nn.Linear(in_channels, out_channels, bias=False)
+
+ @auto_fp16(apply_to=('inputs'), out_fp32=True)
+ def forward(self, inputs):
+ """Forward function.
+
+ Args:
+ inputs (torch.Tensor): Voxels features of shape (N, M, C).
+ N is the number of voxels, M is the number of points in
+ voxels, C is the number of channels of point features.
+
+ Returns:
+ torch.Tensor: Voxel features. There are three mode under which the
+ features have different meaning.
+ - `max_out=False`: Return point-wise features in
+ shape (N, M, C).
+ - `max_out=True` and `cat_max=False`: Return aggregated
+ voxel features in shape (N, C)
+ - `max_out=True` and `cat_max=True`: Return concatenated
+ point-wise features in shape (N, M, C).
+ """
+ # [K, T, 7] tensordot [7, units] = [K, T, units]
+ voxel_count = inputs.shape[1]
+
+ x = self.linear(inputs)
+ x = self.norm(x.permute(0, 2, 1).contiguous()).permute(0, 2,
+ 1).contiguous()
+ pointwise = F.relu(x)
+ # [K, T, units]
+ if self.max_out:
+ aggregated = torch.max(pointwise, dim=1, keepdim=True)[0]
+ else:
+ # this is for fusion layer
+ return pointwise
+
+ if not self.cat_max:
+ return aggregated.squeeze(1)
+ else:
+ # [K, 1, units]
+ repeated = aggregated.repeat(1, voxel_count, 1)
+ concatenated = torch.cat([pointwise, repeated], dim=2)
+ # [K, T, 2 * units]
+ return concatenated
+
+
+class PFNLayer(nn.Module):
+ """Pillar Feature Net Layer.
+
+ The Pillar Feature Net is composed of a series of these layers, but the
+ PointPillars paper results only used a single PFNLayer.
+
+ Args:
+ in_channels (int): Number of input channels.
+ out_channels (int): Number of output channels.
+ norm_cfg (dict, optional): Config dict of normalization layers.
+ Defaults to dict(type='BN1d', eps=1e-3, momentum=0.01).
+ last_layer (bool, optional): If last_layer, there is no
+ concatenation of features. Defaults to False.
+ mode (str, optional): Pooling model to gather features inside voxels.
+ Defaults to 'max'.
+ """
+
+ def __init__(self,
+ in_channels,
+ out_channels,
+ norm_cfg=dict(type='BN1d', eps=1e-3, momentum=0.01),
+ last_layer=False,
+ mode='max'):
+
+ super().__init__()
+ self.fp16_enabled = False
+ self.name = 'PFNLayer'
+ self.last_vfe = last_layer
+ if not self.last_vfe:
+ out_channels = out_channels // 2
+ self.units = out_channels
+
+ self.norm = build_norm_layer(norm_cfg, self.units)[1]
+ self.linear = nn.Linear(in_channels, self.units, bias=False)
+
+ assert mode in ['max', 'avg']
+ self.mode = mode
+
+ @auto_fp16(apply_to=('inputs'), out_fp32=True)
+ def forward(self, inputs, num_voxels=None, aligned_distance=None):
+ """Forward function.
+
+ Args:
+ inputs (torch.Tensor): Pillar/Voxel inputs with shape (N, M, C).
+ N is the number of voxels, M is the number of points in
+ voxels, C is the number of channels of point features.
+ num_voxels (torch.Tensor, optional): Number of points in each
+ voxel. Defaults to None.
+ aligned_distance (torch.Tensor, optional): The distance of
+ each points to the voxel center. Defaults to None.
+
+ Returns:
+ torch.Tensor: Features of Pillars.
+ """
+ x = self.linear(inputs)
+ x = self.norm(x.permute(0, 2, 1).contiguous()).permute(0, 2,
+ 1).contiguous()
+ x = F.relu(x)
+
+ if self.mode == 'max':
+ if aligned_distance is not None:
+ x = x.mul(aligned_distance.unsqueeze(-1))
+ x_max = torch.max(x, dim=1, keepdim=True)[0]
+ elif self.mode == 'avg':
+ if aligned_distance is not None:
+ x = x.mul(aligned_distance.unsqueeze(-1))
+ x_max = x.sum(
+ dim=1, keepdim=True) / num_voxels.type_as(inputs).view(
+ -1, 1, 1)
+
+ if self.last_vfe:
+ return x_max
+ else:
+ x_repeat = x_max.repeat(1, inputs.shape[1], 1)
+ x_concatenated = torch.cat([x, x_repeat], dim=2)
+ return x_concatenated
diff --git a/mmdet3d/models/voxel_encoders/voxel_encoder.py b/mmdet3d/models/voxel_encoders/voxel_encoder.py
new file mode 100644
index 0000000..9f3cf53
--- /dev/null
+++ b/mmdet3d/models/voxel_encoders/voxel_encoder.py
@@ -0,0 +1,489 @@
+# Copyright (c) OpenMMLab. All rights reserved.
+import torch
+from mmcv.cnn import build_norm_layer
+from mmcv.ops import DynamicScatter
+from mmcv.runner import force_fp32
+from torch import nn
+
+from .. import builder
+from ..builder import VOXEL_ENCODERS
+from .utils import VFELayer, get_paddings_indicator
+
+
+@VOXEL_ENCODERS.register_module()
+class HardSimpleVFE(nn.Module):
+ """Simple voxel feature encoder used in SECOND.
+
+ It simply averages the values of points in a voxel.
+
+ Args:
+ num_features (int, optional): Number of features to use. Default: 4.
+ """
+
+ def __init__(self, num_features=4):
+ super(HardSimpleVFE, self).__init__()
+ self.num_features = num_features
+ self.fp16_enabled = False
+
+ @force_fp32(out_fp16=True)
+ def forward(self, features, num_points, coors):
+ """Forward function.
+
+ Args:
+ features (torch.Tensor): Point features in shape
+ (N, M, 3(4)). N is the number of voxels and M is the maximum
+ number of points inside a single voxel.
+ num_points (torch.Tensor): Number of points in each voxel,
+ shape (N, ).
+ coors (torch.Tensor): Coordinates of voxels.
+
+ Returns:
+ torch.Tensor: Mean of points inside each voxel in shape (N, 3(4))
+ """
+ points_mean = features[:, :, :self.num_features].sum(
+ dim=1, keepdim=False) / num_points.type_as(features).view(-1, 1)
+ return points_mean.contiguous()
+
+
+@VOXEL_ENCODERS.register_module()
+class DynamicSimpleVFE(nn.Module):
+ """Simple dynamic voxel feature encoder used in DV-SECOND.
+
+ It simply averages the values of points in a voxel.
+ But the number of points in a voxel is dynamic and varies.
+
+ Args:
+ voxel_size (tupe[float]): Size of a single voxel
+ point_cloud_range (tuple[float]): Range of the point cloud and voxels
+ """
+
+ def __init__(self,
+ voxel_size=(0.2, 0.2, 4),
+ point_cloud_range=(0, -40, -3, 70.4, 40, 1)):
+ super(DynamicSimpleVFE, self).__init__()
+ self.scatter = DynamicScatter(voxel_size, point_cloud_range, True)
+ self.fp16_enabled = False
+
+ @torch.no_grad()
+ @force_fp32(out_fp16=True)
+ def forward(self, features, coors):
+ """Forward function.
+
+ Args:
+ features (torch.Tensor): Point features in shape
+ (N, 3(4)). N is the number of points.
+ coors (torch.Tensor): Coordinates of voxels.
+
+ Returns:
+ torch.Tensor: Mean of points inside each voxel in shape (M, 3(4)).
+ M is the number of voxels.
+ """
+ # This function is used from the start of the voxelnet
+ # num_points: [concated_num_points]
+ features, features_coors = self.scatter(features, coors)
+ return features, features_coors
+
+
+@VOXEL_ENCODERS.register_module()
+class DynamicVFE(nn.Module):
+ """Dynamic Voxel feature encoder used in DV-SECOND.
+
+ It encodes features of voxels and their points. It could also fuse
+ image feature into voxel features in a point-wise manner.
+ The number of points inside the voxel varies.
+
+ Args:
+ in_channels (int, optional): Input channels of VFE. Defaults to 4.
+ feat_channels (list(int), optional): Channels of features in VFE.
+ with_distance (bool, optional): Whether to use the L2 distance of
+ points to the origin point. Defaults to False.
+ with_cluster_center (bool, optional): Whether to use the distance
+ to cluster center of points inside a voxel. Defaults to False.
+ with_voxel_center (bool, optional): Whether to use the distance
+ to center of voxel for each points inside a voxel.
+ Defaults to False.
+ voxel_size (tuple[float], optional): Size of a single voxel.
+ Defaults to (0.2, 0.2, 4).
+ point_cloud_range (tuple[float], optional): The range of points
+ or voxels. Defaults to (0, -40, -3, 70.4, 40, 1).
+ norm_cfg (dict, optional): Config dict of normalization layers.
+ mode (str, optional): The mode when pooling features of points
+ inside a voxel. Available options include 'max' and 'avg'.
+ Defaults to 'max'.
+ fusion_layer (dict, optional): The config dict of fusion
+ layer used in multi-modal detectors. Defaults to None.
+ return_point_feats (bool, optional): Whether to return the features
+ of each points. Defaults to False.
+ """
+
+ def __init__(self,
+ in_channels=4,
+ feat_channels=[],
+ with_distance=False,
+ with_cluster_center=False,
+ with_voxel_center=False,
+ voxel_size=(0.2, 0.2, 4),
+ point_cloud_range=(0, -40, -3, 70.4, 40, 1),
+ norm_cfg=dict(type='BN1d', eps=1e-3, momentum=0.01),
+ mode='max',
+ fusion_layer=None,
+ return_point_feats=False):
+ super(DynamicVFE, self).__init__()
+ assert mode in ['avg', 'max']
+ assert len(feat_channels) > 0
+ if with_cluster_center:
+ in_channels += 3
+ if with_voxel_center:
+ in_channels += 3
+ if with_distance:
+ in_channels += 1
+ self.in_channels = in_channels
+ self._with_distance = with_distance
+ self._with_cluster_center = with_cluster_center
+ self._with_voxel_center = with_voxel_center
+ self.return_point_feats = return_point_feats
+ self.fp16_enabled = False
+
+ # Need pillar (voxel) size and x/y offset in order to calculate offset
+ self.vx = voxel_size[0]
+ self.vy = voxel_size[1]
+ self.vz = voxel_size[2]
+ self.x_offset = self.vx / 2 + point_cloud_range[0]
+ self.y_offset = self.vy / 2 + point_cloud_range[1]
+ self.z_offset = self.vz / 2 + point_cloud_range[2]
+ self.point_cloud_range = point_cloud_range
+ self.scatter = DynamicScatter(voxel_size, point_cloud_range, True)
+
+ feat_channels = [self.in_channels] + list(feat_channels)
+ vfe_layers = []
+ for i in range(len(feat_channels) - 1):
+ in_filters = feat_channels[i]
+ out_filters = feat_channels[i + 1]
+ if i > 0:
+ in_filters *= 2
+ norm_name, norm_layer = build_norm_layer(norm_cfg, out_filters)
+ vfe_layers.append(
+ nn.Sequential(
+ nn.Linear(in_filters, out_filters, bias=False), norm_layer,
+ nn.ReLU(inplace=True)))
+ self.vfe_layers = nn.ModuleList(vfe_layers)
+ self.num_vfe = len(vfe_layers)
+ self.vfe_scatter = DynamicScatter(voxel_size, point_cloud_range,
+ (mode != 'max'))
+ self.cluster_scatter = DynamicScatter(
+ voxel_size, point_cloud_range, average_points=True)
+ self.fusion_layer = None
+ if fusion_layer is not None:
+ self.fusion_layer = builder.build_fusion_layer(fusion_layer)
+
+ def map_voxel_center_to_point(self, pts_coors, voxel_mean, voxel_coors):
+ """Map voxel features to its corresponding points.
+
+ Args:
+ pts_coors (torch.Tensor): Voxel coordinate of each point.
+ voxel_mean (torch.Tensor): Voxel features to be mapped.
+ voxel_coors (torch.Tensor): Coordinates of valid voxels
+
+ Returns:
+ torch.Tensor: Features or centers of each point.
+ """
+ # Step 1: scatter voxel into canvas
+ # Calculate necessary things for canvas creation
+ canvas_z = int(
+ (self.point_cloud_range[5] - self.point_cloud_range[2]) / self.vz)
+ canvas_y = int(
+ (self.point_cloud_range[4] - self.point_cloud_range[1]) / self.vy)
+ canvas_x = int(
+ (self.point_cloud_range[3] - self.point_cloud_range[0]) / self.vx)
+ # canvas_channel = voxel_mean.size(1)
+ batch_size = pts_coors[-1, 0] + 1
+ canvas_len = canvas_z * canvas_y * canvas_x * batch_size
+ # Create the canvas for this sample
+ canvas = voxel_mean.new_zeros(canvas_len, dtype=torch.long)
+ # Only include non-empty pillars
+ indices = (
+ voxel_coors[:, 0] * canvas_z * canvas_y * canvas_x +
+ voxel_coors[:, 1] * canvas_y * canvas_x +
+ voxel_coors[:, 2] * canvas_x + voxel_coors[:, 3])
+ # Scatter the blob back to the canvas
+ canvas[indices.long()] = torch.arange(
+ start=0, end=voxel_mean.size(0), device=voxel_mean.device)
+
+ # Step 2: get voxel mean for each point
+ voxel_index = (
+ pts_coors[:, 0] * canvas_z * canvas_y * canvas_x +
+ pts_coors[:, 1] * canvas_y * canvas_x +
+ pts_coors[:, 2] * canvas_x + pts_coors[:, 3])
+ voxel_inds = canvas[voxel_index.long()]
+ center_per_point = voxel_mean[voxel_inds, ...]
+ return center_per_point
+
+ @force_fp32(out_fp16=True)
+ def forward(self,
+ features,
+ coors,
+ points=None,
+ img_feats=None,
+ img_metas=None):
+ """Forward functions.
+
+ Args:
+ features (torch.Tensor): Features of voxels, shape is NxC.
+ coors (torch.Tensor): Coordinates of voxels, shape is Nx(1+NDim).
+ points (list[torch.Tensor], optional): Raw points used to guide the
+ multi-modality fusion. Defaults to None.
+ img_feats (list[torch.Tensor], optional): Image features used for
+ multi-modality fusion. Defaults to None.
+ img_metas (dict, optional): [description]. Defaults to None.
+
+ Returns:
+ tuple: If `return_point_feats` is False, returns voxel features and
+ its coordinates. If `return_point_feats` is True, returns
+ feature of each points inside voxels.
+ """
+ features_ls = [features]
+ # Find distance of x, y, and z from cluster center
+ if self._with_cluster_center:
+ voxel_mean, mean_coors = self.cluster_scatter(features, coors)
+ points_mean = self.map_voxel_center_to_point(
+ coors, voxel_mean, mean_coors)
+ # TODO: maybe also do cluster for reflectivity
+ f_cluster = features[:, :3] - points_mean[:, :3]
+ features_ls.append(f_cluster)
+
+ # Find distance of x, y, and z from pillar center
+ if self._with_voxel_center:
+ f_center = features.new_zeros(size=(features.size(0), 3))
+ f_center[:, 0] = features[:, 0] - (
+ coors[:, 3].type_as(features) * self.vx + self.x_offset)
+ f_center[:, 1] = features[:, 1] - (
+ coors[:, 2].type_as(features) * self.vy + self.y_offset)
+ f_center[:, 2] = features[:, 2] - (
+ coors[:, 1].type_as(features) * self.vz + self.z_offset)
+ features_ls.append(f_center)
+
+ if self._with_distance:
+ points_dist = torch.norm(features[:, :3], 2, 1, keepdim=True)
+ features_ls.append(points_dist)
+
+ # Combine together feature decorations
+ features = torch.cat(features_ls, dim=-1)
+ for i, vfe in enumerate(self.vfe_layers):
+ point_feats = vfe(features)
+ if (i == len(self.vfe_layers) - 1 and self.fusion_layer is not None
+ and img_feats is not None):
+ point_feats = self.fusion_layer(img_feats, points, point_feats,
+ img_metas)
+ voxel_feats, voxel_coors = self.vfe_scatter(point_feats, coors)
+ if i != len(self.vfe_layers) - 1:
+ # need to concat voxel feats if it is not the last vfe
+ feat_per_point = self.map_voxel_center_to_point(
+ coors, voxel_feats, voxel_coors)
+ features = torch.cat([point_feats, feat_per_point], dim=1)
+
+ if self.return_point_feats:
+ return point_feats
+ return voxel_feats, voxel_coors
+
+
+@VOXEL_ENCODERS.register_module()
+class HardVFE(nn.Module):
+ """Voxel feature encoder used in DV-SECOND.
+
+ It encodes features of voxels and their points. It could also fuse
+ image feature into voxel features in a point-wise manner.
+
+ Args:
+ in_channels (int, optional): Input channels of VFE. Defaults to 4.
+ feat_channels (list(int), optional): Channels of features in VFE.
+ with_distance (bool, optional): Whether to use the L2 distance
+ of points to the origin point. Defaults to False.
+ with_cluster_center (bool, optional): Whether to use the distance
+ to cluster center of points inside a voxel. Defaults to False.
+ with_voxel_center (bool, optional): Whether to use the distance to
+ center of voxel for each points inside a voxel. Defaults to False.
+ voxel_size (tuple[float], optional): Size of a single voxel.
+ Defaults to (0.2, 0.2, 4).
+ point_cloud_range (tuple[float], optional): The range of points
+ or voxels. Defaults to (0, -40, -3, 70.4, 40, 1).
+ norm_cfg (dict, optional): Config dict of normalization layers.
+ mode (str, optional): The mode when pooling features of points inside a
+ voxel. Available options include 'max' and 'avg'.
+ Defaults to 'max'.
+ fusion_layer (dict, optional): The config dict of fusion layer
+ used in multi-modal detectors. Defaults to None.
+ return_point_feats (bool, optional): Whether to return the
+ features of each points. Defaults to False.
+ """
+
+ def __init__(self,
+ in_channels=4,
+ feat_channels=[],
+ with_distance=False,
+ with_cluster_center=False,
+ with_voxel_center=False,
+ voxel_size=(0.2, 0.2, 4),
+ point_cloud_range=(0, -40, -3, 70.4, 40, 1),
+ norm_cfg=dict(type='BN1d', eps=1e-3, momentum=0.01),
+ mode='max',
+ fusion_layer=None,
+ return_point_feats=False):
+ super(HardVFE, self).__init__()
+ assert len(feat_channels) > 0
+ if with_cluster_center:
+ in_channels += 3
+ if with_voxel_center:
+ in_channels += 3
+ if with_distance:
+ in_channels += 1
+ self.in_channels = in_channels
+ self._with_distance = with_distance
+ self._with_cluster_center = with_cluster_center
+ self._with_voxel_center = with_voxel_center
+ self.return_point_feats = return_point_feats
+ self.fp16_enabled = False
+
+ # Need pillar (voxel) size and x/y offset to calculate pillar offset
+ self.vx = voxel_size[0]
+ self.vy = voxel_size[1]
+ self.vz = voxel_size[2]
+ self.x_offset = self.vx / 2 + point_cloud_range[0]
+ self.y_offset = self.vy / 2 + point_cloud_range[1]
+ self.z_offset = self.vz / 2 + point_cloud_range[2]
+ self.point_cloud_range = point_cloud_range
+ self.scatter = DynamicScatter(voxel_size, point_cloud_range, True)
+
+ feat_channels = [self.in_channels] + list(feat_channels)
+ vfe_layers = []
+ for i in range(len(feat_channels) - 1):
+ in_filters = feat_channels[i]
+ out_filters = feat_channels[i + 1]
+ if i > 0:
+ in_filters *= 2
+ # TODO: pass norm_cfg to VFE
+ # norm_name, norm_layer = build_norm_layer(norm_cfg, out_filters)
+ if i == (len(feat_channels) - 2):
+ cat_max = False
+ max_out = True
+ if fusion_layer:
+ max_out = False
+ else:
+ max_out = True
+ cat_max = True
+ vfe_layers.append(
+ VFELayer(
+ in_filters,
+ out_filters,
+ norm_cfg=norm_cfg,
+ max_out=max_out,
+ cat_max=cat_max))
+ self.vfe_layers = nn.ModuleList(vfe_layers)
+ self.num_vfe = len(vfe_layers)
+
+ self.fusion_layer = None
+ if fusion_layer is not None:
+ self.fusion_layer = builder.build_fusion_layer(fusion_layer)
+
+ @force_fp32(out_fp16=True)
+ def forward(self,
+ features,
+ num_points,
+ coors,
+ img_feats=None,
+ img_metas=None):
+ """Forward functions.
+
+ Args:
+ features (torch.Tensor): Features of voxels, shape is MxNxC.
+ num_points (torch.Tensor): Number of points in each voxel.
+ coors (torch.Tensor): Coordinates of voxels, shape is Mx(1+NDim).
+ img_feats (list[torch.Tensor], optional): Image features used for
+ multi-modality fusion. Defaults to None.
+ img_metas (dict, optional): [description]. Defaults to None.
+
+ Returns:
+ tuple: If `return_point_feats` is False, returns voxel features and
+ its coordinates. If `return_point_feats` is True, returns
+ feature of each points inside voxels.
+ """
+ features_ls = [features]
+ # Find distance of x, y, and z from cluster center
+ if self._with_cluster_center:
+ points_mean = (
+ features[:, :, :3].sum(dim=1, keepdim=True) /
+ num_points.type_as(features).view(-1, 1, 1))
+ # TODO: maybe also do cluster for reflectivity
+ f_cluster = features[:, :, :3] - points_mean
+ features_ls.append(f_cluster)
+
+ # Find distance of x, y, and z from pillar center
+ if self._with_voxel_center:
+ f_center = features.new_zeros(
+ size=(features.size(0), features.size(1), 3))
+ f_center[:, :, 0] = features[:, :, 0] - (
+ coors[:, 3].type_as(features).unsqueeze(1) * self.vx +
+ self.x_offset)
+ f_center[:, :, 1] = features[:, :, 1] - (
+ coors[:, 2].type_as(features).unsqueeze(1) * self.vy +
+ self.y_offset)
+ f_center[:, :, 2] = features[:, :, 2] - (
+ coors[:, 1].type_as(features).unsqueeze(1) * self.vz +
+ self.z_offset)
+ features_ls.append(f_center)
+
+ if self._with_distance:
+ points_dist = torch.norm(features[:, :, :3], 2, 2, keepdim=True)
+ features_ls.append(points_dist)
+
+ # Combine together feature decorations
+ voxel_feats = torch.cat(features_ls, dim=-1)
+ # The feature decorations were calculated without regard to whether
+ # pillar was empty.
+ # Need to ensure that empty voxels remain set to zeros.
+ voxel_count = voxel_feats.shape[1]
+ mask = get_paddings_indicator(num_points, voxel_count, axis=0)
+ voxel_feats *= mask.unsqueeze(-1).type_as(voxel_feats)
+
+ for i, vfe in enumerate(self.vfe_layers):
+ voxel_feats = vfe(voxel_feats)
+
+ if (self.fusion_layer is not None and img_feats is not None):
+ voxel_feats = self.fusion_with_mask(features, mask, voxel_feats,
+ coors, img_feats, img_metas)
+
+ return voxel_feats
+
+ def fusion_with_mask(self, features, mask, voxel_feats, coors, img_feats,
+ img_metas):
+ """Fuse image and point features with mask.
+
+ Args:
+ features (torch.Tensor): Features of voxel, usually it is the
+ values of points in voxels.
+ mask (torch.Tensor): Mask indicates valid features in each voxel.
+ voxel_feats (torch.Tensor): Features of voxels.
+ coors (torch.Tensor): Coordinates of each single voxel.
+ img_feats (list[torch.Tensor]): Multi-scale feature maps of image.
+ img_metas (list(dict)): Meta information of image and points.
+
+ Returns:
+ torch.Tensor: Fused features of each voxel.
+ """
+ # the features is consist of a batch of points
+ batch_size = coors[-1, 0] + 1
+ points = []
+ for i in range(batch_size):
+ single_mask = (coors[:, 0] == i)
+ points.append(features[single_mask][mask[single_mask]])
+
+ point_feats = voxel_feats[mask]
+ point_feats = self.fusion_layer(img_feats, points, point_feats,
+ img_metas)
+
+ voxel_canvas = voxel_feats.new_zeros(
+ size=(voxel_feats.size(0), voxel_feats.size(1),
+ point_feats.size(-1)))
+ voxel_canvas[mask] = point_feats
+ out = torch.max(voxel_canvas, dim=1)[0]
+
+ return out
diff --git a/mmdet3d/ops/__init__.py b/mmdet3d/ops/__init__.py
new file mode 100644
index 0000000..88fb74d
--- /dev/null
+++ b/mmdet3d/ops/__init__.py
@@ -0,0 +1,48 @@
+# Copyright (c) OpenMMLab. All rights reserved.
+from mmcv.ops import (RoIAlign, SigmoidFocalLoss, get_compiler_version,
+ get_compiling_cuda_version, nms, roi_align,
+ sigmoid_focal_loss)
+from mmcv.ops.assign_score_withk import assign_score_withk
+from mmcv.ops.ball_query import ball_query
+from mmcv.ops.furthest_point_sample import (furthest_point_sample,
+ furthest_point_sample_with_dist)
+from mmcv.ops.gather_points import gather_points
+from mmcv.ops.group_points import GroupAll, QueryAndGroup, grouping_operation
+from mmcv.ops.knn import knn
+from mmcv.ops.points_in_boxes import (points_in_boxes_all, points_in_boxes_cpu,
+ points_in_boxes_part)
+from mmcv.ops.points_sampler import PointsSampler as Points_Sampler
+from mmcv.ops.roiaware_pool3d import RoIAwarePool3d
+from mmcv.ops.roipoint_pool3d import RoIPointPool3d
+from mmcv.ops.scatter_points import DynamicScatter, dynamic_scatter
+from mmcv.ops.three_interpolate import three_interpolate
+from mmcv.ops.three_nn import three_nn
+from mmcv.ops.voxelize import Voxelization, voxelization
+
+from .dgcnn_modules import DGCNNFAModule, DGCNNFPModule, DGCNNGFModule
+from .norm import NaiveSyncBatchNorm1d, NaiveSyncBatchNorm2d
+from .paconv import PAConv, PAConvCUDA
+from .pointnet_modules import (PAConvCUDASAModule, PAConvCUDASAModuleMSG,
+ PAConvSAModule, PAConvSAModuleMSG,
+ PointFPModule, PointSAModule, PointSAModuleMSG,
+ build_sa_module)
+from .sparse_block import (SparseBasicBlock, SparseBottleneck,
+ make_sparse_convmodule)
+
+__all__ = [
+ 'nms', 'soft_nms', 'RoIAlign', 'roi_align', 'get_compiler_version',
+ 'get_compiling_cuda_version', 'NaiveSyncBatchNorm1d',
+ 'NaiveSyncBatchNorm2d', 'batched_nms', 'Voxelization', 'voxelization',
+ 'dynamic_scatter', 'DynamicScatter', 'sigmoid_focal_loss',
+ 'SigmoidFocalLoss', 'SparseBasicBlock', 'SparseBottleneck',
+ 'RoIAwarePool3d', 'points_in_boxes_part', 'points_in_boxes_cpu',
+ 'make_sparse_convmodule', 'ball_query', 'knn', 'furthest_point_sample',
+ 'furthest_point_sample_with_dist', 'three_interpolate', 'three_nn',
+ 'gather_points', 'grouping_operation', 'GroupAll', 'QueryAndGroup',
+ 'PointSAModule', 'PointSAModuleMSG', 'PointFPModule', 'DGCNNFPModule',
+ 'DGCNNGFModule', 'DGCNNFAModule', 'points_in_boxes_all',
+ 'get_compiler_version', 'assign_score_withk', 'get_compiling_cuda_version',
+ 'Points_Sampler', 'build_sa_module', 'PAConv', 'PAConvCUDA',
+ 'PAConvSAModuleMSG', 'PAConvSAModule', 'PAConvCUDASAModule',
+ 'PAConvCUDASAModuleMSG', 'RoIPointPool3d'
+]
diff --git a/mmdet3d/ops/dgcnn_modules/__init__.py b/mmdet3d/ops/dgcnn_modules/__init__.py
new file mode 100644
index 0000000..67beb09
--- /dev/null
+++ b/mmdet3d/ops/dgcnn_modules/__init__.py
@@ -0,0 +1,6 @@
+# Copyright (c) OpenMMLab. All rights reserved.
+from .dgcnn_fa_module import DGCNNFAModule
+from .dgcnn_fp_module import DGCNNFPModule
+from .dgcnn_gf_module import DGCNNGFModule
+
+__all__ = ['DGCNNFAModule', 'DGCNNFPModule', 'DGCNNGFModule']
diff --git a/mmdet3d/ops/dgcnn_modules/dgcnn_fa_module.py b/mmdet3d/ops/dgcnn_modules/dgcnn_fa_module.py
new file mode 100644
index 0000000..b0975e6
--- /dev/null
+++ b/mmdet3d/ops/dgcnn_modules/dgcnn_fa_module.py
@@ -0,0 +1,68 @@
+# Copyright (c) OpenMMLab. All rights reserved.
+import torch
+from mmcv.cnn import ConvModule
+from mmcv.runner import BaseModule, force_fp32
+from torch import nn as nn
+
+
+class DGCNNFAModule(BaseModule):
+ """Point feature aggregation module used in DGCNN.
+
+ Aggregate all the features of points.
+
+ Args:
+ mlp_channels (list[int]): List of mlp channels.
+ norm_cfg (dict, optional): Type of normalization method.
+ Defaults to dict(type='BN1d').
+ act_cfg (dict, optional): Type of activation method.
+ Defaults to dict(type='ReLU').
+ init_cfg (dict, optional): Initialization config. Defaults to None.
+ """
+
+ def __init__(self,
+ mlp_channels,
+ norm_cfg=dict(type='BN1d'),
+ act_cfg=dict(type='ReLU'),
+ init_cfg=None):
+ super().__init__(init_cfg=init_cfg)
+ self.fp16_enabled = False
+ self.mlps = nn.Sequential()
+ for i in range(len(mlp_channels) - 1):
+ self.mlps.add_module(
+ f'layer{i}',
+ ConvModule(
+ mlp_channels[i],
+ mlp_channels[i + 1],
+ kernel_size=(1, ),
+ stride=(1, ),
+ conv_cfg=dict(type='Conv1d'),
+ norm_cfg=norm_cfg,
+ act_cfg=act_cfg))
+
+ @force_fp32()
+ def forward(self, points):
+ """forward.
+
+ Args:
+ points (List[Tensor]): tensor of the features to be aggregated.
+
+ Returns:
+ Tensor: (B, N, M) M = mlp[-1], tensor of the output points.
+ """
+
+ if len(points) > 1:
+ new_points = torch.cat(points[1:], dim=-1)
+ new_points = new_points.transpose(1, 2).contiguous() # (B, C, N)
+ new_points_copy = new_points
+
+ new_points = self.mlps(new_points)
+
+ new_fa_points = new_points.max(dim=-1, keepdim=True)[0]
+ new_fa_points = new_fa_points.repeat(1, 1, new_points.shape[-1])
+
+ new_points = torch.cat([new_fa_points, new_points_copy], dim=1)
+ new_points = new_points.transpose(1, 2).contiguous()
+ else:
+ new_points = points
+
+ return new_points
diff --git a/mmdet3d/ops/dgcnn_modules/dgcnn_fp_module.py b/mmdet3d/ops/dgcnn_modules/dgcnn_fp_module.py
new file mode 100644
index 0000000..c871721
--- /dev/null
+++ b/mmdet3d/ops/dgcnn_modules/dgcnn_fp_module.py
@@ -0,0 +1,59 @@
+# Copyright (c) OpenMMLab. All rights reserved.
+from mmcv.cnn import ConvModule
+from mmcv.runner import BaseModule, force_fp32
+from torch import nn as nn
+
+
+class DGCNNFPModule(BaseModule):
+ """Point feature propagation module used in DGCNN.
+
+ Propagate the features from one set to another.
+
+ Args:
+ mlp_channels (list[int]): List of mlp channels.
+ norm_cfg (dict, optional): Type of activation method.
+ Defaults to dict(type='BN1d').
+ act_cfg (dict, optional): Type of activation method.
+ Defaults to dict(type='ReLU').
+ init_cfg (dict, optional): Initialization config. Defaults to None.
+ """
+
+ def __init__(self,
+ mlp_channels,
+ norm_cfg=dict(type='BN1d'),
+ act_cfg=dict(type='ReLU'),
+ init_cfg=None):
+ super().__init__(init_cfg=init_cfg)
+ self.fp16_enabled = False
+ self.mlps = nn.Sequential()
+ for i in range(len(mlp_channels) - 1):
+ self.mlps.add_module(
+ f'layer{i}',
+ ConvModule(
+ mlp_channels[i],
+ mlp_channels[i + 1],
+ kernel_size=(1, ),
+ stride=(1, ),
+ conv_cfg=dict(type='Conv1d'),
+ norm_cfg=norm_cfg,
+ act_cfg=act_cfg))
+
+ @force_fp32()
+ def forward(self, points):
+ """forward.
+
+ Args:
+ points (Tensor): (B, N, C) tensor of the input points.
+
+ Returns:
+ Tensor: (B, N, M) M = mlp[-1], tensor of the new points.
+ """
+
+ if points is not None:
+ new_points = points.transpose(1, 2).contiguous() # (B, C, N)
+ new_points = self.mlps(new_points)
+ new_points = new_points.transpose(1, 2).contiguous()
+ else:
+ new_points = points
+
+ return new_points
diff --git a/mmdet3d/ops/dgcnn_modules/dgcnn_gf_module.py b/mmdet3d/ops/dgcnn_modules/dgcnn_gf_module.py
new file mode 100644
index 0000000..96785e7
--- /dev/null
+++ b/mmdet3d/ops/dgcnn_modules/dgcnn_gf_module.py
@@ -0,0 +1,221 @@
+# Copyright (c) OpenMMLab. All rights reserved.
+import torch
+from mmcv.cnn import ConvModule
+from mmcv.ops.group_points import GroupAll, QueryAndGroup, grouping_operation
+from torch import nn as nn
+from torch.nn import functional as F
+
+
+class BaseDGCNNGFModule(nn.Module):
+ """Base module for point graph feature module used in DGCNN.
+
+ Args:
+ radii (list[float]): List of radius in each knn or ball query.
+ sample_nums (list[int]): Number of samples in each knn or ball query.
+ mlp_channels (list[list[int]]): Specify of the dgcnn before
+ the global pooling for each graph feature module.
+ knn_modes (list[str], optional): Type of KNN method, valid mode
+ ['F-KNN', 'D-KNN'], Defaults to ['F-KNN'].
+ dilated_group (bool, optional): Whether to use dilated ball query.
+ Defaults to False.
+ use_xyz (bool, optional): Whether to use xyz as point features.
+ Defaults to True.
+ pool_mode (str, optional): Type of pooling method. Defaults to 'max'.
+ normalize_xyz (bool, optional): If ball query, whether to normalize
+ local XYZ with radius. Defaults to False.
+ grouper_return_grouped_xyz (bool, optional): Whether to return grouped
+ xyz in `QueryAndGroup`. Defaults to False.
+ grouper_return_grouped_idx (bool, optional): Whether to return grouped
+ idx in `QueryAndGroup`. Defaults to False.
+ """
+
+ def __init__(self,
+ radii,
+ sample_nums,
+ mlp_channels,
+ knn_modes=['F-KNN'],
+ dilated_group=False,
+ use_xyz=True,
+ pool_mode='max',
+ normalize_xyz=False,
+ grouper_return_grouped_xyz=False,
+ grouper_return_grouped_idx=False):
+ super(BaseDGCNNGFModule, self).__init__()
+
+ assert len(sample_nums) == len(
+ mlp_channels
+ ), 'Num_samples and mlp_channels should have the same length.'
+ assert pool_mode in ['max', 'avg'
+ ], "Pool_mode should be one of ['max', 'avg']."
+ assert isinstance(knn_modes, list) or isinstance(
+ knn_modes, tuple), 'The type of knn_modes should be list or tuple.'
+
+ if isinstance(mlp_channels, tuple):
+ mlp_channels = list(map(list, mlp_channels))
+ self.mlp_channels = mlp_channels
+
+ self.pool_mode = pool_mode
+ self.groupers = nn.ModuleList()
+ self.mlps = nn.ModuleList()
+ self.knn_modes = knn_modes
+
+ for i in range(len(sample_nums)):
+ sample_num = sample_nums[i]
+ if sample_num is not None:
+ if self.knn_modes[i] == 'D-KNN':
+ grouper = QueryAndGroup(
+ radii[i],
+ sample_num,
+ use_xyz=use_xyz,
+ normalize_xyz=normalize_xyz,
+ return_grouped_xyz=grouper_return_grouped_xyz,
+ return_grouped_idx=True)
+ else:
+ grouper = QueryAndGroup(
+ radii[i],
+ sample_num,
+ use_xyz=use_xyz,
+ normalize_xyz=normalize_xyz,
+ return_grouped_xyz=grouper_return_grouped_xyz,
+ return_grouped_idx=grouper_return_grouped_idx)
+ else:
+ grouper = GroupAll(use_xyz)
+ self.groupers.append(grouper)
+
+ def _pool_features(self, features):
+ """Perform feature aggregation using pooling operation.
+
+ Args:
+ features (torch.Tensor): (B, C, N, K)
+ Features of locally grouped points before pooling.
+
+ Returns:
+ torch.Tensor: (B, C, N)
+ Pooled features aggregating local information.
+ """
+ if self.pool_mode == 'max':
+ # (B, C, N, 1)
+ new_features = F.max_pool2d(
+ features, kernel_size=[1, features.size(3)])
+ elif self.pool_mode == 'avg':
+ # (B, C, N, 1)
+ new_features = F.avg_pool2d(
+ features, kernel_size=[1, features.size(3)])
+ else:
+ raise NotImplementedError
+
+ return new_features.squeeze(-1).contiguous()
+
+ def forward(self, points):
+ """forward.
+
+ Args:
+ points (Tensor): (B, N, C) input points.
+
+ Returns:
+ List[Tensor]: (B, N, C1) new points generated from each graph
+ feature module.
+ """
+ new_points_list = [points]
+
+ for i in range(len(self.groupers)):
+
+ new_points = new_points_list[i]
+ new_points_trans = new_points.transpose(
+ 1, 2).contiguous() # (B, C, N)
+
+ if self.knn_modes[i] == 'D-KNN':
+ # (B, N, C) -> (B, N, K)
+ idx = self.groupers[i](new_points[..., -3:].contiguous(),
+ new_points[..., -3:].contiguous())[-1]
+
+ grouped_results = grouping_operation(
+ new_points_trans, idx) # (B, C, N) -> (B, C, N, K)
+ grouped_results -= new_points_trans.unsqueeze(-1)
+ else:
+ grouped_results = self.groupers[i](
+ new_points, new_points) # (B, N, C) -> (B, C, N, K)
+
+ new_points = new_points_trans.unsqueeze(-1).repeat(
+ 1, 1, 1, grouped_results.shape[-1])
+ new_points = torch.cat([grouped_results, new_points], dim=1)
+
+ # (B, mlp[-1], N, K)
+ new_points = self.mlps[i](new_points)
+
+ # (B, mlp[-1], N)
+ new_points = self._pool_features(new_points)
+ new_points = new_points.transpose(1, 2).contiguous()
+ new_points_list.append(new_points)
+
+ return new_points
+
+
+class DGCNNGFModule(BaseDGCNNGFModule):
+ """Point graph feature module used in DGCNN.
+
+ Args:
+ mlp_channels (list[int]): Specify of the dgcnn before
+ the global pooling for each graph feature module.
+ num_sample (int, optional): Number of samples in each knn or ball
+ query. Defaults to None.
+ knn_mode (str, optional): Type of KNN method, valid mode
+ ['F-KNN', 'D-KNN']. Defaults to 'F-KNN'.
+ radius (float, optional): Radius to group with.
+ Defaults to None.
+ dilated_group (bool, optional): Whether to use dilated ball query.
+ Defaults to False.
+ norm_cfg (dict, optional): Type of normalization method.
+ Defaults to dict(type='BN2d').
+ act_cfg (dict, optional): Type of activation method.
+ Defaults to dict(type='ReLU').
+ use_xyz (bool, optional): Whether to use xyz as point features.
+ Defaults to True.
+ pool_mode (str, optional): Type of pooling method.
+ Defaults to 'max'.
+ normalize_xyz (bool, optional): If ball query, whether to normalize
+ local XYZ with radius. Defaults to False.
+ bias (bool | str, optional): If specified as `auto`, it will be decided
+ by the norm_cfg. Bias will be set as True if `norm_cfg` is None,
+ otherwise False. Defaults to 'auto'.
+ """
+
+ def __init__(self,
+ mlp_channels,
+ num_sample=None,
+ knn_mode='F-KNN',
+ radius=None,
+ dilated_group=False,
+ norm_cfg=dict(type='BN2d'),
+ act_cfg=dict(type='ReLU'),
+ use_xyz=True,
+ pool_mode='max',
+ normalize_xyz=False,
+ bias='auto'):
+ super(DGCNNGFModule, self).__init__(
+ mlp_channels=[mlp_channels],
+ sample_nums=[num_sample],
+ knn_modes=[knn_mode],
+ radii=[radius],
+ use_xyz=use_xyz,
+ pool_mode=pool_mode,
+ normalize_xyz=normalize_xyz,
+ dilated_group=dilated_group)
+
+ for i in range(len(self.mlp_channels)):
+ mlp_channel = self.mlp_channels[i]
+
+ mlp = nn.Sequential()
+ for i in range(len(mlp_channel) - 1):
+ mlp.add_module(
+ f'layer{i}',
+ ConvModule(
+ mlp_channel[i],
+ mlp_channel[i + 1],
+ kernel_size=(1, 1),
+ stride=(1, 1),
+ conv_cfg=dict(type='Conv2d'),
+ norm_cfg=norm_cfg,
+ act_cfg=act_cfg,
+ bias=bias))
+ self.mlps.append(mlp)
diff --git a/mmdet3d/ops/norm.py b/mmdet3d/ops/norm.py
new file mode 100644
index 0000000..98ec7f1
--- /dev/null
+++ b/mmdet3d/ops/norm.py
@@ -0,0 +1,163 @@
+# Copyright (c) OpenMMLab. All rights reserved.
+import torch
+from mmcv.cnn import NORM_LAYERS
+from mmcv.runner import force_fp32
+from torch import distributed as dist
+from torch import nn as nn
+from torch.autograd.function import Function
+
+
+class AllReduce(Function):
+
+ @staticmethod
+ def forward(ctx, input):
+ input_list = [
+ torch.zeros_like(input) for k in range(dist.get_world_size())
+ ]
+ # Use allgather instead of allreduce in-place operations is unreliable
+ dist.all_gather(input_list, input, async_op=False)
+ inputs = torch.stack(input_list, dim=0)
+ return torch.sum(inputs, dim=0)
+
+ @staticmethod
+ def backward(ctx, grad_output):
+ dist.all_reduce(grad_output, async_op=False)
+ return grad_output
+
+
+@NORM_LAYERS.register_module('naiveSyncBN1d')
+class NaiveSyncBatchNorm1d(nn.BatchNorm1d):
+ """Synchronized Batch Normalization for 3D Tensors.
+
+ Note:
+ This implementation is modified from
+ https://github.com/facebookresearch/detectron2/
+
+ `torch.nn.SyncBatchNorm` has known unknown bugs.
+ It produces significantly worse AP (and sometimes goes NaN)
+ when the batch size on each worker is quite different
+ (e.g., when scale augmentation is used).
+ In 3D detection, different workers has points of different shapes,
+ which also cause instability.
+
+ Use this implementation before `nn.SyncBatchNorm` is fixed.
+ It is slower than `nn.SyncBatchNorm`.
+ """
+
+ def __init__(self, *args, **kwargs):
+ super().__init__(*args, **kwargs)
+ self.fp16_enabled = False
+
+ # customized normalization layer still needs this decorator
+ # to force the input to be fp32 and the output to be fp16
+ # TODO: make mmcv fp16 utils handle customized norm layers
+ @force_fp32(out_fp16=True)
+ def forward(self, input):
+ """
+ Args:
+ input (tensor): Has shape (N, C) or (N, C, L), where N is
+ the batch size, C is the number of features or
+ channels, and L is the sequence length
+
+ Returns:
+ tensor: Has shape (N, C) or (N, C, L), has same shape
+ as input.
+ """
+ assert input.dtype == torch.float32, \
+ f'input should be in float32 type, got {input.dtype}'
+ using_dist = dist.is_available() and dist.is_initialized()
+ if (not using_dist) or dist.get_world_size() == 1 \
+ or not self.training:
+ return super().forward(input)
+ assert input.shape[0] > 0, 'SyncBN does not support empty inputs'
+ is_two_dim = input.dim() == 2
+ if is_two_dim:
+ input = input.unsqueeze(2)
+
+ C = input.shape[1]
+ mean = torch.mean(input, dim=[0, 2])
+ meansqr = torch.mean(input * input, dim=[0, 2])
+
+ vec = torch.cat([mean, meansqr], dim=0)
+ vec = AllReduce.apply(vec) * (1.0 / dist.get_world_size())
+
+ mean, meansqr = torch.split(vec, C)
+ var = meansqr - mean * mean
+ self.running_mean += self.momentum * (
+ mean.detach() - self.running_mean)
+ self.running_var += self.momentum * (var.detach() - self.running_var)
+
+ invstd = torch.rsqrt(var + self.eps)
+ scale = self.weight * invstd
+ bias = self.bias - mean * scale
+ scale = scale.reshape(1, -1, 1)
+ bias = bias.reshape(1, -1, 1)
+ output = input * scale + bias
+ if is_two_dim:
+ output = output.squeeze(2)
+ return output
+
+
+@NORM_LAYERS.register_module('naiveSyncBN2d')
+class NaiveSyncBatchNorm2d(nn.BatchNorm2d):
+ """Synchronized Batch Normalization for 4D Tensors.
+
+ Note:
+ This implementation is modified from
+ https://github.com/facebookresearch/detectron2/
+
+ `torch.nn.SyncBatchNorm` has known unknown bugs.
+ It produces significantly worse AP (and sometimes goes NaN)
+ when the batch size on each worker is quite different
+ (e.g., when scale augmentation is used).
+ This phenomenon also occurs when the multi-modality feature fusion
+ modules of multi-modality detectors use SyncBN.
+
+ Use this implementation before `nn.SyncBatchNorm` is fixed.
+ It is slower than `nn.SyncBatchNorm`.
+ """
+
+ def __init__(self, *args, **kwargs):
+ super().__init__(*args, **kwargs)
+ self.fp16_enabled = False
+
+ # customized normalization layer still needs this decorator
+ # to force the input to be fp32 and the output to be fp16
+ # TODO: make mmcv fp16 utils handle customized norm layers
+ @force_fp32(out_fp16=True)
+ def forward(self, input):
+ """
+ Args:
+ Input (tensor): Feature has shape (N, C, H, W).
+
+ Returns:
+ tensor: Has shape (N, C, H, W), same shape as input.
+ """
+ assert input.dtype == torch.float32, \
+ f'input should be in float32 type, got {input.dtype}'
+ using_dist = dist.is_available() and dist.is_initialized()
+ if (not using_dist) or \
+ dist.get_world_size() == 1 or \
+ not self.training:
+ return super().forward(input)
+
+ assert input.shape[0] > 0, 'SyncBN does not support empty inputs'
+ C = input.shape[1]
+ mean = torch.mean(input, dim=[0, 2, 3])
+ meansqr = torch.mean(input * input, dim=[0, 2, 3])
+
+ vec = torch.cat([mean, meansqr], dim=0)
+ vec = AllReduce.apply(vec) * (1.0 / dist.get_world_size())
+
+ mean, meansqr = torch.split(vec, C)
+ var = meansqr - mean * mean
+ self.running_mean += self.momentum * (
+ mean.detach() - self.running_mean)
+ self.running_var += self.momentum * (var.detach() - self.running_var)
+
+ invstd = torch.rsqrt(var + self.eps)
+ scale = self.weight * invstd
+ bias = self.bias - mean * scale
+ scale = scale.reshape(1, -1, 1, 1)
+ bias = bias.reshape(1, -1, 1, 1)
+ return input * scale + bias
diff --git a/mmdet3d/ops/paconv/__init__.py b/mmdet3d/ops/paconv/__init__.py
new file mode 100644
index 0000000..d71c766
--- /dev/null
+++ b/mmdet3d/ops/paconv/__init__.py
@@ -0,0 +1,4 @@
+# Copyright (c) OpenMMLab. All rights reserved.
+from .paconv import PAConv, PAConvCUDA
+
+__all__ = ['PAConv', 'PAConvCUDA']
diff --git a/mmdet3d/ops/paconv/paconv.py b/mmdet3d/ops/paconv/paconv.py
new file mode 100644
index 0000000..bda8bfe
--- /dev/null
+++ b/mmdet3d/ops/paconv/paconv.py
@@ -0,0 +1,392 @@
+# Copyright (c) OpenMMLab. All rights reserved.
+import copy
+
+import torch
+from mmcv.cnn import (ConvModule, build_activation_layer, build_norm_layer,
+ constant_init)
+from mmcv.ops import assign_score_withk as assign_score_cuda
+from torch import nn as nn
+from torch.nn import functional as F
+
+from .utils import assign_kernel_withoutk, assign_score, calc_euclidian_dist
+
+
+class ScoreNet(nn.Module):
+ r"""ScoreNet that outputs coefficient scores to assemble kernel weights in
+ the weight bank according to the relative position of point pairs.
+
+ Args:
+ mlp_channels (List[int]): Hidden unit sizes of SharedMLP layers.
+ last_bn (bool, optional): Whether to use BN on the last output of mlps.
+ Defaults to False.
+ score_norm (str, optional): Normalization function of output scores.
+ Can be 'softmax', 'sigmoid' or 'identity'. Defaults to 'softmax'.
+ temp_factor (float, optional): Temperature factor to scale the output
+ scores before softmax. Defaults to 1.0.
+ norm_cfg (dict, optional): Type of normalization method.
+ Defaults to dict(type='BN2d').
+ bias (bool | str, optional): If specified as `auto`, it will be decided
+ by the norm_cfg. Bias will be set as True if `norm_cfg` is None,
+ otherwise False. Defaults to 'auto'.
+
+ Note:
+ The official code applies xavier_init to all Conv layers in ScoreNet,
+ see `PAConv `_. However in our experiments, we
+ did not find much difference in applying such xavier initialization
+ or not. So we neglect this initialization in our implementation.
+ """
+
+ def __init__(self,
+ mlp_channels,
+ last_bn=False,
+ score_norm='softmax',
+ temp_factor=1.0,
+ norm_cfg=dict(type='BN2d'),
+ bias='auto'):
+ super(ScoreNet, self).__init__()
+
+ assert score_norm in ['softmax', 'sigmoid', 'identity'], \
+ f'unsupported score_norm function {score_norm}'
+
+ self.score_norm = score_norm
+ self.temp_factor = temp_factor
+
+ self.mlps = nn.Sequential()
+ for i in range(len(mlp_channels) - 2):
+ self.mlps.add_module(
+ f'layer{i}',
+ ConvModule(
+ mlp_channels[i],
+ mlp_channels[i + 1],
+ kernel_size=(1, 1),
+ stride=(1, 1),
+ conv_cfg=dict(type='Conv2d'),
+ norm_cfg=norm_cfg,
+ bias=bias))
+
+ # for the last mlp that outputs scores, no relu and possibly no bn
+ i = len(mlp_channels) - 2
+ self.mlps.add_module(
+ f'layer{i}',
+ ConvModule(
+ mlp_channels[i],
+ mlp_channels[i + 1],
+ kernel_size=(1, 1),
+ stride=(1, 1),
+ conv_cfg=dict(type='Conv2d'),
+ norm_cfg=norm_cfg if last_bn else None,
+ act_cfg=None,
+ bias=bias))
+
+ def forward(self, xyz_features):
+ """Forward.
+
+ Args:
+ xyz_features (torch.Tensor): (B, C, N, K), features constructed
+ from xyz coordinates of point pairs. May contain relative
+ positions, Euclidean distance, etc.
+
+ Returns:
+ torch.Tensor: (B, N, K, M), predicted scores for `M` kernels.
+ """
+ scores = self.mlps(xyz_features) # (B, M, N, K)
+
+ # perform score normalization
+ if self.score_norm == 'softmax':
+ scores = F.softmax(scores / self.temp_factor, dim=1)
+ elif self.score_norm == 'sigmoid':
+ scores = torch.sigmoid(scores / self.temp_factor)
+ else: # 'identity'
+ scores = scores
+
+ scores = scores.permute(0, 2, 3, 1) # (B, N, K, M)
+
+ return scores
+
+
+class PAConv(nn.Module):
+ """Non-CUDA version of PAConv.
+
+ PAConv stores a trainable weight bank containing several kernel weights.
+ Given input points and features, it computes coefficient scores to assemble
+ those kernels to form conv kernels, and then runs convolution on the input.
+
+ Args:
+ in_channels (int): Input channels of point features.
+ out_channels (int): Output channels of point features.
+ num_kernels (int): Number of kernel weights in the weight bank.
+ norm_cfg (dict, optional): Type of normalization method.
+ Defaults to dict(type='BN2d', momentum=0.1).
+ act_cfg (dict, optional): Type of activation method.
+ Defaults to dict(type='ReLU', inplace=True).
+ scorenet_input (str, optional): Type of input to ScoreNet.
+ Can be 'identity', 'w_neighbor' or 'w_neighbor_dist'.
+ Defaults to 'w_neighbor_dist'.
+ weight_bank_init (str, optional): Init method of weight bank kernels.
+ Can be 'kaiming' or 'xavier'. Defaults to 'kaiming'.
+ kernel_input (str, optional): Input features to be multiplied with
+ kernel weights. Can be 'identity' or 'w_neighbor'.
+ Defaults to 'w_neighbor'.
+ scorenet_cfg (dict, optional): Config of the ScoreNet module, which
+ may contain the following keys and values:
+
+ - mlp_channels (List[int]): Hidden units of MLPs.
+ - score_norm (str): Normalization function of output scores.
+ Can be 'softmax', 'sigmoid' or 'identity'.
+ - temp_factor (float): Temperature factor to scale the output
+ scores before softmax.
+ - last_bn (bool): Whether to use BN on the last output of mlps.
+ """
+
+ def __init__(self,
+ in_channels,
+ out_channels,
+ num_kernels,
+ norm_cfg=dict(type='BN2d', momentum=0.1),
+ act_cfg=dict(type='ReLU', inplace=True),
+ scorenet_input='w_neighbor_dist',
+ weight_bank_init='kaiming',
+ kernel_input='w_neighbor',
+ scorenet_cfg=dict(
+ mlp_channels=[16, 16, 16],
+ score_norm='softmax',
+ temp_factor=1.0,
+ last_bn=False)):
+ super(PAConv, self).__init__()
+
+ # determine weight kernel size according to used features
+ if kernel_input == 'identity':
+ # only use grouped_features
+ kernel_mul = 1
+ elif kernel_input == 'w_neighbor':
+ # concat of (grouped_features - center_features, grouped_features)
+ kernel_mul = 2
+ else:
+ raise NotImplementedError(
+ f'unsupported kernel_input {kernel_input}')
+ self.kernel_input = kernel_input
+ in_channels = kernel_mul * in_channels
+
+ # determine mlp channels in ScoreNet according to used xyz features
+ if scorenet_input == 'identity':
+ # only use relative position (grouped_xyz - center_xyz)
+ self.scorenet_in_channels = 3
+ elif scorenet_input == 'w_neighbor':
+ # (grouped_xyz - center_xyz, grouped_xyz)
+ self.scorenet_in_channels = 6
+ elif scorenet_input == 'w_neighbor_dist':
+ # (center_xyz, grouped_xyz - center_xyz, Euclidean distance)
+ self.scorenet_in_channels = 7
+ else:
+ raise NotImplementedError(
+ f'unsupported scorenet_input {scorenet_input}')
+ self.scorenet_input = scorenet_input
+
+ # construct kernel weights in weight bank
+ # self.weight_bank is of shape [C, num_kernels * out_c]
+ # where C can be in_c or (2 * in_c)
+ if weight_bank_init == 'kaiming':
+ weight_init = nn.init.kaiming_normal_
+ elif weight_bank_init == 'xavier':
+ weight_init = nn.init.xavier_normal_
+ else:
+ raise NotImplementedError(
+ f'unsupported weight bank init method {weight_bank_init}')
+
+ self.num_kernels = num_kernels # the parameter `m` in the paper
+ weight_bank = weight_init(
+ torch.empty(self.num_kernels, in_channels, out_channels))
+ weight_bank = weight_bank.permute(1, 0, 2).reshape(
+ in_channels, self.num_kernels * out_channels).contiguous()
+ self.weight_bank = nn.Parameter(weight_bank, requires_grad=True)
+
+ # construct ScoreNet
+ scorenet_cfg_ = copy.deepcopy(scorenet_cfg)
+ scorenet_cfg_['mlp_channels'].insert(0, self.scorenet_in_channels)
+ scorenet_cfg_['mlp_channels'].append(self.num_kernels)
+ self.scorenet = ScoreNet(**scorenet_cfg_)
+
+ self.bn = build_norm_layer(norm_cfg, out_channels)[1] if \
+ norm_cfg is not None else None
+ self.activate = build_activation_layer(act_cfg) if \
+ act_cfg is not None else None
+
+ # set some basic attributes of Conv layers
+ self.in_channels = in_channels
+ self.out_channels = out_channels
+
+ self.init_weights()
+
+ def init_weights(self):
+ """Initialize weights of shared MLP layers and BN layers."""
+ if self.bn is not None:
+ constant_init(self.bn, val=1, bias=0)
+
+ def _prepare_scorenet_input(self, points_xyz):
+ """Prepare input point pairs features for self.ScoreNet.
+
+ Args:
+ points_xyz (torch.Tensor): (B, 3, npoint, K)
+ Coordinates of the grouped points.
+
+ Returns:
+ torch.Tensor: (B, C, npoint, K)
+ The generated features per point pair.
+ """
+ B, _, npoint, K = points_xyz.size()
+ center_xyz = points_xyz[..., :1].repeat(1, 1, 1, K)
+ xyz_diff = points_xyz - center_xyz # [B, 3, npoint, K]
+ if self.scorenet_input == 'identity':
+ xyz_features = xyz_diff
+ elif self.scorenet_input == 'w_neighbor':
+ xyz_features = torch.cat((xyz_diff, points_xyz), dim=1)
+ else: # w_neighbor_dist
+ euclidian_dist = calc_euclidian_dist(
+ center_xyz.permute(0, 2, 3, 1).reshape(B * npoint * K, 3),
+ points_xyz.permute(0, 2, 3, 1).reshape(B * npoint * K, 3)).\
+ reshape(B, 1, npoint, K)
+ xyz_features = torch.cat((center_xyz, xyz_diff, euclidian_dist),
+ dim=1)
+ return xyz_features
+
+ def forward(self, inputs):
+ """Forward.
+
+ Args:
+ inputs (tuple(torch.Tensor)):
+
+ - features (torch.Tensor): (B, in_c, npoint, K)
+ Features of the queried points.
+ - points_xyz (torch.Tensor): (B, 3, npoint, K)
+ Coordinates of the grouped points.
+
+ Returns:
+ Tuple[torch.Tensor]:
+
+ - new_features: (B, out_c, npoint, K), features after PAConv.
+ - points_xyz: same as input.
+ """
+ features, points_xyz = inputs
+ B, _, npoint, K = features.size()
+
+ if self.kernel_input == 'w_neighbor':
+ center_features = features[..., :1].repeat(1, 1, 1, K)
+ features_diff = features - center_features
+ # to (B, 2 * in_c, npoint, K)
+ features = torch.cat((features_diff, features), dim=1)
+
+ # prepare features for between each point and its grouping center
+ xyz_features = self._prepare_scorenet_input(points_xyz)
+
+ # scores to assemble kernel weights
+ scores = self.scorenet(xyz_features) # [B, npoint, K, m]
+
+ # first compute out features over all kernels
+ # features is [B, C, npoint, K], weight_bank is [C, m * out_c]
+ new_features = torch.matmul(
+ features.permute(0, 2, 3, 1),
+ self.weight_bank).view(B, npoint, K, self.num_kernels,
+ -1) # [B, npoint, K, m, out_c]
+
+ # then aggregate using scores
+ new_features = assign_score(scores, new_features)
+ # to [B, out_c, npoint, K]
+ new_features = new_features.permute(0, 3, 1, 2).contiguous()
+
+ if self.bn is not None:
+ new_features = self.bn(new_features)
+ if self.activate is not None:
+ new_features = self.activate(new_features)
+
+ # in order to keep input output consistency
+ # so that we can wrap PAConv in Sequential
+ return (new_features, points_xyz)
+
+
+class PAConvCUDA(PAConv):
+ """CUDA version of PAConv that implements a cuda op to efficiently perform
+ kernel assembling.
+
+ Different from vanilla PAConv, the input features of this function is not
+ grouped by centers. Instead, they will be queried on-the-fly by the
+ additional input `points_idx`. This avoids the large intermediate matrix.
+ See the `paper `_ appendix Sec. D for
+ more detailed descriptions.
+ """
+
+ def __init__(self,
+ in_channels,
+ out_channels,
+ num_kernels,
+ norm_cfg=dict(type='BN2d', momentum=0.1),
+ act_cfg=dict(type='ReLU', inplace=True),
+ scorenet_input='w_neighbor_dist',
+ weight_bank_init='kaiming',
+ kernel_input='w_neighbor',
+ scorenet_cfg=dict(
+ mlp_channels=[8, 16, 16],
+ score_norm='softmax',
+ temp_factor=1.0,
+ last_bn=False)):
+ super(PAConvCUDA, self).__init__(
+ in_channels=in_channels,
+ out_channels=out_channels,
+ num_kernels=num_kernels,
+ norm_cfg=norm_cfg,
+ act_cfg=act_cfg,
+ scorenet_input=scorenet_input,
+ weight_bank_init=weight_bank_init,
+ kernel_input=kernel_input,
+ scorenet_cfg=scorenet_cfg)
+
+ assert self.kernel_input == 'w_neighbor', \
+ 'CUDA implemented PAConv only supports w_neighbor kernel_input'
+
+ def forward(self, inputs):
+ """Forward.
+
+ Args:
+ inputs (tuple(torch.Tensor)):
+
+ - features (torch.Tensor): (B, in_c, N)
+ Features of all points in the current point cloud.
+ Different from non-CUDA version PAConv, here the features
+ are not grouped by each center to form a K dim.
+ - points_xyz (torch.Tensor): (B, 3, npoint, K)
+ Coordinates of the grouped points.
+ - points_idx (torch.Tensor): (B, npoint, K)
+ Index of the grouped points.
+
+ Returns:
+ Tuple[torch.Tensor]:
+
+ - new_features: (B, out_c, npoint, K), features after PAConv.
+ - points_xyz: same as input.
+ - points_idx: same as input.
+ """
+ features, points_xyz, points_idx = inputs
+
+ # prepare features for between each point and its grouping center
+ xyz_features = self._prepare_scorenet_input(points_xyz)
+
+ # scores to assemble kernel weights
+ scores = self.scorenet(xyz_features) # [B, npoint, K, m]
+
+ # pre-compute features for points and centers separately
+ # features is [B, in_c, N], weight_bank is [C, m * out_dim]
+ point_feat, center_feat = assign_kernel_withoutk(
+ features, self.weight_bank, self.num_kernels)
+
+ # aggregate features using custom cuda op
+ new_features = assign_score_cuda(
+ scores, point_feat, center_feat, points_idx,
+ 'sum').contiguous() # [B, out_c, npoint, K]
+
+ if self.bn is not None:
+ new_features = self.bn(new_features)
+ if self.activate is not None:
+ new_features = self.activate(new_features)
+
+ # in order to keep input output consistency
+ return (new_features, points_xyz, points_idx)
diff --git a/mmdet3d/ops/paconv/utils.py b/mmdet3d/ops/paconv/utils.py
new file mode 100644
index 0000000..68e71d5
--- /dev/null
+++ b/mmdet3d/ops/paconv/utils.py
@@ -0,0 +1,87 @@
+# Copyright (c) OpenMMLab. All rights reserved.
+import torch
+
+
+def calc_euclidian_dist(xyz1, xyz2):
+ """Calculate the Euclidean distance between two sets of points.
+
+ Args:
+ xyz1 (torch.Tensor): (N, 3), the first set of points.
+ xyz2 (torch.Tensor): (N, 3), the second set of points.
+
+ Returns:
+ torch.Tensor: (N, ), the Euclidean distance between each point pair.
+ """
+ assert xyz1.shape[0] == xyz2.shape[0], 'number of points are not the same'
+ assert xyz1.shape[1] == xyz2.shape[1] == 3, \
+ 'points coordinates dimension is not 3'
+ return torch.norm(xyz1 - xyz2, dim=-1)
+
+
+def assign_score(scores, point_features):
+ """Perform weighted sum to aggregate output features according to scores.
+ This function is used in non-CUDA version of PAConv.
+
+ Compared to the cuda op assigh_score_withk, this pytorch implementation
+ pre-computes output features for the neighbors of all centers, and then
+ performs aggregation. It consumes more GPU memories.
+
+ Args:
+ scores (torch.Tensor): (B, npoint, K, M), predicted scores to
+ aggregate weight matrices in the weight bank.
+ `npoint` is the number of sampled centers.
+ `K` is the number of queried neighbors.
+ `M` is the number of weight matrices in the weight bank.
+ point_features (torch.Tensor): (B, npoint, K, M, out_dim)
+ Pre-computed point features to be aggregated.
+
+ Returns:
+ torch.Tensor: (B, npoint, K, out_dim), the aggregated features.
+ """
+ B, npoint, K, M = scores.size()
+ scores = scores.view(B, npoint, K, 1, M)
+ output = torch.matmul(scores, point_features).view(B, npoint, K, -1)
+ return output
+
+
+def assign_kernel_withoutk(features, kernels, M):
+ """Pre-compute features with weight matrices in weight bank. This function
+ is used before cuda op assign_score_withk in CUDA version PAConv.
+
+ Args:
+ features (torch.Tensor): (B, in_dim, N), input features of all points.
+ `N` is the number of points in current point cloud.
+ kernels (torch.Tensor): (2 * in_dim, M * out_dim), weight matrices in
+ the weight bank, transformed from (M, 2 * in_dim, out_dim).
+ `2 * in_dim` is because the input features are concatenation of
+ (point_features - center_features, point_features).
+ M (int): Number of weight matrices in the weight bank.
+
+ Returns:
+ Tuple[torch.Tensor]: both of shape (B, N, M, out_dim):
+
+ - point_features: Pre-computed features for points.
+ - center_features: Pre-computed features for centers.
+ """
+ B, in_dim, N = features.size()
+ feat_trans = features.permute(0, 2, 1) # [B, N, in_dim]
+ out_feat_half1 = torch.matmul(feat_trans, kernels[:in_dim]).view(
+ B, N, M, -1) # [B, N, M, out_dim]
+ out_feat_half2 = torch.matmul(feat_trans, kernels[in_dim:]).view(
+ B, N, M, -1) # [B, N, M, out_dim]
+
+ # TODO: why this hard-coded if condition?
+ # when the network input is only xyz without additional features
+ # xyz will be used as features, so that features.size(1) == 3 % 2 != 0
+ # we need to compensate center_features because otherwise
+ # `point_features - center_features` will result in all zeros?
+ if features.size(1) % 2 != 0:
+ out_feat_half_coord = torch.matmul(
+ feat_trans[:, :, :3], # [B, N, 3]
+ kernels[in_dim:in_dim + 3]).view(B, N, M, -1) # [B, N, M, out_dim]
+ else:
+ out_feat_half_coord = torch.zeros_like(out_feat_half2)
+
+ point_features = out_feat_half1 + out_feat_half2
+ center_features = out_feat_half1 + out_feat_half_coord
+ return point_features, center_features
diff --git a/mmdet3d/ops/pointnet_modules/__init__.py b/mmdet3d/ops/pointnet_modules/__init__.py
new file mode 100644
index 0000000..99b08eb
--- /dev/null
+++ b/mmdet3d/ops/pointnet_modules/__init__.py
@@ -0,0 +1,12 @@
+# Copyright (c) OpenMMLab. All rights reserved.
+from .builder import build_sa_module
+from .paconv_sa_module import (PAConvCUDASAModule, PAConvCUDASAModuleMSG,
+ PAConvSAModule, PAConvSAModuleMSG)
+from .point_fp_module import PointFPModule
+from .point_sa_module import PointSAModule, PointSAModuleMSG
+
+__all__ = [
+ 'build_sa_module', 'PointSAModuleMSG', 'PointSAModule', 'PointFPModule',
+ 'PAConvSAModule', 'PAConvSAModuleMSG', 'PAConvCUDASAModule',
+ 'PAConvCUDASAModuleMSG'
+]
diff --git a/mmdet3d/ops/pointnet_modules/builder.py b/mmdet3d/ops/pointnet_modules/builder.py
new file mode 100644
index 0000000..6631cb4
--- /dev/null
+++ b/mmdet3d/ops/pointnet_modules/builder.py
@@ -0,0 +1,39 @@
+# Copyright (c) OpenMMLab. All rights reserved.
+from mmcv.utils import Registry
+
+SA_MODULES = Registry('point_sa_module')
+
+
+def build_sa_module(cfg, *args, **kwargs):
+ """Build PointNet2 set abstraction (SA) module.
+
+ Args:
+ cfg (None or dict): The SA module config, which should contain:
+ - type (str): Module type.
+ - module args: Args needed to instantiate an SA module.
+ args (argument list): Arguments passed to the `__init__`
+ method of the corresponding module.
+ kwargs (keyword arguments): Keyword arguments passed to the `__init__`
+ method of the corresponding SA module .
+
+ Returns:
+ nn.Module: Created SA module.
+ """
+ if cfg is None:
+ cfg_ = dict(type='PointSAModule')
+ else:
+ if not isinstance(cfg, dict):
+ raise TypeError('cfg must be a dict')
+ if 'type' not in cfg:
+ raise KeyError('the cfg dict must contain the key "type"')
+ cfg_ = cfg.copy()
+
+ module_type = cfg_.pop('type')
+ if module_type not in SA_MODULES:
+ raise KeyError(f'Unrecognized module type {module_type}')
+ else:
+ sa_module = SA_MODULES.get(module_type)
+
+ module = sa_module(*args, **kwargs, **cfg_)
+
+ return module
diff --git a/mmdet3d/ops/pointnet_modules/paconv_sa_module.py b/mmdet3d/ops/pointnet_modules/paconv_sa_module.py
new file mode 100644
index 0000000..361ecbb
--- /dev/null
+++ b/mmdet3d/ops/pointnet_modules/paconv_sa_module.py
@@ -0,0 +1,342 @@
+# Copyright (c) OpenMMLab. All rights reserved.
+import torch
+from torch import nn as nn
+
+from mmdet3d.ops import PAConv, PAConvCUDA
+from .builder import SA_MODULES
+from .point_sa_module import BasePointSAModule
+
+
+@SA_MODULES.register_module()
+class PAConvSAModuleMSG(BasePointSAModule):
+ r"""Point set abstraction module with multi-scale grouping (MSG) used in
+ PAConv networks.
+
+ Replace the MLPs in `PointSAModuleMSG` with PAConv layers.
+ See the `paper `_ for more details.
+
+ Args:
+ paconv_num_kernels (list[list[int]]): Number of kernel weights in the
+ weight banks of each layer's PAConv.
+ paconv_kernel_input (str, optional): Input features to be multiplied
+ with kernel weights. Can be 'identity' or 'w_neighbor'.
+ Defaults to 'w_neighbor'.
+ scorenet_input (str, optional): Type of the input to ScoreNet.
+ Defaults to 'w_neighbor_dist'. Can be the following values:
+
+ - 'identity': Use xyz coordinates as input.
+ - 'w_neighbor': Use xyz coordinates and the difference with center
+ points as input.
+ - 'w_neighbor_dist': Use xyz coordinates, the difference with
+ center points and the Euclidean distance as input.
+
+ scorenet_cfg (dict, optional): Config of the ScoreNet module, which
+ may contain the following keys and values:
+
+ - mlp_channels (List[int]): Hidden units of MLPs.
+ - score_norm (str): Normalization function of output scores.
+ Can be 'softmax', 'sigmoid' or 'identity'.
+ - temp_factor (float): Temperature factor to scale the output
+ scores before softmax.
+ - last_bn (bool): Whether to use BN on the last output of mlps.
+ """
+
+ def __init__(self,
+ num_point,
+ radii,
+ sample_nums,
+ mlp_channels,
+ paconv_num_kernels,
+ fps_mod=['D-FPS'],
+ fps_sample_range_list=[-1],
+ dilated_group=False,
+ norm_cfg=dict(type='BN2d', momentum=0.1),
+ use_xyz=True,
+ pool_mod='max',
+ normalize_xyz=False,
+ bias='auto',
+ paconv_kernel_input='w_neighbor',
+ scorenet_input='w_neighbor_dist',
+ scorenet_cfg=dict(
+ mlp_channels=[16, 16, 16],
+ score_norm='softmax',
+ temp_factor=1.0,
+ last_bn=False)):
+ super(PAConvSAModuleMSG, self).__init__(
+ num_point=num_point,
+ radii=radii,
+ sample_nums=sample_nums,
+ mlp_channels=mlp_channels,
+ fps_mod=fps_mod,
+ fps_sample_range_list=fps_sample_range_list,
+ dilated_group=dilated_group,
+ use_xyz=use_xyz,
+ pool_mod=pool_mod,
+ normalize_xyz=normalize_xyz,
+ grouper_return_grouped_xyz=True)
+
+ assert len(paconv_num_kernels) == len(mlp_channels)
+ for i in range(len(mlp_channels)):
+ assert len(paconv_num_kernels[i]) == len(mlp_channels[i]) - 1, \
+ 'PAConv number of kernel weights wrong'
+
+ # in PAConv, bias only exists in ScoreNet
+ scorenet_cfg['bias'] = bias
+
+ for i in range(len(self.mlp_channels)):
+ mlp_channel = self.mlp_channels[i]
+ if use_xyz:
+ mlp_channel[0] += 3
+
+ num_kernels = paconv_num_kernels[i]
+
+ mlp = nn.Sequential()
+ for i in range(len(mlp_channel) - 1):
+ mlp.add_module(
+ f'layer{i}',
+ PAConv(
+ mlp_channel[i],
+ mlp_channel[i + 1],
+ num_kernels[i],
+ norm_cfg=norm_cfg,
+ kernel_input=paconv_kernel_input,
+ scorenet_input=scorenet_input,
+ scorenet_cfg=scorenet_cfg))
+ self.mlps.append(mlp)
+
+
+@SA_MODULES.register_module()
+class PAConvSAModule(PAConvSAModuleMSG):
+ r"""Point set abstraction module with single-scale grouping (SSG) used in
+ PAConv networks.
+
+ Replace the MLPs in `PointSAModule` with PAConv layers. See the `paper
+ `_ for more details.
+ """
+
+ def __init__(self,
+ mlp_channels,
+ paconv_num_kernels,
+ num_point=None,
+ radius=None,
+ num_sample=None,
+ norm_cfg=dict(type='BN2d', momentum=0.1),
+ use_xyz=True,
+ pool_mod='max',
+ fps_mod=['D-FPS'],
+ fps_sample_range_list=[-1],
+ normalize_xyz=False,
+ paconv_kernel_input='w_neighbor',
+ scorenet_input='w_neighbor_dist',
+ scorenet_cfg=dict(
+ mlp_channels=[16, 16, 16],
+ score_norm='softmax',
+ temp_factor=1.0,
+ last_bn=False)):
+ super(PAConvSAModule, self).__init__(
+ mlp_channels=[mlp_channels],
+ paconv_num_kernels=[paconv_num_kernels],
+ num_point=num_point,
+ radii=[radius],
+ sample_nums=[num_sample],
+ norm_cfg=norm_cfg,
+ use_xyz=use_xyz,
+ pool_mod=pool_mod,
+ fps_mod=fps_mod,
+ fps_sample_range_list=fps_sample_range_list,
+ normalize_xyz=normalize_xyz,
+ paconv_kernel_input=paconv_kernel_input,
+ scorenet_input=scorenet_input,
+ scorenet_cfg=scorenet_cfg)
+
+
+@SA_MODULES.register_module()
+class PAConvCUDASAModuleMSG(BasePointSAModule):
+ r"""Point set abstraction module with multi-scale grouping (MSG) used in
+ PAConv networks.
+
+ Replace the non CUDA version PAConv with CUDA implemented PAConv for
+ efficient computation. See the `paper `_
+ for more details.
+ """
+
+ def __init__(self,
+ num_point,
+ radii,
+ sample_nums,
+ mlp_channels,
+ paconv_num_kernels,
+ fps_mod=['D-FPS'],
+ fps_sample_range_list=[-1],
+ dilated_group=False,
+ norm_cfg=dict(type='BN2d', momentum=0.1),
+ use_xyz=True,
+ pool_mod='max',
+ normalize_xyz=False,
+ bias='auto',
+ paconv_kernel_input='w_neighbor',
+ scorenet_input='w_neighbor_dist',
+ scorenet_cfg=dict(
+ mlp_channels=[8, 16, 16],
+ score_norm='softmax',
+ temp_factor=1.0,
+ last_bn=False)):
+ super(PAConvCUDASAModuleMSG, self).__init__(
+ num_point=num_point,
+ radii=radii,
+ sample_nums=sample_nums,
+ mlp_channels=mlp_channels,
+ fps_mod=fps_mod,
+ fps_sample_range_list=fps_sample_range_list,
+ dilated_group=dilated_group,
+ use_xyz=use_xyz,
+ pool_mod=pool_mod,
+ normalize_xyz=normalize_xyz,
+ grouper_return_grouped_xyz=True,
+ grouper_return_grouped_idx=True)
+
+ assert len(paconv_num_kernels) == len(mlp_channels)
+ for i in range(len(mlp_channels)):
+ assert len(paconv_num_kernels[i]) == len(mlp_channels[i]) - 1, \
+ 'PAConv number of kernel weights wrong'
+
+ # in PAConv, bias only exists in ScoreNet
+ scorenet_cfg['bias'] = bias
+
+ # we need to manually concat xyz for CUDA implemented PAConv
+ self.use_xyz = use_xyz
+
+ for i in range(len(self.mlp_channels)):
+ mlp_channel = self.mlp_channels[i]
+ if use_xyz:
+ mlp_channel[0] += 3
+
+ num_kernels = paconv_num_kernels[i]
+
+ # can't use `nn.Sequential` for PAConvCUDA because its input and
+ # output have different shapes
+ mlp = nn.ModuleList()
+ for i in range(len(mlp_channel) - 1):
+ mlp.append(
+ PAConvCUDA(
+ mlp_channel[i],
+ mlp_channel[i + 1],
+ num_kernels[i],
+ norm_cfg=norm_cfg,
+ kernel_input=paconv_kernel_input,
+ scorenet_input=scorenet_input,
+ scorenet_cfg=scorenet_cfg))
+ self.mlps.append(mlp)
+
+ def forward(
+ self,
+ points_xyz,
+ features=None,
+ indices=None,
+ target_xyz=None,
+ ):
+ """forward.
+
+ Args:
+ points_xyz (Tensor): (B, N, 3) xyz coordinates of the features.
+ features (Tensor, optional): (B, C, N) features of each point.
+ Default: None.
+ indices (Tensor, optional): (B, num_point) Index of the features.
+ Default: None.
+ target_xyz (Tensor, optional): (B, M, 3) new coords of the outputs.
+ Default: None.
+
+ Returns:
+ Tensor: (B, M, 3) where M is the number of points.
+ New features xyz.
+ Tensor: (B, M, sum_k(mlps[k][-1])) where M is the number
+ of points. New feature descriptors.
+ Tensor: (B, M) where M is the number of points.
+ Index of the features.
+ """
+ new_features_list = []
+
+ # sample points, (B, num_point, 3), (B, num_point)
+ new_xyz, indices = self._sample_points(points_xyz, features, indices,
+ target_xyz)
+
+ for i in range(len(self.groupers)):
+ xyz = points_xyz
+ new_features = features
+ for j in range(len(self.mlps[i])):
+ # we don't use grouped_features here to avoid large GPU memory
+ # _, (B, 3, num_point, nsample), (B, num_point, nsample)
+ _, grouped_xyz, grouped_idx = self.groupers[i](xyz, new_xyz,
+ new_features)
+
+ # concat xyz as additional features
+ if self.use_xyz and j == 0:
+ # (B, C+3, N)
+ new_features = torch.cat(
+ (points_xyz.permute(0, 2, 1), new_features), dim=1)
+
+ # (B, out_c, num_point, nsample)
+ grouped_new_features = self.mlps[i][j](
+ (new_features, grouped_xyz, grouped_idx.long()))[0]
+
+ # different from PointNet++ and non CUDA version of PAConv
+ # CUDA version of PAConv needs to aggregate local features
+ # every time after it passes through a Conv layer
+ # in order to transform to valid input shape
+ # (B, out_c, num_point)
+ new_features = self._pool_features(grouped_new_features)
+
+ # constrain the points to be grouped for next PAConv layer
+ # because new_features only contains sampled centers now
+ # (B, num_point, 3)
+ xyz = new_xyz
+
+ new_features_list.append(new_features)
+
+ return new_xyz, torch.cat(new_features_list, dim=1), indices
+
+
+@SA_MODULES.register_module()
+class PAConvCUDASAModule(PAConvCUDASAModuleMSG):
+ r"""Point set abstraction module with single-scale grouping (SSG) used in
+ PAConv networks.
+
+ Replace the non CUDA version PAConv with CUDA implemented PAConv for
+ efficient computation. See the `paper `_
+ for more details.
+ """
+
+ def __init__(self,
+ mlp_channels,
+ paconv_num_kernels,
+ num_point=None,
+ radius=None,
+ num_sample=None,
+ norm_cfg=dict(type='BN2d', momentum=0.1),
+ use_xyz=True,
+ pool_mod='max',
+ fps_mod=['D-FPS'],
+ fps_sample_range_list=[-1],
+ normalize_xyz=False,
+ paconv_kernel_input='w_neighbor',
+ scorenet_input='w_neighbor_dist',
+ scorenet_cfg=dict(
+ mlp_channels=[8, 16, 16],
+ score_norm='softmax',
+ temp_factor=1.0,
+ last_bn=False)):
+ super(PAConvCUDASAModule, self).__init__(
+ mlp_channels=[mlp_channels],
+ paconv_num_kernels=[paconv_num_kernels],
+ num_point=num_point,
+ radii=[radius],
+ sample_nums=[num_sample],
+ norm_cfg=norm_cfg,
+ use_xyz=use_xyz,
+ pool_mod=pool_mod,
+ fps_mod=fps_mod,
+ fps_sample_range_list=fps_sample_range_list,
+ normalize_xyz=normalize_xyz,
+ paconv_kernel_input=paconv_kernel_input,
+ scorenet_input=scorenet_input,
+ scorenet_cfg=scorenet_cfg)
diff --git a/mmdet3d/ops/pointnet_modules/point_fp_module.py b/mmdet3d/ops/pointnet_modules/point_fp_module.py
new file mode 100644
index 0000000..1bc833e
--- /dev/null
+++ b/mmdet3d/ops/pointnet_modules/point_fp_module.py
@@ -0,0 +1,79 @@
+# Copyright (c) OpenMMLab. All rights reserved.
+from typing import List
+
+import torch
+from mmcv.cnn import ConvModule
+from mmcv.ops import three_interpolate, three_nn
+from mmcv.runner import BaseModule, force_fp32
+from torch import nn as nn
+
+
+class PointFPModule(BaseModule):
+ """Point feature propagation module used in PointNets.
+
+ Propagate the features from one set to another.
+
+ Args:
+ mlp_channels (list[int]): List of mlp channels.
+ norm_cfg (dict, optional): Type of normalization method.
+ Default: dict(type='BN2d').
+ """
+
+ def __init__(self,
+ mlp_channels: List[int],
+ norm_cfg: dict = dict(type='BN2d'),
+ init_cfg=None):
+ super().__init__(init_cfg=init_cfg)
+ self.fp16_enabled = False
+ self.mlps = nn.Sequential()
+ for i in range(len(mlp_channels) - 1):
+ self.mlps.add_module(
+ f'layer{i}',
+ ConvModule(
+ mlp_channels[i],
+ mlp_channels[i + 1],
+ kernel_size=(1, 1),
+ stride=(1, 1),
+ conv_cfg=dict(type='Conv2d'),
+ norm_cfg=norm_cfg))
+
+ @force_fp32()
+ def forward(self, target: torch.Tensor, source: torch.Tensor,
+ target_feats: torch.Tensor,
+ source_feats: torch.Tensor) -> torch.Tensor:
+ """forward.
+
+ Args:
+ target (Tensor): (B, n, 3) tensor of the xyz positions of
+ the target features.
+ source (Tensor): (B, m, 3) tensor of the xyz positions of
+ the source features.
+ target_feats (Tensor): (B, C1, n) tensor of the features to be
+ propagated to.
+ source_feats (Tensor): (B, C2, m) tensor of features
+ to be propagated.
+
+ Return:
+ Tensor: (B, M, N) M = mlp[-1], tensor of the target features.
+ """
+ if source is not None:
+ dist, idx = three_nn(target, source)
+ dist_reciprocal = 1.0 / (dist + 1e-8)
+ norm = torch.sum(dist_reciprocal, dim=2, keepdim=True)
+ weight = dist_reciprocal / norm
+
+ interpolated_feats = three_interpolate(source_feats, idx, weight)
+ else:
+ interpolated_feats = source_feats.expand(*source_feats.size()[0:2],
+ target.size(1))
+
+ if target_feats is not None:
+ new_features = torch.cat([interpolated_feats, target_feats],
+ dim=1) # (B, C2 + C1, n)
+ else:
+ new_features = interpolated_feats
+
+ new_features = new_features.unsqueeze(-1)
+ new_features = self.mlps(new_features)
+
+ return new_features.squeeze(-1)
diff --git a/mmdet3d/ops/pointnet_modules/point_sa_module.py b/mmdet3d/ops/pointnet_modules/point_sa_module.py
new file mode 100644
index 0000000..e33377f
--- /dev/null
+++ b/mmdet3d/ops/pointnet_modules/point_sa_module.py
@@ -0,0 +1,352 @@
+# Copyright (c) OpenMMLab. All rights reserved.
+import torch
+from mmcv.cnn import ConvModule
+from mmcv.ops import GroupAll
+from mmcv.ops import PointsSampler as Points_Sampler
+from mmcv.ops import QueryAndGroup, gather_points
+from torch import nn as nn
+from torch.nn import functional as F
+
+from mmdet3d.ops import PAConv
+from .builder import SA_MODULES
+
+
+class BasePointSAModule(nn.Module):
+ """Base module for point set abstraction module used in PointNets.
+
+ Args:
+ num_point (int): Number of points.
+ radii (list[float]): List of radius in each ball query.
+ sample_nums (list[int]): Number of samples in each ball query.
+ mlp_channels (list[list[int]]): Specify of the pointnet before
+ the global pooling for each scale.
+ fps_mod (list[str], optional): Type of FPS method, valid mod
+ ['F-FPS', 'D-FPS', 'FS'], Default: ['D-FPS'].
+ F-FPS: using feature distances for FPS.
+ D-FPS: using Euclidean distances of points for FPS.
+ FS: using F-FPS and D-FPS simultaneously.
+ fps_sample_range_list (list[int], optional):
+ Range of points to apply FPS. Default: [-1].
+ dilated_group (bool, optional): Whether to use dilated ball query.
+ Default: False.
+ use_xyz (bool, optional): Whether to use xyz.
+ Default: True.
+ pool_mod (str, optional): Type of pooling method.
+ Default: 'max_pool'.
+ normalize_xyz (bool, optional): Whether to normalize local XYZ
+ with radius. Default: False.
+ grouper_return_grouped_xyz (bool, optional): Whether to return
+ grouped xyz in `QueryAndGroup`. Defaults to False.
+ grouper_return_grouped_idx (bool, optional): Whether to return
+ grouped idx in `QueryAndGroup`. Defaults to False.
+ """
+
+ def __init__(self,
+ num_point,
+ radii,
+ sample_nums,
+ mlp_channels,
+ fps_mod=['D-FPS'],
+ fps_sample_range_list=[-1],
+ dilated_group=False,
+ use_xyz=True,
+ pool_mod='max',
+ normalize_xyz=False,
+ grouper_return_grouped_xyz=False,
+ grouper_return_grouped_idx=False):
+ super(BasePointSAModule, self).__init__()
+
+ assert len(radii) == len(sample_nums) == len(mlp_channels)
+ assert pool_mod in ['max', 'avg']
+ assert isinstance(fps_mod, list) or isinstance(fps_mod, tuple)
+ assert isinstance(fps_sample_range_list, list) or isinstance(
+ fps_sample_range_list, tuple)
+ assert len(fps_mod) == len(fps_sample_range_list)
+
+ if isinstance(mlp_channels, tuple):
+ mlp_channels = list(map(list, mlp_channels))
+ self.mlp_channels = mlp_channels
+
+ if isinstance(num_point, int):
+ self.num_point = [num_point]
+ elif isinstance(num_point, list) or isinstance(num_point, tuple):
+ self.num_point = num_point
+ elif num_point is None:
+ self.num_point = None
+ else:
+ raise NotImplementedError('Error type of num_point!')
+
+ self.pool_mod = pool_mod
+ self.groupers = nn.ModuleList()
+ self.mlps = nn.ModuleList()
+ self.fps_mod_list = fps_mod
+ self.fps_sample_range_list = fps_sample_range_list
+
+ if self.num_point is not None:
+ self.points_sampler = Points_Sampler(self.num_point,
+ self.fps_mod_list,
+ self.fps_sample_range_list)
+ else:
+ self.points_sampler = None
+
+ for i in range(len(radii)):
+ radius = radii[i]
+ sample_num = sample_nums[i]
+ if num_point is not None:
+ if dilated_group and i != 0:
+ min_radius = radii[i - 1]
+ else:
+ min_radius = 0
+ grouper = QueryAndGroup(
+ radius,
+ sample_num,
+ min_radius=min_radius,
+ use_xyz=use_xyz,
+ normalize_xyz=normalize_xyz,
+ return_grouped_xyz=grouper_return_grouped_xyz,
+ return_grouped_idx=grouper_return_grouped_idx)
+ else:
+ grouper = GroupAll(use_xyz)
+ self.groupers.append(grouper)
+
+ def _sample_points(self, points_xyz, features, indices, target_xyz):
+ """Perform point sampling based on inputs.
+
+ If `indices` is specified, directly sample corresponding points.
+ Else if `target_xyz` is specified, use is as sampled points.
+ Otherwise sample points using `self.points_sampler`.
+
+ Args:
+ points_xyz (Tensor): (B, N, 3) xyz coordinates of the features.
+ features (Tensor): (B, C, N) features of each point.
+ indices (Tensor): (B, num_point) Index of the features.
+ target_xyz (Tensor): (B, M, 3) new_xyz coordinates of the outputs.
+
+ Returns:
+ Tensor: (B, num_point, 3) sampled xyz coordinates of points.
+ Tensor: (B, num_point) sampled points' index.
+ """
+ xyz_flipped = points_xyz.transpose(1, 2).contiguous()
+ if indices is not None:
+ assert (indices.shape[1] == self.num_point[0])
+ new_xyz = gather_points(xyz_flipped, indices).transpose(
+ 1, 2).contiguous() if self.num_point is not None else None
+ elif target_xyz is not None:
+ new_xyz = target_xyz.contiguous()
+ else:
+ if self.num_point is not None:
+ indices = self.points_sampler(points_xyz, features)
+ new_xyz = gather_points(xyz_flipped,
+ indices).transpose(1, 2).contiguous()
+ else:
+ new_xyz = None
+
+ return new_xyz, indices
+
+ def _pool_features(self, features):
+ """Perform feature aggregation using pooling operation.
+
+ Args:
+ features (torch.Tensor): (B, C, N, K)
+ Features of locally grouped points before pooling.
+
+ Returns:
+ torch.Tensor: (B, C, N)
+ Pooled features aggregating local information.
+ """
+ if self.pool_mod == 'max':
+ # (B, C, N, 1)
+ new_features = F.max_pool2d(
+ features, kernel_size=[1, features.size(3)])
+ elif self.pool_mod == 'avg':
+ # (B, C, N, 1)
+ new_features = F.avg_pool2d(
+ features, kernel_size=[1, features.size(3)])
+ else:
+ raise NotImplementedError
+
+ return new_features.squeeze(-1).contiguous()
+
+ def forward(
+ self,
+ points_xyz,
+ features=None,
+ indices=None,
+ target_xyz=None,
+ ):
+ """forward.
+
+ Args:
+ points_xyz (Tensor): (B, N, 3) xyz coordinates of the features.
+ features (Tensor, optional): (B, C, N) features of each point.
+ Default: None.
+ indices (Tensor, optional): (B, num_point) Index of the features.
+ Default: None.
+ target_xyz (Tensor, optional): (B, M, 3) new coords of the outputs.
+ Default: None.
+
+ Returns:
+ Tensor: (B, M, 3) where M is the number of points.
+ New features xyz.
+ Tensor: (B, M, sum_k(mlps[k][-1])) where M is the number
+ of points. New feature descriptors.
+ Tensor: (B, M) where M is the number of points.
+ Index of the features.
+ """
+ new_features_list = []
+
+ # sample points, (B, num_point, 3), (B, num_point)
+ new_xyz, indices = self._sample_points(points_xyz, features, indices,
+ target_xyz)
+
+ for i in range(len(self.groupers)):
+ # grouped_results may contain:
+ # - grouped_features: (B, C, num_point, nsample)
+ # - grouped_xyz: (B, 3, num_point, nsample)
+ # - grouped_idx: (B, num_point, nsample)
+ grouped_results = self.groupers[i](points_xyz, new_xyz, features)
+
+ # (B, mlp[-1], num_point, nsample)
+ new_features = self.mlps[i](grouped_results)
+
+ # this is a bit hack because PAConv outputs two values
+ # we take the first one as feature
+ if isinstance(self.mlps[i][0], PAConv):
+ assert isinstance(new_features, tuple)
+ new_features = new_features[0]
+
+ # (B, mlp[-1], num_point)
+ new_features = self._pool_features(new_features)
+ new_features_list.append(new_features)
+
+ return new_xyz, torch.cat(new_features_list, dim=1), indices
+
+
+@SA_MODULES.register_module()
+class PointSAModuleMSG(BasePointSAModule):
+ """Point set abstraction module with multi-scale grouping (MSG) used in
+ PointNets.
+
+ Args:
+ num_point (int): Number of points.
+ radii (list[float]): List of radius in each ball query.
+ sample_nums (list[int]): Number of samples in each ball query.
+ mlp_channels (list[list[int]]): Specify of the pointnet before
+ the global pooling for each scale.
+ fps_mod (list[str], optional): Type of FPS method, valid mod
+ ['F-FPS', 'D-FPS', 'FS'], Default: ['D-FPS'].
+ F-FPS: using feature distances for FPS.
+ D-FPS: using Euclidean distances of points for FPS.
+ FS: using F-FPS and D-FPS simultaneously.
+ fps_sample_range_list (list[int], optional): Range of points to
+ apply FPS. Default: [-1].
+ dilated_group (bool, optional): Whether to use dilated ball query.
+ Default: False.
+ norm_cfg (dict, optional): Type of normalization method.
+ Default: dict(type='BN2d').
+ use_xyz (bool, optional): Whether to use xyz.
+ Default: True.
+ pool_mod (str, optional): Type of pooling method.
+ Default: 'max_pool'.
+ normalize_xyz (bool, optional): Whether to normalize local XYZ
+ with radius. Default: False.
+ bias (bool | str, optional): If specified as `auto`, it will be
+ decided by `norm_cfg`. `bias` will be set as True if
+ `norm_cfg` is None, otherwise False. Default: 'auto'.
+ """
+
+ def __init__(self,
+ num_point,
+ radii,
+ sample_nums,
+ mlp_channels,
+ fps_mod=['D-FPS'],
+ fps_sample_range_list=[-1],
+ dilated_group=False,
+ norm_cfg=dict(type='BN2d'),
+ use_xyz=True,
+ pool_mod='max',
+ normalize_xyz=False,
+ bias='auto'):
+ super(PointSAModuleMSG, self).__init__(
+ num_point=num_point,
+ radii=radii,
+ sample_nums=sample_nums,
+ mlp_channels=mlp_channels,
+ fps_mod=fps_mod,
+ fps_sample_range_list=fps_sample_range_list,
+ dilated_group=dilated_group,
+ use_xyz=use_xyz,
+ pool_mod=pool_mod,
+ normalize_xyz=normalize_xyz)
+
+ for i in range(len(self.mlp_channels)):
+ mlp_channel = self.mlp_channels[i]
+ if use_xyz:
+ mlp_channel[0] += 3
+
+ mlp = nn.Sequential()
+ for i in range(len(mlp_channel) - 1):
+ mlp.add_module(
+ f'layer{i}',
+ ConvModule(
+ mlp_channel[i],
+ mlp_channel[i + 1],
+ kernel_size=(1, 1),
+ stride=(1, 1),
+ conv_cfg=dict(type='Conv2d'),
+ norm_cfg=norm_cfg,
+ bias=bias))
+ self.mlps.append(mlp)
+
+
+@SA_MODULES.register_module()
+class PointSAModule(PointSAModuleMSG):
+ """Point set abstraction module with single-scale grouping (SSG) used in
+ PointNets.
+
+ Args:
+ mlp_channels (list[int]): Specify of the pointnet before
+ the global pooling for each scale.
+ num_point (int, optional): Number of points.
+ Default: None.
+ radius (float, optional): Radius to group with.
+ Default: None.
+ num_sample (int, optional): Number of samples in each ball query.
+ Default: None.
+ norm_cfg (dict, optional): Type of normalization method.
+ Default: dict(type='BN2d').
+ use_xyz (bool, optional): Whether to use xyz.
+ Default: True.
+ pool_mod (str, optional): Type of pooling method.
+ Default: 'max_pool'.
+ fps_mod (list[str], optional): Type of FPS method, valid mod
+ ['F-FPS', 'D-FPS', 'FS'], Default: ['D-FPS'].
+ fps_sample_range_list (list[int], optional): Range of points
+ to apply FPS. Default: [-1].
+ normalize_xyz (bool, optional): Whether to normalize local XYZ
+ with radius. Default: False.
+ """
+
+ def __init__(self,
+ mlp_channels,
+ num_point=None,
+ radius=None,
+ num_sample=None,
+ norm_cfg=dict(type='BN2d'),
+ use_xyz=True,
+ pool_mod='max',
+ fps_mod=['D-FPS'],
+ fps_sample_range_list=[-1],
+ normalize_xyz=False):
+ super(PointSAModule, self).__init__(
+ mlp_channels=[mlp_channels],
+ num_point=num_point,
+ radii=[radius],
+ sample_nums=[num_sample],
+ norm_cfg=norm_cfg,
+ use_xyz=use_xyz,
+ pool_mod=pool_mod,
+ fps_mod=fps_mod,
+ fps_sample_range_list=fps_sample_range_list,
+ normalize_xyz=normalize_xyz)
diff --git a/mmdet3d/ops/sparse_block.py b/mmdet3d/ops/sparse_block.py
new file mode 100644
index 0000000..03b18e2
--- /dev/null
+++ b/mmdet3d/ops/sparse_block.py
@@ -0,0 +1,199 @@
+# Copyright (c) OpenMMLab. All rights reserved.
+from mmcv.cnn import build_conv_layer, build_norm_layer
+from torch import nn
+
+from mmdet.models.backbones.resnet import BasicBlock, Bottleneck
+from .spconv import IS_SPCONV2_AVAILABLE
+
+if IS_SPCONV2_AVAILABLE:
+ from spconv.pytorch import SparseModule, SparseSequential
+else:
+ from mmcv.ops import SparseModule, SparseSequential
+
+
+def replace_feature(out, new_features):
+ if 'replace_feature' in out.__dir__():
+ # spconv 2.x behaviour
+ return out.replace_feature(new_features)
+ else:
+ out.features = new_features
+ return out
+
+
+class SparseBottleneck(Bottleneck, SparseModule):
+ """Sparse bottleneck block for PartA^2.
+
+ Bottleneck block implemented with submanifold sparse convolution.
+
+ Args:
+ inplanes (int): inplanes of block.
+ planes (int): planes of block.
+ stride (int, optional): stride of the first block. Default: 1.
+ downsample (Module, optional): down sample module for block.
+ conv_cfg (dict, optional): dictionary to construct and config conv
+ layer. Default: None.
+ norm_cfg (dict, optional): dictionary to construct and config norm
+ layer. Default: dict(type='BN').
+ """
+
+ expansion = 4
+
+ def __init__(self,
+ inplanes,
+ planes,
+ stride=1,
+ downsample=None,
+ conv_cfg=None,
+ norm_cfg=None):
+
+ SparseModule.__init__(self)
+ Bottleneck.__init__(
+ self,
+ inplanes,
+ planes,
+ stride=stride,
+ downsample=downsample,
+ conv_cfg=conv_cfg,
+ norm_cfg=norm_cfg)
+
+ def forward(self, x):
+ identity = x.features
+
+ out = self.conv1(x)
+ out = replace_feature(out, self.bn1(out.features))
+ out = replace_feature(out, self.relu(out.features))
+
+ out = self.conv2(out)
+ out = replace_feature(out, self.bn2(out.features))
+ out = replace_feature(out, self.relu(out.features))
+
+ out = self.conv3(out)
+ out = replace_feature(out, self.bn3(out.features))
+
+ if self.downsample is not None:
+ identity = self.downsample(x)
+
+ out = replace_feature(out, out.features + identity)
+ out = replace_feature(out, self.relu(out.features))
+
+ return out
+
+
+class SparseBasicBlock(BasicBlock, SparseModule):
+ """Sparse basic block for PartA^2.
+
+ Sparse basic block implemented with submanifold sparse convolution.
+
+ Args:
+ inplanes (int): inplanes of block.
+ planes (int): planes of block.
+ stride (int, optional): stride of the first block. Default: 1.
+ downsample (Module, optional): down sample module for block.
+ conv_cfg (dict, optional): dictionary to construct and config conv
+ layer. Default: None.
+ norm_cfg (dict, optional): dictionary to construct and config norm
+ layer. Default: dict(type='BN').
+ """
+
+ expansion = 1
+
+ def __init__(self,
+ inplanes,
+ planes,
+ stride=1,
+ downsample=None,
+ conv_cfg=None,
+ norm_cfg=None):
+ SparseModule.__init__(self)
+ BasicBlock.__init__(
+ self,
+ inplanes,
+ planes,
+ stride=stride,
+ downsample=downsample,
+ conv_cfg=conv_cfg,
+ norm_cfg=norm_cfg)
+
+ def forward(self, x):
+ identity = x.features
+
+ assert x.features.dim() == 2, f'x.features.dim()={x.features.dim()}'
+ out = self.conv1(x)
+ out = replace_feature(out, self.norm1(out.features))
+ out = replace_feature(out, self.relu(out.features))
+
+ out = self.conv2(out)
+ out = replace_feature(out, self.norm2(out.features))
+
+ if self.downsample is not None:
+ identity = self.downsample(x)
+
+ out = replace_feature(out, out.features + identity)
+ out = replace_feature(out, self.relu(out.features))
+
+ return out
+
+
+def make_sparse_convmodule(in_channels,
+ out_channels,
+ kernel_size,
+ indice_key,
+ stride=1,
+ padding=0,
+ conv_type='SubMConv3d',
+ norm_cfg=None,
+ order=('conv', 'norm', 'act')):
+ """Make sparse convolution module.
+
+ Args:
+ in_channels (int): the number of input channels
+ out_channels (int): the number of out channels
+ kernel_size (int|tuple(int)): kernel size of convolution
+ indice_key (str): the indice key used for sparse tensor
+ stride (int|tuple(int)): the stride of convolution
+ padding (int or list[int]): the padding number of input
+ conv_type (str): sparse conv type in spconv
+ norm_cfg (dict[str]): config of normalization layer
+ order (tuple[str]): The order of conv/norm/activation layers. It is a
+ sequence of "conv", "norm" and "act". Common examples are
+ ("conv", "norm", "act") and ("act", "conv", "norm").
+
+ Returns:
+ spconv.SparseSequential: sparse convolution module.
+ """
+ assert isinstance(order, tuple) and len(order) <= 3
+ assert set(order) | {'conv', 'norm', 'act'} == {'conv', 'norm', 'act'}
+
+ conv_cfg = dict(type=conv_type, indice_key=indice_key)
+
+ layers = list()
+ for layer in order:
+ if layer == 'conv':
+ if conv_type not in [
+ 'SparseInverseConv3d', 'SparseInverseConv2d',
+ 'SparseInverseConv1d'
+ ]:
+ layers.append(
+ build_conv_layer(
+ conv_cfg,
+ in_channels,
+ out_channels,
+ kernel_size,
+ stride=stride,
+ padding=padding,
+ bias=False))
+ else:
+ layers.append(
+ build_conv_layer(
+ conv_cfg,
+ in_channels,
+ out_channels,
+ kernel_size,
+ bias=False))
+ elif layer == 'norm':
+ layers.append(build_norm_layer(norm_cfg, out_channels)[1])
+ elif layer == 'act':
+ layers.append(nn.ReLU(inplace=True))
+
+ layers = SparseSequential(*layers)
+ return layers
diff --git a/mmdet3d/ops/spconv/__init__.py b/mmdet3d/ops/spconv/__init__.py
new file mode 100644
index 0000000..561e502
--- /dev/null
+++ b/mmdet3d/ops/spconv/__init__.py
@@ -0,0 +1,14 @@
+# Copyright (c) OpenMMLab. All rights reserved.
+from .overwrite_spconv.write_spconv2 import register_spconv2
+
+try:
+ import spconv
+except ImportError:
+ IS_SPCONV2_AVAILABLE = False
+else:
+ if hasattr(spconv, '__version__') and spconv.__version__ >= '2.0.0':
+ IS_SPCONV2_AVAILABLE = register_spconv2()
+ else:
+ IS_SPCONV2_AVAILABLE = False
+
+__all__ = ['IS_SPCONV2_AVAILABLE']
diff --git a/mmdet3d/ops/spconv/overwrite_spconv/__init__.py b/mmdet3d/ops/spconv/overwrite_spconv/__init__.py
new file mode 100644
index 0000000..2e93d9c
--- /dev/null
+++ b/mmdet3d/ops/spconv/overwrite_spconv/__init__.py
@@ -0,0 +1,4 @@
+# Copyright (c) OpenMMLab. All rights reserved.
+from .write_spconv2 import register_spconv2
+
+__all__ = ['register_spconv2']
diff --git a/mmdet3d/ops/spconv/overwrite_spconv/write_spconv2.py b/mmdet3d/ops/spconv/overwrite_spconv/write_spconv2.py
new file mode 100644
index 0000000..237051e
--- /dev/null
+++ b/mmdet3d/ops/spconv/overwrite_spconv/write_spconv2.py
@@ -0,0 +1,118 @@
+# Copyright (c) OpenMMLab. All rights reserved.
+import itertools
+
+from mmcv.cnn.bricks.registry import CONV_LAYERS
+from torch.nn.parameter import Parameter
+
+
+def register_spconv2():
+ """This func registers spconv2.0 spconv ops to overwrite the default mmcv
+ spconv ops."""
+ try:
+ from spconv.pytorch import (SparseConv2d, SparseConv3d, SparseConv4d,
+ SparseConvTranspose2d,
+ SparseConvTranspose3d, SparseInverseConv2d,
+ SparseInverseConv3d, SparseModule,
+ SubMConv2d, SubMConv3d, SubMConv4d)
+ except ImportError:
+ return False
+ else:
+ CONV_LAYERS._register_module(SparseConv2d, 'SparseConv2d', force=True)
+ CONV_LAYERS._register_module(SparseConv3d, 'SparseConv3d', force=True)
+ CONV_LAYERS._register_module(SparseConv4d, 'SparseConv4d', force=True)
+
+ CONV_LAYERS._register_module(
+ SparseConvTranspose2d, 'SparseConvTranspose2d', force=True)
+ CONV_LAYERS._register_module(
+ SparseConvTranspose3d, 'SparseConvTranspose3d', force=True)
+
+ CONV_LAYERS._register_module(
+ SparseInverseConv2d, 'SparseInverseConv2d', force=True)
+ CONV_LAYERS._register_module(
+ SparseInverseConv3d, 'SparseInverseConv3d', force=True)
+
+ CONV_LAYERS._register_module(SubMConv2d, 'SubMConv2d', force=True)
+ CONV_LAYERS._register_module(SubMConv3d, 'SubMConv3d', force=True)
+ CONV_LAYERS._register_module(SubMConv4d, 'SubMConv4d', force=True)
+ SparseModule._load_from_state_dict = _load_from_state_dict
+ SparseModule._save_to_state_dict = _save_to_state_dict
+ return True
+
+
+def _save_to_state_dict(self, destination, prefix, keep_vars):
+ """Rewrite this func to compat the convolutional kernel weights between
+ spconv 1.x in MMCV and 2.x in spconv2.x.
+
+ Kernel weights in MMCV spconv has shape in (D,H,W,in_channel,out_channel) ,
+ while those in spcon2.x is in (out_channel,D,H,W,in_channel).
+ """
+ for name, param in self._parameters.items():
+ if param is not None:
+ param = param if keep_vars else param.detach()
+ if name == 'weight':
+ dims = list(range(1, len(param.shape))) + [0]
+ param = param.permute(*dims)
+ destination[prefix + name] = param
+ for name, buf in self._buffers.items():
+ if buf is not None and name not in self._non_persistent_buffers_set:
+ destination[prefix + name] = buf if keep_vars else buf.detach()
+
+
+def _load_from_state_dict(self, state_dict, prefix, local_metadata, strict,
+ missing_keys, unexpected_keys, error_msgs):
+ """Rewrite this func to compat the convolutional kernel weights between
+ spconv 1.x in MMCV and 2.x in spconv2.x.
+
+ Kernel weights in MMCV spconv has shape in (D,H,W,in_channel,out_channel) ,
+ while those in spcon2.x is in (out_channel,D,H,W,in_channel).
+ """
+ for hook in self._load_state_dict_pre_hooks.values():
+ hook(state_dict, prefix, local_metadata, strict, missing_keys,
+ unexpected_keys, error_msgs)
+
+ local_name_params = itertools.chain(self._parameters.items(),
+ self._buffers.items())
+ local_state = {k: v.data for k, v in local_name_params if v is not None}
+
+ for name, param in local_state.items():
+ key = prefix + name
+ if key in state_dict:
+ input_param = state_dict[key]
+
+ # Backward compatibility: loading 1-dim tensor from
+ # 0.3.* to version 0.4+
+ if len(param.shape) == 0 and len(input_param.shape) == 1:
+ input_param = input_param[0]
+ dims = [len(input_param.shape) - 1] + list(
+ range(len(input_param.shape) - 1))
+ input_param = input_param.permute(*dims)
+ if input_param.shape != param.shape:
+ # local shape should match the one in checkpoint
+ error_msgs.append(
+ f'size mismatch for {key}: copying a param with '
+ f'shape {key, input_param.shape} from checkpoint,'
+ f'the shape in current model is {param.shape}.')
+ continue
+
+ if isinstance(input_param, Parameter):
+ # backwards compatibility for serialized parameters
+ input_param = input_param.data
+ try:
+ param.copy_(input_param)
+ except Exception:
+ error_msgs.append(
+ f'While copying the parameter named "{key}", whose '
+ f'dimensions in the model are {param.size()} and whose '
+ f'dimensions in the checkpoint are {input_param.size()}.')
+ elif strict:
+ missing_keys.append(key)
+
+ if strict:
+ for key, input_param in state_dict.items():
+ if key.startswith(prefix):
+ input_name = key[len(prefix):]
+ input_name = input_name.split(
+ '.', 1)[0] # get the name of param/buffer/child
+ if input_name not in self._modules \
+ and input_name not in local_state:
+ unexpected_keys.append(key)
diff --git a/mmdet3d/utils/__init__.py b/mmdet3d/utils/__init__.py
new file mode 100644
index 0000000..ad59961
--- /dev/null
+++ b/mmdet3d/utils/__init__.py
@@ -0,0 +1,14 @@
+# Copyright (c) OpenMMLab. All rights reserved.
+from mmcv.utils import Registry, build_from_cfg, print_log
+
+from .collect_env import collect_env
+from .compat_cfg import compat_cfg
+from .logger import get_root_logger
+from .misc import find_latest_checkpoint
+from .setup_env import setup_multi_processes
+
+__all__ = [
+ 'Registry', 'build_from_cfg', 'get_root_logger', 'collect_env',
+ 'print_log', 'setup_multi_processes', 'find_latest_checkpoint',
+ 'compat_cfg'
+]
diff --git a/mmdet3d/utils/collect_env.py b/mmdet3d/utils/collect_env.py
new file mode 100644
index 0000000..1131f12
--- /dev/null
+++ b/mmdet3d/utils/collect_env.py
@@ -0,0 +1,23 @@
+# Copyright (c) OpenMMLab. All rights reserved.
+from mmcv.utils import collect_env as collect_base_env
+from mmcv.utils import get_git_hash
+
+import mmdet
+import mmdet3d
+import mmseg
+from mmdet3d.ops.spconv import IS_SPCONV2_AVAILABLE
+
+
+def collect_env():
+ """Collect the information of the running environments."""
+ env_info = collect_base_env()
+ env_info['MMDetection'] = mmdet.__version__
+ env_info['MMSegmentation'] = mmseg.__version__
+ env_info['MMDetection3D'] = mmdet3d.__version__ + '+' + get_git_hash()[:7]
+ env_info['spconv2.0'] = IS_SPCONV2_AVAILABLE
+ return env_info
+
+
+if __name__ == '__main__':
+ for name, val in collect_env().items():
+ print(f'{name}: {val}')
diff --git a/mmdet3d/utils/compat_cfg.py b/mmdet3d/utils/compat_cfg.py
new file mode 100644
index 0000000..05aa37d
--- /dev/null
+++ b/mmdet3d/utils/compat_cfg.py
@@ -0,0 +1,139 @@
+# Copyright (c) OpenMMLab. All rights reserved.
+import copy
+import warnings
+
+from mmcv import ConfigDict
+
+
+def compat_cfg(cfg):
+ """This function would modify some filed to keep the compatibility of
+ config.
+
+ For example, it will move some args which will be deprecated to the correct
+ fields.
+ """
+ cfg = copy.deepcopy(cfg)
+ cfg = compat_imgs_per_gpu(cfg)
+ cfg = compat_loader_args(cfg)
+ cfg = compat_runner_args(cfg)
+ return cfg
+
+
+def compat_runner_args(cfg):
+ if 'runner' not in cfg:
+ cfg.runner = ConfigDict({
+ 'type': 'EpochBasedRunner',
+ 'max_epochs': cfg.total_epochs
+ })
+ warnings.warn(
+ 'config is now expected to have a `runner` section, '
+ 'please set `runner` in your config.', UserWarning)
+ else:
+ if 'total_epochs' in cfg:
+ assert cfg.total_epochs == cfg.runner.max_epochs
+ return cfg
+
+
+def compat_imgs_per_gpu(cfg):
+ cfg = copy.deepcopy(cfg)
+ if 'imgs_per_gpu' in cfg.data:
+ warnings.warn('"imgs_per_gpu" is deprecated in MMDet V2.0. '
+ 'Please use "samples_per_gpu" instead')
+ if 'samples_per_gpu' in cfg.data:
+ warnings.warn(
+ f'Got "imgs_per_gpu"={cfg.data.imgs_per_gpu} and '
+ f'"samples_per_gpu"={cfg.data.samples_per_gpu}, "imgs_per_gpu"'
+ f'={cfg.data.imgs_per_gpu} is used in this experiments')
+ else:
+ warnings.warn('Automatically set "samples_per_gpu"="imgs_per_gpu"='
+ f'{cfg.data.imgs_per_gpu} in this experiments')
+ cfg.data.samples_per_gpu = cfg.data.imgs_per_gpu
+ return cfg
+
+
+def compat_loader_args(cfg):
+ """Deprecated sample_per_gpu in cfg.data."""
+
+ cfg = copy.deepcopy(cfg)
+ if 'train_dataloader' not in cfg.data:
+ cfg.data['train_dataloader'] = ConfigDict()
+ if 'val_dataloader' not in cfg.data:
+ cfg.data['val_dataloader'] = ConfigDict()
+ if 'test_dataloader' not in cfg.data:
+ cfg.data['test_dataloader'] = ConfigDict()
+
+ # special process for train_dataloader
+ if 'samples_per_gpu' in cfg.data:
+
+ samples_per_gpu = cfg.data.pop('samples_per_gpu')
+ assert 'samples_per_gpu' not in \
+ cfg.data.train_dataloader, ('`samples_per_gpu` are set '
+ 'in `data` field and ` '
+ 'data.train_dataloader` '
+ 'at the same time. '
+ 'Please only set it in '
+ '`data.train_dataloader`. ')
+ cfg.data.train_dataloader['samples_per_gpu'] = samples_per_gpu
+
+ if 'persistent_workers' in cfg.data:
+
+ persistent_workers = cfg.data.pop('persistent_workers')
+ assert 'persistent_workers' not in \
+ cfg.data.train_dataloader, ('`persistent_workers` are set '
+ 'in `data` field and ` '
+ 'data.train_dataloader` '
+ 'at the same time. '
+ 'Please only set it in '
+ '`data.train_dataloader`. ')
+ cfg.data.train_dataloader['persistent_workers'] = persistent_workers
+
+ if 'workers_per_gpu' in cfg.data:
+
+ workers_per_gpu = cfg.data.pop('workers_per_gpu')
+ cfg.data.train_dataloader['workers_per_gpu'] = workers_per_gpu
+ cfg.data.val_dataloader['workers_per_gpu'] = workers_per_gpu
+ cfg.data.test_dataloader['workers_per_gpu'] = workers_per_gpu
+
+ # special process for val_dataloader
+ if 'samples_per_gpu' in cfg.data.val:
+ # keep default value of `sample_per_gpu` is 1
+ assert 'samples_per_gpu' not in \
+ cfg.data.val_dataloader, ('`samples_per_gpu` are set '
+ 'in `data.val` field and ` '
+ 'data.val_dataloader` at '
+ 'the same time. '
+ 'Please only set it in '
+ '`data.val_dataloader`. ')
+ cfg.data.val_dataloader['samples_per_gpu'] = \
+ cfg.data.val.pop('samples_per_gpu')
+ # special process for val_dataloader
+
+ # in case the test dataset is concatenated
+ if isinstance(cfg.data.test, dict):
+ if 'samples_per_gpu' in cfg.data.test:
+ assert 'samples_per_gpu' not in \
+ cfg.data.test_dataloader, ('`samples_per_gpu` are set '
+ 'in `data.test` field and ` '
+ 'data.test_dataloader` '
+ 'at the same time. '
+ 'Please only set it in '
+ '`data.test_dataloader`. ')
+
+ cfg.data.test_dataloader['samples_per_gpu'] = \
+ cfg.data.test.pop('samples_per_gpu')
+
+ elif isinstance(cfg.data.test, list):
+ for ds_cfg in cfg.data.test:
+ if 'samples_per_gpu' in ds_cfg:
+ assert 'samples_per_gpu' not in \
+ cfg.data.test_dataloader, ('`samples_per_gpu` are set '
+ 'in `data.test` field and ` '
+ 'data.test_dataloader` at'
+ ' the same time. '
+ 'Please only set it in '
+ '`data.test_dataloader`. ')
+ samples_per_gpu = max(
+ [ds_cfg.pop('samples_per_gpu', 1) for ds_cfg in cfg.data.test])
+ cfg.data.test_dataloader['samples_per_gpu'] = samples_per_gpu
+
+ return cfg
diff --git a/mmdet3d/utils/logger.py b/mmdet3d/utils/logger.py
new file mode 100644
index 0000000..14295d1
--- /dev/null
+++ b/mmdet3d/utils/logger.py
@@ -0,0 +1,31 @@
+# Copyright (c) OpenMMLab. All rights reserved.
+import logging
+
+from mmcv.utils import get_logger
+
+
+def get_root_logger(log_file=None, log_level=logging.INFO, name='mmdet3d'):
+ """Get root logger and add a keyword filter to it.
+
+ The logger will be initialized if it has not been initialized. By default a
+ StreamHandler will be added. If `log_file` is specified, a FileHandler will
+ also be added. The name of the root logger is the top-level package name,
+ e.g., "mmdet3d".
+
+ Args:
+ log_file (str, optional): File path of log. Defaults to None.
+ log_level (int, optional): The level of logger.
+ Defaults to logging.INFO.
+ name (str, optional): The name of the root logger, also used as a
+ filter keyword. Defaults to 'mmdet3d'.
+
+ Returns:
+ :obj:`logging.Logger`: The obtained logger
+ """
+ logger = get_logger(name=name, log_file=log_file, log_level=log_level)
+
+ # add a logging filter
+ logging_filter = logging.Filter(name)
+ logging_filter.filter = lambda record: record.find(name) != -1
+
+ return logger
diff --git a/mmdet3d/utils/misc.py b/mmdet3d/utils/misc.py
new file mode 100644
index 0000000..08af048
--- /dev/null
+++ b/mmdet3d/utils/misc.py
@@ -0,0 +1,39 @@
+# Copyright (c) OpenMMLab. All rights reserved.
+import glob
+import os.path as osp
+import warnings
+
+
+def find_latest_checkpoint(path, suffix='pth'):
+ """Find the latest checkpoint from the working directory. This function is
+ copied from mmdetection.
+
+ Args:
+ path(str): The path to find checkpoints.
+ suffix(str): File extension.
+ Defaults to pth.
+
+ Returns:
+ latest_path(str | None): File path of the latest checkpoint.
+ References:
+ .. [1] https://github.com/microsoft/SoftTeacher
+ /blob/main/ssod/utils/patch.py
+ """
+ if not osp.exists(path):
+ warnings.warn('The path of checkpoints does not exist.')
+ return None
+ if osp.exists(osp.join(path, f'latest.{suffix}')):
+ return osp.join(path, f'latest.{suffix}')
+
+ checkpoints = glob.glob(osp.join(path, f'*.{suffix}'))
+ if len(checkpoints) == 0:
+ warnings.warn('There are no checkpoints in the path.')
+ return None
+ latest = -1
+ latest_path = None
+ for checkpoint in checkpoints:
+ count = int(osp.basename(checkpoint).split('_')[-1].split('.')[0])
+ if count > latest:
+ latest = count
+ latest_path = checkpoint
+ return latest_path
diff --git a/mmdet3d/utils/setup_env.py b/mmdet3d/utils/setup_env.py
new file mode 100644
index 0000000..8812cb7
--- /dev/null
+++ b/mmdet3d/utils/setup_env.py
@@ -0,0 +1,53 @@
+# Copyright (c) OpenMMLab. All rights reserved.
+import os
+import platform
+import warnings
+
+import cv2
+from torch import multiprocessing as mp
+
+
+def setup_multi_processes(cfg):
+ """Setup multi-processing environment variables."""
+ # set multi-process start method as `fork` to speed up the training
+ if platform.system() != 'Windows':
+ mp_start_method = cfg.get('mp_start_method', 'fork')
+ current_method = mp.get_start_method(allow_none=True)
+ if current_method is not None and current_method != mp_start_method:
+ warnings.warn(
+ f'Multi-processing start method `{mp_start_method}` is '
+ f'different from the previous setting `{current_method}`.'
+ f'It will be force set to `{mp_start_method}`. You can change '
+ f'this behavior by changing `mp_start_method` in your config.')
+ mp.set_start_method(mp_start_method, force=True)
+
+ # disable opencv multithreading to avoid system being overloaded
+ opencv_num_threads = cfg.get('opencv_num_threads', 0)
+ cv2.setNumThreads(opencv_num_threads)
+
+ # setup OMP threads
+ # This code is referred from https://github.com/pytorch/pytorch/blob/master/torch/distributed/run.py # noqa
+ workers_per_gpu = cfg.data.get('workers_per_gpu', 1)
+ if 'train_dataloader' in cfg.data:
+ workers_per_gpu = \
+ max(cfg.data.train_dataloader.get('workers_per_gpu', 1),
+ workers_per_gpu)
+
+ if 'OMP_NUM_THREADS' not in os.environ and workers_per_gpu > 1:
+ omp_num_threads = 1
+ warnings.warn(
+ f'Setting OMP_NUM_THREADS environment variable for each process '
+ f'to be {omp_num_threads} in default, to avoid your system being '
+ f'overloaded, please further tune the variable for optimal '
+ f'performance in your application as needed.')
+ os.environ['OMP_NUM_THREADS'] = str(omp_num_threads)
+
+ # setup MKL threads
+ if 'MKL_NUM_THREADS' not in os.environ and workers_per_gpu > 1:
+ mkl_num_threads = 1
+ warnings.warn(
+ f'Setting MKL_NUM_THREADS environment variable for each process '
+ f'to be {mkl_num_threads} in default, to avoid your system being '
+ f'overloaded, please further tune the variable for optimal '
+ f'performance in your application as needed.')
+ os.environ['MKL_NUM_THREADS'] = str(mkl_num_threads)
diff --git a/mmdet3d/version.py b/mmdet3d/version.py
new file mode 100644
index 0000000..c95fbed
--- /dev/null
+++ b/mmdet3d/version.py
@@ -0,0 +1,19 @@
+# Copyright (c) Open-MMLab. All rights reserved.
+
+__version__ = '1.0.0rc3'
+short_version = __version__
+
+
+def parse_version_info(version_str):
+ version_info = []
+ for x in version_str.split('.'):
+ if x.isdigit():
+ version_info.append(int(x))
+ elif x.find('rc') != -1:
+ patch_version = x.split('rc')
+ version_info.append(int(patch_version[0]))
+ version_info.append(f'rc{patch_version[1]}')
+ return tuple(version_info)
+
+
+version_info = parse_version_info(__version__)
diff --git a/model-index.yml b/model-index.yml
new file mode 100644
index 0000000..091111b
--- /dev/null
+++ b/model-index.yml
@@ -0,0 +1,19 @@
+Import:
+ - configs/3dssd/metafile.yml
+ - configs/centerpoint/metafile.yml
+ - configs/dynamic_voxelization/metafile.yml
+ - configs/free_anchor/metafile.yml
+ - configs/h3dnet/metafile.yml
+ - configs/imvotenet/metafile.yml
+ - configs/mvxnet/metafile.yml
+ - configs/nuimages/metafile.yml
+ - configs/parta2/metafile.yml
+ - configs/pointnet2/metafile.yml
+ - configs/pointpillars/metafile.yml
+ - configs/regnet/metafile.yml
+ - configs/second/metafile.yml
+ - configs/ssn/metafile.yml
+ - configs/votenet/metafile.yml
+ - configs/fcos3d/metafile.yml
+ - configs/imvoxelnet/metafile.yml
+ - configs/pgd/metafile.yml
diff --git a/requirements.txt b/requirements.txt
new file mode 100644
index 0000000..6981bd7
--- /dev/null
+++ b/requirements.txt
@@ -0,0 +1,4 @@
+-r requirements/build.txt
+-r requirements/optional.txt
+-r requirements/runtime.txt
+-r requirements/tests.txt
diff --git a/requirements/build.txt b/requirements/build.txt
new file mode 100644
index 0000000..e69de29
diff --git a/requirements/docs.txt b/requirements/docs.txt
new file mode 100644
index 0000000..a31b771
--- /dev/null
+++ b/requirements/docs.txt
@@ -0,0 +1,8 @@
+docutils==0.16.0
+m2r
+mistune==0.8.4
+myst-parser
+-e git+https://github.com/open-mmlab/pytorch_sphinx_theme.git#egg=pytorch_sphinx_theme
+sphinx==4.0.2
+sphinx-copybutton
+sphinx_markdown_tables
diff --git a/requirements/mminstall.txt b/requirements/mminstall.txt
new file mode 100644
index 0000000..16a8d8b
--- /dev/null
+++ b/requirements/mminstall.txt
@@ -0,0 +1,3 @@
+mmcv-full>=1.4.8,<=1.6.0
+mmdet>=2.24.0,<=3.0.0
+mmsegmentation>=0.20.0,<=1.0.0
diff --git a/requirements/optional.txt b/requirements/optional.txt
new file mode 100644
index 0000000..84cbfa8
--- /dev/null
+++ b/requirements/optional.txt
@@ -0,0 +1,3 @@
+open3d
+spconv
+waymo-open-dataset-tf-2-1-0==1.2.0
diff --git a/requirements/readthedocs.txt b/requirements/readthedocs.txt
new file mode 100644
index 0000000..3ffe9e4
--- /dev/null
+++ b/requirements/readthedocs.txt
@@ -0,0 +1,5 @@
+mmcv>=1.4.8
+mmdet>=2.24.0
+mmsegmentation>=0.20.1
+torch
+torchvision
diff --git a/requirements/runtime.txt b/requirements/runtime.txt
new file mode 100644
index 0000000..a3b2d72
--- /dev/null
+++ b/requirements/runtime.txt
@@ -0,0 +1,10 @@
+lyft_dataset_sdk==0.0.8
+networkx>=2.2,<2.3
+numba==0.53.0
+numpy==1.21.5
+nuscenes-devkit==1.1.9
+plyfile==0.7.4
+scikit-image==0.19.3
+# by default we also use tensorboard to log results
+tensorboard==2.11.2
+trimesh>=2.35.39,<2.35.40
diff --git a/requirements/tests.txt b/requirements/tests.txt
new file mode 100644
index 0000000..303cc37
--- /dev/null
+++ b/requirements/tests.txt
@@ -0,0 +1,13 @@
+asynctest
+codecov
+flake8
+interrogate
+isort
+# Note: used for kwarray.group_items, this may be ported to mmcv in the future.
+kwarray
+pytest
+pytest-cov
+pytest-runner
+ubelt
+xdoctest >= 0.10.0
+yapf
diff --git a/resources/mmdet3d_outdoor_demo.gif b/resources/mmdet3d_outdoor_demo.gif
new file mode 100644
index 0000000..1c7541a
Binary files /dev/null and b/resources/mmdet3d_outdoor_demo.gif differ
diff --git a/resources/nuimages_demo.gif b/resources/nuimages_demo.gif
new file mode 100644
index 0000000..7436fab
Binary files /dev/null and b/resources/nuimages_demo.gif differ
diff --git a/resources/open3d_visual.gif b/resources/open3d_visual.gif
new file mode 100644
index 0000000..02b1f86
Binary files /dev/null and b/resources/open3d_visual.gif differ
diff --git a/setup.cfg b/setup.cfg
new file mode 100644
index 0000000..f617343
--- /dev/null
+++ b/setup.cfg
@@ -0,0 +1,16 @@
+[yapf]
+BASED_ON_STYLE = pep8
+BLANK_LINE_BEFORE_NESTED_CLASS_OR_DEF = true
+SPLIT_BEFORE_EXPRESSION_AFTER_OPENING_PAREN = true
+
+[isort]
+line_length = 79
+multi_line_output = 0
+extra_standard_library = setuptools
+known_first_party = mmdet,mmseg,mmdet3d
+known_third_party = cv2,imageio,indoor3d_util,load_scannet_data,lyft_dataset_sdk,m2r,matplotlib,mmcv,nuimages,numba,numpy,nuscenes,pandas,plyfile,pycocotools,pyquaternion,pytest,pytorch_sphinx_theme,recommonmark,requests,scannet_utils,scipy,seaborn,shapely,skimage,sphinx,tensorflow,terminaltables,torch,trimesh,ts,waymo_open_dataset
+no_lines_before = STDLIB,LOCALFOLDER
+default_section = THIRDPARTY
+
+[codespell]
+ignore-words-list = ans,refridgerator,crate,hist,formating,dout,wan,nd,fo,avod,AVOD
diff --git a/setup.py b/setup.py
new file mode 100644
index 0000000..17ecfdb
--- /dev/null
+++ b/setup.py
@@ -0,0 +1,228 @@
+import os
+import platform
+import shutil
+import sys
+import warnings
+from os import path as osp
+from setuptools import find_packages, setup
+
+import torch
+from torch.utils.cpp_extension import (BuildExtension, CppExtension,
+ CUDAExtension)
+
+
+def readme():
+ with open('README.md', encoding='utf-8') as f:
+ content = f.read()
+ return content
+
+
+version_file = 'mmdet3d/version.py'
+
+
+def get_version():
+ with open(version_file, 'r') as f:
+ exec(compile(f.read(), version_file, 'exec'))
+ import sys
+
+ # return short version for sdist
+ if 'sdist' in sys.argv or 'bdist_wheel' in sys.argv:
+ return locals()['short_version']
+ else:
+ return locals()['__version__']
+
+
+def make_cuda_ext(name,
+ module,
+ sources,
+ sources_cuda=[],
+ extra_args=[],
+ extra_include_path=[]):
+
+ define_macros = []
+ extra_compile_args = {'cxx': [] + extra_args}
+
+ if torch.cuda.is_available() or os.getenv('FORCE_CUDA', '0') == '1':
+ define_macros += [('WITH_CUDA', None)]
+ extension = CUDAExtension
+ extra_compile_args['nvcc'] = extra_args + [
+ '-D__CUDA_NO_HALF_OPERATORS__',
+ '-D__CUDA_NO_HALF_CONVERSIONS__',
+ '-D__CUDA_NO_HALF2_OPERATORS__',
+ ]
+ sources += sources_cuda
+ else:
+ print('Compiling {} without CUDA'.format(name))
+ extension = CppExtension
+ # raise EnvironmentError('CUDA is required to compile MMDetection!')
+
+ return extension(
+ name='{}.{}'.format(module, name),
+ sources=[os.path.join(*module.split('.'), p) for p in sources],
+ include_dirs=extra_include_path,
+ define_macros=define_macros,
+ extra_compile_args=extra_compile_args)
+
+
+def parse_requirements(fname='requirements.txt', with_version=True):
+ """Parse the package dependencies listed in a requirements file but strips
+ specific versioning information.
+
+ Args:
+ fname (str): path to requirements file
+ with_version (bool, default=False): if True include version specs
+
+ Returns:
+ list[str]: list of requirements items
+
+ CommandLine:
+ python -c "import setup; print(setup.parse_requirements())"
+ """
+ import re
+ import sys
+ from os.path import exists
+ require_fpath = fname
+
+ def parse_line(line):
+ """Parse information from a line in a requirements text file."""
+ if line.startswith('-r '):
+ # Allow specifying requirements in other files
+ target = line.split(' ')[1]
+ for info in parse_require_file(target):
+ yield info
+ else:
+ info = {'line': line}
+ if line.startswith('-e '):
+ info['package'] = line.split('#egg=')[1]
+ else:
+ # Remove versioning from the package
+ pat = '(' + '|'.join(['>=', '==', '>']) + ')'
+ parts = re.split(pat, line, maxsplit=1)
+ parts = [p.strip() for p in parts]
+
+ info['package'] = parts[0]
+ if len(parts) > 1:
+ op, rest = parts[1:]
+ if ';' in rest:
+ # Handle platform specific dependencies
+ # http://setuptools.readthedocs.io/en/latest/setuptools.html#declaring-platform-specific-dependencies
+ version, platform_deps = map(str.strip,
+ rest.split(';'))
+ info['platform_deps'] = platform_deps
+ else:
+ version = rest # NOQA
+ info['version'] = (op, version)
+ yield info
+
+ def parse_require_file(fpath):
+ with open(fpath, 'r') as f:
+ for line in f.readlines():
+ line = line.strip()
+ if line and not line.startswith('#'):
+ for info in parse_line(line):
+ yield info
+
+ def gen_packages_items():
+ if exists(require_fpath):
+ for info in parse_require_file(require_fpath):
+ parts = [info['package']]
+ if with_version and 'version' in info:
+ parts.extend(info['version'])
+ if not sys.version.startswith('3.4'):
+ # apparently package_deps are broken in 3.4
+ platform_deps = info.get('platform_deps')
+ if platform_deps is not None:
+ parts.append(';' + platform_deps)
+ item = ''.join(parts)
+ yield item
+
+ packages = list(gen_packages_items())
+ return packages
+
+
+def add_mim_extention():
+ """Add extra files that are required to support MIM into the package.
+
+ These files will be added by creating a symlink to the originals if the
+ package is installed in `editable` mode (e.g. pip install -e .), or by
+ copying from the originals otherwise.
+ """
+
+ # parse installment mode
+ if 'develop' in sys.argv:
+ # installed by `pip install -e .`
+ if platform.system() == 'Windows':
+ # set `copy` mode here since symlink fails on Windows.
+ mode = 'copy'
+ else:
+ mode = 'symlink'
+ elif 'sdist' in sys.argv or 'bdist_wheel' in sys.argv:
+ # installed by `pip install .`
+ # or create source distribution by `python setup.py sdist`
+ mode = 'copy'
+ else:
+ return
+
+ filenames = ['tools', 'configs', 'model-index.yml']
+ repo_path = osp.dirname(__file__)
+ mim_path = osp.join(repo_path, 'mmdet3d', '.mim')
+ os.makedirs(mim_path, exist_ok=True)
+
+ for filename in filenames:
+ if osp.exists(filename):
+ src_path = osp.join(repo_path, filename)
+ tar_path = osp.join(mim_path, filename)
+
+ if osp.isfile(tar_path) or osp.islink(tar_path):
+ os.remove(tar_path)
+ elif osp.isdir(tar_path):
+ shutil.rmtree(tar_path)
+
+ if mode == 'symlink':
+ src_relpath = osp.relpath(src_path, osp.dirname(tar_path))
+ os.symlink(src_relpath, tar_path)
+ elif mode == 'copy':
+ if osp.isfile(src_path):
+ shutil.copyfile(src_path, tar_path)
+ elif osp.isdir(src_path):
+ shutil.copytree(src_path, tar_path)
+ else:
+ warnings.warn(f'Cannot copy file {src_path}.')
+ else:
+ raise ValueError(f'Invalid mode {mode}')
+
+
+if __name__ == '__main__':
+ add_mim_extention()
+ setup(
+ name='mmdet3d',
+ version=get_version(),
+ description=("OpenMMLab's next-generation platform"
+ 'for general 3D object detection.'),
+ long_description=readme(),
+ long_description_content_type='text/markdown',
+ author='MMDetection3D Contributors',
+ author_email='zwwdev@gmail.com',
+ keywords='computer vision, 3D object detection',
+ url='https://github.com/open-mmlab/mmdetection3d',
+ packages=find_packages(),
+ include_package_data=True,
+ package_data={'mmdet3d.ops': ['*/*.so']},
+ classifiers=[
+ 'Development Status :: 4 - Beta',
+ 'License :: OSI Approved :: Apache Software License',
+ 'Operating System :: OS Independent',
+ 'Programming Language :: Python :: 3',
+ 'Programming Language :: Python :: 3.6',
+ 'Programming Language :: Python :: 3.7',
+ ],
+ license='Apache License 2.0',
+ install_requires=parse_requirements('requirements/runtime.txt'),
+ extras_require={
+ 'all': parse_requirements('requirements.txt'),
+ 'tests': parse_requirements('requirements/tests.txt'),
+ 'build': parse_requirements('requirements/build.txt'),
+ 'optional': parse_requirements('requirements/optional.txt'),
+ },
+ cmdclass={'build_ext': BuildExtension},
+ zip_safe=False)
diff --git a/tests/test_data/test_datasets/test_dataset_wrappers.py b/tests/test_data/test_datasets/test_dataset_wrappers.py
new file mode 100644
index 0000000..bcf183f
--- /dev/null
+++ b/tests/test_data/test_datasets/test_dataset_wrappers.py
@@ -0,0 +1,79 @@
+# Copyright (c) OpenMMLab. All rights reserved.
+import numpy as np
+import torch
+
+from mmdet3d.datasets.builder import build_dataset
+
+
+def test_getitem():
+ np.random.seed(1)
+ torch.manual_seed(1)
+ point_cloud_range = [-50, -50, -5, 50, 50, 3]
+ file_client_args = dict(backend='disk')
+ class_names = [
+ 'car', 'truck', 'trailer', 'bus', 'construction_vehicle', 'bicycle',
+ 'motorcycle', 'pedestrian', 'traffic_cone', 'barrier'
+ ]
+ pipeline = [
+ dict(
+ type='LoadPointsFromFile',
+ coord_type='LIDAR',
+ load_dim=5,
+ use_dim=5,
+ file_client_args=file_client_args),
+ dict(
+ type='LoadPointsFromMultiSweeps',
+ sweeps_num=9,
+ use_dim=[0, 1, 2, 3, 4],
+ file_client_args=file_client_args,
+ pad_empty_sweeps=True,
+ remove_close=True,
+ test_mode=True),
+ dict(type='LoadAnnotations3D', with_bbox_3d=True, with_label_3d=True),
+ # dict(type='ObjectSample', db_sampler=db_sampler),
+ dict(
+ type='GlobalRotScaleTrans',
+ rot_range=[-0.3925, 0.3925],
+ scale_ratio_range=[0.95, 1.05],
+ translation_std=[0, 0, 0]),
+ dict(type='RandomFlip3D', flip_ratio_bev_horizontal=0.5),
+ dict(type='PointsRangeFilter', point_cloud_range=point_cloud_range),
+ dict(type='ObjectRangeFilter', point_cloud_range=point_cloud_range),
+ dict(type='ObjectNameFilter', classes=class_names),
+ dict(type='PointShuffle'),
+ dict(type='DefaultFormatBundle3D', class_names=class_names),
+ dict(
+ type='Collect3D', keys=['points', 'gt_bboxes_3d', 'gt_labels_3d'])
+ ]
+ input_modality = dict(
+ use_lidar=True,
+ use_camera=False,
+ use_radar=False,
+ use_map=False,
+ use_external=False)
+ dataset_cfg = dict(
+ type='CBGSDataset',
+ dataset=dict(
+ type='NuScenesDataset',
+ data_root='tests/data/nuscenes',
+ ann_file='tests/data/nuscenes/nus_info.pkl',
+ pipeline=pipeline,
+ classes=class_names,
+ modality=input_modality,
+ test_mode=False,
+ use_valid_flag=True,
+ # we use box_type_3d='LiDAR' in kitti and nuscenes dataset
+ # and box_type_3d='Depth' in sunrgbd and scannet dataset.
+ box_type_3d='LiDAR'))
+ nus_dataset = build_dataset(dataset_cfg)
+ assert len(nus_dataset) == 20
+
+ data = nus_dataset[0]
+ assert data['img_metas'].data['flip'] is True
+ assert data['img_metas'].data['pcd_horizontal_flip'] is True
+ assert data['points']._data.shape == (537, 5)
+
+ data = nus_dataset[2]
+ assert data['img_metas'].data['flip'] is False
+ assert data['img_metas'].data['pcd_horizontal_flip'] is False
+ assert data['points']._data.shape == (901, 5)
diff --git a/tests/test_data/test_datasets/test_kitti_dataset.py b/tests/test_data/test_datasets/test_kitti_dataset.py
new file mode 100644
index 0000000..f71be6b
--- /dev/null
+++ b/tests/test_data/test_datasets/test_kitti_dataset.py
@@ -0,0 +1,478 @@
+# Copyright (c) OpenMMLab. All rights reserved.
+import math
+import os
+import tempfile
+
+import numpy as np
+import pytest
+import torch
+
+from mmdet3d.core.bbox import LiDARInstance3DBoxes, limit_period
+from mmdet3d.datasets import KittiDataset
+
+
+def _generate_kitti_dataset_config():
+ data_root = 'tests/data/kitti'
+ ann_file = 'tests/data/kitti/kitti_infos_train.pkl'
+ classes = ['Pedestrian', 'Cyclist', 'Car']
+ pts_prefix = 'velodyne_reduced'
+ pipeline = [
+ dict(
+ type='LoadPointsFromFile',
+ coord_type='LIDAR',
+ load_dim=4,
+ use_dim=4,
+ file_client_args=dict(backend='disk')),
+ dict(
+ type='MultiScaleFlipAug3D',
+ img_scale=(1333, 800),
+ pts_scale_ratio=1,
+ flip=False,
+ transforms=[
+ dict(
+ type='GlobalRotScaleTrans',
+ rot_range=[0, 0],
+ scale_ratio_range=[1.0, 1.0],
+ translation_std=[0, 0, 0]),
+ dict(type='RandomFlip3D'),
+ dict(
+ type='PointsRangeFilter',
+ point_cloud_range=[0, -40, -3, 70.4, 40, 1]),
+ dict(
+ type='DefaultFormatBundle3D',
+ class_names=classes,
+ with_label=False),
+ dict(type='Collect3D', keys=['points'])
+ ])
+ ]
+ modality = dict(use_lidar=True, use_camera=False)
+ split = 'training'
+ return data_root, ann_file, classes, pts_prefix, pipeline, modality, split
+
+
+def _generate_kitti_multi_modality_dataset_config():
+ data_root = 'tests/data/kitti'
+ ann_file = 'tests/data/kitti/kitti_infos_train.pkl'
+ classes = ['Pedestrian', 'Cyclist', 'Car']
+ pts_prefix = 'velodyne_reduced'
+ img_norm_cfg = dict(
+ mean=[103.530, 116.280, 123.675], std=[1.0, 1.0, 1.0], to_rgb=False)
+ pipeline = [
+ dict(
+ type='LoadPointsFromFile',
+ coord_type='LIDAR',
+ load_dim=4,
+ use_dim=4,
+ file_client_args=dict(backend='disk')),
+ dict(type='LoadImageFromFile'),
+ dict(
+ type='MultiScaleFlipAug3D',
+ img_scale=(1333, 800),
+ pts_scale_ratio=1,
+ flip=False,
+ transforms=[
+ dict(type='Resize', multiscale_mode='value', keep_ratio=True),
+ dict(
+ type='GlobalRotScaleTrans',
+ rot_range=[0, 0],
+ scale_ratio_range=[1., 1.],
+ translation_std=[0, 0, 0]),
+ dict(type='RandomFlip3D'),
+ dict(type='Normalize', **img_norm_cfg),
+ dict(type='Pad', size_divisor=32),
+ dict(
+ type='PointsRangeFilter',
+ point_cloud_range=[0, -40, -3, 70.4, 40, 1]),
+ dict(
+ type='DefaultFormatBundle3D',
+ class_names=classes,
+ with_label=False),
+ dict(type='Collect3D', keys=['points', 'img'])
+ ])
+ ]
+ modality = dict(use_lidar=True, use_camera=True)
+ split = 'training'
+ return data_root, ann_file, classes, pts_prefix, pipeline, modality, split
+
+
+def test_getitem():
+ np.random.seed(0)
+ data_root, ann_file, classes, pts_prefix, \
+ _, modality, split = _generate_kitti_dataset_config()
+ pipeline = [
+ dict(
+ type='LoadPointsFromFile',
+ coord_type='LIDAR',
+ load_dim=4,
+ use_dim=4,
+ file_client_args=dict(backend='disk')),
+ dict(
+ type='LoadAnnotations3D',
+ with_bbox_3d=True,
+ with_label_3d=True,
+ file_client_args=dict(backend='disk')),
+ dict(
+ type='ObjectSample',
+ db_sampler=dict(
+ data_root='tests/data/kitti/',
+ # in coordinate system refactor, this test file is modified
+ info_path='tests/data/kitti/kitti_dbinfos_train.pkl',
+ rate=1.0,
+ prepare=dict(
+ filter_by_difficulty=[-1],
+ filter_by_min_points=dict(Pedestrian=10)),
+ classes=['Pedestrian', 'Cyclist', 'Car'],
+ sample_groups=dict(Pedestrian=6))),
+ dict(
+ type='ObjectNoise',
+ num_try=100,
+ translation_std=[1.0, 1.0, 0.5],
+ global_rot_range=[0.0, 0.0],
+ rot_range=[-0.78539816, 0.78539816]),
+ dict(type='RandomFlip3D', flip_ratio_bev_horizontal=0.5),
+ dict(
+ type='GlobalRotScaleTrans',
+ rot_range=[-0.78539816, 0.78539816],
+ scale_ratio_range=[0.95, 1.05]),
+ dict(
+ type='PointsRangeFilter',
+ point_cloud_range=[0, -40, -3, 70.4, 40, 1]),
+ dict(
+ type='ObjectRangeFilter',
+ point_cloud_range=[0, -40, -3, 70.4, 40, 1]),
+ dict(type='PointShuffle'),
+ dict(
+ type='DefaultFormatBundle3D',
+ class_names=['Pedestrian', 'Cyclist', 'Car']),
+ dict(
+ type='Collect3D', keys=['points', 'gt_bboxes_3d', 'gt_labels_3d'])
+ ]
+ kitti_dataset = KittiDataset(data_root, ann_file, split, pts_prefix,
+ pipeline, classes, modality)
+ data = kitti_dataset[0]
+ points = data['points']._data
+ gt_bboxes_3d = data['gt_bboxes_3d']._data
+ gt_labels_3d = data['gt_labels_3d']._data
+ expected_gt_bboxes_3d = torch.tensor(
+ [[9.5081, -5.2269, -1.1370, 1.2288, 0.4915, 1.9353, 1.9988]])
+ expected_gt_labels_3d = torch.tensor([0])
+ rot_matrix = data['img_metas']._data['pcd_rotation']
+ rot_angle = data['img_metas']._data['pcd_rotation_angle']
+ horizontal_flip = data['img_metas']._data['pcd_horizontal_flip']
+ vertical_flip = data['img_metas']._data['pcd_vertical_flip']
+ expected_rot_matrix = torch.tensor([[0.8018, 0.5976, 0.0000],
+ [-0.5976, 0.8018, 0.0000],
+ [0.0000, 0.0000, 1.0000]])
+ expected_rot_angle = 0.6404654291602163
+ noise_angle = 0.20247319
+ assert torch.allclose(expected_rot_matrix, rot_matrix, atol=1e-4)
+ assert math.isclose(expected_rot_angle, rot_angle, abs_tol=1e-4)
+ assert horizontal_flip is True
+ assert vertical_flip is False
+
+ # after coord system refactor
+ expected_gt_bboxes_3d[:, :3] = \
+ expected_gt_bboxes_3d[:, :3] @ rot_matrix @ rot_matrix
+ expected_gt_bboxes_3d[:, -1:] = -np.pi - expected_gt_bboxes_3d[:, -1:] \
+ + 2 * rot_angle - 2 * noise_angle
+ expected_gt_bboxes_3d[:, -1:] = limit_period(
+ expected_gt_bboxes_3d[:, -1:], period=np.pi * 2)
+ assert points.shape == (780, 4)
+ assert torch.allclose(
+ gt_bboxes_3d.tensor, expected_gt_bboxes_3d, atol=1e-4)
+ assert torch.all(gt_labels_3d == expected_gt_labels_3d)
+
+ # test multi-modality KITTI dataset
+ np.random.seed(0)
+ point_cloud_range = [0, -40, -3, 70.4, 40, 1]
+ img_norm_cfg = dict(
+ mean=[103.530, 116.280, 123.675], std=[1.0, 1.0, 1.0], to_rgb=False)
+ multi_modality_pipeline = [
+ dict(
+ type='LoadPointsFromFile',
+ coord_type='LIDAR',
+ load_dim=4,
+ use_dim=4),
+ dict(type='LoadImageFromFile'),
+ dict(type='LoadAnnotations3D', with_bbox_3d=True, with_label_3d=True),
+ dict(
+ type='Resize',
+ img_scale=[(640, 192), (2560, 768)],
+ multiscale_mode='range',
+ keep_ratio=True),
+ dict(
+ type='GlobalRotScaleTrans',
+ rot_range=[-0.78539816, 0.78539816],
+ scale_ratio_range=[0.95, 1.05],
+ translation_std=[0.2, 0.2, 0.2]),
+ dict(type='RandomFlip3D', flip_ratio_bev_horizontal=0.5),
+ dict(type='PointsRangeFilter', point_cloud_range=point_cloud_range),
+ dict(type='ObjectRangeFilter', point_cloud_range=point_cloud_range),
+ dict(type='PointShuffle'),
+ dict(type='Normalize', **img_norm_cfg),
+ dict(type='Pad', size_divisor=32),
+ dict(type='DefaultFormatBundle3D', class_names=classes),
+ dict(
+ type='Collect3D',
+ keys=['points', 'img', 'gt_bboxes_3d', 'gt_labels_3d']),
+ ]
+ modality = dict(use_lidar=True, use_camera=True)
+ kitti_dataset = KittiDataset(data_root, ann_file, split, pts_prefix,
+ multi_modality_pipeline, classes, modality)
+ data = kitti_dataset[0]
+ img = data['img']._data
+ lidar2img = data['img_metas']._data['lidar2img']
+
+ expected_lidar2img = np.array(
+ [[6.02943726e+02, -7.07913330e+02, -1.22748432e+01, -1.70942719e+02],
+ [1.76777252e+02, 8.80879879e+00, -7.07936157e+02, -1.02568634e+02],
+ [9.99984801e-01, -1.52826728e-03, -5.29071223e-03, -3.27567995e-01],
+ [0.00000000e+00, 0.00000000e+00, 0.00000000e+00, 1.00000000e+00]])
+
+ assert img.shape[:] == (3, 416, 1344)
+ assert np.allclose(lidar2img, expected_lidar2img)
+
+
+def test_evaluate():
+ if not torch.cuda.is_available():
+ pytest.skip('test requires GPU and torch+cuda')
+ data_root, ann_file, classes, pts_prefix, \
+ pipeline, modality, split = _generate_kitti_dataset_config()
+ kitti_dataset = KittiDataset(data_root, ann_file, split, pts_prefix,
+ pipeline, classes, modality)
+ boxes_3d = LiDARInstance3DBoxes(
+ torch.tensor(
+ [[8.7314, -1.8559, -1.5997, 0.4800, 1.2000, 1.8900, 0.0100]]))
+ labels_3d = torch.tensor([
+ 0,
+ ])
+ scores_3d = torch.tensor([0.5])
+ metric = ['mAP']
+ result = dict(boxes_3d=boxes_3d, labels_3d=labels_3d, scores_3d=scores_3d)
+ ap_dict = kitti_dataset.evaluate([result], metric)
+ assert np.isclose(ap_dict['KITTI/Overall_3D_AP11_easy'],
+ 3.0303030303030307)
+ assert np.isclose(ap_dict['KITTI/Overall_3D_AP11_moderate'],
+ 3.0303030303030307)
+ assert np.isclose(ap_dict['KITTI/Overall_3D_AP11_hard'],
+ 3.0303030303030307)
+
+
+def test_show():
+ from os import path as osp
+
+ import mmcv
+
+ from mmdet3d.core.bbox import LiDARInstance3DBoxes
+ tmp_dir = tempfile.TemporaryDirectory()
+ temp_dir = tmp_dir.name
+ data_root, ann_file, classes, pts_prefix, \
+ pipeline, modality, split = _generate_kitti_dataset_config()
+ kitti_dataset = KittiDataset(
+ data_root, ann_file, split=split, modality=modality, pipeline=pipeline)
+ boxes_3d = LiDARInstance3DBoxes(
+ torch.tensor(
+ [[46.1218, -4.6496, -0.9275, 0.5316, 1.4442, 1.7450, 1.1749],
+ [33.3189, 0.1981, 0.3136, 0.5656, 1.2301, 1.7985, 1.5723],
+ [46.1366, -4.6404, -0.9510, 0.5162, 1.6501, 1.7540, 1.3778],
+ [33.2646, 0.2297, 0.3446, 0.5746, 1.3365, 1.7947, 1.5430],
+ [58.9079, 16.6272, -1.5829, 1.5656, 3.9313, 1.4899, 1.5505]]))
+ scores_3d = torch.tensor([0.1815, 0.1663, 0.5792, 0.2194, 0.2780])
+ labels_3d = torch.tensor([0, 0, 1, 1, 2])
+ result = dict(boxes_3d=boxes_3d, scores_3d=scores_3d, labels_3d=labels_3d)
+ results = [result]
+ kitti_dataset.show(results, temp_dir, show=False)
+ pts_file_path = osp.join(temp_dir, '000000', '000000_points.obj')
+ gt_file_path = osp.join(temp_dir, '000000', '000000_gt.obj')
+ pred_file_path = osp.join(temp_dir, '000000', '000000_pred.obj')
+ mmcv.check_file_exist(pts_file_path)
+ mmcv.check_file_exist(gt_file_path)
+ mmcv.check_file_exist(pred_file_path)
+ tmp_dir.cleanup()
+
+ # test show with pipeline
+ eval_pipeline = [
+ dict(
+ type='LoadPointsFromFile',
+ coord_type='LIDAR',
+ load_dim=4,
+ use_dim=4),
+ dict(
+ type='DefaultFormatBundle3D',
+ class_names=classes,
+ with_label=False),
+ dict(type='Collect3D', keys=['points'])
+ ]
+ tmp_dir = tempfile.TemporaryDirectory()
+ temp_dir = tmp_dir.name
+ kitti_dataset.show(results, temp_dir, show=False, pipeline=eval_pipeline)
+ pts_file_path = osp.join(temp_dir, '000000', '000000_points.obj')
+ gt_file_path = osp.join(temp_dir, '000000', '000000_gt.obj')
+ pred_file_path = osp.join(temp_dir, '000000', '000000_pred.obj')
+ mmcv.check_file_exist(pts_file_path)
+ mmcv.check_file_exist(gt_file_path)
+ mmcv.check_file_exist(pred_file_path)
+ tmp_dir.cleanup()
+
+ # test multi-modality show
+ tmp_dir = tempfile.TemporaryDirectory()
+ temp_dir = tmp_dir.name
+ _, _, _, _, multi_modality_pipeline, modality, _ = \
+ _generate_kitti_multi_modality_dataset_config()
+ kitti_dataset = KittiDataset(data_root, ann_file, split, pts_prefix,
+ multi_modality_pipeline, classes, modality)
+ kitti_dataset.show(results, temp_dir, show=False)
+ pts_file_path = osp.join(temp_dir, '000000', '000000_points.obj')
+ gt_file_path = osp.join(temp_dir, '000000', '000000_gt.obj')
+ pred_file_path = osp.join(temp_dir, '000000', '000000_pred.obj')
+ img_file_path = osp.join(temp_dir, '000000', '000000_img.png')
+ img_pred_path = osp.join(temp_dir, '000000', '000000_pred.png')
+ img_gt_file = osp.join(temp_dir, '000000', '000000_gt.png')
+ mmcv.check_file_exist(pts_file_path)
+ mmcv.check_file_exist(gt_file_path)
+ mmcv.check_file_exist(pred_file_path)
+ mmcv.check_file_exist(img_file_path)
+ mmcv.check_file_exist(img_pred_path)
+ mmcv.check_file_exist(img_gt_file)
+ tmp_dir.cleanup()
+
+ # test multi-modality show with pipeline
+ eval_pipeline = [
+ dict(
+ type='LoadPointsFromFile',
+ coord_type='LIDAR',
+ load_dim=4,
+ use_dim=4),
+ dict(type='LoadImageFromFile'),
+ dict(
+ type='DefaultFormatBundle3D',
+ class_names=classes,
+ with_label=False),
+ dict(type='Collect3D', keys=['points', 'img'])
+ ]
+ tmp_dir = tempfile.TemporaryDirectory()
+ temp_dir = tmp_dir.name
+ kitti_dataset.show(results, temp_dir, show=False, pipeline=eval_pipeline)
+ pts_file_path = osp.join(temp_dir, '000000', '000000_points.obj')
+ gt_file_path = osp.join(temp_dir, '000000', '000000_gt.obj')
+ pred_file_path = osp.join(temp_dir, '000000', '000000_pred.obj')
+ img_file_path = osp.join(temp_dir, '000000', '000000_img.png')
+ img_pred_path = osp.join(temp_dir, '000000', '000000_pred.png')
+ img_gt_file = osp.join(temp_dir, '000000', '000000_gt.png')
+ mmcv.check_file_exist(pts_file_path)
+ mmcv.check_file_exist(gt_file_path)
+ mmcv.check_file_exist(pred_file_path)
+ mmcv.check_file_exist(img_file_path)
+ mmcv.check_file_exist(img_pred_path)
+ mmcv.check_file_exist(img_gt_file)
+ tmp_dir.cleanup()
+
+
+def test_format_results():
+ from mmdet3d.core.bbox import LiDARInstance3DBoxes
+ data_root, ann_file, classes, pts_prefix, \
+ pipeline, modality, split = _generate_kitti_dataset_config()
+ kitti_dataset = KittiDataset(data_root, ann_file, split, pts_prefix,
+ pipeline, classes, modality)
+ # coord system refactor
+ boxes_3d = LiDARInstance3DBoxes(
+ torch.tensor(
+ [[8.7314, -1.8559, -1.5997, 1.2000, 0.4800, 1.8900, -1.5808]]))
+ labels_3d = torch.tensor([
+ 0,
+ ])
+ scores_3d = torch.tensor([0.5])
+ result = dict(boxes_3d=boxes_3d, labels_3d=labels_3d, scores_3d=scores_3d)
+ results = [result]
+ result_files, tmp_dir = kitti_dataset.format_results(results)
+ expected_name = np.array(['Pedestrian'])
+ expected_truncated = np.array([0.])
+ expected_occluded = np.array([0])
+ # coord sys refactor
+ expected_alpha = np.array(-3.3410306 + np.pi)
+ expected_bbox = np.array([[710.443, 144.00221, 820.29114, 307.58667]])
+ expected_dimensions = np.array([[1.2, 1.89, 0.48]])
+ expected_location = np.array([[1.8399826, 1.4700007, 8.410018]])
+ expected_rotation_y = np.array([0.0100])
+ expected_score = np.array([0.5])
+ expected_sample_idx = np.array([0])
+ assert np.all(result_files[0]['name'] == expected_name)
+ assert np.allclose(result_files[0]['truncated'], expected_truncated)
+ assert np.all(result_files[0]['occluded'] == expected_occluded)
+ assert np.allclose(result_files[0]['alpha'], expected_alpha, 1e-3)
+ assert np.allclose(result_files[0]['bbox'], expected_bbox)
+ assert np.allclose(result_files[0]['dimensions'], expected_dimensions)
+ assert np.allclose(result_files[0]['location'], expected_location)
+ assert np.allclose(result_files[0]['rotation_y'], expected_rotation_y,
+ 1e-3)
+ assert np.allclose(result_files[0]['score'], expected_score)
+ assert np.allclose(result_files[0]['sample_idx'], expected_sample_idx)
+ tmp_dir.cleanup()
+
+
+def test_bbox2result_kitti():
+ data_root, ann_file, classes, pts_prefix, \
+ pipeline, modality, split = _generate_kitti_dataset_config()
+ kitti_dataset = KittiDataset(data_root, ann_file, split, pts_prefix,
+ pipeline, classes, modality)
+ boxes_3d = LiDARInstance3DBoxes(
+ torch.tensor(
+ [[8.7314, -1.8559, -1.5997, 1.2000, 0.4800, 1.8900, -1.5808]]))
+ labels_3d = torch.tensor([
+ 0,
+ ])
+ scores_3d = torch.tensor([0.5])
+ result = dict(boxes_3d=boxes_3d, labels_3d=labels_3d, scores_3d=scores_3d)
+ results = [result]
+ tmp_dir = tempfile.TemporaryDirectory()
+ temp_kitti_result_dir = tmp_dir.name
+ det_annos = kitti_dataset.bbox2result_kitti(
+ results, classes, submission_prefix=temp_kitti_result_dir)
+ expected_file_path = os.path.join(temp_kitti_result_dir, '000000.txt')
+ expected_name = np.array(['Pedestrian'])
+ expected_dimensions = np.array([1.2000, 1.8900, 0.4800])
+ # coord system refactor (reverse sign)
+ expected_rotation_y = 0.0100
+ expected_score = np.array([0.5])
+ assert np.all(det_annos[0]['name'] == expected_name)
+ assert np.allclose(det_annos[0]['rotation_y'], expected_rotation_y, 1e-3)
+ assert np.allclose(det_annos[0]['score'], expected_score)
+ assert np.allclose(det_annos[0]['dimensions'], expected_dimensions)
+ assert os.path.exists(expected_file_path)
+ tmp_dir.cleanup()
+
+ tmp_dir = tempfile.TemporaryDirectory()
+ temp_kitti_result_dir = tmp_dir.name
+ boxes_3d = LiDARInstance3DBoxes(torch.tensor([]))
+ labels_3d = torch.tensor([])
+ scores_3d = torch.tensor([])
+ empty_result = dict(
+ boxes_3d=boxes_3d, labels_3d=labels_3d, scores_3d=scores_3d)
+ results = [empty_result]
+ det_annos = kitti_dataset.bbox2result_kitti(
+ results, classes, submission_prefix=temp_kitti_result_dir)
+ expected_file_path = os.path.join(temp_kitti_result_dir, '000000.txt')
+ assert os.path.exists(expected_file_path)
+ tmp_dir.cleanup()
+
+
+def test_bbox2result_kitti2d():
+ data_root, ann_file, classes, pts_prefix, \
+ pipeline, modality, split = _generate_kitti_dataset_config()
+ kitti_dataset = KittiDataset(data_root, ann_file, split, pts_prefix,
+ pipeline, classes, modality)
+ bboxes = np.array([[[46.1218, -4.6496, -0.9275, 0.5316, 0.5],
+ [33.3189, 0.1981, 0.3136, 0.5656, 0.5]],
+ [[46.1366, -4.6404, -0.9510, 0.5162, 0.5],
+ [33.2646, 0.2297, 0.3446, 0.5746, 0.5]]])
+ det_annos = kitti_dataset.bbox2result_kitti2d([bboxes], classes)
+ expected_name = np.array(
+ ['Pedestrian', 'Pedestrian', 'Cyclist', 'Cyclist'])
+ expected_bbox = np.array([[46.1218, -4.6496, -0.9275, 0.5316],
+ [33.3189, 0.1981, 0.3136, 0.5656],
+ [46.1366, -4.6404, -0.951, 0.5162],
+ [33.2646, 0.2297, 0.3446, 0.5746]])
+ expected_score = np.array([0.5, 0.5, 0.5, 0.5])
+ assert np.all(det_annos[0]['name'] == expected_name)
+ assert np.allclose(det_annos[0]['bbox'], expected_bbox)
+ assert np.allclose(det_annos[0]['score'], expected_score)
diff --git a/tests/test_data/test_datasets/test_kitti_mono_dataset.py b/tests/test_data/test_datasets/test_kitti_mono_dataset.py
new file mode 100644
index 0000000..22ea10b
--- /dev/null
+++ b/tests/test_data/test_datasets/test_kitti_mono_dataset.py
@@ -0,0 +1,217 @@
+# Copyright (c) OpenMMLab. All rights reserved.
+import mmcv
+import numpy as np
+import pytest
+import torch
+
+from mmdet3d.datasets import KittiMonoDataset
+
+
+def test_getitem():
+ np.random.seed(0)
+ class_names = ['Pedestrian', 'Cyclist', 'Car']
+ img_norm_cfg = dict(
+ mean=[103.530, 116.280, 123.675], std=[1.0, 1.0, 1.0], to_rgb=False)
+ pipeline = [
+ dict(type='LoadImageFromFileMono3D'),
+ dict(
+ type='LoadAnnotations3D',
+ with_bbox=True,
+ with_label=True,
+ with_attr_label=False,
+ with_bbox_3d=True,
+ with_label_3d=True,
+ with_bbox_depth=True),
+ dict(type='Resize', img_scale=(1242, 375), keep_ratio=True),
+ dict(type='RandomFlip3D', flip_ratio_bev_horizontal=1.0),
+ dict(type='Normalize', **img_norm_cfg),
+ dict(type='Pad', size_divisor=32),
+ dict(type='DefaultFormatBundle3D', class_names=class_names),
+ dict(
+ type='Collect3D',
+ keys=[
+ 'img', 'gt_bboxes', 'gt_labels', 'gt_bboxes_3d',
+ 'gt_labels_3d', 'centers2d', 'depths'
+ ]),
+ ]
+
+ kitti_dataset = KittiMonoDataset(
+ ann_file='tests/data/kitti/kitti_infos_mono3d.coco.json',
+ info_file='tests/data/kitti/kitti_infos_mono3d.pkl',
+ pipeline=pipeline,
+ data_root='tests/data/kitti/',
+ img_prefix='tests/data/kitti/',
+ test_mode=False)
+
+ data = kitti_dataset[0]
+ img_metas = data['img_metas']._data
+ filename = img_metas['filename']
+ img_shape = img_metas['img_shape']
+ pad_shape = img_metas['pad_shape']
+ flip = img_metas['flip']
+ bboxes = data['gt_bboxes']._data
+ labels3d = data['gt_labels_3d']._data
+ labels = data['gt_labels']._data
+ centers2d = data['centers2d']._data
+ depths = data['depths']._data
+
+ expected_filename = 'tests/data/kitti/training/image_2/000007.png'
+ expected_img_shape = (375, 1242, 3)
+ expected_pad_shape = (384, 1248, 3)
+ expected_flip = True
+ expected_bboxes = torch.tensor([[625.3445, 175.0120, 676.5177, 224.9605],
+ [729.5906, 179.8571, 760.1503, 202.5390],
+ [676.7557, 175.7334, 699.7753, 193.9447],
+ [886.5021, 176.1380, 911.1581, 213.8148]])
+ expected_labels = torch.tensor([2, 2, 2, 1])
+ expected_centers2d = torch.tensor([[650.6185, 198.3731],
+ [744.2711, 190.7532],
+ [687.8787, 184.5331],
+ [898.4750, 194.4337]])
+ expected_depths = torch.tensor([25.0127, 47.5527, 60.5227, 34.0927])
+
+ assert filename == expected_filename
+ assert img_shape == expected_img_shape
+ assert pad_shape == expected_pad_shape
+ assert flip == expected_flip
+ assert torch.allclose(bboxes, expected_bboxes, 1e-5)
+ assert torch.all(labels == expected_labels)
+ assert torch.all(labels3d == expected_labels)
+ assert torch.allclose(centers2d, expected_centers2d, 1e-5)
+ assert torch.allclose(depths, expected_depths, 1e-5)
+
+
+def test_format_results():
+ root_path = 'tests/data/kitti/'
+ info_file = 'tests/data/kitti/kitti_infos_mono3d.pkl'
+ ann_file = 'tests/data/kitti/kitti_infos_mono3d.coco.json'
+ class_names = ['Pedestrian', 'Cyclist', 'Car']
+ pipeline = [
+ dict(type='LoadImageFromFileMono3D'),
+ dict(
+ type='LoadAnnotations3D',
+ with_bbox=True,
+ with_label=True,
+ with_attr_label=False,
+ with_bbox_3d=True,
+ with_label_3d=True,
+ with_bbox_depth=True),
+ dict(type='Resize', img_scale=(1242, 375), keep_ratio=True),
+ dict(type='Pad', size_divisor=32),
+ dict(type='DefaultFormatBundle3D', class_names=class_names),
+ dict(
+ type='Collect3D',
+ keys=[
+ 'img', 'gt_bboxes', 'gt_labels', 'gt_bboxes_3d',
+ 'gt_labels_3d', 'centers2d', 'depths'
+ ]),
+ ]
+ kitti_dataset = KittiMonoDataset(
+ ann_file=ann_file,
+ info_file=info_file,
+ pipeline=pipeline,
+ data_root=root_path,
+ test_mode=True)
+
+ # format 3D detection results
+ results = mmcv.load('tests/data/kitti/mono3d_sample_results.pkl')
+ result_files, tmp_dir = kitti_dataset.format_results(results)
+ result_data = result_files['img_bbox']
+ assert len(result_data) == 1
+ assert len(result_data[0]['name']) == 4
+ det = result_data[0]
+
+ expected_bbox = torch.tensor([[565.4989, 175.02547, 616.70184, 225.00565],
+ [481.85907, 179.8642, 512.43414, 202.5624],
+ [542.23157, 175.73912, 565.26263, 193.96303],
+ [330.8572, 176.1482, 355.53937, 213.8469]])
+ expected_dims = torch.tensor([[3.201, 1.6110001, 1.661],
+ [3.701, 1.401, 1.511],
+ [4.051, 1.4610001, 1.661],
+ [1.9510001, 1.7210001, 0.501]])
+ expected_rotation = torch.tensor([-1.59, 1.55, 1.56, 1.54])
+ expected_detname = ['Car', 'Car', 'Car', 'Cyclist']
+
+ assert torch.allclose(torch.from_numpy(det['bbox']), expected_bbox, 1e-5)
+ assert torch.allclose(
+ torch.from_numpy(det['dimensions']), expected_dims, 1e-5)
+ assert torch.allclose(
+ torch.from_numpy(det['rotation_y']), expected_rotation, 1e-5)
+ assert det['name'].tolist() == expected_detname
+
+ # format 2D detection results
+ results = mmcv.load('tests/data/kitti/mono3d_sample_results2d.pkl')
+ result_files, tmp_dir = kitti_dataset.format_results(results)
+ result_data = result_files['img_bbox2d']
+ assert len(result_data) == 1
+ assert len(result_data[0]['name']) == 4
+ det = result_data[0]
+
+ expected_bbox = torch.tensor(
+ [[330.84191493, 176.13804312, 355.49885373, 213.81578769],
+ [565.48227204, 175.01202566, 616.65650883, 224.96147091],
+ [481.84967085, 179.85710612, 512.41043776, 202.54001526],
+ [542.22471517, 175.73341152, 565.24534908, 193.94568878]])
+ expected_dims = torch.tensor([[0., 0., 0.], [0., 0., 0.], [0., 0., 0.],
+ [0., 0., 0.]])
+ expected_rotation = torch.tensor([0., 0., 0., 0.])
+ expected_detname = ['Cyclist', 'Car', 'Car', 'Car']
+
+ assert torch.allclose(
+ torch.from_numpy(det['bbox']).float(), expected_bbox, 1e-5)
+ assert torch.allclose(
+ torch.from_numpy(det['dimensions']).float(), expected_dims, 1e-5)
+ assert torch.allclose(
+ torch.from_numpy(det['rotation_y']).float(), expected_rotation, 1e-5)
+ assert det['name'].tolist() == expected_detname
+
+
+def test_evaluate():
+ if not torch.cuda.is_available():
+ pytest.skip('test requires GPU and torch+cuda')
+ root_path = 'tests/data/kitti/'
+ info_file = 'tests/data/kitti/kitti_infos_mono3d.pkl'
+ ann_file = 'tests/data/kitti/kitti_infos_mono3d.coco.json'
+ class_names = ['Pedestrian', 'Cyclist', 'Car']
+ pipeline = [
+ dict(type='LoadImageFromFileMono3D'),
+ dict(
+ type='LoadAnnotations3D',
+ with_bbox=True,
+ with_label=True,
+ with_attr_label=False,
+ with_bbox_3d=True,
+ with_label_3d=True,
+ with_bbox_depth=True),
+ dict(type='Resize', img_scale=(1242, 375), keep_ratio=True),
+ dict(type='Pad', size_divisor=32),
+ dict(type='DefaultFormatBundle3D', class_names=class_names),
+ dict(
+ type='Collect3D',
+ keys=[
+ 'img', 'gt_bboxes', 'gt_labels', 'gt_bboxes_3d',
+ 'gt_labels_3d', 'centers2d', 'depths'
+ ]),
+ ]
+ kitti_dataset = KittiMonoDataset(
+ ann_file=ann_file,
+ info_file=info_file,
+ pipeline=pipeline,
+ data_root=root_path,
+ test_mode=True)
+
+ # format 3D detection results
+ results = mmcv.load('tests/data/kitti/mono3d_sample_results.pkl')
+ results2d = mmcv.load('tests/data/kitti/mono3d_sample_results2d.pkl')
+ results[0]['img_bbox2d'] = results2d[0]['img_bbox2d']
+
+ metric = ['mAP']
+ ap_dict = kitti_dataset.evaluate(results, metric)
+ assert np.isclose(ap_dict['img_bbox/KITTI/Overall_3D_AP11_easy'], 3.0303)
+ assert np.isclose(ap_dict['img_bbox/KITTI/Overall_3D_AP11_moderate'],
+ 6.0606)
+ assert np.isclose(ap_dict['img_bbox/KITTI/Overall_3D_AP11_hard'], 6.0606)
+ assert np.isclose(ap_dict['img_bbox2d/KITTI/Overall_2D_AP11_easy'], 3.0303)
+ assert np.isclose(ap_dict['img_bbox2d/KITTI/Overall_2D_AP11_moderate'],
+ 6.0606)
+ assert np.isclose(ap_dict['img_bbox2d/KITTI/Overall_2D_AP11_hard'], 6.0606)
diff --git a/tests/test_data/test_datasets/test_lyft_dataset.py b/tests/test_data/test_datasets/test_lyft_dataset.py
new file mode 100644
index 0000000..29733e3
--- /dev/null
+++ b/tests/test_data/test_datasets/test_lyft_dataset.py
@@ -0,0 +1,192 @@
+# Copyright (c) OpenMMLab. All rights reserved.
+import tempfile
+
+import mmcv
+import numpy as np
+import torch
+
+from mmdet3d.core import limit_period
+from mmdet3d.datasets import LyftDataset
+
+
+def test_getitem():
+ np.random.seed(0)
+ torch.manual_seed(0)
+ root_path = './tests/data/lyft'
+ # in coordinate system refactor, this test file is modified
+ ann_file = './tests/data/lyft/lyft_infos.pkl'
+ class_names = ('car', 'truck', 'bus', 'emergency_vehicle', 'other_vehicle',
+ 'motorcycle', 'bicycle', 'pedestrian', 'animal')
+ point_cloud_range = [-80, -80, -10, 80, 80, 10]
+ pipelines = [
+ dict(
+ type='LoadPointsFromFile',
+ coord_type='LIDAR',
+ load_dim=5,
+ use_dim=5,
+ file_client_args=dict(backend='disk')),
+ dict(
+ type='LoadPointsFromMultiSweeps',
+ sweeps_num=2,
+ file_client_args=dict(backend='disk')),
+ dict(type='LoadAnnotations3D', with_bbox_3d=True, with_label_3d=True),
+ dict(
+ type='GlobalRotScaleTrans',
+ rot_range=[-0.523599, 0.523599],
+ scale_ratio_range=[0.85, 1.15],
+ translation_std=[0, 0, 0]),
+ dict(type='RandomFlip3D', flip_ratio_bev_horizontal=0.5),
+ dict(type='PointsRangeFilter', point_cloud_range=point_cloud_range),
+ dict(type='ObjectRangeFilter', point_cloud_range=point_cloud_range),
+ dict(type='PointShuffle'),
+ dict(type='DefaultFormatBundle3D', class_names=class_names),
+ dict(
+ type='Collect3D', keys=['points', 'gt_bboxes_3d', 'gt_labels_3d'])
+ ]
+ lyft_dataset = LyftDataset(ann_file, pipelines, root_path)
+ data = lyft_dataset[0]
+ points = data['points']._data
+ gt_bboxes_3d = data['gt_bboxes_3d']._data
+ gt_labels_3d = data['gt_labels_3d']._data
+ pts_filename = data['img_metas']._data['pts_filename']
+ pcd_horizontal_flip = data['img_metas']._data['pcd_horizontal_flip']
+ pcd_scale_factor = data['img_metas']._data['pcd_scale_factor']
+ pcd_rotation = data['img_metas']._data['pcd_rotation']
+ pcd_rotation_angle = data['img_metas']._data['pcd_rotation_angle']
+ sample_idx = data['img_metas']._data['sample_idx']
+ # coord sys refactor
+ pcd_rotation_expected = np.array([[0.99869376, 0.05109515, 0.],
+ [-0.05109515, 0.99869376, 0.],
+ [0., 0., 1.]])
+ assert pts_filename == \
+ 'tests/data/lyft/lidar/host-a017_lidar1_1236118886901125926.bin'
+ assert pcd_horizontal_flip is True
+ assert abs(pcd_scale_factor - 1.0645568099117257) < 1e-5
+ assert np.allclose(pcd_rotation, pcd_rotation_expected, 1e-3)
+ assert sample_idx == \
+ 'b98a05255ba2632e957884758cb31f0e6fcc8d3cd6ee76b6d0ba55b72f08fc54'
+ expected_points = torch.tensor([[61.4785, -3.7393, 6.7699, 0.4001],
+ [47.7904, -3.9887, 6.0926, 0.0000],
+ [52.5683, -4.2178, 6.7179, 0.0000],
+ [52.4867, -4.0315, 6.7057, 0.0000],
+ [59.8372, -1.7366, 6.5864, 0.4001],
+ [53.0842, -3.7064, 6.7811, 0.0000],
+ [60.5549, -3.4978, 6.6578, 0.4001],
+ [59.1695, -1.2910, 7.0296, 0.2000],
+ [53.0702, -3.8868, 6.7807, 0.0000],
+ [47.9579, -4.1648, 5.6219, 0.2000],
+ [59.8226, -1.5522, 6.5867, 0.4001],
+ [61.2858, -4.2254, 7.3089, 0.2000],
+ [49.9896, -4.5202, 5.8823, 0.2000],
+ [61.4597, -4.6402, 7.3340, 0.2000],
+ [59.8244, -1.3499, 6.5895, 0.4001]])
+ expected_gt_bboxes_3d = torch.tensor(
+ [[63.2257, 17.5206, -0.6307, 2.0109, 5.1652, 1.9471, -1.5868],
+ [-25.3804, 27.4598, -2.3297, 2.7412, 8.4792, 3.4343, -1.5939],
+ [-15.2098, -7.0109, -2.2566, 0.7931, 0.8410, 1.7916, 1.5090]])
+ expected_gt_labels = np.array([0, 4, 7])
+ original_classes = lyft_dataset.CLASSES
+
+ # manually go through pipeline
+ expected_points[:, :3] = (
+ (expected_points[:, :3] * torch.tensor([1, -1, 1]))
+ @ pcd_rotation_expected @ pcd_rotation_expected) * torch.tensor(
+ [1, -1, 1])
+ expected_gt_bboxes_3d[:, :3] = (
+ (expected_gt_bboxes_3d[:, :3] * torch.tensor([1, -1, 1]))
+ @ pcd_rotation_expected @ pcd_rotation_expected) * torch.tensor(
+ [1, -1, 1])
+ expected_gt_bboxes_3d[:, 3:6] = expected_gt_bboxes_3d[:, [4, 3, 5]]
+ expected_gt_bboxes_3d[:, 6:] = -expected_gt_bboxes_3d[:, 6:] \
+ - np.pi / 2 - pcd_rotation_angle * 2
+ expected_gt_bboxes_3d[:, 6:] = limit_period(
+ expected_gt_bboxes_3d[:, 6:], period=np.pi * 2)
+
+ assert torch.allclose(points, expected_points, 1e-2)
+ assert torch.allclose(gt_bboxes_3d.tensor, expected_gt_bboxes_3d, 1e-3)
+ assert np.all(gt_labels_3d.numpy() == expected_gt_labels)
+ assert original_classes == class_names
+
+ lyft_dataset = LyftDataset(
+ ann_file, None, root_path, classes=['car', 'pedestrian'])
+ assert lyft_dataset.CLASSES != original_classes
+ assert lyft_dataset.CLASSES == ['car', 'pedestrian']
+
+ lyft_dataset = LyftDataset(
+ ann_file, None, root_path, classes=('car', 'pedestrian'))
+ assert lyft_dataset.CLASSES != original_classes
+ assert lyft_dataset.CLASSES == ('car', 'pedestrian')
+
+ import tempfile
+ with tempfile.TemporaryDirectory() as tmpdir:
+ path = tmpdir + 'classes.txt'
+ with open(path, 'w') as f:
+ f.write('car\npedestrian\n')
+
+ lyft_dataset = LyftDataset(ann_file, None, root_path, classes=path)
+ assert lyft_dataset.CLASSES != original_classes
+ assert lyft_dataset.CLASSES == ['car', 'pedestrian']
+
+
+def test_evaluate():
+ root_path = './tests/data/lyft'
+ # in coordinate system refactor, this test file is modified
+ ann_file = './tests/data/lyft/lyft_infos_val.pkl'
+ lyft_dataset = LyftDataset(ann_file, None, root_path)
+ # in coordinate system refactor, this test file is modified
+ results = mmcv.load('./tests/data/lyft/sample_results.pkl')
+ ap_dict = lyft_dataset.evaluate(results, 'bbox')
+ car_precision = ap_dict['pts_bbox_Lyft/car_AP']
+ assert car_precision == 0.6
+
+
+def test_show():
+ from os import path as osp
+
+ import mmcv
+
+ from mmdet3d.core.bbox import LiDARInstance3DBoxes
+ tmp_dir = tempfile.TemporaryDirectory()
+ temp_dir = tmp_dir.name
+ root_path = './tests/data/lyft'
+ ann_file = './tests/data/lyft/lyft_infos.pkl'
+ class_names = ('car', 'truck', 'bus', 'emergency_vehicle', 'other_vehicle',
+ 'motorcycle', 'bicycle', 'pedestrian', 'animal')
+ eval_pipeline = [
+ dict(
+ type='LoadPointsFromFile',
+ coord_type='LIDAR',
+ load_dim=5,
+ use_dim=5,
+ file_client_args=dict(backend='disk')),
+ dict(
+ type='LoadPointsFromMultiSweeps',
+ sweeps_num=10,
+ file_client_args=dict(backend='disk')),
+ dict(
+ type='DefaultFormatBundle3D',
+ class_names=class_names,
+ with_label=False),
+ dict(type='Collect3D', keys=['points'])
+ ]
+ kitti_dataset = LyftDataset(ann_file, None, root_path)
+ boxes_3d = LiDARInstance3DBoxes(
+ torch.tensor(
+ [[46.1218, -4.6496, -0.9275, 1.4442, 0.5316, 1.7450, -2.7457],
+ [33.3189, 0.1981, 0.3136, 1.2301, 0.5656, 1.7985, 3.1401],
+ [46.1366, -4.6404, -0.9510, 1.6501, 0.5162, 1.7540, -2.9486],
+ [33.2646, 0.2297, 0.3446, 1.3365, 0.5746, 1.7947, -3.1138],
+ [58.9079, 16.6272, -1.5829, 3.9313, 1.5656, 1.4899, -3.1213]]))
+ scores_3d = torch.tensor([0.1815, 0.1663, 0.5792, 0.2194, 0.2780])
+ labels_3d = torch.tensor([0, 0, 1, 1, 2])
+ result = dict(boxes_3d=boxes_3d, scores_3d=scores_3d, labels_3d=labels_3d)
+ results = [dict(pts_bbox=result)]
+ kitti_dataset.show(results, temp_dir, show=False, pipeline=eval_pipeline)
+ file_name = 'host-a017_lidar1_1236118886901125926'
+ pts_file_path = osp.join(temp_dir, file_name, f'{file_name}_points.obj')
+ gt_file_path = osp.join(temp_dir, file_name, f'{file_name}_gt.obj')
+ pred_file_path = osp.join(temp_dir, file_name, f'{file_name}_pred.obj')
+ mmcv.check_file_exist(pts_file_path)
+ mmcv.check_file_exist(gt_file_path)
+ mmcv.check_file_exist(pred_file_path)
+ tmp_dir.cleanup()
diff --git a/tests/test_data/test_datasets/test_nuscene_dataset.py b/tests/test_data/test_datasets/test_nuscene_dataset.py
new file mode 100644
index 0000000..f7b7656
--- /dev/null
+++ b/tests/test_data/test_datasets/test_nuscene_dataset.py
@@ -0,0 +1,118 @@
+# Copyright (c) OpenMMLab. All rights reserved.
+import tempfile
+
+import numpy as np
+import torch
+
+from mmdet3d.datasets import NuScenesDataset
+
+
+def test_getitem():
+ np.random.seed(0)
+ point_cloud_range = [-50, -50, -5, 50, 50, 3]
+ file_client_args = dict(backend='disk')
+ class_names = [
+ 'car', 'truck', 'trailer', 'bus', 'construction_vehicle', 'bicycle',
+ 'motorcycle', 'pedestrian', 'traffic_cone', 'barrier'
+ ]
+ pipeline = [
+ dict(
+ type='LoadPointsFromFile',
+ coord_type='LIDAR',
+ load_dim=5,
+ use_dim=5,
+ file_client_args=file_client_args),
+ dict(
+ type='LoadPointsFromMultiSweeps',
+ sweeps_num=2,
+ file_client_args=file_client_args),
+ dict(
+ type='MultiScaleFlipAug3D',
+ img_scale=(1333, 800),
+ pts_scale_ratio=1,
+ flip=False,
+ transforms=[
+ dict(
+ type='GlobalRotScaleTrans',
+ rot_range=[0, 0],
+ scale_ratio_range=[1., 1.],
+ translation_std=[0, 0, 0]),
+ dict(type='RandomFlip3D'),
+ dict(
+ type='PointsRangeFilter',
+ point_cloud_range=point_cloud_range),
+ dict(
+ type='DefaultFormatBundle3D',
+ class_names=class_names,
+ with_label=False),
+ dict(type='Collect3D', keys=['points'])
+ ])
+ ]
+
+ nus_dataset = NuScenesDataset(
+ 'tests/data/nuscenes/nus_info.pkl',
+ pipeline,
+ 'tests/data/nuscenes',
+ test_mode=True)
+ data = nus_dataset[0]
+ assert data['img_metas'][0].data['flip'] is False
+ assert data['img_metas'][0].data['pcd_horizontal_flip'] is False
+ assert data['points'][0]._data.shape == (100, 4)
+
+ data = nus_dataset[1]
+ assert data['img_metas'][0].data['flip'] is False
+ assert data['img_metas'][0].data['pcd_horizontal_flip'] is False
+ assert data['points'][0]._data.shape == (597, 4)
+
+
+def test_show():
+ from os import path as osp
+
+ import mmcv
+
+ from mmdet3d.core.bbox import LiDARInstance3DBoxes
+ tmp_dir = tempfile.TemporaryDirectory()
+ temp_dir = tmp_dir.name
+ class_names = [
+ 'car', 'truck', 'trailer', 'bus', 'construction_vehicle', 'bicycle',
+ 'motorcycle', 'pedestrian', 'traffic_cone', 'barrier'
+ ]
+ eval_pipeline = [
+ dict(
+ type='LoadPointsFromFile',
+ coord_type='LIDAR',
+ load_dim=5,
+ use_dim=5,
+ file_client_args=dict(backend='disk')),
+ dict(
+ type='LoadPointsFromMultiSweeps',
+ sweeps_num=10,
+ file_client_args=dict(backend='disk')),
+ dict(
+ type='DefaultFormatBundle3D',
+ class_names=class_names,
+ with_label=False),
+ dict(type='Collect3D', keys=['points'])
+ ]
+ nus_dataset = NuScenesDataset('tests/data/nuscenes/nus_info.pkl', None,
+ 'tests/data/nuscenes')
+ boxes_3d = LiDARInstance3DBoxes(
+ torch.tensor(
+ [[46.1218, -4.6496, -0.9275, 0.5316, 1.4442, 1.7450, 1.1749],
+ [33.3189, 0.1981, 0.3136, 0.5656, 1.2301, 1.7985, 1.5723],
+ [46.1366, -4.6404, -0.9510, 0.5162, 1.6501, 1.7540, 1.3778],
+ [33.2646, 0.2297, 0.3446, 0.5746, 1.3365, 1.7947, 1.5430],
+ [58.9079, 16.6272, -1.5829, 1.5656, 3.9313, 1.4899, 1.5505]]))
+ scores_3d = torch.tensor([0.1815, 0.1663, 0.5792, 0.2194, 0.2780])
+ labels_3d = torch.tensor([0, 0, 1, 1, 2])
+ result = dict(boxes_3d=boxes_3d, scores_3d=scores_3d, labels_3d=labels_3d)
+ results = [dict(pts_bbox=result)]
+ nus_dataset.show(results, temp_dir, show=False, pipeline=eval_pipeline)
+ file_name = 'n015-2018-08-02-17-16-37+0800__LIDAR_TOP__1533201470948018'
+ pts_file_path = osp.join(temp_dir, file_name, f'{file_name}_points.obj')
+ gt_file_path = osp.join(temp_dir, file_name, f'{file_name}_gt.obj')
+ pred_file_path = osp.join(temp_dir, file_name, f'{file_name}_pred.obj')
+ mmcv.check_file_exist(pts_file_path)
+ mmcv.check_file_exist(gt_file_path)
+ mmcv.check_file_exist(pred_file_path)
+ tmp_dir.cleanup()
diff --git a/tests/test_data/test_datasets/test_nuscenes_mono_dataset.py b/tests/test_data/test_datasets/test_nuscenes_mono_dataset.py
new file mode 100644
index 0000000..d3a37f4
--- /dev/null
+++ b/tests/test_data/test_datasets/test_nuscenes_mono_dataset.py
@@ -0,0 +1,191 @@
+# Copyright (c) OpenMMLab. All rights reserved.
+import tempfile
+from os import path as osp
+
+import mmcv
+import numpy as np
+import pytest
+import torch
+
+from mmdet3d.datasets import NuScenesMonoDataset
+
+
+def test_getitem():
+ np.random.seed(0)
+ class_names = [
+ 'car', 'truck', 'trailer', 'bus', 'construction_vehicle', 'bicycle',
+ 'motorcycle', 'pedestrian', 'traffic_cone', 'barrier'
+ ]
+ img_norm_cfg = dict(
+ mean=[102.9801, 115.9465, 122.7717], std=[1.0, 1.0, 1.0], to_rgb=False)
+ pipeline = [
+ dict(type='LoadImageFromFileMono3D'),
+ dict(
+ type='LoadAnnotations3D',
+ with_bbox=True,
+ with_label=True,
+ with_attr_label=True,
+ with_bbox_3d=True,
+ with_label_3d=True,
+ with_bbox_depth=True),
+ dict(type='Resize', img_scale=(1600, 900), keep_ratio=True),
+ dict(type='RandomFlip3D', flip_ratio_bev_horizontal=1.0),
+ dict(type='Normalize', **img_norm_cfg),
+ dict(type='Pad', size_divisor=32),
+ dict(type='DefaultFormatBundle3D', class_names=class_names),
+ dict(
+ type='Collect3D',
+ keys=[
+ 'img', 'gt_bboxes', 'gt_labels', 'attr_labels', 'gt_bboxes_3d',
+ 'gt_labels_3d', 'centers2d', 'depths'
+ ]),
+ ]
+
+ nus_dataset = NuScenesMonoDataset(
+ ann_file='tests/data/nuscenes/nus_infos_mono3d.coco.json',
+ pipeline=pipeline,
+ data_root='tests/data/nuscenes/',
+ img_prefix='tests/data/nuscenes/',
+ test_mode=False)
+
+ data = nus_dataset[0]
+ img_metas = data['img_metas']._data
+ filename = img_metas['filename']
+ img_shape = img_metas['img_shape']
+ pad_shape = img_metas['pad_shape']
+ flip = img_metas['flip']
+ bboxes = data['gt_bboxes']._data
+ attrs = data['attr_labels']._data
+ labels3d = data['gt_labels_3d']._data
+ labels = data['gt_labels']._data
+ centers2d = data['centers2d']._data
+ depths = data['depths']._data
+
+ expected_filename = 'tests/data/nuscenes/samples/CAM_BACK_LEFT/' + \
+ 'n015-2018-07-18-11-07-57+0800__CAM_BACK_LEFT__1531883530447423.jpg'
+ expected_img_shape = (900, 1600, 3)
+ expected_pad_shape = (928, 1600, 3)
+ expected_flip = True
+ expected_bboxes = torch.tensor([[485.4207, 513.7568, 515.4637, 576.1393],
+ [748.9482, 512.0452, 776.4941, 571.6310],
+ [432.1318, 427.8805, 508.4290, 578.1468],
+ [367.3779, 427.7682, 439.4244, 578.8904],
+ [592.8713, 515.0040, 623.4984, 575.0945]])
+ expected_attr_labels = torch.tensor([8, 8, 4, 4, 8])
+ expected_labels = torch.tensor([8, 8, 7, 7, 8])
+ expected_centers2d = torch.tensor([[500.6090, 544.6358],
+ [762.8789, 541.5280],
+ [471.1633, 502.2295],
+ [404.1957, 502.5908],
+ [608.3627, 544.7317]])
+ expected_depths = torch.tensor(
+ [15.3193, 15.6073, 14.7567, 14.8803, 15.4923])
+
+ assert filename == expected_filename
+ assert img_shape == expected_img_shape
+ assert pad_shape == expected_pad_shape
+ assert flip == expected_flip
+ assert torch.allclose(bboxes, expected_bboxes, 1e-5)
+ assert torch.all(attrs == expected_attr_labels)
+ assert torch.all(labels == expected_labels)
+ assert torch.all(labels3d == expected_labels)
+ assert torch.allclose(centers2d, expected_centers2d, 1e-5)
+ assert torch.allclose(depths, expected_depths, 1e-5)
+
+
+def test_format_results():
+ if not torch.cuda.is_available():
+ pytest.skip('test requires GPU and torch+cuda')
+ root_path = 'tests/data/nuscenes/'
+ ann_file = 'tests/data/nuscenes/nus_infos_mono3d.coco.json'
+ class_names = [
+ 'car', 'truck', 'trailer', 'bus', 'construction_vehicle', 'bicycle',
+ 'motorcycle', 'pedestrian', 'traffic_cone', 'barrier'
+ ]
+ pipeline = [
+ dict(type='LoadImageFromFileMono3D'),
+ dict(
+ type='LoadAnnotations3D',
+ with_bbox=True,
+ with_label=True,
+ with_attr_label=True,
+ with_bbox_3d=True,
+ with_label_3d=True,
+ with_bbox_depth=True),
+ dict(type='Resize', img_scale=(1600, 900), keep_ratio=True),
+ dict(type='Pad', size_divisor=32),
+ dict(type='DefaultFormatBundle3D', class_names=class_names),
+ dict(
+ type='Collect3D',
+ keys=[
+ 'img', 'gt_bboxes', 'gt_labels', 'attr_labels', 'gt_bboxes_3d',
+ 'gt_labels_3d', 'centers2d', 'depths'
+ ]),
+ ]
+ nus_dataset = NuScenesMonoDataset(
+ ann_file=ann_file,
+ pipeline=pipeline,
+ data_root=root_path,
+ test_mode=True)
+ results = mmcv.load('tests/data/nuscenes/mono3d_sample_results.pkl')
+ result_files, tmp_dir = nus_dataset.format_results(results)
+ result_data = mmcv.load(result_files['img_bbox'])
+ assert len(result_data['results'].keys()) == 1
+ assert len(result_data['results']['e93e98b63d3b40209056d129dc53ceee']) == 8
+ det = result_data['results']['e93e98b63d3b40209056d129dc53ceee'][0]
+
+ expected_token = 'e93e98b63d3b40209056d129dc53ceee'
+ expected_trans = torch.tensor(
+ [1018.753821915645, 605.190386124652, 0.7266818822266328])
+ expected_size = torch.tensor([1.440000057220459, 1.6380000114440918, 4.25])
+ expected_rotation = torch.tensor([-0.5717, -0.0014, 0.0170, -0.8203])
+ expected_detname = 'car'
+ expected_attr = 'vehicle.moving'
+
+ assert det['sample_token'] == expected_token
+ assert torch.allclose(
+ torch.tensor(det['translation']), expected_trans, 1e-5)
+ assert torch.allclose(torch.tensor(det['size']), expected_size, 1e-5)
+ assert torch.allclose(
+ torch.tensor(det['rotation']), expected_rotation, atol=1e-4)
+ assert det['detection_name'] == expected_detname
+ assert det['attribute_name'] == expected_attr
+
+
+def test_show():
+ root_path = 'tests/data/nuscenes/'
+ ann_file = 'tests/data/nuscenes/nus_infos_mono3d.coco.json'
+ class_names = [
+ 'car', 'truck', 'trailer', 'bus', 'construction_vehicle', 'bicycle',
+ 'motorcycle', 'pedestrian', 'traffic_cone', 'barrier'
+ ]
+ eval_pipeline = [
+ dict(type='LoadImageFromFileMono3D'),
+ dict(
+ type='DefaultFormatBundle3D',
+ class_names=class_names,
+ with_label=False),
+ dict(type='Collect3D', keys=['img'])
+ ]
+ nus_dataset = NuScenesMonoDataset(
+ data_root=root_path,
+ ann_file=ann_file,
+ img_prefix='tests/data/nuscenes/',
+ test_mode=True,
+ pipeline=eval_pipeline)
+ results = mmcv.load('tests/data/nuscenes/mono3d_sample_results.pkl')
+ results = [results[0]]
+
+ # show with eval_pipeline
+ tmp_dir = tempfile.TemporaryDirectory()
+ temp_dir = tmp_dir.name
+ nus_dataset.show(results, temp_dir, show=False)
+ file_name = 'n015-2018-07-18-11-07-57+0800__' \
+ 'CAM_BACK_LEFT__1531883530447423'
+ img_file_path = osp.join(temp_dir, file_name, f'{file_name}_img.png')
+ gt_file_path = osp.join(temp_dir, file_name, f'{file_name}_gt.png')
+ pred_file_path = osp.join(temp_dir, file_name, f'{file_name}_pred.png')
+ mmcv.check_file_exist(img_file_path)
+ mmcv.check_file_exist(gt_file_path)
+ mmcv.check_file_exist(pred_file_path)
+ tmp_dir.cleanup()
diff --git a/tests/test_data/test_datasets/test_s3dis_dataset.py b/tests/test_data/test_datasets/test_s3dis_dataset.py
new file mode 100644
index 0000000..9466c30
--- /dev/null
+++ b/tests/test_data/test_datasets/test_s3dis_dataset.py
@@ -0,0 +1,404 @@
+# Copyright (c) OpenMMLab. All rights reserved.
+import numpy as np
+import pytest
+import torch
+
+from mmdet3d.datasets import (
+ S3DISDataset, S3DISSegDataset, S3DISInstanceSegDataset)
+
+
+def test_getitem():
+ np.random.seed(0)
+ root_path = './tests/data/s3dis/'
+ ann_file = './tests/data/s3dis/s3dis_infos.pkl'
+ class_names = ('table', 'chair', 'sofa', 'bookcase', 'board')
+ pipeline = [
+ dict(
+ type='LoadPointsFromFile',
+ coord_type='DEPTH',
+ shift_height=False,
+ load_dim=6,
+ use_dim=[0, 1, 2, 3, 4, 5]),
+ dict(type='LoadAnnotations3D', with_bbox_3d=True, with_label_3d=True),
+ dict(type='PointSample', num_points=40000),
+ dict(type='DefaultFormatBundle3D', class_names=class_names),
+ dict(
+ type='Collect3D', keys=['points', 'gt_bboxes_3d', 'gt_labels_3d'])
+ ]
+ s3dis_dataset = S3DISDataset(
+ data_root=root_path, ann_file=ann_file, pipeline=pipeline)
+
+ data = s3dis_dataset[0]
+ points = data['points']._data
+ gt_bboxes_3d = data['gt_bboxes_3d']._data
+ gt_labels_3d = data['gt_labels_3d']._data
+ expected_gt_bboxes_3d = torch.tensor(
+ [[2.3080, 2.4175, 0.2010, 0.8820, 0.8690, 0.6970, 0.0000],
+ [2.4730, 0.7090, 0.2010, 0.9080, 0.9620, 0.7030, 0.0000],
+ [5.3235, 0.4910, 0.0740, 0.8410, 0.9020, 0.8790, 0.0000]])
+ expected_gt_labels = np.array([1, 1, 3, 1, 2, 0, 0, 0, 3])
+
+ assert tuple(points.shape) == (40000, 6)
+ assert torch.allclose(gt_bboxes_3d[:3].tensor, expected_gt_bboxes_3d, 1e-2)
+ assert np.all(gt_labels_3d.numpy() == expected_gt_labels)
+
+
+def test_evaluate():
+ if not torch.cuda.is_available():
+ pytest.skip()
+ from mmdet3d.core.bbox.structures import DepthInstance3DBoxes
+ root_path = './tests/data/s3dis'
+ ann_file = './tests/data/s3dis/s3dis_infos.pkl'
+ s3dis_dataset = S3DISDataset(root_path, ann_file)
+ results = []
+ pred_boxes = dict()
+ pred_boxes['boxes_3d'] = DepthInstance3DBoxes(
+ torch.tensor([[2.3080, 2.4175, 0.2010, 0.8820, 0.8690, 0.6970, 0.0000],
+ [2.4730, 0.7090, 0.2010, 0.9080, 0.9620, 0.7030, 0.0000],
+ [5.3235, 0.4910, 0.0740, 0.8410, 0.9020, 0.8790,
+ 0.0000]]))
+ pred_boxes['labels_3d'] = torch.tensor([1, 1, 3])
+ pred_boxes['scores_3d'] = torch.tensor([0.5, 1.0, 1.0])
+ results.append(pred_boxes)
+ ret_dict = s3dis_dataset.evaluate(results)
+ assert abs(ret_dict['chair_AP_0.25'] - 0.666) < 0.01
+ assert abs(ret_dict['chair_AP_0.50'] - 0.666) < 0.01
+ assert abs(ret_dict['bookcase_AP_0.25'] - 0.5) < 0.01
+ assert abs(ret_dict['bookcase_AP_0.50'] - 0.5) < 0.01
+
+
+def test_seg_getitem():
+ np.random.seed(0)
+ root_path = './tests/data/s3dis/'
+ ann_file = './tests/data/s3dis/s3dis_infos.pkl'
+ class_names = ('ceiling', 'floor', 'wall', 'beam', 'column', 'window',
+ 'door', 'table', 'chair', 'sofa', 'bookcase', 'board',
+ 'clutter')
+ palette = [[0, 255, 0], [0, 0, 255], [0, 255, 255], [255, 255, 0],
+ [255, 0, 255], [100, 100, 255], [200, 200, 100],
+ [170, 120, 200], [255, 0, 0], [200, 100, 100], [10, 200, 100],
+ [200, 200, 200], [50, 50, 50]]
+ scene_idxs = [0 for _ in range(20)]
+
+ pipelines = [
+ dict(
+ type='LoadPointsFromFile',
+ coord_type='DEPTH',
+ shift_height=False,
+ use_color=True,
+ load_dim=6,
+ use_dim=[0, 1, 2, 3, 4, 5]),
+ dict(
+ type='LoadAnnotations3D',
+ with_bbox_3d=False,
+ with_label_3d=False,
+ with_mask_3d=False,
+ with_seg_3d=True),
+ dict(
+ type='PointSegClassMapping',
+ valid_cat_ids=tuple(range(len(class_names))),
+ max_cat_id=13),
+ dict(
+ type='IndoorPatchPointSample',
+ num_points=5,
+ block_size=1.0,
+ ignore_index=len(class_names),
+ use_normalized_coord=True,
+ enlarge_size=0.2,
+ min_unique_num=None),
+ dict(type='NormalizePointsColor', color_mean=None),
+ dict(type='DefaultFormatBundle3D', class_names=class_names),
+ dict(
+ type='Collect3D',
+ keys=['points', 'pts_semantic_mask'],
+ meta_keys=['file_name', 'sample_idx'])
+ ]
+
+ s3dis_dataset = S3DISSegDataset(
+ data_root=root_path,
+ ann_files=ann_file,
+ pipeline=pipelines,
+ classes=None,
+ palette=None,
+ modality=None,
+ test_mode=False,
+ ignore_index=None,
+ scene_idxs=scene_idxs)
+
+ data = s3dis_dataset[0]
+ points = data['points']._data
+ pts_semantic_mask = data['pts_semantic_mask']._data
+
+ file_name = data['img_metas']._data['file_name']
+ sample_idx = data['img_metas']._data['sample_idx']
+
+ assert file_name == './tests/data/s3dis/points/Area_1_office_2.bin'
+ assert sample_idx == 'Area_1_office_2'
+ expected_points = torch.tensor([[
+ 0.0000, 0.0000, 3.1720, 0.4706, 0.4431, 0.3725, 0.4624, 0.7502, 0.9543
+ ], [
+ 0.2880, -0.5900, 0.0650, 0.3451, 0.3373, 0.3490, 0.5119, 0.5518, 0.0196
+ ], [
+ 0.1570, 0.6000, 3.1700, 0.4941, 0.4667, 0.3569, 0.4893, 0.9519, 0.9537
+ ], [
+ -0.1320, 0.3950, 0.2720, 0.3216, 0.2863, 0.2275, 0.4397, 0.8830, 0.0818
+ ],
+ [
+ -0.4860, -0.0640, 3.1710, 0.3843,
+ 0.3725, 0.3059, 0.3789, 0.7286, 0.9540
+ ]])
+ expected_pts_semantic_mask = np.array([0, 1, 0, 8, 0])
+ original_classes = s3dis_dataset.CLASSES
+ original_palette = s3dis_dataset.PALETTE
+
+ assert s3dis_dataset.CLASSES == class_names
+ assert s3dis_dataset.ignore_index == 13
+ assert torch.allclose(points, expected_points, 1e-2)
+ assert np.all(pts_semantic_mask.numpy() == expected_pts_semantic_mask)
+ assert original_classes == class_names
+ assert original_palette == palette
+ assert s3dis_dataset.scene_idxs.dtype == np.int32
+ assert np.all(s3dis_dataset.scene_idxs == np.array(scene_idxs))
+
+ # test dataset with selected classes
+ s3dis_dataset = S3DISSegDataset(
+ data_root=root_path,
+ ann_files=ann_file,
+ pipeline=None,
+ classes=['beam', 'window'],
+ scene_idxs=scene_idxs)
+
+ label_map = {i: 13 for i in range(14)}
+ label_map.update({3: 0, 5: 1})
+
+ assert s3dis_dataset.CLASSES != original_classes
+ assert s3dis_dataset.CLASSES == ['beam', 'window']
+ assert s3dis_dataset.PALETTE == [palette[3], palette[5]]
+ assert s3dis_dataset.VALID_CLASS_IDS == [3, 5]
+ assert s3dis_dataset.label_map == label_map
+ assert s3dis_dataset.label2cat == {0: 'beam', 1: 'window'}
+
+ # test load classes from file
+ import tempfile
+ with tempfile.TemporaryDirectory() as tmpdir:
+ path = tmpdir + 'classes.txt'
+ with open(path, 'w') as f:
+ f.write('beam\nwindow\n')
+
+ s3dis_dataset = S3DISSegDataset(
+ data_root=root_path,
+ ann_files=ann_file,
+ pipeline=None,
+ classes=path,
+ scene_idxs=scene_idxs)
+ assert s3dis_dataset.CLASSES != original_classes
+ assert s3dis_dataset.CLASSES == ['beam', 'window']
+ assert s3dis_dataset.PALETTE == [palette[3], palette[5]]
+ assert s3dis_dataset.VALID_CLASS_IDS == [3, 5]
+ assert s3dis_dataset.label_map == label_map
+ assert s3dis_dataset.label2cat == {0: 'beam', 1: 'window'}
+
+ # test scene_idxs in dataset
+ # we should input scene_idxs in train mode
+ with pytest.raises(NotImplementedError):
+ s3dis_dataset = S3DISSegDataset(
+ data_root=root_path,
+ ann_files=ann_file,
+ pipeline=None,
+ scene_idxs=None)
+
+ # test mode
+ s3dis_dataset = S3DISSegDataset(
+ data_root=root_path,
+ ann_files=ann_file,
+ pipeline=None,
+ test_mode=True,
+ scene_idxs=scene_idxs)
+ assert np.all(s3dis_dataset.scene_idxs == np.array([0]))
+
+
+def test_seg_evaluate():
+ if not torch.cuda.is_available():
+ pytest.skip()
+ root_path = './tests/data/s3dis'
+ ann_file = './tests/data/s3dis/s3dis_infos.pkl'
+ s3dis_dataset = S3DISSegDataset(
+ data_root=root_path, ann_files=ann_file, test_mode=True)
+ results = []
+ pred_sem_mask = dict(
+ semantic_mask=torch.tensor([
+ 2, 3, 1, 2, 2, 6, 1, 0, 1, 1, 9, 12, 3, 0, 2, 0, 2, 0, 8, 3, 1, 2,
+ 0, 2, 1, 7, 2, 10, 2, 0, 0, 0, 2, 3, 2, 2, 2, 2, 2, 3, 0, 0, 4, 6,
+ 7, 2, 1, 2, 0, 1, 7, 0, 2, 2, 2, 0, 2, 2, 1, 12, 0, 2, 2, 2, 2, 7,
+ 2, 2, 0, 2, 6, 2, 12, 6, 3, 12, 2, 1, 6, 1, 2, 6, 8, 2, 10, 1, 11,
+ 0, 6, 9, 4, 3, 0, 0, 12, 1, 1, 5, 3, 2
+ ]).long())
+ results.append(pred_sem_mask)
+ ret_dict = s3dis_dataset.evaluate(results)
+ assert abs(ret_dict['miou'] - 0.7625) < 0.01
+ assert abs(ret_dict['acc'] - 0.9) < 0.01
+ assert abs(ret_dict['acc_cls'] - 0.9074) < 0.01
+
+
+def test_seg_show():
+ import tempfile
+ from os import path as osp
+
+ import mmcv
+
+ tmp_dir = tempfile.TemporaryDirectory()
+ temp_dir = tmp_dir.name
+ root_path = './tests/data/s3dis'
+ ann_file = './tests/data/s3dis/s3dis_infos.pkl'
+ s3dis_dataset = S3DISSegDataset(
+ data_root=root_path, ann_files=ann_file, scene_idxs=[0])
+ result = dict(
+ semantic_mask=torch.tensor([
+ 2, 2, 1, 2, 2, 5, 1, 0, 1, 1, 9, 12, 3, 0, 2, 0, 2, 0, 8, 2, 0, 2,
+ 0, 2, 1, 7, 2, 10, 2, 0, 0, 0, 2, 2, 2, 2, 2, 1, 2, 2, 0, 0, 4, 6,
+ 7, 2, 1, 2, 0, 1, 7, 0, 2, 2, 2, 0, 2, 2, 1, 12, 0, 2, 2, 2, 2, 7,
+ 2, 2, 0, 2, 6, 2, 12, 6, 2, 12, 2, 1, 6, 1, 2, 6, 8, 2, 10, 1, 10,
+ 0, 6, 9, 4, 3, 0, 0, 12, 1, 1, 5, 2, 2
+ ]).long())
+ results = [result]
+ s3dis_dataset.show(results, temp_dir, show=False)
+ pts_file_path = osp.join(temp_dir, 'Area_1_office_2',
+ 'Area_1_office_2_points.obj')
+ gt_file_path = osp.join(temp_dir, 'Area_1_office_2',
+ 'Area_1_office_2_gt.obj')
+ pred_file_path = osp.join(temp_dir, 'Area_1_office_2',
+ 'Area_1_office_2_pred.obj')
+ mmcv.check_file_exist(pts_file_path)
+ mmcv.check_file_exist(gt_file_path)
+ mmcv.check_file_exist(pred_file_path)
+ tmp_dir.cleanup()
+ # test show with pipeline
+ tmp_dir = tempfile.TemporaryDirectory()
+ temp_dir = tmp_dir.name
+ class_names = ('ceiling', 'floor', 'wall', 'beam', 'column', 'window',
+ 'door', 'table', 'chair', 'sofa', 'bookcase', 'board',
+ 'clutter')
+ eval_pipeline = [
+ dict(
+ type='LoadPointsFromFile',
+ coord_type='DEPTH',
+ shift_height=False,
+ use_color=True,
+ load_dim=6,
+ use_dim=[0, 1, 2, 3, 4, 5]),
+ dict(
+ type='LoadAnnotations3D',
+ with_bbox_3d=False,
+ with_label_3d=False,
+ with_mask_3d=False,
+ with_seg_3d=True),
+ dict(
+ type='PointSegClassMapping',
+ valid_cat_ids=tuple(range(len(class_names))),
+ max_cat_id=13),
+ dict(
+ type='DefaultFormatBundle3D',
+ with_label=False,
+ class_names=class_names),
+ dict(type='Collect3D', keys=['points', 'pts_semantic_mask'])
+ ]
+ s3dis_dataset.show(results, temp_dir, show=False, pipeline=eval_pipeline)
+ pts_file_path = osp.join(temp_dir, 'Area_1_office_2',
+ 'Area_1_office_2_points.obj')
+ gt_file_path = osp.join(temp_dir, 'Area_1_office_2',
+ 'Area_1_office_2_gt.obj')
+ pred_file_path = osp.join(temp_dir, 'Area_1_office_2',
+ 'Area_1_office_2_pred.obj')
+ mmcv.check_file_exist(pts_file_path)
+ mmcv.check_file_exist(gt_file_path)
+ mmcv.check_file_exist(pred_file_path)
+ tmp_dir.cleanup()
+
+
+def test_multi_areas():
+ # S3DIS dataset has 6 areas, we often train on several of them
+ # need to verify the concat function of S3DISSegDataset
+ root_path = './tests/data/s3dis'
+ ann_file = './tests/data/s3dis/s3dis_infos.pkl'
+ class_names = ('ceiling', 'floor', 'wall', 'beam', 'column', 'window',
+ 'door', 'table', 'chair', 'sofa', 'bookcase', 'board',
+ 'clutter')
+ palette = [[0, 255, 0], [0, 0, 255], [0, 255, 255], [255, 255, 0],
+ [255, 0, 255], [100, 100, 255], [200, 200, 100],
+ [170, 120, 200], [255, 0, 0], [200, 100, 100], [10, 200, 100],
+ [200, 200, 200], [50, 50, 50]]
+ scene_idxs = [0 for _ in range(20)]
+
+ # repeat
+ repeat_num = 3
+ s3dis_dataset = S3DISSegDataset(
+ data_root=root_path,
+ ann_files=[ann_file for _ in range(repeat_num)],
+ scene_idxs=scene_idxs)
+ assert s3dis_dataset.CLASSES == class_names
+ assert s3dis_dataset.PALETTE == palette
+ assert len(s3dis_dataset.data_infos) == repeat_num
+ assert np.all(s3dis_dataset.scene_idxs == np.concatenate(
+ [np.array(scene_idxs) + i for i in range(repeat_num)]))
+
+ # different scene_idxs input
+ s3dis_dataset = S3DISSegDataset(
+ data_root=root_path,
+ ann_files=[ann_file for _ in range(repeat_num)],
+ scene_idxs=[[0, 0, 1, 2, 2], [0, 1, 2, 3, 3, 4], [0, 1, 1, 2, 2, 2]])
+ assert np.all(s3dis_dataset.scene_idxs == np.array(
+ [0, 0, 1, 2, 2, 3, 4, 5, 6, 6, 7, 8, 9, 9, 10, 10, 10]))
+
+
+def test_instance_seg_evaluate():
+ np.random.seed(0)
+ root_path = './tests/data/s3dis/'
+ ann_file = './tests/data/s3dis/s3dis_infos.pkl'
+ class_names = ('table', 'chair', 'sofa', 'bookcase', 'board')
+ pipeline = [
+ dict(
+ type='LoadPointsFromFile',
+ coord_type='DEPTH',
+ shift_height=False,
+ use_color=True,
+ load_dim=6,
+ use_dim=[0, 1, 2, 3, 4, 5]),
+ dict(type='NormalizePointsColor', color_mean=None),
+ dict(type='DefaultFormatBundle3D', class_names=class_names),
+ dict(type='Collect3D', keys=['points'])]
+ s3dis_dataset = S3DISInstanceSegDataset(
+ data_root=root_path,
+ ann_file=ann_file,
+ pipeline=pipeline,
+ test_mode=True)
+
+ pred_mask = torch.tensor([
+ 1, 3, 2, 1, 3, 20, 2, 17, 2, 2, 23, 21, 18, 17, 22, 17, 3, 17,
+ 19, 14, 17, 3, 17, 3, 2, 8, 22, 9, 22, 17, 17, 17, 14, 3, 14, 22,
+ 3, 2, 22, 3, 17, 17, 24, 27, 11, 22, 2, 3, 17, 2, 11, 17, 22, 22,
+ 1, 17, 14, 22, 2, 13, 17, 3, 3, 1, 3, 10, 3, 1, 17, 22, 27, 3,
+ 11, 27, 22, 11, 22, 2, 27, 2, 3, 27, 19, 3, 9, 2, 9, 17, 27, 23,
+ 24, 18, 17, 17, 16, 2, 2, 20, 3, 22]).long()
+ pred_mask = torch.nn.functional.one_hot(pred_mask + 1).T[1:].bool()
+ pred_labels = torch.tensor(
+ [0, 0, 0, 0, 0, 0, 0, 0, 1, 3, 0, 0, 0, 0, 0, 0, 0, 0, 0, 1, 0, 0,
+ 0, 2, 0, 0, 0, 0]).long()
+ pred_scores = torch.tensor([.99 for _ in range(len(pred_labels))])
+ results = [
+ dict(
+ instance_mask=pred_mask,
+ instance_label=pred_labels,
+ instance_score=torch.tensor(pred_scores))
+ ]
+ # We add options here as default min_region_size
+ # is much bigger than test instances.
+ ret_dict = s3dis_dataset.evaluate(
+ results,
+ options=dict(min_region_sizes=np.array([1])))
+ assert abs(ret_dict['all_ap'] - 0.6875) < 0.001
+ assert abs(ret_dict['all_ap_50%'] - 0.68755) < 0.001
+ assert abs(ret_dict['all_ap_25%'] - 0.7812) < 0.001
+ assert abs(ret_dict['classes']['table']['ap25%'] - 0.3750) < 0.001
+ assert abs(ret_dict['classes']['table']['ap50%'] - 0.0) < 0.001
+ assert abs(ret_dict['classes']['chair']['ap25%'] - 0.75) < 0.001
+ assert abs(ret_dict['classes']['chair']['ap50%'] - 0.75) < 0.001
diff --git a/tests/test_data/test_datasets/test_scannet_dataset.py b/tests/test_data/test_datasets/test_scannet_dataset.py
new file mode 100644
index 0000000..c322c6f
--- /dev/null
+++ b/tests/test_data/test_datasets/test_scannet_dataset.py
@@ -0,0 +1,899 @@
+# Copyright (c) OpenMMLab. All rights reserved.
+import copy
+
+import numpy as np
+import pytest
+import torch
+
+from mmdet3d.datasets import (ScanNetDataset, ScanNetInstanceSegDataset,
+ ScanNetSegDataset, ScanNetInstanceSegV2Dataset)
+
+
+def test_getitem():
+ np.random.seed(0)
+ root_path = './tests/data/scannet/'
+ ann_file = './tests/data/scannet/scannet_infos.pkl'
+ class_names = ('cabinet', 'bed', 'chair', 'sofa', 'table', 'door',
+ 'window', 'bookshelf', 'picture', 'counter', 'desk',
+ 'curtain', 'refrigerator', 'showercurtrain', 'toilet',
+ 'sink', 'bathtub', 'garbagebin')
+ pipelines = [
+ dict(
+ type='LoadPointsFromFile',
+ coord_type='DEPTH',
+ shift_height=True,
+ load_dim=6,
+ use_dim=[0, 1, 2]),
+ dict(
+ type='LoadAnnotations3D',
+ with_bbox_3d=True,
+ with_label_3d=True,
+ with_mask_3d=True,
+ with_seg_3d=True),
+ dict(type='GlobalAlignment', rotation_axis=2),
+ dict(
+ type='PointSegClassMapping',
+ valid_cat_ids=(3, 4, 5, 6, 7, 8, 9, 10, 11, 12, 14, 16, 24, 28, 33,
+ 34, 36, 39)),
+ dict(type='PointSample', num_points=5),
+ dict(
+ type='RandomFlip3D',
+ sync_2d=False,
+ flip_ratio_bev_horizontal=1.0,
+ flip_ratio_bev_vertical=1.0),
+ dict(
+ type='GlobalRotScaleTrans',
+ rot_range=[-0.087266, 0.087266],
+ scale_ratio_range=[1.0, 1.0],
+ shift_height=True),
+ dict(type='DefaultFormatBundle3D', class_names=class_names),
+ dict(
+ type='Collect3D',
+ keys=[
+ 'points', 'gt_bboxes_3d', 'gt_labels_3d', 'pts_semantic_mask',
+ 'pts_instance_mask'
+ ],
+ meta_keys=['file_name', 'sample_idx', 'pcd_rotation']),
+ ]
+
+ scannet_dataset = ScanNetDataset(root_path, ann_file, pipelines)
+ data = scannet_dataset[0]
+ points = data['points']._data
+ gt_bboxes_3d = data['gt_bboxes_3d']._data
+ gt_labels = data['gt_labels_3d']._data
+ pts_semantic_mask = data['pts_semantic_mask']._data
+ pts_instance_mask = data['pts_instance_mask']._data
+ file_name = data['img_metas']._data['file_name']
+ pcd_rotation = data['img_metas']._data['pcd_rotation']
+ sample_idx = data['img_metas']._data['sample_idx']
+ expected_rotation = np.array([[0.99654, 0.08311407, 0.],
+ [-0.08311407, 0.99654, 0.], [0., 0., 1.]])
+ assert file_name == './tests/data/scannet/points/scene0000_00.bin'
+ assert np.allclose(pcd_rotation, expected_rotation, 1e-3)
+ assert sample_idx == 'scene0000_00'
+ expected_points = torch.tensor(
+ [[1.8339e+00, 2.1093e+00, 2.2900e+00, 2.3895e+00],
+ [3.6079e+00, 1.4592e-01, 2.0687e+00, 2.1682e+00],
+ [4.1886e+00, 5.0614e+00, -1.0841e-01, -8.8736e-03],
+ [6.8790e+00, 1.5086e+00, -9.3154e-02, 6.3816e-03],
+ [4.8253e+00, 2.6668e-01, 1.4917e+00, 1.5912e+00]])
+ expected_gt_bboxes_3d = torch.tensor(
+ [[-1.1835, -3.6317, 1.5704, 1.7577, 0.3761, 0.5724, 0.0000],
+ [-3.1832, 3.2269, 1.1911, 0.6727, 0.2251, 0.6715, 0.0000],
+ [-0.9598, -2.2864, 0.0093, 0.7506, 2.5709, 1.2145, 0.0000],
+ [-2.6988, -2.7354, 0.8288, 0.7680, 1.8877, 0.2870, 0.0000],
+ [3.2989, 0.2885, -0.0090, 0.7600, 3.8814, 2.1603, 0.0000]])
+ expected_gt_labels = np.array([
+ 6, 6, 4, 9, 11, 11, 10, 0, 15, 17, 17, 17, 3, 12, 4, 4, 14, 1, 0, 0, 0,
+ 0, 0, 0, 5, 5, 5
+ ])
+ expected_pts_semantic_mask = np.array([0, 18, 18, 18, 18])
+ expected_pts_instance_mask = np.array([44, 22, 10, 10, 57])
+ original_classes = scannet_dataset.CLASSES
+
+ assert scannet_dataset.CLASSES == class_names
+ assert torch.allclose(points, expected_points, 1e-2)
+ assert gt_bboxes_3d.tensor[:5].shape == (5, 7)
+ assert torch.allclose(gt_bboxes_3d.tensor[:5], expected_gt_bboxes_3d, 1e-2)
+ assert np.all(gt_labels.numpy() == expected_gt_labels)
+ assert np.all(pts_semantic_mask.numpy() == expected_pts_semantic_mask)
+ assert np.all(pts_instance_mask.numpy() == expected_pts_instance_mask)
+ assert original_classes == class_names
+
+ scannet_dataset = ScanNetDataset(
+ root_path, ann_file, pipeline=None, classes=['cabinet', 'bed'])
+ assert scannet_dataset.CLASSES != original_classes
+ assert scannet_dataset.CLASSES == ['cabinet', 'bed']
+
+ scannet_dataset = ScanNetDataset(
+ root_path, ann_file, pipeline=None, classes=('cabinet', 'bed'))
+ assert scannet_dataset.CLASSES != original_classes
+ assert scannet_dataset.CLASSES == ('cabinet', 'bed')
+
+ # Test load classes from file
+ import tempfile
+ with tempfile.TemporaryDirectory() as tmpdir:
+ path = tmpdir + 'classes.txt'
+ with open(path, 'w') as f:
+ f.write('cabinet\nbed\n')
+
+ scannet_dataset = ScanNetDataset(
+ root_path, ann_file, pipeline=None, classes=path)
+ assert scannet_dataset.CLASSES != original_classes
+ assert scannet_dataset.CLASSES == ['cabinet', 'bed']
+
+
+def test_evaluate():
+ if not torch.cuda.is_available():
+ pytest.skip()
+ from mmdet3d.core.bbox.structures import DepthInstance3DBoxes
+ root_path = './tests/data/scannet'
+ ann_file = './tests/data/scannet/scannet_infos.pkl'
+ scannet_dataset = ScanNetDataset(root_path, ann_file)
+ results = []
+ pred_boxes = dict()
+ pred_boxes['boxes_3d'] = DepthInstance3DBoxes(
+ torch.tensor([[
+ 1.4813e+00, 3.5207e+00, 1.5704e+00, 1.7445e+00, 2.3196e-01,
+ 5.7235e-01, 0.0000e+00
+ ],
+ [
+ 2.9040e+00, -3.4803e+00, 1.1911e+00, 6.6078e-01,
+ 1.7072e-01, 6.7154e-01, 0.0000e+00
+ ],
+ [
+ 1.1466e+00, 2.1987e+00, 9.2576e-03, 5.4184e-01,
+ 2.5346e+00, 1.2145e+00, 0.0000e+00
+ ],
+ [
+ 2.9168e+00, 2.5016e+00, 8.2875e-01, 6.1697e-01,
+ 1.8428e+00, 2.8697e-01, 0.0000e+00
+ ],
+ [
+ -3.3114e+00, -1.3351e-02, -8.9524e-03, 4.4082e-01,
+ 3.8582e+00, 2.1603e+00, 0.0000e+00
+ ],
+ [
+ -2.0135e+00, -3.4857e+00, 9.3848e-01, 1.9911e+00,
+ 2.1603e-01, 1.2767e+00, 0.0000e+00
+ ],
+ [
+ -2.1945e+00, -3.1402e+00, -3.8165e-02, 1.4801e+00,
+ 6.8676e-01, 1.0586e+00, 0.0000e+00
+ ],
+ [
+ -2.7553e+00, 2.4055e+00, -2.9972e-02, 1.4764e+00,
+ 1.4927e+00, 2.3380e+00, 0.0000e+00
+ ]]))
+ pred_boxes['labels_3d'] = torch.tensor([6, 6, 4, 9, 11, 11])
+ pred_boxes['scores_3d'] = torch.tensor([0.5, 1.0, 1.0, 1.0, 1.0, 0.5])
+ results.append(pred_boxes)
+ metric = [0.25, 0.5]
+ ret_dict = scannet_dataset.evaluate(results, metric)
+ assert abs(ret_dict['table_AP_0.25'] - 0.3333) < 0.01
+ assert abs(ret_dict['window_AP_0.25'] - 1.0) < 0.01
+ assert abs(ret_dict['counter_AP_0.25'] - 1.0) < 0.01
+ assert abs(ret_dict['curtain_AP_0.25'] - 1.0) < 0.01
+
+ # test evaluate with pipeline
+ class_names = ('cabinet', 'bed', 'chair', 'sofa', 'table', 'door',
+ 'window', 'bookshelf', 'picture', 'counter', 'desk',
+ 'curtain', 'refrigerator', 'showercurtrain', 'toilet',
+ 'sink', 'bathtub', 'garbagebin')
+ eval_pipeline = [
+ dict(
+ type='LoadPointsFromFile',
+ coord_type='DEPTH',
+ shift_height=False,
+ load_dim=6,
+ use_dim=[0, 1, 2]),
+ dict(type='GlobalAlignment', rotation_axis=2),
+ dict(
+ type='DefaultFormatBundle3D',
+ class_names=class_names,
+ with_label=False),
+ dict(type='Collect3D', keys=['points'])
+ ]
+ ret_dict = scannet_dataset.evaluate(
+ results, metric, pipeline=eval_pipeline)
+ assert abs(ret_dict['table_AP_0.25'] - 0.3333) < 0.01
+ assert abs(ret_dict['window_AP_0.25'] - 1.0) < 0.01
+ assert abs(ret_dict['counter_AP_0.25'] - 1.0) < 0.01
+ assert abs(ret_dict['curtain_AP_0.25'] - 1.0) < 0.01
+
+
+def test_show():
+ import tempfile
+ from os import path as osp
+
+ import mmcv
+
+ from mmdet3d.core.bbox import DepthInstance3DBoxes
+ tmp_dir = tempfile.TemporaryDirectory()
+ temp_dir = tmp_dir.name
+ root_path = './tests/data/scannet'
+ ann_file = './tests/data/scannet/scannet_infos.pkl'
+ scannet_dataset = ScanNetDataset(root_path, ann_file)
+ boxes_3d = DepthInstance3DBoxes(
+ torch.tensor([[
+ -2.4053e+00, 9.2295e-01, 8.0661e-02, 2.4054e+00, 2.1468e+00,
+ 8.5990e-01, 0.0000e+00
+ ],
+ [
+ -1.9341e+00, -2.0741e+00, 3.0698e-03, 3.2206e-01,
+ 2.5322e-01, 3.5144e-01, 0.0000e+00
+ ],
+ [
+ -3.6908e+00, 8.0684e-03, 2.6201e-01, 4.1515e-01,
+ 7.6489e-01, 5.3585e-01, 0.0000e+00
+ ],
+ [
+ 2.6332e+00, 8.5143e-01, -4.9964e-03, 3.0367e-01,
+ 1.3448e+00, 1.8329e+00, 0.0000e+00
+ ],
+ [
+ 2.0221e-02, 2.6153e+00, 1.5109e-02, 7.3335e-01,
+ 1.0429e+00, 1.0251e+00, 0.0000e+00
+ ]]))
+ scores_3d = torch.tensor(
+ [1.2058e-04, 2.3012e-03, 6.2324e-06, 6.6139e-06, 6.7965e-05])
+ labels_3d = torch.tensor([0, 0, 0, 0, 0])
+ result = dict(boxes_3d=boxes_3d, scores_3d=scores_3d, labels_3d=labels_3d)
+ results = [result]
+ scannet_dataset.show(results, temp_dir, show=False)
+ pts_file_path = osp.join(temp_dir, 'scene0000_00',
+ 'scene0000_00_points.obj')
+ gt_file_path = osp.join(temp_dir, 'scene0000_00', 'scene0000_00_gt.obj')
+ pred_file_path = osp.join(temp_dir, 'scene0000_00',
+ 'scene0000_00_pred.obj')
+ mmcv.check_file_exist(pts_file_path)
+ mmcv.check_file_exist(gt_file_path)
+ mmcv.check_file_exist(pred_file_path)
+ tmp_dir.cleanup()
+
+ # show function with pipeline
+ class_names = ('cabinet', 'bed', 'chair', 'sofa', 'table', 'door',
+ 'window', 'bookshelf', 'picture', 'counter', 'desk',
+ 'curtain', 'refrigerator', 'showercurtrain', 'toilet',
+ 'sink', 'bathtub', 'garbagebin')
+ eval_pipeline = [
+ dict(
+ type='LoadPointsFromFile',
+ coord_type='DEPTH',
+ shift_height=False,
+ load_dim=6,
+ use_dim=[0, 1, 2]),
+ dict(type='GlobalAlignment', rotation_axis=2),
+ dict(
+ type='DefaultFormatBundle3D',
+ class_names=class_names,
+ with_label=False),
+ dict(type='Collect3D', keys=['points'])
+ ]
+ tmp_dir = tempfile.TemporaryDirectory()
+ temp_dir = tmp_dir.name
+ scannet_dataset.show(results, temp_dir, show=False, pipeline=eval_pipeline)
+ pts_file_path = osp.join(temp_dir, 'scene0000_00',
+ 'scene0000_00_points.obj')
+ gt_file_path = osp.join(temp_dir, 'scene0000_00', 'scene0000_00_gt.obj')
+ pred_file_path = osp.join(temp_dir, 'scene0000_00',
+ 'scene0000_00_pred.obj')
+ mmcv.check_file_exist(pts_file_path)
+ mmcv.check_file_exist(gt_file_path)
+ mmcv.check_file_exist(pred_file_path)
+ tmp_dir.cleanup()
+
+
+def test_seg_getitem():
+ np.random.seed(0)
+ root_path = './tests/data/scannet/'
+ ann_file = './tests/data/scannet/scannet_infos.pkl'
+ class_names = ('wall', 'floor', 'cabinet', 'bed', 'chair', 'sofa', 'table',
+ 'door', 'window', 'bookshelf', 'picture', 'counter', 'desk',
+ 'curtain', 'refrigerator', 'showercurtrain', 'toilet',
+ 'sink', 'bathtub', 'otherfurniture')
+ palette = [
+ [174, 199, 232],
+ [152, 223, 138],
+ [31, 119, 180],
+ [255, 187, 120],
+ [188, 189, 34],
+ [140, 86, 75],
+ [255, 152, 150],
+ [214, 39, 40],
+ [197, 176, 213],
+ [148, 103, 189],
+ [196, 156, 148],
+ [23, 190, 207],
+ [247, 182, 210],
+ [219, 219, 141],
+ [255, 127, 14],
+ [158, 218, 229],
+ [44, 160, 44],
+ [112, 128, 144],
+ [227, 119, 194],
+ [82, 84, 163],
+ ]
+ scene_idxs = [0 for _ in range(20)]
+
+ # test network inputs are (xyz, rgb, normalized_xyz)
+ pipelines = [
+ dict(
+ type='LoadPointsFromFile',
+ coord_type='DEPTH',
+ shift_height=False,
+ use_color=True,
+ load_dim=6,
+ use_dim=[0, 1, 2, 3, 4, 5]),
+ dict(
+ type='LoadAnnotations3D',
+ with_bbox_3d=False,
+ with_label_3d=False,
+ with_mask_3d=False,
+ with_seg_3d=True),
+ dict(
+ type='PointSegClassMapping',
+ valid_cat_ids=(1, 2, 3, 4, 5, 6, 7, 8, 9, 10, 11, 12, 14, 16, 24,
+ 28, 33, 34, 36, 39),
+ max_cat_id=40),
+ dict(
+ type='IndoorPatchPointSample',
+ num_points=5,
+ block_size=1.5,
+ ignore_index=len(class_names),
+ use_normalized_coord=True,
+ enlarge_size=0.2,
+ min_unique_num=None),
+ dict(type='NormalizePointsColor', color_mean=None),
+ dict(type='DefaultFormatBundle3D', class_names=class_names),
+ dict(
+ type='Collect3D',
+ keys=['points', 'pts_semantic_mask'],
+ meta_keys=['file_name', 'sample_idx'])
+ ]
+
+ scannet_dataset = ScanNetSegDataset(
+ data_root=root_path,
+ ann_file=ann_file,
+ pipeline=pipelines,
+ classes=None,
+ palette=None,
+ modality=None,
+ test_mode=False,
+ ignore_index=None,
+ scene_idxs=scene_idxs)
+
+ data = scannet_dataset[0]
+ points = data['points']._data
+ pts_semantic_mask = data['pts_semantic_mask']._data
+ file_name = data['img_metas']._data['file_name']
+ sample_idx = data['img_metas']._data['sample_idx']
+
+ assert file_name == './tests/data/scannet/points/scene0000_00.bin'
+ assert sample_idx == 'scene0000_00'
+ expected_points = torch.tensor([[
+ 0.0000, 0.0000, 1.2427, 0.6118, 0.5529, 0.4471, -0.6462, -1.0046,
+ 0.4280
+ ],
+ [
+ 0.1553, -0.0074, 1.6077, 0.5882,
+ 0.6157, 0.5569, -0.6001, -1.0068,
+ 0.5537
+ ],
+ [
+ 0.1518, 0.6016, 0.6548, 0.1490, 0.1059,
+ 0.0431, -0.6012, -0.8309, 0.2255
+ ],
+ [
+ -0.7494, 0.1033, 0.6756, 0.5216,
+ 0.4353, 0.3333, -0.8687, -0.9748,
+ 0.2327
+ ],
+ [
+ -0.6836, -0.0203, 0.5884, 0.5765,
+ 0.5020, 0.4510, -0.8491, -1.0105,
+ 0.2027
+ ]])
+ expected_pts_semantic_mask = np.array([13, 13, 12, 2, 0])
+ original_classes = scannet_dataset.CLASSES
+ original_palette = scannet_dataset.PALETTE
+
+ assert scannet_dataset.CLASSES == class_names
+ assert scannet_dataset.ignore_index == 20
+ assert torch.allclose(points, expected_points, 1e-2)
+ assert np.all(pts_semantic_mask.numpy() == expected_pts_semantic_mask)
+ assert original_classes == class_names
+ assert original_palette == palette
+ assert scannet_dataset.scene_idxs.dtype == np.int32
+ assert np.all(scannet_dataset.scene_idxs == np.array(scene_idxs))
+
+ # test network inputs are (xyz, rgb)
+ np.random.seed(0)
+ new_pipelines = copy.deepcopy(pipelines)
+ new_pipelines[3] = dict(
+ type='IndoorPatchPointSample',
+ num_points=5,
+ block_size=1.5,
+ ignore_index=len(class_names),
+ use_normalized_coord=False,
+ enlarge_size=0.2,
+ min_unique_num=None)
+ scannet_dataset = ScanNetSegDataset(
+ data_root=root_path,
+ ann_file=ann_file,
+ pipeline=new_pipelines,
+ scene_idxs=scene_idxs)
+
+ data = scannet_dataset[0]
+ points = data['points']._data
+ assert torch.allclose(points, expected_points[:, :6], 1e-2)
+
+ # test network inputs are (xyz, normalized_xyz)
+ np.random.seed(0)
+ new_pipelines = copy.deepcopy(pipelines)
+ new_pipelines[0] = dict(
+ type='LoadPointsFromFile',
+ coord_type='DEPTH',
+ shift_height=False,
+ use_color=False,
+ load_dim=6,
+ use_dim=[0, 1, 2])
+ new_pipelines.remove(new_pipelines[4])
+ scannet_dataset = ScanNetSegDataset(
+ data_root=root_path,
+ ann_file=ann_file,
+ pipeline=new_pipelines,
+ scene_idxs=scene_idxs)
+
+ data = scannet_dataset[0]
+ points = data['points']._data
+ assert torch.allclose(points, expected_points[:, [0, 1, 2, 6, 7, 8]], 1e-2)
+
+ # test network inputs are (xyz,)
+ np.random.seed(0)
+ new_pipelines = copy.deepcopy(pipelines)
+ new_pipelines[0] = dict(
+ type='LoadPointsFromFile',
+ coord_type='DEPTH',
+ shift_height=False,
+ use_color=False,
+ load_dim=6,
+ use_dim=[0, 1, 2])
+ new_pipelines[3] = dict(
+ type='IndoorPatchPointSample',
+ num_points=5,
+ block_size=1.5,
+ ignore_index=len(class_names),
+ use_normalized_coord=False,
+ enlarge_size=0.2,
+ min_unique_num=None)
+ new_pipelines.remove(new_pipelines[4])
+ scannet_dataset = ScanNetSegDataset(
+ data_root=root_path,
+ ann_file=ann_file,
+ pipeline=new_pipelines,
+ scene_idxs=scene_idxs)
+
+ data = scannet_dataset[0]
+ points = data['points']._data
+ assert torch.allclose(points, expected_points[:, :3], 1e-2)
+
+ # test dataset with selected classes
+ scannet_dataset = ScanNetSegDataset(
+ data_root=root_path,
+ ann_file=ann_file,
+ pipeline=None,
+ classes=['cabinet', 'chair'],
+ scene_idxs=scene_idxs)
+
+ label_map = {i: 20 for i in range(41)}
+ label_map.update({3: 0, 5: 1})
+
+ assert scannet_dataset.CLASSES != original_classes
+ assert scannet_dataset.CLASSES == ['cabinet', 'chair']
+ assert scannet_dataset.PALETTE == [palette[2], palette[4]]
+ assert scannet_dataset.VALID_CLASS_IDS == [3, 5]
+ assert scannet_dataset.label_map == label_map
+ assert scannet_dataset.label2cat == {0: 'cabinet', 1: 'chair'}
+
+ # test load classes from file
+ import tempfile
+ with tempfile.TemporaryDirectory() as tmpdir:
+ path = tmpdir + 'classes.txt'
+ with open(path, 'w') as f:
+ f.write('cabinet\nchair\n')
+
+ scannet_dataset = ScanNetSegDataset(
+ data_root=root_path,
+ ann_file=ann_file,
+ pipeline=None,
+ classes=path,
+ scene_idxs=scene_idxs)
+ assert scannet_dataset.CLASSES != original_classes
+ assert scannet_dataset.CLASSES == ['cabinet', 'chair']
+ assert scannet_dataset.PALETTE == [palette[2], palette[4]]
+ assert scannet_dataset.VALID_CLASS_IDS == [3, 5]
+ assert scannet_dataset.label_map == label_map
+ assert scannet_dataset.label2cat == {0: 'cabinet', 1: 'chair'}
+
+ # test scene_idxs in dataset
+ # we should input scene_idxs in train mode
+ with pytest.raises(NotImplementedError):
+ scannet_dataset = ScanNetSegDataset(
+ data_root=root_path,
+ ann_file=ann_file,
+ pipeline=None,
+ scene_idxs=None)
+
+ # test mode
+ scannet_dataset = ScanNetSegDataset(
+ data_root=root_path,
+ ann_file=ann_file,
+ pipeline=None,
+ test_mode=True,
+ scene_idxs=scene_idxs)
+ assert np.all(scannet_dataset.scene_idxs == np.array([0]))
+
+
+def test_seg_evaluate():
+ if not torch.cuda.is_available():
+ pytest.skip()
+ root_path = './tests/data/scannet'
+ ann_file = './tests/data/scannet/scannet_infos.pkl'
+ scannet_dataset = ScanNetSegDataset(
+ data_root=root_path, ann_file=ann_file, test_mode=True)
+ results = []
+ pred_sem_mask = dict(
+ semantic_mask=torch.tensor([
+ 13, 5, 1, 2, 6, 2, 13, 1, 14, 2, 0, 0, 5, 5, 3, 0, 1, 14, 0, 0, 0,
+ 18, 6, 15, 13, 0, 2, 4, 0, 3, 16, 6, 13, 5, 13, 0, 0, 0, 0, 1, 7,
+ 3, 19, 12, 8, 0, 11, 0, 0, 1, 2, 13, 17, 1, 1, 1, 6, 2, 13, 19, 4,
+ 17, 0, 14, 1, 7, 2, 1, 7, 2, 0, 5, 17, 5, 0, 0, 3, 6, 5, 11, 1, 13,
+ 13, 2, 3, 1, 0, 13, 19, 1, 14, 5, 3, 1, 13, 1, 2, 3, 2, 1
+ ]).long())
+ results.append(pred_sem_mask)
+
+ class_names = ('wall', 'floor', 'cabinet', 'bed', 'chair', 'sofa', 'table',
+ 'door', 'window', 'bookshelf', 'picture', 'counter', 'desk',
+ 'curtain', 'refrigerator', 'showercurtrain', 'toilet',
+ 'sink', 'bathtub', 'otherfurniture')
+ eval_pipeline = [
+ dict(
+ type='LoadPointsFromFile',
+ coord_type='DEPTH',
+ shift_height=False,
+ use_color=True,
+ load_dim=6,
+ use_dim=[0, 1, 2, 3, 4, 5]),
+ dict(
+ type='LoadAnnotations3D',
+ with_bbox_3d=False,
+ with_label_3d=False,
+ with_mask_3d=False,
+ with_seg_3d=True),
+ dict(
+ type='PointSegClassMapping',
+ valid_cat_ids=(1, 2, 3, 4, 5, 6, 7, 8, 9, 10, 11, 12, 14, 16, 24,
+ 28, 33, 34, 36, 39),
+ max_cat_id=40),
+ dict(type='DefaultFormatBundle3D', class_names=class_names),
+ dict(type='Collect3D', keys=['points', 'pts_semantic_mask'])
+ ]
+ ret_dict = scannet_dataset.evaluate(results, pipeline=eval_pipeline)
+ assert abs(ret_dict['miou'] - 0.5308) < 0.01
+ assert abs(ret_dict['acc'] - 0.8219) < 0.01
+ assert abs(ret_dict['acc_cls'] - 0.7649) < 0.01
+
+
+def test_seg_show():
+ import tempfile
+ from os import path as osp
+
+ import mmcv
+
+ tmp_dir = tempfile.TemporaryDirectory()
+ temp_dir = tmp_dir.name
+ root_path = './tests/data/scannet'
+ ann_file = './tests/data/scannet/scannet_infos.pkl'
+ scannet_dataset = ScanNetSegDataset(
+ data_root=root_path, ann_file=ann_file, scene_idxs=[0])
+ result = dict(
+ semantic_mask=torch.tensor([
+ 13, 5, 1, 2, 6, 2, 13, 1, 14, 2, 0, 0, 5, 5, 3, 0, 1, 14, 0, 0, 0,
+ 18, 6, 15, 13, 0, 2, 4, 0, 3, 16, 6, 13, 5, 13, 0, 0, 0, 0, 1, 7,
+ 3, 19, 12, 8, 0, 11, 0, 0, 1, 2, 13, 17, 1, 1, 1, 6, 2, 13, 19, 4,
+ 17, 0, 14, 1, 7, 2, 1, 7, 2, 0, 5, 17, 5, 0, 0, 3, 6, 5, 11, 1, 13,
+ 13, 2, 3, 1, 0, 13, 19, 1, 14, 5, 3, 1, 13, 1, 2, 3, 2, 1
+ ]).long())
+ results = [result]
+ scannet_dataset.show(results, temp_dir, show=False)
+ pts_file_path = osp.join(temp_dir, 'scene0000_00',
+ 'scene0000_00_points.obj')
+ gt_file_path = osp.join(temp_dir, 'scene0000_00', 'scene0000_00_gt.obj')
+ pred_file_path = osp.join(temp_dir, 'scene0000_00',
+ 'scene0000_00_pred.obj')
+ mmcv.check_file_exist(pts_file_path)
+ mmcv.check_file_exist(gt_file_path)
+ mmcv.check_file_exist(pred_file_path)
+ tmp_dir.cleanup()
+ # test show with pipeline
+ tmp_dir = tempfile.TemporaryDirectory()
+ temp_dir = tmp_dir.name
+ class_names = ('wall', 'floor', 'cabinet', 'bed', 'chair', 'sofa', 'table',
+ 'door', 'window', 'bookshelf', 'picture', 'counter', 'desk',
+ 'curtain', 'refrigerator', 'showercurtrain', 'toilet',
+ 'sink', 'bathtub', 'otherfurniture')
+ eval_pipeline = [
+ dict(
+ type='LoadPointsFromFile',
+ coord_type='DEPTH',
+ shift_height=False,
+ use_color=True,
+ load_dim=6,
+ use_dim=[0, 1, 2, 3, 4, 5]),
+ dict(
+ type='LoadAnnotations3D',
+ with_bbox_3d=False,
+ with_label_3d=False,
+ with_mask_3d=False,
+ with_seg_3d=True),
+ dict(
+ type='PointSegClassMapping',
+ valid_cat_ids=(1, 2, 3, 4, 5, 6, 7, 8, 9, 10, 11, 12, 14, 16, 24,
+ 28, 33, 34, 36, 39),
+ max_cat_id=40),
+ dict(type='DefaultFormatBundle3D', class_names=class_names),
+ dict(type='Collect3D', keys=['points', 'pts_semantic_mask'])
+ ]
+ scannet_dataset.show(results, temp_dir, show=False, pipeline=eval_pipeline)
+ pts_file_path = osp.join(temp_dir, 'scene0000_00',
+ 'scene0000_00_points.obj')
+ gt_file_path = osp.join(temp_dir, 'scene0000_00', 'scene0000_00_gt.obj')
+ pred_file_path = osp.join(temp_dir, 'scene0000_00',
+ 'scene0000_00_pred.obj')
+ mmcv.check_file_exist(pts_file_path)
+ mmcv.check_file_exist(gt_file_path)
+ mmcv.check_file_exist(pred_file_path)
+ tmp_dir.cleanup()
+
+
+def test_seg_format_results():
+ from os import path as osp
+
+ import mmcv
+
+ root_path = './tests/data/scannet'
+ ann_file = './tests/data/scannet/scannet_infos.pkl'
+ scannet_dataset = ScanNetSegDataset(
+ data_root=root_path, ann_file=ann_file, test_mode=True)
+ results = []
+ pred_sem_mask = dict(
+ semantic_mask=torch.tensor([
+ 13, 5, 1, 2, 6, 2, 13, 1, 14, 2, 0, 0, 5, 5, 3, 0, 1, 14, 0, 0, 0,
+ 18, 6, 15, 13, 0, 2, 4, 0, 3, 16, 6, 13, 5, 13, 0, 0, 0, 0, 1, 7,
+ 3, 19, 12, 8, 0, 11, 0, 0, 1, 2, 13, 17, 1, 1, 1, 6, 2, 13, 19, 4,
+ 17, 0, 14, 1, 7, 2, 1, 7, 2, 0, 5, 17, 5, 0, 0, 3, 6, 5, 11, 1, 13,
+ 13, 2, 3, 1, 0, 13, 19, 1, 14, 5, 3, 1, 13, 1, 2, 3, 2, 1
+ ]).long())
+ results.append(pred_sem_mask)
+ result_files, tmp_dir = scannet_dataset.format_results(results)
+
+ expected_label = np.array([
+ 16, 6, 2, 3, 7, 3, 16, 2, 24, 3, 1, 1, 6, 6, 4, 1, 2, 24, 1, 1, 1, 36,
+ 7, 28, 16, 1, 3, 5, 1, 4, 33, 7, 16, 6, 16, 1, 1, 1, 1, 2, 8, 4, 39,
+ 14, 9, 1, 12, 1, 1, 2, 3, 16, 34, 2, 2, 2, 7, 3, 16, 39, 5, 34, 1, 24,
+ 2, 8, 3, 2, 8, 3, 1, 6, 34, 6, 1, 1, 4, 7, 6, 12, 2, 16, 16, 3, 4, 2,
+ 1, 16, 39, 2, 24, 6, 4, 2, 16, 2, 3, 4, 3, 2
+ ])
+ expected_txt_path = osp.join(tmp_dir.name, 'results', 'scene0000_00.txt')
+ assert np.all(result_files[0]['seg_mask'] == expected_label)
+ mmcv.check_file_exist(expected_txt_path)
+
+
+def test_instance_seg_getitem():
+ np.random.seed(0)
+ root_path = './tests/data/scannet/'
+ ann_file = './tests/data/scannet/scannet_infos.pkl'
+ class_names = ('cabinet', 'bed', 'chair', 'sofa', 'table', 'door',
+ 'window', 'bookshelf', 'picture', 'counter', 'desk',
+ 'curtain', 'refrigerator', 'showercurtrain', 'toilet',
+ 'sink', 'bathtub', 'garbagebin')
+ train_pipeline = [
+ dict(
+ type='LoadPointsFromFile',
+ coord_type='DEPTH',
+ shift_height=False,
+ use_color=True,
+ load_dim=6,
+ use_dim=[0, 1, 2, 3, 4, 5]),
+ dict(
+ type='LoadAnnotations3D',
+ with_bbox_3d=False,
+ with_label_3d=False,
+ with_mask_3d=True,
+ with_seg_3d=True),
+ dict(
+ type='PointSegClassMapping',
+ valid_cat_ids=(3, 4, 5, 6, 7, 8, 9, 10, 11, 12, 14, 16, 24, 28, 33,
+ 34, 36, 39),
+ max_cat_id=40),
+ dict(type='NormalizePointsColor', color_mean=None),
+ dict(type='DefaultFormatBundle3D', class_names=class_names),
+ dict(
+ type='Collect3D',
+ keys=['points', 'pts_semantic_mask', 'pts_instance_mask'])
+ ]
+ scannet_dataset = ScanNetInstanceSegDataset(
+ data_root=root_path,
+ ann_file=ann_file,
+ pipeline=train_pipeline,
+ classes=class_names,
+ test_mode=False)
+ expected_points = torch.tensor([[
+ -3.4742e+00, 7.8792e-01, 1.7397e+00, 3.3725e-01, 3.5294e-01, 3.0588e-01
+ ], [
+ 2.7216e+00, 3.4164e+00, 2.4572e+00, 6.6275e-01, 6.2745e-01, 5.1373e-01
+ ],
+ [
+ 1.3404e+00, -1.4675e+00, -4.4059e-02,
+ 3.8431e-01, 3.6078e-01, 3.5686e-01
+ ],
+ [
+ -3.0335e+00, 2.7273e+00, 1.5181e+00,
+ 2.3137e-01, 1.6078e-01, 8.2353e-02
+ ],
+ [
+ -4.3207e-01, 1.8154e+00, 1.7455e-01,
+ 4.0392e-01, 3.8039e-01, 4.1961e-01
+ ]])
+
+ data = scannet_dataset[0]
+
+ points = data['points']._data[:5]
+ pts_semantic_mask = data['pts_semantic_mask']._data[:5]
+ pts_instance_mask = data['pts_instance_mask']._data[:5]
+ expected_semantic_mask = np.array([11, 18, 18, 0, 4])
+ expected_instance_mask = np.array([6, 56, 10, 9, 35])
+
+ assert torch.allclose(points, expected_points, 1e-2)
+ assert np.all(pts_semantic_mask.numpy() == expected_semantic_mask)
+ assert np.all(pts_instance_mask.numpy() == expected_instance_mask)
+
+
+def test_instance_seg_evaluate():
+ root_path = './tests/data/scannet'
+ ann_file = './tests/data/scannet/scannet_infos.pkl'
+ class_names = ('cabinet', 'bed', 'chair', 'sofa', 'table', 'door',
+ 'window', 'bookshelf', 'picture', 'counter', 'desk',
+ 'curtain', 'refrigerator', 'showercurtrain', 'toilet',
+ 'sink', 'bathtub', 'garbagebin')
+ test_pipeline = [
+ dict(
+ type='LoadPointsFromFile',
+ coord_type='DEPTH',
+ shift_height=False,
+ use_color=True,
+ load_dim=6,
+ use_dim=[0, 1, 2, 3, 4, 5]),
+ dict(type='NormalizePointsColor', color_mean=None),
+ dict(type='DefaultFormatBundle3D', class_names=class_names),
+ dict(type='Collect3D', keys=['points'])
+ ]
+ scannet_dataset = ScanNetInstanceSegDataset(
+ data_root=root_path,
+ ann_file=ann_file,
+ pipeline=test_pipeline,
+ test_mode=True)
+
+ pred_mask = torch.tensor([
+ 1, -1, -1, -1, 7, 11, 2, -1, 1, 10, -1, -1, 5, -1, -1, -1, -1, 1, -1,
+ -1, -1, -1, 0, -1, 1, -1, 12, -1, -1, -1, 8, 5, 1, 5, 2, -1, -1, -1,
+ -1, -1, -1, -1, -1, -1, -1, -1, -1, -1, -1, -1, -1, 1, 8, -1, -1, -1,
+ 0, 4, 3, -1, 9, -1, -1, 6, -1, -1, -1, -1, 13, -1, -1, 5, -1, 5, -1,
+ -1, 9, 0, 5, -1, -1, 2, 3, 4, -1, -1, -1, 2, -1, -1, -1, 5, 9, -1, 1,
+ -1, 4, 10, 4, -1
+ ]).long()
+ pred_labels = torch.tensor(
+ [4, 11, 11, 10, 0, 3, 12, 4, 14, 1, 0, 0, 0, 5, 5]).long()
+ pred_scores = torch.tensor([.99 for _ in range(len(pred_labels))])
+ results = [
+ dict(
+ instance_mask=pred_mask,
+ instance_label=pred_labels,
+ instance_score=torch.tensor(pred_scores))
+ ]
+ eval_pipeline = [
+ dict(
+ type='LoadPointsFromFile',
+ coord_type='DEPTH',
+ shift_height=False,
+ use_color=True,
+ load_dim=6,
+ use_dim=[0, 1, 2, 3, 4, 5]),
+ dict(
+ type='LoadAnnotations3D',
+ with_bbox_3d=False,
+ with_label_3d=False,
+ with_mask_3d=True,
+ with_seg_3d=True),
+ dict(
+ type='PointSegClassMapping',
+ valid_cat_ids=(3, 4, 5, 6, 7, 8, 9, 10, 11, 12, 14, 16, 24, 28, 33,
+ 34, 36, 39),
+ max_cat_id=40),
+ dict(type='NormalizePointsColor', color_mean=None),
+ dict(type='DefaultFormatBundle3D', class_names=class_names),
+ dict(
+ type='Collect3D',
+ keys=['points', 'pts_semantic_mask', 'pts_instance_mask'])
+ ]
+ # We add options here as default min_region_size
+ # is much bigger than test instances.
+ ret_dict = scannet_dataset.evaluate(
+ results,
+ pipeline=eval_pipeline,
+ options=dict(min_region_sizes=np.array([1])))
+ assert abs(ret_dict['all_ap'] - 0.90625) < 0.001
+ assert abs(ret_dict['all_ap_50%'] - 0.90625) < 0.001
+ assert abs(ret_dict['all_ap_25%'] - 0.94444) < 0.001
+ assert abs(ret_dict['classes']['cabinet']['ap25%'] - 1.0) < 0.001
+ assert abs(ret_dict['classes']['cabinet']['ap50%'] - 0.65625) < 0.001
+ assert abs(ret_dict['classes']['door']['ap25%'] - 0.5) < 0.001
+ assert abs(ret_dict['classes']['door']['ap50%'] - 0.5) < 0.001
+
+
+def test_instance_seg_evaluate_v2():
+ root_path = './tests/data/scannet'
+ ann_file = './tests/data/scannet/scannet_infos.pkl'
+ class_names = ('cabinet', 'bed', 'chair', 'sofa', 'table', 'door',
+ 'window', 'bookshelf', 'picture', 'counter', 'desk',
+ 'curtain', 'refrigerator', 'showercurtrain', 'toilet',
+ 'sink', 'bathtub', 'garbagebin')
+ test_pipeline = [
+ dict(
+ type='LoadPointsFromFile',
+ coord_type='DEPTH',
+ shift_height=False,
+ use_color=True,
+ load_dim=6,
+ use_dim=[0, 1, 2, 3, 4, 5]),
+ dict(type='NormalizePointsColor', color_mean=None),
+ dict(type='DefaultFormatBundle3D', class_names=class_names),
+ dict(type='Collect3D', keys=['points'])
+ ]
+ scannet_dataset = ScanNetInstanceSegV2Dataset(
+ data_root=root_path,
+ ann_file=ann_file,
+ pipeline=test_pipeline,
+ test_mode=True)
+
+ pred_mask = torch.tensor([
+ 1, -1, -1, -1, 7, 11, 2, -1, 1, 10, -1, -1, 5, -1, -1, -1, -1, 1, -1,
+ -1, -1, -1, 0, -1, 1, -1, 12, -1, -1, -1, 8, 5, 1, 5, 2, -1, -1, -1,
+ -1, -1, -1, -1, -1, -1, -1, -1, -1, -1, -1, -1, -1, 1, 8, -1, -1, -1,
+ 0, 4, 3, -1, 9, -1, -1, 6, -1, -1, -1, -1, 13, -1, -1, 5, -1, 5, -1,
+ -1, 9, 0, 5, -1, -1, 2, 3, 4, -1, -1, -1, 2, -1, -1, -1, 5, 9, -1, 1,
+ -1, 4, 10, 4, -1
+ ]).long()
+ pred_mask = torch.nn.functional.one_hot(pred_mask + 1).T[1:].bool()
+ pred_labels = torch.tensor(
+ [4, 11, 11, 10, 0, 3, 12, 4, 14, 1, 0, 0, 0, 5, 5]).long()
+ pred_scores = torch.tensor([.99 for _ in range(len(pred_labels))])
+ results = [
+ dict(
+ instance_mask=pred_mask,
+ instance_label=pred_labels,
+ instance_score=torch.tensor(pred_scores))
+ ]
+ # We add options here as default min_region_size
+ # is much bigger than test instances.
+ ret_dict = scannet_dataset.evaluate(
+ results,
+ options=dict(min_region_sizes=np.array([1])))
+ assert abs(ret_dict['all_ap'] - 0.90625) < 0.001
+ assert abs(ret_dict['all_ap_50%'] - 0.90625) < 0.001
+ assert abs(ret_dict['all_ap_25%'] - 0.94444) < 0.001
+ assert abs(ret_dict['classes']['cabinet']['ap25%'] - 1.0) < 0.001
+ assert abs(ret_dict['classes']['cabinet']['ap50%'] - 0.65625) < 0.001
+ assert abs(ret_dict['classes']['door']['ap25%'] - 0.5) < 0.001
+ assert abs(ret_dict['classes']['door']['ap50%'] - 0.5) < 0.001
diff --git a/tests/test_data/test_datasets/test_semantickitti_dataset.py b/tests/test_data/test_datasets/test_semantickitti_dataset.py
new file mode 100644
index 0000000..908363a
--- /dev/null
+++ b/tests/test_data/test_datasets/test_semantickitti_dataset.py
@@ -0,0 +1,53 @@
+# Copyright (c) OpenMMLab. All rights reserved.
+import numpy as np
+
+from mmdet3d.datasets import SemanticKITTIDataset
+
+
+def test_getitem():
+ np.random.seed(0)
+ root_path = './tests/data/semantickitti/'
+ ann_file = './tests/data/semantickitti/semantickitti_infos.pkl'
+ class_names = ('unlabeled', 'car', 'bicycle', 'motorcycle', 'truck', 'bus',
+ 'person', 'bicyclist', 'motorcyclist', 'road', 'parking',
+ 'sidewalk', 'other-ground', 'building', 'fence',
+ 'vegetation', 'trunck', 'terrian', 'pole', 'traffic-sign')
+ pipelines = [
+ dict(
+ type='LoadPointsFromFile',
+ coord_type='LIDAR',
+ shift_height=True,
+ load_dim=4,
+ use_dim=[0, 1, 2]),
+ dict(
+ type='LoadAnnotations3D',
+ with_bbox_3d=False,
+ with_label_3d=False,
+ with_mask_3d=False,
+ with_seg_3d=True,
+ seg_3d_dtype=np.int32),
+ dict(
+ type='RandomFlip3D',
+ sync_2d=False,
+ flip_ratio_bev_horizontal=1.0,
+ flip_ratio_bev_vertical=1.0),
+ dict(
+ type='GlobalRotScaleTrans',
+ rot_range=[-0.087266, 0.087266],
+ scale_ratio_range=[1.0, 1.0],
+ shift_height=True),
+ dict(type='DefaultFormatBundle3D', class_names=class_names),
+ dict(
+ type='Collect3D',
+ keys=[
+ 'points',
+ 'pts_semantic_mask',
+ ],
+ meta_keys=['file_name', 'sample_idx', 'pcd_rotation']),
+ ]
+
+ semantickitti_dataset = SemanticKITTIDataset(root_path, ann_file,
+ pipelines)
+ data = semantickitti_dataset[0]
+ assert data['points']._data.shape[0] == data[
+ 'pts_semantic_mask']._data.shape[0]
diff --git a/tests/test_data/test_datasets/test_sunrgbd_dataset.py b/tests/test_data/test_datasets/test_sunrgbd_dataset.py
new file mode 100644
index 0000000..9d3333b
--- /dev/null
+++ b/tests/test_data/test_datasets/test_sunrgbd_dataset.py
@@ -0,0 +1,328 @@
+# Copyright (c) OpenMMLab. All rights reserved.
+import numpy as np
+import pytest
+import torch
+
+from mmdet3d.datasets import SUNRGBDDataset
+
+
+def _generate_sunrgbd_dataset_config():
+ root_path = './tests/data/sunrgbd'
+ # in coordinate system refactor, this test file is modified
+ ann_file = './tests/data/sunrgbd/sunrgbd_infos.pkl'
+ class_names = ('bed', 'table', 'sofa', 'chair', 'toilet', 'desk',
+ 'dresser', 'night_stand', 'bookshelf', 'bathtub')
+ pipelines = [
+ dict(
+ type='LoadPointsFromFile',
+ coord_type='DEPTH',
+ shift_height=True,
+ load_dim=6,
+ use_dim=[0, 1, 2]),
+ dict(type='LoadAnnotations3D'),
+ dict(
+ type='RandomFlip3D',
+ sync_2d=False,
+ flip_ratio_bev_horizontal=0.5,
+ ),
+ dict(
+ type='GlobalRotScaleTrans',
+ rot_range=[-0.523599, 0.523599],
+ scale_ratio_range=[0.85, 1.15],
+ shift_height=True),
+ dict(type='PointSample', num_points=5),
+ dict(type='DefaultFormatBundle3D', class_names=class_names),
+ dict(
+ type='Collect3D',
+ keys=['points', 'gt_bboxes_3d', 'gt_labels_3d'],
+ meta_keys=[
+ 'file_name', 'pcd_horizontal_flip', 'sample_idx',
+ 'pcd_scale_factor', 'pcd_rotation'
+ ]),
+ ]
+ modality = dict(use_lidar=True, use_camera=False)
+ return root_path, ann_file, class_names, pipelines, modality
+
+
+def _generate_sunrgbd_multi_modality_dataset_config():
+ root_path = './tests/data/sunrgbd'
+ ann_file = './tests/data/sunrgbd/sunrgbd_infos.pkl'
+ class_names = ('bed', 'table', 'sofa', 'chair', 'toilet', 'desk',
+ 'dresser', 'night_stand', 'bookshelf', 'bathtub')
+ img_norm_cfg = dict(
+ mean=[103.530, 116.280, 123.675], std=[1.0, 1.0, 1.0], to_rgb=False)
+ pipelines = [
+ dict(
+ type='LoadPointsFromFile',
+ coord_type='DEPTH',
+ shift_height=True,
+ load_dim=6,
+ use_dim=[0, 1, 2]),
+ dict(type='LoadImageFromFile'),
+ dict(type='LoadAnnotations3D'),
+ dict(type='LoadAnnotations', with_bbox=True),
+ dict(type='Resize', img_scale=(1333, 600), keep_ratio=True),
+ dict(type='RandomFlip', flip_ratio=0.0),
+ dict(type='Normalize', **img_norm_cfg),
+ dict(type='Pad', size_divisor=32),
+ dict(
+ type='RandomFlip3D',
+ sync_2d=False,
+ flip_ratio_bev_horizontal=0.5,
+ ),
+ dict(
+ type='GlobalRotScaleTrans',
+ rot_range=[-0.523599, 0.523599],
+ scale_ratio_range=[0.85, 1.15],
+ shift_height=True),
+ dict(type='PointSample', num_points=5),
+ dict(type='DefaultFormatBundle3D', class_names=class_names),
+ dict(
+ type='Collect3D',
+ keys=[
+ 'img', 'gt_bboxes', 'gt_labels', 'points', 'gt_bboxes_3d',
+ 'gt_labels_3d'
+ ])
+ ]
+ modality = dict(use_lidar=True, use_camera=True)
+ return root_path, ann_file, class_names, pipelines, modality
+
+
+def test_getitem():
+
+ from os import path as osp
+
+ np.random.seed(0)
+ root_path, ann_file, class_names, pipelines, modality = \
+ _generate_sunrgbd_dataset_config()
+
+ sunrgbd_dataset = SUNRGBDDataset(
+ root_path, ann_file, pipelines, modality=modality)
+ data = sunrgbd_dataset[0]
+ points = data['points']._data
+ gt_bboxes_3d = data['gt_bboxes_3d']._data
+ gt_labels_3d = data['gt_labels_3d']._data
+ file_name = data['img_metas']._data['file_name']
+ pcd_horizontal_flip = data['img_metas']._data['pcd_horizontal_flip']
+ pcd_scale_factor = data['img_metas']._data['pcd_scale_factor']
+ pcd_rotation = data['img_metas']._data['pcd_rotation']
+ sample_idx = data['img_metas']._data['sample_idx']
+ pcd_rotation_expected = np.array([[0.99889565, 0.04698427, 0.],
+ [-0.04698427, 0.99889565, 0.],
+ [0., 0., 1.]])
+ expected_file_name = osp.join('./tests/data/sunrgbd', 'points/000001.bin')
+ assert file_name == expected_file_name
+ assert pcd_horizontal_flip is False
+ assert abs(pcd_scale_factor - 0.9770964398016714) < 1e-5
+ assert np.allclose(pcd_rotation, pcd_rotation_expected, 1e-3)
+ assert sample_idx == 1
+ expected_points = torch.tensor([[-0.9904, 1.2596, 0.1105, 0.0905],
+ [-0.9948, 1.2758, 0.0437, 0.0238],
+ [-0.9866, 1.2641, 0.0504, 0.0304],
+ [-0.9915, 1.2586, 0.1265, 0.1065],
+ [-0.9890, 1.2561, 0.1216, 0.1017]])
+ expected_gt_bboxes_3d = torch.tensor(
+ [[0.8308, 4.1168, -1.2035, 2.2493, 1.8444, 1.9245, 1.6486],
+ [2.3002, 4.8149, -1.2442, 0.5718, 0.8629, 0.9510, 1.6030],
+ [-1.1477, 1.8090, -1.1725, 0.6965, 1.5273, 2.0563, 0.0552]])
+ # coord sys refactor (rotation is correct but yaw has to be reversed)
+ expected_gt_bboxes_3d[:, 6:] = -expected_gt_bboxes_3d[:, 6:]
+ expected_gt_labels = np.array([0, 7, 6])
+ original_classes = sunrgbd_dataset.CLASSES
+
+ assert torch.allclose(points, expected_points, 1e-2)
+ assert torch.allclose(gt_bboxes_3d.tensor, expected_gt_bboxes_3d, 1e-3)
+ assert np.all(gt_labels_3d.numpy() == expected_gt_labels)
+ assert original_classes == class_names
+
+ SUNRGBD_dataset = SUNRGBDDataset(
+ root_path, ann_file, pipeline=None, classes=['bed', 'table'])
+ assert SUNRGBD_dataset.CLASSES != original_classes
+ assert SUNRGBD_dataset.CLASSES == ['bed', 'table']
+
+ SUNRGBD_dataset = SUNRGBDDataset(
+ root_path, ann_file, pipeline=None, classes=('bed', 'table'))
+ assert SUNRGBD_dataset.CLASSES != original_classes
+ assert SUNRGBD_dataset.CLASSES == ('bed', 'table')
+
+ import tempfile
+ with tempfile.TemporaryDirectory() as tmpdir:
+ path = tmpdir + 'classes.txt'
+ with open(path, 'w') as f:
+ f.write('bed\ntable\n')
+
+ SUNRGBD_dataset = SUNRGBDDataset(
+ root_path, ann_file, pipeline=None, classes=path)
+ assert SUNRGBD_dataset.CLASSES != original_classes
+ assert SUNRGBD_dataset.CLASSES == ['bed', 'table']
+
+ # test multi-modality SUN RGB-D dataset
+ np.random.seed(0)
+ root_path, ann_file, class_names, multi_modality_pipelines, modality = \
+ _generate_sunrgbd_multi_modality_dataset_config()
+ sunrgbd_dataset = SUNRGBDDataset(
+ root_path, ann_file, multi_modality_pipelines, modality=modality)
+ data = sunrgbd_dataset[0]
+
+ points = data['points']._data
+ gt_bboxes_3d = data['gt_bboxes_3d']._data
+ gt_labels_3d = data['gt_labels_3d']._data
+ img = data['img']._data
+ depth2img = data['img_metas']._data['depth2img']
+
+ expected_rt_mat = np.array([[0.97959, 0.012593, -0.20061],
+ [0.012593, 0.99223, 0.12377],
+ [0.20061, -0.12377, 0.97182]])
+ expected_k_mat = np.array([[529.5, 0., 0.], [0., 529.5, 0.],
+ [365., 265., 1.]])
+ rt_mat = np.array([[1, 0, 0], [0, 0, -1], [0, 1, 0]
+ ]) @ expected_rt_mat.transpose(1, 0)
+ expected_depth2img = expected_k_mat @ rt_mat
+
+ assert torch.allclose(points, expected_points, 1e-2)
+ assert torch.allclose(gt_bboxes_3d.tensor, expected_gt_bboxes_3d, 1e-3)
+ assert np.all(gt_labels_3d.numpy() == expected_gt_labels)
+ assert img.shape[:] == (3, 608, 832)
+ assert np.allclose(depth2img, expected_depth2img)
+
+
+def test_evaluate():
+ if not torch.cuda.is_available():
+ pytest.skip()
+ from mmdet3d.core.bbox.structures import DepthInstance3DBoxes
+ root_path, ann_file, _, pipelines, modality = \
+ _generate_sunrgbd_dataset_config()
+ sunrgbd_dataset = SUNRGBDDataset(
+ root_path, ann_file, pipelines, modality=modality)
+ results = []
+ pred_boxes = dict()
+ pred_boxes['boxes_3d'] = DepthInstance3DBoxes(
+ torch.tensor(
+ [[1.0473, 4.1687, -1.2317, 2.3021, 1.8876, 1.9696, 1.6956],
+ [2.5831, 4.8117, -1.2733, 0.5852, 0.8832, 0.9733, 1.6500],
+ [-1.0864, 1.9045, -1.2000, 0.7128, 1.5631, 2.1045, 0.1022]]))
+ pred_boxes['labels_3d'] = torch.tensor([0, 7, 6])
+ pred_boxes['scores_3d'] = torch.tensor([0.5, 1.0, 1.0])
+ results.append(pred_boxes)
+ metric = [0.25, 0.5]
+ ap_dict = sunrgbd_dataset.evaluate(results, metric)
+ bed_precision_25 = ap_dict['bed_AP_0.25']
+ dresser_precision_25 = ap_dict['dresser_AP_0.25']
+ night_stand_precision_25 = ap_dict['night_stand_AP_0.25']
+ assert abs(bed_precision_25 - 1) < 0.01
+ assert abs(dresser_precision_25 - 1) < 0.01
+ assert abs(night_stand_precision_25 - 1) < 0.01
+
+
+def test_show():
+ import tempfile
+ from os import path as osp
+
+ import mmcv
+
+ from mmdet3d.core.bbox import DepthInstance3DBoxes
+ tmp_dir = tempfile.TemporaryDirectory()
+ temp_dir = tmp_dir.name
+ root_path, ann_file, class_names, pipelines, modality = \
+ _generate_sunrgbd_dataset_config()
+ sunrgbd_dataset = SUNRGBDDataset(
+ root_path, ann_file, pipelines, modality=modality)
+ boxes_3d = DepthInstance3DBoxes(
+ torch.tensor(
+ [[1.1500, 4.2614, -1.0669, 1.3219, 2.1593, 1.0267, 1.6473],
+ [-0.9583, 2.1916, -1.0881, 0.6213, 1.3022, 1.6275, -3.0720],
+ [2.5697, 4.8152, -1.1157, 0.5421, 0.7019, 0.7896, 1.6712],
+ [0.7283, 2.5448, -1.0356, 0.7691, 0.9056, 0.5771, 1.7121],
+ [-0.9860, 3.2413, -1.2349, 0.5110, 0.9940, 1.1245, 0.3295]]))
+ scores_3d = torch.tensor(
+ [1.5280e-01, 1.6682e-03, 6.2811e-04, 1.2860e-03, 9.4229e-06])
+ labels_3d = torch.tensor([0, 0, 0, 0, 0])
+ result = dict(boxes_3d=boxes_3d, scores_3d=scores_3d, labels_3d=labels_3d)
+ results = [result]
+ sunrgbd_dataset.show(results, temp_dir, show=False)
+ pts_file_path = osp.join(temp_dir, '000001', '000001_points.obj')
+ gt_file_path = osp.join(temp_dir, '000001', '000001_gt.obj')
+ pred_file_path = osp.join(temp_dir, '000001', '000001_pred.obj')
+ mmcv.check_file_exist(pts_file_path)
+ mmcv.check_file_exist(gt_file_path)
+ mmcv.check_file_exist(pred_file_path)
+ tmp_dir.cleanup()
+
+ # test show with pipeline
+ eval_pipeline = [
+ dict(
+ type='LoadPointsFromFile',
+ coord_type='DEPTH',
+ shift_height=True,
+ load_dim=6,
+ use_dim=[0, 1, 2]),
+ dict(
+ type='DefaultFormatBundle3D',
+ class_names=class_names,
+ with_label=False),
+ dict(type='Collect3D', keys=['points'])
+ ]
+ tmp_dir = tempfile.TemporaryDirectory()
+ temp_dir = tmp_dir.name
+ sunrgbd_dataset.show(results, temp_dir, show=False, pipeline=eval_pipeline)
+ pts_file_path = osp.join(temp_dir, '000001', '000001_points.obj')
+ gt_file_path = osp.join(temp_dir, '000001', '000001_gt.obj')
+ pred_file_path = osp.join(temp_dir, '000001', '000001_pred.obj')
+ mmcv.check_file_exist(pts_file_path)
+ mmcv.check_file_exist(gt_file_path)
+ mmcv.check_file_exist(pred_file_path)
+ tmp_dir.cleanup()
+
+ # test multi-modality show
+ tmp_dir = tempfile.TemporaryDirectory()
+ temp_dir = tmp_dir.name
+ root_path, ann_file, class_names, multi_modality_pipelines, modality = \
+ _generate_sunrgbd_multi_modality_dataset_config()
+ sunrgbd_dataset = SUNRGBDDataset(
+ root_path, ann_file, multi_modality_pipelines, modality=modality)
+ sunrgbd_dataset.show(results, temp_dir, False, multi_modality_pipelines)
+ pts_file_path = osp.join(temp_dir, '000001', '000001_points.obj')
+ gt_file_path = osp.join(temp_dir, '000001', '000001_gt.obj')
+ pred_file_path = osp.join(temp_dir, '000001', '000001_pred.obj')
+ img_file_path = osp.join(temp_dir, '000001', '000001_img.png')
+ img_pred_path = osp.join(temp_dir, '000001', '000001_pred.png')
+ img_gt_file = osp.join(temp_dir, '000001', '000001_gt.png')
+ mmcv.check_file_exist(pts_file_path)
+ mmcv.check_file_exist(gt_file_path)
+ mmcv.check_file_exist(pred_file_path)
+ mmcv.check_file_exist(img_file_path)
+ mmcv.check_file_exist(img_pred_path)
+ mmcv.check_file_exist(img_gt_file)
+ tmp_dir.cleanup()
+
+ # test multi-modality show with pipeline
+ eval_pipeline = [
+ dict(type='LoadImageFromFile'),
+ dict(
+ type='LoadPointsFromFile',
+ coord_type='DEPTH',
+ shift_height=True,
+ load_dim=6,
+ use_dim=[0, 1, 2]),
+ dict(
+ type='DefaultFormatBundle3D',
+ class_names=class_names,
+ with_label=False),
+ dict(type='Collect3D', keys=['points', 'img'])
+ ]
+ tmp_dir = tempfile.TemporaryDirectory()
+ temp_dir = tmp_dir.name
+ sunrgbd_dataset.show(results, temp_dir, show=False, pipeline=eval_pipeline)
+ pts_file_path = osp.join(temp_dir, '000001', '000001_points.obj')
+ gt_file_path = osp.join(temp_dir, '000001', '000001_gt.obj')
+ pred_file_path = osp.join(temp_dir, '000001', '000001_pred.obj')
+ img_file_path = osp.join(temp_dir, '000001', '000001_img.png')
+ img_pred_path = osp.join(temp_dir, '000001', '000001_pred.png')
+ img_gt_file = osp.join(temp_dir, '000001', '000001_gt.png')
+ mmcv.check_file_exist(pts_file_path)
+ mmcv.check_file_exist(gt_file_path)
+ mmcv.check_file_exist(pred_file_path)
+ mmcv.check_file_exist(img_file_path)
+ mmcv.check_file_exist(img_pred_path)
+ mmcv.check_file_exist(img_gt_file)
+ tmp_dir.cleanup()
diff --git a/tests/test_data/test_datasets/test_waymo_dataset.py b/tests/test_data/test_datasets/test_waymo_dataset.py
new file mode 100644
index 0000000..6199a65
--- /dev/null
+++ b/tests/test_data/test_datasets/test_waymo_dataset.py
@@ -0,0 +1,268 @@
+# Copyright (c) OpenMMLab. All rights reserved.
+import tempfile
+
+import numpy as np
+import pytest
+import torch
+
+from mmdet3d.datasets import WaymoDataset
+
+
+def _generate_waymo_train_dataset_config():
+ data_root = 'tests/data/waymo/kitti_format/'
+ ann_file = 'tests/data/waymo/kitti_format/waymo_infos_train.pkl'
+ classes = ['Car', 'Pedestrian', 'Cyclist']
+ pts_prefix = 'velodyne'
+ point_cloud_range = [-74.88, -74.88, -2, 74.88, 74.88, 4]
+ file_client_args = dict(backend='disk')
+ db_sampler = dict(
+ data_root=data_root,
+ # in coordinate system refactor, this test file is modified
+ info_path=data_root + 'waymo_dbinfos_train.pkl',
+ rate=1.0,
+ prepare=dict(
+ filter_by_difficulty=[-1], filter_by_min_points=dict(Car=5)),
+ classes=classes,
+ sample_groups=dict(Car=15),
+ points_loader=dict(
+ type='LoadPointsFromFile',
+ coord_type='LIDAR',
+ load_dim=6,
+ use_dim=[0, 1, 2, 3, 4],
+ file_client_args=file_client_args))
+ pipeline = [
+ dict(
+ type='LoadPointsFromFile',
+ coord_type='LIDAR',
+ load_dim=6,
+ use_dim=5,
+ file_client_args=file_client_args),
+ dict(
+ type='LoadAnnotations3D',
+ with_bbox_3d=True,
+ with_label_3d=True,
+ file_client_args=file_client_args),
+ dict(type='ObjectSample', db_sampler=db_sampler),
+ dict(
+ type='RandomFlip3D',
+ sync_2d=False,
+ flip_ratio_bev_horizontal=0.5,
+ flip_ratio_bev_vertical=0.5),
+ dict(
+ type='GlobalRotScaleTrans',
+ rot_range=[-0.78539816, 0.78539816],
+ scale_ratio_range=[0.95, 1.05]),
+ dict(type='PointsRangeFilter', point_cloud_range=point_cloud_range),
+ dict(type='ObjectRangeFilter', point_cloud_range=point_cloud_range),
+ dict(type='PointShuffle'),
+ dict(type='DefaultFormatBundle3D', class_names=classes),
+ dict(
+ type='Collect3D', keys=['points', 'gt_bboxes_3d', 'gt_labels_3d'])
+ ]
+ modality = dict(use_lidar=True, use_camera=False)
+ split = 'training'
+ return data_root, ann_file, classes, pts_prefix, pipeline, modality, split
+
+
+def _generate_waymo_val_dataset_config():
+ data_root = 'tests/data/waymo/kitti_format/'
+ ann_file = 'tests/data/waymo/kitti_format/waymo_infos_val.pkl'
+ classes = ['Car', 'Pedestrian', 'Cyclist']
+ pts_prefix = 'velodyne'
+ point_cloud_range = [-74.88, -74.88, -2, 74.88, 74.88, 4]
+ file_client_args = dict(backend='disk')
+ pipeline = [
+ dict(
+ type='LoadPointsFromFile',
+ coord_type='LIDAR',
+ load_dim=6,
+ use_dim=5,
+ file_client_args=file_client_args),
+ dict(
+ type='MultiScaleFlipAug3D',
+ img_scale=(1333, 800),
+ pts_scale_ratio=1,
+ flip=False,
+ transforms=[
+ dict(
+ type='GlobalRotScaleTrans',
+ rot_range=[0, 0],
+ scale_ratio_range=[1., 1.],
+ translation_std=[0, 0, 0]),
+ dict(type='RandomFlip3D'),
+ dict(
+ type='PointsRangeFilter',
+ point_cloud_range=point_cloud_range),
+ dict(
+ type='DefaultFormatBundle3D',
+ class_names=classes,
+ with_label=False),
+ dict(type='Collect3D', keys=['points'])
+ ])
+ ]
+ modality = dict(use_lidar=True, use_camera=False)
+ split = 'training'
+ return data_root, ann_file, classes, pts_prefix, pipeline, modality, split
+
+
+def test_getitem():
+ np.random.seed(0)
+ data_root, ann_file, classes, pts_prefix, pipeline, \
+ modality, split = _generate_waymo_train_dataset_config()
+ waymo_dataset = WaymoDataset(data_root, ann_file, split, pts_prefix,
+ pipeline, classes, modality)
+ data = waymo_dataset[0]
+ points = data['points']._data
+ gt_bboxes_3d = data['gt_bboxes_3d']._data
+ gt_labels_3d = data['gt_labels_3d']._data
+ expected_gt_bboxes_3d = torch.tensor(
+ [[31.8048, -0.1002, 2.1857, 6.0931, 2.3519, 3.1756, -0.1403]])
+ expected_gt_labels_3d = torch.tensor([0])
+ assert points.shape == (765, 5)
+ assert torch.allclose(
+ gt_bboxes_3d.tensor, expected_gt_bboxes_3d, atol=1e-4)
+ assert torch.all(gt_labels_3d == expected_gt_labels_3d)
+
+
+def test_evaluate():
+ if not torch.cuda.is_available():
+ pytest.skip('test requires GPU and torch+cuda')
+ from mmdet3d.core.bbox import LiDARInstance3DBoxes
+ data_root, ann_file, classes, pts_prefix, pipeline, \
+ modality, split = _generate_waymo_val_dataset_config()
+ waymo_dataset = WaymoDataset(data_root, ann_file, split, pts_prefix,
+ pipeline, classes, modality)
+ boxes_3d = LiDARInstance3DBoxes(
+ torch.tensor([[
+ 6.9684e+01, 3.3335e+01, 4.1465e-02, 4.3600e+00, 2.0100e+00,
+ 1.4600e+00, 9.0000e-02 - np.pi / 2
+ ]]))
+ labels_3d = torch.tensor([0])
+ scores_3d = torch.tensor([0.5])
+ result = dict(boxes_3d=boxes_3d, labels_3d=labels_3d, scores_3d=scores_3d)
+
+ # kitti protocol
+ metric = ['kitti']
+ ap_dict = waymo_dataset.evaluate([result], metric=metric)
+ assert np.isclose(ap_dict['KITTI/Overall_3D_AP11_easy'],
+ 3.0303030303030307)
+ assert np.isclose(ap_dict['KITTI/Overall_3D_AP11_moderate'],
+ 3.0303030303030307)
+ assert np.isclose(ap_dict['KITTI/Overall_3D_AP11_hard'],
+ 3.0303030303030307)
+
+ # waymo protocol
+ metric = ['waymo']
+ boxes_3d = LiDARInstance3DBoxes(
+ torch.tensor([[
+ 6.9684e+01, 3.3335e+01, 4.1465e-02, 4.3600e+00, 2.0100e+00,
+ 1.4600e+00, 9.0000e-02 - np.pi / 2
+ ]]))
+ labels_3d = torch.tensor([0])
+ scores_3d = torch.tensor([0.8])
+ result = dict(boxes_3d=boxes_3d, labels_3d=labels_3d, scores_3d=scores_3d)
+ ap_dict = waymo_dataset.evaluate([result], metric=metric)
+ assert np.isclose(ap_dict['Overall/L1 mAP'], 0.3333333333333333)
+ assert np.isclose(ap_dict['Overall/L2 mAP'], 0.3333333333333333)
+ assert np.isclose(ap_dict['Overall/L1 mAPH'], 0.3333333333333333)
+ assert np.isclose(ap_dict['Overall/L2 mAPH'], 0.3333333333333333)
+
+
+def test_show():
+ from os import path as osp
+
+ import mmcv
+
+ from mmdet3d.core.bbox import LiDARInstance3DBoxes
+
+ # Waymo shares show function with KITTI so I just copy it here
+ tmp_dir = tempfile.TemporaryDirectory()
+ temp_dir = tmp_dir.name
+ data_root, ann_file, classes, pts_prefix, pipeline, \
+ modality, split = _generate_waymo_val_dataset_config()
+ waymo_dataset = WaymoDataset(
+ data_root, ann_file, split=split, modality=modality, pipeline=pipeline)
+ boxes_3d = LiDARInstance3DBoxes(
+ torch.tensor(
+ [[46.1218, -4.6496, -0.9275, 1.4442, 0.5316, 1.7450, 1.1749],
+ [33.3189, 0.1981, 0.3136, 1.2301, 0.5656, 1.7985, 1.5723],
+ [46.1366, -4.6404, -0.9510, 1.6501, 0.5162, 1.7540, 1.3778],
+ [33.2646, 0.2297, 0.3446, 1.3365, 0.5746, 1.7947, 1.5430],
+ [58.9079, 16.6272, -1.5829, 3.9313, 1.5656, 1.4899, 1.5505]]))
+ scores_3d = torch.tensor([0.1815, 0.1663, 0.5792, 0.2194, 0.2780])
+ labels_3d = torch.tensor([0, 0, 1, 1, 2])
+ result = dict(boxes_3d=boxes_3d, scores_3d=scores_3d, labels_3d=labels_3d)
+ results = [result]
+ waymo_dataset.show(results, temp_dir, show=False)
+ pts_file_path = osp.join(temp_dir, '1000000', '1000000_points.obj')
+ gt_file_path = osp.join(temp_dir, '1000000', '1000000_gt.obj')
+ pred_file_path = osp.join(temp_dir, '1000000', '1000000_pred.obj')
+ mmcv.check_file_exist(pts_file_path)
+ mmcv.check_file_exist(gt_file_path)
+ mmcv.check_file_exist(pred_file_path)
+ tmp_dir.cleanup()
+
+ # test show with pipeline
+ tmp_dir = tempfile.TemporaryDirectory()
+ temp_dir = tmp_dir.name
+ eval_pipeline = [
+ dict(
+ type='LoadPointsFromFile',
+ coord_type='LIDAR',
+ load_dim=6,
+ use_dim=5),
+ dict(
+ type='DefaultFormatBundle3D',
+ class_names=classes,
+ with_label=False),
+ dict(type='Collect3D', keys=['points'])
+ ]
+ waymo_dataset.show(results, temp_dir, show=False, pipeline=eval_pipeline)
+ pts_file_path = osp.join(temp_dir, '1000000', '1000000_points.obj')
+ gt_file_path = osp.join(temp_dir, '1000000', '1000000_gt.obj')
+ pred_file_path = osp.join(temp_dir, '1000000', '1000000_pred.obj')
+ mmcv.check_file_exist(pts_file_path)
+ mmcv.check_file_exist(gt_file_path)
+ mmcv.check_file_exist(pred_file_path)
+ tmp_dir.cleanup()
+
+
+def test_format_results():
+ if not torch.cuda.is_available():
+ pytest.skip('test requires GPU and torch+cuda')
+ from mmdet3d.core.bbox import LiDARInstance3DBoxes
+ data_root, ann_file, classes, pts_prefix, pipeline, \
+ modality, split = _generate_waymo_val_dataset_config()
+ waymo_dataset = WaymoDataset(data_root, ann_file, split, pts_prefix,
+ pipeline, classes, modality)
+ boxes_3d = LiDARInstance3DBoxes(
+ torch.tensor([[
+ 6.9684e+01, 3.3335e+01, 4.1465e-02, 4.3600e+00, 2.0100e+00,
+ 1.4600e+00, 9.0000e-02 - np.pi / 2
+ ]]))
+ labels_3d = torch.tensor([0])
+ scores_3d = torch.tensor([0.5])
+ result = dict(boxes_3d=boxes_3d, labels_3d=labels_3d, scores_3d=scores_3d)
+ result_files, tmp_dir = waymo_dataset.format_results([result],
+ data_format='waymo')
+ expected_name = np.array(['Car'])
+ expected_truncated = np.array([0.])
+ expected_occluded = np.array([0])
+ expected_alpha = np.array([0.35619745])
+ expected_bbox = np.array([[0., 673.59814, 37.07779, 719.7537]])
+ expected_dimensions = np.array([[4.36, 1.46, 2.01]])
+ expected_location = np.array([[-33.000042, 2.4999967, 68.29972]])
+ expected_rotation_y = np.array([-0.09])
+ expected_score = np.array([0.5])
+ expected_sample_idx = np.array([1000000])
+ assert np.all(result_files[0]['name'] == expected_name)
+ assert np.allclose(result_files[0]['truncated'], expected_truncated)
+ assert np.all(result_files[0]['occluded'] == expected_occluded)
+ assert np.allclose(result_files[0]['bbox'], expected_bbox, 1e-3)
+ assert np.allclose(result_files[0]['dimensions'], expected_dimensions)
+ assert np.allclose(result_files[0]['location'], expected_location)
+ assert np.allclose(result_files[0]['rotation_y'], expected_rotation_y)
+ assert np.allclose(result_files[0]['score'], expected_score)
+ assert np.allclose(result_files[0]['sample_idx'], expected_sample_idx)
+ assert np.allclose(result_files[0]['alpha'], expected_alpha)
+ tmp_dir.cleanup()
diff --git a/tests/test_data/test_pipelines/test_augmentations/test_data_augment_utils.py b/tests/test_data/test_pipelines/test_augmentations/test_data_augment_utils.py
new file mode 100644
index 0000000..60dca9e
--- /dev/null
+++ b/tests/test_data/test_pipelines/test_augmentations/test_data_augment_utils.py
@@ -0,0 +1,72 @@
+# Copyright (c) OpenMMLab. All rights reserved.
+import mmcv
+import numpy as np
+
+from mmdet3d.datasets.pipelines.data_augment_utils import (
+ noise_per_object_v3_, points_transform_)
+
+
+def test_noise_per_object_v3_():
+ np.random.seed(0)
+ points = np.fromfile(
+ './tests/data/kitti/training/velodyne_reduced/000000.bin',
+ np.float32).reshape(-1, 4)
+ annos = mmcv.load('./tests/data/kitti/kitti_infos_train.pkl')
+ info = annos[0]
+ annos = info['annos']
+ loc = annos['location']
+ dims = annos['dimensions']
+ rots = annos['rotation_y']
+ gt_bboxes_3d = np.concatenate([loc, dims, rots[..., np.newaxis]],
+ axis=1).astype(np.float32)
+
+ noise_per_object_v3_(gt_boxes=gt_bboxes_3d, points=points)
+ expected_gt_bboxes_3d = np.array(
+ [[3.3430212, 2.1475432, 9.388738, 1.2, 1.89, 0.48, 0.05056486]])
+
+ assert points.shape == (800, 4)
+ assert np.allclose(gt_bboxes_3d, expected_gt_bboxes_3d)
+
+
+def test_points_transform():
+ points = np.array([[46.5090, 6.1140, -0.7790, 0.0000],
+ [42.9490, 6.4050, -0.7050, 0.0000],
+ [42.9010, 6.5360, -0.7050, 0.0000],
+ [46.1960, 6.0960, -1.0100, 0.0000],
+ [43.3080, 6.2680, -0.9360, 0.0000]])
+ gt_boxes = np.array([[
+ 1.5340e+01, 8.4691e+00, -1.6855e+00, 1.6400e+00, 3.7000e+00,
+ 1.4900e+00, 3.1300e+00
+ ],
+ [
+ 1.7999e+01, 8.2386e+00, -1.5802e+00, 1.5500e+00,
+ 4.0200e+00, 1.5200e+00, 3.1300e+00
+ ],
+ [
+ 2.9620e+01, 8.2617e+00, -1.6185e+00, 1.7800e+00,
+ 4.2500e+00, 1.9000e+00, -3.1200e+00
+ ],
+ [
+ 4.8218e+01, 7.8035e+00, -1.3790e+00, 1.6400e+00,
+ 3.7000e+00, 1.5200e+00, -1.0000e-02
+ ],
+ [
+ 3.3079e+01, -8.4817e+00, -1.3092e+00, 4.3000e-01,
+ 1.7000e+00, 1.6200e+00, -1.5700e+00
+ ]])
+ point_masks = np.array([[False, False, False, False, False],
+ [False, False, False, False, False],
+ [False, False, False, False, False],
+ [False, False, False, False, False],
+ [False, False, False, False, False]])
+ loc_transforms = np.array([[-1.8635, -0.2774, -0.1774],
+ [-1.0297, -1.0302, -0.3062],
+ [1.6680, 0.2597, 0.0551],
+ [0.2230, 0.7257, -0.0097],
+ [-0.1403, 0.8300, 0.3431]])
+ rot_transforms = np.array([0.6888, -0.3858, 0.1910, -0.0044, -0.0036])
+ valid_mask = np.array([True, True, True, True, True])
+ points_transform_(points, gt_boxes[:, :3], point_masks, loc_transforms,
+ rot_transforms, valid_mask)
+ assert points.shape == (5, 4)
+ assert gt_boxes.shape == (5, 7)
diff --git a/tests/test_data/test_pipelines/test_augmentations/test_test_augment_utils.py b/tests/test_data/test_pipelines/test_augmentations/test_test_augment_utils.py
new file mode 100644
index 0000000..4e621d3
--- /dev/null
+++ b/tests/test_data/test_pipelines/test_augmentations/test_test_augment_utils.py
@@ -0,0 +1,62 @@
+# Copyright (c) OpenMMLab. All rights reserved.
+import numpy as np
+import torch
+
+from mmdet3d.core.points import DepthPoints
+from mmdet3d.datasets.pipelines import MultiScaleFlipAug3D
+
+
+def test_multi_scale_flip_aug_3D():
+ np.random.seed(0)
+ transforms = [{
+ 'type': 'GlobalRotScaleTrans',
+ 'rot_range': [-0.1, 0.1],
+ 'scale_ratio_range': [0.9, 1.1],
+ 'translation_std': [0, 0, 0]
+ }, {
+ 'type': 'RandomFlip3D',
+ 'sync_2d': False,
+ 'flip_ratio_bev_horizontal': 0.5
+ }, {
+ 'type': 'PointSample',
+ 'num_points': 5
+ }, {
+ 'type':
+ 'DefaultFormatBundle3D',
+ 'class_names': ('bed', 'table', 'sofa', 'chair', 'toilet', 'desk',
+ 'dresser', 'night_stand', 'bookshelf', 'bathtub'),
+ 'with_label':
+ False
+ }, {
+ 'type': 'Collect3D',
+ 'keys': ['points']
+ }]
+ img_scale = (1333, 800)
+ pts_scale_ratio = 1
+ multi_scale_flip_aug_3D = MultiScaleFlipAug3D(transforms, img_scale,
+ pts_scale_ratio)
+ pts_file_name = 'tests/data/sunrgbd/points/000001.bin'
+ sample_idx = 4
+ file_name = 'tests/data/sunrgbd/points/000001.bin'
+ bbox3d_fields = []
+ points = np.array([[0.20397437, 1.4267826, -1.0503972, 0.16195858],
+ [-2.2095256, 3.3159535, -0.7706928, 0.4416629],
+ [1.5090443, 3.2764456, -1.1913797, 0.02097607],
+ [-1.373904, 3.8711405, 0.8524302, 2.064786],
+ [-1.8139812, 3.538856, -1.0056694, 0.20668638]])
+ points = DepthPoints(points, points_dim=4, attribute_dims=dict(height=3))
+ results = dict(
+ points=points,
+ pts_file_name=pts_file_name,
+ sample_idx=sample_idx,
+ file_name=file_name,
+ bbox3d_fields=bbox3d_fields)
+ results = multi_scale_flip_aug_3D(results)
+ expected_points = torch.tensor(
+ [[-2.2418, 3.2942, -0.7707, 0.4417], [-1.4116, 3.8575, 0.8524, 2.0648],
+ [-1.8484, 3.5210, -1.0057, 0.2067], [0.1900, 1.4287, -1.0504, 0.1620],
+ [1.4770, 3.2910, -1.1914, 0.0210]],
+ dtype=torch.float32)
+
+ assert torch.allclose(
+ results['points'][0]._data, expected_points, atol=1e-4)
diff --git a/tests/test_data/test_pipelines/test_augmentations/test_transforms_3d.py b/tests/test_data/test_pipelines/test_augmentations/test_transforms_3d.py
new file mode 100644
index 0000000..759210e
--- /dev/null
+++ b/tests/test_data/test_pipelines/test_augmentations/test_transforms_3d.py
@@ -0,0 +1,852 @@
+# Copyright (c) OpenMMLab. All rights reserved.
+import mmcv
+import numpy as np
+import pytest
+import torch
+
+from mmdet3d.core import (Box3DMode, CameraInstance3DBoxes,
+ DepthInstance3DBoxes, LiDARInstance3DBoxes)
+from mmdet3d.core.bbox import Coord3DMode
+from mmdet3d.core.points import DepthPoints, LiDARPoints
+# yapf: disable
+from mmdet3d.datasets import (AffineResize, BackgroundPointsFilter,
+ GlobalAlignment, GlobalRotScaleTrans,
+ ObjectNameFilter, ObjectNoise, ObjectRangeFilter,
+ ObjectSample, PointSample, PointShuffle,
+ PointsRangeFilter, RandomDropPointsColor,
+ RandomFlip3D, RandomJitterPoints,
+ RandomShiftScale, VoxelBasedPointSampler)
+
+
+def test_remove_points_in_boxes():
+ points = np.array([[68.1370, 3.3580, 2.5160, 0.0000],
+ [67.6970, 3.5500, 2.5010, 0.0000],
+ [67.6490, 3.7600, 2.5000, 0.0000],
+ [66.4140, 3.9010, 2.4590, 0.0000],
+ [66.0120, 4.0850, 2.4460, 0.0000],
+ [65.8340, 4.1780, 2.4400, 0.0000],
+ [65.8410, 4.3860, 2.4400, 0.0000],
+ [65.7450, 4.5870, 2.4380, 0.0000],
+ [65.5510, 4.7800, 2.4320, 0.0000],
+ [65.4860, 4.9820, 2.4300, 0.0000]])
+
+ boxes = np.array(
+ [[30.0285, 10.5110, -1.5304, 0.5100, 0.8700, 1.6000, 1.6400],
+ [7.8369, 1.6053, -1.5605, 0.5800, 1.2300, 1.8200, -3.1000],
+ [10.8740, -1.0827, -1.3310, 0.6000, 0.5200, 1.7100, 1.3500],
+ [14.9783, 2.2466, -1.4950, 0.6100, 0.7300, 1.5300, -1.9200],
+ [11.0656, 0.6195, -1.5202, 0.6600, 1.0100, 1.7600, -1.4600],
+ [10.5994, -7.9049, -1.4980, 0.5300, 1.9600, 1.6800, 1.5600],
+ [28.7068, -8.8244, -1.1485, 0.6500, 1.7900, 1.7500, 3.1200],
+ [20.2630, 5.1947, -1.4799, 0.7300, 1.7600, 1.7300, 1.5100],
+ [18.2496, 3.1887, -1.6109, 0.5600, 1.6800, 1.7100, 1.5600],
+ [7.7396, -4.3245, -1.5801, 0.5600, 1.7900, 1.8000, -0.8300]])
+ points = LiDARPoints(points, points_dim=4)
+ points = ObjectSample.remove_points_in_boxes(points, boxes)
+ assert points.tensor.numpy().shape == (10, 4)
+
+
+def test_object_sample():
+ db_sampler = mmcv.ConfigDict({
+ 'data_root': './tests/data/kitti/',
+ 'info_path': './tests/data/kitti/kitti_dbinfos_train.pkl',
+ 'rate': 1.0,
+ 'prepare': {
+ 'filter_by_difficulty': [-1],
+ 'filter_by_min_points': {
+ 'Pedestrian': 10
+ }
+ },
+ 'classes': ['Pedestrian', 'Cyclist', 'Car'],
+ 'sample_groups': {
+ 'Pedestrian': 6
+ }
+ })
+ np.random.seed(0)
+ object_sample = ObjectSample(db_sampler)
+ points = np.fromfile(
+ './tests/data/kitti/training/velodyne_reduced/000000.bin',
+ np.float32).reshape(-1, 4)
+ annos = mmcv.load('./tests/data/kitti/kitti_infos_train.pkl')
+ info = annos[0]
+ rect = info['calib']['R0_rect'].astype(np.float32)
+ Trv2c = info['calib']['Tr_velo_to_cam'].astype(np.float32)
+ annos = info['annos']
+ loc = annos['location']
+ dims = annos['dimensions']
+ rots = annos['rotation_y']
+ gt_names = annos['name']
+
+ gt_bboxes_3d = np.concatenate([loc, dims, rots[..., np.newaxis]],
+ axis=1).astype(np.float32)
+ gt_bboxes_3d = CameraInstance3DBoxes(gt_bboxes_3d).convert_to(
+ Box3DMode.LIDAR, np.linalg.inv(rect @ Trv2c))
+ CLASSES = ('Pedestrian', 'Cyclist', 'Car')
+ gt_labels = []
+ for cat in gt_names:
+ if cat in CLASSES:
+ gt_labels.append(CLASSES.index(cat))
+ else:
+ gt_labels.append(-1)
+ gt_labels = np.array(gt_labels, dtype=np.int64)
+ points = LiDARPoints(points, points_dim=4)
+ input_dict = dict(
+ points=points, gt_bboxes_3d=gt_bboxes_3d, gt_labels_3d=gt_labels)
+ input_dict = object_sample(input_dict)
+ points = input_dict['points']
+ gt_bboxes_3d = input_dict['gt_bboxes_3d']
+ gt_labels_3d = input_dict['gt_labels_3d']
+ repr_str = repr(object_sample)
+ expected_repr_str = 'ObjectSample sample_2d=False, ' \
+ 'data_root=./tests/data/kitti/, ' \
+ 'info_path=./tests/data/kitti/kitti' \
+ '_dbinfos_train.pkl, rate=1.0, ' \
+ 'prepare={\'filter_by_difficulty\': [-1], ' \
+ '\'filter_by_min_points\': {\'Pedestrian\': 10}}, ' \
+ 'classes=[\'Pedestrian\', \'Cyclist\', \'Car\'], ' \
+ 'sample_groups={\'Pedestrian\': 6}'
+ assert repr_str == expected_repr_str
+ assert points.tensor.numpy().shape == (800, 4)
+ assert gt_bboxes_3d.tensor.shape == (1, 7)
+ assert np.all(gt_labels_3d == [0])
+
+
+def test_object_noise():
+ np.random.seed(0)
+ object_noise = ObjectNoise()
+ points = np.fromfile(
+ './tests/data/kitti/training/velodyne_reduced/000000.bin',
+ np.float32).reshape(-1, 4)
+ annos = mmcv.load('./tests/data/kitti/kitti_infos_train.pkl')
+ info = annos[0]
+ rect = info['calib']['R0_rect'].astype(np.float32)
+ Trv2c = info['calib']['Tr_velo_to_cam'].astype(np.float32)
+ annos = info['annos']
+ loc = annos['location']
+ dims = annos['dimensions']
+ rots = annos['rotation_y']
+ gt_bboxes_3d = np.concatenate([loc, dims, rots[..., np.newaxis]],
+ axis=1).astype(np.float32)
+ gt_bboxes_3d = CameraInstance3DBoxes(gt_bboxes_3d).convert_to(
+ Box3DMode.LIDAR, np.linalg.inv(rect @ Trv2c))
+ points = LiDARPoints(points, points_dim=4)
+ input_dict = dict(points=points, gt_bboxes_3d=gt_bboxes_3d)
+ input_dict = object_noise(input_dict)
+ points = input_dict['points']
+ gt_bboxes_3d = input_dict['gt_bboxes_3d'].tensor
+
+ # coord sys refactor (lidar2cam)
+ expected_gt_bboxes_3d = torch.tensor([[
+ 9.1724, -1.7559, -1.3550, 1.2000, 0.4800, 1.8900,
+ 0.0505 - float(rots) * 2 - np.pi / 2
+ ]])
+ repr_str = repr(object_noise)
+ expected_repr_str = 'ObjectNoise(num_try=100, ' \
+ 'translation_std=[0.25, 0.25, 0.25], ' \
+ 'global_rot_range=[0.0, 0.0], ' \
+ 'rot_range=[-0.15707963267, 0.15707963267])'
+
+ assert repr_str == expected_repr_str
+ assert points.tensor.numpy().shape == (800, 4)
+ assert torch.allclose(gt_bboxes_3d, expected_gt_bboxes_3d, 1e-3)
+
+
+def test_object_name_filter():
+ class_names = ['Pedestrian']
+ object_name_filter = ObjectNameFilter(class_names)
+
+ annos = mmcv.load('./tests/data/kitti/kitti_infos_train.pkl')
+ info = annos[0]
+ rect = info['calib']['R0_rect'].astype(np.float32)
+ Trv2c = info['calib']['Tr_velo_to_cam'].astype(np.float32)
+ annos = info['annos']
+ loc = annos['location']
+ dims = annos['dimensions']
+ rots = annos['rotation_y']
+ gt_names = annos['name']
+
+ gt_bboxes_3d = np.concatenate([loc, dims, rots[..., np.newaxis]],
+ axis=1).astype(np.float32)
+ gt_bboxes_3d = CameraInstance3DBoxes(gt_bboxes_3d).convert_to(
+ Box3DMode.LIDAR, np.linalg.inv(rect @ Trv2c))
+ CLASSES = ('Pedestrian', 'Cyclist', 'Car')
+ gt_labels = []
+ for cat in gt_names:
+ if cat in CLASSES:
+ gt_labels.append(CLASSES.index(cat))
+ else:
+ gt_labels.append(-1)
+ gt_labels = np.array(gt_labels, dtype=np.int64)
+ input_dict = dict(
+ gt_bboxes_3d=gt_bboxes_3d.clone(), gt_labels_3d=gt_labels.copy())
+
+ results = object_name_filter(input_dict)
+ bboxes_3d = results['gt_bboxes_3d']
+ labels_3d = results['gt_labels_3d']
+ keep_mask = np.array([name in class_names for name in gt_names])
+ assert torch.allclose(gt_bboxes_3d.tensor[keep_mask], bboxes_3d.tensor)
+ assert np.all(gt_labels[keep_mask] == labels_3d)
+
+ repr_str = repr(object_name_filter)
+ expected_repr_str = f'ObjectNameFilter(classes={class_names})'
+ assert repr_str == expected_repr_str
+
+
+def test_point_shuffle():
+ np.random.seed(0)
+ torch.manual_seed(0)
+ point_shuffle = PointShuffle()
+
+ points = np.fromfile('tests/data/scannet/points/scene0000_00.bin',
+ np.float32).reshape(-1, 6)
+ ins_mask = np.fromfile('tests/data/scannet/instance_mask/scene0000_00.bin',
+ np.int64)
+ sem_mask = np.fromfile('tests/data/scannet/semantic_mask/scene0000_00.bin',
+ np.int64)
+
+ points = DepthPoints(
+ points.copy(), points_dim=6, attribute_dims=dict(color=[3, 4, 5]))
+ input_dict = dict(
+ points=points.clone(),
+ pts_instance_mask=ins_mask.copy(),
+ pts_semantic_mask=sem_mask.copy())
+ results = point_shuffle(input_dict)
+
+ shuffle_pts = results['points']
+ shuffle_ins_mask = results['pts_instance_mask']
+ shuffle_sem_mask = results['pts_semantic_mask']
+
+ shuffle_idx = np.array([
+ 44, 19, 93, 90, 71, 69, 37, 95, 53, 91, 81, 42, 80, 85, 74, 56, 76, 63,
+ 82, 40, 26, 92, 57, 10, 16, 66, 89, 41, 97, 8, 31, 24, 35, 30, 65, 7,
+ 98, 23, 20, 29, 78, 61, 94, 15, 4, 52, 59, 5, 54, 46, 3, 28, 2, 70, 6,
+ 60, 49, 68, 55, 72, 79, 77, 45, 1, 32, 34, 11, 0, 22, 12, 87, 50, 25,
+ 47, 36, 96, 9, 83, 62, 84, 18, 17, 75, 67, 13, 48, 39, 21, 64, 88, 38,
+ 27, 14, 73, 33, 58, 86, 43, 99, 51
+ ])
+ expected_pts = points.tensor.numpy()[shuffle_idx]
+ expected_ins_mask = ins_mask[shuffle_idx]
+ expected_sem_mask = sem_mask[shuffle_idx]
+
+ assert np.allclose(shuffle_pts.tensor.numpy(), expected_pts)
+ assert np.all(shuffle_ins_mask == expected_ins_mask)
+ assert np.all(shuffle_sem_mask == expected_sem_mask)
+
+ repr_str = repr(point_shuffle)
+ expected_repr_str = 'PointShuffle'
+ assert repr_str == expected_repr_str
+
+
+def test_points_range_filter():
+ pcd_range = [0.0, 0.0, 0.0, 3.0, 3.0, 3.0]
+ points_range_filter = PointsRangeFilter(pcd_range)
+
+ points = np.fromfile('tests/data/scannet/points/scene0000_00.bin',
+ np.float32).reshape(-1, 6)
+ ins_mask = np.fromfile('tests/data/scannet/instance_mask/scene0000_00.bin',
+ np.int64)
+ sem_mask = np.fromfile('tests/data/scannet/semantic_mask/scene0000_00.bin',
+ np.int64)
+
+ points = DepthPoints(
+ points.copy(), points_dim=6, attribute_dims=dict(color=[3, 4, 5]))
+ input_dict = dict(
+ points=points.clone(),
+ pts_instance_mask=ins_mask.copy(),
+ pts_semantic_mask=sem_mask.copy())
+ results = points_range_filter(input_dict)
+ shuffle_pts = results['points']
+ shuffle_ins_mask = results['pts_instance_mask']
+ shuffle_sem_mask = results['pts_semantic_mask']
+
+ select_idx = np.array(
+ [5, 11, 22, 26, 27, 33, 46, 47, 56, 63, 74, 78, 79, 91])
+ expected_pts = points.tensor.numpy()[select_idx]
+ expected_ins_mask = ins_mask[select_idx]
+ expected_sem_mask = sem_mask[select_idx]
+
+ assert np.allclose(shuffle_pts.tensor.numpy(), expected_pts)
+ assert np.all(shuffle_ins_mask == expected_ins_mask)
+ assert np.all(shuffle_sem_mask == expected_sem_mask)
+
+ repr_str = repr(points_range_filter)
+ expected_repr_str = f'PointsRangeFilter(point_cloud_range={pcd_range})'
+ assert repr_str == expected_repr_str
+
+
+def test_object_range_filter():
+ point_cloud_range = [0, -40, -3, 70.4, 40, 1]
+ object_range_filter = ObjectRangeFilter(point_cloud_range)
+
+ bbox = np.array(
+ [[8.7314, -1.8559, -0.6547, 0.4800, 1.2000, 1.8900, 0.0100],
+ [28.7314, -18.559, 0.6547, 2.4800, 1.6000, 1.9200, 5.0100],
+ [-2.54, -1.8559, -0.6547, 0.4800, 1.2000, 1.8900, 0.0100],
+ [72.7314, -18.559, 0.6547, 6.4800, 11.6000, 4.9200, -0.0100],
+ [18.7314, -18.559, 20.6547, 6.4800, 8.6000, 3.9200, -1.0100],
+ [3.7314, 42.559, -0.6547, 6.4800, 8.6000, 2.9200, 3.0100]])
+ gt_bboxes_3d = LiDARInstance3DBoxes(bbox, origin=(0.5, 0.5, 0.5))
+ gt_labels_3d = np.array([0, 2, 1, 1, 2, 0], dtype=np.int64)
+
+ input_dict = dict(
+ gt_bboxes_3d=gt_bboxes_3d.clone(), gt_labels_3d=gt_labels_3d.copy())
+ results = object_range_filter(input_dict)
+ bboxes_3d = results['gt_bboxes_3d']
+ labels_3d = results['gt_labels_3d']
+ keep_mask = np.array([True, True, False, False, True, False])
+ expected_bbox = gt_bboxes_3d.tensor[keep_mask]
+ expected_bbox[1, 6] -= 2 * np.pi # limit yaw
+
+ assert torch.allclose(expected_bbox, bboxes_3d.tensor)
+ assert np.all(gt_labels_3d[keep_mask] == labels_3d)
+
+ repr_str = repr(object_range_filter)
+ expected_repr_str = 'ObjectRangeFilter(point_cloud_range=' \
+ '[0.0, -40.0, -3.0, 70.4000015258789, 40.0, 1.0])'
+ assert repr_str == expected_repr_str
+
+
+def test_global_alignment():
+ np.random.seed(0)
+ global_alignment = GlobalAlignment(rotation_axis=2)
+
+ points = np.fromfile('tests/data/scannet/points/scene0000_00.bin',
+ np.float32).reshape(-1, 6)
+ annos = mmcv.load('tests/data/scannet/scannet_infos.pkl')
+ info = annos[0]
+ axis_align_matrix = info['annos']['axis_align_matrix']
+
+ depth_points = DepthPoints(points.copy(), points_dim=6)
+
+ input_dict = dict(
+ points=depth_points.clone(),
+ ann_info=dict(axis_align_matrix=axis_align_matrix))
+
+ input_dict = global_alignment(input_dict)
+ trans_depth_points = input_dict['points']
+
+ # construct expected transformed points by affine transformation
+ pts = np.ones((points.shape[0], 4))
+ pts[:, :3] = points[:, :3]
+ trans_pts = np.dot(pts, axis_align_matrix.T)
+ expected_points = np.concatenate([trans_pts[:, :3], points[:, 3:]], axis=1)
+
+ assert np.allclose(
+ trans_depth_points.tensor.numpy(), expected_points, atol=1e-6)
+
+ repr_str = repr(global_alignment)
+ expected_repr_str = 'GlobalAlignment(rotation_axis=2)'
+ assert repr_str == expected_repr_str
+
+
+def test_global_rot_scale_trans():
+ angle = 0.78539816
+ scale = [0.95, 1.05]
+ trans_std = 1.0
+
+ # rot_range should be a number or seq of numbers
+ with pytest.raises(AssertionError):
+ global_rot_scale_trans = GlobalRotScaleTrans(rot_range='0.0')
+
+ # scale_ratio_range should be seq of numbers
+ with pytest.raises(AssertionError):
+ global_rot_scale_trans = GlobalRotScaleTrans(scale_ratio_range=1.0)
+
+ # translation_std should be a positive number or seq of positive numbers
+ with pytest.raises(AssertionError):
+ global_rot_scale_trans = GlobalRotScaleTrans(translation_std='0.0')
+ with pytest.raises(AssertionError):
+ global_rot_scale_trans = GlobalRotScaleTrans(translation_std=-1.0)
+
+ global_rot_scale_trans = GlobalRotScaleTrans(
+ rot_range=angle,
+ scale_ratio_range=scale,
+ translation_std=trans_std,
+ shift_height=False)
+
+ np.random.seed(0)
+ points = np.fromfile('tests/data/scannet/points/scene0000_00.bin',
+ np.float32).reshape(-1, 6)
+ annos = mmcv.load('tests/data/scannet/scannet_infos.pkl')
+ info = annos[0]
+ gt_bboxes_3d = info['annos']['gt_boxes_upright_depth']
+
+ depth_points = DepthPoints(
+ points.copy(), points_dim=6, attribute_dims=dict(color=[3, 4, 5]))
+ gt_bboxes_3d = DepthInstance3DBoxes(
+ gt_bboxes_3d.copy(),
+ box_dim=gt_bboxes_3d.shape[-1],
+ with_yaw=False,
+ origin=(0.5, 0.5, 0.5))
+
+ input_dict = dict(
+ points=depth_points.clone(),
+ bbox3d_fields=['gt_bboxes_3d'],
+ gt_bboxes_3d=gt_bboxes_3d.clone())
+
+ input_dict = global_rot_scale_trans(input_dict)
+ trans_depth_points = input_dict['points']
+ trans_bboxes_3d = input_dict['gt_bboxes_3d']
+
+ noise_rot = 0.07667607233534723
+ scale_factor = 1.021518936637242
+ trans_factor = np.array([0.97873798, 2.2408932, 1.86755799])
+
+ true_depth_points = depth_points.clone()
+ true_bboxes_3d = gt_bboxes_3d.clone()
+ true_depth_points, noise_rot_mat_T = true_bboxes_3d.rotate(
+ noise_rot, true_depth_points)
+ true_bboxes_3d.scale(scale_factor)
+ true_bboxes_3d.translate(trans_factor)
+ true_depth_points.scale(scale_factor)
+ true_depth_points.translate(trans_factor)
+
+ assert torch.allclose(
+ trans_depth_points.tensor, true_depth_points.tensor, atol=1e-6)
+ assert torch.allclose(
+ trans_bboxes_3d.tensor, true_bboxes_3d.tensor, atol=1e-6)
+ assert input_dict['pcd_scale_factor'] == scale_factor
+ assert torch.allclose(
+ input_dict['pcd_rotation'], noise_rot_mat_T, atol=1e-6)
+ assert np.allclose(input_dict['pcd_trans'], trans_factor)
+
+ repr_str = repr(global_rot_scale_trans)
+ expected_repr_str = f'GlobalRotScaleTrans(rot_range={[-angle, angle]},' \
+ f' scale_ratio_range={scale},' \
+ f' translation_std={[trans_std for _ in range(3)]},' \
+ f' shift_height=False)'
+ assert repr_str == expected_repr_str
+
+ # points with shift_height but no bbox
+ global_rot_scale_trans = GlobalRotScaleTrans(
+ rot_range=angle,
+ scale_ratio_range=scale,
+ translation_std=trans_std,
+ shift_height=True)
+
+ # points should have height attribute when shift_height=True
+ with pytest.raises(AssertionError):
+ input_dict = global_rot_scale_trans(input_dict)
+
+ np.random.seed(0)
+ shift_height = points[:, 2:3] * 0.99
+ points = np.concatenate([points, shift_height], axis=1)
+ depth_points = DepthPoints(
+ points.copy(),
+ points_dim=7,
+ attribute_dims=dict(color=[3, 4, 5], height=6))
+
+ input_dict = dict(points=depth_points.clone(), bbox3d_fields=[])
+
+ input_dict = global_rot_scale_trans(input_dict)
+ trans_depth_points = input_dict['points']
+ true_shift_height = shift_height * scale_factor
+
+ assert np.allclose(
+ trans_depth_points.tensor.numpy(),
+ np.concatenate([true_depth_points.tensor.numpy(), true_shift_height],
+ axis=1),
+ atol=1e-6)
+
+
+def test_random_drop_points_color():
+ # drop_ratio should be in [0, 1]
+ with pytest.raises(AssertionError):
+ random_drop_points_color = RandomDropPointsColor(drop_ratio=1.1)
+
+ # 100% drop
+ random_drop_points_color = RandomDropPointsColor(drop_ratio=1)
+
+ points = np.fromfile('tests/data/scannet/points/scene0000_00.bin',
+ np.float32).reshape(-1, 6)
+ depth_points = DepthPoints(
+ points.copy(), points_dim=6, attribute_dims=dict(color=[3, 4, 5]))
+
+ input_dict = dict(points=depth_points.clone())
+
+ input_dict = random_drop_points_color(input_dict)
+ trans_depth_points = input_dict['points']
+ trans_color = trans_depth_points.color
+ assert torch.all(trans_color == trans_color.new_zeros(trans_color.shape))
+
+ # 0% drop
+ random_drop_points_color = RandomDropPointsColor(drop_ratio=0)
+ input_dict = dict(points=depth_points.clone())
+
+ input_dict = random_drop_points_color(input_dict)
+ trans_depth_points = input_dict['points']
+ trans_color = trans_depth_points.color
+ assert torch.allclose(trans_color, depth_points.tensor[:, 3:6])
+
+ random_drop_points_color = RandomDropPointsColor(drop_ratio=0.5)
+ repr_str = repr(random_drop_points_color)
+ expected_repr_str = 'RandomDropPointsColor(drop_ratio=0.5)'
+ assert repr_str == expected_repr_str
+
+
+def test_random_flip_3d():
+ random_flip_3d = RandomFlip3D(
+ flip_ratio_bev_horizontal=1.0, flip_ratio_bev_vertical=1.0)
+ points = np.array([[22.7035, 9.3901, -0.2848, 0.0000],
+ [21.9826, 9.1766, -0.2698, 0.0000],
+ [21.4329, 9.0209, -0.2578, 0.0000],
+ [21.3068, 9.0205, -0.2558, 0.0000],
+ [21.3400, 9.1305, -0.2578, 0.0000],
+ [21.3291, 9.2099, -0.2588, 0.0000],
+ [21.2759, 9.2599, -0.2578, 0.0000],
+ [21.2686, 9.2982, -0.2588, 0.0000],
+ [21.2334, 9.3607, -0.2588, 0.0000],
+ [21.2179, 9.4372, -0.2598, 0.0000]])
+ bbox3d_fields = ['gt_bboxes_3d']
+ img_fields = []
+ box_type_3d = LiDARInstance3DBoxes
+ gt_bboxes_3d = LiDARInstance3DBoxes(
+ torch.tensor(
+ [[38.9229, 18.4417, -1.1459, 0.7100, 1.7600, 1.8600, -2.2652],
+ [12.7768, 0.5795, -2.2682, 0.5700, 0.9900, 1.7200, -2.5029],
+ [12.7557, 2.2996, -1.4869, 0.6100, 1.1100, 1.9000, -1.9390],
+ [10.6677, 0.8064, -1.5435, 0.7900, 0.9600, 1.7900, 1.0856],
+ [5.0903, 5.1004, -1.2694, 0.7100, 1.7000, 1.8300, -1.9136]]))
+ points = LiDARPoints(points, points_dim=4)
+ input_dict = dict(
+ points=points,
+ bbox3d_fields=bbox3d_fields,
+ box_type_3d=box_type_3d,
+ img_fields=img_fields,
+ gt_bboxes_3d=gt_bboxes_3d)
+ input_dict = random_flip_3d(input_dict)
+ points = input_dict['points'].tensor.numpy()
+ gt_bboxes_3d = input_dict['gt_bboxes_3d'].tensor
+ expected_points = np.array([[22.7035, -9.3901, -0.2848, 0.0000],
+ [21.9826, -9.1766, -0.2698, 0.0000],
+ [21.4329, -9.0209, -0.2578, 0.0000],
+ [21.3068, -9.0205, -0.2558, 0.0000],
+ [21.3400, -9.1305, -0.2578, 0.0000],
+ [21.3291, -9.2099, -0.2588, 0.0000],
+ [21.2759, -9.2599, -0.2578, 0.0000],
+ [21.2686, -9.2982, -0.2588, 0.0000],
+ [21.2334, -9.3607, -0.2588, 0.0000],
+ [21.2179, -9.4372, -0.2598, 0.0000]])
+ expected_gt_bboxes_3d = torch.tensor(
+ [[38.9229, -18.4417, -1.1459, 0.7100, 1.7600, 1.8600, 2.2652],
+ [12.7768, -0.5795, -2.2682, 0.5700, 0.9900, 1.7200, 2.5029],
+ [12.7557, -2.2996, -1.4869, 0.6100, 1.1100, 1.9000, 1.9390],
+ [10.6677, -0.8064, -1.5435, 0.7900, 0.9600, 1.7900, -1.0856],
+ [5.0903, -5.1004, -1.2694, 0.7100, 1.7000, 1.8300, 1.9136]])
+ repr_str = repr(random_flip_3d)
+ expected_repr_str = 'RandomFlip3D(sync_2d=True,' \
+ ' flip_ratio_bev_vertical=1.0)'
+ assert np.allclose(points, expected_points)
+ assert torch.allclose(gt_bboxes_3d, expected_gt_bboxes_3d)
+ assert repr_str == expected_repr_str
+
+
+def test_random_jitter_points():
+ # jitter_std should be a number or seq of numbers
+ with pytest.raises(AssertionError):
+ random_jitter_points = RandomJitterPoints(jitter_std='0.0')
+
+ # clip_range should be a number or seq of numbers
+ with pytest.raises(AssertionError):
+ random_jitter_points = RandomJitterPoints(clip_range='0.0')
+
+ random_jitter_points = RandomJitterPoints(jitter_std=0.01, clip_range=0.05)
+ np.random.seed(0)
+ points = np.fromfile('tests/data/scannet/points/scene0000_00.bin',
+ np.float32).reshape(-1, 6)[:10]
+ depth_points = DepthPoints(
+ points.copy(), points_dim=6, attribute_dims=dict(color=[3, 4, 5]))
+
+ input_dict = dict(points=depth_points.clone())
+
+ input_dict = random_jitter_points(input_dict)
+ trans_depth_points = input_dict['points']
+
+ jitter_noise = np.array([[0.01764052, 0.00400157, 0.00978738],
+ [0.02240893, 0.01867558, -0.00977278],
+ [0.00950088, -0.00151357, -0.00103219],
+ [0.00410598, 0.00144044, 0.01454273],
+ [0.00761038, 0.00121675, 0.00443863],
+ [0.00333674, 0.01494079, -0.00205158],
+ [0.00313068, -0.00854096, -0.0255299],
+ [0.00653619, 0.00864436, -0.00742165],
+ [0.02269755, -0.01454366, 0.00045759],
+ [-0.00187184, 0.01532779, 0.01469359]])
+
+ trans_depth_points = trans_depth_points.tensor.numpy()
+ expected_depth_points = points
+ expected_depth_points[:, :3] += jitter_noise
+ assert np.allclose(trans_depth_points, expected_depth_points)
+
+ repr_str = repr(random_jitter_points)
+ jitter_std = [0.01, 0.01, 0.01]
+ clip_range = [-0.05, 0.05]
+ expected_repr_str = f'RandomJitterPoints(jitter_std={jitter_std},' \
+ f' clip_range={clip_range})'
+ assert repr_str == expected_repr_str
+
+ # test clipping very large noise
+ random_jitter_points = RandomJitterPoints(jitter_std=1.0, clip_range=0.05)
+ input_dict = dict(points=depth_points.clone())
+
+ input_dict = random_jitter_points(input_dict)
+ trans_depth_points = input_dict['points']
+ assert (trans_depth_points.tensor - depth_points.tensor).max().item() <= \
+ 0.05 + 1e-6
+ assert (trans_depth_points.tensor - depth_points.tensor).min().item() >= \
+ -0.05 - 1e-6
+
+
+def test_background_points_filter():
+ np.random.seed(0)
+ background_points_filter = BackgroundPointsFilter((0.5, 2.0, 0.5))
+ points = np.fromfile(
+ './tests/data/kitti/training/velodyne_reduced/000000.bin',
+ np.float32).reshape(-1, 4)
+ orig_points = points.copy()
+ annos = mmcv.load('./tests/data/kitti/kitti_infos_train.pkl')
+ info = annos[0]
+ rect = info['calib']['R0_rect'].astype(np.float32)
+ Trv2c = info['calib']['Tr_velo_to_cam'].astype(np.float32)
+ annos = info['annos']
+ loc = annos['location']
+ dims = annos['dimensions']
+ rots = annos['rotation_y']
+ gt_bboxes_3d = np.concatenate([loc, dims, rots[..., np.newaxis]],
+ axis=1).astype(np.float32)
+ gt_bboxes_3d = CameraInstance3DBoxes(gt_bboxes_3d).convert_to(
+ Box3DMode.LIDAR, np.linalg.inv(rect @ Trv2c))
+ extra_points = gt_bboxes_3d.corners.reshape(8, 3)[[1, 2, 5, 6], :]
+ extra_points[:, 2] += 0.1
+ extra_points = torch.cat([extra_points, extra_points.new_zeros(4, 1)], 1)
+ points = np.concatenate([points, extra_points.numpy()], 0)
+ points = LiDARPoints(points, points_dim=4)
+ input_dict = dict(points=points, gt_bboxes_3d=gt_bboxes_3d)
+ origin_gt_bboxes_3d = gt_bboxes_3d.clone()
+ input_dict = background_points_filter(input_dict)
+
+ points = input_dict['points'].tensor.numpy()
+ repr_str = repr(background_points_filter)
+ expected_repr_str = 'BackgroundPointsFilter(bbox_enlarge_range=' \
+ '[[0.5, 2.0, 0.5]])'
+ assert repr_str == expected_repr_str
+ assert points.shape == (800, 4)
+ assert np.equal(orig_points, points).all()
+ assert np.equal(input_dict['gt_bboxes_3d'].tensor.numpy(),
+ origin_gt_bboxes_3d.tensor.numpy()).all()
+
+ # test single float config
+ BackgroundPointsFilter(0.5)
+
+ # The length of bbox_enlarge_range should be 3
+ with pytest.raises(AssertionError):
+ BackgroundPointsFilter((0.5, 2.0))
+
+
+def test_voxel_based_point_filter():
+ np.random.seed(0)
+ cur_sweep_cfg = dict(
+ voxel_size=[0.1, 0.1, 0.1],
+ point_cloud_range=[-50, -50, -4, 50, 50, 2],
+ max_num_points=1,
+ max_voxels=1024)
+ prev_sweep_cfg = dict(
+ voxel_size=[0.1, 0.1, 0.1],
+ point_cloud_range=[-50, -50, -4, 50, 50, 2],
+ max_num_points=1,
+ max_voxels=1024)
+ voxel_based_points_filter = VoxelBasedPointSampler(
+ cur_sweep_cfg, prev_sweep_cfg, time_dim=3)
+ points = np.stack([
+ np.random.rand(4096) * 120 - 60,
+ np.random.rand(4096) * 120 - 60,
+ np.random.rand(4096) * 10 - 6
+ ],
+ axis=-1)
+
+ input_time = np.concatenate([np.zeros([2048, 1]), np.ones([2048, 1])], 0)
+ input_points = np.concatenate([points, input_time], 1)
+ input_points = LiDARPoints(input_points, points_dim=4)
+ input_dict = dict(
+ points=input_points, pts_mask_fields=[], pts_seg_fields=[])
+ input_dict = voxel_based_points_filter(input_dict)
+
+ points = input_dict['points']
+ repr_str = repr(voxel_based_points_filter)
+ expected_repr_str = """VoxelBasedPointSampler(
+ num_cur_sweep=1024,
+ num_prev_sweep=1024,
+ time_dim=3,
+ cur_voxel_generator=
+ VoxelGenerator(voxel_size=[0.1 0.1 0.1],
+ point_cloud_range=[-50.0, -50.0, -4.0, 50.0, 50.0, 2.0],
+ max_num_points=1,
+ max_voxels=1024,
+ grid_size=[1000, 1000, 60]),
+ prev_voxel_generator=
+ VoxelGenerator(voxel_size=[0.1 0.1 0.1],
+ point_cloud_range=[-50.0, -50.0, -4.0, 50.0, 50.0, 2.0],
+ max_num_points=1,
+ max_voxels=1024,
+ grid_size=[1000, 1000, 60]))"""
+
+ assert repr_str == expected_repr_str
+ assert points.shape == (2048, 4)
+ assert (points.tensor[:, :3].min(0)[0].numpy() <
+ cur_sweep_cfg['point_cloud_range'][0:3]).sum() == 0
+ assert (points.tensor[:, :3].max(0)[0].numpy() >
+ cur_sweep_cfg['point_cloud_range'][3:6]).sum() == 0
+
+ # Test instance mask and semantic mask
+ input_dict = dict(points=input_points)
+ input_dict['pts_instance_mask'] = np.random.randint(0, 10, [4096])
+ input_dict['pts_semantic_mask'] = np.random.randint(0, 6, [4096])
+ input_dict['pts_mask_fields'] = ['pts_instance_mask']
+ input_dict['pts_seg_fields'] = ['pts_semantic_mask']
+
+ input_dict = voxel_based_points_filter(input_dict)
+ pts_instance_mask = input_dict['pts_instance_mask']
+ pts_semantic_mask = input_dict['pts_semantic_mask']
+ assert pts_instance_mask.shape == (2048, )
+ assert pts_semantic_mask.shape == (2048, )
+ assert pts_instance_mask.max() < 10
+ assert pts_instance_mask.min() >= 0
+ assert pts_semantic_mask.max() < 6
+ assert pts_semantic_mask.min() >= 0
+
+
+def test_points_sample():
+ np.random.seed(0)
+ points = np.fromfile(
+ './tests/data/kitti/training/velodyne_reduced/000000.bin',
+ np.float32).reshape(-1, 4)
+ annos = mmcv.load('./tests/data/kitti/kitti_infos_train.pkl')
+ info = annos[0]
+ rect = torch.tensor(info['calib']['R0_rect'].astype(np.float32))
+ Trv2c = torch.tensor(info['calib']['Tr_velo_to_cam'].astype(np.float32))
+
+ points = LiDARPoints(
+ points.copy(), points_dim=4).convert_to(Coord3DMode.CAM, rect @ Trv2c)
+ num_points = 20
+ sample_range = 40
+ input_dict = dict(points=points.clone())
+
+ point_sample = PointSample(
+ num_points=num_points, sample_range=sample_range)
+ sampled_pts = point_sample(input_dict)['points']
+
+ select_idx = np.array([
+ 622, 146, 231, 444, 504, 533, 80, 401, 379, 2, 707, 562, 176, 491, 496,
+ 464, 15, 590, 194, 449
+ ])
+ expected_pts = points.tensor.numpy()[select_idx]
+ assert np.allclose(sampled_pts.tensor.numpy(), expected_pts)
+
+ repr_str = repr(point_sample)
+ expected_repr_str = f'PointSample(num_points={num_points}, ' \
+ f'sample_range={sample_range}, ' \
+ 'replace=False)'
+ assert repr_str == expected_repr_str
+
+ # test when number of far points are larger than number of sampled points
+ np.random.seed(0)
+ point_sample = PointSample(num_points=2, sample_range=sample_range)
+ input_dict = dict(points=points.clone())
+ sampled_pts = point_sample(input_dict)['points']
+
+ select_idx = np.array([449, 444])
+ expected_pts = points.tensor.numpy()[select_idx]
+ assert np.allclose(sampled_pts.tensor.numpy(), expected_pts)
+
+
+def test_affine_resize():
+
+ def create_random_bboxes(num_bboxes, img_w, img_h):
+ bboxes_left_top = np.random.uniform(0, 0.5, size=(num_bboxes, 2))
+ bboxes_right_bottom = np.random.uniform(0.5, 1, size=(num_bboxes, 2))
+ bboxes = np.concatenate((bboxes_left_top, bboxes_right_bottom), 1)
+ bboxes = (bboxes * np.array([img_w, img_h, img_w, img_h])).astype(
+ np.float32)
+ return bboxes
+
+ affine_reszie = AffineResize(img_scale=(1290, 384), down_ratio=4)
+
+ # test the situation: not use Random_Scale_Shift before AffineResize
+ results = dict()
+ img = mmcv.imread('./tests/data/kitti/training/image_2/000000.png',
+ 'color')
+ results['img'] = img
+ results['bbox_fields'] = ['gt_bboxes']
+ results['bbox3d_fields'] = ['gt_bboxes_3d']
+
+ h, w, _ = img.shape
+ gt_bboxes = create_random_bboxes(8, w, h)
+ gt_bboxes_3d = CameraInstance3DBoxes(torch.randn((8, 7)))
+ results['gt_labels'] = np.ones(gt_bboxes.shape[0], dtype=np.int64)
+ results['gt_labels3d'] = results['gt_labels']
+ results['gt_bboxes'] = gt_bboxes
+ results['gt_bboxes_3d'] = gt_bboxes_3d
+ results['depths'] = np.random.randn(gt_bboxes.shape[0])
+ centers2d_x = (gt_bboxes[:, [0]] + gt_bboxes[:, [2]]) / 2
+ centers2d_y = (gt_bboxes[:, [1]] + gt_bboxes[:, [3]]) / 2
+ centers2d = np.concatenate((centers2d_x, centers2d_y), axis=1)
+ results['centers2d'] = centers2d
+
+ results = affine_reszie(results)
+
+ assert results['gt_labels'].shape[0] == results['centers2d'].shape[0]
+ assert results['gt_labels3d'].shape[0] == results['centers2d'].shape[0]
+ assert results['gt_bboxes'].shape[0] == results['centers2d'].shape[0]
+ assert results['gt_bboxes_3d'].tensor.shape[0] == \
+ results['centers2d'].shape[0]
+ assert results['affine_aug'] is False
+
+ # test the situation: not use Random_Scale_Shift before AffineResize
+ results = dict()
+ img = mmcv.imread('./tests/data/kitti/training/image_2/000000.png',
+ 'color')
+ results['img'] = img
+ results['bbox_fields'] = ['gt_bboxes']
+ results['bbox3d_fields'] = ['gt_bboxes_3d']
+ h, w, _ = img.shape
+ center = np.array([w / 2, h / 2], dtype=np.float32)
+ size = np.array([w, h], dtype=np.float32)
+
+ results['center'] = center
+ results['size'] = size
+ results['affine_aug'] = False
+
+ gt_bboxes = create_random_bboxes(8, w, h)
+ gt_bboxes_3d = CameraInstance3DBoxes(torch.randn((8, 7)))
+ results['gt_labels'] = np.ones(gt_bboxes.shape[0], dtype=np.int64)
+ results['gt_labels3d'] = results['gt_labels']
+ results['gt_bboxes'] = gt_bboxes
+ results['gt_bboxes_3d'] = gt_bboxes_3d
+ results['depths'] = np.random.randn(gt_bboxes.shape[0])
+ centers2d_x = (gt_bboxes[:, [0]] + gt_bboxes[:, [2]]) / 2
+ centers2d_y = (gt_bboxes[:, [1]] + gt_bboxes[:, [3]]) / 2
+ centers2d = np.concatenate((centers2d_x, centers2d_y), axis=1)
+ results['centers2d'] = centers2d
+
+ results = affine_reszie(results)
+
+ assert results['gt_labels'].shape[0] == results['centers2d'].shape[0]
+ assert results['gt_labels3d'].shape[0] == results['centers2d'].shape[0]
+ assert results['gt_bboxes'].shape[0] == results['centers2d'].shape[0]
+ assert results['gt_bboxes_3d'].tensor.shape[0] == results[
+ 'centers2d'].shape[0]
+ assert 'center' in results
+ assert 'size' in results
+ assert 'affine_aug' in results
+
+
+def test_random_shift_scale():
+ random_shift_scale = RandomShiftScale(shift_scale=(0.2, 0.4), aug_prob=0.3)
+ results = dict()
+ img = mmcv.imread('./tests/data/kitti/training/image_2/000000.png',
+ 'color')
+ results['img'] = img
+ results = random_shift_scale(results)
+ assert results['center'].dtype == np.float32
+ assert results['size'].dtype == np.float32
+ assert 'affine_aug' in results
diff --git a/tests/test_data/test_pipelines/test_indoor_pipeline.py b/tests/test_data/test_pipelines/test_indoor_pipeline.py
new file mode 100644
index 0000000..bfe1b41
--- /dev/null
+++ b/tests/test_data/test_pipelines/test_indoor_pipeline.py
@@ -0,0 +1,341 @@
+# Copyright (c) OpenMMLab. All rights reserved.
+from os import path as osp
+
+import mmcv
+import numpy as np
+import torch
+
+from mmdet3d.core.bbox import DepthInstance3DBoxes
+from mmdet3d.datasets.pipelines import Compose
+
+
+def test_scannet_pipeline():
+ class_names = ('cabinet', 'bed', 'chair', 'sofa', 'table', 'door',
+ 'window', 'bookshelf', 'picture', 'counter', 'desk',
+ 'curtain', 'refrigerator', 'showercurtrain', 'toilet',
+ 'sink', 'bathtub', 'garbagebin')
+
+ np.random.seed(0)
+ pipelines = [
+ dict(
+ type='LoadPointsFromFile',
+ coord_type='DEPTH',
+ shift_height=True,
+ load_dim=6,
+ use_dim=[0, 1, 2]),
+ dict(
+ type='LoadAnnotations3D',
+ with_bbox_3d=True,
+ with_label_3d=True,
+ with_mask_3d=True,
+ with_seg_3d=True),
+ dict(type='GlobalAlignment', rotation_axis=2),
+ dict(
+ type='PointSegClassMapping',
+ valid_cat_ids=(3, 4, 5, 6, 7, 8, 9, 10, 11, 12, 14, 16, 24, 28, 33,
+ 34, 36, 39)),
+ dict(type='PointSample', num_points=5),
+ dict(
+ type='RandomFlip3D',
+ sync_2d=False,
+ flip_ratio_bev_horizontal=1.0,
+ flip_ratio_bev_vertical=1.0),
+ dict(
+ type='GlobalRotScaleTrans',
+ rot_range=[-0.087266, 0.087266],
+ scale_ratio_range=[1.0, 1.0],
+ shift_height=True),
+ dict(type='DefaultFormatBundle3D', class_names=class_names),
+ dict(
+ type='Collect3D',
+ keys=[
+ 'points', 'gt_bboxes_3d', 'gt_labels_3d', 'pts_semantic_mask',
+ 'pts_instance_mask'
+ ]),
+ ]
+ pipeline = Compose(pipelines)
+ info = mmcv.load('./tests/data/scannet/scannet_infos.pkl')[0]
+ results = dict()
+ data_path = './tests/data/scannet'
+ results['pts_filename'] = osp.join(data_path, info['pts_path'])
+ if info['annos']['gt_num'] != 0:
+ scannet_gt_bboxes_3d = info['annos']['gt_boxes_upright_depth'].astype(
+ np.float32)
+ scannet_gt_labels_3d = info['annos']['class'].astype(np.int64)
+ else:
+ scannet_gt_bboxes_3d = np.zeros((1, 6), dtype=np.float32)
+ scannet_gt_labels_3d = np.zeros((1, ), dtype=np.int64)
+ results['ann_info'] = dict()
+ results['ann_info']['pts_instance_mask_path'] = osp.join(
+ data_path, info['pts_instance_mask_path'])
+ results['ann_info']['pts_semantic_mask_path'] = osp.join(
+ data_path, info['pts_semantic_mask_path'])
+ results['ann_info']['gt_bboxes_3d'] = DepthInstance3DBoxes(
+ scannet_gt_bboxes_3d, box_dim=6, with_yaw=False)
+ results['ann_info']['gt_labels_3d'] = scannet_gt_labels_3d
+ results['ann_info']['axis_align_matrix'] = \
+ info['annos']['axis_align_matrix']
+
+ results['img_fields'] = []
+ results['bbox3d_fields'] = []
+ results['pts_mask_fields'] = []
+ results['pts_seg_fields'] = []
+
+ results = pipeline(results)
+
+ points = results['points']._data
+ gt_bboxes_3d = results['gt_bboxes_3d']._data
+ gt_labels_3d = results['gt_labels_3d']._data
+ pts_semantic_mask = results['pts_semantic_mask']._data
+ pts_instance_mask = results['pts_instance_mask']._data
+ expected_points = torch.tensor(
+ [[1.8339e+00, 2.1093e+00, 2.2900e+00, 2.3895e+00],
+ [3.6079e+00, 1.4592e-01, 2.0687e+00, 2.1682e+00],
+ [4.1886e+00, 5.0614e+00, -1.0841e-01, -8.8736e-03],
+ [6.8790e+00, 1.5086e+00, -9.3154e-02, 6.3816e-03],
+ [4.8253e+00, 2.6668e-01, 1.4917e+00, 1.5912e+00]])
+ expected_gt_bboxes_3d = torch.tensor(
+ [[-1.1835, -3.6317, 1.8565, 1.7577, 0.3761, 0.5724, 0.0000],
+ [-3.1832, 3.2269, 1.5268, 0.6727, 0.2251, 0.6715, 0.0000],
+ [-0.9598, -2.2864, 0.6165, 0.7506, 2.5709, 1.2145, 0.0000],
+ [-2.6988, -2.7354, 0.9722, 0.7680, 1.8877, 0.2870, 0.0000],
+ [3.2989, 0.2885, 1.0712, 0.7600, 3.8814, 2.1603, 0.0000]])
+ expected_gt_labels_3d = np.array([
+ 6, 6, 4, 9, 11, 11, 10, 0, 15, 17, 17, 17, 3, 12, 4, 4, 14, 1, 0, 0, 0,
+ 0, 0, 0, 5, 5, 5
+ ])
+ expected_pts_semantic_mask = np.array([0, 18, 18, 18, 18])
+ expected_pts_instance_mask = np.array([44, 22, 10, 10, 57])
+ assert torch.allclose(points, expected_points, 1e-2)
+ assert torch.allclose(gt_bboxes_3d.tensor[:5, :], expected_gt_bboxes_3d,
+ 1e-2)
+ assert np.all(gt_labels_3d.numpy() == expected_gt_labels_3d)
+ assert np.all(pts_semantic_mask.numpy() == expected_pts_semantic_mask)
+ assert np.all(pts_instance_mask.numpy() == expected_pts_instance_mask)
+
+
+def test_scannet_seg_pipeline():
+ class_names = ('wall', 'floor', 'cabinet', 'bed', 'chair', 'sofa', 'table',
+ 'door', 'window', 'bookshelf', 'picture', 'counter', 'desk',
+ 'curtain', 'refrigerator', 'showercurtrain', 'toilet',
+ 'sink', 'bathtub', 'otherfurniture')
+
+ np.random.seed(0)
+ pipelines = [
+ dict(
+ type='LoadPointsFromFile',
+ coord_type='DEPTH',
+ shift_height=False,
+ use_color=True,
+ load_dim=6,
+ use_dim=[0, 1, 2, 3, 4, 5]),
+ dict(
+ type='LoadAnnotations3D',
+ with_bbox_3d=False,
+ with_label_3d=False,
+ with_mask_3d=False,
+ with_seg_3d=True),
+ dict(
+ type='PointSegClassMapping',
+ valid_cat_ids=(1, 2, 3, 4, 5, 6, 7, 8, 9, 10, 11, 12, 14, 16, 24,
+ 28, 33, 34, 36, 39),
+ max_cat_id=40),
+ dict(
+ type='IndoorPatchPointSample',
+ num_points=5,
+ block_size=1.5,
+ ignore_index=len(class_names),
+ use_normalized_coord=True,
+ enlarge_size=0.2,
+ min_unique_num=None),
+ dict(type='NormalizePointsColor', color_mean=None),
+ dict(type='DefaultFormatBundle3D', class_names=class_names),
+ dict(type='Collect3D', keys=['points', 'pts_semantic_mask'])
+ ]
+ pipeline = Compose(pipelines)
+ info = mmcv.load('./tests/data/scannet/scannet_infos.pkl')[0]
+ results = dict()
+ data_path = './tests/data/scannet'
+ results['pts_filename'] = osp.join(data_path, info['pts_path'])
+ results['ann_info'] = dict()
+ results['ann_info']['pts_semantic_mask_path'] = osp.join(
+ data_path, info['pts_semantic_mask_path'])
+
+ results['pts_seg_fields'] = []
+
+ results = pipeline(results)
+
+ points = results['points']._data
+ pts_semantic_mask = results['pts_semantic_mask']._data
+
+ # build sampled points
+ scannet_points = np.fromfile(
+ osp.join(data_path, info['pts_path']), dtype=np.float32).reshape(
+ (-1, 6))
+ scannet_choices = np.array([87, 34, 58, 9, 18])
+ scannet_center = np.array([-2.1772466, -3.4789145, 1.242711])
+ scannet_center[2] = 0.0
+ scannet_coord_max = np.amax(scannet_points[:, :3], axis=0)
+ expected_points = np.concatenate([
+ scannet_points[scannet_choices, :3] - scannet_center,
+ scannet_points[scannet_choices, 3:] / 255.,
+ scannet_points[scannet_choices, :3] / scannet_coord_max
+ ],
+ axis=1)
+ expected_pts_semantic_mask = np.array([13, 13, 12, 2, 0])
+ assert np.allclose(points.numpy(), expected_points, atol=1e-6)
+ assert np.all(pts_semantic_mask.numpy() == expected_pts_semantic_mask)
+
+
+def test_s3dis_seg_pipeline():
+ class_names = ('ceiling', 'floor', 'wall', 'beam', 'column', 'window',
+ 'door', 'table', 'chair', 'sofa', 'bookcase', 'board',
+ 'clutter')
+
+ np.random.seed(0)
+ pipelines = [
+ dict(
+ type='LoadPointsFromFile',
+ coord_type='DEPTH',
+ shift_height=False,
+ use_color=True,
+ load_dim=6,
+ use_dim=[0, 1, 2, 3, 4, 5]),
+ dict(
+ type='LoadAnnotations3D',
+ with_bbox_3d=False,
+ with_label_3d=False,
+ with_mask_3d=False,
+ with_seg_3d=True),
+ dict(
+ type='PointSegClassMapping',
+ valid_cat_ids=tuple(range(len(class_names))),
+ max_cat_id=13),
+ dict(
+ type='IndoorPatchPointSample',
+ num_points=5,
+ block_size=1.0,
+ ignore_index=len(class_names),
+ use_normalized_coord=True,
+ enlarge_size=0.2,
+ min_unique_num=None),
+ dict(type='NormalizePointsColor', color_mean=None),
+ dict(type='DefaultFormatBundle3D', class_names=class_names),
+ dict(type='Collect3D', keys=['points', 'pts_semantic_mask'])
+ ]
+ pipeline = Compose(pipelines)
+ info = mmcv.load('./tests/data/s3dis/s3dis_infos.pkl')[0]
+ results = dict()
+ data_path = './tests/data/s3dis'
+ results['pts_filename'] = osp.join(data_path, info['pts_path'])
+ results['ann_info'] = dict()
+ results['ann_info']['pts_semantic_mask_path'] = osp.join(
+ data_path, info['pts_semantic_mask_path'])
+
+ results['pts_seg_fields'] = []
+
+ results = pipeline(results)
+
+ points = results['points']._data
+ pts_semantic_mask = results['pts_semantic_mask']._data
+
+ # build sampled points
+ s3dis_points = np.fromfile(
+ osp.join(data_path, info['pts_path']), dtype=np.float32).reshape(
+ (-1, 6))
+ s3dis_choices = np.array([87, 37, 60, 18, 31])
+ s3dis_center = np.array([2.691, 2.231, 3.172])
+ s3dis_center[2] = 0.0
+ s3dis_coord_max = np.amax(s3dis_points[:, :3], axis=0)
+ expected_points = np.concatenate([
+ s3dis_points[s3dis_choices, :3] - s3dis_center,
+ s3dis_points[s3dis_choices, 3:] / 255.,
+ s3dis_points[s3dis_choices, :3] / s3dis_coord_max
+ ],
+ axis=1)
+ expected_pts_semantic_mask = np.array([0, 1, 0, 8, 0])
+ assert np.allclose(points.numpy(), expected_points, atol=1e-6)
+ assert np.all(pts_semantic_mask.numpy() == expected_pts_semantic_mask)
+
+
+def test_sunrgbd_pipeline():
+ class_names = ('bed', 'table', 'sofa', 'chair', 'toilet', 'desk',
+ 'dresser', 'night_stand', 'bookshelf', 'bathtub')
+ np.random.seed(0)
+ pipelines = [
+ dict(
+ type='LoadPointsFromFile',
+ coord_type='DEPTH',
+ shift_height=True,
+ load_dim=6,
+ use_dim=[0, 1, 2]),
+ dict(type='LoadAnnotations3D'),
+ dict(
+ type='RandomFlip3D',
+ sync_2d=False,
+ flip_ratio_bev_horizontal=1.0,
+ ),
+ dict(
+ type='GlobalRotScaleTrans',
+ rot_range=[-0.523599, 0.523599],
+ scale_ratio_range=[0.85, 1.15],
+ shift_height=True),
+ dict(type='PointSample', num_points=5),
+ dict(type='DefaultFormatBundle3D', class_names=class_names),
+ dict(
+ type='Collect3D', keys=['points', 'gt_bboxes_3d', 'gt_labels_3d']),
+ ]
+ pipeline = Compose(pipelines)
+ results = dict()
+ info = mmcv.load('./tests/data/sunrgbd/sunrgbd_infos.pkl')[0]
+ data_path = './tests/data/sunrgbd'
+ results['pts_filename'] = osp.join(data_path, info['pts_path'])
+
+ if info['annos']['gt_num'] != 0:
+ gt_bboxes_3d = info['annos']['gt_boxes_upright_depth'].astype(
+ np.float32)
+ gt_labels_3d = info['annos']['class'].astype(np.int64)
+ else:
+ gt_bboxes_3d = np.zeros((1, 7), dtype=np.float32)
+ gt_labels_3d = np.zeros((1, ), dtype=np.int64)
+
+ # prepare input of pipeline
+ results['ann_info'] = dict()
+ results['ann_info']['gt_bboxes_3d'] = DepthInstance3DBoxes(gt_bboxes_3d)
+ results['ann_info']['gt_labels_3d'] = gt_labels_3d
+ results['img_fields'] = []
+ results['bbox3d_fields'] = []
+ results['pts_mask_fields'] = []
+ results['pts_seg_fields'] = []
+
+ results = pipeline(results)
+ points = results['points']._data
+ gt_bboxes_3d = results['gt_bboxes_3d']._data
+ gt_labels_3d = results['gt_labels_3d']._data
+ expected_points = torch.tensor([[0.8678, 1.3470, 0.1105, 0.0905],
+ [0.8707, 1.3635, 0.0437, 0.0238],
+ [0.8636, 1.3511, 0.0504, 0.0304],
+ [0.8690, 1.3461, 0.1265, 0.1065],
+ [0.8668, 1.3434, 0.1216, 0.1017]])
+ # Depth coordinate system update: only yaw changes since rotation in depth
+ # is counter-clockwise and yaw angle is clockwise originally
+ # But heading angles in sunrgbd data also reverses the sign
+ # and after horizontal flip the sign reverse again
+ rotation_angle = info['annos']['rotation_y']
+ expected_gt_bboxes_3d = torch.tensor(
+ [[
+ -1.2136, 4.0206, -0.2412, 2.2493, 1.8444, 1.9245,
+ 1.3989 + 0.047001579467984445 * 2 - 2 * rotation_angle[0]
+ ],
+ [
+ -2.7420, 4.5777, -0.7686, 0.5718, 0.8629, 0.9510,
+ 1.4446 + 0.047001579467984445 * 2 - 2 * rotation_angle[1]
+ ],
+ [
+ 0.9729, 1.9087, -0.1443, 0.6965, 1.5273, 2.0563,
+ 2.9924 + 0.047001579467984445 * 2 - 2 * rotation_angle[2]
+ ]]).float()
+ expected_gt_labels_3d = np.array([0, 7, 6])
+ assert torch.allclose(gt_bboxes_3d.tensor, expected_gt_bboxes_3d, 1e-3)
+ assert np.allclose(gt_labels_3d.flatten(), expected_gt_labels_3d)
+ assert torch.allclose(points, expected_points, 1e-2)
diff --git a/tests/test_data/test_pipelines/test_indoor_sample.py b/tests/test_data/test_pipelines/test_indoor_sample.py
new file mode 100644
index 0000000..2407f52
--- /dev/null
+++ b/tests/test_data/test_pipelines/test_indoor_sample.py
@@ -0,0 +1,200 @@
+# Copyright (c) OpenMMLab. All rights reserved.
+import numpy as np
+
+from mmdet3d.core.points import DepthPoints
+from mmdet3d.datasets.pipelines import (IndoorPatchPointSample, PointSample,
+ PointSegClassMapping)
+
+
+def test_indoor_sample():
+ np.random.seed(0)
+ scannet_sample_points = PointSample(5)
+ scannet_results = dict()
+ scannet_points = np.array([[1.0719866, -0.7870435, 0.8408122, 0.9196809],
+ [1.103661, 0.81065744, 2.6616862, 2.7405548],
+ [1.0276475, 1.5061463, 2.6174362, 2.6963048],
+ [-0.9709588, 0.6750515, 0.93901765, 1.0178864],
+ [1.0578915, 1.1693821, 0.87503505, 0.95390373],
+ [0.05560996, -1.5688863, 1.2440368, 1.3229055],
+ [-0.15731563, -1.7735453, 2.7535574, 2.832426],
+ [1.1188195, -0.99211365, 2.5551798, 2.6340485],
+ [-0.9186557, -1.7041215, 2.0562649, 2.1351335],
+ [-1.0128691, -1.3394243, 0.040936, 0.1198047]])
+ scannet_results['points'] = DepthPoints(
+ scannet_points, points_dim=4, attribute_dims=dict(height=3))
+ scannet_pts_instance_mask = np.array(
+ [15, 12, 11, 38, 0, 18, 17, 12, 17, 0])
+ scannet_results['pts_instance_mask'] = scannet_pts_instance_mask
+ scannet_pts_semantic_mask = np.array([38, 1, 1, 40, 0, 40, 1, 1, 1, 0])
+ scannet_results['pts_semantic_mask'] = scannet_pts_semantic_mask
+ scannet_results = scannet_sample_points(scannet_results)
+ scannet_points_result = scannet_results['points'].tensor.numpy()
+ scannet_instance_labels_result = scannet_results['pts_instance_mask']
+ scannet_semantic_labels_result = scannet_results['pts_semantic_mask']
+ scannet_choices = np.array([2, 8, 4, 9, 1])
+ assert np.allclose(scannet_points[scannet_choices], scannet_points_result)
+ assert np.all(scannet_pts_instance_mask[scannet_choices] ==
+ scannet_instance_labels_result)
+ assert np.all(scannet_pts_semantic_mask[scannet_choices] ==
+ scannet_semantic_labels_result)
+
+ np.random.seed(0)
+ sunrgbd_sample_points = PointSample(5)
+ sunrgbd_results = dict()
+ sunrgbd_point_cloud = np.array(
+ [[-1.8135729e-01, 1.4695230e+00, -1.2780589e+00, 7.8938007e-03],
+ [1.2581362e-03, 2.0561588e+00, -1.0341064e+00, 2.5184631e-01],
+ [6.8236995e-01, 3.3611867e+00, -9.2599887e-01, 3.5995382e-01],
+ [-2.9432583e-01, 1.8714852e+00, -9.0929651e-01, 3.7665617e-01],
+ [-0.5024875, 1.8032674, -1.1403012, 0.14565146],
+ [-0.520559, 1.6324949, -0.9896099, 0.2963428],
+ [0.95929825, 2.9402404, -0.8746674, 0.41128528],
+ [-0.74624217, 1.5244724, -0.8678476, 0.41810507],
+ [0.56485355, 1.5747732, -0.804522, 0.4814307],
+ [-0.0913099, 1.3673826, -1.2800645, 0.00588822]])
+ sunrgbd_results['points'] = DepthPoints(
+ sunrgbd_point_cloud, points_dim=4, attribute_dims=dict(height=3))
+ sunrgbd_results = sunrgbd_sample_points(sunrgbd_results)
+ sunrgbd_choices = np.array([2, 8, 4, 9, 1])
+ sunrgbd_points_result = sunrgbd_results['points'].tensor.numpy()
+ repr_str = repr(sunrgbd_sample_points)
+ expected_repr_str = 'PointSample(num_points=5, ' \
+ 'sample_range=None, ' \
+ 'replace=False)'
+ assert repr_str == expected_repr_str
+ assert np.allclose(sunrgbd_point_cloud[sunrgbd_choices],
+ sunrgbd_points_result)
+
+
+def test_indoor_seg_sample():
+ # test the train time behavior of IndoorPatchPointSample
+ np.random.seed(0)
+ scannet_patch_sample_points = IndoorPatchPointSample(
+ 5, 1.5, ignore_index=20, use_normalized_coord=True)
+ scannet_seg_class_mapping = \
+ PointSegClassMapping((1, 2, 3, 4, 5, 6, 7, 8, 9, 10, 11, 12, 14, 16,
+ 24, 28, 33, 34, 36, 39), 40)
+ scannet_results = dict()
+ scannet_points = np.fromfile(
+ './tests/data/scannet/points/scene0000_00.bin',
+ dtype=np.float32).reshape((-1, 6))
+ scannet_results['points'] = DepthPoints(
+ scannet_points, points_dim=6, attribute_dims=dict(color=[3, 4, 5]))
+
+ scannet_pts_semantic_mask = np.fromfile(
+ './tests/data/scannet/semantic_mask/scene0000_00.bin', dtype=np.int64)
+ scannet_results['pts_semantic_mask'] = scannet_pts_semantic_mask
+
+ scannet_results = scannet_seg_class_mapping(scannet_results)
+ scannet_results = scannet_patch_sample_points(scannet_results)
+ scannet_points_result = scannet_results['points']
+ scannet_semantic_labels_result = scannet_results['pts_semantic_mask']
+
+ # manually constructed sampled points
+ scannet_choices = np.array([87, 34, 58, 9, 18])
+ scannet_center = np.array([-2.1772466, -3.4789145, 1.242711])
+ scannet_center[2] = 0.0
+ scannet_coord_max = np.amax(scannet_points[:, :3], axis=0)
+ scannet_input_points = np.concatenate([
+ scannet_points[scannet_choices, :3] - scannet_center,
+ scannet_points[scannet_choices, 3:],
+ scannet_points[scannet_choices, :3] / scannet_coord_max
+ ], 1)
+
+ assert scannet_points_result.points_dim == 9
+ assert scannet_points_result.attribute_dims == dict(
+ color=[3, 4, 5], normalized_coord=[6, 7, 8])
+ scannet_points_result = scannet_points_result.tensor.numpy()
+ assert np.allclose(scannet_input_points, scannet_points_result, atol=1e-6)
+ assert np.all(
+ np.array([13, 13, 12, 2, 0]) == scannet_semantic_labels_result)
+
+ repr_str = repr(scannet_patch_sample_points)
+ expected_repr_str = 'IndoorPatchPointSample(num_points=5, ' \
+ 'block_size=1.5, ' \
+ 'ignore_index=20, ' \
+ 'use_normalized_coord=True, ' \
+ 'num_try=10, ' \
+ 'enlarge_size=0.2, ' \
+ 'min_unique_num=None, ' \
+ 'eps=0.01)'
+ assert repr_str == expected_repr_str
+
+ # when enlarge_size and min_unique_num are set
+ np.random.seed(0)
+ scannet_patch_sample_points = IndoorPatchPointSample(
+ 5,
+ 1.0,
+ ignore_index=20,
+ use_normalized_coord=False,
+ num_try=1000,
+ enlarge_size=None,
+ min_unique_num=5)
+ # this patch is within [0, 1] and has 5 unique points
+ # it should be selected
+ scannet_points = np.random.rand(5, 6)
+ scannet_points[0, :3] = np.array([0.5, 0.5, 0.5])
+ # generate points smaller than `min_unique_num` in local patches
+ # they won't be sampled
+ for i in range(2, 11, 2):
+ scannet_points = np.concatenate(
+ [scannet_points, np.random.rand(4, 6) + i], axis=0)
+ scannet_results = dict(
+ points=DepthPoints(
+ scannet_points, points_dim=6,
+ attribute_dims=dict(color=[3, 4, 5])),
+ pts_semantic_mask=np.random.randint(0, 20,
+ (scannet_points.shape[0], )))
+ scannet_results = scannet_patch_sample_points(scannet_results)
+ scannet_points_result = scannet_results['points']
+
+ # manually constructed sampled points
+ scannet_choices = np.array([2, 4, 3, 1, 0])
+ scannet_center = np.array([0.56804454, 0.92559665, 0.07103606])
+ scannet_center[2] = 0.0
+ scannet_input_points = np.concatenate([
+ scannet_points[scannet_choices, :3] - scannet_center,
+ scannet_points[scannet_choices, 3:],
+ ], 1)
+
+ assert scannet_points_result.points_dim == 6
+ assert scannet_points_result.attribute_dims == dict(color=[3, 4, 5])
+ scannet_points_result = scannet_points_result.tensor.numpy()
+ assert np.allclose(scannet_input_points, scannet_points_result, atol=1e-6)
+
+ # test on S3DIS dataset
+ np.random.seed(0)
+ s3dis_patch_sample_points = IndoorPatchPointSample(
+ 5, 1.0, ignore_index=None, use_normalized_coord=True)
+ s3dis_results = dict()
+ s3dis_points = np.fromfile(
+ './tests/data/s3dis/points/Area_1_office_2.bin',
+ dtype=np.float32).reshape((-1, 6))
+ s3dis_results['points'] = DepthPoints(
+ s3dis_points, points_dim=6, attribute_dims=dict(color=[3, 4, 5]))
+
+ s3dis_pts_semantic_mask = np.fromfile(
+ './tests/data/s3dis/semantic_mask/Area_1_office_2.bin', dtype=np.int64)
+ s3dis_results['pts_semantic_mask'] = s3dis_pts_semantic_mask
+
+ s3dis_results = s3dis_patch_sample_points(s3dis_results)
+ s3dis_points_result = s3dis_results['points']
+ s3dis_semantic_labels_result = s3dis_results['pts_semantic_mask']
+
+ # manually constructed sampled points
+ s3dis_choices = np.array([87, 37, 60, 18, 31])
+ s3dis_center = np.array([2.691, 2.231, 3.172])
+ s3dis_center[2] = 0.0
+ s3dis_coord_max = np.amax(s3dis_points[:, :3], axis=0)
+ s3dis_input_points = np.concatenate([
+ s3dis_points[s3dis_choices, :3] - s3dis_center,
+ s3dis_points[s3dis_choices,
+ 3:], s3dis_points[s3dis_choices, :3] / s3dis_coord_max
+ ], 1)
+
+ assert s3dis_points_result.points_dim == 9
+ assert s3dis_points_result.attribute_dims == dict(
+ color=[3, 4, 5], normalized_coord=[6, 7, 8])
+ s3dis_points_result = s3dis_points_result.tensor.numpy()
+ assert np.allclose(s3dis_input_points, s3dis_points_result, atol=1e-6)
+ assert np.all(np.array([0, 1, 0, 8, 0]) == s3dis_semantic_labels_result)
diff --git a/tests/test_data/test_pipelines/test_loadings/test_load_images_from_multi_views.py b/tests/test_data/test_pipelines/test_loadings/test_load_images_from_multi_views.py
new file mode 100644
index 0000000..925c949
--- /dev/null
+++ b/tests/test_data/test_pipelines/test_loadings/test_load_images_from_multi_views.py
@@ -0,0 +1,46 @@
+# Copyright (c) OpenMMLab. All rights reserved.
+import numpy as np
+import torch
+from mmcv.parallel import DataContainer
+
+from mmdet3d.datasets.pipelines import (DefaultFormatBundle,
+ LoadMultiViewImageFromFiles)
+
+
+def test_load_multi_view_image_from_files():
+ multi_view_img_loader = LoadMultiViewImageFromFiles(to_float32=True)
+
+ num_views = 6
+ filename = 'tests/data/waymo/kitti_format/training/image_0/0000000.png'
+ filenames = [filename for _ in range(num_views)]
+
+ input_dict = dict(img_filename=filenames)
+ results = multi_view_img_loader(input_dict)
+ img = results['img']
+ img0 = img[0]
+ img_norm_cfg = results['img_norm_cfg']
+
+ assert isinstance(img, list)
+ assert len(img) == num_views
+ assert img0.dtype == np.float32
+ assert results['filename'] == filenames
+ assert results['img_shape'] == results['ori_shape'] == \
+ results['pad_shape'] == (1280, 1920, 3, num_views)
+ assert results['scale_factor'] == 1.0
+ assert np.all(img_norm_cfg['mean'] == np.zeros(3, dtype=np.float32))
+ assert np.all(img_norm_cfg['std'] == np.ones(3, dtype=np.float32))
+ assert not img_norm_cfg['to_rgb']
+
+ repr_str = repr(multi_view_img_loader)
+ expected_str = 'LoadMultiViewImageFromFiles(to_float32=True, ' \
+ "color_type='unchanged')"
+ assert repr_str == expected_str
+
+ # test LoadMultiViewImageFromFiles's compatibility with DefaultFormatBundle
+ # refer to https://github.com/open-mmlab/mmdetection3d/issues/227
+ default_format_bundle = DefaultFormatBundle()
+ results = default_format_bundle(results)
+ img = results['img']
+
+ assert isinstance(img, DataContainer)
+ assert img._data.shape == torch.Size((num_views, 3, 1280, 1920))
diff --git a/tests/test_data/test_pipelines/test_loadings/test_load_points_from_multi_sweeps.py b/tests/test_data/test_pipelines/test_loadings/test_load_points_from_multi_sweeps.py
new file mode 100644
index 0000000..88d8b32
--- /dev/null
+++ b/tests/test_data/test_pipelines/test_loadings/test_load_points_from_multi_sweeps.py
@@ -0,0 +1,69 @@
+# Copyright (c) OpenMMLab. All rights reserved.
+import numpy as np
+
+from mmdet3d.core.points import LiDARPoints
+from mmdet3d.datasets.pipelines.loading import LoadPointsFromMultiSweeps
+
+
+def test_load_points_from_multi_sweeps():
+ np.random.seed(0)
+
+ file_client_args = dict(backend='disk')
+ load_points_from_multi_sweeps_1 = LoadPointsFromMultiSweeps(
+ sweeps_num=9,
+ use_dim=[0, 1, 2, 3, 4],
+ file_client_args=file_client_args)
+
+ load_points_from_multi_sweeps_2 = LoadPointsFromMultiSweeps(
+ sweeps_num=9,
+ use_dim=[0, 1, 2, 3, 4],
+ file_client_args=file_client_args,
+ pad_empty_sweeps=True,
+ remove_close=True)
+
+ load_points_from_multi_sweeps_3 = LoadPointsFromMultiSweeps(
+ sweeps_num=9,
+ use_dim=[0, 1, 2, 3, 4],
+ file_client_args=file_client_args,
+ pad_empty_sweeps=True,
+ remove_close=True,
+ test_mode=True)
+
+ points = np.random.random([100, 5]) * 2
+ points = LiDARPoints(points, points_dim=5)
+ input_results = dict(points=points, sweeps=[], timestamp=None)
+ results = load_points_from_multi_sweeps_1(input_results)
+ assert results['points'].tensor.numpy().shape == (100, 5)
+
+ input_results = dict(points=points, sweeps=[], timestamp=None)
+ results = load_points_from_multi_sweeps_2(input_results)
+ assert results['points'].tensor.numpy().shape == (775, 5)
+
+ sensor2lidar_rotation = np.array(
+ [[9.99999967e-01, 1.13183067e-05, 2.56845368e-04],
+ [-1.12839618e-05, 9.99999991e-01, -1.33719456e-04],
+ [-2.56846879e-04, 1.33716553e-04, 9.99999958e-01]])
+ sensor2lidar_translation = np.array([-0.0009198, -0.03964854, -0.00190136])
+ sweep = dict(
+ data_path='tests/data/nuscenes/sweeps/LIDAR_TOP/'
+ 'n008-2018-09-18-12-07-26-0400__LIDAR_TOP__'
+ '1537287083900561.pcd.bin',
+ sensor2lidar_rotation=sensor2lidar_rotation,
+ sensor2lidar_translation=sensor2lidar_translation,
+ timestamp=0)
+
+ input_results = dict(points=points, sweeps=[sweep], timestamp=1.0)
+ results = load_points_from_multi_sweeps_1(input_results)
+ assert results['points'].tensor.numpy().shape == (500, 5)
+
+ input_results = dict(points=points, sweeps=[sweep], timestamp=1.0)
+ results = load_points_from_multi_sweeps_2(input_results)
+ assert results['points'].tensor.numpy().shape == (451, 5)
+
+ input_results = dict(points=points, sweeps=[sweep] * 10, timestamp=1.0)
+ results = load_points_from_multi_sweeps_2(input_results)
+ assert results['points'].tensor.numpy().shape == (3259, 5)
+
+ input_results = dict(points=points, sweeps=[sweep] * 10, timestamp=1.0)
+ results = load_points_from_multi_sweeps_3(input_results)
+ assert results['points'].tensor.numpy().shape == (3259, 5)
diff --git a/tests/test_data/test_pipelines/test_loadings/test_loading.py b/tests/test_data/test_pipelines/test_loadings/test_loading.py
new file mode 100644
index 0000000..396c1df
--- /dev/null
+++ b/tests/test_data/test_pipelines/test_loadings/test_loading.py
@@ -0,0 +1,376 @@
+# Copyright (c) OpenMMLab. All rights reserved.
+from os import path as osp
+
+import mmcv
+import numpy as np
+import pytest
+
+from mmdet3d.core.bbox import DepthInstance3DBoxes
+from mmdet3d.core.points import DepthPoints, LiDARPoints
+# yapf: disable
+from mmdet3d.datasets.pipelines import (LoadAnnotations3D,
+ LoadImageFromFileMono3D,
+ LoadPointsFromFile,
+ LoadPointsFromMultiSweeps,
+ NormalizePointsColor,
+ PointSegClassMapping)
+
+# yapf: enable
+
+
+def test_load_points_from_indoor_file():
+ # test on SUN RGB-D dataset with shifted height
+ sunrgbd_info = mmcv.load('./tests/data/sunrgbd/sunrgbd_infos.pkl')
+ sunrgbd_load_points_from_file = LoadPointsFromFile(
+ coord_type='DEPTH', load_dim=6, shift_height=True)
+ sunrgbd_results = dict()
+ data_path = './tests/data/sunrgbd'
+ sunrgbd_info = sunrgbd_info[0]
+ sunrgbd_results['pts_filename'] = osp.join(data_path,
+ sunrgbd_info['pts_path'])
+ sunrgbd_results = sunrgbd_load_points_from_file(sunrgbd_results)
+ sunrgbd_point_cloud = sunrgbd_results['points'].tensor.numpy()
+ assert sunrgbd_point_cloud.shape == (100, 4)
+
+ scannet_info = mmcv.load('./tests/data/scannet/scannet_infos.pkl')
+ scannet_load_data = LoadPointsFromFile(
+ coord_type='DEPTH', shift_height=True)
+ scannet_results = dict()
+ data_path = './tests/data/scannet'
+ scannet_info = scannet_info[0]
+
+ # test on ScanNet dataset with shifted height
+ scannet_results['pts_filename'] = osp.join(data_path,
+ scannet_info['pts_path'])
+ scannet_results = scannet_load_data(scannet_results)
+ scannet_point_cloud = scannet_results['points'].tensor.numpy()
+ repr_str = repr(scannet_load_data)
+ expected_repr_str = 'LoadPointsFromFile(shift_height=True, ' \
+ 'use_color=False, ' \
+ 'file_client_args={\'backend\': \'disk\'}, ' \
+ 'load_dim=6, use_dim=[0, 1, 2])'
+ assert repr_str == expected_repr_str
+ assert scannet_point_cloud.shape == (100, 4)
+
+ # test load point cloud with both shifted height and color
+ scannet_load_data = LoadPointsFromFile(
+ coord_type='DEPTH',
+ load_dim=6,
+ use_dim=[0, 1, 2, 3, 4, 5],
+ shift_height=True,
+ use_color=True)
+
+ scannet_results = dict()
+
+ scannet_results['pts_filename'] = osp.join(data_path,
+ scannet_info['pts_path'])
+ scannet_results = scannet_load_data(scannet_results)
+ scannet_point_cloud = scannet_results['points']
+ assert scannet_point_cloud.points_dim == 7
+ assert scannet_point_cloud.attribute_dims == dict(
+ height=3, color=[4, 5, 6])
+
+ scannet_point_cloud = scannet_point_cloud.tensor.numpy()
+ assert scannet_point_cloud.shape == (100, 7)
+
+ # test load point cloud on S3DIS with color
+ data_path = './tests/data/s3dis'
+ s3dis_info = mmcv.load('./tests/data/s3dis/s3dis_infos.pkl')
+ s3dis_info = s3dis_info[0]
+ s3dis_load_data = LoadPointsFromFile(
+ coord_type='DEPTH',
+ load_dim=6,
+ use_dim=[0, 1, 2, 3, 4, 5],
+ shift_height=False,
+ use_color=True)
+
+ s3dis_results = dict()
+
+ s3dis_results['pts_filename'] = osp.join(data_path, s3dis_info['pts_path'])
+ s3dis_results = s3dis_load_data(s3dis_results)
+ s3dis_point_cloud = s3dis_results['points']
+ assert s3dis_point_cloud.points_dim == 6
+ assert s3dis_point_cloud.attribute_dims == dict(color=[3, 4, 5])
+
+ s3dis_point_cloud = s3dis_point_cloud.tensor.numpy()
+ assert s3dis_point_cloud.shape == (100, 6)
+
+
+def test_load_points_from_outdoor_file():
+ data_path = 'tests/data/kitti/a.bin'
+ load_points_from_file = LoadPointsFromFile(
+ coord_type='LIDAR', load_dim=4, use_dim=4)
+ results = dict()
+ results['pts_filename'] = data_path
+ results = load_points_from_file(results)
+ points = results['points'].tensor.numpy()
+ assert points.shape == (50, 4)
+ assert np.allclose(points.sum(), 2637.479)
+
+ load_points_from_file = LoadPointsFromFile(
+ coord_type='LIDAR', load_dim=4, use_dim=[0, 1, 2, 3])
+ results = dict()
+ results['pts_filename'] = data_path
+ results = load_points_from_file(results)
+ new_points = results['points'].tensor.numpy()
+ assert new_points.shape == (50, 4)
+ assert np.allclose(points.sum(), 2637.479)
+ np.equal(points, new_points)
+
+ with pytest.raises(AssertionError):
+ LoadPointsFromFile(coord_type='LIDAR', load_dim=4, use_dim=5)
+
+
+def test_load_annotations3D():
+ # Test scannet LoadAnnotations3D
+ scannet_info = mmcv.load('./tests/data/scannet/scannet_infos.pkl')[0]
+ scannet_load_annotations3D = LoadAnnotations3D(
+ with_bbox_3d=True,
+ with_label_3d=True,
+ with_mask_3d=True,
+ with_seg_3d=True)
+ scannet_results = dict()
+ data_path = './tests/data/scannet'
+
+ if scannet_info['annos']['gt_num'] != 0:
+ scannet_gt_bboxes_3d = scannet_info['annos']['gt_boxes_upright_depth']
+ scannet_gt_labels_3d = scannet_info['annos']['class']
+ else:
+ scannet_gt_bboxes_3d = np.zeros((1, 6), dtype=np.float32)
+ scannet_gt_labels_3d = np.zeros((1, ))
+
+ # prepare input of loading pipeline
+ scannet_results['ann_info'] = dict()
+ scannet_results['ann_info']['pts_instance_mask_path'] = osp.join(
+ data_path, scannet_info['pts_instance_mask_path'])
+ scannet_results['ann_info']['pts_semantic_mask_path'] = osp.join(
+ data_path, scannet_info['pts_semantic_mask_path'])
+ scannet_results['ann_info']['gt_bboxes_3d'] = DepthInstance3DBoxes(
+ scannet_gt_bboxes_3d, box_dim=6, with_yaw=False)
+ scannet_results['ann_info']['gt_labels_3d'] = scannet_gt_labels_3d
+
+ scannet_results['bbox3d_fields'] = []
+ scannet_results['pts_mask_fields'] = []
+ scannet_results['pts_seg_fields'] = []
+
+ scannet_results = scannet_load_annotations3D(scannet_results)
+ scannet_gt_boxes = scannet_results['gt_bboxes_3d']
+ scannet_gt_labels = scannet_results['gt_labels_3d']
+
+ scannet_pts_instance_mask = scannet_results['pts_instance_mask']
+ scannet_pts_semantic_mask = scannet_results['pts_semantic_mask']
+ repr_str = repr(scannet_load_annotations3D)
+ expected_repr_str = 'LoadAnnotations3D(\n with_bbox_3d=True, ' \
+ 'with_label_3d=True, with_attr_label=False, ' \
+ 'with_mask_3d=True, with_seg_3d=True, ' \
+ 'with_bbox=False, with_label=False, ' \
+ 'with_mask=False, with_seg=False, ' \
+ 'with_bbox_depth=False, poly2mask=True)'
+ assert repr_str == expected_repr_str
+ assert scannet_gt_boxes.tensor.shape == (27, 7)
+ assert scannet_gt_labels.shape == (27, )
+ assert scannet_pts_instance_mask.shape == (100, )
+ assert scannet_pts_semantic_mask.shape == (100, )
+
+ # Test s3dis LoadAnnotations3D
+ s3dis_info = mmcv.load('./tests/data/s3dis/s3dis_infos.pkl')[0]
+ s3dis_load_annotations3D = LoadAnnotations3D(
+ with_bbox_3d=False,
+ with_label_3d=False,
+ with_mask_3d=True,
+ with_seg_3d=True)
+ s3dis_results = dict()
+ data_path = './tests/data/s3dis'
+
+ # prepare input of loading pipeline
+ s3dis_results['ann_info'] = dict()
+ s3dis_results['ann_info']['pts_instance_mask_path'] = osp.join(
+ data_path, s3dis_info['pts_instance_mask_path'])
+ s3dis_results['ann_info']['pts_semantic_mask_path'] = osp.join(
+ data_path, s3dis_info['pts_semantic_mask_path'])
+
+ s3dis_results['pts_mask_fields'] = []
+ s3dis_results['pts_seg_fields'] = []
+
+ s3dis_results = s3dis_load_annotations3D(s3dis_results)
+
+ s3dis_pts_instance_mask = s3dis_results['pts_instance_mask']
+ s3dis_pts_semantic_mask = s3dis_results['pts_semantic_mask']
+ assert s3dis_pts_instance_mask.shape == (100, )
+ assert s3dis_pts_semantic_mask.shape == (100, )
+
+
+def test_load_segmentation_mask():
+ # Test loading semantic segmentation mask on ScanNet dataset
+ scannet_info = mmcv.load('./tests/data/scannet/scannet_infos.pkl')[0]
+ scannet_load_annotations3D = LoadAnnotations3D(
+ with_bbox_3d=False,
+ with_label_3d=False,
+ with_mask_3d=False,
+ with_seg_3d=True)
+ scannet_results = dict()
+ data_path = './tests/data/scannet'
+
+ # prepare input of loading pipeline
+ scannet_results['ann_info'] = dict()
+ scannet_results['ann_info']['pts_semantic_mask_path'] = osp.join(
+ data_path, scannet_info['pts_semantic_mask_path'])
+ scannet_results['pts_seg_fields'] = []
+
+ scannet_results = scannet_load_annotations3D(scannet_results)
+ scannet_pts_semantic_mask = scannet_results['pts_semantic_mask']
+ assert scannet_pts_semantic_mask.shape == (100, )
+
+ # Convert class_id to label and assign ignore_index
+ scannet_seg_class_mapping = \
+ PointSegClassMapping((1, 2, 3, 4, 5, 6, 7, 8, 9, 10, 11, 12, 14, 16,
+ 24, 28, 33, 34, 36, 39), 40)
+ scannet_results = scannet_seg_class_mapping(scannet_results)
+ scannet_pts_semantic_mask = scannet_results['pts_semantic_mask']
+
+ assert np.all(scannet_pts_semantic_mask == np.array([
+ 13, 20, 1, 2, 6, 2, 13, 1, 13, 2, 0, 20, 5, 20, 2, 0, 1, 13, 0, 0, 0,
+ 20, 6, 20, 13, 20, 2, 20, 20, 2, 16, 5, 13, 5, 13, 0, 20, 0, 0, 1, 7,
+ 20, 20, 20, 20, 20, 20, 20, 0, 1, 2, 13, 16, 1, 1, 1, 6, 2, 12, 20, 3,
+ 20, 20, 14, 1, 20, 2, 1, 7, 2, 0, 5, 20, 5, 20, 20, 3, 6, 5, 20, 0, 13,
+ 12, 2, 20, 0, 0, 13, 20, 1, 20, 5, 3, 0, 13, 1, 2, 2, 2, 1
+ ]))
+
+ # Test on S3DIS dataset
+ s3dis_info = mmcv.load('./tests/data/s3dis/s3dis_infos.pkl')[0]
+ s3dis_load_annotations3D = LoadAnnotations3D(
+ with_bbox_3d=False,
+ with_label_3d=False,
+ with_mask_3d=False,
+ with_seg_3d=True)
+ s3dis_results = dict()
+ data_path = './tests/data/s3dis'
+
+ # prepare input of loading pipeline
+ s3dis_results['ann_info'] = dict()
+ s3dis_results['ann_info']['pts_semantic_mask_path'] = osp.join(
+ data_path, s3dis_info['pts_semantic_mask_path'])
+ s3dis_results['pts_seg_fields'] = []
+
+ s3dis_results = s3dis_load_annotations3D(s3dis_results)
+ s3dis_pts_semantic_mask = s3dis_results['pts_semantic_mask']
+ assert s3dis_pts_semantic_mask.shape == (100, )
+
+ # Convert class_id to label and assign ignore_index
+ s3dis_seg_class_mapping = PointSegClassMapping(tuple(range(13)), 13)
+ s3dis_results = s3dis_seg_class_mapping(s3dis_results)
+ s3dis_pts_semantic_mask = s3dis_results['pts_semantic_mask']
+
+ assert np.all(s3dis_pts_semantic_mask == np.array([
+ 2, 2, 1, 2, 2, 5, 1, 0, 1, 1, 9, 12, 3, 0, 2, 0, 2, 0, 8, 2, 0, 2, 0,
+ 2, 1, 7, 2, 10, 2, 0, 0, 0, 2, 2, 2, 2, 2, 1, 2, 2, 0, 0, 4, 6, 7, 2,
+ 1, 2, 0, 1, 7, 0, 2, 2, 2, 0, 2, 2, 1, 12, 0, 2, 2, 2, 2, 7, 2, 2, 0,
+ 2, 6, 2, 12, 6, 2, 12, 2, 1, 6, 1, 2, 6, 8, 2, 10, 1, 10, 0, 6, 9, 4,
+ 3, 0, 0, 12, 1, 1, 5, 2, 2
+ ]))
+
+
+def test_load_points_from_multi_sweeps():
+ load_points_from_multi_sweeps = LoadPointsFromMultiSweeps()
+ sweep = dict(
+ data_path='./tests/data/nuscenes/sweeps/LIDAR_TOP/'
+ 'n008-2018-09-18-12-07-26-0400__LIDAR_TOP__1537287083900561.pcd.bin',
+ timestamp=1537290014899034,
+ sensor2lidar_translation=[-0.02344713, -3.88266051, -0.17151584],
+ sensor2lidar_rotation=np.array(
+ [[9.99979347e-01, 3.99870769e-04, 6.41441690e-03],
+ [-4.42034222e-04, 9.99978299e-01, 6.57316197e-03],
+ [-6.41164929e-03, -6.57586161e-03, 9.99957824e-01]]))
+ points = LiDARPoints(
+ np.array([[1., 2., 3., 4., 5.], [1., 2., 3., 4., 5.],
+ [1., 2., 3., 4., 5.]]),
+ points_dim=5)
+ results = dict(points=points, timestamp=1537290014899034, sweeps=[sweep])
+
+ results = load_points_from_multi_sweeps(results)
+ points = results['points'].tensor.numpy()
+ repr_str = repr(load_points_from_multi_sweeps)
+ expected_repr_str = 'LoadPointsFromMultiSweeps(sweeps_num=10)'
+ assert repr_str == expected_repr_str
+ assert points.shape == (403, 4)
+
+
+def test_load_image_from_file_mono_3d():
+ load_image_from_file_mono_3d = LoadImageFromFileMono3D()
+ filename = 'tests/data/nuscenes/samples/CAM_BACK_LEFT/' \
+ 'n015-2018-07-18-11-07-57+0800__CAM_BACK_LEFT__1531883530447423.jpg'
+ cam_intrinsic = np.array([[1256.74, 0.0, 792.11], [0.0, 1256.74, 492.78],
+ [0.0, 0.0, 1.0]])
+ input_dict = dict(
+ img_prefix=None,
+ img_info=dict(filename=filename, cam_intrinsic=cam_intrinsic.copy()))
+ results = load_image_from_file_mono_3d(input_dict)
+ assert results['img'].shape == (900, 1600, 3)
+ assert np.all(results['cam2img'] == cam_intrinsic)
+
+ repr_str = repr(load_image_from_file_mono_3d)
+ expected_repr_str = 'LoadImageFromFileMono3D(to_float32=False, ' \
+ "color_type='color', channel_order='bgr', " \
+ "file_client_args={'backend': 'disk'})"
+ assert repr_str == expected_repr_str
+
+
+def test_point_seg_class_mapping():
+ # max_cat_id should larger tham max id in valid_cat_ids
+ with pytest.raises(AssertionError):
+ point_seg_class_mapping = PointSegClassMapping([1, 2, 5], 4)
+
+ sem_mask = np.array([
+ 16, 22, 2, 3, 7, 3, 16, 2, 16, 3, 1, 0, 6, 22, 3, 1, 2, 16, 1, 1, 1,
+ 38, 7, 25, 16, 25, 3, 40, 38, 3, 33, 6, 16, 6, 16, 1, 38, 1, 1, 2, 8,
+ 0, 18, 15, 0, 0, 40, 40, 1, 2, 3, 16, 33, 2, 2, 2, 7, 3, 14, 22, 4, 22,
+ 15, 24, 2, 40, 3, 2, 8, 3, 1, 6, 40, 6, 0, 15, 4, 7, 6, 0, 1, 16, 14,
+ 3, 0, 1, 1, 16, 38, 2, 15, 6, 4, 1, 16, 2, 3, 3, 3, 2
+ ])
+ valid_cat_ids = (1, 2, 3, 4, 5, 6, 7, 8, 9, 10, 11, 12, 14, 16, 24, 28, 33,
+ 34, 36, 39)
+ point_seg_class_mapping = PointSegClassMapping(valid_cat_ids, 40)
+ input_dict = dict(pts_semantic_mask=sem_mask)
+ results = point_seg_class_mapping(input_dict)
+ mapped_sem_mask = results['pts_semantic_mask']
+ expected_sem_mask = np.array([
+ 13, 20, 1, 2, 6, 2, 13, 1, 13, 2, 0, 20, 5, 20, 2, 0, 1, 13, 0, 0, 0,
+ 20, 6, 20, 13, 20, 2, 20, 20, 2, 16, 5, 13, 5, 13, 0, 20, 0, 0, 1, 7,
+ 20, 20, 20, 20, 20, 20, 20, 0, 1, 2, 13, 16, 1, 1, 1, 6, 2, 12, 20, 3,
+ 20, 20, 14, 1, 20, 2, 1, 7, 2, 0, 5, 20, 5, 20, 20, 3, 6, 5, 20, 0, 13,
+ 12, 2, 20, 0, 0, 13, 20, 1, 20, 5, 3, 0, 13, 1, 2, 2, 2, 1
+ ])
+ repr_str = repr(point_seg_class_mapping)
+ expected_repr_str = f'PointSegClassMapping(valid_cat_ids={valid_cat_ids}'\
+ ', max_cat_id=40)'
+
+ assert np.all(mapped_sem_mask == expected_sem_mask)
+ assert repr_str == expected_repr_str
+
+
+def test_normalize_points_color():
+ coord = np.array([[68.137, 3.358, 2.516], [67.697, 3.55, 2.501],
+ [67.649, 3.76, 2.5], [66.414, 3.901, 2.459],
+ [66.012, 4.085, 2.446], [65.834, 4.178, 2.44],
+ [65.841, 4.386, 2.44], [65.745, 4.587, 2.438],
+ [65.551, 4.78, 2.432], [65.486, 4.982, 2.43]])
+ color = np.array([[131, 95, 138], [71, 185, 253], [169, 47, 41],
+ [174, 161, 88], [6, 158, 213], [6, 86, 78],
+ [118, 161, 78], [72, 195, 138], [180, 170, 32],
+ [197, 85, 27]])
+ points = np.concatenate([coord, color], axis=1)
+ points = DepthPoints(
+ points, points_dim=6, attribute_dims=dict(color=[3, 4, 5]))
+ input_dict = dict(points=points)
+
+ color_mean = [100, 150, 200]
+ points_color_normalizer = NormalizePointsColor(color_mean=color_mean)
+ input_dict = points_color_normalizer(input_dict)
+ points = input_dict['points']
+ repr_str = repr(points_color_normalizer)
+ expected_repr_str = f'NormalizePointsColor(color_mean={color_mean})'
+
+ assert repr_str == expected_repr_str
+ assert np.allclose(points.coord, coord)
+ assert np.allclose(points.color,
+ (color - np.array(color_mean)[None, :]) / 255.0)
diff --git a/tests/test_data/test_pipelines/test_outdoor_pipeline.py b/tests/test_data/test_pipelines/test_outdoor_pipeline.py
new file mode 100644
index 0000000..9d5a591
--- /dev/null
+++ b/tests/test_data/test_pipelines/test_outdoor_pipeline.py
@@ -0,0 +1,309 @@
+# Copyright (c) OpenMMLab. All rights reserved.
+import numpy as np
+import torch
+
+from mmdet3d.core.bbox import LiDARInstance3DBoxes
+from mmdet3d.datasets.pipelines import Compose
+
+
+def test_outdoor_aug_pipeline():
+ point_cloud_range = [0, -40, -3, 70.4, 40, 1]
+ class_names = ['Car']
+ np.random.seed(0)
+
+ train_pipeline = [
+ dict(
+ type='LoadPointsFromFile',
+ coord_type='LIDAR',
+ load_dim=4,
+ use_dim=4),
+ dict(type='LoadAnnotations3D', with_bbox_3d=True, with_label_3d=True),
+ dict(
+ type='ObjectNoise',
+ num_try=100,
+ translation_std=[1.0, 1.0, 0.5],
+ global_rot_range=[0.0, 0.0],
+ rot_range=[-0.78539816, 0.78539816]),
+ dict(type='RandomFlip3D', flip_ratio_bev_horizontal=0.5),
+ dict(
+ type='GlobalRotScaleTrans',
+ rot_range=[-0.78539816, 0.78539816],
+ scale_ratio_range=[0.95, 1.05]),
+ dict(type='PointsRangeFilter', point_cloud_range=point_cloud_range),
+ dict(type='ObjectRangeFilter', point_cloud_range=point_cloud_range),
+ dict(type='PointShuffle'),
+ dict(type='DefaultFormatBundle3D', class_names=class_names),
+ dict(
+ type='Collect3D', keys=['points', 'gt_bboxes_3d', 'gt_labels_3d'])
+ ]
+ pipeline = Compose(train_pipeline)
+
+ # coord sys refactor: reverse sign of yaw
+ gt_bboxes_3d = LiDARInstance3DBoxes(
+ torch.tensor([
+ [
+ 2.16902428e+01, -4.06038128e-02, -1.61906636e+00,
+ 1.65999997e+00, 3.20000005e+00, 1.61000001e+00, 1.53999996e+00
+ ],
+ [
+ 7.05006886e+00, -6.57459593e+00, -1.60107934e+00,
+ 2.27999997e+00, 1.27799997e+01, 3.66000009e+00, -1.54999995e+00
+ ],
+ [
+ 2.24698811e+01, -6.69203758e+00, -1.50118136e+00,
+ 2.31999993e+00, 1.47299995e+01, 3.64000010e+00, -1.59000003e+00
+ ],
+ [
+ 3.48291969e+01, -7.09058380e+00, -1.36622977e+00,
+ 2.31999993e+00, 1.00400000e+01, 3.60999990e+00, -1.61000001e+00
+ ],
+ [
+ 4.62394600e+01, -7.75838804e+00, -1.32405007e+00,
+ 2.33999991e+00, 1.28299999e+01, 3.63000011e+00, -1.63999999e+00
+ ],
+ [
+ 2.82966995e+01, -5.55755794e-01, -1.30332506e+00,
+ 1.47000003e+00, 2.23000002e+00, 1.48000002e+00, 1.57000005e+00
+ ],
+ [
+ 2.66690197e+01, 2.18230209e+01, -1.73605704e+00,
+ 1.55999994e+00, 3.48000002e+00, 1.39999998e+00, 1.69000006e+00
+ ],
+ [
+ 3.13197803e+01, 8.16214371e+00, -1.62177873e+00,
+ 1.74000001e+00, 3.76999998e+00, 1.48000002e+00, -2.78999996e+00
+ ],
+ [
+ 4.34395561e+01, -1.95209332e+01, -1.20757008e+00,
+ 1.69000006e+00, 4.09999990e+00, 1.40999997e+00, 1.53999996e+00
+ ],
+ [
+ 3.29882965e+01, -3.79360509e+00, -1.69245458e+00,
+ 1.74000001e+00, 4.09000015e+00, 1.49000001e+00, 1.52999997e+00
+ ],
+ [
+ 3.85469360e+01, 8.35060215e+00, -1.31423414e+00,
+ 1.59000003e+00, 4.28000021e+00, 1.45000005e+00, -1.73000002e+00
+ ],
+ [
+ 2.22492104e+01, -1.13536005e+01, -1.38272512e+00,
+ 1.62000000e+00, 3.55999994e+00, 1.71000004e+00, -2.48000002e+00
+ ],
+ [
+ 3.36115799e+01, -1.97708054e+01, -4.92827654e-01,
+ 1.64999998e+00, 3.54999995e+00, 1.79999995e+00, 1.57000005e+00
+ ],
+ [
+ 9.85029602e+00, -1.51294518e+00, -1.66834795e+00,
+ 1.59000003e+00, 3.17000008e+00, 1.38999999e+00, 8.39999974e-01
+ ]
+ ],
+ dtype=torch.float32))
+ gt_labels_3d = np.array([0, -1, -1, -1, -1, 0, 0, 0, 0, 0, 0, 0, 0, 0])
+ results = dict(
+ pts_filename='tests/data/kitti/a.bin',
+ ann_info=dict(gt_bboxes_3d=gt_bboxes_3d, gt_labels_3d=gt_labels_3d),
+ bbox3d_fields=[],
+ img_fields=[])
+
+ origin_center = gt_bboxes_3d.tensor[:, :3].clone()
+ origin_angle = gt_bboxes_3d.tensor[:, 6].clone()
+
+ output = pipeline(results)
+
+ # manually go through the pipeline
+ rotation_angle = output['img_metas']._data['pcd_rotation_angle']
+ rotation_matrix = output['img_metas']._data['pcd_rotation']
+ noise_angle = torch.tensor([
+ 0.70853819, -0.19160091, -0.71116999, 0.49571753, -0.12447527,
+ -0.4690133, -0.34776965, -0.65692282, -0.52442831, -0.01575567,
+ -0.61849673, 0.6572608, 0.30312288, -0.19182971
+ ])
+ noise_trans = torch.tensor([[1.7641e+00, 4.0016e-01, 4.8937e-01],
+ [-1.3065e+00, 1.6581e+00, -5.9082e-02],
+ [-1.5504e+00, 4.1732e-01, -4.7218e-01],
+ [-5.2158e-01, -1.1847e+00, 4.8035e-01],
+ [-8.9637e-01, -1.9627e+00, 7.9241e-01],
+ [1.3240e-02, -1.2194e-01, 1.6953e-01],
+ [8.1798e-01, -2.7891e-01, 7.1578e-01],
+ [-4.1733e-04, 3.7416e-01, 2.0478e-01],
+ [1.5218e-01, -3.7413e-01, -6.7257e-03],
+ [-1.9138e+00, -2.2855e+00, -8.0092e-01],
+ [1.5933e+00, 5.6872e-01, -5.7244e-02],
+ [-1.8523e+00, -7.1333e-01, -8.8111e-01],
+ [5.2678e-01, 1.0106e-01, -1.9432e-01],
+ [-7.2449e-01, -8.0292e-01, -1.1334e-02]])
+ angle = -origin_angle - noise_angle + torch.tensor(rotation_angle)
+ angle -= 2 * np.pi * (angle >= np.pi)
+ angle += 2 * np.pi * (angle < -np.pi)
+ scale = output['img_metas']._data['pcd_scale_factor']
+
+ expected_tensor = torch.tensor(
+ [[20.6514, -8.8250, -1.0816, 1.5893, 3.0637, 1.5414],
+ [7.9374, 4.9457, -1.2008, 2.1829, 12.2357, 3.5041],
+ [20.8115, -2.0273, -1.8893, 2.2212, 14.1026, 3.4850],
+ [32.3850, -5.2135, -1.1321, 2.2212, 9.6124, 3.4562],
+ [43.7022, -7.8316, -0.5090, 2.2403, 12.2836, 3.4754],
+ [25.3300, -9.6670, -1.0855, 1.4074, 2.1350, 1.4170],
+ [16.5414, -29.0583, -0.9768, 1.4936, 3.3318, 1.3404],
+ [24.6548, -18.9226, -1.3567, 1.6659, 3.6094, 1.4170],
+ [45.8403, 1.8183, -1.1626, 1.6180, 3.9254, 1.3499],
+ [30.6288, -8.4497, -1.4881, 1.6659, 3.9158, 1.4265],
+ [32.3316, -22.4611, -1.3131, 1.5223, 4.0977, 1.3882],
+ [22.4492, 3.2944, -2.1674, 1.5510, 3.4084, 1.6372],
+ [37.3824, 5.0472, -0.6579, 1.5797, 3.3988, 1.7233],
+ [8.9259, -1.2578, -1.6081, 1.5223, 3.0350, 1.3308]])
+
+ expected_tensor[:, :3] = ((
+ (origin_center + noise_trans) * torch.tensor([1, -1, 1]))
+ @ rotation_matrix) * scale
+
+ expected_tensor = torch.cat([expected_tensor, angle.unsqueeze(-1)], dim=-1)
+ assert torch.allclose(
+ output['gt_bboxes_3d']._data.tensor, expected_tensor, atol=1e-3)
+
+
+def test_outdoor_velocity_aug_pipeline():
+ point_cloud_range = [-50, -50, -5, 50, 50, 3]
+ class_names = [
+ 'car', 'truck', 'trailer', 'bus', 'construction_vehicle', 'bicycle',
+ 'motorcycle', 'pedestrian', 'traffic_cone', 'barrier'
+ ]
+ np.random.seed(0)
+
+ train_pipeline = [
+ dict(
+ type='LoadPointsFromFile',
+ coord_type='LIDAR',
+ load_dim=4,
+ use_dim=4),
+ dict(type='LoadAnnotations3D', with_bbox_3d=True, with_label_3d=True),
+ dict(
+ type='GlobalRotScaleTrans',
+ rot_range=[-0.3925, 0.3925],
+ scale_ratio_range=[0.95, 1.05],
+ translation_std=[0, 0, 0]),
+ dict(type='RandomFlip3D', flip_ratio_bev_horizontal=0.5),
+ dict(type='PointsRangeFilter', point_cloud_range=point_cloud_range),
+ dict(type='ObjectRangeFilter', point_cloud_range=point_cloud_range),
+ dict(type='PointShuffle'),
+ dict(type='DefaultFormatBundle3D', class_names=class_names),
+ dict(
+ type='Collect3D', keys=['points', 'gt_bboxes_3d', 'gt_labels_3d'])
+ ]
+ pipeline = Compose(train_pipeline)
+
+ gt_bboxes_3d = LiDARInstance3DBoxes(
+ torch.tensor(
+ [[
+ -5.2422e+00, 4.0021e+01, -4.7643e-01, 2.0620e+00, 4.4090e+00,
+ 1.5480e+00, -1.4880e+00, 8.5338e-03, 4.4934e-02
+ ],
+ [
+ -2.6675e+01, 5.5950e+00, -1.3053e+00, 3.4300e-01, 4.5800e-01,
+ 7.8200e-01, -4.6276e+00, -4.3284e-04, -1.8543e-03
+ ],
+ [
+ -5.8098e+00, 3.5409e+01, -6.6511e-01, 2.3960e+00, 3.9690e+00,
+ 1.7320e+00, -4.6520e+00, 0.0000e+00, 0.0000e+00
+ ],
+ [
+ -3.1309e+01, 1.0901e+00, -1.0561e+00, 1.9440e+00, 3.8570e+00,
+ 1.7230e+00, -2.8143e+00, -2.7606e-02, -8.0573e-02
+ ],
+ [
+ -4.5642e+01, 2.0136e+01, -2.4681e-02, 1.9870e+00, 4.4400e+00,
+ 1.9420e+00, 2.8336e-01, 0.0000e+00, 0.0000e+00
+ ],
+ [
+ -5.1617e+00, 1.8305e+01, -1.0879e+00, 2.3230e+00, 4.8510e+00,
+ 1.3710e+00, -1.5803e+00, 0.0000e+00, 0.0000e+00
+ ],
+ [
+ -2.5285e+01, 4.1442e+00, -1.2713e+00, 1.7550e+00, 1.9890e+00,
+ 2.2200e+00, -4.4900e+00, -3.1784e-02, -1.5291e-01
+ ],
+ [
+ -2.2611e+00, 1.9170e+01, -1.1452e+00, 9.1900e-01, 1.1230e+00,
+ 1.9310e+00, 4.7790e-02, 6.7684e-02, -1.7537e+00
+ ],
+ [
+ -6.5878e+01, 1.3500e+01, -2.2528e-01, 1.8200e+00, 3.8520e+00,
+ 1.5450e+00, -2.8757e+00, 0.0000e+00, 0.0000e+00
+ ],
+ [
+ -5.4490e+00, 2.8363e+01, -7.7275e-01, 2.2360e+00, 3.7540e+00,
+ 1.5590e+00, -4.6520e+00, -7.9736e-03, 7.7207e-03
+ ]],
+ dtype=torch.float32),
+ box_dim=9)
+
+ gt_labels_3d = np.array([0, 8, 0, 0, 0, 0, -1, 7, 0, 0])
+ results = dict(
+ pts_filename='tests/data/kitti/a.bin',
+ ann_info=dict(gt_bboxes_3d=gt_bboxes_3d, gt_labels_3d=gt_labels_3d),
+ bbox3d_fields=[],
+ img_fields=[])
+
+ origin_center = gt_bboxes_3d.tensor[:, :3].clone()
+ origin_angle = gt_bboxes_3d.tensor[:, 6].clone(
+ ) # TODO: ObjectNoise modifies tensor!!
+ origin_velo = gt_bboxes_3d.tensor[:, 7:9].clone()
+
+ output = pipeline(results)
+
+ expected_tensor = torch.tensor(
+ [[
+ -3.7849e+00, -4.1057e+01, -4.8668e-01, 2.1064e+00, 4.5039e+00,
+ 1.5813e+00, -1.6919e+00, 1.0469e-02, -4.5533e-02
+ ],
+ [
+ -2.7010e+01, -6.7551e+00, -1.3334e+00, 3.5038e-01, 4.6786e-01,
+ 7.9883e-01, 1.4477e+00, -5.1440e-04, 1.8758e-03
+ ],
+ [
+ -4.5448e+00, -3.6372e+01, -6.7942e-01, 2.4476e+00, 4.0544e+00,
+ 1.7693e+00, 1.4721e+00, 0.0000e+00, -0.0000e+00
+ ],
+ [
+ -3.1916e+01, -2.3379e+00, -1.0788e+00, 1.9858e+00, 3.9400e+00,
+ 1.7601e+00, -3.6564e-01, -3.1333e-02, 8.1166e-02
+ ],
+ [
+ -4.5802e+01, -2.2340e+01, -2.5213e-02, 2.0298e+00, 4.5355e+00,
+ 1.9838e+00, 2.8199e+00, 0.0000e+00, -0.0000e+00
+ ],
+ [
+ -4.5526e+00, -1.8887e+01, -1.1114e+00, 2.3730e+00, 4.9554e+00,
+ 1.4005e+00, -1.5997e+00, 0.0000e+00, -0.0000e+00
+ ],
+ [
+ -2.5648e+01, -5.2197e+00, -1.2987e+00, 1.7928e+00, 2.0318e+00,
+ 2.2678e+00, 1.3100e+00, -3.8428e-02, 1.5485e-01
+ ],
+ [
+ -1.5578e+00, -1.9657e+01, -1.1699e+00, 9.3878e-01, 1.1472e+00,
+ 1.9726e+00, 3.0555e+00, 4.5907e-04, 1.7928e+00
+ ],
+ [
+ -4.4522e+00, -2.9166e+01, -7.8938e-01, 2.2841e+00, 3.8348e+00,
+ 1.5925e+00, 1.4721e+00, -7.8371e-03, -8.1931e-03
+ ]])
+ # coord sys refactor (manually go through pipeline)
+ rotation_angle = output['img_metas']._data['pcd_rotation_angle']
+ rotation_matrix = output['img_metas']._data['pcd_rotation']
+ expected_tensor[:, :3] = ((origin_center @ rotation_matrix) *
+ output['img_metas']._data['pcd_scale_factor'] *
+ torch.tensor([1, -1, 1]))[[
+ 0, 1, 2, 3, 4, 5, 6, 7, 9
+ ]]
+ angle = -origin_angle - rotation_angle
+ angle -= 2 * np.pi * (angle >= np.pi)
+ angle += 2 * np.pi * (angle < -np.pi)
+ expected_tensor[:, 6:7] = angle.unsqueeze(-1)[[0, 1, 2, 3, 4, 5, 6, 7, 9]]
+ expected_tensor[:,
+ 7:9] = ((origin_velo @ rotation_matrix[:2, :2]) *
+ output['img_metas']._data['pcd_scale_factor'] *
+ torch.tensor([1, -1]))[[0, 1, 2, 3, 4, 5, 6, 7, 9]]
+ assert torch.allclose(
+ output['gt_bboxes_3d']._data.tensor, expected_tensor, atol=1e-3)
diff --git a/tests/test_metrics/test_indoor_eval.py b/tests/test_metrics/test_indoor_eval.py
new file mode 100644
index 0000000..2842c58
--- /dev/null
+++ b/tests/test_metrics/test_indoor_eval.py
@@ -0,0 +1,188 @@
+# Copyright (c) OpenMMLab. All rights reserved.
+import numpy as np
+import pytest
+import torch
+
+from mmdet3d.core.evaluation.indoor_eval import average_precision, indoor_eval
+
+
+def test_indoor_eval():
+ if not torch.cuda.is_available():
+ pytest.skip()
+ from mmdet3d.core.bbox.structures import Box3DMode, DepthInstance3DBoxes
+ det_infos = [{
+ 'labels_3d':
+ torch.tensor([0, 1, 2, 2, 0, 3, 1, 2, 3, 2]),
+ 'boxes_3d':
+ DepthInstance3DBoxes(
+ torch.tensor([[
+ -2.4089e-03, -3.3174e+00, 4.9438e-01, 2.1668e+00, 2.8431e-01,
+ 1.6506e+00, 0.0000e+00
+ ],
+ [
+ -3.4269e-01, -2.7565e+00, 2.8144e-02, 6.8554e-01,
+ 9.6854e-01, 6.1755e-01, 0.0000e+00
+ ],
+ [
+ -3.8320e+00, -1.0646e+00, 1.7074e-01, 2.4981e-01,
+ 4.4708e-01, 6.2538e-01, 0.0000e+00
+ ],
+ [
+ 4.1073e-01, 3.3757e+00, 3.4311e-01, 8.0617e-01,
+ 2.8679e-01, 1.6060e+00, 0.0000e+00
+ ],
+ [
+ 6.1199e-01, -3.1041e+00, 4.1873e-01, 1.2310e+00,
+ 4.0162e-01, 1.7303e+00, 0.0000e+00
+ ],
+ [
+ -5.9877e-01, -2.6011e+00, 1.1148e+00, 1.5704e-01,
+ 7.5957e-01, 9.6930e-01, 0.0000e+00
+ ],
+ [
+ 2.7462e-01, -3.0088e+00, 6.5231e-02, 8.1208e-01,
+ 4.1861e-01, 3.7339e-01, 0.0000e+00
+ ],
+ [
+ -1.4704e+00, -2.0024e+00, 2.7479e-01, 1.7888e+00,
+ 1.0566e+00, 1.3704e+00, 0.0000e+00
+ ],
+ [
+ 8.2727e-02, -3.1160e+00, 2.5690e-01, 1.4054e+00,
+ 2.0772e-01, 9.6792e-01, 0.0000e+00
+ ],
+ [
+ 2.6896e+00, 1.9881e+00, 1.1566e+00, 9.9885e-02,
+ 3.5713e-01, 4.5638e-01, 0.0000e+00
+ ]]),
+ origin=(0.5, 0.5, 0)),
+ 'scores_3d':
+ torch.tensor([
+ 1.7516e-05, 1.0167e-06, 8.4486e-07, 7.1048e-02, 6.4274e-05,
+ 1.5003e-07, 5.8102e-06, 1.9399e-08, 5.3126e-07, 1.8630e-09
+ ])
+ }]
+
+ label2cat = {
+ 0: 'cabinet',
+ 1: 'bed',
+ 2: 'chair',
+ 3: 'sofa',
+ }
+ gt_annos = [{
+ 'gt_num':
+ 10,
+ 'gt_boxes_upright_depth':
+ np.array([[
+ -2.4089e-03, -3.3174e+00, 4.9438e-01, 2.1668e+00, 2.8431e-01,
+ 1.6506e+00, 0.0000e+00
+ ],
+ [
+ -3.4269e-01, -2.7565e+00, 2.8144e-02, 6.8554e-01,
+ 9.6854e-01, 6.1755e-01, 0.0000e+00
+ ],
+ [
+ -3.8320e+00, -1.0646e+00, 1.7074e-01, 2.4981e-01,
+ 4.4708e-01, 6.2538e-01, 0.0000e+00
+ ],
+ [
+ 4.1073e-01, 3.3757e+00, 3.4311e-01, 8.0617e-01,
+ 2.8679e-01, 1.6060e+00, 0.0000e+00
+ ],
+ [
+ 6.1199e-01, -3.1041e+00, 4.1873e-01, 1.2310e+00,
+ 4.0162e-01, 1.7303e+00, 0.0000e+00
+ ],
+ [
+ -5.9877e-01, -2.6011e+00, 1.1148e+00, 1.5704e-01,
+ 7.5957e-01, 9.6930e-01, 0.0000e+00
+ ],
+ [
+ 2.7462e-01, -3.0088e+00, 6.5231e-02, 8.1208e-01,
+ 4.1861e-01, 3.7339e-01, 0.0000e+00
+ ],
+ [
+ -1.4704e+00, -2.0024e+00, 2.7479e-01, 1.7888e+00,
+ 1.0566e+00, 1.3704e+00, 0.0000e+00
+ ],
+ [
+ 8.2727e-02, -3.1160e+00, 2.5690e-01, 1.4054e+00,
+ 2.0772e-01, 9.6792e-01, 0.0000e+00
+ ],
+ [
+ 2.6896e+00, 1.9881e+00, 1.1566e+00, 9.9885e-02,
+ 3.5713e-01, 4.5638e-01, 0.0000e+00
+ ]]),
+ 'class':
+ np.array([0, 1, 2, 0, 0, 3, 1, 3, 3, 2])
+ }]
+
+ ret_value = indoor_eval(
+ gt_annos,
+ det_infos, [0.25, 0.5],
+ label2cat,
+ box_type_3d=DepthInstance3DBoxes,
+ box_mode_3d=Box3DMode.DEPTH)
+
+ assert np.isclose(ret_value['cabinet_AP_0.25'], 0.666667)
+ assert np.isclose(ret_value['bed_AP_0.25'], 1.0)
+ assert np.isclose(ret_value['chair_AP_0.25'], 0.5)
+ assert np.isclose(ret_value['mAP_0.25'], 0.708333)
+ assert np.isclose(ret_value['mAR_0.25'], 0.833333)
+
+
+def test_indoor_eval_less_classes():
+ if not torch.cuda.is_available():
+ pytest.skip()
+ from mmdet3d.core.bbox.structures import Box3DMode, DepthInstance3DBoxes
+ det_infos = [{
+ 'labels_3d':
+ torch.tensor([0]),
+ 'boxes_3d':
+ DepthInstance3DBoxes(torch.tensor([[1., 1., 1., 1., 1., 1., 1.]])),
+ 'scores_3d':
+ torch.tensor([.5])
+ }, {
+ 'labels_3d':
+ torch.tensor([1]),
+ 'boxes_3d':
+ DepthInstance3DBoxes(torch.tensor([[1., 1., 1., 1., 1., 1., 1.]])),
+ 'scores_3d':
+ torch.tensor([.5])
+ }]
+
+ label2cat = {0: 'cabinet', 1: 'bed', 2: 'chair'}
+ gt_annos = [{
+ 'gt_num':
+ 2,
+ 'gt_boxes_upright_depth':
+ np.array([[0., 0., 0., 1., 1., 1., 1.], [1., 1., 1., 1., 1., 1., 1.]]),
+ 'class':
+ np.array([2, 0])
+ }, {
+ 'gt_num':
+ 1,
+ 'gt_boxes_upright_depth':
+ np.array([
+ [1., 1., 1., 1., 1., 1., 1.],
+ ]),
+ 'class':
+ np.array([1])
+ }]
+
+ ret_value = indoor_eval(
+ gt_annos,
+ det_infos, [0.25, 0.5],
+ label2cat,
+ box_type_3d=DepthInstance3DBoxes,
+ box_mode_3d=Box3DMode.DEPTH)
+
+ assert np.isclose(ret_value['mAP_0.25'], 0.666667)
+ assert np.isclose(ret_value['mAR_0.25'], 0.666667)
+
+
+def test_average_precision():
+ ap = average_precision(
+ np.array([[0.25, 0.5, 0.75], [0.25, 0.5, 0.75]]),
+ np.array([[1., 1., 1.], [1., 1., 1.]]), '11points')
+ assert abs(ap[0] - 0.06611571) < 0.001
diff --git a/tests/test_metrics/test_instance_seg_eval.py b/tests/test_metrics/test_instance_seg_eval.py
new file mode 100644
index 0000000..a73483a
--- /dev/null
+++ b/tests/test_metrics/test_instance_seg_eval.py
@@ -0,0 +1,75 @@
+# Copyright (c) OpenMMLab. All rights reserved.
+import numpy as np
+import torch
+
+from mmdet3d.core import instance_seg_eval
+
+
+def test_instance_seg_eval():
+ valid_class_ids = (3, 4, 5, 6, 7, 8, 9, 10, 11, 12, 14, 16, 24, 28, 33, 34,
+ 36, 39)
+ class_labels = ('cabinet', 'bed', 'chair', 'sofa', 'table', 'door',
+ 'window', 'bookshelf', 'picture', 'counter', 'desk',
+ 'curtain', 'refrigerator', 'showercurtrain', 'toilet',
+ 'sink', 'bathtub', 'garbagebin')
+ n_points_list = [3300, 3000]
+ gt_labels_list = [[0, 0, 0, 0, 0, 0, 14, 14, 2, 1],
+ [13, 13, 2, 1, 3, 3, 0, 0, 0]]
+ gt_instance_masks = []
+ gt_semantic_masks = []
+ pred_instance_masks = []
+ pred_instance_labels = []
+ pred_instance_scores = []
+ for n_points, gt_labels in zip(n_points_list, gt_labels_list):
+ gt_instance_mask = np.ones(n_points, dtype=np.int) * -1
+ gt_semantic_mask = np.ones(n_points, dtype=np.int) * -1
+ pred_instance_mask = np.ones(n_points, dtype=np.int) * -1
+ labels = []
+ scores = []
+ for i, gt_label in enumerate(gt_labels):
+ begin = i * 300
+ end = begin + 300
+ gt_instance_mask[begin:end] = i
+ gt_semantic_mask[begin:end] = gt_label
+ pred_instance_mask[begin:end] = i
+ labels.append(gt_label)
+ scores.append(.99)
+ gt_instance_masks.append(torch.tensor(gt_instance_mask))
+ gt_semantic_masks.append(torch.tensor(gt_semantic_mask))
+ pred_instance_masks.append(torch.tensor(pred_instance_mask))
+ pred_instance_labels.append(torch.tensor(labels))
+ pred_instance_scores.append(torch.tensor(scores))
+
+ ret_value = instance_seg_eval(
+ gt_semantic_masks=gt_semantic_masks,
+ gt_instance_masks=gt_instance_masks,
+ pred_instance_masks=pred_instance_masks,
+ pred_instance_labels=pred_instance_labels,
+ pred_instance_scores=pred_instance_scores,
+ valid_class_ids=valid_class_ids,
+ class_labels=class_labels)
+ for label in [
+ 'cabinet', 'bed', 'chair', 'sofa', 'showercurtrain', 'toilet'
+ ]:
+ metrics = ret_value['classes'][label]
+ assert metrics['ap'] == 1.0
+ assert metrics['ap50%'] == 1.0
+ assert metrics['ap25%'] == 1.0
+
+ pred_instance_masks[1][2240:2700] = -1
+ pred_instance_masks[0][2700:3000] = 8
+ pred_instance_labels[0][9] = 2
+ ret_value = instance_seg_eval(
+ gt_semantic_masks=gt_semantic_masks,
+ gt_instance_masks=gt_instance_masks,
+ pred_instance_masks=pred_instance_masks,
+ pred_instance_labels=pred_instance_labels,
+ pred_instance_scores=pred_instance_scores,
+ valid_class_ids=valid_class_ids,
+ class_labels=class_labels)
+ assert abs(ret_value['classes']['cabinet']['ap50%'] - 0.72916) < 0.01
+ assert abs(ret_value['classes']['cabinet']['ap25%'] - 0.88888) < 0.01
+ assert abs(ret_value['classes']['bed']['ap50%'] - 0.5) < 0.01
+ assert abs(ret_value['classes']['bed']['ap25%'] - 0.5) < 0.01
+ assert abs(ret_value['classes']['chair']['ap50%'] - 0.375) < 0.01
+ assert abs(ret_value['classes']['chair']['ap25%'] - 1.0) < 0.01
diff --git a/tests/test_metrics/test_kitti_eval.py b/tests/test_metrics/test_kitti_eval.py
new file mode 100644
index 0000000..7447ceb
--- /dev/null
+++ b/tests/test_metrics/test_kitti_eval.py
@@ -0,0 +1,267 @@
+# Copyright (c) OpenMMLab. All rights reserved.
+import numpy as np
+import pytest
+import torch
+
+from mmdet3d.core.evaluation.kitti_utils.eval import (do_eval, eval_class,
+ kitti_eval)
+
+
+def test_do_eval():
+ if not torch.cuda.is_available():
+ pytest.skip('test requires GPU and CUDA')
+ gt_name = np.array(
+ ['Pedestrian', 'Cyclist', 'Car', 'Car', 'Car', 'DontCare', 'DontCare'])
+ gt_truncated = np.array([0., 0., 0., -1., -1., -1., -1.])
+ gt_occluded = np.array([0, 0, 3, -1, -1, -1, -1])
+ gt_alpha = np.array([-1.57, 1.85, -1.65, -10., -10., -10., -10.])
+ gt_bbox = np.array([[674.9179, 165.48549, 693.23694, 193.42134],
+ [676.21954, 165.70988, 691.63745, 193.83748],
+ [389.4093, 182.48041, 421.49072, 202.13422],
+ [232.0577, 186.16724, 301.94623, 217.4024],
+ [758.6537, 172.98509, 816.32434, 212.76743],
+ [532.37, 176.35, 542.68, 185.27],
+ [559.62, 175.83, 575.4, 183.15]])
+ gt_dimensions = np.array([[12.34, 2.85, 2.63], [3.69, 1.67, 1.87],
+ [2.02, 1.86, 0.6], [-1., -1., -1.],
+ [-1., -1., -1.], [-1., -1., -1.],
+ [-1., -1., -1.]])
+ gt_location = np.array([[4.700e-01, 1.490e+00, 6.944e+01],
+ [-1.653e+01, 2.390e+00, 5.849e+01],
+ [4.590e+00, 1.320e+00, 4.584e+01],
+ [-1.000e+03, -1.000e+03, -1.000e+03],
+ [-1.000e+03, -1.000e+03, -1.000e+03],
+ [-1.000e+03, -1.000e+03, -1.000e+03],
+ [-1.000e+03, -1.000e+03, -1.000e+03]])
+ gt_rotation_y = [-1.56, 1.57, -1.55, -10., -10., -10., -10.]
+ gt_anno = dict(
+ name=gt_name,
+ truncated=gt_truncated,
+ occluded=gt_occluded,
+ alpha=gt_alpha,
+ bbox=gt_bbox,
+ dimensions=gt_dimensions,
+ location=gt_location,
+ rotation_y=gt_rotation_y)
+
+ dt_name = np.array(['Pedestrian', 'Cyclist', 'Car', 'Car', 'Car'])
+ dt_truncated = np.array([0., 0., 0., 0., 0.])
+ dt_occluded = np.array([0, 0, 0, 0, 0])
+ dt_alpha = np.array([1.0744612, 1.2775835, 1.82563, 2.1145396, -1.7676563])
+ dt_dimensions = np.array([[1.4441837, 1.7450154, 0.53160036],
+ [1.6501029, 1.7540325, 0.5162356],
+ [3.9313498, 1.4899347, 1.5655756],
+ [4.0111866, 1.5350999, 1.585221],
+ [3.7337692, 1.5117968, 1.5515774]])
+ dt_location = np.array([[4.6671643, 1.285098, 45.836895],
+ [4.658241, 1.3088846, 45.85148],
+ [-16.598526, 2.298814, 58.618088],
+ [-18.629122, 2.2990575, 39.305355],
+ [7.0964046, 1.5178275, 29.32426]])
+ dt_rotation_y = np.array(
+ [1.174933, 1.3778262, 1.550529, 1.6742425, -1.5330327])
+ dt_bbox = np.array([[674.9179, 165.48549, 693.23694, 193.42134],
+ [676.21954, 165.70988, 691.63745, 193.83748],
+ [389.4093, 182.48041, 421.49072, 202.13422],
+ [232.0577, 186.16724, 301.94623, 217.4024],
+ [758.6537, 172.98509, 816.32434, 212.76743]])
+ dt_score = np.array(
+ [0.18151495, 0.57920843, 0.27795696, 0.23100418, 0.21541929])
+ dt_anno = dict(
+ name=dt_name,
+ truncated=dt_truncated,
+ occluded=dt_occluded,
+ alpha=dt_alpha,
+ bbox=dt_bbox,
+ dimensions=dt_dimensions,
+ location=dt_location,
+ rotation_y=dt_rotation_y,
+ score=dt_score)
+ current_classes = [1, 2, 0]
+ min_overlaps = np.array([[[0.5, 0.5, 0.7], [0.5, 0.5, 0.7],
+ [0.5, 0.5, 0.7]],
+ [[0.5, 0.5, 0.7], [0.25, 0.25, 0.5],
+ [0.25, 0.25, 0.5]]])
+ eval_types = ['bbox', 'bev', '3d', 'aos']
+ mAP11_bbox, mAP11_bev, mAP11_3d, mAP11_aos, mAP40_bbox,\
+ mAP40_bev, mAP40_3d, mAP40_aos = do_eval([gt_anno], [dt_anno],
+ current_classes, min_overlaps,
+ eval_types)
+ expected_mAP11_bbox = np.array([[[0., 0.], [9.09090909, 9.09090909],
+ [9.09090909, 9.09090909]],
+ [[0., 0.], [9.09090909, 9.09090909],
+ [9.09090909, 9.09090909]],
+ [[0., 0.], [9.09090909, 9.09090909],
+ [9.09090909, 9.09090909]]])
+ expected_mAP40_bbox = np.array([[[0., 0.], [0., 0.], [0., 0.]],
+ [[0., 0.], [0., 0.], [0., 0.]],
+ [[0., 0.], [2.5, 2.5], [2.5, 2.5]]])
+ expected_mAP11_bev = np.array([[[0., 0.], [0., 0.], [0., 0.]],
+ [[0., 0.], [0., 0.], [0., 0.]],
+ [[0., 0.], [0., 0.], [0., 0.]]])
+ expected_mAP40_bev = np.array([[[0., 0.], [0., 0.], [0., 0.]],
+ [[0., 0.], [0., 0.], [0., 0.]],
+ [[0., 0.], [0., 0.], [0., 0.]]])
+ expected_mAP11_3d = np.array([[[0., 0.], [0., 0.], [0., 0.]],
+ [[0., 0.], [0., 0.], [0., 0.]],
+ [[0., 0.], [0., 0.], [0., 0.]]])
+ expected_mAP40_3d = np.array([[[0., 0.], [0., 0.], [0., 0.]],
+ [[0., 0.], [0., 0.], [0., 0.]],
+ [[0., 0.], [0., 0.], [0., 0.]]])
+ expected_mAP11_aos = np.array([[[0., 0.], [0.55020816, 0.55020816],
+ [0.55020816, 0.55020816]],
+ [[0., 0.], [8.36633862, 8.36633862],
+ [8.36633862, 8.36633862]],
+ [[0., 0.], [8.63476893, 8.63476893],
+ [8.63476893, 8.63476893]]])
+ expected_mAP40_aos = np.array([[[0., 0.], [0., 0.], [0., 0.]],
+ [[0., 0.], [0., 0.], [0., 0.]],
+ [[0., 0.], [1.58140643, 1.58140643],
+ [1.58140643, 1.58140643]]])
+ assert np.allclose(mAP11_bbox, expected_mAP11_bbox)
+ assert np.allclose(mAP11_bev, expected_mAP11_bev)
+ assert np.allclose(mAP11_3d, expected_mAP11_3d)
+ assert np.allclose(mAP11_aos, expected_mAP11_aos)
+ assert np.allclose(mAP40_bbox, expected_mAP40_bbox)
+ assert np.allclose(mAP40_bev, expected_mAP40_bev)
+ assert np.allclose(mAP40_3d, expected_mAP40_3d)
+ assert np.allclose(mAP40_aos, expected_mAP40_aos)
+
+
+def test_kitti_eval():
+ if not torch.cuda.is_available():
+ pytest.skip('test requires GPU and CUDA')
+ gt_name = np.array(
+ ['Pedestrian', 'Cyclist', 'Car', 'Car', 'Car', 'DontCare', 'DontCare'])
+ gt_truncated = np.array([0., 0., 0., -1., -1., -1., -1.])
+ gt_occluded = np.array([0, 0, 3, -1, -1, -1, -1])
+ gt_alpha = np.array([-1.57, 1.85, -1.65, -10., -10., -10., -10.])
+ gt_bbox = np.array([[674.9179, 165.48549, 693.23694, 193.42134],
+ [676.21954, 165.70988, 691.63745, 193.83748],
+ [389.4093, 182.48041, 421.49072, 202.13422],
+ [232.0577, 186.16724, 301.94623, 217.4024],
+ [758.6537, 172.98509, 816.32434, 212.76743],
+ [532.37, 176.35, 542.68, 185.27],
+ [559.62, 175.83, 575.4, 183.15]])
+ gt_dimensions = np.array([[12.34, 2.85, 2.63], [3.69, 1.67, 1.87],
+ [2.02, 1.86, 0.6], [-1., -1., -1.],
+ [-1., -1., -1.], [-1., -1., -1.],
+ [-1., -1., -1.]])
+ gt_location = np.array([[4.700e-01, 1.490e+00, 6.944e+01],
+ [-1.653e+01, 2.390e+00, 5.849e+01],
+ [4.590e+00, 1.320e+00, 4.584e+01],
+ [-1.000e+03, -1.000e+03, -1.000e+03],
+ [-1.000e+03, -1.000e+03, -1.000e+03],
+ [-1.000e+03, -1.000e+03, -1.000e+03],
+ [-1.000e+03, -1.000e+03, -1.000e+03]])
+ gt_rotation_y = [-1.56, 1.57, -1.55, -10., -10., -10., -10.]
+ gt_anno = dict(
+ name=gt_name,
+ truncated=gt_truncated,
+ occluded=gt_occluded,
+ alpha=gt_alpha,
+ bbox=gt_bbox,
+ dimensions=gt_dimensions,
+ location=gt_location,
+ rotation_y=gt_rotation_y)
+
+ dt_name = np.array(['Pedestrian', 'Cyclist', 'Car', 'Car', 'Car'])
+ dt_truncated = np.array([0., 0., 0., 0., 0.])
+ dt_occluded = np.array([0, 0, 0, 0, 0])
+ dt_alpha = np.array([1.0744612, 1.2775835, 1.82563, 2.1145396, -1.7676563])
+ dt_dimensions = np.array([[1.4441837, 1.7450154, 0.53160036],
+ [1.6501029, 1.7540325, 0.5162356],
+ [3.9313498, 1.4899347, 1.5655756],
+ [4.0111866, 1.5350999, 1.585221],
+ [3.7337692, 1.5117968, 1.5515774]])
+ dt_location = np.array([[4.6671643, 1.285098, 45.836895],
+ [4.658241, 1.3088846, 45.85148],
+ [-16.598526, 2.298814, 58.618088],
+ [-18.629122, 2.2990575, 39.305355],
+ [7.0964046, 1.5178275, 29.32426]])
+ dt_rotation_y = np.array(
+ [1.174933, 1.3778262, 1.550529, 1.6742425, -1.5330327])
+ dt_bbox = np.array([[674.9179, 165.48549, 693.23694, 193.42134],
+ [676.21954, 165.70988, 691.63745, 193.83748],
+ [389.4093, 182.48041, 421.49072, 202.13422],
+ [232.0577, 186.16724, 301.94623, 217.4024],
+ [758.6537, 172.98509, 816.32434, 212.76743]])
+ dt_score = np.array(
+ [0.18151495, 0.57920843, 0.27795696, 0.23100418, 0.21541929])
+ dt_anno = dict(
+ name=dt_name,
+ truncated=dt_truncated,
+ occluded=dt_occluded,
+ alpha=dt_alpha,
+ bbox=dt_bbox,
+ dimensions=dt_dimensions,
+ location=dt_location,
+ rotation_y=dt_rotation_y,
+ score=dt_score)
+
+ current_classes = [1, 2, 0]
+ result, ret_dict = kitti_eval([gt_anno], [dt_anno], current_classes)
+ assert np.isclose(ret_dict['KITTI/Overall_2D_AP11_moderate'],
+ 9.090909090909092)
+ assert np.isclose(ret_dict['KITTI/Overall_2D_AP11_hard'],
+ 9.090909090909092)
+ assert np.isclose(ret_dict['KITTI/Overall_2D_AP40_moderate'],
+ 0.8333333333333334)
+ assert np.isclose(ret_dict['KITTI/Overall_2D_AP40_hard'],
+ 0.8333333333333334)
+
+
+def test_eval_class():
+ gt_name = np.array(
+ ['Pedestrian', 'Cyclist', 'Car', 'Car', 'Car', 'DontCare', 'DontCare'])
+ gt_truncated = np.array([0., 0., 0., -1., -1., -1., -1.])
+ gt_occluded = np.array([0, 0, 3, -1, -1, -1, -1])
+ gt_alpha = np.array([-1.57, 1.85, -1.65, -10., -10., -10., -10.])
+ gt_bbox = np.array([[674.9179, 165.48549, 693.23694, 193.42134],
+ [676.21954, 165.70988, 691.63745, 193.83748],
+ [389.4093, 182.48041, 421.49072, 202.13422],
+ [232.0577, 186.16724, 301.94623, 217.4024],
+ [758.6537, 172.98509, 816.32434, 212.76743],
+ [532.37, 176.35, 542.68, 185.27],
+ [559.62, 175.83, 575.4, 183.15]])
+ gt_anno = dict(
+ name=gt_name,
+ truncated=gt_truncated,
+ occluded=gt_occluded,
+ alpha=gt_alpha,
+ bbox=gt_bbox)
+
+ dt_name = np.array(['Pedestrian', 'Cyclist', 'Car', 'Car', 'Car'])
+ dt_truncated = np.array([0., 0., 0., 0., 0.])
+ dt_occluded = np.array([0, 0, 0, 0, 0])
+ dt_alpha = np.array([1.0744612, 1.2775835, 1.82563, 2.1145396, -1.7676563])
+ dt_bbox = np.array([[674.9179, 165.48549, 693.23694, 193.42134],
+ [676.21954, 165.70988, 691.63745, 193.83748],
+ [389.4093, 182.48041, 421.49072, 202.13422],
+ [232.0577, 186.16724, 301.94623, 217.4024],
+ [758.6537, 172.98509, 816.32434, 212.76743]])
+ dt_score = np.array(
+ [0.18151495, 0.57920843, 0.27795696, 0.23100418, 0.21541929])
+ dt_anno = dict(
+ name=dt_name,
+ truncated=dt_truncated,
+ occluded=dt_occluded,
+ alpha=dt_alpha,
+ bbox=dt_bbox,
+ score=dt_score)
+ current_classes = [1, 2, 0]
+ difficultys = [0, 1, 2]
+ metric = 0
+ min_overlaps = np.array([[[0.5, 0.5, 0.7], [0.5, 0.5, 0.7],
+ [0.5, 0.5, 0.7]],
+ [[0.5, 0.5, 0.7], [0.25, 0.25, 0.5],
+ [0.25, 0.25, 0.5]]])
+
+ ret_dict = eval_class([gt_anno], [dt_anno], current_classes, difficultys,
+ metric, min_overlaps, True, 1)
+ recall_sum = np.sum(ret_dict['recall'])
+ precision_sum = np.sum(ret_dict['precision'])
+ orientation_sum = np.sum(ret_dict['orientation'])
+ assert np.isclose(recall_sum, 16)
+ assert np.isclose(precision_sum, 16)
+ assert np.isclose(orientation_sum, 10.252829201850309)
diff --git a/tests/test_metrics/test_losses.py b/tests/test_metrics/test_losses.py
new file mode 100644
index 0000000..c7f128c
--- /dev/null
+++ b/tests/test_metrics/test_losses.py
@@ -0,0 +1,211 @@
+# Copyright (c) OpenMMLab. All rights reserved.
+import numpy as np
+import pytest
+import torch
+from torch import nn as nn
+
+from mmdet3d.models.builder import build_loss
+
+
+def test_chamfer_disrance():
+ from mmdet3d.models.losses import ChamferDistance, chamfer_distance
+
+ with pytest.raises(AssertionError):
+ # test invalid mode
+ ChamferDistance(mode='smoothl1')
+ # test invalid type of reduction
+ ChamferDistance(mode='l2', reduction=None)
+
+ self = ChamferDistance(
+ mode='l2', reduction='sum', loss_src_weight=1.0, loss_dst_weight=1.0)
+ source = torch.tensor([[[-0.9888, 0.9683, -0.8494],
+ [-6.4536, 4.5146,
+ 1.6861], [2.0482, 5.6936, -1.4701],
+ [-0.5173, 5.6472, 2.1748],
+ [-2.8010, 5.4423, -1.2158],
+ [2.4018, 2.4389, -0.2403],
+ [-2.8811, 3.8486, 1.4750],
+ [-0.2031, 3.8969,
+ -1.5245], [1.3827, 4.9295, 1.1537],
+ [-2.6961, 2.2621, -1.0976]],
+ [[0.3692, 1.8409,
+ -1.4983], [1.9995, 6.3602, 0.1798],
+ [-2.1317, 4.6011,
+ -0.7028], [2.4158, 3.1482, 0.3169],
+ [-0.5836, 3.6250, -1.2650],
+ [-1.9862, 1.6182, -1.4901],
+ [2.5992, 1.2847, -0.8471],
+ [-0.3467, 5.3681, -1.4755],
+ [-0.8576, 3.3400, -1.7399],
+ [2.7447, 4.6349, 0.1994]]])
+
+ target = torch.tensor([[[-0.4758, 1.0094, -0.8645],
+ [-0.3130, 0.8564, -0.9061],
+ [-0.1560, 2.0394, -0.8936],
+ [-0.3685, 1.6467, -0.8271],
+ [-0.2740, 2.2212, -0.7980]],
+ [[1.4856, 2.5299,
+ -1.0047], [2.3262, 3.3065, -0.9475],
+ [2.4593, 2.5870,
+ -0.9423], [0.0000, 0.0000, 0.0000],
+ [0.0000, 0.0000, 0.0000]]])
+
+ loss_source, loss_target, indices1, indices2 = self(
+ source, target, return_indices=True)
+
+ assert torch.allclose(loss_source, torch.tensor(219.5936))
+ assert torch.allclose(loss_target, torch.tensor(22.3705))
+
+ expected_inds1 = [[0, 4, 4, 4, 4, 2, 4, 4, 4, 3],
+ [0, 1, 0, 1, 0, 4, 2, 0, 0, 1]]
+ expected_inds2 = [[0, 4, 4, 4, 4, 2, 4, 4, 4, 3],
+ [0, 1, 0, 1, 0, 3, 2, 0, 0, 1]]
+ assert (torch.equal(indices1, indices1.new_tensor(expected_inds1))
+ or torch.equal(indices1, indices1.new_tensor(expected_inds2)))
+ assert torch.equal(indices2,
+ indices2.new_tensor([[0, 0, 0, 0, 0], [0, 3, 6, 0, 0]]))
+
+ loss_source, loss_target, indices1, indices2 = chamfer_distance(
+ source, target, reduction='sum')
+
+ assert torch.allclose(loss_source, torch.tensor(219.5936))
+ assert torch.allclose(loss_target, torch.tensor(22.3705))
+ assert (torch.equal(indices1, indices1.new_tensor(expected_inds1))
+ or torch.equal(indices1, indices1.new_tensor(expected_inds2)))
+ assert (indices2 == indices2.new_tensor([[0, 0, 0, 0, 0], [0, 3, 6, 0,
+ 0]])).all()
+
+
+def test_paconv_regularization_loss():
+ from mmdet3d.models.losses import PAConvRegularizationLoss
+ from mmdet3d.ops import PAConv, PAConvCUDA
+ from mmdet.apis import set_random_seed
+
+ class ToyModel(nn.Module):
+
+ def __init__(self):
+ super(ToyModel, self).__init__()
+
+ self.paconvs = nn.ModuleList()
+ self.paconvs.append(PAConv(8, 16, 8))
+ self.paconvs.append(PAConv(8, 16, 8, kernel_input='identity'))
+ self.paconvs.append(PAConvCUDA(8, 16, 8))
+
+ self.conv1 = nn.Conv1d(3, 8, 1)
+
+ set_random_seed(0, True)
+ model = ToyModel()
+
+ # reduction should be in ['none', 'mean', 'sum']
+ with pytest.raises(AssertionError):
+ paconv_corr_loss = PAConvRegularizationLoss(reduction='l2')
+
+ paconv_corr_loss = PAConvRegularizationLoss(reduction='mean')
+ mean_corr_loss = paconv_corr_loss(model.modules())
+ assert mean_corr_loss >= 0
+ assert mean_corr_loss.requires_grad
+
+ sum_corr_loss = paconv_corr_loss(model.modules(), reduction_override='sum')
+ assert torch.allclose(sum_corr_loss, mean_corr_loss * 3)
+
+ none_corr_loss = paconv_corr_loss(
+ model.modules(), reduction_override='none')
+ assert none_corr_loss.shape[0] == 3
+ assert torch.allclose(none_corr_loss.mean(), mean_corr_loss)
+
+
+def test_uncertain_smooth_l1_loss():
+ from mmdet3d.models.losses import UncertainL1Loss, UncertainSmoothL1Loss
+
+ # reduction should be in ['none', 'mean', 'sum']
+ with pytest.raises(AssertionError):
+ uncertain_l1_loss = UncertainL1Loss(reduction='l2')
+ with pytest.raises(AssertionError):
+ uncertain_smooth_l1_loss = UncertainSmoothL1Loss(reduction='l2')
+
+ pred = torch.tensor([1.5783, 0.5972, 1.4821, 0.9488])
+ target = torch.tensor([1.0813, -0.3466, -1.1404, -0.9665])
+ sigma = torch.tensor([-1.0053, 0.4710, -1.7784, -0.8603])
+
+ # test uncertain l1 loss
+ uncertain_l1_loss_cfg = dict(
+ type='UncertainL1Loss', alpha=1.0, reduction='mean', loss_weight=1.0)
+ uncertain_l1_loss = build_loss(uncertain_l1_loss_cfg)
+ mean_l1_loss = uncertain_l1_loss(pred, target, sigma)
+ expected_l1_loss = torch.tensor(4.7069)
+ assert torch.allclose(mean_l1_loss, expected_l1_loss, atol=1e-4)
+
+ # test uncertain smooth l1 loss
+ uncertain_smooth_l1_loss_cfg = dict(
+ type='UncertainSmoothL1Loss',
+ alpha=1.0,
+ beta=0.5,
+ reduction='mean',
+ loss_weight=1.0)
+ uncertain_smooth_l1_loss = build_loss(uncertain_smooth_l1_loss_cfg)
+ mean_smooth_l1_loss = uncertain_smooth_l1_loss(pred, target, sigma)
+ expected_smooth_l1_loss = torch.tensor(3.9795)
+ assert torch.allclose(
+ mean_smooth_l1_loss, expected_smooth_l1_loss, atol=1e-4)
+
+
+def test_multibin_loss():
+ from mmdet3d.models.losses import MultiBinLoss
+
+ # reduction should be in ['none', 'mean', 'sum']
+ with pytest.raises(AssertionError):
+ multibin_loss = MultiBinLoss(reduction='l2')
+
+ pred = torch.tensor([[
+ 0.81, 0.32, 0.78, 0.52, 0.24, 0.12, 0.32, 0.11, 1.20, 1.30, 0.20, 0.11,
+ 0.12, 0.11, 0.23, 0.31
+ ],
+ [
+ 0.02, 0.19, 0.78, 0.22, 0.31, 0.12, 0.22, 0.11,
+ 1.20, 1.30, 0.45, 0.51, 0.12, 0.11, 0.13, 0.61
+ ]])
+ target = torch.tensor([[1, 1, 0, 0, 2.14, 3.12, 0.68, -2.15],
+ [1, 1, 0, 0, 3.12, 3.12, 2.34, 1.23]])
+ multibin_loss_cfg = dict(
+ type='MultiBinLoss', reduction='none', loss_weight=1.0)
+ multibin_loss = build_loss(multibin_loss_cfg)
+ output_multibin_loss = multibin_loss(pred, target, num_dir_bins=4)
+ expected_multibin_loss = torch.tensor(2.1120)
+ assert torch.allclose(
+ output_multibin_loss, expected_multibin_loss, atol=1e-4)
+
+
+def test_axis_aligned_iou_loss():
+ from mmdet3d.models.losses import AxisAlignedIoULoss
+
+ boxes1 = torch.tensor([[0., 0., 0., 1., 1., 1.], [0., 0., 0., 1., 1., 1.],
+ [0., 0., 0., 1., 1., 1.]])
+ boxes2 = torch.tensor([[0., 0., 0., 1., 1.,
+ 1.], [.5, .5, .5, 1.5, 1.5, 1.5],
+ [1., 1., 1., 2., 2., 2.]])
+
+ expect_ious = torch.tensor([[0., 14 / 15, 1.]])
+ ious = AxisAlignedIoULoss(reduction='none')(boxes1, boxes2)
+ assert torch.allclose(ious, expect_ious, atol=1e-4)
+
+
+@pytest.mark.skipif(
+ not torch.cuda.is_available(), reason='requires CUDA support')
+def test_rotated_iou_3d_loss():
+ # adapted from mmcv.tests.test_ops.test_diff_iou_rotated
+ from mmdet3d.models.losses import RotatedIoU3DLoss
+
+ boxes1 = torch.tensor([[.5, .5, .5, 1., 1., 1., .0],
+ [.5, .5, .5, 1., 1., 1., .0],
+ [.5, .5, .5, 1., 1., 1., .0],
+ [.5, .5, .5, 1., 1., 1., .0],
+ [.5, .5, .5, 1., 1., 1., .0]]).cuda()
+ boxes2 = torch.tensor([[.5, .5, .5, 1., 1., 1., .0],
+ [.5, .5, .5, 1., 1., 2., np.pi / 2],
+ [.5, .5, .5, 1., 1., 1., np.pi / 4],
+ [1., 1., 1., 1., 1., 1., .0],
+ [-1.5, -1.5, -1.5, 2.5, 2.5, 2.5, .0]]).cuda()
+
+ expect_ious = 1 - torch.tensor([[1., .5, .7071, 1 / 15, .0]]).cuda()
+ ious = RotatedIoU3DLoss(reduction='none')(boxes1, boxes2)
+ assert torch.allclose(ious, expect_ious, atol=1e-4)
diff --git a/tests/test_metrics/test_seg_eval.py b/tests/test_metrics/test_seg_eval.py
new file mode 100644
index 0000000..193fc89
--- /dev/null
+++ b/tests/test_metrics/test_seg_eval.py
@@ -0,0 +1,39 @@
+# Copyright (c) OpenMMLab. All rights reserved.
+import numpy as np
+import pytest
+import torch
+
+from mmdet3d.core.evaluation.seg_eval import seg_eval
+
+
+def test_indoor_eval():
+ if not torch.cuda.is_available():
+ pytest.skip()
+ seg_preds = [
+ torch.Tensor([
+ 0, 0, 1, 0, 0, 2, 1, 3, 1, 2, 1, 0, 2, 2, 2, 2, 1, 3, 0, 3, 3, 3, 3
+ ])
+ ]
+ gt_labels = [
+ torch.Tensor([
+ 0, 0, 0, 255, 0, 0, 1, 1, 1, 255, 1, 1, 2, 2, 2, 2, 2, 3, 3, 3, 3,
+ 3, 255
+ ])
+ ]
+
+ label2cat = {
+ 0: 'car',
+ 1: 'bicycle',
+ 2: 'motorcycle',
+ 3: 'truck',
+ }
+ ret_value = seg_eval(gt_labels, seg_preds, label2cat, ignore_index=255)
+
+ assert np.isclose(ret_value['car'], 0.428571429)
+ assert np.isclose(ret_value['bicycle'], 0.428571429)
+ assert np.isclose(ret_value['motorcycle'], 0.6666667)
+ assert np.isclose(ret_value['truck'], 0.6666667)
+
+ assert np.isclose(ret_value['acc'], 0.7)
+ assert np.isclose(ret_value['acc_cls'], 0.7)
+ assert np.isclose(ret_value['miou'], 0.547619048)
diff --git a/tests/test_models/test_backbones.py b/tests/test_models/test_backbones.py
new file mode 100644
index 0000000..c755044
--- /dev/null
+++ b/tests/test_models/test_backbones.py
@@ -0,0 +1,407 @@
+# Copyright (c) OpenMMLab. All rights reserved.
+import numpy as np
+import pytest
+import torch
+
+from mmdet3d.models import build_backbone
+
+
+def test_pointnet2_sa_ssg():
+ if not torch.cuda.is_available():
+ pytest.skip()
+
+ cfg = dict(
+ type='PointNet2SASSG',
+ in_channels=6,
+ num_points=(32, 16),
+ radius=(0.8, 1.2),
+ num_samples=(16, 8),
+ sa_channels=((8, 16), (16, 16)),
+ fp_channels=((16, 16), (16, 16)))
+ self = build_backbone(cfg)
+ self.cuda()
+ assert self.SA_modules[0].mlps[0].layer0.conv.in_channels == 6
+ assert self.SA_modules[0].mlps[0].layer0.conv.out_channels == 8
+ assert self.SA_modules[0].mlps[0].layer1.conv.out_channels == 16
+ assert self.SA_modules[1].mlps[0].layer1.conv.out_channels == 16
+ assert self.FP_modules[0].mlps.layer0.conv.in_channels == 32
+ assert self.FP_modules[0].mlps.layer0.conv.out_channels == 16
+ assert self.FP_modules[1].mlps.layer0.conv.in_channels == 19
+
+ xyz = np.fromfile('tests/data/sunrgbd/points/000001.bin', dtype=np.float32)
+ xyz = torch.from_numpy(xyz).view(1, -1, 6).cuda() # (B, N, 6)
+ # test forward
+ ret_dict = self(xyz)
+ fp_xyz = ret_dict['fp_xyz']
+ fp_features = ret_dict['fp_features']
+ fp_indices = ret_dict['fp_indices']
+ sa_xyz = ret_dict['sa_xyz']
+ sa_features = ret_dict['sa_features']
+ sa_indices = ret_dict['sa_indices']
+ assert len(fp_xyz) == len(fp_features) == len(fp_indices) == 3
+ assert len(sa_xyz) == len(sa_features) == len(sa_indices) == 3
+ assert fp_xyz[0].shape == torch.Size([1, 16, 3])
+ assert fp_xyz[1].shape == torch.Size([1, 32, 3])
+ assert fp_xyz[2].shape == torch.Size([1, 100, 3])
+ assert fp_features[0].shape == torch.Size([1, 16, 16])
+ assert fp_features[1].shape == torch.Size([1, 16, 32])
+ assert fp_features[2].shape == torch.Size([1, 16, 100])
+ assert fp_indices[0].shape == torch.Size([1, 16])
+ assert fp_indices[1].shape == torch.Size([1, 32])
+ assert fp_indices[2].shape == torch.Size([1, 100])
+ assert sa_xyz[0].shape == torch.Size([1, 100, 3])
+ assert sa_xyz[1].shape == torch.Size([1, 32, 3])
+ assert sa_xyz[2].shape == torch.Size([1, 16, 3])
+ assert sa_features[0].shape == torch.Size([1, 3, 100])
+ assert sa_features[1].shape == torch.Size([1, 16, 32])
+ assert sa_features[2].shape == torch.Size([1, 16, 16])
+ assert sa_indices[0].shape == torch.Size([1, 100])
+ assert sa_indices[1].shape == torch.Size([1, 32])
+ assert sa_indices[2].shape == torch.Size([1, 16])
+
+ # test only xyz input without features
+ cfg['in_channels'] = 3
+ self = build_backbone(cfg)
+ self.cuda()
+ ret_dict = self(xyz[..., :3])
+ assert len(fp_xyz) == len(fp_features) == len(fp_indices) == 3
+ assert len(sa_xyz) == len(sa_features) == len(sa_indices) == 3
+ assert fp_features[0].shape == torch.Size([1, 16, 16])
+ assert fp_features[1].shape == torch.Size([1, 16, 32])
+ assert fp_features[2].shape == torch.Size([1, 16, 100])
+ assert sa_features[0].shape == torch.Size([1, 3, 100])
+ assert sa_features[1].shape == torch.Size([1, 16, 32])
+ assert sa_features[2].shape == torch.Size([1, 16, 16])
+
+
+def test_multi_backbone():
+ if not torch.cuda.is_available():
+ pytest.skip()
+
+ # test list config
+ cfg_list = dict(
+ type='MultiBackbone',
+ num_streams=4,
+ suffixes=['net0', 'net1', 'net2', 'net3'],
+ backbones=[
+ dict(
+ type='PointNet2SASSG',
+ in_channels=4,
+ num_points=(256, 128, 64, 32),
+ radius=(0.2, 0.4, 0.8, 1.2),
+ num_samples=(64, 32, 16, 16),
+ sa_channels=((64, 64, 128), (128, 128, 256), (128, 128, 256),
+ (128, 128, 256)),
+ fp_channels=((256, 256), (256, 256)),
+ norm_cfg=dict(type='BN2d')),
+ dict(
+ type='PointNet2SASSG',
+ in_channels=4,
+ num_points=(256, 128, 64, 32),
+ radius=(0.2, 0.4, 0.8, 1.2),
+ num_samples=(64, 32, 16, 16),
+ sa_channels=((64, 64, 128), (128, 128, 256), (128, 128, 256),
+ (128, 128, 256)),
+ fp_channels=((256, 256), (256, 256)),
+ norm_cfg=dict(type='BN2d')),
+ dict(
+ type='PointNet2SASSG',
+ in_channels=4,
+ num_points=(256, 128, 64, 32),
+ radius=(0.2, 0.4, 0.8, 1.2),
+ num_samples=(64, 32, 16, 16),
+ sa_channels=((64, 64, 128), (128, 128, 256), (128, 128, 256),
+ (128, 128, 256)),
+ fp_channels=((256, 256), (256, 256)),
+ norm_cfg=dict(type='BN2d')),
+ dict(
+ type='PointNet2SASSG',
+ in_channels=4,
+ num_points=(256, 128, 64, 32),
+ radius=(0.2, 0.4, 0.8, 1.2),
+ num_samples=(64, 32, 16, 16),
+ sa_channels=((64, 64, 128), (128, 128, 256), (128, 128, 256),
+ (128, 128, 256)),
+ fp_channels=((256, 256), (256, 256)),
+ norm_cfg=dict(type='BN2d'))
+ ])
+
+ self = build_backbone(cfg_list)
+ self.cuda()
+
+ assert len(self.backbone_list) == 4
+
+ xyz = np.fromfile('tests/data/sunrgbd/points/000001.bin', dtype=np.float32)
+ xyz = torch.from_numpy(xyz).view(1, -1, 6).cuda() # (B, N, 6)
+ # test forward
+ ret_dict = self(xyz[:, :, :4])
+
+ assert ret_dict['hd_feature'].shape == torch.Size([1, 256, 128])
+ assert ret_dict['fp_xyz_net0'][-1].shape == torch.Size([1, 128, 3])
+ assert ret_dict['fp_features_net0'][-1].shape == torch.Size([1, 256, 128])
+
+ # test dict config
+ cfg_dict = dict(
+ type='MultiBackbone',
+ num_streams=2,
+ suffixes=['net0', 'net1'],
+ aggregation_mlp_channels=[512, 128],
+ backbones=dict(
+ type='PointNet2SASSG',
+ in_channels=4,
+ num_points=(256, 128, 64, 32),
+ radius=(0.2, 0.4, 0.8, 1.2),
+ num_samples=(64, 32, 16, 16),
+ sa_channels=((64, 64, 128), (128, 128, 256), (128, 128, 256),
+ (128, 128, 256)),
+ fp_channels=((256, 256), (256, 256)),
+ norm_cfg=dict(type='BN2d')))
+
+ self = build_backbone(cfg_dict)
+ self.cuda()
+
+ assert len(self.backbone_list) == 2
+
+ # test forward
+ ret_dict = self(xyz[:, :, :4])
+
+ assert ret_dict['hd_feature'].shape == torch.Size([1, 128, 128])
+ assert ret_dict['fp_xyz_net0'][-1].shape == torch.Size([1, 128, 3])
+ assert ret_dict['fp_features_net0'][-1].shape == torch.Size([1, 256, 128])
+
+ # Length of backbone configs list should be equal to num_streams
+ with pytest.raises(AssertionError):
+ cfg_list['num_streams'] = 3
+ build_backbone(cfg_list)
+
+ # Length of suffixes list should be equal to num_streams
+ with pytest.raises(AssertionError):
+ cfg_dict['suffixes'] = ['net0', 'net1', 'net2']
+ build_backbone(cfg_dict)
+
+ # Type of 'backbones' should be Dict or List[Dict].
+ with pytest.raises(AssertionError):
+ cfg_dict['backbones'] = 'PointNet2SASSG'
+ build_backbone(cfg_dict)
+
+
+def test_pointnet2_sa_msg():
+ if not torch.cuda.is_available():
+ pytest.skip()
+
+ # PN2MSG used in 3DSSD
+ cfg = dict(
+ type='PointNet2SAMSG',
+ in_channels=4,
+ num_points=(256, 64, (32, 32)),
+ radii=((0.2, 0.4, 0.8), (0.4, 0.8, 1.6), (1.6, 3.2, 4.8)),
+ num_samples=((8, 8, 16), (8, 8, 16), (8, 8, 8)),
+ sa_channels=(((8, 8, 16), (8, 8, 16),
+ (8, 8, 16)), ((16, 16, 32), (16, 16, 32), (16, 24, 32)),
+ ((32, 32, 64), (32, 24, 64), (32, 64, 64))),
+ aggregation_channels=(16, 32, 64),
+ fps_mods=(('D-FPS'), ('FS'), ('F-FPS', 'D-FPS')),
+ fps_sample_range_lists=((-1), (-1), (64, -1)),
+ norm_cfg=dict(type='BN2d'),
+ sa_cfg=dict(
+ type='PointSAModuleMSG',
+ pool_mod='max',
+ use_xyz=True,
+ normalize_xyz=False))
+
+ self = build_backbone(cfg)
+ self.cuda()
+ assert self.SA_modules[0].mlps[0].layer0.conv.in_channels == 4
+ assert self.SA_modules[0].mlps[0].layer0.conv.out_channels == 8
+ assert self.SA_modules[0].mlps[1].layer1.conv.out_channels == 8
+ assert self.SA_modules[2].mlps[2].layer2.conv.out_channels == 64
+
+ xyz = np.fromfile('tests/data/sunrgbd/points/000001.bin', dtype=np.float32)
+ xyz = torch.from_numpy(xyz).view(1, -1, 6).cuda() # (B, N, 6)
+ # test forward
+ ret_dict = self(xyz[:, :, :4])
+ sa_xyz = ret_dict['sa_xyz'][-1]
+ sa_features = ret_dict['sa_features'][-1]
+ sa_indices = ret_dict['sa_indices'][-1]
+
+ assert sa_xyz.shape == torch.Size([1, 64, 3])
+ assert sa_features.shape == torch.Size([1, 64, 64])
+ assert sa_indices.shape == torch.Size([1, 64])
+
+ # out_indices should smaller than the length of SA Modules.
+ with pytest.raises(AssertionError):
+ build_backbone(
+ dict(
+ type='PointNet2SAMSG',
+ in_channels=4,
+ num_points=(256, 64, (32, 32)),
+ radii=((0.2, 0.4, 0.8), (0.4, 0.8, 1.6), (1.6, 3.2, 4.8)),
+ num_samples=((8, 8, 16), (8, 8, 16), (8, 8, 8)),
+ sa_channels=(((8, 8, 16), (8, 8, 16), (8, 8, 16)),
+ ((16, 16, 32), (16, 16, 32), (16, 24, 32)),
+ ((32, 32, 64), (32, 24, 64), (32, 64, 64))),
+ aggregation_channels=(16, 32, 64),
+ fps_mods=(('D-FPS'), ('FS'), ('F-FPS', 'D-FPS')),
+ fps_sample_range_lists=((-1), (-1), (64, -1)),
+ out_indices=(2, 3),
+ norm_cfg=dict(type='BN2d'),
+ sa_cfg=dict(
+ type='PointSAModuleMSG',
+ pool_mod='max',
+ use_xyz=True,
+ normalize_xyz=False)))
+
+ # PN2MSG used in segmentation
+ cfg = dict(
+ type='PointNet2SAMSG',
+ in_channels=6, # [xyz, rgb]
+ num_points=(1024, 256, 64, 16),
+ radii=((0.05, 0.1), (0.1, 0.2), (0.2, 0.4), (0.4, 0.8)),
+ num_samples=((16, 32), (16, 32), (16, 32), (16, 32)),
+ sa_channels=(((16, 16, 32), (32, 32, 64)), ((64, 64, 128), (64, 96,
+ 128)),
+ ((128, 196, 256), (128, 196, 256)), ((256, 256, 512),
+ (256, 384, 512))),
+ aggregation_channels=(None, None, None, None),
+ fps_mods=(('D-FPS'), ('D-FPS'), ('D-FPS'), ('D-FPS')),
+ fps_sample_range_lists=((-1), (-1), (-1), (-1)),
+ dilated_group=(False, False, False, False),
+ out_indices=(0, 1, 2, 3),
+ norm_cfg=dict(type='BN2d'),
+ sa_cfg=dict(
+ type='PointSAModuleMSG',
+ pool_mod='max',
+ use_xyz=True,
+ normalize_xyz=False))
+
+ self = build_backbone(cfg)
+ self.cuda()
+ ret_dict = self(xyz)
+ sa_xyz = ret_dict['sa_xyz']
+ sa_features = ret_dict['sa_features']
+ sa_indices = ret_dict['sa_indices']
+
+ assert len(sa_xyz) == len(sa_features) == len(sa_indices) == 5
+ assert sa_xyz[0].shape == torch.Size([1, 100, 3])
+ assert sa_xyz[1].shape == torch.Size([1, 1024, 3])
+ assert sa_xyz[2].shape == torch.Size([1, 256, 3])
+ assert sa_xyz[3].shape == torch.Size([1, 64, 3])
+ assert sa_xyz[4].shape == torch.Size([1, 16, 3])
+ assert sa_features[0].shape == torch.Size([1, 3, 100])
+ assert sa_features[1].shape == torch.Size([1, 96, 1024])
+ assert sa_features[2].shape == torch.Size([1, 256, 256])
+ assert sa_features[3].shape == torch.Size([1, 512, 64])
+ assert sa_features[4].shape == torch.Size([1, 1024, 16])
+ assert sa_indices[0].shape == torch.Size([1, 100])
+ assert sa_indices[1].shape == torch.Size([1, 1024])
+ assert sa_indices[2].shape == torch.Size([1, 256])
+ assert sa_indices[3].shape == torch.Size([1, 64])
+ assert sa_indices[4].shape == torch.Size([1, 16])
+
+
+def test_dgcnn_gf():
+ if not torch.cuda.is_available():
+ pytest.skip()
+
+ # DGCNNGF used in segmentation
+ cfg = dict(
+ type='DGCNNBackbone',
+ in_channels=6,
+ num_samples=(20, 20, 20),
+ knn_modes=['D-KNN', 'F-KNN', 'F-KNN'],
+ radius=(None, None, None),
+ gf_channels=((64, 64), (64, 64), (64, )),
+ fa_channels=(1024, ),
+ act_cfg=dict(type='ReLU'))
+
+ self = build_backbone(cfg)
+ self.cuda()
+
+ xyz = np.fromfile('tests/data/sunrgbd/points/000001.bin', dtype=np.float32)
+ xyz = torch.from_numpy(xyz).view(1, -1, 6).cuda() # (B, N, 6)
+ # test forward
+ ret_dict = self(xyz)
+ gf_points = ret_dict['gf_points']
+ fa_points = ret_dict['fa_points']
+
+ assert len(gf_points) == 4
+ assert gf_points[0].shape == torch.Size([1, 100, 6])
+ assert gf_points[1].shape == torch.Size([1, 100, 64])
+ assert gf_points[2].shape == torch.Size([1, 100, 64])
+ assert gf_points[3].shape == torch.Size([1, 100, 64])
+ assert fa_points.shape == torch.Size([1, 100, 1216])
+
+
+def test_dla_net():
+ # test DLANet used in SMOKE
+ # test list config
+ cfg = dict(
+ type='DLANet',
+ depth=34,
+ in_channels=3,
+ norm_cfg=dict(type='GN', num_groups=32))
+
+ img = torch.randn((4, 3, 32, 32))
+ self = build_backbone(cfg)
+ self.init_weights()
+
+ results = self(img)
+ assert len(results) == 6
+ assert results[0].shape == torch.Size([4, 16, 32, 32])
+ assert results[1].shape == torch.Size([4, 32, 16, 16])
+ assert results[2].shape == torch.Size([4, 64, 8, 8])
+ assert results[3].shape == torch.Size([4, 128, 4, 4])
+ assert results[4].shape == torch.Size([4, 256, 2, 2])
+ assert results[5].shape == torch.Size([4, 512, 1, 1])
+
+
+def test_mink_resnet():
+ if not torch.cuda.is_available():
+ pytest.skip('test requires GPU and torch+cuda')
+
+ try:
+ import MinkowskiEngine as ME
+ except ImportError:
+ pytest.skip('test requires MinkowskiEngine installation')
+
+ coordinates, features = [], []
+ np.random.seed(42)
+ # batch of 2 point clouds
+ for i in range(2):
+ c = torch.from_numpy(np.random.rand(500, 3) * 100)
+ coordinates.append(c.float().cuda())
+ f = torch.from_numpy(np.random.rand(500, 3))
+ features.append(f.float().cuda())
+ tensor_coordinates, tensor_features = ME.utils.sparse_collate(
+ coordinates, features)
+ x = ME.SparseTensor(
+ features=tensor_features, coordinates=tensor_coordinates)
+
+ # MinkResNet34 with 4 outputs
+ cfg = dict(type='MinkResNet', depth=34, in_channels=3)
+ self = build_backbone(cfg).cuda()
+ self.init_weights()
+
+ y = self(x)
+ assert len(y) == 4
+ assert y[0].F.shape == torch.Size([900, 64])
+ assert y[0].tensor_stride[0] == 8
+ assert y[1].F.shape == torch.Size([472, 128])
+ assert y[1].tensor_stride[0] == 16
+ assert y[2].F.shape == torch.Size([105, 256])
+ assert y[2].tensor_stride[0] == 32
+ assert y[3].F.shape == torch.Size([16, 512])
+ assert y[3].tensor_stride[0] == 64
+
+ # MinkResNet50 with 2 outputs
+ cfg = dict(
+ type='MinkResNet', depth=34, in_channels=3, num_stages=2, pool=False)
+ self = build_backbone(cfg).cuda()
+ self.init_weights()
+
+ y = self(x)
+ assert len(y) == 2
+ assert y[0].F.shape == torch.Size([985, 64])
+ assert y[0].tensor_stride[0] == 4
+ assert y[1].F.shape == torch.Size([900, 128])
+ assert y[1].tensor_stride[0] == 8
diff --git a/tests/test_models/test_common_modules/test_dgcnn_modules.py b/tests/test_models/test_common_modules/test_dgcnn_modules.py
new file mode 100644
index 0000000..6b56120
--- /dev/null
+++ b/tests/test_models/test_common_modules/test_dgcnn_modules.py
@@ -0,0 +1,92 @@
+# Copyright (c) OpenMMLab. All rights reserved.
+import numpy as np
+import pytest
+import torch
+
+
+def test_dgcnn_gf_module():
+ if not torch.cuda.is_available():
+ pytest.skip()
+ from mmdet3d.ops import DGCNNGFModule
+
+ self = DGCNNGFModule(
+ mlp_channels=[18, 64, 64],
+ num_sample=20,
+ knn_mode='D-KNN',
+ radius=None,
+ norm_cfg=dict(type='BN2d'),
+ act_cfg=dict(type='ReLU'),
+ pool_mode='max').cuda()
+
+ assert self.mlps[0].layer0.conv.in_channels == 18
+ assert self.mlps[0].layer0.conv.out_channels == 64
+
+ xyz = np.fromfile('tests/data/sunrgbd/points/000001.bin', np.float32)
+
+ # (B, N, C)
+ xyz = torch.from_numpy(xyz).view(1, -1, 3).cuda()
+ points = xyz.repeat([1, 1, 3])
+
+ # test forward
+ new_points = self(points)
+
+ assert new_points.shape == torch.Size([1, 200, 64])
+
+ # test F-KNN mod
+ self = DGCNNGFModule(
+ mlp_channels=[6, 64, 64],
+ num_sample=20,
+ knn_mode='F-KNN',
+ radius=None,
+ norm_cfg=dict(type='BN2d'),
+ act_cfg=dict(type='ReLU'),
+ pool_mode='max').cuda()
+
+ # test forward
+ new_points = self(xyz)
+ assert new_points.shape == torch.Size([1, 200, 64])
+
+ # test ball query
+ self = DGCNNGFModule(
+ mlp_channels=[6, 64, 64],
+ num_sample=20,
+ knn_mode='F-KNN',
+ radius=0.2,
+ norm_cfg=dict(type='BN2d'),
+ act_cfg=dict(type='ReLU'),
+ pool_mode='max').cuda()
+
+
+def test_dgcnn_fa_module():
+ if not torch.cuda.is_available():
+ pytest.skip()
+ from mmdet3d.ops import DGCNNFAModule
+
+ self = DGCNNFAModule(mlp_channels=[24, 16]).cuda()
+ assert self.mlps.layer0.conv.in_channels == 24
+ assert self.mlps.layer0.conv.out_channels == 16
+
+ points = [torch.rand(1, 200, 12).float().cuda() for _ in range(3)]
+
+ fa_points = self(points)
+ assert fa_points.shape == torch.Size([1, 200, 40])
+
+
+def test_dgcnn_fp_module():
+ if not torch.cuda.is_available():
+ pytest.skip()
+ from mmdet3d.ops import DGCNNFPModule
+
+ self = DGCNNFPModule(mlp_channels=[24, 16]).cuda()
+ assert self.mlps.layer0.conv.in_channels == 24
+ assert self.mlps.layer0.conv.out_channels == 16
+
+ xyz = np.fromfile('tests/data/sunrgbd/points/000001.bin',
+ np.float32).reshape((-1, 6))
+
+ # (B, N, 3)
+ xyz = torch.from_numpy(xyz).view(1, -1, 3).cuda()
+ points = xyz.repeat([1, 1, 8]).cuda()
+
+ fp_points = self(points)
+ assert fp_points.shape == torch.Size([1, 200, 16])
diff --git a/tests/test_models/test_common_modules/test_middle_encoders.py b/tests/test_models/test_common_modules/test_middle_encoders.py
new file mode 100644
index 0000000..1fe0e22
--- /dev/null
+++ b/tests/test_models/test_common_modules/test_middle_encoders.py
@@ -0,0 +1,49 @@
+# Copyright (c) OpenMMLab. All rights reserved.
+import pytest
+import torch
+
+from mmdet3d.models.builder import build_middle_encoder
+
+
+def test_sparse_encoder():
+ if not torch.cuda.is_available():
+ pytest.skip('test requires GPU and torch+cuda')
+ sparse_encoder_cfg = dict(
+ type='SparseEncoder',
+ in_channels=5,
+ sparse_shape=[40, 1024, 1024],
+ order=('conv', 'norm', 'act'),
+ encoder_channels=((16, 16, 32), (32, 32, 64), (64, 64, 128), (128,
+ 128)),
+ encoder_paddings=((1, 1, 1), (1, 1, 1), (1, 1, 1), (1, 1, 1), (1, 1,
+ 1)),
+ block_type='basicblock')
+
+ sparse_encoder = build_middle_encoder(sparse_encoder_cfg).cuda()
+ voxel_features = torch.rand([207842, 5]).cuda()
+ coors = torch.randint(0, 4, [207842, 4]).cuda()
+
+ ret = sparse_encoder(voxel_features, coors, 4)
+ assert ret.shape == torch.Size([4, 256, 128, 128])
+
+
+def test_sparse_encoder_for_ssd():
+ if not torch.cuda.is_available():
+ pytest.skip('test requires GPU and torch+cuda')
+ sparse_encoder_for_ssd_cfg = dict(
+ type='SparseEncoderSASSD',
+ in_channels=5,
+ sparse_shape=[40, 1024, 1024],
+ order=('conv', 'norm', 'act'),
+ encoder_channels=((16, 16, 32), (32, 32, 64), (64, 64, 128), (128,
+ 128)),
+ encoder_paddings=((1, 1, 1), (1, 1, 1), (1, 1, 1), (1, 1, 1), (1, 1,
+ 1)),
+ block_type='basicblock')
+
+ sparse_encoder = build_middle_encoder(sparse_encoder_for_ssd_cfg).cuda()
+ voxel_features = torch.rand([207842, 5]).cuda()
+ coors = torch.randint(0, 4, [207842, 4]).cuda()
+
+ ret, _ = sparse_encoder(voxel_features, coors, 4, True)
+ assert ret.shape == torch.Size([4, 256, 128, 128])
diff --git a/tests/test_models/test_common_modules/test_paconv_modules.py b/tests/test_models/test_common_modules/test_paconv_modules.py
new file mode 100644
index 0000000..2ca1274
--- /dev/null
+++ b/tests/test_models/test_common_modules/test_paconv_modules.py
@@ -0,0 +1,300 @@
+# Copyright (c) OpenMMLab. All rights reserved.
+import numpy as np
+import pytest
+import torch
+
+
+def test_paconv_sa_module_msg():
+ if not torch.cuda.is_available():
+ pytest.skip()
+ from mmdet3d.ops import PAConvSAModuleMSG
+
+ # paconv_num_kernels should have same length as mlp_channels
+ with pytest.raises(AssertionError):
+ self = PAConvSAModuleMSG(
+ num_point=16,
+ radii=[0.2, 0.4],
+ sample_nums=[4, 8],
+ mlp_channels=[[12, 16], [12, 32]],
+ paconv_num_kernels=[[4]]).cuda()
+
+ # paconv_num_kernels inner num should match as mlp_channels
+ with pytest.raises(AssertionError):
+ self = PAConvSAModuleMSG(
+ num_point=16,
+ radii=[0.2, 0.4],
+ sample_nums=[4, 8],
+ mlp_channels=[[12, 16], [12, 32]],
+ paconv_num_kernels=[[4, 4], [8, 8]]).cuda()
+
+ self = PAConvSAModuleMSG(
+ num_point=16,
+ radii=[0.2, 0.4],
+ sample_nums=[4, 8],
+ mlp_channels=[[12, 16], [12, 32]],
+ paconv_num_kernels=[[4], [8]],
+ norm_cfg=dict(type='BN2d'),
+ use_xyz=False,
+ pool_mod='max',
+ paconv_kernel_input='w_neighbor').cuda()
+
+ assert self.mlps[0].layer0.in_channels == 12 * 2
+ assert self.mlps[0].layer0.out_channels == 16
+ assert self.mlps[1].layer0.in_channels == 12 * 2
+ assert self.mlps[1].layer0.out_channels == 32
+ assert self.mlps[0].layer0.bn.num_features == 16
+ assert self.mlps[1].layer0.bn.num_features == 32
+
+ assert self.mlps[0].layer0.scorenet.mlps.layer0.conv.in_channels == 7
+ assert self.mlps[0].layer0.scorenet.mlps.layer3.conv.out_channels == 4
+ assert self.mlps[1].layer0.scorenet.mlps.layer0.conv.in_channels == 7
+ assert self.mlps[1].layer0.scorenet.mlps.layer3.conv.out_channels == 8
+
+ # last conv in ScoreNet has neither bn nor relu
+ with pytest.raises(AttributeError):
+ _ = self.mlps[0].layer0.scorenet.mlps.layer3.bn
+ with pytest.raises(AttributeError):
+ _ = self.mlps[0].layer0.scorenet.mlps.layer3.activate
+
+ xyz = np.fromfile('tests/data/sunrgbd/points/000001.bin', np.float32)
+
+ # (B, N, 3)
+ xyz = torch.from_numpy(xyz).view(1, -1, 3).cuda()
+ # (B, C, N)
+ features = xyz.repeat([1, 1, 4]).transpose(1, 2).contiguous().cuda()
+
+ # test forward
+ new_xyz, new_features, inds = self(xyz, features)
+ assert new_xyz.shape == torch.Size([1, 16, 3])
+ assert new_features.shape == torch.Size([1, 48, 16])
+ assert inds.shape == torch.Size([1, 16])
+
+ # test with identity kernel input
+ self = PAConvSAModuleMSG(
+ num_point=16,
+ radii=[0.2, 0.4],
+ sample_nums=[4, 8],
+ mlp_channels=[[12, 16], [12, 32]],
+ paconv_num_kernels=[[4], [8]],
+ norm_cfg=dict(type='BN2d'),
+ use_xyz=False,
+ pool_mod='max',
+ paconv_kernel_input='identity').cuda()
+
+ assert self.mlps[0].layer0.in_channels == 12 * 1
+ assert self.mlps[0].layer0.out_channels == 16
+ assert self.mlps[0].layer0.num_kernels == 4
+ assert self.mlps[1].layer0.in_channels == 12 * 1
+ assert self.mlps[1].layer0.out_channels == 32
+ assert self.mlps[1].layer0.num_kernels == 8
+
+ xyz = np.fromfile('tests/data/sunrgbd/points/000001.bin', np.float32)
+
+ # (B, N, 3)
+ xyz = torch.from_numpy(xyz).view(1, -1, 3).cuda()
+ # (B, C, N)
+ features = xyz.repeat([1, 1, 4]).transpose(1, 2).contiguous().cuda()
+
+ # test forward
+ new_xyz, new_features, inds = self(xyz, features)
+ assert new_xyz.shape == torch.Size([1, 16, 3])
+ assert new_features.shape == torch.Size([1, 48, 16])
+ assert inds.shape == torch.Size([1, 16])
+
+
+def test_paconv_sa_module():
+ if not torch.cuda.is_available():
+ pytest.skip()
+ from mmdet3d.ops import build_sa_module
+ sa_cfg = dict(
+ type='PAConvSAModule',
+ num_point=16,
+ radius=0.2,
+ num_sample=8,
+ mlp_channels=[12, 32],
+ paconv_num_kernels=[8],
+ norm_cfg=dict(type='BN2d'),
+ use_xyz=True,
+ pool_mod='max',
+ paconv_kernel_input='w_neighbor')
+ self = build_sa_module(sa_cfg).cuda()
+
+ assert self.mlps[0].layer0.in_channels == 15 * 2
+ assert self.mlps[0].layer0.out_channels == 32
+ assert self.mlps[0].layer0.num_kernels == 8
+
+ xyz = np.fromfile('tests/data/sunrgbd/points/000001.bin', np.float32)
+
+ # (B, N, 3)
+ xyz = torch.from_numpy(xyz[..., :3]).view(1, -1, 3).cuda()
+ # (B, C, N)
+ features = xyz.repeat([1, 1, 4]).transpose(1, 2).contiguous().cuda()
+
+ # test forward
+ new_xyz, new_features, inds = self(xyz, features)
+ assert new_xyz.shape == torch.Size([1, 16, 3])
+ assert new_features.shape == torch.Size([1, 32, 16])
+ assert inds.shape == torch.Size([1, 16])
+
+ # test kNN sampling when radius is None
+ sa_cfg = dict(
+ type='PAConvSAModule',
+ num_point=16,
+ radius=None,
+ num_sample=8,
+ mlp_channels=[12, 32],
+ paconv_num_kernels=[8],
+ norm_cfg=dict(type='BN2d'),
+ use_xyz=True,
+ pool_mod='max',
+ paconv_kernel_input='identity')
+ self = build_sa_module(sa_cfg).cuda()
+ assert self.mlps[0].layer0.in_channels == 15 * 1
+
+ xyz = np.fromfile('tests/data/sunrgbd/points/000001.bin', np.float32)
+
+ xyz = torch.from_numpy(xyz[..., :3]).view(1, -1, 3).cuda()
+ features = xyz.repeat([1, 1, 4]).transpose(1, 2).contiguous().cuda()
+ new_xyz, new_features, inds = self(xyz, features)
+ assert new_xyz.shape == torch.Size([1, 16, 3])
+ assert new_features.shape == torch.Size([1, 32, 16])
+ assert inds.shape == torch.Size([1, 16])
+
+
+def test_paconv_cuda_sa_module_msg():
+ if not torch.cuda.is_available():
+ pytest.skip()
+ from mmdet3d.ops import PAConvCUDASAModuleMSG
+
+ # paconv_num_kernels should have same length as mlp_channels
+ with pytest.raises(AssertionError):
+ self = PAConvCUDASAModuleMSG(
+ num_point=16,
+ radii=[0.2, 0.4],
+ sample_nums=[4, 8],
+ mlp_channels=[[12, 16], [12, 32]],
+ paconv_num_kernels=[[4]]).cuda()
+
+ # paconv_num_kernels inner num should match as mlp_channels
+ with pytest.raises(AssertionError):
+ self = PAConvCUDASAModuleMSG(
+ num_point=16,
+ radii=[0.2, 0.4],
+ sample_nums=[4, 8],
+ mlp_channels=[[12, 16], [12, 32]],
+ paconv_num_kernels=[[4, 4], [8, 8]]).cuda()
+
+ self = PAConvCUDASAModuleMSG(
+ num_point=16,
+ radii=[0.2, 0.4],
+ sample_nums=[4, 8],
+ mlp_channels=[[12, 16], [12, 32]],
+ paconv_num_kernels=[[4], [8]],
+ norm_cfg=dict(type='BN2d'),
+ use_xyz=False,
+ pool_mod='max',
+ paconv_kernel_input='w_neighbor').cuda()
+
+ assert self.mlps[0][0].in_channels == 12 * 2
+ assert self.mlps[0][0].out_channels == 16
+ assert self.mlps[0][0].num_kernels == 4
+ assert self.mlps[0][0].bn.num_features == 16
+ assert self.mlps[1][0].in_channels == 12 * 2
+ assert self.mlps[1][0].out_channels == 32
+ assert self.mlps[1][0].num_kernels == 8
+ assert self.mlps[1][0].bn.num_features == 32
+
+ assert self.mlps[0][0].scorenet.mlps.layer0.conv.in_channels == 7
+ assert self.mlps[0][0].scorenet.mlps.layer3.conv.out_channels == 4
+ assert self.mlps[1][0].scorenet.mlps.layer0.conv.in_channels == 7
+ assert self.mlps[1][0].scorenet.mlps.layer3.conv.out_channels == 8
+
+ # last conv in ScoreNet has neither bn nor relu
+ with pytest.raises(AttributeError):
+ _ = self.mlps[0][0].scorenet.mlps.layer3.bn
+ with pytest.raises(AttributeError):
+ _ = self.mlps[0][0].scorenet.mlps.layer3.activate
+
+ xyz = np.fromfile('tests/data/sunrgbd/points/000001.bin', np.float32)
+
+ # (B, N, 3)
+ xyz = torch.from_numpy(xyz).view(1, -1, 3).cuda()
+ # (B, C, N)
+ features = xyz.repeat([1, 1, 4]).transpose(1, 2).contiguous().cuda()
+
+ # test forward
+ new_xyz, new_features, inds = self(xyz, features)
+ assert new_xyz.shape == torch.Size([1, 16, 3])
+ assert new_features.shape == torch.Size([1, 48, 16])
+ assert inds.shape == torch.Size([1, 16])
+
+ # CUDA PAConv only supports w_neighbor kernel_input
+ with pytest.raises(AssertionError):
+ self = PAConvCUDASAModuleMSG(
+ num_point=16,
+ radii=[0.2, 0.4],
+ sample_nums=[4, 8],
+ mlp_channels=[[12, 16], [12, 32]],
+ paconv_num_kernels=[[4], [8]],
+ norm_cfg=dict(type='BN2d'),
+ use_xyz=False,
+ pool_mod='max',
+ paconv_kernel_input='identity').cuda()
+
+
+def test_paconv_cuda_sa_module():
+ if not torch.cuda.is_available():
+ pytest.skip()
+ from mmdet3d.ops import build_sa_module
+ sa_cfg = dict(
+ type='PAConvCUDASAModule',
+ num_point=16,
+ radius=0.2,
+ num_sample=8,
+ mlp_channels=[12, 32],
+ paconv_num_kernels=[8],
+ norm_cfg=dict(type='BN2d'),
+ use_xyz=True,
+ pool_mod='max',
+ paconv_kernel_input='w_neighbor')
+ self = build_sa_module(sa_cfg).cuda()
+
+ assert self.mlps[0][0].in_channels == 15 * 2
+ assert self.mlps[0][0].out_channels == 32
+ assert self.mlps[0][0].num_kernels == 8
+
+ xyz = np.fromfile('tests/data/sunrgbd/points/000001.bin', np.float32)
+
+ # (B, N, 3)
+ xyz = torch.from_numpy(xyz[..., :3]).view(1, -1, 3).cuda()
+ # (B, C, N)
+ features = xyz.repeat([1, 1, 4]).transpose(1, 2).contiguous().cuda()
+
+ # test forward
+ new_xyz, new_features, inds = self(xyz, features)
+ assert new_xyz.shape == torch.Size([1, 16, 3])
+ assert new_features.shape == torch.Size([1, 32, 16])
+ assert inds.shape == torch.Size([1, 16])
+
+ # test kNN sampling when radius is None
+ sa_cfg = dict(
+ type='PAConvCUDASAModule',
+ num_point=16,
+ radius=None,
+ num_sample=8,
+ mlp_channels=[12, 32],
+ paconv_num_kernels=[8],
+ norm_cfg=dict(type='BN2d'),
+ use_xyz=True,
+ pool_mod='max',
+ paconv_kernel_input='w_neighbor')
+ self = build_sa_module(sa_cfg).cuda()
+
+ xyz = np.fromfile('tests/data/sunrgbd/points/000001.bin', np.float32)
+
+ xyz = torch.from_numpy(xyz[..., :3]).view(1, -1, 3).cuda()
+ features = xyz.repeat([1, 1, 4]).transpose(1, 2).contiguous().cuda()
+ new_xyz, new_features, inds = self(xyz, features)
+ assert new_xyz.shape == torch.Size([1, 16, 3])
+ assert new_features.shape == torch.Size([1, 32, 16])
+ assert inds.shape == torch.Size([1, 16])
diff --git a/tests/test_models/test_common_modules/test_paconv_ops.py b/tests/test_models/test_common_modules/test_paconv_ops.py
new file mode 100644
index 0000000..1aab6da
--- /dev/null
+++ b/tests/test_models/test_common_modules/test_paconv_ops.py
@@ -0,0 +1,49 @@
+# Copyright (c) OpenMMLab. All rights reserved.
+import pytest
+import torch
+
+from mmdet3d.ops import PAConv, PAConvCUDA
+
+
+def test_paconv():
+ B = 2
+ in_channels = 6
+ out_channels = 12
+ npoint = 4
+ K = 3
+ num_kernels = 4
+ points_xyz = torch.randn(B, 3, npoint, K)
+ features = torch.randn(B, in_channels, npoint, K)
+
+ paconv = PAConv(in_channels, out_channels, num_kernels)
+ assert paconv.weight_bank.shape == torch.Size(
+ [in_channels * 2, out_channels * num_kernels])
+
+ with torch.no_grad():
+ new_features, _ = paconv((features, points_xyz))
+
+ assert new_features.shape == torch.Size([B, out_channels, npoint, K])
+
+
+def test_paconv_cuda():
+ if not torch.cuda.is_available():
+ pytest.skip()
+ B = 2
+ in_channels = 6
+ out_channels = 12
+ N = 32
+ npoint = 4
+ K = 3
+ num_kernels = 4
+ points_xyz = torch.randn(B, 3, npoint, K).float().cuda()
+ features = torch.randn(B, in_channels, N).float().cuda()
+ points_idx = torch.randint(0, N, (B, npoint, K)).long().cuda()
+
+ paconv = PAConvCUDA(in_channels, out_channels, num_kernels).cuda()
+ assert paconv.weight_bank.shape == torch.Size(
+ [in_channels * 2, out_channels * num_kernels])
+
+ with torch.no_grad():
+ new_features, _, _ = paconv((features, points_xyz, points_idx))
+
+ assert new_features.shape == torch.Size([B, out_channels, npoint, K])
diff --git a/tests/test_models/test_common_modules/test_pointnet_modules.py b/tests/test_models/test_common_modules/test_pointnet_modules.py
new file mode 100644
index 0000000..66c21b1
--- /dev/null
+++ b/tests/test_models/test_common_modules/test_pointnet_modules.py
@@ -0,0 +1,234 @@
+# Copyright (c) OpenMMLab. All rights reserved.
+import numpy as np
+import pytest
+import torch
+
+
+def test_pointnet_sa_module_msg():
+ if not torch.cuda.is_available():
+ pytest.skip()
+ from mmdet3d.ops import PointSAModuleMSG
+
+ self = PointSAModuleMSG(
+ num_point=16,
+ radii=[0.2, 0.4],
+ sample_nums=[4, 8],
+ mlp_channels=[[12, 16], [12, 32]],
+ norm_cfg=dict(type='BN2d'),
+ use_xyz=False,
+ pool_mod='max').cuda()
+
+ assert self.mlps[0].layer0.conv.in_channels == 12
+ assert self.mlps[0].layer0.conv.out_channels == 16
+ assert self.mlps[1].layer0.conv.in_channels == 12
+ assert self.mlps[1].layer0.conv.out_channels == 32
+
+ xyz = np.fromfile('tests/data/sunrgbd/points/000001.bin', np.float32)
+
+ # (B, N, 3)
+ xyz = torch.from_numpy(xyz).view(1, -1, 3).cuda()
+ # (B, C, N)
+ features = xyz.repeat([1, 1, 4]).transpose(1, 2).contiguous().cuda()
+
+ # test forward
+ new_xyz, new_features, inds = self(xyz, features)
+ assert new_xyz.shape == torch.Size([1, 16, 3])
+ assert new_features.shape == torch.Size([1, 48, 16])
+ assert inds.shape == torch.Size([1, 16])
+
+ # test D-FPS mod
+ self = PointSAModuleMSG(
+ num_point=16,
+ radii=[0.2, 0.4],
+ sample_nums=[4, 8],
+ mlp_channels=[[12, 16], [12, 32]],
+ norm_cfg=dict(type='BN2d'),
+ use_xyz=False,
+ pool_mod='max',
+ fps_mod=['D-FPS'],
+ fps_sample_range_list=[-1]).cuda()
+
+ # test forward
+ new_xyz, new_features, inds = self(xyz, features)
+ assert new_xyz.shape == torch.Size([1, 16, 3])
+ assert new_features.shape == torch.Size([1, 48, 16])
+ assert inds.shape == torch.Size([1, 16])
+
+ # test F-FPS mod
+ self = PointSAModuleMSG(
+ num_point=16,
+ radii=[0.2, 0.4],
+ sample_nums=[4, 8],
+ mlp_channels=[[12, 16], [12, 32]],
+ norm_cfg=dict(type='BN2d'),
+ use_xyz=False,
+ pool_mod='max',
+ fps_mod=['F-FPS'],
+ fps_sample_range_list=[-1]).cuda()
+
+ # test forward
+ new_xyz, new_features, inds = self(xyz, features)
+ assert new_xyz.shape == torch.Size([1, 16, 3])
+ assert new_features.shape == torch.Size([1, 48, 16])
+ assert inds.shape == torch.Size([1, 16])
+
+ # test FS mod
+ self = PointSAModuleMSG(
+ num_point=8,
+ radii=[0.2, 0.4],
+ sample_nums=[4, 8],
+ mlp_channels=[[12, 16], [12, 32]],
+ norm_cfg=dict(type='BN2d'),
+ use_xyz=False,
+ pool_mod='max',
+ fps_mod=['FS'],
+ fps_sample_range_list=[-1]).cuda()
+
+ # test forward
+ new_xyz, new_features, inds = self(xyz, features)
+ assert new_xyz.shape == torch.Size([1, 16, 3])
+ assert new_features.shape == torch.Size([1, 48, 16])
+ assert inds.shape == torch.Size([1, 16])
+
+ # test using F-FPS mod and D-FPS mod simultaneously
+ self = PointSAModuleMSG(
+ num_point=[8, 12],
+ radii=[0.2, 0.4],
+ sample_nums=[4, 8],
+ mlp_channels=[[12, 16], [12, 32]],
+ norm_cfg=dict(type='BN2d'),
+ use_xyz=False,
+ pool_mod='max',
+ fps_mod=['F-FPS', 'D-FPS'],
+ fps_sample_range_list=[64, -1]).cuda()
+
+ # test forward
+ new_xyz, new_features, inds = self(xyz, features)
+ assert new_xyz.shape == torch.Size([1, 20, 3])
+ assert new_features.shape == torch.Size([1, 48, 20])
+ assert inds.shape == torch.Size([1, 20])
+
+ # test num_points = None
+ self = PointSAModuleMSG(
+ num_point=None,
+ radii=[0.2, 0.4],
+ sample_nums=[4, 8],
+ mlp_channels=[[12, 16], [12, 32]],
+ norm_cfg=dict(type='BN2d'),
+ use_xyz=False,
+ pool_mod='max').cuda()
+
+ # test forward
+ new_xyz, new_features, inds = self(xyz, features)
+ assert new_features.shape == torch.Size([1, 48, 1])
+
+ # length of 'fps_mod' should be same as 'fps_sample_range_list'
+ with pytest.raises(AssertionError):
+ PointSAModuleMSG(
+ num_point=8,
+ radii=[0.2, 0.4],
+ sample_nums=[4, 8],
+ mlp_channels=[[12, 16], [12, 32]],
+ norm_cfg=dict(type='BN2d'),
+ use_xyz=False,
+ pool_mod='max',
+ fps_mod=['F-FPS', 'D-FPS'],
+ fps_sample_range_list=[-1]).cuda()
+
+ # length of 'num_point' should be same as 'fps_sample_range_list'
+ with pytest.raises(AssertionError):
+ PointSAModuleMSG(
+ num_point=[8, 8],
+ radii=[0.2, 0.4],
+ sample_nums=[4, 8],
+ mlp_channels=[[12, 16], [12, 32]],
+ norm_cfg=dict(type='BN2d'),
+ use_xyz=False,
+ pool_mod='max',
+ fps_mod=['F-FPS'],
+ fps_sample_range_list=[-1]).cuda()
+
+
+def test_pointnet_sa_module():
+ if not torch.cuda.is_available():
+ pytest.skip()
+ from mmdet3d.ops import build_sa_module
+ sa_cfg = dict(
+ type='PointSAModule',
+ num_point=16,
+ radius=0.2,
+ num_sample=8,
+ mlp_channels=[12, 32],
+ norm_cfg=dict(type='BN2d'),
+ use_xyz=True,
+ pool_mod='max')
+ self = build_sa_module(sa_cfg).cuda()
+
+ assert self.mlps[0].layer0.conv.in_channels == 15
+ assert self.mlps[0].layer0.conv.out_channels == 32
+
+ xyz = np.fromfile('tests/data/sunrgbd/points/000001.bin', np.float32)
+
+ # (B, N, 3)
+ xyz = torch.from_numpy(xyz[..., :3]).view(1, -1, 3).cuda()
+ # (B, C, N)
+ features = xyz.repeat([1, 1, 4]).transpose(1, 2).contiguous().cuda()
+
+ # test forward
+ new_xyz, new_features, inds = self(xyz, features)
+ assert new_xyz.shape == torch.Size([1, 16, 3])
+ assert new_features.shape == torch.Size([1, 32, 16])
+ assert inds.shape == torch.Size([1, 16])
+
+ # can't set normalize_xyz when radius is None
+ with pytest.raises(AssertionError):
+ sa_cfg = dict(
+ type='PointSAModule',
+ num_point=16,
+ radius=None,
+ num_sample=8,
+ mlp_channels=[12, 32],
+ norm_cfg=dict(type='BN2d'),
+ use_xyz=True,
+ pool_mod='max',
+ normalize_xyz=True)
+ self = build_sa_module(sa_cfg)
+
+ # test kNN sampling when radius is None
+ sa_cfg['normalize_xyz'] = False
+ self = build_sa_module(sa_cfg).cuda()
+
+ xyz = np.fromfile('tests/data/sunrgbd/points/000001.bin', np.float32)
+
+ xyz = torch.from_numpy(xyz[..., :3]).view(1, -1, 3).cuda()
+ features = xyz.repeat([1, 1, 4]).transpose(1, 2).contiguous().cuda()
+ new_xyz, new_features, inds = self(xyz, features)
+ assert new_xyz.shape == torch.Size([1, 16, 3])
+ assert new_features.shape == torch.Size([1, 32, 16])
+ assert inds.shape == torch.Size([1, 16])
+
+
+def test_pointnet_fp_module():
+ if not torch.cuda.is_available():
+ pytest.skip()
+ from mmdet3d.ops import PointFPModule
+
+ self = PointFPModule(mlp_channels=[24, 16]).cuda()
+ assert self.mlps.layer0.conv.in_channels == 24
+ assert self.mlps.layer0.conv.out_channels == 16
+
+ xyz = np.fromfile('tests/data/sunrgbd/points/000001.bin',
+ np.float32).reshape((-1, 6))
+
+ # (B, N, 3)
+ xyz1 = torch.from_numpy(xyz[0::2, :3]).view(1, -1, 3).cuda()
+ # (B, C1, N)
+ features1 = xyz1.repeat([1, 1, 4]).transpose(1, 2).contiguous().cuda()
+
+ # (B, M, 3)
+ xyz2 = torch.from_numpy(xyz[1::3, :3]).view(1, -1, 3).cuda()
+ # (B, C2, N)
+ features2 = xyz2.repeat([1, 1, 4]).transpose(1, 2).contiguous().cuda()
+
+ fp_features = self(xyz1, xyz2, features1, features2)
+ assert fp_features.shape == torch.Size([1, 16, 50])
diff --git a/tests/test_models/test_common_modules/test_sparse_unet.py b/tests/test_models/test_common_modules/test_sparse_unet.py
new file mode 100644
index 0000000..1b7eff9
--- /dev/null
+++ b/tests/test_models/test_common_modules/test_sparse_unet.py
@@ -0,0 +1,145 @@
+# Copyright (c) OpenMMLab. All rights reserved.
+import pytest
+import torch
+from mmcv.ops import (SparseConv3d, SparseConvTensor, SparseInverseConv3d,
+ SubMConv3d)
+
+from mmdet3d.ops import SparseBasicBlock
+
+
+def test_SparseUNet():
+ if not torch.cuda.is_available():
+ pytest.skip('test requires GPU and torch+cuda')
+ from mmdet3d.models.middle_encoders.sparse_unet import SparseUNet
+ self = SparseUNet(in_channels=4, sparse_shape=[41, 1600, 1408]).cuda()
+
+ # test encoder layers
+ assert len(self.encoder_layers) == 4
+ assert self.encoder_layers.encoder_layer1[0][0].in_channels == 16
+ assert self.encoder_layers.encoder_layer1[0][0].out_channels == 16
+ assert isinstance(self.encoder_layers.encoder_layer1[0][0], SubMConv3d)
+ assert isinstance(self.encoder_layers.encoder_layer1[0][1],
+ torch.nn.modules.batchnorm.BatchNorm1d)
+ assert isinstance(self.encoder_layers.encoder_layer1[0][2],
+ torch.nn.modules.activation.ReLU)
+ assert self.encoder_layers.encoder_layer4[0][0].in_channels == 64
+ assert self.encoder_layers.encoder_layer4[0][0].out_channels == 64
+ assert isinstance(self.encoder_layers.encoder_layer4[0][0], SparseConv3d)
+ assert isinstance(self.encoder_layers.encoder_layer4[2][0], SubMConv3d)
+
+ # test decoder layers
+ assert isinstance(self.lateral_layer1, SparseBasicBlock)
+ assert isinstance(self.merge_layer1[0], SubMConv3d)
+ assert isinstance(self.upsample_layer1[0], SubMConv3d)
+ assert isinstance(self.upsample_layer2[0], SparseInverseConv3d)
+
+ voxel_features = torch.tensor(
+ [[6.56126, 0.9648336, -1.7339306, 0.315],
+ [6.8162713, -2.480431, -1.3616394, 0.36],
+ [11.643568, -4.744306, -1.3580885, 0.16],
+ [23.482342, 6.5036807, 0.5806964, 0.35]],
+ dtype=torch.float32).cuda() # n, point_features
+ coordinates = torch.tensor(
+ [[0, 12, 819, 131], [0, 16, 750, 136], [1, 16, 705, 232],
+ [1, 35, 930, 469]],
+ dtype=torch.int32).cuda() # n, 4(batch, ind_x, ind_y, ind_z)
+
+ unet_ret_dict = self.forward(voxel_features, coordinates, 2)
+ seg_features = unet_ret_dict['seg_features']
+ spatial_features = unet_ret_dict['spatial_features']
+
+ assert seg_features.shape == torch.Size([4, 16])
+ assert spatial_features.shape == torch.Size([2, 256, 200, 176])
+
+
+def test_SparseBasicBlock():
+ if not torch.cuda.is_available():
+ pytest.skip('test requires GPU and torch+cuda')
+ voxel_features = torch.tensor(
+ [[6.56126, 0.9648336, -1.7339306, 0.315],
+ [6.8162713, -2.480431, -1.3616394, 0.36],
+ [11.643568, -4.744306, -1.3580885, 0.16],
+ [23.482342, 6.5036807, 0.5806964, 0.35]],
+ dtype=torch.float32).cuda() # n, point_features
+ coordinates = torch.tensor(
+ [[0, 12, 819, 131], [0, 16, 750, 136], [1, 16, 705, 232],
+ [1, 35, 930, 469]],
+ dtype=torch.int32).cuda() # n, 4(batch, ind_x, ind_y, ind_z)
+
+ # test
+ input_sp_tensor = SparseConvTensor(voxel_features, coordinates,
+ [41, 1600, 1408], 2)
+ self = SparseBasicBlock(
+ 4,
+ 4,
+ conv_cfg=dict(type='SubMConv3d', indice_key='subm1'),
+ norm_cfg=dict(type='BN1d', eps=1e-3, momentum=0.01)).cuda()
+ # test conv and bn layer
+ assert isinstance(self.conv1, SubMConv3d)
+ assert self.conv1.in_channels == 4
+ assert self.conv1.out_channels == 4
+ assert isinstance(self.conv2, SubMConv3d)
+ assert self.conv2.out_channels == 4
+ assert self.conv2.out_channels == 4
+ assert self.bn1.eps == 1e-3
+ assert self.bn1.momentum == 0.01
+
+ out_features = self(input_sp_tensor)
+ assert out_features.features.shape == torch.Size([4, 4])
+
+
+def test_make_sparse_convmodule():
+ if not torch.cuda.is_available():
+ pytest.skip('test requires GPU and torch+cuda')
+ from mmdet3d.ops import make_sparse_convmodule
+
+ voxel_features = torch.tensor(
+ [[6.56126, 0.9648336, -1.7339306, 0.315],
+ [6.8162713, -2.480431, -1.3616394, 0.36],
+ [11.643568, -4.744306, -1.3580885, 0.16],
+ [23.482342, 6.5036807, 0.5806964, 0.35]],
+ dtype=torch.float32).cuda() # n, point_features
+ coordinates = torch.tensor(
+ [[0, 12, 819, 131], [0, 16, 750, 136], [1, 16, 705, 232],
+ [1, 35, 930, 469]],
+ dtype=torch.int32).cuda() # n, 4(batch, ind_x, ind_y, ind_z)
+
+ # test
+ input_sp_tensor = SparseConvTensor(voxel_features, coordinates,
+ [41, 1600, 1408], 2)
+
+ sparse_block0 = make_sparse_convmodule(
+ 4,
+ 16,
+ 3,
+ 'test0',
+ stride=1,
+ padding=0,
+ conv_type='SubMConv3d',
+ norm_cfg=dict(type='BN1d', eps=1e-3, momentum=0.01),
+ order=('conv', 'norm', 'act')).cuda()
+ assert isinstance(sparse_block0[0], SubMConv3d)
+ assert sparse_block0[0].in_channels == 4
+ assert sparse_block0[0].out_channels == 16
+ assert isinstance(sparse_block0[1], torch.nn.BatchNorm1d)
+ assert sparse_block0[1].eps == 0.001
+ assert sparse_block0[1].momentum == 0.01
+ assert isinstance(sparse_block0[2], torch.nn.ReLU)
+
+ # test forward
+ out_features = sparse_block0(input_sp_tensor)
+ assert out_features.features.shape == torch.Size([4, 16])
+
+ sparse_block1 = make_sparse_convmodule(
+ 4,
+ 16,
+ 3,
+ 'test1',
+ stride=1,
+ padding=0,
+ conv_type='SparseInverseConv3d',
+ norm_cfg=dict(type='BN1d', eps=1e-3, momentum=0.01),
+ order=('norm', 'act', 'conv'))
+ assert isinstance(sparse_block1[0], torch.nn.BatchNorm1d)
+ assert isinstance(sparse_block1[1], torch.nn.ReLU)
+ assert isinstance(sparse_block1[2], SparseInverseConv3d)
diff --git a/tests/test_models/test_common_modules/test_vote_module.py b/tests/test_models/test_common_modules/test_vote_module.py
new file mode 100644
index 0000000..30e6b93
--- /dev/null
+++ b/tests/test_models/test_common_modules/test_vote_module.py
@@ -0,0 +1,39 @@
+# Copyright (c) OpenMMLab. All rights reserved.
+import torch
+
+
+def test_vote_module():
+ from mmdet3d.models.model_utils import VoteModule
+
+ vote_loss = dict(
+ type='ChamferDistance',
+ mode='l1',
+ reduction='none',
+ loss_dst_weight=10.0)
+ self = VoteModule(vote_per_seed=3, in_channels=8, vote_loss=vote_loss)
+
+ seed_xyz = torch.rand([2, 64, 3], dtype=torch.float32) # (b, npoints, 3)
+ seed_features = torch.rand(
+ [2, 8, 64], dtype=torch.float32) # (b, in_channels, npoints)
+
+ # test forward
+ vote_xyz, vote_features, vote_offset = self(seed_xyz, seed_features)
+ assert vote_xyz.shape == torch.Size([2, 192, 3])
+ assert vote_features.shape == torch.Size([2, 8, 192])
+ assert vote_offset.shape == torch.Size([2, 3, 192])
+
+ # test clip offset and without feature residual
+ self = VoteModule(
+ vote_per_seed=1,
+ in_channels=8,
+ num_points=32,
+ with_res_feat=False,
+ vote_xyz_range=(2.0, 2.0, 2.0))
+
+ vote_xyz, vote_features, vote_offset = self(seed_xyz, seed_features)
+ assert vote_xyz.shape == torch.Size([2, 32, 3])
+ assert vote_features.shape == torch.Size([2, 8, 32])
+ assert vote_offset.shape == torch.Size([2, 3, 32])
+ assert torch.allclose(seed_features[..., :32], vote_features)
+ assert vote_offset.max() <= 2.0
+ assert vote_offset.min() >= -2.0
diff --git a/tests/test_models/test_detectors.py b/tests/test_models/test_detectors.py
new file mode 100644
index 0000000..155e569
--- /dev/null
+++ b/tests/test_models/test_detectors.py
@@ -0,0 +1,608 @@
+# Copyright (c) OpenMMLab. All rights reserved.
+import copy
+import random
+from os.path import dirname, exists, join
+
+import numpy as np
+import pytest
+import torch
+
+from mmdet3d.core.bbox import (CameraInstance3DBoxes, DepthInstance3DBoxes,
+ LiDARInstance3DBoxes)
+from mmdet3d.models.builder import build_detector
+
+
+def _setup_seed(seed):
+ torch.manual_seed(seed)
+ torch.cuda.manual_seed_all(seed)
+ np.random.seed(seed)
+ random.seed(seed)
+ torch.backends.cudnn.deterministic = True
+
+
+def _get_config_directory():
+ """Find the predefined detector config directory."""
+ try:
+ # Assume we are running in the source mmdetection3d repo
+ repo_dpath = dirname(dirname(dirname(__file__)))
+ except NameError:
+ # For IPython development when this __file__ is not defined
+ import mmdet3d
+ repo_dpath = dirname(dirname(mmdet3d.__file__))
+ config_dpath = join(repo_dpath, 'configs')
+ if not exists(config_dpath):
+ raise Exception('Cannot find config path')
+ return config_dpath
+
+
+def _get_config_module(fname):
+ """Load a configuration as a python module."""
+ from mmcv import Config
+ config_dpath = _get_config_directory()
+ config_fpath = join(config_dpath, fname)
+ config_mod = Config.fromfile(config_fpath)
+ return config_mod
+
+
+def _get_model_cfg(fname):
+ """Grab configs necessary to create a model.
+
+ These are deep copied to allow for safe modification of parameters without
+ influencing other tests.
+ """
+ config = _get_config_module(fname)
+ model = copy.deepcopy(config.model)
+
+ return model
+
+
+def _get_detector_cfg(fname):
+ """Grab configs necessary to create a detector.
+
+ These are deep copied to allow for safe modification of parameters without
+ influencing other tests.
+ """
+ import mmcv
+ config = _get_config_module(fname)
+ model = copy.deepcopy(config.model)
+ train_cfg = mmcv.Config(copy.deepcopy(config.model.train_cfg))
+ test_cfg = mmcv.Config(copy.deepcopy(config.model.test_cfg))
+
+ model.update(train_cfg=train_cfg)
+ model.update(test_cfg=test_cfg)
+ return model
+
+
+def test_get_dynamic_voxelnet():
+ if not torch.cuda.is_available():
+ pytest.skip('test requires GPU and torch+cuda')
+
+ dynamic_voxelnet_cfg = _get_model_cfg(
+ 'dynamic_voxelization/dv_second_secfpn_6x8_80e_kitti-3d-car.py')
+ self = build_detector(dynamic_voxelnet_cfg).cuda()
+ points_0 = torch.rand([2010, 4], device='cuda')
+ points_1 = torch.rand([2020, 4], device='cuda')
+ points = [points_0, points_1]
+ feats = self.extract_feat(points, None)
+ assert feats[0].shape == torch.Size([2, 512, 200, 176])
+
+
+def test_voxel_net():
+ if not torch.cuda.is_available():
+ pytest.skip('test requires GPU and torch+cuda')
+ _setup_seed(0)
+ voxel_net_cfg = _get_detector_cfg(
+ 'second/hv_second_secfpn_6x8_80e_kitti-3d-3class.py')
+
+ self = build_detector(voxel_net_cfg).cuda()
+ points_0 = torch.rand([2010, 4], device='cuda')
+ points_1 = torch.rand([2020, 4], device='cuda')
+ points = [points_0, points_1]
+ gt_bbox_0 = LiDARInstance3DBoxes(torch.rand([10, 7], device='cuda'))
+ gt_bbox_1 = LiDARInstance3DBoxes(torch.rand([10, 7], device='cuda'))
+ gt_bboxes = [gt_bbox_0, gt_bbox_1]
+ gt_labels_0 = torch.randint(0, 3, [10], device='cuda')
+ gt_labels_1 = torch.randint(0, 3, [10], device='cuda')
+ gt_labels = [gt_labels_0, gt_labels_1]
+ img_meta_0 = dict(box_type_3d=LiDARInstance3DBoxes)
+ img_meta_1 = dict(box_type_3d=LiDARInstance3DBoxes)
+ img_metas = [img_meta_0, img_meta_1]
+
+ # test forward_train
+ losses = self.forward_train(points, img_metas, gt_bboxes, gt_labels)
+ assert losses['loss_cls'][0] >= 0
+ assert losses['loss_bbox'][0] >= 0
+ assert losses['loss_dir'][0] >= 0
+
+ # test simple_test
+ with torch.no_grad():
+ results = self.simple_test(points, img_metas)
+ boxes_3d = results[0]['boxes_3d']
+ scores_3d = results[0]['scores_3d']
+ labels_3d = results[0]['labels_3d']
+ assert boxes_3d.tensor.shape == (50, 7)
+ assert scores_3d.shape == torch.Size([50])
+ assert labels_3d.shape == torch.Size([50])
+
+
+def test_3dssd():
+ if not torch.cuda.is_available():
+ pytest.skip('test requires GPU and torch+cuda')
+ _setup_seed(0)
+ ssd3d_cfg = _get_detector_cfg('3dssd/3dssd_4x4_kitti-3d-car.py')
+ self = build_detector(ssd3d_cfg).cuda()
+ points_0 = torch.rand([2000, 4], device='cuda')
+ points_1 = torch.rand([2000, 4], device='cuda')
+ points = [points_0, points_1]
+ img_meta_0 = dict(box_type_3d=DepthInstance3DBoxes)
+ img_meta_1 = dict(box_type_3d=DepthInstance3DBoxes)
+ img_metas = [img_meta_0, img_meta_1]
+ gt_bbox_0 = DepthInstance3DBoxes(torch.rand([10, 7], device='cuda'))
+ gt_bbox_1 = DepthInstance3DBoxes(torch.rand([10, 7], device='cuda'))
+ gt_bboxes = [gt_bbox_0, gt_bbox_1]
+ gt_labels_0 = torch.zeros([10], device='cuda').long()
+ gt_labels_1 = torch.zeros([10], device='cuda').long()
+ gt_labels = [gt_labels_0, gt_labels_1]
+
+ # test forward_train
+ losses = self.forward_train(points, img_metas, gt_bboxes, gt_labels)
+ assert losses['vote_loss'] >= 0
+ assert losses['centerness_loss'] >= 0
+ assert losses['center_loss'] >= 0
+ assert losses['dir_class_loss'] >= 0
+ assert losses['dir_res_loss'] >= 0
+ assert losses['corner_loss'] >= 0
+ assert losses['size_res_loss'] >= 0
+
+ # test simple_test
+ with torch.no_grad():
+ results = self.simple_test(points, img_metas)
+ boxes_3d = results[0]['boxes_3d']
+ scores_3d = results[0]['scores_3d']
+ labels_3d = results[0]['labels_3d']
+ assert boxes_3d.tensor.shape[0] >= 0
+ assert boxes_3d.tensor.shape[1] == 7
+ assert scores_3d.shape[0] >= 0
+ assert labels_3d.shape[0] >= 0
+
+
+def test_vote_net():
+ if not torch.cuda.is_available():
+ pytest.skip('test requires GPU and torch+cuda')
+
+ _setup_seed(0)
+ vote_net_cfg = _get_detector_cfg(
+ 'votenet/votenet_16x8_sunrgbd-3d-10class.py')
+ self = build_detector(vote_net_cfg).cuda()
+ points_0 = torch.rand([2000, 4], device='cuda')
+ points_1 = torch.rand([2000, 4], device='cuda')
+ points = [points_0, points_1]
+ img_meta_0 = dict(box_type_3d=DepthInstance3DBoxes)
+ img_meta_1 = dict(box_type_3d=DepthInstance3DBoxes)
+ img_metas = [img_meta_0, img_meta_1]
+ gt_bbox_0 = DepthInstance3DBoxes(torch.rand([10, 7], device='cuda'))
+ gt_bbox_1 = DepthInstance3DBoxes(torch.rand([10, 7], device='cuda'))
+ gt_bboxes = [gt_bbox_0, gt_bbox_1]
+ gt_labels_0 = torch.randint(0, 10, [10], device='cuda')
+ gt_labels_1 = torch.randint(0, 10, [10], device='cuda')
+ gt_labels = [gt_labels_0, gt_labels_1]
+
+ # test forward_train
+ losses = self.forward_train(points, img_metas, gt_bboxes, gt_labels)
+ assert losses['vote_loss'] >= 0
+ assert losses['objectness_loss'] >= 0
+ assert losses['semantic_loss'] >= 0
+ assert losses['center_loss'] >= 0
+ assert losses['dir_class_loss'] >= 0
+ assert losses['dir_res_loss'] >= 0
+ assert losses['size_class_loss'] >= 0
+ assert losses['size_res_loss'] >= 0
+
+ # test simple_test
+ with torch.no_grad():
+ results = self.simple_test(points, img_metas)
+ boxes_3d = results[0]['boxes_3d']
+ scores_3d = results[0]['scores_3d']
+ labels_3d = results[0]['labels_3d']
+ assert boxes_3d.tensor.shape[0] >= 0
+ assert boxes_3d.tensor.shape[1] == 7
+ assert scores_3d.shape[0] >= 0
+ assert labels_3d.shape[0] >= 0
+
+
+def test_parta2():
+ if not torch.cuda.is_available():
+ pytest.skip('test requires GPU and torch+cuda')
+ _setup_seed(0)
+ parta2 = _get_detector_cfg(
+ 'parta2/hv_PartA2_secfpn_2x8_cyclic_80e_kitti-3d-3class.py')
+ self = build_detector(parta2).cuda()
+ points_0 = torch.rand([1000, 4], device='cuda')
+ points_1 = torch.rand([1000, 4], device='cuda')
+ points = [points_0, points_1]
+ img_meta_0 = dict(box_type_3d=LiDARInstance3DBoxes)
+ img_meta_1 = dict(box_type_3d=LiDARInstance3DBoxes)
+ img_metas = [img_meta_0, img_meta_1]
+ gt_bbox_0 = LiDARInstance3DBoxes(torch.rand([10, 7], device='cuda'))
+ gt_bbox_1 = LiDARInstance3DBoxes(torch.rand([10, 7], device='cuda'))
+ gt_bboxes = [gt_bbox_0, gt_bbox_1]
+ gt_labels_0 = torch.randint(0, 3, [10], device='cuda')
+ gt_labels_1 = torch.randint(0, 3, [10], device='cuda')
+ gt_labels = [gt_labels_0, gt_labels_1]
+
+ # test_forward_train
+ losses = self.forward_train(points, img_metas, gt_bboxes, gt_labels)
+ assert losses['loss_rpn_cls'][0] >= 0
+ assert losses['loss_rpn_bbox'][0] >= 0
+ assert losses['loss_rpn_dir'][0] >= 0
+ assert losses['loss_seg'] >= 0
+ assert losses['loss_part'] >= 0
+ assert losses['loss_cls'] >= 0
+ assert losses['loss_bbox'] >= 0
+ assert losses['loss_corner'] >= 0
+
+ # test_simple_test
+ with torch.no_grad():
+ results = self.simple_test(points, img_metas)
+ boxes_3d = results[0]['boxes_3d']
+ scores_3d = results[0]['scores_3d']
+ labels_3d = results[0]['labels_3d']
+ assert boxes_3d.tensor.shape[0] >= 0
+ assert boxes_3d.tensor.shape[1] == 7
+ assert scores_3d.shape[0] >= 0
+ assert labels_3d.shape[0] >= 0
+
+
+def test_centerpoint():
+ if not torch.cuda.is_available():
+ pytest.skip('test requires GPU and torch+cuda')
+ centerpoint = _get_detector_cfg(
+ 'centerpoint/centerpoint_0075voxel_second_secfpn_'
+ 'dcn_4x8_cyclic_flip-tta_20e_nus.py')
+ self = build_detector(centerpoint).cuda()
+ points_0 = torch.rand([1000, 5], device='cuda')
+ points_1 = torch.rand([1000, 5], device='cuda')
+ points = [points_0, points_1]
+ img_meta_0 = dict(
+ box_type_3d=LiDARInstance3DBoxes,
+ flip=True,
+ pcd_horizontal_flip=True,
+ pcd_vertical_flip=False)
+ img_meta_1 = dict(
+ box_type_3d=LiDARInstance3DBoxes,
+ flip=True,
+ pcd_horizontal_flip=False,
+ pcd_vertical_flip=True)
+ img_metas = [img_meta_0, img_meta_1]
+ gt_bbox_0 = LiDARInstance3DBoxes(
+ torch.rand([10, 9], device='cuda'), box_dim=9)
+ gt_bbox_1 = LiDARInstance3DBoxes(
+ torch.rand([10, 9], device='cuda'), box_dim=9)
+ gt_bboxes = [gt_bbox_0, gt_bbox_1]
+ gt_labels_0 = torch.randint(0, 3, [10], device='cuda')
+ gt_labels_1 = torch.randint(0, 3, [10], device='cuda')
+ gt_labels = [gt_labels_0, gt_labels_1]
+
+ # test_forward_train
+ losses = self.forward_train(points, img_metas, gt_bboxes, gt_labels)
+ for key, value in losses.items():
+ assert value >= 0
+
+ # test_simple_test
+ with torch.no_grad():
+ results = self.simple_test(points, img_metas)
+ boxes_3d_0 = results[0]['pts_bbox']['boxes_3d']
+ scores_3d_0 = results[0]['pts_bbox']['scores_3d']
+ labels_3d_0 = results[0]['pts_bbox']['labels_3d']
+ assert boxes_3d_0.tensor.shape[0] >= 0
+ assert boxes_3d_0.tensor.shape[1] == 9
+ assert scores_3d_0.shape[0] >= 0
+ assert labels_3d_0.shape[0] >= 0
+ boxes_3d_1 = results[1]['pts_bbox']['boxes_3d']
+ scores_3d_1 = results[1]['pts_bbox']['scores_3d']
+ labels_3d_1 = results[1]['pts_bbox']['labels_3d']
+ assert boxes_3d_1.tensor.shape[0] >= 0
+ assert boxes_3d_1.tensor.shape[1] == 9
+ assert scores_3d_1.shape[0] >= 0
+ assert labels_3d_1.shape[0] >= 0
+
+ # test_aug_test
+ points = [[torch.rand([1000, 5], device='cuda')]]
+ img_metas = [[
+ dict(
+ box_type_3d=LiDARInstance3DBoxes,
+ pcd_scale_factor=1.0,
+ flip=True,
+ pcd_horizontal_flip=True,
+ pcd_vertical_flip=False)
+ ]]
+ with torch.no_grad():
+ results = self.aug_test(points, img_metas)
+ boxes_3d_0 = results[0]['pts_bbox']['boxes_3d']
+ scores_3d_0 = results[0]['pts_bbox']['scores_3d']
+ labels_3d_0 = results[0]['pts_bbox']['labels_3d']
+ assert boxes_3d_0.tensor.shape[0] >= 0
+ assert boxes_3d_0.tensor.shape[1] == 9
+ assert scores_3d_0.shape[0] >= 0
+ assert labels_3d_0.shape[0] >= 0
+
+
+def test_fcos3d():
+ if not torch.cuda.is_available():
+ pytest.skip('test requires GPU and torch+cuda')
+
+ _setup_seed(0)
+ fcos3d_cfg = _get_detector_cfg(
+ 'fcos3d/fcos3d_r101_caffe_fpn_gn-head_dcn_2x8_1x_nus-mono3d.py')
+ self = build_detector(fcos3d_cfg).cuda()
+ imgs = torch.rand([1, 3, 928, 1600], dtype=torch.float32).cuda()
+ gt_bboxes = [torch.rand([3, 4], dtype=torch.float32).cuda()]
+ gt_bboxes_3d = CameraInstance3DBoxes(
+ torch.rand([3, 9], device='cuda'), box_dim=9)
+ gt_labels = [torch.randint(0, 10, [3], device='cuda')]
+ gt_labels_3d = gt_labels
+ centers2d = [torch.rand([3, 2], dtype=torch.float32).cuda()]
+ depths = [torch.rand([3], dtype=torch.float32).cuda()]
+ attr_labels = [torch.randint(0, 9, [3], device='cuda')]
+ img_metas = [
+ dict(
+ cam2img=[[1260.8474446004698, 0.0, 807.968244525554],
+ [0.0, 1260.8474446004698, 495.3344268742088],
+ [0.0, 0.0, 1.0]],
+ scale_factor=np.array([1., 1., 1., 1.], dtype=np.float32),
+ box_type_3d=CameraInstance3DBoxes)
+ ]
+
+ # test forward_train
+ losses = self.forward_train(imgs, img_metas, gt_bboxes, gt_labels,
+ gt_bboxes_3d, gt_labels_3d, centers2d, depths,
+ attr_labels)
+ assert losses['loss_cls'] >= 0
+ assert losses['loss_offset'] >= 0
+ assert losses['loss_depth'] >= 0
+ assert losses['loss_size'] >= 0
+ assert losses['loss_rotsin'] >= 0
+ assert losses['loss_centerness'] >= 0
+ assert losses['loss_velo'] >= 0
+ assert losses['loss_dir'] >= 0
+ assert losses['loss_attr'] >= 0
+
+ # test simple_test
+ with torch.no_grad():
+ results = self.simple_test(imgs, img_metas)
+ boxes_3d = results[0]['img_bbox']['boxes_3d']
+ scores_3d = results[0]['img_bbox']['scores_3d']
+ labels_3d = results[0]['img_bbox']['labels_3d']
+ attrs_3d = results[0]['img_bbox']['attrs_3d']
+ assert boxes_3d.tensor.shape[0] >= 0
+ assert boxes_3d.tensor.shape[1] == 9
+ assert scores_3d.shape[0] >= 0
+ assert labels_3d.shape[0] >= 0
+ assert attrs_3d.shape[0] >= 0
+
+
+def test_groupfree3dnet():
+ if not torch.cuda.is_available():
+ pytest.skip('test requires GPU and torch+cuda')
+
+ _setup_seed(0)
+ groupfree3d_cfg = _get_detector_cfg(
+ 'groupfree3d/groupfree3d_8x4_scannet-3d-18class-L6-O256.py')
+ self = build_detector(groupfree3d_cfg).cuda()
+
+ points_0 = torch.rand([50000, 3], device='cuda')
+ points_1 = torch.rand([50000, 3], device='cuda')
+ points = [points_0, points_1]
+ img_meta_0 = dict(box_type_3d=DepthInstance3DBoxes)
+ img_meta_1 = dict(box_type_3d=DepthInstance3DBoxes)
+ img_metas = [img_meta_0, img_meta_1]
+ gt_bbox_0 = DepthInstance3DBoxes(torch.rand([10, 7], device='cuda'))
+ gt_bbox_1 = DepthInstance3DBoxes(torch.rand([10, 7], device='cuda'))
+ gt_bboxes = [gt_bbox_0, gt_bbox_1]
+ gt_labels_0 = torch.randint(0, 18, [10], device='cuda')
+ gt_labels_1 = torch.randint(0, 18, [10], device='cuda')
+ gt_labels = [gt_labels_0, gt_labels_1]
+ pts_instance_mask_1 = torch.randint(0, 10, [50000], device='cuda')
+ pts_instance_mask_2 = torch.randint(0, 10, [50000], device='cuda')
+ pts_instance_mask = [pts_instance_mask_1, pts_instance_mask_2]
+ pts_semantic_mask_1 = torch.randint(0, 19, [50000], device='cuda')
+ pts_semantic_mask_2 = torch.randint(0, 19, [50000], device='cuda')
+ pts_semantic_mask = [pts_semantic_mask_1, pts_semantic_mask_2]
+
+ # test forward_train
+ losses = self.forward_train(points, img_metas, gt_bboxes, gt_labels,
+ pts_semantic_mask, pts_instance_mask)
+
+ assert losses['sampling_objectness_loss'] >= 0
+ assert losses['s5.objectness_loss'] >= 0
+ assert losses['s5.semantic_loss'] >= 0
+ assert losses['s5.center_loss'] >= 0
+ assert losses['s5.dir_class_loss'] >= 0
+ assert losses['s5.dir_res_loss'] >= 0
+ assert losses['s5.size_class_loss'] >= 0
+ assert losses['s5.size_res_loss'] >= 0
+
+ # test simple_test
+ with torch.no_grad():
+ results = self.simple_test(points, img_metas)
+ boxes_3d = results[0]['boxes_3d']
+ scores_3d = results[0]['scores_3d']
+ labels_3d = results[0]['labels_3d']
+ assert boxes_3d.tensor.shape[0] >= 0
+ assert boxes_3d.tensor.shape[1] == 7
+ assert scores_3d.shape[0] >= 0
+ assert labels_3d.shape[0] >= 0
+
+
+def test_imvoxelnet():
+ if not torch.cuda.is_available():
+ pytest.skip('test requires GPU and torch+cuda')
+
+ imvoxelnet_cfg = _get_detector_cfg(
+ 'imvoxelnet/imvoxelnet_4x8_kitti-3d-car.py')
+ self = build_detector(imvoxelnet_cfg).cuda()
+ imgs = torch.rand([1, 3, 384, 1280], dtype=torch.float32).cuda()
+ gt_bboxes_3d = [LiDARInstance3DBoxes(torch.rand([3, 7], device='cuda'))]
+ gt_labels_3d = [torch.zeros([3], dtype=torch.long, device='cuda')]
+ img_metas = [
+ dict(
+ box_type_3d=LiDARInstance3DBoxes,
+ lidar2img=np.array([[6.0e+02, -7.2e+02, -1.2e+00, -1.2e+02],
+ [1.8e+02, 7.6e+00, -7.1e+02, -1.0e+02],
+ [9.9e-01, 1.2e-04, 1.0e-02, -2.6e-01],
+ [0.0e+00, 0.0e+00, 0.0e+00, 1.0e+00]],
+ dtype=np.float32),
+ img_shape=(384, 1272, 3))
+ ]
+
+ # test forward_train
+ losses = self.forward_train(imgs, img_metas, gt_bboxes_3d, gt_labels_3d)
+ assert losses['loss_cls'][0] >= 0
+ assert losses['loss_bbox'][0] >= 0
+ assert losses['loss_dir'][0] >= 0
+
+ # test simple_test
+ with torch.no_grad():
+ results = self.simple_test(imgs, img_metas)
+ boxes_3d = results[0]['boxes_3d']
+ scores_3d = results[0]['scores_3d']
+ labels_3d = results[0]['labels_3d']
+ assert boxes_3d.tensor.shape[0] >= 0
+ assert boxes_3d.tensor.shape[1] == 7
+ assert scores_3d.shape[0] >= 0
+ assert labels_3d.shape[0] >= 0
+
+
+def test_point_rcnn():
+ if not torch.cuda.is_available():
+ pytest.skip('test requires GPU and torch+cuda')
+ pointrcnn_cfg = _get_detector_cfg(
+ 'point_rcnn/point_rcnn_2x8_kitti-3d-3classes.py')
+ self = build_detector(pointrcnn_cfg).cuda()
+ points_0 = torch.rand([1000, 4], device='cuda')
+ points_1 = torch.rand([1000, 4], device='cuda')
+ points = [points_0, points_1]
+
+ img_meta_0 = dict(box_type_3d=LiDARInstance3DBoxes)
+ img_meta_1 = dict(box_type_3d=LiDARInstance3DBoxes)
+ img_metas = [img_meta_0, img_meta_1]
+ gt_bbox_0 = LiDARInstance3DBoxes(torch.rand([10, 7], device='cuda'))
+ gt_bbox_1 = LiDARInstance3DBoxes(torch.rand([10, 7], device='cuda'))
+ gt_bboxes = [gt_bbox_0, gt_bbox_1]
+ gt_labels_0 = torch.randint(0, 3, [10], device='cuda')
+ gt_labels_1 = torch.randint(0, 3, [10], device='cuda')
+ gt_labels = [gt_labels_0, gt_labels_1]
+
+ # test_forward_train
+ losses = self.forward_train(points, img_metas, gt_bboxes, gt_labels)
+ assert losses['bbox_loss'] >= 0
+ assert losses['semantic_loss'] >= 0
+ assert losses['loss_cls'] >= 0
+ assert losses['loss_bbox'] >= 0
+ assert losses['loss_corner'] >= 0
+
+
+def test_smoke():
+ if not torch.cuda.is_available():
+ pytest.skip('test requires GPU and torch+cuda')
+
+ _setup_seed(0)
+ smoke_cfg = _get_detector_cfg(
+ 'smoke/smoke_dla34_pytorch_dlaneck_gn-all_8x4_6x_kitti-mono3d.py')
+ self = build_detector(smoke_cfg).cuda()
+ imgs = torch.rand([1, 3, 384, 1280], dtype=torch.float32).cuda()
+ gt_bboxes = [
+ torch.Tensor([[563.63122442, 175.02195182, 614.81298184, 224.97763099],
+ [480.89676358, 179.86272635, 511.53017463, 202.54645962],
+ [541.48322272, 175.73767011, 564.55208966, 193.95009791],
+ [329.51448848, 176.14566789, 354.24670848,
+ 213.82599081]]).cuda()
+ ]
+ gt_bboxes_3d = [
+ CameraInstance3DBoxes(
+ torch.Tensor([[-0.69, 1.69, 25.01, 3.20, 1.61, 1.66, -1.59],
+ [-7.43, 1.88, 47.55, 3.70, 1.40, 1.51, 1.55],
+ [-4.71, 1.71, 60.52, 4.05, 1.46, 1.66, 1.56],
+ [-12.63, 1.88, 34.09, 1.95, 1.72, 0.50,
+ 1.54]]).cuda(),
+ box_dim=7)
+ ]
+ gt_labels = [torch.tensor([0, 0, 0, 1]).cuda()]
+ gt_labels_3d = gt_labels
+ centers2d = [
+ torch.Tensor([[589.6528477, 198.3862263], [496.8143155, 190.75967182],
+ [553.40528354, 184.53785991],
+ [342.23690317, 194.44298819]]).cuda()
+ ]
+ # depths is actually not used in smoke head loss computation
+ depths = [torch.rand([3], dtype=torch.float32).cuda()]
+ attr_labels = None
+ img_metas = [
+ dict(
+ cam2img=[[721.5377, 0., 609.5593, 0.], [0., 721.5377, 172.854, 0.],
+ [0., 0., 1., 0.], [0., 0., 0., 1.]],
+ scale_factor=np.array([1., 1., 1., 1.], dtype=np.float32),
+ pad_shape=[384, 1280],
+ trans_mat=np.array([[0.25, 0., 0.], [0., 0.25, 0], [0., 0., 1.]],
+ dtype=np.float32),
+ affine_aug=False,
+ box_type_3d=CameraInstance3DBoxes)
+ ]
+
+ # test forward_train
+ losses = self.forward_train(imgs, img_metas, gt_bboxes, gt_labels,
+ gt_bboxes_3d, gt_labels_3d, centers2d, depths,
+ attr_labels)
+
+ assert losses['loss_cls'] >= 0
+ assert losses['loss_bbox'] >= 0
+
+ # test simple_test
+ with torch.no_grad():
+ results = self.simple_test(imgs, img_metas)
+ boxes_3d = results[0]['img_bbox']['boxes_3d']
+ scores_3d = results[0]['img_bbox']['scores_3d']
+ labels_3d = results[0]['img_bbox']['labels_3d']
+ assert boxes_3d.tensor.shape[0] >= 0
+ assert boxes_3d.tensor.shape[1] == 7
+ assert scores_3d.shape[0] >= 0
+ assert labels_3d.shape[0] >= 0
+
+
+def test_sassd():
+ if not torch.cuda.is_available():
+ pytest.skip('test requires GPU and torch+cuda')
+ _setup_seed(0)
+ sassd_cfg = _get_detector_cfg('sassd/sassd_6x8_80e_kitti-3d-3class.py')
+
+ self = build_detector(sassd_cfg).cuda()
+ points_0 = torch.rand([2010, 4], device='cuda')
+ points_1 = torch.rand([2020, 4], device='cuda')
+ points = [points_0, points_1]
+ gt_bbox_0 = LiDARInstance3DBoxes(torch.rand([10, 7], device='cuda'))
+ gt_bbox_1 = LiDARInstance3DBoxes(torch.rand([10, 7], device='cuda'))
+ gt_bboxes = [gt_bbox_0, gt_bbox_1]
+ gt_labels_0 = torch.randint(0, 3, [10], device='cuda')
+ gt_labels_1 = torch.randint(0, 3, [10], device='cuda')
+ gt_labels = [gt_labels_0, gt_labels_1]
+ img_meta_0 = dict(box_type_3d=LiDARInstance3DBoxes)
+ img_meta_1 = dict(box_type_3d=LiDARInstance3DBoxes)
+ img_metas = [img_meta_0, img_meta_1]
+
+ # test forward_train
+ losses = self.forward_train(points, img_metas, gt_bboxes, gt_labels)
+ assert losses['loss_cls'][0] >= 0
+ assert losses['loss_bbox'][0] >= 0
+ assert losses['loss_dir'][0] >= 0
+ assert losses['aux_loss_cls'][0] >= 0
+ assert losses['aux_loss_reg'][0] >= 0
+
+ # test simple_test
+ with torch.no_grad():
+ results = self.simple_test(points, img_metas)
+ boxes_3d = results[0]['boxes_3d']
+ scores_3d = results[0]['scores_3d']
+ labels_3d = results[0]['labels_3d']
+ assert boxes_3d.tensor.shape == (50, 7)
+ assert scores_3d.shape == torch.Size([50])
+ assert labels_3d.shape == torch.Size([50])
diff --git a/tests/test_models/test_forward.py b/tests/test_models/test_forward.py
new file mode 100644
index 0000000..d6f4f10
--- /dev/null
+++ b/tests/test_models/test_forward.py
@@ -0,0 +1,209 @@
+# Copyright (c) OpenMMLab. All rights reserved.
+"""Test model forward process.
+
+CommandLine:
+ pytest tests/test_models/test_forward.py
+ xdoctest tests/test_models/test_forward.py zero
+"""
+import copy
+from os.path import dirname, exists, join
+
+import numpy as np
+import torch
+
+
+def _get_config_directory():
+ """Find the predefined detector config directory."""
+ try:
+ # Assume we are running in the source mmdetection3d repo
+ repo_dpath = dirname(dirname(dirname(__file__)))
+ except NameError:
+ # For IPython development when this __file__ is not defined
+ import mmdet3d
+ repo_dpath = dirname(dirname(mmdet3d.__file__))
+ config_dpath = join(repo_dpath, 'configs')
+ if not exists(config_dpath):
+ raise Exception('Cannot find config path')
+ return config_dpath
+
+
+def _get_config_module(fname):
+ """Load a configuration as a python module."""
+ from mmcv import Config
+ config_dpath = _get_config_directory()
+ config_fpath = join(config_dpath, fname)
+ config_mod = Config.fromfile(config_fpath)
+ return config_mod
+
+
+def _get_detector_cfg(fname):
+ """Grab configs necessary to create a detector.
+
+ These are deep copied to allow for safe modification of parameters without
+ influencing other tests.
+ """
+ config = _get_config_module(fname)
+ model = copy.deepcopy(config.model)
+ return model
+
+
+def _test_two_stage_forward(cfg_file):
+ model = _get_detector_cfg(cfg_file)
+ model['pretrained'] = None
+
+ from mmdet.models import build_detector
+ detector = build_detector(model)
+
+ input_shape = (1, 3, 256, 256)
+
+ # Test forward train with a non-empty truth batch
+ mm_inputs = _demo_mm_inputs(input_shape, num_items=[10])
+ imgs = mm_inputs.pop('imgs')
+ img_metas = mm_inputs.pop('img_metas')
+ gt_bboxes = mm_inputs['gt_bboxes']
+ gt_labels = mm_inputs['gt_labels']
+ gt_masks = mm_inputs['gt_masks']
+ losses = detector.forward(
+ imgs,
+ img_metas,
+ gt_bboxes=gt_bboxes,
+ gt_labels=gt_labels,
+ gt_masks=gt_masks,
+ return_loss=True)
+ assert isinstance(losses, dict)
+ loss, _ = detector._parse_losses(losses)
+ loss.requires_grad_(True)
+ assert float(loss.item()) > 0
+ loss.backward()
+
+ # Test forward train with an empty truth batch
+ mm_inputs = _demo_mm_inputs(input_shape, num_items=[0])
+ imgs = mm_inputs.pop('imgs')
+ img_metas = mm_inputs.pop('img_metas')
+ gt_bboxes = mm_inputs['gt_bboxes']
+ gt_labels = mm_inputs['gt_labels']
+ gt_masks = mm_inputs['gt_masks']
+ losses = detector.forward(
+ imgs,
+ img_metas,
+ gt_bboxes=gt_bboxes,
+ gt_labels=gt_labels,
+ gt_masks=gt_masks,
+ return_loss=True)
+ assert isinstance(losses, dict)
+ loss, _ = detector._parse_losses(losses)
+ assert float(loss.item()) > 0
+ loss.backward()
+
+ # Test forward test
+ with torch.no_grad():
+ img_list = [g[None, :] for g in imgs]
+ batch_results = []
+ for one_img, one_meta in zip(img_list, img_metas):
+ result = detector.forward([one_img], [[one_meta]],
+ return_loss=False)
+ batch_results.append(result)
+
+
+def _test_single_stage_forward(cfg_file):
+ model = _get_detector_cfg(cfg_file)
+ model['pretrained'] = None
+
+ from mmdet.models import build_detector
+ detector = build_detector(model)
+
+ input_shape = (1, 3, 300, 300)
+ mm_inputs = _demo_mm_inputs(input_shape)
+
+ imgs = mm_inputs.pop('imgs')
+ img_metas = mm_inputs.pop('img_metas')
+
+ # Test forward train
+ gt_bboxes = mm_inputs['gt_bboxes']
+ gt_labels = mm_inputs['gt_labels']
+ losses = detector.forward(
+ imgs,
+ img_metas,
+ gt_bboxes=gt_bboxes,
+ gt_labels=gt_labels,
+ return_loss=True)
+ assert isinstance(losses, dict)
+ loss, _ = detector._parse_losses(losses)
+ assert float(loss.item()) > 0
+
+ # Test forward test
+ with torch.no_grad():
+ img_list = [g[None, :] for g in imgs]
+ batch_results = []
+ for one_img, one_meta in zip(img_list, img_metas):
+ result = detector.forward([one_img], [[one_meta]],
+ return_loss=False)
+ batch_results.append(result)
+
+
+def _demo_mm_inputs(input_shape=(1, 3, 300, 300),
+ num_items=None, num_classes=10): # yapf: disable
+ """Create a superset of inputs needed to run test or train batches.
+
+ Args:
+ input_shape (tuple):
+ input batch dimensions
+
+ num_items (List[int]):
+ specifies the number of boxes in each batch item
+
+ num_classes (int):
+ number of different labels a box might have
+ """
+ from mmdet.core import BitmapMasks
+
+ (N, C, H, W) = input_shape
+
+ rng = np.random.RandomState(0)
+
+ imgs = rng.rand(*input_shape)
+
+ img_metas = [{
+ 'img_shape': (H, W, C),
+ 'ori_shape': (H, W, C),
+ 'pad_shape': (H, W, C),
+ 'filename': '.png',
+ 'scale_factor': 1.0,
+ 'flip': False,
+ } for _ in range(N)]
+
+ gt_bboxes = []
+ gt_labels = []
+ gt_masks = []
+
+ for batch_idx in range(N):
+ if num_items is None:
+ num_boxes = rng.randint(1, 10)
+ else:
+ num_boxes = num_items[batch_idx]
+
+ cx, cy, bw, bh = rng.rand(num_boxes, 4).T
+
+ tl_x = ((cx * W) - (W * bw / 2)).clip(0, W)
+ tl_y = ((cy * H) - (H * bh / 2)).clip(0, H)
+ br_x = ((cx * W) + (W * bw / 2)).clip(0, W)
+ br_y = ((cy * H) + (H * bh / 2)).clip(0, H)
+
+ boxes = np.vstack([tl_x, tl_y, br_x, br_y]).T
+ class_idxs = rng.randint(1, num_classes, size=num_boxes)
+
+ gt_bboxes.append(torch.FloatTensor(boxes))
+ gt_labels.append(torch.LongTensor(class_idxs))
+
+ mask = np.random.randint(0, 2, (len(boxes), H, W), dtype=np.uint8)
+ gt_masks.append(BitmapMasks(mask, H, W))
+
+ mm_inputs = {
+ 'imgs': torch.FloatTensor(imgs).requires_grad_(True),
+ 'img_metas': img_metas,
+ 'gt_bboxes': gt_bboxes,
+ 'gt_labels': gt_labels,
+ 'gt_bboxes_ignore': None,
+ 'gt_masks': gt_masks,
+ }
+ return mm_inputs
diff --git a/tests/test_models/test_fusion/test_fusion_coord_trans.py b/tests/test_models/test_fusion/test_fusion_coord_trans.py
new file mode 100644
index 0000000..4df6382
--- /dev/null
+++ b/tests/test_models/test_fusion/test_fusion_coord_trans.py
@@ -0,0 +1,137 @@
+# Copyright (c) OpenMMLab. All rights reserved.
+"""Tests coords transformation in fusion modules.
+
+CommandLine:
+ pytest tests/test_models/test_fusion/test_fusion_coord_trans.py
+"""
+
+import torch
+
+from mmdet3d.models.fusion_layers import apply_3d_transformation
+
+
+def test_coords_transformation():
+ """Test the transformation of 3d coords."""
+
+ # H+R+S+T, not reverse, depth
+ img_meta = {
+ 'pcd_scale_factor':
+ 1.2311e+00,
+ 'pcd_rotation': [[8.660254e-01, 0.5, 0], [-0.5, 8.660254e-01, 0],
+ [0, 0, 1.0e+00]],
+ 'pcd_trans': [1.111e-02, -8.88e-03, 0.0],
+ 'pcd_horizontal_flip':
+ True,
+ 'transformation_3d_flow': ['HF', 'R', 'S', 'T']
+ }
+
+ pcd = torch.tensor([[-5.2422e+00, -2.9757e-01, 4.0021e+01],
+ [-9.1435e-01, 2.6675e+01, -5.5950e+00],
+ [2.0089e-01, 5.8098e+00, -3.5409e+01],
+ [-1.9461e-01, 3.1309e+01, -1.0901e+00]])
+
+ pcd_transformed = apply_3d_transformation(
+ pcd, 'DEPTH', img_meta, reverse=False)
+
+ expected_tensor = torch.tensor(
+ [[5.78332345e+00, 2.900697e+00, 4.92698531e+01],
+ [-1.5433839e+01, 2.8993850e+01, -6.8880045e+00],
+ [-3.77929405e+00, 6.061661e+00, -4.35920199e+01],
+ [-1.9053658e+01, 3.3491436e+01, -1.34202211e+00]])
+
+ assert torch.allclose(expected_tensor, pcd_transformed, 1e-4)
+
+ # H+R+S+T, reverse, depth
+ img_meta = {
+ 'pcd_scale_factor':
+ 7.07106781e-01,
+ 'pcd_rotation': [[7.07106781e-01, 7.07106781e-01, 0.0],
+ [-7.07106781e-01, 7.07106781e-01, 0.0],
+ [0.0, 0.0, 1.0e+00]],
+ 'pcd_trans': [0.0, 0.0, 0.0],
+ 'pcd_horizontal_flip':
+ False,
+ 'transformation_3d_flow': ['HF', 'R', 'S', 'T']
+ }
+
+ pcd = torch.tensor([[-5.2422e+00, -2.9757e-01, 4.0021e+01],
+ [-9.1435e+01, 2.6675e+01, -5.5950e+00],
+ [6.061661e+00, -0.0, -1.0e+02]])
+
+ pcd_transformed = apply_3d_transformation(
+ pcd, 'DEPTH', img_meta, reverse=True)
+
+ expected_tensor = torch.tensor(
+ [[-5.53977e+00, 4.94463e+00, 5.65982409e+01],
+ [-6.476e+01, 1.1811e+02, -7.91252488e+00],
+ [6.061661e+00, -6.061661e+00, -1.41421356e+02]])
+ assert torch.allclose(expected_tensor, pcd_transformed, 1e-4)
+
+ # H+R+S+T, not reverse, camera
+ img_meta = {
+ 'pcd_scale_factor':
+ 1.0 / 7.07106781e-01,
+ 'pcd_rotation': [[7.07106781e-01, 0.0, 7.07106781e-01],
+ [0.0, 1.0e+00, 0.0],
+ [-7.07106781e-01, 0.0, 7.07106781e-01]],
+ 'pcd_trans': [1.0e+00, -1.0e+00, 0.0],
+ 'pcd_horizontal_flip':
+ True,
+ 'transformation_3d_flow': ['HF', 'S', 'R', 'T']
+ }
+
+ pcd = torch.tensor([[-5.2422e+00, 4.0021e+01, -2.9757e-01],
+ [-9.1435e+01, -5.5950e+00, 2.6675e+01],
+ [6.061661e+00, -1.0e+02, -0.0]])
+
+ pcd_transformed = apply_3d_transformation(
+ pcd, 'CAMERA', img_meta, reverse=False)
+
+ expected_tensor = torch.tensor(
+ [[6.53977e+00, 5.55982409e+01, 4.94463e+00],
+ [6.576e+01, -8.91252488e+00, 1.1811e+02],
+ [-5.061661e+00, -1.42421356e+02, -6.061661e+00]])
+
+ assert torch.allclose(expected_tensor, pcd_transformed, 1e-4)
+
+ # V, reverse, camera
+ img_meta = {'pcd_vertical_flip': True, 'transformation_3d_flow': ['VF']}
+
+ pcd_transformed = apply_3d_transformation(
+ pcd, 'CAMERA', img_meta, reverse=True)
+
+ expected_tensor = torch.tensor([[-5.2422e+00, 4.0021e+01, 2.9757e-01],
+ [-9.1435e+01, -5.5950e+00, -2.6675e+01],
+ [6.061661e+00, -1.0e+02, 0.0]])
+
+ assert torch.allclose(expected_tensor, pcd_transformed, 1e-4)
+
+ # V+H, not reverse, depth
+ img_meta = {
+ 'pcd_vertical_flip': True,
+ 'pcd_horizontal_flip': True,
+ 'transformation_3d_flow': ['VF', 'HF']
+ }
+
+ pcd_transformed = apply_3d_transformation(
+ pcd, 'DEPTH', img_meta, reverse=False)
+
+ expected_tensor = torch.tensor([[5.2422e+00, -4.0021e+01, -2.9757e-01],
+ [9.1435e+01, 5.5950e+00, 2.6675e+01],
+ [-6.061661e+00, 1.0e+02, 0.0]])
+ assert torch.allclose(expected_tensor, pcd_transformed, 1e-4)
+
+ # V+H, reverse, lidar
+ img_meta = {
+ 'pcd_vertical_flip': True,
+ 'pcd_horizontal_flip': True,
+ 'transformation_3d_flow': ['VF', 'HF']
+ }
+
+ pcd_transformed = apply_3d_transformation(
+ pcd, 'LIDAR', img_meta, reverse=True)
+
+ expected_tensor = torch.tensor([[5.2422e+00, -4.0021e+01, -2.9757e-01],
+ [9.1435e+01, 5.5950e+00, 2.6675e+01],
+ [-6.061661e+00, 1.0e+02, 0.0]])
+ assert torch.allclose(expected_tensor, pcd_transformed, 1e-4)
diff --git a/tests/test_models/test_fusion/test_point_fusion.py b/tests/test_models/test_fusion/test_point_fusion.py
new file mode 100644
index 0000000..d976a74
--- /dev/null
+++ b/tests/test_models/test_fusion/test_point_fusion.py
@@ -0,0 +1,61 @@
+# Copyright (c) OpenMMLab. All rights reserved.
+"""Tests the core function of point fusion.
+
+CommandLine:
+ pytest tests/test_models/test_fusion/test_point_fusion.py
+"""
+
+import torch
+
+from mmdet3d.models.fusion_layers import PointFusion
+
+
+def test_sample_single():
+ # this function makes sure the rewriting of 3d coords transformation
+ # in point fusion does not change the original behaviour
+ lidar2img = torch.tensor(
+ [[6.0294e+02, -7.0791e+02, -1.2275e+01, -1.7094e+02],
+ [1.7678e+02, 8.8088e+00, -7.0794e+02, -1.0257e+02],
+ [9.9998e-01, -1.5283e-03, -5.2907e-03, -3.2757e-01],
+ [0.0000e+00, 0.0000e+00, 0.0000e+00, 1.0000e+00]])
+
+ # all use default
+ img_meta = {
+ 'transformation_3d_flow': ['R', 'S', 'T', 'HF'],
+ 'input_shape': [370, 1224],
+ 'img_shape': [370, 1224],
+ 'lidar2img': lidar2img,
+ }
+
+ # dummy parameters
+ fuse = PointFusion(1, 1, 1, 1)
+ img_feat = torch.arange(370 * 1224)[None, ...].view(
+ 370, 1224)[None, None, ...].float() / (370 * 1224)
+ pts = torch.tensor([[8.356, -4.312, -0.445], [11.777, -6.724, -0.564],
+ [6.453, 2.53, -1.612], [6.227, -3.839, -0.563]])
+ out = fuse.sample_single(img_feat, pts, img_meta)
+
+ expected_tensor = torch.tensor(
+ [0.5560822, 0.5476625, 0.9687978, 0.6241757])
+ assert torch.allclose(expected_tensor, out, 1e-4)
+
+ pcd_rotation = torch.tensor([[8.660254e-01, 0.5, 0],
+ [-0.5, 8.660254e-01, 0], [0, 0, 1.0e+00]])
+ pcd_scale_factor = 1.111
+ pcd_trans = torch.tensor([1.0, -1.0, 0.5])
+ pts = pts @ pcd_rotation
+ pts *= pcd_scale_factor
+ pts += pcd_trans
+ pts[:, 1] = -pts[:, 1]
+
+ # not use default
+ img_meta.update({
+ 'pcd_scale_factor': pcd_scale_factor,
+ 'pcd_rotation': pcd_rotation,
+ 'pcd_trans': pcd_trans,
+ 'pcd_horizontal_flip': True
+ })
+ out = fuse.sample_single(img_feat, pts, img_meta)
+ expected_tensor = torch.tensor(
+ [0.5560822, 0.5476625, 0.9687978, 0.6241757])
+ assert torch.allclose(expected_tensor, out, 1e-4)
diff --git a/tests/test_models/test_fusion/test_vote_fusion.py b/tests/test_models/test_fusion/test_vote_fusion.py
new file mode 100644
index 0000000..a4c2e05
--- /dev/null
+++ b/tests/test_models/test_fusion/test_vote_fusion.py
@@ -0,0 +1,322 @@
+# Copyright (c) OpenMMLab. All rights reserved.
+"""Tests the core function of vote fusion.
+
+CommandLine:
+ pytest tests/test_models/test_fusion/test_vote_fusion.py
+"""
+
+import torch
+
+from mmdet3d.models.fusion_layers import VoteFusion
+
+
+def test_vote_fusion():
+ img_meta = {
+ 'ori_shape': (530, 730, 3),
+ 'img_shape': (600, 826, 3),
+ 'pad_shape': (608, 832, 3),
+ 'scale_factor':
+ torch.tensor([1.1315, 1.1321, 1.1315, 1.1321]),
+ 'flip':
+ False,
+ 'pcd_horizontal_flip':
+ False,
+ 'pcd_vertical_flip':
+ False,
+ 'pcd_trans':
+ torch.tensor([0., 0., 0.]),
+ 'pcd_scale_factor':
+ 1.0308290128214932,
+ 'pcd_rotation':
+ torch.tensor([[0.9747, 0.2234, 0.0000], [-0.2234, 0.9747, 0.0000],
+ [0.0000, 0.0000, 1.0000]]),
+ 'transformation_3d_flow': ['HF', 'R', 'S', 'T']
+ }
+
+ rt_mat = torch.tensor([[0.979570, 0.047954, -0.195330],
+ [0.047954, 0.887470, 0.458370],
+ [0.195330, -0.458370, 0.867030]])
+ k_mat = torch.tensor([[529.5000, 0.0000, 365.0000],
+ [0.0000, 529.5000, 265.0000],
+ [0.0000, 0.0000, 1.0000]])
+ rt_mat = rt_mat.new_tensor([[1, 0, 0], [0, 0, -1], [0, 1, 0]
+ ]) @ rt_mat.transpose(1, 0)
+ depth2img = k_mat @ rt_mat
+ img_meta['depth2img'] = depth2img
+
+ bboxes = torch.tensor([[[
+ 5.4286e+02, 9.8283e+01, 6.1700e+02, 1.6742e+02, 9.7922e-01, 3.0000e+00
+ ], [
+ 4.2613e+02, 8.4646e+01, 4.9091e+02, 1.6237e+02, 9.7848e-01, 3.0000e+00
+ ], [
+ 2.5606e+02, 7.3244e+01, 3.7883e+02, 1.8471e+02, 9.7317e-01, 3.0000e+00
+ ], [
+ 6.0104e+02, 1.0648e+02, 6.6757e+02, 1.9216e+02, 8.4607e-01, 3.0000e+00
+ ], [
+ 2.2923e+02, 1.4984e+02, 7.0163e+02, 4.6537e+02, 3.5719e-01, 0.0000e+00
+ ], [
+ 2.5614e+02, 7.4965e+01, 3.3275e+02, 1.5908e+02, 2.8688e-01, 3.0000e+00
+ ], [
+ 9.8718e+00, 1.4142e+02, 2.0213e+02, 3.3878e+02, 1.0935e-01, 3.0000e+00
+ ], [
+ 6.1930e+02, 1.1768e+02, 6.8505e+02, 2.0318e+02, 1.0720e-01, 3.0000e+00
+ ]]])
+
+ seeds_3d = torch.tensor([[[0.044544, 1.675476, -1.531831],
+ [2.500625, 7.238662, -0.737675],
+ [-0.600003, 4.827733, -0.084022],
+ [1.396212, 3.994484, -1.551180],
+ [-2.054746, 2.012759, -0.357472],
+ [-0.582477, 6.580470, -1.466052],
+ [1.313331, 5.722039, 0.123904],
+ [-1.107057, 3.450359, -1.043422],
+ [1.759746, 5.655951, -1.519564],
+ [-0.203003, 6.453243, 0.137703],
+ [-0.910429, 0.904407, -0.512307],
+ [0.434049, 3.032374, -0.763842],
+ [1.438146, 2.289263, -1.546332],
+ [0.575622, 5.041906, -0.891143],
+ [-1.675931, 1.417597, -1.588347]]])
+
+ imgs = torch.linspace(
+ -1, 1, steps=608 * 832).reshape(1, 608, 832).repeat(3, 1, 1)[None]
+
+ expected_tensor1 = torch.tensor(
+ [[[
+ 0.000000e+00, -0.000000e+00, 0.000000e+00, -0.000000e+00,
+ 0.000000e+00, 1.193706e-01, -0.000000e+00, -2.879214e-01,
+ -0.000000e+00, 0.000000e+00, 1.422463e-01, -6.474612e-01,
+ -0.000000e+00, 1.490057e-02, 0.000000e+00
+ ],
+ [
+ 0.000000e+00, -0.000000e+00, -0.000000e+00, 0.000000e+00,
+ 0.000000e+00, -1.873745e+00, -0.000000e+00, 1.576240e-01,
+ 0.000000e+00, -0.000000e+00, -3.646177e-02, -7.751858e-01,
+ 0.000000e+00, 9.593642e-02, 0.000000e+00
+ ],
+ [
+ 0.000000e+00, 0.000000e+00, 0.000000e+00, 0.000000e+00,
+ 0.000000e+00, -6.263277e-02, 0.000000e+00, -3.646387e-01,
+ 0.000000e+00, 0.000000e+00, -5.875812e-01, -6.263450e-02,
+ 0.000000e+00, 1.149264e-01, 0.000000e+00
+ ],
+ [
+ 0.000000e+00, 0.000000e+00, 0.000000e+00, 0.000000e+00,
+ 0.000000e+00, 8.899736e-01, 0.000000e+00, 9.019017e-01,
+ 0.000000e+00, 0.000000e+00, 6.917775e-01, 8.899733e-01,
+ 0.000000e+00, 9.812444e-01, 0.000000e+00
+ ],
+ [
+ -0.000000e+00, -0.000000e+00, -0.000000e+00, -0.000000e+00,
+ -0.000000e+00, -4.516903e-01, -0.000000e+00, -2.315422e-01,
+ -0.000000e+00, -0.000000e+00, -4.197519e-01, -4.516906e-01,
+ -0.000000e+00, -1.547615e-01, -0.000000e+00
+ ],
+ [
+ 0.000000e+00, 0.000000e+00, 0.000000e+00, 0.000000e+00,
+ 0.000000e+00, 3.571937e-01, 0.000000e+00, 0.000000e+00,
+ 0.000000e+00, 0.000000e+00, 0.000000e+00, 3.571937e-01,
+ 0.000000e+00, 0.000000e+00, 0.000000e+00
+ ],
+ [
+ 0.000000e+00, 0.000000e+00, 0.000000e+00, 0.000000e+00,
+ 0.000000e+00, 0.000000e+00, 0.000000e+00, 0.000000e+00,
+ 0.000000e+00, 0.000000e+00, 0.000000e+00, 0.000000e+00,
+ 0.000000e+00, 0.000000e+00, 0.000000e+00
+ ],
+ [
+ 0.000000e+00, 0.000000e+00, 0.000000e+00, 0.000000e+00,
+ 0.000000e+00, 0.000000e+00, 0.000000e+00, 0.000000e+00,
+ 0.000000e+00, 0.000000e+00, 0.000000e+00, 0.000000e+00,
+ 0.000000e+00, 0.000000e+00, 0.000000e+00
+ ],
+ [
+ 0.000000e+00, 0.000000e+00, 0.000000e+00, 0.000000e+00,
+ 0.000000e+00, 0.000000e+00, 0.000000e+00, 9.731653e-01,
+ 0.000000e+00, 0.000000e+00, 1.093455e-01, 0.000000e+00,
+ 0.000000e+00, 8.460656e-01, 0.000000e+00
+ ],
+ [
+ 0.000000e+00, 0.000000e+00, 0.000000e+00, 0.000000e+00,
+ 0.000000e+00, 0.000000e+00, 0.000000e+00, 0.000000e+00,
+ 0.000000e+00, 0.000000e+00, 0.000000e+00, 0.000000e+00,
+ 0.000000e+00, 0.000000e+00, 0.000000e+00
+ ],
+ [
+ 0.000000e+00, 0.000000e+00, 0.000000e+00, 0.000000e+00,
+ 0.000000e+00, 0.000000e+00, 0.000000e+00, 0.000000e+00,
+ 0.000000e+00, 0.000000e+00, 0.000000e+00, 0.000000e+00,
+ 0.000000e+00, 0.000000e+00, 0.000000e+00
+ ],
+ [
+ 0.000000e+00, 0.000000e+00, 0.000000e+00, 0.000000e+00,
+ 0.000000e+00, 0.000000e+00, 0.000000e+00, 0.000000e+00,
+ 0.000000e+00, 0.000000e+00, 0.000000e+00, 0.000000e+00,
+ 0.000000e+00, 0.000000e+00, 0.000000e+00
+ ],
+ [
+ 0.000000e+00, 0.000000e+00, 0.000000e+00, 0.000000e+00,
+ 0.000000e+00, 0.000000e+00, 0.000000e+00, 0.000000e+00,
+ 0.000000e+00, 0.000000e+00, 0.000000e+00, 0.000000e+00,
+ 0.000000e+00, 0.000000e+00, 0.000000e+00
+ ],
+ [
+ 0.000000e+00, 0.000000e+00, 0.000000e+00, 0.000000e+00,
+ 0.000000e+00, 0.000000e+00, 0.000000e+00, 0.000000e+00,
+ 0.000000e+00, 0.000000e+00, 0.000000e+00, 0.000000e+00,
+ 0.000000e+00, 0.000000e+00, 0.000000e+00
+ ],
+ [
+ 0.000000e+00, 0.000000e+00, 0.000000e+00, 0.000000e+00,
+ 0.000000e+00, 0.000000e+00, 0.000000e+00, 0.000000e+00,
+ 0.000000e+00, 0.000000e+00, 0.000000e+00, 0.000000e+00,
+ 0.000000e+00, 0.000000e+00, 0.000000e+00
+ ],
+ [
+ 2.316288e-03, -1.948284e-03, -3.694394e-03, 2.176163e-04,
+ -3.882605e-03, -1.901490e-03, -3.355042e-03, -1.774631e-03,
+ -6.981542e-04, -3.886823e-03, -1.302233e-03, -1.189933e-03,
+ 2.540967e-03, -1.834944e-03, 1.032048e-03
+ ],
+ [
+ 2.316288e-03, -1.948284e-03, -3.694394e-03, 2.176163e-04,
+ -3.882605e-03, -1.901490e-03, -3.355042e-03, -1.774631e-03,
+ -6.981542e-04, -3.886823e-03, -1.302233e-03, -1.189933e-03,
+ 2.540967e-03, -1.834944e-03, 1.032048e-03
+ ],
+ [
+ 2.316288e-03, -1.948284e-03, -3.694394e-03, 2.176163e-04,
+ -3.882605e-03, -1.901490e-03, -3.355042e-03, -1.774631e-03,
+ -6.981542e-04, -3.886823e-03, -1.302233e-03, -1.189933e-03,
+ 2.540967e-03, -1.834944e-03, 1.032048e-03
+ ]]])
+
+ expected_tensor2 = torch.tensor([[
+ False, False, False, False, False, True, False, True, False, False,
+ True, True, False, True, False, False, False, False, False, False,
+ False, False, True, False, False, False, False, False, True, False,
+ False, False, False, False, False, False, False, False, False, False,
+ False, False, False, True, False
+ ]])
+
+ expected_tensor3 = torch.tensor(
+ [[[
+ -0.000000e+00, -0.000000e+00, -0.000000e+00, -0.000000e+00,
+ 0.000000e+00, -0.000000e+00, -0.000000e+00, 0.000000e+00,
+ -0.000000e+00, -0.000000e+00, 0.000000e+00, -0.000000e+00,
+ -0.000000e+00, 1.720988e-01, 0.000000e+00
+ ],
+ [
+ 0.000000e+00, -0.000000e+00, -0.000000e+00, 0.000000e+00,
+ -0.000000e+00, 0.000000e+00, -0.000000e+00, 0.000000e+00,
+ 0.000000e+00, -0.000000e+00, 0.000000e+00, 0.000000e+00,
+ 0.000000e+00, 4.824460e-02, 0.000000e+00
+ ],
+ [
+ -0.000000e+00, -0.000000e+00, -0.000000e+00, -0.000000e+00,
+ -0.000000e+00, -0.000000e+00, -0.000000e+00, 0.000000e+00,
+ -0.000000e+00, -0.000000e+00, -0.000000e+00, -0.000000e+00,
+ -0.000000e+00, 1.447314e-01, -0.000000e+00
+ ],
+ [
+ 0.000000e+00, 0.000000e+00, 0.000000e+00, 0.000000e+00,
+ 0.000000e+00, 0.000000e+00, 0.000000e+00, 0.000000e+00,
+ 0.000000e+00, 0.000000e+00, 0.000000e+00, 0.000000e+00,
+ 0.000000e+00, 9.759269e-01, 0.000000e+00
+ ],
+ [
+ -0.000000e+00, -0.000000e+00, -0.000000e+00, -0.000000e+00,
+ -0.000000e+00, -0.000000e+00, -0.000000e+00, -0.000000e+00,
+ -0.000000e+00, -0.000000e+00, -0.000000e+00, -0.000000e+00,
+ -0.000000e+00, -1.631542e-01, -0.000000e+00
+ ],
+ [
+ 0.000000e+00, 0.000000e+00, 0.000000e+00, 0.000000e+00,
+ 0.000000e+00, 0.000000e+00, 0.000000e+00, 0.000000e+00,
+ 0.000000e+00, 0.000000e+00, 0.000000e+00, 0.000000e+00,
+ 0.000000e+00, 0.000000e+00, 0.000000e+00
+ ],
+ [
+ 0.000000e+00, 0.000000e+00, 0.000000e+00, 0.000000e+00,
+ 0.000000e+00, 0.000000e+00, 0.000000e+00, 0.000000e+00,
+ 0.000000e+00, 0.000000e+00, 0.000000e+00, 0.000000e+00,
+ 0.000000e+00, 0.000000e+00, 0.000000e+00
+ ],
+ [
+ 0.000000e+00, 0.000000e+00, 0.000000e+00, 0.000000e+00,
+ 0.000000e+00, 0.000000e+00, 0.000000e+00, 0.000000e+00,
+ 0.000000e+00, 0.000000e+00, 0.000000e+00, 0.000000e+00,
+ 0.000000e+00, 0.000000e+00, 0.000000e+00
+ ],
+ [
+ 0.000000e+00, 0.000000e+00, 0.000000e+00, 0.000000e+00,
+ 0.000000e+00, 0.000000e+00, 0.000000e+00, 0.000000e+00,
+ 0.000000e+00, 0.000000e+00, 0.000000e+00, 0.000000e+00,
+ 0.000000e+00, 1.072001e-01, 0.000000e+00
+ ],
+ [
+ 0.000000e+00, 0.000000e+00, 0.000000e+00, 0.000000e+00,
+ 0.000000e+00, 0.000000e+00, 0.000000e+00, 0.000000e+00,
+ 0.000000e+00, 0.000000e+00, 0.000000e+00, 0.000000e+00,
+ 0.000000e+00, 0.000000e+00, 0.000000e+00
+ ],
+ [
+ 0.000000e+00, 0.000000e+00, 0.000000e+00, 0.000000e+00,
+ 0.000000e+00, 0.000000e+00, 0.000000e+00, 0.000000e+00,
+ 0.000000e+00, 0.000000e+00, 0.000000e+00, 0.000000e+00,
+ 0.000000e+00, 0.000000e+00, 0.000000e+00
+ ],
+ [
+ 0.000000e+00, 0.000000e+00, 0.000000e+00, 0.000000e+00,
+ 0.000000e+00, 0.000000e+00, 0.000000e+00, 0.000000e+00,
+ 0.000000e+00, 0.000000e+00, 0.000000e+00, 0.000000e+00,
+ 0.000000e+00, 0.000000e+00, 0.000000e+00
+ ],
+ [
+ 0.000000e+00, 0.000000e+00, 0.000000e+00, 0.000000e+00,
+ 0.000000e+00, 0.000000e+00, 0.000000e+00, 0.000000e+00,
+ 0.000000e+00, 0.000000e+00, 0.000000e+00, 0.000000e+00,
+ 0.000000e+00, 0.000000e+00, 0.000000e+00
+ ],
+ [
+ 0.000000e+00, 0.000000e+00, 0.000000e+00, 0.000000e+00,
+ 0.000000e+00, 0.000000e+00, 0.000000e+00, 0.000000e+00,
+ 0.000000e+00, 0.000000e+00, 0.000000e+00, 0.000000e+00,
+ 0.000000e+00, 0.000000e+00, 0.000000e+00
+ ],
+ [
+ 0.000000e+00, 0.000000e+00, 0.000000e+00, 0.000000e+00,
+ 0.000000e+00, 0.000000e+00, 0.000000e+00, 0.000000e+00,
+ 0.000000e+00, 0.000000e+00, 0.000000e+00, 0.000000e+00,
+ 0.000000e+00, 0.000000e+00, 0.000000e+00
+ ],
+ [
+ 2.316288e-03, -1.948284e-03, -3.694394e-03, 2.176163e-04,
+ -3.882605e-03, -1.901490e-03, -3.355042e-03, -1.774631e-03,
+ -6.981542e-04, -3.886823e-03, -1.302233e-03, -1.189933e-03,
+ 2.540967e-03, -1.834944e-03, 1.032048e-03
+ ],
+ [
+ 2.316288e-03, -1.948284e-03, -3.694394e-03, 2.176163e-04,
+ -3.882605e-03, -1.901490e-03, -3.355042e-03, -1.774631e-03,
+ -6.981542e-04, -3.886823e-03, -1.302233e-03, -1.189933e-03,
+ 2.540967e-03, -1.834944e-03, 1.032048e-03
+ ],
+ [
+ 2.316288e-03, -1.948284e-03, -3.694394e-03, 2.176163e-04,
+ -3.882605e-03, -1.901490e-03, -3.355042e-03, -1.774631e-03,
+ -6.981542e-04, -3.886823e-03, -1.302233e-03, -1.189933e-03,
+ 2.540967e-03, -1.834944e-03, 1.032048e-03
+ ]]])
+
+ fusion = VoteFusion()
+ out1, out2 = fusion(imgs, bboxes, seeds_3d, [img_meta])
+ assert torch.allclose(expected_tensor1, out1[:, :, :15], 1e-3)
+ assert torch.allclose(expected_tensor2.float(), out2.float(), 1e-3)
+ assert torch.allclose(expected_tensor3, out1[:, :, 30:45], 1e-3)
+
+ out1, out2 = fusion(imgs, bboxes[:, :2], seeds_3d, [img_meta])
+ out1 = out1[:, :15, 30:45]
+ out2 = out2[:, 30:45].float()
+ assert torch.allclose(torch.zeros_like(out1), out1, 1e-3)
+ assert torch.allclose(torch.zeros_like(out2), out2, 1e-3)
diff --git a/tests/test_models/test_heads/test_dgcnn_decode_head.py b/tests/test_models/test_heads/test_dgcnn_decode_head.py
new file mode 100644
index 0000000..6d1f149
--- /dev/null
+++ b/tests/test_models/test_heads/test_dgcnn_decode_head.py
@@ -0,0 +1,68 @@
+# Copyright (c) OpenMMLab. All rights reserved.
+import numpy as np
+import pytest
+import torch
+from mmcv.cnn.bricks import ConvModule
+
+from mmdet3d.models.builder import build_head
+
+
+def test_dgcnn_decode_head_loss():
+ if not torch.cuda.is_available():
+ pytest.skip('test requires GPU and torch+cuda')
+ dgcnn_decode_head_cfg = dict(
+ type='DGCNNHead',
+ fp_channels=(1024, 512),
+ channels=256,
+ num_classes=13,
+ dropout_ratio=0.5,
+ conv_cfg=dict(type='Conv1d'),
+ norm_cfg=dict(type='BN1d'),
+ act_cfg=dict(type='LeakyReLU', negative_slope=0.2),
+ loss_decode=dict(
+ type='CrossEntropyLoss',
+ use_sigmoid=False,
+ class_weight=None,
+ loss_weight=1.0),
+ ignore_index=13)
+
+ self = build_head(dgcnn_decode_head_cfg)
+ self.cuda()
+ assert isinstance(self.conv_seg, torch.nn.Conv1d)
+ assert self.conv_seg.in_channels == 256
+ assert self.conv_seg.out_channels == 13
+ assert self.conv_seg.kernel_size == (1, )
+ assert isinstance(self.pre_seg_conv, ConvModule)
+ assert isinstance(self.pre_seg_conv.conv, torch.nn.Conv1d)
+ assert self.pre_seg_conv.conv.in_channels == 512
+ assert self.pre_seg_conv.conv.out_channels == 256
+ assert self.pre_seg_conv.conv.kernel_size == (1, )
+ assert isinstance(self.pre_seg_conv.bn, torch.nn.BatchNorm1d)
+ assert self.pre_seg_conv.bn.num_features == 256
+
+ # test forward
+ fa_points = torch.rand(2, 4096, 1024).float().cuda()
+ input_dict = dict(fa_points=fa_points)
+ seg_logits = self(input_dict)
+ assert seg_logits.shape == torch.Size([2, 13, 4096])
+
+ # test loss
+ pts_semantic_mask = torch.randint(0, 13, (2, 4096)).long().cuda()
+ losses = self.losses(seg_logits, pts_semantic_mask)
+ assert losses['loss_sem_seg'].item() > 0
+
+ # test loss with ignore_index
+ ignore_index_mask = torch.ones_like(pts_semantic_mask) * 13
+ losses = self.losses(seg_logits, ignore_index_mask)
+ assert losses['loss_sem_seg'].item() == 0
+
+ # test loss with class_weight
+ dgcnn_decode_head_cfg['loss_decode'] = dict(
+ type='CrossEntropyLoss',
+ use_sigmoid=False,
+ class_weight=np.random.rand(13),
+ loss_weight=1.0)
+ self = build_head(dgcnn_decode_head_cfg)
+ self.cuda()
+ losses = self.losses(seg_logits, pts_semantic_mask)
+ assert losses['loss_sem_seg'].item() > 0
diff --git a/tests/test_models/test_heads/test_heads.py b/tests/test_models/test_heads/test_heads.py
new file mode 100644
index 0000000..5f27644
--- /dev/null
+++ b/tests/test_models/test_heads/test_heads.py
@@ -0,0 +1,1654 @@
+# Copyright (c) OpenMMLab. All rights reserved.
+import copy
+import random
+from os.path import dirname, exists, join
+
+import mmcv
+import numpy as np
+import pytest
+import torch
+
+from mmdet3d.core.bbox import (Box3DMode, CameraInstance3DBoxes,
+ DepthInstance3DBoxes, LiDARInstance3DBoxes)
+from mmdet3d.models.builder import build_head
+from mmdet.apis import set_random_seed
+
+
+def _setup_seed(seed):
+ torch.manual_seed(seed)
+ torch.cuda.manual_seed_all(seed)
+ np.random.seed(seed)
+ random.seed(seed)
+ torch.backends.cudnn.deterministic = True
+
+
+def _get_config_directory():
+ """Find the predefined detector config directory."""
+ try:
+ # Assume we are running in the source mmdetection3d repo
+ repo_dpath = dirname(dirname(dirname(dirname(__file__))))
+ except NameError:
+ # For IPython development when this __file__ is not defined
+ import mmdet3d
+ repo_dpath = dirname(dirname(mmdet3d.__file__))
+ config_dpath = join(repo_dpath, 'configs')
+ if not exists(config_dpath):
+ raise Exception('Cannot find config path')
+ return config_dpath
+
+
+def _get_config_module(fname):
+ """Load a configuration as a python module."""
+ from mmcv import Config
+ config_dpath = _get_config_directory()
+ config_fpath = join(config_dpath, fname)
+ config_mod = Config.fromfile(config_fpath)
+ return config_mod
+
+
+def _get_head_cfg(fname):
+ """Grab configs necessary to create a bbox_head.
+
+ These are deep copied to allow for safe modification of parameters without
+ influencing other tests.
+ """
+ config = _get_config_module(fname)
+ model = copy.deepcopy(config.model)
+ train_cfg = mmcv.Config(copy.deepcopy(config.model.train_cfg))
+ test_cfg = mmcv.Config(copy.deepcopy(config.model.test_cfg))
+
+ bbox_head = model.bbox_head
+ bbox_head.update(train_cfg=train_cfg)
+ bbox_head.update(test_cfg=test_cfg)
+ return bbox_head
+
+
+def _get_rpn_head_cfg(fname):
+ """Grab configs necessary to create a rpn_head.
+
+ These are deep copied to allow for safe modification of parameters without
+ influencing other tests.
+ """
+ config = _get_config_module(fname)
+ model = copy.deepcopy(config.model)
+ train_cfg = mmcv.Config(copy.deepcopy(config.model.train_cfg))
+ test_cfg = mmcv.Config(copy.deepcopy(config.model.test_cfg))
+
+ rpn_head = model.rpn_head
+ rpn_head.update(train_cfg=train_cfg.rpn)
+ rpn_head.update(test_cfg=test_cfg.rpn)
+ return rpn_head, train_cfg.rpn_proposal
+
+
+def _get_roi_head_cfg(fname):
+ """Grab configs necessary to create a roi_head.
+
+ These are deep copied to allow for safe modification of parameters without
+ influencing other tests.
+ """
+ config = _get_config_module(fname)
+ model = copy.deepcopy(config.model)
+ train_cfg = mmcv.Config(copy.deepcopy(config.model.train_cfg))
+ test_cfg = mmcv.Config(copy.deepcopy(config.model.test_cfg))
+
+ roi_head = model.roi_head
+ roi_head.update(train_cfg=train_cfg.rcnn)
+ roi_head.update(test_cfg=test_cfg.rcnn)
+ return roi_head
+
+
+def _get_pts_bbox_head_cfg(fname):
+ """Grab configs necessary to create a pts_bbox_head.
+
+ These are deep copied to allow for safe modification of parameters without
+ influencing other tests.
+ """
+ config = _get_config_module(fname)
+ model = copy.deepcopy(config.model)
+ train_cfg = mmcv.Config(copy.deepcopy(config.model.train_cfg.pts))
+ test_cfg = mmcv.Config(copy.deepcopy(config.model.test_cfg.pts))
+
+ pts_bbox_head = model.pts_bbox_head
+ pts_bbox_head.update(train_cfg=train_cfg)
+ pts_bbox_head.update(test_cfg=test_cfg)
+ return pts_bbox_head
+
+
+def _get_pointrcnn_rpn_head_cfg(fname):
+ """Grab configs necessary to create a rpn_head.
+
+ These are deep copied to allow for safe modification of parameters without
+ influencing other tests.
+ """
+ config = _get_config_module(fname)
+ model = copy.deepcopy(config.model)
+ train_cfg = mmcv.Config(copy.deepcopy(config.model.train_cfg))
+ test_cfg = mmcv.Config(copy.deepcopy(config.model.test_cfg))
+
+ rpn_head = model.rpn_head
+ rpn_head.update(train_cfg=train_cfg.rpn)
+ rpn_head.update(test_cfg=test_cfg.rpn)
+ return rpn_head, train_cfg.rpn
+
+
+def _get_vote_head_cfg(fname):
+ """Grab configs necessary to create a vote_head.
+
+ These are deep copied to allow for safe modification of parameters without
+ influencing other tests.
+ """
+ config = _get_config_module(fname)
+ model = copy.deepcopy(config.model)
+ train_cfg = mmcv.Config(copy.deepcopy(config.model.train_cfg))
+ test_cfg = mmcv.Config(copy.deepcopy(config.model.test_cfg))
+
+ vote_head = model.bbox_head
+ vote_head.update(train_cfg=train_cfg)
+ vote_head.update(test_cfg=test_cfg)
+ return vote_head
+
+
+def _get_parta2_bbox_head_cfg(fname):
+ """Grab configs necessary to create a parta2_bbox_head.
+
+ These are deep copied to allow for safe modification of parameters without
+ influencing other tests.
+ """
+ config = _get_config_module(fname)
+ model = copy.deepcopy(config.model)
+
+ vote_head = model.roi_head.bbox_head
+ return vote_head
+
+
+def _get_pointrcnn_bbox_head_cfg(fname):
+ config = _get_config_module(fname)
+ model = copy.deepcopy(config.model)
+
+ vote_head = model.roi_head.bbox_head
+ return vote_head
+
+
+def test_anchor3d_head_loss():
+ if not torch.cuda.is_available():
+ pytest.skip('test requires GPU and torch+cuda')
+ bbox_head_cfg = _get_head_cfg(
+ 'second/hv_second_secfpn_6x8_80e_kitti-3d-3class.py')
+
+ from mmdet3d.models.builder import build_head
+ self = build_head(bbox_head_cfg)
+ self.cuda()
+ assert isinstance(self.conv_cls, torch.nn.modules.conv.Conv2d)
+ assert self.conv_cls.in_channels == 512
+ assert self.conv_cls.out_channels == 18
+ assert self.conv_reg.out_channels == 42
+ assert self.conv_dir_cls.out_channels == 12
+
+ # test forward
+ feats = list()
+ feats.append(torch.rand([2, 512, 200, 176], dtype=torch.float32).cuda())
+ (cls_score, bbox_pred, dir_cls_preds) = self.forward(feats)
+ assert cls_score[0].shape == torch.Size([2, 18, 200, 176])
+ assert bbox_pred[0].shape == torch.Size([2, 42, 200, 176])
+ assert dir_cls_preds[0].shape == torch.Size([2, 12, 200, 176])
+
+ # test loss
+ gt_bboxes = list(
+ torch.tensor(
+ [[[6.4118, -3.4305, -1.7291, 1.7033, 3.4693, 1.6197, -0.9091]],
+ [[16.9107, 9.7925, -1.9201, 1.6097, 3.2786, 1.5307, -2.4056]]],
+ dtype=torch.float32).cuda())
+ gt_labels = list(torch.tensor([[0], [1]], dtype=torch.int64).cuda())
+ input_metas = [{
+ 'sample_idx': 1234
+ }, {
+ 'sample_idx': 2345
+ }] # fake input_metas
+
+ losses = self.loss(cls_score, bbox_pred, dir_cls_preds, gt_bboxes,
+ gt_labels, input_metas)
+ assert losses['loss_cls'][0] > 0
+ assert losses['loss_bbox'][0] > 0
+ assert losses['loss_dir'][0] > 0
+
+ # test empty ground truth case
+ gt_bboxes = list(torch.empty((2, 0, 7)).cuda())
+ gt_labels = list(torch.empty((2, 0)).cuda())
+ empty_gt_losses = self.loss(cls_score, bbox_pred, dir_cls_preds, gt_bboxes,
+ gt_labels, input_metas)
+ assert empty_gt_losses['loss_cls'][0] > 0
+ assert empty_gt_losses['loss_bbox'][0] == 0
+ assert empty_gt_losses['loss_dir'][0] == 0
+
+
+def test_anchor3d_head_getboxes():
+ if not torch.cuda.is_available():
+ pytest.skip('test requires GPU and torch+cuda')
+ bbox_head_cfg = _get_head_cfg(
+ 'second/hv_second_secfpn_6x8_80e_kitti-3d-3class.py')
+
+ from mmdet3d.models.builder import build_head
+ self = build_head(bbox_head_cfg)
+ self.cuda()
+
+ feats = list()
+ feats.append(torch.rand([2, 512, 200, 176], dtype=torch.float32).cuda())
+ # fake input_metas
+ input_metas = [{
+ 'sample_idx': 1234,
+ 'box_type_3d': LiDARInstance3DBoxes,
+ 'box_mode_3d': Box3DMode.LIDAR
+ }, {
+ 'sample_idx': 2345,
+ 'box_type_3d': LiDARInstance3DBoxes,
+ 'box_mode_3d': Box3DMode.LIDAR
+ }]
+ (cls_score, bbox_pred, dir_cls_preds) = self.forward(feats)
+
+ # test get_boxes
+ cls_score[0] -= 1.5 # too many positive samples may cause cuda oom
+ result_list = self.get_bboxes(cls_score, bbox_pred, dir_cls_preds,
+ input_metas)
+ assert (result_list[0][1] > 0.3).all()
+
+
+def test_parta2_rpnhead_getboxes():
+ if not torch.cuda.is_available():
+ pytest.skip('test requires GPU and torch+cuda')
+ rpn_head_cfg, proposal_cfg = _get_rpn_head_cfg(
+ 'parta2/hv_PartA2_secfpn_2x8_cyclic_80e_kitti-3d-3class.py')
+
+ self = build_head(rpn_head_cfg)
+ self.cuda()
+
+ feats = list()
+ feats.append(torch.rand([2, 512, 200, 176], dtype=torch.float32).cuda())
+ # fake input_metas
+ input_metas = [{
+ 'sample_idx': 1234,
+ 'box_type_3d': LiDARInstance3DBoxes,
+ 'box_mode_3d': Box3DMode.LIDAR
+ }, {
+ 'sample_idx': 2345,
+ 'box_type_3d': LiDARInstance3DBoxes,
+ 'box_mode_3d': Box3DMode.LIDAR
+ }]
+ (cls_score, bbox_pred, dir_cls_preds) = self.forward(feats)
+
+ # test get_boxes
+ cls_score[0] -= 1.5 # too many positive samples may cause cuda oom
+ result_list = self.get_bboxes(cls_score, bbox_pred, dir_cls_preds,
+ input_metas, proposal_cfg)
+ assert result_list[0]['scores_3d'].shape == torch.Size([512])
+ assert result_list[0]['labels_3d'].shape == torch.Size([512])
+ assert result_list[0]['cls_preds'].shape == torch.Size([512, 3])
+ assert result_list[0]['boxes_3d'].tensor.shape == torch.Size([512, 7])
+
+
+def test_point_rcnn_rpnhead_getboxes():
+ if not torch.cuda.is_available():
+ pytest.skip('test requires GPU and torch+cuda')
+ rpn_head_cfg, proposal_cfg = _get_pointrcnn_rpn_head_cfg(
+ './point_rcnn/point_rcnn_2x8_kitti-3d-3classes.py')
+ self = build_head(rpn_head_cfg)
+ self.cuda()
+
+ fp_features = torch.rand([2, 128, 1024], dtype=torch.float32).cuda()
+ feats = {'fp_features': fp_features}
+ # fake input_metas
+ input_metas = [{
+ 'sample_idx': 1234,
+ 'box_type_3d': LiDARInstance3DBoxes,
+ 'box_mode_3d': Box3DMode.LIDAR
+ }, {
+ 'sample_idx': 2345,
+ 'box_type_3d': LiDARInstance3DBoxes,
+ 'box_mode_3d': Box3DMode.LIDAR
+ }]
+ (bbox_preds, cls_preds) = self.forward(feats)
+ assert bbox_preds.shape == (2, 1024, 8)
+ assert cls_preds.shape == (2, 1024, 3)
+ points = torch.rand([2, 1024, 3], dtype=torch.float32).cuda()
+ result_list = self.get_bboxes(points, bbox_preds, cls_preds, input_metas)
+ max_num = proposal_cfg.nms_cfg.nms_post
+ bbox, score_selected, labels, cls_preds_selected = result_list[0]
+ assert bbox.tensor.shape == (max_num, 7)
+ assert score_selected.shape == (max_num, )
+ assert labels.shape == (max_num, )
+ assert cls_preds_selected.shape == (max_num, 3)
+
+
+def test_vote_head():
+ if not torch.cuda.is_available():
+ pytest.skip('test requires GPU and torch+cuda')
+ _setup_seed(0)
+ vote_head_cfg = _get_vote_head_cfg(
+ 'votenet/votenet_8x8_scannet-3d-18class.py')
+ self = build_head(vote_head_cfg).cuda()
+ fp_xyz = [torch.rand([2, 256, 3], dtype=torch.float32).cuda()]
+ fp_features = [torch.rand([2, 256, 256], dtype=torch.float32).cuda()]
+ fp_indices = [torch.randint(0, 128, [2, 256]).cuda()]
+
+ input_dict = dict(
+ fp_xyz=fp_xyz, fp_features=fp_features, fp_indices=fp_indices)
+
+ # test forward
+ ret_dict = self(input_dict, 'vote')
+ assert ret_dict['center'].shape == torch.Size([2, 256, 3])
+ assert ret_dict['obj_scores'].shape == torch.Size([2, 256, 2])
+ assert ret_dict['size_res'].shape == torch.Size([2, 256, 18, 3])
+ assert ret_dict['dir_res'].shape == torch.Size([2, 256, 1])
+
+ # test loss
+ points = [torch.rand([40000, 4], device='cuda') for i in range(2)]
+ gt_bbox1 = LiDARInstance3DBoxes(torch.rand([10, 7], device='cuda'))
+ gt_bbox2 = LiDARInstance3DBoxes(torch.rand([10, 7], device='cuda'))
+ gt_bboxes = [gt_bbox1, gt_bbox2]
+ gt_labels = [torch.randint(0, 18, [10], device='cuda') for i in range(2)]
+ pts_semantic_mask = [
+ torch.randint(0, 18, [40000], device='cuda') for i in range(2)
+ ]
+ pts_instance_mask = [
+ torch.randint(0, 10, [40000], device='cuda') for i in range(2)
+ ]
+ losses = self.loss(ret_dict, points, gt_bboxes, gt_labels,
+ pts_semantic_mask, pts_instance_mask)
+ assert losses['vote_loss'] >= 0
+ assert losses['objectness_loss'] >= 0
+ assert losses['semantic_loss'] >= 0
+ assert losses['center_loss'] >= 0
+ assert losses['dir_class_loss'] >= 0
+ assert losses['dir_res_loss'] >= 0
+ assert losses['size_class_loss'] >= 0
+ assert losses['size_res_loss'] >= 0
+
+ # test multiclass_nms_single
+ obj_scores = torch.rand([256], device='cuda')
+ sem_scores = torch.rand([256, 18], device='cuda')
+ points = torch.rand([40000, 3], device='cuda')
+ bbox = torch.rand([256, 7], device='cuda')
+ input_meta = dict(box_type_3d=DepthInstance3DBoxes)
+ bbox_selected, score_selected, labels = self.multiclass_nms_single(
+ obj_scores, sem_scores, bbox, points, input_meta)
+ assert bbox_selected.shape[0] >= 0
+ assert bbox_selected.shape[1] == 7
+ assert score_selected.shape[0] >= 0
+ assert labels.shape[0] >= 0
+
+ # test get_boxes
+ points = torch.rand([1, 40000, 4], device='cuda')
+ seed_points = torch.rand([1, 1024, 3], device='cuda')
+ seed_indices = torch.randint(0, 40000, [1, 1024], device='cuda')
+ vote_points = torch.rand([1, 1024, 3], device='cuda')
+ vote_features = torch.rand([1, 256, 1024], device='cuda')
+ aggregated_points = torch.rand([1, 256, 3], device='cuda')
+ aggregated_indices = torch.range(0, 256, device='cuda')
+ obj_scores = torch.rand([1, 256, 2], device='cuda')
+ center = torch.rand([1, 256, 3], device='cuda')
+ dir_class = torch.rand([1, 256, 1], device='cuda')
+ dir_res_norm = torch.rand([1, 256, 1], device='cuda')
+ dir_res = torch.rand([1, 256, 1], device='cuda')
+ size_class = torch.rand([1, 256, 18], device='cuda')
+ size_res = torch.rand([1, 256, 18, 3], device='cuda')
+ sem_scores = torch.rand([1, 256, 18], device='cuda')
+ bbox_preds = dict(
+ seed_points=seed_points,
+ seed_indices=seed_indices,
+ vote_points=vote_points,
+ vote_features=vote_features,
+ aggregated_points=aggregated_points,
+ aggregated_indices=aggregated_indices,
+ obj_scores=obj_scores,
+ center=center,
+ dir_class=dir_class,
+ dir_res_norm=dir_res_norm,
+ dir_res=dir_res,
+ size_class=size_class,
+ size_res=size_res,
+ sem_scores=sem_scores)
+ results = self.get_bboxes(points, bbox_preds, [input_meta])
+ assert results[0][0].tensor.shape[0] >= 0
+ assert results[0][0].tensor.shape[1] == 7
+ assert results[0][1].shape[0] >= 0
+ assert results[0][2].shape[0] >= 0
+
+
+def test_smoke_mono3d_head():
+
+ head_cfg = dict(
+ type='SMOKEMono3DHead',
+ num_classes=3,
+ in_channels=64,
+ dim_channel=[3, 4, 5],
+ ori_channel=[6, 7],
+ stacked_convs=0,
+ feat_channels=64,
+ use_direction_classifier=False,
+ diff_rad_by_sin=False,
+ pred_attrs=False,
+ pred_velo=False,
+ dir_offset=0,
+ strides=None,
+ group_reg_dims=(8, ),
+ cls_branch=(256, ),
+ reg_branch=((256, ), ),
+ num_attrs=0,
+ bbox_code_size=7,
+ dir_branch=(),
+ attr_branch=(),
+ bbox_coder=dict(
+ type='SMOKECoder',
+ base_depth=(28.01, 16.32),
+ base_dims=((0.88, 1.73, 0.67), (1.78, 1.70, 0.58), (3.88, 1.63,
+ 1.53)),
+ code_size=7),
+ loss_cls=dict(type='GaussianFocalLoss', loss_weight=1.0),
+ loss_bbox=dict(type='L1Loss', reduction='sum', loss_weight=1 / 300),
+ loss_dir=dict(
+ type='CrossEntropyLoss', use_sigmoid=False, loss_weight=1.0),
+ loss_attr=None,
+ conv_bias=True,
+ dcn_on_last_conv=False)
+
+ self = build_head(head_cfg)
+
+ feats = [torch.rand([2, 64, 32, 32], dtype=torch.float32)]
+
+ # test forward
+ ret_dict = self(feats)
+
+ assert len(ret_dict) == 2
+ assert len(ret_dict[0]) == 1
+ assert ret_dict[0][0].shape == torch.Size([2, 3, 32, 32])
+ assert ret_dict[1][0].shape == torch.Size([2, 8, 32, 32])
+
+ # test loss
+ gt_bboxes = [
+ torch.Tensor([[1.0, 2.0, 20.0, 40.0], [45.0, 50.0, 80.0, 70.1],
+ [34.0, 39.0, 65.0, 64.0]]),
+ torch.Tensor([[11.0, 22.0, 29.0, 31.0], [41.0, 55.0, 60.0, 99.0],
+ [29.0, 29.0, 65.0, 56.0]])
+ ]
+ gt_bboxes_3d = [
+ CameraInstance3DBoxes(torch.rand([3, 7]), box_dim=7),
+ CameraInstance3DBoxes(torch.rand([3, 7]), box_dim=7)
+ ]
+ gt_labels = [torch.randint(0, 3, [3]) for i in range(2)]
+ gt_labels_3d = gt_labels
+ centers2d = [torch.randint(0, 60, (3, 2)), torch.randint(0, 40, (3, 2))]
+ depths = [
+ torch.rand([3], dtype=torch.float32),
+ torch.rand([3], dtype=torch.float32)
+ ]
+ attr_labels = None
+ img_metas = [
+ dict(
+ cam2img=[[1260.8474446004698, 0.0, 807.968244525554, 40.1111],
+ [0.0, 1260.8474446004698, 495.3344268742088, 2.34422],
+ [0.0, 0.0, 1.0, 0.00333333], [0.0, 0.0, 0.0, 1.0]],
+ scale_factor=np.array([1., 1., 1., 1.], dtype=np.float32),
+ pad_shape=[128, 128],
+ trans_mat=np.array([[0.25, 0., 0.], [0., 0.25, 0], [0., 0., 1.]],
+ dtype=np.float32),
+ affine_aug=False,
+ box_type_3d=CameraInstance3DBoxes) for i in range(2)
+ ]
+ losses = self.loss(*ret_dict, gt_bboxes, gt_labels, gt_bboxes_3d,
+ gt_labels_3d, centers2d, depths, attr_labels, img_metas)
+
+ assert losses['loss_cls'] >= 0
+ assert losses['loss_bbox'] >= 0
+
+ # test get_boxes
+ results = self.get_bboxes(*ret_dict, img_metas)
+ assert len(results) == 2
+ assert len(results[0]) == 4
+ assert results[0][0].tensor.shape == torch.Size([100, 7])
+ assert results[0][1].shape == torch.Size([100])
+ assert results[0][2].shape == torch.Size([100])
+ assert results[0][3] is None
+
+
+def test_parta2_bbox_head():
+ if not torch.cuda.is_available():
+ pytest.skip('test requires GPU and torch+cuda')
+ parta2_bbox_head_cfg = _get_parta2_bbox_head_cfg(
+ './parta2/hv_PartA2_secfpn_2x8_cyclic_80e_kitti-3d-3class.py')
+ self = build_head(parta2_bbox_head_cfg).cuda()
+ seg_feats = torch.rand([256, 14, 14, 14, 16]).cuda()
+ part_feats = torch.rand([256, 14, 14, 14, 4]).cuda()
+
+ cls_score, bbox_pred = self.forward(seg_feats, part_feats)
+ assert cls_score.shape == (256, 1)
+ assert bbox_pred.shape == (256, 7)
+
+
+def test_point_rcnn_bbox_head():
+ if not torch.cuda.is_available():
+ pytest.skip('test requires GPU and torch+cuda')
+ pointrcnn_bbox_head_cfg = _get_pointrcnn_bbox_head_cfg(
+ './point_rcnn/point_rcnn_2x8_kitti-3d-3classes.py')
+ self = build_head(pointrcnn_bbox_head_cfg).cuda()
+ feats = torch.rand([100, 512, 133]).cuda()
+ rcnn_cls, rcnn_reg = self.forward(feats)
+ assert rcnn_cls.shape == (100, 1)
+ assert rcnn_reg.shape == (100, 7)
+
+
+def test_part_aggregation_ROI_head():
+ if not torch.cuda.is_available():
+ pytest.skip('test requires GPU and torch+cuda')
+
+ roi_head_cfg = _get_roi_head_cfg(
+ 'parta2/hv_PartA2_secfpn_2x8_cyclic_80e_kitti-3d-3class.py')
+ self = build_head(roi_head_cfg).cuda()
+
+ features = np.load('./tests/test_samples/parta2_roihead_inputs.npz')
+ seg_features = torch.tensor(
+ features['seg_features'], dtype=torch.float32, device='cuda')
+ feats_dict = dict(seg_features=seg_features)
+
+ voxels = torch.tensor(
+ features['voxels'], dtype=torch.float32, device='cuda')
+ num_points = torch.ones([500], device='cuda')
+ coors = torch.zeros([500, 4], device='cuda')
+ voxel_centers = torch.zeros([500, 3], device='cuda')
+ box_type_3d = LiDARInstance3DBoxes
+ img_metas = [dict(box_type_3d=box_type_3d)]
+ voxels_dict = dict(
+ voxels=voxels,
+ num_points=num_points,
+ coors=coors,
+ voxel_centers=voxel_centers)
+
+ pred_bboxes = LiDARInstance3DBoxes(
+ torch.tensor(
+ [[0.3990, 0.5167, 0.0249, 0.9401, 0.9459, 0.7967, 0.4150],
+ [0.8203, 0.2290, 0.9096, 0.1183, 0.0752, 0.4092, 0.9601],
+ [0.2093, 0.1940, 0.8909, 0.4387, 0.3570, 0.5454, 0.8299],
+ [0.2099, 0.7684, 0.4290, 0.2117, 0.6606, 0.1654, 0.4250],
+ [0.9927, 0.6964, 0.2472, 0.7028, 0.7494, 0.9303, 0.0494]],
+ dtype=torch.float32,
+ device='cuda'))
+ pred_scores = torch.tensor([0.9722, 0.7910, 0.4690, 0.3300, 0.3345],
+ dtype=torch.float32,
+ device='cuda')
+ pred_labels = torch.tensor([0, 1, 0, 2, 1],
+ dtype=torch.int64,
+ device='cuda')
+ pred_clses = torch.tensor(
+ [[0.7874, 0.1344, 0.2190], [0.8193, 0.6969, 0.7304],
+ [0.2328, 0.9028, 0.3900], [0.6177, 0.5012, 0.2330],
+ [0.8985, 0.4894, 0.7152]],
+ dtype=torch.float32,
+ device='cuda')
+ proposal = dict(
+ boxes_3d=pred_bboxes,
+ scores_3d=pred_scores,
+ labels_3d=pred_labels,
+ cls_preds=pred_clses)
+ proposal_list = [proposal]
+ gt_bboxes_3d = [LiDARInstance3DBoxes(torch.rand([5, 7], device='cuda'))]
+ gt_labels_3d = [torch.randint(0, 3, [5], device='cuda')]
+
+ losses = self.forward_train(feats_dict, voxels_dict, {}, proposal_list,
+ gt_bboxes_3d, gt_labels_3d)
+ assert losses['loss_seg'] >= 0
+ assert losses['loss_part'] >= 0
+ assert losses['loss_cls'] >= 0
+ assert losses['loss_bbox'] >= 0
+ assert losses['loss_corner'] >= 0
+
+ bbox_results = self.simple_test(feats_dict, voxels_dict, img_metas,
+ proposal_list)
+ boxes_3d = bbox_results[0]['boxes_3d']
+ scores_3d = bbox_results[0]['scores_3d']
+ labels_3d = bbox_results[0]['labels_3d']
+ assert boxes_3d.tensor.shape == (12, 7)
+ assert scores_3d.shape == (12, )
+ assert labels_3d.shape == (12, )
+
+
+def test_point_rcnn_roi_head():
+ if not torch.cuda.is_available():
+ pytest.skip('test requires GPU and torch+cuda')
+
+ roi_head_cfg = _get_roi_head_cfg(
+ './point_rcnn/point_rcnn_2x8_kitti-3d-3classes.py')
+
+ self = build_head(roi_head_cfg).cuda()
+
+ features = torch.rand([3, 128, 16384]).cuda()
+ points = torch.rand([3, 16384, 3]).cuda()
+ points_cls_preds = torch.rand([3, 16384, 3]).cuda()
+ rcnn_feats = {
+ 'features': features,
+ 'points': points,
+ 'points_cls_preds': points_cls_preds
+ }
+ boxes_3d = LiDARInstance3DBoxes(torch.rand(50, 7).cuda())
+ labels_3d = torch.randint(low=0, high=2, size=[50]).cuda()
+ proposal = {'boxes_3d': boxes_3d, 'labels_3d': labels_3d}
+ proposal_list = [proposal for i in range(3)]
+ gt_bboxes_3d = [
+ LiDARInstance3DBoxes(torch.rand([5, 7], device='cuda'))
+ for i in range(3)
+ ]
+ gt_labels_3d = [torch.randint(0, 2, [5], device='cuda') for i in range(3)]
+ box_type_3d = LiDARInstance3DBoxes
+ img_metas = [dict(box_type_3d=box_type_3d) for i in range(3)]
+
+ losses = self.forward_train(rcnn_feats, img_metas, proposal_list,
+ gt_bboxes_3d, gt_labels_3d)
+ assert losses['loss_cls'] >= 0
+ assert losses['loss_bbox'] >= 0
+ assert losses['loss_corner'] >= 0
+
+ bbox_results = self.simple_test(rcnn_feats, img_metas, proposal_list)
+ boxes_3d = bbox_results[0]['boxes_3d']
+ scores_3d = bbox_results[0]['scores_3d']
+ labels_3d = bbox_results[0]['labels_3d']
+ assert boxes_3d.tensor.shape[1] == 7
+ assert boxes_3d.tensor.shape[0] == scores_3d.shape[0]
+ assert scores_3d.shape[0] == labels_3d.shape[0]
+
+
+def test_free_anchor_3D_head():
+ if not torch.cuda.is_available():
+ pytest.skip('test requires GPU and torch+cuda')
+ _setup_seed(0)
+ pts_bbox_head_cfg = _get_pts_bbox_head_cfg(
+ './free_anchor/hv_pointpillars_fpn_sbn-all_'
+ 'free-anchor_4x8_2x_nus-3d.py')
+ self = build_head(pts_bbox_head_cfg)
+ cls_scores = [
+ torch.rand([4, 80, 200, 200], device='cuda') for i in range(3)
+ ]
+ bbox_preds = [
+ torch.rand([4, 72, 200, 200], device='cuda') for i in range(3)
+ ]
+ dir_cls_preds = [
+ torch.rand([4, 16, 200, 200], device='cuda') for i in range(3)
+ ]
+ gt_bboxes = [
+ LiDARInstance3DBoxes(torch.rand([8, 9], device='cuda'), box_dim=9)
+ for i in range(4)
+ ]
+ gt_labels = [
+ torch.randint(0, 10, [8], device='cuda', dtype=torch.long)
+ for i in range(4)
+ ]
+ input_metas = [0]
+ losses = self.loss(cls_scores, bbox_preds, dir_cls_preds, gt_bboxes,
+ gt_labels, input_metas, None)
+ assert losses['positive_bag_loss'] >= 0
+ assert losses['negative_bag_loss'] >= 0
+
+
+def test_primitive_head():
+ if not torch.cuda.is_available():
+ pytest.skip('test requires GPU and torch+cuda')
+ _setup_seed(0)
+
+ primitive_head_cfg = dict(
+ type='PrimitiveHead',
+ num_dims=2,
+ num_classes=18,
+ primitive_mode='z',
+ vote_module_cfg=dict(
+ in_channels=256,
+ vote_per_seed=1,
+ gt_per_seed=1,
+ conv_channels=(256, 256),
+ conv_cfg=dict(type='Conv1d'),
+ norm_cfg=dict(type='BN1d'),
+ norm_feats=True,
+ vote_loss=dict(
+ type='ChamferDistance',
+ mode='l1',
+ reduction='none',
+ loss_dst_weight=10.0)),
+ vote_aggregation_cfg=dict(
+ type='PointSAModule',
+ num_point=64,
+ radius=0.3,
+ num_sample=16,
+ mlp_channels=[256, 128, 128, 128],
+ use_xyz=True,
+ normalize_xyz=True),
+ feat_channels=(128, 128),
+ conv_cfg=dict(type='Conv1d'),
+ norm_cfg=dict(type='BN1d'),
+ objectness_loss=dict(
+ type='CrossEntropyLoss',
+ class_weight=[0.4, 0.6],
+ reduction='mean',
+ loss_weight=1.0),
+ center_loss=dict(
+ type='ChamferDistance',
+ mode='l1',
+ reduction='sum',
+ loss_src_weight=1.0,
+ loss_dst_weight=1.0),
+ semantic_reg_loss=dict(
+ type='ChamferDistance',
+ mode='l1',
+ reduction='sum',
+ loss_src_weight=1.0,
+ loss_dst_weight=1.0),
+ semantic_cls_loss=dict(
+ type='CrossEntropyLoss', reduction='sum', loss_weight=1.0),
+ train_cfg=dict(
+ dist_thresh=0.2,
+ var_thresh=1e-2,
+ lower_thresh=1e-6,
+ num_point=100,
+ num_point_line=10,
+ line_thresh=0.2))
+
+ self = build_head(primitive_head_cfg).cuda()
+ fp_xyz = [torch.rand([2, 64, 3], dtype=torch.float32).cuda()]
+ hd_features = torch.rand([2, 256, 64], dtype=torch.float32).cuda()
+ fp_indices = [torch.randint(0, 64, [2, 64]).cuda()]
+ input_dict = dict(
+ fp_xyz_net0=fp_xyz, hd_feature=hd_features, fp_indices_net0=fp_indices)
+
+ # test forward
+ ret_dict = self(input_dict, 'vote')
+ assert ret_dict['center_z'].shape == torch.Size([2, 64, 3])
+ assert ret_dict['size_residuals_z'].shape == torch.Size([2, 64, 2])
+ assert ret_dict['sem_cls_scores_z'].shape == torch.Size([2, 64, 18])
+ assert ret_dict['aggregated_points_z'].shape == torch.Size([2, 64, 3])
+
+ # test loss
+ points = torch.rand([2, 1024, 3], dtype=torch.float32).cuda()
+ ret_dict['seed_points'] = fp_xyz[0]
+ ret_dict['seed_indices'] = fp_indices[0]
+
+ from mmdet3d.core.bbox import DepthInstance3DBoxes
+ gt_bboxes_3d = [
+ DepthInstance3DBoxes(torch.rand([4, 7], dtype=torch.float32).cuda()),
+ DepthInstance3DBoxes(torch.rand([4, 7], dtype=torch.float32).cuda())
+ ]
+ gt_labels_3d = torch.randint(0, 18, [2, 4]).cuda()
+ gt_labels_3d = [gt_labels_3d[0], gt_labels_3d[1]]
+ pts_semantic_mask = torch.randint(0, 19, [2, 1024]).cuda()
+ pts_semantic_mask = [pts_semantic_mask[0], pts_semantic_mask[1]]
+ pts_instance_mask = torch.randint(0, 4, [2, 1024]).cuda()
+ pts_instance_mask = [pts_instance_mask[0], pts_instance_mask[1]]
+
+ loss_input_dict = dict(
+ bbox_preds=ret_dict,
+ points=points,
+ gt_bboxes_3d=gt_bboxes_3d,
+ gt_labels_3d=gt_labels_3d,
+ pts_semantic_mask=pts_semantic_mask,
+ pts_instance_mask=pts_instance_mask)
+ losses_dict = self.loss(**loss_input_dict)
+
+ assert losses_dict['flag_loss_z'] >= 0
+ assert losses_dict['vote_loss_z'] >= 0
+ assert losses_dict['center_loss_z'] >= 0
+ assert losses_dict['size_loss_z'] >= 0
+ assert losses_dict['sem_loss_z'] >= 0
+
+ # 'Primitive_mode' should be one of ['z', 'xy', 'line']
+ with pytest.raises(AssertionError):
+ primitive_head_cfg['vote_module_cfg']['in_channels'] = 'xyz'
+ build_head(primitive_head_cfg)
+
+
+def test_h3d_head():
+ if not torch.cuda.is_available():
+ pytest.skip('test requires GPU and torch+cuda')
+ _setup_seed(0)
+
+ h3d_head_cfg = _get_roi_head_cfg('h3dnet/h3dnet_3x8_scannet-3d-18class.py')
+
+ num_point = 128
+ num_proposal = 64
+ h3d_head_cfg.primitive_list[0].vote_aggregation_cfg.num_point = num_point
+ h3d_head_cfg.primitive_list[1].vote_aggregation_cfg.num_point = num_point
+ h3d_head_cfg.primitive_list[2].vote_aggregation_cfg.num_point = num_point
+ h3d_head_cfg.bbox_head.num_proposal = num_proposal
+ self = build_head(h3d_head_cfg).cuda()
+
+ # prepare RoI outputs
+ fp_xyz = [torch.rand([1, num_point, 3], dtype=torch.float32).cuda()]
+ hd_features = torch.rand([1, 256, num_point], dtype=torch.float32).cuda()
+ fp_indices = [torch.randint(0, 128, [1, num_point]).cuda()]
+ aggregated_points = torch.rand([1, num_proposal, 3],
+ dtype=torch.float32).cuda()
+ aggregated_features = torch.rand([1, 128, num_proposal],
+ dtype=torch.float32).cuda()
+ proposal_list = torch.cat([
+ torch.rand([1, num_proposal, 3], dtype=torch.float32).cuda() * 4 - 2,
+ torch.rand([1, num_proposal, 3], dtype=torch.float32).cuda() * 4,
+ torch.zeros([1, num_proposal, 1]).cuda()
+ ],
+ dim=-1)
+
+ input_dict = dict(
+ fp_xyz_net0=fp_xyz,
+ hd_feature=hd_features,
+ aggregated_points=aggregated_points,
+ aggregated_features=aggregated_features,
+ seed_points=fp_xyz[0],
+ seed_indices=fp_indices[0],
+ proposal_list=proposal_list)
+
+ # prepare gt label
+ from mmdet3d.core.bbox import DepthInstance3DBoxes
+ gt_bboxes_3d = [
+ DepthInstance3DBoxes(torch.rand([4, 7], dtype=torch.float32).cuda()),
+ DepthInstance3DBoxes(torch.rand([4, 7], dtype=torch.float32).cuda())
+ ]
+ gt_labels_3d = torch.randint(0, 18, [1, 4]).cuda()
+ gt_labels_3d = [gt_labels_3d[0]]
+ pts_semantic_mask = torch.randint(0, 19, [1, num_point]).cuda()
+ pts_semantic_mask = [pts_semantic_mask[0]]
+ pts_instance_mask = torch.randint(0, 4, [1, num_point]).cuda()
+ pts_instance_mask = [pts_instance_mask[0]]
+ points = torch.rand([1, num_point, 3], dtype=torch.float32).cuda()
+
+ # prepare rpn targets
+ vote_targets = torch.rand([1, num_point, 9], dtype=torch.float32).cuda()
+ vote_target_masks = torch.rand([1, num_point], dtype=torch.float32).cuda()
+ size_class_targets = torch.rand([1, num_proposal],
+ dtype=torch.float32).cuda().long()
+ size_res_targets = torch.rand([1, num_proposal, 3],
+ dtype=torch.float32).cuda()
+ dir_class_targets = torch.rand([1, num_proposal],
+ dtype=torch.float32).cuda().long()
+ dir_res_targets = torch.rand([1, num_proposal], dtype=torch.float32).cuda()
+ center_targets = torch.rand([1, 4, 3], dtype=torch.float32).cuda()
+ mask_targets = torch.rand([1, num_proposal],
+ dtype=torch.float32).cuda().long()
+ valid_gt_masks = torch.rand([1, 4], dtype=torch.float32).cuda()
+ objectness_targets = torch.rand([1, num_proposal],
+ dtype=torch.float32).cuda().long()
+ objectness_weights = torch.rand([1, num_proposal],
+ dtype=torch.float32).cuda()
+ box_loss_weights = torch.rand([1, num_proposal],
+ dtype=torch.float32).cuda()
+ valid_gt_weights = torch.rand([1, 4], dtype=torch.float32).cuda()
+
+ targets = (vote_targets, vote_target_masks, size_class_targets,
+ size_res_targets, dir_class_targets, dir_res_targets,
+ center_targets, None, mask_targets, valid_gt_masks,
+ objectness_targets, objectness_weights, box_loss_weights,
+ valid_gt_weights)
+
+ input_dict['targets'] = targets
+
+ # train forward
+ ret_dict = self.forward_train(
+ input_dict,
+ points=points,
+ gt_bboxes_3d=gt_bboxes_3d,
+ gt_labels_3d=gt_labels_3d,
+ pts_semantic_mask=pts_semantic_mask,
+ pts_instance_mask=pts_instance_mask,
+ img_metas=None)
+
+ assert ret_dict['flag_loss_z'] >= 0
+ assert ret_dict['vote_loss_z'] >= 0
+ assert ret_dict['center_loss_z'] >= 0
+ assert ret_dict['size_loss_z'] >= 0
+ assert ret_dict['sem_loss_z'] >= 0
+ assert ret_dict['objectness_loss_optimized'] >= 0
+ assert ret_dict['primitive_sem_matching_loss'] >= 0
+
+
+def test_center_head():
+ tasks = [
+ dict(num_class=1, class_names=['car']),
+ dict(num_class=2, class_names=['truck', 'construction_vehicle']),
+ dict(num_class=2, class_names=['bus', 'trailer']),
+ dict(num_class=1, class_names=['barrier']),
+ dict(num_class=2, class_names=['motorcycle', 'bicycle']),
+ dict(num_class=2, class_names=['pedestrian', 'traffic_cone']),
+ ]
+ bbox_cfg = dict(
+ type='CenterPointBBoxCoder',
+ post_center_range=[-61.2, -61.2, -10.0, 61.2, 61.2, 10.0],
+ max_num=500,
+ score_threshold=0.1,
+ pc_range=[-51.2, -51.2],
+ out_size_factor=8,
+ voxel_size=[0.2, 0.2])
+ train_cfg = dict(
+ grid_size=[1024, 1024, 40],
+ point_cloud_range=[-51.2, -51.2, -5., 51.2, 51.2, 3.],
+ voxel_size=[0.1, 0.1, 0.2],
+ out_size_factor=8,
+ dense_reg=1,
+ gaussian_overlap=0.1,
+ max_objs=500,
+ code_weights=[1.0, 1.0, 1.0, 1.0, 1.0, 1.0, 0.2, 0.2, 1.0, 1.0],
+ min_radius=2)
+ test_cfg = dict(
+ post_center_limit_range=[-61.2, -61.2, -10.0, 61.2, 61.2, 10.0],
+ max_per_img=500,
+ max_pool_nms=False,
+ min_radius=[4, 12, 10, 1, 0.85, 0.175],
+ post_max_size=83,
+ score_threshold=0.1,
+ pc_range=[-51.2, -51.2],
+ out_size_factor=8,
+ voxel_size=[0.2, 0.2],
+ nms_type='circle')
+ center_head_cfg = dict(
+ type='CenterHead',
+ in_channels=sum([256, 256]),
+ tasks=tasks,
+ train_cfg=train_cfg,
+ test_cfg=test_cfg,
+ bbox_coder=bbox_cfg,
+ common_heads=dict(
+ reg=(2, 2), height=(1, 2), dim=(3, 2), rot=(2, 2), vel=(2, 2)),
+ share_conv_channel=64,
+ norm_bbox=True)
+
+ center_head = build_head(center_head_cfg)
+
+ x = torch.rand([2, 512, 128, 128])
+ output = center_head([x])
+ for i in range(6):
+ assert output[i][0]['reg'].shape == torch.Size([2, 2, 128, 128])
+ assert output[i][0]['height'].shape == torch.Size([2, 1, 128, 128])
+ assert output[i][0]['dim'].shape == torch.Size([2, 3, 128, 128])
+ assert output[i][0]['rot'].shape == torch.Size([2, 2, 128, 128])
+ assert output[i][0]['vel'].shape == torch.Size([2, 2, 128, 128])
+ assert output[i][0]['heatmap'].shape == torch.Size(
+ [2, tasks[i]['num_class'], 128, 128])
+
+ # test get_bboxes
+ img_metas = [
+ dict(box_type_3d=LiDARInstance3DBoxes),
+ dict(box_type_3d=LiDARInstance3DBoxes)
+ ]
+ ret_lists = center_head.get_bboxes(output, img_metas)
+ for ret_list in ret_lists:
+ assert ret_list[0].tensor.shape[0] <= 500
+ assert ret_list[1].shape[0] <= 500
+ assert ret_list[2].shape[0] <= 500
+
+
+def test_dcn_center_head():
+ if not torch.cuda.is_available():
+ pytest.skip('test requires GPU and CUDA')
+ set_random_seed(0)
+ tasks = [
+ dict(num_class=1, class_names=['car']),
+ dict(num_class=2, class_names=['truck', 'construction_vehicle']),
+ dict(num_class=2, class_names=['bus', 'trailer']),
+ dict(num_class=1, class_names=['barrier']),
+ dict(num_class=2, class_names=['motorcycle', 'bicycle']),
+ dict(num_class=2, class_names=['pedestrian', 'traffic_cone']),
+ ]
+ voxel_size = [0.2, 0.2, 8]
+ dcn_center_head_cfg = dict(
+ type='CenterHead',
+ in_channels=sum([128, 128, 128]),
+ tasks=[
+ dict(num_class=1, class_names=['car']),
+ dict(num_class=2, class_names=['truck', 'construction_vehicle']),
+ dict(num_class=2, class_names=['bus', 'trailer']),
+ dict(num_class=1, class_names=['barrier']),
+ dict(num_class=2, class_names=['motorcycle', 'bicycle']),
+ dict(num_class=2, class_names=['pedestrian', 'traffic_cone']),
+ ],
+ common_heads={
+ 'reg': (2, 2),
+ 'height': (1, 2),
+ 'dim': (3, 2),
+ 'rot': (2, 2),
+ 'vel': (2, 2)
+ },
+ share_conv_channel=64,
+ bbox_coder=dict(
+ type='CenterPointBBoxCoder',
+ post_center_range=[-61.2, -61.2, -10.0, 61.2, 61.2, 10.0],
+ max_num=500,
+ score_threshold=0.1,
+ pc_range=[-51.2, -51.2],
+ out_size_factor=4,
+ voxel_size=voxel_size[:2],
+ code_size=9),
+ separate_head=dict(
+ type='DCNSeparateHead',
+ dcn_config=dict(
+ type='DCN',
+ in_channels=64,
+ out_channels=64,
+ kernel_size=3,
+ padding=1,
+ groups=4,
+ bias=False), # mmcv 1.2.6 doesn't support bias=True anymore
+ init_bias=-2.19,
+ final_kernel=3),
+ loss_cls=dict(type='GaussianFocalLoss', reduction='mean'),
+ loss_bbox=dict(type='L1Loss', reduction='none', loss_weight=0.25),
+ norm_bbox=True)
+ # model training and testing settings
+ train_cfg = dict(
+ grid_size=[512, 512, 1],
+ point_cloud_range=[-51.2, -51.2, -5., 51.2, 51.2, 3.],
+ voxel_size=voxel_size,
+ out_size_factor=4,
+ dense_reg=1,
+ gaussian_overlap=0.1,
+ max_objs=500,
+ min_radius=2,
+ code_weights=[1.0, 1.0, 1.0, 1.0, 1.0, 1.0, 0.2, 0.2, 1.0, 1.0])
+
+ test_cfg = dict(
+ post_center_limit_range=[-61.2, -61.2, -10.0, 61.2, 61.2, 10.0],
+ max_per_img=500,
+ max_pool_nms=False,
+ min_radius=[4, 12, 10, 1, 0.85, 0.175],
+ post_max_size=83,
+ score_threshold=0.1,
+ pc_range=[-51.2, -51.2],
+ out_size_factor=4,
+ voxel_size=voxel_size[:2],
+ nms_type='circle')
+ dcn_center_head_cfg.update(train_cfg=train_cfg, test_cfg=test_cfg)
+
+ dcn_center_head = build_head(dcn_center_head_cfg).cuda()
+
+ x = torch.ones([2, 384, 128, 128]).cuda()
+ output = dcn_center_head([x])
+ for i in range(6):
+ assert output[i][0]['reg'].shape == torch.Size([2, 2, 128, 128])
+ assert output[i][0]['height'].shape == torch.Size([2, 1, 128, 128])
+ assert output[i][0]['dim'].shape == torch.Size([2, 3, 128, 128])
+ assert output[i][0]['rot'].shape == torch.Size([2, 2, 128, 128])
+ assert output[i][0]['vel'].shape == torch.Size([2, 2, 128, 128])
+ assert output[i][0]['heatmap'].shape == torch.Size(
+ [2, tasks[i]['num_class'], 128, 128])
+
+ # Test loss.
+ gt_bboxes_0 = LiDARInstance3DBoxes(torch.rand([10, 9]).cuda(), box_dim=9)
+ gt_bboxes_1 = LiDARInstance3DBoxes(torch.rand([20, 9]).cuda(), box_dim=9)
+ gt_labels_0 = torch.randint(1, 11, [10]).cuda()
+ gt_labels_1 = torch.randint(1, 11, [20]).cuda()
+ gt_bboxes_3d = [gt_bboxes_0, gt_bboxes_1]
+ gt_labels_3d = [gt_labels_0, gt_labels_1]
+ loss = dcn_center_head.loss(gt_bboxes_3d, gt_labels_3d, output)
+ for key, item in loss.items():
+ if 'heatmap' in key:
+ assert item >= 0
+ else:
+ assert torch.sum(item) >= 0
+
+ # test get_bboxes
+ img_metas = [
+ dict(box_type_3d=LiDARInstance3DBoxes),
+ dict(box_type_3d=LiDARInstance3DBoxes)
+ ]
+ ret_lists = dcn_center_head.get_bboxes(output, img_metas)
+ for ret_list in ret_lists:
+ assert ret_list[0].tensor.shape[0] <= 500
+ assert ret_list[1].shape[0] <= 500
+ assert ret_list[2].shape[0] <= 500
+
+
+def test_ssd3d_head():
+ if not torch.cuda.is_available():
+ pytest.skip('test requires GPU and torch+cuda')
+ _setup_seed(0)
+ ssd3d_head_cfg = _get_vote_head_cfg('3dssd/3dssd_4x4_kitti-3d-car.py')
+ ssd3d_head_cfg.vote_module_cfg.num_points = 64
+ self = build_head(ssd3d_head_cfg).cuda()
+ sa_xyz = [torch.rand([2, 128, 3], dtype=torch.float32).cuda()]
+ sa_features = [torch.rand([2, 256, 128], dtype=torch.float32).cuda()]
+ sa_indices = [torch.randint(0, 64, [2, 128]).cuda()]
+
+ input_dict = dict(
+ sa_xyz=sa_xyz, sa_features=sa_features, sa_indices=sa_indices)
+
+ # test forward
+ ret_dict = self(input_dict, 'spec')
+ assert ret_dict['center'].shape == torch.Size([2, 64, 3])
+ assert ret_dict['obj_scores'].shape == torch.Size([2, 1, 64])
+ assert ret_dict['size'].shape == torch.Size([2, 64, 3])
+ assert ret_dict['dir_res'].shape == torch.Size([2, 64, 12])
+
+ # test loss
+ points = [torch.rand([4000, 3], device='cuda') for i in range(2)]
+ gt_bbox1 = LiDARInstance3DBoxes(torch.rand([5, 7], device='cuda'))
+ gt_bbox2 = LiDARInstance3DBoxes(torch.rand([5, 7], device='cuda'))
+ gt_bboxes = [gt_bbox1, gt_bbox2]
+ gt_labels = [
+ torch.zeros([5], dtype=torch.long, device='cuda') for i in range(2)
+ ]
+ img_metas = [dict(box_type_3d=LiDARInstance3DBoxes) for i in range(2)]
+ losses = self.loss(
+ ret_dict, points, gt_bboxes, gt_labels, img_metas=img_metas)
+
+ assert losses['centerness_loss'] >= 0
+ assert losses['center_loss'] >= 0
+ assert losses['dir_class_loss'] >= 0
+ assert losses['dir_res_loss'] >= 0
+ assert losses['size_res_loss'] >= 0
+ assert losses['corner_loss'] >= 0
+ assert losses['vote_loss'] >= 0
+
+ # test multiclass_nms_single
+ sem_scores = ret_dict['obj_scores'].transpose(1, 2)[0]
+ obj_scores = sem_scores.max(-1)[0]
+ bbox = self.bbox_coder.decode(ret_dict)[0]
+ input_meta = img_metas[0]
+ bbox_selected, score_selected, labels = self.multiclass_nms_single(
+ obj_scores, sem_scores, bbox, points[0], input_meta)
+ assert bbox_selected.shape[0] >= 0
+ assert bbox_selected.shape[1] == 7
+ assert score_selected.shape[0] >= 0
+ assert labels.shape[0] >= 0
+
+ # test get_boxes
+ points = torch.stack(points, 0)
+ results = self.get_bboxes(points, ret_dict, img_metas)
+ assert results[0][0].tensor.shape[0] >= 0
+ assert results[0][0].tensor.shape[1] == 7
+ assert results[0][1].shape[0] >= 0
+ assert results[0][2].shape[0] >= 0
+
+
+def test_shape_aware_head_loss():
+ if not torch.cuda.is_available():
+ pytest.skip('test requires GPU and torch+cuda')
+ bbox_head_cfg = _get_pts_bbox_head_cfg(
+ 'ssn/hv_ssn_secfpn_sbn-all_2x16_2x_lyft-3d.py')
+ # modify bn config to avoid bugs caused by syncbn
+ for task in bbox_head_cfg['tasks']:
+ task['norm_cfg'] = dict(type='BN2d')
+
+ from mmdet3d.models.builder import build_head
+ self = build_head(bbox_head_cfg)
+ self.cuda()
+ assert len(self.heads) == 4
+ assert isinstance(self.heads[0].conv_cls, torch.nn.modules.conv.Conv2d)
+ assert self.heads[0].conv_cls.in_channels == 64
+ assert self.heads[0].conv_cls.out_channels == 36
+ assert self.heads[0].conv_reg.out_channels == 28
+ assert self.heads[0].conv_dir_cls.out_channels == 8
+
+ # test forward
+ feats = list()
+ feats.append(torch.rand([2, 384, 200, 200], dtype=torch.float32).cuda())
+ (cls_score, bbox_pred, dir_cls_preds) = self.forward(feats)
+ assert cls_score[0].shape == torch.Size([2, 420000, 9])
+ assert bbox_pred[0].shape == torch.Size([2, 420000, 7])
+ assert dir_cls_preds[0].shape == torch.Size([2, 420000, 2])
+
+ # test loss
+ gt_bboxes = [
+ LiDARInstance3DBoxes(
+ torch.tensor(
+ [[-14.5695, -6.4169, -2.1054, 1.8830, 4.6720, 1.4840, 1.5587],
+ [25.7215, 3.4581, -1.3456, 1.6720, 4.4090, 1.5830, 1.5301]],
+ dtype=torch.float32).cuda()),
+ LiDARInstance3DBoxes(
+ torch.tensor(
+ [[-50.763, -3.5517, -0.99658, 1.7430, 4.4020, 1.6990, 1.7874],
+ [-68.720, 0.033, -0.75276, 1.7860, 4.9100, 1.6610, 1.7525]],
+ dtype=torch.float32).cuda())
+ ]
+ gt_labels = list(torch.tensor([[4, 4], [4, 4]], dtype=torch.int64).cuda())
+ input_metas = [{
+ 'sample_idx': 1234
+ }, {
+ 'sample_idx': 2345
+ }] # fake input_metas
+
+ losses = self.loss(cls_score, bbox_pred, dir_cls_preds, gt_bboxes,
+ gt_labels, input_metas)
+
+ assert losses['loss_cls'][0] > 0
+ assert losses['loss_bbox'][0] > 0
+ assert losses['loss_dir'][0] > 0
+
+ # test empty ground truth case
+ gt_bboxes = list(torch.empty((2, 0, 7)).cuda())
+ gt_labels = list(torch.empty((2, 0)).cuda())
+ empty_gt_losses = self.loss(cls_score, bbox_pred, dir_cls_preds, gt_bboxes,
+ gt_labels, input_metas)
+ assert empty_gt_losses['loss_cls'][0] > 0
+ assert empty_gt_losses['loss_bbox'][0] == 0
+ assert empty_gt_losses['loss_dir'][0] == 0
+
+
+def test_shape_aware_head_getboxes():
+ if not torch.cuda.is_available():
+ pytest.skip('test requires GPU and torch+cuda')
+ bbox_head_cfg = _get_pts_bbox_head_cfg(
+ 'ssn/hv_ssn_secfpn_sbn-all_2x16_2x_lyft-3d.py')
+ # modify bn config to avoid bugs caused by syncbn
+ for task in bbox_head_cfg['tasks']:
+ task['norm_cfg'] = dict(type='BN2d')
+
+ from mmdet3d.models.builder import build_head
+ self = build_head(bbox_head_cfg)
+ self.cuda()
+
+ feats = list()
+ feats.append(torch.rand([2, 384, 200, 200], dtype=torch.float32).cuda())
+ # fake input_metas
+ input_metas = [{
+ 'sample_idx': 1234,
+ 'box_type_3d': LiDARInstance3DBoxes,
+ 'box_mode_3d': Box3DMode.LIDAR
+ }, {
+ 'sample_idx': 2345,
+ 'box_type_3d': LiDARInstance3DBoxes,
+ 'box_mode_3d': Box3DMode.LIDAR
+ }]
+ (cls_score, bbox_pred, dir_cls_preds) = self.forward(feats)
+
+ # test get_bboxes
+ cls_score[0] -= 1.5 # too many positive samples may cause cuda oom
+ result_list = self.get_bboxes(cls_score, bbox_pred, dir_cls_preds,
+ input_metas)
+ assert len(result_list[0][1]) > 0 # ensure not all boxes are filtered
+ assert (result_list[0][1] > 0.3).all()
+
+
+def test_fcos_mono3d_head():
+ if not torch.cuda.is_available():
+ pytest.skip('test requires GPU and torch+cuda')
+ _setup_seed(0)
+ fcos3d_head_cfg = _get_head_cfg(
+ 'fcos3d/fcos3d_r101_caffe_fpn_gn-head_dcn_2x8_1x_nus-mono3d.py')
+ self = build_head(fcos3d_head_cfg).cuda()
+
+ feats = [
+ torch.rand([2, 256, 116, 200], dtype=torch.float32).cuda(),
+ torch.rand([2, 256, 58, 100], dtype=torch.float32).cuda(),
+ torch.rand([2, 256, 29, 50], dtype=torch.float32).cuda(),
+ torch.rand([2, 256, 15, 25], dtype=torch.float32).cuda(),
+ torch.rand([2, 256, 8, 13], dtype=torch.float32).cuda()
+ ]
+
+ # test forward
+ ret_dict = self(feats)
+ assert len(ret_dict) == 5
+ assert len(ret_dict[0]) == 5
+ assert ret_dict[0][0].shape == torch.Size([2, 10, 116, 200])
+
+ # test loss
+ gt_bboxes = [
+ torch.rand([3, 4], dtype=torch.float32).cuda(),
+ torch.rand([3, 4], dtype=torch.float32).cuda()
+ ]
+ gt_bboxes_3d = CameraInstance3DBoxes(
+ torch.rand([3, 9], device='cuda'), box_dim=9)
+ gt_labels = [torch.randint(0, 10, [3], device='cuda') for i in range(2)]
+ gt_labels_3d = gt_labels
+ centers2d = [
+ torch.rand([3, 2], dtype=torch.float32).cuda(),
+ torch.rand([3, 2], dtype=torch.float32).cuda()
+ ]
+ depths = [
+ torch.rand([3], dtype=torch.float32).cuda(),
+ torch.rand([3], dtype=torch.float32).cuda()
+ ]
+ attr_labels = [torch.randint(0, 9, [3], device='cuda') for i in range(2)]
+ img_metas = [
+ dict(
+ cam2img=[[1260.8474446004698, 0.0, 807.968244525554],
+ [0.0, 1260.8474446004698, 495.3344268742088],
+ [0.0, 0.0, 1.0]],
+ scale_factor=np.array([1., 1., 1., 1.], dtype=np.float32),
+ box_type_3d=CameraInstance3DBoxes) for i in range(2)
+ ]
+ losses = self.loss(*ret_dict, gt_bboxes, gt_labels, gt_bboxes_3d,
+ gt_labels_3d, centers2d, depths, attr_labels, img_metas)
+ assert losses['loss_cls'] >= 0
+ assert losses['loss_offset'] >= 0
+ assert losses['loss_depth'] >= 0
+ assert losses['loss_size'] >= 0
+ assert losses['loss_rotsin'] >= 0
+ assert losses['loss_centerness'] >= 0
+ assert losses['loss_velo'] >= 0
+ assert losses['loss_dir'] >= 0
+ assert losses['loss_attr'] >= 0
+
+ # test get_boxes
+ results = self.get_bboxes(*ret_dict, img_metas)
+ assert len(results) == 2
+ assert len(results[0]) == 4
+ assert results[0][0].tensor.shape == torch.Size([200, 9])
+ assert results[0][1].shape == torch.Size([200])
+ assert results[0][2].shape == torch.Size([200])
+ assert results[0][3].shape == torch.Size([200])
+
+
+def test_groupfree3d_head():
+ if not torch.cuda.is_available():
+ pytest.skip('test requires GPU and torch+cuda')
+ _setup_seed(0)
+ vote_head_cfg = _get_vote_head_cfg(
+ 'groupfree3d/groupfree3d_8x4_scannet-3d-18class-L6-O256.py')
+ self = build_head(vote_head_cfg).cuda()
+
+ fp_xyz = [torch.rand([2, 256, 3], dtype=torch.float32).cuda()]
+ fp_features = [torch.rand([2, 288, 256], dtype=torch.float32).cuda()]
+ fp_indices = [torch.randint(0, 128, [2, 256]).cuda()]
+
+ input_dict = dict(
+ fp_xyz=fp_xyz, fp_features=fp_features, fp_indices=fp_indices)
+
+ # test forward
+ ret_dict = self(input_dict, 'kps')
+ assert ret_dict['seeds_obj_cls_logits'].shape == torch.Size([2, 1, 256])
+ assert ret_dict['s5.center'].shape == torch.Size([2, 256, 3])
+ assert ret_dict['s5.dir_class'].shape == torch.Size([2, 256, 1])
+ assert ret_dict['s5.dir_res'].shape == torch.Size([2, 256, 1])
+ assert ret_dict['s5.size_class'].shape == torch.Size([2, 256, 18])
+ assert ret_dict['s5.size_res'].shape == torch.Size([2, 256, 18, 3])
+ assert ret_dict['s5.obj_scores'].shape == torch.Size([2, 256, 1])
+ assert ret_dict['s5.sem_scores'].shape == torch.Size([2, 256, 18])
+
+ # test losses
+ points = [torch.rand([5000, 4], device='cuda') for i in range(2)]
+ gt_bbox1 = torch.rand([10, 7], dtype=torch.float32).cuda()
+ gt_bbox2 = torch.rand([10, 7], dtype=torch.float32).cuda()
+
+ gt_bbox1 = DepthInstance3DBoxes(gt_bbox1)
+ gt_bbox2 = DepthInstance3DBoxes(gt_bbox2)
+ gt_bboxes = [gt_bbox1, gt_bbox2]
+
+ pts_instance_mask_1 = torch.randint(0, 10, [5000], device='cuda')
+ pts_instance_mask_2 = torch.randint(0, 10, [5000], device='cuda')
+ pts_instance_mask = [pts_instance_mask_1, pts_instance_mask_2]
+
+ pts_semantic_mask_1 = torch.randint(0, 19, [5000], device='cuda')
+ pts_semantic_mask_2 = torch.randint(0, 19, [5000], device='cuda')
+ pts_semantic_mask = [pts_semantic_mask_1, pts_semantic_mask_2]
+
+ labels_1 = torch.randint(0, 18, [10], device='cuda')
+ labels_2 = torch.randint(0, 18, [10], device='cuda')
+ gt_labels = [labels_1, labels_2]
+
+ losses = self.loss(ret_dict, points, gt_bboxes, gt_labels,
+ pts_semantic_mask, pts_instance_mask)
+
+ assert losses['s5.objectness_loss'] >= 0
+ assert losses['s5.semantic_loss'] >= 0
+ assert losses['s5.center_loss'] >= 0
+ assert losses['s5.dir_class_loss'] >= 0
+ assert losses['s5.dir_res_loss'] >= 0
+ assert losses['s5.size_class_loss'] >= 0
+ assert losses['s5.size_res_loss'] >= 0
+
+ # test multiclass_nms_single
+ obj_scores = torch.rand([256], device='cuda')
+ sem_scores = torch.rand([256, 18], device='cuda')
+ points = torch.rand([5000, 3], device='cuda')
+ bbox = torch.rand([256, 7], device='cuda')
+ input_meta = dict(box_type_3d=DepthInstance3DBoxes)
+ bbox_selected, score_selected, labels = \
+ self.multiclass_nms_single(obj_scores,
+ sem_scores,
+ bbox,
+ points,
+ input_meta)
+ assert bbox_selected.shape[0] >= 0
+ assert bbox_selected.shape[1] == 7
+ assert score_selected.shape[0] >= 0
+ assert labels.shape[0] >= 0
+
+ # test get_boxes
+ points = torch.rand([1, 5000, 3], device='cuda')
+ seed_points = torch.rand([1, 1024, 3], device='cuda')
+ seed_indices = torch.randint(0, 5000, [1, 1024], device='cuda')
+ obj_scores = torch.rand([1, 256, 1], device='cuda')
+ center = torch.rand([1, 256, 3], device='cuda')
+ dir_class = torch.rand([1, 256, 1], device='cuda')
+ dir_res_norm = torch.rand([1, 256, 1], device='cuda')
+ dir_res = torch.rand([1, 256, 1], device='cuda')
+ size_class = torch.rand([1, 256, 18], device='cuda')
+ size_res = torch.rand([1, 256, 18, 3], device='cuda')
+ sem_scores = torch.rand([1, 256, 18], device='cuda')
+ bbox_preds = dict()
+ bbox_preds['seed_points'] = seed_points
+ bbox_preds['seed_indices'] = seed_indices
+ bbox_preds['s5.obj_scores'] = obj_scores
+ bbox_preds['s5.center'] = center
+ bbox_preds['s5.dir_class'] = dir_class
+ bbox_preds['s5.dir_res_norm'] = dir_res_norm
+ bbox_preds['s5.dir_res'] = dir_res
+ bbox_preds['s5.size_class'] = size_class
+ bbox_preds['s5.size_res'] = size_res
+ bbox_preds['s5.sem_scores'] = sem_scores
+
+ self.test_cfg['prediction_stages'] = 'last'
+ results = self.get_bboxes(points, bbox_preds, [input_meta])
+ assert results[0][0].tensor.shape[0] >= 0
+ assert results[0][0].tensor.shape[1] == 7
+ assert results[0][1].shape[0] >= 0
+ assert results[0][2].shape[0] >= 0
+
+
+def test_pgd_head():
+ if not torch.cuda.is_available():
+ pytest.skip('test requires GPU and torch+cuda')
+ _setup_seed(0)
+ pgd_head_cfg = _get_head_cfg(
+ 'pgd/pgd_r101_caffe_fpn_gn-head_3x4_4x_kitti-mono3d.py')
+ self = build_head(pgd_head_cfg).cuda()
+
+ feats = [
+ torch.rand([2, 256, 96, 312], dtype=torch.float32).cuda(),
+ torch.rand([2, 256, 48, 156], dtype=torch.float32).cuda(),
+ torch.rand([2, 256, 24, 78], dtype=torch.float32).cuda(),
+ torch.rand([2, 256, 12, 39], dtype=torch.float32).cuda(),
+ ]
+
+ # test forward
+ ret_dict = self(feats)
+ assert len(ret_dict) == 7
+ assert len(ret_dict[0]) == 4
+ assert ret_dict[0][0].shape == torch.Size([2, 3, 96, 312])
+
+ # test loss
+ gt_bboxes = [
+ torch.rand([3, 4], dtype=torch.float32).cuda(),
+ torch.rand([3, 4], dtype=torch.float32).cuda()
+ ]
+ gt_bboxes_3d = CameraInstance3DBoxes(
+ torch.rand([3, 7], device='cuda'), box_dim=7)
+ gt_labels = [torch.randint(0, 3, [3], device='cuda') for i in range(2)]
+ gt_labels_3d = gt_labels
+ centers2d = [
+ torch.rand([3, 2], dtype=torch.float32).cuda(),
+ torch.rand([3, 2], dtype=torch.float32).cuda()
+ ]
+ depths = [
+ torch.rand([3], dtype=torch.float32).cuda(),
+ torch.rand([3], dtype=torch.float32).cuda()
+ ]
+ attr_labels = None
+ img_metas = [
+ dict(
+ img_shape=[384, 1248],
+ cam2img=[[721.5377, 0.0, 609.5593, 44.85728],
+ [0.0, 721.5377, 172.854, 0.2163791],
+ [0.0, 0.0, 1.0, 0.002745884], [0.0, 0.0, 0.0, 1.0]],
+ scale_factor=np.array([1., 1., 1., 1.], dtype=np.float32),
+ box_type_3d=CameraInstance3DBoxes) for i in range(2)
+ ]
+ losses = self.loss(*ret_dict, gt_bboxes, gt_labels, gt_bboxes_3d,
+ gt_labels_3d, centers2d, depths, attr_labels, img_metas)
+ assert losses['loss_cls'] >= 0
+ assert losses['loss_offset'] >= 0
+ assert losses['loss_depth'] >= 0
+ assert losses['loss_size'] >= 0
+ assert losses['loss_rotsin'] >= 0
+ assert losses['loss_centerness'] >= 0
+ assert losses['loss_kpts'] >= 0
+ assert losses['loss_bbox2d'] >= 0
+ assert losses['loss_consistency'] >= 0
+ assert losses['loss_dir'] >= 0
+
+ # test get_boxes
+ results = self.get_bboxes(*ret_dict, img_metas)
+ assert len(results) == 2
+ assert len(results[0]) == 5
+ assert results[0][0].tensor.shape == torch.Size([20, 7])
+ assert results[0][1].shape == torch.Size([20])
+ assert results[0][2].shape == torch.Size([20])
+ assert results[0][3] is None
+ assert results[0][4].shape == torch.Size([20, 5])
+
+
+def test_monoflex_head():
+
+ head_cfg = dict(
+ type='MonoFlexHead',
+ num_classes=3,
+ in_channels=64,
+ use_edge_fusion=True,
+ edge_fusion_inds=[(1, 0)],
+ edge_heatmap_ratio=1 / 8,
+ stacked_convs=0,
+ feat_channels=64,
+ use_direction_classifier=False,
+ diff_rad_by_sin=False,
+ pred_attrs=False,
+ pred_velo=False,
+ dir_offset=0,
+ strides=None,
+ group_reg_dims=((4, ), (2, ), (20, ), (3, ), (3, ), (8, 8), (1, ),
+ (1, )),
+ cls_branch=(256, ),
+ reg_branch=((256, ), (256, ), (256, ), (256, ), (256, ), (256, ),
+ (256, ), (256, )),
+ num_attrs=0,
+ bbox_code_size=7,
+ dir_branch=(),
+ attr_branch=(),
+ bbox_coder=dict(
+ type='MonoFlexCoder',
+ depth_mode='exp',
+ base_depth=(26.494627, 16.05988),
+ depth_range=[0.1, 100],
+ combine_depth=True,
+ uncertainty_range=[-10, 10],
+ base_dims=((3.8840, 1.5261, 1.6286, 0.4259, 0.1367, 0.1022),
+ (0.8423, 1.7607, 0.6602, 0.2349, 0.1133, 0.1427),
+ (1.7635, 1.7372, 0.5968, 0.1766, 0.0948, 0.1242)),
+ dims_mode='linear',
+ multibin=True,
+ num_dir_bins=4,
+ bin_centers=[0, np.pi / 2, np.pi, -np.pi / 2],
+ bin_margin=np.pi / 6,
+ code_size=7),
+ conv_bias=True,
+ dcn_on_last_conv=False)
+
+ self = build_head(head_cfg)
+
+ feats = [torch.rand([2, 64, 32, 32], dtype=torch.float32)]
+
+ input_metas = [
+ dict(img_shape=(110, 110), pad_shape=(128, 128)),
+ dict(img_shape=(98, 110), pad_shape=(128, 128))
+ ]
+ cls_score, out_reg = self(feats, input_metas)
+
+ assert cls_score[0].shape == torch.Size([2, 3, 32, 32])
+ assert out_reg[0].shape == torch.Size([2, 50, 32, 32])
+
+
+def test_fcaf3d_head():
+ if not torch.cuda.is_available():
+ pytest.skip('test requires GPU and torch+cuda')
+
+ try:
+ import MinkowskiEngine as ME
+ except ImportError:
+ pytest.skip('test requires MinkowskiEngine installation')
+
+ _setup_seed(0)
+
+ coordinates, features = [], []
+ # batch of 2 point clouds
+ for i in range(2):
+ c = torch.from_numpy(np.random.rand(500, 3) * 100)
+ coordinates.append(c.float().cuda())
+ f = torch.from_numpy(np.random.rand(500, 3))
+ features.append(f.float().cuda())
+ tensor_coordinates, tensor_features = ME.utils.sparse_collate(
+ coordinates, features)
+ x = ME.SparseTensor(
+ features=tensor_features, coordinates=tensor_coordinates)
+
+ # backbone
+ conv1 = ME.MinkowskiConvolution(
+ 3, 64, kernel_size=3, stride=2, dimension=3).cuda()
+ conv2 = ME.MinkowskiConvolution(
+ 64, 128, kernel_size=3, stride=2, dimension=3).cuda()
+ conv3 = ME.MinkowskiConvolution(
+ 128, 256, kernel_size=3, stride=2, dimension=3).cuda()
+ conv4 = ME.MinkowskiConvolution(
+ 256, 512, kernel_size=3, stride=2, dimension=3).cuda()
+
+ # backbone outputs of 4 levels
+ x1 = conv1(x)
+ x2 = conv2(x1)
+ x3 = conv3(x2)
+ x4 = conv4(x3)
+ x = (x1, x2, x3, x4)
+
+ # build head
+ cfg = dict(
+ type='FCAF3DHead',
+ in_channels=(64, 128, 256, 512),
+ out_channels=128,
+ voxel_size=1.,
+ pts_prune_threshold=1000,
+ pts_assign_threshold=27,
+ pts_center_threshold=18,
+ n_classes=18,
+ n_reg_outs=6)
+ test_cfg = mmcv.Config(dict(nms_pre=1000, iou_thr=.5, score_thr=.01))
+ cfg.update(test_cfg=test_cfg)
+ head = build_head(cfg).cuda()
+
+ # test forward train
+ gt_bboxes = [
+ DepthInstance3DBoxes(
+ torch.tensor([[10., 10., 10., 10., 10., 10.],
+ [30., 30., 30., 30., 30., 30.]]),
+ box_dim=6,
+ with_yaw=False),
+ DepthInstance3DBoxes(
+ torch.tensor([[20., 20., 20., 20., 20., 20.],
+ [40., 40., 40., 40., 40., 40.]]),
+ box_dim=6,
+ with_yaw=False)
+ ]
+ gt_labels = [torch.tensor([2, 4]).cuda(), torch.tensor([3, 5]).cuda()]
+ img_metas = [
+ dict(box_type_3d=DepthInstance3DBoxes),
+ dict(box_type_3d=DepthInstance3DBoxes)
+ ]
+
+ losses = head.forward_train(x, gt_bboxes, gt_labels, img_metas)
+ assert losses['center_loss'].shape == torch.Size([])
+ assert losses['bbox_loss'].shape == torch.Size([])
+ assert losses['cls_loss'].shape == torch.Size([])
+
+ # test forward test
+ bbox_list = head.forward_test(x, img_metas)
+ assert len(bbox_list) == 2
+ for bboxes, scores, labels in bbox_list:
+ n, dim = bboxes.tensor.shape
+ assert n > 0
+ assert dim == 7
+ assert scores.shape == torch.Size([n])
+ assert labels.shape == torch.Size([n])
diff --git a/tests/test_models/test_heads/test_paconv_decode_head.py b/tests/test_models/test_heads/test_paconv_decode_head.py
new file mode 100644
index 0000000..e5e57c8
--- /dev/null
+++ b/tests/test_models/test_heads/test_paconv_decode_head.py
@@ -0,0 +1,83 @@
+# Copyright (c) OpenMMLab. All rights reserved.
+import numpy as np
+import pytest
+import torch
+from mmcv.cnn.bricks import ConvModule
+
+from mmdet3d.models.builder import build_head
+
+
+def test_paconv_decode_head_loss():
+ if not torch.cuda.is_available():
+ pytest.skip('test requires GPU and torch+cuda')
+ paconv_decode_head_cfg = dict(
+ type='PAConvHead',
+ fp_channels=((768, 256, 256), (384, 256, 256), (320, 256, 128),
+ (128 + 6, 128, 128, 128)),
+ channels=128,
+ num_classes=20,
+ dropout_ratio=0.5,
+ conv_cfg=dict(type='Conv1d'),
+ norm_cfg=dict(type='BN1d'),
+ act_cfg=dict(type='ReLU'),
+ loss_decode=dict(
+ type='CrossEntropyLoss',
+ use_sigmoid=False,
+ class_weight=None,
+ loss_weight=1.0),
+ ignore_index=20)
+
+ self = build_head(paconv_decode_head_cfg)
+ self.cuda()
+ assert isinstance(self.conv_seg, torch.nn.Conv1d)
+ assert self.conv_seg.in_channels == 128
+ assert self.conv_seg.out_channels == 20
+ assert self.conv_seg.kernel_size == (1, )
+ assert isinstance(self.pre_seg_conv, ConvModule)
+ assert isinstance(self.pre_seg_conv.conv, torch.nn.Conv1d)
+ assert self.pre_seg_conv.conv.in_channels == 128
+ assert self.pre_seg_conv.conv.out_channels == 128
+ assert self.pre_seg_conv.conv.kernel_size == (1, )
+ assert isinstance(self.pre_seg_conv.bn, torch.nn.BatchNorm1d)
+ assert self.pre_seg_conv.bn.num_features == 128
+ assert isinstance(self.pre_seg_conv.activate, torch.nn.ReLU)
+
+ # test forward
+ sa_xyz = [
+ torch.rand(2, 4096, 3).float().cuda(),
+ torch.rand(2, 1024, 3).float().cuda(),
+ torch.rand(2, 256, 3).float().cuda(),
+ torch.rand(2, 64, 3).float().cuda(),
+ torch.rand(2, 16, 3).float().cuda(),
+ ]
+ sa_features = [
+ torch.rand(2, 6, 4096).float().cuda(),
+ torch.rand(2, 64, 1024).float().cuda(),
+ torch.rand(2, 128, 256).float().cuda(),
+ torch.rand(2, 256, 64).float().cuda(),
+ torch.rand(2, 512, 16).float().cuda(),
+ ]
+ input_dict = dict(sa_xyz=sa_xyz, sa_features=sa_features)
+ seg_logits = self(input_dict)
+ assert seg_logits.shape == torch.Size([2, 20, 4096])
+
+ # test loss
+ pts_semantic_mask = torch.randint(0, 20, (2, 4096)).long().cuda()
+ losses = self.losses(seg_logits, pts_semantic_mask)
+ assert losses['loss_sem_seg'].item() > 0
+
+ # test loss with ignore_index
+ ignore_index_mask = torch.ones_like(pts_semantic_mask) * 20
+ losses = self.losses(seg_logits, ignore_index_mask)
+ assert losses['loss_sem_seg'].item() == 0
+
+ # test loss with class_weight
+ paconv_decode_head_cfg['loss_decode'] = dict(
+ type='CrossEntropyLoss',
+ use_sigmoid=False,
+ class_weight=np.random.rand(20),
+ loss_weight=1.0)
+ self = build_head(paconv_decode_head_cfg)
+ self.cuda()
+ losses = self.losses(seg_logits, pts_semantic_mask)
+ assert losses['loss_sem_seg'].item() > 0
diff --git a/tests/test_models/test_heads/test_parta2_bbox_head.py b/tests/test_models/test_heads/test_parta2_bbox_head.py
new file mode 100644
index 0000000..5b1a603
--- /dev/null
+++ b/tests/test_models/test_heads/test_parta2_bbox_head.py
@@ -0,0 +1,493 @@
+# Copyright (c) OpenMMLab. All rights reserved.
+import pytest
+import torch
+from mmcv import Config
+from mmcv.ops import SubMConv3d
+from torch.nn import BatchNorm1d, ReLU
+
+from mmdet3d.core.bbox import Box3DMode, LiDARInstance3DBoxes
+from mmdet3d.core.bbox.samplers import IoUNegPiecewiseSampler
+from mmdet3d.models import PartA2BboxHead
+from mmdet3d.ops import make_sparse_convmodule
+
+
+def test_loss():
+ self = PartA2BboxHead(
+ num_classes=3,
+ seg_in_channels=16,
+ part_in_channels=4,
+ seg_conv_channels=[64, 64],
+ part_conv_channels=[64, 64],
+ merge_conv_channels=[128, 128],
+ down_conv_channels=[128, 256],
+ shared_fc_channels=[256, 512, 512, 512],
+ cls_channels=[256, 256],
+ reg_channels=[256, 256])
+
+ cls_score = torch.Tensor([[-3.6810], [-3.9413], [-5.3971], [-17.1281],
+ [-5.9434], [-6.2251]])
+ bbox_pred = torch.Tensor(
+ [[
+ -6.3016e-03, -5.2294e-03, -1.2793e-02, -1.0602e-02, -7.4086e-04,
+ 9.2471e-03, 7.3514e-03
+ ],
+ [
+ -1.1975e-02, -1.1578e-02, -3.1219e-02, 2.7754e-02, 6.9775e-03,
+ 9.4042e-04, 9.0472e-04
+ ],
+ [
+ 3.7539e-03, -9.1897e-03, -5.3666e-03, -1.0380e-05, 4.3467e-03,
+ 4.2470e-03, 1.8355e-03
+ ],
+ [
+ -7.6093e-02, -1.2497e-01, -9.2942e-02, 2.1404e-02, 2.3750e-02,
+ 1.0365e-01, -1.3042e-02
+ ],
+ [
+ 2.7577e-03, -1.1514e-02, -1.1097e-02, -2.4946e-03, 2.3268e-03,
+ 1.6797e-03, -1.4076e-03
+ ],
+ [
+ 3.9635e-03, -7.8551e-03, -3.5125e-03, 2.1229e-04, 9.7042e-03,
+ 1.7499e-03, -5.1254e-03
+ ]])
+ rois = torch.Tensor([
+ [0.0000, 13.3711, -12.5483, -1.9306, 1.7027, 4.2836, 1.4283, -1.1499],
+ [0.0000, 19.2472, -7.2655, -10.6641, 3.3078, 83.1976, 29.3337, 2.4501],
+ [0.0000, 13.8012, -10.9791, -3.0617, 0.2504, 1.2518, 0.8807, 3.1034],
+ [0.0000, 16.2736, -9.0284, -2.0494, 8.2697, 31.2336, 9.1006, 1.9208],
+ [0.0000, 10.4462, -13.6879, -3.1869, 7.3366, 0.3518, 1.7199, -0.7225],
+ [0.0000, 11.3374, -13.6671, -3.2332, 4.9934, 0.3750, 1.6033, -0.9665]
+ ])
+ labels = torch.Tensor([0.7100, 0.0000, 0.0000, 0.0000, 0.0000, 0.0000])
+ bbox_targets = torch.Tensor(
+ [[0.0598, 0.0243, -0.0984, -0.0454, 0.0066, 0.1114, 0.1714]])
+ pos_gt_bboxes = torch.Tensor(
+ [[13.6686, -12.5586, -2.1553, 1.6271, 4.3119, 1.5966, 2.1631]])
+ reg_mask = torch.Tensor([1, 0, 0, 0, 0, 0])
+ label_weights = torch.Tensor(
+ [0.0078, 0.0078, 0.0078, 0.0078, 0.0078, 0.0078])
+ bbox_weights = torch.Tensor([1., 0., 0., 0., 0., 0.])
+
+ loss = self.loss(cls_score, bbox_pred, rois, labels, bbox_targets,
+ pos_gt_bboxes, reg_mask, label_weights, bbox_weights)
+
+ expected_loss_cls = torch.Tensor([
+ 2.0579e-02, 1.5005e-04, 3.5252e-05, 0.0000e+00, 2.0433e-05, 1.5422e-05
+ ])
+ expected_loss_bbox = torch.as_tensor(0.0622)
+ expected_loss_corner = torch.Tensor([0.1374])
+
+ assert torch.allclose(loss['loss_cls'], expected_loss_cls, 1e-3)
+ assert torch.allclose(loss['loss_bbox'], expected_loss_bbox, 1e-3)
+ assert torch.allclose(loss['loss_corner'], expected_loss_corner, 1e-3)
+
+
+def test_get_targets():
+ self = PartA2BboxHead(
+ num_classes=3,
+ seg_in_channels=16,
+ part_in_channels=4,
+ seg_conv_channels=[64, 64],
+ part_conv_channels=[64, 64],
+ merge_conv_channels=[128, 128],
+ down_conv_channels=[128, 256],
+ shared_fc_channels=[256, 512, 512, 512],
+ cls_channels=[256, 256],
+ reg_channels=[256, 256])
+
+ sampling_result = IoUNegPiecewiseSampler(
+ 1,
+ pos_fraction=0.55,
+ neg_piece_fractions=[0.8, 0.2],
+ neg_iou_piece_thrs=[0.55, 0.1],
+ return_iou=True)
+ sampling_result.pos_bboxes = torch.Tensor(
+ [[8.1517, 0.0384, -1.9496, 1.5271, 4.1131, 1.4879, 1.2076]])
+ sampling_result.pos_gt_bboxes = torch.Tensor(
+ [[7.8417, -0.1405, -1.9652, 1.6122, 3.2838, 1.5331, -2.0835]])
+ sampling_result.iou = torch.Tensor([
+ 6.7787e-01, 0.0000e+00, 0.0000e+00, 0.0000e+00, 0.0000e+00, 0.0000e+00,
+ 0.0000e+00, 1.2839e-01, 0.0000e+00, 0.0000e+00, 0.0000e+00, 0.0000e+00,
+ 0.0000e+00, 0.0000e+00, 0.0000e+00, 7.0261e-04, 0.0000e+00, 0.0000e+00,
+ 0.0000e+00, 0.0000e+00, 5.8915e-02, 0.0000e+00, 0.0000e+00, 0.0000e+00,
+ 0.0000e+00, 0.0000e+00, 0.0000e+00, 0.0000e+00, 0.0000e+00, 5.6628e-06,
+ 5.0271e-02, 0.0000e+00, 1.9608e-01, 0.0000e+00, 0.0000e+00, 2.3519e-01,
+ 1.6589e-02, 0.0000e+00, 1.0162e-01, 2.1634e-02, 0.0000e+00, 0.0000e+00,
+ 0.0000e+00, 0.0000e+00, 0.0000e+00, 0.0000e+00, 0.0000e+00, 5.6326e-02,
+ 1.3810e-01, 0.0000e+00, 0.0000e+00, 0.0000e+00, 0.0000e+00, 0.0000e+00,
+ 0.0000e+00, 0.0000e+00, 0.0000e+00, 0.0000e+00, 0.0000e+00, 0.0000e+00,
+ 4.5455e-02, 0.0000e+00, 1.0929e-03, 0.0000e+00, 8.8191e-02, 1.1012e-01,
+ 0.0000e+00, 0.0000e+00, 0.0000e+00, 1.6236e-01, 0.0000e+00, 1.1342e-01,
+ 1.0636e-01, 9.9803e-02, 5.7394e-02, 0.0000e+00, 1.6773e-01, 0.0000e+00,
+ 0.0000e+00, 0.0000e+00, 0.0000e+00, 0.0000e+00, 0.0000e+00, 6.3464e-03,
+ 0.0000e+00, 2.7977e-01, 0.0000e+00, 3.1252e-01, 2.1642e-01, 2.2945e-01,
+ 0.0000e+00, 1.8297e-01, 0.0000e+00, 2.1908e-01, 1.1661e-01, 1.3513e-01,
+ 1.5898e-01, 7.4368e-03, 1.2523e-01, 1.4735e-04, 0.0000e+00, 0.0000e+00,
+ 0.0000e+00, 1.0948e-01, 2.5889e-01, 4.4585e-04, 8.6483e-02, 1.6376e-01,
+ 0.0000e+00, 2.2894e-01, 2.7489e-01, 0.0000e+00, 0.0000e+00, 0.0000e+00,
+ 1.8334e-01, 1.0193e-01, 2.3389e-01, 1.1035e-01, 3.3700e-01, 1.4397e-01,
+ 1.0379e-01, 0.0000e+00, 1.1226e-01, 0.0000e+00, 0.0000e+00, 1.6201e-01,
+ 0.0000e+00, 1.3569e-01
+ ])
+
+ rcnn_train_cfg = Config({
+ 'assigner': [{
+ 'type': 'MaxIoUAssigner',
+ 'iou_calculator': {
+ 'type': 'BboxOverlaps3D',
+ 'coordinate': 'lidar'
+ },
+ 'pos_iou_thr': 0.55,
+ 'neg_iou_thr': 0.55,
+ 'min_pos_iou': 0.55,
+ 'ignore_iof_thr': -1
+ }, {
+ 'type': 'MaxIoUAssigner',
+ 'iou_calculator': {
+ 'type': 'BboxOverlaps3D',
+ 'coordinate': 'lidar'
+ },
+ 'pos_iou_thr': 0.55,
+ 'neg_iou_thr': 0.55,
+ 'min_pos_iou': 0.55,
+ 'ignore_iof_thr': -1
+ }, {
+ 'type': 'MaxIoUAssigner',
+ 'iou_calculator': {
+ 'type': 'BboxOverlaps3D',
+ 'coordinate': 'lidar'
+ },
+ 'pos_iou_thr': 0.55,
+ 'neg_iou_thr': 0.55,
+ 'min_pos_iou': 0.55,
+ 'ignore_iof_thr': -1
+ }],
+ 'sampler': {
+ 'type': 'IoUNegPiecewiseSampler',
+ 'num': 128,
+ 'pos_fraction': 0.55,
+ 'neg_piece_fractions': [0.8, 0.2],
+ 'neg_iou_piece_thrs': [0.55, 0.1],
+ 'neg_pos_ub': -1,
+ 'add_gt_as_proposals': False,
+ 'return_iou': True
+ },
+ 'cls_pos_thr':
+ 0.75,
+ 'cls_neg_thr':
+ 0.25
+ })
+
+ label, bbox_targets, pos_gt_bboxes, reg_mask, label_weights, bbox_weights\
+ = self.get_targets([sampling_result], rcnn_train_cfg)
+
+ expected_label = torch.Tensor([
+ 0.8557, 0.0000, 0.0000, 0.0000, 0.0000, 0.0000, 0.0000, 0.0000, 0.0000,
+ 0.0000, 0.0000, 0.0000, 0.0000, 0.0000, 0.0000, 0.0000, 0.0000, 0.0000,
+ 0.0000, 0.0000, 0.0000, 0.0000, 0.0000, 0.0000, 0.0000, 0.0000, 0.0000,
+ 0.0000, 0.0000, 0.0000, 0.0000, 0.0000, 0.0000, 0.0000, 0.0000, 0.0000,
+ 0.0000, 0.0000, 0.0000, 0.0000, 0.0000, 0.0000, 0.0000, 0.0000, 0.0000,
+ 0.0000, 0.0000, 0.0000, 0.0000, 0.0000, 0.0000, 0.0000, 0.0000, 0.0000,
+ 0.0000, 0.0000, 0.0000, 0.0000, 0.0000, 0.0000, 0.0000, 0.0000, 0.0000,
+ 0.0000, 0.0000, 0.0000, 0.0000, 0.0000, 0.0000, 0.0000, 0.0000, 0.0000,
+ 0.0000, 0.0000, 0.0000, 0.0000, 0.0000, 0.0000, 0.0000, 0.0000, 0.0000,
+ 0.0000, 0.0000, 0.0000, 0.0000, 0.0595, 0.0000, 0.1250, 0.0000, 0.0000,
+ 0.0000, 0.0000, 0.0000, 0.0000, 0.0000, 0.0000, 0.0000, 0.0000, 0.0000,
+ 0.0000, 0.0000, 0.0000, 0.0000, 0.0000, 0.0178, 0.0000, 0.0000, 0.0000,
+ 0.0000, 0.0000, 0.0498, 0.0000, 0.0000, 0.0000, 0.0000, 0.0000, 0.0000,
+ 0.0000, 0.1740, 0.0000, 0.0000, 0.0000, 0.0000, 0.0000, 0.0000, 0.0000,
+ 0.0000, 0.0000
+ ])
+
+ expected_bbox_targets = torch.Tensor(
+ [[-0.0632, 0.0516, 0.0047, 0.0542, -0.2252, 0.0299, -0.1495]])
+
+ expected_pos_gt_bboxes = torch.Tensor(
+ [[7.8417, -0.1405, -1.9652, 1.6122, 3.2838, 1.5331, -2.0835]])
+
+ expected_reg_mask = torch.LongTensor([
+ 1, 0, 0, 0, 0, 0, 0, 0, 0, 0, 0, 0, 0, 0, 0, 0, 0, 0, 0, 0, 0, 0, 0, 0,
+ 0, 0, 0, 0, 0, 0, 0, 0, 0, 0, 0, 0, 0, 0, 0, 0, 0, 0, 0, 0, 0, 0, 0, 0,
+ 0, 0, 0, 0, 0, 0, 0, 0, 0, 0, 0, 0, 0, 0, 0, 0, 0, 0, 0, 0, 0, 0, 0, 0,
+ 0, 0, 0, 0, 0, 0, 0, 0, 0, 0, 0, 0, 0, 0, 0, 0, 0, 0, 0, 0, 0, 0, 0, 0,
+ 0, 0, 0, 0, 0, 0, 0, 0, 0, 0, 0, 0, 0, 0, 0, 0, 0, 0, 0, 0, 0, 0, 0, 0,
+ 0, 0, 0, 0, 0, 0, 0, 0
+ ])
+
+ expected_label_weights = torch.Tensor([
+ 0.0078, 0.0078, 0.0078, 0.0078, 0.0078, 0.0078, 0.0078, 0.0078, 0.0078,
+ 0.0078, 0.0078, 0.0078, 0.0078, 0.0078, 0.0078, 0.0078, 0.0078, 0.0078,
+ 0.0078, 0.0078, 0.0078, 0.0078, 0.0078, 0.0078, 0.0078, 0.0078, 0.0078,
+ 0.0078, 0.0078, 0.0078, 0.0078, 0.0078, 0.0078, 0.0078, 0.0078, 0.0078,
+ 0.0078, 0.0078, 0.0078, 0.0078, 0.0078, 0.0078, 0.0078, 0.0078, 0.0078,
+ 0.0078, 0.0078, 0.0078, 0.0078, 0.0078, 0.0078, 0.0078, 0.0078, 0.0078,
+ 0.0078, 0.0078, 0.0078, 0.0078, 0.0078, 0.0078, 0.0078, 0.0078, 0.0078,
+ 0.0078, 0.0078, 0.0078, 0.0078, 0.0078, 0.0078, 0.0078, 0.0078, 0.0078,
+ 0.0078, 0.0078, 0.0078, 0.0078, 0.0078, 0.0078, 0.0078, 0.0078, 0.0078,
+ 0.0078, 0.0078, 0.0078, 0.0078, 0.0078, 0.0078, 0.0078, 0.0078, 0.0078,
+ 0.0078, 0.0078, 0.0078, 0.0078, 0.0078, 0.0078, 0.0078, 0.0078, 0.0078,
+ 0.0078, 0.0078, 0.0078, 0.0078, 0.0078, 0.0078, 0.0078, 0.0078, 0.0078,
+ 0.0078, 0.0078, 0.0078, 0.0078, 0.0078, 0.0078, 0.0078, 0.0078, 0.0078,
+ 0.0078, 0.0078, 0.0078, 0.0078, 0.0078, 0.0078, 0.0078, 0.0078, 0.0078,
+ 0.0078, 0.0078
+ ])
+
+ expected_bbox_weights = torch.Tensor([
+ 1., 0., 0., 0., 0., 0., 0., 0., 0., 0., 0., 0., 0., 0., 0., 0., 0., 0.,
+ 0., 0., 0., 0., 0., 0., 0., 0., 0., 0., 0., 0., 0., 0., 0., 0., 0., 0.,
+ 0., 0., 0., 0., 0., 0., 0., 0., 0., 0., 0., 0., 0., 0., 0., 0., 0., 0.,
+ 0., 0., 0., 0., 0., 0., 0., 0., 0., 0., 0., 0., 0., 0., 0., 0., 0., 0.,
+ 0., 0., 0., 0., 0., 0., 0., 0., 0., 0., 0., 0., 0., 0., 0., 0., 0., 0.,
+ 0., 0., 0., 0., 0., 0., 0., 0., 0., 0., 0., 0., 0., 0., 0., 0., 0., 0.,
+ 0., 0., 0., 0., 0., 0., 0., 0., 0., 0., 0., 0., 0., 0., 0., 0., 0., 0.,
+ 0., 0.
+ ])
+
+ assert torch.allclose(label, expected_label, 1e-2)
+ assert torch.allclose(bbox_targets, expected_bbox_targets, 1e-2)
+ assert torch.allclose(pos_gt_bboxes, expected_pos_gt_bboxes)
+ assert torch.all(reg_mask == expected_reg_mask)
+ assert torch.allclose(label_weights, expected_label_weights, 1e-2)
+ assert torch.allclose(bbox_weights, expected_bbox_weights)
+
+
+def test_get_bboxes():
+ if not torch.cuda.is_available():
+ pytest.skip()
+ self = PartA2BboxHead(
+ num_classes=3,
+ seg_in_channels=16,
+ part_in_channels=4,
+ seg_conv_channels=[64, 64],
+ part_conv_channels=[64, 64],
+ merge_conv_channels=[128, 128],
+ down_conv_channels=[128, 256],
+ shared_fc_channels=[256, 512, 512, 512],
+ cls_channels=[256, 256],
+ reg_channels=[256, 256])
+
+ rois = torch.Tensor([[
+ 0.0000e+00, 5.6284e+01, 2.5712e+01, -1.3196e+00, 1.5943e+00,
+ 3.7509e+00, 1.4969e+00, 1.2105e-03
+ ],
+ [
+ 0.0000e+00, 5.4685e+01, 2.9132e+01, -1.9178e+00,
+ 1.6337e+00, 4.1116e+00, 1.5472e+00, -1.7312e+00
+ ],
+ [
+ 0.0000e+00, 5.5927e+01, 2.5830e+01, -1.4099e+00,
+ 1.5958e+00, 3.8861e+00, 1.4911e+00, -2.9276e+00
+ ],
+ [
+ 0.0000e+00, 5.6306e+01, 2.6310e+01, -1.3729e+00,
+ 1.5893e+00, 3.7448e+00, 1.4924e+00, 1.6071e-01
+ ],
+ [
+ 0.0000e+00, 3.1633e+01, -5.8557e+00, -1.2541e+00,
+ 1.6517e+00, 4.1829e+00, 1.5593e+00, -1.6037e+00
+ ],
+ [
+ 0.0000e+00, 3.1789e+01, -5.5308e+00, -1.3012e+00,
+ 1.6412e+00, 4.1070e+00, 1.5487e+00, -1.6517e+00
+ ]]).cuda()
+
+ cls_score = torch.Tensor([[-2.2061], [-2.1121], [-1.4478], [-2.9614],
+ [-0.1761], [0.7357]]).cuda()
+
+ bbox_pred = torch.Tensor(
+ [[
+ -4.7917e-02, -1.6504e-02, -2.2340e-02, 5.1296e-03, -2.0984e-02,
+ 1.0598e-02, -1.1907e-01
+ ],
+ [
+ -1.6261e-02, -5.4005e-02, 6.2480e-03, 1.5496e-03, -1.3285e-02,
+ 8.1482e-03, -2.2707e-03
+ ],
+ [
+ -3.9423e-02, 2.0151e-02, -2.1138e-02, -1.1845e-03, -1.5343e-02,
+ 5.7208e-03, 8.5646e-03
+ ],
+ [
+ 6.3104e-02, -3.9307e-02, 2.3005e-02, -7.0528e-03, -9.2637e-05,
+ 2.2656e-02, 1.6358e-02
+ ],
+ [
+ -1.4864e-03, 5.6840e-02, 5.8247e-03, -3.5541e-03, -4.9658e-03,
+ 2.5036e-03, 3.0302e-02
+ ],
+ [
+ -4.3259e-02, -1.9963e-02, 3.5004e-02, 3.7546e-03, 1.0876e-02,
+ -3.9637e-04, 2.0445e-02
+ ]]).cuda()
+
+ class_labels = [torch.Tensor([2, 2, 2, 2, 2, 2]).cuda()]
+
+ class_pred = [
+ torch.Tensor([[1.0877e-05, 1.0318e-05, 2.6599e-01],
+ [1.3105e-05, 1.1904e-05, 2.4432e-01],
+ [1.4530e-05, 1.4619e-05, 2.4395e-01],
+ [1.3251e-05, 1.3038e-05, 2.3703e-01],
+ [2.9156e-05, 2.5521e-05, 2.2826e-01],
+ [3.1665e-05, 2.9054e-05, 2.2077e-01]]).cuda()
+ ]
+
+ cfg = Config(
+ dict(
+ use_rotate_nms=True,
+ use_raw_score=True,
+ nms_thr=0.01,
+ score_thr=0.1))
+ input_meta = dict(
+ box_type_3d=LiDARInstance3DBoxes, box_mode_3d=Box3DMode.LIDAR)
+ result_list = self.get_bboxes(rois, cls_score, bbox_pred, class_labels,
+ class_pred, [input_meta], cfg)
+ selected_bboxes, selected_scores, selected_label_preds = result_list[0]
+
+ expected_selected_bboxes = torch.Tensor(
+ [[56.0888, 25.6445, -1.3610, 1.6025, 3.6730, 1.5128, -0.1179],
+ [54.4606, 29.2412, -1.9145, 1.6362, 4.0573, 1.5599, -1.7335],
+ [31.8887, -5.8574, -1.2470, 1.6458, 4.1622, 1.5632, -1.5734]]).cuda()
+ expected_selected_scores = torch.Tensor([-2.2061, -2.1121, -0.1761]).cuda()
+ expected_selected_label_preds = torch.Tensor([2., 2., 2.]).cuda()
+ assert torch.allclose(selected_bboxes.tensor, expected_selected_bboxes,
+ 1e-3)
+ assert torch.allclose(selected_scores, expected_selected_scores, 1e-3)
+ assert torch.allclose(selected_label_preds, expected_selected_label_preds)
+
+
+def test_multi_class_nms():
+ if not torch.cuda.is_available():
+ pytest.skip()
+
+ self = PartA2BboxHead(
+ num_classes=3,
+ seg_in_channels=16,
+ part_in_channels=4,
+ seg_conv_channels=[64, 64],
+ part_conv_channels=[64, 64],
+ merge_conv_channels=[128, 128],
+ down_conv_channels=[128, 256],
+ shared_fc_channels=[256, 512, 512, 512],
+ cls_channels=[256, 256],
+ reg_channels=[256, 256])
+
+ box_probs = torch.Tensor([[1.0877e-05, 1.0318e-05, 2.6599e-01],
+ [1.3105e-05, 1.1904e-05, 2.4432e-01],
+ [1.4530e-05, 1.4619e-05, 2.4395e-01],
+ [1.3251e-05, 1.3038e-05, 2.3703e-01],
+ [2.9156e-05, 2.5521e-05, 2.2826e-01],
+ [3.1665e-05, 2.9054e-05, 2.2077e-01],
+ [5.5738e-06, 6.2453e-06, 2.1978e-01],
+ [9.0193e-06, 9.2154e-06, 2.1418e-01],
+ [1.4004e-05, 1.3209e-05, 2.1316e-01],
+ [7.9210e-06, 8.1767e-06, 2.1304e-01]]).cuda()
+
+ box_preds = torch.Tensor(
+ [[
+ 5.6217e+01, 2.5908e+01, -1.3611e+00, 1.6025e+00, 3.6730e+00,
+ 1.5129e+00, 1.1786e-01
+ ],
+ [
+ 5.4653e+01, 2.8885e+01, -1.9145e+00, 1.6362e+00, 4.0574e+00,
+ 1.5599e+00, 1.7335e+00
+ ],
+ [
+ 5.5809e+01, 2.5686e+01, -1.4457e+00, 1.5939e+00, 3.8270e+00,
+ 1.4997e+00, 2.9191e+00
+ ],
+ [
+ 5.6107e+01, 2.6082e+01, -1.3557e+00, 1.5782e+00, 3.7444e+00,
+ 1.5266e+00, -1.7707e-01
+ ],
+ [
+ 3.1618e+01, -5.6004e+00, -1.2470e+00, 1.6459e+00, 4.1622e+00,
+ 1.5632e+00, 1.5734e+00
+ ],
+ [
+ 3.1605e+01, -5.6342e+00, -1.2467e+00, 1.6474e+00, 4.1519e+00,
+ 1.5481e+00, 1.6313e+00
+ ],
+ [
+ 5.6211e+01, 2.7294e+01, -1.5350e+00, 1.5422e+00, 3.7733e+00,
+ 1.5140e+00, -9.5846e-02
+ ],
+ [
+ 5.5907e+01, 2.7155e+01, -1.4712e+00, 1.5416e+00, 3.7611e+00,
+ 1.5142e+00, 5.2059e-02
+ ],
+ [
+ 5.4000e+01, 3.0585e+01, -1.6874e+00, 1.6495e+00, 4.0376e+00,
+ 1.5554e+00, 1.7900e+00
+ ],
+ [
+ 5.6007e+01, 2.6300e+01, -1.3945e+00, 1.5716e+00, 3.7064e+00,
+ 1.4715e+00, 2.9639e+00
+ ]]).cuda()
+
+ input_meta = dict(
+ box_type_3d=LiDARInstance3DBoxes, box_mode_3d=Box3DMode.LIDAR)
+ selected = self.multi_class_nms(box_probs, box_preds, 0.1, 0.001,
+ input_meta)
+ expected_selected = torch.Tensor([0, 1, 4, 8]).cuda()
+
+ assert torch.all(selected == expected_selected)
+
+
+def test_make_sparse_convmodule():
+ with pytest.raises(AssertionError):
+ # assert invalid order setting
+ make_sparse_convmodule(
+ in_channels=4,
+ out_channels=8,
+ kernel_size=3,
+ indice_key='rcnn_part2',
+ norm_cfg=dict(type='BN1d'),
+ order=('norm', 'act', 'conv', 'norm'))
+
+ # assert invalid type of order
+ make_sparse_convmodule(
+ in_channels=4,
+ out_channels=8,
+ kernel_size=3,
+ indice_key='rcnn_part2',
+ norm_cfg=dict(type='BN1d'),
+ order=['norm', 'conv'])
+
+ # assert invalid elements of order
+ make_sparse_convmodule(
+ in_channels=4,
+ out_channels=8,
+ kernel_size=3,
+ indice_key='rcnn_part2',
+ norm_cfg=dict(type='BN1d'),
+ order=('conv', 'normal', 'activate'))
+
+ sparse_convmodule = make_sparse_convmodule(
+ in_channels=4,
+ out_channels=64,
+ kernel_size=3,
+ padding=1,
+ indice_key='rcnn_part0',
+ norm_cfg=dict(type='BN1d', eps=0.001, momentum=0.01))
+
+ assert isinstance(sparse_convmodule[0], SubMConv3d)
+ assert isinstance(sparse_convmodule[1], BatchNorm1d)
+ assert isinstance(sparse_convmodule[2], ReLU)
+ assert sparse_convmodule[1].num_features == 64
+ assert sparse_convmodule[1].eps == 0.001
+ assert sparse_convmodule[1].affine is True
+ assert sparse_convmodule[1].track_running_stats is True
+ assert isinstance(sparse_convmodule[2], ReLU)
+ assert sparse_convmodule[2].inplace is True
+
+ pre_act = make_sparse_convmodule(
+ in_channels=4,
+ out_channels=8,
+ kernel_size=3,
+ indice_key='rcnn_part1',
+ norm_cfg=dict(type='BN1d'),
+ order=('norm', 'act', 'conv'))
+ assert isinstance(pre_act[0], BatchNorm1d)
+ assert isinstance(pre_act[1], ReLU)
+ assert isinstance(pre_act[2], SubMConv3d)
diff --git a/tests/test_models/test_heads/test_pointnet2_decode_head.py b/tests/test_models/test_heads/test_pointnet2_decode_head.py
new file mode 100644
index 0000000..5e6e40f
--- /dev/null
+++ b/tests/test_models/test_heads/test_pointnet2_decode_head.py
@@ -0,0 +1,83 @@
+# Copyright (c) OpenMMLab. All rights reserved.
+import numpy as np
+import pytest
+import torch
+from mmcv.cnn.bricks import ConvModule
+
+from mmdet3d.models.builder import build_head
+
+
+def test_pn2_decode_head_loss():
+ if not torch.cuda.is_available():
+ pytest.skip('test requires GPU and torch+cuda')
+ pn2_decode_head_cfg = dict(
+ type='PointNet2Head',
+ fp_channels=((768, 256, 256), (384, 256, 256), (320, 256, 128),
+ (128, 128, 128, 128)),
+ channels=128,
+ num_classes=20,
+ dropout_ratio=0.5,
+ conv_cfg=dict(type='Conv1d'),
+ norm_cfg=dict(type='BN1d'),
+ act_cfg=dict(type='ReLU'),
+ loss_decode=dict(
+ type='CrossEntropyLoss',
+ use_sigmoid=False,
+ class_weight=None,
+ loss_weight=1.0),
+ ignore_index=20)
+
+ self = build_head(pn2_decode_head_cfg)
+ self.cuda()
+ assert isinstance(self.conv_seg, torch.nn.Conv1d)
+ assert self.conv_seg.in_channels == 128
+ assert self.conv_seg.out_channels == 20
+ assert self.conv_seg.kernel_size == (1, )
+ assert isinstance(self.pre_seg_conv, ConvModule)
+ assert isinstance(self.pre_seg_conv.conv, torch.nn.Conv1d)
+ assert self.pre_seg_conv.conv.in_channels == 128
+ assert self.pre_seg_conv.conv.out_channels == 128
+ assert self.pre_seg_conv.conv.kernel_size == (1, )
+ assert isinstance(self.pre_seg_conv.bn, torch.nn.BatchNorm1d)
+ assert self.pre_seg_conv.bn.num_features == 128
+ assert isinstance(self.pre_seg_conv.activate, torch.nn.ReLU)
+
+ # test forward
+ sa_xyz = [
+ torch.rand(2, 4096, 3).float().cuda(),
+ torch.rand(2, 1024, 3).float().cuda(),
+ torch.rand(2, 256, 3).float().cuda(),
+ torch.rand(2, 64, 3).float().cuda(),
+ torch.rand(2, 16, 3).float().cuda(),
+ ]
+ sa_features = [
+ torch.rand(2, 6, 4096).float().cuda(),
+ torch.rand(2, 64, 1024).float().cuda(),
+ torch.rand(2, 128, 256).float().cuda(),
+ torch.rand(2, 256, 64).float().cuda(),
+ torch.rand(2, 512, 16).float().cuda(),
+ ]
+ input_dict = dict(sa_xyz=sa_xyz, sa_features=sa_features)
+ seg_logits = self(input_dict)
+ assert seg_logits.shape == torch.Size([2, 20, 4096])
+
+ # test loss
+ pts_semantic_mask = torch.randint(0, 20, (2, 4096)).long().cuda()
+ losses = self.losses(seg_logits, pts_semantic_mask)
+ assert losses['loss_sem_seg'].item() > 0
+
+ # test loss with ignore_index
+ ignore_index_mask = torch.ones_like(pts_semantic_mask) * 20
+ losses = self.losses(seg_logits, ignore_index_mask)
+ assert losses['loss_sem_seg'].item() == 0
+
+ # test loss with class_weight
+ pn2_decode_head_cfg['loss_decode'] = dict(
+ type='CrossEntropyLoss',
+ use_sigmoid=False,
+ class_weight=np.random.rand(20),
+ loss_weight=1.0)
+ self = build_head(pn2_decode_head_cfg)
+ self.cuda()
+ losses = self.losses(seg_logits, pts_semantic_mask)
+ assert losses['loss_sem_seg'].item() > 0
diff --git a/tests/test_models/test_heads/test_roi_extractors.py b/tests/test_models/test_heads/test_roi_extractors.py
new file mode 100644
index 0000000..842b768
--- /dev/null
+++ b/tests/test_models/test_heads/test_roi_extractors.py
@@ -0,0 +1,56 @@
+# Copyright (c) OpenMMLab. All rights reserved.
+import numpy as np
+import pytest
+import torch
+
+from mmdet3d.models.roi_heads.roi_extractors import (Single3DRoIAwareExtractor,
+ Single3DRoIPointExtractor)
+
+
+def test_single_roiaware_extractor():
+ if not torch.cuda.is_available():
+ pytest.skip('test requires GPU and torch+cuda')
+
+ roi_layer_cfg = dict(
+ type='RoIAwarePool3d', out_size=4, max_pts_per_voxel=128, mode='max')
+
+ self = Single3DRoIAwareExtractor(roi_layer=roi_layer_cfg)
+ feats = torch.tensor(
+ [[1, 2, 3.3], [1.2, 2.5, 3.0], [0.8, 2.1, 3.5], [1.6, 2.6, 3.6],
+ [0.8, 1.2, 3.9], [-9.2, 21.0, 18.2], [3.8, 7.9, 6.3],
+ [4.7, 3.5, -12.2], [3.8, 7.6, -2], [-10.6, -12.9, -20], [-16, -18, 9],
+ [-21.3, -52, -5], [0, 0, 0], [6, 7, 8], [-2, -3, -4]],
+ dtype=torch.float32).cuda()
+ coordinate = feats.clone()
+ batch_inds = torch.zeros(feats.shape[0]).cuda()
+ rois = torch.tensor([[0, 1.0, 2.0, 3.0, 5.0, 4.0, 6.0, -0.3 - np.pi / 2],
+ [0, -10.0, 23.0, 16.0, 20, 10, 20, -0.5 - np.pi / 2]],
+ dtype=torch.float32).cuda()
+ # test forward
+ pooled_feats = self(feats, coordinate, batch_inds, rois)
+ assert pooled_feats.shape == torch.Size([2, 4, 4, 4, 3])
+ assert torch.allclose(pooled_feats.sum(),
+ torch.tensor(51.100).cuda(), 1e-3)
+
+
+def test_single_roipoint_extractor():
+ if not torch.cuda.is_available():
+ pytest.skip('test requires GPU and torch+cuda')
+
+ roi_layer_cfg = dict(type='RoIPointPool3d', num_sampled_points=512)
+
+ self = Single3DRoIPointExtractor(roi_layer=roi_layer_cfg)
+
+ feats = torch.tensor(
+ [[1, 2, 3.3], [1.2, 2.5, 3.0], [0.8, 2.1, 3.5], [1.6, 2.6, 3.6],
+ [0.8, 1.2, 3.9], [-9.2, 21.0, 18.2], [3.8, 7.9, 6.3],
+ [4.7, 3.5, -12.2], [3.8, 7.6, -2], [-10.6, -12.9, -20], [-16, -18, 9],
+ [-21.3, -52, -5], [0, 0, 0], [6, 7, 8], [-2, -3, -4]],
+ dtype=torch.float32).unsqueeze(0).cuda()
+ points = feats.clone()
+ batch_inds = feats.shape[0]
+ rois = torch.tensor([[0, 1.0, 2.0, 3.0, 4.0, 5.0, 6.0, 0.3],
+ [0, -10.0, 23.0, 16.0, 10, 20, 20, 0.5]],
+ dtype=torch.float32).cuda()
+ pooled_feats = self(feats, points, batch_inds, rois)
+ assert pooled_feats.shape == torch.Size([2, 512, 6])
diff --git a/tests/test_models/test_heads/test_semantic_heads.py b/tests/test_models/test_heads/test_semantic_heads.py
new file mode 100644
index 0000000..e259ecb
--- /dev/null
+++ b/tests/test_models/test_heads/test_semantic_heads.py
@@ -0,0 +1,82 @@
+# Copyright (c) OpenMMLab. All rights reserved.
+import pytest
+import torch
+
+from mmdet3d.core.bbox import LiDARInstance3DBoxes
+
+
+def test_PointwiseSemanticHead():
+ # PointwiseSemanticHead only support gpu version currently.
+ if not torch.cuda.is_available():
+ pytest.skip('test requires GPU and torch+cuda')
+ from mmdet3d.models.builder import build_head
+
+ head_cfg = dict(
+ type='PointwiseSemanticHead',
+ in_channels=8,
+ extra_width=0.2,
+ seg_score_thr=0.3,
+ num_classes=3,
+ loss_seg=dict(
+ type='FocalLoss',
+ use_sigmoid=True,
+ reduction='sum',
+ gamma=2.0,
+ alpha=0.25,
+ loss_weight=1.0),
+ loss_part=dict(
+ type='CrossEntropyLoss', use_sigmoid=True, loss_weight=1.0))
+
+ self = build_head(head_cfg)
+ self.cuda()
+
+ # test forward
+ voxel_features = torch.rand([4, 8], dtype=torch.float32).cuda()
+ feats_dict = self.forward(voxel_features)
+ assert feats_dict['seg_preds'].shape == torch.Size(
+ [voxel_features.shape[0], 1])
+ assert feats_dict['part_preds'].shape == torch.Size(
+ [voxel_features.shape[0], 3])
+ assert feats_dict['part_feats'].shape == torch.Size(
+ [voxel_features.shape[0], 4])
+
+ voxel_centers = torch.tensor(
+ [[6.56126, 0.9648336, -1.7339306], [6.8162713, -2.480431, -1.3616394],
+ [11.643568, -4.744306, -1.3580885], [23.482342, 6.5036807, 0.5806964]
+ ],
+ dtype=torch.float32).cuda() # n, point_features
+ coordinates = torch.tensor(
+ [[0, 12, 819, 131], [0, 16, 750, 136], [1, 16, 705, 232],
+ [1, 35, 930, 469]],
+ dtype=torch.int32).cuda() # n, 4(batch, ind_x, ind_y, ind_z)
+ voxel_dict = dict(voxel_centers=voxel_centers, coors=coordinates)
+ gt_bboxes = [
+ LiDARInstance3DBoxes(
+ torch.tensor(
+ [[6.4118, -3.4305, -1.7291, 1.7033, 3.4693, 1.6197, 0.9091]],
+ dtype=torch.float32).cuda()),
+ LiDARInstance3DBoxes(
+ torch.tensor(
+ [[16.9107, 9.7925, -1.9201, 1.6097, 3.2786, 1.5307, 2.4056]],
+ dtype=torch.float32).cuda())
+ ]
+ # batch size is 2 in the unit test
+ gt_labels = list(torch.tensor([[0], [1]], dtype=torch.int64).cuda())
+
+ # test get_targets
+ target_dict = self.get_targets(voxel_dict, gt_bboxes, gt_labels)
+
+ assert target_dict['seg_targets'].shape == torch.Size(
+ [voxel_features.shape[0]])
+ assert torch.allclose(target_dict['seg_targets'],
+ target_dict['seg_targets'].new_tensor([3, -1, 3, 3]))
+ assert target_dict['part_targets'].shape == torch.Size(
+ [voxel_features.shape[0], 3])
+ assert target_dict['part_targets'].sum() == 0
+
+ # test loss
+ loss_dict = self.loss(feats_dict, target_dict)
+ assert loss_dict['loss_seg'] > 0
+ assert loss_dict['loss_part'] == 0 # no points in gt_boxes
+ total_loss = loss_dict['loss_seg'] + loss_dict['loss_part']
+ total_loss.backward()
diff --git a/tests/test_models/test_necks/test_fpn.py b/tests/test_models/test_necks/test_fpn.py
new file mode 100644
index 0000000..5dadbd2
--- /dev/null
+++ b/tests/test_models/test_necks/test_fpn.py
@@ -0,0 +1,38 @@
+# Copyright (c) OpenMMLab. All rights reserved.
+import pytest
+
+
+def test_secfpn():
+ neck_cfg = dict(
+ type='SECONDFPN',
+ in_channels=[2, 3],
+ upsample_strides=[1, 2],
+ out_channels=[4, 6],
+ )
+ from mmdet3d.models.builder import build_neck
+ neck = build_neck(neck_cfg)
+ assert neck.deblocks[0][0].in_channels == 2
+ assert neck.deblocks[1][0].in_channels == 3
+ assert neck.deblocks[0][0].out_channels == 4
+ assert neck.deblocks[1][0].out_channels == 6
+ assert neck.deblocks[0][0].stride == (1, 1)
+ assert neck.deblocks[1][0].stride == (2, 2)
+ assert neck is not None
+
+ neck_cfg = dict(
+ type='SECONDFPN',
+ in_channels=[2, 2],
+ upsample_strides=[1, 2, 4],
+ out_channels=[2, 2],
+ )
+ with pytest.raises(AssertionError):
+ build_neck(neck_cfg)
+
+ neck_cfg = dict(
+ type='SECONDFPN',
+ in_channels=[2, 2, 4],
+ upsample_strides=[1, 2, 4],
+ out_channels=[2, 2],
+ )
+ with pytest.raises(AssertionError):
+ build_neck(neck_cfg)
diff --git a/tests/test_models/test_necks/test_necks.py b/tests/test_models/test_necks/test_necks.py
new file mode 100644
index 0000000..3095519
--- /dev/null
+++ b/tests/test_models/test_necks/test_necks.py
@@ -0,0 +1,134 @@
+# Copyright (c) OpenMMLab. All rights reserved.
+import pytest
+import torch
+
+from mmdet3d.models.builder import build_backbone, build_neck
+
+
+def test_centerpoint_fpn():
+
+ second_cfg = dict(
+ type='SECOND',
+ in_channels=64,
+ out_channels=[64, 128, 256],
+ layer_nums=[3, 5, 5],
+ layer_strides=[2, 2, 2],
+ norm_cfg=dict(type='BN', eps=1e-3, momentum=0.01),
+ conv_cfg=dict(type='Conv2d', bias=False))
+
+ second = build_backbone(second_cfg)
+
+ # centerpoint usage of fpn
+ centerpoint_fpn_cfg = dict(
+ type='SECONDFPN',
+ in_channels=[64, 128, 256],
+ out_channels=[128, 128, 128],
+ upsample_strides=[0.5, 1, 2],
+ norm_cfg=dict(type='BN', eps=1e-3, momentum=0.01),
+ upsample_cfg=dict(type='deconv', bias=False),
+ use_conv_for_no_stride=True)
+
+ # original usage of fpn
+ fpn_cfg = dict(
+ type='SECONDFPN',
+ in_channels=[64, 128, 256],
+ upsample_strides=[1, 2, 4],
+ out_channels=[128, 128, 128])
+
+ second_fpn = build_neck(fpn_cfg)
+
+ centerpoint_second_fpn = build_neck(centerpoint_fpn_cfg)
+
+ input = torch.rand([4, 64, 512, 512])
+ sec_output = second(input)
+ centerpoint_output = centerpoint_second_fpn(sec_output)
+ second_output = second_fpn(sec_output)
+ assert centerpoint_output[0].shape == torch.Size([4, 384, 128, 128])
+ assert second_output[0].shape == torch.Size([4, 384, 256, 256])
+
+
+def test_imvoxel_neck():
+ if not torch.cuda.is_available():
+ pytest.skip('test requires GPU and torch+cuda')
+
+ neck_cfg = dict(
+ type='OutdoorImVoxelNeck', in_channels=64, out_channels=256)
+ neck = build_neck(neck_cfg).cuda()
+ inputs = torch.rand([1, 64, 216, 248, 12], device='cuda')
+ outputs = neck(inputs)
+ assert outputs[0].shape == (1, 256, 248, 216)
+
+
+def test_fp_neck():
+ if not torch.cuda.is_available():
+ pytest.skip()
+
+ xyzs = [16384, 4096, 1024, 256, 64]
+ feat_channels = [1, 96, 256, 512, 1024]
+ channel_num = 5
+
+ sa_xyz = [torch.rand(3, xyzs[i], 3) for i in range(channel_num)]
+ sa_features = [
+ torch.rand(3, feat_channels[i], xyzs[i]) for i in range(channel_num)
+ ]
+
+ neck_cfg = dict(
+ type='PointNetFPNeck',
+ fp_channels=((1536, 512, 512), (768, 512, 512), (608, 256, 256),
+ (257, 128, 128)))
+
+ neck = build_neck(neck_cfg)
+ neck.init_weights()
+
+ if torch.cuda.is_available():
+ sa_xyz = [x.cuda() for x in sa_xyz]
+ sa_features = [x.cuda() for x in sa_features]
+ neck.cuda()
+
+ feats_sa = {'sa_xyz': sa_xyz, 'sa_features': sa_features}
+ outputs = neck(feats_sa)
+ assert outputs['fp_xyz'].cpu().numpy().shape == (3, 16384, 3)
+ assert outputs['fp_features'].detach().cpu().numpy().shape == (3, 128,
+ 16384)
+
+
+def test_dla_neck():
+
+ s = 32
+ in_channels = [16, 32, 64, 128, 256, 512]
+ feat_sizes = [s // 2**i for i in range(6)] # [32, 16, 8, 4, 2, 1]
+
+ if torch.cuda.is_available():
+ # Test DLA Neck with DCNv2 on GPU
+ neck_cfg = dict(
+ type='DLANeck',
+ in_channels=[16, 32, 64, 128, 256, 512],
+ start_level=2,
+ end_level=5,
+ norm_cfg=dict(type='GN', num_groups=32))
+ neck = build_neck(neck_cfg)
+ neck.init_weights()
+ neck.cuda()
+ feats = [
+ torch.rand(4, in_channels[i], feat_sizes[i], feat_sizes[i]).cuda()
+ for i in range(len(in_channels))
+ ]
+ outputs = neck(feats)
+ assert outputs[0].shape == (4, 64, 8, 8)
+ else:
+ # Test DLA Neck without DCNv2 on CPU
+ neck_cfg = dict(
+ type='DLANeck',
+ in_channels=[16, 32, 64, 128, 256, 512],
+ start_level=2,
+ end_level=5,
+ norm_cfg=dict(type='GN', num_groups=32),
+ use_dcn=False)
+ neck = build_neck(neck_cfg)
+ neck.init_weights()
+ feats = [
+ torch.rand(4, in_channels[i], feat_sizes[i], feat_sizes[i])
+ for i in range(len(in_channels))
+ ]
+ outputs = neck(feats)
+ assert outputs[0].shape == (4, 64, 8, 8)
diff --git a/tests/test_models/test_segmentors.py b/tests/test_models/test_segmentors.py
new file mode 100644
index 0000000..73904e6
--- /dev/null
+++ b/tests/test_models/test_segmentors.py
@@ -0,0 +1,352 @@
+# Copyright (c) OpenMMLab. All rights reserved.
+import copy
+from os.path import dirname, exists, join
+
+import numpy as np
+import pytest
+import torch
+
+from mmdet3d.models.builder import build_segmentor
+from mmdet.apis import set_random_seed
+
+
+def _get_config_directory():
+ """Find the predefined detector config directory."""
+ try:
+ # Assume we are running in the source mmdetection3d repo
+ repo_dpath = dirname(dirname(dirname(__file__)))
+ except NameError:
+ # For IPython development when this __file__ is not defined
+ import mmdet3d
+ repo_dpath = dirname(dirname(mmdet3d.__file__))
+ config_dpath = join(repo_dpath, 'configs')
+ if not exists(config_dpath):
+ raise Exception('Cannot find config path')
+ return config_dpath
+
+
+def _get_config_module(fname):
+ """Load a configuration as a python module."""
+ from mmcv import Config
+ config_dpath = _get_config_directory()
+ config_fpath = join(config_dpath, fname)
+ config_mod = Config.fromfile(config_fpath)
+ return config_mod
+
+
+def _get_segmentor_cfg(fname):
+ """Grab configs necessary to create a segmentor.
+
+ These are deep copied to allow for safe modification of parameters without
+ influencing other tests.
+ """
+ import mmcv
+ config = _get_config_module(fname)
+ model = copy.deepcopy(config.model)
+ train_cfg = mmcv.Config(copy.deepcopy(config.model.train_cfg))
+ test_cfg = mmcv.Config(copy.deepcopy(config.model.test_cfg))
+
+ model.update(train_cfg=train_cfg)
+ model.update(test_cfg=test_cfg)
+ return model
+
+
+def test_pointnet2_ssg():
+ if not torch.cuda.is_available():
+ pytest.skip('test requires GPU and torch+cuda')
+
+ set_random_seed(0, True)
+ pn2_ssg_cfg = _get_segmentor_cfg(
+ 'pointnet2/pointnet2_ssg_16x2_cosine_200e_scannet_seg-3d-20class.py')
+ pn2_ssg_cfg.test_cfg.num_points = 32
+ self = build_segmentor(pn2_ssg_cfg).cuda()
+ points = [torch.rand(1024, 6).float().cuda() for _ in range(2)]
+ img_metas = [dict(), dict()]
+ gt_masks = [torch.randint(0, 20, (1024, )).long().cuda() for _ in range(2)]
+
+ # test forward_train
+ losses = self.forward_train(points, img_metas, gt_masks)
+ assert losses['decode.loss_sem_seg'].item() >= 0
+
+ # test forward function
+ set_random_seed(0, True)
+ data_dict = dict(
+ points=points, img_metas=img_metas, pts_semantic_mask=gt_masks)
+ forward_losses = self.forward(return_loss=True, **data_dict)
+ assert np.allclose(losses['decode.loss_sem_seg'].item(),
+ forward_losses['decode.loss_sem_seg'].item())
+
+ # test loss with ignore_index
+ ignore_masks = [torch.ones_like(gt_masks[0]) * 20 for _ in range(2)]
+ losses = self.forward_train(points, img_metas, ignore_masks)
+ assert losses['decode.loss_sem_seg'].item() == 0
+
+ # test simple_test
+ self.eval()
+ with torch.no_grad():
+ scene_points = [
+ torch.randn(500, 6).float().cuda() * 3.0,
+ torch.randn(200, 6).float().cuda() * 2.5
+ ]
+ results = self.simple_test(scene_points, img_metas)
+ assert results[0]['semantic_mask'].shape == torch.Size([500])
+ assert results[1]['semantic_mask'].shape == torch.Size([200])
+
+ # test forward function calling simple_test
+ with torch.no_grad():
+ data_dict = dict(points=[scene_points], img_metas=[img_metas])
+ results = self.forward(return_loss=False, **data_dict)
+ assert results[0]['semantic_mask'].shape == torch.Size([500])
+ assert results[1]['semantic_mask'].shape == torch.Size([200])
+
+ # test aug_test
+ with torch.no_grad():
+ scene_points = [
+ torch.randn(2, 500, 6).float().cuda() * 3.0,
+ torch.randn(2, 200, 6).float().cuda() * 2.5
+ ]
+ img_metas = [[dict(), dict()], [dict(), dict()]]
+ results = self.aug_test(scene_points, img_metas)
+ assert results[0]['semantic_mask'].shape == torch.Size([500])
+ assert results[1]['semantic_mask'].shape == torch.Size([200])
+
+ # test forward function calling aug_test
+ with torch.no_grad():
+ data_dict = dict(points=scene_points, img_metas=img_metas)
+ results = self.forward(return_loss=False, **data_dict)
+ assert results[0]['semantic_mask'].shape == torch.Size([500])
+ assert results[1]['semantic_mask'].shape == torch.Size([200])
+
+
+def test_pointnet2_msg():
+ if not torch.cuda.is_available():
+ pytest.skip('test requires GPU and torch+cuda')
+
+ set_random_seed(0, True)
+ pn2_msg_cfg = _get_segmentor_cfg(
+ 'pointnet2/pointnet2_msg_16x2_cosine_250e_scannet_seg-3d-20class.py')
+ pn2_msg_cfg.test_cfg.num_points = 32
+ self = build_segmentor(pn2_msg_cfg).cuda()
+ points = [torch.rand(1024, 6).float().cuda() for _ in range(2)]
+ img_metas = [dict(), dict()]
+ gt_masks = [torch.randint(0, 20, (1024, )).long().cuda() for _ in range(2)]
+
+ # test forward_train
+ losses = self.forward_train(points, img_metas, gt_masks)
+ assert losses['decode.loss_sem_seg'].item() >= 0
+
+ # test loss with ignore_index
+ ignore_masks = [torch.ones_like(gt_masks[0]) * 20 for _ in range(2)]
+ losses = self.forward_train(points, img_metas, ignore_masks)
+ assert losses['decode.loss_sem_seg'].item() == 0
+
+ # test simple_test
+ self.eval()
+ with torch.no_grad():
+ scene_points = [
+ torch.randn(500, 6).float().cuda() * 3.0,
+ torch.randn(200, 6).float().cuda() * 2.5
+ ]
+ results = self.simple_test(scene_points, img_metas)
+ assert results[0]['semantic_mask'].shape == torch.Size([500])
+ assert results[1]['semantic_mask'].shape == torch.Size([200])
+
+ # test aug_test
+ with torch.no_grad():
+ scene_points = [
+ torch.randn(2, 500, 6).float().cuda() * 3.0,
+ torch.randn(2, 200, 6).float().cuda() * 2.5
+ ]
+ img_metas = [[dict(), dict()], [dict(), dict()]]
+ results = self.aug_test(scene_points, img_metas)
+ assert results[0]['semantic_mask'].shape == torch.Size([500])
+ assert results[1]['semantic_mask'].shape == torch.Size([200])
+
+
+def test_paconv_ssg():
+ if not torch.cuda.is_available():
+ pytest.skip('test requires GPU and torch+cuda')
+
+ set_random_seed(0, True)
+ paconv_ssg_cfg = _get_segmentor_cfg(
+ 'paconv/paconv_ssg_8x8_cosine_150e_s3dis_seg-3d-13class.py')
+ # for GPU memory consideration
+ paconv_ssg_cfg.backbone.num_points = (256, 64, 16, 4)
+ paconv_ssg_cfg.test_cfg.num_points = 32
+ self = build_segmentor(paconv_ssg_cfg).cuda()
+ points = [torch.rand(1024, 9).float().cuda() for _ in range(2)]
+ img_metas = [dict(), dict()]
+ gt_masks = [torch.randint(0, 13, (1024, )).long().cuda() for _ in range(2)]
+
+ # test forward_train
+ losses = self.forward_train(points, img_metas, gt_masks)
+ assert losses['decode.loss_sem_seg'].item() >= 0
+ assert losses['regularize.loss_regularize'].item() >= 0
+
+ # test forward function
+ set_random_seed(0, True)
+ data_dict = dict(
+ points=points, img_metas=img_metas, pts_semantic_mask=gt_masks)
+ forward_losses = self.forward(return_loss=True, **data_dict)
+ assert np.allclose(losses['decode.loss_sem_seg'].item(),
+ forward_losses['decode.loss_sem_seg'].item())
+ assert np.allclose(losses['regularize.loss_regularize'].item(),
+ forward_losses['regularize.loss_regularize'].item())
+
+ # test loss with ignore_index
+ ignore_masks = [torch.ones_like(gt_masks[0]) * 13 for _ in range(2)]
+ losses = self.forward_train(points, img_metas, ignore_masks)
+ assert losses['decode.loss_sem_seg'].item() == 0
+
+ # test simple_test
+ self.eval()
+ with torch.no_grad():
+ scene_points = [
+ torch.randn(200, 6).float().cuda() * 3.0,
+ torch.randn(100, 6).float().cuda() * 2.5
+ ]
+ results = self.simple_test(scene_points, img_metas)
+ assert results[0]['semantic_mask'].shape == torch.Size([200])
+ assert results[1]['semantic_mask'].shape == torch.Size([100])
+
+ # test forward function calling simple_test
+ with torch.no_grad():
+ data_dict = dict(points=[scene_points], img_metas=[img_metas])
+ results = self.forward(return_loss=False, **data_dict)
+ assert results[0]['semantic_mask'].shape == torch.Size([200])
+ assert results[1]['semantic_mask'].shape == torch.Size([100])
+
+ # test aug_test
+ with torch.no_grad():
+ scene_points = [
+ torch.randn(2, 200, 6).float().cuda() * 3.0,
+ torch.randn(2, 100, 6).float().cuda() * 2.5
+ ]
+ img_metas = [[dict(), dict()], [dict(), dict()]]
+ results = self.aug_test(scene_points, img_metas)
+ assert results[0]['semantic_mask'].shape == torch.Size([200])
+ assert results[1]['semantic_mask'].shape == torch.Size([100])
+
+ # test forward function calling aug_test
+ with torch.no_grad():
+ data_dict = dict(points=scene_points, img_metas=img_metas)
+ results = self.forward(return_loss=False, **data_dict)
+ assert results[0]['semantic_mask'].shape == torch.Size([200])
+ assert results[1]['semantic_mask'].shape == torch.Size([100])
+
+
+def test_paconv_cuda_ssg():
+ if not torch.cuda.is_available():
+ pytest.skip('test requires GPU and torch+cuda')
+
+ set_random_seed(0, True)
+ paconv_cuda_ssg_cfg = _get_segmentor_cfg(
+ 'paconv/paconv_cuda_ssg_8x8_cosine_200e_s3dis_seg-3d-13class.py')
+ # for GPU memory consideration
+ paconv_cuda_ssg_cfg.backbone.num_points = (256, 64, 16, 4)
+ paconv_cuda_ssg_cfg.test_cfg.num_points = 32
+ self = build_segmentor(paconv_cuda_ssg_cfg).cuda()
+ points = [torch.rand(1024, 9).float().cuda() for _ in range(2)]
+ img_metas = [dict(), dict()]
+ gt_masks = [torch.randint(0, 13, (1024, )).long().cuda() for _ in range(2)]
+
+ # test forward_train
+ losses = self.forward_train(points, img_metas, gt_masks)
+ assert losses['decode.loss_sem_seg'].item() >= 0
+ assert losses['regularize.loss_regularize'].item() >= 0
+
+ # test forward function
+ set_random_seed(0, True)
+ data_dict = dict(
+ points=points, img_metas=img_metas, pts_semantic_mask=gt_masks)
+ forward_losses = self.forward(return_loss=True, **data_dict)
+ assert np.allclose(losses['decode.loss_sem_seg'].item(),
+ forward_losses['decode.loss_sem_seg'].item())
+ assert np.allclose(losses['regularize.loss_regularize'].item(),
+ forward_losses['regularize.loss_regularize'].item())
+
+ # test loss with ignore_index
+ ignore_masks = [torch.ones_like(gt_masks[0]) * 13 for _ in range(2)]
+ losses = self.forward_train(points, img_metas, ignore_masks)
+ assert losses['decode.loss_sem_seg'].item() == 0
+
+ # test simple_test
+ self.eval()
+ with torch.no_grad():
+ scene_points = [
+ torch.randn(200, 6).float().cuda() * 3.0,
+ torch.randn(100, 6).float().cuda() * 2.5
+ ]
+ results = self.simple_test(scene_points, img_metas)
+ assert results[0]['semantic_mask'].shape == torch.Size([200])
+ assert results[1]['semantic_mask'].shape == torch.Size([100])
+
+ # test forward function calling simple_test
+ with torch.no_grad():
+ data_dict = dict(points=[scene_points], img_metas=[img_metas])
+ results = self.forward(return_loss=False, **data_dict)
+ assert results[0]['semantic_mask'].shape == torch.Size([200])
+ assert results[1]['semantic_mask'].shape == torch.Size([100])
+
+ # test aug_test
+ with torch.no_grad():
+ scene_points = [
+ torch.randn(2, 200, 6).float().cuda() * 3.0,
+ torch.randn(2, 100, 6).float().cuda() * 2.5
+ ]
+ img_metas = [[dict(), dict()], [dict(), dict()]]
+ results = self.aug_test(scene_points, img_metas)
+ assert results[0]['semantic_mask'].shape == torch.Size([200])
+ assert results[1]['semantic_mask'].shape == torch.Size([100])
+
+ # test forward function calling aug_test
+ with torch.no_grad():
+ data_dict = dict(points=scene_points, img_metas=img_metas)
+ results = self.forward(return_loss=False, **data_dict)
+ assert results[0]['semantic_mask'].shape == torch.Size([200])
+ assert results[1]['semantic_mask'].shape == torch.Size([100])
+
+
+def test_dgcnn():
+ if not torch.cuda.is_available():
+ pytest.skip('test requires GPU and torch+cuda')
+
+ set_random_seed(0, True)
+ dgcnn_cfg = _get_segmentor_cfg(
+ 'dgcnn/dgcnn_32x4_cosine_100e_s3dis_seg-3d-13class.py')
+ dgcnn_cfg.test_cfg.num_points = 32
+ self = build_segmentor(dgcnn_cfg).cuda()
+ points = [torch.rand(4096, 9).float().cuda() for _ in range(2)]
+ img_metas = [dict(), dict()]
+ gt_masks = [torch.randint(0, 13, (4096, )).long().cuda() for _ in range(2)]
+
+ # test forward_train
+ losses = self.forward_train(points, img_metas, gt_masks)
+ assert losses['decode.loss_sem_seg'].item() >= 0
+
+ # test loss with ignore_index
+ ignore_masks = [torch.ones_like(gt_masks[0]) * 13 for _ in range(2)]
+ losses = self.forward_train(points, img_metas, ignore_masks)
+ assert losses['decode.loss_sem_seg'].item() == 0
+
+ # test simple_test
+ self.eval()
+ with torch.no_grad():
+ scene_points = [
+ torch.randn(500, 6).float().cuda() * 3.0,
+ torch.randn(200, 6).float().cuda() * 2.5
+ ]
+ results = self.simple_test(scene_points, img_metas)
+ assert results[0]['semantic_mask'].shape == torch.Size([500])
+ assert results[1]['semantic_mask'].shape == torch.Size([200])
+
+ # test aug_test
+ with torch.no_grad():
+ scene_points = [
+ torch.randn(2, 500, 6).float().cuda() * 3.0,
+ torch.randn(2, 200, 6).float().cuda() * 2.5
+ ]
+ img_metas = [[dict(), dict()], [dict(), dict()]]
+ results = self.aug_test(scene_points, img_metas)
+ assert results[0]['semantic_mask'].shape == torch.Size([500])
+ assert results[1]['semantic_mask'].shape == torch.Size([200])
diff --git a/tests/test_models/test_voxel_encoder/test_voxel_encoders.py b/tests/test_models/test_voxel_encoder/test_voxel_encoders.py
new file mode 100644
index 0000000..9bee9c9
--- /dev/null
+++ b/tests/test_models/test_voxel_encoder/test_voxel_encoders.py
@@ -0,0 +1,34 @@
+# Copyright (c) OpenMMLab. All rights reserved.
+import torch
+
+from mmdet3d.models.builder import build_voxel_encoder
+
+
+def test_pillar_feature_net():
+ pillar_feature_net_cfg = dict(
+ type='PillarFeatureNet',
+ in_channels=5,
+ feat_channels=[64],
+ with_distance=False,
+ voxel_size=(0.2, 0.2, 8),
+ point_cloud_range=(-51.2, -51.2, -5.0, 51.2, 51.2, 3.0),
+ norm_cfg=dict(type='BN1d', eps=1e-3, momentum=0.01))
+
+ pillar_feature_net = build_voxel_encoder(pillar_feature_net_cfg)
+
+ features = torch.rand([97297, 20, 5])
+ num_voxels = torch.randint(1, 100, [97297])
+ coors = torch.randint(0, 100, [97297, 4])
+
+ features = pillar_feature_net(features, num_voxels, coors)
+ assert features.shape == torch.Size([97297, 64])
+
+
+def test_hard_simple_VFE():
+ hard_simple_VFE_cfg = dict(type='HardSimpleVFE', num_features=5)
+ hard_simple_VFE = build_voxel_encoder(hard_simple_VFE_cfg)
+ features = torch.rand([240000, 10, 5])
+ num_voxels = torch.randint(1, 10, [240000])
+
+ outputs = hard_simple_VFE(features, num_voxels, None)
+ assert outputs.shape == torch.Size([240000, 5])
diff --git a/tests/test_models/test_voxel_encoder/test_voxel_generator.py b/tests/test_models/test_voxel_encoder/test_voxel_generator.py
new file mode 100644
index 0000000..123abb5
--- /dev/null
+++ b/tests/test_models/test_voxel_encoder/test_voxel_generator.py
@@ -0,0 +1,22 @@
+# Copyright (c) OpenMMLab. All rights reserved.
+import numpy as np
+
+from mmdet3d.core.voxel.voxel_generator import VoxelGenerator
+
+
+def test_voxel_generator():
+ np.random.seed(0)
+ voxel_size = [0.5, 0.5, 0.5]
+ point_cloud_range = [0, -40, -3, 70.4, 40, 1]
+ max_num_points = 1000
+ self = VoxelGenerator(voxel_size, point_cloud_range, max_num_points)
+ points = np.random.rand(1000, 4)
+ voxels = self.generate(points)
+ voxels, coors, num_points_per_voxel = voxels
+ expected_coors = np.array([[7, 81, 1], [6, 81, 0], [7, 80, 1], [6, 81, 1],
+ [7, 81, 0], [6, 80, 1], [7, 80, 0], [6, 80, 0]])
+ expected_num_points_per_voxel = np.array(
+ [120, 121, 127, 134, 115, 127, 125, 131])
+ assert voxels.shape == (8, 1000, 4)
+ assert np.all(coors == expected_coors)
+ assert np.all(num_points_per_voxel == expected_num_points_per_voxel)
diff --git a/tests/test_runtime/test_apis.py b/tests/test_runtime/test_apis.py
new file mode 100644
index 0000000..1f2255b
--- /dev/null
+++ b/tests/test_runtime/test_apis.py
@@ -0,0 +1,362 @@
+# Copyright (c) OpenMMLab. All rights reserved.
+import os
+import tempfile
+from os.path import dirname, exists, join
+
+import numpy as np
+import pytest
+import torch
+from mmcv.parallel import MMDataParallel
+
+from mmdet3d.apis import (convert_SyncBN, inference_detector,
+ inference_mono_3d_detector,
+ inference_multi_modality_detector,
+ inference_segmentor, init_model, show_result_meshlab,
+ single_gpu_test)
+from mmdet3d.core import Box3DMode
+from mmdet3d.core.bbox import (CameraInstance3DBoxes, DepthInstance3DBoxes,
+ LiDARInstance3DBoxes)
+from mmdet3d.datasets import build_dataloader, build_dataset
+from mmdet3d.models import build_model
+
+
+def _get_config_directory():
+ """Find the predefined detector config directory."""
+ try:
+ # Assume we are running in the source mmdetection3d repo
+ repo_dpath = dirname(dirname(dirname(__file__)))
+ except NameError:
+ # For IPython development when this __file__ is not defined
+ import mmdet3d
+ repo_dpath = dirname(dirname(mmdet3d.__file__))
+ config_dpath = join(repo_dpath, 'configs')
+ if not exists(config_dpath):
+ raise Exception('Cannot find config path')
+ return config_dpath
+
+
+def _get_config_module(fname):
+ """Load a configuration as a python module."""
+ from mmcv import Config
+ config_dpath = _get_config_directory()
+ config_fpath = join(config_dpath, fname)
+ config_mod = Config.fromfile(config_fpath)
+ return config_mod
+
+
+def test_convert_SyncBN():
+ cfg = _get_config_module(
+ 'pointpillars/hv_pointpillars_fpn_sbn-all_4x8_2x_nus-3d.py')
+ model_cfg = cfg.model
+ convert_SyncBN(model_cfg)
+ assert model_cfg['pts_voxel_encoder']['norm_cfg']['type'] == 'BN1d'
+ assert model_cfg['pts_backbone']['norm_cfg']['type'] == 'BN2d'
+ assert model_cfg['pts_neck']['norm_cfg']['type'] == 'BN2d'
+
+
+def test_show_result_meshlab():
+ pcd = 'tests/data/nuscenes/samples/LIDAR_TOP/n015-2018-08-02-17-16-37+' \
+ '0800__LIDAR_TOP__1533201470948018.pcd.bin'
+ box_3d = LiDARInstance3DBoxes(
+ torch.tensor(
+ [[8.7314, -1.8559, -1.5997, 0.4800, 1.2000, 1.8900, 0.0100]]))
+ labels_3d = torch.tensor([0])
+ scores_3d = torch.tensor([0.5])
+ points = np.random.rand(100, 4)
+ img_meta = dict(
+ pts_filename=pcd, boxes_3d=box_3d, box_mode_3d=Box3DMode.LIDAR)
+ data = dict(points=[[torch.tensor(points)]], img_metas=[[img_meta]])
+ result = [
+ dict(
+ pts_bbox=dict(
+ boxes_3d=box_3d, labels_3d=labels_3d, scores_3d=scores_3d))
+ ]
+ tmp_dir = tempfile.TemporaryDirectory()
+ temp_out_dir = tmp_dir.name
+ out_dir, file_name = show_result_meshlab(data, result, temp_out_dir)
+ expected_outfile_pred = file_name + '_pred.obj'
+ expected_outfile_pts = file_name + '_points.obj'
+ expected_outfile_pred_path = os.path.join(out_dir, file_name,
+ expected_outfile_pred)
+ expected_outfile_pts_path = os.path.join(out_dir, file_name,
+ expected_outfile_pts)
+ assert os.path.exists(expected_outfile_pred_path)
+ assert os.path.exists(expected_outfile_pts_path)
+ tmp_dir.cleanup()
+
+ # test multi-modality show
+ # indoor scene
+ pcd = 'tests/data/sunrgbd/points/000001.bin'
+ filename = 'tests/data/sunrgbd/sunrgbd_trainval/image/000001.jpg'
+ box_3d = DepthInstance3DBoxes(
+ torch.tensor(
+ [[-1.1580, 3.3041, -0.9961, 0.3829, 0.4647, 0.5574, 1.1213]]))
+ img = np.random.randn(1, 3, 608, 832)
+ k_mat = np.array([[529.5000, 0.0000, 365.0000],
+ [0.0000, 529.5000, 265.0000], [0.0000, 0.0000, 1.0000]])
+ rt_mat = np.array([[0.9980, 0.0058, -0.0634], [0.0058, 0.9835, 0.1808],
+ [0.0634, -0.1808, 0.9815]])
+ rt_mat = np.array([[1, 0, 0], [0, 0, -1], [0, 1, 0]]) @ rt_mat.transpose(
+ 1, 0)
+ depth2img = k_mat @ rt_mat
+ img_meta = dict(
+ filename=filename,
+ depth2img=depth2img,
+ pcd_horizontal_flip=False,
+ pcd_vertical_flip=False,
+ box_mode_3d=Box3DMode.DEPTH,
+ box_type_3d=DepthInstance3DBoxes,
+ pcd_trans=np.array([0., 0., 0.]),
+ pcd_scale_factor=1.0,
+ pts_filename=pcd,
+ transformation_3d_flow=['R', 'S', 'T'])
+ data = dict(
+ points=[[torch.tensor(points)]], img_metas=[[img_meta]], img=[img])
+ result = [dict(boxes_3d=box_3d, labels_3d=labels_3d, scores_3d=scores_3d)]
+ tmp_dir = tempfile.TemporaryDirectory()
+ temp_out_dir = tmp_dir.name
+ out_dir, file_name = show_result_meshlab(
+ data, result, temp_out_dir, 0.3, task='multi_modality-det')
+ expected_outfile_pred = file_name + '_pred.obj'
+ expected_outfile_pts = file_name + '_points.obj'
+ expected_outfile_png = file_name + '_img.png'
+ expected_outfile_proj = file_name + '_pred.png'
+ expected_outfile_pred_path = os.path.join(out_dir, file_name,
+ expected_outfile_pred)
+ expected_outfile_pts_path = os.path.join(out_dir, file_name,
+ expected_outfile_pts)
+ expected_outfile_png_path = os.path.join(out_dir, file_name,
+ expected_outfile_png)
+ expected_outfile_proj_path = os.path.join(out_dir, file_name,
+ expected_outfile_proj)
+ assert os.path.exists(expected_outfile_pred_path)
+ assert os.path.exists(expected_outfile_pts_path)
+ assert os.path.exists(expected_outfile_png_path)
+ assert os.path.exists(expected_outfile_proj_path)
+ tmp_dir.cleanup()
+ # outdoor scene
+ pcd = 'tests/data/kitti/training/velodyne_reduced/000000.bin'
+ filename = 'tests/data/kitti/training/image_2/000000.png'
+ box_3d = LiDARInstance3DBoxes(
+ torch.tensor(
+ [[6.4495, -3.9097, -1.7409, 1.5063, 3.1819, 1.4716, 1.8782]]))
+ img = np.random.randn(1, 3, 384, 1280)
+ lidar2img = np.array(
+ [[6.09695435e+02, -7.21421631e+02, -1.25125790e+00, -1.23041824e+02],
+ [1.80384201e+02, 7.64479828e+00, -7.19651550e+02, -1.01016693e+02],
+ [9.99945343e-01, 1.24365499e-04, 1.04513029e-02, -2.69386917e-01],
+ [0.00000000e+00, 0.00000000e+00, 0.00000000e+00, 1.00000000e+00]])
+ img_meta = dict(
+ filename=filename,
+ pcd_horizontal_flip=False,
+ pcd_vertical_flip=False,
+ box_mode_3d=Box3DMode.LIDAR,
+ box_type_3d=LiDARInstance3DBoxes,
+ pcd_trans=np.array([0., 0., 0.]),
+ pcd_scale_factor=1.0,
+ pts_filename=pcd,
+ lidar2img=lidar2img)
+ data = dict(
+ points=[[torch.tensor(points)]], img_metas=[[img_meta]], img=[img])
+ result = [
+ dict(
+ pts_bbox=dict(
+ boxes_3d=box_3d, labels_3d=labels_3d, scores_3d=scores_3d))
+ ]
+ out_dir, file_name = show_result_meshlab(
+ data, result, temp_out_dir, 0.1, task='multi_modality-det')
+ tmp_dir = tempfile.TemporaryDirectory()
+ temp_out_dir = tmp_dir.name
+ expected_outfile_pred = file_name + '_pred.obj'
+ expected_outfile_pts = file_name + '_points.obj'
+ expected_outfile_png = file_name + '_img.png'
+ expected_outfile_proj = file_name + '_pred.png'
+ expected_outfile_pred_path = os.path.join(out_dir, file_name,
+ expected_outfile_pred)
+ expected_outfile_pts_path = os.path.join(out_dir, file_name,
+ expected_outfile_pts)
+ expected_outfile_png_path = os.path.join(out_dir, file_name,
+ expected_outfile_png)
+ expected_outfile_proj_path = os.path.join(out_dir, file_name,
+ expected_outfile_proj)
+ assert os.path.exists(expected_outfile_pred_path)
+ assert os.path.exists(expected_outfile_pts_path)
+ assert os.path.exists(expected_outfile_png_path)
+ assert os.path.exists(expected_outfile_proj_path)
+ tmp_dir.cleanup()
+ # test mono-3d show
+ filename = 'tests/data/nuscenes/samples/CAM_BACK_LEFT/n015-2018-' \
+ '07-18-11-07-57+0800__CAM_BACK_LEFT__1531883530447423.jpg'
+ box_3d = CameraInstance3DBoxes(
+ torch.tensor(
+ [[6.4495, -3.9097, -1.7409, 1.5063, 3.1819, 1.4716, 1.8782]]))
+ img = np.random.randn(1, 3, 384, 1280)
+ cam2img = np.array([[100.0, 0.0, 50.0], [0.0, 100.0, 50.0],
+ [0.0, 0.0, 1.0]])
+ img_meta = dict(
+ filename=filename,
+ pcd_horizontal_flip=False,
+ pcd_vertical_flip=False,
+ box_mode_3d=Box3DMode.CAM,
+ box_type_3d=CameraInstance3DBoxes,
+ pcd_trans=np.array([0., 0., 0.]),
+ pcd_scale_factor=1.0,
+ cam2img=cam2img)
+ data = dict(
+ points=[[torch.tensor(points)]], img_metas=[[img_meta]], img=[img])
+ result = [
+ dict(
+ img_bbox=dict(
+ boxes_3d=box_3d, labels_3d=labels_3d, scores_3d=scores_3d))
+ ]
+ out_dir, file_name = show_result_meshlab(
+ data, result, temp_out_dir, 0.1, task='mono-det')
+ tmp_dir = tempfile.TemporaryDirectory()
+ temp_out_dir = tmp_dir.name
+ expected_outfile_png = file_name + '_img.png'
+ expected_outfile_proj = file_name + '_pred.png'
+ expected_outfile_png_path = os.path.join(out_dir, file_name,
+ expected_outfile_png)
+ expected_outfile_proj_path = os.path.join(out_dir, file_name,
+ expected_outfile_proj)
+ assert os.path.exists(expected_outfile_png_path)
+ assert os.path.exists(expected_outfile_proj_path)
+ tmp_dir.cleanup()
+
+ # test seg show
+ pcd = 'tests/data/scannet/points/scene0000_00.bin'
+ points = np.random.rand(100, 6)
+ img_meta = dict(pts_filename=pcd)
+ data = dict(points=[[torch.tensor(points)]], img_metas=[[img_meta]])
+ pred_seg = torch.randint(0, 20, (100, ))
+ result = [dict(semantic_mask=pred_seg)]
+ tmp_dir = tempfile.TemporaryDirectory()
+ temp_out_dir = tmp_dir.name
+ out_dir, file_name = show_result_meshlab(
+ data, result, temp_out_dir, task='seg')
+ expected_outfile_pred = file_name + '_pred.obj'
+ expected_outfile_pts = file_name + '_points.obj'
+ expected_outfile_pred_path = os.path.join(out_dir, file_name,
+ expected_outfile_pred)
+ expected_outfile_pts_path = os.path.join(out_dir, file_name,
+ expected_outfile_pts)
+ assert os.path.exists(expected_outfile_pred_path)
+ assert os.path.exists(expected_outfile_pts_path)
+ tmp_dir.cleanup()
+
+
+def test_inference_detector():
+ if not torch.cuda.is_available():
+ pytest.skip('test requires GPU and torch+cuda')
+
+ pcd = 'tests/data/kitti/training/velodyne_reduced/000000.bin'
+ detector_cfg = 'configs/pointpillars/hv_pointpillars_secfpn_' \
+ '6x8_160e_kitti-3d-3class.py'
+ detector = init_model(detector_cfg, device='cuda:0')
+ results = inference_detector(detector, pcd)
+ bboxes_3d = results[0][0]['boxes_3d']
+ scores_3d = results[0][0]['scores_3d']
+ labels_3d = results[0][0]['labels_3d']
+ assert bboxes_3d.tensor.shape[0] >= 0
+ assert bboxes_3d.tensor.shape[1] == 7
+ assert scores_3d.shape[0] >= 0
+ assert labels_3d.shape[0] >= 0
+
+
+def test_inference_multi_modality_detector():
+ # these two multi-modality models both only have GPU implementations
+ if not torch.cuda.is_available():
+ pytest.skip('test requires GPU and torch+cuda')
+ # indoor scene
+ pcd = 'tests/data/sunrgbd/points/000001.bin'
+ img = 'tests/data/sunrgbd/sunrgbd_trainval/image/000001.jpg'
+ ann_file = 'tests/data/sunrgbd/sunrgbd_infos.pkl'
+ detector_cfg = 'configs/imvotenet/imvotenet_stage2_'\
+ '16x8_sunrgbd-3d-10class.py'
+ detector = init_model(detector_cfg, device='cuda:0')
+ results = inference_multi_modality_detector(detector, pcd, img, ann_file)
+ bboxes_3d = results[0][0]['boxes_3d']
+ scores_3d = results[0][0]['scores_3d']
+ labels_3d = results[0][0]['labels_3d']
+ assert bboxes_3d.tensor.shape[0] >= 0
+ assert bboxes_3d.tensor.shape[1] == 7
+ assert scores_3d.shape[0] >= 0
+ assert labels_3d.shape[0] >= 0
+
+ # outdoor scene
+ pcd = 'tests/data/kitti/training/velodyne_reduced/000000.bin'
+ img = 'tests/data/kitti/training/image_2/000000.png'
+ ann_file = 'tests/data/kitti/kitti_infos_train.pkl'
+ detector_cfg = 'configs/mvxnet/dv_mvx-fpn_second_secfpn_adamw_' \
+ '2x8_80e_kitti-3d-3class.py'
+ detector = init_model(detector_cfg, device='cuda:0')
+ results = inference_multi_modality_detector(detector, pcd, img, ann_file)
+ bboxes_3d = results[0][0]['pts_bbox']['boxes_3d']
+ scores_3d = results[0][0]['pts_bbox']['scores_3d']
+ labels_3d = results[0][0]['pts_bbox']['labels_3d']
+ assert bboxes_3d.tensor.shape[0] >= 0
+ assert bboxes_3d.tensor.shape[1] == 7
+ assert scores_3d.shape[0] >= 0
+ assert labels_3d.shape[0] >= 0
+
+
+def test_inference_mono_3d_detector():
+ # FCOS3D only has GPU implementations
+ if not torch.cuda.is_available():
+ pytest.skip('test requires GPU and torch+cuda')
+ img = 'tests/data/nuscenes/samples/CAM_BACK_LEFT/' \
+ 'n015-2018-07-18-11-07-57+0800__CAM_BACK_LEFT__1531883530447423.jpg'
+ ann_file = 'tests/data/nuscenes/nus_infos_mono3d.coco.json'
+ detector_cfg = 'configs/fcos3d/fcos3d_r101_caffe_fpn_gn-head_dcn_' \
+ '2x8_1x_nus-mono3d.py'
+ detector = init_model(detector_cfg, device='cuda:0')
+ results = inference_mono_3d_detector(detector, img, ann_file)
+ bboxes_3d = results[0][0]['img_bbox']['boxes_3d']
+ scores_3d = results[0][0]['img_bbox']['scores_3d']
+ labels_3d = results[0][0]['img_bbox']['labels_3d']
+ assert bboxes_3d.tensor.shape[0] >= 0
+ assert bboxes_3d.tensor.shape[1] == 9
+ assert scores_3d.shape[0] >= 0
+ assert labels_3d.shape[0] >= 0
+
+
+def test_inference_segmentor():
+ # PN2 only has GPU implementations
+ if not torch.cuda.is_available():
+ pytest.skip('test requires GPU and torch+cuda')
+ pcd = 'tests/data/scannet/points/scene0000_00.bin'
+ segmentor_cfg = 'configs/pointnet2/pointnet2_ssg_' \
+ '16x2_cosine_200e_scannet_seg-3d-20class.py'
+ segmentor = init_model(segmentor_cfg, device='cuda:0')
+ results = inference_segmentor(segmentor, pcd)
+ seg_3d = results[0][0]['semantic_mask']
+ assert seg_3d.shape == torch.Size([100])
+ assert seg_3d.min() >= 0
+ assert seg_3d.max() <= 19
+
+
+def test_single_gpu_test():
+ if not torch.cuda.is_available():
+ pytest.skip('test requires GPU and torch+cuda')
+ cfg = _get_config_module('votenet/votenet_16x8_sunrgbd-3d-10class.py')
+ cfg.model.train_cfg = None
+ model = build_model(cfg.model, test_cfg=cfg.get('test_cfg'))
+ dataset_cfg = cfg.data.test
+ dataset_cfg.data_root = './tests/data/sunrgbd'
+ dataset_cfg.ann_file = 'tests/data/sunrgbd/sunrgbd_infos.pkl'
+ dataset = build_dataset(dataset_cfg)
+ data_loader = build_dataloader(
+ dataset,
+ samples_per_gpu=1,
+ workers_per_gpu=cfg.data.workers_per_gpu,
+ dist=False,
+ shuffle=False)
+ model = MMDataParallel(model, device_ids=[0])
+ results = single_gpu_test(model, data_loader)
+ bboxes_3d = results[0]['boxes_3d']
+ scores_3d = results[0]['scores_3d']
+ labels_3d = results[0]['labels_3d']
+ assert bboxes_3d.tensor.shape[0] >= 0
+ assert bboxes_3d.tensor.shape[1] == 7
+ assert scores_3d.shape[0] >= 0
+ assert labels_3d.shape[0] >= 0
diff --git a/tests/test_runtime/test_config.py b/tests/test_runtime/test_config.py
new file mode 100644
index 0000000..6a9d8f5
--- /dev/null
+++ b/tests/test_runtime/test_config.py
@@ -0,0 +1,307 @@
+# Copyright (c) OpenMMLab. All rights reserved.
+from os.path import dirname, exists, join, relpath
+
+
+def _get_config_directory():
+ """Find the predefined detector config directory."""
+ try:
+ # Assume we are running in the source mmdetection3d repo
+ repo_dpath = dirname(dirname(dirname(__file__)))
+ except NameError:
+ # For IPython development when this __file__ is not defined
+ import mmdet3d
+ repo_dpath = dirname(dirname(mmdet3d.__file__))
+ config_dpath = join(repo_dpath, 'configs')
+ if not exists(config_dpath):
+ raise Exception('Cannot find config path')
+ return config_dpath
+
+
+def test_config_build_model():
+ """Test that all detection models defined in the configs can be
+ initialized."""
+ from mmcv import Config
+
+ from mmdet3d.models import build_model
+
+ config_dpath = _get_config_directory()
+ print('Found config_dpath = {!r}'.format(config_dpath))
+
+ import glob
+ config_fpaths = list(glob.glob(join(config_dpath, '**', '*.py')))
+ config_fpaths = [p for p in config_fpaths if p.find('_base_') == -1]
+ config_names = [relpath(p, config_dpath) for p in config_fpaths]
+
+ print('Using {} config files'.format(len(config_names)))
+
+ for config_fname in config_names:
+ config_fpath = join(config_dpath, config_fname)
+ config_mod = Config.fromfile(config_fpath)
+
+ config_mod.model
+ config_mod.model.train_cfg
+ config_mod.model.test_cfg
+ print('Building detector, config_fpath = {!r}'.format(config_fpath))
+
+ # Remove pretrained keys to allow for testing in an offline environment
+ if 'pretrained' in config_mod.model:
+ config_mod.model['pretrained'] = None
+
+ # We skip detectors based on MikowskiEngine as it is an external
+ # dependency and may be not installed by the user.
+ if config_fname.startswith('fcaf3d'):
+ continue
+
+ detector = build_model(config_mod.model)
+ assert detector is not None
+
+ if 'roi_head' in config_mod.model.keys():
+ # for two stage detector
+ # detectors must have bbox head
+ assert detector.roi_head.with_bbox and detector.with_bbox
+ assert detector.roi_head.with_mask == detector.with_mask
+
+ head_config = config_mod.model['roi_head']
+ if head_config.type == 'PartAggregationROIHead':
+ check_parta2_roi_head(head_config, detector.roi_head)
+ elif head_config.type == 'H3DRoIHead':
+ check_h3d_roi_head(head_config, detector.roi_head)
+ elif head_config.type == 'PointRCNNRoIHead':
+ check_pointrcnn_roi_head(head_config, detector.roi_head)
+ else:
+ _check_roi_head(head_config, detector.roi_head)
+ # else:
+ # # for single stage detector
+ # # detectors must have bbox head
+ # # assert detector.with_bbox
+ # head_config = config_mod.model['bbox_head']
+ # _check_bbox_head(head_config, detector.bbox_head)
+
+
+def test_config_build_pipeline():
+ """Test that all detection models defined in the configs can be
+ initialized."""
+ from mmcv import Config
+
+ from mmdet3d.datasets.pipelines import Compose
+
+ config_dpath = _get_config_directory()
+ print('Found config_dpath = {!r}'.format(config_dpath))
+
+ # Other configs needs database sampler.
+ config_names = [
+ 'pointpillars/hv_pointpillars_secfpn_sbn-all_4x8_2x_nus-3d.py',
+ ]
+
+ print('Using {} config files'.format(len(config_names)))
+
+ for config_fname in config_names:
+ config_fpath = join(config_dpath, config_fname)
+ config_mod = Config.fromfile(config_fpath)
+
+ # build train_pipeline
+ train_pipeline = Compose(config_mod.train_pipeline)
+ test_pipeline = Compose(config_mod.test_pipeline)
+ assert train_pipeline is not None
+ assert test_pipeline is not None
+
+
+def _check_roi_head(config, head):
+ # check consistency between head_config and roi_head
+ assert config['type'] == head.__class__.__name__
+
+ # check roi_align
+ bbox_roi_cfg = config.bbox_roi_extractor
+ bbox_roi_extractor = head.bbox_roi_extractor
+ _check_roi_extractor(bbox_roi_cfg, bbox_roi_extractor)
+
+ # check bbox head infos
+ bbox_cfg = config.bbox_head
+ bbox_head = head.bbox_head
+ _check_bbox_head(bbox_cfg, bbox_head)
+
+ if head.with_mask:
+ # check roi_align
+ if config.mask_roi_extractor:
+ mask_roi_cfg = config.mask_roi_extractor
+ mask_roi_extractor = head.mask_roi_extractor
+ _check_roi_extractor(mask_roi_cfg, mask_roi_extractor,
+ bbox_roi_extractor)
+
+ # check mask head infos
+ mask_head = head.mask_head
+ mask_cfg = config.mask_head
+ _check_mask_head(mask_cfg, mask_head)
+
+
+def _check_roi_extractor(config, roi_extractor, prev_roi_extractor=None):
+ from torch import nn as nn
+ if isinstance(roi_extractor, nn.ModuleList):
+ if prev_roi_extractor:
+ prev_roi_extractor = prev_roi_extractor[0]
+ roi_extractor = roi_extractor[0]
+
+ assert (len(config.featmap_strides) == len(roi_extractor.roi_layers))
+ assert (config.out_channels == roi_extractor.out_channels)
+ from torch.nn.modules.utils import _pair
+ assert (_pair(config.roi_layer.output_size) ==
+ roi_extractor.roi_layers[0].output_size)
+
+ if 'use_torchvision' in config.roi_layer:
+ assert (config.roi_layer.use_torchvision ==
+ roi_extractor.roi_layers[0].use_torchvision)
+ elif 'aligned' in config.roi_layer:
+ assert (
+ config.roi_layer.aligned == roi_extractor.roi_layers[0].aligned)
+
+ if prev_roi_extractor:
+ assert (roi_extractor.roi_layers[0].aligned ==
+ prev_roi_extractor.roi_layers[0].aligned)
+ assert (roi_extractor.roi_layers[0].use_torchvision ==
+ prev_roi_extractor.roi_layers[0].use_torchvision)
+
+
+def _check_mask_head(mask_cfg, mask_head):
+ from torch import nn as nn
+ if isinstance(mask_cfg, list):
+ for single_mask_cfg, single_mask_head in zip(mask_cfg, mask_head):
+ _check_mask_head(single_mask_cfg, single_mask_head)
+ elif isinstance(mask_head, nn.ModuleList):
+ for single_mask_head in mask_head:
+ _check_mask_head(mask_cfg, single_mask_head)
+ else:
+ assert mask_cfg['type'] == mask_head.__class__.__name__
+ assert mask_cfg.in_channels == mask_head.in_channels
+ assert (
+ mask_cfg.conv_out_channels == mask_head.conv_logits.in_channels)
+ class_agnostic = mask_cfg.get('class_agnostic', False)
+ out_dim = (1 if class_agnostic else mask_cfg.num_classes)
+ assert mask_head.conv_logits.out_channels == out_dim
+
+
+def _check_bbox_head(bbox_cfg, bbox_head):
+ from torch import nn as nn
+ if isinstance(bbox_cfg, list):
+ for single_bbox_cfg, single_bbox_head in zip(bbox_cfg, bbox_head):
+ _check_bbox_head(single_bbox_cfg, single_bbox_head)
+ elif isinstance(bbox_head, nn.ModuleList):
+ for single_bbox_head in bbox_head:
+ _check_bbox_head(bbox_cfg, single_bbox_head)
+ else:
+ assert bbox_cfg['type'] == bbox_head.__class__.__name__
+ assert bbox_cfg.in_channels == bbox_head.in_channels
+ with_cls = bbox_cfg.get('with_cls', True)
+ if with_cls:
+ fc_out_channels = bbox_cfg.get('fc_out_channels', 2048)
+ assert (fc_out_channels == bbox_head.fc_cls.in_features)
+ assert bbox_cfg.num_classes + 1 == bbox_head.fc_cls.out_features
+
+ with_reg = bbox_cfg.get('with_reg', True)
+ if with_reg:
+ out_dim = (4 if bbox_cfg.reg_class_agnostic else 4 *
+ bbox_cfg.num_classes)
+ assert bbox_head.fc_reg.out_features == out_dim
+
+
+def check_parta2_roi_head(config, head):
+ assert config['type'] == head.__class__.__name__
+
+ # check seg_roi_extractor
+ seg_roi_cfg = config.seg_roi_extractor
+ seg_roi_extractor = head.seg_roi_extractor
+ _check_parta2_roi_extractor(seg_roi_cfg, seg_roi_extractor)
+
+ # check part_roi_extractor
+ part_roi_cfg = config.part_roi_extractor
+ part_roi_extractor = head.part_roi_extractor
+ _check_parta2_roi_extractor(part_roi_cfg, part_roi_extractor)
+
+ # check bbox head infos
+ bbox_cfg = config.bbox_head
+ bbox_head = head.bbox_head
+ _check_parta2_bbox_head(bbox_cfg, bbox_head)
+
+
+def _check_parta2_roi_extractor(config, roi_extractor):
+ assert config['type'] == roi_extractor.__class__.__name__
+ assert (config.roi_layer.out_size == roi_extractor.roi_layer.out_size)
+ assert (config.roi_layer.max_pts_per_voxel ==
+ roi_extractor.roi_layer.max_pts_per_voxel)
+
+
+def _check_parta2_bbox_head(bbox_cfg, bbox_head):
+ from torch import nn as nn
+ if isinstance(bbox_cfg, list):
+ for single_bbox_cfg, single_bbox_head in zip(bbox_cfg, bbox_head):
+ _check_bbox_head(single_bbox_cfg, single_bbox_head)
+ elif isinstance(bbox_head, nn.ModuleList):
+ for single_bbox_head in bbox_head:
+ _check_bbox_head(bbox_cfg, single_bbox_head)
+ else:
+ assert bbox_cfg['type'] == bbox_head.__class__.__name__
+ assert bbox_cfg.seg_in_channels == bbox_head.seg_conv[0][0].in_channels
+ assert bbox_cfg.part_in_channels == bbox_head.part_conv[0][
+ 0].in_channels
+
+
+def check_h3d_roi_head(config, head):
+ assert config['type'] == head.__class__.__name__
+
+ # check seg_roi_extractor
+ primitive_z_cfg = config.primitive_list[0]
+ primitive_z_extractor = head.primitive_z
+ _check_primitive_extractor(primitive_z_cfg, primitive_z_extractor)
+
+ primitive_xy_cfg = config.primitive_list[1]
+ primitive_xy_extractor = head.primitive_xy
+ _check_primitive_extractor(primitive_xy_cfg, primitive_xy_extractor)
+
+ primitive_line_cfg = config.primitive_list[2]
+ primitive_line_extractor = head.primitive_line
+ _check_primitive_extractor(primitive_line_cfg, primitive_line_extractor)
+
+ # check bbox head infos
+ bbox_cfg = config.bbox_head
+ bbox_head = head.bbox_head
+ _check_h3d_bbox_head(bbox_cfg, bbox_head)
+
+
+def _check_primitive_extractor(config, primitive_extractor):
+ assert config['type'] == primitive_extractor.__class__.__name__
+ assert (config.num_dims == primitive_extractor.num_dims)
+ assert (config.num_classes == primitive_extractor.num_classes)
+
+
+def _check_h3d_bbox_head(bbox_cfg, bbox_head):
+ assert bbox_cfg['type'] == bbox_head.__class__.__name__
+ assert bbox_cfg.num_proposal * \
+ 6 == bbox_head.surface_center_matcher.num_point[0]
+ assert bbox_cfg.num_proposal * \
+ 12 == bbox_head.line_center_matcher.num_point[0]
+ assert bbox_cfg.suface_matching_cfg.mlp_channels[-1] * \
+ 18 == bbox_head.bbox_pred[0].in_channels
+
+
+def check_pointrcnn_roi_head(config, head):
+ assert config['type'] == head.__class__.__name__
+
+ # check point_roi_extractor
+ point_roi_cfg = config.point_roi_extractor
+ point_roi_extractor = head.point_roi_extractor
+ _check_pointrcnn_roi_extractor(point_roi_cfg, point_roi_extractor)
+ # check pointrcnn rcnn bboxhead
+ bbox_cfg = config.bbox_head
+ bbox_head = head.bbox_head
+ _check_pointrcnn_bbox_head(bbox_cfg, bbox_head)
+
+
+def _check_pointrcnn_roi_extractor(config, roi_extractor):
+ assert config['type'] == roi_extractor.__class__.__name__
+ assert config.roi_layer.num_sampled_points == \
+ roi_extractor.roi_layer.num_sampled_points
+
+
+def _check_pointrcnn_bbox_head(bbox_cfg, bbox_head):
+ assert bbox_cfg['type'] == bbox_head.__class__.__name__
+ assert bbox_cfg.num_classes == bbox_head.num_classes
+ assert bbox_cfg.with_corner_loss == bbox_head.with_corner_loss
diff --git a/tests/test_samples/parta2_roihead_inputs.npz b/tests/test_samples/parta2_roihead_inputs.npz
new file mode 100644
index 0000000..8dc8e54
Binary files /dev/null and b/tests/test_samples/parta2_roihead_inputs.npz differ
diff --git a/tests/test_utils/test_anchors.py b/tests/test_utils/test_anchors.py
new file mode 100644
index 0000000..5de25e2
--- /dev/null
+++ b/tests/test_utils/test_anchors.py
@@ -0,0 +1,239 @@
+# Copyright (c) OpenMMLab. All rights reserved.
+"""
+CommandLine:
+ pytest tests/test_utils/test_anchor.py
+ xdoctest tests/test_utils/test_anchor.py zero
+
+"""
+import torch
+
+from mmdet3d.core.anchor import build_prior_generator
+
+
+def test_anchor_3d_range_generator():
+ if torch.cuda.is_available():
+ device = 'cuda'
+ else:
+ device = 'cpu'
+ anchor_generator_cfg = dict(
+ type='Anchor3DRangeGenerator',
+ ranges=[
+ [0, -39.68, -0.6, 70.4, 39.68, -0.6],
+ [0, -39.68, -0.6, 70.4, 39.68, -0.6],
+ [0, -39.68, -1.78, 70.4, 39.68, -1.78],
+ ],
+ sizes=[[0.8, 0.6, 1.73], [1.76, 0.6, 1.73], [3.9, 1.6, 1.56]],
+ rotations=[0, 1.57],
+ reshape_out=False)
+
+ anchor_generator = build_prior_generator(anchor_generator_cfg)
+ repr_str = repr(anchor_generator)
+ expected_repr_str = 'Anchor3DRangeGenerator(anchor_range=' \
+ '[[0, -39.68, -0.6, 70.4, 39.68, -0.6], ' \
+ '[0, -39.68, -0.6, 70.4, 39.68, -0.6], ' \
+ '[0, -39.68, -1.78, 70.4, 39.68, -1.78]],' \
+ '\nscales=[1],\nsizes=[[0.8, 0.6, 1.73], ' \
+ '[1.76, 0.6, 1.73], [3.9, 1.6, 1.56]],' \
+ '\nrotations=[0, 1.57],\nreshape_out=False,' \
+ '\nsize_per_range=True)'
+ assert repr_str == expected_repr_str
+ featmap_size = (256, 256)
+ mr_anchors = anchor_generator.single_level_grid_anchors(
+ featmap_size, 1.1, device=device)
+ assert mr_anchors.shape == torch.Size([1, 256, 256, 3, 2, 7])
+
+
+def test_aligned_anchor_generator():
+ if torch.cuda.is_available():
+ device = 'cuda'
+ else:
+ device = 'cpu'
+
+ anchor_generator_cfg = dict(
+ type='AlignedAnchor3DRangeGenerator',
+ ranges=[[-51.2, -51.2, -1.80, 51.2, 51.2, -1.80]],
+ scales=[1, 2, 4],
+ sizes=[
+ [2.5981, 0.8660, 1.], # 1.5/sqrt(3)
+ [1.7321, 0.5774, 1.], # 1/sqrt(3)
+ [1., 1., 1.],
+ [0.4, 0.4, 1],
+ ],
+ custom_values=[0, 0],
+ rotations=[0, 1.57],
+ size_per_range=False,
+ reshape_out=True)
+
+ featmap_sizes = [(256, 256), (128, 128), (64, 64)]
+ anchor_generator = build_prior_generator(anchor_generator_cfg)
+ assert anchor_generator.num_base_anchors == 8
+
+ # check base anchors
+ expected_grid_anchors = [
+ torch.tensor([[
+ -51.0000, -51.0000, -1.8000, 2.5981, 0.8660, 1.0000, 0.0000,
+ 0.0000, 0.0000
+ ],
+ [
+ -51.0000, -51.0000, -1.8000, 0.4000, 0.4000, 1.0000,
+ 1.5700, 0.0000, 0.0000
+ ],
+ [
+ -50.6000, -51.0000, -1.8000, 0.4000, 0.4000, 1.0000,
+ 0.0000, 0.0000, 0.0000
+ ],
+ [
+ -50.2000, -51.0000, -1.8000, 1.0000, 1.0000, 1.0000,
+ 1.5700, 0.0000, 0.0000
+ ],
+ [
+ -49.8000, -51.0000, -1.8000, 1.0000, 1.0000, 1.0000,
+ 0.0000, 0.0000, 0.0000
+ ],
+ [
+ -49.4000, -51.0000, -1.8000, 1.7321, 0.5774, 1.0000,
+ 1.5700, 0.0000, 0.0000
+ ],
+ [
+ -49.0000, -51.0000, -1.8000, 1.7321, 0.5774, 1.0000,
+ 0.0000, 0.0000, 0.0000
+ ],
+ [
+ -48.6000, -51.0000, -1.8000, 2.5981, 0.8660, 1.0000,
+ 1.5700, 0.0000, 0.0000
+ ]],
+ device=device),
+ torch.tensor([[
+ -50.8000, -50.8000, -1.8000, 5.1962, 1.7320, 2.0000, 0.0000,
+ 0.0000, 0.0000
+ ],
+ [
+ -50.8000, -50.8000, -1.8000, 0.8000, 0.8000, 2.0000,
+ 1.5700, 0.0000, 0.0000
+ ],
+ [
+ -50.0000, -50.8000, -1.8000, 0.8000, 0.8000, 2.0000,
+ 0.0000, 0.0000, 0.0000
+ ],
+ [
+ -49.2000, -50.8000, -1.8000, 2.0000, 2.0000, 2.0000,
+ 1.5700, 0.0000, 0.0000
+ ],
+ [
+ -48.4000, -50.8000, -1.8000, 2.0000, 2.0000, 2.0000,
+ 0.0000, 0.0000, 0.0000
+ ],
+ [
+ -47.6000, -50.8000, -1.8000, 3.4642, 1.1548, 2.0000,
+ 1.5700, 0.0000, 0.0000
+ ],
+ [
+ -46.8000, -50.8000, -1.8000, 3.4642, 1.1548, 2.0000,
+ 0.0000, 0.0000, 0.0000
+ ],
+ [
+ -46.0000, -50.8000, -1.8000, 5.1962, 1.7320, 2.0000,
+ 1.5700, 0.0000, 0.0000
+ ]],
+ device=device),
+ torch.tensor([[
+ -50.4000, -50.4000, -1.8000, 10.3924, 3.4640, 4.0000, 0.0000,
+ 0.0000, 0.0000
+ ],
+ [
+ -50.4000, -50.4000, -1.8000, 1.6000, 1.6000, 4.0000,
+ 1.5700, 0.0000, 0.0000
+ ],
+ [
+ -48.8000, -50.4000, -1.8000, 1.6000, 1.6000, 4.0000,
+ 0.0000, 0.0000, 0.0000
+ ],
+ [
+ -47.2000, -50.4000, -1.8000, 4.0000, 4.0000, 4.0000,
+ 1.5700, 0.0000, 0.0000
+ ],
+ [
+ -45.6000, -50.4000, -1.8000, 4.0000, 4.0000, 4.0000,
+ 0.0000, 0.0000, 0.0000
+ ],
+ [
+ -44.0000, -50.4000, -1.8000, 6.9284, 2.3096, 4.0000,
+ 1.5700, 0.0000, 0.0000
+ ],
+ [
+ -42.4000, -50.4000, -1.8000, 6.9284, 2.3096, 4.0000,
+ 0.0000, 0.0000, 0.0000
+ ],
+ [
+ -40.8000, -50.4000, -1.8000, 10.3924, 3.4640, 4.0000,
+ 1.5700, 0.0000, 0.0000
+ ]],
+ device=device)
+ ]
+ multi_level_anchors = anchor_generator.grid_anchors(
+ featmap_sizes, device=device)
+ expected_multi_level_shapes = [
+ torch.Size([524288, 9]),
+ torch.Size([131072, 9]),
+ torch.Size([32768, 9])
+ ]
+ for i, single_level_anchor in enumerate(multi_level_anchors):
+ assert single_level_anchor.shape == expected_multi_level_shapes[i]
+ # set [:56:7] thus it could cover 8 (len(size) * len(rotations))
+ # anchors on 8 location
+ assert single_level_anchor[:56:7].allclose(expected_grid_anchors[i])
+
+
+def test_aligned_anchor_generator_per_cls():
+ if torch.cuda.is_available():
+ device = 'cuda'
+ else:
+ device = 'cpu'
+
+ anchor_generator_cfg = dict(
+ type='AlignedAnchor3DRangeGeneratorPerCls',
+ ranges=[[-100, -100, -1.80, 100, 100, -1.80],
+ [-100, -100, -1.30, 100, 100, -1.30]],
+ sizes=[[1.76, 0.63, 1.44], [2.35, 0.96, 1.59]],
+ custom_values=[0, 0],
+ rotations=[0, 1.57],
+ reshape_out=False)
+
+ featmap_sizes = [(100, 100), (50, 50)]
+ anchor_generator = build_prior_generator(anchor_generator_cfg)
+
+ # check base anchors
+ expected_grid_anchors = [[
+ torch.tensor([[
+ -99.0000, -99.0000, -1.8000, 1.7600, 0.6300, 1.4400, 0.0000,
+ 0.0000, 0.0000
+ ],
+ [
+ -99.0000, -99.0000, -1.8000, 1.7600, 0.6300, 1.4400,
+ 1.5700, 0.0000, 0.0000
+ ]],
+ device=device),
+ torch.tensor([[
+ -98.0000, -98.0000, -1.3000, 2.3500, 0.9600, 1.5900, 0.0000,
+ 0.0000, 0.0000
+ ],
+ [
+ -98.0000, -98.0000, -1.3000, 2.3500, 0.9600, 1.5900,
+ 1.5700, 0.0000, 0.0000
+ ]],
+ device=device)
+ ]]
+ multi_level_anchors = anchor_generator.grid_anchors(
+ featmap_sizes, device=device)
+ expected_multi_level_shapes = [[
+ torch.Size([20000, 9]), torch.Size([5000, 9])
+ ]]
+ for i, single_level_anchor in enumerate(multi_level_anchors):
+ assert len(single_level_anchor) == len(expected_multi_level_shapes[i])
+ # set [:2*interval:interval] thus it could cover
+ # 2 (len(size) * len(rotations)) anchors on 2 location
+ # Note that len(size) for each class is always 1 in this case
+ for j in range(len(single_level_anchor)):
+ interval = int(expected_multi_level_shapes[i][j][0] / 2)
+ assert single_level_anchor[j][:2 * interval:interval].allclose(
+ expected_grid_anchors[i][j])
diff --git a/tests/test_utils/test_assigners.py b/tests/test_utils/test_assigners.py
new file mode 100644
index 0000000..a2e910e
--- /dev/null
+++ b/tests/test_utils/test_assigners.py
@@ -0,0 +1,150 @@
+# Copyright (c) OpenMMLab. All rights reserved.
+"""Tests the Assigner objects.
+
+CommandLine:
+ pytest tests/test_utils/test_assigner.py
+ xdoctest tests/test_utils/test_assigner.py zero
+"""
+import torch
+
+from mmdet3d.core.bbox.assigners import MaxIoUAssigner
+
+
+def test_max_iou_assigner():
+ self = MaxIoUAssigner(
+ pos_iou_thr=0.5,
+ neg_iou_thr=0.5,
+ )
+ bboxes = torch.FloatTensor([
+ [0, 0, 10, 10],
+ [10, 10, 20, 20],
+ [5, 5, 15, 15],
+ [32, 32, 38, 42],
+ ])
+ gt_bboxes = torch.FloatTensor([
+ [0, 0, 10, 9],
+ [0, 10, 10, 19],
+ ])
+ gt_labels = torch.LongTensor([2, 3])
+ assign_result = self.assign(bboxes, gt_bboxes, gt_labels=gt_labels)
+ assert len(assign_result.gt_inds) == 4
+ assert len(assign_result.labels) == 4
+
+ expected_gt_inds = torch.LongTensor([1, 0, 2, 0])
+ assert torch.all(assign_result.gt_inds == expected_gt_inds)
+
+
+def test_max_iou_assigner_with_ignore():
+ self = MaxIoUAssigner(
+ pos_iou_thr=0.5,
+ neg_iou_thr=0.5,
+ ignore_iof_thr=0.5,
+ ignore_wrt_candidates=False,
+ )
+ bboxes = torch.FloatTensor([
+ [0, 0, 10, 10],
+ [10, 10, 20, 20],
+ [5, 5, 15, 15],
+ [30, 32, 40, 42],
+ ])
+ gt_bboxes = torch.FloatTensor([
+ [0, 0, 10, 9],
+ [0, 10, 10, 19],
+ ])
+ gt_bboxes_ignore = torch.Tensor([
+ [30, 30, 40, 40],
+ ])
+ assign_result = self.assign(
+ bboxes, gt_bboxes, gt_bboxes_ignore=gt_bboxes_ignore)
+
+ expected_gt_inds = torch.LongTensor([1, 0, 2, -1])
+ assert torch.all(assign_result.gt_inds == expected_gt_inds)
+
+
+def test_max_iou_assigner_with_empty_gt():
+ """Test corner case where an image might have no true detections."""
+ self = MaxIoUAssigner(
+ pos_iou_thr=0.5,
+ neg_iou_thr=0.5,
+ )
+ bboxes = torch.FloatTensor([
+ [0, 0, 10, 10],
+ [10, 10, 20, 20],
+ [5, 5, 15, 15],
+ [32, 32, 38, 42],
+ ])
+ gt_bboxes = torch.FloatTensor(size=(0, 4))
+ assign_result = self.assign(bboxes, gt_bboxes)
+
+ expected_gt_inds = torch.LongTensor([0, 0, 0, 0])
+ assert torch.all(assign_result.gt_inds == expected_gt_inds)
+
+
+def test_max_iou_assigner_with_empty_boxes():
+ """Test corner case where an network might predict no boxes."""
+ self = MaxIoUAssigner(
+ pos_iou_thr=0.5,
+ neg_iou_thr=0.5,
+ )
+ bboxes = torch.empty((0, 4))
+ gt_bboxes = torch.FloatTensor([
+ [0, 0, 10, 9],
+ [0, 10, 10, 19],
+ ])
+ gt_labels = torch.LongTensor([2, 3])
+
+ # Test with gt_labels
+ assign_result = self.assign(bboxes, gt_bboxes, gt_labels=gt_labels)
+ assert len(assign_result.gt_inds) == 0
+ assert tuple(assign_result.labels.shape) == (0, )
+
+ # Test without gt_labels
+ assign_result = self.assign(bboxes, gt_bboxes, gt_labels=None)
+ assert len(assign_result.gt_inds) == 0
+ assert assign_result.labels is None
+
+
+def test_max_iou_assigner_with_empty_boxes_and_ignore():
+ """Test corner case where an network might predict no boxes and
+ ignore_iof_thr is on."""
+ self = MaxIoUAssigner(
+ pos_iou_thr=0.5,
+ neg_iou_thr=0.5,
+ ignore_iof_thr=0.5,
+ )
+ bboxes = torch.empty((0, 4))
+ gt_bboxes = torch.FloatTensor([
+ [0, 0, 10, 9],
+ [0, 10, 10, 19],
+ ])
+ gt_bboxes_ignore = torch.Tensor([
+ [30, 30, 40, 40],
+ ])
+ gt_labels = torch.LongTensor([2, 3])
+
+ # Test with gt_labels
+ assign_result = self.assign(
+ bboxes,
+ gt_bboxes,
+ gt_labels=gt_labels,
+ gt_bboxes_ignore=gt_bboxes_ignore)
+ assert len(assign_result.gt_inds) == 0
+ assert tuple(assign_result.labels.shape) == (0, )
+
+ # Test without gt_labels
+ assign_result = self.assign(
+ bboxes, gt_bboxes, gt_labels=None, gt_bboxes_ignore=gt_bboxes_ignore)
+ assert len(assign_result.gt_inds) == 0
+ assert assign_result.labels is None
+
+
+def test_max_iou_assigner_with_empty_boxes_and_gt():
+ """Test corner case where an network might predict no boxes and no gt."""
+ self = MaxIoUAssigner(
+ pos_iou_thr=0.5,
+ neg_iou_thr=0.5,
+ )
+ bboxes = torch.empty((0, 4))
+ gt_bboxes = torch.empty((0, 4))
+ assign_result = self.assign(bboxes, gt_bboxes)
+ assert len(assign_result.gt_inds) == 0
diff --git a/tests/test_utils/test_bbox_coders.py b/tests/test_utils/test_bbox_coders.py
new file mode 100644
index 0000000..f16bee0
--- /dev/null
+++ b/tests/test_utils/test_bbox_coders.py
@@ -0,0 +1,665 @@
+# Copyright (c) OpenMMLab. All rights reserved.
+import numpy as np
+import torch
+from mmcv.cnn import Scale
+from torch import nn as nn
+
+from mmdet3d.core.bbox import (CameraInstance3DBoxes, DepthInstance3DBoxes,
+ LiDARInstance3DBoxes)
+from mmdet.core import build_bbox_coder
+
+
+def test_partial_bin_based_box_coder():
+ box_coder_cfg = dict(
+ type='PartialBinBasedBBoxCoder',
+ num_sizes=10,
+ num_dir_bins=12,
+ with_rot=True,
+ mean_sizes=[[2.114256, 1.620300, 0.927272],
+ [0.791118, 1.279516, 0.718182],
+ [0.923508, 1.867419, 0.845495],
+ [0.591958, 0.552978, 0.827272],
+ [0.699104, 0.454178, 0.75625],
+ [0.69519, 1.346299, 0.736364],
+ [0.528526, 1.002642, 1.172878],
+ [0.500618, 0.632163, 0.683424],
+ [0.404671, 1.071108, 1.688889],
+ [0.76584, 1.398258, 0.472728]])
+ box_coder = build_bbox_coder(box_coder_cfg)
+
+ # test eocode
+ gt_bboxes = DepthInstance3DBoxes(
+ [[0.8308, 4.1168, -1.2035, 2.2493, 1.8444, 1.9245, 1.6486],
+ [2.3002, 4.8149, -1.2442, 0.5718, 0.8629, 0.9510, 1.6030],
+ [-1.1477, 1.8090, -1.1725, 0.6965, 1.5273, 2.0563, 0.0552]])
+
+ gt_labels = torch.tensor([0, 1, 2])
+ center_target, size_class_target, size_res_target, dir_class_target, \
+ dir_res_target = box_coder.encode(gt_bboxes, gt_labels)
+ expected_center_target = torch.tensor([[0.8308, 4.1168, -0.2413],
+ [2.3002, 4.8149, -0.7687],
+ [-1.1477, 1.8090, -0.1444]])
+ expected_size_class_target = torch.tensor([0, 1, 2])
+ expected_size_res_target = torch.tensor([[0.1350, 0.2241, 0.9972],
+ [-0.2193, -0.4166, 0.2328],
+ [-0.2270, -0.3401, 1.2108]])
+ expected_dir_class_target = torch.tensor([3, 3, 0])
+ expected_dir_res_target = torch.tensor([0.0778, 0.0322, 0.0552])
+ assert torch.allclose(center_target, expected_center_target, atol=1e-4)
+ assert torch.all(size_class_target == expected_size_class_target)
+ assert torch.allclose(size_res_target, expected_size_res_target, atol=1e-4)
+ assert torch.all(dir_class_target == expected_dir_class_target)
+ assert torch.allclose(dir_res_target, expected_dir_res_target, atol=1e-4)
+
+ # test decode
+ center = torch.tensor([[[0.8014, 3.4134,
+ -0.6133], [2.6375, 8.4191, 2.0438],
+ [4.2017, 5.2504,
+ -0.7851], [-1.0088, 5.4107, 1.6293],
+ [1.4837, 4.0268, 0.6222]]])
+
+ size_class = torch.tensor([[[
+ -1.0061, -2.2788, 1.1322, -4.4380, -11.0526, -2.8113, -2.0642, -7.5886,
+ -4.8627, -5.0437
+ ],
+ [
+ -2.2058, -0.3527, -1.9976, 0.8815, -2.7980,
+ -1.9053, -0.5097, -2.0232, -1.4242, -4.1192
+ ],
+ [
+ -1.4783, -0.1009, -1.1537, 0.3052, -4.3147,
+ -2.6529, 0.2729, -0.3755, -2.6479, -3.7548
+ ],
+ [
+ -6.1809, -3.5024, -8.3273, 1.1252, -4.3315,
+ -7.8288, -4.6091, -5.8153, 0.7480, -10.1396
+ ],
+ [
+ -9.0424, -3.7883, -6.0788, -1.8855,
+ -10.2493, -9.7164, -1.0658, -4.1713,
+ 1.1173, -10.6204
+ ]]])
+
+ size_res = torch.tensor([[[[-9.8976e-02, -5.2152e-01, -7.6421e-02],
+ [1.4593e-01, 5.6099e-01, 8.9421e-02],
+ [5.1481e-02, 3.9280e-01, 1.2705e-01],
+ [3.6869e-01, 7.0558e-01, 1.4647e-01],
+ [4.7683e-01, 3.3644e-01, 2.3481e-01],
+ [8.7346e-02, 8.4987e-01, 3.3265e-01],
+ [2.1393e-01, 8.5585e-01, 9.8948e-02],
+ [7.8530e-02, 5.9694e-02, -8.7211e-02],
+ [1.8551e-01, 1.1308e+00, -5.1864e-01],
+ [3.6485e-01, 7.3757e-01, 1.5264e-01]],
+ [[-9.5593e-01, -5.0455e-01, 1.9554e-01],
+ [-1.0870e-01, 1.8025e-01, 1.0228e-01],
+ [-8.2882e-02, -4.3771e-01, 9.2135e-02],
+ [-4.0840e-02, -5.9841e-02, 1.1982e-01],
+ [7.3448e-02, 5.2045e-02, 1.7301e-01],
+ [-4.0440e-02, 4.9532e-02, 1.1266e-01],
+ [3.5857e-02, 1.3564e-02, 1.0212e-01],
+ [-1.0407e-01, -5.9321e-02, 9.2622e-02],
+ [7.4691e-03, 9.3080e-02, -4.4077e-01],
+ [-6.0121e-02, -1.3381e-01, -6.8083e-02]],
+ [[-9.3970e-01, -9.7823e-01, -5.1075e-02],
+ [-1.2843e-01, -1.8381e-01, 7.1327e-02],
+ [-1.2247e-01, -8.1115e-01, 3.6495e-02],
+ [4.9154e-02, -4.5440e-02, 8.9520e-02],
+ [1.5653e-01, 3.5990e-02, 1.6414e-01],
+ [-5.9621e-02, 4.9357e-03, 1.4264e-01],
+ [8.5235e-04, -1.0030e-01, -3.0712e-02],
+ [-3.7255e-02, 2.8996e-02, 5.5545e-02],
+ [3.9298e-02, -4.7420e-02, -4.9147e-01],
+ [-1.1548e-01, -1.5895e-01, -3.9155e-02]],
+ [[-1.8725e+00, -7.4102e-01, 1.0524e+00],
+ [-3.3210e-01, 4.7828e-02, -3.2666e-02],
+ [-2.7949e-01, 5.5541e-02, -1.0059e-01],
+ [-8.5533e-02, 1.4870e-01, -1.6709e-01],
+ [3.8283e-01, 2.6609e-01, 2.1361e-01],
+ [-4.2156e-01, 3.2455e-01, 6.7309e-01],
+ [-2.4336e-02, -8.3366e-02, 3.9913e-01],
+ [8.2142e-03, 4.8323e-02, -1.5247e-01],
+ [-4.8142e-02, -3.0074e-01, -1.6829e-01],
+ [1.3274e-01, -2.3825e-01, -1.8127e-01]],
+ [[-1.2576e+00, -6.1550e-01, 7.9430e-01],
+ [-4.7222e-01, 1.5634e+00, -5.9460e-02],
+ [-3.5367e-01, 1.3616e+00, -1.6421e-01],
+ [-1.6611e-02, 2.4231e-01, -9.6188e-02],
+ [5.4486e-01, 4.6833e-01, 5.1151e-01],
+ [-6.1755e-01, 1.0292e+00, 1.2458e+00],
+ [-6.8152e-02, 2.4786e-01, 9.5088e-01],
+ [-4.8745e-02, 1.5134e-01, -9.9962e-02],
+ [2.4485e-03, -7.5991e-02, 1.3545e-01],
+ [4.1608e-01, -1.2093e-01, -3.1643e-01]]]])
+
+ dir_class = torch.tensor([[[
+ -1.0230, -5.1965, -5.2195, 2.4030, -2.7661, -7.3399, -1.1640, -4.0630,
+ -5.2940, 0.8245, -3.1869, -6.1743
+ ],
+ [
+ -1.9503, -1.6940, -0.8716, -1.1494, -0.8196,
+ 0.2862, -0.2921, -0.7894, -0.2481, -0.9916,
+ -1.4304, -1.2466
+ ],
+ [
+ -1.7435, -1.2043, -0.1265, 0.5083, -0.0717,
+ -0.9560, -1.6171, -2.6463, -2.3863, -2.1358,
+ -1.8812, -2.3117
+ ],
+ [
+ -1.9282, 0.3792, -1.8426, -1.4587, -0.8582,
+ -3.4639, -3.2133, -3.7867, -7.6781, -6.4459,
+ -6.2455, -5.4797
+ ],
+ [
+ -3.1869, 0.4456, -0.5824, 0.9994, -1.0554,
+ -8.4232, -7.7019, -7.1382, -10.2724,
+ -7.8229, -8.1860, -8.6194
+ ]]])
+
+ dir_res = torch.tensor(
+ [[[
+ 1.1022e-01, -2.3750e-01, 2.0381e-01, 1.2177e-01, -2.8501e-01,
+ 1.5351e-01, 1.2218e-01, -2.0677e-01, 1.4468e-01, 1.1593e-01,
+ -2.6864e-01, 1.1290e-01
+ ],
+ [
+ -1.5788e-02, 4.1538e-02, -2.2857e-04, -1.4011e-02, 4.2560e-02,
+ -3.1186e-03, -5.0343e-02, 6.8110e-03, -2.6728e-02, -3.2781e-02,
+ 3.6889e-02, -1.5609e-03
+ ],
+ [
+ 1.9004e-02, 5.7105e-03, 6.0329e-02, 1.3074e-02, -2.5546e-02,
+ -1.1456e-02, -3.2484e-02, -3.3487e-02, 1.6609e-03, 1.7095e-02,
+ 1.2647e-05, 2.4814e-02
+ ],
+ [
+ 1.4482e-01, -6.3083e-02, 5.8307e-02, 9.1396e-02, -8.4571e-02,
+ 4.5890e-02, 5.6243e-02, -1.2448e-01, -9.5244e-02, 4.5746e-02,
+ -1.7390e-02, 9.0267e-02
+ ],
+ [
+ 1.8065e-01, -2.0078e-02, 8.5401e-02, 1.0784e-01, -1.2495e-01,
+ 2.2796e-02, 1.1310e-01, -8.4364e-02, -1.1904e-01, 6.1180e-02,
+ -1.8109e-02, 1.1229e-01
+ ]]])
+ bbox_out = dict(
+ center=center,
+ size_class=size_class,
+ size_res=size_res,
+ dir_class=dir_class,
+ dir_res=dir_res)
+
+ bbox3d = box_coder.decode(bbox_out)
+ expected_bbox3d = torch.tensor(
+ [[[0.8014, 3.4134, -0.6133, 0.9750, 2.2602, 0.9725, 1.6926],
+ [2.6375, 8.4191, 2.0438, 0.5511, 0.4931, 0.9471, 2.6149],
+ [4.2017, 5.2504, -0.7851, 0.6411, 0.5075, 0.9168, 1.5839],
+ [-1.0088, 5.4107, 1.6293, 0.5064, 0.7017, 0.6602, 0.4605],
+ [1.4837, 4.0268, 0.6222, 0.4071, 0.9951, 1.8243, 1.6786]]])
+ assert torch.allclose(bbox3d, expected_bbox3d, atol=1e-4)
+
+ # test split_pred
+ cls_preds = torch.rand(2, 12, 256)
+ reg_preds = torch.rand(2, 67, 256)
+ base_xyz = torch.rand(2, 256, 3)
+ results = box_coder.split_pred(cls_preds, reg_preds, base_xyz)
+ obj_scores = results['obj_scores']
+ center = results['center']
+ dir_class = results['dir_class']
+ dir_res_norm = results['dir_res_norm']
+ dir_res = results['dir_res']
+ size_class = results['size_class']
+ size_res_norm = results['size_res_norm']
+ size_res = results['size_res']
+ sem_scores = results['sem_scores']
+ assert obj_scores.shape == torch.Size([2, 256, 2])
+ assert center.shape == torch.Size([2, 256, 3])
+ assert dir_class.shape == torch.Size([2, 256, 12])
+ assert dir_res_norm.shape == torch.Size([2, 256, 12])
+ assert dir_res.shape == torch.Size([2, 256, 12])
+ assert size_class.shape == torch.Size([2, 256, 10])
+ assert size_res_norm.shape == torch.Size([2, 256, 10, 3])
+ assert size_res.shape == torch.Size([2, 256, 10, 3])
+ assert sem_scores.shape == torch.Size([2, 256, 10])
+
+
+def test_anchor_free_box_coder():
+ box_coder_cfg = dict(
+ type='AnchorFreeBBoxCoder', num_dir_bins=12, with_rot=True)
+ box_coder = build_bbox_coder(box_coder_cfg)
+
+ # test encode
+ gt_bboxes = LiDARInstance3DBoxes([[
+ 2.1227e+00, 5.7951e+00, -9.9900e-01, 1.6736e+00, 4.2419e+00,
+ 1.5473e+00, -1.5501e+00
+ ],
+ [
+ 1.1791e+01, 9.0276e+00, -8.5772e-01,
+ 1.6210e+00, 3.5367e+00, 1.4841e+00,
+ -1.7369e+00
+ ],
+ [
+ 2.3638e+01, 9.6997e+00, -5.6713e-01,
+ 1.7578e+00, 4.6103e+00, 1.5999e+00,
+ -1.4556e+00
+ ]])
+ gt_labels = torch.tensor([0, 0, 0])
+
+ (center_targets, size_targets, dir_class_targets,
+ dir_res_targets) = box_coder.encode(gt_bboxes, gt_labels)
+
+ expected_center_target = torch.tensor([[2.1227, 5.7951, -0.2253],
+ [11.7908, 9.0276, -0.1156],
+ [23.6380, 9.6997, 0.2328]])
+ expected_size_targets = torch.tensor([[0.8368, 2.1210, 0.7736],
+ [0.8105, 1.7683, 0.7421],
+ [0.8789, 2.3052, 0.8000]])
+ expected_dir_class_target = torch.tensor([9, 9, 9])
+ expected_dir_res_target = torch.tensor([0.0394, -0.3172, 0.2199])
+ assert torch.allclose(center_targets, expected_center_target, atol=1e-4)
+ assert torch.allclose(size_targets, expected_size_targets, atol=1e-4)
+ assert torch.all(dir_class_targets == expected_dir_class_target)
+ assert torch.allclose(dir_res_targets, expected_dir_res_target, atol=1e-3)
+
+ # test decode
+ center = torch.tensor([[[14.5954, 6.3312, 0.7671],
+ [67.5245, 22.4422, 1.5610],
+ [47.7693, -6.7980, 1.4395]]])
+
+ size_res = torch.tensor([[[-1.0752, 1.8760, 0.7715],
+ [-0.8016, 1.1754, 0.0102],
+ [-1.2789, 0.5948, 0.4728]]])
+
+ dir_class = torch.tensor([[[
+ 0.1512, 1.7914, -1.7658, 2.1572, -0.9215, 1.2139, 0.1749, 0.8606,
+ 1.1743, -0.7679, -1.6005, 0.4623
+ ],
+ [
+ -0.3957, 1.2026, -1.2677, 1.3863, -0.5754,
+ 1.7083, 0.2601, 0.1129, 0.7146, -0.1367,
+ -1.2892, -0.0083
+ ],
+ [
+ -0.8862, 1.2050, -1.3881, 1.6604, -0.9087,
+ 1.1907, -0.0280, 0.2027, 1.0644, -0.7205,
+ -1.0738, 0.4748
+ ]]])
+
+ dir_res = torch.tensor([[[
+ 1.1151, 0.5535, -0.2053, -0.6582, -0.1616, -0.1821, 0.4675, 0.6621,
+ 0.8146, -0.0448, -0.7253, -0.7171
+ ],
+ [
+ 0.7888, 0.2478, -0.1962, -0.7267, 0.0573,
+ -0.2398, 0.6984, 0.5859, 0.7507, -0.1980,
+ -0.6538, -0.6602
+ ],
+ [
+ 0.9039, 0.6109, 0.1960, -0.5016, 0.0551,
+ -0.4086, 0.3398, 0.2759, 0.7247, -0.0655,
+ -0.5052, -0.9026
+ ]]])
+ bbox_out = dict(
+ center=center, size=size_res, dir_class=dir_class, dir_res=dir_res)
+
+ bbox3d = box_coder.decode(bbox_out)
+ expected_bbox3d = torch.tensor(
+ [[[14.5954, 6.3312, 0.7671, 0.1000, 3.7521, 1.5429, 0.9126],
+ [67.5245, 22.4422, 1.5610, 0.1000, 2.3508, 0.1000, 2.3782],
+ [47.7693, -6.7980, 1.4395, 0.1000, 1.1897, 0.9456, 1.0692]]])
+ assert torch.allclose(bbox3d, expected_bbox3d, atol=1e-4)
+
+ # test split_pred
+ cls_preds = torch.rand(2, 1, 256)
+ reg_preds = torch.rand(2, 30, 256)
+ base_xyz = torch.rand(2, 256, 3)
+ results = box_coder.split_pred(cls_preds, reg_preds, base_xyz)
+ obj_scores = results['obj_scores']
+ center = results['center']
+ center_offset = results['center_offset']
+ dir_class = results['dir_class']
+ dir_res_norm = results['dir_res_norm']
+ dir_res = results['dir_res']
+ size = results['size']
+ assert obj_scores.shape == torch.Size([2, 1, 256])
+ assert center.shape == torch.Size([2, 256, 3])
+ assert center_offset.shape == torch.Size([2, 256, 3])
+ assert dir_class.shape == torch.Size([2, 256, 12])
+ assert dir_res_norm.shape == torch.Size([2, 256, 12])
+ assert dir_res.shape == torch.Size([2, 256, 12])
+ assert size.shape == torch.Size([2, 256, 3])
+
+
+def test_centerpoint_bbox_coder():
+ bbox_coder_cfg = dict(
+ type='CenterPointBBoxCoder',
+ post_center_range=[-61.2, -61.2, -10.0, 61.2, 61.2, 10.0],
+ max_num=500,
+ score_threshold=0.1,
+ pc_range=[-51.2, -51.2],
+ out_size_factor=4,
+ voxel_size=[0.2, 0.2])
+
+ bbox_coder = build_bbox_coder(bbox_coder_cfg)
+
+ batch_dim = torch.rand([2, 3, 128, 128])
+ batch_hei = torch.rand([2, 1, 128, 128])
+ batch_hm = torch.rand([2, 2, 128, 128])
+ batch_reg = torch.rand([2, 2, 128, 128])
+ batch_rotc = torch.rand([2, 1, 128, 128])
+ batch_rots = torch.rand([2, 1, 128, 128])
+ batch_vel = torch.rand([2, 2, 128, 128])
+
+ temp = bbox_coder.decode(batch_hm, batch_rots, batch_rotc, batch_hei,
+ batch_dim, batch_vel, batch_reg, 5)
+ for i in range(len(temp)):
+ assert temp[i]['bboxes'].shape == torch.Size([500, 9])
+ assert temp[i]['scores'].shape == torch.Size([500])
+ assert temp[i]['labels'].shape == torch.Size([500])
+
+
+def test_point_xyzwhlr_bbox_coder():
+ bbox_coder_cfg = dict(
+ type='PointXYZWHLRBBoxCoder',
+ use_mean_size=True,
+ mean_size=[[3.9, 1.6, 1.56], [0.8, 0.6, 1.73], [1.76, 0.6, 1.73]])
+ boxcoder = build_bbox_coder(bbox_coder_cfg)
+
+ # test encode
+ gt_bboxes_3d = torch.tensor(
+ [[13.3329, 2.3514, -0.7004, 1.7508, 0.4702, 1.7909, -3.0522],
+ [2.2068, -2.6994, -0.3277, 3.8703, 1.6602, 1.6913, -1.9057],
+ [5.5269, 2.5085, -1.0129, 1.1496, 0.8006, 1.8887, 2.1756]])
+
+ points = torch.tensor([[13.70, 2.40, 0.12], [3.20, -3.00, 0.2],
+ [5.70, 2.20, -0.4]])
+
+ gt_labels_3d = torch.tensor([2, 0, 1])
+
+ bbox_target = boxcoder.encode(gt_bboxes_3d, points, gt_labels_3d)
+ expected_bbox_target = torch.tensor([[
+ -0.1974, -0.0261, -0.4742, -0.0052, -0.2438, 0.0346, -0.9960, -0.0893
+ ], [-0.2356, 0.0713, -0.3383, -0.0076, 0.0369, 0.0808, -0.3287, -0.9444
+ ], [-0.1731, 0.3085, -0.3543, 0.3626, 0.2884, 0.0878, -0.5686,
+ 0.8226]])
+ assert torch.allclose(expected_bbox_target, bbox_target, atol=1e-4)
+ # test decode
+ bbox3d_out = boxcoder.decode(bbox_target, points, gt_labels_3d)
+ assert torch.allclose(bbox3d_out, gt_bboxes_3d, atol=1e-4)
+
+
+def test_fcos3d_bbox_coder():
+ # test a config without priors
+ bbox_coder_cfg = dict(
+ type='FCOS3DBBoxCoder',
+ base_depths=None,
+ base_dims=None,
+ code_size=7,
+ norm_on_bbox=True)
+ bbox_coder = build_bbox_coder(bbox_coder_cfg)
+
+ # test decode
+ # [2, 7, 1, 1]
+ batch_bbox = torch.tensor([[[[0.3130]], [[0.7094]], [[0.8743]], [[0.0570]],
+ [[0.5579]], [[0.1593]], [[0.4553]]],
+ [[[0.7758]], [[0.2298]], [[0.3925]], [[0.6307]],
+ [[0.4377]], [[0.3339]], [[0.1966]]]])
+ batch_scale = nn.ModuleList([Scale(1.0) for _ in range(3)])
+ stride = 2
+ training = False
+ cls_score = torch.randn([2, 2, 1, 1]).sigmoid()
+ decode_bbox = bbox_coder.decode(batch_bbox, batch_scale, stride, training,
+ cls_score)
+
+ expected_bbox = torch.tensor([[[[0.6261]], [[1.4188]], [[2.3971]],
+ [[1.0586]], [[1.7470]], [[1.1727]],
+ [[0.4553]]],
+ [[[1.5516]], [[0.4596]], [[1.4806]],
+ [[1.8790]], [[1.5492]], [[1.3965]],
+ [[0.1966]]]])
+ assert torch.allclose(decode_bbox, expected_bbox, atol=1e-3)
+
+ # test a config with priors
+ prior_bbox_coder_cfg = dict(
+ type='FCOS3DBBoxCoder',
+ base_depths=((28., 13.), (25., 12.)),
+ base_dims=((2., 3., 1.), (1., 2., 3.)),
+ code_size=7,
+ norm_on_bbox=True)
+ prior_bbox_coder = build_bbox_coder(prior_bbox_coder_cfg)
+
+ # test decode
+ batch_bbox = torch.tensor([[[[0.3130]], [[0.7094]], [[0.8743]], [[0.0570]],
+ [[0.5579]], [[0.1593]], [[0.4553]]],
+ [[[0.7758]], [[0.2298]], [[0.3925]], [[0.6307]],
+ [[0.4377]], [[0.3339]], [[0.1966]]]])
+ batch_scale = nn.ModuleList([Scale(1.0) for _ in range(3)])
+ stride = 2
+ training = False
+ cls_score = torch.tensor([[[[0.5811]], [[0.6198]]], [[[0.4889]],
+ [[0.8142]]]])
+ decode_bbox = prior_bbox_coder.decode(batch_bbox, batch_scale, stride,
+ training, cls_score)
+ expected_bbox = torch.tensor([[[[0.6260]], [[1.4188]], [[35.4916]],
+ [[1.0587]], [[3.4940]], [[3.5181]],
+ [[0.4553]]],
+ [[[1.5516]], [[0.4596]], [[29.7100]],
+ [[1.8789]], [[3.0983]], [[4.1892]],
+ [[0.1966]]]])
+ assert torch.allclose(decode_bbox, expected_bbox, atol=1e-3)
+
+ # test decode_yaw
+ decode_bbox = decode_bbox.permute(0, 2, 3, 1).view(-1, 7)
+ batch_centers2d = torch.tensor([[100., 150.], [200., 100.]])
+ batch_dir_cls = torch.tensor([0., 1.])
+ dir_offset = 0.7854
+ cam2img = torch.tensor([[700., 0., 450., 0.], [0., 700., 200., 0.],
+ [0., 0., 1., 0.], [0., 0., 0., 1.]])
+ decode_bbox = prior_bbox_coder.decode_yaw(decode_bbox, batch_centers2d,
+ batch_dir_cls, dir_offset,
+ cam2img)
+ expected_bbox = torch.tensor(
+ [[0.6260, 1.4188, 35.4916, 1.0587, 3.4940, 3.5181, 3.1332],
+ [1.5516, 0.4596, 29.7100, 1.8789, 3.0983, 4.1892, 6.1368]])
+ assert torch.allclose(decode_bbox, expected_bbox, atol=1e-3)
+
+
+def test_pgd_bbox_coder():
+ # test a config without priors
+ bbox_coder_cfg = dict(
+ type='PGDBBoxCoder',
+ base_depths=None,
+ base_dims=None,
+ code_size=7,
+ norm_on_bbox=True)
+ bbox_coder = build_bbox_coder(bbox_coder_cfg)
+
+ # test decode_2d
+ # [2, 27, 1, 1]
+ batch_bbox = torch.tensor([[[[0.0103]], [[0.7394]], [[0.3296]], [[0.4708]],
+ [[0.1439]], [[0.0778]], [[0.9399]], [[0.8366]],
+ [[0.1264]], [[0.3030]], [[0.1898]], [[0.0714]],
+ [[0.4144]], [[0.4341]], [[0.6442]], [[0.2951]],
+ [[0.2890]], [[0.4486]], [[0.2848]], [[0.1071]],
+ [[0.9530]], [[0.9460]], [[0.3822]], [[0.9320]],
+ [[0.2611]], [[0.5580]], [[0.0397]]],
+ [[[0.8612]], [[0.1680]], [[0.5167]], [[0.8502]],
+ [[0.0377]], [[0.3615]], [[0.9550]], [[0.5219]],
+ [[0.1402]], [[0.6843]], [[0.2121]], [[0.9468]],
+ [[0.6238]], [[0.7918]], [[0.1646]], [[0.0500]],
+ [[0.6290]], [[0.3956]], [[0.2901]], [[0.4612]],
+ [[0.7333]], [[0.1194]], [[0.6999]], [[0.3980]],
+ [[0.3262]], [[0.7185]], [[0.4474]]]])
+ batch_scale = nn.ModuleList([Scale(1.0) for _ in range(5)])
+ stride = 2
+ training = False
+ cls_score = torch.randn([2, 2, 1, 1]).sigmoid()
+ decode_bbox = bbox_coder.decode(batch_bbox, batch_scale, stride, training,
+ cls_score)
+ max_regress_range = 16
+ pred_keypoints = True
+ pred_bbox2d = True
+ decode_bbox_w2d = bbox_coder.decode_2d(decode_bbox, batch_scale, stride,
+ max_regress_range, training,
+ pred_keypoints, pred_bbox2d)
+ expected_decode_bbox_w2d = torch.tensor(
+ [[[[0.0206]], [[1.4788]],
+ [[1.3904]], [[1.6013]], [[1.1548]], [[1.0809]], [[0.9399]],
+ [[10.9441]], [[2.0117]], [[4.7049]], [[3.0009]], [[1.1405]],
+ [[6.2752]], [[6.5399]], [[9.0840]], [[4.5892]], [[4.4994]],
+ [[6.7320]], [[4.4375]], [[1.7071]], [[11.8582]], [[11.8075]],
+ [[5.8339]], [[1.8640]], [[0.5222]], [[1.1160]], [[0.0794]]],
+ [[[1.7224]], [[0.3360]], [[1.6765]], [[2.3401]], [[1.0384]],
+ [[1.4355]], [[0.9550]], [[7.6666]], [[2.2286]], [[9.5089]],
+ [[3.3436]], [[11.8133]], [[8.8603]], [[10.5508]], [[2.6101]],
+ [[0.7993]], [[8.9178]], [[6.0188]], [[4.5156]], [[6.8970]],
+ [[10.0013]], [[1.9014]], [[9.6689]], [[0.7960]], [[0.6524]],
+ [[1.4370]], [[0.8948]]]])
+ assert torch.allclose(expected_decode_bbox_w2d, decode_bbox_w2d, atol=1e-3)
+
+ # test decode_prob_depth
+ # [10, 8]
+ depth_cls_preds = torch.tensor([
+ [-0.4383, 0.7207, -0.4092, 0.4649, 0.8526, 0.6186, -1.4312, -0.7150],
+ [0.0621, 0.2369, 0.5170, 0.8484, -0.1099, 0.1829, -0.0072, 1.0618],
+ [-1.6114, -0.1057, 0.5721, -0.5986, -2.0471, 0.8140, -0.8385, -0.4822],
+ [0.0742, -0.3261, 0.4607, 1.8155, -0.3571, -0.0234, 0.3787, 2.3251],
+ [1.0492, -0.6881, -0.0136, -1.8291, 0.8460, -1.0171, 2.5691, -0.8114],
+ [0.0968, -0.5601, 1.0458, 0.2560, 1.3018, 0.1635, 0.0680, -1.0263],
+ [-0.0765, 0.1498, -2.7321, 1.0047, -0.2505, 0.0871, -0.4820, -0.3003],
+ [-0.4123, 0.2298, -0.1330, -0.6008, 0.6526, 0.7118, 0.9728, -0.7793],
+ [1.6940, 0.3355, 1.4661, 0.5477, 0.8667, 0.0527, -0.9975, -0.0689],
+ [0.4724, -0.3632, -0.0654, 0.4034, -0.3494, -0.7548, 0.7297, 1.2754]
+ ])
+ depth_range = (0, 70)
+ depth_unit = 10
+ num_depth_cls = 8
+ uniform_prob_depth_preds = bbox_coder.decode_prob_depth(
+ depth_cls_preds, depth_range, depth_unit, 'uniform', num_depth_cls)
+ expected_preds = torch.tensor([
+ 32.0441, 38.4689, 36.1831, 48.2096, 46.1560, 32.7973, 33.2155, 39.9822,
+ 21.9905, 43.0161
+ ])
+ assert torch.allclose(uniform_prob_depth_preds, expected_preds, atol=1e-3)
+
+ linear_prob_depth_preds = bbox_coder.decode_prob_depth(
+ depth_cls_preds, depth_range, depth_unit, 'linear', num_depth_cls)
+ expected_preds = torch.tensor([
+ 21.1431, 30.2421, 25.8964, 41.6116, 38.6234, 21.4582, 23.2993, 30.1111,
+ 13.9273, 36.8419
+ ])
+ assert torch.allclose(linear_prob_depth_preds, expected_preds, atol=1e-3)
+
+ log_prob_depth_preds = bbox_coder.decode_prob_depth(
+ depth_cls_preds, depth_range, depth_unit, 'log', num_depth_cls)
+ expected_preds = torch.tensor([
+ 12.6458, 24.2487, 17.4015, 36.9375, 27.5982, 12.5510, 15.6635, 19.8408,
+ 9.1605, 31.3765
+ ])
+ assert torch.allclose(log_prob_depth_preds, expected_preds, atol=1e-3)
+
+ loguniform_prob_depth_preds = bbox_coder.decode_prob_depth(
+ depth_cls_preds, depth_range, depth_unit, 'loguniform', num_depth_cls)
+ expected_preds = torch.tensor([
+ 6.9925, 10.3273, 8.9895, 18.6524, 16.4667, 7.3196, 7.5078, 11.3207,
+ 3.7987, 13.6095
+ ])
+ assert torch.allclose(
+ loguniform_prob_depth_preds, expected_preds, atol=1e-3)
+
+
+def test_smoke_bbox_coder():
+ bbox_coder_cfg = dict(
+ type='SMOKECoder',
+ base_depth=(28.01, 16.32),
+ base_dims=((3.88, 1.63, 1.53), (1.78, 1.70, 0.58), (0.88, 1.73, 0.67)),
+ code_size=7)
+
+ bbox_coder = build_bbox_coder(bbox_coder_cfg)
+ regression = torch.rand([200, 8])
+ points = torch.rand([200, 2])
+ labels = torch.ones([2, 100])
+ cam2imgs = torch.rand([2, 4, 4])
+ trans_mats = torch.rand([2, 3, 3])
+
+ img_metas = [dict(box_type_3d=CameraInstance3DBoxes) for i in range(2)]
+ locations, dimensions, orientations = bbox_coder.decode(
+ regression, points, labels, cam2imgs, trans_mats)
+ assert locations.shape == torch.Size([200, 3])
+ assert dimensions.shape == torch.Size([200, 3])
+ assert orientations.shape == torch.Size([200, 1])
+ bboxes = bbox_coder.encode(locations, dimensions, orientations, img_metas)
+ assert bboxes.tensor.shape == torch.Size([200, 7])
+
+ # specically designed to test orientation decode function's
+ # special cases.
+ ori_vector = torch.tensor([[-0.9, -0.01], [-0.9, 0.01]])
+ locations = torch.tensor([[15., 2., 1.], [15., 2., -1.]])
+ orientations = bbox_coder._decode_orientation(ori_vector, locations)
+ assert orientations.shape == torch.Size([2, 1])
+
+
+def test_monoflex_bbox_coder():
+ bbox_coder_cfg = dict(
+ type='MonoFlexCoder',
+ depth_mode='exp',
+ base_depth=(26.494627, 16.05988),
+ depth_range=[0.1, 100],
+ combine_depth=True,
+ uncertainty_range=[-10, 10],
+ base_dims=((3.8840, 1.5261, 1.6286, 0.4259, 0.1367,
+ 0.1022), (0.8423, 1.7607, 0.6602, 0.2349, 0.1133, 0.1427),
+ (1.7635, 1.7372, 0.5968, 0.1766, 0.0948, 0.1242)),
+ dims_mode='linear',
+ multibin=True,
+ num_dir_bins=4,
+ bin_centers=[0, np.pi / 2, np.pi, -np.pi / 2],
+ bin_margin=np.pi / 6,
+ code_size=7)
+ bbox_coder = build_bbox_coder(bbox_coder_cfg)
+ gt_bboxes_3d = CameraInstance3DBoxes(torch.rand([6, 7]))
+ orientation_target = bbox_coder.encode(gt_bboxes_3d)
+ assert orientation_target.shape == torch.Size([6, 8])
+
+ regression = torch.rand([100, 50])
+ base_centers2d = torch.rand([100, 2])
+ labels = torch.ones([100])
+ downsample_ratio = 4
+ cam2imgs = torch.rand([100, 4, 4])
+
+ preds = bbox_coder.decode(regression, base_centers2d, labels,
+ downsample_ratio, cam2imgs)
+
+ assert preds['bboxes2d'].shape == torch.Size([100, 4])
+ assert preds['dimensions'].shape == torch.Size([100, 3])
+ assert preds['offsets2d'].shape == torch.Size([100, 2])
+ assert preds['keypoints2d'].shape == torch.Size([100, 10, 2])
+ assert preds['orientations'].shape == torch.Size([100, 16])
+ assert preds['direct_depth'].shape == torch.Size([
+ 100,
+ ])
+ assert preds['keypoints_depth'].shape == torch.Size([100, 3])
+ assert preds['combined_depth'].shape == torch.Size([
+ 100,
+ ])
+ assert preds['direct_depth_uncertainty'].shape == torch.Size([
+ 100,
+ ])
+ assert preds['keypoints_depth_uncertainty'].shape == torch.Size([100, 3])
+
+ offsets_2d = torch.randn([100, 2])
+ depths = torch.randn([
+ 100,
+ ])
+ locations = bbox_coder.decode_location(base_centers2d, offsets_2d, depths,
+ cam2imgs, downsample_ratio)
+ assert locations.shape == torch.Size([100, 3])
+
+ orientations = torch.randn([100, 16])
+ yaws, local_yaws = bbox_coder.decode_orientation(orientations, locations)
+ assert yaws.shape == torch.Size([
+ 100,
+ ])
+ assert local_yaws.shape == torch.Size([
+ 100,
+ ])
diff --git a/tests/test_utils/test_box3d.py b/tests/test_utils/test_box3d.py
new file mode 100644
index 0000000..69d8b31
--- /dev/null
+++ b/tests/test_utils/test_box3d.py
@@ -0,0 +1,1797 @@
+# Copyright (c) OpenMMLab. All rights reserved.
+import unittest
+
+import numpy as np
+import pytest
+import torch
+
+from mmdet3d.core.bbox import (BaseInstance3DBoxes, Box3DMode,
+ CameraInstance3DBoxes, Coord3DMode,
+ DepthInstance3DBoxes, LiDARInstance3DBoxes,
+ bbox3d2roi, bbox3d_mapping_back)
+from mmdet3d.core.bbox.structures.utils import (get_box_type, limit_period,
+ points_cam2img,
+ rotation_3d_in_axis,
+ xywhr2xyxyr)
+from mmdet3d.core.points import CameraPoints, DepthPoints, LiDARPoints
+
+
+def test_bbox3d_mapping_back():
+ bboxes = BaseInstance3DBoxes(
+ [[
+ -5.24223238e+00, 4.00209696e+01, 2.97570381e-01, 2.06200000e+00,
+ 4.40900000e+00, 1.54800000e+00, -1.48801203e+00
+ ],
+ [
+ -2.66751588e+01, 5.59499564e+00, -9.14345860e-01, 3.43000000e-01,
+ 4.58000000e-01, 7.82000000e-01, -4.62759755e+00
+ ],
+ [
+ -5.80979675e+00, 3.54092357e+01, 2.00889888e-01, 2.39600000e+00,
+ 3.96900000e+00, 1.73200000e+00, -4.65203216e+00
+ ],
+ [
+ -3.13086877e+01, 1.09007628e+00, -1.94612112e-01, 1.94400000e+00,
+ 3.85700000e+00, 1.72300000e+00, -2.81427027e+00
+ ]])
+ new_bboxes = bbox3d_mapping_back(bboxes, 1.1, True, True)
+ expected_new_bboxes = torch.tensor(
+ [[-4.7657, 36.3827, 0.2705, 1.8745, 4.0082, 1.4073, -1.4880],
+ [-24.2501, 5.0864, -0.8312, 0.3118, 0.4164, 0.7109, -4.6276],
+ [-5.2816, 32.1902, 0.1826, 2.1782, 3.6082, 1.5745, -4.6520],
+ [-28.4624, 0.9910, -0.1769, 1.7673, 3.5064, 1.5664, -2.8143]])
+ assert torch.allclose(new_bboxes.tensor, expected_new_bboxes, atol=1e-4)
+
+
+def test_bbox3d2roi():
+ bbox_0 = torch.tensor(
+ [[-5.2422, 4.0020, 2.9757, 2.0620, 4.4090, 1.5480, -1.4880],
+ [-5.8097, 3.5409, 2.0088, 2.3960, 3.9690, 1.7320, -4.6520]])
+ bbox_1 = torch.tensor(
+ [[-2.6675, 5.5949, -9.1434, 3.4300, 4.5800, 7.8200, -4.6275],
+ [-3.1308, 1.0900, -1.9461, 1.9440, 3.8570, 1.7230, -2.8142]])
+ bbox_list = [bbox_0, bbox_1]
+ rois = bbox3d2roi(bbox_list)
+ expected_rois = torch.tensor(
+ [[0.0000, -5.2422, 4.0020, 2.9757, 2.0620, 4.4090, 1.5480, -1.4880],
+ [0.0000, -5.8097, 3.5409, 2.0088, 2.3960, 3.9690, 1.7320, -4.6520],
+ [1.0000, -2.6675, 5.5949, -9.1434, 3.4300, 4.5800, 7.8200, -4.6275],
+ [1.0000, -3.1308, 1.0900, -1.9461, 1.9440, 3.8570, 1.7230, -2.8142]])
+ assert torch.all(torch.eq(rois, expected_rois))
+
+
+def test_base_boxes3d():
+ # test empty initialization
+ empty_boxes = []
+ boxes = BaseInstance3DBoxes(empty_boxes)
+ assert boxes.tensor.shape[0] == 0
+ assert boxes.tensor.shape[1] == 7
+
+ # Test init with origin
+ gravity_center_box = np.array(
+ [[
+ -5.24223238e+00, 4.00209696e+01, 2.97570381e-01, 2.06200000e+00,
+ 4.40900000e+00, 1.54800000e+00, -1.48801203e+00
+ ],
+ [
+ -2.66751588e+01, 5.59499564e+00, -9.14345860e-01, 3.43000000e-01,
+ 4.58000000e-01, 7.82000000e-01, -4.62759755e+00
+ ],
+ [
+ -5.80979675e+00, 3.54092357e+01, 2.00889888e-01, 2.39600000e+00,
+ 3.96900000e+00, 1.73200000e+00, -4.65203216e+00
+ ],
+ [
+ -3.13086877e+01, 1.09007628e+00, -1.94612112e-01, 1.94400000e+00,
+ 3.85700000e+00, 1.72300000e+00, -2.81427027e+00
+ ]],
+ dtype=np.float32)
+
+ bottom_center_box = BaseInstance3DBoxes(
+ gravity_center_box, origin=(0.5, 0.5, 0.5))
+
+ assert bottom_center_box.yaw.shape[0] == 4
+
+
+def test_lidar_boxes3d():
+ # test empty initialization
+ empty_boxes = []
+ boxes = LiDARInstance3DBoxes(empty_boxes)
+ assert boxes.tensor.shape[0] == 0
+ assert boxes.tensor.shape[1] == 7
+
+ # Test init with origin
+ gravity_center_box = np.array(
+ [[
+ -5.24223238e+00, 4.00209696e+01, 2.97570381e-01, 2.06200000e+00,
+ 4.40900000e+00, 1.54800000e+00, -1.48801203e+00
+ ],
+ [
+ -2.66751588e+01, 5.59499564e+00, -9.14345860e-01, 3.43000000e-01,
+ 4.58000000e-01, 7.82000000e-01, -4.62759755e+00
+ ],
+ [
+ -5.80979675e+00, 3.54092357e+01, 2.00889888e-01, 2.39600000e+00,
+ 3.96900000e+00, 1.73200000e+00, -4.65203216e+00
+ ],
+ [
+ -3.13086877e+01, 1.09007628e+00, -1.94612112e-01, 1.94400000e+00,
+ 3.85700000e+00, 1.72300000e+00, -2.81427027e+00
+ ]],
+ dtype=np.float32)
+ bottom_center_box = LiDARInstance3DBoxes(
+ gravity_center_box, origin=(0.5, 0.5, 0.5))
+ expected_tensor = torch.tensor(
+ [[
+ -5.24223238e+00, 4.00209696e+01, -4.76429619e-01, 2.06200000e+00,
+ 4.40900000e+00, 1.54800000e+00, -1.48801203e+00
+ ],
+ [
+ -2.66751588e+01, 5.59499564e+00, -1.30534586e+00, 3.43000000e-01,
+ 4.58000000e-01, 7.82000000e-01, -4.62759755e+00
+ ],
+ [
+ -5.80979675e+00, 3.54092357e+01, -6.65110112e-01, 2.39600000e+00,
+ 3.96900000e+00, 1.73200000e+00, -4.65203216e+00
+ ],
+ [
+ -3.13086877e+01, 1.09007628e+00, -1.05611211e+00, 1.94400000e+00,
+ 3.85700000e+00, 1.72300000e+00, -2.81427027e+00
+ ]])
+ assert torch.allclose(expected_tensor, bottom_center_box.tensor)
+
+ # Test init with numpy array
+ np_boxes = np.array([[
+ 1.7802081, 2.516249, -1.7501148, 1.75, 3.39, 1.65,
+ 1.48 - 0.13603681398218053 * 4
+ ],
+ [
+ 8.959413, 2.4567227, -1.6357126, 1.54, 4.01, 1.57,
+ 1.62 - 0.13603681398218053 * 4
+ ]],
+ dtype=np.float32)
+ boxes_1 = LiDARInstance3DBoxes(np_boxes)
+ assert torch.allclose(boxes_1.tensor, torch.from_numpy(np_boxes))
+
+ # test properties
+ assert boxes_1.volume.size(0) == 2
+ assert (boxes_1.center == boxes_1.bottom_center).all()
+ assert repr(boxes) == (
+ 'LiDARInstance3DBoxes(\n tensor([], size=(0, 7)))')
+
+ # test init with torch.Tensor
+ th_boxes = torch.tensor(
+ [[
+ 28.29669987, -0.5557558, -1.30332506, 1.47000003, 2.23000002,
+ 1.48000002, -1.57000005 - 0.13603681398218053 * 4
+ ],
+ [
+ 26.66901946, 21.82302134, -1.73605708, 1.55999994, 3.48000002,
+ 1.39999998, -1.69000006 - 0.13603681398218053 * 4
+ ],
+ [
+ 31.31977974, 8.16214412, -1.62177875, 1.74000001, 3.76999998,
+ 1.48000002, 2.78999996 - 0.13603681398218053 * 4
+ ]],
+ dtype=torch.float32)
+ boxes_2 = LiDARInstance3DBoxes(th_boxes)
+ assert torch.allclose(boxes_2.tensor, th_boxes)
+
+ # test clone/to/device
+ boxes_2 = boxes_2.clone()
+ boxes_1 = boxes_1.to(boxes_2.device)
+
+ # test box concatenation
+ expected_tensor = torch.tensor([[
+ 1.7802081, 2.516249, -1.7501148, 1.75, 3.39, 1.65,
+ 1.48 - 0.13603681398218053 * 4
+ ],
+ [
+ 8.959413, 2.4567227, -1.6357126, 1.54,
+ 4.01, 1.57,
+ 1.62 - 0.13603681398218053 * 4
+ ],
+ [
+ 28.2967, -0.5557558, -1.303325, 1.47,
+ 2.23, 1.48,
+ -1.57 - 0.13603681398218053 * 4
+ ],
+ [
+ 26.66902, 21.82302, -1.736057, 1.56,
+ 3.48, 1.4,
+ -1.69 - 0.13603681398218053 * 4
+ ],
+ [
+ 31.31978, 8.162144, -1.6217787, 1.74,
+ 3.77, 1.48,
+ 2.79 - 0.13603681398218053 * 4
+ ]])
+ boxes = LiDARInstance3DBoxes.cat([boxes_1, boxes_2])
+ assert torch.allclose(boxes.tensor, expected_tensor)
+ # concatenate empty list
+ empty_boxes = LiDARInstance3DBoxes.cat([])
+ assert empty_boxes.tensor.shape[0] == 0
+ assert empty_boxes.tensor.shape[-1] == 7
+
+ # test box flip
+ points = torch.tensor([[1.2559, -0.6762, -1.4658],
+ [4.7814, -0.8784,
+ -1.3857], [6.7053, 0.2517, -0.9697],
+ [0.6533, -0.5520, -0.5265],
+ [4.5870, 0.5358, -1.4741]])
+ expected_tensor = torch.tensor(
+ [[
+ 1.7802081, -2.516249, -1.7501148, 1.75, 3.39, 1.65,
+ 1.6615927 - np.pi + 0.13603681398218053 * 4
+ ],
+ [
+ 8.959413, -2.4567227, -1.6357126, 1.54, 4.01, 1.57,
+ 1.5215927 - np.pi + 0.13603681398218053 * 4
+ ],
+ [
+ 28.2967, 0.5557558, -1.303325, 1.47, 2.23, 1.48,
+ 4.7115927 - np.pi + 0.13603681398218053 * 4
+ ],
+ [
+ 26.66902, -21.82302, -1.736057, 1.56, 3.48, 1.4,
+ 4.8315926 - np.pi + 0.13603681398218053 * 4
+ ],
+ [
+ 31.31978, -8.162144, -1.6217787, 1.74, 3.77, 1.48,
+ 0.35159278 - np.pi + 0.13603681398218053 * 4
+ ]])
+ expected_points = torch.tensor([[1.2559, 0.6762, -1.4658],
+ [4.7814, 0.8784, -1.3857],
+ [6.7053, -0.2517, -0.9697],
+ [0.6533, 0.5520, -0.5265],
+ [4.5870, -0.5358, -1.4741]])
+ points = boxes.flip('horizontal', points)
+ assert torch.allclose(boxes.tensor, expected_tensor)
+ assert torch.allclose(points, expected_points, 1e-3)
+
+ expected_tensor = torch.tensor(
+ [[
+ -1.7802, -2.5162, -1.7501, 1.7500, 3.3900, 1.6500,
+ -1.6616 + np.pi * 2 - 0.13603681398218053 * 4
+ ],
+ [
+ -8.9594, -2.4567, -1.6357, 1.5400, 4.0100, 1.5700,
+ -1.5216 + np.pi * 2 - 0.13603681398218053 * 4
+ ],
+ [
+ -28.2967, 0.5558, -1.3033, 1.4700, 2.2300, 1.4800,
+ -4.7116 + np.pi * 2 - 0.13603681398218053 * 4
+ ],
+ [
+ -26.6690, -21.8230, -1.7361, 1.5600, 3.4800, 1.4000,
+ -4.8316 + np.pi * 2 - 0.13603681398218053 * 4
+ ],
+ [
+ -31.3198, -8.1621, -1.6218, 1.7400, 3.7700, 1.4800,
+ -0.3516 + np.pi * 2 - 0.13603681398218053 * 4
+ ]])
+ boxes_flip_vert = boxes.clone()
+ points = boxes_flip_vert.flip('vertical', points)
+ expected_points = torch.tensor([[-1.2559, 0.6762, -1.4658],
+ [-4.7814, 0.8784, -1.3857],
+ [-6.7053, -0.2517, -0.9697],
+ [-0.6533, 0.5520, -0.5265],
+ [-4.5870, -0.5358, -1.4741]])
+ assert torch.allclose(boxes_flip_vert.tensor, expected_tensor, 1e-4)
+ assert torch.allclose(points, expected_points)
+
+ # test box rotation
+ # with input torch.Tensor points and angle
+ expected_tensor = torch.tensor(
+ [[
+ 1.4225, -2.7344, -1.7501, 1.7500, 3.3900, 1.6500,
+ 1.7976 - np.pi + 0.13603681398218053 * 2
+ ],
+ [
+ 8.5435, -3.6491, -1.6357, 1.5400, 4.0100, 1.5700,
+ 1.6576 - np.pi + 0.13603681398218053 * 2
+ ],
+ [
+ 28.1106, -3.2869, -1.3033, 1.4700, 2.2300, 1.4800,
+ 4.8476 - np.pi + 0.13603681398218053 * 2
+ ],
+ [
+ 23.4630, -25.2382, -1.7361, 1.5600, 3.4800, 1.4000,
+ 4.9676 - np.pi + 0.13603681398218053 * 2
+ ],
+ [
+ 29.9235, -12.3342, -1.6218, 1.7400, 3.7700, 1.4800,
+ 0.4876 - np.pi + 0.13603681398218053 * 2
+ ]])
+ points, rot_mat_T = boxes.rotate(-0.13603681398218053, points)
+ expected_points = torch.tensor([[-1.1526, 0.8403, -1.4658],
+ [-4.6181, 1.5187, -1.3857],
+ [-6.6775, 0.6600, -0.9697],
+ [-0.5724, 0.6355, -0.5265],
+ [-4.6173, 0.0912, -1.4741]])
+ expected_rot_mat_T = torch.tensor([[0.9908, -0.1356, 0.0000],
+ [0.1356, 0.9908, 0.0000],
+ [0.0000, 0.0000, 1.0000]])
+ assert torch.allclose(boxes.tensor, expected_tensor, 1e-3)
+ assert torch.allclose(points, expected_points, 1e-3)
+ assert torch.allclose(rot_mat_T, expected_rot_mat_T, 1e-3)
+
+ # with input torch.Tensor points and rotation matrix
+ points, rot_mat_T = boxes.rotate(0.13603681398218053, points) # back
+ rot_mat = np.array([[0.99076125, -0.13561762, 0.],
+ [0.13561762, 0.99076125, 0.], [0., 0., 1.]])
+ points, rot_mat_T = boxes.rotate(rot_mat, points)
+ assert torch.allclose(boxes.tensor, expected_tensor, 1e-3)
+ assert torch.allclose(points, expected_points, 1e-3)
+ assert torch.allclose(rot_mat_T, expected_rot_mat_T, 1e-3)
+
+ # with input np.ndarray points and angle
+ points_np = np.array([[-1.0280, 0.9888,
+ -1.4658], [-4.3695, 2.1310, -1.3857],
+ [-6.5263, 1.5595,
+ -0.9697], [-0.4809, 0.7073, -0.5265],
+ [-4.5623, 0.7166, -1.4741]])
+ points_np, rot_mat_T_np = boxes.rotate(-0.13603681398218053, points_np)
+ expected_points_np = np.array([[-0.8844, 1.1191, -1.4658],
+ [-4.0401, 2.7039, -1.3857],
+ [-6.2545, 2.4302, -0.9697],
+ [-0.3805, 0.7660, -0.5265],
+ [-4.4230, 1.3287, -1.4741]])
+ expected_rot_mat_T_np = np.array([[0.9908, -0.1356, 0.0000],
+ [0.1356, 0.9908, 0.0000],
+ [0.0000, 0.0000, 1.0000]])
+
+ assert np.allclose(points_np, expected_points_np, 1e-3)
+ assert np.allclose(rot_mat_T_np, expected_rot_mat_T_np, 1e-3)
+
+ # with input LiDARPoints and rotation matrix
+ points_np, rot_mat_T_np = boxes.rotate(0.13603681398218053, points_np)
+ lidar_points = LiDARPoints(points_np)
+ lidar_points, rot_mat_T_np = boxes.rotate(rot_mat, lidar_points)
+ points_np = lidar_points.tensor.numpy()
+
+ assert np.allclose(points_np, expected_points_np, 1e-3)
+ assert np.allclose(rot_mat_T_np, expected_rot_mat_T_np, 1e-3)
+
+ # test box scaling
+ expected_tensor = torch.tensor([[
+ 1.0443488, -2.9183323, -1.7599131, 1.7597977, 3.4089797, 1.6592377,
+ 1.9336663 - np.pi
+ ],
+ [
+ 8.014273, -4.8007393, -1.6448704,
+ 1.5486219, 4.0324507, 1.57879,
+ 1.7936664 - np.pi
+ ],
+ [
+ 27.558605, -7.1084175, -1.310622,
+ 1.4782301, 2.242485, 1.488286,
+ 4.9836664 - np.pi
+ ],
+ [
+ 19.934517, -28.344835, -1.7457767,
+ 1.5687338, 3.4994833, 1.4078381,
+ 5.1036663 - np.pi
+ ],
+ [
+ 28.130915, -16.369587, -1.6308585,
+ 1.7497417, 3.791107, 1.488286,
+ 0.6236664 - np.pi
+ ]])
+ boxes.scale(1.00559866335275)
+ assert torch.allclose(boxes.tensor, expected_tensor)
+
+ # test box translation
+ expected_tensor = torch.tensor([[
+ 1.1281544, -3.0507944, -1.9169292, 1.7597977, 3.4089797, 1.6592377,
+ 1.9336663 - np.pi
+ ],
+ [
+ 8.098079, -4.9332013, -1.8018866,
+ 1.5486219, 4.0324507, 1.57879,
+ 1.7936664 - np.pi
+ ],
+ [
+ 27.64241, -7.2408795, -1.4676381,
+ 1.4782301, 2.242485, 1.488286,
+ 4.9836664 - np.pi
+ ],
+ [
+ 20.018322, -28.477297, -1.9027928,
+ 1.5687338, 3.4994833, 1.4078381,
+ 5.1036663 - np.pi
+ ],
+ [
+ 28.21472, -16.502048, -1.7878747,
+ 1.7497417, 3.791107, 1.488286,
+ 0.6236664 - np.pi
+ ]])
+ boxes.translate([0.0838056, -0.13246193, -0.15701613])
+ assert torch.allclose(boxes.tensor, expected_tensor)
+
+ # test bbox in_range_bev
+ expected_tensor = torch.tensor(
+ [[1.1282, -3.0508, 1.7598, 3.4090, -1.2079],
+ [8.0981, -4.9332, 1.5486, 4.0325, -1.3479],
+ [27.6424, -7.2409, 1.4782, 2.2425, 1.8421],
+ [20.0183, -28.4773, 1.5687, 3.4995, 1.9621],
+ [28.2147, -16.5020, 1.7497, 3.7911, -2.5179]])
+ assert torch.allclose(boxes.bev, expected_tensor, atol=1e-3)
+ expected_tensor = torch.tensor([1, 1, 1, 1, 1], dtype=torch.bool)
+ mask = boxes.in_range_bev([0., -40., 70.4, 40.])
+ assert (mask == expected_tensor).all()
+ mask = boxes.nonempty()
+ assert (mask == expected_tensor).all()
+
+ # test bbox in_range
+ expected_tensor = torch.tensor([1, 1, 0, 0, 0], dtype=torch.bool)
+ mask = boxes.in_range_3d([0, -20, -2, 22, 2, 5])
+ assert (mask == expected_tensor).all()
+
+ # test bbox indexing
+ index_boxes = boxes[2:5]
+ expected_tensor = torch.tensor([[
+ 27.64241, -7.2408795, -1.4676381, 1.4782301, 2.242485, 1.488286,
+ 4.9836664 - np.pi
+ ],
+ [
+ 20.018322, -28.477297, -1.9027928,
+ 1.5687338, 3.4994833, 1.4078381,
+ 5.1036663 - np.pi
+ ],
+ [
+ 28.21472, -16.502048, -1.7878747,
+ 1.7497417, 3.791107, 1.488286,
+ 0.6236664 - np.pi
+ ]])
+ assert len(index_boxes) == 3
+ assert torch.allclose(index_boxes.tensor, expected_tensor)
+
+ index_boxes = boxes[2]
+ expected_tensor = torch.tensor([[
+ 27.64241, -7.2408795, -1.4676381, 1.4782301, 2.242485, 1.488286,
+ 4.9836664 - np.pi
+ ]])
+ assert len(index_boxes) == 1
+ assert torch.allclose(index_boxes.tensor, expected_tensor)
+
+ index_boxes = boxes[[2, 4]]
+ expected_tensor = torch.tensor([[
+ 27.64241, -7.2408795, -1.4676381, 1.4782301, 2.242485, 1.488286,
+ 4.9836664 - np.pi
+ ],
+ [
+ 28.21472, -16.502048, -1.7878747,
+ 1.7497417, 3.791107, 1.488286,
+ 0.6236664 - np.pi
+ ]])
+ assert len(index_boxes) == 2
+ assert torch.allclose(index_boxes.tensor, expected_tensor)
+
+ # test iteration
+ for i, box in enumerate(index_boxes):
+ torch.allclose(box, expected_tensor[i])
+
+ # test properties
+ assert torch.allclose(boxes.bottom_center, boxes.tensor[:, :3])
+ expected_tensor = (
+ boxes.tensor[:, :3] - boxes.tensor[:, 3:6] *
+ (torch.tensor([0.5, 0.5, 0]) - torch.tensor([0.5, 0.5, 0.5])))
+ assert torch.allclose(boxes.gravity_center, expected_tensor)
+
+ boxes.limit_yaw()
+ assert (boxes.tensor[:, 6] <= np.pi / 2).all()
+ assert (boxes.tensor[:, 6] >= -np.pi / 2).all()
+
+ Box3DMode.convert(boxes, Box3DMode.LIDAR, Box3DMode.LIDAR)
+ expected_tensor = boxes.tensor.clone()
+ assert torch.allclose(expected_tensor, boxes.tensor)
+
+ boxes.flip()
+ boxes.flip()
+ boxes.limit_yaw()
+ assert torch.allclose(expected_tensor, boxes.tensor)
+
+ # test nearest_bev
+ expected_tensor = torch.tensor([[-0.5763, -3.9307, 2.8326, -2.1709],
+ [6.0819, -5.7075, 10.1143, -4.1589],
+ [26.5212, -7.9800, 28.7637, -6.5018],
+ [18.2686, -29.2617, 21.7681, -27.6929],
+ [27.3398, -18.3976, 29.0896, -14.6065]])
+ assert torch.allclose(
+ boxes.nearest_bev, expected_tensor, rtol=1e-4, atol=1e-7)
+
+ expected_tensor = torch.tensor([[[-7.7767e-01, -2.8332e+00, -1.9169e+00],
+ [-7.7767e-01, -2.8332e+00, -2.5769e-01],
+ [2.4093e+00, -1.6232e+00, -2.5769e-01],
+ [2.4093e+00, -1.6232e+00, -1.9169e+00],
+ [-1.5301e-01, -4.4784e+00, -1.9169e+00],
+ [-1.5301e-01, -4.4784e+00, -2.5769e-01],
+ [3.0340e+00, -3.2684e+00, -2.5769e-01],
+ [3.0340e+00, -3.2684e+00, -1.9169e+00]],
+ [[5.9606e+00, -4.6237e+00, -1.8019e+00],
+ [5.9606e+00, -4.6237e+00, -2.2310e-01],
+ [9.8933e+00, -3.7324e+00, -2.2310e-01],
+ [9.8933e+00, -3.7324e+00, -1.8019e+00],
+ [6.3029e+00, -6.1340e+00, -1.8019e+00],
+ [6.3029e+00, -6.1340e+00, -2.2310e-01],
+ [1.0236e+01, -5.2427e+00, -2.2310e-01],
+ [1.0236e+01, -5.2427e+00, -1.8019e+00]],
+ [[2.6364e+01, -6.8292e+00, -1.4676e+00],
+ [2.6364e+01, -6.8292e+00, 2.0648e-02],
+ [2.8525e+01, -6.2283e+00, 2.0648e-02],
+ [2.8525e+01, -6.2283e+00, -1.4676e+00],
+ [2.6760e+01, -8.2534e+00, -1.4676e+00],
+ [2.6760e+01, -8.2534e+00, 2.0648e-02],
+ [2.8921e+01, -7.6525e+00, 2.0648e-02],
+ [2.8921e+01, -7.6525e+00, -1.4676e+00]],
+ [[1.8102e+01, -2.8420e+01, -1.9028e+00],
+ [1.8102e+01, -2.8420e+01, -4.9495e-01],
+ [2.1337e+01, -2.7085e+01, -4.9495e-01],
+ [2.1337e+01, -2.7085e+01, -1.9028e+00],
+ [1.8700e+01, -2.9870e+01, -1.9028e+00],
+ [1.8700e+01, -2.9870e+01, -4.9495e-01],
+ [2.1935e+01, -2.8535e+01, -4.9495e-01],
+ [2.1935e+01, -2.8535e+01, -1.9028e+00]],
+ [[2.8612e+01, -1.8552e+01, -1.7879e+00],
+ [2.8612e+01, -1.8552e+01, -2.9959e-01],
+ [2.6398e+01, -1.5474e+01, -2.9959e-01],
+ [2.6398e+01, -1.5474e+01, -1.7879e+00],
+ [3.0032e+01, -1.7530e+01, -1.7879e+00],
+ [3.0032e+01, -1.7530e+01, -2.9959e-01],
+ [2.7818e+01, -1.4452e+01, -2.9959e-01],
+ [2.7818e+01, -1.4452e+01, -1.7879e+00]]])
+
+ assert torch.allclose(boxes.corners, expected_tensor, rtol=1e-4, atol=1e-7)
+
+ # test new_box
+ new_box1 = boxes.new_box([[1, 2, 3, 4, 5, 6, 7]])
+ assert torch.allclose(
+ new_box1.tensor,
+ torch.tensor([[1, 2, 3, 4, 5, 6, 7]], dtype=boxes.tensor.dtype))
+ assert new_box1.device == boxes.device
+ assert new_box1.with_yaw == boxes.with_yaw
+ assert new_box1.box_dim == boxes.box_dim
+
+ new_box2 = boxes.new_box(np.array([[1, 2, 3, 4, 5, 6, 7]]))
+ assert torch.allclose(
+ new_box2.tensor,
+ torch.tensor([[1, 2, 3, 4, 5, 6, 7]], dtype=boxes.tensor.dtype))
+
+ new_box3 = boxes.new_box(torch.tensor([[1, 2, 3, 4, 5, 6, 7]]))
+ assert torch.allclose(
+ new_box3.tensor,
+ torch.tensor([[1, 2, 3, 4, 5, 6, 7]], dtype=boxes.tensor.dtype))
+
+
+def test_boxes_conversion():
+ """Test the conversion of boxes between different modes.
+
+ ComandLine:
+ xdoctest tests/test_box3d.py::test_boxes_conversion zero
+ """
+ lidar_boxes = LiDARInstance3DBoxes(
+ [[1.7802081, 2.516249, -1.7501148, 1.75, 3.39, 1.65, 1.48],
+ [8.959413, 2.4567227, -1.6357126, 1.54, 4.01, 1.57, 1.62],
+ [28.2967, -0.5557558, -1.303325, 1.47, 2.23, 1.48, -1.57],
+ [26.66902, 21.82302, -1.736057, 1.56, 3.48, 1.4, -1.69],
+ [31.31978, 8.162144, -1.6217787, 1.74, 3.77, 1.48, 2.79]])
+ cam_box_tensor = Box3DMode.convert(lidar_boxes.tensor, Box3DMode.LIDAR,
+ Box3DMode.CAM)
+ expected_box = lidar_boxes.convert_to(Box3DMode.CAM)
+ assert torch.equal(expected_box.tensor, cam_box_tensor)
+
+ # Some properties should be the same
+ cam_boxes = CameraInstance3DBoxes(cam_box_tensor)
+ assert torch.equal(cam_boxes.height, lidar_boxes.height)
+ assert torch.equal(cam_boxes.top_height, -lidar_boxes.top_height)
+ assert torch.equal(cam_boxes.bottom_height, -lidar_boxes.bottom_height)
+ assert torch.allclose(cam_boxes.volume, lidar_boxes.volume)
+
+ lidar_box_tensor = Box3DMode.convert(cam_box_tensor, Box3DMode.CAM,
+ Box3DMode.LIDAR)
+ expected_tensor = torch.tensor(
+ [[1.7802081, 2.516249, -1.7501148, 1.75, 3.39, 1.65, 1.48],
+ [8.959413, 2.4567227, -1.6357126, 1.54, 4.01, 1.57, 1.62],
+ [28.2967, -0.5557558, -1.303325, 1.47, 2.23, 1.48, -1.57],
+ [26.66902, 21.82302, -1.736057, 1.56, 3.48, 1.4, -1.69],
+ [31.31978, 8.162144, -1.6217787, 1.74, 3.77, 1.48, 2.79]])
+
+ assert torch.allclose(expected_tensor, lidar_box_tensor)
+ assert torch.allclose(lidar_boxes.tensor, lidar_box_tensor)
+
+ depth_box_tensor = Box3DMode.convert(cam_box_tensor, Box3DMode.CAM,
+ Box3DMode.DEPTH)
+ depth_to_cam_box_tensor = Box3DMode.convert(depth_box_tensor,
+ Box3DMode.DEPTH, Box3DMode.CAM)
+ assert torch.allclose(cam_box_tensor, depth_to_cam_box_tensor)
+
+ # test similar mode conversion
+ same_results = Box3DMode.convert(depth_box_tensor, Box3DMode.DEPTH,
+ Box3DMode.DEPTH)
+ assert torch.equal(same_results, depth_box_tensor)
+
+ # test conversion with a given rt_mat
+ camera_boxes = CameraInstance3DBoxes(
+ [[0.06, 1.77, 21.4, 3.2, 1.61, 1.66, -1.54],
+ [6.59, 1.53, 6.76, 12.78, 3.66, 2.28, 1.55],
+ [6.71, 1.59, 22.18, 14.73, 3.64, 2.32, 1.59],
+ [7.11, 1.58, 34.54, 10.04, 3.61, 2.32, 1.61],
+ [7.78, 1.65, 45.95, 12.83, 3.63, 2.34, 1.64]])
+
+ rect = torch.tensor(
+ [[0.9999239, 0.00983776, -0.00744505, 0.],
+ [-0.0098698, 0.9999421, -0.00427846, 0.],
+ [0.00740253, 0.00435161, 0.9999631, 0.], [0., 0., 0., 1.]],
+ dtype=torch.float32)
+
+ Trv2c = torch.tensor(
+ [[7.533745e-03, -9.999714e-01, -6.166020e-04, -4.069766e-03],
+ [1.480249e-02, 7.280733e-04, -9.998902e-01, -7.631618e-02],
+ [9.998621e-01, 7.523790e-03, 1.480755e-02, -2.717806e-01],
+ [0.000000e+00, 0.000000e+00, 0.000000e+00, 1.000000e+00]],
+ dtype=torch.float32)
+
+ # coord sys refactor (reverse sign of yaw)
+ expected_tensor = torch.tensor(
+ [[
+ 2.16902434e+01, -4.06038554e-02, -1.61906639e+00, 3.20000005e+00,
+ 1.65999997e+00, 1.61000001e+00, 1.53999996e+00 - np.pi / 2
+ ],
+ [
+ 7.05006905e+00, -6.57459601e+00, -1.60107949e+00, 1.27799997e+01,
+ 2.27999997e+00, 3.66000009e+00, -1.54999995e+00 - np.pi / 2
+ ],
+ [
+ 2.24698818e+01, -6.69203759e+00, -1.50118145e+00, 1.47299995e+01,
+ 2.31999993e+00, 3.64000010e+00, -1.59000003e+00 + 3 * np.pi / 2
+ ],
+ [
+ 3.48291965e+01, -7.09058388e+00, -1.36622983e+00, 1.00400000e+01,
+ 2.31999993e+00, 3.60999990e+00, -1.61000001e+00 + 3 * np.pi / 2
+ ],
+ [
+ 4.62394617e+01, -7.75838800e+00, -1.32405020e+00, 1.28299999e+01,
+ 2.33999991e+00, 3.63000011e+00, -1.63999999e+00 + 3 * np.pi / 2
+ ]],
+ dtype=torch.float32)
+
+ rt_mat = rect @ Trv2c
+ # test conversion with Box type
+ cam_to_lidar_box = Box3DMode.convert(camera_boxes, Box3DMode.CAM,
+ Box3DMode.LIDAR, rt_mat.inverse())
+ assert torch.allclose(cam_to_lidar_box.tensor, expected_tensor)
+
+ lidar_to_cam_box = Box3DMode.convert(cam_to_lidar_box.tensor,
+ Box3DMode.LIDAR, Box3DMode.CAM,
+ rt_mat)
+ assert torch.allclose(lidar_to_cam_box, camera_boxes.tensor)
+
+ # test numpy convert
+ cam_to_lidar_box = Box3DMode.convert(camera_boxes.tensor.numpy(),
+ Box3DMode.CAM, Box3DMode.LIDAR,
+ rt_mat.inverse().numpy())
+ assert np.allclose(cam_to_lidar_box, expected_tensor.numpy())
+
+ # test list convert
+ cam_to_lidar_box = Box3DMode.convert(
+ camera_boxes.tensor[0].numpy().tolist(), Box3DMode.CAM,
+ Box3DMode.LIDAR,
+ rt_mat.inverse().numpy())
+ assert np.allclose(np.array(cam_to_lidar_box), expected_tensor[0].numpy())
+
+ # test convert from depth to lidar
+ depth_boxes = torch.tensor(
+ [[2.4593, 2.5870, -0.4321, 0.8597, 0.6193, 1.0204, 3.0693],
+ [1.4856, 2.5299, -0.5570, 0.9385, 2.1404, 0.8954, 3.0601]],
+ dtype=torch.float32)
+ depth_boxes = DepthInstance3DBoxes(depth_boxes)
+ depth_to_lidar_box = depth_boxes.convert_to(Box3DMode.LIDAR)
+ expected_box = depth_to_lidar_box.convert_to(Box3DMode.DEPTH)
+ assert torch.equal(depth_boxes.tensor, expected_box.tensor)
+
+ lidar_to_depth_box = Box3DMode.convert(depth_to_lidar_box, Box3DMode.LIDAR,
+ Box3DMode.DEPTH)
+ assert torch.allclose(depth_boxes.tensor, lidar_to_depth_box.tensor)
+ assert torch.allclose(depth_boxes.volume, lidar_to_depth_box.volume)
+
+ # test convert from depth to camera
+ depth_to_cam_box = Box3DMode.convert(depth_boxes, Box3DMode.DEPTH,
+ Box3DMode.CAM)
+ cam_to_depth_box = Box3DMode.convert(depth_to_cam_box, Box3DMode.CAM,
+ Box3DMode.DEPTH)
+ expected_tensor = depth_to_cam_box.convert_to(Box3DMode.DEPTH)
+ assert torch.equal(expected_tensor.tensor, cam_to_depth_box.tensor)
+ assert torch.allclose(depth_boxes.tensor, cam_to_depth_box.tensor)
+ assert torch.allclose(depth_boxes.volume, cam_to_depth_box.volume)
+
+ with pytest.raises(NotImplementedError):
+ # assert invalid convert mode
+ Box3DMode.convert(depth_boxes, Box3DMode.DEPTH, 3)
+
+
+def test_camera_boxes3d():
+ # Test init with numpy array
+ np_boxes = np.array([[
+ 1.7802081, 2.516249, -1.7501148, 1.75, 3.39, 1.65,
+ 1.48 - 0.13603681398218053 * 4 - 2 * np.pi
+ ],
+ [
+ 8.959413, 2.4567227, -1.6357126, 1.54, 4.01, 1.57,
+ 1.62 - 0.13603681398218053 * 4 - 2 * np.pi
+ ]],
+ dtype=np.float32)
+
+ boxes_1 = Box3DMode.convert(
+ LiDARInstance3DBoxes(np_boxes), Box3DMode.LIDAR, Box3DMode.CAM)
+ assert isinstance(boxes_1, CameraInstance3DBoxes)
+
+ cam_np_boxes = Box3DMode.convert(np_boxes, Box3DMode.LIDAR, Box3DMode.CAM)
+ assert torch.allclose(boxes_1.tensor,
+ boxes_1.tensor.new_tensor(cam_np_boxes))
+
+ # test init with torch.Tensor
+ th_boxes = torch.tensor(
+ [[
+ 28.29669987, -0.5557558, -1.30332506, 1.47000003, 2.23000002,
+ 1.48000002, -1.57000005 - 0.13603681398218053 * 4 - 2 * np.pi
+ ],
+ [
+ 26.66901946, 21.82302134, -1.73605708, 1.55999994, 3.48000002,
+ 1.39999998, -1.69000006 - 0.13603681398218053 * 4 - 2 * np.pi
+ ],
+ [
+ 31.31977974, 8.16214412, -1.62177875, 1.74000001, 3.76999998,
+ 1.48000002, 2.78999996 - 0.13603681398218053 * 4 - 2 * np.pi
+ ]],
+ dtype=torch.float32)
+ cam_th_boxes = Box3DMode.convert(th_boxes, Box3DMode.LIDAR, Box3DMode.CAM)
+ boxes_2 = CameraInstance3DBoxes(cam_th_boxes)
+ assert torch.allclose(boxes_2.tensor, cam_th_boxes)
+
+ # test clone/to/device
+ boxes_2 = boxes_2.clone()
+ boxes_1 = boxes_1.to(boxes_2.device)
+
+ # test box concatenation
+ expected_tensor = Box3DMode.convert(
+ torch.tensor([[
+ 1.7802081, 2.516249, -1.7501148, 1.75, 3.39, 1.65,
+ 1.48 - 0.13603681398218053 * 4 - 2 * np.pi
+ ],
+ [
+ 8.959413, 2.4567227, -1.6357126, 1.54, 4.01, 1.57,
+ 1.62 - 0.13603681398218053 * 4 - 2 * np.pi
+ ],
+ [
+ 28.2967, -0.5557558, -1.303325, 1.47, 2.23, 1.48,
+ -1.57 - 0.13603681398218053 * 4 - 2 * np.pi
+ ],
+ [
+ 26.66902, 21.82302, -1.736057, 1.56, 3.48, 1.4,
+ -1.69 - 0.13603681398218053 * 4 - 2 * np.pi
+ ],
+ [
+ 31.31978, 8.162144, -1.6217787, 1.74, 3.77, 1.48,
+ 2.79 - 0.13603681398218053 * 4 - 2 * np.pi
+ ]]), Box3DMode.LIDAR, Box3DMode.CAM)
+ boxes = CameraInstance3DBoxes.cat([boxes_1, boxes_2])
+ assert torch.allclose(boxes.tensor, expected_tensor)
+
+ # test box flip
+ points = torch.tensor([[0.6762, 1.4658, 1.2559], [0.8784, 1.3857, 4.7814],
+ [-0.2517, 0.9697, 6.7053], [0.5520, 0.5265, 0.6533],
+ [-0.5358, 1.4741, 4.5870]])
+ expected_tensor = Box3DMode.convert(
+ torch.tensor([[
+ 1.7802081, -2.516249, -1.7501148, 1.75, 3.39, 1.65,
+ 1.6615927 + 0.13603681398218053 * 4 - np.pi
+ ],
+ [
+ 8.959413, -2.4567227, -1.6357126, 1.54, 4.01, 1.57,
+ 1.5215927 + 0.13603681398218053 * 4 - np.pi
+ ],
+ [
+ 28.2967, 0.5557558, -1.303325, 1.47, 2.23, 1.48,
+ 4.7115927 + 0.13603681398218053 * 4 - np.pi
+ ],
+ [
+ 26.66902, -21.82302, -1.736057, 1.56, 3.48, 1.4,
+ 4.8315926 + 0.13603681398218053 * 4 - np.pi
+ ],
+ [
+ 31.31978, -8.162144, -1.6217787, 1.74, 3.77, 1.48,
+ 0.35159278 + 0.13603681398218053 * 4 - np.pi
+ ]]), Box3DMode.LIDAR, Box3DMode.CAM)
+ points = boxes.flip('horizontal', points)
+ expected_points = torch.tensor([[-0.6762, 1.4658, 1.2559],
+ [-0.8784, 1.3857, 4.7814],
+ [0.2517, 0.9697, 6.7053],
+ [-0.5520, 0.5265, 0.6533],
+ [0.5358, 1.4741, 4.5870]])
+
+ yaw_normalized_tensor = boxes.tensor.clone()
+ yaw_normalized_tensor[:, -1:] = limit_period(
+ yaw_normalized_tensor[:, -1:], period=np.pi * 2)
+ assert torch.allclose(yaw_normalized_tensor, expected_tensor, 1e-3)
+ assert torch.allclose(points, expected_points, 1e-3)
+
+ expected_tensor = torch.tensor(
+ [[
+ 2.5162, 1.7501, -1.7802, 1.7500, 1.6500, 3.3900,
+ 1.6616 + 0.13603681398218053 * 4 - np.pi / 2
+ ],
+ [
+ 2.4567, 1.6357, -8.9594, 1.5400, 1.5700, 4.0100,
+ 1.5216 + 0.13603681398218053 * 4 - np.pi / 2
+ ],
+ [
+ -0.5558, 1.3033, -28.2967, 1.4700, 1.4800, 2.2300,
+ 4.7116 + 0.13603681398218053 * 4 - np.pi / 2
+ ],
+ [
+ 21.8230, 1.7361, -26.6690, 1.5600, 1.4000, 3.4800,
+ 4.8316 + 0.13603681398218053 * 4 - np.pi / 2
+ ],
+ [
+ 8.1621, 1.6218, -31.3198, 1.7400, 1.4800, 3.7700,
+ 0.3516 + 0.13603681398218053 * 4 - np.pi / 2
+ ]])
+ boxes_flip_vert = boxes.clone()
+ points = boxes_flip_vert.flip('vertical', points)
+ expected_points = torch.tensor([[-0.6762, 1.4658, -1.2559],
+ [-0.8784, 1.3857, -4.7814],
+ [0.2517, 0.9697, -6.7053],
+ [-0.5520, 0.5265, -0.6533],
+ [0.5358, 1.4741, -4.5870]])
+
+ yaw_normalized_tensor = boxes_flip_vert.tensor.clone()
+ yaw_normalized_tensor[:, -1:] = limit_period(
+ yaw_normalized_tensor[:, -1:], period=np.pi * 2)
+ expected_tensor[:, -1:] = limit_period(
+ expected_tensor[:, -1:], period=np.pi * 2)
+ assert torch.allclose(yaw_normalized_tensor, expected_tensor, 1e-4)
+ assert torch.allclose(points, expected_points)
+
+ # test box rotation
+ # with input torch.Tensor points and angle
+ expected_tensor = Box3DMode.convert(
+ torch.tensor([[
+ 1.4225, -2.7344, -1.7501, 1.7500, 3.3900, 1.6500,
+ 1.7976 + 0.13603681398218053 * 2 - np.pi
+ ],
+ [
+ 8.5435, -3.6491, -1.6357, 1.5400, 4.0100, 1.5700,
+ 1.6576 + 0.13603681398218053 * 2 - np.pi
+ ],
+ [
+ 28.1106, -3.2869, -1.3033, 1.4700, 2.2300, 1.4800,
+ 4.8476 + 0.13603681398218053 * 2 - np.pi
+ ],
+ [
+ 23.4630, -25.2382, -1.7361, 1.5600, 3.4800, 1.4000,
+ 4.9676 + 0.13603681398218053 * 2 - np.pi
+ ],
+ [
+ 29.9235, -12.3342, -1.6218, 1.7400, 3.7700, 1.4800,
+ 0.4876 + 0.13603681398218053 * 2 - np.pi
+ ]]), Box3DMode.LIDAR, Box3DMode.CAM)
+ points, rot_mat_T = boxes.rotate(torch.tensor(0.13603681398218053), points)
+ expected_points = torch.tensor([[-0.8403, 1.4658, -1.1526],
+ [-1.5187, 1.3857, -4.6181],
+ [-0.6600, 0.9697, -6.6775],
+ [-0.6355, 0.5265, -0.5724],
+ [-0.0912, 1.4741, -4.6173]])
+ expected_rot_mat_T = torch.tensor([[0.9908, 0.0000, -0.1356],
+ [0.0000, 1.0000, 0.0000],
+ [0.1356, 0.0000, 0.9908]])
+ yaw_normalized_tensor = boxes.tensor.clone()
+ yaw_normalized_tensor[:, -1:] = limit_period(
+ yaw_normalized_tensor[:, -1:], period=np.pi * 2)
+ expected_tensor[:, -1:] = limit_period(
+ expected_tensor[:, -1:], period=np.pi * 2)
+ assert torch.allclose(yaw_normalized_tensor, expected_tensor, 1e-3)
+ assert torch.allclose(points, expected_points, 1e-3)
+ assert torch.allclose(rot_mat_T, expected_rot_mat_T, 1e-3)
+
+ # with input torch.Tensor points and rotation matrix
+ points, rot_mat_T = boxes.rotate(
+ torch.tensor(-0.13603681398218053), points) # back
+ rot_mat = np.array([[0.99076125, 0., -0.13561762], [0., 1., 0.],
+ [0.13561762, 0., 0.99076125]])
+ points, rot_mat_T = boxes.rotate(rot_mat, points)
+ yaw_normalized_tensor = boxes.tensor.clone()
+ yaw_normalized_tensor[:, -1:] = limit_period(
+ yaw_normalized_tensor[:, -1:], period=np.pi * 2)
+ assert torch.allclose(yaw_normalized_tensor, expected_tensor, 1e-3)
+ assert torch.allclose(points, expected_points, 1e-3)
+ assert torch.allclose(rot_mat_T, expected_rot_mat_T, 1e-3)
+
+ # with input np.ndarray points and angle
+ points_np = np.array([[0.6762, 1.2559, -1.4658, 2.5359],
+ [0.8784, 4.7814, -1.3857, 0.7167],
+ [-0.2517, 6.7053, -0.9697, 0.5599],
+ [0.5520, 0.6533, -0.5265, 1.0032],
+ [-0.5358, 4.5870, -1.4741, 0.0556]])
+ points_np, rot_mat_T_np = boxes.rotate(
+ torch.tensor(0.13603681398218053), points_np)
+ expected_points_np = np.array([[0.4712, 1.2559, -1.5440, 2.5359],
+ [0.6824, 4.7814, -1.4920, 0.7167],
+ [-0.3809, 6.7053, -0.9266, 0.5599],
+ [0.4755, 0.6533, -0.5965, 1.0032],
+ [-0.7308, 4.5870, -1.3878, 0.0556]])
+ expected_rot_mat_T_np = np.array([[0.9908, 0.0000, -0.1356],
+ [0.0000, 1.0000, 0.0000],
+ [0.1356, 0.0000, 0.9908]])
+
+ assert np.allclose(points_np, expected_points_np, 1e-3)
+ assert np.allclose(rot_mat_T_np, expected_rot_mat_T_np, 1e-3)
+
+ # with input CameraPoints and rotation matrix
+ points_np, rot_mat_T_np = boxes.rotate(
+ torch.tensor(-0.13603681398218053), points_np)
+ camera_points = CameraPoints(points_np, points_dim=4)
+ camera_points, rot_mat_T_np = boxes.rotate(rot_mat, camera_points)
+ points_np = camera_points.tensor.numpy()
+ assert np.allclose(points_np, expected_points_np, 1e-3)
+ assert np.allclose(rot_mat_T_np, expected_rot_mat_T_np, 1e-3)
+
+ # test box scaling
+ expected_tensor = Box3DMode.convert(
+ torch.tensor([[
+ 1.0443488, -2.9183323, -1.7599131, 1.7597977, 3.4089797, 1.6592377,
+ 1.9336663 - np.pi
+ ],
+ [
+ 8.014273, -4.8007393, -1.6448704, 1.5486219,
+ 4.0324507, 1.57879, 1.7936664 - np.pi
+ ],
+ [
+ 27.558605, -7.1084175, -1.310622, 1.4782301,
+ 2.242485, 1.488286, 4.9836664 - np.pi
+ ],
+ [
+ 19.934517, -28.344835, -1.7457767, 1.5687338,
+ 3.4994833, 1.4078381, 5.1036663 - np.pi
+ ],
+ [
+ 28.130915, -16.369587, -1.6308585, 1.7497417,
+ 3.791107, 1.488286, 0.6236664 - np.pi
+ ]]), Box3DMode.LIDAR, Box3DMode.CAM)
+ boxes.scale(1.00559866335275)
+ yaw_normalized_tensor = boxes.tensor.clone()
+ yaw_normalized_tensor[:, -1:] = limit_period(
+ yaw_normalized_tensor[:, -1:], period=np.pi * 2)
+ expected_tensor[:, -1:] = limit_period(
+ expected_tensor[:, -1:], period=np.pi * 2)
+ assert torch.allclose(yaw_normalized_tensor, expected_tensor)
+
+ # test box translation
+ expected_tensor = Box3DMode.convert(
+ torch.tensor([[
+ 1.1281544, -3.0507944, -1.9169292, 1.7597977, 3.4089797, 1.6592377,
+ 1.9336663 - np.pi
+ ],
+ [
+ 8.098079, -4.9332013, -1.8018866, 1.5486219,
+ 4.0324507, 1.57879, 1.7936664 - np.pi
+ ],
+ [
+ 27.64241, -7.2408795, -1.4676381, 1.4782301,
+ 2.242485, 1.488286, 4.9836664 - np.pi
+ ],
+ [
+ 20.018322, -28.477297, -1.9027928, 1.5687338,
+ 3.4994833, 1.4078381, 5.1036663 - np.pi
+ ],
+ [
+ 28.21472, -16.502048, -1.7878747, 1.7497417,
+ 3.791107, 1.488286, 0.6236664 - np.pi
+ ]]), Box3DMode.LIDAR, Box3DMode.CAM)
+ boxes.translate(torch.tensor([0.13246193, 0.15701613, 0.0838056]))
+ yaw_normalized_tensor = boxes.tensor.clone()
+ yaw_normalized_tensor[:, -1:] = limit_period(
+ yaw_normalized_tensor[:, -1:], period=np.pi * 2)
+ expected_tensor[:, -1:] = limit_period(
+ expected_tensor[:, -1:], period=np.pi * 2)
+ assert torch.allclose(yaw_normalized_tensor, expected_tensor)
+
+ # test bbox in_range_bev
+ expected_tensor = torch.tensor([1, 1, 1, 1, 1], dtype=torch.bool)
+ mask = boxes.in_range_bev([0., -40., 70.4, 40.])
+ assert (mask == expected_tensor).all()
+ mask = boxes.nonempty()
+ assert (mask == expected_tensor).all()
+
+ # test bbox in_range
+ expected_tensor = torch.tensor([1, 1, 0, 0, 0], dtype=torch.bool)
+ mask = boxes.in_range_3d([-2, -5, 0, 20, 2, 22])
+ assert (mask == expected_tensor).all()
+
+ expected_tensor = torch.tensor(
+ [[3.0508, 1.1282, 1.7598, 3.4090, -5.9203],
+ [4.9332, 8.0981, 1.5486, 4.0325, -6.0603],
+ [7.2409, 27.6424, 1.4782, 2.2425, -2.8703],
+ [28.4773, 20.0183, 1.5687, 3.4995, -2.7503],
+ [16.5020, 28.2147, 1.7497, 3.7911, -0.9471]])
+ assert torch.allclose(boxes.bev, expected_tensor, atol=1e-3)
+
+ # test properties
+ assert torch.allclose(boxes.bottom_center, boxes.tensor[:, :3])
+ expected_tensor = (
+ boxes.tensor[:, :3] - boxes.tensor[:, 3:6] *
+ (torch.tensor([0.5, 1.0, 0.5]) - torch.tensor([0.5, 0.5, 0.5])))
+ assert torch.allclose(boxes.gravity_center, expected_tensor)
+
+ boxes.limit_yaw()
+ assert (boxes.tensor[:, 6] <= np.pi / 2).all()
+ assert (boxes.tensor[:, 6] >= -np.pi / 2).all()
+
+ Box3DMode.convert(boxes, Box3DMode.LIDAR, Box3DMode.LIDAR)
+ expected_tensor = boxes.tensor.clone()
+ assert torch.allclose(expected_tensor, boxes.tensor)
+
+ boxes.flip()
+ boxes.flip()
+ boxes.limit_yaw()
+ assert torch.allclose(expected_tensor, boxes.tensor)
+
+ # test nearest_bev
+ # BEV box in lidar coordinates (x, y)
+ lidar_expected_tensor = torch.tensor(
+ [[-0.5763, -3.9307, 2.8326, -2.1709],
+ [6.0819, -5.7075, 10.1143, -4.1589],
+ [26.5212, -7.9800, 28.7637, -6.5018],
+ [18.2686, -29.2617, 21.7681, -27.6929],
+ [27.3398, -18.3976, 29.0896, -14.6065]])
+ # BEV box in camera coordinate (-y, x)
+ expected_tensor = lidar_expected_tensor.clone()
+ expected_tensor[:, 0::2] = -lidar_expected_tensor[:, [3, 1]]
+ expected_tensor[:, 1::2] = lidar_expected_tensor[:, 0::2]
+ assert torch.allclose(
+ boxes.nearest_bev, expected_tensor, rtol=1e-4, atol=1e-7)
+
+ expected_tensor = torch.tensor([[[2.8332e+00, 2.5769e-01, -7.7767e-01],
+ [1.6232e+00, 2.5769e-01, 2.4093e+00],
+ [1.6232e+00, 1.9169e+00, 2.4093e+00],
+ [2.8332e+00, 1.9169e+00, -7.7767e-01],
+ [4.4784e+00, 2.5769e-01, -1.5302e-01],
+ [3.2684e+00, 2.5769e-01, 3.0340e+00],
+ [3.2684e+00, 1.9169e+00, 3.0340e+00],
+ [4.4784e+00, 1.9169e+00, -1.5302e-01]],
+ [[4.6237e+00, 2.2310e-01, 5.9606e+00],
+ [3.7324e+00, 2.2310e-01, 9.8933e+00],
+ [3.7324e+00, 1.8019e+00, 9.8933e+00],
+ [4.6237e+00, 1.8019e+00, 5.9606e+00],
+ [6.1340e+00, 2.2310e-01, 6.3029e+00],
+ [5.2427e+00, 2.2310e-01, 1.0236e+01],
+ [5.2427e+00, 1.8019e+00, 1.0236e+01],
+ [6.1340e+00, 1.8019e+00, 6.3029e+00]],
+ [[6.8292e+00, -2.0648e-02, 2.6364e+01],
+ [6.2283e+00, -2.0648e-02, 2.8525e+01],
+ [6.2283e+00, 1.4676e+00, 2.8525e+01],
+ [6.8292e+00, 1.4676e+00, 2.6364e+01],
+ [8.2534e+00, -2.0648e-02, 2.6760e+01],
+ [7.6525e+00, -2.0648e-02, 2.8921e+01],
+ [7.6525e+00, 1.4676e+00, 2.8921e+01],
+ [8.2534e+00, 1.4676e+00, 2.6760e+01]],
+ [[2.8420e+01, 4.9495e-01, 1.8102e+01],
+ [2.7085e+01, 4.9495e-01, 2.1337e+01],
+ [2.7085e+01, 1.9028e+00, 2.1337e+01],
+ [2.8420e+01, 1.9028e+00, 1.8102e+01],
+ [2.9870e+01, 4.9495e-01, 1.8700e+01],
+ [2.8535e+01, 4.9495e-01, 2.1935e+01],
+ [2.8535e+01, 1.9028e+00, 2.1935e+01],
+ [2.9870e+01, 1.9028e+00, 1.8700e+01]],
+ [[1.4452e+01, 2.9959e-01, 2.7818e+01],
+ [1.7530e+01, 2.9959e-01, 3.0032e+01],
+ [1.7530e+01, 1.7879e+00, 3.0032e+01],
+ [1.4452e+01, 1.7879e+00, 2.7818e+01],
+ [1.5474e+01, 2.9959e-01, 2.6398e+01],
+ [1.8552e+01, 2.9959e-01, 2.8612e+01],
+ [1.8552e+01, 1.7879e+00, 2.8612e+01],
+ [1.5474e+01, 1.7879e+00, 2.6398e+01]]])
+
+ assert torch.allclose(boxes.corners, expected_tensor, rtol=1e-3, atol=1e-4)
+
+ th_boxes = torch.tensor(
+ [[
+ 28.29669987, -0.5557558, -1.30332506, 1.47000003, 2.23000002,
+ 1.48000002, -1.57000005
+ ],
+ [
+ 26.66901946, 21.82302134, -1.73605708, 1.55999994, 3.48000002,
+ 1.39999998, -1.69000006
+ ],
+ [
+ 31.31977974, 8.16214412, -1.62177875, 1.74000001, 3.76999998,
+ 1.48000002, 2.78999996
+ ]],
+ dtype=torch.float32)
+
+ # test init with a given origin
+ boxes_origin_given = CameraInstance3DBoxes(
+ th_boxes.clone(), box_dim=7, origin=(0.5, 0.5, 0.5))
+ expected_tensor = th_boxes.clone()
+ expected_tensor[:, :3] = th_boxes[:, :3] + th_boxes[:, 3:6] * (
+ th_boxes.new_tensor((0.5, 1.0, 0.5)) - th_boxes.new_tensor(
+ (0.5, 0.5, 0.5)))
+ assert torch.allclose(boxes_origin_given.tensor, expected_tensor)
+
+
+def test_boxes3d_overlaps():
+ """Test the iou calculation of boxes in different modes.
+
+ ComandLine:
+ xdoctest tests/test_box3d.py::test_boxes3d_overlaps zero
+ """
+ if not torch.cuda.is_available():
+ pytest.skip('test requires GPU and torch+cuda')
+
+ # Test LiDAR boxes 3D overlaps
+ boxes1_tensor = torch.tensor(
+ [[1.8, -2.5, -1.8, 1.75, 3.39, 1.65, -1.6615927],
+ [8.9, -2.5, -1.6, 1.54, 4.01, 1.57, -1.5215927],
+ [28.3, 0.5, -1.3, 1.47, 2.23, 1.48, -4.7115927],
+ [31.3, -8.2, -1.6, 1.74, 3.77, 1.48, -0.35]],
+ device='cuda')
+ boxes1 = LiDARInstance3DBoxes(boxes1_tensor)
+
+ boxes2_tensor = torch.tensor([[1.2, -3.0, -1.9, 1.8, 3.4, 1.7, -1.9],
+ [8.1, -2.9, -1.8, 1.5, 4.1, 1.6, -1.8],
+ [31.3, -8.2, -1.6, 1.74, 3.77, 1.48, -0.35],
+ [20.1, -28.5, -1.9, 1.6, 3.5, 1.4, -5.1]],
+ device='cuda')
+ boxes2 = LiDARInstance3DBoxes(boxes2_tensor)
+
+ expected_iou_tensor = torch.tensor(
+ [[0.3710, 0.0000, 0.0000, 0.0000], [0.0000, 0.3322, 0.0000, 0.0000],
+ [0.0000, 0.0000, 0.0000, 0.0000], [0.0000, 0.0000, 1.0000, 0.0000]],
+ device='cuda')
+ overlaps_3d_iou = boxes1.overlaps(boxes1, boxes2)
+ assert torch.allclose(
+ expected_iou_tensor, overlaps_3d_iou, rtol=1e-4, atol=1e-7)
+
+ expected_iof_tensor = torch.tensor(
+ [[0.5582, 0.0000, 0.0000, 0.0000], [0.0000, 0.5025, 0.0000, 0.0000],
+ [0.0000, 0.0000, 0.0000, 0.0000], [0.0000, 0.0000, 1.0000, 0.0000]],
+ device='cuda')
+ overlaps_3d_iof = boxes1.overlaps(boxes1, boxes2, mode='iof')
+ assert torch.allclose(
+ expected_iof_tensor, overlaps_3d_iof, rtol=1e-4, atol=1e-7)
+
+ empty_boxes = []
+ boxes3 = LiDARInstance3DBoxes(empty_boxes)
+ overlaps_3d_empty = boxes1.overlaps(boxes3, boxes2)
+ assert overlaps_3d_empty.shape[0] == 0
+ assert overlaps_3d_empty.shape[1] == 4
+ # Test camera boxes 3D overlaps
+ cam_boxes1_tensor = Box3DMode.convert(boxes1_tensor, Box3DMode.LIDAR,
+ Box3DMode.CAM)
+ cam_boxes1 = CameraInstance3DBoxes(cam_boxes1_tensor)
+
+ cam_boxes2_tensor = Box3DMode.convert(boxes2_tensor, Box3DMode.LIDAR,
+ Box3DMode.CAM)
+ cam_boxes2 = CameraInstance3DBoxes(cam_boxes2_tensor)
+ cam_overlaps_3d = cam_boxes1.overlaps(cam_boxes1, cam_boxes2)
+
+ # same boxes under different coordinates should have the same iou
+ assert torch.allclose(
+ expected_iou_tensor, cam_overlaps_3d, rtol=1e-3, atol=1e-4)
+ assert torch.allclose(
+ cam_overlaps_3d, overlaps_3d_iou, rtol=1e-3, atol=1e-4)
+
+ with pytest.raises(AssertionError):
+ cam_boxes1.overlaps(cam_boxes1, boxes1)
+ with pytest.raises(AssertionError):
+ boxes1.overlaps(cam_boxes1, boxes1)
+
+
+def test_depth_boxes3d():
+ # test empty initialization
+ empty_boxes = []
+ boxes = DepthInstance3DBoxes(empty_boxes)
+ assert boxes.tensor.shape[0] == 0
+ assert boxes.tensor.shape[1] == 7
+
+ # Test init with numpy array
+ np_boxes = np.array(
+ [[1.4856, 2.5299, -0.5570, 0.9385, 2.1404, 0.8954, 3.0601],
+ [2.3262, 3.3065, --0.44255, 0.8234, 0.5325, 1.0099, 2.9971]],
+ dtype=np.float32)
+ boxes_1 = DepthInstance3DBoxes(np_boxes)
+ assert torch.allclose(boxes_1.tensor, torch.from_numpy(np_boxes))
+
+ # test properties
+
+ assert boxes_1.volume.size(0) == 2
+ assert (boxes_1.center == boxes_1.bottom_center).all()
+ expected_tensor = torch.tensor([[1.4856, 2.5299, -0.1093],
+ [2.3262, 3.3065, 0.9475]])
+ assert torch.allclose(boxes_1.gravity_center, expected_tensor)
+ expected_tensor = torch.tensor([[1.4856, 2.5299, 0.9385, 2.1404, 3.0601],
+ [2.3262, 3.3065, 0.8234, 0.5325, 2.9971]])
+ assert torch.allclose(boxes_1.bev, expected_tensor)
+ expected_tensor = torch.tensor([[1.0164, 1.4597, 1.9548, 3.6001],
+ [1.9145, 3.0402, 2.7379, 3.5728]])
+ assert torch.allclose(boxes_1.nearest_bev, expected_tensor, 1e-4)
+ assert repr(boxes) == (
+ 'DepthInstance3DBoxes(\n tensor([], size=(0, 7)))')
+
+ # test init with torch.Tensor
+ th_boxes = torch.tensor(
+ [[2.4593, 2.5870, -0.4321, 0.8597, 0.6193, 1.0204, 3.0693],
+ [1.4856, 2.5299, -0.5570, 0.9385, 2.1404, 0.8954, 3.0601]],
+ dtype=torch.float32)
+ boxes_2 = DepthInstance3DBoxes(th_boxes)
+ assert torch.allclose(boxes_2.tensor, th_boxes)
+
+ # test clone/to/device
+ boxes_2 = boxes_2.clone()
+ boxes_1 = boxes_1.to(boxes_2.device)
+
+ # test box concatenation
+ expected_tensor = torch.tensor(
+ [[1.4856, 2.5299, -0.5570, 0.9385, 2.1404, 0.8954, 3.0601],
+ [2.3262, 3.3065, 0.44255, 0.8234, 0.5325, 1.0099, 2.9971],
+ [2.4593, 2.5870, -0.4321, 0.8597, 0.6193, 1.0204, 3.0693],
+ [1.4856, 2.5299, -0.5570, 0.9385, 2.1404, 0.8954, 3.0601]])
+ boxes = DepthInstance3DBoxes.cat([boxes_1, boxes_2])
+ assert torch.allclose(boxes.tensor, expected_tensor)
+ # concatenate empty list
+ empty_boxes = DepthInstance3DBoxes.cat([])
+ assert empty_boxes.tensor.shape[0] == 0
+ assert empty_boxes.tensor.shape[-1] == 7
+
+ # test box flip
+ points = torch.tensor([[0.6762, 1.2559, -1.4658, 2.5359],
+ [0.8784, 4.7814, -1.3857, 0.7167],
+ [-0.2517, 6.7053, -0.9697, 0.5599],
+ [0.5520, 0.6533, -0.5265, 1.0032],
+ [-0.5358, 4.5870, -1.4741, 0.0556]])
+ expected_tensor = torch.tensor(
+ [[-1.4856, 2.5299, -0.5570, 0.9385, 2.1404, 0.8954, 0.0815],
+ [-2.3262, 3.3065, 0.4426, 0.8234, 0.5325, 1.0099, 0.1445],
+ [-2.4593, 2.5870, -0.4321, 0.8597, 0.6193, 1.0204, 0.0723],
+ [-1.4856, 2.5299, -0.5570, 0.9385, 2.1404, 0.8954, 0.0815]])
+ points = boxes.flip(bev_direction='horizontal', points=points)
+ expected_points = torch.tensor([[-0.6762, 1.2559, -1.4658, 2.5359],
+ [-0.8784, 4.7814, -1.3857, 0.7167],
+ [0.2517, 6.7053, -0.9697, 0.5599],
+ [-0.5520, 0.6533, -0.5265, 1.0032],
+ [0.5358, 4.5870, -1.4741, 0.0556]])
+ assert torch.allclose(boxes.tensor, expected_tensor, 1e-3)
+ assert torch.allclose(points, expected_points)
+ expected_tensor = torch.tensor(
+ [[-1.4856, -2.5299, -0.5570, 0.9385, 2.1404, 0.8954, -0.0815],
+ [-2.3262, -3.3065, 0.4426, 0.8234, 0.5325, 1.0099, -0.1445],
+ [-2.4593, -2.5870, -0.4321, 0.8597, 0.6193, 1.0204, -0.0723],
+ [-1.4856, -2.5299, -0.5570, 0.9385, 2.1404, 0.8954, -0.0815]])
+ points = boxes.flip(bev_direction='vertical', points=points)
+ expected_points = torch.tensor([[-0.6762, -1.2559, -1.4658, 2.5359],
+ [-0.8784, -4.7814, -1.3857, 0.7167],
+ [0.2517, -6.7053, -0.9697, 0.5599],
+ [-0.5520, -0.6533, -0.5265, 1.0032],
+ [0.5358, -4.5870, -1.4741, 0.0556]])
+ assert torch.allclose(boxes.tensor, expected_tensor, 1e-3)
+ assert torch.allclose(points, expected_points)
+
+ # test box rotation
+ # with input torch.Tensor points and angle
+ boxes_rot = boxes.clone()
+ expected_tensor = torch.tensor(
+ [[-1.5434, -2.4951, -0.5570, 0.9385, 2.1404, 0.8954, -0.0585],
+ [-2.4016, -3.2521, 0.4426, 0.8234, 0.5325, 1.0099, -0.1215],
+ [-2.5181, -2.5298, -0.4321, 0.8597, 0.6193, 1.0204, -0.0493],
+ [-1.5434, -2.4951, -0.5570, 0.9385, 2.1404, 0.8954, -0.0585]])
+ expected_tensor[:, -1:] -= 0.022998953275003075 * 2
+ points, rot_mat_T = boxes_rot.rotate(-0.022998953275003075, points)
+ expected_points = torch.tensor([[-0.7049, -1.2400, -1.4658, 2.5359],
+ [-0.9881, -4.7599, -1.3857, 0.7167],
+ [0.0974, -6.7093, -0.9697, 0.5599],
+ [-0.5669, -0.6404, -0.5265, 1.0032],
+ [0.4302, -4.5981, -1.4741, 0.0556]])
+ expected_rot_mat_T = torch.tensor([[0.9997, -0.0230, 0.0000],
+ [0.0230, 0.9997, 0.0000],
+ [0.0000, 0.0000, 1.0000]])
+ assert torch.allclose(boxes_rot.tensor, expected_tensor, 1e-3)
+ assert torch.allclose(points, expected_points, 1e-3)
+ assert torch.allclose(rot_mat_T, expected_rot_mat_T, 1e-3)
+
+ # with input torch.Tensor points and rotation matrix
+ points, rot_mat_T = boxes.rotate(-0.022998953275003075, points) # back
+ rot_mat = np.array([[0.99973554, 0.02299693, 0.],
+ [-0.02299693, 0.99973554, 0.], [0., 0., 1.]])
+ points, rot_mat_T = boxes.rotate(rot_mat, points)
+ expected_rot_mat_T = torch.tensor([[0.99973554, 0.02299693, 0.0000],
+ [-0.02299693, 0.99973554, 0.0000],
+ [0.0000, 0.0000, 1.0000]])
+ assert torch.allclose(boxes_rot.tensor, expected_tensor, 1e-3)
+ assert torch.allclose(points, expected_points, 1e-3)
+ assert torch.allclose(rot_mat_T, expected_rot_mat_T, 1e-3)
+
+ # with input np.ndarray points and angle
+ points_np = np.array([[0.6762, 1.2559, -1.4658, 2.5359],
+ [0.8784, 4.7814, -1.3857, 0.7167],
+ [-0.2517, 6.7053, -0.9697, 0.5599],
+ [0.5520, 0.6533, -0.5265, 1.0032],
+ [-0.5358, 4.5870, -1.4741, 0.0556]])
+ points_np, rot_mat_T_np = boxes.rotate(-0.022998953275003075, points_np)
+ expected_points_np = np.array([[0.7049, 1.2400, -1.4658, 2.5359],
+ [0.9881, 4.7599, -1.3857, 0.7167],
+ [-0.0974, 6.7093, -0.9697, 0.5599],
+ [0.5669, 0.6404, -0.5265, 1.0032],
+ [-0.4302, 4.5981, -1.4741, 0.0556]])
+ expected_rot_mat_T_np = np.array([[0.99973554, -0.02299693, 0.0000],
+ [0.02299693, 0.99973554, 0.0000],
+ [0.0000, 0.0000, 1.0000]])
+ expected_tensor = torch.tensor(
+ [[-1.5434, -2.4951, -0.5570, 0.9385, 2.1404, 0.8954, -0.0585],
+ [-2.4016, -3.2521, 0.4426, 0.8234, 0.5325, 1.0099, -0.1215],
+ [-2.5181, -2.5298, -0.4321, 0.8597, 0.6193, 1.0204, -0.0493],
+ [-1.5434, -2.4951, -0.5570, 0.9385, 2.1404, 0.8954, -0.0585]])
+ expected_tensor[:, -1:] -= 0.022998953275003075 * 2
+ assert torch.allclose(boxes.tensor, expected_tensor, 1e-3)
+ assert np.allclose(points_np, expected_points_np, 1e-3)
+ assert np.allclose(rot_mat_T_np, expected_rot_mat_T_np, 1e-3)
+
+ # with input DepthPoints and rotation matrix
+ points_np, rot_mat_T_np = boxes.rotate(-0.022998953275003075, points_np)
+ depth_points = DepthPoints(points_np, points_dim=4)
+ depth_points, rot_mat_T_np = boxes.rotate(rot_mat, depth_points)
+ points_np = depth_points.tensor.numpy()
+ expected_rot_mat_T_np = expected_rot_mat_T_np.T
+ assert torch.allclose(boxes.tensor, expected_tensor, 1e-3)
+ assert np.allclose(points_np, expected_points_np, 1e-3)
+ assert np.allclose(rot_mat_T_np, expected_rot_mat_T_np, 1e-3)
+
+ expected_tensor = torch.tensor([[[-2.1217, -3.5105, -0.5570],
+ [-2.1217, -3.5105, 0.3384],
+ [-1.8985, -1.3818, 0.3384],
+ [-1.8985, -1.3818, -0.5570],
+ [-1.1883, -3.6084, -0.5570],
+ [-1.1883, -3.6084, 0.3384],
+ [-0.9651, -1.4796, 0.3384],
+ [-0.9651, -1.4796, -0.5570]],
+ [[-2.8519, -3.4460, 0.4426],
+ [-2.8519, -3.4460, 1.4525],
+ [-2.7632, -2.9210, 1.4525],
+ [-2.7632, -2.9210, 0.4426],
+ [-2.0401, -3.5833, 0.4426],
+ [-2.0401, -3.5833, 1.4525],
+ [-1.9513, -3.0582, 1.4525],
+ [-1.9513, -3.0582, 0.4426]],
+ [[-2.9755, -2.7971, -0.4321],
+ [-2.9755, -2.7971, 0.5883],
+ [-2.9166, -2.1806, 0.5883],
+ [-2.9166, -2.1806, -0.4321],
+ [-2.1197, -2.8789, -0.4321],
+ [-2.1197, -2.8789, 0.5883],
+ [-2.0608, -2.2624, 0.5883],
+ [-2.0608, -2.2624, -0.4321]],
+ [[-2.1217, -3.5105, -0.5570],
+ [-2.1217, -3.5105, 0.3384],
+ [-1.8985, -1.3818, 0.3384],
+ [-1.8985, -1.3818, -0.5570],
+ [-1.1883, -3.6084, -0.5570],
+ [-1.1883, -3.6084, 0.3384],
+ [-0.9651, -1.4796, 0.3384],
+ [-0.9651, -1.4796, -0.5570]]])
+
+ assert torch.allclose(boxes.corners, expected_tensor, 1e-3)
+
+ th_boxes = torch.tensor(
+ [[0.61211395, 0.8129094, 0.10563634, 1.497534, 0.16927195, 0.27956772],
+ [1.430009, 0.49797538, 0.9382923, 0.07694054, 0.9312509, 1.8919173]],
+ dtype=torch.float32)
+ boxes = DepthInstance3DBoxes(th_boxes, box_dim=6, with_yaw=False)
+ expected_tensor = torch.tensor([[
+ 0.64884546, 0.78390356, 0.10563634, 1.50373348, 0.23795205, 0.27956772,
+ 0
+ ],
+ [
+ 1.45139421, 0.43169443, 0.93829232,
+ 0.11967964, 0.93380373, 1.89191735, 0
+ ]])
+ boxes_3 = boxes.clone()
+ boxes_3.rotate(-0.04599790655000615)
+ assert torch.allclose(boxes_3.tensor, expected_tensor)
+ boxes.rotate(torch.tensor(-0.04599790655000615))
+ assert torch.allclose(boxes.tensor, expected_tensor)
+
+ # test bbox in_range_bev
+ expected_tensor = torch.tensor([1, 1], dtype=torch.bool)
+ mask = boxes.in_range_bev([0., -40., 70.4, 40.])
+ assert (mask == expected_tensor).all()
+ mask = boxes.nonempty()
+ assert (mask == expected_tensor).all()
+
+ # test bbox in_range
+ expected_tensor = torch.tensor([0, 1], dtype=torch.bool)
+ mask = boxes.in_range_3d([1, 0, -2, 2, 1, 5])
+ assert (mask == expected_tensor).all()
+
+ expected_tensor = torch.tensor([[[-0.1030, 0.6649, 0.1056],
+ [-0.1030, 0.6649, 0.3852],
+ [-0.1030, 0.9029, 0.3852],
+ [-0.1030, 0.9029, 0.1056],
+ [1.4007, 0.6649, 0.1056],
+ [1.4007, 0.6649, 0.3852],
+ [1.4007, 0.9029, 0.3852],
+ [1.4007, 0.9029, 0.1056]],
+ [[1.3916, -0.0352, 0.9383],
+ [1.3916, -0.0352, 2.8302],
+ [1.3916, 0.8986, 2.8302],
+ [1.3916, 0.8986, 0.9383],
+ [1.5112, -0.0352, 0.9383],
+ [1.5112, -0.0352, 2.8302],
+ [1.5112, 0.8986, 2.8302],
+ [1.5112, 0.8986, 0.9383]]])
+ assert torch.allclose(boxes.corners, expected_tensor, 1e-3)
+
+ # test points in boxes
+ if torch.cuda.is_available():
+ box_idxs_of_pts = boxes.points_in_boxes_all(points.cuda())
+ expected_idxs_of_pts = torch.tensor(
+ [[0, 0], [0, 0], [0, 0], [0, 0], [0, 0]],
+ device='cuda:0',
+ dtype=torch.int32)
+ assert torch.all(box_idxs_of_pts == expected_idxs_of_pts)
+
+ # test get_surface_line_center
+ boxes = torch.tensor(
+ [[0.3294, 1.0359, 0.1171, 1.0822, 1.1247, 1.3721, -0.4916],
+ [-2.4630, -2.6324, -0.1616, 0.9202, 1.7896, 0.1992, -0.3185]])
+ boxes = DepthInstance3DBoxes(
+ boxes, box_dim=boxes.shape[-1], with_yaw=True, origin=(0.5, 0.5, 0.5))
+ surface_center, line_center = boxes.get_surface_line_center()
+
+ expected_surface_center = torch.tensor([[0.3294, 1.0359, 0.8031],
+ [0.3294, 1.0359, -0.5689],
+ [0.5949, 1.5317, 0.1171],
+ [0.1533, 0.5018, 0.1171],
+ [0.8064, 0.7805, 0.1171],
+ [-0.1845, 1.2053, 0.1171],
+ [-2.4630, -2.6324, -0.0620],
+ [-2.4630, -2.6324, -0.2612],
+ [-2.0406, -1.8436, -0.1616],
+ [-2.7432, -3.4822, -0.1616],
+ [-2.0574, -2.8496, -0.1616],
+ [-2.9000, -2.4883, -0.1616]])
+
+ expected_line_center = torch.tensor([[0.8064, 0.7805, 0.8031],
+ [-0.1845, 1.2053, 0.8031],
+ [0.5949, 1.5317, 0.8031],
+ [0.1533, 0.5018, 0.8031],
+ [0.8064, 0.7805, -0.5689],
+ [-0.1845, 1.2053, -0.5689],
+ [0.5949, 1.5317, -0.5689],
+ [0.1533, 0.5018, -0.5689],
+ [1.0719, 1.2762, 0.1171],
+ [0.6672, 0.3324, 0.1171],
+ [0.1178, 1.7871, 0.1171],
+ [-0.3606, 0.6713, 0.1171],
+ [-2.0574, -2.8496, -0.0620],
+ [-2.9000, -2.4883, -0.0620],
+ [-2.0406, -1.8436, -0.0620],
+ [-2.7432, -3.4822, -0.0620],
+ [-2.0574, -2.8496, -0.2612],
+ [-2.9000, -2.4883, -0.2612],
+ [-2.0406, -1.8436, -0.2612],
+ [-2.7432, -3.4822, -0.2612],
+ [-1.6350, -2.0607, -0.1616],
+ [-2.3062, -3.6263, -0.1616],
+ [-2.4462, -1.6264, -0.1616],
+ [-3.1802, -3.3381, -0.1616]])
+
+ assert torch.allclose(surface_center, expected_surface_center, atol=1e-04)
+ assert torch.allclose(line_center, expected_line_center, atol=1e-04)
+
+
+def test_rotation_3d_in_axis():
+ # clockwise
+ points = torch.tensor([[[-0.4599, -0.0471, 0.0000],
+ [-0.4599, -0.0471, 1.8433],
+ [-0.4599, 0.0471, 1.8433]],
+ [[-0.2555, -0.2683, 0.0000],
+ [-0.2555, -0.2683, 0.9072],
+ [-0.2555, 0.2683, 0.9072]]])
+ rotated = rotation_3d_in_axis(
+ points,
+ torch.tensor([-np.pi / 10, np.pi / 10]),
+ axis=0,
+ clockwise=True)
+ expected_rotated = torch.tensor(
+ [[[-0.4599, -0.0448, -0.0146], [-0.4599, -0.6144, 1.7385],
+ [-0.4599, -0.5248, 1.7676]],
+ [[-0.2555, -0.2552, 0.0829], [-0.2555, 0.0252, 0.9457],
+ [-0.2555, 0.5355, 0.7799]]],
+ dtype=torch.float32)
+ assert torch.allclose(rotated, expected_rotated, atol=1e-3)
+
+ # anti-clockwise with return rotation mat
+ points = torch.tensor([[[-0.4599, -0.0471, 0.0000],
+ [-0.4599, -0.0471, 1.8433]]])
+ rotated = rotation_3d_in_axis(points, torch.tensor([np.pi / 2]), axis=0)
+ expected_rotated = torch.tensor([[[-0.4599, 0.0000, -0.0471],
+ [-0.4599, -1.8433, -0.0471]]])
+ assert torch.allclose(rotated, expected_rotated, 1e-3)
+
+ points = torch.tensor([[[-0.4599, -0.0471, 0.0000],
+ [-0.4599, -0.0471, 1.8433]]])
+ rotated, mat = rotation_3d_in_axis(
+ points, torch.tensor([np.pi / 2]), axis=0, return_mat=True)
+ expected_rotated = torch.tensor([[[-0.4599, 0.0000, -0.0471],
+ [-0.4599, -1.8433, -0.0471]]])
+ expected_mat = torch.tensor([[[1, 0, 0], [0, 0, 1], [0, -1, 0]]]).float()
+ assert torch.allclose(rotated, expected_rotated, atol=1e-6)
+ assert torch.allclose(mat, expected_mat, atol=1e-6)
+
+ points = torch.tensor([[[-0.4599, -0.0471, 0.0000],
+ [-0.4599, -0.0471, 1.8433]],
+ [[-0.2555, -0.2683, 0.0000],
+ [-0.2555, -0.2683, 0.9072]]])
+ rotated = rotation_3d_in_axis(points, np.pi / 2, axis=0)
+ expected_rotated = torch.tensor([[[-0.4599, 0.0000, -0.0471],
+ [-0.4599, -1.8433, -0.0471]],
+ [[-0.2555, 0.0000, -0.2683],
+ [-0.2555, -0.9072, -0.2683]]])
+ assert torch.allclose(rotated, expected_rotated, atol=1e-3)
+
+ points = np.array([[[-0.4599, -0.0471, 0.0000], [-0.4599, -0.0471,
+ 1.8433]],
+ [[-0.2555, -0.2683, 0.0000],
+ [-0.2555, -0.2683, 0.9072]]]).astype(np.float32)
+
+ rotated = rotation_3d_in_axis(points, np.pi / 2, axis=0)
+ expected_rotated = np.array([[[-0.4599, 0.0000, -0.0471],
+ [-0.4599, -1.8433, -0.0471]],
+ [[-0.2555, 0.0000, -0.2683],
+ [-0.2555, -0.9072, -0.2683]]])
+ assert np.allclose(rotated, expected_rotated, atol=1e-3)
+
+ points = torch.tensor([[[-0.4599, -0.0471, 0.0000],
+ [-0.4599, -0.0471, 1.8433]],
+ [[-0.2555, -0.2683, 0.0000],
+ [-0.2555, -0.2683, 0.9072]]])
+ angles = [np.pi / 2, -np.pi / 2]
+ rotated = rotation_3d_in_axis(points, angles, axis=0).numpy()
+ expected_rotated = np.array([[[-0.4599, 0.0000, -0.0471],
+ [-0.4599, -1.8433, -0.0471]],
+ [[-0.2555, 0.0000, 0.2683],
+ [-0.2555, 0.9072, 0.2683]]])
+ assert np.allclose(rotated, expected_rotated, atol=1e-3)
+
+ points = torch.tensor([[[-0.4599, -0.0471, 0.0000],
+ [-0.4599, -0.0471, 1.8433]],
+ [[-0.2555, -0.2683, 0.0000],
+ [-0.2555, -0.2683, 0.9072]]])
+ angles = [np.pi / 2, -np.pi / 2]
+ rotated = rotation_3d_in_axis(points, angles, axis=1).numpy()
+ expected_rotated = np.array([[[0.0000, -0.0471, 0.4599],
+ [1.8433, -0.0471, 0.4599]],
+ [[0.0000, -0.2683, -0.2555],
+ [-0.9072, -0.2683, -0.2555]]])
+ assert np.allclose(rotated, expected_rotated, atol=1e-3)
+
+ points = torch.tensor([[[-0.4599, -0.0471, 0.0000],
+ [-0.4599, 0.0471, 1.8433]],
+ [[-0.2555, -0.2683, 0.0000],
+ [0.2555, -0.2683, 0.9072]]])
+ angles = [np.pi / 2, -np.pi / 2]
+ rotated = rotation_3d_in_axis(points, angles, axis=2).numpy()
+ expected_rotated = np.array([[[0.0471, -0.4599, 0.0000],
+ [-0.0471, -0.4599, 1.8433]],
+ [[-0.2683, 0.2555, 0.0000],
+ [-0.2683, -0.2555, 0.9072]]])
+ assert np.allclose(rotated, expected_rotated, atol=1e-3)
+
+ points = torch.tensor([[[-0.0471, 0.0000], [-0.0471, 1.8433]],
+ [[-0.2683, 0.0000], [-0.2683, 0.9072]]])
+ angles = [np.pi / 2, -np.pi / 2]
+ rotated = rotation_3d_in_axis(points, angles)
+ expected_rotated = np.array([[[0.0000, -0.0471], [-1.8433, -0.0471]],
+ [[0.0000, 0.2683], [0.9072, 0.2683]]])
+ assert np.allclose(rotated, expected_rotated, atol=1e-3)
+
+
+def test_rotation_2d():
+ angles = np.array([3.14])
+ corners = np.array([[[-0.235, -0.49], [-0.235, 0.49], [0.235, 0.49],
+ [0.235, -0.49]]])
+ corners_rotated = rotation_3d_in_axis(corners, angles)
+ expected_corners = np.array([[[0.2357801, 0.48962511],
+ [0.2342193, -0.49037365],
+ [-0.2357801, -0.48962511],
+ [-0.2342193, 0.49037365]]])
+ assert np.allclose(corners_rotated, expected_corners)
+
+
+def test_limit_period():
+ torch.manual_seed(0)
+ val = torch.rand([5, 1])
+ result = limit_period(val)
+ expected_result = torch.tensor([[0.4963], [0.7682], [0.0885], [0.1320],
+ [0.3074]])
+ assert torch.allclose(result, expected_result, 1e-3)
+
+ val = val.numpy()
+ result = limit_period(val)
+ expected_result = expected_result.numpy()
+ assert np.allclose(result, expected_result, 1e-3)
+
+
+def test_xywhr2xyxyr():
+ torch.manual_seed(0)
+ xywhr = torch.tensor([[1., 2., 3., 4., 5.], [0., 1., 2., 3., 4.]])
+ xyxyr = xywhr2xyxyr(xywhr)
+ expected_xyxyr = torch.tensor([[-0.5000, 0.0000, 2.5000, 4.0000, 5.0000],
+ [-1.0000, -0.5000, 1.0000, 2.5000, 4.0000]])
+
+ assert torch.allclose(xyxyr, expected_xyxyr)
+
+
+class test_get_box_type(unittest.TestCase):
+
+ def test_get_box_type(self):
+ box_type_3d, box_mode_3d = get_box_type('camera')
+ assert box_type_3d == CameraInstance3DBoxes
+ assert box_mode_3d == Box3DMode.CAM
+
+ box_type_3d, box_mode_3d = get_box_type('depth')
+ assert box_type_3d == DepthInstance3DBoxes
+ assert box_mode_3d == Box3DMode.DEPTH
+
+ box_type_3d, box_mode_3d = get_box_type('lidar')
+ assert box_type_3d == LiDARInstance3DBoxes
+ assert box_mode_3d == Box3DMode.LIDAR
+
+ def test_bad_box_type(self):
+ self.assertRaises(ValueError, get_box_type, 'test')
+
+
+def test_points_cam2img():
+ torch.manual_seed(0)
+ points = torch.rand([5, 3])
+ proj_mat = torch.rand([4, 4])
+ point_2d_res = points_cam2img(points, proj_mat)
+ expected_point_2d_res = torch.tensor([[0.5832, 0.6496], [0.6146, 0.7910],
+ [0.6994, 0.7782], [0.5623, 0.6303],
+ [0.4359, 0.6532]])
+ assert torch.allclose(point_2d_res, expected_point_2d_res, 1e-3)
+
+ points = points.numpy()
+ proj_mat = proj_mat.numpy()
+ point_2d_res = points_cam2img(points, proj_mat)
+ expected_point_2d_res = expected_point_2d_res.numpy()
+ assert np.allclose(point_2d_res, expected_point_2d_res, 1e-3)
+
+ points = torch.from_numpy(points)
+ point_2d_res = points_cam2img(points, proj_mat)
+ expected_point_2d_res = torch.from_numpy(expected_point_2d_res)
+ assert torch.allclose(point_2d_res, expected_point_2d_res, 1e-3)
+
+ point_2d_res = points_cam2img(points, proj_mat, with_depth=True)
+ expected_point_2d_res = torch.tensor([[0.5832, 0.6496, 1.7577],
+ [0.6146, 0.7910, 1.5477],
+ [0.6994, 0.7782, 2.0091],
+ [0.5623, 0.6303, 1.8739],
+ [0.4359, 0.6532, 1.2056]])
+ assert torch.allclose(point_2d_res, expected_point_2d_res, 1e-3)
+
+
+def test_points_in_boxes():
+ if not torch.cuda.is_available():
+ pytest.skip('test requires GPU and torch+cuda')
+ lidar_pts = torch.tensor([[1.0, 4.3, 0.1], [1.0, 4.4,
+ 0.1], [1.1, 4.3, 0.1],
+ [0.9, 4.3, 0.1], [1.0, -0.3, 0.1],
+ [1.0, -0.4, 0.1], [2.9, 0.1, 6.0],
+ [-0.9, 3.9, 6.0]]).cuda()
+ lidar_boxes = torch.tensor([[1.0, 2.0, 0.0, 4.0, 4.0, 6.0, np.pi / 6],
+ [1.0, 2.0, 0.0, 4.0, 4.0, 6.0, np.pi / 2],
+ [1.0, 2.0, 0.0, 4.0, 4.0, 6.0, 7 * np.pi / 6],
+ [1.0, 2.0, 0.0, 4.0, 4.0, 6.0, -np.pi / 6]],
+ dtype=torch.float32).cuda()
+ lidar_boxes = LiDARInstance3DBoxes(lidar_boxes)
+
+ point_indices = lidar_boxes.points_in_boxes_all(lidar_pts)
+ expected_point_indices = torch.tensor(
+ [[1, 0, 1, 1], [0, 0, 0, 0], [1, 0, 1, 0], [0, 0, 0, 1], [1, 0, 1, 1],
+ [0, 0, 0, 0], [0, 1, 0, 0], [0, 1, 0, 0]],
+ dtype=torch.int32).cuda()
+ assert point_indices.shape == torch.Size([8, 4])
+ assert (point_indices == expected_point_indices).all()
+
+ lidar_pts = torch.tensor([[1.0, 4.3, 0.1], [1.0, 4.4,
+ 0.1], [1.1, 4.3, 0.1],
+ [0.9, 4.3, 0.1], [1.0, -0.3, 0.1],
+ [1.0, -0.4, 0.1], [2.9, 0.1, 6.0],
+ [-0.9, 3.9, 6.0]]).cuda()
+ lidar_boxes = torch.tensor([[1.0, 2.0, 0.0, 4.0, 4.0, 6.0, np.pi / 6],
+ [1.0, 2.0, 0.0, 4.0, 4.0, 6.0, np.pi / 2],
+ [1.0, 2.0, 0.0, 4.0, 4.0, 6.0, 7 * np.pi / 6],
+ [1.0, 2.0, 0.0, 4.0, 4.0, 6.0, -np.pi / 6]],
+ dtype=torch.float32).cuda()
+ lidar_boxes = LiDARInstance3DBoxes(lidar_boxes)
+
+ point_indices = lidar_boxes.points_in_boxes_part(lidar_pts)
+ expected_point_indices = torch.tensor([0, -1, 0, 3, 0, -1, 1, 1],
+ dtype=torch.int32).cuda()
+ assert point_indices.shape == torch.Size([8])
+ assert (point_indices == expected_point_indices).all()
+
+ depth_boxes = torch.tensor([[1.0, 2.0, 3.0, 4.0, 5.0, 6.0, 0.3],
+ [-10.0, 23.0, 16.0, 10, 20, 20, 0.5]],
+ dtype=torch.float32).cuda()
+ depth_boxes = DepthInstance3DBoxes(depth_boxes)
+ depth_pts = torch.tensor(
+ [[[1, 2, 3.3], [1.2, 2.5, 3.0], [0.8, 2.1, 3.5], [1.6, 2.6, 3.6],
+ [0.8, 1.2, 3.9], [-9.2, 21.0, 18.2], [3.8, 7.9, 6.3],
+ [4.7, 3.5, -12.2], [3.8, 7.6, -2], [-10.6, -12.9, -20], [
+ -16, -18, 9
+ ], [-21.3, -52, -5], [0, 0, 0], [6, 7, 8], [-2, -3, -4]]],
+ dtype=torch.float32).cuda()
+
+ point_indices = depth_boxes.points_in_boxes_all(depth_pts)
+ expected_point_indices = torch.tensor(
+ [[1, 0], [1, 0], [1, 0], [1, 0], [1, 0], [0, 1], [0, 0], [0, 0],
+ [0, 0], [0, 0], [0, 0], [0, 0], [0, 0], [0, 0], [0, 0]],
+ dtype=torch.int32).cuda()
+ assert point_indices.shape == torch.Size([15, 2])
+ assert (point_indices == expected_point_indices).all()
+
+ point_indices = depth_boxes.points_in_boxes_part(depth_pts)
+ expected_point_indices = torch.tensor(
+ [0, 0, 0, 0, 0, 1, -1, -1, -1, -1, -1, -1, -1, -1, -1],
+ dtype=torch.int32).cuda()
+ assert point_indices.shape == torch.Size([15])
+ assert (point_indices == expected_point_indices).all()
+
+ depth_boxes = torch.tensor([[1.0, 2.0, 3.0, 4.0, 5.0, 6.0, 0.3],
+ [-10.0, 23.0, 16.0, 10, 20, 20, 0.5],
+ [1.0, 2.0, 0.0, 4.0, 4.0, 6.0, np.pi / 6],
+ [1.0, 2.0, 0.0, 4.0, 4.0, 6.0, np.pi / 2],
+ [1.0, 2.0, 0.0, 4.0, 4.0, 6.0, 7 * np.pi / 6],
+ [1.0, 2.0, 0.0, 4.0, 4.0, 6.0, -np.pi / 6]],
+ dtype=torch.float32).cuda()
+ cam_boxes = DepthInstance3DBoxes(depth_boxes).convert_to(Box3DMode.CAM)
+ depth_pts = torch.tensor(
+ [[1, 2, 3.3], [1.2, 2.5, 3.0], [0.8, 2.1, 3.5], [1.6, 2.6, 3.6],
+ [0.8, 1.2, 3.9], [-9.2, 21.0, 18.2], [3.8, 7.9, 6.3],
+ [4.7, 3.5, -12.2], [3.8, 7.6, -2], [-10.6, -12.9, -20], [-16, -18, 9],
+ [-21.3, -52, -5], [0, 0, 0], [6, 7, 8], [-2, -3, -4], [1.0, 4.3, 0.1],
+ [1.0, 4.4, 0.1], [1.1, 4.3, 0.1], [0.9, 4.3, 0.1], [1.0, -0.3, 0.1],
+ [1.0, -0.4, 0.1], [2.9, 0.1, 6.0], [-0.9, 3.9, 6.0]],
+ dtype=torch.float32).cuda()
+
+ cam_pts = DepthPoints(depth_pts).convert_to(Coord3DMode.CAM).tensor
+
+ point_indices = cam_boxes.points_in_boxes_all(cam_pts)
+ expected_point_indices = torch.tensor(
+ [[1, 0, 1, 1, 1, 1], [1, 0, 1, 1, 1, 1], [1, 0, 1, 1, 1, 1],
+ [1, 0, 1, 1, 1, 1], [1, 0, 1, 1, 1, 1], [0, 1, 0, 0, 0, 0],
+ [0, 0, 0, 0, 0, 0], [0, 0, 0, 0, 0, 0], [0, 0, 0, 0, 0, 0],
+ [0, 0, 0, 0, 0, 0], [0, 0, 0, 0, 0, 0], [0, 0, 0, 0, 0, 0],
+ [0, 0, 0, 1, 0, 1], [0, 0, 0, 0, 0, 0], [0, 0, 0, 0, 0, 0],
+ [0, 0, 1, 1, 1, 1], [0, 0, 0, 1, 0, 0], [0, 0, 0, 1, 0, 1],
+ [0, 0, 1, 1, 1, 0], [0, 0, 1, 1, 1, 1], [0, 0, 0, 1, 0, 0],
+ [1, 0, 0, 0, 0, 0], [1, 0, 0, 0, 0, 0]],
+ dtype=torch.int32).cuda()
+ assert point_indices.shape == torch.Size([23, 6])
+ assert (point_indices == expected_point_indices).all()
+
+ point_indices = cam_boxes.points_in_boxes_batch(cam_pts)
+ assert (point_indices == expected_point_indices).all()
+
+ point_indices = cam_boxes.points_in_boxes_part(cam_pts)
+ expected_point_indices = torch.tensor([
+ 0, 0, 0, 0, 0, 1, -1, -1, -1, -1, -1, -1, 3, -1, -1, 2, 3, 3, 2, 2, 3,
+ 0, 0
+ ],
+ dtype=torch.int32).cuda()
+ assert point_indices.shape == torch.Size([23])
+ assert (point_indices == expected_point_indices).all()
+
+ point_indices = cam_boxes.points_in_boxes(cam_pts)
+ assert (point_indices == expected_point_indices).all()
diff --git a/tests/test_utils/test_box_np_ops.py b/tests/test_utils/test_box_np_ops.py
new file mode 100644
index 0000000..1c6275d
--- /dev/null
+++ b/tests/test_utils/test_box_np_ops.py
@@ -0,0 +1,83 @@
+# Copyright (c) OpenMMLab. All rights reserved.
+import numpy as np
+
+
+def test_camera_to_lidar():
+ from mmdet3d.core.bbox.box_np_ops import camera_to_lidar
+ points = np.array([[1.84, 1.47, 8.41]])
+ rect = np.array([[0.9999128, 0.01009263, -0.00851193, 0.],
+ [-0.01012729, 0.9999406, -0.00403767, 0.],
+ [0.00847068, 0.00412352, 0.9999556, 0.], [0., 0., 0.,
+ 1.]])
+ Trv2c = np.array([[0.00692796, -0.9999722, -0.00275783, -0.02457729],
+ [-0.00116298, 0.00274984, -0.9999955, -0.06127237],
+ [0.9999753, 0.00693114, -0.0011439, -0.3321029],
+ [0., 0., 0., 1.]])
+ points_lidar = camera_to_lidar(points, rect, Trv2c)
+ expected_points = np.array([[8.73138192, -1.85591746, -1.59969933]])
+ assert np.allclose(points_lidar, expected_points)
+
+
+def test_box_camera_to_lidar():
+ from mmdet3d.core.bbox.box_np_ops import box_camera_to_lidar
+ box = np.array([[1.84, 1.47, 8.41, 1.2, 1.89, 0.48, -0.01]])
+ rect = np.array([[0.9999128, 0.01009263, -0.00851193, 0.],
+ [-0.01012729, 0.9999406, -0.00403767, 0.],
+ [0.00847068, 0.00412352, 0.9999556, 0.], [0., 0., 0.,
+ 1.]])
+ Trv2c = np.array([[0.00692796, -0.9999722, -0.00275783, -0.02457729],
+ [-0.00116298, 0.00274984, -0.9999955, -0.06127237],
+ [0.9999753, 0.00693114, -0.0011439, -0.3321029],
+ [0., 0., 0., 1.]])
+ box_lidar = box_camera_to_lidar(box, rect, Trv2c)
+ expected_box = np.array([[
+ 8.73138192, -1.85591746, -1.59969933, 1.2, 0.48, 1.89, 0.01 - np.pi / 2
+ ]])
+ assert np.allclose(box_lidar, expected_box)
+
+
+def test_corners_nd():
+ from mmdet3d.core.bbox.box_np_ops import corners_nd
+ dims = np.array([[0.47, 0.98]])
+ corners = corners_nd(dims)
+ expected_corners = np.array([[[-0.235, -0.49], [-0.235, 0.49],
+ [0.235, 0.49], [0.235, -0.49]]])
+ assert np.allclose(corners, expected_corners)
+
+
+def test_center_to_corner_box2d():
+ from mmdet3d.core.bbox.box_np_ops import center_to_corner_box2d
+ center = np.array([[9.348705, -3.6271024]])
+ dims = np.array([[0.47, 0.98]])
+ angles = np.array([3.14])
+ corner = center_to_corner_box2d(center, dims, angles)
+ expected_corner = np.array([[[9.584485, -3.1374772], [9.582925, -4.117476],
+ [9.112926, -4.1167274],
+ [9.114486, -3.1367288]]])
+ assert np.allclose(corner, expected_corner)
+
+ center = np.array([[-0.0, 0.0]])
+ dims = np.array([[4.0, 8.0]])
+ angles = np.array([-0.785398]) # -45 degrees
+ corner = center_to_corner_box2d(center, dims, angles)
+ expected_corner = np.array([[[-4.24264, -1.41421], [1.41421, 4.24264],
+ [4.24264, 1.41421], [-1.41421, -4.24264]]])
+ assert np.allclose(corner, expected_corner)
+
+
+def test_points_in_convex_polygon_jit():
+ from mmdet3d.core.bbox.box_np_ops import points_in_convex_polygon_jit
+ points = np.array([[0.4, 0.4], [0.5, 0.5], [0.6, 0.6]])
+ polygons = np.array([[[1.0, 0.0], [0.0, 1.0], [0.0, 0.5], [0.0, 0.0]],
+ [[1.0, 0.0], [1.0, 1.0], [0.5, 1.0], [0.0, 1.0]],
+ [[1.0, 0.0], [0.0, 1.0], [-1.0, 0.0], [0.0, -1.0]]])
+ res = points_in_convex_polygon_jit(points, polygons)
+ expected_res = np.array([[1, 0, 1], [0, 0, 0], [0, 1, 0]]).astype(np.bool)
+ assert np.allclose(res, expected_res)
+
+ polygons = np.array([[[0.0, 0.0], [0.0, 1.0], [0.5, 0.5], [1.0, 0.0]],
+ [[0.0, 1.0], [1.0, 1.0], [1.0, 0.5], [1.0, 0.0]],
+ [[1.0, 0.0], [0.0, -1.0], [-1.0, 0.0], [0.0, 1.1]]])
+ res = points_in_convex_polygon_jit(points, polygons, clockwise=True)
+ expected_res = np.array([[1, 0, 1], [0, 0, 1], [0, 1, 0]]).astype(np.bool)
+ assert np.allclose(res, expected_res)
diff --git a/tests/test_utils/test_compat_cfg.py b/tests/test_utils/test_compat_cfg.py
new file mode 100644
index 0000000..3689e15
--- /dev/null
+++ b/tests/test_utils/test_compat_cfg.py
@@ -0,0 +1,113 @@
+import pytest
+from mmcv import ConfigDict
+
+from mmdet3d.utils.compat_cfg import (compat_imgs_per_gpu, compat_loader_args,
+ compat_runner_args)
+
+
+def test_compat_runner_args():
+ cfg = ConfigDict(dict(total_epochs=12))
+ with pytest.warns(None) as record:
+ cfg = compat_runner_args(cfg)
+ assert len(record) == 1
+ assert 'runner' in record.list[0].message.args[0]
+ assert 'runner' in cfg
+ assert cfg.runner.type == 'EpochBasedRunner'
+ assert cfg.runner.max_epochs == cfg.total_epochs
+
+
+def test_compat_loader_args():
+ cfg = ConfigDict(dict(data=dict(val=dict(), test=dict(), train=dict())))
+ cfg = compat_loader_args(cfg)
+ # auto fill loader args
+ assert 'val_dataloader' in cfg.data
+ assert 'train_dataloader' in cfg.data
+ assert 'test_dataloader' in cfg.data
+ cfg = ConfigDict(
+ dict(
+ data=dict(
+ samples_per_gpu=1,
+ persistent_workers=True,
+ workers_per_gpu=1,
+ val=dict(samples_per_gpu=3),
+ test=dict(samples_per_gpu=2),
+ train=dict())))
+ cfg = compat_loader_args(cfg)
+
+ assert cfg.data.train_dataloader.workers_per_gpu == 1
+ assert cfg.data.train_dataloader.samples_per_gpu == 1
+ assert cfg.data.train_dataloader.persistent_workers
+ assert cfg.data.val_dataloader.workers_per_gpu == 1
+ assert cfg.data.val_dataloader.samples_per_gpu == 3
+ assert cfg.data.test_dataloader.workers_per_gpu == 1
+ assert cfg.data.test_dataloader.samples_per_gpu == 2
+
+ # test test is a list
+ cfg = ConfigDict(
+ dict(
+ data=dict(
+ samples_per_gpu=1,
+ persistent_workers=True,
+ workers_per_gpu=1,
+ val=dict(samples_per_gpu=3),
+ test=[dict(samples_per_gpu=2),
+ dict(samples_per_gpu=3)],
+ train=dict())))
+
+ cfg = compat_loader_args(cfg)
+
+ # assert can not set args at the same time
+ cfg = ConfigDict(
+ dict(
+ data=dict(
+ samples_per_gpu=1,
+ persistent_workers=True,
+ workers_per_gpu=1,
+ val=dict(samples_per_gpu=3),
+ test=dict(samples_per_gpu=2),
+ train=dict(),
+ train_dataloader=dict(samples_per_gpu=2))))
+ # samples_per_gpu can not be set in `train_dataloader`
+ # and data field at the same time
+ with pytest.raises(AssertionError):
+ compat_loader_args(cfg)
+ cfg = ConfigDict(
+ dict(
+ data=dict(
+ samples_per_gpu=1,
+ persistent_workers=True,
+ workers_per_gpu=1,
+ val=dict(samples_per_gpu=3),
+ test=dict(samples_per_gpu=2),
+ train=dict(),
+ val_dataloader=dict(samples_per_gpu=2))))
+ # samples_per_gpu can not be set in `val_dataloader`
+ # and data field at the same time
+ with pytest.raises(AssertionError):
+ compat_loader_args(cfg)
+ cfg = ConfigDict(
+ dict(
+ data=dict(
+ samples_per_gpu=1,
+ persistent_workers=True,
+ workers_per_gpu=1,
+ val=dict(samples_per_gpu=3),
+ test=dict(samples_per_gpu=2),
+ test_dataloader=dict(samples_per_gpu=2))))
+ # samples_per_gpu can not be set in `test_dataloader`
+ # and data field at the same time
+ with pytest.raises(AssertionError):
+ compat_loader_args(cfg)
+
+
+def test_compat_imgs_per_gpu():
+ cfg = ConfigDict(
+ dict(
+ data=dict(
+ imgs_per_gpu=1,
+ samples_per_gpu=2,
+ val=dict(),
+ test=dict(),
+ train=dict())))
+ cfg = compat_imgs_per_gpu(cfg)
+ assert cfg.data.samples_per_gpu == cfg.data.imgs_per_gpu
diff --git a/tests/test_utils/test_coord_3d_mode.py b/tests/test_utils/test_coord_3d_mode.py
new file mode 100644
index 0000000..24f0e19
--- /dev/null
+++ b/tests/test_utils/test_coord_3d_mode.py
@@ -0,0 +1,351 @@
+# Copyright (c) OpenMMLab. All rights reserved.
+import numpy as np
+import torch
+
+from mmdet3d.core.bbox import (CameraInstance3DBoxes, Coord3DMode,
+ DepthInstance3DBoxes, LiDARInstance3DBoxes,
+ limit_period)
+from mmdet3d.core.points import CameraPoints, DepthPoints, LiDARPoints
+
+
+def test_points_conversion():
+ """Test the conversion of points between different modes."""
+ points_np = np.array([[
+ -5.24223238e+00, 4.00209696e+01, 2.97570381e-01, 0.6666, 0.1956,
+ 0.4974, 0.9409
+ ],
+ [
+ -2.66751588e+01, 5.59499564e+00, -9.14345860e-01,
+ 0.1502, 0.3707, 0.1086, 0.6297
+ ],
+ [
+ -5.80979675e+00, 3.54092357e+01, 2.00889888e-01,
+ 0.6565, 0.6248, 0.6954, 0.2538
+ ],
+ [
+ -3.13086877e+01, 1.09007628e+00, -1.94612112e-01,
+ 0.2803, 0.0258, 0.4896, 0.3269
+ ]],
+ dtype=np.float32)
+
+ # test CAM to LIDAR and DEPTH
+ cam_points = CameraPoints(
+ points_np,
+ points_dim=7,
+ attribute_dims=dict(color=[3, 4, 5], height=6))
+
+ convert_lidar_points = cam_points.convert_to(Coord3DMode.LIDAR)
+ expected_tensor = torch.tensor([[
+ 2.9757e-01, 5.2422e+00, -4.0021e+01, 6.6660e-01, 1.9560e-01,
+ 4.9740e-01, 9.4090e-01
+ ],
+ [
+ -9.1435e-01, 2.6675e+01, -5.5950e+00,
+ 1.5020e-01, 3.7070e-01, 1.0860e-01,
+ 6.2970e-01
+ ],
+ [
+ 2.0089e-01, 5.8098e+00, -3.5409e+01,
+ 6.5650e-01, 6.2480e-01, 6.9540e-01,
+ 2.5380e-01
+ ],
+ [
+ -1.9461e-01, 3.1309e+01, -1.0901e+00,
+ 2.8030e-01, 2.5800e-02, 4.8960e-01,
+ 3.2690e-01
+ ]])
+
+ lidar_point_tensor = Coord3DMode.convert_point(cam_points.tensor,
+ Coord3DMode.CAM,
+ Coord3DMode.LIDAR)
+ assert torch.allclose(expected_tensor, convert_lidar_points.tensor, 1e-4)
+ assert torch.allclose(lidar_point_tensor, convert_lidar_points.tensor,
+ 1e-4)
+
+ convert_depth_points = cam_points.convert_to(Coord3DMode.DEPTH)
+ expected_tensor = torch.tensor([[
+ -5.2422e+00, 2.9757e-01, -4.0021e+01, 6.6660e-01, 1.9560e-01,
+ 4.9740e-01, 9.4090e-01
+ ],
+ [
+ -2.6675e+01, -9.1435e-01, -5.5950e+00,
+ 1.5020e-01, 3.7070e-01, 1.0860e-01,
+ 6.2970e-01
+ ],
+ [
+ -5.8098e+00, 2.0089e-01, -3.5409e+01,
+ 6.5650e-01, 6.2480e-01, 6.9540e-01,
+ 2.5380e-01
+ ],
+ [
+ -3.1309e+01, -1.9461e-01, -1.0901e+00,
+ 2.8030e-01, 2.5800e-02, 4.8960e-01,
+ 3.2690e-01
+ ]])
+
+ depth_point_tensor = Coord3DMode.convert_point(cam_points.tensor,
+ Coord3DMode.CAM,
+ Coord3DMode.DEPTH)
+ assert torch.allclose(expected_tensor, convert_depth_points.tensor, 1e-4)
+ assert torch.allclose(depth_point_tensor, convert_depth_points.tensor,
+ 1e-4)
+
+ # test LIDAR to CAM and DEPTH
+ lidar_points = LiDARPoints(
+ points_np,
+ points_dim=7,
+ attribute_dims=dict(color=[3, 4, 5], height=6))
+
+ convert_cam_points = lidar_points.convert_to(Coord3DMode.CAM)
+ expected_tensor = torch.tensor([[
+ -4.0021e+01, -2.9757e-01, -5.2422e+00, 6.6660e-01, 1.9560e-01,
+ 4.9740e-01, 9.4090e-01
+ ],
+ [
+ -5.5950e+00, 9.1435e-01, -2.6675e+01,
+ 1.5020e-01, 3.7070e-01, 1.0860e-01,
+ 6.2970e-01
+ ],
+ [
+ -3.5409e+01, -2.0089e-01, -5.8098e+00,
+ 6.5650e-01, 6.2480e-01, 6.9540e-01,
+ 2.5380e-01
+ ],
+ [
+ -1.0901e+00, 1.9461e-01, -3.1309e+01,
+ 2.8030e-01, 2.5800e-02, 4.8960e-01,
+ 3.2690e-01
+ ]])
+
+ cam_point_tensor = Coord3DMode.convert_point(lidar_points.tensor,
+ Coord3DMode.LIDAR,
+ Coord3DMode.CAM)
+ assert torch.allclose(expected_tensor, convert_cam_points.tensor, 1e-4)
+ assert torch.allclose(cam_point_tensor, convert_cam_points.tensor, 1e-4)
+
+ convert_depth_points = lidar_points.convert_to(Coord3DMode.DEPTH)
+ expected_tensor = torch.tensor([[
+ -4.0021e+01, -5.2422e+00, 2.9757e-01, 6.6660e-01, 1.9560e-01,
+ 4.9740e-01, 9.4090e-01
+ ],
+ [
+ -5.5950e+00, -2.6675e+01, -9.1435e-01,
+ 1.5020e-01, 3.7070e-01, 1.0860e-01,
+ 6.2970e-01
+ ],
+ [
+ -3.5409e+01, -5.8098e+00, 2.0089e-01,
+ 6.5650e-01, 6.2480e-01, 6.9540e-01,
+ 2.5380e-01
+ ],
+ [
+ -1.0901e+00, -3.1309e+01, -1.9461e-01,
+ 2.8030e-01, 2.5800e-02, 4.8960e-01,
+ 3.2690e-01
+ ]])
+
+ depth_point_tensor = Coord3DMode.convert_point(lidar_points.tensor,
+ Coord3DMode.LIDAR,
+ Coord3DMode.DEPTH)
+ assert torch.allclose(expected_tensor, convert_depth_points.tensor, 1e-4)
+ assert torch.allclose(depth_point_tensor, convert_depth_points.tensor,
+ 1e-4)
+
+ # test DEPTH to CAM and LIDAR
+ depth_points = DepthPoints(
+ points_np,
+ points_dim=7,
+ attribute_dims=dict(color=[3, 4, 5], height=6))
+
+ convert_cam_points = depth_points.convert_to(Coord3DMode.CAM)
+ expected_tensor = torch.tensor([[
+ -5.2422e+00, -2.9757e-01, 4.0021e+01, 6.6660e-01, 1.9560e-01,
+ 4.9740e-01, 9.4090e-01
+ ],
+ [
+ -2.6675e+01, 9.1435e-01, 5.5950e+00,
+ 1.5020e-01, 3.7070e-01, 1.0860e-01,
+ 6.2970e-01
+ ],
+ [
+ -5.8098e+00, -2.0089e-01, 3.5409e+01,
+ 6.5650e-01, 6.2480e-01, 6.9540e-01,
+ 2.5380e-01
+ ],
+ [
+ -3.1309e+01, 1.9461e-01, 1.0901e+00,
+ 2.8030e-01, 2.5800e-02, 4.8960e-01,
+ 3.2690e-01
+ ]])
+
+ cam_point_tensor = Coord3DMode.convert_point(depth_points.tensor,
+ Coord3DMode.DEPTH,
+ Coord3DMode.CAM)
+ assert torch.allclose(expected_tensor, convert_cam_points.tensor, 1e-4)
+ assert torch.allclose(cam_point_tensor, convert_cam_points.tensor, 1e-4)
+
+ rt_mat_provided = torch.tensor([[0.99789, -0.012698, -0.063678],
+ [-0.012698, 0.92359, -0.38316],
+ [0.063678, 0.38316, 0.92148]])
+
+ depth_points_new = torch.cat([
+ depth_points.tensor[:, :3] @ rt_mat_provided.t(),
+ depth_points.tensor[:, 3:]
+ ],
+ dim=1)
+ mat = rt_mat_provided.new_tensor([[1, 0, 0], [0, 0, -1], [0, 1, 0]])
+ rt_mat_provided = mat @ rt_mat_provided.transpose(1, 0)
+ cam_point_tensor_new = Coord3DMode.convert_point(
+ depth_points_new,
+ Coord3DMode.DEPTH,
+ Coord3DMode.CAM,
+ rt_mat=rt_mat_provided)
+ assert torch.allclose(expected_tensor, cam_point_tensor_new, 1e-4)
+
+ convert_lidar_points = depth_points.convert_to(Coord3DMode.LIDAR)
+ expected_tensor = torch.tensor([[
+ 4.0021e+01, 5.2422e+00, 2.9757e-01, 6.6660e-01, 1.9560e-01, 4.9740e-01,
+ 9.4090e-01
+ ],
+ [
+ 5.5950e+00, 2.6675e+01, -9.1435e-01,
+ 1.5020e-01, 3.7070e-01, 1.0860e-01,
+ 6.2970e-01
+ ],
+ [
+ 3.5409e+01, 5.8098e+00, 2.0089e-01,
+ 6.5650e-01, 6.2480e-01, 6.9540e-01,
+ 2.5380e-01
+ ],
+ [
+ 1.0901e+00, 3.1309e+01, -1.9461e-01,
+ 2.8030e-01, 2.5800e-02, 4.8960e-01,
+ 3.2690e-01
+ ]])
+
+ lidar_point_tensor = Coord3DMode.convert_point(depth_points.tensor,
+ Coord3DMode.DEPTH,
+ Coord3DMode.LIDAR)
+ assert torch.allclose(lidar_point_tensor, convert_lidar_points.tensor,
+ 1e-4)
+ assert torch.allclose(lidar_point_tensor, convert_lidar_points.tensor,
+ 1e-4)
+
+
+def test_boxes_conversion():
+ # test CAM to LIDAR and DEPTH
+ cam_boxes = CameraInstance3DBoxes(
+ [[1.7802081, 2.516249, -1.7501148, 1.75, 3.39, 1.65, 1.48],
+ [8.959413, 2.4567227, -1.6357126, 1.54, 4.01, 1.57, 1.62],
+ [28.2967, -0.5557558, -1.303325, 1.47, 2.23, 1.48, -1.57],
+ [26.66902, 21.82302, -1.736057, 1.56, 3.48, 1.4, -1.69],
+ [31.31978, 8.162144, -1.6217787, 1.74, 3.77, 1.48, 2.79]])
+ convert_lidar_boxes = Coord3DMode.convert(cam_boxes, Coord3DMode.CAM,
+ Coord3DMode.LIDAR)
+
+ expected_tensor = torch.tensor([[
+ -1.7501, -1.7802, -2.5162, 1.7500, 1.6500, 3.3900, -1.4800 - np.pi / 2
+ ], [
+ -1.6357, -8.9594, -2.4567, 1.5400, 1.5700, 4.0100, -1.6200 - np.pi / 2
+ ], [-1.3033, -28.2967, 0.5558, 1.4700, 1.4800, 2.2300, 1.5700 - np.pi / 2],
+ [
+ -1.7361, -26.6690, -21.8230, 1.5600,
+ 1.4000, 3.4800, 1.6900 - np.pi / 2
+ ],
+ [
+ -1.6218, -31.3198, -8.1621, 1.7400,
+ 1.4800, 3.7700, -2.7900 - np.pi / 2
+ ]])
+ expected_tensor[:, -1:] = limit_period(
+ expected_tensor[:, -1:], period=np.pi * 2)
+ assert torch.allclose(expected_tensor, convert_lidar_boxes.tensor, 1e-3)
+
+ convert_depth_boxes = Coord3DMode.convert(cam_boxes, Coord3DMode.CAM,
+ Coord3DMode.DEPTH)
+ expected_tensor = torch.tensor(
+ [[1.7802, -1.7501, -2.5162, 1.7500, 1.6500, 3.3900, -1.4800],
+ [8.9594, -1.6357, -2.4567, 1.5400, 1.5700, 4.0100, -1.6200],
+ [28.2967, -1.3033, 0.5558, 1.4700, 1.4800, 2.2300, 1.5700],
+ [26.6690, -1.7361, -21.8230, 1.5600, 1.4000, 3.4800, 1.6900],
+ [31.3198, -1.6218, -8.1621, 1.7400, 1.4800, 3.7700, -2.7900]])
+ assert torch.allclose(expected_tensor, convert_depth_boxes.tensor, 1e-3)
+
+ # test LIDAR to CAM and DEPTH
+ lidar_boxes = LiDARInstance3DBoxes(
+ [[1.7802081, 2.516249, -1.7501148, 1.75, 3.39, 1.65, 1.48],
+ [8.959413, 2.4567227, -1.6357126, 1.54, 4.01, 1.57, 1.62],
+ [28.2967, -0.5557558, -1.303325, 1.47, 2.23, 1.48, -1.57],
+ [26.66902, 21.82302, -1.736057, 1.56, 3.48, 1.4, -1.69],
+ [31.31978, 8.162144, -1.6217787, 1.74, 3.77, 1.48, 2.79]])
+ convert_cam_boxes = Coord3DMode.convert(lidar_boxes, Coord3DMode.LIDAR,
+ Coord3DMode.CAM)
+ expected_tensor = torch.tensor([
+ [-2.5162, 1.7501, 1.7802, 1.7500, 1.6500, 3.3900, -1.4800 - np.pi / 2],
+ [-2.4567, 1.6357, 8.9594, 1.5400, 1.5700, 4.0100, -1.6200 - np.pi / 2],
+ [0.5558, 1.3033, 28.2967, 1.4700, 1.4800, 2.2300, 1.5700 - np.pi / 2],
+ [
+ -21.8230, 1.7361, 26.6690, 1.5600, 1.4000, 3.4800,
+ 1.6900 - np.pi / 2
+ ],
+ [
+ -8.1621, 1.6218, 31.3198, 1.7400, 1.4800, 3.7700,
+ -2.7900 - np.pi / 2
+ ]
+ ])
+ expected_tensor[:, -1:] = limit_period(
+ expected_tensor[:, -1:], period=np.pi * 2)
+ assert torch.allclose(expected_tensor, convert_cam_boxes.tensor, 1e-3)
+
+ convert_depth_boxes = Coord3DMode.convert(lidar_boxes, Coord3DMode.LIDAR,
+ Coord3DMode.DEPTH)
+ expected_tensor = torch.tensor([[
+ -2.5162, 1.7802, -1.7501, 1.7500, 3.3900, 1.6500, 1.4800 + np.pi / 2
+ ], [-2.4567, 8.9594, -1.6357, 1.5400, 4.0100, 1.5700, 1.6200 + np.pi / 2],
+ [
+ 0.5558, 28.2967, -1.3033, 1.4700,
+ 2.2300, 1.4800, -1.5700 + np.pi / 2
+ ],
+ [
+ -21.8230, 26.6690, -1.7361, 1.5600,
+ 3.4800, 1.4000, -1.6900 + np.pi / 2
+ ],
+ [
+ -8.1621, 31.3198, -1.6218, 1.7400,
+ 3.7700, 1.4800, 2.7900 + np.pi / 2
+ ]])
+ expected_tensor[:, -1:] = limit_period(
+ expected_tensor[:, -1:], period=np.pi * 2)
+ assert torch.allclose(expected_tensor, convert_depth_boxes.tensor, 1e-3)
+
+ # test DEPTH to CAM and LIDAR
+ depth_boxes = DepthInstance3DBoxes(
+ [[1.7802081, 2.516249, -1.7501148, 1.75, 3.39, 1.65, 1.48],
+ [8.959413, 2.4567227, -1.6357126, 1.54, 4.01, 1.57, 1.62],
+ [28.2967, -0.5557558, -1.303325, 1.47, 2.23, 1.48, -1.57],
+ [26.66902, 21.82302, -1.736057, 1.56, 3.48, 1.4, -1.69],
+ [31.31978, 8.162144, -1.6217787, 1.74, 3.77, 1.48, 2.79]])
+ convert_cam_boxes = Coord3DMode.convert(depth_boxes, Coord3DMode.DEPTH,
+ Coord3DMode.CAM)
+ expected_tensor = torch.tensor(
+ [[1.7802, 1.7501, 2.5162, 1.7500, 1.6500, 3.3900, -1.4800],
+ [8.9594, 1.6357, 2.4567, 1.5400, 1.5700, 4.0100, -1.6200],
+ [28.2967, 1.3033, -0.5558, 1.4700, 1.4800, 2.2300, 1.5700],
+ [26.6690, 1.7361, 21.8230, 1.5600, 1.4000, 3.4800, 1.6900],
+ [31.3198, 1.6218, 8.1621, 1.7400, 1.4800, 3.7700, -2.7900]])
+ assert torch.allclose(expected_tensor, convert_cam_boxes.tensor, 1e-3)
+
+ convert_lidar_boxes = Coord3DMode.convert(depth_boxes, Coord3DMode.DEPTH,
+ Coord3DMode.LIDAR)
+ expected_tensor = torch.tensor([[
+ 2.5162, -1.7802, -1.7501, 1.7500, 3.3900, 1.6500, 1.4800 - np.pi / 2
+ ], [
+ 2.4567, -8.9594, -1.6357, 1.5400, 4.0100, 1.5700, 1.6200 - np.pi / 2
+ ], [
+ -0.5558, -28.2967, -1.3033, 1.4700, 2.2300, 1.4800, -1.5700 - np.pi / 2
+ ], [
+ 21.8230, -26.6690, -1.7361, 1.5600, 3.4800, 1.4000, -1.6900 - np.pi / 2
+ ], [8.1621, -31.3198, -1.6218, 1.7400, 3.7700, 1.4800,
+ 2.7900 - np.pi / 2]])
+ expected_tensor[:, -1:] = limit_period(
+ expected_tensor[:, -1:], period=np.pi * 2)
+ assert torch.allclose(expected_tensor, convert_lidar_boxes.tensor, 1e-3)
diff --git a/tests/test_utils/test_merge_augs.py b/tests/test_utils/test_merge_augs.py
new file mode 100644
index 0000000..c9ea5dc
--- /dev/null
+++ b/tests/test_utils/test_merge_augs.py
@@ -0,0 +1,67 @@
+# Copyright (c) OpenMMLab. All rights reserved.
+import mmcv
+import pytest
+import torch
+
+from mmdet3d.core import merge_aug_bboxes_3d
+from mmdet3d.core.bbox import DepthInstance3DBoxes
+
+
+def test_merge_aug_bboxes_3d():
+ if not torch.cuda.is_available():
+ pytest.skip('test requires GPU and torch+cuda')
+ img_meta_0 = dict(
+ pcd_horizontal_flip=False,
+ pcd_vertical_flip=True,
+ pcd_scale_factor=1.0)
+ img_meta_1 = dict(
+ pcd_horizontal_flip=True,
+ pcd_vertical_flip=False,
+ pcd_scale_factor=1.0)
+ img_meta_2 = dict(
+ pcd_horizontal_flip=False,
+ pcd_vertical_flip=False,
+ pcd_scale_factor=0.5)
+ img_metas = [[img_meta_0], [img_meta_1], [img_meta_2]]
+ boxes_3d = DepthInstance3DBoxes(
+ torch.tensor(
+ [[1.0473, 4.1687, -1.2317, 2.3021, 1.8876, 1.9696, 1.6956],
+ [2.5831, 4.8117, -1.2733, 0.5852, 0.8832, 0.9733, 1.6500],
+ [-1.0864, 1.9045, -1.2000, 0.7128, 1.5631, 2.1045, 0.1022]],
+ device='cuda'))
+ labels_3d = torch.tensor([0, 7, 6], device='cuda')
+ scores_3d_1 = torch.tensor([0.3, 0.6, 0.9], device='cuda')
+ scores_3d_2 = torch.tensor([0.2, 0.5, 0.8], device='cuda')
+ scores_3d_3 = torch.tensor([0.1, 0.4, 0.7], device='cuda')
+ aug_result_1 = dict(
+ boxes_3d=boxes_3d, labels_3d=labels_3d, scores_3d=scores_3d_1)
+ aug_result_2 = dict(
+ boxes_3d=boxes_3d, labels_3d=labels_3d, scores_3d=scores_3d_2)
+ aug_result_3 = dict(
+ boxes_3d=boxes_3d, labels_3d=labels_3d, scores_3d=scores_3d_3)
+ aug_results = [aug_result_1, aug_result_2, aug_result_3]
+ test_cfg = mmcv.ConfigDict(
+ use_rotate_nms=True,
+ nms_across_levels=False,
+ nms_thr=0.01,
+ score_thr=0.1,
+ min_bbox_size=0,
+ nms_pre=100,
+ max_num=50)
+ results = merge_aug_bboxes_3d(aug_results, img_metas, test_cfg)
+ expected_boxes_3d = torch.tensor(
+ [[-1.0864, -1.9045, -1.2000, 0.7128, 1.5631, 2.1045, -0.1022],
+ [1.0864, 1.9045, -1.2000, 0.7128, 1.5631, 2.1045, 3.0394],
+ [-2.1728, 3.8090, -2.4000, 1.4256, 3.1262, 4.2090, 0.1022],
+ [2.5831, -4.8117, -1.2733, 0.5852, 0.8832, 0.9733, -1.6500],
+ [-2.5831, 4.8117, -1.2733, 0.5852, 0.8832, 0.9733, 1.4916],
+ [5.1662, 9.6234, -2.5466, 1.1704, 1.7664, 1.9466, 1.6500],
+ [1.0473, -4.1687, -1.2317, 2.3021, 1.8876, 1.9696, -1.6956],
+ [-1.0473, 4.1687, -1.2317, 2.3021, 1.8876, 1.9696, 1.4460],
+ [2.0946, 8.3374, -2.4634, 4.6042, 3.7752, 3.9392, 1.6956]])
+ expected_scores_3d = torch.tensor(
+ [0.9, 0.8, 0.7, 0.6, 0.5, 0.4, 0.3, 0.2, 0.1])
+ expected_labels_3d = torch.tensor([6, 6, 6, 7, 7, 7, 0, 0, 0])
+ assert torch.allclose(results['boxes_3d'].tensor, expected_boxes_3d)
+ assert torch.allclose(results['scores_3d'], expected_scores_3d)
+ assert torch.all(results['labels_3d'] == expected_labels_3d)
diff --git a/tests/test_utils/test_nms.py b/tests/test_utils/test_nms.py
new file mode 100644
index 0000000..8ae38c4
--- /dev/null
+++ b/tests/test_utils/test_nms.py
@@ -0,0 +1,114 @@
+# Copyright (c) OpenMMLab. All rights reserved.
+import numpy as np
+import pytest
+import torch
+
+
+def test_aligned_3d_nms():
+ from mmdet3d.core.post_processing import aligned_3d_nms
+
+ boxes = torch.tensor([[1.2261, 0.6679, -1.2678, 2.6547, 1.0428, 0.1000],
+ [5.0919, 0.6512, 0.7238, 5.4821, 1.2451, 2.1095],
+ [6.8392, -1.2205, 0.8570, 7.6920, 0.3220, 3.2223],
+ [3.6900, -0.4235, -1.0380, 4.4415, 0.2671, -0.1442],
+ [4.8071, -1.4311, 0.7004, 5.5788, -0.6837, 1.2487],
+ [2.1807, -1.5811, -1.1289, 3.0151, -0.1346, -0.5351],
+ [4.4631, -4.2588, -1.1403, 5.3012, -3.4463, -0.3212],
+ [4.7607, -3.3311, 0.5993, 5.2976, -2.7874, 1.2273],
+ [3.1265, 0.7113, -0.0296, 3.8944, 1.3532, 0.9785],
+ [5.5828, -3.5350, 1.0105, 8.2841, -0.0405, 3.3614],
+ [3.0003, -2.1099, -1.0608, 5.3423, 0.0328, 0.6252],
+ [2.7148, 0.6082, -1.1738, 3.6995, 1.2375, -0.0209],
+ [4.9263, -0.2152, 0.2889, 5.6963, 0.3416, 1.3471],
+ [5.0713, 1.3459, -0.2598, 5.6278, 1.9300, 1.2835],
+ [4.5985, -2.3996, -0.3393, 5.2705, -1.7306, 0.5698],
+ [4.1386, 0.5658, 0.0422, 4.8937, 1.1983, 0.9911],
+ [2.7694, -1.9822, -1.0637, 4.0691, 0.3575, -0.1393],
+ [4.6464, -3.0123, -1.0694, 5.1421, -2.4450, -0.3758],
+ [3.4754, 0.4443, -1.1282, 4.6727, 1.3786, 0.2550],
+ [2.5905, -0.3504, -1.1202, 3.1599, 0.1153, -0.3036],
+ [4.1336, -3.4813, 1.1477, 6.2091, -0.8776, 2.6757],
+ [3.9966, 0.2069, -1.1148, 5.0841, 1.0525, -0.0648],
+ [4.3216, -1.8647, 0.4733, 6.2069, 0.6671, 3.3363],
+ [4.7683, 0.4286, -0.0500, 5.5642, 1.2906, 0.8902],
+ [1.7337, 0.7625, -1.0058, 3.0675, 1.3617, 0.3849],
+ [4.7193, -3.3687, -0.9635, 5.1633, -2.7656, 1.1001],
+ [4.4704, -2.7744, -1.1127, 5.0971, -2.0228, -0.3150],
+ [2.7027, 0.6122, -0.9169, 3.3083, 1.2117, 0.6129],
+ [4.8789, -2.0025, 0.8385, 5.5214, -1.3668, 1.3552],
+ [3.7856, -1.7582, -0.1738, 5.3373, -0.6300, 0.5558]])
+
+ scores = torch.tensor([
+ 3.6414e-03, 2.2901e-02, 2.7576e-04, 1.2238e-02, 5.9310e-04, 1.2659e-01,
+ 2.4104e-02, 5.0742e-03, 2.3581e-03, 2.0946e-07, 8.8039e-01, 1.9127e-01,
+ 5.0469e-05, 9.3638e-03, 3.0663e-03, 9.4350e-03, 5.3380e-02, 1.7895e-01,
+ 2.0048e-01, 1.1294e-03, 3.0304e-08, 2.0237e-01, 1.0894e-08, 6.7972e-02,
+ 6.7156e-01, 9.3986e-04, 7.9470e-01, 3.9736e-01, 1.8000e-04, 7.9151e-04
+ ])
+
+ cls = torch.tensor([
+ 8, 8, 8, 3, 3, 1, 3, 3, 7, 8, 0, 6, 7, 8, 3, 7, 2, 7, 6, 3, 8, 6, 6, 7,
+ 6, 8, 7, 6, 3, 1
+ ])
+
+ pick = aligned_3d_nms(boxes, scores, cls, 0.25)
+ expected_pick = torch.tensor([
+ 10, 26, 24, 27, 21, 18, 17, 5, 23, 16, 6, 1, 3, 15, 13, 7, 0, 14, 8,
+ 19, 25, 29, 4, 2, 28, 12, 9, 20, 22
+ ])
+
+ assert torch.all(pick == expected_pick)
+
+
+def test_circle_nms():
+ from mmdet3d.core.post_processing import circle_nms
+ boxes = torch.tensor([[-11.1100, 2.1300, 0.8823],
+ [-11.2810, 2.2422, 0.8914],
+ [-10.3966, -0.3198, 0.8643],
+ [-10.2906, -13.3159,
+ 0.8401], [5.6518, 9.9791, 0.8271],
+ [-11.2652, 13.3637, 0.8267],
+ [4.7768, -13.0409, 0.7810], [5.6621, 9.0422, 0.7753],
+ [-10.5561, 18.9627, 0.7518],
+ [-10.5643, 13.2293, 0.7200]])
+ keep = circle_nms(boxes.numpy(), 0.175)
+ expected_keep = [1, 2, 3, 4, 5, 6, 7, 8, 9]
+ assert np.all(keep == expected_keep)
+
+
+# copied from tests/test_ops/test_iou3d.py from mmcv<=1.5
+@pytest.mark.skipif(
+ not torch.cuda.is_available(), reason='requires CUDA support')
+def test_nms_bev():
+ from mmdet3d.core.post_processing import nms_bev
+
+ np_boxes = np.array(
+ [[6.0, 3.0, 8.0, 7.0, 2.0], [3.0, 6.0, 9.0, 11.0, 1.0],
+ [3.0, 7.0, 10.0, 12.0, 1.0], [1.0, 4.0, 13.0, 7.0, 3.0]],
+ dtype=np.float32)
+ np_scores = np.array([0.6, 0.9, 0.7, 0.2], dtype=np.float32)
+ np_inds = np.array([1, 0, 3])
+ boxes = torch.from_numpy(np_boxes)
+ scores = torch.from_numpy(np_scores)
+ inds = nms_bev(boxes.cuda(), scores.cuda(), thresh=0.3)
+
+ assert np.allclose(inds.cpu().numpy(), np_inds)
+
+
+# copied from tests/test_ops/test_iou3d.py from mmcv<=1.5
+@pytest.mark.skipif(
+ not torch.cuda.is_available(), reason='requires CUDA support')
+def test_nms_normal_bev():
+ from mmdet3d.core.post_processing import nms_normal_bev
+
+ np_boxes = np.array(
+ [[6.0, 3.0, 8.0, 7.0, 2.0], [3.0, 6.0, 9.0, 11.0, 1.0],
+ [3.0, 7.0, 10.0, 12.0, 1.0], [1.0, 4.0, 13.0, 7.0, 3.0]],
+ dtype=np.float32)
+ np_scores = np.array([0.6, 0.9, 0.7, 0.2], dtype=np.float32)
+ np_inds = np.array([1, 0, 3])
+ boxes = torch.from_numpy(np_boxes)
+ scores = torch.from_numpy(np_scores)
+ inds = nms_normal_bev(boxes.cuda(), scores.cuda(), thresh=0.3)
+
+ assert np.allclose(inds.cpu().numpy(), np_inds)
diff --git a/tests/test_utils/test_points.py b/tests/test_utils/test_points.py
new file mode 100644
index 0000000..20af27f
--- /dev/null
+++ b/tests/test_utils/test_points.py
@@ -0,0 +1,1100 @@
+# Copyright (c) OpenMMLab. All rights reserved.
+import numpy as np
+import pytest
+import torch
+
+from mmdet3d.core.points import (BasePoints, CameraPoints, DepthPoints,
+ LiDARPoints)
+
+
+def test_base_points():
+ # test empty initialization
+ empty_boxes = []
+ points = BasePoints(empty_boxes)
+ assert points.tensor.shape[0] == 0
+ assert points.tensor.shape[1] == 3
+
+ # Test init with origin
+ points_np = np.array([[-5.24223238e+00, 4.00209696e+01, 2.97570381e-01],
+ [-2.66751588e+01, 5.59499564e+00, -9.14345860e-01],
+ [-5.80979675e+00, 3.54092357e+01, 2.00889888e-01],
+ [-3.13086877e+01, 1.09007628e+00, -1.94612112e-01]],
+ dtype=np.float32)
+ base_points = BasePoints(points_np, points_dim=3)
+ assert base_points.tensor.shape[0] == 4
+
+ # Test init with color and height
+ points_np = np.array([[
+ -5.24223238e+00, 4.00209696e+01, 2.97570381e-01, 0.6666, 0.1956,
+ 0.4974, 0.9409
+ ],
+ [
+ -2.66751588e+01, 5.59499564e+00, -9.14345860e-01,
+ 0.1502, 0.3707, 0.1086, 0.6297
+ ],
+ [
+ -5.80979675e+00, 3.54092357e+01, 2.00889888e-01,
+ 0.6565, 0.6248, 0.6954, 0.2538
+ ],
+ [
+ -3.13086877e+01, 1.09007628e+00, -1.94612112e-01,
+ 0.2803, 0.0258, 0.4896, 0.3269
+ ]],
+ dtype=np.float32)
+ base_points = BasePoints(
+ points_np,
+ points_dim=7,
+ attribute_dims=dict(color=[3, 4, 5], height=6))
+ expected_tensor = torch.tensor([[
+ -5.24223238e+00, 4.00209696e+01, 2.97570381e-01, 0.6666, 0.1956,
+ 0.4974, 0.9409
+ ],
+ [
+ -2.66751588e+01, 5.59499564e+00,
+ -9.14345860e-01, 0.1502, 0.3707,
+ 0.1086, 0.6297
+ ],
+ [
+ -5.80979675e+00, 3.54092357e+01,
+ 2.00889888e-01, 0.6565, 0.6248, 0.6954,
+ 0.2538
+ ],
+ [
+ -3.13086877e+01, 1.09007628e+00,
+ -1.94612112e-01, 0.2803, 0.0258,
+ 0.4896, 0.3269
+ ]])
+
+ assert torch.allclose(expected_tensor, base_points.tensor)
+ assert torch.allclose(expected_tensor[:, :2], base_points.bev)
+ assert torch.allclose(expected_tensor[:, :3], base_points.coord)
+ assert torch.allclose(expected_tensor[:, 3:6], base_points.color)
+ assert torch.allclose(expected_tensor[:, 6], base_points.height)
+
+ # test points clone
+ new_base_points = base_points.clone()
+ assert torch.allclose(new_base_points.tensor, base_points.tensor)
+
+ # test points shuffle
+ new_base_points.shuffle()
+ assert new_base_points.tensor.shape == torch.Size([4, 7])
+
+ # test points rotation
+ rot_mat = torch.tensor([[0.93629336, -0.27509585, 0.21835066],
+ [0.28962948, 0.95642509, -0.03695701],
+ [-0.19866933, 0.0978434, 0.97517033]])
+
+ base_points.rotate(rot_mat)
+ expected_tensor = torch.tensor([[
+ 6.6239e+00, 3.9748e+01, -2.3335e+00, 6.6660e-01, 1.9560e-01,
+ 4.9740e-01, 9.4090e-01
+ ],
+ [
+ -2.3174e+01, 1.2600e+01, -6.9230e+00,
+ 1.5020e-01, 3.7070e-01, 1.0860e-01,
+ 6.2970e-01
+ ],
+ [
+ 4.7760e+00, 3.5484e+01, -2.3813e+00,
+ 6.5650e-01, 6.2480e-01, 6.9540e-01,
+ 2.5380e-01
+ ],
+ [
+ -2.8960e+01, 9.6364e+00, -7.0663e+00,
+ 2.8030e-01, 2.5800e-02, 4.8960e-01,
+ 3.2690e-01
+ ]])
+ assert torch.allclose(expected_tensor, base_points.tensor, 1e-3)
+
+ new_base_points = base_points.clone()
+ new_base_points.rotate(0.1, axis=2)
+ expected_tensor = torch.tensor([[
+ 2.6226e+00, 4.0211e+01, -2.3335e+00, 6.6660e-01, 1.9560e-01,
+ 4.9740e-01, 9.4090e-01
+ ],
+ [
+ -2.4316e+01, 1.0224e+01, -6.9230e+00,
+ 1.5020e-01, 3.7070e-01, 1.0860e-01,
+ 6.2970e-01
+ ],
+ [
+ 1.2096e+00, 3.5784e+01, -2.3813e+00,
+ 6.5650e-01, 6.2480e-01, 6.9540e-01,
+ 2.5380e-01
+ ],
+ [
+ -2.9777e+01, 6.6971e+00, -7.0663e+00,
+ 2.8030e-01, 2.5800e-02, 4.8960e-01,
+ 3.2690e-01
+ ]])
+ assert torch.allclose(expected_tensor, new_base_points.tensor, 1e-3)
+
+ # test points translation
+ translation_vector = torch.tensor([0.93629336, -0.27509585, 0.21835066])
+ base_points.translate(translation_vector)
+ expected_tensor = torch.tensor([[
+ 7.5602e+00, 3.9473e+01, -2.1152e+00, 6.6660e-01, 1.9560e-01,
+ 4.9740e-01, 9.4090e-01
+ ],
+ [
+ -2.2237e+01, 1.2325e+01, -6.7046e+00,
+ 1.5020e-01, 3.7070e-01, 1.0860e-01,
+ 6.2970e-01
+ ],
+ [
+ 5.7123e+00, 3.5209e+01, -2.1629e+00,
+ 6.5650e-01, 6.2480e-01, 6.9540e-01,
+ 2.5380e-01
+ ],
+ [
+ -2.8023e+01, 9.3613e+00, -6.8480e+00,
+ 2.8030e-01, 2.5800e-02, 4.8960e-01,
+ 3.2690e-01
+ ]])
+ assert torch.allclose(expected_tensor, base_points.tensor, 1e-4)
+
+ # test points filter
+ point_range = [-10, -40, -10, 10, 40, 10]
+ in_range_flags = base_points.in_range_3d(point_range)
+ expected_flags = torch.tensor([True, False, True, False])
+ assert torch.all(in_range_flags == expected_flags)
+
+ # test points scale
+ base_points.scale(1.2)
+ expected_tensor = torch.tensor([[
+ 9.0722e+00, 4.7368e+01, -2.5382e+00, 6.6660e-01, 1.9560e-01,
+ 4.9740e-01, 9.4090e-01
+ ],
+ [
+ -2.6685e+01, 1.4790e+01, -8.0455e+00,
+ 1.5020e-01, 3.7070e-01, 1.0860e-01,
+ 6.2970e-01
+ ],
+ [
+ 6.8547e+00, 4.2251e+01, -2.5955e+00,
+ 6.5650e-01, 6.2480e-01, 6.9540e-01,
+ 2.5380e-01
+ ],
+ [
+ -3.3628e+01, 1.1234e+01, -8.2176e+00,
+ 2.8030e-01, 2.5800e-02, 4.8960e-01,
+ 3.2690e-01
+ ]])
+ assert torch.allclose(expected_tensor, base_points.tensor, 1e-3)
+
+ # test get_item
+ expected_tensor = torch.tensor(
+ [[-26.6848, 14.7898, -8.0455, 0.1502, 0.3707, 0.1086, 0.6297]])
+ assert torch.allclose(expected_tensor, base_points[1].tensor, 1e-4)
+ expected_tensor = torch.tensor(
+ [[-26.6848, 14.7898, -8.0455, 0.1502, 0.3707, 0.1086, 0.6297],
+ [6.8547, 42.2509, -2.5955, 0.6565, 0.6248, 0.6954, 0.2538]])
+ assert torch.allclose(expected_tensor, base_points[1:3].tensor, 1e-4)
+ mask = torch.tensor([True, False, True, False])
+ expected_tensor = torch.tensor(
+ [[9.0722, 47.3678, -2.5382, 0.6666, 0.1956, 0.4974, 0.9409],
+ [6.8547, 42.2509, -2.5955, 0.6565, 0.6248, 0.6954, 0.2538]])
+ assert torch.allclose(expected_tensor, base_points[mask].tensor, 1e-4)
+ expected_tensor = torch.tensor([[0.6666], [0.1502], [0.6565], [0.2803]])
+ assert torch.allclose(expected_tensor, base_points[:, 3].tensor, 1e-4)
+
+ # test length
+ assert len(base_points) == 4
+
+ # test repr
+ expected_repr = 'BasePoints(\n '\
+ 'tensor([[ 9.0722e+00, 4.7368e+01, -2.5382e+00, '\
+ '6.6660e-01, 1.9560e-01,\n 4.9740e-01, '\
+ '9.4090e-01],\n '\
+ '[-2.6685e+01, 1.4790e+01, -8.0455e+00, 1.5020e-01, '\
+ '3.7070e-01,\n '\
+ '1.0860e-01, 6.2970e-01],\n '\
+ '[ 6.8547e+00, 4.2251e+01, -2.5955e+00, 6.5650e-01, '\
+ '6.2480e-01,\n '\
+ '6.9540e-01, 2.5380e-01],\n '\
+ '[-3.3628e+01, 1.1234e+01, -8.2176e+00, 2.8030e-01, '\
+ '2.5800e-02,\n '\
+ '4.8960e-01, 3.2690e-01]]))'
+ assert expected_repr == str(base_points)
+
+ # test concatenate
+ base_points_clone = base_points.clone()
+ cat_points = BasePoints.cat([base_points, base_points_clone])
+ assert torch.allclose(cat_points.tensor[:len(base_points)],
+ base_points.tensor)
+
+ # test iteration
+ for i, point in enumerate(base_points):
+ assert torch.allclose(point, base_points.tensor[i])
+
+ # test new_point
+ new_points = base_points.new_point([[1, 2, 3, 4, 5, 6, 7]])
+ assert torch.allclose(
+ new_points.tensor,
+ torch.tensor([[1, 2, 3, 4, 5, 6, 7]], dtype=base_points.tensor.dtype))
+
+ # test BasePoint indexing
+ base_points = BasePoints(
+ points_np,
+ points_dim=7,
+ attribute_dims=dict(height=3, color=[4, 5, 6]))
+ assert torch.all(base_points[:, 3:].tensor == torch.tensor(points_np[:,
+ 3:]))
+
+ # test set and get function for BasePoint color and height
+ base_points = BasePoints(points_np[:, :3])
+ assert base_points.attribute_dims is None
+ base_points.height = points_np[:, 3]
+ assert base_points.attribute_dims == dict(height=3)
+ base_points.color = points_np[:, 4:]
+ assert base_points.attribute_dims == dict(height=3, color=[4, 5, 6])
+ assert torch.allclose(base_points.height,
+ torch.tensor([0.6666, 0.1502, 0.6565, 0.2803]))
+ assert torch.allclose(
+ base_points.color,
+ torch.tensor([[0.1956, 0.4974, 0.9409], [0.3707, 0.1086, 0.6297],
+ [0.6248, 0.6954, 0.2538], [0.0258, 0.4896, 0.3269]]))
+ # values to be set should have correct shape (e.g. number of points)
+ with pytest.raises(ValueError):
+ base_points.coord = np.random.rand(5, 3)
+ with pytest.raises(ValueError):
+ base_points.height = np.random.rand(3)
+ with pytest.raises(ValueError):
+ base_points.color = np.random.rand(4, 2)
+ base_points.coord = points_np[:, [1, 2, 3]]
+ base_points.height = points_np[:, 0]
+ base_points.color = points_np[:, [4, 5, 6]]
+ assert np.allclose(base_points.coord, points_np[:, 1:4])
+ assert np.allclose(base_points.height, points_np[:, 0])
+ assert np.allclose(base_points.color, points_np[:, 4:])
+
+
+def test_cam_points():
+ # test empty initialization
+ empty_boxes = []
+ points = CameraPoints(empty_boxes)
+ assert points.tensor.shape[0] == 0
+ assert points.tensor.shape[1] == 3
+
+ # Test init with origin
+ points_np = np.array([[-5.24223238e+00, 4.00209696e+01, 2.97570381e-01],
+ [-2.66751588e+01, 5.59499564e+00, -9.14345860e-01],
+ [-5.80979675e+00, 3.54092357e+01, 2.00889888e-01],
+ [-3.13086877e+01, 1.09007628e+00, -1.94612112e-01]],
+ dtype=np.float32)
+ cam_points = CameraPoints(points_np, points_dim=3)
+ assert cam_points.tensor.shape[0] == 4
+
+ # Test init with color and height
+ points_np = np.array([[
+ -5.24223238e+00, 4.00209696e+01, 2.97570381e-01, 0.6666, 0.1956,
+ 0.4974, 0.9409
+ ],
+ [
+ -2.66751588e+01, 5.59499564e+00, -9.14345860e-01,
+ 0.1502, 0.3707, 0.1086, 0.6297
+ ],
+ [
+ -5.80979675e+00, 3.54092357e+01, 2.00889888e-01,
+ 0.6565, 0.6248, 0.6954, 0.2538
+ ],
+ [
+ -3.13086877e+01, 1.09007628e+00, -1.94612112e-01,
+ 0.2803, 0.0258, 0.4896, 0.3269
+ ]],
+ dtype=np.float32)
+ cam_points = CameraPoints(
+ points_np,
+ points_dim=7,
+ attribute_dims=dict(color=[3, 4, 5], height=6))
+ expected_tensor = torch.tensor([[
+ -5.24223238e+00, 4.00209696e+01, 2.97570381e-01, 0.6666, 0.1956,
+ 0.4974, 0.9409
+ ],
+ [
+ -2.66751588e+01, 5.59499564e+00,
+ -9.14345860e-01, 0.1502, 0.3707,
+ 0.1086, 0.6297
+ ],
+ [
+ -5.80979675e+00, 3.54092357e+01,
+ 2.00889888e-01, 0.6565, 0.6248, 0.6954,
+ 0.2538
+ ],
+ [
+ -3.13086877e+01, 1.09007628e+00,
+ -1.94612112e-01, 0.2803, 0.0258,
+ 0.4896, 0.3269
+ ]])
+
+ assert torch.allclose(expected_tensor, cam_points.tensor)
+ assert torch.allclose(expected_tensor[:, [0, 2]], cam_points.bev)
+ assert torch.allclose(expected_tensor[:, :3], cam_points.coord)
+ assert torch.allclose(expected_tensor[:, 3:6], cam_points.color)
+ assert torch.allclose(expected_tensor[:, 6], cam_points.height)
+
+ # test points clone
+ new_cam_points = cam_points.clone()
+ assert torch.allclose(new_cam_points.tensor, cam_points.tensor)
+
+ # test points shuffle
+ new_cam_points.shuffle()
+ assert new_cam_points.tensor.shape == torch.Size([4, 7])
+
+ # test points rotation
+ rot_mat = torch.tensor([[0.93629336, -0.27509585, 0.21835066],
+ [0.28962948, 0.95642509, -0.03695701],
+ [-0.19866933, 0.0978434, 0.97517033]])
+ cam_points.rotate(rot_mat)
+ expected_tensor = torch.tensor([[
+ 6.6239e+00, 3.9748e+01, -2.3335e+00, 6.6660e-01, 1.9560e-01,
+ 4.9740e-01, 9.4090e-01
+ ],
+ [
+ -2.3174e+01, 1.2600e+01, -6.9230e+00,
+ 1.5020e-01, 3.7070e-01, 1.0860e-01,
+ 6.2970e-01
+ ],
+ [
+ 4.7760e+00, 3.5484e+01, -2.3813e+00,
+ 6.5650e-01, 6.2480e-01, 6.9540e-01,
+ 2.5380e-01
+ ],
+ [
+ -2.8960e+01, 9.6364e+00, -7.0663e+00,
+ 2.8030e-01, 2.5800e-02, 4.8960e-01,
+ 3.2690e-01
+ ]])
+ assert torch.allclose(expected_tensor, cam_points.tensor, 1e-3)
+
+ new_cam_points = cam_points.clone()
+ new_cam_points.rotate(0.1, axis=2)
+ expected_tensor = torch.tensor([[
+ 2.6226e+00, 4.0211e+01, -2.3335e+00, 6.6660e-01, 1.9560e-01,
+ 4.9740e-01, 9.4090e-01
+ ],
+ [
+ -2.4316e+01, 1.0224e+01, -6.9230e+00,
+ 1.5020e-01, 3.7070e-01, 1.0860e-01,
+ 6.2970e-01
+ ],
+ [
+ 1.2096e+00, 3.5784e+01, -2.3813e+00,
+ 6.5650e-01, 6.2480e-01, 6.9540e-01,
+ 2.5380e-01
+ ],
+ [
+ -2.9777e+01, 6.6971e+00, -7.0663e+00,
+ 2.8030e-01, 2.5800e-02, 4.8960e-01,
+ 3.2690e-01
+ ]])
+ assert torch.allclose(expected_tensor, new_cam_points.tensor, 1e-3)
+
+ # test points translation
+ translation_vector = torch.tensor([0.93629336, -0.27509585, 0.21835066])
+ cam_points.translate(translation_vector)
+ expected_tensor = torch.tensor([[
+ 7.5602e+00, 3.9473e+01, -2.1152e+00, 6.6660e-01, 1.9560e-01,
+ 4.9740e-01, 9.4090e-01
+ ],
+ [
+ -2.2237e+01, 1.2325e+01, -6.7046e+00,
+ 1.5020e-01, 3.7070e-01, 1.0860e-01,
+ 6.2970e-01
+ ],
+ [
+ 5.7123e+00, 3.5209e+01, -2.1629e+00,
+ 6.5650e-01, 6.2480e-01, 6.9540e-01,
+ 2.5380e-01
+ ],
+ [
+ -2.8023e+01, 9.3613e+00, -6.8480e+00,
+ 2.8030e-01, 2.5800e-02, 4.8960e-01,
+ 3.2690e-01
+ ]])
+ assert torch.allclose(expected_tensor, cam_points.tensor, 1e-4)
+
+ # test points filter
+ point_range = [-10, -40, -10, 10, 40, 10]
+ in_range_flags = cam_points.in_range_3d(point_range)
+ expected_flags = torch.tensor([True, False, True, False])
+ assert torch.all(in_range_flags == expected_flags)
+
+ # test points scale
+ cam_points.scale(1.2)
+ expected_tensor = torch.tensor([[
+ 9.0722e+00, 4.7368e+01, -2.5382e+00, 6.6660e-01, 1.9560e-01,
+ 4.9740e-01, 9.4090e-01
+ ],
+ [
+ -2.6685e+01, 1.4790e+01, -8.0455e+00,
+ 1.5020e-01, 3.7070e-01, 1.0860e-01,
+ 6.2970e-01
+ ],
+ [
+ 6.8547e+00, 4.2251e+01, -2.5955e+00,
+ 6.5650e-01, 6.2480e-01, 6.9540e-01,
+ 2.5380e-01
+ ],
+ [
+ -3.3628e+01, 1.1234e+01, -8.2176e+00,
+ 2.8030e-01, 2.5800e-02, 4.8960e-01,
+ 3.2690e-01
+ ]])
+ assert torch.allclose(expected_tensor, cam_points.tensor, 1e-3)
+
+ # test get_item
+ expected_tensor = torch.tensor(
+ [[-26.6848, 14.7898, -8.0455, 0.1502, 0.3707, 0.1086, 0.6297]])
+ assert torch.allclose(expected_tensor, cam_points[1].tensor, 1e-4)
+ expected_tensor = torch.tensor(
+ [[-26.6848, 14.7898, -8.0455, 0.1502, 0.3707, 0.1086, 0.6297],
+ [6.8547, 42.2509, -2.5955, 0.6565, 0.6248, 0.6954, 0.2538]])
+ assert torch.allclose(expected_tensor, cam_points[1:3].tensor, 1e-4)
+ mask = torch.tensor([True, False, True, False])
+ expected_tensor = torch.tensor(
+ [[9.0722, 47.3678, -2.5382, 0.6666, 0.1956, 0.4974, 0.9409],
+ [6.8547, 42.2509, -2.5955, 0.6565, 0.6248, 0.6954, 0.2538]])
+ assert torch.allclose(expected_tensor, cam_points[mask].tensor, 1e-4)
+ expected_tensor = torch.tensor([[0.6666], [0.1502], [0.6565], [0.2803]])
+ assert torch.allclose(expected_tensor, cam_points[:, 3].tensor, 1e-4)
+
+ # test length
+ assert len(cam_points) == 4
+
+ # test repr
+ expected_repr = 'CameraPoints(\n '\
+ 'tensor([[ 9.0722e+00, 4.7368e+01, -2.5382e+00, '\
+ '6.6660e-01, 1.9560e-01,\n 4.9740e-01, '\
+ '9.4090e-01],\n '\
+ '[-2.6685e+01, 1.4790e+01, -8.0455e+00, 1.5020e-01, '\
+ '3.7070e-01,\n '\
+ '1.0860e-01, 6.2970e-01],\n '\
+ '[ 6.8547e+00, 4.2251e+01, -2.5955e+00, 6.5650e-01, '\
+ '6.2480e-01,\n '\
+ '6.9540e-01, 2.5380e-01],\n '\
+ '[-3.3628e+01, 1.1234e+01, -8.2176e+00, 2.8030e-01, '\
+ '2.5800e-02,\n '\
+ '4.8960e-01, 3.2690e-01]]))'
+ assert expected_repr == str(cam_points)
+
+ # test concatenate
+ cam_points_clone = cam_points.clone()
+ cat_points = CameraPoints.cat([cam_points, cam_points_clone])
+ assert torch.allclose(cat_points.tensor[:len(cam_points)],
+ cam_points.tensor)
+
+ # test iteration
+ for i, point in enumerate(cam_points):
+ assert torch.allclose(point, cam_points.tensor[i])
+
+ # test new_point
+ new_points = cam_points.new_point([[1, 2, 3, 4, 5, 6, 7]])
+ assert torch.allclose(
+ new_points.tensor,
+ torch.tensor([[1, 2, 3, 4, 5, 6, 7]], dtype=cam_points.tensor.dtype))
+
+ # test in_range_bev
+ point_bev_range = [-10, -10, 10, 10]
+ in_range_flags = cam_points.in_range_bev(point_bev_range)
+ expected_flags = torch.tensor([True, False, True, False])
+ assert torch.all(in_range_flags == expected_flags)
+
+ # test flip
+ cam_points.flip(bev_direction='horizontal')
+ expected_tensor = torch.tensor([[
+ -9.0722e+00, 4.7368e+01, -2.5382e+00, 6.6660e-01, 1.9560e-01,
+ 4.9740e-01, 9.4090e-01
+ ],
+ [
+ 2.6685e+01, 1.4790e+01, -8.0455e+00,
+ 1.5020e-01, 3.7070e-01, 1.0860e-01,
+ 6.2970e-01
+ ],
+ [
+ -6.8547e+00, 4.2251e+01, -2.5955e+00,
+ 6.5650e-01, 6.2480e-01, 6.9540e-01,
+ 2.5380e-01
+ ],
+ [
+ 3.3628e+01, 1.1234e+01, -8.2176e+00,
+ 2.8030e-01, 2.5800e-02, 4.8960e-01,
+ 3.2690e-01
+ ]])
+ assert torch.allclose(expected_tensor, cam_points.tensor, 1e-4)
+
+ cam_points.flip(bev_direction='vertical')
+ expected_tensor = torch.tensor([[
+ -9.0722e+00, 4.7368e+01, 2.5382e+00, 6.6660e-01, 1.9560e-01,
+ 4.9740e-01, 9.4090e-01
+ ],
+ [
+ 2.6685e+01, 1.4790e+01, 8.0455e+00,
+ 1.5020e-01, 3.7070e-01, 1.0860e-01,
+ 6.2970e-01
+ ],
+ [
+ -6.8547e+00, 4.2251e+01, 2.5955e+00,
+ 6.5650e-01, 6.2480e-01, 6.9540e-01,
+ 2.5380e-01
+ ],
+ [
+ 3.3628e+01, 1.1234e+01, 8.2176e+00,
+ 2.8030e-01, 2.5800e-02, 4.8960e-01,
+ 3.2690e-01
+ ]])
+ assert torch.allclose(expected_tensor, cam_points.tensor, 1e-4)
+
+
+def test_lidar_points():
+ # test empty initialization
+ empty_boxes = []
+ points = LiDARPoints(empty_boxes)
+ assert points.tensor.shape[0] == 0
+ assert points.tensor.shape[1] == 3
+
+ # Test init with origin
+ points_np = np.array([[-5.24223238e+00, 4.00209696e+01, 2.97570381e-01],
+ [-2.66751588e+01, 5.59499564e+00, -9.14345860e-01],
+ [-5.80979675e+00, 3.54092357e+01, 2.00889888e-01],
+ [-3.13086877e+01, 1.09007628e+00, -1.94612112e-01]],
+ dtype=np.float32)
+ lidar_points = LiDARPoints(points_np, points_dim=3)
+ assert lidar_points.tensor.shape[0] == 4
+
+ # Test init with color and height
+ points_np = np.array([[
+ -5.24223238e+00, 4.00209696e+01, 2.97570381e-01, 0.6666, 0.1956,
+ 0.4974, 0.9409
+ ],
+ [
+ -2.66751588e+01, 5.59499564e+00, -9.14345860e-01,
+ 0.1502, 0.3707, 0.1086, 0.6297
+ ],
+ [
+ -5.80979675e+00, 3.54092357e+01, 2.00889888e-01,
+ 0.6565, 0.6248, 0.6954, 0.2538
+ ],
+ [
+ -3.13086877e+01, 1.09007628e+00, -1.94612112e-01,
+ 0.2803, 0.0258, 0.4896, 0.3269
+ ]],
+ dtype=np.float32)
+ lidar_points = LiDARPoints(
+ points_np,
+ points_dim=7,
+ attribute_dims=dict(color=[3, 4, 5], height=6))
+ expected_tensor = torch.tensor([[
+ -5.24223238e+00, 4.00209696e+01, 2.97570381e-01, 0.6666, 0.1956,
+ 0.4974, 0.9409
+ ],
+ [
+ -2.66751588e+01, 5.59499564e+00,
+ -9.14345860e-01, 0.1502, 0.3707,
+ 0.1086, 0.6297
+ ],
+ [
+ -5.80979675e+00, 3.54092357e+01,
+ 2.00889888e-01, 0.6565, 0.6248, 0.6954,
+ 0.2538
+ ],
+ [
+ -3.13086877e+01, 1.09007628e+00,
+ -1.94612112e-01, 0.2803, 0.0258,
+ 0.4896, 0.3269
+ ]])
+
+ assert torch.allclose(expected_tensor, lidar_points.tensor)
+ assert torch.allclose(expected_tensor[:, :2], lidar_points.bev)
+ assert torch.allclose(expected_tensor[:, :3], lidar_points.coord)
+ assert torch.allclose(expected_tensor[:, 3:6], lidar_points.color)
+ assert torch.allclose(expected_tensor[:, 6], lidar_points.height)
+
+ # test points clone
+ new_lidar_points = lidar_points.clone()
+ assert torch.allclose(new_lidar_points.tensor, lidar_points.tensor)
+
+ # test points shuffle
+ new_lidar_points.shuffle()
+ assert new_lidar_points.tensor.shape == torch.Size([4, 7])
+
+ # test points rotation
+ rot_mat = torch.tensor([[0.93629336, -0.27509585, 0.21835066],
+ [0.28962948, 0.95642509, -0.03695701],
+ [-0.19866933, 0.0978434, 0.97517033]])
+ lidar_points.rotate(rot_mat)
+ expected_tensor = torch.tensor([[
+ 6.6239e+00, 3.9748e+01, -2.3335e+00, 6.6660e-01, 1.9560e-01,
+ 4.9740e-01, 9.4090e-01
+ ],
+ [
+ -2.3174e+01, 1.2600e+01, -6.9230e+00,
+ 1.5020e-01, 3.7070e-01, 1.0860e-01,
+ 6.2970e-01
+ ],
+ [
+ 4.7760e+00, 3.5484e+01, -2.3813e+00,
+ 6.5650e-01, 6.2480e-01, 6.9540e-01,
+ 2.5380e-01
+ ],
+ [
+ -2.8960e+01, 9.6364e+00, -7.0663e+00,
+ 2.8030e-01, 2.5800e-02, 4.8960e-01,
+ 3.2690e-01
+ ]])
+ assert torch.allclose(expected_tensor, lidar_points.tensor, 1e-3)
+
+ new_lidar_points = lidar_points.clone()
+ new_lidar_points.rotate(0.1, axis=2)
+ expected_tensor = torch.tensor([[
+ 2.6226e+00, 4.0211e+01, -2.3335e+00, 6.6660e-01, 1.9560e-01,
+ 4.9740e-01, 9.4090e-01
+ ],
+ [
+ -2.4316e+01, 1.0224e+01, -6.9230e+00,
+ 1.5020e-01, 3.7070e-01, 1.0860e-01,
+ 6.2970e-01
+ ],
+ [
+ 1.2096e+00, 3.5784e+01, -2.3813e+00,
+ 6.5650e-01, 6.2480e-01, 6.9540e-01,
+ 2.5380e-01
+ ],
+ [
+ -2.9777e+01, 6.6971e+00, -7.0663e+00,
+ 2.8030e-01, 2.5800e-02, 4.8960e-01,
+ 3.2690e-01
+ ]])
+ assert torch.allclose(expected_tensor, new_lidar_points.tensor, 1e-3)
+
+ # test points translation
+ translation_vector = torch.tensor([0.93629336, -0.27509585, 0.21835066])
+ lidar_points.translate(translation_vector)
+ expected_tensor = torch.tensor([[
+ 7.5602e+00, 3.9473e+01, -2.1152e+00, 6.6660e-01, 1.9560e-01,
+ 4.9740e-01, 9.4090e-01
+ ],
+ [
+ -2.2237e+01, 1.2325e+01, -6.7046e+00,
+ 1.5020e-01, 3.7070e-01, 1.0860e-01,
+ 6.2970e-01
+ ],
+ [
+ 5.7123e+00, 3.5209e+01, -2.1629e+00,
+ 6.5650e-01, 6.2480e-01, 6.9540e-01,
+ 2.5380e-01
+ ],
+ [
+ -2.8023e+01, 9.3613e+00, -6.8480e+00,
+ 2.8030e-01, 2.5800e-02, 4.8960e-01,
+ 3.2690e-01
+ ]])
+ assert torch.allclose(expected_tensor, lidar_points.tensor, 1e-4)
+
+ # test points filter
+ point_range = [-10, -40, -10, 10, 40, 10]
+ in_range_flags = lidar_points.in_range_3d(point_range)
+ expected_flags = torch.tensor([True, False, True, False])
+ assert torch.all(in_range_flags == expected_flags)
+
+ # test points scale
+ lidar_points.scale(1.2)
+ expected_tensor = torch.tensor([[
+ 9.0722e+00, 4.7368e+01, -2.5382e+00, 6.6660e-01, 1.9560e-01,
+ 4.9740e-01, 9.4090e-01
+ ],
+ [
+ -2.6685e+01, 1.4790e+01, -8.0455e+00,
+ 1.5020e-01, 3.7070e-01, 1.0860e-01,
+ 6.2970e-01
+ ],
+ [
+ 6.8547e+00, 4.2251e+01, -2.5955e+00,
+ 6.5650e-01, 6.2480e-01, 6.9540e-01,
+ 2.5380e-01
+ ],
+ [
+ -3.3628e+01, 1.1234e+01, -8.2176e+00,
+ 2.8030e-01, 2.5800e-02, 4.8960e-01,
+ 3.2690e-01
+ ]])
+ assert torch.allclose(expected_tensor, lidar_points.tensor, 1e-3)
+
+ # test get_item
+ expected_tensor = torch.tensor(
+ [[-26.6848, 14.7898, -8.0455, 0.1502, 0.3707, 0.1086, 0.6297]])
+ assert torch.allclose(expected_tensor, lidar_points[1].tensor, 1e-4)
+ expected_tensor = torch.tensor(
+ [[-26.6848, 14.7898, -8.0455, 0.1502, 0.3707, 0.1086, 0.6297],
+ [6.8547, 42.2509, -2.5955, 0.6565, 0.6248, 0.6954, 0.2538]])
+ assert torch.allclose(expected_tensor, lidar_points[1:3].tensor, 1e-4)
+ mask = torch.tensor([True, False, True, False])
+ expected_tensor = torch.tensor(
+ [[9.0722, 47.3678, -2.5382, 0.6666, 0.1956, 0.4974, 0.9409],
+ [6.8547, 42.2509, -2.5955, 0.6565, 0.6248, 0.6954, 0.2538]])
+ assert torch.allclose(expected_tensor, lidar_points[mask].tensor, 1e-4)
+ expected_tensor = torch.tensor([[0.6666], [0.1502], [0.6565], [0.2803]])
+ assert torch.allclose(expected_tensor, lidar_points[:, 3].tensor, 1e-4)
+
+ # test length
+ assert len(lidar_points) == 4
+
+ # test repr
+ expected_repr = 'LiDARPoints(\n '\
+ 'tensor([[ 9.0722e+00, 4.7368e+01, -2.5382e+00, '\
+ '6.6660e-01, 1.9560e-01,\n 4.9740e-01, '\
+ '9.4090e-01],\n '\
+ '[-2.6685e+01, 1.4790e+01, -8.0455e+00, 1.5020e-01, '\
+ '3.7070e-01,\n '\
+ '1.0860e-01, 6.2970e-01],\n '\
+ '[ 6.8547e+00, 4.2251e+01, -2.5955e+00, 6.5650e-01, '\
+ '6.2480e-01,\n '\
+ '6.9540e-01, 2.5380e-01],\n '\
+ '[-3.3628e+01, 1.1234e+01, -8.2176e+00, 2.8030e-01, '\
+ '2.5800e-02,\n '\
+ '4.8960e-01, 3.2690e-01]]))'
+ assert expected_repr == str(lidar_points)
+
+ # test concatenate
+ lidar_points_clone = lidar_points.clone()
+ cat_points = LiDARPoints.cat([lidar_points, lidar_points_clone])
+ assert torch.allclose(cat_points.tensor[:len(lidar_points)],
+ lidar_points.tensor)
+
+ # test iteration
+ for i, point in enumerate(lidar_points):
+ assert torch.allclose(point, lidar_points.tensor[i])
+
+ # test new_point
+ new_points = lidar_points.new_point([[1, 2, 3, 4, 5, 6, 7]])
+ assert torch.allclose(
+ new_points.tensor,
+ torch.tensor([[1, 2, 3, 4, 5, 6, 7]], dtype=lidar_points.tensor.dtype))
+
+ # test in_range_bev
+ point_bev_range = [-30, -40, 30, 40]
+ in_range_flags = lidar_points.in_range_bev(point_bev_range)
+ expected_flags = torch.tensor([False, True, False, False])
+ assert torch.all(in_range_flags == expected_flags)
+
+ # test flip
+ lidar_points.flip(bev_direction='horizontal')
+ expected_tensor = torch.tensor([[
+ 9.0722e+00, -4.7368e+01, -2.5382e+00, 6.6660e-01, 1.9560e-01,
+ 4.9740e-01, 9.4090e-01
+ ],
+ [
+ -2.6685e+01, -1.4790e+01, -8.0455e+00,
+ 1.5020e-01, 3.7070e-01, 1.0860e-01,
+ 6.2970e-01
+ ],
+ [
+ 6.8547e+00, -4.2251e+01, -2.5955e+00,
+ 6.5650e-01, 6.2480e-01, 6.9540e-01,
+ 2.5380e-01
+ ],
+ [
+ -3.3628e+01, -1.1234e+01, -8.2176e+00,
+ 2.8030e-01, 2.5800e-02, 4.8960e-01,
+ 3.2690e-01
+ ]])
+ assert torch.allclose(expected_tensor, lidar_points.tensor, 1e-4)
+
+ lidar_points.flip(bev_direction='vertical')
+ expected_tensor = torch.tensor([[
+ -9.0722e+00, -4.7368e+01, -2.5382e+00, 6.6660e-01, 1.9560e-01,
+ 4.9740e-01, 9.4090e-01
+ ],
+ [
+ 2.6685e+01, -1.4790e+01, -8.0455e+00,
+ 1.5020e-01, 3.7070e-01, 1.0860e-01,
+ 6.2970e-01
+ ],
+ [
+ -6.8547e+00, -4.2251e+01, -2.5955e+00,
+ 6.5650e-01, 6.2480e-01, 6.9540e-01,
+ 2.5380e-01
+ ],
+ [
+ 3.3628e+01, -1.1234e+01, -8.2176e+00,
+ 2.8030e-01, 2.5800e-02, 4.8960e-01,
+ 3.2690e-01
+ ]])
+ assert torch.allclose(expected_tensor, lidar_points.tensor, 1e-4)
+
+
+def test_depth_points():
+ # test empty initialization
+ empty_boxes = []
+ points = DepthPoints(empty_boxes)
+ assert points.tensor.shape[0] == 0
+ assert points.tensor.shape[1] == 3
+
+ # Test init with origin
+ points_np = np.array([[-5.24223238e+00, 4.00209696e+01, 2.97570381e-01],
+ [-2.66751588e+01, 5.59499564e+00, -9.14345860e-01],
+ [-5.80979675e+00, 3.54092357e+01, 2.00889888e-01],
+ [-3.13086877e+01, 1.09007628e+00, -1.94612112e-01]],
+ dtype=np.float32)
+ depth_points = DepthPoints(points_np, points_dim=3)
+ assert depth_points.tensor.shape[0] == 4
+
+ # Test init with color and height
+ points_np = np.array([[
+ -5.24223238e+00, 4.00209696e+01, 2.97570381e-01, 0.6666, 0.1956,
+ 0.4974, 0.9409
+ ],
+ [
+ -2.66751588e+01, 5.59499564e+00, -9.14345860e-01,
+ 0.1502, 0.3707, 0.1086, 0.6297
+ ],
+ [
+ -5.80979675e+00, 3.54092357e+01, 2.00889888e-01,
+ 0.6565, 0.6248, 0.6954, 0.2538
+ ],
+ [
+ -3.13086877e+01, 1.09007628e+00, -1.94612112e-01,
+ 0.2803, 0.0258, 0.4896, 0.3269
+ ]],
+ dtype=np.float32)
+ depth_points = DepthPoints(
+ points_np,
+ points_dim=7,
+ attribute_dims=dict(color=[3, 4, 5], height=6))
+ expected_tensor = torch.tensor([[
+ -5.24223238e+00, 4.00209696e+01, 2.97570381e-01, 0.6666, 0.1956,
+ 0.4974, 0.9409
+ ],
+ [
+ -2.66751588e+01, 5.59499564e+00,
+ -9.14345860e-01, 0.1502, 0.3707,
+ 0.1086, 0.6297
+ ],
+ [
+ -5.80979675e+00, 3.54092357e+01,
+ 2.00889888e-01, 0.6565, 0.6248, 0.6954,
+ 0.2538
+ ],
+ [
+ -3.13086877e+01, 1.09007628e+00,
+ -1.94612112e-01, 0.2803, 0.0258,
+ 0.4896, 0.3269
+ ]])
+
+ assert torch.allclose(expected_tensor, depth_points.tensor)
+ assert torch.allclose(expected_tensor[:, :2], depth_points.bev)
+ assert torch.allclose(expected_tensor[:, :3], depth_points.coord)
+ assert torch.allclose(expected_tensor[:, 3:6], depth_points.color)
+ assert torch.allclose(expected_tensor[:, 6], depth_points.height)
+
+ # test points clone
+ new_depth_points = depth_points.clone()
+ assert torch.allclose(new_depth_points.tensor, depth_points.tensor)
+
+ # test points shuffle
+ new_depth_points.shuffle()
+ assert new_depth_points.tensor.shape == torch.Size([4, 7])
+
+ # test points rotation
+ rot_mat = torch.tensor([[0.93629336, -0.27509585, 0.21835066],
+ [0.28962948, 0.95642509, -0.03695701],
+ [-0.19866933, 0.0978434, 0.97517033]])
+ depth_points.rotate(rot_mat)
+ expected_tensor = torch.tensor([[
+ 6.6239e+00, 3.9748e+01, -2.3335e+00, 6.6660e-01, 1.9560e-01,
+ 4.9740e-01, 9.4090e-01
+ ],
+ [
+ -2.3174e+01, 1.2600e+01, -6.9230e+00,
+ 1.5020e-01, 3.7070e-01, 1.0860e-01,
+ 6.2970e-01
+ ],
+ [
+ 4.7760e+00, 3.5484e+01, -2.3813e+00,
+ 6.5650e-01, 6.2480e-01, 6.9540e-01,
+ 2.5380e-01
+ ],
+ [
+ -2.8960e+01, 9.6364e+00, -7.0663e+00,
+ 2.8030e-01, 2.5800e-02, 4.8960e-01,
+ 3.2690e-01
+ ]])
+ assert torch.allclose(expected_tensor, depth_points.tensor, 1e-3)
+
+ new_depth_points = depth_points.clone()
+ new_depth_points.rotate(0.1, axis=2)
+ expected_tensor = torch.tensor([[
+ 2.6226e+00, 4.0211e+01, -2.3335e+00, 6.6660e-01, 1.9560e-01,
+ 4.9740e-01, 9.4090e-01
+ ],
+ [
+ -2.4316e+01, 1.0224e+01, -6.9230e+00,
+ 1.5020e-01, 3.7070e-01, 1.0860e-01,
+ 6.2970e-01
+ ],
+ [
+ 1.2096e+00, 3.5784e+01, -2.3813e+00,
+ 6.5650e-01, 6.2480e-01, 6.9540e-01,
+ 2.5380e-01
+ ],
+ [
+ -2.9777e+01, 6.6971e+00, -7.0663e+00,
+ 2.8030e-01, 2.5800e-02, 4.8960e-01,
+ 3.2690e-01
+ ]])
+ assert torch.allclose(expected_tensor, new_depth_points.tensor, 1e-3)
+
+ # test points translation
+ translation_vector = torch.tensor([0.93629336, -0.27509585, 0.21835066])
+ depth_points.translate(translation_vector)
+ expected_tensor = torch.tensor([[
+ 7.5602e+00, 3.9473e+01, -2.1152e+00, 6.6660e-01, 1.9560e-01,
+ 4.9740e-01, 9.4090e-01
+ ],
+ [
+ -2.2237e+01, 1.2325e+01, -6.7046e+00,
+ 1.5020e-01, 3.7070e-01, 1.0860e-01,
+ 6.2970e-01
+ ],
+ [
+ 5.7123e+00, 3.5209e+01, -2.1629e+00,
+ 6.5650e-01, 6.2480e-01, 6.9540e-01,
+ 2.5380e-01
+ ],
+ [
+ -2.8023e+01, 9.3613e+00, -6.8480e+00,
+ 2.8030e-01, 2.5800e-02, 4.8960e-01,
+ 3.2690e-01
+ ]])
+ assert torch.allclose(expected_tensor, depth_points.tensor, 1e-4)
+
+ # test points filter
+ point_range = [-10, -40, -10, 10, 40, 10]
+ in_range_flags = depth_points.in_range_3d(point_range)
+ expected_flags = torch.tensor([True, False, True, False])
+ assert torch.all(in_range_flags == expected_flags)
+
+ # test points scale
+ depth_points.scale(1.2)
+ expected_tensor = torch.tensor([[
+ 9.0722e+00, 4.7368e+01, -2.5382e+00, 6.6660e-01, 1.9560e-01,
+ 4.9740e-01, 9.4090e-01
+ ],
+ [
+ -2.6685e+01, 1.4790e+01, -8.0455e+00,
+ 1.5020e-01, 3.7070e-01, 1.0860e-01,
+ 6.2970e-01
+ ],
+ [
+ 6.8547e+00, 4.2251e+01, -2.5955e+00,
+ 6.5650e-01, 6.2480e-01, 6.9540e-01,
+ 2.5380e-01
+ ],
+ [
+ -3.3628e+01, 1.1234e+01, -8.2176e+00,
+ 2.8030e-01, 2.5800e-02, 4.8960e-01,
+ 3.2690e-01
+ ]])
+ assert torch.allclose(expected_tensor, depth_points.tensor, 1e-3)
+
+ # test get_item
+ expected_tensor = torch.tensor(
+ [[-26.6848, 14.7898, -8.0455, 0.1502, 0.3707, 0.1086, 0.6297]])
+ assert torch.allclose(expected_tensor, depth_points[1].tensor, 1e-4)
+ expected_tensor = torch.tensor(
+ [[-26.6848, 14.7898, -8.0455, 0.1502, 0.3707, 0.1086, 0.6297],
+ [6.8547, 42.2509, -2.5955, 0.6565, 0.6248, 0.6954, 0.2538]])
+ assert torch.allclose(expected_tensor, depth_points[1:3].tensor, 1e-4)
+ mask = torch.tensor([True, False, True, False])
+ expected_tensor = torch.tensor(
+ [[9.0722, 47.3678, -2.5382, 0.6666, 0.1956, 0.4974, 0.9409],
+ [6.8547, 42.2509, -2.5955, 0.6565, 0.6248, 0.6954, 0.2538]])
+ assert torch.allclose(expected_tensor, depth_points[mask].tensor, 1e-4)
+ expected_tensor = torch.tensor([[0.6666], [0.1502], [0.6565], [0.2803]])
+ assert torch.allclose(expected_tensor, depth_points[:, 3].tensor, 1e-4)
+
+ # test length
+ assert len(depth_points) == 4
+
+ # test repr
+ expected_repr = 'DepthPoints(\n '\
+ 'tensor([[ 9.0722e+00, 4.7368e+01, -2.5382e+00, '\
+ '6.6660e-01, 1.9560e-01,\n 4.9740e-01, '\
+ '9.4090e-01],\n '\
+ '[-2.6685e+01, 1.4790e+01, -8.0455e+00, 1.5020e-01, '\
+ '3.7070e-01,\n '\
+ '1.0860e-01, 6.2970e-01],\n '\
+ '[ 6.8547e+00, 4.2251e+01, -2.5955e+00, 6.5650e-01, '\
+ '6.2480e-01,\n '\
+ '6.9540e-01, 2.5380e-01],\n '\
+ '[-3.3628e+01, 1.1234e+01, -8.2176e+00, 2.8030e-01, '\
+ '2.5800e-02,\n '\
+ '4.8960e-01, 3.2690e-01]]))'
+ assert expected_repr == str(depth_points)
+
+ # test concatenate
+ depth_points_clone = depth_points.clone()
+ cat_points = DepthPoints.cat([depth_points, depth_points_clone])
+ assert torch.allclose(cat_points.tensor[:len(depth_points)],
+ depth_points.tensor)
+
+ # test iteration
+ for i, point in enumerate(depth_points):
+ assert torch.allclose(point, depth_points.tensor[i])
+
+ # test new_point
+ new_points = depth_points.new_point([[1, 2, 3, 4, 5, 6, 7]])
+ assert torch.allclose(
+ new_points.tensor,
+ torch.tensor([[1, 2, 3, 4, 5, 6, 7]], dtype=depth_points.tensor.dtype))
+
+ # test in_range_bev
+ point_bev_range = [-30, -40, 30, 40]
+ in_range_flags = depth_points.in_range_bev(point_bev_range)
+ expected_flags = torch.tensor([False, True, False, False])
+ assert torch.all(in_range_flags == expected_flags)
+
+ # test flip
+ depth_points.flip(bev_direction='horizontal')
+ expected_tensor = torch.tensor([[
+ -9.0722e+00, 4.7368e+01, -2.5382e+00, 6.6660e-01, 1.9560e-01,
+ 4.9740e-01, 9.4090e-01
+ ],
+ [
+ 2.6685e+01, 1.4790e+01, -8.0455e+00,
+ 1.5020e-01, 3.7070e-01, 1.0860e-01,
+ 6.2970e-01
+ ],
+ [
+ -6.8547e+00, 4.2251e+01, -2.5955e+00,
+ 6.5650e-01, 6.2480e-01, 6.9540e-01,
+ 2.5380e-01
+ ],
+ [
+ 3.3628e+01, 1.1234e+01, -8.2176e+00,
+ 2.8030e-01, 2.5800e-02, 4.8960e-01,
+ 3.2690e-01
+ ]])
+ assert torch.allclose(expected_tensor, depth_points.tensor, 1e-4)
+
+ depth_points.flip(bev_direction='vertical')
+ expected_tensor = torch.tensor([[
+ -9.0722e+00, -4.7368e+01, -2.5382e+00, 6.6660e-01, 1.9560e-01,
+ 4.9740e-01, 9.4090e-01
+ ],
+ [
+ 2.6685e+01, -1.4790e+01, -8.0455e+00,
+ 1.5020e-01, 3.7070e-01, 1.0860e-01,
+ 6.2970e-01
+ ],
+ [
+ -6.8547e+00, -4.2251e+01, -2.5955e+00,
+ 6.5650e-01, 6.2480e-01, 6.9540e-01,
+ 2.5380e-01
+ ],
+ [
+ 3.3628e+01, -1.1234e+01, -8.2176e+00,
+ 2.8030e-01, 2.5800e-02, 4.8960e-01,
+ 3.2690e-01
+ ]])
+ assert torch.allclose(expected_tensor, depth_points.tensor, 1e-4)
diff --git a/tests/test_utils/test_samplers.py b/tests/test_utils/test_samplers.py
new file mode 100644
index 0000000..236a6bd
--- /dev/null
+++ b/tests/test_utils/test_samplers.py
@@ -0,0 +1,44 @@
+# Copyright (c) OpenMMLab. All rights reserved.
+import pytest
+import torch
+
+from mmdet3d.core.bbox.assigners import MaxIoUAssigner
+from mmdet3d.core.bbox.samplers import IoUNegPiecewiseSampler
+
+
+def test_iou_piecewise_sampler():
+ if not torch.cuda.is_available():
+ pytest.skip()
+ assigner = MaxIoUAssigner(
+ pos_iou_thr=0.55,
+ neg_iou_thr=0.55,
+ min_pos_iou=0.55,
+ ignore_iof_thr=-1,
+ iou_calculator=dict(type='BboxOverlaps3D', coordinate='lidar'))
+ bboxes = torch.tensor(
+ [[32, 32, 16, 8, 38, 42, -0.3], [32, 32, 16, 8, 38, 42, -0.3],
+ [32, 32, 16, 8, 38, 42, -0.3], [32, 32, 16, 8, 38, 42, -0.3],
+ [0, 0, 0, 10, 10, 10, 0.2], [10, 10, 10, 20, 20, 15, 0.6],
+ [5, 5, 5, 15, 15, 15, 0.7], [5, 5, 5, 15, 15, 15, 0.7],
+ [5, 5, 5, 15, 15, 15, 0.7], [32, 32, 16, 8, 38, 42, -0.3],
+ [32, 32, 16, 8, 38, 42, -0.3], [32, 32, 16, 8, 38, 42, -0.3]],
+ dtype=torch.float32).cuda()
+ gt_bboxes = torch.tensor(
+ [[0, 0, 0, 10, 10, 9, 0.2], [5, 10, 10, 20, 20, 15, 0.6]],
+ dtype=torch.float32).cuda()
+ gt_labels = torch.tensor([1, 1], dtype=torch.int64).cuda()
+ assign_result = assigner.assign(bboxes, gt_bboxes, gt_labels=gt_labels)
+
+ sampler = IoUNegPiecewiseSampler(
+ num=10,
+ pos_fraction=0.55,
+ neg_piece_fractions=[0.8, 0.2],
+ neg_iou_piece_thrs=[0.55, 0.1],
+ neg_pos_ub=-1,
+ add_gt_as_proposals=False)
+
+ sample_result = sampler.sample(assign_result, bboxes, gt_bboxes, gt_labels)
+
+ assert sample_result.pos_inds == 4
+ assert len(sample_result.pos_bboxes) == len(sample_result.pos_inds)
+ assert len(sample_result.neg_bboxes) == len(sample_result.neg_inds)
diff --git a/tests/test_utils/test_setup_env.py b/tests/test_utils/test_setup_env.py
new file mode 100644
index 0000000..0c070c9
--- /dev/null
+++ b/tests/test_utils/test_setup_env.py
@@ -0,0 +1,68 @@
+# Copyright (c) OpenMMLab. All rights reserved.
+import multiprocessing as mp
+import os
+import platform
+
+import cv2
+from mmcv import Config
+
+from mmdet3d.utils import setup_multi_processes
+
+
+def test_setup_multi_processes():
+ # temp save system setting
+ sys_start_mehod = mp.get_start_method(allow_none=True)
+ sys_cv_threads = cv2.getNumThreads()
+ # pop and temp save system env vars
+ sys_omp_threads = os.environ.pop('OMP_NUM_THREADS', default=None)
+ sys_mkl_threads = os.environ.pop('MKL_NUM_THREADS', default=None)
+
+ # test config without setting env
+ config = dict(data=dict(workers_per_gpu=2))
+ cfg = Config(config)
+ setup_multi_processes(cfg)
+ assert os.getenv('OMP_NUM_THREADS') == '1'
+ assert os.getenv('MKL_NUM_THREADS') == '1'
+ # when set to 0, the num threads will be 1
+ assert cv2.getNumThreads() == 1
+ if platform.system() != 'Windows':
+ assert mp.get_start_method() == 'fork'
+
+ # test num workers <= 1
+ os.environ.pop('OMP_NUM_THREADS')
+ os.environ.pop('MKL_NUM_THREADS')
+ config = dict(data=dict(workers_per_gpu=0))
+ cfg = Config(config)
+ setup_multi_processes(cfg)
+ assert 'OMP_NUM_THREADS' not in os.environ
+ assert 'MKL_NUM_THREADS' not in os.environ
+
+ # test manually set env var
+ os.environ['OMP_NUM_THREADS'] = '4'
+ config = dict(data=dict(workers_per_gpu=2))
+ cfg = Config(config)
+ setup_multi_processes(cfg)
+ assert os.getenv('OMP_NUM_THREADS') == '4'
+
+ # test manually set opencv threads and mp start method
+ config = dict(
+ data=dict(workers_per_gpu=2),
+ opencv_num_threads=4,
+ mp_start_method='spawn')
+ cfg = Config(config)
+ setup_multi_processes(cfg)
+ assert cv2.getNumThreads() == 4
+ assert mp.get_start_method() == 'spawn'
+
+ # revert setting to avoid affecting other programs
+ if sys_start_mehod:
+ mp.set_start_method(sys_start_mehod, force=True)
+ cv2.setNumThreads(sys_cv_threads)
+ if sys_omp_threads:
+ os.environ['OMP_NUM_THREADS'] = sys_omp_threads
+ else:
+ os.environ.pop('OMP_NUM_THREADS')
+ if sys_mkl_threads:
+ os.environ['MKL_NUM_THREADS'] = sys_mkl_threads
+ else:
+ os.environ.pop('MKL_NUM_THREADS')
diff --git a/tests/test_utils/test_utils.py b/tests/test_utils/test_utils.py
new file mode 100644
index 0000000..c68e43a
--- /dev/null
+++ b/tests/test_utils/test_utils.py
@@ -0,0 +1,288 @@
+# Copyright (c) OpenMMLab. All rights reserved.
+import numpy as np
+import pytest
+import torch
+
+from mmdet3d.core import array_converter, draw_heatmap_gaussian, points_img2cam
+from mmdet3d.core.bbox import CameraInstance3DBoxes
+from mmdet3d.models.utils import (filter_outside_objs, get_edge_indices,
+ get_keypoints, handle_proj_objs)
+
+
+def test_gaussian():
+ heatmap = torch.zeros((128, 128))
+ ct_int = torch.tensor([64, 64], dtype=torch.int32)
+ radius = 2
+ draw_heatmap_gaussian(heatmap, ct_int, radius)
+ assert torch.isclose(torch.sum(heatmap), torch.tensor(4.3505), atol=1e-3)
+
+
+def test_array_converter():
+ # to torch
+ @array_converter(to_torch=True, apply_to=('array_a', 'array_b'))
+ def test_func_1(array_a, array_b, container):
+ container.append(array_a)
+ container.append(array_b)
+ return array_a.clone(), array_b.clone()
+
+ np_array_a = np.array([0.0])
+ np_array_b = np.array([0.0])
+ container = []
+ new_array_a, new_array_b = test_func_1(np_array_a, np_array_b, container)
+
+ assert isinstance(new_array_a, np.ndarray)
+ assert isinstance(new_array_b, np.ndarray)
+ assert isinstance(container[0], torch.Tensor)
+ assert isinstance(container[1], torch.Tensor)
+
+ # one to torch and one not
+ @array_converter(to_torch=True, apply_to=('array_a', ))
+ def test_func_2(array_a, array_b):
+ return torch.cat([array_a, array_b])
+
+ with pytest.raises(TypeError):
+ _ = test_func_2(np_array_a, np_array_b)
+
+ # wrong template_arg_name_
+ @array_converter(
+ to_torch=True, apply_to=('array_a', ), template_arg_name_='array_c')
+ def test_func_3(array_a, array_b):
+ return torch.cat([array_a, array_b])
+
+ with pytest.raises(ValueError):
+ _ = test_func_3(np_array_a, np_array_b)
+
+ # wrong apply_to
+ @array_converter(to_torch=True, apply_to=('array_a', 'array_c'))
+ def test_func_4(array_a, array_b):
+ return torch.cat([array_a, array_b])
+
+ with pytest.raises(ValueError):
+ _ = test_func_4(np_array_a, np_array_b)
+
+ # to numpy
+ @array_converter(to_torch=False, apply_to=('array_a', 'array_b'))
+ def test_func_5(array_a, array_b, container):
+ container.append(array_a)
+ container.append(array_b)
+ return array_a.copy(), array_b.copy()
+
+ pt_array_a = torch.tensor([0.0])
+ pt_array_b = torch.tensor([0.0])
+ container = []
+ new_array_a, new_array_b = test_func_5(pt_array_a, pt_array_b, container)
+
+ assert isinstance(container[0], np.ndarray)
+ assert isinstance(container[1], np.ndarray)
+ assert isinstance(new_array_a, torch.Tensor)
+ assert isinstance(new_array_b, torch.Tensor)
+
+ # apply_to = None
+ @array_converter(to_torch=False)
+ def test_func_6(array_a, array_b, container):
+ container.append(array_a)
+ container.append(array_b)
+ return array_a.clone(), array_b.clone()
+
+ container = []
+ new_array_a, new_array_b = test_func_6(pt_array_a, pt_array_b, container)
+
+ assert isinstance(container[0], torch.Tensor)
+ assert isinstance(container[1], torch.Tensor)
+ assert isinstance(new_array_a, torch.Tensor)
+ assert isinstance(new_array_b, torch.Tensor)
+
+ # with default arg
+ @array_converter(to_torch=True, apply_to=('array_a', 'array_b'))
+ def test_func_7(array_a, container, array_b=np.array([2.])):
+ container.append(array_a)
+ container.append(array_b)
+ return array_a.clone(), array_b.clone()
+
+ container = []
+ new_array_a, new_array_b = test_func_7(np_array_a, container)
+
+ assert isinstance(container[0], torch.Tensor)
+ assert isinstance(container[1], torch.Tensor)
+ assert isinstance(new_array_a, np.ndarray)
+ assert isinstance(new_array_b, np.ndarray)
+ assert np.allclose(new_array_b, np.array([2.]), 1e-3)
+
+ # override default arg
+
+ container = []
+ new_array_a, new_array_b = test_func_7(np_array_a, container,
+ np.array([4.]))
+
+ assert isinstance(container[0], torch.Tensor)
+ assert isinstance(container[1], torch.Tensor)
+ assert isinstance(new_array_a, np.ndarray)
+ assert np.allclose(new_array_b, np.array([4.]), 1e-3)
+
+ # list arg
+ @array_converter(to_torch=True, apply_to=('array_a', 'array_b'))
+ def test_func_8(container, array_a, array_b=[2.]):
+ container.append(array_a)
+ container.append(array_b)
+ return array_a.clone(), array_b.clone()
+
+ container = []
+ new_array_a, new_array_b = test_func_8(container, [3.])
+
+ assert isinstance(container[0], torch.Tensor)
+ assert isinstance(container[1], torch.Tensor)
+ assert np.allclose(new_array_a, np.array([3.]), 1e-3)
+ assert np.allclose(new_array_b, np.array([2.]), 1e-3)
+
+ # number arg
+ @array_converter(to_torch=True, apply_to=('array_a', 'array_b'))
+ def test_func_9(container, array_a, array_b=1):
+ container.append(array_a)
+ container.append(array_b)
+ return array_a.clone(), array_b.clone()
+
+ container = []
+ new_array_a, new_array_b = test_func_9(container, np_array_a)
+
+ assert isinstance(container[0], torch.FloatTensor)
+ assert isinstance(container[1], torch.FloatTensor)
+ assert np.allclose(new_array_a, np_array_a, 1e-3)
+ assert np.allclose(new_array_b, np.array(1.0), 1e-3)
+
+ # feed kwargs
+ container = []
+ kwargs = {'array_a': [5.], 'array_b': [6.]}
+ new_array_a, new_array_b = test_func_8(container, **kwargs)
+
+ assert isinstance(container[0], torch.Tensor)
+ assert isinstance(container[1], torch.Tensor)
+ assert np.allclose(new_array_a, np.array([5.]), 1e-3)
+ assert np.allclose(new_array_b, np.array([6.]), 1e-3)
+
+ # feed args and kwargs
+ container = []
+ kwargs = {'array_b': [7.]}
+ args = (container, [8.])
+ new_array_a, new_array_b = test_func_8(*args, **kwargs)
+
+ assert isinstance(container[0], torch.Tensor)
+ assert isinstance(container[1], torch.Tensor)
+ assert np.allclose(new_array_a, np.array([8.]), 1e-3)
+ assert np.allclose(new_array_b, np.array([7.]), 1e-3)
+
+ # wrong template arg type
+ with pytest.raises(TypeError):
+ new_array_a, new_array_b = test_func_9(container, 3 + 4j)
+
+ with pytest.raises(TypeError):
+ new_array_a, new_array_b = test_func_9(container, {})
+
+ # invalid template arg list
+ with pytest.raises(TypeError):
+ new_array_a, new_array_b = test_func_9(container,
+ [True, np.array([3.0])])
+
+
+def test_points_img2cam():
+ points = torch.tensor([[0.5764, 0.9109, 0.7576], [0.6656, 0.5498, 0.9813]])
+ cam2img = torch.tensor([[700., 0., 450., 0.], [0., 700., 200., 0.],
+ [0., 0., 1., 0.]])
+ xyzs = points_img2cam(points, cam2img)
+ expected_xyzs = torch.tensor([[-0.4864, -0.2155, 0.7576],
+ [-0.6299, -0.2796, 0.9813]])
+ assert torch.allclose(xyzs, expected_xyzs, atol=1e-3)
+
+
+def test_generate_edge_indices():
+
+ input_metas = [
+ dict(img_shape=(110, 110), pad_shape=(128, 128)),
+ dict(img_shape=(98, 110), pad_shape=(128, 128))
+ ]
+ downsample_ratio = 4
+ edge_indices_list = get_edge_indices(input_metas, downsample_ratio)
+
+ assert edge_indices_list[0].shape[0] == 108
+ assert edge_indices_list[1].shape[0] == 102
+
+
+def test_truncation_hanlde():
+
+ centers2d_list = [
+ torch.tensor([[-99.86, 199.45], [499.50, 399.20], [201.20, 99.86]])
+ ]
+
+ gt_bboxes_list = [
+ torch.tensor([[0.25, 99.8, 99.8, 199.6], [300.2, 250.1, 399.8, 299.6],
+ [100.2, 20.1, 300.8, 180.7]])
+ ]
+ img_metas = [dict(img_shape=[300, 400])]
+ centers2d_target_list, offsets2d_list, trunc_mask_list = \
+ handle_proj_objs(centers2d_list, gt_bboxes_list, img_metas)
+
+ centers2d_target = torch.tensor([[0., 166.30435501], [379.03437877, 299.],
+ [201.2, 99.86]])
+
+ offsets2d = torch.tensor([[-99.86, 33.45], [120.5, 100.2], [0.2, -0.14]])
+ trunc_mask = torch.tensor([True, True, False])
+
+ assert torch.allclose(centers2d_target_list[0], centers2d_target)
+ assert torch.allclose(offsets2d_list[0], offsets2d, atol=1e-4)
+ assert torch.all(trunc_mask_list[0] == trunc_mask)
+ assert torch.allclose(
+ centers2d_target_list[0].round().int() + offsets2d_list[0],
+ centers2d_list[0])
+
+
+def test_filter_outside_objs():
+
+ centers2d_list = [
+ torch.tensor([[-99.86, 199.45], [499.50, 399.20], [201.20, 99.86]]),
+ torch.tensor([[-47.86, 199.45], [410.50, 399.20], [401.20, 349.86]])
+ ]
+ gt_bboxes_list = [
+ torch.rand([3, 4], dtype=torch.float32),
+ torch.rand([3, 4], dtype=torch.float32)
+ ]
+ gt_bboxes_3d_list = [
+ CameraInstance3DBoxes(torch.rand([3, 7]), box_dim=7),
+ CameraInstance3DBoxes(torch.rand([3, 7]), box_dim=7)
+ ]
+ gt_labels_list = [torch.tensor([0, 1, 2]), torch.tensor([2, 0, 0])]
+ gt_labels_3d_list = [torch.tensor([0, 1, 2]), torch.tensor([2, 0, 0])]
+ img_metas = [dict(img_shape=[300, 400]), dict(img_shape=[500, 450])]
+ filter_outside_objs(gt_bboxes_list, gt_labels_list, gt_bboxes_3d_list,
+ gt_labels_3d_list, centers2d_list, img_metas)
+
+ assert len(centers2d_list[0]) == len(gt_bboxes_3d_list[0]) == \
+ len(gt_bboxes_list[0]) == len(gt_labels_3d_list[0]) == \
+ len(gt_labels_list[0]) == 1
+
+ assert len(centers2d_list[1]) == len(gt_bboxes_3d_list[1]) == \
+ len(gt_bboxes_list[1]) == len(gt_labels_3d_list[1]) == \
+ len(gt_labels_list[1]) == 2
+
+
+def test_generate_keypoints():
+
+ centers2d_list = [
+ torch.tensor([[-99.86, 199.45], [499.50, 399.20], [201.20, 99.86]]),
+ torch.tensor([[-47.86, 199.45], [410.50, 399.20], [401.20, 349.86]])
+ ]
+ gt_bboxes_3d_list = [
+ CameraInstance3DBoxes(torch.rand([3, 7])),
+ CameraInstance3DBoxes(torch.rand([3, 7]))
+ ]
+ img_metas = [
+ dict(
+ cam2img=[[1260.8474446004698, 0.0, 807.968244525554, 40.1111],
+ [0.0, 1260.8474446004698, 495.3344268742088, 2.34422],
+ [0.0, 0.0, 1.0, 0.00333333], [0.0, 0.0, 0.0, 1.0]],
+ img_shape=(300, 400)) for i in range(2)
+ ]
+
+ keypoints2d_list, keypoints_depth_mask_list = \
+ get_keypoints(gt_bboxes_3d_list, centers2d_list, img_metas)
+
+ assert keypoints2d_list[0].shape == (3, 10, 3)
+ assert keypoints_depth_mask_list[0].shape == (3, 3)
diff --git a/tools/analysis_tools/analyze_logs.py b/tools/analysis_tools/analyze_logs.py
new file mode 100644
index 0000000..1885846
--- /dev/null
+++ b/tools/analysis_tools/analyze_logs.py
@@ -0,0 +1,202 @@
+# Copyright (c) OpenMMLab. All rights reserved.
+import argparse
+import json
+from collections import defaultdict
+
+import numpy as np
+import seaborn as sns
+from matplotlib import pyplot as plt
+
+
+def cal_train_time(log_dicts, args):
+ for i, log_dict in enumerate(log_dicts):
+ print(f'{"-" * 5}Analyze train time of {args.json_logs[i]}{"-" * 5}')
+ all_times = []
+ for epoch in log_dict.keys():
+ if args.include_outliers:
+ all_times.append(log_dict[epoch]['time'])
+ else:
+ all_times.append(log_dict[epoch]['time'][1:])
+ all_times = np.array(all_times)
+ epoch_ave_time = all_times.mean(-1)
+ slowest_epoch = epoch_ave_time.argmax()
+ fastest_epoch = epoch_ave_time.argmin()
+ std_over_epoch = epoch_ave_time.std()
+ print(f'slowest epoch {slowest_epoch + 1}, '
+ f'average time is {epoch_ave_time[slowest_epoch]:.4f}')
+ print(f'fastest epoch {fastest_epoch + 1}, '
+ f'average time is {epoch_ave_time[fastest_epoch]:.4f}')
+ print(f'time std over epochs is {std_over_epoch:.4f}')
+ print(f'average iter time: {np.mean(all_times):.4f} s/iter')
+ print()
+
+
+def plot_curve(log_dicts, args):
+ if args.backend is not None:
+ plt.switch_backend(args.backend)
+ sns.set_style(args.style)
+ # if legend is None, use {filename}_{key} as legend
+ legend = args.legend
+ if legend is None:
+ legend = []
+ for json_log in args.json_logs:
+ for metric in args.keys:
+ legend.append(f'{json_log}_{metric}')
+ assert len(legend) == (len(args.json_logs) * len(args.keys))
+ metrics = args.keys
+
+ num_metrics = len(metrics)
+ for i, log_dict in enumerate(log_dicts):
+ epochs = list(log_dict.keys())
+ for j, metric in enumerate(metrics):
+ print(f'plot curve of {args.json_logs[i]}, metric is {metric}')
+ if metric not in log_dict[epochs[args.interval - 1]]:
+ raise KeyError(
+ f'{args.json_logs[i]} does not contain metric {metric}')
+
+ if args.mode == 'eval':
+ if min(epochs) == args.interval:
+ x0 = args.interval
+ else:
+ # if current training is resumed from previous checkpoint
+ # we lost information in early epochs
+ # `xs` should start according to `min(epochs)`
+ if min(epochs) % args.interval == 0:
+ x0 = min(epochs)
+ else:
+ # find the first epoch that do eval
+ x0 = min(epochs) + args.interval - \
+ min(epochs) % args.interval
+ xs = np.arange(x0, max(epochs) + 1, args.interval)
+ ys = []
+ for epoch in epochs[args.interval - 1::args.interval]:
+ ys += log_dict[epoch][metric]
+
+ # if training is aborted before eval of the last epoch
+ # `xs` and `ys` will have different length and cause an error
+ # check if `ys[-1]` is empty here
+ if not log_dict[epoch][metric]:
+ xs = xs[:-1]
+
+ ax = plt.gca()
+ ax.set_xticks(xs)
+ plt.xlabel('epoch')
+ plt.plot(xs, ys, label=legend[i * num_metrics + j], marker='o')
+ else:
+ xs = []
+ ys = []
+ num_iters_per_epoch = \
+ log_dict[epochs[args.interval-1]]['iter'][-1]
+ for epoch in epochs[args.interval - 1::args.interval]:
+ iters = log_dict[epoch]['iter']
+ if log_dict[epoch]['mode'][-1] == 'val':
+ iters = iters[:-1]
+ xs.append(
+ np.array(iters) + (epoch - 1) * num_iters_per_epoch)
+ ys.append(np.array(log_dict[epoch][metric][:len(iters)]))
+ xs = np.concatenate(xs)
+ ys = np.concatenate(ys)
+ plt.xlabel('iter')
+ plt.plot(
+ xs, ys, label=legend[i * num_metrics + j], linewidth=0.5)
+ plt.legend()
+ if args.title is not None:
+ plt.title(args.title)
+ if args.out is None:
+ plt.show()
+ else:
+ print(f'save curve to: {args.out}')
+ plt.savefig(args.out)
+ plt.cla()
+
+
+def add_plot_parser(subparsers):
+ parser_plt = subparsers.add_parser(
+ 'plot_curve', help='parser for plotting curves')
+ parser_plt.add_argument(
+ 'json_logs',
+ type=str,
+ nargs='+',
+ help='path of train log in json format')
+ parser_plt.add_argument(
+ '--keys',
+ type=str,
+ nargs='+',
+ default=['mAP_0.25'],
+ help='the metric that you want to plot')
+ parser_plt.add_argument('--title', type=str, help='title of figure')
+ parser_plt.add_argument(
+ '--legend',
+ type=str,
+ nargs='+',
+ default=None,
+ help='legend of each plot')
+ parser_plt.add_argument(
+ '--backend', type=str, default=None, help='backend of plt')
+ parser_plt.add_argument(
+ '--style', type=str, default='dark', help='style of plt')
+ parser_plt.add_argument('--out', type=str, default=None)
+ parser_plt.add_argument('--mode', type=str, default='train')
+ parser_plt.add_argument('--interval', type=int, default=1)
+
+
+def add_time_parser(subparsers):
+ parser_time = subparsers.add_parser(
+ 'cal_train_time',
+ help='parser for computing the average time per training iteration')
+ parser_time.add_argument(
+ 'json_logs',
+ type=str,
+ nargs='+',
+ help='path of train log in json format')
+ parser_time.add_argument(
+ '--include-outliers',
+ action='store_true',
+ help='include the first value of every epoch when computing '
+ 'the average time')
+
+
+def parse_args():
+ parser = argparse.ArgumentParser(description='Analyze Json Log')
+ # currently only support plot curve and calculate average train time
+ subparsers = parser.add_subparsers(dest='task', help='task parser')
+ add_plot_parser(subparsers)
+ add_time_parser(subparsers)
+ args = parser.parse_args()
+ return args
+
+
+def load_json_logs(json_logs):
+ # load and convert json_logs to log_dict, key is epoch, value is a sub dict
+ # keys of sub dict is different metrics, e.g. memory, bbox_mAP
+ # value of sub dict is a list of corresponding values of all iterations
+ log_dicts = [dict() for _ in json_logs]
+ for json_log, log_dict in zip(json_logs, log_dicts):
+ with open(json_log, 'r') as log_file:
+ for line in log_file:
+ log = json.loads(line.strip())
+ # skip lines without `epoch` field
+ if 'epoch' not in log:
+ continue
+ epoch = log.pop('epoch')
+ if epoch not in log_dict:
+ log_dict[epoch] = defaultdict(list)
+ for k, v in log.items():
+ log_dict[epoch][k].append(v)
+ return log_dicts
+
+
+def main():
+ args = parse_args()
+
+ json_logs = args.json_logs
+ for json_log in json_logs:
+ assert json_log.endswith('.json')
+
+ log_dicts = load_json_logs(json_logs)
+
+ eval(args.task)(log_dicts, args)
+
+
+if __name__ == '__main__':
+ main()
diff --git a/tools/analysis_tools/benchmark.py b/tools/analysis_tools/benchmark.py
new file mode 100644
index 0000000..b31c9f0
--- /dev/null
+++ b/tools/analysis_tools/benchmark.py
@@ -0,0 +1,96 @@
+# Copyright (c) OpenMMLab. All rights reserved.
+import argparse
+import time
+
+import torch
+from mmcv import Config
+from mmcv.parallel import MMDataParallel
+from mmcv.runner import load_checkpoint, wrap_fp16_model
+
+from mmdet3d.datasets import build_dataloader, build_dataset
+from mmdet3d.models import build_detector
+from tools.misc.fuse_conv_bn import fuse_module
+
+
+def parse_args():
+ parser = argparse.ArgumentParser(description='MMDet benchmark a model')
+ parser.add_argument('config', help='test config file path')
+ parser.add_argument('checkpoint', help='checkpoint file')
+ parser.add_argument('--samples', default=2000, help='samples to benchmark')
+ parser.add_argument(
+ '--log-interval', default=50, help='interval of logging')
+ parser.add_argument(
+ '--fuse-conv-bn',
+ action='store_true',
+ help='Whether to fuse conv and bn, this will slightly increase'
+ 'the inference speed')
+ args = parser.parse_args()
+ return args
+
+
+def main():
+ args = parse_args()
+
+ cfg = Config.fromfile(args.config)
+ # set cudnn_benchmark
+ if cfg.get('cudnn_benchmark', False):
+ torch.backends.cudnn.benchmark = True
+ cfg.model.pretrained = None
+ cfg.data.test.test_mode = True
+
+ # build the dataloader
+ # TODO: support multiple images per gpu (only minor changes are needed)
+ dataset = build_dataset(cfg.data.test)
+ data_loader = build_dataloader(
+ dataset,
+ samples_per_gpu=1,
+ workers_per_gpu=cfg.data.workers_per_gpu,
+ dist=False,
+ shuffle=False)
+
+ # build the model and load checkpoint
+ cfg.model.train_cfg = None
+ model = build_detector(cfg.model, test_cfg=cfg.get('test_cfg'))
+ fp16_cfg = cfg.get('fp16', None)
+ if fp16_cfg is not None:
+ wrap_fp16_model(model)
+ load_checkpoint(model, args.checkpoint, map_location='cpu')
+ if args.fuse_conv_bn:
+ model = fuse_module(model)
+
+ model = MMDataParallel(model, device_ids=[0])
+
+ model.eval()
+
+ # the first several iterations may be very slow so skip them
+ num_warmup = 5
+ pure_inf_time = 0
+
+ # benchmark with several samples and take the average
+ for i, data in enumerate(data_loader):
+
+ torch.cuda.synchronize()
+ start_time = time.perf_counter()
+
+ with torch.no_grad():
+ model(return_loss=False, rescale=True, **data)
+
+ torch.cuda.synchronize()
+ elapsed = time.perf_counter() - start_time
+
+ if i >= num_warmup:
+ pure_inf_time += elapsed
+ if (i + 1) % args.log_interval == 0:
+ fps = (i + 1 - num_warmup) / pure_inf_time
+ print(f'Done image [{i + 1:<3}/ {args.samples}], '
+ f'fps: {fps:.1f} img / s')
+
+ if (i + 1) == args.samples:
+ pure_inf_time += elapsed
+ fps = (i + 1 - num_warmup) / pure_inf_time
+ print(f'Overall fps: {fps:.1f} img / s')
+ break
+
+
+if __name__ == '__main__':
+ main()
diff --git a/tools/analysis_tools/get_flops.py b/tools/analysis_tools/get_flops.py
new file mode 100644
index 0000000..f45ed80
--- /dev/null
+++ b/tools/analysis_tools/get_flops.py
@@ -0,0 +1,92 @@
+# Copyright (c) OpenMMLab. All rights reserved.
+import argparse
+
+import torch
+from mmcv import Config, DictAction
+
+from mmdet3d.models import build_model
+
+try:
+ from mmcv.cnn import get_model_complexity_info
+except ImportError:
+ raise ImportError('Please upgrade mmcv to >0.6.2')
+
+
+def parse_args():
+ parser = argparse.ArgumentParser(description='Train a detector')
+ parser.add_argument('config', help='train config file path')
+ parser.add_argument(
+ '--shape',
+ type=int,
+ nargs='+',
+ default=[40000, 4],
+ help='input point cloud size')
+ parser.add_argument(
+ '--modality',
+ type=str,
+ default='point',
+ choices=['point', 'image', 'multi'],
+ help='input data modality')
+ parser.add_argument(
+ '--cfg-options',
+ nargs='+',
+ action=DictAction,
+ help='override some settings in the used config, the key-value pair '
+ 'in xxx=yyy format will be merged into config file. If the value to '
+ 'be overwritten is a list, it should be like key="[a,b]" or key=a,b '
+ 'It also allows nested list/tuple values, e.g. key="[(a,b),(c,d)]" '
+ 'Note that the quotation marks are necessary and that no white space '
+ 'is allowed.')
+ args = parser.parse_args()
+ return args
+
+
+def main():
+
+ args = parse_args()
+
+ if args.modality == 'point':
+ assert len(args.shape) == 2, 'invalid input shape'
+ input_shape = tuple(args.shape)
+ elif args.modality == 'image':
+ if len(args.shape) == 1:
+ input_shape = (3, args.shape[0], args.shape[0])
+ elif len(args.shape) == 2:
+ input_shape = (3, ) + tuple(args.shape)
+ else:
+ raise ValueError('invalid input shape')
+ elif args.modality == 'multi':
+ raise NotImplementedError(
+ 'FLOPs counter is currently not supported for models with '
+ 'multi-modality input')
+
+ cfg = Config.fromfile(args.config)
+ if args.cfg_options is not None:
+ cfg.merge_from_dict(args.cfg_options)
+
+ model = build_model(
+ cfg.model,
+ train_cfg=cfg.get('train_cfg'),
+ test_cfg=cfg.get('test_cfg'))
+ if torch.cuda.is_available():
+ model.cuda()
+ model.eval()
+
+ if hasattr(model, 'forward_dummy'):
+ model.forward = model.forward_dummy
+ else:
+ raise NotImplementedError(
+ 'FLOPs counter is currently not supported for {}'.format(
+ model.__class__.__name__))
+
+ flops, params = get_model_complexity_info(model, input_shape)
+ split_line = '=' * 30
+ print(f'{split_line}\nInput shape: {input_shape}\n'
+ f'Flops: {flops}\nParams: {params}\n{split_line}')
+ print('!!!Please be cautious if you use the results in papers. '
+ 'You may need to check if all ops are supported and verify that the '
+ 'flops computation is correct.')
+
+
+if __name__ == '__main__':
+ main()
diff --git a/tools/create_data.py b/tools/create_data.py
new file mode 100644
index 0000000..bb23018
--- /dev/null
+++ b/tools/create_data.py
@@ -0,0 +1,322 @@
+# Copyright (c) OpenMMLab. All rights reserved.
+import argparse
+from os import path as osp
+
+from tools.data_converter import indoor_converter as indoor
+from tools.data_converter import kitti_converter as kitti
+from tools.data_converter import lyft_converter as lyft_converter
+from tools.data_converter import nuscenes_converter as nuscenes_converter
+from tools.data_converter.create_gt_database import (
+ GTDatabaseCreater, create_groundtruth_database)
+
+
+def kitti_data_prep(root_path,
+ info_prefix,
+ version,
+ out_dir,
+ with_plane=False):
+ """Prepare data related to Kitti dataset.
+
+ Related data consists of '.pkl' files recording basic infos,
+ 2D annotations and groundtruth database.
+
+ Args:
+ root_path (str): Path of dataset root.
+ info_prefix (str): The prefix of info filenames.
+ version (str): Dataset version.
+ out_dir (str): Output directory of the groundtruth database info.
+ with_plane (bool, optional): Whether to use plane information.
+ Default: False.
+ """
+ kitti.create_kitti_info_file(root_path, info_prefix, with_plane)
+ kitti.create_reduced_point_cloud(root_path, info_prefix)
+
+ info_train_path = osp.join(root_path, f'{info_prefix}_infos_train.pkl')
+ info_val_path = osp.join(root_path, f'{info_prefix}_infos_val.pkl')
+ info_trainval_path = osp.join(root_path,
+ f'{info_prefix}_infos_trainval.pkl')
+ info_test_path = osp.join(root_path, f'{info_prefix}_infos_test.pkl')
+ kitti.export_2d_annotation(root_path, info_train_path)
+ kitti.export_2d_annotation(root_path, info_val_path)
+ kitti.export_2d_annotation(root_path, info_trainval_path)
+ kitti.export_2d_annotation(root_path, info_test_path)
+
+ create_groundtruth_database(
+ 'KittiDataset',
+ root_path,
+ info_prefix,
+ f'{out_dir}/{info_prefix}_infos_train.pkl',
+ relative_path=False,
+ mask_anno_path='instances_train.json',
+ with_mask=(version == 'mask'))
+
+
+def nuscenes_data_prep(root_path,
+ info_prefix,
+ version,
+ dataset_name,
+ out_dir,
+ max_sweeps=10):
+ """Prepare data related to nuScenes dataset.
+
+ Related data consists of '.pkl' files recording basic infos,
+ 2D annotations and groundtruth database.
+
+ Args:
+ root_path (str): Path of dataset root.
+ info_prefix (str): The prefix of info filenames.
+ version (str): Dataset version.
+ dataset_name (str): The dataset class name.
+ out_dir (str): Output directory of the groundtruth database info.
+ max_sweeps (int, optional): Number of input consecutive frames.
+ Default: 10
+ """
+ nuscenes_converter.create_nuscenes_infos(
+ root_path, info_prefix, version=version, max_sweeps=max_sweeps)
+
+ if version == 'v1.0-test':
+ info_test_path = osp.join(root_path, f'{info_prefix}_infos_test.pkl')
+ nuscenes_converter.export_2d_annotation(
+ root_path, info_test_path, version=version)
+ return
+
+ info_train_path = osp.join(root_path, f'{info_prefix}_infos_train.pkl')
+ info_val_path = osp.join(root_path, f'{info_prefix}_infos_val.pkl')
+ nuscenes_converter.export_2d_annotation(
+ root_path, info_train_path, version=version)
+ nuscenes_converter.export_2d_annotation(
+ root_path, info_val_path, version=version)
+ create_groundtruth_database(dataset_name, root_path, info_prefix,
+ f'{out_dir}/{info_prefix}_infos_train.pkl')
+
+
+def lyft_data_prep(root_path, info_prefix, version, max_sweeps=10):
+ """Prepare data related to Lyft dataset.
+
+ Related data consists of '.pkl' files recording basic infos.
+ Although the ground truth database and 2D annotations are not used in
+ Lyft, it can also be generated like nuScenes.
+
+ Args:
+ root_path (str): Path of dataset root.
+ info_prefix (str): The prefix of info filenames.
+ version (str): Dataset version.
+ max_sweeps (int, optional): Number of input consecutive frames.
+ Defaults to 10.
+ """
+ lyft_converter.create_lyft_infos(
+ root_path, info_prefix, version=version, max_sweeps=max_sweeps)
+
+
+def scannet_data_prep(root_path, info_prefix, out_dir, workers):
+ """Prepare the info file for scannet dataset.
+
+ Args:
+ root_path (str): Path of dataset root.
+ info_prefix (str): The prefix of info filenames.
+ out_dir (str): Output directory of the generated info file.
+ workers (int): Number of threads to be used.
+ """
+ indoor.create_indoor_info_file(
+ root_path, info_prefix, out_dir, workers=workers)
+
+
+def scannet200_data_prep(root_path, info_prefix, out_dir, workers):
+ """Prepare the info file for scannet200 dataset.
+
+ Args:
+ root_path (str): Path of dataset root.
+ info_prefix (str): The prefix of info filenames.
+ out_dir (str): Output directory of the generated info file.
+ workers (int): Number of threads to be used.
+ """
+ indoor.create_indoor_info_file(
+ root_path, info_prefix, out_dir, workers=workers)
+
+
+def s3dis_data_prep(root_path, info_prefix, out_dir, workers):
+ """Prepare the info file for s3dis dataset.
+
+ Args:
+ root_path (str): Path of dataset root.
+ info_prefix (str): The prefix of info filenames.
+ out_dir (str): Output directory of the generated info file.
+ workers (int): Number of threads to be used.
+ """
+ indoor.create_indoor_info_file(
+ root_path, info_prefix, out_dir, workers=workers)
+
+
+def sunrgbd_data_prep(root_path, info_prefix, out_dir, workers):
+ """Prepare the info file for sunrgbd dataset.
+
+ Args:
+ root_path (str): Path of dataset root.
+ info_prefix (str): The prefix of info filenames.
+ out_dir (str): Output directory of the generated info file.
+ workers (int): Number of threads to be used.
+ """
+ indoor.create_indoor_info_file(
+ root_path, info_prefix, out_dir, workers=workers)
+
+
+def waymo_data_prep(root_path,
+ info_prefix,
+ version,
+ out_dir,
+ workers,
+ max_sweeps=5):
+ """Prepare the info file for waymo dataset.
+
+ Args:
+ root_path (str): Path of dataset root.
+ info_prefix (str): The prefix of info filenames.
+ out_dir (str): Output directory of the generated info file.
+ workers (int): Number of threads to be used.
+ max_sweeps (int, optional): Number of input consecutive frames.
+ Default: 5. Here we store pose information of these frames
+ for later use.
+ """
+ from tools.data_converter import waymo_converter as waymo
+
+ splits = ['training', 'validation', 'testing']
+ for i, split in enumerate(splits):
+ load_dir = osp.join(root_path, 'waymo_format', split)
+ if split == 'validation':
+ save_dir = osp.join(out_dir, 'kitti_format', 'training')
+ else:
+ save_dir = osp.join(out_dir, 'kitti_format', split)
+ converter = waymo.Waymo2KITTI(
+ load_dir,
+ save_dir,
+ prefix=str(i),
+ workers=workers,
+ test_mode=(split == 'testing'))
+ converter.convert()
+ # Generate waymo infos
+ out_dir = osp.join(out_dir, 'kitti_format')
+ kitti.create_waymo_info_file(
+ out_dir, info_prefix, max_sweeps=max_sweeps, workers=workers)
+ GTDatabaseCreater(
+ 'WaymoDataset',
+ out_dir,
+ info_prefix,
+ f'{out_dir}/{info_prefix}_infos_train.pkl',
+ relative_path=False,
+ with_mask=False,
+ num_worker=workers).create()
+
+
+parser = argparse.ArgumentParser(description='Data converter arg parser')
+parser.add_argument('dataset', metavar='kitti', help='name of the dataset')
+parser.add_argument(
+ '--root-path',
+ type=str,
+ default='./data/kitti',
+ help='specify the root path of dataset')
+parser.add_argument(
+ '--version',
+ type=str,
+ default='v1.0',
+ required=False,
+ help='specify the dataset version, no need for kitti')
+parser.add_argument(
+ '--max-sweeps',
+ type=int,
+ default=10,
+ required=False,
+ help='specify sweeps of lidar per example')
+parser.add_argument(
+ '--with-plane',
+ action='store_true',
+ help='Whether to use plane information for kitti.')
+parser.add_argument(
+ '--out-dir',
+ type=str,
+ default='./data/kitti',
+ required=False,
+ help='name of info pkl')
+parser.add_argument('--extra-tag', type=str, default='kitti')
+parser.add_argument(
+ '--workers', type=int, default=4, help='number of threads to be used')
+args = parser.parse_args()
+
+if __name__ == '__main__':
+ if args.dataset == 'kitti':
+ kitti_data_prep(
+ root_path=args.root_path,
+ info_prefix=args.extra_tag,
+ version=args.version,
+ out_dir=args.out_dir,
+ with_plane=args.with_plane)
+ elif args.dataset == 'nuscenes' and args.version != 'v1.0-mini':
+ train_version = f'{args.version}-trainval'
+ nuscenes_data_prep(
+ root_path=args.root_path,
+ info_prefix=args.extra_tag,
+ version=train_version,
+ dataset_name='NuScenesDataset',
+ out_dir=args.out_dir,
+ max_sweeps=args.max_sweeps)
+ test_version = f'{args.version}-test'
+ nuscenes_data_prep(
+ root_path=args.root_path,
+ info_prefix=args.extra_tag,
+ version=test_version,
+ dataset_name='NuScenesDataset',
+ out_dir=args.out_dir,
+ max_sweeps=args.max_sweeps)
+ elif args.dataset == 'nuscenes' and args.version == 'v1.0-mini':
+ train_version = f'{args.version}'
+ nuscenes_data_prep(
+ root_path=args.root_path,
+ info_prefix=args.extra_tag,
+ version=train_version,
+ dataset_name='NuScenesDataset',
+ out_dir=args.out_dir,
+ max_sweeps=args.max_sweeps)
+ elif args.dataset == 'lyft':
+ train_version = f'{args.version}-train'
+ lyft_data_prep(
+ root_path=args.root_path,
+ info_prefix=args.extra_tag,
+ version=train_version,
+ max_sweeps=args.max_sweeps)
+ test_version = f'{args.version}-test'
+ lyft_data_prep(
+ root_path=args.root_path,
+ info_prefix=args.extra_tag,
+ version=test_version,
+ max_sweeps=args.max_sweeps)
+ elif args.dataset == 'waymo':
+ waymo_data_prep(
+ root_path=args.root_path,
+ info_prefix=args.extra_tag,
+ version=args.version,
+ out_dir=args.out_dir,
+ workers=args.workers,
+ max_sweeps=args.max_sweeps)
+ elif args.dataset == 'scannet':
+ scannet_data_prep(
+ root_path=args.root_path,
+ info_prefix=args.extra_tag,
+ out_dir=args.out_dir,
+ workers=args.workers)
+ elif args.dataset == 's3dis':
+ s3dis_data_prep(
+ root_path=args.root_path,
+ info_prefix=args.extra_tag,
+ out_dir=args.out_dir,
+ workers=args.workers)
+ elif args.dataset == 'sunrgbd':
+ sunrgbd_data_prep(
+ root_path=args.root_path,
+ info_prefix=args.extra_tag,
+ out_dir=args.out_dir,
+ workers=args.workers)
+ elif args.dataset == 'scannet200':
+ scannet200_data_prep(
+ root_path=args.root_path,
+ info_prefix=args.extra_tag,
+ out_dir=args.out_dir,
+ workers=args.workers)
diff --git a/tools/create_data.sh b/tools/create_data.sh
new file mode 100755
index 0000000..9a57852
--- /dev/null
+++ b/tools/create_data.sh
@@ -0,0 +1,24 @@
+#!/usr/bin/env bash
+
+set -x
+export PYTHONPATH=`pwd`:$PYTHONPATH
+
+PARTITION=$1
+JOB_NAME=$2
+DATASET=$3
+GPUS=${GPUS:-1}
+GPUS_PER_NODE=${GPUS_PER_NODE:-1}
+SRUN_ARGS=${SRUN_ARGS:-""}
+JOB_NAME=create_data
+
+srun -p ${PARTITION} \
+ --job-name=${JOB_NAME} \
+ --gres=gpu:${GPUS_PER_NODE} \
+ --ntasks=${GPUS} \
+ --ntasks-per-node=${GPUS_PER_NODE} \
+ --kill-on-bad-exit=1 \
+ ${SRUN_ARGS} \
+ python -u tools/create_data.py ${DATASET} \
+ --root-path ./data/${DATASET} \
+ --out-dir ./data/${DATASET} \
+ --extra-tag ${DATASET}
diff --git a/tools/data_converter/__init__.py b/tools/data_converter/__init__.py
new file mode 100644
index 0000000..ef101fe
--- /dev/null
+++ b/tools/data_converter/__init__.py
@@ -0,0 +1 @@
+# Copyright (c) OpenMMLab. All rights reserved.
diff --git a/tools/data_converter/create_gt_database.py b/tools/data_converter/create_gt_database.py
new file mode 100644
index 0000000..210f0e8
--- /dev/null
+++ b/tools/data_converter/create_gt_database.py
@@ -0,0 +1,624 @@
+# Copyright (c) OpenMMLab. All rights reserved.
+import pickle
+from os import path as osp
+
+import mmcv
+import numpy as np
+from mmcv import track_iter_progress
+from mmcv.ops import roi_align
+from pycocotools import mask as maskUtils
+from pycocotools.coco import COCO
+
+from mmdet3d.core.bbox import box_np_ops as box_np_ops
+from mmdet3d.datasets import build_dataset
+from mmdet.core.evaluation.bbox_overlaps import bbox_overlaps
+
+
+def _poly2mask(mask_ann, img_h, img_w):
+ if isinstance(mask_ann, list):
+ # polygon -- a single object might consist of multiple parts
+ # we merge all parts into one mask rle code
+ rles = maskUtils.frPyObjects(mask_ann, img_h, img_w)
+ rle = maskUtils.merge(rles)
+ elif isinstance(mask_ann['counts'], list):
+ # uncompressed RLE
+ rle = maskUtils.frPyObjects(mask_ann, img_h, img_w)
+ else:
+ # rle
+ rle = mask_ann
+ mask = maskUtils.decode(rle)
+ return mask
+
+
+def _parse_coco_ann_info(ann_info):
+ gt_bboxes = []
+ gt_labels = []
+ gt_bboxes_ignore = []
+ gt_masks_ann = []
+
+ for i, ann in enumerate(ann_info):
+ if ann.get('ignore', False):
+ continue
+ x1, y1, w, h = ann['bbox']
+ if ann['area'] <= 0:
+ continue
+ bbox = [x1, y1, x1 + w, y1 + h]
+ if ann.get('iscrowd', False):
+ gt_bboxes_ignore.append(bbox)
+ else:
+ gt_bboxes.append(bbox)
+ gt_masks_ann.append(ann['segmentation'])
+
+ if gt_bboxes:
+ gt_bboxes = np.array(gt_bboxes, dtype=np.float32)
+ gt_labels = np.array(gt_labels, dtype=np.int64)
+ else:
+ gt_bboxes = np.zeros((0, 4), dtype=np.float32)
+ gt_labels = np.array([], dtype=np.int64)
+
+ if gt_bboxes_ignore:
+ gt_bboxes_ignore = np.array(gt_bboxes_ignore, dtype=np.float32)
+ else:
+ gt_bboxes_ignore = np.zeros((0, 4), dtype=np.float32)
+
+ ann = dict(
+ bboxes=gt_bboxes, bboxes_ignore=gt_bboxes_ignore, masks=gt_masks_ann)
+
+ return ann
+
+
+def crop_image_patch_v2(pos_proposals, pos_assigned_gt_inds, gt_masks):
+ import torch
+ from torch.nn.modules.utils import _pair
+ device = pos_proposals.device
+ num_pos = pos_proposals.size(0)
+ fake_inds = (
+ torch.arange(num_pos,
+ device=device).to(dtype=pos_proposals.dtype)[:, None])
+ rois = torch.cat([fake_inds, pos_proposals], dim=1) # Nx5
+ mask_size = _pair(28)
+ rois = rois.to(device=device)
+ gt_masks_th = (
+ torch.from_numpy(gt_masks).to(device).index_select(
+ 0, pos_assigned_gt_inds).to(dtype=rois.dtype))
+ # Use RoIAlign could apparently accelerate the training (~0.1s/iter)
+ targets = (
+ roi_align(gt_masks_th, rois, mask_size[::-1], 1.0, 0, True).squeeze(1))
+ return targets
+
+
+def crop_image_patch(pos_proposals, gt_masks, pos_assigned_gt_inds, org_img):
+ num_pos = pos_proposals.shape[0]
+ masks = []
+ img_patches = []
+ for i in range(num_pos):
+ gt_mask = gt_masks[pos_assigned_gt_inds[i]]
+ bbox = pos_proposals[i, :].astype(np.int32)
+ x1, y1, x2, y2 = bbox
+ w = np.maximum(x2 - x1 + 1, 1)
+ h = np.maximum(y2 - y1 + 1, 1)
+
+ mask_patch = gt_mask[y1:y1 + h, x1:x1 + w]
+ masked_img = gt_mask[..., None] * org_img
+ img_patch = masked_img[y1:y1 + h, x1:x1 + w]
+
+ img_patches.append(img_patch)
+ masks.append(mask_patch)
+ return img_patches, masks
+
+
+def create_groundtruth_database(dataset_class_name,
+ data_path,
+ info_prefix,
+ info_path=None,
+ mask_anno_path=None,
+ used_classes=None,
+ database_save_path=None,
+ db_info_save_path=None,
+ relative_path=True,
+ add_rgb=False,
+ lidar_only=False,
+ bev_only=False,
+ coors_range=None,
+ with_mask=False):
+ """Given the raw data, generate the ground truth database.
+
+ Args:
+ dataset_class_name (str): Name of the input dataset.
+ data_path (str): Path of the data.
+ info_prefix (str): Prefix of the info file.
+ info_path (str, optional): Path of the info file.
+ Default: None.
+ mask_anno_path (str, optional): Path of the mask_anno.
+ Default: None.
+ used_classes (list[str], optional): Classes have been used.
+ Default: None.
+ database_save_path (str, optional): Path to save database.
+ Default: None.
+ db_info_save_path (str, optional): Path to save db_info.
+ Default: None.
+ relative_path (bool, optional): Whether to use relative path.
+ Default: True.
+ with_mask (bool, optional): Whether to use mask.
+ Default: False.
+ """
+ print(f'Create GT Database of {dataset_class_name}')
+ dataset_cfg = dict(
+ type=dataset_class_name, data_root=data_path, ann_file=info_path)
+ if dataset_class_name == 'KittiDataset':
+ file_client_args = dict(backend='disk')
+ dataset_cfg.update(
+ test_mode=False,
+ split='training',
+ modality=dict(
+ use_lidar=True,
+ use_depth=False,
+ use_lidar_intensity=True,
+ use_camera=with_mask,
+ ),
+ pipeline=[
+ dict(
+ type='LoadPointsFromFile',
+ coord_type='LIDAR',
+ load_dim=4,
+ use_dim=4,
+ file_client_args=file_client_args),
+ dict(
+ type='LoadAnnotations3D',
+ with_bbox_3d=True,
+ with_label_3d=True,
+ file_client_args=file_client_args)
+ ])
+
+ elif dataset_class_name == 'NuScenesDataset':
+ dataset_cfg.update(
+ use_valid_flag=True,
+ pipeline=[
+ dict(
+ type='LoadPointsFromFile',
+ coord_type='LIDAR',
+ load_dim=5,
+ use_dim=5),
+ dict(
+ type='LoadPointsFromMultiSweeps',
+ sweeps_num=10,
+ use_dim=[0, 1, 2, 3, 4],
+ pad_empty_sweeps=True,
+ remove_close=True),
+ dict(
+ type='LoadAnnotations3D',
+ with_bbox_3d=True,
+ with_label_3d=True)
+ ])
+
+ elif dataset_class_name == 'WaymoDataset':
+ file_client_args = dict(backend='disk')
+ dataset_cfg.update(
+ test_mode=False,
+ split='training',
+ modality=dict(
+ use_lidar=True,
+ use_depth=False,
+ use_lidar_intensity=True,
+ use_camera=False,
+ ),
+ pipeline=[
+ dict(
+ type='LoadPointsFromFile',
+ coord_type='LIDAR',
+ load_dim=6,
+ use_dim=6,
+ file_client_args=file_client_args),
+ dict(
+ type='LoadAnnotations3D',
+ with_bbox_3d=True,
+ with_label_3d=True,
+ file_client_args=file_client_args)
+ ])
+
+ dataset = build_dataset(dataset_cfg)
+
+ if database_save_path is None:
+ database_save_path = osp.join(data_path, f'{info_prefix}_gt_database')
+ if db_info_save_path is None:
+ db_info_save_path = osp.join(data_path,
+ f'{info_prefix}_dbinfos_train.pkl')
+ mmcv.mkdir_or_exist(database_save_path)
+ all_db_infos = dict()
+ if with_mask:
+ coco = COCO(osp.join(data_path, mask_anno_path))
+ imgIds = coco.getImgIds()
+ file2id = dict()
+ for i in imgIds:
+ info = coco.loadImgs([i])[0]
+ file2id.update({info['file_name']: i})
+
+ group_counter = 0
+ for j in track_iter_progress(list(range(len(dataset)))):
+ input_dict = dataset.get_data_info(j)
+ dataset.pre_pipeline(input_dict)
+ example = dataset.pipeline(input_dict)
+ annos = example['ann_info']
+ image_idx = example['sample_idx']
+ points = example['points'].tensor.numpy()
+ gt_boxes_3d = annos['gt_bboxes_3d'].tensor.numpy()
+ names = annos['gt_names']
+ group_dict = dict()
+ if 'group_ids' in annos:
+ group_ids = annos['group_ids']
+ else:
+ group_ids = np.arange(gt_boxes_3d.shape[0], dtype=np.int64)
+ difficulty = np.zeros(gt_boxes_3d.shape[0], dtype=np.int32)
+ if 'difficulty' in annos:
+ difficulty = annos['difficulty']
+
+ num_obj = gt_boxes_3d.shape[0]
+ point_indices = box_np_ops.points_in_rbbox(points, gt_boxes_3d)
+
+ if with_mask:
+ # prepare masks
+ gt_boxes = annos['gt_bboxes']
+ img_path = osp.split(example['img_info']['filename'])[-1]
+ if img_path not in file2id.keys():
+ print(f'skip image {img_path} for empty mask')
+ continue
+ img_id = file2id[img_path]
+ kins_annIds = coco.getAnnIds(imgIds=img_id)
+ kins_raw_info = coco.loadAnns(kins_annIds)
+ kins_ann_info = _parse_coco_ann_info(kins_raw_info)
+ h, w = annos['img_shape'][:2]
+ gt_masks = [
+ _poly2mask(mask, h, w) for mask in kins_ann_info['masks']
+ ]
+ # get mask inds based on iou mapping
+ bbox_iou = bbox_overlaps(kins_ann_info['bboxes'], gt_boxes)
+ mask_inds = bbox_iou.argmax(axis=0)
+ valid_inds = (bbox_iou.max(axis=0) > 0.5)
+
+ # mask the image
+ # use more precise crop when it is ready
+ # object_img_patches = np.ascontiguousarray(
+ # np.stack(object_img_patches, axis=0).transpose(0, 3, 1, 2))
+ # crop image patches using roi_align
+ # object_img_patches = crop_image_patch_v2(
+ # torch.Tensor(gt_boxes),
+ # torch.Tensor(mask_inds).long(), object_img_patches)
+ object_img_patches, object_masks = crop_image_patch(
+ gt_boxes, gt_masks, mask_inds, annos['img'])
+
+ for i in range(num_obj):
+ filename = f'{image_idx}_{names[i]}_{i}.bin'
+ abs_filepath = osp.join(database_save_path, filename)
+ rel_filepath = osp.join(f'{info_prefix}_gt_database', filename)
+
+ # save point clouds and image patches for each object
+ gt_points = points[point_indices[:, i]]
+ gt_points[:, :3] -= gt_boxes_3d[i, :3]
+
+ if with_mask:
+ if object_masks[i].sum() == 0 or not valid_inds[i]:
+ # Skip object for empty or invalid mask
+ continue
+ img_patch_path = abs_filepath + '.png'
+ mask_patch_path = abs_filepath + '.mask.png'
+ mmcv.imwrite(object_img_patches[i], img_patch_path)
+ mmcv.imwrite(object_masks[i], mask_patch_path)
+
+ with open(abs_filepath, 'w') as f:
+ gt_points.tofile(f)
+
+ if (used_classes is None) or names[i] in used_classes:
+ db_info = {
+ 'name': names[i],
+ 'path': rel_filepath,
+ 'image_idx': image_idx,
+ 'gt_idx': i,
+ 'box3d_lidar': gt_boxes_3d[i],
+ 'num_points_in_gt': gt_points.shape[0],
+ 'difficulty': difficulty[i],
+ }
+ local_group_id = group_ids[i]
+ # if local_group_id >= 0:
+ if local_group_id not in group_dict:
+ group_dict[local_group_id] = group_counter
+ group_counter += 1
+ db_info['group_id'] = group_dict[local_group_id]
+ if 'score' in annos:
+ db_info['score'] = annos['score'][i]
+ if with_mask:
+ db_info.update({'box2d_camera': gt_boxes[i]})
+ if names[i] in all_db_infos:
+ all_db_infos[names[i]].append(db_info)
+ else:
+ all_db_infos[names[i]] = [db_info]
+
+ for k, v in all_db_infos.items():
+ print(f'load {len(v)} {k} database infos')
+
+ with open(db_info_save_path, 'wb') as f:
+ pickle.dump(all_db_infos, f)
+
+
+class GTDatabaseCreater:
+ """Given the raw data, generate the ground truth database. This is the
+ parallel version. For serialized version, please refer to
+ `create_groundtruth_database`
+
+ Args:
+ dataset_class_name (str): Name of the input dataset.
+ data_path (str): Path of the data.
+ info_prefix (str): Prefix of the info file.
+ info_path (str, optional): Path of the info file.
+ Default: None.
+ mask_anno_path (str, optional): Path of the mask_anno.
+ Default: None.
+ used_classes (list[str], optional): Classes have been used.
+ Default: None.
+ database_save_path (str, optional): Path to save database.
+ Default: None.
+ db_info_save_path (str, optional): Path to save db_info.
+ Default: None.
+ relative_path (bool, optional): Whether to use relative path.
+ Default: True.
+ with_mask (bool, optional): Whether to use mask.
+ Default: False.
+ num_worker (int, optional): the number of parallel workers to use.
+ Default: 8.
+ """
+
+ def __init__(self,
+ dataset_class_name,
+ data_path,
+ info_prefix,
+ info_path=None,
+ mask_anno_path=None,
+ used_classes=None,
+ database_save_path=None,
+ db_info_save_path=None,
+ relative_path=True,
+ add_rgb=False,
+ lidar_only=False,
+ bev_only=False,
+ coors_range=None,
+ with_mask=False,
+ num_worker=8) -> None:
+ self.dataset_class_name = dataset_class_name
+ self.data_path = data_path
+ self.info_prefix = info_prefix
+ self.info_path = info_path
+ self.mask_anno_path = mask_anno_path
+ self.used_classes = used_classes
+ self.database_save_path = database_save_path
+ self.db_info_save_path = db_info_save_path
+ self.relative_path = relative_path
+ self.add_rgb = add_rgb
+ self.lidar_only = lidar_only
+ self.bev_only = bev_only
+ self.coors_range = coors_range
+ self.with_mask = with_mask
+ self.num_worker = num_worker
+ self.pipeline = None
+
+ def create_single(self, input_dict):
+ group_counter = 0
+ single_db_infos = dict()
+ example = self.pipeline(input_dict)
+ annos = example['ann_info']
+ image_idx = example['sample_idx']
+ points = example['points'].tensor.numpy()
+ gt_boxes_3d = annos['gt_bboxes_3d'].tensor.numpy()
+ names = annos['gt_names']
+ group_dict = dict()
+ if 'group_ids' in annos:
+ group_ids = annos['group_ids']
+ else:
+ group_ids = np.arange(gt_boxes_3d.shape[0], dtype=np.int64)
+ difficulty = np.zeros(gt_boxes_3d.shape[0], dtype=np.int32)
+ if 'difficulty' in annos:
+ difficulty = annos['difficulty']
+
+ num_obj = gt_boxes_3d.shape[0]
+ point_indices = box_np_ops.points_in_rbbox(points, gt_boxes_3d)
+
+ if self.with_mask:
+ # prepare masks
+ gt_boxes = annos['gt_bboxes']
+ img_path = osp.split(example['img_info']['filename'])[-1]
+ if img_path not in self.file2id.keys():
+ print(f'skip image {img_path} for empty mask')
+ return single_db_infos
+ img_id = self.file2id[img_path]
+ kins_annIds = self.coco.getAnnIds(imgIds=img_id)
+ kins_raw_info = self.coco.loadAnns(kins_annIds)
+ kins_ann_info = _parse_coco_ann_info(kins_raw_info)
+ h, w = annos['img_shape'][:2]
+ gt_masks = [
+ _poly2mask(mask, h, w) for mask in kins_ann_info['masks']
+ ]
+ # get mask inds based on iou mapping
+ bbox_iou = bbox_overlaps(kins_ann_info['bboxes'], gt_boxes)
+ mask_inds = bbox_iou.argmax(axis=0)
+ valid_inds = (bbox_iou.max(axis=0) > 0.5)
+
+ # mask the image
+ # use more precise crop when it is ready
+ # object_img_patches = np.ascontiguousarray(
+ # np.stack(object_img_patches, axis=0).transpose(0, 3, 1, 2))
+ # crop image patches using roi_align
+ # object_img_patches = crop_image_patch_v2(
+ # torch.Tensor(gt_boxes),
+ # torch.Tensor(mask_inds).long(), object_img_patches)
+ object_img_patches, object_masks = crop_image_patch(
+ gt_boxes, gt_masks, mask_inds, annos['img'])
+
+ for i in range(num_obj):
+ filename = f'{image_idx}_{names[i]}_{i}.bin'
+ abs_filepath = osp.join(self.database_save_path, filename)
+ rel_filepath = osp.join(f'{self.info_prefix}_gt_database',
+ filename)
+
+ # save point clouds and image patches for each object
+ gt_points = points[point_indices[:, i]]
+ gt_points[:, :3] -= gt_boxes_3d[i, :3]
+
+ if self.with_mask:
+ if object_masks[i].sum() == 0 or not valid_inds[i]:
+ # Skip object for empty or invalid mask
+ continue
+ img_patch_path = abs_filepath + '.png'
+ mask_patch_path = abs_filepath + '.mask.png'
+ mmcv.imwrite(object_img_patches[i], img_patch_path)
+ mmcv.imwrite(object_masks[i], mask_patch_path)
+
+ with open(abs_filepath, 'w') as f:
+ gt_points.tofile(f)
+
+ if (self.used_classes is None) or names[i] in self.used_classes:
+ db_info = {
+ 'name': names[i],
+ 'path': rel_filepath,
+ 'image_idx': image_idx,
+ 'gt_idx': i,
+ 'box3d_lidar': gt_boxes_3d[i],
+ 'num_points_in_gt': gt_points.shape[0],
+ 'difficulty': difficulty[i],
+ }
+ local_group_id = group_ids[i]
+ # if local_group_id >= 0:
+ if local_group_id not in group_dict:
+ group_dict[local_group_id] = group_counter
+ group_counter += 1
+ db_info['group_id'] = group_dict[local_group_id]
+ if 'score' in annos:
+ db_info['score'] = annos['score'][i]
+ if self.with_mask:
+ db_info.update({'box2d_camera': gt_boxes[i]})
+ if names[i] in single_db_infos:
+ single_db_infos[names[i]].append(db_info)
+ else:
+ single_db_infos[names[i]] = [db_info]
+
+ return single_db_infos
+
+ def create(self):
+ print(f'Create GT Database of {self.dataset_class_name}')
+ dataset_cfg = dict(
+ type=self.dataset_class_name,
+ data_root=self.data_path,
+ ann_file=self.info_path)
+ if self.dataset_class_name == 'KittiDataset':
+ file_client_args = dict(backend='disk')
+ dataset_cfg.update(
+ test_mode=False,
+ split='training',
+ modality=dict(
+ use_lidar=True,
+ use_depth=False,
+ use_lidar_intensity=True,
+ use_camera=self.with_mask,
+ ),
+ pipeline=[
+ dict(
+ type='LoadPointsFromFile',
+ coord_type='LIDAR',
+ load_dim=4,
+ use_dim=4,
+ file_client_args=file_client_args),
+ dict(
+ type='LoadAnnotations3D',
+ with_bbox_3d=True,
+ with_label_3d=True,
+ file_client_args=file_client_args)
+ ])
+
+ elif self.dataset_class_name == 'NuScenesDataset':
+ dataset_cfg.update(
+ use_valid_flag=True,
+ pipeline=[
+ dict(
+ type='LoadPointsFromFile',
+ coord_type='LIDAR',
+ load_dim=5,
+ use_dim=5),
+ dict(
+ type='LoadPointsFromMultiSweeps',
+ sweeps_num=10,
+ use_dim=[0, 1, 2, 3, 4],
+ pad_empty_sweeps=True,
+ remove_close=True),
+ dict(
+ type='LoadAnnotations3D',
+ with_bbox_3d=True,
+ with_label_3d=True)
+ ])
+
+ elif self.dataset_class_name == 'WaymoDataset':
+ file_client_args = dict(backend='disk')
+ dataset_cfg.update(
+ test_mode=False,
+ split='training',
+ modality=dict(
+ use_lidar=True,
+ use_depth=False,
+ use_lidar_intensity=True,
+ use_camera=False,
+ ),
+ pipeline=[
+ dict(
+ type='LoadPointsFromFile',
+ coord_type='LIDAR',
+ load_dim=6,
+ use_dim=6,
+ file_client_args=file_client_args),
+ dict(
+ type='LoadAnnotations3D',
+ with_bbox_3d=True,
+ with_label_3d=True,
+ file_client_args=file_client_args)
+ ])
+
+ dataset = build_dataset(dataset_cfg)
+ self.pipeline = dataset.pipeline
+ if self.database_save_path is None:
+ self.database_save_path = osp.join(
+ self.data_path, f'{self.info_prefix}_gt_database')
+ if self.db_info_save_path is None:
+ self.db_info_save_path = osp.join(
+ self.data_path, f'{self.info_prefix}_dbinfos_train.pkl')
+ mmcv.mkdir_or_exist(self.database_save_path)
+ if self.with_mask:
+ self.coco = COCO(osp.join(self.data_path, self.mask_anno_path))
+ imgIds = self.coco.getImgIds()
+ self.file2id = dict()
+ for i in imgIds:
+ info = self.coco.loadImgs([i])[0]
+ self.file2id.update({info['file_name']: i})
+
+ def loop_dataset(i):
+ input_dict = dataset.get_data_info(i)
+ dataset.pre_pipeline(input_dict)
+ return input_dict
+
+ multi_db_infos = mmcv.track_parallel_progress(
+ self.create_single, ((loop_dataset(i)
+ for i in range(len(dataset))), len(dataset)),
+ self.num_worker)
+ print('Make global unique group id')
+ group_counter_offset = 0
+ all_db_infos = dict()
+ for single_db_infos in track_iter_progress(multi_db_infos):
+ group_id = -1
+ for name, name_db_infos in single_db_infos.items():
+ for db_info in name_db_infos:
+ group_id = max(group_id, db_info['group_id'])
+ db_info['group_id'] += group_counter_offset
+ if name not in all_db_infos:
+ all_db_infos[name] = []
+ all_db_infos[name].extend(name_db_infos)
+ group_counter_offset += (group_id + 1)
+
+ for k, v in all_db_infos.items():
+ print(f'load {len(v)} {k} database infos')
+
+ with open(self.db_info_save_path, 'wb') as f:
+ pickle.dump(all_db_infos, f)
diff --git a/tools/data_converter/indoor_converter.py b/tools/data_converter/indoor_converter.py
new file mode 100644
index 0000000..ce7d309
--- /dev/null
+++ b/tools/data_converter/indoor_converter.py
@@ -0,0 +1,120 @@
+# Copyright (c) OpenMMLab. All rights reserved.
+import os
+
+import mmcv
+import numpy as np
+
+from tools.data_converter.s3dis_data_utils import S3DISData, S3DISSegData
+from tools.data_converter.scannet_data_utils import ScanNetData, ScanNetSegData
+from tools.data_converter.sunrgbd_data_utils import SUNRGBDData
+
+
+def create_indoor_info_file(data_path,
+ pkl_prefix='sunrgbd',
+ save_path=None,
+ use_v1=False,
+ workers=4):
+ """Create indoor information file.
+
+ Get information of the raw data and save it to the pkl file.
+
+ Args:
+ data_path (str): Path of the data.
+ pkl_prefix (str, optional): Prefix of the pkl to be saved.
+ Default: 'sunrgbd'.
+ save_path (str, optional): Path of the pkl to be saved. Default: None.
+ use_v1 (bool, optional): Whether to use v1. Default: False.
+ workers (int, optional): Number of threads to be used. Default: 4.
+ """
+ assert os.path.exists(data_path)
+ assert pkl_prefix in ['sunrgbd', 'scannet', 's3dis', 'scannet200'], \
+ f'unsupported indoor dataset {pkl_prefix}'
+ save_path = data_path if save_path is None else save_path
+ assert os.path.exists(save_path)
+
+ # generate infos for both detection and segmentation task
+ if pkl_prefix in ['sunrgbd', 'scannet', 'scannet200']:
+ train_filename = os.path.join(save_path,
+ f'{pkl_prefix}_infos_train.pkl')
+ val_filename = os.path.join(save_path, f'{pkl_prefix}_infos_val.pkl')
+ if pkl_prefix == 'sunrgbd':
+ # SUN RGB-D has a train-val split
+ train_dataset = SUNRGBDData(
+ root_path=data_path, split='train', use_v1=use_v1)
+ val_dataset = SUNRGBDData(
+ root_path=data_path, split='val', use_v1=use_v1)
+ elif pkl_prefix == 'scannet':
+ # ScanNet has a train-val-test split
+ train_dataset = ScanNetData(root_path=data_path, split='train')
+ val_dataset = ScanNetData(root_path=data_path, split='val')
+ test_dataset = ScanNetData(root_path=data_path, split='test')
+ test_filename = os.path.join(save_path,
+ f'{pkl_prefix}_infos_test.pkl')
+ else: #scannet200
+ # ScanNet has a train-val-test split
+ train_dataset = ScanNetData(root_path=data_path, split='train',
+ scannet200=True, save_path=save_path)
+ val_dataset = ScanNetData(root_path=data_path, split='val',
+ scannet200=True, save_path=save_path)
+ test_dataset = ScanNetData(root_path=data_path, split='test',
+ scannet200=True, save_path=save_path)
+ test_filename = os.path.join(save_path,
+ f'{pkl_prefix}_infos_test.pkl')
+
+ infos_train = train_dataset.get_infos(
+ num_workers=workers, has_label=True)
+ mmcv.dump(infos_train, train_filename, 'pkl')
+ print(f'{pkl_prefix} info train file is saved to {train_filename}')
+
+ infos_val = val_dataset.get_infos(num_workers=workers, has_label=True)
+ mmcv.dump(infos_val, val_filename, 'pkl')
+ print(f'{pkl_prefix} info val file is saved to {val_filename}')
+
+ if pkl_prefix == 'scannet' or pkl_prefix == 'scannet200':
+ infos_test = test_dataset.get_infos(
+ num_workers=workers, has_label=False)
+ mmcv.dump(infos_test, test_filename, 'pkl')
+ print(f'{pkl_prefix} info test file is saved to {test_filename}')
+
+ # generate infos for the semantic segmentation task
+ # e.g. re-sampled scene indexes and label weights
+ # scene indexes are used to re-sample rooms with different number of points
+ # label weights are used to balance classes with different number of points
+ if pkl_prefix == 'scannet':
+ # label weight computation function is adopted from
+ # https://github.com/charlesq34/pointnet2/blob/master/scannet/scannet_dataset.py#L24
+ train_dataset = ScanNetSegData(
+ data_root=data_path,
+ ann_file=train_filename,
+ split='train',
+ num_points=8192,
+ label_weight_func=lambda x: 1.0 / np.log(1.2 + x))
+ # TODO: do we need to generate on val set?
+ val_dataset = ScanNetSegData(
+ data_root=data_path,
+ ann_file=val_filename,
+ split='val',
+ num_points=8192,
+ label_weight_func=lambda x: 1.0 / np.log(1.2 + x))
+ # no need to generate for test set
+ train_dataset.get_seg_infos()
+ val_dataset.get_seg_infos()
+ elif pkl_prefix == 's3dis':
+ # S3DIS doesn't have a fixed train-val split
+ # it has 6 areas instead, so we generate info file for each of them
+ # in training, we will use dataset to wrap different areas
+ splits = [f'Area_{i}' for i in [1, 2, 3, 4, 5, 6]]
+ for split in splits:
+ dataset = S3DISData(root_path=data_path, split=split)
+ info = dataset.get_infos(num_workers=workers, has_label=True)
+ filename = os.path.join(save_path,
+ f'{pkl_prefix}_infos_{split}.pkl')
+ mmcv.dump(info, filename, 'pkl')
+ print(f'{pkl_prefix} info {split} file is saved to {filename}')
+ seg_dataset = S3DISSegData(
+ data_root=data_path,
+ ann_file=filename,
+ split=split,
+ num_points=4096,
+ label_weight_func=lambda x: 1.0 / np.log(1.2 + x))
+ seg_dataset.get_seg_infos()
diff --git a/tools/data_converter/kitti_converter.py b/tools/data_converter/kitti_converter.py
new file mode 100644
index 0000000..2db461d
--- /dev/null
+++ b/tools/data_converter/kitti_converter.py
@@ -0,0 +1,624 @@
+# Copyright (c) OpenMMLab. All rights reserved.
+from collections import OrderedDict
+from pathlib import Path
+
+import mmcv
+import numpy as np
+from nuscenes.utils.geometry_utils import view_points
+
+from mmdet3d.core.bbox import box_np_ops, points_cam2img
+from .kitti_data_utils import WaymoInfoGatherer, get_kitti_image_info
+from .nuscenes_converter import post_process_coords
+
+kitti_categories = ('Pedestrian', 'Cyclist', 'Car')
+
+
+def convert_to_kitti_info_version2(info):
+ """convert kitti info v1 to v2 if possible.
+
+ Args:
+ info (dict): Info of the input kitti data.
+ - image (dict): image info
+ - calib (dict): calibration info
+ - point_cloud (dict): point cloud info
+ """
+ if 'image' not in info or 'calib' not in info or 'point_cloud' not in info:
+ info['image'] = {
+ 'image_shape': info['img_shape'],
+ 'image_idx': info['image_idx'],
+ 'image_path': info['img_path'],
+ }
+ info['calib'] = {
+ 'R0_rect': info['calib/R0_rect'],
+ 'Tr_velo_to_cam': info['calib/Tr_velo_to_cam'],
+ 'P2': info['calib/P2'],
+ }
+ info['point_cloud'] = {
+ 'velodyne_path': info['velodyne_path'],
+ }
+
+
+def _read_imageset_file(path):
+ with open(path, 'r') as f:
+ lines = f.readlines()
+ return [int(line) for line in lines]
+
+
+class _NumPointsInGTCalculater:
+ """Calculate the number of points inside the ground truth box. This is the
+ parallel version. For the serialized version, please refer to
+ `_calculate_num_points_in_gt`.
+
+ Args:
+ data_path (str): Path of the data.
+ relative_path (bool): Whether to use relative path.
+ remove_outside (bool, optional): Whether to remove points which are
+ outside of image. Default: True.
+ num_features (int, optional): Number of features per point.
+ Default: False.
+ num_worker (int, optional): the number of parallel workers to use.
+ Default: 8.
+ """
+
+ def __init__(self,
+ data_path,
+ relative_path,
+ remove_outside=True,
+ num_features=4,
+ num_worker=8) -> None:
+ self.data_path = data_path
+ self.relative_path = relative_path
+ self.remove_outside = remove_outside
+ self.num_features = num_features
+ self.num_worker = num_worker
+
+ def calculate_single(self, info):
+ pc_info = info['point_cloud']
+ image_info = info['image']
+ calib = info['calib']
+ if self.relative_path:
+ v_path = str(Path(self.data_path) / pc_info['velodyne_path'])
+ else:
+ v_path = pc_info['velodyne_path']
+ points_v = np.fromfile(
+ v_path, dtype=np.float32,
+ count=-1).reshape([-1, self.num_features])
+ rect = calib['R0_rect']
+ Trv2c = calib['Tr_velo_to_cam']
+ P2 = calib['P2']
+ if self.remove_outside:
+ points_v = box_np_ops.remove_outside_points(
+ points_v, rect, Trv2c, P2, image_info['image_shape'])
+ annos = info['annos']
+ num_obj = len([n for n in annos['name'] if n != 'DontCare'])
+ dims = annos['dimensions'][:num_obj]
+ loc = annos['location'][:num_obj]
+ rots = annos['rotation_y'][:num_obj]
+ gt_boxes_camera = np.concatenate([loc, dims, rots[..., np.newaxis]],
+ axis=1)
+ gt_boxes_lidar = box_np_ops.box_camera_to_lidar(
+ gt_boxes_camera, rect, Trv2c)
+ indices = box_np_ops.points_in_rbbox(points_v[:, :3], gt_boxes_lidar)
+ num_points_in_gt = indices.sum(0)
+ num_ignored = len(annos['dimensions']) - num_obj
+ num_points_in_gt = np.concatenate(
+ [num_points_in_gt, -np.ones([num_ignored])])
+ annos['num_points_in_gt'] = num_points_in_gt.astype(np.int32)
+ return info
+
+ def calculate(self, infos):
+ ret_infos = mmcv.track_parallel_progress(self.calculate_single, infos,
+ self.num_worker)
+ for i, ret_info in enumerate(ret_infos):
+ infos[i] = ret_info
+
+
+def _calculate_num_points_in_gt(data_path,
+ infos,
+ relative_path,
+ remove_outside=True,
+ num_features=4):
+ for info in mmcv.track_iter_progress(infos):
+ pc_info = info['point_cloud']
+ image_info = info['image']
+ calib = info['calib']
+ if relative_path:
+ v_path = str(Path(data_path) / pc_info['velodyne_path'])
+ else:
+ v_path = pc_info['velodyne_path']
+ points_v = np.fromfile(
+ v_path, dtype=np.float32, count=-1).reshape([-1, num_features])
+ rect = calib['R0_rect']
+ Trv2c = calib['Tr_velo_to_cam']
+ P2 = calib['P2']
+ if remove_outside:
+ points_v = box_np_ops.remove_outside_points(
+ points_v, rect, Trv2c, P2, image_info['image_shape'])
+
+ # points_v = points_v[points_v[:, 0] > 0]
+ annos = info['annos']
+ num_obj = len([n for n in annos['name'] if n != 'DontCare'])
+ # annos = kitti.filter_kitti_anno(annos, ['DontCare'])
+ dims = annos['dimensions'][:num_obj]
+ loc = annos['location'][:num_obj]
+ rots = annos['rotation_y'][:num_obj]
+ gt_boxes_camera = np.concatenate([loc, dims, rots[..., np.newaxis]],
+ axis=1)
+ gt_boxes_lidar = box_np_ops.box_camera_to_lidar(
+ gt_boxes_camera, rect, Trv2c)
+ indices = box_np_ops.points_in_rbbox(points_v[:, :3], gt_boxes_lidar)
+ num_points_in_gt = indices.sum(0)
+ num_ignored = len(annos['dimensions']) - num_obj
+ num_points_in_gt = np.concatenate(
+ [num_points_in_gt, -np.ones([num_ignored])])
+ annos['num_points_in_gt'] = num_points_in_gt.astype(np.int32)
+
+
+def create_kitti_info_file(data_path,
+ pkl_prefix='kitti',
+ with_plane=False,
+ save_path=None,
+ relative_path=True):
+ """Create info file of KITTI dataset.
+
+ Given the raw data, generate its related info file in pkl format.
+
+ Args:
+ data_path (str): Path of the data root.
+ pkl_prefix (str, optional): Prefix of the info file to be generated.
+ Default: 'kitti'.
+ with_plane (bool, optional): Whether to use plane information.
+ Default: False.
+ save_path (str, optional): Path to save the info file.
+ Default: None.
+ relative_path (bool, optional): Whether to use relative path.
+ Default: True.
+ """
+ imageset_folder = Path(data_path) / 'ImageSets'
+ train_img_ids = _read_imageset_file(str(imageset_folder / 'train.txt'))
+ val_img_ids = _read_imageset_file(str(imageset_folder / 'val.txt'))
+ test_img_ids = _read_imageset_file(str(imageset_folder / 'test.txt'))
+
+ print('Generate info. this may take several minutes.')
+ if save_path is None:
+ save_path = Path(data_path)
+ else:
+ save_path = Path(save_path)
+ kitti_infos_train = get_kitti_image_info(
+ data_path,
+ training=True,
+ velodyne=True,
+ calib=True,
+ with_plane=with_plane,
+ image_ids=train_img_ids,
+ relative_path=relative_path)
+ _calculate_num_points_in_gt(data_path, kitti_infos_train, relative_path)
+ filename = save_path / f'{pkl_prefix}_infos_train.pkl'
+ print(f'Kitti info train file is saved to {filename}')
+ mmcv.dump(kitti_infos_train, filename)
+ kitti_infos_val = get_kitti_image_info(
+ data_path,
+ training=True,
+ velodyne=True,
+ calib=True,
+ with_plane=with_plane,
+ image_ids=val_img_ids,
+ relative_path=relative_path)
+ _calculate_num_points_in_gt(data_path, kitti_infos_val, relative_path)
+ filename = save_path / f'{pkl_prefix}_infos_val.pkl'
+ print(f'Kitti info val file is saved to {filename}')
+ mmcv.dump(kitti_infos_val, filename)
+ filename = save_path / f'{pkl_prefix}_infos_trainval.pkl'
+ print(f'Kitti info trainval file is saved to {filename}')
+ mmcv.dump(kitti_infos_train + kitti_infos_val, filename)
+
+ kitti_infos_test = get_kitti_image_info(
+ data_path,
+ training=False,
+ label_info=False,
+ velodyne=True,
+ calib=True,
+ with_plane=False,
+ image_ids=test_img_ids,
+ relative_path=relative_path)
+ filename = save_path / f'{pkl_prefix}_infos_test.pkl'
+ print(f'Kitti info test file is saved to {filename}')
+ mmcv.dump(kitti_infos_test, filename)
+
+
+def create_waymo_info_file(data_path,
+ pkl_prefix='waymo',
+ save_path=None,
+ relative_path=True,
+ max_sweeps=5,
+ workers=8):
+ """Create info file of waymo dataset.
+
+ Given the raw data, generate its related info file in pkl format.
+
+ Args:
+ data_path (str): Path of the data root.
+ pkl_prefix (str, optional): Prefix of the info file to be generated.
+ Default: 'waymo'.
+ save_path (str, optional): Path to save the info file.
+ Default: None.
+ relative_path (bool, optional): Whether to use relative path.
+ Default: True.
+ max_sweeps (int, optional): Max sweeps before the detection frame
+ to be used. Default: 5.
+ """
+ imageset_folder = Path(data_path) / 'ImageSets'
+ train_img_ids = _read_imageset_file(str(imageset_folder / 'train.txt'))
+ val_img_ids = _read_imageset_file(str(imageset_folder / 'val.txt'))
+ test_img_ids = _read_imageset_file(str(imageset_folder / 'test.txt'))
+
+ print('Generate info. this may take several minutes.')
+ if save_path is None:
+ save_path = Path(data_path)
+ else:
+ save_path = Path(save_path)
+ waymo_infos_gatherer_trainval = WaymoInfoGatherer(
+ data_path,
+ training=True,
+ velodyne=True,
+ calib=True,
+ pose=True,
+ relative_path=relative_path,
+ max_sweeps=max_sweeps,
+ num_worker=workers)
+ waymo_infos_gatherer_test = WaymoInfoGatherer(
+ data_path,
+ training=False,
+ label_info=False,
+ velodyne=True,
+ calib=True,
+ pose=True,
+ relative_path=relative_path,
+ max_sweeps=max_sweeps,
+ num_worker=workers)
+ num_points_in_gt_calculater = _NumPointsInGTCalculater(
+ data_path,
+ relative_path,
+ num_features=6,
+ remove_outside=False,
+ num_worker=workers)
+
+ waymo_infos_train = waymo_infos_gatherer_trainval.gather(train_img_ids)
+ num_points_in_gt_calculater.calculate(waymo_infos_train)
+ filename = save_path / f'{pkl_prefix}_infos_train.pkl'
+ print(f'Waymo info train file is saved to {filename}')
+ mmcv.dump(waymo_infos_train, filename)
+ waymo_infos_val = waymo_infos_gatherer_trainval.gather(val_img_ids)
+ num_points_in_gt_calculater.calculate(waymo_infos_val)
+ filename = save_path / f'{pkl_prefix}_infos_val.pkl'
+ print(f'Waymo info val file is saved to {filename}')
+ mmcv.dump(waymo_infos_val, filename)
+ filename = save_path / f'{pkl_prefix}_infos_trainval.pkl'
+ print(f'Waymo info trainval file is saved to {filename}')
+ mmcv.dump(waymo_infos_train + waymo_infos_val, filename)
+ waymo_infos_test = waymo_infos_gatherer_test.gather(test_img_ids)
+ filename = save_path / f'{pkl_prefix}_infos_test.pkl'
+ print(f'Waymo info test file is saved to {filename}')
+ mmcv.dump(waymo_infos_test, filename)
+
+
+def _create_reduced_point_cloud(data_path,
+ info_path,
+ save_path=None,
+ back=False,
+ num_features=4,
+ front_camera_id=2):
+ """Create reduced point clouds for given info.
+
+ Args:
+ data_path (str): Path of original data.
+ info_path (str): Path of data info.
+ save_path (str, optional): Path to save reduced point cloud
+ data. Default: None.
+ back (bool, optional): Whether to flip the points to back.
+ Default: False.
+ num_features (int, optional): Number of point features. Default: 4.
+ front_camera_id (int, optional): The referenced/front camera ID.
+ Default: 2.
+ """
+ kitti_infos = mmcv.load(info_path)
+
+ for info in mmcv.track_iter_progress(kitti_infos):
+ pc_info = info['point_cloud']
+ image_info = info['image']
+ calib = info['calib']
+
+ v_path = pc_info['velodyne_path']
+ v_path = Path(data_path) / v_path
+ points_v = np.fromfile(
+ str(v_path), dtype=np.float32,
+ count=-1).reshape([-1, num_features])
+ rect = calib['R0_rect']
+ if front_camera_id == 2:
+ P2 = calib['P2']
+ else:
+ P2 = calib[f'P{str(front_camera_id)}']
+ Trv2c = calib['Tr_velo_to_cam']
+ # first remove z < 0 points
+ # keep = points_v[:, -1] > 0
+ # points_v = points_v[keep]
+ # then remove outside.
+ if back:
+ points_v[:, 0] = -points_v[:, 0]
+ points_v = box_np_ops.remove_outside_points(points_v, rect, Trv2c, P2,
+ image_info['image_shape'])
+ if save_path is None:
+ save_dir = v_path.parent.parent / (v_path.parent.stem + '_reduced')
+ if not save_dir.exists():
+ save_dir.mkdir()
+ save_filename = save_dir / v_path.name
+ # save_filename = str(v_path) + '_reduced'
+ if back:
+ save_filename += '_back'
+ else:
+ save_filename = str(Path(save_path) / v_path.name)
+ if back:
+ save_filename += '_back'
+ with open(save_filename, 'w') as f:
+ points_v.tofile(f)
+
+
+def create_reduced_point_cloud(data_path,
+ pkl_prefix,
+ train_info_path=None,
+ val_info_path=None,
+ test_info_path=None,
+ save_path=None,
+ with_back=False):
+ """Create reduced point clouds for training/validation/testing.
+
+ Args:
+ data_path (str): Path of original data.
+ pkl_prefix (str): Prefix of info files.
+ train_info_path (str, optional): Path of training set info.
+ Default: None.
+ val_info_path (str, optional): Path of validation set info.
+ Default: None.
+ test_info_path (str, optional): Path of test set info.
+ Default: None.
+ save_path (str, optional): Path to save reduced point cloud data.
+ Default: None.
+ with_back (bool, optional): Whether to flip the points to back.
+ Default: False.
+ """
+ if train_info_path is None:
+ train_info_path = Path(data_path) / f'{pkl_prefix}_infos_train.pkl'
+ if val_info_path is None:
+ val_info_path = Path(data_path) / f'{pkl_prefix}_infos_val.pkl'
+ if test_info_path is None:
+ test_info_path = Path(data_path) / f'{pkl_prefix}_infos_test.pkl'
+
+ print('create reduced point cloud for training set')
+ _create_reduced_point_cloud(data_path, train_info_path, save_path)
+ print('create reduced point cloud for validation set')
+ _create_reduced_point_cloud(data_path, val_info_path, save_path)
+ print('create reduced point cloud for testing set')
+ _create_reduced_point_cloud(data_path, test_info_path, save_path)
+ if with_back:
+ _create_reduced_point_cloud(
+ data_path, train_info_path, save_path, back=True)
+ _create_reduced_point_cloud(
+ data_path, val_info_path, save_path, back=True)
+ _create_reduced_point_cloud(
+ data_path, test_info_path, save_path, back=True)
+
+
+def export_2d_annotation(root_path, info_path, mono3d=True):
+ """Export 2d annotation from the info file and raw data.
+
+ Args:
+ root_path (str): Root path of the raw data.
+ info_path (str): Path of the info file.
+ mono3d (bool, optional): Whether to export mono3d annotation.
+ Default: True.
+ """
+ # get bbox annotations for camera
+ kitti_infos = mmcv.load(info_path)
+ cat2Ids = [
+ dict(id=kitti_categories.index(cat_name), name=cat_name)
+ for cat_name in kitti_categories
+ ]
+ coco_ann_id = 0
+ coco_2d_dict = dict(annotations=[], images=[], categories=cat2Ids)
+ from os import path as osp
+ for info in mmcv.track_iter_progress(kitti_infos):
+ coco_infos = get_2d_boxes(info, occluded=[0, 1, 2, 3], mono3d=mono3d)
+ (height, width,
+ _) = mmcv.imread(osp.join(root_path,
+ info['image']['image_path'])).shape
+ coco_2d_dict['images'].append(
+ dict(
+ file_name=info['image']['image_path'],
+ id=info['image']['image_idx'],
+ Tri2v=info['calib']['Tr_imu_to_velo'],
+ Trv2c=info['calib']['Tr_velo_to_cam'],
+ rect=info['calib']['R0_rect'],
+ cam_intrinsic=info['calib']['P2'],
+ width=width,
+ height=height))
+ for coco_info in coco_infos:
+ if coco_info is None:
+ continue
+ # add an empty key for coco format
+ coco_info['segmentation'] = []
+ coco_info['id'] = coco_ann_id
+ coco_2d_dict['annotations'].append(coco_info)
+ coco_ann_id += 1
+ if mono3d:
+ json_prefix = f'{info_path[:-4]}_mono3d'
+ else:
+ json_prefix = f'{info_path[:-4]}'
+ mmcv.dump(coco_2d_dict, f'{json_prefix}.coco.json')
+
+
+def get_2d_boxes(info, occluded, mono3d=True):
+ """Get the 2D annotation records for a given info.
+
+ Args:
+ info: Information of the given sample data.
+ occluded: Integer (0, 1, 2, 3) indicating occlusion state:
+ 0 = fully visible, 1 = partly occluded, 2 = largely occluded,
+ 3 = unknown, -1 = DontCare
+ mono3d (bool): Whether to get boxes with mono3d annotation.
+
+ Return:
+ list[dict]: List of 2D annotation record that belongs to the input
+ `sample_data_token`.
+ """
+ # Get calibration information
+ P2 = info['calib']['P2']
+
+ repro_recs = []
+ # if no annotations in info (test dataset), then return
+ if 'annos' not in info:
+ return repro_recs
+
+ # Get all the annotation with the specified visibilties.
+ ann_dicts = info['annos']
+ mask = [(ocld in occluded) for ocld in ann_dicts['occluded']]
+ for k in ann_dicts.keys():
+ ann_dicts[k] = ann_dicts[k][mask]
+
+ # convert dict of list to list of dict
+ ann_recs = []
+ for i in range(len(ann_dicts['occluded'])):
+ ann_rec = {}
+ for k in ann_dicts.keys():
+ ann_rec[k] = ann_dicts[k][i]
+ ann_recs.append(ann_rec)
+
+ for ann_idx, ann_rec in enumerate(ann_recs):
+ # Augment sample_annotation with token information.
+ ann_rec['sample_annotation_token'] = \
+ f"{info['image']['image_idx']}.{ann_idx}"
+ ann_rec['sample_data_token'] = info['image']['image_idx']
+ sample_data_token = info['image']['image_idx']
+
+ loc = ann_rec['location'][np.newaxis, :]
+ dim = ann_rec['dimensions'][np.newaxis, :]
+ rot = ann_rec['rotation_y'][np.newaxis, np.newaxis]
+ # transform the center from [0.5, 1.0, 0.5] to [0.5, 0.5, 0.5]
+ dst = np.array([0.5, 0.5, 0.5])
+ src = np.array([0.5, 1.0, 0.5])
+ loc = loc + dim * (dst - src)
+ offset = (info['calib']['P2'][0, 3] - info['calib']['P0'][0, 3]) \
+ / info['calib']['P2'][0, 0]
+ loc_3d = np.copy(loc)
+ loc_3d[0, 0] += offset
+ gt_bbox_3d = np.concatenate([loc, dim, rot], axis=1).astype(np.float32)
+
+ # Filter out the corners that are not in front of the calibrated
+ # sensor.
+ corners_3d = box_np_ops.center_to_corner_box3d(
+ gt_bbox_3d[:, :3],
+ gt_bbox_3d[:, 3:6],
+ gt_bbox_3d[:, 6], [0.5, 0.5, 0.5],
+ axis=1)
+ corners_3d = corners_3d[0].T # (1, 8, 3) -> (3, 8)
+ in_front = np.argwhere(corners_3d[2, :] > 0).flatten()
+ corners_3d = corners_3d[:, in_front]
+
+ # Project 3d box to 2d.
+ camera_intrinsic = P2
+ corner_coords = view_points(corners_3d, camera_intrinsic,
+ True).T[:, :2].tolist()
+
+ # Keep only corners that fall within the image.
+ final_coords = post_process_coords(corner_coords)
+
+ # Skip if the convex hull of the re-projected corners
+ # does not intersect the image canvas.
+ if final_coords is None:
+ continue
+ else:
+ min_x, min_y, max_x, max_y = final_coords
+
+ # Generate dictionary record to be included in the .json file.
+ repro_rec = generate_record(ann_rec, min_x, min_y, max_x, max_y,
+ sample_data_token,
+ info['image']['image_path'])
+
+ # If mono3d=True, add 3D annotations in camera coordinates
+ if mono3d and (repro_rec is not None):
+ repro_rec['bbox_cam3d'] = np.concatenate(
+ [loc_3d, dim, rot],
+ axis=1).astype(np.float32).squeeze().tolist()
+ repro_rec['velo_cam3d'] = -1 # no velocity in KITTI
+
+ center3d = np.array(loc).reshape([1, 3])
+ center2d = points_cam2img(
+ center3d, camera_intrinsic, with_depth=True)
+ repro_rec['center2d'] = center2d.squeeze().tolist()
+ # normalized center2D + depth
+ # samples with depth < 0 will be removed
+ if repro_rec['center2d'][2] <= 0:
+ continue
+
+ repro_rec['attribute_name'] = -1 # no attribute in KITTI
+ repro_rec['attribute_id'] = -1
+
+ repro_recs.append(repro_rec)
+
+ return repro_recs
+
+
+def generate_record(ann_rec, x1, y1, x2, y2, sample_data_token, filename):
+ """Generate one 2D annotation record given various information on top of
+ the 2D bounding box coordinates.
+
+ Args:
+ ann_rec (dict): Original 3d annotation record.
+ x1 (float): Minimum value of the x coordinate.
+ y1 (float): Minimum value of the y coordinate.
+ x2 (float): Maximum value of the x coordinate.
+ y2 (float): Maximum value of the y coordinate.
+ sample_data_token (str): Sample data token.
+ filename (str):The corresponding image file where the annotation
+ is present.
+
+ Returns:
+ dict: A sample 2D annotation record.
+ - file_name (str): file name
+ - image_id (str): sample data token
+ - area (float): 2d box area
+ - category_name (str): category name
+ - category_id (int): category id
+ - bbox (list[float]): left x, top y, x_size, y_size of 2d box
+ - iscrowd (int): whether the area is crowd
+ """
+ repro_rec = OrderedDict()
+ repro_rec['sample_data_token'] = sample_data_token
+ coco_rec = dict()
+
+ key_mapping = {
+ 'name': 'category_name',
+ 'num_points_in_gt': 'num_lidar_pts',
+ 'sample_annotation_token': 'sample_annotation_token',
+ 'sample_data_token': 'sample_data_token',
+ }
+
+ for key, value in ann_rec.items():
+ if key in key_mapping.keys():
+ repro_rec[key_mapping[key]] = value
+
+ repro_rec['bbox_corners'] = [x1, y1, x2, y2]
+ repro_rec['filename'] = filename
+
+ coco_rec['file_name'] = filename
+ coco_rec['image_id'] = sample_data_token
+ coco_rec['area'] = (y2 - y1) * (x2 - x1)
+
+ if repro_rec['category_name'] not in kitti_categories:
+ return None
+ cat_name = repro_rec['category_name']
+ coco_rec['category_name'] = cat_name
+ coco_rec['category_id'] = kitti_categories.index(cat_name)
+ coco_rec['bbox'] = [x1, y1, x2 - x1, y2 - y1]
+ coco_rec['iscrowd'] = 0
+
+ return coco_rec
diff --git a/tools/data_converter/kitti_data_utils.py b/tools/data_converter/kitti_data_utils.py
new file mode 100644
index 0000000..cae84cc
--- /dev/null
+++ b/tools/data_converter/kitti_data_utils.py
@@ -0,0 +1,619 @@
+# Copyright (c) OpenMMLab. All rights reserved.
+from collections import OrderedDict
+from concurrent import futures as futures
+from os import path as osp
+from pathlib import Path
+
+import mmcv
+import numpy as np
+from PIL import Image
+from skimage import io
+
+
+def get_image_index_str(img_idx, use_prefix_id=False):
+ if use_prefix_id:
+ return '{:07d}'.format(img_idx)
+ else:
+ return '{:06d}'.format(img_idx)
+
+
+def get_kitti_info_path(idx,
+ prefix,
+ info_type='image_2',
+ file_tail='.png',
+ training=True,
+ relative_path=True,
+ exist_check=True,
+ use_prefix_id=False):
+ img_idx_str = get_image_index_str(idx, use_prefix_id)
+ img_idx_str += file_tail
+ prefix = Path(prefix)
+ if training:
+ file_path = Path('training') / info_type / img_idx_str
+ else:
+ file_path = Path('testing') / info_type / img_idx_str
+ if exist_check and not (prefix / file_path).exists():
+ raise ValueError('file not exist: {}'.format(file_path))
+ if relative_path:
+ return str(file_path)
+ else:
+ return str(prefix / file_path)
+
+
+def get_image_path(idx,
+ prefix,
+ training=True,
+ relative_path=True,
+ exist_check=True,
+ info_type='image_2',
+ use_prefix_id=False):
+ return get_kitti_info_path(idx, prefix, info_type, '.png', training,
+ relative_path, exist_check, use_prefix_id)
+
+
+def get_label_path(idx,
+ prefix,
+ training=True,
+ relative_path=True,
+ exist_check=True,
+ info_type='label_2',
+ use_prefix_id=False):
+ return get_kitti_info_path(idx, prefix, info_type, '.txt', training,
+ relative_path, exist_check, use_prefix_id)
+
+
+def get_plane_path(idx,
+ prefix,
+ training=True,
+ relative_path=True,
+ exist_check=True,
+ info_type='planes',
+ use_prefix_id=False):
+ return get_kitti_info_path(idx, prefix, info_type, '.txt', training,
+ relative_path, exist_check, use_prefix_id)
+
+
+def get_velodyne_path(idx,
+ prefix,
+ training=True,
+ relative_path=True,
+ exist_check=True,
+ use_prefix_id=False):
+ return get_kitti_info_path(idx, prefix, 'velodyne', '.bin', training,
+ relative_path, exist_check, use_prefix_id)
+
+
+def get_calib_path(idx,
+ prefix,
+ training=True,
+ relative_path=True,
+ exist_check=True,
+ use_prefix_id=False):
+ return get_kitti_info_path(idx, prefix, 'calib', '.txt', training,
+ relative_path, exist_check, use_prefix_id)
+
+
+def get_pose_path(idx,
+ prefix,
+ training=True,
+ relative_path=True,
+ exist_check=True,
+ use_prefix_id=False):
+ return get_kitti_info_path(idx, prefix, 'pose', '.txt', training,
+ relative_path, exist_check, use_prefix_id)
+
+
+def get_timestamp_path(idx,
+ prefix,
+ training=True,
+ relative_path=True,
+ exist_check=True,
+ use_prefix_id=False):
+ return get_kitti_info_path(idx, prefix, 'timestamp', '.txt', training,
+ relative_path, exist_check, use_prefix_id)
+
+
+def get_label_anno(label_path):
+ annotations = {}
+ annotations.update({
+ 'name': [],
+ 'truncated': [],
+ 'occluded': [],
+ 'alpha': [],
+ 'bbox': [],
+ 'dimensions': [],
+ 'location': [],
+ 'rotation_y': []
+ })
+ with open(label_path, 'r') as f:
+ lines = f.readlines()
+ # if len(lines) == 0 or len(lines[0]) < 15:
+ # content = []
+ # else:
+ content = [line.strip().split(' ') for line in lines]
+ num_objects = len([x[0] for x in content if x[0] != 'DontCare'])
+ annotations['name'] = np.array([x[0] for x in content])
+ num_gt = len(annotations['name'])
+ annotations['truncated'] = np.array([float(x[1]) for x in content])
+ annotations['occluded'] = np.array([int(x[2]) for x in content])
+ annotations['alpha'] = np.array([float(x[3]) for x in content])
+ annotations['bbox'] = np.array([[float(info) for info in x[4:8]]
+ for x in content]).reshape(-1, 4)
+ # dimensions will convert hwl format to standard lhw(camera) format.
+ annotations['dimensions'] = np.array([[float(info) for info in x[8:11]]
+ for x in content
+ ]).reshape(-1, 3)[:, [2, 0, 1]]
+ annotations['location'] = np.array([[float(info) for info in x[11:14]]
+ for x in content]).reshape(-1, 3)
+ annotations['rotation_y'] = np.array([float(x[14])
+ for x in content]).reshape(-1)
+ if len(content) != 0 and len(content[0]) == 16: # have score
+ annotations['score'] = np.array([float(x[15]) for x in content])
+ else:
+ annotations['score'] = np.zeros((annotations['bbox'].shape[0], ))
+ index = list(range(num_objects)) + [-1] * (num_gt - num_objects)
+ annotations['index'] = np.array(index, dtype=np.int32)
+ annotations['group_ids'] = np.arange(num_gt, dtype=np.int32)
+ return annotations
+
+
+def _extend_matrix(mat):
+ mat = np.concatenate([mat, np.array([[0., 0., 0., 1.]])], axis=0)
+ return mat
+
+
+def get_kitti_image_info(path,
+ training=True,
+ label_info=True,
+ velodyne=False,
+ calib=False,
+ with_plane=False,
+ image_ids=7481,
+ extend_matrix=True,
+ num_worker=8,
+ relative_path=True,
+ with_imageshape=True):
+ """
+ KITTI annotation format version 2:
+ {
+ [optional]points: [N, 3+] point cloud
+ [optional, for kitti]image: {
+ image_idx: ...
+ image_path: ...
+ image_shape: ...
+ }
+ point_cloud: {
+ num_features: 4
+ velodyne_path: ...
+ }
+ [optional, for kitti]calib: {
+ R0_rect: ...
+ Tr_velo_to_cam: ...
+ P2: ...
+ }
+ annos: {
+ location: [num_gt, 3] array
+ dimensions: [num_gt, 3] array
+ rotation_y: [num_gt] angle array
+ name: [num_gt] ground truth name array
+ [optional]difficulty: kitti difficulty
+ [optional]group_ids: used for multi-part object
+ }
+ }
+ """
+ root_path = Path(path)
+ if not isinstance(image_ids, list):
+ image_ids = list(range(image_ids))
+
+ def map_func(idx):
+ info = {}
+ pc_info = {'num_features': 4}
+ calib_info = {}
+
+ image_info = {'image_idx': idx}
+ annotations = None
+ if velodyne:
+ pc_info['velodyne_path'] = get_velodyne_path(
+ idx, path, training, relative_path)
+ image_info['image_path'] = get_image_path(idx, path, training,
+ relative_path)
+ if with_imageshape:
+ img_path = image_info['image_path']
+ if relative_path:
+ img_path = str(root_path / img_path)
+ image_info['image_shape'] = np.array(
+ io.imread(img_path).shape[:2], dtype=np.int32)
+ if label_info:
+ label_path = get_label_path(idx, path, training, relative_path)
+ if relative_path:
+ label_path = str(root_path / label_path)
+ annotations = get_label_anno(label_path)
+ info['image'] = image_info
+ info['point_cloud'] = pc_info
+ if calib:
+ calib_path = get_calib_path(
+ idx, path, training, relative_path=False)
+ with open(calib_path, 'r') as f:
+ lines = f.readlines()
+ P0 = np.array([float(info) for info in lines[0].split(' ')[1:13]
+ ]).reshape([3, 4])
+ P1 = np.array([float(info) for info in lines[1].split(' ')[1:13]
+ ]).reshape([3, 4])
+ P2 = np.array([float(info) for info in lines[2].split(' ')[1:13]
+ ]).reshape([3, 4])
+ P3 = np.array([float(info) for info in lines[3].split(' ')[1:13]
+ ]).reshape([3, 4])
+ if extend_matrix:
+ P0 = _extend_matrix(P0)
+ P1 = _extend_matrix(P1)
+ P2 = _extend_matrix(P2)
+ P3 = _extend_matrix(P3)
+ R0_rect = np.array([
+ float(info) for info in lines[4].split(' ')[1:10]
+ ]).reshape([3, 3])
+ if extend_matrix:
+ rect_4x4 = np.zeros([4, 4], dtype=R0_rect.dtype)
+ rect_4x4[3, 3] = 1.
+ rect_4x4[:3, :3] = R0_rect
+ else:
+ rect_4x4 = R0_rect
+
+ Tr_velo_to_cam = np.array([
+ float(info) for info in lines[5].split(' ')[1:13]
+ ]).reshape([3, 4])
+ Tr_imu_to_velo = np.array([
+ float(info) for info in lines[6].split(' ')[1:13]
+ ]).reshape([3, 4])
+ if extend_matrix:
+ Tr_velo_to_cam = _extend_matrix(Tr_velo_to_cam)
+ Tr_imu_to_velo = _extend_matrix(Tr_imu_to_velo)
+ calib_info['P0'] = P0
+ calib_info['P1'] = P1
+ calib_info['P2'] = P2
+ calib_info['P3'] = P3
+ calib_info['R0_rect'] = rect_4x4
+ calib_info['Tr_velo_to_cam'] = Tr_velo_to_cam
+ calib_info['Tr_imu_to_velo'] = Tr_imu_to_velo
+ info['calib'] = calib_info
+
+ if with_plane:
+ plane_path = get_plane_path(idx, path, training, relative_path)
+ if relative_path:
+ plane_path = str(root_path / plane_path)
+ lines = mmcv.list_from_file(plane_path)
+ info['plane'] = np.array([float(i) for i in lines[3].split()])
+
+ if annotations is not None:
+ info['annos'] = annotations
+ add_difficulty_to_annos(info)
+ return info
+
+ with futures.ThreadPoolExecutor(num_worker) as executor:
+ image_infos = executor.map(map_func, image_ids)
+
+ return list(image_infos)
+
+
+class WaymoInfoGatherer:
+ """
+ Parallel version of waymo dataset information gathering.
+ Waymo annotation format version like KITTI:
+ {
+ [optional]points: [N, 3+] point cloud
+ [optional, for kitti]image: {
+ image_idx: ...
+ image_path: ...
+ image_shape: ...
+ }
+ point_cloud: {
+ num_features: 6
+ velodyne_path: ...
+ }
+ [optional, for kitti]calib: {
+ R0_rect: ...
+ Tr_velo_to_cam0: ...
+ P0: ...
+ }
+ annos: {
+ location: [num_gt, 3] array
+ dimensions: [num_gt, 3] array
+ rotation_y: [num_gt] angle array
+ name: [num_gt] ground truth name array
+ [optional]difficulty: kitti difficulty
+ [optional]group_ids: used for multi-part object
+ }
+ }
+ """
+
+ def __init__(self,
+ path,
+ training=True,
+ label_info=True,
+ velodyne=False,
+ calib=False,
+ pose=False,
+ extend_matrix=True,
+ num_worker=8,
+ relative_path=True,
+ with_imageshape=True,
+ max_sweeps=5) -> None:
+ self.path = path
+ self.training = training
+ self.label_info = label_info
+ self.velodyne = velodyne
+ self.calib = calib
+ self.pose = pose
+ self.extend_matrix = extend_matrix
+ self.num_worker = num_worker
+ self.relative_path = relative_path
+ self.with_imageshape = with_imageshape
+ self.max_sweeps = max_sweeps
+
+ def gather_single(self, idx):
+ root_path = Path(self.path)
+ info = {}
+ pc_info = {'num_features': 6}
+ calib_info = {}
+
+ image_info = {'image_idx': idx}
+ annotations = None
+ if self.velodyne:
+ pc_info['velodyne_path'] = get_velodyne_path(
+ idx,
+ self.path,
+ self.training,
+ self.relative_path,
+ use_prefix_id=True)
+ with open(
+ get_timestamp_path(
+ idx,
+ self.path,
+ self.training,
+ relative_path=False,
+ use_prefix_id=True)) as f:
+ info['timestamp'] = np.int64(f.read())
+ image_info['image_path'] = get_image_path(
+ idx,
+ self.path,
+ self.training,
+ self.relative_path,
+ info_type='image_0',
+ use_prefix_id=True)
+ if self.with_imageshape:
+ img_path = image_info['image_path']
+ if self.relative_path:
+ img_path = str(root_path / img_path)
+ # io using PIL is significantly faster than skimage
+ w, h = Image.open(img_path).size
+ image_info['image_shape'] = np.array((h, w), dtype=np.int32)
+ if self.label_info:
+ label_path = get_label_path(
+ idx,
+ self.path,
+ self.training,
+ self.relative_path,
+ info_type='label_all',
+ use_prefix_id=True)
+ if self.relative_path:
+ label_path = str(root_path / label_path)
+ annotations = get_label_anno(label_path)
+ info['image'] = image_info
+ info['point_cloud'] = pc_info
+ if self.calib:
+ calib_path = get_calib_path(
+ idx,
+ self.path,
+ self.training,
+ relative_path=False,
+ use_prefix_id=True)
+ with open(calib_path, 'r') as f:
+ lines = f.readlines()
+ P0 = np.array([float(info) for info in lines[0].split(' ')[1:13]
+ ]).reshape([3, 4])
+ P1 = np.array([float(info) for info in lines[1].split(' ')[1:13]
+ ]).reshape([3, 4])
+ P2 = np.array([float(info) for info in lines[2].split(' ')[1:13]
+ ]).reshape([3, 4])
+ P3 = np.array([float(info) for info in lines[3].split(' ')[1:13]
+ ]).reshape([3, 4])
+ P4 = np.array([float(info) for info in lines[4].split(' ')[1:13]
+ ]).reshape([3, 4])
+ if self.extend_matrix:
+ P0 = _extend_matrix(P0)
+ P1 = _extend_matrix(P1)
+ P2 = _extend_matrix(P2)
+ P3 = _extend_matrix(P3)
+ P4 = _extend_matrix(P4)
+ R0_rect = np.array([
+ float(info) for info in lines[5].split(' ')[1:10]
+ ]).reshape([3, 3])
+ if self.extend_matrix:
+ rect_4x4 = np.zeros([4, 4], dtype=R0_rect.dtype)
+ rect_4x4[3, 3] = 1.
+ rect_4x4[:3, :3] = R0_rect
+ else:
+ rect_4x4 = R0_rect
+
+ Tr_velo_to_cam = np.array([
+ float(info) for info in lines[6].split(' ')[1:13]
+ ]).reshape([3, 4])
+ if self.extend_matrix:
+ Tr_velo_to_cam = _extend_matrix(Tr_velo_to_cam)
+ calib_info['P0'] = P0
+ calib_info['P1'] = P1
+ calib_info['P2'] = P2
+ calib_info['P3'] = P3
+ calib_info['P4'] = P4
+ calib_info['R0_rect'] = rect_4x4
+ calib_info['Tr_velo_to_cam'] = Tr_velo_to_cam
+ info['calib'] = calib_info
+ if self.pose:
+ pose_path = get_pose_path(
+ idx,
+ self.path,
+ self.training,
+ relative_path=False,
+ use_prefix_id=True)
+ info['pose'] = np.loadtxt(pose_path)
+
+ if annotations is not None:
+ info['annos'] = annotations
+ info['annos']['camera_id'] = info['annos'].pop('score')
+ add_difficulty_to_annos(info)
+
+ sweeps = []
+ prev_idx = idx
+ while len(sweeps) < self.max_sweeps:
+ prev_info = {}
+ prev_idx -= 1
+ prev_info['velodyne_path'] = get_velodyne_path(
+ prev_idx,
+ self.path,
+ self.training,
+ self.relative_path,
+ exist_check=False,
+ use_prefix_id=True)
+ if_prev_exists = osp.exists(
+ Path(self.path) / prev_info['velodyne_path'])
+ if if_prev_exists:
+ with open(
+ get_timestamp_path(
+ prev_idx,
+ self.path,
+ self.training,
+ relative_path=False,
+ use_prefix_id=True)) as f:
+ prev_info['timestamp'] = np.int64(f.read())
+ prev_pose_path = get_pose_path(
+ prev_idx,
+ self.path,
+ self.training,
+ relative_path=False,
+ use_prefix_id=True)
+ prev_info['pose'] = np.loadtxt(prev_pose_path)
+ sweeps.append(prev_info)
+ else:
+ break
+ info['sweeps'] = sweeps
+
+ return info
+
+ def gather(self, image_ids):
+ if not isinstance(image_ids, list):
+ image_ids = list(range(image_ids))
+ image_infos = mmcv.track_parallel_progress(self.gather_single,
+ image_ids, self.num_worker)
+ return list(image_infos)
+
+
+def kitti_anno_to_label_file(annos, folder):
+ folder = Path(folder)
+ for anno in annos:
+ image_idx = anno['metadata']['image_idx']
+ label_lines = []
+ for j in range(anno['bbox'].shape[0]):
+ label_dict = {
+ 'name': anno['name'][j],
+ 'alpha': anno['alpha'][j],
+ 'bbox': anno['bbox'][j],
+ 'location': anno['location'][j],
+ 'dimensions': anno['dimensions'][j],
+ 'rotation_y': anno['rotation_y'][j],
+ 'score': anno['score'][j],
+ }
+ label_line = kitti_result_line(label_dict)
+ label_lines.append(label_line)
+ label_file = folder / f'{get_image_index_str(image_idx)}.txt'
+ label_str = '\n'.join(label_lines)
+ with open(label_file, 'w') as f:
+ f.write(label_str)
+
+
+def add_difficulty_to_annos(info):
+ min_height = [40, 25,
+ 25] # minimum height for evaluated groundtruth/detections
+ max_occlusion = [
+ 0, 1, 2
+ ] # maximum occlusion level of the groundtruth used for evaluation
+ max_trunc = [
+ 0.15, 0.3, 0.5
+ ] # maximum truncation level of the groundtruth used for evaluation
+ annos = info['annos']
+ dims = annos['dimensions'] # lhw format
+ bbox = annos['bbox']
+ height = bbox[:, 3] - bbox[:, 1]
+ occlusion = annos['occluded']
+ truncation = annos['truncated']
+ diff = []
+ easy_mask = np.ones((len(dims), ), dtype=np.bool)
+ moderate_mask = np.ones((len(dims), ), dtype=np.bool)
+ hard_mask = np.ones((len(dims), ), dtype=np.bool)
+ i = 0
+ for h, o, t in zip(height, occlusion, truncation):
+ if o > max_occlusion[0] or h <= min_height[0] or t > max_trunc[0]:
+ easy_mask[i] = False
+ if o > max_occlusion[1] or h <= min_height[1] or t > max_trunc[1]:
+ moderate_mask[i] = False
+ if o > max_occlusion[2] or h <= min_height[2] or t > max_trunc[2]:
+ hard_mask[i] = False
+ i += 1
+ is_easy = easy_mask
+ is_moderate = np.logical_xor(easy_mask, moderate_mask)
+ is_hard = np.logical_xor(hard_mask, moderate_mask)
+
+ for i in range(len(dims)):
+ if is_easy[i]:
+ diff.append(0)
+ elif is_moderate[i]:
+ diff.append(1)
+ elif is_hard[i]:
+ diff.append(2)
+ else:
+ diff.append(-1)
+ annos['difficulty'] = np.array(diff, np.int32)
+ return diff
+
+
+def kitti_result_line(result_dict, precision=4):
+ prec_float = '{' + ':.{}f'.format(precision) + '}'
+ res_line = []
+ all_field_default = OrderedDict([
+ ('name', None),
+ ('truncated', -1),
+ ('occluded', -1),
+ ('alpha', -10),
+ ('bbox', None),
+ ('dimensions', [-1, -1, -1]),
+ ('location', [-1000, -1000, -1000]),
+ ('rotation_y', -10),
+ ('score', 0.0),
+ ])
+ res_dict = [(key, None) for key, val in all_field_default.items()]
+ res_dict = OrderedDict(res_dict)
+ for key, val in result_dict.items():
+ if all_field_default[key] is None and val is None:
+ raise ValueError('you must specify a value for {}'.format(key))
+ res_dict[key] = val
+
+ for key, val in res_dict.items():
+ if key == 'name':
+ res_line.append(val)
+ elif key in ['truncated', 'alpha', 'rotation_y', 'score']:
+ if val is None:
+ res_line.append(str(all_field_default[key]))
+ else:
+ res_line.append(prec_float.format(val))
+ elif key == 'occluded':
+ if val is None:
+ res_line.append(str(all_field_default[key]))
+ else:
+ res_line.append('{}'.format(val))
+ elif key in ['bbox', 'dimensions', 'location']:
+ if val is None:
+ res_line += [str(v) for v in all_field_default[key]]
+ else:
+ res_line += [prec_float.format(v) for v in val]
+ else:
+ raise ValueError('unknown key. supported key:{}'.format(
+ res_dict.keys()))
+ return ' '.join(res_line)
diff --git a/tools/data_converter/lyft_converter.py b/tools/data_converter/lyft_converter.py
new file mode 100644
index 0000000..c6a89d0
--- /dev/null
+++ b/tools/data_converter/lyft_converter.py
@@ -0,0 +1,271 @@
+# Copyright (c) OpenMMLab. All rights reserved.
+import os
+from logging import warning
+from os import path as osp
+
+import mmcv
+import numpy as np
+from lyft_dataset_sdk.lyftdataset import LyftDataset as Lyft
+from pyquaternion import Quaternion
+
+from mmdet3d.datasets import LyftDataset
+from .nuscenes_converter import (get_2d_boxes, get_available_scenes,
+ obtain_sensor2top)
+
+lyft_categories = ('car', 'truck', 'bus', 'emergency_vehicle', 'other_vehicle',
+ 'motorcycle', 'bicycle', 'pedestrian', 'animal')
+
+
+def create_lyft_infos(root_path,
+ info_prefix,
+ version='v1.01-train',
+ max_sweeps=10):
+ """Create info file of lyft dataset.
+
+ Given the raw data, generate its related info file in pkl format.
+
+ Args:
+ root_path (str): Path of the data root.
+ info_prefix (str): Prefix of the info file to be generated.
+ version (str, optional): Version of the data.
+ Default: 'v1.01-train'.
+ max_sweeps (int, optional): Max number of sweeps.
+ Default: 10.
+ """
+ lyft = Lyft(
+ data_path=osp.join(root_path, version),
+ json_path=osp.join(root_path, version, version),
+ verbose=True)
+ available_vers = ['v1.01-train', 'v1.01-test']
+ assert version in available_vers
+ if version == 'v1.01-train':
+ train_scenes = mmcv.list_from_file('data/lyft/train.txt')
+ val_scenes = mmcv.list_from_file('data/lyft/val.txt')
+ elif version == 'v1.01-test':
+ train_scenes = mmcv.list_from_file('data/lyft/test.txt')
+ val_scenes = []
+ else:
+ raise ValueError('unknown')
+
+ # filter existing scenes.
+ available_scenes = get_available_scenes(lyft)
+ available_scene_names = [s['name'] for s in available_scenes]
+ train_scenes = list(
+ filter(lambda x: x in available_scene_names, train_scenes))
+ val_scenes = list(filter(lambda x: x in available_scene_names, val_scenes))
+ train_scenes = set([
+ available_scenes[available_scene_names.index(s)]['token']
+ for s in train_scenes
+ ])
+ val_scenes = set([
+ available_scenes[available_scene_names.index(s)]['token']
+ for s in val_scenes
+ ])
+
+ test = 'test' in version
+ if test:
+ print(f'test scene: {len(train_scenes)}')
+ else:
+ print(f'train scene: {len(train_scenes)}, \
+ val scene: {len(val_scenes)}')
+ train_lyft_infos, val_lyft_infos = _fill_trainval_infos(
+ lyft, train_scenes, val_scenes, test, max_sweeps=max_sweeps)
+
+ metadata = dict(version=version)
+ if test:
+ print(f'test sample: {len(train_lyft_infos)}')
+ data = dict(infos=train_lyft_infos, metadata=metadata)
+ info_name = f'{info_prefix}_infos_test'
+ info_path = osp.join(root_path, f'{info_name}.pkl')
+ mmcv.dump(data, info_path)
+ else:
+ print(f'train sample: {len(train_lyft_infos)}, \
+ val sample: {len(val_lyft_infos)}')
+ data = dict(infos=train_lyft_infos, metadata=metadata)
+ train_info_name = f'{info_prefix}_infos_train'
+ info_path = osp.join(root_path, f'{train_info_name}.pkl')
+ mmcv.dump(data, info_path)
+ data['infos'] = val_lyft_infos
+ val_info_name = f'{info_prefix}_infos_val'
+ info_val_path = osp.join(root_path, f'{val_info_name}.pkl')
+ mmcv.dump(data, info_val_path)
+
+
+def _fill_trainval_infos(lyft,
+ train_scenes,
+ val_scenes,
+ test=False,
+ max_sweeps=10):
+ """Generate the train/val infos from the raw data.
+
+ Args:
+ lyft (:obj:`LyftDataset`): Dataset class in the Lyft dataset.
+ train_scenes (list[str]): Basic information of training scenes.
+ val_scenes (list[str]): Basic information of validation scenes.
+ test (bool, optional): Whether use the test mode. In the test mode, no
+ annotations can be accessed. Default: False.
+ max_sweeps (int, optional): Max number of sweeps. Default: 10.
+
+ Returns:
+ tuple[list[dict]]: Information of training set and
+ validation set that will be saved to the info file.
+ """
+ train_lyft_infos = []
+ val_lyft_infos = []
+
+ for sample in mmcv.track_iter_progress(lyft.sample):
+ lidar_token = sample['data']['LIDAR_TOP']
+ sd_rec = lyft.get('sample_data', sample['data']['LIDAR_TOP'])
+ cs_record = lyft.get('calibrated_sensor',
+ sd_rec['calibrated_sensor_token'])
+ pose_record = lyft.get('ego_pose', sd_rec['ego_pose_token'])
+ abs_lidar_path, boxes, _ = lyft.get_sample_data(lidar_token)
+ # nuScenes devkit returns more convenient relative paths while
+ # lyft devkit returns absolute paths
+ abs_lidar_path = str(abs_lidar_path) # absolute path
+ lidar_path = abs_lidar_path.split(f'{os.getcwd()}/')[-1]
+ # relative path
+
+ mmcv.check_file_exist(lidar_path)
+
+ info = {
+ 'lidar_path': lidar_path,
+ 'token': sample['token'],
+ 'sweeps': [],
+ 'cams': dict(),
+ 'lidar2ego_translation': cs_record['translation'],
+ 'lidar2ego_rotation': cs_record['rotation'],
+ 'ego2global_translation': pose_record['translation'],
+ 'ego2global_rotation': pose_record['rotation'],
+ 'timestamp': sample['timestamp'],
+ }
+
+ l2e_r = info['lidar2ego_rotation']
+ l2e_t = info['lidar2ego_translation']
+ e2g_r = info['ego2global_rotation']
+ e2g_t = info['ego2global_translation']
+ l2e_r_mat = Quaternion(l2e_r).rotation_matrix
+ e2g_r_mat = Quaternion(e2g_r).rotation_matrix
+
+ # obtain 6 image's information per frame
+ camera_types = [
+ 'CAM_FRONT',
+ 'CAM_FRONT_RIGHT',
+ 'CAM_FRONT_LEFT',
+ 'CAM_BACK',
+ 'CAM_BACK_LEFT',
+ 'CAM_BACK_RIGHT',
+ ]
+ for cam in camera_types:
+ cam_token = sample['data'][cam]
+ cam_path, _, cam_intrinsic = lyft.get_sample_data(cam_token)
+ cam_info = obtain_sensor2top(lyft, cam_token, l2e_t, l2e_r_mat,
+ e2g_t, e2g_r_mat, cam)
+ cam_info.update(cam_intrinsic=cam_intrinsic)
+ info['cams'].update({cam: cam_info})
+
+ # obtain sweeps for a single key-frame
+ sd_rec = lyft.get('sample_data', sample['data']['LIDAR_TOP'])
+ sweeps = []
+ while len(sweeps) < max_sweeps:
+ if not sd_rec['prev'] == '':
+ sweep = obtain_sensor2top(lyft, sd_rec['prev'], l2e_t,
+ l2e_r_mat, e2g_t, e2g_r_mat, 'lidar')
+ sweeps.append(sweep)
+ sd_rec = lyft.get('sample_data', sd_rec['prev'])
+ else:
+ break
+ info['sweeps'] = sweeps
+ # obtain annotation
+ if not test:
+ annotations = [
+ lyft.get('sample_annotation', token)
+ for token in sample['anns']
+ ]
+ locs = np.array([b.center for b in boxes]).reshape(-1, 3)
+ dims = np.array([b.wlh for b in boxes]).reshape(-1, 3)
+ rots = np.array([b.orientation.yaw_pitch_roll[0]
+ for b in boxes]).reshape(-1, 1)
+
+ names = [b.name for b in boxes]
+ for i in range(len(names)):
+ if names[i] in LyftDataset.NameMapping:
+ names[i] = LyftDataset.NameMapping[names[i]]
+ names = np.array(names)
+
+ # we need to convert box size to
+ # the format of our lidar coordinate system
+ # which is x_size, y_size, z_size (corresponding to l, w, h)
+ gt_boxes = np.concatenate([locs, dims[:, [1, 0, 2]], rots], axis=1)
+ assert len(gt_boxes) == len(
+ annotations), f'{len(gt_boxes)}, {len(annotations)}'
+ info['gt_boxes'] = gt_boxes
+ info['gt_names'] = names
+ info['num_lidar_pts'] = np.array(
+ [a['num_lidar_pts'] for a in annotations])
+ info['num_radar_pts'] = np.array(
+ [a['num_radar_pts'] for a in annotations])
+
+ if sample['scene_token'] in train_scenes:
+ train_lyft_infos.append(info)
+ else:
+ val_lyft_infos.append(info)
+
+ return train_lyft_infos, val_lyft_infos
+
+
+def export_2d_annotation(root_path, info_path, version):
+ """Export 2d annotation from the info file and raw data.
+
+ Args:
+ root_path (str): Root path of the raw data.
+ info_path (str): Path of the info file.
+ version (str): Dataset version.
+ """
+ warning.warn('DeprecationWarning: 2D annotations are not used on the '
+ 'Lyft dataset. The function export_2d_annotation will be '
+ 'deprecated.')
+ # get bbox annotations for camera
+ camera_types = [
+ 'CAM_FRONT',
+ 'CAM_FRONT_RIGHT',
+ 'CAM_FRONT_LEFT',
+ 'CAM_BACK',
+ 'CAM_BACK_LEFT',
+ 'CAM_BACK_RIGHT',
+ ]
+ lyft_infos = mmcv.load(info_path)['infos']
+ lyft = Lyft(
+ data_path=osp.join(root_path, version),
+ json_path=osp.join(root_path, version, version),
+ verbose=True)
+ # info_2d_list = []
+ cat2Ids = [
+ dict(id=lyft_categories.index(cat_name), name=cat_name)
+ for cat_name in lyft_categories
+ ]
+ coco_ann_id = 0
+ coco_2d_dict = dict(annotations=[], images=[], categories=cat2Ids)
+ for info in mmcv.track_iter_progress(lyft_infos):
+ for cam in camera_types:
+ cam_info = info['cams'][cam]
+ coco_infos = get_2d_boxes(
+ lyft,
+ cam_info['sample_data_token'],
+ visibilities=['', '1', '2', '3', '4'])
+ (height, width, _) = mmcv.imread(cam_info['data_path']).shape
+ coco_2d_dict['images'].append(
+ dict(
+ file_name=cam_info['data_path'],
+ id=cam_info['sample_data_token'],
+ width=width,
+ height=height))
+ for coco_info in coco_infos:
+ if coco_info is None:
+ continue
+ # add an empty key for coco format
+ coco_info['segmentation'] = []
+ coco_info['id'] = coco_ann_id
+ coco_2d_dict['annotations'].append(coco_info)
+ coco_ann_id += 1
+ mmcv.dump(coco_2d_dict, f'{info_path[:-4]}.coco.json')
diff --git a/tools/data_converter/lyft_data_fixer.py b/tools/data_converter/lyft_data_fixer.py
new file mode 100644
index 0000000..5510351
--- /dev/null
+++ b/tools/data_converter/lyft_data_fixer.py
@@ -0,0 +1,39 @@
+# Copyright (c) OpenMMLab. All rights reserved.
+import argparse
+import os
+
+import numpy as np
+
+
+def fix_lyft(root_folder='./data/lyft', version='v1.01'):
+ # refer to https://www.kaggle.com/c/3d-object-detection-for-autonomous-vehicles/discussion/110000 # noqa
+ lidar_path = 'lidar/host-a011_lidar1_1233090652702363606.bin'
+ root_folder = os.path.join(root_folder, f'{version}-train')
+ lidar_path = os.path.join(root_folder, lidar_path)
+ assert os.path.isfile(lidar_path), f'Please download the complete Lyft ' \
+ f'dataset and make sure {lidar_path} is present.'
+ points = np.fromfile(lidar_path, dtype=np.float32, count=-1)
+ try:
+ points.reshape([-1, 5])
+ print(f'This fix is not required for version {version}.')
+ except ValueError:
+ new_points = np.array(list(points) + [100.0, 1.0], dtype='float32')
+ new_points.tofile(lidar_path)
+ print(f'Appended 100.0 and 1.0 to the end of {lidar_path}.')
+
+
+parser = argparse.ArgumentParser(description='Lyft dataset fixer arg parser')
+parser.add_argument(
+ '--root-folder',
+ type=str,
+ default='./data/lyft',
+ help='specify the root path of Lyft dataset')
+parser.add_argument(
+ '--version',
+ type=str,
+ default='v1.01',
+ help='specify Lyft dataset version')
+args = parser.parse_args()
+
+if __name__ == '__main__':
+ fix_lyft(root_folder=args.root_folder, version=args.version)
diff --git a/tools/data_converter/nuimage_converter.py b/tools/data_converter/nuimage_converter.py
new file mode 100644
index 0000000..a46015a
--- /dev/null
+++ b/tools/data_converter/nuimage_converter.py
@@ -0,0 +1,226 @@
+# Copyright (c) OpenMMLab. All rights reserved.
+import argparse
+import base64
+from os import path as osp
+
+import mmcv
+import numpy as np
+from nuimages import NuImages
+from nuimages.utils.utils import mask_decode, name_to_index_mapping
+
+nus_categories = ('car', 'truck', 'trailer', 'bus', 'construction_vehicle',
+ 'bicycle', 'motorcycle', 'pedestrian', 'traffic_cone',
+ 'barrier')
+
+NAME_MAPPING = {
+ 'movable_object.barrier': 'barrier',
+ 'vehicle.bicycle': 'bicycle',
+ 'vehicle.bus.bendy': 'bus',
+ 'vehicle.bus.rigid': 'bus',
+ 'vehicle.car': 'car',
+ 'vehicle.construction': 'construction_vehicle',
+ 'vehicle.motorcycle': 'motorcycle',
+ 'human.pedestrian.adult': 'pedestrian',
+ 'human.pedestrian.child': 'pedestrian',
+ 'human.pedestrian.construction_worker': 'pedestrian',
+ 'human.pedestrian.police_officer': 'pedestrian',
+ 'movable_object.trafficcone': 'traffic_cone',
+ 'vehicle.trailer': 'trailer',
+ 'vehicle.truck': 'truck',
+}
+
+
+def parse_args():
+ parser = argparse.ArgumentParser(description='Data converter arg parser')
+ parser.add_argument(
+ '--data-root',
+ type=str,
+ default='./data/nuimages',
+ help='specify the root path of dataset')
+ parser.add_argument(
+ '--version',
+ type=str,
+ nargs='+',
+ default=['v1.0-mini'],
+ required=False,
+ help='specify the dataset version')
+ parser.add_argument(
+ '--out-dir',
+ type=str,
+ default='./data/nuimages/annotations/',
+ required=False,
+ help='path to save the exported json')
+ parser.add_argument(
+ '--nproc',
+ type=int,
+ default=4,
+ required=False,
+ help='workers to process semantic masks')
+ parser.add_argument('--extra-tag', type=str, default='nuimages')
+ args = parser.parse_args()
+ return args
+
+
+def get_img_annos(nuim, img_info, cat2id, out_dir, data_root, seg_root):
+ """Get semantic segmentation map for an image.
+
+ Args:
+ nuim (obj:`NuImages`): NuImages dataset object
+ img_info (dict): Meta information of img
+
+ Returns:
+ np.ndarray: Semantic segmentation map of the image
+ """
+ sd_token = img_info['token']
+ image_id = img_info['id']
+ name_to_index = name_to_index_mapping(nuim.category)
+
+ # Get image data.
+ width, height = img_info['width'], img_info['height']
+ semseg_mask = np.zeros((height, width)).astype('uint8')
+
+ # Load stuff / surface regions.
+ surface_anns = [
+ o for o in nuim.surface_ann if o['sample_data_token'] == sd_token
+ ]
+
+ # Draw stuff / surface regions.
+ for ann in surface_anns:
+ # Get color and mask.
+ category_token = ann['category_token']
+ category_name = nuim.get('category', category_token)['name']
+ if ann['mask'] is None:
+ continue
+ mask = mask_decode(ann['mask'])
+
+ # Draw mask for semantic segmentation.
+ semseg_mask[mask == 1] = name_to_index[category_name]
+
+ # Load object instances.
+ object_anns = [
+ o for o in nuim.object_ann if o['sample_data_token'] == sd_token
+ ]
+
+ # Sort by token to ensure that objects always appear in the
+ # instance mask in the same order.
+ object_anns = sorted(object_anns, key=lambda k: k['token'])
+
+ # Draw object instances.
+ # The 0 index is reserved for background; thus, the instances
+ # should start from index 1.
+ annotations = []
+ for i, ann in enumerate(object_anns, start=1):
+ # Get color, box, mask and name.
+ category_token = ann['category_token']
+ category_name = nuim.get('category', category_token)['name']
+ if ann['mask'] is None:
+ continue
+ mask = mask_decode(ann['mask'])
+
+ # Draw masks for semantic segmentation and instance segmentation.
+ semseg_mask[mask == 1] = name_to_index[category_name]
+
+ if category_name in NAME_MAPPING:
+ cat_name = NAME_MAPPING[category_name]
+ cat_id = cat2id[cat_name]
+
+ x_min, y_min, x_max, y_max = ann['bbox']
+ # encode calibrated instance mask
+ mask_anno = dict()
+ mask_anno['counts'] = base64.b64decode(
+ ann['mask']['counts']).decode()
+ mask_anno['size'] = ann['mask']['size']
+
+ data_anno = dict(
+ image_id=image_id,
+ category_id=cat_id,
+ bbox=[x_min, y_min, x_max - x_min, y_max - y_min],
+ area=(x_max - x_min) * (y_max - y_min),
+ segmentation=mask_anno,
+ iscrowd=0)
+ annotations.append(data_anno)
+
+ # after process, save semantic masks
+ img_filename = img_info['file_name']
+ seg_filename = img_filename.replace('jpg', 'png')
+ seg_filename = osp.join(seg_root, seg_filename)
+ mmcv.imwrite(semseg_mask, seg_filename)
+ return annotations, np.max(semseg_mask)
+
+
+def export_nuim_to_coco(nuim, data_root, out_dir, extra_tag, version, nproc):
+ print('Process category information')
+ categories = []
+ categories = [
+ dict(id=nus_categories.index(cat_name), name=cat_name)
+ for cat_name in nus_categories
+ ]
+ cat2id = {k_v['name']: k_v['id'] for k_v in categories}
+
+ images = []
+ print('Process image meta information...')
+ for sample_info in mmcv.track_iter_progress(nuim.sample_data):
+ if sample_info['is_key_frame']:
+ img_idx = len(images)
+ images.append(
+ dict(
+ id=img_idx,
+ token=sample_info['token'],
+ file_name=sample_info['filename'],
+ width=sample_info['width'],
+ height=sample_info['height']))
+
+ seg_root = f'{out_dir}semantic_masks'
+ mmcv.mkdir_or_exist(seg_root)
+ mmcv.mkdir_or_exist(osp.join(data_root, 'calibrated'))
+
+ global process_img_anno
+
+ def process_img_anno(img_info):
+ single_img_annos, max_cls_id = get_img_annos(nuim, img_info, cat2id,
+ out_dir, data_root,
+ seg_root)
+ return single_img_annos, max_cls_id
+
+ print('Process img annotations...')
+ if nproc > 1:
+ outputs = mmcv.track_parallel_progress(
+ process_img_anno, images, nproc=nproc)
+ else:
+ outputs = []
+ for img_info in mmcv.track_iter_progress(images):
+ outputs.append(process_img_anno(img_info))
+
+ # Determine the index of object annotation
+ print('Process annotation information...')
+ annotations = []
+ max_cls_ids = []
+ for single_img_annos, max_cls_id in outputs:
+ max_cls_ids.append(max_cls_id)
+ for img_anno in single_img_annos:
+ img_anno.update(id=len(annotations))
+ annotations.append(img_anno)
+
+ max_cls_id = max(max_cls_ids)
+ print(f'Max ID of class in the semantic map: {max_cls_id}')
+
+ coco_format_json = dict(
+ images=images, annotations=annotations, categories=categories)
+
+ mmcv.mkdir_or_exist(out_dir)
+ out_file = osp.join(out_dir, f'{extra_tag}_{version}.json')
+ print(f'Annotation dumped to {out_file}')
+ mmcv.dump(coco_format_json, out_file)
+
+
+def main():
+ args = parse_args()
+ for version in args.version:
+ nuim = NuImages(
+ dataroot=args.data_root, version=version, verbose=True, lazy=True)
+ export_nuim_to_coco(nuim, args.data_root, args.out_dir, args.extra_tag,
+ version, args.nproc)
+
+
+if __name__ == '__main__':
+ main()
diff --git a/tools/data_converter/nuscenes_converter.py b/tools/data_converter/nuscenes_converter.py
new file mode 100644
index 0000000..c6140fc
--- /dev/null
+++ b/tools/data_converter/nuscenes_converter.py
@@ -0,0 +1,628 @@
+# Copyright (c) OpenMMLab. All rights reserved.
+import os
+from collections import OrderedDict
+from os import path as osp
+from typing import List, Tuple, Union
+
+import mmcv
+import numpy as np
+from nuscenes.nuscenes import NuScenes
+from nuscenes.utils.geometry_utils import view_points
+from pyquaternion import Quaternion
+from shapely.geometry import MultiPoint, box
+
+from mmdet3d.core.bbox import points_cam2img
+from mmdet3d.datasets import NuScenesDataset
+
+nus_categories = ('car', 'truck', 'trailer', 'bus', 'construction_vehicle',
+ 'bicycle', 'motorcycle', 'pedestrian', 'traffic_cone',
+ 'barrier')
+
+nus_attributes = ('cycle.with_rider', 'cycle.without_rider',
+ 'pedestrian.moving', 'pedestrian.standing',
+ 'pedestrian.sitting_lying_down', 'vehicle.moving',
+ 'vehicle.parked', 'vehicle.stopped', 'None')
+
+
+def create_nuscenes_infos(root_path,
+ info_prefix,
+ version='v1.0-trainval',
+ max_sweeps=10):
+ """Create info file of nuscene dataset.
+
+ Given the raw data, generate its related info file in pkl format.
+
+ Args:
+ root_path (str): Path of the data root.
+ info_prefix (str): Prefix of the info file to be generated.
+ version (str, optional): Version of the data.
+ Default: 'v1.0-trainval'.
+ max_sweeps (int, optional): Max number of sweeps.
+ Default: 10.
+ """
+ from nuscenes.nuscenes import NuScenes
+ nusc = NuScenes(version=version, dataroot=root_path, verbose=True)
+ from nuscenes.utils import splits
+ available_vers = ['v1.0-trainval', 'v1.0-test', 'v1.0-mini']
+ assert version in available_vers
+ if version == 'v1.0-trainval':
+ train_scenes = splits.train
+ val_scenes = splits.val
+ elif version == 'v1.0-test':
+ train_scenes = splits.test
+ val_scenes = []
+ elif version == 'v1.0-mini':
+ train_scenes = splits.mini_train
+ val_scenes = splits.mini_val
+ else:
+ raise ValueError('unknown')
+
+ # filter existing scenes.
+ available_scenes = get_available_scenes(nusc)
+ available_scene_names = [s['name'] for s in available_scenes]
+ train_scenes = list(
+ filter(lambda x: x in available_scene_names, train_scenes))
+ val_scenes = list(filter(lambda x: x in available_scene_names, val_scenes))
+ train_scenes = set([
+ available_scenes[available_scene_names.index(s)]['token']
+ for s in train_scenes
+ ])
+ val_scenes = set([
+ available_scenes[available_scene_names.index(s)]['token']
+ for s in val_scenes
+ ])
+
+ test = 'test' in version
+ if test:
+ print('test scene: {}'.format(len(train_scenes)))
+ else:
+ print('train scene: {}, val scene: {}'.format(
+ len(train_scenes), len(val_scenes)))
+ train_nusc_infos, val_nusc_infos = _fill_trainval_infos(
+ nusc, train_scenes, val_scenes, test, max_sweeps=max_sweeps)
+
+ metadata = dict(version=version)
+ if test:
+ print('test sample: {}'.format(len(train_nusc_infos)))
+ data = dict(infos=train_nusc_infos, metadata=metadata)
+ info_path = osp.join(root_path,
+ '{}_infos_test.pkl'.format(info_prefix))
+ mmcv.dump(data, info_path)
+ else:
+ print('train sample: {}, val sample: {}'.format(
+ len(train_nusc_infos), len(val_nusc_infos)))
+ data = dict(infos=train_nusc_infos, metadata=metadata)
+ info_path = osp.join(root_path,
+ '{}_infos_train.pkl'.format(info_prefix))
+ mmcv.dump(data, info_path)
+ data['infos'] = val_nusc_infos
+ info_val_path = osp.join(root_path,
+ '{}_infos_val.pkl'.format(info_prefix))
+ mmcv.dump(data, info_val_path)
+
+
+def get_available_scenes(nusc):
+ """Get available scenes from the input nuscenes class.
+
+ Given the raw data, get the information of available scenes for
+ further info generation.
+
+ Args:
+ nusc (class): Dataset class in the nuScenes dataset.
+
+ Returns:
+ available_scenes (list[dict]): List of basic information for the
+ available scenes.
+ """
+ available_scenes = []
+ print('total scene num: {}'.format(len(nusc.scene)))
+ for scene in nusc.scene:
+ scene_token = scene['token']
+ scene_rec = nusc.get('scene', scene_token)
+ sample_rec = nusc.get('sample', scene_rec['first_sample_token'])
+ sd_rec = nusc.get('sample_data', sample_rec['data']['LIDAR_TOP'])
+ has_more_frames = True
+ scene_not_exist = False
+ while has_more_frames:
+ lidar_path, boxes, _ = nusc.get_sample_data(sd_rec['token'])
+ lidar_path = str(lidar_path)
+ if os.getcwd() in lidar_path:
+ # path from lyftdataset is absolute path
+ lidar_path = lidar_path.split(f'{os.getcwd()}/')[-1]
+ # relative path
+ if not mmcv.is_filepath(lidar_path):
+ scene_not_exist = True
+ break
+ else:
+ break
+ if scene_not_exist:
+ continue
+ available_scenes.append(scene)
+ print('exist scene num: {}'.format(len(available_scenes)))
+ return available_scenes
+
+
+def _fill_trainval_infos(nusc,
+ train_scenes,
+ val_scenes,
+ test=False,
+ max_sweeps=10):
+ """Generate the train/val infos from the raw data.
+
+ Args:
+ nusc (:obj:`NuScenes`): Dataset class in the nuScenes dataset.
+ train_scenes (list[str]): Basic information of training scenes.
+ val_scenes (list[str]): Basic information of validation scenes.
+ test (bool, optional): Whether use the test mode. In test mode, no
+ annotations can be accessed. Default: False.
+ max_sweeps (int, optional): Max number of sweeps. Default: 10.
+
+ Returns:
+ tuple[list[dict]]: Information of training set and validation set
+ that will be saved to the info file.
+ """
+ train_nusc_infos = []
+ val_nusc_infos = []
+
+ for sample in mmcv.track_iter_progress(nusc.sample):
+ lidar_token = sample['data']['LIDAR_TOP']
+ sd_rec = nusc.get('sample_data', sample['data']['LIDAR_TOP'])
+ cs_record = nusc.get('calibrated_sensor',
+ sd_rec['calibrated_sensor_token'])
+ pose_record = nusc.get('ego_pose', sd_rec['ego_pose_token'])
+ lidar_path, boxes, _ = nusc.get_sample_data(lidar_token)
+
+ mmcv.check_file_exist(lidar_path)
+
+ info = {
+ 'lidar_path': lidar_path,
+ 'token': sample['token'],
+ 'sweeps': [],
+ 'cams': dict(),
+ 'lidar2ego_translation': cs_record['translation'],
+ 'lidar2ego_rotation': cs_record['rotation'],
+ 'ego2global_translation': pose_record['translation'],
+ 'ego2global_rotation': pose_record['rotation'],
+ 'timestamp': sample['timestamp'],
+ }
+
+ l2e_r = info['lidar2ego_rotation']
+ l2e_t = info['lidar2ego_translation']
+ e2g_r = info['ego2global_rotation']
+ e2g_t = info['ego2global_translation']
+ l2e_r_mat = Quaternion(l2e_r).rotation_matrix
+ e2g_r_mat = Quaternion(e2g_r).rotation_matrix
+
+ # obtain 6 image's information per frame
+ camera_types = [
+ 'CAM_FRONT',
+ 'CAM_FRONT_RIGHT',
+ 'CAM_FRONT_LEFT',
+ 'CAM_BACK',
+ 'CAM_BACK_LEFT',
+ 'CAM_BACK_RIGHT',
+ ]
+ for cam in camera_types:
+ cam_token = sample['data'][cam]
+ cam_path, _, cam_intrinsic = nusc.get_sample_data(cam_token)
+ cam_info = obtain_sensor2top(nusc, cam_token, l2e_t, l2e_r_mat,
+ e2g_t, e2g_r_mat, cam)
+ cam_info.update(cam_intrinsic=cam_intrinsic)
+ info['cams'].update({cam: cam_info})
+
+ # obtain sweeps for a single key-frame
+ sd_rec = nusc.get('sample_data', sample['data']['LIDAR_TOP'])
+ sweeps = []
+ while len(sweeps) < max_sweeps:
+ if not sd_rec['prev'] == '':
+ sweep = obtain_sensor2top(nusc, sd_rec['prev'], l2e_t,
+ l2e_r_mat, e2g_t, e2g_r_mat, 'lidar')
+ sweeps.append(sweep)
+ sd_rec = nusc.get('sample_data', sd_rec['prev'])
+ else:
+ break
+ info['sweeps'] = sweeps
+ # obtain annotation
+ if not test:
+ annotations = [
+ nusc.get('sample_annotation', token)
+ for token in sample['anns']
+ ]
+ locs = np.array([b.center for b in boxes]).reshape(-1, 3)
+ dims = np.array([b.wlh for b in boxes]).reshape(-1, 3)
+ rots = np.array([b.orientation.yaw_pitch_roll[0]
+ for b in boxes]).reshape(-1, 1)
+ velocity = np.array(
+ [nusc.box_velocity(token)[:2] for token in sample['anns']])
+ valid_flag = np.array(
+ [(anno['num_lidar_pts'] + anno['num_radar_pts']) > 0
+ for anno in annotations],
+ dtype=bool).reshape(-1)
+ # convert velo from global to lidar
+ for i in range(len(boxes)):
+ velo = np.array([*velocity[i], 0.0])
+ velo = velo @ np.linalg.inv(e2g_r_mat).T @ np.linalg.inv(
+ l2e_r_mat).T
+ velocity[i] = velo[:2]
+
+ names = [b.name for b in boxes]
+ for i in range(len(names)):
+ if names[i] in NuScenesDataset.NameMapping:
+ names[i] = NuScenesDataset.NameMapping[names[i]]
+ names = np.array(names)
+ # we need to convert box size to
+ # the format of our lidar coordinate system
+ # which is x_size, y_size, z_size (corresponding to l, w, h)
+ gt_boxes = np.concatenate([locs, dims[:, [1, 0, 2]], rots], axis=1)
+ assert len(gt_boxes) == len(
+ annotations), f'{len(gt_boxes)}, {len(annotations)}'
+ info['gt_boxes'] = gt_boxes
+ info['gt_names'] = names
+ info['gt_velocity'] = velocity.reshape(-1, 2)
+ info['num_lidar_pts'] = np.array(
+ [a['num_lidar_pts'] for a in annotations])
+ info['num_radar_pts'] = np.array(
+ [a['num_radar_pts'] for a in annotations])
+ info['valid_flag'] = valid_flag
+
+ if sample['scene_token'] in train_scenes:
+ train_nusc_infos.append(info)
+ else:
+ val_nusc_infos.append(info)
+
+ return train_nusc_infos, val_nusc_infos
+
+
+def obtain_sensor2top(nusc,
+ sensor_token,
+ l2e_t,
+ l2e_r_mat,
+ e2g_t,
+ e2g_r_mat,
+ sensor_type='lidar'):
+ """Obtain the info with RT matric from general sensor to Top LiDAR.
+
+ Args:
+ nusc (class): Dataset class in the nuScenes dataset.
+ sensor_token (str): Sample data token corresponding to the
+ specific sensor type.
+ l2e_t (np.ndarray): Translation from lidar to ego in shape (1, 3).
+ l2e_r_mat (np.ndarray): Rotation matrix from lidar to ego
+ in shape (3, 3).
+ e2g_t (np.ndarray): Translation from ego to global in shape (1, 3).
+ e2g_r_mat (np.ndarray): Rotation matrix from ego to global
+ in shape (3, 3).
+ sensor_type (str, optional): Sensor to calibrate. Default: 'lidar'.
+
+ Returns:
+ sweep (dict): Sweep information after transformation.
+ """
+ sd_rec = nusc.get('sample_data', sensor_token)
+ cs_record = nusc.get('calibrated_sensor',
+ sd_rec['calibrated_sensor_token'])
+ pose_record = nusc.get('ego_pose', sd_rec['ego_pose_token'])
+ data_path = str(nusc.get_sample_data_path(sd_rec['token']))
+ if os.getcwd() in data_path: # path from lyftdataset is absolute path
+ data_path = data_path.split(f'{os.getcwd()}/')[-1] # relative path
+ sweep = {
+ 'data_path': data_path,
+ 'type': sensor_type,
+ 'sample_data_token': sd_rec['token'],
+ 'sensor2ego_translation': cs_record['translation'],
+ 'sensor2ego_rotation': cs_record['rotation'],
+ 'ego2global_translation': pose_record['translation'],
+ 'ego2global_rotation': pose_record['rotation'],
+ 'timestamp': sd_rec['timestamp']
+ }
+ l2e_r_s = sweep['sensor2ego_rotation']
+ l2e_t_s = sweep['sensor2ego_translation']
+ e2g_r_s = sweep['ego2global_rotation']
+ e2g_t_s = sweep['ego2global_translation']
+
+ # obtain the RT from sensor to Top LiDAR
+ # sweep->ego->global->ego'->lidar
+ l2e_r_s_mat = Quaternion(l2e_r_s).rotation_matrix
+ e2g_r_s_mat = Quaternion(e2g_r_s).rotation_matrix
+ R = (l2e_r_s_mat.T @ e2g_r_s_mat.T) @ (
+ np.linalg.inv(e2g_r_mat).T @ np.linalg.inv(l2e_r_mat).T)
+ T = (l2e_t_s @ e2g_r_s_mat.T + e2g_t_s) @ (
+ np.linalg.inv(e2g_r_mat).T @ np.linalg.inv(l2e_r_mat).T)
+ T -= e2g_t @ (np.linalg.inv(e2g_r_mat).T @ np.linalg.inv(l2e_r_mat).T
+ ) + l2e_t @ np.linalg.inv(l2e_r_mat).T
+ sweep['sensor2lidar_rotation'] = R.T # points @ R.T + T
+ sweep['sensor2lidar_translation'] = T
+ return sweep
+
+
+def export_2d_annotation(root_path, info_path, version, mono3d=True):
+ """Export 2d annotation from the info file and raw data.
+
+ Args:
+ root_path (str): Root path of the raw data.
+ info_path (str): Path of the info file.
+ version (str): Dataset version.
+ mono3d (bool, optional): Whether to export mono3d annotation.
+ Default: True.
+ """
+ # get bbox annotations for camera
+ camera_types = [
+ 'CAM_FRONT',
+ 'CAM_FRONT_RIGHT',
+ 'CAM_FRONT_LEFT',
+ 'CAM_BACK',
+ 'CAM_BACK_LEFT',
+ 'CAM_BACK_RIGHT',
+ ]
+ nusc_infos = mmcv.load(info_path)['infos']
+ nusc = NuScenes(version=version, dataroot=root_path, verbose=True)
+ # info_2d_list = []
+ cat2Ids = [
+ dict(id=nus_categories.index(cat_name), name=cat_name)
+ for cat_name in nus_categories
+ ]
+ coco_ann_id = 0
+ coco_2d_dict = dict(annotations=[], images=[], categories=cat2Ids)
+ for info in mmcv.track_iter_progress(nusc_infos):
+ for cam in camera_types:
+ cam_info = info['cams'][cam]
+ coco_infos = get_2d_boxes(
+ nusc,
+ cam_info['sample_data_token'],
+ visibilities=['', '1', '2', '3', '4'],
+ mono3d=mono3d)
+ (height, width, _) = mmcv.imread(cam_info['data_path']).shape
+ coco_2d_dict['images'].append(
+ dict(
+ file_name=cam_info['data_path'].split('data/nuscenes/')
+ [-1],
+ id=cam_info['sample_data_token'],
+ token=info['token'],
+ cam2ego_rotation=cam_info['sensor2ego_rotation'],
+ cam2ego_translation=cam_info['sensor2ego_translation'],
+ ego2global_rotation=info['ego2global_rotation'],
+ ego2global_translation=info['ego2global_translation'],
+ cam_intrinsic=cam_info['cam_intrinsic'],
+ width=width,
+ height=height))
+ for coco_info in coco_infos:
+ if coco_info is None:
+ continue
+ # add an empty key for coco format
+ coco_info['segmentation'] = []
+ coco_info['id'] = coco_ann_id
+ coco_2d_dict['annotations'].append(coco_info)
+ coco_ann_id += 1
+ if mono3d:
+ json_prefix = f'{info_path[:-4]}_mono3d'
+ else:
+ json_prefix = f'{info_path[:-4]}'
+ mmcv.dump(coco_2d_dict, f'{json_prefix}.coco.json')
+
+
+def get_2d_boxes(nusc,
+ sample_data_token: str,
+ visibilities: List[str],
+ mono3d=True):
+ """Get the 2D annotation records for a given `sample_data_token`.
+
+ Args:
+ sample_data_token (str): Sample data token belonging to a camera
+ keyframe.
+ visibilities (list[str]): Visibility filter.
+ mono3d (bool): Whether to get boxes with mono3d annotation.
+
+ Return:
+ list[dict]: List of 2D annotation record that belongs to the input
+ `sample_data_token`.
+ """
+
+ # Get the sample data and the sample corresponding to that sample data.
+ sd_rec = nusc.get('sample_data', sample_data_token)
+
+ assert sd_rec[
+ 'sensor_modality'] == 'camera', 'Error: get_2d_boxes only works' \
+ ' for camera sample_data!'
+ if not sd_rec['is_key_frame']:
+ raise ValueError(
+ 'The 2D re-projections are available only for keyframes.')
+
+ s_rec = nusc.get('sample', sd_rec['sample_token'])
+
+ # Get the calibrated sensor and ego pose
+ # record to get the transformation matrices.
+ cs_rec = nusc.get('calibrated_sensor', sd_rec['calibrated_sensor_token'])
+ pose_rec = nusc.get('ego_pose', sd_rec['ego_pose_token'])
+ camera_intrinsic = np.array(cs_rec['camera_intrinsic'])
+
+ # Get all the annotation with the specified visibilties.
+ ann_recs = [
+ nusc.get('sample_annotation', token) for token in s_rec['anns']
+ ]
+ ann_recs = [
+ ann_rec for ann_rec in ann_recs
+ if (ann_rec['visibility_token'] in visibilities)
+ ]
+
+ repro_recs = []
+
+ for ann_rec in ann_recs:
+ # Augment sample_annotation with token information.
+ ann_rec['sample_annotation_token'] = ann_rec['token']
+ ann_rec['sample_data_token'] = sample_data_token
+
+ # Get the box in global coordinates.
+ box = nusc.get_box(ann_rec['token'])
+
+ # Move them to the ego-pose frame.
+ box.translate(-np.array(pose_rec['translation']))
+ box.rotate(Quaternion(pose_rec['rotation']).inverse)
+
+ # Move them to the calibrated sensor frame.
+ box.translate(-np.array(cs_rec['translation']))
+ box.rotate(Quaternion(cs_rec['rotation']).inverse)
+
+ # Filter out the corners that are not in front of the calibrated
+ # sensor.
+ corners_3d = box.corners()
+ in_front = np.argwhere(corners_3d[2, :] > 0).flatten()
+ corners_3d = corners_3d[:, in_front]
+
+ # Project 3d box to 2d.
+ corner_coords = view_points(corners_3d, camera_intrinsic,
+ True).T[:, :2].tolist()
+
+ # Keep only corners that fall within the image.
+ final_coords = post_process_coords(corner_coords)
+
+ # Skip if the convex hull of the re-projected corners
+ # does not intersect the image canvas.
+ if final_coords is None:
+ continue
+ else:
+ min_x, min_y, max_x, max_y = final_coords
+
+ # Generate dictionary record to be included in the .json file.
+ repro_rec = generate_record(ann_rec, min_x, min_y, max_x, max_y,
+ sample_data_token, sd_rec['filename'])
+
+ # If mono3d=True, add 3D annotations in camera coordinates
+ if mono3d and (repro_rec is not None):
+ loc = box.center.tolist()
+
+ dim = box.wlh
+ dim[[0, 1, 2]] = dim[[1, 2, 0]] # convert wlh to our lhw
+ dim = dim.tolist()
+
+ rot = box.orientation.yaw_pitch_roll[0]
+ rot = [-rot] # convert the rot to our cam coordinate
+
+ global_velo2d = nusc.box_velocity(box.token)[:2]
+ global_velo3d = np.array([*global_velo2d, 0.0])
+ e2g_r_mat = Quaternion(pose_rec['rotation']).rotation_matrix
+ c2e_r_mat = Quaternion(cs_rec['rotation']).rotation_matrix
+ cam_velo3d = global_velo3d @ np.linalg.inv(
+ e2g_r_mat).T @ np.linalg.inv(c2e_r_mat).T
+ velo = cam_velo3d[0::2].tolist()
+
+ repro_rec['bbox_cam3d'] = loc + dim + rot
+ repro_rec['velo_cam3d'] = velo
+
+ center3d = np.array(loc).reshape([1, 3])
+ center2d = points_cam2img(
+ center3d, camera_intrinsic, with_depth=True)
+ repro_rec['center2d'] = center2d.squeeze().tolist()
+ # normalized center2D + depth
+ # if samples with depth < 0 will be removed
+ if repro_rec['center2d'][2] <= 0:
+ continue
+
+ ann_token = nusc.get('sample_annotation',
+ box.token)['attribute_tokens']
+ if len(ann_token) == 0:
+ attr_name = 'None'
+ else:
+ attr_name = nusc.get('attribute', ann_token[0])['name']
+ attr_id = nus_attributes.index(attr_name)
+ repro_rec['attribute_name'] = attr_name
+ repro_rec['attribute_id'] = attr_id
+
+ repro_recs.append(repro_rec)
+
+ return repro_recs
+
+
+def post_process_coords(
+ corner_coords: List, imsize: Tuple[int, int] = (1600, 900)
+) -> Union[Tuple[float, float, float, float], None]:
+ """Get the intersection of the convex hull of the reprojected bbox corners
+ and the image canvas, return None if no intersection.
+
+ Args:
+ corner_coords (list[int]): Corner coordinates of reprojected
+ bounding box.
+ imsize (tuple[int]): Size of the image canvas.
+
+ Return:
+ tuple [float]: Intersection of the convex hull of the 2D box
+ corners and the image canvas.
+ """
+ polygon_from_2d_box = MultiPoint(corner_coords).convex_hull
+ img_canvas = box(0, 0, imsize[0], imsize[1])
+
+ if polygon_from_2d_box.intersects(img_canvas):
+ img_intersection = polygon_from_2d_box.intersection(img_canvas)
+ intersection_coords = np.array(
+ [coord for coord in img_intersection.exterior.coords])
+
+ min_x = min(intersection_coords[:, 0])
+ min_y = min(intersection_coords[:, 1])
+ max_x = max(intersection_coords[:, 0])
+ max_y = max(intersection_coords[:, 1])
+
+ return min_x, min_y, max_x, max_y
+ else:
+ return None
+
+
+def generate_record(ann_rec: dict, x1: float, y1: float, x2: float, y2: float,
+ sample_data_token: str, filename: str) -> OrderedDict:
+ """Generate one 2D annotation record given various information on top of
+ the 2D bounding box coordinates.
+
+ Args:
+ ann_rec (dict): Original 3d annotation record.
+ x1 (float): Minimum value of the x coordinate.
+ y1 (float): Minimum value of the y coordinate.
+ x2 (float): Maximum value of the x coordinate.
+ y2 (float): Maximum value of the y coordinate.
+ sample_data_token (str): Sample data token.
+ filename (str):The corresponding image file where the annotation
+ is present.
+
+ Returns:
+ dict: A sample 2D annotation record.
+ - file_name (str): file name
+ - image_id (str): sample data token
+ - area (float): 2d box area
+ - category_name (str): category name
+ - category_id (int): category id
+ - bbox (list[float]): left x, top y, dx, dy of 2d box
+ - iscrowd (int): whether the area is crowd
+ """
+ repro_rec = OrderedDict()
+ repro_rec['sample_data_token'] = sample_data_token
+ coco_rec = dict()
+
+ relevant_keys = [
+ 'attribute_tokens',
+ 'category_name',
+ 'instance_token',
+ 'next',
+ 'num_lidar_pts',
+ 'num_radar_pts',
+ 'prev',
+ 'sample_annotation_token',
+ 'sample_data_token',
+ 'visibility_token',
+ ]
+
+ for key, value in ann_rec.items():
+ if key in relevant_keys:
+ repro_rec[key] = value
+
+ repro_rec['bbox_corners'] = [x1, y1, x2, y2]
+ repro_rec['filename'] = filename
+
+ coco_rec['file_name'] = filename
+ coco_rec['image_id'] = sample_data_token
+ coco_rec['area'] = (y2 - y1) * (x2 - x1)
+
+ if repro_rec['category_name'] not in NuScenesDataset.NameMapping:
+ return None
+ cat_name = NuScenesDataset.NameMapping[repro_rec['category_name']]
+ coco_rec['category_name'] = cat_name
+ coco_rec['category_id'] = nus_categories.index(cat_name)
+ coco_rec['bbox'] = [x1, y1, x2 - x1, y2 - y1]
+ coco_rec['iscrowd'] = 0
+
+ return coco_rec
diff --git a/tools/data_converter/s3dis_data_utils.py b/tools/data_converter/s3dis_data_utils.py
new file mode 100644
index 0000000..48f3788
--- /dev/null
+++ b/tools/data_converter/s3dis_data_utils.py
@@ -0,0 +1,245 @@
+# Copyright (c) OpenMMLab. All rights reserved.
+import os
+from concurrent import futures as futures
+from os import path as osp
+
+import mmcv
+import numpy as np
+
+
+class S3DISData(object):
+ """S3DIS data.
+
+ Generate s3dis infos for s3dis_converter.
+
+ Args:
+ root_path (str): Root path of the raw data.
+ split (str, optional): Set split type of the data. Default: 'Area_1'.
+ """
+
+ def __init__(self, root_path, split='Area_1'):
+ self.root_dir = root_path
+ self.split = split
+ self.data_dir = osp.join(root_path,
+ 'Stanford3dDataset_v1.2_Aligned_Version')
+
+ # Following `GSDN `_, use 5 furniture
+ # classes for detection: table, chair, sofa, bookcase, board.
+ self.cat_ids = np.array([7, 8, 9, 10, 11])
+ self.cat_ids2class = {
+ cat_id: i
+ for i, cat_id in enumerate(list(self.cat_ids))
+ }
+
+ assert split in [
+ 'Area_1', 'Area_2', 'Area_3', 'Area_4', 'Area_5', 'Area_6'
+ ]
+ self.sample_id_list = os.listdir(osp.join(self.data_dir,
+ split)) # conferenceRoom_1
+ for sample_id in self.sample_id_list:
+ if os.path.isfile(osp.join(self.data_dir, split, sample_id)):
+ self.sample_id_list.remove(sample_id)
+
+ def __len__(self):
+ return len(self.sample_id_list)
+
+ def get_infos(self, num_workers=4, has_label=True, sample_id_list=None):
+ """Get data infos.
+
+ This method gets information from the raw data.
+
+ Args:
+ num_workers (int, optional): Number of threads to be used.
+ Default: 4.
+ has_label (bool, optional): Whether the data has label.
+ Default: True.
+ sample_id_list (list[int], optional): Index list of the sample.
+ Default: None.
+
+ Returns:
+ infos (list[dict]): Information of the raw data.
+ """
+
+ def process_single_scene(sample_idx):
+ print(f'{self.split} sample_idx: {sample_idx}')
+ info = dict()
+ pc_info = {
+ 'num_features': 6,
+ 'lidar_idx': f'{self.split}_{sample_idx}'
+ }
+ info['point_cloud'] = pc_info
+ pts_filename = osp.join(self.root_dir, 's3dis_data',
+ f'{self.split}_{sample_idx}_point.npy')
+ pts_instance_mask_path = osp.join(
+ self.root_dir, 's3dis_data',
+ f'{self.split}_{sample_idx}_ins_label.npy')
+ pts_semantic_mask_path = osp.join(
+ self.root_dir, 's3dis_data',
+ f'{self.split}_{sample_idx}_sem_label.npy')
+
+ points = np.load(pts_filename).astype(np.float32)
+ pts_instance_mask = np.load(pts_instance_mask_path).astype(np.int)
+ pts_semantic_mask = np.load(pts_semantic_mask_path).astype(np.int)
+
+ mmcv.mkdir_or_exist(osp.join(self.root_dir, 'points'))
+ mmcv.mkdir_or_exist(osp.join(self.root_dir, 'instance_mask'))
+ mmcv.mkdir_or_exist(osp.join(self.root_dir, 'semantic_mask'))
+
+ points.tofile(
+ osp.join(self.root_dir, 'points',
+ f'{self.split}_{sample_idx}.bin'))
+ pts_instance_mask.tofile(
+ osp.join(self.root_dir, 'instance_mask',
+ f'{self.split}_{sample_idx}.bin'))
+ pts_semantic_mask.tofile(
+ osp.join(self.root_dir, 'semantic_mask',
+ f'{self.split}_{sample_idx}.bin'))
+
+ info['pts_path'] = osp.join('points',
+ f'{self.split}_{sample_idx}.bin')
+ info['pts_instance_mask_path'] = osp.join(
+ 'instance_mask', f'{self.split}_{sample_idx}.bin')
+ info['pts_semantic_mask_path'] = osp.join(
+ 'semantic_mask', f'{self.split}_{sample_idx}.bin')
+ info['annos'] = self.get_bboxes(points, pts_instance_mask,
+ pts_semantic_mask)
+
+ return info
+
+ sample_id_list = sample_id_list if sample_id_list is not None \
+ else self.sample_id_list
+ with futures.ThreadPoolExecutor(num_workers) as executor:
+ infos = executor.map(process_single_scene, sample_id_list)
+ return list(infos)
+
+ def get_bboxes(self, points, pts_instance_mask, pts_semantic_mask):
+ """Convert instance masks to axis-aligned bounding boxes.
+
+ Args:
+ points (np.array): Scene points of shape (n, 6).
+ pts_instance_mask (np.ndarray): Instance labels of shape (n,).
+ pts_semantic_mask (np.ndarray): Semantic labels of shape (n,).
+
+ Returns:
+ dict: A dict containing detection infos with following keys:
+
+ - gt_boxes_upright_depth (np.ndarray): Bounding boxes
+ of shape (n, 6)
+ - class (np.ndarray): Box labels of shape (n,)
+ - gt_num (int): Number of boxes.
+ """
+ bboxes, labels = [], []
+ for i in range(1, pts_instance_mask.max() + 1):
+ ids = pts_instance_mask == i
+ # check if all instance points have same semantic label
+ assert pts_semantic_mask[ids].min() == pts_semantic_mask[ids].max()
+ label = pts_semantic_mask[ids][0]
+ # keep only furniture objects
+ if label in self.cat_ids2class:
+ labels.append(self.cat_ids2class[pts_semantic_mask[ids][0]])
+ pts = points[:, :3][ids]
+ min_pts = pts.min(axis=0)
+ max_pts = pts.max(axis=0)
+ locations = (min_pts + max_pts) / 2
+ dimensions = max_pts - min_pts
+ bboxes.append(np.concatenate((locations, dimensions)))
+ annotation = dict()
+ # follow ScanNet and SUN RGB-D keys
+ annotation['gt_boxes_upright_depth'] = np.array(bboxes)
+ annotation['class'] = np.array(labels)
+ annotation['gt_num'] = len(labels)
+ return annotation
+
+
+class S3DISSegData(object):
+ """S3DIS dataset used to generate infos for semantic segmentation task.
+
+ Args:
+ data_root (str): Root path of the raw data.
+ ann_file (str): The generated scannet infos.
+ split (str, optional): Set split type of the data. Default: 'train'.
+ num_points (int, optional): Number of points in each data input.
+ Default: 8192.
+ label_weight_func (function, optional): Function to compute the
+ label weight. Default: None.
+ """
+
+ def __init__(self,
+ data_root,
+ ann_file,
+ split='Area_1',
+ num_points=4096,
+ label_weight_func=None):
+ self.data_root = data_root
+ self.data_infos = mmcv.load(ann_file)
+ self.split = split
+ self.num_points = num_points
+
+ self.all_ids = np.arange(13) # all possible ids
+ self.cat_ids = np.array([0, 1, 2, 3, 4, 5, 6, 7, 8, 9, 10, 11,
+ 12]) # used for seg task
+ self.ignore_index = len(self.cat_ids)
+
+ self.cat_id2class = np.ones((self.all_ids.shape[0],), dtype=np.int) * \
+ self.ignore_index
+ for i, cat_id in enumerate(self.cat_ids):
+ self.cat_id2class[cat_id] = i
+
+ # label weighting function is taken from
+ # https://github.com/charlesq34/pointnet2/blob/master/scannet/scannet_dataset.py#L24
+ self.label_weight_func = (lambda x: 1.0 / np.log(1.2 + x)) if \
+ label_weight_func is None else label_weight_func
+
+ def get_seg_infos(self):
+ scene_idxs, label_weight = self.get_scene_idxs_and_label_weight()
+ save_folder = osp.join(self.data_root, 'seg_info')
+ mmcv.mkdir_or_exist(save_folder)
+ np.save(
+ osp.join(save_folder, f'{self.split}_resampled_scene_idxs.npy'),
+ scene_idxs)
+ np.save(
+ osp.join(save_folder, f'{self.split}_label_weight.npy'),
+ label_weight)
+ print(f'{self.split} resampled scene index and label weight saved')
+
+ def _convert_to_label(self, mask):
+ """Convert class_id in loaded segmentation mask to label."""
+ if isinstance(mask, str):
+ if mask.endswith('npy'):
+ mask = np.load(mask)
+ else:
+ mask = np.fromfile(mask, dtype=np.int64)
+ label = self.cat_id2class[mask]
+ return label
+
+ def get_scene_idxs_and_label_weight(self):
+ """Compute scene_idxs for data sampling and label weight for loss
+ calculation.
+
+ We sample more times for scenes with more points. Label_weight is
+ inversely proportional to number of class points.
+ """
+ num_classes = len(self.cat_ids)
+ num_point_all = []
+ label_weight = np.zeros((num_classes + 1, )) # ignore_index
+ for data_info in self.data_infos:
+ label = self._convert_to_label(
+ osp.join(self.data_root, data_info['pts_semantic_mask_path']))
+ num_point_all.append(label.shape[0])
+ class_count, _ = np.histogram(label, range(num_classes + 2))
+ label_weight += class_count
+
+ # repeat scene_idx for num_scene_point // num_sample_point times
+ sample_prob = np.array(num_point_all) / float(np.sum(num_point_all))
+ num_iter = int(np.sum(num_point_all) / float(self.num_points))
+ scene_idxs = []
+ for idx in range(len(self.data_infos)):
+ scene_idxs.extend([idx] * int(round(sample_prob[idx] * num_iter)))
+ scene_idxs = np.array(scene_idxs).astype(np.int32)
+
+ # calculate label weight, adopted from PointNet++
+ label_weight = label_weight[:-1].astype(np.float32)
+ label_weight = label_weight / label_weight.sum()
+ label_weight = self.label_weight_func(label_weight).astype(np.float32)
+
+ return scene_idxs, label_weight
diff --git a/tools/data_converter/scannet_data_utils.py b/tools/data_converter/scannet_data_utils.py
new file mode 100644
index 0000000..f41b3b7
--- /dev/null
+++ b/tools/data_converter/scannet_data_utils.py
@@ -0,0 +1,320 @@
+# Copyright (c) OpenMMLab. All rights reserved.
+import os
+from concurrent import futures as futures
+from os import path as osp
+
+import mmcv
+import numpy as np
+
+
+class ScanNetData(object):
+ """ScanNet data.
+
+ Generate scannet infos for scannet_converter.
+
+ Args:
+ root_path (str): Root path of the raw data.
+ split (str, optional): Set split type of the data. Default: 'train'.
+ """
+
+ def __init__(self, root_path, split='train', scannet200=False, save_path=None):
+ self.root_dir = root_path
+ self.save_path = root_path if save_path is None else save_path
+ self.split = split
+ self.split_dir = osp.join(root_path)
+ self.scannet200 = scannet200
+ if self.scannet200:
+ self.classes = [
+ 'chair', 'table', 'door', 'couch', 'cabinet', 'shelf', 'desk', 'office chair', 'bed', 'pillow', 'sink', 'picture', 'window', 'toilet', 'bookshelf', 'monitor', 'curtain', 'book', 'armchair', 'coffee table', 'box',
+ 'refrigerator', 'lamp', 'kitchen cabinet', 'towel', 'clothes', 'tv', 'nightstand', 'counter', 'dresser', 'stool', 'cushion', 'plant', 'ceiling', 'bathtub', 'end table', 'dining table', 'keyboard', 'bag', 'backpack', 'toilet paper',
+ 'printer', 'tv stand', 'whiteboard', 'blanket', 'shower curtain', 'trash can', 'closet', 'stairs', 'microwave', 'stove', 'shoe', 'computer tower', 'bottle', 'bin', 'ottoman', 'bench', 'board', 'washing machine', 'mirror', 'copier',
+ 'basket', 'sofa chair', 'file cabinet', 'fan', 'laptop', 'shower', 'paper', 'person', 'paper towel dispenser', 'oven', 'blinds', 'rack', 'plate', 'blackboard', 'piano', 'suitcase', 'rail', 'radiator', 'recycling bin', 'container',
+ 'wardrobe', 'soap dispenser', 'telephone', 'bucket', 'clock', 'stand', 'light', 'laundry basket', 'pipe', 'clothes dryer', 'guitar', 'toilet paper holder', 'seat', 'speaker', 'column', 'bicycle', 'ladder', 'bathroom stall', 'shower wall',
+ 'cup', 'jacket', 'storage bin', 'coffee maker', 'dishwasher', 'paper towel roll', 'machine', 'mat', 'windowsill', 'bar', 'toaster', 'bulletin board', 'ironing board', 'fireplace', 'soap dish', 'kitchen counter', 'doorframe',
+ 'toilet paper dispenser', 'mini fridge', 'fire extinguisher', 'ball', 'hat', 'shower curtain rod', 'water cooler', 'paper cutter', 'tray', 'shower door', 'pillar', 'ledge', 'toaster oven', 'mouse', 'toilet seat cover dispenser',
+ 'furniture', 'cart', 'storage container', 'scale', 'tissue box', 'light switch', 'crate', 'power outlet', 'decoration', 'sign', 'projector', 'closet door', 'vacuum cleaner', 'candle', 'plunger', 'stuffed animal', 'headphones', 'dish rack',
+ 'broom', 'guitar case', 'range hood', 'dustpan', 'hair dryer', 'water bottle', 'handicap bar', 'purse', 'vent', 'shower floor', 'water pitcher', 'mailbox', 'bowl', 'paper bag', 'alarm clock', 'music stand', 'projector screen', 'divider',
+ 'laundry detergent', 'bathroom counter', 'object', 'bathroom vanity', 'closet wall', 'laundry hamper', 'bathroom stall door', 'ceiling light', 'trash bin', 'dumbbell', 'stair rail', 'tube', 'bathroom cabinet', 'cd case', 'closet rod',
+ 'coffee kettle', 'structure', 'shower head', 'keyboard piano', 'case of water bottles', 'coat rack', 'storage organizer', 'folded chair', 'fire alarm', 'power strip', 'calendar', 'poster', 'potted plant', 'luggage', 'mattress'
+ ]
+ self.cat_ids = np.array(
+ [2, 4, 5, 6, 7, 8, 9, 10, 11, 13, 14, 15, 16, 17, 18, 19, 21, 22, 23, 24, 26, 27, 28, 29, 31, 32, 33, 34, 35, 36, 38, 39, 40, 41, 42, 44, 45, 46, 47, 48, 49, 50, 51, 52, 54, 55, 56, 57, 58, 59, 62, 63, 64, 65, 66, 67, 68, 69, 70, 71,
+ 72, 73, 74, 75, 76, 77, 78, 79, 80, 82, 84, 86, 87, 88, 89, 90, 93, 95, 96, 97, 98, 99, 100, 101, 102, 103, 104, 105, 106, 107, 110, 112, 115, 116, 118, 120, 121, 122, 125, 128, 130, 131, 132, 134, 136, 138, 139, 140, 141, 145, 148, 154,
+ 155, 156, 157, 159, 161, 163, 165, 166, 168, 169, 170, 177, 180, 185, 188, 191, 193, 195, 202, 208, 213, 214, 221, 229, 230, 232, 233, 242, 250, 261, 264, 276, 283, 286, 300, 304, 312, 323, 325, 331, 342, 356, 370, 392, 395, 399, 408, 417,
+ 488, 540, 562, 570, 572, 581, 609, 748, 776, 1156, 1163, 1164, 1165, 1166, 1167, 1168, 1169, 1170, 1171, 1172, 1173, 1174, 1175, 1176, 1178, 1179, 1180, 1181, 1182, 1183, 1184, 1185, 1186, 1187, 1188, 1189, 1190, 1191])
+ else:
+ self.classes = [
+ 'cabinet', 'bed', 'chair', 'sofa', 'table', 'door', 'window',
+ 'bookshelf', 'picture', 'counter', 'desk', 'curtain',
+ 'refrigerator', 'showercurtrain', 'toilet', 'sink', 'bathtub',
+ 'garbagebin'
+ ]
+ self.cat_ids = np.array(
+ [3, 4, 5, 6, 7, 8, 9, 10, 11, 12, 14, 16, 24, 28, 33, 34, 36, 39])
+
+ self.cat2label = {cat: self.classes.index(cat) for cat in self.classes}
+ self.label2cat = {self.cat2label[t]: t for t in self.cat2label}
+ self.cat_ids2class = {
+ nyu40id: i
+ for i, nyu40id in enumerate(list(self.cat_ids))
+ }
+ assert split in ['train', 'val', 'test']
+ split_file = osp.join(self.root_dir, 'meta_data',
+ f'scannetv2_{split}.txt')
+ mmcv.check_file_exist(split_file)
+ self.sample_id_list = mmcv.list_from_file(split_file)
+ self.test_mode = (split == 'test')
+
+ def __len__(self):
+ return len(self.sample_id_list)
+
+ def get_aligned_box_label(self, idx):
+ box_file = osp.join(self.root_dir, 'scannet_instance_data',
+ f'{idx}_aligned_bbox.npy')
+ mmcv.check_file_exist(box_file)
+ return np.load(box_file)
+
+ def get_unaligned_box_label(self, idx):
+ box_file = osp.join(self.root_dir, 'scannet_instance_data',
+ f'{idx}_unaligned_bbox.npy')
+ mmcv.check_file_exist(box_file)
+ return np.load(box_file)
+
+ def get_axis_align_matrix(self, idx):
+ matrix_file = osp.join(self.root_dir, 'scannet_instance_data',
+ f'{idx}_axis_align_matrix.npy')
+ mmcv.check_file_exist(matrix_file)
+ return np.load(matrix_file)
+
+ def get_images(self, idx):
+ paths = []
+ path = osp.join(self.root_dir, 'posed_images', idx)
+ for file in sorted(os.listdir(path)):
+ if file.endswith('.jpg'):
+ paths.append(osp.join('posed_images', idx, file))
+ return paths
+
+ def get_extrinsics(self, idx):
+ extrinsics = []
+ path = osp.join(self.root_dir, 'posed_images', idx)
+ for file in sorted(os.listdir(path)):
+ if file.endswith('.txt') and not file == 'intrinsic.txt':
+ extrinsics.append(np.loadtxt(osp.join(path, file)))
+ return extrinsics
+
+ def get_intrinsics(self, idx):
+ matrix_file = osp.join(self.root_dir, 'posed_images', idx,
+ 'intrinsic.txt')
+ mmcv.check_file_exist(matrix_file)
+ return np.loadtxt(matrix_file)
+
+ def get_infos(self, num_workers=4, has_label=True, sample_id_list=None):
+ """Get data infos.
+
+ This method gets information from the raw data.
+
+ Args:
+ num_workers (int, optional): Number of threads to be used.
+ Default: 4.
+ has_label (bool, optional): Whether the data has label.
+ Default: True.
+ sample_id_list (list[int], optional): Index list of the sample.
+ Default: None.
+
+ Returns:
+ infos (list[dict]): Information of the raw data.
+ """
+
+ def process_single_scene(sample_idx):
+ print(f'{self.split} sample_idx: {sample_idx}')
+ info = dict()
+ pc_info = {'num_features': 6, 'lidar_idx': sample_idx}
+ info['point_cloud'] = pc_info
+ pts_filename = osp.join(self.root_dir, 'scannet_instance_data',
+ f'{sample_idx}_vert.npy')
+ points = np.load(pts_filename)
+ mmcv.mkdir_or_exist(osp.join(self.save_path, 'points'))
+ points.tofile(
+ osp.join(self.save_path, 'points', f'{sample_idx}.bin'))
+ info['pts_path'] = osp.join('points', f'{sample_idx}.bin')
+
+ # update with RGB image paths if exist
+ if os.path.exists(osp.join(self.root_dir, 'posed_images')):
+ info['intrinsics'] = self.get_intrinsics(sample_idx)
+ all_extrinsics = self.get_extrinsics(sample_idx)
+ all_img_paths = self.get_images(sample_idx)
+ # some poses in ScanNet are invalid
+ extrinsics, img_paths = [], []
+ for extrinsic, img_path in zip(all_extrinsics, all_img_paths):
+ if np.all(np.isfinite(extrinsic)):
+ img_paths.append(img_path)
+ extrinsics.append(extrinsic)
+ info['extrinsics'] = extrinsics
+ info['img_paths'] = img_paths
+
+ if not self.test_mode:
+ pts_instance_mask_path = osp.join(
+ self.root_dir, 'scannet_instance_data',
+ f'{sample_idx}_ins_label.npy')
+ pts_semantic_mask_path = osp.join(
+ self.root_dir, 'scannet_instance_data',
+ f'{sample_idx}_sem_label.npy')
+
+ pts_instance_mask = np.load(pts_instance_mask_path).astype(
+ np.int64)
+ pts_semantic_mask = np.load(pts_semantic_mask_path).astype(
+ np.int64)
+
+ mmcv.mkdir_or_exist(osp.join(self.save_path, 'instance_mask'))
+ mmcv.mkdir_or_exist(osp.join(self.save_path, 'semantic_mask'))
+
+ pts_instance_mask.tofile(
+ osp.join(self.save_path, 'instance_mask',
+ f'{sample_idx}.bin'))
+ pts_semantic_mask.tofile(
+ osp.join(self.save_path, 'semantic_mask',
+ f'{sample_idx}.bin'))
+
+ info['pts_instance_mask_path'] = osp.join(
+ 'instance_mask', f'{sample_idx}.bin')
+ info['pts_semantic_mask_path'] = osp.join(
+ 'semantic_mask', f'{sample_idx}.bin')
+
+ if has_label:
+ annotations = {}
+ # box is of shape [k, 6 + class]
+ aligned_box_label = self.get_aligned_box_label(sample_idx)
+ unaligned_box_label = self.get_unaligned_box_label(sample_idx)
+ annotations['gt_num'] = aligned_box_label.shape[0]
+ if annotations['gt_num'] != 0:
+ aligned_box = aligned_box_label[:, :-1] # k, 6
+ unaligned_box = unaligned_box_label[:, :-1]
+ classes = aligned_box_label[:, -1] # k
+ annotations['name'] = np.array([
+ self.label2cat[self.cat_ids2class[classes[i]]]
+ for i in range(annotations['gt_num'])
+ ])
+ # default names are given to aligned bbox for compatibility
+ # we also save unaligned bbox info with marked names
+ annotations['location'] = aligned_box[:, :3]
+ annotations['dimensions'] = aligned_box[:, 3:6]
+ annotations['gt_boxes_upright_depth'] = aligned_box
+ annotations['unaligned_location'] = unaligned_box[:, :3]
+ annotations['unaligned_dimensions'] = unaligned_box[:, 3:6]
+ annotations[
+ 'unaligned_gt_boxes_upright_depth'] = unaligned_box
+ annotations['index'] = np.arange(
+ annotations['gt_num'], dtype=np.int32)
+ annotations['class'] = np.array([
+ self.cat_ids2class[classes[i]]
+ for i in range(annotations['gt_num'])
+ ])
+ axis_align_matrix = self.get_axis_align_matrix(sample_idx)
+ annotations['axis_align_matrix'] = axis_align_matrix # 4x4
+ info['annos'] = annotations
+ return info
+
+ sample_id_list = sample_id_list if sample_id_list is not None \
+ else self.sample_id_list
+ with futures.ThreadPoolExecutor(num_workers) as executor:
+ infos = executor.map(process_single_scene, sample_id_list)
+ return list(infos)
+
+
+class ScanNetSegData(object):
+ """ScanNet dataset used to generate infos for semantic segmentation task.
+
+ Args:
+ data_root (str): Root path of the raw data.
+ ann_file (str): The generated scannet infos.
+ split (str, optional): Set split type of the data. Default: 'train'.
+ num_points (int, optional): Number of points in each data input.
+ Default: 8192.
+ label_weight_func (function, optional): Function to compute the
+ label weight. Default: None.
+ """
+
+ def __init__(self,
+ data_root,
+ ann_file,
+ split='train',
+ num_points=8192,
+ label_weight_func=None):
+ self.data_root = data_root
+ self.data_infos = mmcv.load(ann_file)
+ self.split = split
+ assert split in ['train', 'val', 'test']
+ self.num_points = num_points
+
+ self.all_ids = np.arange(41) # all possible ids
+ self.cat_ids = np.array([
+ 1, 2, 3, 4, 5, 6, 7, 8, 9, 10, 11, 12, 14, 16, 24, 28, 33, 34, 36,
+ 39
+ ]) # used for seg task
+ self.ignore_index = len(self.cat_ids)
+
+ self.cat_id2class = np.ones((self.all_ids.shape[0],), dtype=np.int) * \
+ self.ignore_index
+ for i, cat_id in enumerate(self.cat_ids):
+ self.cat_id2class[cat_id] = i
+
+ # label weighting function is taken from
+ # https://github.com/charlesq34/pointnet2/blob/master/scannet/scannet_dataset.py#L24
+ self.label_weight_func = (lambda x: 1.0 / np.log(1.2 + x)) if \
+ label_weight_func is None else label_weight_func
+
+ def get_seg_infos(self):
+ if self.split == 'test':
+ return
+ scene_idxs, label_weight = self.get_scene_idxs_and_label_weight()
+ save_folder = osp.join(self.data_root, 'seg_info')
+ mmcv.mkdir_or_exist(save_folder)
+ np.save(
+ osp.join(save_folder, f'{self.split}_resampled_scene_idxs.npy'),
+ scene_idxs)
+ np.save(
+ osp.join(save_folder, f'{self.split}_label_weight.npy'),
+ label_weight)
+ print(f'{self.split} resampled scene index and label weight saved')
+
+ def _convert_to_label(self, mask):
+ """Convert class_id in loaded segmentation mask to label."""
+ if isinstance(mask, str):
+ if mask.endswith('npy'):
+ mask = np.load(mask)
+ else:
+ mask = np.fromfile(mask, dtype=np.int64)
+ label = self.cat_id2class[mask]
+ return label
+
+ def get_scene_idxs_and_label_weight(self):
+ """Compute scene_idxs for data sampling and label weight for loss
+ calculation.
+
+ We sample more times for scenes with more points. Label_weight is
+ inversely proportional to number of class points.
+ """
+ num_classes = len(self.cat_ids)
+ num_point_all = []
+ label_weight = np.zeros((num_classes + 1, )) # ignore_index
+ for data_info in self.data_infos:
+ label = self._convert_to_label(
+ osp.join(self.data_root, data_info['pts_semantic_mask_path']))
+ num_point_all.append(label.shape[0])
+ class_count, _ = np.histogram(label, range(num_classes + 2))
+ label_weight += class_count
+
+ # repeat scene_idx for num_scene_point // num_sample_point times
+ sample_prob = np.array(num_point_all) / float(np.sum(num_point_all))
+ num_iter = int(np.sum(num_point_all) / float(self.num_points))
+ scene_idxs = []
+ for idx in range(len(self.data_infos)):
+ scene_idxs.extend([idx] * int(round(sample_prob[idx] * num_iter)))
+ scene_idxs = np.array(scene_idxs).astype(np.int32)
+
+ # calculate label weight, adopted from PointNet++
+ label_weight = label_weight[:-1].astype(np.float32)
+ label_weight = label_weight / label_weight.sum()
+ label_weight = self.label_weight_func(label_weight).astype(np.float32)
+
+ return scene_idxs, label_weight
diff --git a/tools/data_converter/sunrgbd_data_utils.py b/tools/data_converter/sunrgbd_data_utils.py
new file mode 100644
index 0000000..152ea42
--- /dev/null
+++ b/tools/data_converter/sunrgbd_data_utils.py
@@ -0,0 +1,226 @@
+# Copyright (c) OpenMMLab. All rights reserved.
+from concurrent import futures as futures
+from os import path as osp
+
+import mmcv
+import numpy as np
+from scipy import io as sio
+
+
+def random_sampling(points, num_points, replace=None, return_choices=False):
+ """Random sampling.
+
+ Sampling point cloud to a certain number of points.
+
+ Args:
+ points (ndarray): Point cloud.
+ num_points (int): The number of samples.
+ replace (bool): Whether the sample is with or without replacement.
+ return_choices (bool): Whether to return choices.
+
+ Returns:
+ points (ndarray): Point cloud after sampling.
+ """
+
+ if replace is None:
+ replace = (points.shape[0] < num_points)
+ choices = np.random.choice(points.shape[0], num_points, replace=replace)
+ if return_choices:
+ return points[choices], choices
+ else:
+ return points[choices]
+
+
+class SUNRGBDInstance(object):
+
+ def __init__(self, line):
+ data = line.split(' ')
+ data[1:] = [float(x) for x in data[1:]]
+ self.classname = data[0]
+ self.xmin = data[1]
+ self.ymin = data[2]
+ self.xmax = data[1] + data[3]
+ self.ymax = data[2] + data[4]
+ self.box2d = np.array([self.xmin, self.ymin, self.xmax, self.ymax])
+ self.centroid = np.array([data[5], data[6], data[7]])
+ self.width = data[8]
+ self.length = data[9]
+ self.height = data[10]
+ # data[9] is x_size (length), data[8] is y_size (width), data[10] is
+ # z_size (height) in our depth coordinate system,
+ # l corresponds to the size along the x axis
+ self.size = np.array([data[9], data[8], data[10]]) * 2
+ self.orientation = np.zeros((3, ))
+ self.orientation[0] = data[11]
+ self.orientation[1] = data[12]
+ self.heading_angle = np.arctan2(self.orientation[1],
+ self.orientation[0])
+ self.box3d = np.concatenate(
+ [self.centroid, self.size, self.heading_angle[None]])
+
+
+class SUNRGBDData(object):
+ """SUNRGBD data.
+
+ Generate scannet infos for sunrgbd_converter.
+
+ Args:
+ root_path (str): Root path of the raw data.
+ split (str, optional): Set split type of the data. Default: 'train'.
+ use_v1 (bool, optional): Whether to use v1. Default: False.
+ """
+
+ def __init__(self, root_path, split='train', use_v1=False):
+ self.root_dir = root_path
+ self.split = split
+ self.split_dir = osp.join(root_path, 'sunrgbd_trainval')
+ self.classes = [
+ 'bed', 'table', 'sofa', 'chair', 'toilet', 'desk', 'dresser',
+ 'night_stand', 'bookshelf', 'bathtub'
+ ]
+ self.cat2label = {cat: self.classes.index(cat) for cat in self.classes}
+ self.label2cat = {
+ label: self.classes[label]
+ for label in range(len(self.classes))
+ }
+ assert split in ['train', 'val', 'test']
+ split_file = osp.join(self.split_dir, f'{split}_data_idx.txt')
+ mmcv.check_file_exist(split_file)
+ self.sample_id_list = map(int, mmcv.list_from_file(split_file))
+ self.image_dir = osp.join(self.split_dir, 'image')
+ self.calib_dir = osp.join(self.split_dir, 'calib')
+ self.depth_dir = osp.join(self.split_dir, 'depth')
+ if use_v1:
+ self.label_dir = osp.join(self.split_dir, 'label_v1')
+ else:
+ self.label_dir = osp.join(self.split_dir, 'label')
+
+ def __len__(self):
+ return len(self.sample_id_list)
+
+ def get_image(self, idx):
+ img_filename = osp.join(self.image_dir, f'{idx:06d}.jpg')
+ return mmcv.imread(img_filename)
+
+ def get_image_shape(self, idx):
+ image = self.get_image(idx)
+ return np.array(image.shape[:2], dtype=np.int32)
+
+ def get_depth(self, idx):
+ depth_filename = osp.join(self.depth_dir, f'{idx:06d}.mat')
+ depth = sio.loadmat(depth_filename)['instance']
+ return depth
+
+ def get_calibration(self, idx):
+ calib_filepath = osp.join(self.calib_dir, f'{idx:06d}.txt')
+ lines = [line.rstrip() for line in open(calib_filepath)]
+ Rt = np.array([float(x) for x in lines[0].split(' ')])
+ Rt = np.reshape(Rt, (3, 3), order='F').astype(np.float32)
+ K = np.array([float(x) for x in lines[1].split(' ')])
+ K = np.reshape(K, (3, 3), order='F').astype(np.float32)
+ return K, Rt
+
+ def get_label_objects(self, idx):
+ label_filename = osp.join(self.label_dir, f'{idx:06d}.txt')
+ lines = [line.rstrip() for line in open(label_filename)]
+ objects = [SUNRGBDInstance(line) for line in lines]
+ return objects
+
+ def get_infos(self, num_workers=4, has_label=True, sample_id_list=None):
+ """Get data infos.
+
+ This method gets information from the raw data.
+
+ Args:
+ num_workers (int, optional): Number of threads to be used.
+ Default: 4.
+ has_label (bool, optional): Whether the data has label.
+ Default: True.
+ sample_id_list (list[int], optional): Index list of the sample.
+ Default: None.
+
+ Returns:
+ infos (list[dict]): Information of the raw data.
+ """
+
+ def process_single_scene(sample_idx):
+ print(f'{self.split} sample_idx: {sample_idx}')
+ # convert depth to points
+ SAMPLE_NUM = 50000
+ # TODO: Check whether can move the point
+ # sampling process during training.
+ pc_upright_depth = self.get_depth(sample_idx)
+ pc_upright_depth_subsampled = random_sampling(
+ pc_upright_depth, SAMPLE_NUM)
+
+ info = dict()
+ pc_info = {'num_features': 6, 'lidar_idx': sample_idx}
+ info['point_cloud'] = pc_info
+
+ mmcv.mkdir_or_exist(osp.join(self.root_dir, 'points'))
+ pc_upright_depth_subsampled.tofile(
+ osp.join(self.root_dir, 'points', f'{sample_idx:06d}.bin'))
+
+ info['pts_path'] = osp.join('points', f'{sample_idx:06d}.bin')
+ img_path = osp.join('image', f'{sample_idx:06d}.jpg')
+ image_info = {
+ 'image_idx': sample_idx,
+ 'image_shape': self.get_image_shape(sample_idx),
+ 'image_path': img_path
+ }
+ info['image'] = image_info
+
+ K, Rt = self.get_calibration(sample_idx)
+ calib_info = {'K': K, 'Rt': Rt}
+ info['calib'] = calib_info
+
+ if has_label:
+ obj_list = self.get_label_objects(sample_idx)
+ annotations = {}
+ annotations['gt_num'] = len([
+ obj.classname for obj in obj_list
+ if obj.classname in self.cat2label.keys()
+ ])
+ if annotations['gt_num'] != 0:
+ annotations['name'] = np.array([
+ obj.classname for obj in obj_list
+ if obj.classname in self.cat2label.keys()
+ ])
+ annotations['bbox'] = np.concatenate([
+ obj.box2d.reshape(1, 4) for obj in obj_list
+ if obj.classname in self.cat2label.keys()
+ ],
+ axis=0)
+ annotations['location'] = np.concatenate([
+ obj.centroid.reshape(1, 3) for obj in obj_list
+ if obj.classname in self.cat2label.keys()
+ ],
+ axis=0)
+ annotations['dimensions'] = 2 * np.array([
+ [obj.length, obj.width, obj.height] for obj in obj_list
+ if obj.classname in self.cat2label.keys()
+ ]) # lwh (depth) format
+ annotations['rotation_y'] = np.array([
+ obj.heading_angle for obj in obj_list
+ if obj.classname in self.cat2label.keys()
+ ])
+ annotations['index'] = np.arange(
+ len(obj_list), dtype=np.int32)
+ annotations['class'] = np.array([
+ self.cat2label[obj.classname] for obj in obj_list
+ if obj.classname in self.cat2label.keys()
+ ])
+ annotations['gt_boxes_upright_depth'] = np.stack(
+ [
+ obj.box3d for obj in obj_list
+ if obj.classname in self.cat2label.keys()
+ ],
+ axis=0) # (K,8)
+ info['annos'] = annotations
+ return info
+
+ sample_id_list = sample_id_list if \
+ sample_id_list is not None else self.sample_id_list
+ with futures.ThreadPoolExecutor(num_workers) as executor:
+ infos = executor.map(process_single_scene, sample_id_list)
+ return list(infos)
diff --git a/tools/data_converter/waymo_converter.py b/tools/data_converter/waymo_converter.py
new file mode 100644
index 0000000..f991514
--- /dev/null
+++ b/tools/data_converter/waymo_converter.py
@@ -0,0 +1,556 @@
+# Copyright (c) OpenMMLab. All rights reserved.
+r"""Adapted from `Waymo to KITTI converter
+ `_.
+"""
+
+try:
+ from waymo_open_dataset import dataset_pb2
+except ImportError:
+ raise ImportError(
+ 'Please run "pip install waymo-open-dataset-tf-2-1-0==1.2.0" '
+ 'to install the official devkit first.')
+
+from glob import glob
+from os.path import join
+
+import mmcv
+import numpy as np
+import tensorflow as tf
+from waymo_open_dataset.utils import range_image_utils, transform_utils
+from waymo_open_dataset.utils.frame_utils import \
+ parse_range_image_and_camera_projection
+
+
+class Waymo2KITTI(object):
+ """Waymo to KITTI converter.
+
+ This class serves as the converter to change the waymo raw data to KITTI
+ format.
+
+ Args:
+ load_dir (str): Directory to load waymo raw data.
+ save_dir (str): Directory to save data in KITTI format.
+ prefix (str): Prefix of filename. In general, 0 for training, 1 for
+ validation and 2 for testing.
+ workers (int, optional): Number of workers for the parallel process.
+ test_mode (bool, optional): Whether in the test_mode. Default: False.
+ """
+
+ def __init__(self,
+ load_dir,
+ save_dir,
+ prefix,
+ workers=64,
+ test_mode=False):
+ self.filter_empty_3dboxes = True
+ self.filter_no_label_zone_points = True
+
+ self.selected_waymo_classes = ['VEHICLE', 'PEDESTRIAN', 'CYCLIST']
+
+ # Only data collected in specific locations will be converted
+ # If set None, this filter is disabled
+ # Available options: location_sf (main dataset)
+ self.selected_waymo_locations = None
+ self.save_track_id = False
+
+ # turn on eager execution for older tensorflow versions
+ if int(tf.__version__.split('.')[0]) < 2:
+ tf.enable_eager_execution()
+
+ self.lidar_list = [
+ '_FRONT', '_FRONT_RIGHT', '_FRONT_LEFT', '_SIDE_RIGHT',
+ '_SIDE_LEFT'
+ ]
+ self.type_list = [
+ 'UNKNOWN', 'VEHICLE', 'PEDESTRIAN', 'SIGN', 'CYCLIST'
+ ]
+ self.waymo_to_kitti_class_map = {
+ 'UNKNOWN': 'DontCare',
+ 'PEDESTRIAN': 'Pedestrian',
+ 'VEHICLE': 'Car',
+ 'CYCLIST': 'Cyclist',
+ 'SIGN': 'Sign' # not in kitti
+ }
+
+ self.load_dir = load_dir
+ self.save_dir = save_dir
+ self.prefix = prefix
+ self.workers = int(workers)
+ self.test_mode = test_mode
+
+ self.tfrecord_pathnames = sorted(
+ glob(join(self.load_dir, '*.tfrecord')))
+
+ self.label_save_dir = f'{self.save_dir}/label_'
+ self.label_all_save_dir = f'{self.save_dir}/label_all'
+ self.image_save_dir = f'{self.save_dir}/image_'
+ self.calib_save_dir = f'{self.save_dir}/calib'
+ self.point_cloud_save_dir = f'{self.save_dir}/velodyne'
+ self.pose_save_dir = f'{self.save_dir}/pose'
+ self.timestamp_save_dir = f'{self.save_dir}/timestamp'
+
+ self.create_folder()
+
+ def convert(self):
+ """Convert action."""
+ print('Start converting ...')
+ mmcv.track_parallel_progress(self.convert_one, range(len(self)),
+ self.workers)
+ print('\nFinished ...')
+
+ def convert_one(self, file_idx):
+ """Convert action for single file.
+
+ Args:
+ file_idx (int): Index of the file to be converted.
+ """
+ pathname = self.tfrecord_pathnames[file_idx]
+ dataset = tf.data.TFRecordDataset(pathname, compression_type='')
+
+ for frame_idx, data in enumerate(dataset):
+
+ frame = dataset_pb2.Frame()
+ frame.ParseFromString(bytearray(data.numpy()))
+ if (self.selected_waymo_locations is not None
+ and frame.context.stats.location
+ not in self.selected_waymo_locations):
+ continue
+
+ self.save_image(frame, file_idx, frame_idx)
+ self.save_calib(frame, file_idx, frame_idx)
+ self.save_lidar(frame, file_idx, frame_idx)
+ self.save_pose(frame, file_idx, frame_idx)
+ self.save_timestamp(frame, file_idx, frame_idx)
+
+ if not self.test_mode:
+ self.save_label(frame, file_idx, frame_idx)
+
+ def __len__(self):
+ """Length of the filename list."""
+ return len(self.tfrecord_pathnames)
+
+ def save_image(self, frame, file_idx, frame_idx):
+ """Parse and save the images in png format.
+
+ Args:
+ frame (:obj:`Frame`): Open dataset frame proto.
+ file_idx (int): Current file index.
+ frame_idx (int): Current frame index.
+ """
+ for img in frame.images:
+ img_path = f'{self.image_save_dir}{str(img.name - 1)}/' + \
+ f'{self.prefix}{str(file_idx).zfill(3)}' + \
+ f'{str(frame_idx).zfill(3)}.png'
+ img = mmcv.imfrombytes(img.image)
+ mmcv.imwrite(img, img_path)
+
+ def save_calib(self, frame, file_idx, frame_idx):
+ """Parse and save the calibration data.
+
+ Args:
+ frame (:obj:`Frame`): Open dataset frame proto.
+ file_idx (int): Current file index.
+ frame_idx (int): Current frame index.
+ """
+ # waymo front camera to kitti reference camera
+ T_front_cam_to_ref = np.array([[0.0, -1.0, 0.0], [0.0, 0.0, -1.0],
+ [1.0, 0.0, 0.0]])
+ camera_calibs = []
+ R0_rect = [f'{i:e}' for i in np.eye(3).flatten()]
+ Tr_velo_to_cams = []
+ calib_context = ''
+
+ for camera in frame.context.camera_calibrations:
+ # extrinsic parameters
+ T_cam_to_vehicle = np.array(camera.extrinsic.transform).reshape(
+ 4, 4)
+ T_vehicle_to_cam = np.linalg.inv(T_cam_to_vehicle)
+ Tr_velo_to_cam = \
+ self.cart_to_homo(T_front_cam_to_ref) @ T_vehicle_to_cam
+ if camera.name == 1: # FRONT = 1, see dataset.proto for details
+ self.T_velo_to_front_cam = Tr_velo_to_cam.copy()
+ Tr_velo_to_cam = Tr_velo_to_cam[:3, :].reshape((12, ))
+ Tr_velo_to_cams.append([f'{i:e}' for i in Tr_velo_to_cam])
+
+ # intrinsic parameters
+ camera_calib = np.zeros((3, 4))
+ camera_calib[0, 0] = camera.intrinsic[0]
+ camera_calib[1, 1] = camera.intrinsic[1]
+ camera_calib[0, 2] = camera.intrinsic[2]
+ camera_calib[1, 2] = camera.intrinsic[3]
+ camera_calib[2, 2] = 1
+ camera_calib = list(camera_calib.reshape(12))
+ camera_calib = [f'{i:e}' for i in camera_calib]
+ camera_calibs.append(camera_calib)
+
+ # all camera ids are saved as id-1 in the result because
+ # camera 0 is unknown in the proto
+ for i in range(5):
+ calib_context += 'P' + str(i) + ': ' + \
+ ' '.join(camera_calibs[i]) + '\n'
+ calib_context += 'R0_rect' + ': ' + ' '.join(R0_rect) + '\n'
+ for i in range(5):
+ calib_context += 'Tr_velo_to_cam_' + str(i) + ': ' + \
+ ' '.join(Tr_velo_to_cams[i]) + '\n'
+
+ with open(
+ f'{self.calib_save_dir}/{self.prefix}' +
+ f'{str(file_idx).zfill(3)}{str(frame_idx).zfill(3)}.txt',
+ 'w+') as fp_calib:
+ fp_calib.write(calib_context)
+ fp_calib.close()
+
+ def save_lidar(self, frame, file_idx, frame_idx):
+ """Parse and save the lidar data in psd format.
+
+ Args:
+ frame (:obj:`Frame`): Open dataset frame proto.
+ file_idx (int): Current file index.
+ frame_idx (int): Current frame index.
+ """
+ range_images, camera_projections, range_image_top_pose = \
+ parse_range_image_and_camera_projection(frame)
+
+ # First return
+ points_0, cp_points_0, intensity_0, elongation_0, mask_indices_0 = \
+ self.convert_range_image_to_point_cloud(
+ frame,
+ range_images,
+ camera_projections,
+ range_image_top_pose,
+ ri_index=0
+ )
+ points_0 = np.concatenate(points_0, axis=0)
+ intensity_0 = np.concatenate(intensity_0, axis=0)
+ elongation_0 = np.concatenate(elongation_0, axis=0)
+ mask_indices_0 = np.concatenate(mask_indices_0, axis=0)
+
+ # Second return
+ points_1, cp_points_1, intensity_1, elongation_1, mask_indices_1 = \
+ self.convert_range_image_to_point_cloud(
+ frame,
+ range_images,
+ camera_projections,
+ range_image_top_pose,
+ ri_index=1
+ )
+ points_1 = np.concatenate(points_1, axis=0)
+ intensity_1 = np.concatenate(intensity_1, axis=0)
+ elongation_1 = np.concatenate(elongation_1, axis=0)
+ mask_indices_1 = np.concatenate(mask_indices_1, axis=0)
+
+ points = np.concatenate([points_0, points_1], axis=0)
+ intensity = np.concatenate([intensity_0, intensity_1], axis=0)
+ elongation = np.concatenate([elongation_0, elongation_1], axis=0)
+ mask_indices = np.concatenate([mask_indices_0, mask_indices_1], axis=0)
+
+ # timestamp = frame.timestamp_micros * np.ones_like(intensity)
+
+ # concatenate x,y,z, intensity, elongation, timestamp (6-dim)
+ point_cloud = np.column_stack(
+ (points, intensity, elongation, mask_indices))
+
+ pc_path = f'{self.point_cloud_save_dir}/{self.prefix}' + \
+ f'{str(file_idx).zfill(3)}{str(frame_idx).zfill(3)}.bin'
+ point_cloud.astype(np.float32).tofile(pc_path)
+
+ def save_label(self, frame, file_idx, frame_idx):
+ """Parse and save the label data in txt format.
+ The relation between waymo and kitti coordinates is noteworthy:
+ 1. x, y, z correspond to l, w, h (waymo) -> l, h, w (kitti)
+ 2. x-y-z: front-left-up (waymo) -> right-down-front(kitti)
+ 3. bbox origin at volumetric center (waymo) -> bottom center (kitti)
+ 4. rotation: +x around y-axis (kitti) -> +x around z-axis (waymo)
+
+ Args:
+ frame (:obj:`Frame`): Open dataset frame proto.
+ file_idx (int): Current file index.
+ frame_idx (int): Current frame index.
+ """
+ fp_label_all = open(
+ f'{self.label_all_save_dir}/{self.prefix}' +
+ f'{str(file_idx).zfill(3)}{str(frame_idx).zfill(3)}.txt', 'w+')
+ id_to_bbox = dict()
+ id_to_name = dict()
+ for labels in frame.projected_lidar_labels:
+ name = labels.name
+ for label in labels.labels:
+ # TODO: need a workaround as bbox may not belong to front cam
+ bbox = [
+ label.box.center_x - label.box.length / 2,
+ label.box.center_y - label.box.width / 2,
+ label.box.center_x + label.box.length / 2,
+ label.box.center_y + label.box.width / 2
+ ]
+ id_to_bbox[label.id] = bbox
+ id_to_name[label.id] = name - 1
+
+ for obj in frame.laser_labels:
+ bounding_box = None
+ name = None
+ id = obj.id
+ for lidar in self.lidar_list:
+ if id + lidar in id_to_bbox:
+ bounding_box = id_to_bbox.get(id + lidar)
+ name = str(id_to_name.get(id + lidar))
+ break
+
+ if bounding_box is None or name is None:
+ name = '0'
+ bounding_box = (0, 0, 0, 0)
+
+ my_type = self.type_list[obj.type]
+
+ if my_type not in self.selected_waymo_classes:
+ continue
+
+ if self.filter_empty_3dboxes and obj.num_lidar_points_in_box < 1:
+ continue
+
+ my_type = self.waymo_to_kitti_class_map[my_type]
+
+ height = obj.box.height
+ width = obj.box.width
+ length = obj.box.length
+
+ x = obj.box.center_x
+ y = obj.box.center_y
+ z = obj.box.center_z - height / 2
+
+ # project bounding box to the virtual reference frame
+ pt_ref = self.T_velo_to_front_cam @ \
+ np.array([x, y, z, 1]).reshape((4, 1))
+ x, y, z, _ = pt_ref.flatten().tolist()
+
+ rotation_y = -obj.box.heading - np.pi / 2
+ track_id = obj.id
+
+ # not available
+ truncated = 0
+ occluded = 0
+ alpha = -10
+
+ line = my_type + \
+ ' {} {} {} {} {} {} {} {} {} {} {} {} {} {}\n'.format(
+ round(truncated, 2), occluded, round(alpha, 2),
+ round(bounding_box[0], 2), round(bounding_box[1], 2),
+ round(bounding_box[2], 2), round(bounding_box[3], 2),
+ round(height, 2), round(width, 2), round(length, 2),
+ round(x, 2), round(y, 2), round(z, 2),
+ round(rotation_y, 2))
+
+ if self.save_track_id:
+ line_all = line[:-1] + ' ' + name + ' ' + track_id + '\n'
+ else:
+ line_all = line[:-1] + ' ' + name + '\n'
+
+ fp_label = open(
+ f'{self.label_save_dir}{name}/{self.prefix}' +
+ f'{str(file_idx).zfill(3)}{str(frame_idx).zfill(3)}.txt', 'a')
+ fp_label.write(line)
+ fp_label.close()
+
+ fp_label_all.write(line_all)
+
+ fp_label_all.close()
+
+ def save_pose(self, frame, file_idx, frame_idx):
+ """Parse and save the pose data.
+
+ Note that SDC's own pose is not included in the regular training
+ of KITTI dataset. KITTI raw dataset contains ego motion files
+ but are not often used. Pose is important for algorithms that
+ take advantage of the temporal information.
+
+ Args:
+ frame (:obj:`Frame`): Open dataset frame proto.
+ file_idx (int): Current file index.
+ frame_idx (int): Current frame index.
+ """
+ pose = np.array(frame.pose.transform).reshape(4, 4)
+ np.savetxt(
+ join(f'{self.pose_save_dir}/{self.prefix}' +
+ f'{str(file_idx).zfill(3)}{str(frame_idx).zfill(3)}.txt'),
+ pose)
+
+ def save_timestamp(self, frame, file_idx, frame_idx):
+ """Save the timestamp data in a separate file instead of the
+ pointcloud.
+
+ Note that SDC's own pose is not included in the regular training
+ of KITTI dataset. KITTI raw dataset contains ego motion files
+ but are not often used. Pose is important for algorithms that
+ take advantage of the temporal information.
+
+ Args:
+ frame (:obj:`Frame`): Open dataset frame proto.
+ file_idx (int): Current file index.
+ frame_idx (int): Current frame index.
+ """
+ with open(
+ join(f'{self.timestamp_save_dir}/{self.prefix}' +
+ f'{str(file_idx).zfill(3)}{str(frame_idx).zfill(3)}.txt'),
+ 'w') as f:
+ f.write(str(frame.timestamp_micros))
+
+ def create_folder(self):
+ """Create folder for data preprocessing."""
+ if not self.test_mode:
+ dir_list1 = [
+ self.label_all_save_dir, self.calib_save_dir,
+ self.point_cloud_save_dir, self.pose_save_dir,
+ self.timestamp_save_dir
+ ]
+ dir_list2 = [self.label_save_dir, self.image_save_dir]
+ else:
+ dir_list1 = [
+ self.calib_save_dir, self.point_cloud_save_dir,
+ self.pose_save_dir, self.timestamp_save_dir
+ ]
+ dir_list2 = [self.image_save_dir]
+ for d in dir_list1:
+ mmcv.mkdir_or_exist(d)
+ for d in dir_list2:
+ for i in range(5):
+ mmcv.mkdir_or_exist(f'{d}{str(i)}')
+
+ def convert_range_image_to_point_cloud(self,
+ frame,
+ range_images,
+ camera_projections,
+ range_image_top_pose,
+ ri_index=0):
+ """Convert range images to point cloud.
+
+ Args:
+ frame (:obj:`Frame`): Open dataset frame.
+ range_images (dict): Mapping from laser_name to list of two
+ range images corresponding with two returns.
+ camera_projections (dict): Mapping from laser_name to list of two
+ camera projections corresponding with two returns.
+ range_image_top_pose (:obj:`Transform`): Range image pixel pose for
+ top lidar.
+ ri_index (int, optional): 0 for the first return,
+ 1 for the second return. Default: 0.
+
+ Returns:
+ tuple[list[np.ndarray]]: (List of points with shape [N, 3],
+ camera projections of points with shape [N, 6], intensity
+ with shape [N, 1], elongation with shape [N, 1], points'
+ position in the depth map (element offset if points come from
+ the main lidar otherwise -1) with shape[N, 1]). All the
+ lists have the length of lidar numbers (5).
+ """
+ calibrations = sorted(
+ frame.context.laser_calibrations, key=lambda c: c.name)
+ points = []
+ cp_points = []
+ intensity = []
+ elongation = []
+ mask_indices = []
+
+ frame_pose = tf.convert_to_tensor(
+ value=np.reshape(np.array(frame.pose.transform), [4, 4]))
+ # [H, W, 6]
+ range_image_top_pose_tensor = tf.reshape(
+ tf.convert_to_tensor(value=range_image_top_pose.data),
+ range_image_top_pose.shape.dims)
+ # [H, W, 3, 3]
+ range_image_top_pose_tensor_rotation = \
+ transform_utils.get_rotation_matrix(
+ range_image_top_pose_tensor[..., 0],
+ range_image_top_pose_tensor[..., 1],
+ range_image_top_pose_tensor[..., 2])
+ range_image_top_pose_tensor_translation = \
+ range_image_top_pose_tensor[..., 3:]
+ range_image_top_pose_tensor = transform_utils.get_transform(
+ range_image_top_pose_tensor_rotation,
+ range_image_top_pose_tensor_translation)
+ for c in calibrations:
+ range_image = range_images[c.name][ri_index]
+ if len(c.beam_inclinations) == 0:
+ beam_inclinations = range_image_utils.compute_inclination(
+ tf.constant(
+ [c.beam_inclination_min, c.beam_inclination_max]),
+ height=range_image.shape.dims[0])
+ else:
+ beam_inclinations = tf.constant(c.beam_inclinations)
+
+ beam_inclinations = tf.reverse(beam_inclinations, axis=[-1])
+ extrinsic = np.reshape(np.array(c.extrinsic.transform), [4, 4])
+
+ range_image_tensor = tf.reshape(
+ tf.convert_to_tensor(value=range_image.data),
+ range_image.shape.dims)
+ pixel_pose_local = None
+ frame_pose_local = None
+ if c.name == dataset_pb2.LaserName.TOP:
+ pixel_pose_local = range_image_top_pose_tensor
+ pixel_pose_local = tf.expand_dims(pixel_pose_local, axis=0)
+ frame_pose_local = tf.expand_dims(frame_pose, axis=0)
+ range_image_mask = range_image_tensor[..., 0] > 0
+
+ if self.filter_no_label_zone_points:
+ nlz_mask = range_image_tensor[..., 3] != 1.0 # 1.0: in NLZ
+ range_image_mask = range_image_mask & nlz_mask
+
+ range_image_cartesian = \
+ range_image_utils.extract_point_cloud_from_range_image(
+ tf.expand_dims(range_image_tensor[..., 0], axis=0),
+ tf.expand_dims(extrinsic, axis=0),
+ tf.expand_dims(tf.convert_to_tensor(
+ value=beam_inclinations), axis=0),
+ pixel_pose=pixel_pose_local,
+ frame_pose=frame_pose_local)
+
+ mask_index = tf.where(range_image_mask)
+
+ range_image_cartesian = tf.squeeze(range_image_cartesian, axis=0)
+ points_tensor = tf.gather_nd(range_image_cartesian, mask_index)
+
+ cp = camera_projections[c.name][ri_index]
+ cp_tensor = tf.reshape(
+ tf.convert_to_tensor(value=cp.data), cp.shape.dims)
+ cp_points_tensor = tf.gather_nd(cp_tensor, mask_index)
+ points.append(points_tensor.numpy())
+ cp_points.append(cp_points_tensor.numpy())
+
+ intensity_tensor = tf.gather_nd(range_image_tensor[..., 1],
+ mask_index)
+ intensity.append(intensity_tensor.numpy())
+
+ elongation_tensor = tf.gather_nd(range_image_tensor[..., 2],
+ mask_index)
+ elongation.append(elongation_tensor.numpy())
+ if c.name == 1:
+ mask_index = (ri_index * range_image_mask.shape[0] +
+ mask_index[:, 0]
+ ) * range_image_mask.shape[1] + mask_index[:, 1]
+ mask_index = mask_index.numpy().astype(elongation[-1].dtype)
+ else:
+ mask_index = np.full_like(elongation[-1], -1)
+
+ mask_indices.append(mask_index)
+
+ return points, cp_points, intensity, elongation, mask_indices
+
+ def cart_to_homo(self, mat):
+ """Convert transformation matrix in Cartesian coordinates to
+ homogeneous format.
+
+ Args:
+ mat (np.ndarray): Transformation matrix in Cartesian.
+ The input matrix shape is 3x3 or 3x4.
+
+ Returns:
+ np.ndarray: Transformation matrix in homogeneous format.
+ The matrix shape is 4x4.
+ """
+ ret = np.eye(4)
+ if mat.shape == (3, 3):
+ ret[:3, :3] = mat
+ elif mat.shape == (3, 4):
+ ret[:3, :] = mat
+ else:
+ raise ValueError(mat.shape)
+ return ret
diff --git a/tools/deployment/mmdet3d2torchserve.py b/tools/deployment/mmdet3d2torchserve.py
new file mode 100644
index 0000000..df7e608
--- /dev/null
+++ b/tools/deployment/mmdet3d2torchserve.py
@@ -0,0 +1,111 @@
+# Copyright (c) OpenMMLab. All rights reserved.
+from argparse import ArgumentParser, Namespace
+from pathlib import Path
+from tempfile import TemporaryDirectory
+
+import mmcv
+
+try:
+ from model_archiver.model_packaging import package_model
+ from model_archiver.model_packaging_utils import ModelExportUtils
+except ImportError:
+ package_model = None
+
+
+def mmdet3d2torchserve(
+ config_file: str,
+ checkpoint_file: str,
+ output_folder: str,
+ model_name: str,
+ model_version: str = '1.0',
+ force: bool = False,
+):
+ """Converts MMDetection3D model (config + checkpoint) to TorchServe `.mar`.
+
+ Args:
+ config_file (str):
+ In MMDetection3D config format.
+ The contents vary for each task repository.
+ checkpoint_file (str):
+ In MMDetection3D checkpoint format.
+ The contents vary for each task repository.
+ output_folder (str):
+ Folder where `{model_name}.mar` will be created.
+ The file created will be in TorchServe archive format.
+ model_name (str):
+ If not None, used for naming the `{model_name}.mar` file
+ that will be created under `output_folder`.
+ If None, `{Path(checkpoint_file).stem}` will be used.
+ model_version (str, optional):
+ Model's version. Default: '1.0'.
+ force (bool, optional):
+ If True, if there is an existing `{model_name}.mar`
+ file under `output_folder` it will be overwritten.
+ Default: False.
+ """
+ mmcv.mkdir_or_exist(output_folder)
+
+ config = mmcv.Config.fromfile(config_file)
+
+ with TemporaryDirectory() as tmpdir:
+ config.dump(f'{tmpdir}/config.py')
+
+ args = Namespace(
+ **{
+ 'model_file': f'{tmpdir}/config.py',
+ 'serialized_file': checkpoint_file,
+ 'handler': f'{Path(__file__).parent}/mmdet3d_handler.py',
+ 'model_name': model_name or Path(checkpoint_file).stem,
+ 'version': model_version,
+ 'export_path': output_folder,
+ 'force': force,
+ 'requirements_file': None,
+ 'extra_files': None,
+ 'runtime': 'python',
+ 'archive_format': 'default'
+ })
+ manifest = ModelExportUtils.generate_manifest_json(args)
+ package_model(args, manifest)
+
+
+def parse_args():
+ parser = ArgumentParser(
+ description='Convert MMDetection models to TorchServe `.mar` format.')
+ parser.add_argument('config', type=str, help='config file path')
+ parser.add_argument('checkpoint', type=str, help='checkpoint file path')
+ parser.add_argument(
+ '--output-folder',
+ type=str,
+ required=True,
+ help='Folder where `{model_name}.mar` will be created.')
+ parser.add_argument(
+ '--model-name',
+ type=str,
+ default=None,
+ help='If not None, used for naming the `{model_name}.mar`'
+ 'file that will be created under `output_folder`.'
+ 'If None, `{Path(checkpoint_file).stem}` will be used.')
+ parser.add_argument(
+ '--model-version',
+ type=str,
+ default='1.0',
+ help='Number used for versioning.')
+ parser.add_argument(
+ '-f',
+ '--force',
+ action='store_true',
+ help='overwrite the existing `{model_name}.mar`')
+ args = parser.parse_args()
+
+ return args
+
+
+if __name__ == '__main__':
+ args = parse_args()
+
+ if package_model is None:
+ raise ImportError('`torch-model-archiver` is required.'
+ 'Try: pip install torch-model-archiver')
+
+ mmdet3d2torchserve(args.config, args.checkpoint, args.output_folder,
+ args.model_name, args.model_version, args.force)
diff --git a/tools/deployment/mmdet3d_handler.py b/tools/deployment/mmdet3d_handler.py
new file mode 100644
index 0000000..8b526cd
--- /dev/null
+++ b/tools/deployment/mmdet3d_handler.py
@@ -0,0 +1,120 @@
+# Copyright (c) OpenMMLab. All rights reserved.
+import base64
+import os
+
+import numpy as np
+import torch
+from ts.torch_handler.base_handler import BaseHandler
+
+from mmdet3d.apis import inference_detector, init_model
+from mmdet3d.core.points import get_points_type
+
+
+class MMdet3dHandler(BaseHandler):
+ """MMDetection3D Handler used in TorchServe.
+
+ Handler to load models in MMDetection3D, and it will process data to get
+ predicted results. For now, it only supports SECOND.
+ """
+ threshold = 0.5
+ load_dim = 4
+ use_dim = [0, 1, 2, 3]
+ coord_type = 'LIDAR'
+ attribute_dims = None
+
+ def initialize(self, context):
+ """Initialize function loads the model in MMDetection3D.
+
+ Args:
+ context (context): It is a JSON Object containing information
+ pertaining to the model artifacts parameters.
+ """
+ properties = context.system_properties
+ self.map_location = 'cuda' if torch.cuda.is_available() else 'cpu'
+ self.device = torch.device(self.map_location + ':' +
+ str(properties.get('gpu_id')) if torch.cuda.
+ is_available() else self.map_location)
+ self.manifest = context.manifest
+
+ model_dir = properties.get('model_dir')
+ serialized_file = self.manifest['model']['serializedFile']
+ checkpoint = os.path.join(model_dir, serialized_file)
+ self.config_file = os.path.join(model_dir, 'config.py')
+ self.model = init_model(self.config_file, checkpoint, self.device)
+ self.initialized = True
+
+ def preprocess(self, data):
+ """Preprocess function converts data into LiDARPoints class.
+
+ Args:
+ data (List): Input data from the request.
+
+ Returns:
+ `LiDARPoints` : The preprocess function returns the input
+ point cloud data as LiDARPoints class.
+ """
+ for row in data:
+ # Compat layer: normally the envelope should just return the data
+ # directly, but older versions of Torchserve didn't have envelope.
+ pts = row.get('data') or row.get('body')
+ if isinstance(pts, str):
+ pts = base64.b64decode(pts)
+
+ points = np.frombuffer(pts, dtype=np.float32)
+ points = points.reshape(-1, self.load_dim)
+ points = points[:, self.use_dim]
+ points_class = get_points_type(self.coord_type)
+ points = points_class(
+ points,
+ points_dim=points.shape[-1],
+ attribute_dims=self.attribute_dims)
+
+ return points
+
+ def inference(self, data):
+ """Inference Function.
+
+ This function is used to make a prediction call on the
+ given input request.
+
+ Args:
+ data (`LiDARPoints`): LiDARPoints class passed to make
+ the inference request.
+
+ Returns:
+ List(dict) : The predicted result is returned in this function.
+ """
+ results, _ = inference_detector(self.model, data)
+ return results
+
+ def postprocess(self, data):
+ """Postprocess function.
+
+ This function makes use of the output from the inference and
+ converts it into a torchserve supported response output.
+
+ Args:
+ data (List[dict]): The data received from the prediction
+ output of the model.
+
+ Returns:
+ List: The post process function returns a list of the predicted
+ output.
+ """
+ output = []
+ for pts_index, result in enumerate(data):
+ output.append([])
+ if 'pts_bbox' in result.keys():
+ pred_bboxes = result['pts_bbox']['boxes_3d'].tensor.numpy()
+ pred_scores = result['pts_bbox']['scores_3d'].numpy()
+ else:
+ pred_bboxes = result['boxes_3d'].tensor.numpy()
+ pred_scores = result['scores_3d'].numpy()
+
+ index = pred_scores > self.threshold
+ bbox_coords = pred_bboxes[index].tolist()
+ score = pred_scores[index].tolist()
+
+ output[pts_index].append({'3dbbox': bbox_coords, 'score': score})
+
+ return output
diff --git a/tools/deployment/test_torchserver.py b/tools/deployment/test_torchserver.py
new file mode 100644
index 0000000..613f9e4
--- /dev/null
+++ b/tools/deployment/test_torchserver.py
@@ -0,0 +1,56 @@
+from argparse import ArgumentParser
+
+import numpy as np
+import requests
+
+from mmdet3d.apis import inference_detector, init_model
+
+
+def parse_args():
+ parser = ArgumentParser()
+ parser.add_argument('pcd', help='Point cloud file')
+ parser.add_argument('config', help='Config file')
+ parser.add_argument('checkpoint', help='Checkpoint file')
+ parser.add_argument('model_name', help='The model name in the server')
+ parser.add_argument(
+ '--inference-addr',
+ default='127.0.0.1:8080',
+ help='Address and port of the inference server')
+ parser.add_argument(
+ '--device', default='cuda:0', help='Device used for inference')
+ parser.add_argument(
+ '--score-thr', type=float, default=0.5, help='3d bbox score threshold')
+ args = parser.parse_args()
+ return args
+
+
+def parse_result(input):
+ bbox = input[0]['3dbbox']
+ result = np.array(bbox)
+ return result
+
+
+def main(args):
+ # build the model from a config file and a checkpoint file
+ model = init_model(args.config, args.checkpoint, device=args.device)
+ # test a single point cloud file
+ model_result, _ = inference_detector(model, args.pcd)
+ # filter the 3d bboxes whose scores > 0.5
+ if 'pts_bbox' in model_result[0].keys():
+ pred_bboxes = model_result[0]['pts_bbox']['boxes_3d'].tensor.numpy()
+ pred_scores = model_result[0]['pts_bbox']['scores_3d'].numpy()
+ else:
+ pred_bboxes = model_result[0]['boxes_3d'].tensor.numpy()
+ pred_scores = model_result[0]['scores_3d'].numpy()
+ model_result = pred_bboxes[pred_scores > 0.5]
+
+ url = 'http://' + args.inference_addr + '/predictions/' + args.model_name
+ with open(args.pcd, 'rb') as points:
+ response = requests.post(url, points)
+ server_result = parse_result(response.json())
+ assert np.allclose(model_result, server_result)
+
+
+if __name__ == '__main__':
+ args = parse_args()
+ main(args)
diff --git a/tools/dist_test.sh b/tools/dist_test.sh
new file mode 100755
index 0000000..dea131b
--- /dev/null
+++ b/tools/dist_test.sh
@@ -0,0 +1,22 @@
+#!/usr/bin/env bash
+
+CONFIG=$1
+CHECKPOINT=$2
+GPUS=$3
+NNODES=${NNODES:-1}
+NODE_RANK=${NODE_RANK:-0}
+PORT=${PORT:-29500}
+MASTER_ADDR=${MASTER_ADDR:-"127.0.0.1"}
+
+PYTHONPATH="$(dirname $0)/..":$PYTHONPATH \
+python -m torch.distributed.launch \
+ --nnodes=$NNODES \
+ --node_rank=$NODE_RANK \
+ --master_addr=$MASTER_ADDR \
+ --nproc_per_node=$GPUS \
+ --master_port=$PORT \
+ $(dirname "$0")/test.py \
+ $CONFIG \
+ $CHECKPOINT \
+ --launcher pytorch \
+ ${@:4}
diff --git a/tools/dist_train.sh b/tools/dist_train.sh
new file mode 100755
index 0000000..aa71bf4
--- /dev/null
+++ b/tools/dist_train.sh
@@ -0,0 +1,20 @@
+#!/usr/bin/env bash
+
+CONFIG=$1
+GPUS=$2
+NNODES=${NNODES:-1}
+NODE_RANK=${NODE_RANK:-0}
+PORT=${PORT:-29500}
+MASTER_ADDR=${MASTER_ADDR:-"127.0.0.1"}
+
+PYTHONPATH="$(dirname $0)/..":$PYTHONPATH \
+python -m torch.distributed.launch \
+ --nnodes=$NNODES \
+ --node_rank=$NODE_RANK \
+ --master_addr=$MASTER_ADDR \
+ --nproc_per_node=$GPUS \
+ --master_port=$PORT \
+ $(dirname "$0")/train.py \
+ $CONFIG \
+ --seed 0 \
+ --launcher pytorch ${@:3}
diff --git a/tools/misc/browse_dataset.py b/tools/misc/browse_dataset.py
new file mode 100644
index 0000000..e4451b1
--- /dev/null
+++ b/tools/misc/browse_dataset.py
@@ -0,0 +1,232 @@
+# Copyright (c) OpenMMLab. All rights reserved.
+import argparse
+import warnings
+from os import path as osp
+from pathlib import Path
+
+import mmcv
+import numpy as np
+from mmcv import Config, DictAction, mkdir_or_exist
+
+from mmdet3d.core.bbox import (Box3DMode, CameraInstance3DBoxes, Coord3DMode,
+ DepthInstance3DBoxes, LiDARInstance3DBoxes)
+from mmdet3d.core.visualizer import (show_multi_modality_result, show_result,
+ show_seg_result)
+from mmdet3d.datasets import build_dataset
+
+
+def parse_args():
+ parser = argparse.ArgumentParser(description='Browse a dataset')
+ parser.add_argument('config', help='train config file path')
+ parser.add_argument(
+ '--skip-type',
+ type=str,
+ nargs='+',
+ default=['Normalize'],
+ help='skip some useless pipeline')
+ parser.add_argument(
+ '--output-dir',
+ default=None,
+ type=str,
+ help='If there is no display interface, you can save it')
+ parser.add_argument(
+ '--task',
+ type=str,
+ choices=['det', 'seg', 'multi_modality-det', 'mono-det'],
+ help='Determine the visualization method depending on the task.')
+ parser.add_argument(
+ '--aug',
+ action='store_true',
+ help='Whether to visualize augmented datasets or original dataset.')
+ parser.add_argument(
+ '--online',
+ action='store_true',
+ help='Whether to perform online visualization. Note that you often '
+ 'need a monitor to do so.')
+ parser.add_argument(
+ '--cfg-options',
+ nargs='+',
+ action=DictAction,
+ help='override some settings in the used config, the key-value pair '
+ 'in xxx=yyy format will be merged into config file. If the value to '
+ 'be overwritten is a list, it should be like key="[a,b]" or key=a,b '
+ 'It also allows nested list/tuple values, e.g. key="[(a,b),(c,d)]" '
+ 'Note that the quotation marks are necessary and that no white space '
+ 'is allowed.')
+ args = parser.parse_args()
+ return args
+
+
+def build_data_cfg(config_path, skip_type, aug, cfg_options):
+ """Build data config for loading visualization data."""
+
+ cfg = Config.fromfile(config_path)
+ if cfg_options is not None:
+ cfg.merge_from_dict(cfg_options)
+ # extract inner dataset of `RepeatDataset` as `cfg.data.train`
+ # so we don't need to worry about it later
+ if cfg.data.train['type'] == 'RepeatDataset':
+ cfg.data.train = cfg.data.train.dataset
+ # use only first dataset for `ConcatDataset`
+ if cfg.data.train['type'] == 'ConcatDataset':
+ cfg.data.train = cfg.data.train.datasets[0]
+ train_data_cfg = cfg.data.train
+
+ if aug:
+ show_pipeline = cfg.train_pipeline
+ else:
+ show_pipeline = cfg.eval_pipeline
+ for i in range(len(cfg.train_pipeline)):
+ if cfg.train_pipeline[i]['type'] == 'LoadAnnotations3D':
+ show_pipeline.insert(i, cfg.train_pipeline[i])
+ # Collect points as well as labels
+ if cfg.train_pipeline[i]['type'] == 'Collect3D':
+ if show_pipeline[-1]['type'] == 'Collect3D':
+ show_pipeline[-1] = cfg.train_pipeline[i]
+ else:
+ show_pipeline.append(cfg.train_pipeline[i])
+
+ train_data_cfg['pipeline'] = [
+ x for x in show_pipeline if x['type'] not in skip_type
+ ]
+
+ return cfg
+
+
+def to_depth_mode(points, bboxes):
+ """Convert points and bboxes to Depth Coord and Depth Box mode."""
+ if points is not None:
+ points = Coord3DMode.convert_point(points.copy(), Coord3DMode.LIDAR,
+ Coord3DMode.DEPTH)
+ if bboxes is not None:
+ bboxes = Box3DMode.convert(bboxes.clone(), Box3DMode.LIDAR,
+ Box3DMode.DEPTH)
+ return points, bboxes
+
+
+def show_det_data(input, out_dir, show=False):
+ """Visualize 3D point cloud and 3D bboxes."""
+ img_metas = input['img_metas']._data
+ points = input['points']._data.numpy()
+ gt_bboxes = input['gt_bboxes_3d']._data.tensor
+ if img_metas['box_mode_3d'] != Box3DMode.DEPTH:
+ points, gt_bboxes = to_depth_mode(points, gt_bboxes)
+ filename = osp.splitext(osp.basename(img_metas['pts_filename']))[0]
+ show_result(
+ points,
+ gt_bboxes.clone(),
+ None,
+ out_dir,
+ filename,
+ show=show,
+ snapshot=True)
+
+
+def show_seg_data(input, out_dir, show=False):
+ """Visualize 3D point cloud and segmentation mask."""
+ img_metas = input['img_metas']._data
+ points = input['points']._data.numpy()
+ gt_seg = input['pts_semantic_mask']._data.numpy()
+ filename = osp.splitext(osp.basename(img_metas['pts_filename']))[0]
+ show_seg_result(
+ points,
+ gt_seg.copy(),
+ None,
+ out_dir,
+ filename,
+ np.array(img_metas['PALETTE']),
+ img_metas['ignore_index'],
+ show=show,
+ snapshot=True)
+
+
+def show_proj_bbox_img(input, out_dir, show=False, is_nus_mono=False):
+ """Visualize 3D bboxes on 2D image by projection."""
+ gt_bboxes = input['gt_bboxes_3d']._data
+ img_metas = input['img_metas']._data
+ img = input['img']._data.numpy()
+ # need to transpose channel to first dim
+ img = img.transpose(1, 2, 0)
+ # no 3D gt bboxes, just show img
+ if gt_bboxes.tensor.shape[0] == 0:
+ gt_bboxes = None
+ filename = Path(img_metas['filename']).name
+ if isinstance(gt_bboxes, DepthInstance3DBoxes):
+ show_multi_modality_result(
+ img,
+ gt_bboxes,
+ None,
+ None,
+ out_dir,
+ filename,
+ box_mode='depth',
+ img_metas=img_metas,
+ show=show)
+ elif isinstance(gt_bboxes, LiDARInstance3DBoxes):
+ show_multi_modality_result(
+ img,
+ gt_bboxes,
+ None,
+ img_metas['lidar2img'],
+ out_dir,
+ filename,
+ box_mode='lidar',
+ img_metas=img_metas,
+ show=show)
+ elif isinstance(gt_bboxes, CameraInstance3DBoxes):
+ show_multi_modality_result(
+ img,
+ gt_bboxes,
+ None,
+ img_metas['cam2img'],
+ out_dir,
+ filename,
+ box_mode='camera',
+ img_metas=img_metas,
+ show=show)
+ else:
+ # can't project, just show img
+ warnings.warn(
+ f'unrecognized gt box type {type(gt_bboxes)}, only show image')
+ show_multi_modality_result(
+ img, None, None, None, out_dir, filename, show=show)
+
+
+def main():
+ args = parse_args()
+
+ if args.output_dir is not None:
+ mkdir_or_exist(args.output_dir)
+
+ cfg = build_data_cfg(args.config, args.skip_type, args.aug,
+ args.cfg_options)
+ try:
+ dataset = build_dataset(
+ cfg.data.train, default_args=dict(filter_empty_gt=False))
+ except TypeError: # seg dataset doesn't have `filter_empty_gt` key
+ dataset = build_dataset(cfg.data.train)
+
+ dataset_type = cfg.dataset_type
+ # configure visualization mode
+ vis_task = args.task # 'det', 'seg', 'multi_modality-det', 'mono-det'
+ progress_bar = mmcv.ProgressBar(len(dataset))
+
+ for input in dataset:
+ if vis_task in ['det', 'multi_modality-det']:
+ # show 3D bboxes on 3D point clouds
+ show_det_data(input, args.output_dir, show=args.online)
+ if vis_task in ['multi_modality-det', 'mono-det']:
+ # project 3D bboxes to 2D image
+ show_proj_bbox_img(
+ input,
+ args.output_dir,
+ show=args.online,
+ is_nus_mono=(dataset_type == 'NuScenesMonoDataset'))
+ elif vis_task in ['seg']:
+ # show 3D segmentation mask on 3D point clouds
+ show_seg_data(input, args.output_dir, show=args.online)
+ progress_bar.update()
+
+
+if __name__ == '__main__':
+ main()
diff --git a/tools/misc/fuse_conv_bn.py b/tools/misc/fuse_conv_bn.py
new file mode 100644
index 0000000..9aff402
--- /dev/null
+++ b/tools/misc/fuse_conv_bn.py
@@ -0,0 +1,68 @@
+# Copyright (c) OpenMMLab. All rights reserved.
+import argparse
+
+import torch
+from mmcv.runner import save_checkpoint
+from torch import nn as nn
+
+from mmdet3d.apis import init_model
+
+
+def fuse_conv_bn(conv, bn):
+ """During inference, the functionary of batch norm layers is turned off but
+ only the mean and var alone channels are used, which exposes the chance to
+ fuse it with the preceding conv layers to save computations and simplify
+ network structures."""
+ conv_w = conv.weight
+ conv_b = conv.bias if conv.bias is not None else torch.zeros_like(
+ bn.running_mean)
+
+ factor = bn.weight / torch.sqrt(bn.running_var + bn.eps)
+ conv.weight = nn.Parameter(conv_w *
+ factor.reshape([conv.out_channels, 1, 1, 1]))
+ conv.bias = nn.Parameter((conv_b - bn.running_mean) * factor + bn.bias)
+ return conv
+
+
+def fuse_module(m):
+ last_conv = None
+ last_conv_name = None
+
+ for name, child in m.named_children():
+ if isinstance(child, (nn.BatchNorm2d, nn.SyncBatchNorm)):
+ if last_conv is None: # only fuse BN that is after Conv
+ continue
+ fused_conv = fuse_conv_bn(last_conv, child)
+ m._modules[last_conv_name] = fused_conv
+ # To reduce changes, set BN as Identity instead of deleting it.
+ m._modules[name] = nn.Identity()
+ last_conv = None
+ elif isinstance(child, nn.Conv2d):
+ last_conv = child
+ last_conv_name = name
+ else:
+ fuse_module(child)
+ return m
+
+
+def parse_args():
+ parser = argparse.ArgumentParser(
+ description='fuse Conv and BN layers in a model')
+ parser.add_argument('config', help='config file path')
+ parser.add_argument('checkpoint', help='checkpoint file path')
+ parser.add_argument('out', help='output path of the converted model')
+ args = parser.parse_args()
+ return args
+
+
+def main():
+ args = parse_args()
+ # build the model from a config file and a checkpoint file
+ model = init_model(args.config, args.checkpoint)
+ # fuse conv and bn layers of the model
+ fused_model = fuse_module(model)
+ save_checkpoint(fused_model, args.out)
+
+
+if __name__ == '__main__':
+ main()
diff --git a/tools/misc/print_config.py b/tools/misc/print_config.py
new file mode 100644
index 0000000..c3538ef
--- /dev/null
+++ b/tools/misc/print_config.py
@@ -0,0 +1,27 @@
+# Copyright (c) OpenMMLab. All rights reserved.
+import argparse
+
+from mmcv import Config, DictAction
+
+
+def parse_args():
+ parser = argparse.ArgumentParser(description='Print the whole config')
+ parser.add_argument('config', help='config file path')
+ parser.add_argument(
+ '--options', nargs='+', action=DictAction, help='arguments in dict')
+ args = parser.parse_args()
+
+ return args
+
+
+def main():
+ args = parse_args()
+
+ cfg = Config.fromfile(args.config)
+ if args.options is not None:
+ cfg.merge_from_dict(args.options)
+ print(f'Config:\n{cfg.pretty_text}')
+
+
+if __name__ == '__main__':
+ main()
diff --git a/tools/misc/visualize_results.py b/tools/misc/visualize_results.py
new file mode 100644
index 0000000..c59445f
--- /dev/null
+++ b/tools/misc/visualize_results.py
@@ -0,0 +1,50 @@
+# Copyright (c) OpenMMLab. All rights reserved.
+import argparse
+
+import mmcv
+from mmcv import Config
+
+from mmdet3d.datasets import build_dataset
+
+
+def parse_args():
+ parser = argparse.ArgumentParser(
+ description='MMDet3D visualize the results')
+ parser.add_argument('config', help='test config file path')
+ parser.add_argument('--result', help='results file in pickle format')
+ parser.add_argument(
+ '--show-dir', help='directory where visualize results will be saved')
+ args = parser.parse_args()
+
+ return args
+
+
+def main():
+ args = parse_args()
+
+ if args.result is not None and \
+ not args.result.endswith(('.pkl', '.pickle')):
+ raise ValueError('The results file must be a pkl file.')
+
+ cfg = Config.fromfile(args.config)
+ cfg.data.test.test_mode = True
+
+ # build the dataset
+ dataset = build_dataset(cfg.data.test)
+ results = mmcv.load(args.result)
+
+ if getattr(dataset, 'show', None) is not None:
+ # data loading pipeline for showing
+ eval_pipeline = cfg.get('eval_pipeline', {})
+ if eval_pipeline:
+ dataset.show(results, args.show_dir, pipeline=eval_pipeline)
+ else:
+ dataset.show(results, args.show_dir) # use default pipeline
+ else:
+ raise NotImplementedError(
+ 'Show is not implemented for dataset {}!'.format(
+ type(dataset).__name__))
+
+
+if __name__ == '__main__':
+ main()
diff --git a/tools/model_converters/convert_h3dnet_checkpoints.py b/tools/model_converters/convert_h3dnet_checkpoints.py
new file mode 100644
index 0000000..2ede340
--- /dev/null
+++ b/tools/model_converters/convert_h3dnet_checkpoints.py
@@ -0,0 +1,177 @@
+# Copyright (c) OpenMMLab. All rights reserved.
+import argparse
+import tempfile
+
+import torch
+from mmcv import Config
+from mmcv.runner import load_state_dict
+
+from mmdet3d.models import build_detector
+
+
+def parse_args():
+ parser = argparse.ArgumentParser(
+ description='MMDet3D upgrade model version(before v0.6.0) of H3DNet')
+ parser.add_argument('checkpoint', help='checkpoint file')
+ parser.add_argument('--out', help='path of the output checkpoint file')
+ args = parser.parse_args()
+ return args
+
+
+def parse_config(config_strings):
+ """Parse config from strings.
+
+ Args:
+ config_strings (string): strings of model config.
+
+ Returns:
+ Config: model config
+ """
+ temp_file = tempfile.NamedTemporaryFile()
+ config_path = f'{temp_file.name}.py'
+ with open(config_path, 'w') as f:
+ f.write(config_strings)
+
+ config = Config.fromfile(config_path)
+
+ # Update backbone config
+ if 'pool_mod' in config.model.backbone.backbones:
+ config.model.backbone.backbones.pop('pool_mod')
+
+ if 'sa_cfg' not in config.model.backbone:
+ config.model.backbone['sa_cfg'] = dict(
+ type='PointSAModule',
+ pool_mod='max',
+ use_xyz=True,
+ normalize_xyz=True)
+
+ if 'type' not in config.model.rpn_head.vote_aggregation_cfg:
+ config.model.rpn_head.vote_aggregation_cfg['type'] = 'PointSAModule'
+
+ # Update rpn_head config
+ if 'pred_layer_cfg' not in config.model.rpn_head:
+ config.model.rpn_head['pred_layer_cfg'] = dict(
+ in_channels=128, shared_conv_channels=(128, 128), bias=True)
+
+ if 'feat_channels' in config.model.rpn_head:
+ config.model.rpn_head.pop('feat_channels')
+
+ if 'vote_moudule_cfg' in config.model.rpn_head:
+ config.model.rpn_head['vote_module_cfg'] = config.model.rpn_head.pop(
+ 'vote_moudule_cfg')
+
+ if config.model.rpn_head.vote_aggregation_cfg.use_xyz:
+ config.model.rpn_head.vote_aggregation_cfg.mlp_channels[0] -= 3
+
+ for cfg in config.model.roi_head.primitive_list:
+ cfg['vote_module_cfg'] = cfg.pop('vote_moudule_cfg')
+ cfg.vote_aggregation_cfg.mlp_channels[0] -= 3
+ if 'type' not in cfg.vote_aggregation_cfg:
+ cfg.vote_aggregation_cfg['type'] = 'PointSAModule'
+
+ if 'type' not in config.model.roi_head.bbox_head.suface_matching_cfg:
+ config.model.roi_head.bbox_head.suface_matching_cfg[
+ 'type'] = 'PointSAModule'
+
+ if config.model.roi_head.bbox_head.suface_matching_cfg.use_xyz:
+ config.model.roi_head.bbox_head.suface_matching_cfg.mlp_channels[
+ 0] -= 3
+
+ if 'type' not in config.model.roi_head.bbox_head.line_matching_cfg:
+ config.model.roi_head.bbox_head.line_matching_cfg[
+ 'type'] = 'PointSAModule'
+
+ if config.model.roi_head.bbox_head.line_matching_cfg.use_xyz:
+ config.model.roi_head.bbox_head.line_matching_cfg.mlp_channels[0] -= 3
+
+ if 'proposal_module_cfg' in config.model.roi_head.bbox_head:
+ config.model.roi_head.bbox_head.pop('proposal_module_cfg')
+
+ temp_file.close()
+
+ return config
+
+
+def main():
+ """Convert keys in checkpoints for VoteNet.
+
+ There can be some breaking changes during the development of mmdetection3d,
+ and this tool is used for upgrading checkpoints trained with old versions
+ (before v0.6.0) to the latest one.
+ """
+ args = parse_args()
+ checkpoint = torch.load(args.checkpoint)
+ cfg = parse_config(checkpoint['meta']['config'])
+ # Build the model and load checkpoint
+ model = build_detector(
+ cfg.model,
+ train_cfg=cfg.get('train_cfg'),
+ test_cfg=cfg.get('test_cfg'))
+ orig_ckpt = checkpoint['state_dict']
+ converted_ckpt = orig_ckpt.copy()
+
+ if cfg['dataset_type'] == 'ScanNetDataset':
+ NUM_CLASSES = 18
+ elif cfg['dataset_type'] == 'SUNRGBDDataset':
+ NUM_CLASSES = 10
+ else:
+ raise NotImplementedError
+
+ RENAME_PREFIX = {
+ 'rpn_head.conv_pred.0': 'rpn_head.conv_pred.shared_convs.layer0',
+ 'rpn_head.conv_pred.1': 'rpn_head.conv_pred.shared_convs.layer1'
+ }
+
+ DEL_KEYS = [
+ 'rpn_head.conv_pred.0.bn.num_batches_tracked',
+ 'rpn_head.conv_pred.1.bn.num_batches_tracked'
+ ]
+
+ EXTRACT_KEYS = {
+ 'rpn_head.conv_pred.conv_cls.weight':
+ ('rpn_head.conv_pred.conv_out.weight', [(0, 2), (-NUM_CLASSES, -1)]),
+ 'rpn_head.conv_pred.conv_cls.bias':
+ ('rpn_head.conv_pred.conv_out.bias', [(0, 2), (-NUM_CLASSES, -1)]),
+ 'rpn_head.conv_pred.conv_reg.weight':
+ ('rpn_head.conv_pred.conv_out.weight', [(2, -NUM_CLASSES)]),
+ 'rpn_head.conv_pred.conv_reg.bias':
+ ('rpn_head.conv_pred.conv_out.bias', [(2, -NUM_CLASSES)])
+ }
+
+ # Delete some useless keys
+ for key in DEL_KEYS:
+ converted_ckpt.pop(key)
+
+ # Rename keys with specific prefix
+ RENAME_KEYS = dict()
+ for old_key in converted_ckpt.keys():
+ for rename_prefix in RENAME_PREFIX.keys():
+ if rename_prefix in old_key:
+ new_key = old_key.replace(rename_prefix,
+ RENAME_PREFIX[rename_prefix])
+ RENAME_KEYS[new_key] = old_key
+ for new_key, old_key in RENAME_KEYS.items():
+ converted_ckpt[new_key] = converted_ckpt.pop(old_key)
+
+ # Extract weights and rename the keys
+ for new_key, (old_key, indices) in EXTRACT_KEYS.items():
+ cur_layers = orig_ckpt[old_key]
+ converted_layers = []
+ for (start, end) in indices:
+ if end != -1:
+ converted_layers.append(cur_layers[start:end])
+ else:
+ converted_layers.append(cur_layers[start:])
+ converted_layers = torch.cat(converted_layers, 0)
+ converted_ckpt[new_key] = converted_layers
+ if old_key in converted_ckpt.keys():
+ converted_ckpt.pop(old_key)
+
+ # Check the converted checkpoint by loading to the model
+ load_state_dict(model, converted_ckpt, strict=True)
+ checkpoint['state_dict'] = converted_ckpt
+ torch.save(checkpoint, args.out)
+
+
+if __name__ == '__main__':
+ main()
diff --git a/tools/model_converters/convert_votenet_checkpoints.py b/tools/model_converters/convert_votenet_checkpoints.py
new file mode 100644
index 0000000..7264e31
--- /dev/null
+++ b/tools/model_converters/convert_votenet_checkpoints.py
@@ -0,0 +1,153 @@
+# Copyright (c) OpenMMLab. All rights reserved.
+import argparse
+import tempfile
+
+import torch
+from mmcv import Config
+from mmcv.runner import load_state_dict
+
+from mmdet3d.models import build_detector
+
+
+def parse_args():
+ parser = argparse.ArgumentParser(
+ description='MMDet3D upgrade model version(before v0.6.0) of VoteNet')
+ parser.add_argument('checkpoint', help='checkpoint file')
+ parser.add_argument('--out', help='path of the output checkpoint file')
+ args = parser.parse_args()
+ return args
+
+
+def parse_config(config_strings):
+ """Parse config from strings.
+
+ Args:
+ config_strings (string): strings of model config.
+
+ Returns:
+ Config: model config
+ """
+ temp_file = tempfile.NamedTemporaryFile()
+ config_path = f'{temp_file.name}.py'
+ with open(config_path, 'w') as f:
+ f.write(config_strings)
+
+ config = Config.fromfile(config_path)
+
+ # Update backbone config
+ if 'pool_mod' in config.model.backbone:
+ config.model.backbone.pop('pool_mod')
+
+ if 'sa_cfg' not in config.model.backbone:
+ config.model.backbone['sa_cfg'] = dict(
+ type='PointSAModule',
+ pool_mod='max',
+ use_xyz=True,
+ normalize_xyz=True)
+
+ if 'type' not in config.model.bbox_head.vote_aggregation_cfg:
+ config.model.bbox_head.vote_aggregation_cfg['type'] = 'PointSAModule'
+
+ # Update bbox_head config
+ if 'pred_layer_cfg' not in config.model.bbox_head:
+ config.model.bbox_head['pred_layer_cfg'] = dict(
+ in_channels=128, shared_conv_channels=(128, 128), bias=True)
+
+ if 'feat_channels' in config.model.bbox_head:
+ config.model.bbox_head.pop('feat_channels')
+
+ if 'vote_moudule_cfg' in config.model.bbox_head:
+ config.model.bbox_head['vote_module_cfg'] = config.model.bbox_head.pop(
+ 'vote_moudule_cfg')
+
+ if config.model.bbox_head.vote_aggregation_cfg.use_xyz:
+ config.model.bbox_head.vote_aggregation_cfg.mlp_channels[0] -= 3
+
+ temp_file.close()
+
+ return config
+
+
+def main():
+ """Convert keys in checkpoints for VoteNet.
+
+ There can be some breaking changes during the development of mmdetection3d,
+ and this tool is used for upgrading checkpoints trained with old versions
+ (before v0.6.0) to the latest one.
+ """
+ args = parse_args()
+ checkpoint = torch.load(args.checkpoint)
+ cfg = parse_config(checkpoint['meta']['config'])
+ # Build the model and load checkpoint
+ model = build_detector(
+ cfg.model,
+ train_cfg=cfg.get('train_cfg'),
+ test_cfg=cfg.get('test_cfg'))
+ orig_ckpt = checkpoint['state_dict']
+ converted_ckpt = orig_ckpt.copy()
+
+ if cfg['dataset_type'] == 'ScanNetDataset':
+ NUM_CLASSES = 18
+ elif cfg['dataset_type'] == 'SUNRGBDDataset':
+ NUM_CLASSES = 10
+ else:
+ raise NotImplementedError
+
+ RENAME_PREFIX = {
+ 'bbox_head.conv_pred.0': 'bbox_head.conv_pred.shared_convs.layer0',
+ 'bbox_head.conv_pred.1': 'bbox_head.conv_pred.shared_convs.layer1'
+ }
+
+ DEL_KEYS = [
+ 'bbox_head.conv_pred.0.bn.num_batches_tracked',
+ 'bbox_head.conv_pred.1.bn.num_batches_tracked'
+ ]
+
+ EXTRACT_KEYS = {
+ 'bbox_head.conv_pred.conv_cls.weight':
+ ('bbox_head.conv_pred.conv_out.weight', [(0, 2), (-NUM_CLASSES, -1)]),
+ 'bbox_head.conv_pred.conv_cls.bias':
+ ('bbox_head.conv_pred.conv_out.bias', [(0, 2), (-NUM_CLASSES, -1)]),
+ 'bbox_head.conv_pred.conv_reg.weight':
+ ('bbox_head.conv_pred.conv_out.weight', [(2, -NUM_CLASSES)]),
+ 'bbox_head.conv_pred.conv_reg.bias':
+ ('bbox_head.conv_pred.conv_out.bias', [(2, -NUM_CLASSES)])
+ }
+
+ # Delete some useless keys
+ for key in DEL_KEYS:
+ converted_ckpt.pop(key)
+
+ # Rename keys with specific prefix
+ RENAME_KEYS = dict()
+ for old_key in converted_ckpt.keys():
+ for rename_prefix in RENAME_PREFIX.keys():
+ if rename_prefix in old_key:
+ new_key = old_key.replace(rename_prefix,
+ RENAME_PREFIX[rename_prefix])
+ RENAME_KEYS[new_key] = old_key
+ for new_key, old_key in RENAME_KEYS.items():
+ converted_ckpt[new_key] = converted_ckpt.pop(old_key)
+
+ # Extract weights and rename the keys
+ for new_key, (old_key, indices) in EXTRACT_KEYS.items():
+ cur_layers = orig_ckpt[old_key]
+ converted_layers = []
+ for (start, end) in indices:
+ if end != -1:
+ converted_layers.append(cur_layers[start:end])
+ else:
+ converted_layers.append(cur_layers[start:])
+ converted_layers = torch.cat(converted_layers, 0)
+ converted_ckpt[new_key] = converted_layers
+ if old_key in converted_ckpt.keys():
+ converted_ckpt.pop(old_key)
+
+ # Check the converted checkpoint by loading to the model
+ load_state_dict(model, converted_ckpt, strict=True)
+ checkpoint['state_dict'] = converted_ckpt
+ torch.save(checkpoint, args.out)
+
+
+if __name__ == '__main__':
+ main()
diff --git a/tools/model_converters/publish_model.py b/tools/model_converters/publish_model.py
new file mode 100644
index 0000000..e266057
--- /dev/null
+++ b/tools/model_converters/publish_model.py
@@ -0,0 +1,36 @@
+# Copyright (c) OpenMMLab. All rights reserved.
+import argparse
+import subprocess
+
+import torch
+
+
+def parse_args():
+ parser = argparse.ArgumentParser(
+ description='Process a checkpoint to be published')
+ parser.add_argument('in_file', help='input checkpoint filename')
+ parser.add_argument('out_file', help='output checkpoint filename')
+ args = parser.parse_args()
+ return args
+
+
+def process_checkpoint(in_file, out_file):
+ checkpoint = torch.load(in_file, map_location='cpu')
+ # remove optimizer for smaller file size
+ if 'optimizer' in checkpoint:
+ del checkpoint['optimizer']
+ # if it is necessary to remove some sensitive data in checkpoint['meta'],
+ # add the code here.
+ torch.save(checkpoint, out_file)
+ sha = subprocess.check_output(['sha256sum', out_file]).decode()
+ final_file = out_file.rstrip('.pth') + '-{}.pth'.format(sha[:8])
+ subprocess.Popen(['mv', out_file, final_file])
+
+
+def main():
+ args = parse_args()
+ process_checkpoint(args.in_file, args.out_file)
+
+
+if __name__ == '__main__':
+ main()
diff --git a/tools/model_converters/regnet2mmdet.py b/tools/model_converters/regnet2mmdet.py
new file mode 100644
index 0000000..fbf8c8f
--- /dev/null
+++ b/tools/model_converters/regnet2mmdet.py
@@ -0,0 +1,90 @@
+# Copyright (c) OpenMMLab. All rights reserved.
+import argparse
+from collections import OrderedDict
+
+import torch
+
+
+def convert_stem(model_key, model_weight, state_dict, converted_names):
+ new_key = model_key.replace('stem.conv', 'conv1')
+ new_key = new_key.replace('stem.bn', 'bn1')
+ state_dict[new_key] = model_weight
+ converted_names.add(model_key)
+ print(f'Convert {model_key} to {new_key}')
+
+
+def convert_head(model_key, model_weight, state_dict, converted_names):
+ new_key = model_key.replace('head.fc', 'fc')
+ state_dict[new_key] = model_weight
+ converted_names.add(model_key)
+ print(f'Convert {model_key} to {new_key}')
+
+
+def convert_reslayer(model_key, model_weight, state_dict, converted_names):
+ split_keys = model_key.split('.')
+ layer, block, module = split_keys[:3]
+ block_id = int(block[1:])
+ layer_name = f'layer{int(layer[1:])}'
+ block_name = f'{block_id - 1}'
+
+ if block_id == 1 and module == 'bn':
+ new_key = f'{layer_name}.{block_name}.downsample.1.{split_keys[-1]}'
+ elif block_id == 1 and module == 'proj':
+ new_key = f'{layer_name}.{block_name}.downsample.0.{split_keys[-1]}'
+ elif module == 'f':
+ if split_keys[3] == 'a_bn':
+ module_name = 'bn1'
+ elif split_keys[3] == 'b_bn':
+ module_name = 'bn2'
+ elif split_keys[3] == 'c_bn':
+ module_name = 'bn3'
+ elif split_keys[3] == 'a':
+ module_name = 'conv1'
+ elif split_keys[3] == 'b':
+ module_name = 'conv2'
+ elif split_keys[3] == 'c':
+ module_name = 'conv3'
+ new_key = f'{layer_name}.{block_name}.{module_name}.{split_keys[-1]}'
+ else:
+ raise ValueError(f'Unsupported conversion of key {model_key}')
+ print(f'Convert {model_key} to {new_key}')
+ state_dict[new_key] = model_weight
+ converted_names.add(model_key)
+
+
+def convert(src, dst):
+ """Convert keys in pycls pretrained RegNet models to mmdet style."""
+ # load caffe model
+ regnet_model = torch.load(src)
+ blobs = regnet_model['model_state']
+ # convert to pytorch style
+ state_dict = OrderedDict()
+ converted_names = set()
+ for key, weight in blobs.items():
+ if 'stem' in key:
+ convert_stem(key, weight, state_dict, converted_names)
+ elif 'head' in key:
+ convert_head(key, weight, state_dict, converted_names)
+ elif key.startswith('s'):
+ convert_reslayer(key, weight, state_dict, converted_names)
+
+ # check if all layers are converted
+ for key in blobs:
+ if key not in converted_names:
+ print(f'not converted: {key}')
+ # save checkpoint
+ checkpoint = dict()
+ checkpoint['state_dict'] = state_dict
+ torch.save(checkpoint, dst)
+
+
+def main():
+ parser = argparse.ArgumentParser(description='Convert model keys')
+ parser.add_argument('src', help='src detectron model path')
+ parser.add_argument('dst', help='save path')
+ args = parser.parse_args()
+ convert(args.src, args.dst)
+
+
+if __name__ == '__main__':
+ main()
diff --git a/tools/slurm_test.sh b/tools/slurm_test.sh
new file mode 100755
index 0000000..6dd67e5
--- /dev/null
+++ b/tools/slurm_test.sh
@@ -0,0 +1,24 @@
+#!/usr/bin/env bash
+
+set -x
+
+PARTITION=$1
+JOB_NAME=$2
+CONFIG=$3
+CHECKPOINT=$4
+GPUS=${GPUS:-8}
+GPUS_PER_NODE=${GPUS_PER_NODE:-8}
+CPUS_PER_TASK=${CPUS_PER_TASK:-5}
+PY_ARGS=${@:5}
+SRUN_ARGS=${SRUN_ARGS:-""}
+
+PYTHONPATH="$(dirname $0)/..":$PYTHONPATH \
+srun -p ${PARTITION} \
+ --job-name=${JOB_NAME} \
+ --gres=gpu:${GPUS_PER_NODE} \
+ --ntasks=${GPUS} \
+ --ntasks-per-node=${GPUS_PER_NODE} \
+ --cpus-per-task=${CPUS_PER_TASK} \
+ --kill-on-bad-exit=1 \
+ ${SRUN_ARGS} \
+ python -u tools/test.py ${CONFIG} ${CHECKPOINT} --launcher="slurm" ${PY_ARGS}
diff --git a/tools/slurm_train.sh b/tools/slurm_train.sh
new file mode 100755
index 0000000..b3feb3d
--- /dev/null
+++ b/tools/slurm_train.sh
@@ -0,0 +1,24 @@
+#!/usr/bin/env bash
+
+set -x
+
+PARTITION=$1
+JOB_NAME=$2
+CONFIG=$3
+WORK_DIR=$4
+GPUS=${GPUS:-8}
+GPUS_PER_NODE=${GPUS_PER_NODE:-8}
+CPUS_PER_TASK=${CPUS_PER_TASK:-5}
+SRUN_ARGS=${SRUN_ARGS:-""}
+PY_ARGS=${@:5}
+
+PYTHONPATH="$(dirname $0)/..":$PYTHONPATH \
+srun -p ${PARTITION} \
+ --job-name=${JOB_NAME} \
+ --gres=gpu:${GPUS_PER_NODE} \
+ --ntasks=${GPUS} \
+ --ntasks-per-node=${GPUS_PER_NODE} \
+ --cpus-per-task=${CPUS_PER_TASK} \
+ --kill-on-bad-exit=1 \
+ ${SRUN_ARGS} \
+ python -u tools/train.py ${CONFIG} --work-dir=${WORK_DIR} --launcher="slurm" ${PY_ARGS}
diff --git a/tools/test.py b/tools/test.py
new file mode 100644
index 0000000..291c40b
--- /dev/null
+++ b/tools/test.py
@@ -0,0 +1,260 @@
+# Copyright (c) OpenMMLab. All rights reserved.
+import argparse
+import os
+import warnings
+
+import mmcv
+import torch
+from mmcv import Config, DictAction
+from mmcv.cnn import fuse_conv_bn
+from mmcv.parallel import MMDataParallel, MMDistributedDataParallel
+from mmcv.runner import (get_dist_info, init_dist, load_checkpoint,
+ wrap_fp16_model)
+
+import mmdet
+from mmdet3d.apis import single_gpu_test
+from mmdet3d.datasets import build_dataloader, build_dataset
+from mmdet3d.models import build_model
+from mmdet.apis import multi_gpu_test, set_random_seed
+from mmdet.datasets import replace_ImageToTensor
+
+if mmdet.__version__ > '2.23.0':
+ # If mmdet version > 2.23.0, setup_multi_processes would be imported and
+ # used from mmdet instead of mmdet3d.
+ from mmdet.utils import setup_multi_processes
+else:
+ from mmdet3d.utils import setup_multi_processes
+
+try:
+ # If mmdet version > 2.23.0, compat_cfg would be imported and
+ # used from mmdet instead of mmdet3d.
+ from mmdet.utils import compat_cfg
+except ImportError:
+ from mmdet3d.utils import compat_cfg
+
+
+def parse_args():
+ parser = argparse.ArgumentParser(
+ description='MMDet test (and eval) a model')
+ parser.add_argument('config', help='test config file path')
+ parser.add_argument('checkpoint', help='checkpoint file')
+ parser.add_argument('--out', help='output result file in pickle format')
+ parser.add_argument(
+ '--fuse-conv-bn',
+ action='store_true',
+ help='Whether to fuse conv and bn, this will slightly increase'
+ 'the inference speed')
+ parser.add_argument(
+ '--gpu-ids',
+ type=int,
+ nargs='+',
+ help='(Deprecated, please use --gpu-id) ids of gpus to use '
+ '(only applicable to non-distributed training)')
+ parser.add_argument(
+ '--gpu-id',
+ type=int,
+ default=0,
+ help='id of gpu to use '
+ '(only applicable to non-distributed testing)')
+ parser.add_argument(
+ '--format-only',
+ action='store_true',
+ help='Format the output results without perform evaluation. It is'
+ 'useful when you want to format the result to a specific format and '
+ 'submit it to the test server')
+ parser.add_argument(
+ '--eval',
+ type=str,
+ nargs='+',
+ help='evaluation metrics, which depends on the dataset, e.g., "bbox",'
+ ' "segm", "proposal" for COCO, and "mAP", "recall" for PASCAL VOC')
+ parser.add_argument('--show', action='store_true', help='show results')
+ parser.add_argument(
+ '--show-dir', help='directory where results will be saved')
+ parser.add_argument(
+ '--gpu-collect',
+ action='store_true',
+ help='whether to use gpu to collect results.')
+ parser.add_argument(
+ '--tmpdir',
+ help='tmp directory used for collecting results from multiple '
+ 'workers, available when gpu-collect is not specified')
+ parser.add_argument('--seed', type=int, default=0, help='random seed')
+ parser.add_argument(
+ '--deterministic',
+ action='store_true',
+ help='whether to set deterministic options for CUDNN backend.')
+ parser.add_argument(
+ '--cfg-options',
+ nargs='+',
+ action=DictAction,
+ help='override some settings in the used config, the key-value pair '
+ 'in xxx=yyy format will be merged into config file. If the value to '
+ 'be overwritten is a list, it should be like key="[a,b]" or key=a,b '
+ 'It also allows nested list/tuple values, e.g. key="[(a,b),(c,d)]" '
+ 'Note that the quotation marks are necessary and that no white space '
+ 'is allowed.')
+ parser.add_argument(
+ '--options',
+ nargs='+',
+ action=DictAction,
+ help='custom options for evaluation, the key-value pair in xxx=yyy '
+ 'format will be kwargs for dataset.evaluate() function (deprecate), '
+ 'change to --eval-options instead.')
+ parser.add_argument(
+ '--eval-options',
+ nargs='+',
+ action=DictAction,
+ help='custom options for evaluation, the key-value pair in xxx=yyy '
+ 'format will be kwargs for dataset.evaluate() function')
+ parser.add_argument(
+ '--launcher',
+ choices=['none', 'pytorch', 'slurm', 'mpi'],
+ default='none',
+ help='job launcher')
+ parser.add_argument('--local_rank', type=int, default=0)
+ args = parser.parse_args()
+ if 'LOCAL_RANK' not in os.environ:
+ os.environ['LOCAL_RANK'] = str(args.local_rank)
+
+ if args.options and args.eval_options:
+ raise ValueError(
+ '--options and --eval-options cannot be both specified, '
+ '--options is deprecated in favor of --eval-options')
+ if args.options:
+ warnings.warn('--options is deprecated in favor of --eval-options')
+ args.eval_options = args.options
+ return args
+
+
+def main():
+ args = parse_args()
+
+ assert args.out or args.eval or args.format_only or args.show \
+ or args.show_dir, \
+ ('Please specify at least one operation (save/eval/format/show the '
+ 'results / save the results) with the argument "--out", "--eval"'
+ ', "--format-only", "--show" or "--show-dir"')
+
+ if args.eval and args.format_only:
+ raise ValueError('--eval and --format_only cannot be both specified')
+
+ if args.out is not None and not args.out.endswith(('.pkl', '.pickle')):
+ raise ValueError('The output file must be a pkl file.')
+
+ cfg = Config.fromfile(args.config)
+ if args.cfg_options is not None:
+ cfg.merge_from_dict(args.cfg_options)
+
+ cfg = compat_cfg(cfg)
+
+ # set multi-process settings
+ setup_multi_processes(cfg)
+
+ # set cudnn_benchmark
+ if cfg.get('cudnn_benchmark', False):
+ torch.backends.cudnn.benchmark = True
+
+ cfg.model.pretrained = None
+
+ if args.gpu_ids is not None:
+ cfg.gpu_ids = args.gpu_ids[0:1]
+ warnings.warn('`--gpu-ids` is deprecated, please use `--gpu-id`. '
+ 'Because we only support single GPU mode in '
+ 'non-distributed testing. Use the first GPU '
+ 'in `gpu_ids` now.')
+ else:
+ cfg.gpu_ids = [args.gpu_id]
+
+ # init distributed env first, since logger depends on the dist info.
+ if args.launcher == 'none':
+ distributed = False
+ else:
+ distributed = True
+ init_dist(args.launcher, **cfg.dist_params)
+
+ test_dataloader_default_args = dict(
+ samples_per_gpu=1, workers_per_gpu=2, dist=distributed, shuffle=False)
+
+ # in case the test dataset is concatenated
+ if isinstance(cfg.data.test, dict):
+ cfg.data.test.test_mode = True
+ if cfg.data.test_dataloader.get('samples_per_gpu', 1) > 1:
+ # Replace 'ImageToTensor' to 'DefaultFormatBundle'
+ cfg.data.test.pipeline = replace_ImageToTensor(
+ cfg.data.test.pipeline)
+ elif isinstance(cfg.data.test, list):
+ for ds_cfg in cfg.data.test:
+ ds_cfg.test_mode = True
+ if cfg.data.test_dataloader.get('samples_per_gpu', 1) > 1:
+ for ds_cfg in cfg.data.test:
+ ds_cfg.pipeline = replace_ImageToTensor(ds_cfg.pipeline)
+
+ test_loader_cfg = {
+ **test_dataloader_default_args,
+ **cfg.data.get('test_dataloader', {})
+ }
+
+ # set random seeds
+ if args.seed is not None:
+ set_random_seed(args.seed, deterministic=args.deterministic)
+
+ # build the dataloader
+ dataset = build_dataset(cfg.data.test)
+ data_loader = build_dataloader(dataset, **test_loader_cfg)
+
+ # build the model and load checkpoint
+ cfg.model.train_cfg = None
+ model = build_model(cfg.model, test_cfg=cfg.get('test_cfg'))
+ fp16_cfg = cfg.get('fp16', None)
+ if fp16_cfg is not None:
+ wrap_fp16_model(model)
+ checkpoint = load_checkpoint(model, args.checkpoint, map_location='cpu')
+ if args.fuse_conv_bn:
+ model = fuse_conv_bn(model)
+ # old versions did not save class info in checkpoints, this walkaround is
+ # for backward compatibility
+ if 'CLASSES' in checkpoint.get('meta', {}):
+ model.CLASSES = checkpoint['meta']['CLASSES']
+ else:
+ model.CLASSES = dataset.CLASSES
+ # palette for visualization in segmentation tasks
+ if 'PALETTE' in checkpoint.get('meta', {}):
+ model.PALETTE = checkpoint['meta']['PALETTE']
+ elif hasattr(dataset, 'PALETTE'):
+ # segmentation dataset has `PALETTE` attribute
+ model.PALETTE = dataset.PALETTE
+
+ if not distributed:
+ model = MMDataParallel(model, device_ids=cfg.gpu_ids)
+ outputs = single_gpu_test(model, data_loader)
+ else:
+ model = MMDistributedDataParallel(
+ model.cuda(),
+ device_ids=[torch.cuda.current_device()],
+ broadcast_buffers=False)
+ outputs = multi_gpu_test(model, data_loader, args.tmpdir,
+ args.gpu_collect)
+
+ rank, _ = get_dist_info()
+ if rank == 0:
+ if args.out:
+ print(f'\nwriting results to {args.out}')
+ mmcv.dump(outputs, args.out)
+ kwargs = {} if args.eval_options is None else args.eval_options
+ if args.format_only:
+ dataset.format_results(outputs, **kwargs)
+ if args.eval:
+ eval_kwargs = cfg.get('evaluation', {}).copy()
+ # hard-code way to remove EvalHook args
+ for key in [
+ 'interval', 'tmpdir', 'start', 'gpu_collect', 'save_best',
+ 'rule'
+ ]:
+ eval_kwargs.pop(key, None)
+ eval_kwargs.update(dict(metric=args.eval, **kwargs))
+ print(dataset.evaluate(outputs, show=args.show, out_dir=args.show_dir, **eval_kwargs))
+
+
+if __name__ == '__main__':
+ main()
diff --git a/tools/train.py b/tools/train.py
new file mode 100644
index 0000000..ed9c2a6
--- /dev/null
+++ b/tools/train.py
@@ -0,0 +1,263 @@
+# Copyright (c) OpenMMLab. All rights reserved.
+from __future__ import division
+import argparse
+import copy
+import os
+import time
+import warnings
+from os import path as osp
+
+import mmcv
+import torch
+import torch.distributed as dist
+from mmcv import Config, DictAction
+from mmcv.runner import get_dist_info, init_dist
+
+from mmdet import __version__ as mmdet_version
+from mmdet3d import __version__ as mmdet3d_version
+from mmdet3d.apis import init_random_seed, train_model
+from mmdet3d.datasets import build_dataset
+from mmdet3d.models import build_model
+from mmdet3d.utils import collect_env, get_root_logger
+from mmdet.apis import set_random_seed
+from mmseg import __version__ as mmseg_version
+
+try:
+ # If mmdet version > 2.20.0, setup_multi_processes would be imported and
+ # used from mmdet instead of mmdet3d.
+ from mmdet.utils import setup_multi_processes
+except ImportError:
+ from mmdet3d.utils import setup_multi_processes
+
+
+def parse_args():
+ parser = argparse.ArgumentParser(description='Train a detector')
+ parser.add_argument('config', help='train config file path')
+ parser.add_argument('--work-dir', help='the dir to save logs and models')
+ parser.add_argument(
+ '--resume-from', help='the checkpoint file to resume from')
+ parser.add_argument(
+ '--auto-resume',
+ action='store_true',
+ help='resume from the latest checkpoint automatically')
+ parser.add_argument(
+ '--no-validate',
+ action='store_true',
+ help='whether not to evaluate the checkpoint during training')
+ group_gpus = parser.add_mutually_exclusive_group()
+ group_gpus.add_argument(
+ '--gpus',
+ type=int,
+ help='(Deprecated, please use --gpu-id) number of gpus to use '
+ '(only applicable to non-distributed training)')
+ group_gpus.add_argument(
+ '--gpu-ids',
+ type=int,
+ nargs='+',
+ help='(Deprecated, please use --gpu-id) ids of gpus to use '
+ '(only applicable to non-distributed training)')
+ group_gpus.add_argument(
+ '--gpu-id',
+ type=int,
+ default=0,
+ help='number of gpus to use '
+ '(only applicable to non-distributed training)')
+ parser.add_argument('--seed', type=int, default=0, help='random seed')
+ parser.add_argument(
+ '--diff-seed',
+ action='store_true',
+ help='Whether or not set different seeds for different ranks')
+ parser.add_argument(
+ '--deterministic',
+ action='store_true',
+ help='whether to set deterministic options for CUDNN backend.')
+ parser.add_argument(
+ '--options',
+ nargs='+',
+ action=DictAction,
+ help='override some settings in the used config, the key-value pair '
+ 'in xxx=yyy format will be merged into config file (deprecate), '
+ 'change to --cfg-options instead.')
+ parser.add_argument(
+ '--cfg-options',
+ nargs='+',
+ action=DictAction,
+ help='override some settings in the used config, the key-value pair '
+ 'in xxx=yyy format will be merged into config file. If the value to '
+ 'be overwritten is a list, it should be like key="[a,b]" or key=a,b '
+ 'It also allows nested list/tuple values, e.g. key="[(a,b),(c,d)]" '
+ 'Note that the quotation marks are necessary and that no white space '
+ 'is allowed.')
+ parser.add_argument(
+ '--launcher',
+ choices=['none', 'pytorch', 'slurm', 'mpi'],
+ default='none',
+ help='job launcher')
+ parser.add_argument('--local_rank', type=int, default=0)
+ parser.add_argument(
+ '--autoscale-lr',
+ action='store_true',
+ help='automatically scale lr with the number of gpus')
+ args = parser.parse_args()
+ if 'LOCAL_RANK' not in os.environ:
+ os.environ['LOCAL_RANK'] = str(args.local_rank)
+
+ if args.options and args.cfg_options:
+ raise ValueError(
+ '--options and --cfg-options cannot be both specified, '
+ '--options is deprecated in favor of --cfg-options')
+ if args.options:
+ warnings.warn('--options is deprecated in favor of --cfg-options')
+ args.cfg_options = args.options
+
+ return args
+
+
+def main():
+ args = parse_args()
+
+ cfg = Config.fromfile(args.config)
+ if args.cfg_options is not None:
+ cfg.merge_from_dict(args.cfg_options)
+
+ # set multi-process settings
+ setup_multi_processes(cfg)
+
+ # set cudnn_benchmark
+ if cfg.get('cudnn_benchmark', False):
+ torch.backends.cudnn.benchmark = True
+
+ # work_dir is determined in this priority: CLI > segment in file > filename
+ if args.work_dir is not None:
+ # update configs according to CLI args if args.work_dir is not None
+ cfg.work_dir = args.work_dir
+ elif cfg.get('work_dir', None) is None:
+ # use config filename as default work_dir if cfg.work_dir is None
+ cfg.work_dir = osp.join('./work_dirs',
+ osp.splitext(osp.basename(args.config))[0])
+ if args.resume_from is not None:
+ cfg.resume_from = args.resume_from
+
+ if args.auto_resume:
+ cfg.auto_resume = args.auto_resume
+ warnings.warn('`--auto-resume` is only supported when mmdet'
+ 'version >= 2.20.0 for 3D detection model or'
+ 'mmsegmentation verision >= 0.21.0 for 3D'
+ 'segmentation model')
+
+ if args.gpus is not None:
+ cfg.gpu_ids = range(1)
+ warnings.warn('`--gpus` is deprecated because we only support '
+ 'single GPU mode in non-distributed training. '
+ 'Use `gpus=1` now.')
+ if args.gpu_ids is not None:
+ cfg.gpu_ids = args.gpu_ids[0:1]
+ warnings.warn('`--gpu-ids` is deprecated, please use `--gpu-id`. '
+ 'Because we only support single GPU mode in '
+ 'non-distributed training. Use the first GPU '
+ 'in `gpu_ids` now.')
+ if args.gpus is None and args.gpu_ids is None:
+ cfg.gpu_ids = [args.gpu_id]
+
+ if args.autoscale_lr:
+ # apply the linear scaling rule (https://arxiv.org/abs/1706.02677)
+ cfg.optimizer['lr'] = cfg.optimizer['lr'] * len(cfg.gpu_ids) / 8
+
+ # init distributed env first, since logger depends on the dist info.
+ if args.launcher == 'none':
+ distributed = False
+ else:
+ distributed = True
+ init_dist(args.launcher, **cfg.dist_params)
+ # re-set gpu_ids with distributed training mode
+ _, world_size = get_dist_info()
+ cfg.gpu_ids = range(world_size)
+
+ # create work_dir
+ mmcv.mkdir_or_exist(osp.abspath(cfg.work_dir))
+ # dump config
+ cfg.dump(osp.join(cfg.work_dir, osp.basename(args.config)))
+ # init the logger before other steps
+ timestamp = time.strftime('%Y%m%d_%H%M%S', time.localtime())
+ log_file = osp.join(cfg.work_dir, f'{timestamp}.log')
+ # specify logger name, if we still use 'mmdet', the output info will be
+ # filtered and won't be saved in the log_file
+ # TODO: ugly workaround to judge whether we are training det or seg model
+ if cfg.model.type in ['EncoderDecoder3D']:
+ logger_name = 'mmseg'
+ else:
+ logger_name = 'mmdet'
+ logger = get_root_logger(
+ log_file=log_file, log_level=cfg.log_level, name=logger_name)
+
+ # init the meta dict to record some important information such as
+ # environment info and seed, which will be logged
+ meta = dict()
+ # log env info
+ env_info_dict = collect_env()
+ env_info = '\n'.join([(f'{k}: {v}') for k, v in env_info_dict.items()])
+ dash_line = '-' * 60 + '\n'
+ logger.info('Environment info:\n' + dash_line + env_info + '\n' +
+ dash_line)
+ meta['env_info'] = env_info
+ meta['config'] = cfg.pretty_text
+
+ # log some basic info
+ logger.info(f'Distributed training: {distributed}')
+ logger.info(f'Config:\n{cfg.pretty_text}')
+
+ # set random seeds
+ seed = init_random_seed(args.seed)
+ seed = seed + dist.get_rank() if args.diff_seed else seed
+ logger.info(f'Set random seed to {seed}, '
+ f'deterministic: {args.deterministic}')
+ set_random_seed(seed, deterministic=args.deterministic)
+ cfg.seed = seed
+ meta['seed'] = seed
+ meta['exp_name'] = osp.basename(args.config)
+
+ model = build_model(
+ cfg.model,
+ train_cfg=cfg.get('train_cfg'),
+ test_cfg=cfg.get('test_cfg'))
+ model.init_weights()
+
+ logger.info(f'Model:\n{model}')
+ datasets = [build_dataset(cfg.data.train)]
+ if len(cfg.workflow) == 2:
+ val_dataset = copy.deepcopy(cfg.data.val)
+ # in case we use a dataset wrapper
+ if 'dataset' in cfg.data.train:
+ val_dataset.pipeline = cfg.data.train.dataset.pipeline
+ else:
+ val_dataset.pipeline = cfg.data.train.pipeline
+ # set test_mode=False here in deep copied config
+ # which do not affect AP/AR calculation later
+ # refer to https://mmdetection3d.readthedocs.io/en/latest/tutorials/customize_runtime.html#customize-workflow # noqa
+ val_dataset.test_mode = False
+ datasets.append(build_dataset(val_dataset))
+ if cfg.checkpoint_config is not None:
+ # save mmdet version, config file content and class names in
+ # checkpoints as meta data
+ cfg.checkpoint_config.meta = dict(
+ mmdet_version=mmdet_version,
+ mmseg_version=mmseg_version,
+ mmdet3d_version=mmdet3d_version,
+ config=cfg.pretty_text,
+ CLASSES=datasets[0].CLASSES,
+ PALETTE=datasets[0].PALETTE # for segmentors
+ if hasattr(datasets[0], 'PALETTE') else None)
+ # add an attribute for visualization convenience
+ model.CLASSES = datasets[0].CLASSES
+ train_model(
+ model,
+ datasets,
+ cfg,
+ distributed=distributed,
+ validate=(not args.no_validate),
+ timestamp=timestamp,
+ meta=meta)
+
+
+if __name__ == '__main__':
+ main()
diff --git a/tools/update_data_coords.py b/tools/update_data_coords.py
new file mode 100644
index 0000000..94728bc
--- /dev/null
+++ b/tools/update_data_coords.py
@@ -0,0 +1,168 @@
+import argparse
+import time
+from os import path as osp
+
+import mmcv
+import numpy as np
+
+from mmdet3d.core.bbox import limit_period
+
+
+def update_sunrgbd_infos(root_dir, out_dir, pkl_files):
+ print(f'{pkl_files} will be modified because '
+ f'of the refactor of the Depth coordinate system.')
+ if root_dir == out_dir:
+ print(f'Warning, you are overwriting '
+ f'the original data under {root_dir}.')
+ time.sleep(3)
+ for pkl_file in pkl_files:
+ in_path = osp.join(root_dir, pkl_file)
+ print(f'Reading from input file: {in_path}.')
+ a = mmcv.load(in_path)
+ print('Start updating:')
+ for item in mmcv.track_iter_progress(a):
+ if 'rotation_y' in item['annos']:
+ item['annos']['rotation_y'] = -item['annos']['rotation_y']
+ item['annos']['gt_boxes_upright_depth'][:, -1:] = \
+ -item['annos']['gt_boxes_upright_depth'][:, -1:]
+
+ out_path = osp.join(out_dir, pkl_file)
+ print(f'Writing to output file: {out_path}.')
+ mmcv.dump(a, out_path, 'pkl')
+
+
+def update_outdoor_dbinfos(root_dir, out_dir, pkl_files):
+ print(f'{pkl_files} will be modified because '
+ f'of the refactor of the LIDAR coordinate system.')
+ if root_dir == out_dir:
+ print(f'Warning, you are overwriting '
+ f'the original data under {root_dir}.')
+ time.sleep(3)
+ for pkl_file in pkl_files:
+ in_path = osp.join(root_dir, pkl_file)
+ print(f'Reading from input file: {in_path}.')
+ a = mmcv.load(in_path)
+ print('Start updating:')
+ for k in a.keys():
+ print(f'Updating samples of class {k}:')
+ for item in mmcv.track_iter_progress(a[k]):
+ boxes = item['box3d_lidar'].copy()
+ # swap l, w (or dx, dy)
+ item['box3d_lidar'][3] = boxes[4]
+ item['box3d_lidar'][4] = boxes[3]
+ # change yaw
+ item['box3d_lidar'][6] = -boxes[6] - np.pi / 2
+ item['box3d_lidar'][6] = limit_period(
+ item['box3d_lidar'][6], period=np.pi * 2)
+
+ out_path = osp.join(out_dir, pkl_file)
+ print(f'Writing to output file: {out_path}.')
+ mmcv.dump(a, out_path, 'pkl')
+
+
+def update_nuscenes_or_lyft_infos(root_dir, out_dir, pkl_files):
+
+ print(f'{pkl_files} will be modified because '
+ f'of the refactor of the LIDAR coordinate system.')
+ if root_dir == out_dir:
+ print(f'Warning, you are overwriting '
+ f'the original data under {root_dir}.')
+ time.sleep(3)
+ for pkl_file in pkl_files:
+ in_path = osp.join(root_dir, pkl_file)
+ print(f'Reading from input file: {in_path}.')
+ a = mmcv.load(in_path)
+ print('Start updating:')
+ for item in mmcv.track_iter_progress(a['infos']):
+ boxes = item['gt_boxes'].copy()
+ # swap l, w (or dx, dy)
+ item['gt_boxes'][:, 3] = boxes[:, 4]
+ item['gt_boxes'][:, 4] = boxes[:, 3]
+ # change yaw
+ item['gt_boxes'][:, 6] = -boxes[:, 6] - np.pi / 2
+ item['gt_boxes'][:, 6] = limit_period(
+ item['gt_boxes'][:, 6], period=np.pi * 2)
+
+ out_path = osp.join(out_dir, pkl_file)
+ print(f'Writing to output file: {out_path}.')
+ mmcv.dump(a, out_path, 'pkl')
+
+
+parser = argparse.ArgumentParser(description='Arg parser for data coords '
+ 'update due to coords sys refactor.')
+parser.add_argument('dataset', metavar='kitti', help='name of the dataset')
+parser.add_argument(
+ '--root-dir',
+ type=str,
+ default='./data/kitti',
+ help='specify the root dir of dataset')
+parser.add_argument(
+ '--version',
+ type=str,
+ default='v1.0',
+ required=False,
+ help='specify the dataset version, no need for kitti')
+parser.add_argument(
+ '--out-dir',
+ type=str,
+ default=None,
+ required=False,
+ help='name of info pkl')
+args = parser.parse_args()
+
+if __name__ == '__main__':
+ if args.out_dir is None:
+ args.out_dir = args.root_dir
+ if args.dataset == 'kitti':
+ # KITTI infos is in CAM coord sys (unchanged)
+ # KITTI dbinfos is in LIDAR coord sys (changed)
+ # so we only update dbinfos
+ pkl_files = ['kitti_dbinfos_train.pkl']
+ update_outdoor_dbinfos(
+ root_dir=args.root_dir, out_dir=args.out_dir, pkl_files=pkl_files)
+ elif args.dataset == 'nuscenes':
+ # nuScenes infos is in LIDAR coord sys (changed)
+ # nuScenes dbinfos is in LIDAR coord sys (changed)
+ # so we update both infos and dbinfos
+ pkl_files = ['nuscenes_infos_val.pkl']
+ if args.version != 'v1.0-mini':
+ pkl_files.append('nuscenes_infos_train.pkl')
+ else:
+ pkl_files.append('nuscenes_infos_train_tiny.pkl')
+ update_nuscenes_or_lyft_infos(
+ root_dir=args.root_dir, out_dir=args.out_dir, pkl_files=pkl_files)
+ if args.version != 'v1.0-mini':
+ pkl_files = ['nuscenes_dbinfos_train.pkl']
+ update_outdoor_dbinfos(
+ root_dir=args.root_dir,
+ out_dir=args.out_dir,
+ pkl_files=pkl_files)
+ elif args.dataset == 'lyft':
+ # Lyft infos is in LIDAR coord sys (changed)
+ # Lyft has no dbinfos
+ # so we update infos
+ pkl_files = ['lyft_infos_train.pkl', 'lyft_infos_val.pkl']
+ update_nuscenes_or_lyft_infos(
+ root_dir=args.root_dir, out_dir=args.out_dir, pkl_files=pkl_files)
+ elif args.dataset == 'waymo':
+ # Waymo infos is in CAM coord sys (unchanged)
+ # Waymo dbinfos is in LIDAR coord sys (changed)
+ # so we only update dbinfos
+ pkl_files = ['waymo_dbinfos_train.pkl']
+ update_outdoor_dbinfos(
+ root_dir=args.root_dir, out_dir=args.out_dir, pkl_files=pkl_files)
+ elif args.dataset == 'scannet':
+ # ScanNet infos is in DEPTH coord sys (changed)
+ # but bbox is without yaw
+ # so ScanNet is unaffected
+ pass
+ elif args.dataset == 's3dis':
+ # Segmentation datasets are not affected
+ pass
+ elif args.dataset == 'sunrgbd':
+ # SUNRGBD infos is in DEPTH coord sys (changed)
+ # and bbox is with yaw
+ # so we update infos
+ pkl_files = ['sunrgbd_infos_train.pkl', 'sunrgbd_infos_val.pkl']
+ update_sunrgbd_infos(
+ root_dir=args.root_dir, out_dir=args.out_dir, pkl_files=pkl_files)
diff --git a/tools/update_data_coords.sh b/tools/update_data_coords.sh
new file mode 100644
index 0000000..bd8db62
--- /dev/null
+++ b/tools/update_data_coords.sh
@@ -0,0 +1,22 @@
+#!/usr/bin/env bash
+
+set -x
+export PYTHONPATH=`pwd`:$PYTHONPATH
+
+PARTITION=$1
+DATASET=$2
+GPUS=${GPUS:-1}
+GPUS_PER_NODE=${GPUS_PER_NODE:-1}
+SRUN_ARGS=${SRUN_ARGS:-""}
+JOB_NAME=update_data_coords
+
+srun -p ${PARTITION} \
+ --job-name=${JOB_NAME} \
+ --gres=gpu:${GPUS_PER_NODE} \
+ --ntasks=${GPUS} \
+ --ntasks-per-node=${GPUS_PER_NODE} \
+ --kill-on-bad-exit=1 \
+ ${SRUN_ARGS} \
+ python -u tools/update_data_coords.py ${DATASET} \
+ --root-dir ./data/${DATASET} \
+ --out-dir ./data/${DATASET}